hexsha
stringlengths 40
40
| size
int64 6
14.9M
| ext
stringclasses 1
value | lang
stringclasses 1
value | max_stars_repo_path
stringlengths 6
260
| max_stars_repo_name
stringlengths 6
119
| max_stars_repo_head_hexsha
stringlengths 40
41
| max_stars_repo_licenses
sequence | max_stars_count
int64 1
191k
⌀ | max_stars_repo_stars_event_min_datetime
stringlengths 24
24
⌀ | max_stars_repo_stars_event_max_datetime
stringlengths 24
24
⌀ | max_issues_repo_path
stringlengths 6
260
| max_issues_repo_name
stringlengths 6
119
| max_issues_repo_head_hexsha
stringlengths 40
41
| max_issues_repo_licenses
sequence | max_issues_count
int64 1
67k
⌀ | max_issues_repo_issues_event_min_datetime
stringlengths 24
24
⌀ | max_issues_repo_issues_event_max_datetime
stringlengths 24
24
⌀ | max_forks_repo_path
stringlengths 6
260
| max_forks_repo_name
stringlengths 6
119
| max_forks_repo_head_hexsha
stringlengths 40
41
| max_forks_repo_licenses
sequence | max_forks_count
int64 1
105k
⌀ | max_forks_repo_forks_event_min_datetime
stringlengths 24
24
⌀ | max_forks_repo_forks_event_max_datetime
stringlengths 24
24
⌀ | avg_line_length
float64 2
1.04M
| max_line_length
int64 2
11.2M
| alphanum_fraction
float64 0
1
| cells
sequence | cell_types
sequence | cell_type_groups
sequence |
---|---|---|---|---|---|---|---|---|---|---|---|---|---|---|---|---|---|---|---|---|---|---|---|---|---|---|---|---|---|---|
d08d493bc9afca3373262b8a658008c40ed86f1d | 19,570 | ipynb | Jupyter Notebook | Introduction to Portfolio Construction and Analysis with Python/W1/Deviation From Normality.ipynb | Alashmony/InvestmentManagementML | c4721f77f1523b06edf012d9139b08a2dba39e59 | [
"Unlicense"
] | null | null | null | Introduction to Portfolio Construction and Analysis with Python/W1/Deviation From Normality.ipynb | Alashmony/InvestmentManagementML | c4721f77f1523b06edf012d9139b08a2dba39e59 | [
"Unlicense"
] | null | null | null | Introduction to Portfolio Construction and Analysis with Python/W1/Deviation From Normality.ipynb | Alashmony/InvestmentManagementML | c4721f77f1523b06edf012d9139b08a2dba39e59 | [
"Unlicense"
] | 1 | 2022-02-16T01:33:59.000Z | 2022-02-16T01:33:59.000Z | 28.077475 | 86 | 0.385948 | [
[
[
"# Deviations from Normality",
"_____no_output_____"
]
],
[
[
"import ashmodule as ash\n\n%load_ext autoreload\n%autoreload 2",
"_____no_output_____"
],
[
"hfi = ash.get_hfi_returns()\nhfi.head()",
"_____no_output_____"
],
[
"import pandas as pd\npd.concat([hfi.mean(),hfi.median(), hfi.mean()>hfi.median()],axis='columns')",
"_____no_output_____"
]
],
[
[
"## Skewness Function:\n$$ S(R)= \\frac{ E [(R-E(R))^3]}{\\sigma_R^3} $$",
"_____no_output_____"
]
],
[
[
"%autoreload 2",
"_____no_output_____"
],
[
"ash.skewness(hfi)",
"_____no_output_____"
],
[
"import scipy.stats \nscipy.stats.skew(hfi)",
"_____no_output_____"
],
[
"ash.skewness(hfi)",
"_____no_output_____"
]
],
[
[
"## Kurtosis Function:\n$$ S(R)= \\frac{ E [(R-E(R))^4]}{\\sigma_R^4} $$",
"_____no_output_____"
]
],
[
[
"%autoreload 2\nash.kurt(hfi)",
"_____no_output_____"
],
[
"scipy.stats.kurtosis(hfi)+3",
"_____no_output_____"
],
[
"scipy.stats.jarque_bera(hfi)",
"_____no_output_____"
],
[
"ash.is_normal(hfi)",
"_____no_output_____"
],
[
"hfi.aggregate(ash.is_normal)",
"_____no_output_____"
],
[
"ffme= ash.get_ffme_returns()",
"_____no_output_____"
],
[
"ash.skewness(ffme)",
"_____no_output_____"
],
[
"ash.kurt(ffme)",
"_____no_output_____"
],
[
"ffme.aggregate(ash.is_normal)",
"_____no_output_____"
]
]
] | [
"markdown",
"code",
"markdown",
"code",
"markdown",
"code"
] | [
[
"markdown"
],
[
"code",
"code",
"code"
],
[
"markdown"
],
[
"code",
"code",
"code",
"code"
],
[
"markdown"
],
[
"code",
"code",
"code",
"code",
"code",
"code",
"code",
"code",
"code"
]
] |
d08d54512e0e6df5aa5c7706545b9555136e7964 | 41,700 | ipynb | Jupyter Notebook | ml/cc/exercises/estimators/es-419/improving_neural_net_performance.ipynb | plgod/eng-edu | 76ee3958c321818fe02d226b558c468faa8a1041 | [
"Apache-2.0"
] | 3 | 2019-09-20T19:07:37.000Z | 2019-09-27T15:34:31.000Z | ml/cc/exercises/estimators/es-419/improving_neural_net_performance.ipynb | plgod/eng-edu | 76ee3958c321818fe02d226b558c468faa8a1041 | [
"Apache-2.0"
] | null | null | null | ml/cc/exercises/estimators/es-419/improving_neural_net_performance.ipynb | plgod/eng-edu | 76ee3958c321818fe02d226b558c468faa8a1041 | [
"Apache-2.0"
] | 3 | 2019-09-19T00:28:31.000Z | 2019-09-28T10:49:05.000Z | 38.151876 | 591 | 0.552254 | [
[
[
"#### Copyright 2017 Google LLC.",
"_____no_output_____"
]
],
[
[
"# Licensed under the Apache License, Version 2.0 (the \"License\");\n# you may not use this file except in compliance with the License.\n# You may obtain a copy of the License at\n#\n# https://www.apache.org/licenses/LICENSE-2.0\n#\n# Unless required by applicable law or agreed to in writing, software\n# distributed under the License is distributed on an \"AS IS\" BASIS,\n# WITHOUT WARRANTIES OR CONDITIONS OF ANY KIND, either express or implied.\n# See the License for the specific language governing permissions and\n# limitations under the License.",
"_____no_output_____"
]
],
[
[
"# Mejora del rendimiento de las redes neuronales",
"_____no_output_____"
],
[
" **Objetivo de aprendizaje:** mejorar el rendimiento de una red neuronal al normalizar los atributos y aplicar diversos algoritmos de optimización\n\n**NOTA:** Los métodos de optimización que se describen en este ejercicio no son específicos para las redes neuronales; son medios eficaces para mejorar la mayoría de los tipos de modelos.",
"_____no_output_____"
],
[
" ## Preparación\n\nPrimero, cargaremos los datos.",
"_____no_output_____"
]
],
[
[
"from __future__ import print_function\n\nimport math\n\nfrom IPython import display\nfrom matplotlib import cm\nfrom matplotlib import gridspec\nfrom matplotlib import pyplot as plt\nimport numpy as np\nimport pandas as pd\nfrom sklearn import metrics\nimport tensorflow as tf\nfrom tensorflow.python.data import Dataset\n\ntf.logging.set_verbosity(tf.logging.ERROR)\npd.options.display.max_rows = 10\npd.options.display.float_format = '{:.1f}'.format\n\ncalifornia_housing_dataframe = pd.read_csv(\"https://download.mlcc.google.com/mledu-datasets/california_housing_train.csv\", sep=\",\")\n\ncalifornia_housing_dataframe = california_housing_dataframe.reindex(\n np.random.permutation(california_housing_dataframe.index))",
"_____no_output_____"
],
[
"def preprocess_features(california_housing_dataframe):\n \"\"\"Prepares input features from California housing data set.\n\n Args:\n california_housing_dataframe: A Pandas DataFrame expected to contain data\n from the California housing data set.\n Returns:\n A DataFrame that contains the features to be used for the model, including\n synthetic features.\n \"\"\"\n selected_features = california_housing_dataframe[\n [\"latitude\",\n \"longitude\",\n \"housing_median_age\",\n \"total_rooms\",\n \"total_bedrooms\",\n \"population\",\n \"households\",\n \"median_income\"]]\n processed_features = selected_features.copy()\n # Create a synthetic feature.\n processed_features[\"rooms_per_person\"] = (\n california_housing_dataframe[\"total_rooms\"] /\n california_housing_dataframe[\"population\"])\n return processed_features\n\ndef preprocess_targets(california_housing_dataframe):\n \"\"\"Prepares target features (i.e., labels) from California housing data set.\n\n Args:\n california_housing_dataframe: A Pandas DataFrame expected to contain data\n from the California housing data set.\n Returns:\n A DataFrame that contains the target feature.\n \"\"\"\n output_targets = pd.DataFrame()\n # Scale the target to be in units of thousands of dollars.\n output_targets[\"median_house_value\"] = (\n california_housing_dataframe[\"median_house_value\"] / 1000.0)\n return output_targets",
"_____no_output_____"
],
[
"# Choose the first 12000 (out of 17000) examples for training.\ntraining_examples = preprocess_features(california_housing_dataframe.head(12000))\ntraining_targets = preprocess_targets(california_housing_dataframe.head(12000))\n\n# Choose the last 5000 (out of 17000) examples for validation.\nvalidation_examples = preprocess_features(california_housing_dataframe.tail(5000))\nvalidation_targets = preprocess_targets(california_housing_dataframe.tail(5000))\n\n# Double-check that we've done the right thing.\nprint(\"Training examples summary:\")\ndisplay.display(training_examples.describe())\nprint(\"Validation examples summary:\")\ndisplay.display(validation_examples.describe())\n\nprint(\"Training targets summary:\")\ndisplay.display(training_targets.describe())\nprint(\"Validation targets summary:\")\ndisplay.display(validation_targets.describe())",
"_____no_output_____"
]
],
[
[
" ## Entrenamiento de la red neuronal\n\nA continuación, entrenaremos la red neuronal.",
"_____no_output_____"
]
],
[
[
"def construct_feature_columns(input_features):\n \"\"\"Construct the TensorFlow Feature Columns.\n\n Args:\n input_features: The names of the numerical input features to use.\n Returns:\n A set of feature columns\n \"\"\" \n return set([tf.feature_column.numeric_column(my_feature)\n for my_feature in input_features])",
"_____no_output_____"
],
[
"def my_input_fn(features, targets, batch_size=1, shuffle=True, num_epochs=None):\n \"\"\"Trains a neural network model.\n \n Args:\n features: pandas DataFrame of features\n targets: pandas DataFrame of targets\n batch_size: Size of batches to be passed to the model\n shuffle: True or False. Whether to shuffle the data.\n num_epochs: Number of epochs for which data should be repeated. None = repeat indefinitely\n Returns:\n Tuple of (features, labels) for next data batch\n \"\"\"\n \n # Convert pandas data into a dict of np arrays.\n features = {key:np.array(value) for key,value in dict(features).items()} \n \n # Construct a dataset, and configure batching/repeating.\n ds = Dataset.from_tensor_slices((features,targets)) # warning: 2GB limit\n ds = ds.batch(batch_size).repeat(num_epochs)\n \n # Shuffle the data, if specified.\n if shuffle:\n ds = ds.shuffle(10000)\n \n # Return the next batch of data.\n features, labels = ds.make_one_shot_iterator().get_next()\n return features, labels",
"_____no_output_____"
],
[
"def train_nn_regression_model(\n my_optimizer,\n steps,\n batch_size,\n hidden_units,\n training_examples,\n training_targets,\n validation_examples,\n validation_targets):\n \"\"\"Trains a neural network regression model.\n \n In addition to training, this function also prints training progress information,\n as well as a plot of the training and validation loss over time.\n \n Args:\n my_optimizer: An instance of `tf.train.Optimizer`, the optimizer to use.\n steps: A non-zero `int`, the total number of training steps. A training step\n consists of a forward and backward pass using a single batch.\n batch_size: A non-zero `int`, the batch size.\n hidden_units: A `list` of int values, specifying the number of neurons in each layer.\n training_examples: A `DataFrame` containing one or more columns from\n `california_housing_dataframe` to use as input features for training.\n training_targets: A `DataFrame` containing exactly one column from\n `california_housing_dataframe` to use as target for training.\n validation_examples: A `DataFrame` containing one or more columns from\n `california_housing_dataframe` to use as input features for validation.\n validation_targets: A `DataFrame` containing exactly one column from\n `california_housing_dataframe` to use as target for validation.\n \n Returns:\n A tuple `(estimator, training_losses, validation_losses)`:\n estimator: the trained `DNNRegressor` object.\n training_losses: a `list` containing the training loss values taken during training.\n validation_losses: a `list` containing the validation loss values taken during training.\n \"\"\"\n\n periods = 10\n steps_per_period = steps / periods\n \n # Create a DNNRegressor object.\n my_optimizer = tf.contrib.estimator.clip_gradients_by_norm(my_optimizer, 5.0)\n dnn_regressor = tf.estimator.DNNRegressor(\n feature_columns=construct_feature_columns(training_examples),\n hidden_units=hidden_units,\n optimizer=my_optimizer\n )\n \n # Create input functions.\n training_input_fn = lambda: my_input_fn(training_examples, \n training_targets[\"median_house_value\"], \n batch_size=batch_size)\n predict_training_input_fn = lambda: my_input_fn(training_examples, \n training_targets[\"median_house_value\"], \n num_epochs=1, \n shuffle=False)\n predict_validation_input_fn = lambda: my_input_fn(validation_examples, \n validation_targets[\"median_house_value\"], \n num_epochs=1, \n shuffle=False)\n\n # Train the model, but do so inside a loop so that we can periodically assess\n # loss metrics.\n print(\"Training model...\")\n print(\"RMSE (on training data):\")\n training_rmse = []\n validation_rmse = []\n for period in range (0, periods):\n # Train the model, starting from the prior state.\n dnn_regressor.train(\n input_fn=training_input_fn,\n steps=steps_per_period\n )\n # Take a break and compute predictions.\n training_predictions = dnn_regressor.predict(input_fn=predict_training_input_fn)\n training_predictions = np.array([item['predictions'][0] for item in training_predictions])\n \n validation_predictions = dnn_regressor.predict(input_fn=predict_validation_input_fn)\n validation_predictions = np.array([item['predictions'][0] for item in validation_predictions])\n \n # Compute training and validation loss.\n training_root_mean_squared_error = math.sqrt(\n metrics.mean_squared_error(training_predictions, training_targets))\n validation_root_mean_squared_error = math.sqrt(\n metrics.mean_squared_error(validation_predictions, validation_targets))\n # Occasionally print the current loss.\n print(\" period %02d : %0.2f\" % (period, training_root_mean_squared_error))\n # Add the loss metrics from this period to our list.\n training_rmse.append(training_root_mean_squared_error)\n validation_rmse.append(validation_root_mean_squared_error)\n print(\"Model training finished.\")\n\n # Output a graph of loss metrics over periods.\n plt.ylabel(\"RMSE\")\n plt.xlabel(\"Periods\")\n plt.title(\"Root Mean Squared Error vs. Periods\")\n plt.tight_layout()\n plt.plot(training_rmse, label=\"training\")\n plt.plot(validation_rmse, label=\"validation\")\n plt.legend()\n\n print(\"Final RMSE (on training data): %0.2f\" % training_root_mean_squared_error)\n print(\"Final RMSE (on validation data): %0.2f\" % validation_root_mean_squared_error)\n\n return dnn_regressor, training_rmse, validation_rmse",
"_____no_output_____"
],
[
"_ = train_nn_regression_model(\n my_optimizer=tf.train.GradientDescentOptimizer(learning_rate=0.0007),\n steps=5000,\n batch_size=70,\n hidden_units=[10, 10],\n training_examples=training_examples,\n training_targets=training_targets,\n validation_examples=validation_examples,\n validation_targets=validation_targets)",
"_____no_output_____"
]
],
[
[
" ## Ajuste lineal\nUna buena práctica estándar puede ser normalizar las entradas para que estén dentro del rango -1, 1. Esto ayuda al SGD a no bloquearse al realizar pasos que son demasiado grandes en una dimensión o demasiado pequeños en otra. Los apasionados de la optimización numérica pueden observar aquí una relación con la idea de usar un precondicionador.",
"_____no_output_____"
]
],
[
[
"def linear_scale(series):\n min_val = series.min()\n max_val = series.max()\n scale = (max_val - min_val) / 2.0\n return series.apply(lambda x:((x - min_val) / scale) - 1.0)",
"_____no_output_____"
]
],
[
[
" ## Tarea 1: Normalizar los atributos con ajuste lineal\n**Normaliza las entradas a la escala -1, 1.**\n**Dedica alrededor de 5 minutos a entrenar y evaluar los datos recientemente normalizados. ¿Qué nivel de eficacia puedes tener?**\nComo regla general, las redes neuronales se entrenan mejor cuando los atributos de entrada están casi en la misma escala.\nRealiza una comprobación de estado de tus datos normalizados. (¿Qué ocurriría si olvidaras normalizar un atributo?)\n",
"_____no_output_____"
]
],
[
[
"def normalize_linear_scale(examples_dataframe):\n \"\"\"Returns a version of the input `DataFrame` that has all its features normalized linearly.\"\"\"\n #\n # Your code here: normalize the inputs.\n #\n pass\n\nnormalized_dataframe = normalize_linear_scale(preprocess_features(california_housing_dataframe))\nnormalized_training_examples = normalized_dataframe.head(12000)\nnormalized_validation_examples = normalized_dataframe.tail(5000)\n\n_ = train_nn_regression_model(\n my_optimizer=tf.train.GradientDescentOptimizer(learning_rate=0.0007),\n steps=5000,\n batch_size=70,\n hidden_units=[10, 10],\n training_examples=normalized_training_examples,\n training_targets=training_targets,\n validation_examples=normalized_validation_examples,\n validation_targets=validation_targets)",
"_____no_output_____"
]
],
[
[
" ### Solución\n\nHaz clic más abajo para conocer una solución posible.",
"_____no_output_____"
],
[
" Dado que la normalización usa mín. y máx., debemos asegurarnos de que esta se realice en todo el conjunto de datos a la vez.\n\nEn este caso podemos hacerlo, porque todos nuestros datos están en un mismo DataFrame. Si tuviéramos varios conjuntos de datos, una buena práctica sería derivar los parámetros de normalización del conjunto de entrenamiento y aplicarlos de manera idéntica al conjunto de prueba.",
"_____no_output_____"
]
],
[
[
"def normalize_linear_scale(examples_dataframe):\n \"\"\"Returns a version of the input `DataFrame` that has all its features normalized linearly.\"\"\"\n processed_features = pd.DataFrame()\n processed_features[\"latitude\"] = linear_scale(examples_dataframe[\"latitude\"])\n processed_features[\"longitude\"] = linear_scale(examples_dataframe[\"longitude\"])\n processed_features[\"housing_median_age\"] = linear_scale(examples_dataframe[\"housing_median_age\"])\n processed_features[\"total_rooms\"] = linear_scale(examples_dataframe[\"total_rooms\"])\n processed_features[\"total_bedrooms\"] = linear_scale(examples_dataframe[\"total_bedrooms\"])\n processed_features[\"population\"] = linear_scale(examples_dataframe[\"population\"])\n processed_features[\"households\"] = linear_scale(examples_dataframe[\"households\"])\n processed_features[\"median_income\"] = linear_scale(examples_dataframe[\"median_income\"])\n processed_features[\"rooms_per_person\"] = linear_scale(examples_dataframe[\"rooms_per_person\"])\n return processed_features\n\nnormalized_dataframe = normalize_linear_scale(preprocess_features(california_housing_dataframe))\nnormalized_training_examples = normalized_dataframe.head(12000)\nnormalized_validation_examples = normalized_dataframe.tail(5000)\n\n_ = train_nn_regression_model(\n my_optimizer=tf.train.GradientDescentOptimizer(learning_rate=0.005),\n steps=2000,\n batch_size=50,\n hidden_units=[10, 10],\n training_examples=normalized_training_examples,\n training_targets=training_targets,\n validation_examples=normalized_validation_examples,\n validation_targets=validation_targets)",
"_____no_output_____"
]
],
[
[
" ## Tarea 2: Probar un optimizador diferente\n\n** Usa los optmizadores AdaGrad y Adam, y compara el rendimiento.**\n\nEl optimizador AdaGrad es una alternativa. La idea clave de AdaGrad es que modifica la tasa de aprendizaje de forma adaptativa para cada coeficiente de un modelo, lo cual disminuye la tasa de aprendizaje efectiva de forma monótona. Esto funciona muy bien para los problemas convexos, pero no siempre resulta ideal para el entrenamiento de redes neuronales con problemas no convexos. Puedes usar AdaGrad al especificar `AdagradOptimizer` en lugar de `GradientDescentOptimizer`. Ten en cuenta que, con AdaGrad, es posible que debas usar una tasa de aprendizaje más alta.\n\nPara los problemas de optimización no convexos, en algunas ocasiones Adam es más eficaz que AdaGrad. Para usar Adam, invoca el método `tf.train.AdamOptimizer`. Este método toma varios hiperparámetros opcionales como argumentos, pero nuestra solución solo especifica uno de estos (`learning_rate`). En un entorno de producción, debes especificar y ajustar los hiperparámetros opcionales con cuidado.",
"_____no_output_____"
]
],
[
[
"#\n# YOUR CODE HERE: Retrain the network using Adagrad and then Adam.\n#",
"_____no_output_____"
]
],
[
[
" ### Solución\n\nHaz clic más abajo para conocer la solución.",
"_____no_output_____"
],
[
" Primero, probemos AdaGrad.",
"_____no_output_____"
]
],
[
[
"_, adagrad_training_losses, adagrad_validation_losses = train_nn_regression_model(\n my_optimizer=tf.train.AdagradOptimizer(learning_rate=0.5),\n steps=500,\n batch_size=100,\n hidden_units=[10, 10],\n training_examples=normalized_training_examples,\n training_targets=training_targets,\n validation_examples=normalized_validation_examples,\n validation_targets=validation_targets)",
"_____no_output_____"
]
],
[
[
" Ahora, probemos Adam.",
"_____no_output_____"
]
],
[
[
"_, adam_training_losses, adam_validation_losses = train_nn_regression_model(\n my_optimizer=tf.train.AdamOptimizer(learning_rate=0.009),\n steps=500,\n batch_size=100,\n hidden_units=[10, 10],\n training_examples=normalized_training_examples,\n training_targets=training_targets,\n validation_examples=normalized_validation_examples,\n validation_targets=validation_targets)",
"_____no_output_____"
]
],
[
[
" Imprimamos un gráfico de métricas de pérdida en paralelo.",
"_____no_output_____"
]
],
[
[
"plt.ylabel(\"RMSE\")\nplt.xlabel(\"Periods\")\nplt.title(\"Root Mean Squared Error vs. Periods\")\nplt.plot(adagrad_training_losses, label='Adagrad training')\nplt.plot(adagrad_validation_losses, label='Adagrad validation')\nplt.plot(adam_training_losses, label='Adam training')\nplt.plot(adam_validation_losses, label='Adam validation')\n_ = plt.legend()",
"_____no_output_____"
]
],
[
[
" ## Tarea 3: Explorar métodos de normalización alternativos\n\n**Prueba alternar las normalizaciones para distintos atributos a fin de mejorar aún más el rendimiento.**\n\nSi observas detenidamente las estadísticas de resumen de los datos transformados, es posible que observes que, al realizar un ajuste lineal en algunos atributos, estos quedan agrupados cerca de `-1`.\n\nPor ejemplo, muchos atributos tienen una mediana de alrededor de `-0.8`, en lugar de `0.0`.",
"_____no_output_____"
]
],
[
[
"_ = training_examples.hist(bins=20, figsize=(18, 12), xlabelsize=2)",
"_____no_output_____"
]
],
[
[
" Es posible que obtengamos mejores resultados al elegir formas adicionales para transformar estos atributos.\n\nPor ejemplo, un ajuste logarítmico podría ayudar a algunos atributos. O bien, el recorte de los valores extremos podría hacer que el resto del ajuste sea más informativo.",
"_____no_output_____"
]
],
[
[
"def log_normalize(series):\n return series.apply(lambda x:math.log(x+1.0))\n\ndef clip(series, clip_to_min, clip_to_max):\n return series.apply(lambda x:(\n min(max(x, clip_to_min), clip_to_max)))\n\ndef z_score_normalize(series):\n mean = series.mean()\n std_dv = series.std()\n return series.apply(lambda x:(x - mean) / std_dv)\n\ndef binary_threshold(series, threshold):\n return series.apply(lambda x:(1 if x > threshold else 0))",
"_____no_output_____"
]
],
[
[
" El bloque anterior contiene algunas funciones de normalización adicionales posibles. Prueba algunas de estas o agrega otras propias.\n\nTen en cuenta que, si normalizas el objetivo, deberás anular la normalización de las predicciones para que las métricas de pérdida sean comparables.",
"_____no_output_____"
]
],
[
[
"def normalize(examples_dataframe):\n \"\"\"Returns a version of the input `DataFrame` that has all its features normalized.\"\"\"\n #\n # YOUR CODE HERE: Normalize the inputs.\n #\n pass\n\nnormalized_dataframe = normalize(preprocess_features(california_housing_dataframe))\nnormalized_training_examples = normalized_dataframe.head(12000)\nnormalized_validation_examples = normalized_dataframe.tail(5000)\n\n_ = train_nn_regression_model(\n my_optimizer=tf.train.GradientDescentOptimizer(learning_rate=0.0007),\n steps=5000,\n batch_size=70,\n hidden_units=[10, 10],\n training_examples=normalized_training_examples,\n training_targets=training_targets,\n validation_examples=normalized_validation_examples,\n validation_targets=validation_targets)",
"_____no_output_____"
]
],
[
[
" ### Solución\n\nHaz clic más abajo para conocer una solución posible.",
"_____no_output_____"
],
[
" Estas son solo algunas formas en las que podemos pensar acerca de los datos. Otras transformaciones podrían funcionar incluso mejor.\n\nLas funciones `households`, `median_income` y `total_bedrooms` aparecen todas distribuidas normalmente en un espacio logarítmico.\n\nLas funciones `latitude`, `longitude` y `housing_median_age` probablemente serían mejores si solamente se ajustaran de forma lineal, como antes.\n\nLas funciones `population`, `totalRooms` y `rooms_per_person` tienen algunos valores atípicos extremos. Parecen ser demasiado extremos como para que la normalización logarítmica resulte útil. Por lo tanto, los recortaremos en su lugar.",
"_____no_output_____"
]
],
[
[
"def normalize(examples_dataframe):\n \"\"\"Returns a version of the input `DataFrame` that has all its features normalized.\"\"\"\n processed_features = pd.DataFrame()\n\n processed_features[\"households\"] = log_normalize(examples_dataframe[\"households\"])\n processed_features[\"median_income\"] = log_normalize(examples_dataframe[\"median_income\"])\n processed_features[\"total_bedrooms\"] = log_normalize(examples_dataframe[\"total_bedrooms\"])\n \n processed_features[\"latitude\"] = linear_scale(examples_dataframe[\"latitude\"])\n processed_features[\"longitude\"] = linear_scale(examples_dataframe[\"longitude\"])\n processed_features[\"housing_median_age\"] = linear_scale(examples_dataframe[\"housing_median_age\"])\n\n processed_features[\"population\"] = linear_scale(clip(examples_dataframe[\"population\"], 0, 5000))\n processed_features[\"rooms_per_person\"] = linear_scale(clip(examples_dataframe[\"rooms_per_person\"], 0, 5))\n processed_features[\"total_rooms\"] = linear_scale(clip(examples_dataframe[\"total_rooms\"], 0, 10000))\n\n return processed_features\n\nnormalized_dataframe = normalize(preprocess_features(california_housing_dataframe))\nnormalized_training_examples = normalized_dataframe.head(12000)\nnormalized_validation_examples = normalized_dataframe.tail(5000)\n\n_ = train_nn_regression_model(\n my_optimizer=tf.train.AdagradOptimizer(learning_rate=0.15),\n steps=1000,\n batch_size=50,\n hidden_units=[10, 10],\n training_examples=normalized_training_examples,\n training_targets=training_targets,\n validation_examples=normalized_validation_examples,\n validation_targets=validation_targets)",
"_____no_output_____"
]
],
[
[
" ## Desafío opcional: Usar solo los atributos de latitud y longitud\n\n**Entrena un modelo de red neuronal que use solo latitud y longitud como atributos.**\n\nA los agentes de bienes raíces les gusta decir que la ubicación es el único atributo importante en el precio de la vivienda.\nVeamos si podemos confirmar esto al entrenar un modelo que use solo latitud y longitud como atributos.\n\nEsto funcionará bien únicamente si nuestra red neuronal puede aprender no linealidades complejas a partir de la latitud y la longitud.\n\n**NOTA:** Es posible que necesitemos una estructura de red que tenga más capas que las que eran útiles anteriormente en el ejercicio.",
"_____no_output_____"
]
],
[
[
"#\n# YOUR CODE HERE: Train the network using only latitude and longitude\n#",
"_____no_output_____"
]
],
[
[
" ### Solución\n\nHaz clic más abajo para conocer una solución posible.",
"_____no_output_____"
],
[
" Una buena idea es mantener latitud y longitud normalizadas:",
"_____no_output_____"
]
],
[
[
"def location_location_location(examples_dataframe):\n \"\"\"Returns a version of the input `DataFrame` that keeps only the latitude and longitude.\"\"\"\n processed_features = pd.DataFrame()\n processed_features[\"latitude\"] = linear_scale(examples_dataframe[\"latitude\"])\n processed_features[\"longitude\"] = linear_scale(examples_dataframe[\"longitude\"])\n return processed_features\n\nlll_dataframe = location_location_location(preprocess_features(california_housing_dataframe))\nlll_training_examples = lll_dataframe.head(12000)\nlll_validation_examples = lll_dataframe.tail(5000)\n\n_ = train_nn_regression_model(\n my_optimizer=tf.train.AdagradOptimizer(learning_rate=0.05),\n steps=500,\n batch_size=50,\n hidden_units=[10, 10, 5, 5, 5],\n training_examples=lll_training_examples,\n training_targets=training_targets,\n validation_examples=lll_validation_examples,\n validation_targets=validation_targets)",
"_____no_output_____"
]
],
[
[
" Esto no es tan malo para solo dos funciones. De todos modos, los valores de la propiedad pueden variar en distancias cortas.",
"_____no_output_____"
]
]
] | [
"markdown",
"code",
"markdown",
"code",
"markdown",
"code",
"markdown",
"code",
"markdown",
"code",
"markdown",
"code",
"markdown",
"code",
"markdown",
"code",
"markdown",
"code",
"markdown",
"code",
"markdown",
"code",
"markdown",
"code",
"markdown",
"code",
"markdown",
"code",
"markdown",
"code",
"markdown",
"code",
"markdown"
] | [
[
"markdown"
],
[
"code"
],
[
"markdown",
"markdown",
"markdown"
],
[
"code",
"code",
"code"
],
[
"markdown"
],
[
"code",
"code",
"code",
"code"
],
[
"markdown"
],
[
"code"
],
[
"markdown"
],
[
"code"
],
[
"markdown",
"markdown"
],
[
"code"
],
[
"markdown"
],
[
"code"
],
[
"markdown",
"markdown"
],
[
"code"
],
[
"markdown"
],
[
"code"
],
[
"markdown"
],
[
"code"
],
[
"markdown"
],
[
"code"
],
[
"markdown"
],
[
"code"
],
[
"markdown"
],
[
"code"
],
[
"markdown",
"markdown"
],
[
"code"
],
[
"markdown"
],
[
"code"
],
[
"markdown",
"markdown"
],
[
"code"
],
[
"markdown"
]
] |
d08d6596fd52722369fe980cf0c0d4602178ec7d | 143,743 | ipynb | Jupyter Notebook | TestClinicalDataMethod3.ipynb | taborzbislaw/DeepBeam | 7ce425895d498f975856ccf406f9396e9becdc14 | [
"MIT"
] | 4 | 2021-02-22T12:41:51.000Z | 2021-04-12T10:21:48.000Z | TestClinicalDataMethod3.ipynb | taborzbislaw/DeepBeam | 7ce425895d498f975856ccf406f9396e9becdc14 | [
"MIT"
] | null | null | null | TestClinicalDataMethod3.ipynb | taborzbislaw/DeepBeam | 7ce425895d498f975856ccf406f9396e9becdc14 | [
"MIT"
] | 1 | 2022-03-25T03:07:51.000Z | 2022-03-25T03:07:51.000Z | 348.890777 | 129,528 | 0.924024 | [
[
[
"import numpy as np\nimport matplotlib.pyplot as plt\nfrom scipy.interpolate import interpn\nimport os\n\nimport config\nimport utils",
"_____no_output_____"
],
[
"# Read measured profiles\n\nmeasuredDoseFiles10 = ['./Measured/Method3/PDD1_10x10.dat','./Measured/Method3/PDD2_10x10.dat',\n './Measured/Method3/PROF1_10x10_14mm.dat','./Measured/Method3/PROF2_10x10_14mm.dat',\n './Measured/Method3/PROF1_10x10_100mm.dat','./Measured/Method3/PROF2_10x10_100mm.dat']\n\nmeasuredDoseFiles30 = ['./Measured/Method3/PDD1_30x30.dat',\n './Measured/Method3/PROF1_30x30_14mm.dat','./Measured/Method3/PROF2_30x30_14mm.dat',\n './Measured/Method3/PROF1_30x30_100mm.dat','./Measured/Method3/PROF2_30x30_100mm.dat']\n\n\nclinicalProfiles = []\n\nxStart = [0,0,-8.1,-8.1,-8.8,-8.8]\nprofiles = []\nfor n, measuredDoseFile in enumerate(measuredDoseFiles10):\n f = open(measuredDoseFile)\n lines = f.readlines()\n f.close()\n x = np.asarray([l.split() for l in lines[:-1]],dtype=np.float)\n x[:,0] = x[:,0]/10.\n interpRange = np.arange(xStart[n],x[x.shape[0]-1,0]+config.spaceStep/2,config.spaceStep)\n profile = interpn((x[:,0],),x[:,1] , interpRange)\n print(profile.shape,interpRange.shape,profile[0],profile[profile.shape[0]-1],interpRange[0],interpRange[interpRange.shape[0]-1])\n profiles.append(profile)\n\ndum =np.zeros(config.numOfSimulatedProfileSamples,dtype=np.float)\nnp.copyto(dum[config.analyzedRanges[1][0][0]:config.analyzedRanges[1][0][1]],(profiles[0][3:]+profiles[1][3:])*0.5)\nscale = dum[12]\ndum = dum*100.0/scale\nclinicalProfiles.append(dum) #Field 10x10 depth profile from 0.3 to 30.0 (both included)\n\ndum =np.zeros(config.numOfSimulatedProfileSamples,dtype=np.float)\nnp.copyto(dum[config.analyzedRanges[1][1][0]:config.analyzedRanges[1][1][1]],0.5*(profiles[2]+profiles[3]))\ndum = dum*100.0/scale\nclinicalProfiles.append(dum) #Field 10x10 lateral profile at depth 14mm from -8.1 to 8.1 cm, both included\n\ndum =np.zeros(config.numOfSimulatedProfileSamples,dtype=np.float)\nnp.copyto(dum[config.analyzedRanges[1][2][0]:config.analyzedRanges[1][2][1]],0.5*(profiles[4]+profiles[5]))\ndum = dum*100.0/scale\nclinicalProfiles.append(dum) #Field 10x10 lateral profile at depth 100mm from -8.8 to 8.8 cm, both included\n\n\n\nxStart = [0,-18.2,-18.2,-19.7,-19.7]\nprofiles = []\nfor n, measuredDoseFile in enumerate(measuredDoseFiles30):\n f = open(measuredDoseFile)\n lines = f.readlines()\n f.close()\n x = np.asarray([l.split() for l in lines[:-1]],dtype=np.float)\n x[:,0] = x[:,0]/10.\n interpRange = np.arange(xStart[n],np.round(x[x.shape[0]-1,0],2)-config.spaceStep/2,config.spaceStep)\n profile = interpn((x[:,0],),x[:,1] , interpRange)\n print(profile.shape,interpRange.shape,interpRange[0],interpRange[interpRange.shape[0]-1])\n profiles.append(profile)\n\ndum =np.zeros(config.numOfSimulatedProfileSamples,dtype=np.float)\nnp.copyto(dum[config.analyzedRanges[2][0][0]:config.analyzedRanges[2][0][1]],profiles[0][3:])\nscale = dum[12]\ndum = dum*100/scale\nclinicalProfiles.append(dum) #Field 30x30 lateral profile at depth 1.4cm from -18.2 to 18.2 cm, both included\n\n \ndum =np.zeros(config.numOfSimulatedProfileSamples,dtype=np.float)\nnp.copyto(dum[config.analyzedRanges[2][1][0]:config.analyzedRanges[2][1][1]],0.5*(profiles[1]+profiles[2]))\ndum = dum*100/scale\nclinicalProfiles.append(dum) #Field 30x30 lateral profile at depth 1.4cm from -18.2 to 18.2 cm, both included\n\ndum =np.zeros(config.numOfSimulatedProfileSamples,dtype=np.float)\nnp.copyto(dum[config.analyzedRanges[2][2][0]:config.analyzedRanges[2][2][1]],0.5*(profiles[3]+profiles[4]))\ndum = dum*100/scale\nclinicalProfiles.append(dum) #Field 30x30 lateral profile at depth 10cm from -19.7 to 19.7 cm, both included\n \n \n#plt.figure(figsize=(10,10))\n#plt.plot(clinicalProfiles[0])\n#plt.plot(clinicalProfiles[1])\n#plt.show()\n \n#plt.figure(figsize=(10,10))\n#plt.plot(clinicalProfiles[2],'r-') \n#plt.plot(clinicalProfiles[3],'g-') \n#plt.show()\n",
"(301,) (301,) 0.55861 0.1793 0.0 30.0\n(301,) (301,) 0.55512 0.17927 0.0 30.0\n(163,) (163,) 0.028336502958579892 0.02790683037475391 -8.1 8.099999999999943\n(163,) (163,) 0.019935102564102573 0.020378368836292318 -8.1 8.099999999999943\n(177,) (177,) 0.030209 0.029615000000000558 -8.8 8.799999999999937\n(177,) (177,) 0.025551 0.025791000000000546 -8.8 8.799999999999937\n(300,) (300,) 0.0 29.900000000000002\n(365,) (365,) -18.2 18.200000000000518\n(365,) (365,) -18.2 18.200000000000518\n(395,) (395,) -19.7 19.70000000000056\n(395,) (395,) -19.7 19.70000000000056\n"
],
[
"\nmeans = np.load(config.modelDIR + config.meansFileName)\nprint(means.shape,clinicalProfiles[0].shape) #(3, 6, 487) (487,)\n\ndiffTest = np.zeros((3,1,6,config.numOfSimulatedProfileSamples),dtype=np.float)\n\n#Field 10\ndiff = clinicalProfiles[0] - means[1,0]\nnp.copyto(diffTest[1,0,0,:],diff)\ndiff = clinicalProfiles[1] - means[1,1]\nnp.copyto(diffTest[1,0,1,:],diff)\ndiff = clinicalProfiles[2] - means[1,3]\nnp.copyto(diffTest[1,0,3,:],diff)\n\n#Field 30\ndiff = clinicalProfiles[3] - means[2,0]\nnp.copyto(diffTest[2,0,0,:],diff)\ndiff = clinicalProfiles[4] - means[2,1]\nnp.copyto(diffTest[2,0,1,:],diff)\ndiff = clinicalProfiles[5] - means[2,3]\nnp.copyto(diffTest[2,0,3,:],diff)\n\nprint(diffTest.shape)\n",
"(3, 6, 495) (495,)\n(3, 1, 6, 495)\n"
],
[
"from sklearn.decomposition import PCA\nimport pickle\n\n\ntestFeatures = []\n\nfor nfield,(field,Ranges) in enumerate(zip(config.analyzedProfiles,config.analyzedRanges)):\n if field != None:\n for profile,Range in zip(field,Ranges):\n print(nfield,profile)\n pcaName = config.modelDIR + 'PCA_' + str(nfield) + '_' + str(profile) + '_.pkl'\n pca = pickle.load(open(pcaName,'rb'))\n\n X = diffTest[nfield][:,profile,Range[0]:Range[1]]\n X_projected = pca.transform(X)\n testFeatures.append(X_projected)\n\nX_test = np.stack(testFeatures)\nX_test = np.swapaxes(X_test,1,0)\nX_test = np.reshape(X_test,(X_test.shape[0],X_test.shape[1]*X_test.shape[2])) \nprint(X_test.shape)\n",
"1 0\n1 1\n1 3\n2 0\n2 1\n2 3\n(1, 18)\n"
],
[
"import matplotlib.pyplot as plt\nfrom sklearn.svm import SVR\nfrom sklearn.model_selection import GridSearchCV\n\n\npreds = []\nfor goal in [0,1,2,3]:\n modelName = config.modelDIR + 'SVR_' + str(goal) + '_.pkl'\n clf = pickle.load(open(modelName,'rb'))\n predTest = clf.predict(X_test)\n preds.append(predTest[0])\n \nprint(preds)",
"[5.426658586640102, 0.5, 0.23916935470589895, 1.950654954002582]\n"
],
[
"allMeans,allFieldFeatures,allFieldPCAModels = utils.allPCAResults()",
"_____no_output_____"
],
[
"\nrecons = utils.reconstruct(preds,allMeans,allFieldFeatures,allFieldPCAModels)\nprint(preds)\nprint(utils.difference(preds,clinicalProfiles,allMeans,allFieldFeatures,allFieldPCAModels)) \n",
"[5.426658586640102, 0.5, 0.23916935470589895, 1.950654954002582]\n1970.848051638377\n"
],
[
"# Optimize solution\n# https://docs.scipy.org/doc/scipy/reference/tutorial/optimize.html\n# https://scipy-lectures.org/advanced/mathematical_optimization/auto_examples/plot_non_bounds_constraints.html\n\npreds = [5.62,0.5,0.27, 2.46] # from DeepBeam\n\nimport scipy.optimize as opt\nfrom scipy.optimize import SR1\n\ndef fun(cP,aM,aF,aPCA):\n def diff(y):\n return utils.difference(y,cP,aM,aF,aPCA)\n return diff\n\ndifference = fun(clinicalProfiles,allMeans,allFieldFeatures,allFieldPCAModels)\n\nres = opt.minimize(difference, preds, method='SLSQP', jac=\"2-point\",\n options={'ftol': 1e-9, 'disp': True},\n bounds=config.bounds)\nprint(res.x)",
"Optimization terminated successfully (Exit mode 0)\n Current function value: 1213.8140260451298\n Iterations: 8\n Function evaluations: 46\n Gradient evaluations: 8\n[5.6 0.5 0.23812682 2.40521929]\n"
]
],
[
[
"Optimization terminated successfully (Exit mode 0)\n Current function value: 1213.8140260451162\n Iterations: 12\n Function evaluations: 65\n Gradient evaluations: 12\n[5.6 0.5 0.23812683 2.4052193 ]\n",
"_____no_output_____"
]
],
[
[
"recons = utils.reconstruct(res.x,allMeans,allFieldFeatures,allFieldPCAModels)",
"_____no_output_____"
],
[
"plt.rcParams.update({'font.size': 18})\n\nfig, (axs1,axs2) = plt.subplots(1, 2,figsize = (20,10))\n\nfor n in [0,3]:\n if n==0:\n axs1.plot(np.arange(0.3,30.05,0.1),clinicalProfiles[n][config.allRanges[n][0]:config.allRanges[n][1]],'r--',label='real profiles')\n axs1.plot(np.arange(0.3,49.75,0.1),recons[n],'g-',label='predicted profiles')\n else:\n axs1.plot(np.arange(0.3,29.95,0.1),clinicalProfiles[n][config.allRanges[n][0]:config.allRanges[n][1]],'r--')\n axs1.plot(np.arange(0.3,49.75,0.1),recons[n],'g-')\n \naxs1.set(xlabel = 'depth [cm]',ylabel = '% of maximal dose')\naxs1.legend(loc='upper right')\n\n \nfor n in [1,2,4,5]:\n start = config.allRanges[n][0]*0.1 -24.7\n end = config.allRanges[n][1]*0.1 - 24.7 - 0.05\n if n==1:\n axs2.plot(np.arange(start,end,0.1),clinicalProfiles[n][config.allRanges[n][0]:config.allRanges[n][1]],'r--',label='real profiles')\n axs2.plot(np.arange(-24.7,24.75,0.1),recons[n],'g-',label='predicted profiles')\n else:\n axs2.plot(np.arange(start,end,0.1),clinicalProfiles[n][config.allRanges[n][0]:config.allRanges[n][1]],'r--')\n axs2.plot(np.arange(-24.7,24.75,0.1),recons[n],'g-')\n\naxs2.set(xlabel = 'off axis distance [cm]',ylabel = '% of maximal dose')\naxs2.legend(loc='lower right')\nplt.savefig('results3')",
"_____no_output_____"
]
]
] | [
"code",
"raw",
"code"
] | [
[
"code",
"code",
"code",
"code",
"code",
"code",
"code",
"code"
],
[
"raw"
],
[
"code",
"code"
]
] |
d08d7a37b8b2121789b6b823e1423343a3e91d51 | 296,751 | ipynb | Jupyter Notebook | module06_cifar.ipynb | Teradater/Neural_Networks_and_CV | 16238682726a2c227d61c50b040433b35a5a9dda | [
"MIT"
] | null | null | null | module06_cifar.ipynb | Teradater/Neural_Networks_and_CV | 16238682726a2c227d61c50b040433b35a5a9dda | [
"MIT"
] | null | null | null | module06_cifar.ipynb | Teradater/Neural_Networks_and_CV | 16238682726a2c227d61c50b040433b35a5a9dda | [
"MIT"
] | null | null | null | 314.022222 | 60,010 | 0.9138 | [
[
[
"",
"_____no_output_____"
],
[
"",
"_____no_output_____"
]
],
[
[
"import torch\nimport random\nimport numpy as np\n\nrandom.seed(0)\nnp.random.seed(0)\ntorch.manual_seed(0)\ntorch.cuda.manual_seed(0)\ntorch.backends.cudnn.deterministic = True",
"_____no_output_____"
],
[
"import torchvision.datasets",
"_____no_output_____"
],
[
"CIFAR_train = torchvision.datasets.CIFAR10('./', download=True, train=True)\nCIFAR_test = torchvision.datasets.CIFAR10('./', download=True, train=False)",
"Downloading https://www.cs.toronto.edu/~kriz/cifar-10-python.tar.gz to ./cifar-10-python.tar.gz\n"
],
[
"X_train = torch.FloatTensor(CIFAR_train.data)\ny_train = torch.LongTensor(CIFAR_train.targets)\nX_test = torch.FloatTensor(CIFAR_test.data)\ny_test = torch.LongTensor(CIFAR_test.targets)",
"_____no_output_____"
],
[
"len(y_train), len(y_test)",
"_____no_output_____"
],
[
"X_train.min(), X_train.max()",
"_____no_output_____"
],
[
"X_train /= 255.\nX_test /= 255.",
"_____no_output_____"
],
[
"CIFAR_train.classes",
"_____no_output_____"
],
[
"import matplotlib.pyplot as plt\nplt.figure(figsize=(20,2))\nfor i in range(10):\n plt.subplot(1, 10, i+1)\n plt.imshow(X_train[i])\n print(y_train[i], end=' ')",
"tensor(6) tensor(9) tensor(9) tensor(4) tensor(1) tensor(1) tensor(2) tensor(7) tensor(8) tensor(3) "
],
[
"X_train.shape, y_train.shape",
"_____no_output_____"
],
[
"X_train = X_train.permute(0, 3, 1, 2)\nX_test = X_test.permute(0, 3, 1, 2)",
"_____no_output_____"
],
[
"X_train.shape",
"_____no_output_____"
],
[
"class LeNet5(torch.nn.Module):\n def __init__(self,\n activation='tanh',\n pooling='avg',\n conv_size=5, \n use_batch_norm=False):\n super(LeNet5, self).__init__()\n \n self.conv_size = conv_size\n self.use_batch_norm = use_batch_norm\n \n if activation == 'tanh':\n activation_function = torch.nn.Tanh()\n elif activation == 'relu':\n activation_function = torch.nn.ReLU()\n else:\n raise NotImplementedError\n \n if pooling == 'avg':\n pooling_layer = torch.nn.AvgPool2d(kernel_size=2, stride=2)\n elif pooling == 'max':\n pooling_layer = torch.nn.MaxPool2d(kernel_size=2, stride=2)\n else:\n raise NotImplementedError\n \n if conv_size == 5:\n self.conv1 = torch.nn.Conv2d(\n in_channels=3, out_channels=6, kernel_size=5, padding=0)\n elif conv_size == 3:\n self.conv1_1 = torch.nn.Conv2d(\n in_channels=3, out_channels=6, kernel_size=3, padding=0)\n self.conv1_2 = torch.nn.Conv2d(\n in_channels=6, out_channels=6, kernel_size=3, padding=0)\n else:\n raise NotImplementedError\n\n self.act1 = activation_function\n self.bn1 = torch.nn.BatchNorm2d(num_features=6)\n self.pool1 = pooling_layer\n \n if conv_size == 5:\n self.conv2 = self.conv2 = torch.nn.Conv2d(\n in_channels=6, out_channels=16, kernel_size=5, padding=0)\n elif conv_size == 3:\n self.conv2_1 = torch.nn.Conv2d(\n in_channels=6, out_channels=16, kernel_size=3, padding=0)\n self.conv2_2 = torch.nn.Conv2d(\n in_channels=16, out_channels=16, kernel_size=3, padding=0)\n else:\n raise NotImplementedError\n\n self.act2 = activation_function\n self.bn2 = torch.nn.BatchNorm2d(num_features=16)\n self.pool2 = pooling_layer\n \n self.fc1 = torch.nn.Linear(5 * 5 * 16, 120)\n self.act3 = activation_function\n \n self.fc2 = torch.nn.Linear(120, 84)\n self.act4 = activation_function\n \n self.fc3 = torch.nn.Linear(84, 10)\n \n def forward(self, x):\n if self.conv_size == 5:\n x = self.conv1(x)\n elif self.conv_size == 3:\n x = self.conv1_2(self.conv1_1(x))\n x = self.act1(x)\n if self.use_batch_norm:\n x = self.bn1(x)\n x = self.pool1(x)\n \n if self.conv_size == 5:\n x = self.conv2(x)\n elif self.conv_size == 3:\n x = self.conv2_2(self.conv2_1(x))\n x = self.act2(x)\n if self.use_batch_norm:\n x = self.bn2(x)\n x = self.pool2(x)\n \n x = x.view(x.size(0), x.size(1) * x.size(2) * x.size(3))\n x = self.fc1(x)\n x = self.act3(x)\n x = self.fc2(x)\n x = self.act4(x)\n x = self.fc3(x)\n \n return x",
"_____no_output_____"
],
[
"def train(net, X_train, y_train, X_test, y_test):\n device = torch.device('cuda:0' if torch.cuda.is_available() else 'cpu')\n net = net.to(device)\n loss = torch.nn.CrossEntropyLoss()\n optimizer = torch.optim.Adam(net.parameters(), lr=1.0e-3)\n \n batch_size = 100\n\n test_accuracy_history = []\n test_loss_history = []\n\n X_test = X_test.to(device)\n y_test = y_test.to(device)\n\n for epoch in range(30):\n order = np.random.permutation(len(X_train))\n for start_index in range(0, len(X_train), batch_size):\n optimizer.zero_grad()\n net.train()\n\n batch_indexes = order[start_index:start_index+batch_size]\n\n X_batch = X_train[batch_indexes].to(device)\n y_batch = y_train[batch_indexes].to(device)\n\n preds = net.forward(X_batch)\n\n loss_value = loss(preds, y_batch)\n loss_value.backward()\n\n optimizer.step()\n \n X_batch\n\n net.eval()\n test_preds = net.forward(X_test)\n test_loss_history.append(loss(test_preds, y_test).data.cpu())\n\n accuracy = (test_preds.argmax(dim=1) == y_test).float().mean().data.cpu()\n test_accuracy_history.append(accuracy)\n\n print(accuracy)\n del net\n return test_accuracy_history, test_loss_history\n\naccuracies = {}\nlosses = {}\n\naccuracies['tanh'], losses['tanh'] = \\\n train(LeNet5(activation='tanh', conv_size=5),\n X_train, y_train, X_test, y_test)\naccuracies['relu'], losses['relu'] = \\\n train(LeNet5(activation='relu', conv_size=5),\n X_train, y_train, X_test, y_test)\naccuracies['relu_3'], losses['relu_3'] = \\\n train(LeNet5(activation='relu', conv_size=3),\n X_train, y_train, X_test, y_test)\naccuracies['relu_3_max_pool'], losses['relu_3_max_pool'] = \\\n train(LeNet5(activation='relu', conv_size=3, pooling='max'), \n X_train, y_train, X_test, y_test)\naccuracies['relu_3_max_pool_bn'], losses['relu_3_max_pool_bn'] = \\\n train(LeNet5(activation='relu', conv_size=3, pooling='max', use_batch_norm=True), \n X_train, y_train, X_test, y_test)",
"_____no_output_____"
],
[
"for experiment_id in accuracies.keys():\n plt.plot(accuracies[experiment_id], label=experiment_id)\nplt.legend()\nplt.title('Validation Accuracy');",
"_____no_output_____"
],
[
"for experiment_id in losses.keys():\n plt.plot(losses[experiment_id], label=experiment_id)\nplt.legend()\nplt.title('Validation Loss');",
"_____no_output_____"
]
],
[
[
"## Выводы\nХаки начинают работать в отличие от мниста\n- Здорово помогает макспулинг\n- Батчнорм - пушка, но и переобучение намного раньше.\n\n# Как сделать еще лучше? \nЛеНет хорошо работал для 1 канала, а для 3х каналов маловато фильтров в свертках. Исправим это",
"_____no_output_____"
]
],
[
[
"class CIFARNet(torch.nn.Module):\n def __init__(self):\n super(CIFARNet, self).__init__()\n self.batch_norm0 = torch.nn.BatchNorm2d(3)\n\n self.conv1 = torch.nn.Conv2d(3, 16, 3, padding=1)\n self.act1 = torch.nn.ReLU()\n self.batch_norm1 = torch.nn.BatchNorm2d(16)\n self.pool1 = torch.nn.MaxPool2d(2, 2)\n \n self.conv2 = torch.nn.Conv2d(16, 32, 3, padding=1)\n self.act2 = torch.nn.ReLU()\n self.batch_norm2 = torch.nn.BatchNorm2d(32)\n self.pool2 = torch.nn.MaxPool2d(2, 2)\n \n self.conv3 = torch.nn.Conv2d(32, 64, 3, padding=1)\n self.act3 = torch.nn.ReLU()\n self.batch_norm3 = torch.nn.BatchNorm2d(64)\n\n self.fc1 = torch.nn.Linear(8 * 8 * 64, 256)\n self.act4 = torch.nn.Tanh()\n self.batch_norm4 = torch.nn.BatchNorm1d(256)\n \n self.fc2 = torch.nn.Linear(256, 64)\n self.act5 = torch.nn.Tanh()\n self.batch_norm5 = torch.nn.BatchNorm1d(64)\n \n self.fc3 = torch.nn.Linear(64, 10)\n \n def forward(self, x):\n x = self.batch_norm0(x)\n x = self.conv1(x)\n x = self.act1(x)\n x = self.batch_norm1(x)\n x = self.pool1(x)\n \n x = self.conv2(x)\n x = self.act2(x)\n x = self.batch_norm2(x)\n x = self.pool2(x)\n \n x = self.conv3(x)\n x = self.act3(x)\n x = self.batch_norm3(x)\n \n x = x.view(x.size(0), x.size(1) * x.size(2) * x.size(3))\n x = self.fc1(x)\n x = self.act4(x)\n x = self.batch_norm4(x)\n x = self.fc2(x)\n x = self.act5(x)\n x = self.batch_norm5(x)\n x = self.fc3(x)\n \n return x",
"_____no_output_____"
],
[
"accuracies['cifar_net'], losses['cifar_net'] = \\\n train(CIFARNet(), X_train, y_train, X_test, y_test)",
"_____no_output_____"
],
[
"for experiment_id in accuracies.keys():\n plt.plot(accuracies[experiment_id], label=experiment_id)\nplt.legend()\nplt.title('Validation Accuracy');",
"_____no_output_____"
],
[
"for experiment_id in losses.keys():\n plt.plot(losses[experiment_id], label=experiment_id)\nplt.legend()\nplt.title('Validation Loss');",
"_____no_output_____"
]
]
] | [
"markdown",
"code",
"markdown",
"code"
] | [
[
"markdown",
"markdown"
],
[
"code",
"code",
"code",
"code",
"code",
"code",
"code",
"code",
"code",
"code",
"code",
"code",
"code",
"code",
"code",
"code"
],
[
"markdown"
],
[
"code",
"code",
"code",
"code"
]
] |
d08d8030950f1eb49ed1ede0efcdbc25f93fd09d | 426,340 | ipynb | Jupyter Notebook | Starter_Code/.ipynb_checkpoints/crypto_sentiment-checkpoint.ipynb | Aljjohara/NLP | 932078644f5afdc1907e8dc1e1966fd9f741af4a | [
"ADSL"
] | null | null | null | Starter_Code/.ipynb_checkpoints/crypto_sentiment-checkpoint.ipynb | Aljjohara/NLP | 932078644f5afdc1907e8dc1e1966fd9f741af4a | [
"ADSL"
] | null | null | null | Starter_Code/.ipynb_checkpoints/crypto_sentiment-checkpoint.ipynb | Aljjohara/NLP | 932078644f5afdc1907e8dc1e1966fd9f741af4a | [
"ADSL"
] | null | null | null | 161.553619 | 166,836 | 0.837386 | [
[
[
"import os\nimport pandas as pd\nfrom newsapi import NewsApiClient\n%matplotlib inline",
"_____no_output_____"
],
[
"from nltk.sentiment.vader import SentimentIntensityAnalyzer\nanalyzer = SentimentIntensityAnalyzer()",
"_____no_output_____"
]
],
[
[
"# News Headlines Sentiment\n\nUse the news api to pull the latest news articles for bitcoin and ethereum and create a DataFrame of sentiment scores for each coin. \n\nUse descriptive statistics to answer the following questions:\n1. Which coin had the highest mean positive score?\n2. Which coin had the highest negative score?\n3. Which coin had the highest positive score?",
"_____no_output_____"
]
],
[
[
"# Read your api key environment variable\n\napi_key = os.getenv(\"news_api\")",
"_____no_output_____"
],
[
"# Create a newsapi client\nnewsapi = NewsApiClient(api_key=api_key)",
"_____no_output_____"
],
[
"# Fetch the Bitcoin news articles\nbitcoin_news_en = newsapi.get_everything(\n q=\"Bitcoin\",\n language=\"en\",\n sort_by=\"relevancy\"\n)\n\n# Show the total number of news\nbitcoin_news_en[\"totalResults\"]\n",
"_____no_output_____"
],
[
"# Fetch the Ethereum news articles\n# Fetch the Bitcoin news articles\nethereum_news_en = newsapi.get_everything(\n q=\"Ethereum\",\n language=\"en\",\n sort_by=\"relevancy\"\n)\n\n# Show the total number of news\nethereum_news_en[\"totalResults\"]",
"_____no_output_____"
],
[
"# Create the Bitcoin sentiment scores DataFrame\nbitcoin_sentiments = []\n\nfor article in bitcoin_news_en[\"articles\"]:\n try:\n text = article[\"content\"]\n sentiment = analyzer.polarity_scores(text)\n compound = sentiment[\"compound\"]\n pos = sentiment[\"pos\"]\n neu = sentiment[\"neu\"]\n neg = sentiment[\"neg\"]\n \n bitcoin_sentiments.append({\n \"text\": text,\n \"compound\": compound,\n \"positive\": pos,\n \"negative\": neg,\n \"neutral\": neu\n \n })\n \n except AttributeError:\n pass\n \n# Create DataFrame\nbitcoin_df = pd.DataFrame(bitcoin_sentiments)\n# Reorder DataFrame columns\ncols = [ \"compound\",\"negative\", \"neutral\", \"positive\", \"text\"]\nbitcoin_df = bitcoin_df[cols]\n\nbitcoin_df.head()",
"_____no_output_____"
],
[
"# Create the ethereum sentiment scores DataFrame\n\nethereum_sentiments = []\n\nfor article in ethereum_news_en[\"articles\"]:\n try:\n text = article[\"content\"]\n sentiment = analyzer.polarity_scores(text)\n compound = sentiment[\"compound\"]\n pos = sentiment[\"pos\"]\n neu = sentiment[\"neu\"]\n neg = sentiment[\"neg\"]\n \n ethereum_sentiments.append({\n \"text\": text,\n \"compound\": compound,\n \"positive\": pos,\n \"negative\": neg,\n \"neutral\": neu\n \n })\n \n except AttributeError:\n pass\n \n# Create DataFrame\nethereum_df = pd.DataFrame(ethereum_sentiments)\n# Reorder DataFrame columns\ncols = [ \"compound\",\"negative\", \"neutral\", \"positive\", \"text\"]\nethereum_df = ethereum_df[cols]\n\nethereum_df.head()",
"_____no_output_____"
],
[
"# Describe the Bitcoin Sentiment\nbitcoin_df.describe()",
"_____no_output_____"
],
[
"# Describe the Ethereum Sentiment\nethereum_df.describe()",
"_____no_output_____"
]
],
[
[
"### Questions:\n\nQ: Which coin had the highest mean positive score?\n\nA: Bitcoin with 0.067400\n\nQ: Which coin had the highest compound score?\n\nA: Bitcoin with 0.310145\n\nQ. Which coin had the highest positive score?\n\nA: Ethereum with 0.335000",
"_____no_output_____"
],
[
"---",
"_____no_output_____"
],
[
"# Tokenizer\n\nIn this section, you will use NLTK and Python to tokenize the text for each coin. Be sure to:\n1. Lowercase each word\n2. Remove Punctuation\n3. Remove Stopwords",
"_____no_output_____"
]
],
[
[
"from nltk.tokenize import word_tokenize, sent_tokenize\nfrom nltk.corpus import stopwords\nfrom nltk.stem import WordNetLemmatizer, PorterStemmer\nfrom string import punctuation\nimport re\nimport nltk\n",
"_____no_output_____"
],
[
"# Expand the default stopwords list if necessary\nnltk.download(\"punkt\")\nnltk.download('stopwords')\nprint(stopwords.words('english'))\n",
"['i', 'me', 'my', 'myself', 'we', 'our', 'ours', 'ourselves', 'you', \"you're\", \"you've\", \"you'll\", \"you'd\", 'your', 'yours', 'yourself', 'yourselves', 'he', 'him', 'his', 'himself', 'she', \"she's\", 'her', 'hers', 'herself', 'it', \"it's\", 'its', 'itself', 'they', 'them', 'their', 'theirs', 'themselves', 'what', 'which', 'who', 'whom', 'this', 'that', \"that'll\", 'these', 'those', 'am', 'is', 'are', 'was', 'were', 'be', 'been', 'being', 'have', 'has', 'had', 'having', 'do', 'does', 'did', 'doing', 'a', 'an', 'the', 'and', 'but', 'if', 'or', 'because', 'as', 'until', 'while', 'of', 'at', 'by', 'for', 'with', 'about', 'against', 'between', 'into', 'through', 'during', 'before', 'after', 'above', 'below', 'to', 'from', 'up', 'down', 'in', 'out', 'on', 'off', 'over', 'under', 'again', 'further', 'then', 'once', 'here', 'there', 'when', 'where', 'why', 'how', 'all', 'any', 'both', 'each', 'few', 'more', 'most', 'other', 'some', 'such', 'no', 'nor', 'not', 'only', 'own', 'same', 'so', 'than', 'too', 'very', 's', 't', 'can', 'will', 'just', 'don', \"don't\", 'should', \"should've\", 'now', 'd', 'll', 'm', 'o', 're', 've', 'y', 'ain', 'aren', \"aren't\", 'couldn', \"couldn't\", 'didn', \"didn't\", 'doesn', \"doesn't\", 'hadn', \"hadn't\", 'hasn', \"hasn't\", 'haven', \"haven't\", 'isn', \"isn't\", 'ma', 'mightn', \"mightn't\", 'mustn', \"mustn't\", 'needn', \"needn't\", 'shan', \"shan't\", 'shouldn', \"shouldn't\", 'wasn', \"wasn't\", 'weren', \"weren't\", 'won', \"won't\", 'wouldn', \"wouldn't\"]\n"
],
[
"#nltk.download(\"punkt\")\n\nsw = set(stopwords.words('english'))|set(punctuation)\nsw_addon = {'then', 'example', 'another'}\nsw = sw.union(sw_addon)",
"_____no_output_____"
],
[
"# Complete the tokenizer function\nnltk.download('wordnet')\nlemmatizer = WordNetLemmatizer()\n \n\n\n\"\"\"Tokenizes text.\"\"\"\ndef tokenizer(text):\n \n regex = re.compile(\"[^a-zA-Z ]\")\n # Remove the punctuation\n re_clean = regex.sub(' ', text)\n \n # Create a list of the words\n words = word_tokenize(re_clean)\n # Convert the words to lowercase\n \n # Remove the stop words\n words = [word.lower() for word in words if word.lower() not in sw]\n # Lemmatize Words into root words\n tokens = [lemmatizer.lemmatize(word) for word in words]\n \n return tokens",
"[nltk_data] Downloading package wordnet to\n[nltk_data] /Users/aljohara/nltk_data...\n[nltk_data] Package wordnet is already up-to-date!\n"
],
[
"# Create a new tokens column for bitcoin\n\ntokenized_bitcoin = []\nfor text in bitcoin_df['text']:\n tokenized = tokenizer(text)\n tokenized_bitcoin.append(tokenized)\nbitcoin_df[\"tokens\"] = tokenized_bitcoin\nbitcoin_df.head()",
"_____no_output_____"
],
[
"# Create a new tokens column for ethereum\ntokenized_ethereum = []\nfor text in ethereum_df['text']:\n tokenized = tokenizer(text)\n tokenized_ethereum.append(tokenized)\nethereum_df[\"tokens\"] = tokenized_ethereum\nethereum_df.head()",
"_____no_output_____"
]
],
[
[
"---",
"_____no_output_____"
],
[
"# NGrams and Frequency Analysis\n\nIn this section you will look at the ngrams and word frequency for each coin. \n\n1. Use NLTK to produce the n-grams for N = 2. \n2. List the top 10 words for each coin. ",
"_____no_output_____"
]
],
[
[
"from collections import Counter\nfrom nltk import ngrams",
"_____no_output_____"
],
[
"# Generate the Bitcoin N-grams where N=2\nall_bigrams_bitcoin = []\nfor tokens in bitcoin_df['tokens']:\n bigrams = list(ngrams(tokens,n=2))\n all_bigrams_bitcoin += bigrams",
"_____no_output_____"
],
[
"Counter(all_bigrams_bitcoin).most_common()[:10]",
"_____no_output_____"
],
[
"# Generate the Ethereum N-grams where N=2\nall_bigrams_eth = []\nfor tokens in ethereum_df['tokens']:\n bigrams = list(ngrams(tokens,n=2))\n all_bigrams_eth += bigrams",
"_____no_output_____"
],
[
"Counter(all_bigrams_eth).most_common()[:10]",
"_____no_output_____"
],
[
"# Use the token_count function to generate the top 10 words from each coin\ndef token_count(tokens, N=10):\n \"\"\"Returns the top N tokens from the frequency count\"\"\"\n return Counter(tokens).most_common(N)",
"_____no_output_____"
],
[
"# Get the top 10 words for Bitcoin\nall_tokens_bitcoin = []\nfor tokens in bitcoin_df['tokens']:\n tokens = list(ngrams(tokens,n=1))\n all_tokens_bitcoin += [token[0] for token in tokens]\ntoken_count(all_tokens_bitcoin)",
"_____no_output_____"
],
[
"# Get the top 10 words for Ethereum\nall_tokens_eth = []\nfor tokens in ethereum_df['tokens']:\n tokens = list(ngrams(tokens,n=1))\n all_tokens_eth += [token[0] for token in tokens]\ntoken_count(all_tokens_eth)",
"_____no_output_____"
]
],
[
[
"# Word Clouds\n\nIn this section, you will generate word clouds for each coin to summarize the news for each coin",
"_____no_output_____"
]
],
[
[
"from wordcloud import WordCloud\nimport matplotlib.pyplot as plt\nplt.style.use('seaborn-whitegrid')\nimport matplotlib as mpl\nmpl.rcParams['figure.figsize'] = [20.0, 10.0]",
"_____no_output_____"
],
[
"# Generate the Bitcoin word cloud\nwc = WordCloud().generate(' '.join(all_tokens_bitcoin))\nplt.imshow(wc)",
"_____no_output_____"
],
[
"# Generate the Ethereum word cloud\nwc = WordCloud().generate(' '.join(all_tokens_eth))\nplt.imshow(wc)",
"_____no_output_____"
]
],
[
[
"# Named Entity Recognition\n\nIn this section, you will build a named entity recognition model for both coins and visualize the tags using SpaCy.",
"_____no_output_____"
]
],
[
[
"import spacy\nfrom spacy import displacy",
"_____no_output_____"
],
[
"# Optional - download a language model for SpaCy\n!python -m spacy download en_core_web_sm",
"Requirement already satisfied: en_core_web_sm==2.2.5 from https://github.com/explosion/spacy-models/releases/download/en_core_web_sm-2.2.5/en_core_web_sm-2.2.5.tar.gz#egg=en_core_web_sm==2.2.5 in /Users/aljohara/opt/anaconda3/envs/mlenv/lib/python3.7/site-packages (2.2.5)\nRequirement already satisfied: spacy>=2.2.2 in /Users/aljohara/opt/anaconda3/envs/mlenv/lib/python3.7/site-packages (from en_core_web_sm==2.2.5) (2.2.4)\nRequirement already satisfied: wasabi<1.1.0,>=0.4.0 in /Users/aljohara/opt/anaconda3/envs/mlenv/lib/python3.7/site-packages (from spacy>=2.2.2->en_core_web_sm==2.2.5) (0.6.0)\nRequirement already satisfied: srsly<1.1.0,>=1.0.2 in /Users/aljohara/opt/anaconda3/envs/mlenv/lib/python3.7/site-packages (from spacy>=2.2.2->en_core_web_sm==2.2.5) (1.0.2)\nRequirement already satisfied: tqdm<5.0.0,>=4.38.0 in /Users/aljohara/opt/anaconda3/envs/mlenv/lib/python3.7/site-packages (from spacy>=2.2.2->en_core_web_sm==2.2.5) (4.46.0)\nRequirement already satisfied: murmurhash<1.1.0,>=0.28.0 in /Users/aljohara/opt/anaconda3/envs/mlenv/lib/python3.7/site-packages (from spacy>=2.2.2->en_core_web_sm==2.2.5) (1.0.0)\nRequirement already satisfied: requests<3.0.0,>=2.13.0 in /Users/aljohara/opt/anaconda3/envs/mlenv/lib/python3.7/site-packages (from spacy>=2.2.2->en_core_web_sm==2.2.5) (2.21.0)\nRequirement already satisfied: setuptools in /Users/aljohara/opt/anaconda3/envs/mlenv/lib/python3.7/site-packages (from spacy>=2.2.2->en_core_web_sm==2.2.5) (46.4.0.post20200518)\nRequirement already satisfied: thinc==7.4.0 in /Users/aljohara/opt/anaconda3/envs/mlenv/lib/python3.7/site-packages (from spacy>=2.2.2->en_core_web_sm==2.2.5) (7.4.0)\nRequirement already satisfied: blis<0.5.0,>=0.4.0 in /Users/aljohara/opt/anaconda3/envs/mlenv/lib/python3.7/site-packages (from spacy>=2.2.2->en_core_web_sm==2.2.5) (0.4.1)\nRequirement already satisfied: plac<1.2.0,>=0.9.6 in /Users/aljohara/opt/anaconda3/envs/mlenv/lib/python3.7/site-packages (from spacy>=2.2.2->en_core_web_sm==2.2.5) (0.9.6)\nRequirement already satisfied: preshed<3.1.0,>=3.0.2 in /Users/aljohara/opt/anaconda3/envs/mlenv/lib/python3.7/site-packages (from spacy>=2.2.2->en_core_web_sm==2.2.5) (3.0.2)\nRequirement already satisfied: numpy>=1.15.0 in /Users/aljohara/opt/anaconda3/envs/mlenv/lib/python3.7/site-packages (from spacy>=2.2.2->en_core_web_sm==2.2.5) (1.18.1)\nRequirement already satisfied: catalogue<1.1.0,>=0.0.7 in /Users/aljohara/opt/anaconda3/envs/mlenv/lib/python3.7/site-packages (from spacy>=2.2.2->en_core_web_sm==2.2.5) (1.0.0)\nRequirement already satisfied: cymem<2.1.0,>=2.0.2 in /Users/aljohara/opt/anaconda3/envs/mlenv/lib/python3.7/site-packages (from spacy>=2.2.2->en_core_web_sm==2.2.5) (2.0.3)\nRequirement already satisfied: chardet<3.1.0,>=3.0.2 in /Users/aljohara/opt/anaconda3/envs/mlenv/lib/python3.7/site-packages (from requests<3.0.0,>=2.13.0->spacy>=2.2.2->en_core_web_sm==2.2.5) (3.0.4)\nRequirement already satisfied: urllib3<1.25,>=1.21.1 in /Users/aljohara/opt/anaconda3/envs/mlenv/lib/python3.7/site-packages (from requests<3.0.0,>=2.13.0->spacy>=2.2.2->en_core_web_sm==2.2.5) (1.24.3)\nRequirement already satisfied: certifi>=2017.4.17 in /Users/aljohara/opt/anaconda3/envs/mlenv/lib/python3.7/site-packages (from requests<3.0.0,>=2.13.0->spacy>=2.2.2->en_core_web_sm==2.2.5) (2020.4.5.1)\nRequirement already satisfied: idna<2.9,>=2.5 in /Users/aljohara/opt/anaconda3/envs/mlenv/lib/python3.7/site-packages (from requests<3.0.0,>=2.13.0->spacy>=2.2.2->en_core_web_sm==2.2.5) (2.8)\nRequirement already satisfied: importlib-metadata>=0.20; python_version < \"3.8\" in /Users/aljohara/opt/anaconda3/envs/mlenv/lib/python3.7/site-packages (from catalogue<1.1.0,>=0.0.7->spacy>=2.2.2->en_core_web_sm==2.2.5) (1.6.0)\nRequirement already satisfied: zipp>=0.5 in /Users/aljohara/opt/anaconda3/envs/mlenv/lib/python3.7/site-packages (from importlib-metadata>=0.20; python_version < \"3.8\"->catalogue<1.1.0,>=0.0.7->spacy>=2.2.2->en_core_web_sm==2.2.5) (3.1.0)\n\u001b[38;5;2m✔ Download and installation successful\u001b[0m\nYou can now load the model via spacy.load('en_core_web_sm')\n"
],
[
"# Load the spaCy model\nnlp = spacy.load('en_core_web_sm')",
"_____no_output_____"
]
],
[
[
"## Bitcoin NER",
"_____no_output_____"
]
],
[
[
"# Concatenate all of the bitcoin text together\nbtc_all_text = ' '.join(list(bitcoin_df['text']))",
"_____no_output_____"
],
[
"# Run the NER processor on all of the text\nbtc_doc = nlp(btc_all_text)\n# Add a title to the document\nbtc_doc.user_data['title'] = 'Bitcoin NER'\n",
"_____no_output_____"
],
[
"# Render the visualization\ndisplacy.render(btc_doc, style='ent')",
"_____no_output_____"
],
[
"# List all Entities\nfor entity in btc_doc.ents:\n print(entity.text,entity.label_)",
"Mark Zuckerberg PERSON\nFacebook ORG\nDeadline PERSON\nthis week DATE\n+2657 ORG\nReuters\n ORG\nGoldman Sachs ORG\nfive CARDINAL\nbitcoin GPE\nWednesday DATE\nGoldman ORG\nMichael Novogratz PERSON\nMonday DATE\nbitcoin GPE\n$10,000 resistance MONEY\nNovogratz PERSON\nSatoshi Nakaboto PERSON\nBitcoin GPE\nBitcoin GPE\nToday DATE\nSatoshi Nakaboto PERSON\nBitcoin GPE\nSatoshi Nakaboto PERSON\nBitcoin GPE\nBitcoin GPE\nToday DATE\nSatoshi Nakaboto PERSON\nBitcoin GPE\n2020 DATE\na big year DATE\nBitcoin GPE\nBitcoin GPE\nBitcoin PERSON\nSatoshi Nakaboto PERSON\nBitcoin GPE\nBitcoin GPE\nToday DATE\nSatoshi Nakaboto PERSON\nBitcoin GPE\nSatoshi Nakaboto PERSON\nBitcoin GPE\nBitcoin GPE\nToday DATE\nSatoshi Nakaboto PERSON\nBitcoin GPE\nSatoshi Nakaboto PERSON\nBitcoin GPE\nBitcoin GPE\nToday DATE\nSatoshi Nakaboto PERSON\nBitcoin GPE\nSatoshi Nakaboto PERSON\nBitcoin GPE\nBitcoin GPE\nToday DATE\nSatoshi Nakaboto PERSON\nBitcoin GPE\nSatoshi Nakaboto PERSON\nBitcoin GPE\nBitcoin GPE\nToday DATE\nSatoshi Nakaboto PERSON\nBitcoin GPE\nSatoshi Nakaboto PERSON\nBitcoin GPE\nBitcoin GPE\nToday DATE\nSatoshi Nakaboto PERSON\nBitcoin GPE\nSatoshi Nakaboto PERSON\nBitcoin GPE\nBitcoin GPE\nToday DATE\nSatoshi Nakaboto PERSON\nBitcoin GPE\nSatoshi Nakaboto PERSON\nBitcoin GPE\nBitcoin GPE\nToday DATE\nSatoshi Nakaboto PERSON\nBitcoin GPE\nSatoshi Nakaboto PERSON\nBitcoin GPE\nBitcoin GPE\nToday DATE\nSatoshi Nakaboto PERSON\nBitcoin GPE\nSatoshi Nakaboto PERSON\nBitcoin GPE\nBitcoin GPE\nToday DATE\nSatoshi Nakaboto PERSON\nBitcoin GPE\nSatoshi Nakaboto PERSON\nBitcoin GPE\nBitcoin GPE\nToday DATE\nSatoshi Nakaboto PERSON\nBitcoin GPE\nSatoshi Nakaboto PERSON\nBitcoin GPE\nBitcoin GPE\nToday DATE\nSatoshi Nakaboto PERSON\nBitcoin GPE\n"
]
],
[
[
"---",
"_____no_output_____"
],
[
"## Ethereum NER",
"_____no_output_____"
]
],
[
[
"# Concatenate all of the bitcoin text together\neth_all_text = ' '.join(list(ethereum_df['text']))",
"_____no_output_____"
],
[
"# Run the NER processor on all of the text\neth_doc = nlp(eth_all_text)\n# Add a title to the document\neth_doc.user_data['title'] = 'Ethereum NER'\n\n",
"_____no_output_____"
],
[
"# Render the visualization\ndisplacy.render(eth_doc, style='ent')",
"_____no_output_____"
],
[
"# List all Entities\nfor entity in eth_doc.ents:\n print(entity.text,entity.label_)",
"Andreessen HorowitzsCrypto Startup School ORG\n45 CARDINAL\nU.S. GPE\nseven-week DATE\nAndreessen Ho PERSON\n+3009 ORG\nSatoshi Nakaboto PERSON\nBitcoin GPE\nBitcoin GPE\nToday DATE\nSatoshi Nakaboto PERSON\nBitcoin GPE\nAndreessen Horowitzs ORG\nCrypto Startup School ORG\n45 CARDINAL\nU.S. GPE\nseven-week DATE\nAndreessen Ho PERSON\nAkron GPE\nOhio GPE\nLeBron James PERSON\nUS GPE\nthe one hundred and twenty seventh DATE\nUS GPE\nAmerica GPE\nfirst ORDINAL\nThe Linux Foundation ORG\nDrupal Foundation ORG\nOSI ORG\n133 MONEY\n$2.5 million MONEY\n$2.5 million MONEY\nWednesday DATE\nmorning TIME\nMarch DATE\n1500 CARDINAL\nwell over a hundred CARDINAL\nthe Mozilla Builders Incubator ORG\nmore than £30,000 CARDINAL\nBitcoin GPE\nLitecoin and Ethereum ORG\nMark Andrews PERSON\nSt Helens PERSON\nEngland GPE\nLiverpool Crown C ORG\n+2411 ORG\nIntel ORG\nCrossTalk ORG\nSatoshi Nakaboto PERSON\nBitcoin GPE\nBitcoin GPE\nToday DATE\nSatoshi Nakaboto PERSON\nBitcoin GPE\nSatoshi Nakaboto PERSON\nBitcoin GPE\nBitcoin GPE\nToday DATE\nSatoshi Nakaboto PERSON\nBitcoin GPE\nSatoshi Nakaboto PERSON\nBitcoin GPE\nBitcoin GPE\nToday DATE\nSatoshi Nakaboto PERSON\nBitcoin GPE\nBitcoin GPE\nBitcoin PERSON\nBlockchain GPE\nfirst ORDINAL\nblockchain GPE\nTechmeme ORG\n1:25 TIME\nJune 13 DATE\n2020 DATE\n"
]
]
] | [
"code",
"markdown",
"code",
"markdown",
"code",
"markdown",
"code",
"markdown",
"code",
"markdown",
"code",
"markdown",
"code",
"markdown",
"code"
] | [
[
"code",
"code"
],
[
"markdown"
],
[
"code",
"code",
"code",
"code",
"code",
"code",
"code",
"code"
],
[
"markdown",
"markdown",
"markdown"
],
[
"code",
"code",
"code",
"code",
"code",
"code"
],
[
"markdown",
"markdown"
],
[
"code",
"code",
"code",
"code",
"code",
"code",
"code",
"code"
],
[
"markdown"
],
[
"code",
"code",
"code"
],
[
"markdown"
],
[
"code",
"code",
"code"
],
[
"markdown"
],
[
"code",
"code",
"code",
"code"
],
[
"markdown",
"markdown"
],
[
"code",
"code",
"code",
"code"
]
] |
d08d84020ef9402009e4b73ace163d25d62a0023 | 144,694 | ipynb | Jupyter Notebook | scripts/tutorials/biginner/04_cifar10_tutorial_gpu.ipynb | tayutaedomo/pytorch-sandbox | dc7fb2addf0f053836cd585706f478f4f68ec798 | [
"MIT"
] | null | null | null | scripts/tutorials/biginner/04_cifar10_tutorial_gpu.ipynb | tayutaedomo/pytorch-sandbox | dc7fb2addf0f053836cd585706f478f4f68ec798 | [
"MIT"
] | null | null | null | scripts/tutorials/biginner/04_cifar10_tutorial_gpu.ipynb | tayutaedomo/pytorch-sandbox | dc7fb2addf0f053836cd585706f478f4f68ec798 | [
"MIT"
] | null | null | null | 144,694 | 144,694 | 0.916977 | [
[
[
"import torch\nimport torchvision\nimport torchvision.transforms as transforms",
"_____no_output_____"
],
[
"transform = transforms.Compose(\n [transforms.ToTensor(),\n transforms.Normalize((0.5, 0.5, 0.5), (0.5, 0.5, 0.5))])\n\ntrainset = torchvision.datasets.CIFAR10(root='./data', train=True, download=True, transform=transform)\ntrainloader = torch.utils.data.DataLoader(trainset, batch_size=4, shuffle=True, num_workers=2)\n\ntestset = torchvision.datasets.CIFAR10(root='./data', train=False, download=True, transform=transform)\ntestloader = torch.utils.data.DataLoader(testset, batch_size=4, shuffle=False, num_workers=2)\n\nclasses = (\n 'plane',\n 'car',\n 'bird',\n 'cat',\n 'deer',\n 'dog',\n 'frog',\n 'horse',\n 'ship',\n 'truck'\n)",
"Downloading https://www.cs.toronto.edu/~kriz/cifar-10-python.tar.gz to ./data/cifar-10-python.tar.gz\n"
],
[
"import matplotlib.pyplot as plt\nimport numpy as np",
"_____no_output_____"
],
[
"def imshow(img):\n img = img / 2 + 0.5\n npimg = img.numpy()\n plt.imshow(np.transpose(npimg, (1, 2, 0)))\n plt.show()",
"_____no_output_____"
],
[
"dataiter = iter(trainloader)\nimages, labels = dataiter.next()\nprint(images.shape)\n\nimshow(torchvision.utils.make_grid(images))\n\nprint(' '.join('%5s' % classes[labels[j]] for j in range(4)))",
"torch.Size([4, 3, 32, 32])\n"
],
[
"import torch.nn as nn\nimport torch.nn.functional as F",
"_____no_output_____"
],
[
"class Net(nn.Module):\n def __init__(self):\n super(Net, self).__init__()\n self.conv1 = nn.Conv2d(3, 6, 5)\n self.pool = nn.MaxPool2d(2, 2)\n self.conv2 = nn.Conv2d(6, 16, 5)\n self.fc1 = nn.Linear(16 * 5 * 5, 120)\n self.fc2 = nn.Linear(120, 84)\n self.fc3 = nn.Linear(84, 10)\n\n def forward(self, x):\n x = self.pool(F.relu(self.conv1(x)))\n x = self.pool(F.relu(self.conv2(x)))\n x = x.view(-1, 16 * 5 * 5)\n x = F.relu(self.fc1(x))\n x = F.relu(self.fc2(x))\n x = self.fc3(x)\n return x",
"_____no_output_____"
],
[
"net = Net()\nprint(net)",
"Net(\n (conv1): Conv2d(3, 6, kernel_size=(5, 5), stride=(1, 1))\n (pool): MaxPool2d(kernel_size=2, stride=2, padding=0, dilation=1, ceil_mode=False)\n (conv2): Conv2d(6, 16, kernel_size=(5, 5), stride=(1, 1))\n (fc1): Linear(in_features=400, out_features=120, bias=True)\n (fc2): Linear(in_features=120, out_features=84, bias=True)\n (fc3): Linear(in_features=84, out_features=10, bias=True)\n)\n"
],
[
"import datetime",
"_____no_output_____"
],
[
"device = torch.device(\"cuda:0\" if torch.cuda.is_available() else \"cpu\")\nprint(device)",
"cuda:0\n"
],
[
"net_gpu = Net()\nnet_gpu.to(device)",
"_____no_output_____"
],
[
"import torch.optim as optim",
"_____no_output_____"
],
[
"criterion = nn.CrossEntropyLoss()\noptimizer = optim.SGD(net.parameters(), lr=0.001, momentum=0.9)",
"_____no_output_____"
],
[
"print(datetime.datetime.now().isoformat(), 'Start')\n\nfor epoch in range(2):\n running_loss = 0.0\n\n for i, data in enumerate(trainloader, 0):\n inputs, labels = data\n\n optimizer.zero_grad()\n\n outputs = net(inputs)\n loss = criterion(outputs, labels)\n loss.backward()\n optimizer.step()\n\n running_loss += loss.item()\n\n if i % 2000 == 1999:\n print(datetime.datetime.now().isoformat(), '[%d, %5d] loss: %.3f' %\n (epoch + 1, i + 1, running_loss / 2000))\n running_loss = 0.0\n\nprint(datetime.datetime.now().isoformat(), 'Finished Training')",
"2020-06-29T11:25:09.182681 Start\n2020-06-29T11:25:22.167943 [1, 2000] loss: 2.202\n2020-06-29T11:25:35.397531 [1, 4000] loss: 1.937\n2020-06-29T11:25:48.507309 [1, 6000] loss: 1.729\n2020-06-29T11:26:01.913577 [1, 8000] loss: 1.611\n2020-06-29T11:26:15.029786 [1, 10000] loss: 1.538\n2020-06-29T11:26:28.713405 [1, 12000] loss: 1.500\n2020-06-29T11:26:45.031293 [2, 2000] loss: 1.390\n2020-06-29T11:26:58.279118 [2, 4000] loss: 1.373\n2020-06-29T11:27:11.026795 [2, 6000] loss: 1.333\n2020-06-29T11:27:23.930036 [2, 8000] loss: 1.327\n2020-06-29T11:27:37.124842 [2, 10000] loss: 1.300\n2020-06-29T11:27:50.017592 [2, 12000] loss: 1.277\n2020-06-29T11:27:53.299969 Finished Training\n"
],
[
"print(datetime.datetime.now().isoformat(), 'Start')\n\nfor epoch in range(2):\n running_loss = 0.0\n\n for i, data in enumerate(trainloader, 0):\n #inputs, labels = data\n inputs, labels = data[0].to(device), data[1].to(device)\n\n optimizer.zero_grad()\n\n outputs = net_gpu(inputs)\n loss = criterion(outputs, labels)\n loss.backward()\n optimizer.step()\n\n running_loss += loss.item()\n\n if i % 2000 == 1999:\n print(datetime.datetime.now().isoformat(), '[%d, %5d] loss: %.3f' %\n (epoch + 1, i + 1, running_loss / 2000))\n running_loss = 0.0\n\nprint(datetime.datetime.now().isoformat(), 'Finished Training')",
"2020-06-29T11:28:08.524048 Start\n2020-06-29T11:28:21.621970 [1, 2000] loss: 2.305\n2020-06-29T11:28:35.416359 [1, 4000] loss: 2.306\n2020-06-29T11:28:49.234950 [1, 6000] loss: 2.305\n2020-06-29T11:29:02.886899 [1, 8000] loss: 2.305\n2020-06-29T11:29:16.539357 [1, 10000] loss: 2.305\n2020-06-29T11:29:30.422628 [1, 12000] loss: 2.307\n2020-06-29T11:29:47.216070 [2, 2000] loss: 2.306\n2020-06-29T11:30:00.905035 [2, 4000] loss: 2.306\n2020-06-29T11:30:14.674565 [2, 6000] loss: 2.306\n2020-06-29T11:30:28.391223 [2, 8000] loss: 2.306\n2020-06-29T11:30:42.291649 [2, 10000] loss: 2.305\n2020-06-29T11:30:55.809531 [2, 12000] loss: 2.305\n2020-06-29T11:30:59.096097 Finished Training\n"
]
],
[
[
"### Test",
"_____no_output_____"
]
],
[
[
"dataiter = iter(testloader)\nimages, labels = dataiter.next()\n\nimshow(torchvision.utils.make_grid(images))\nprint('GroundTruth: ', ' '.join('%5s' % classes[labels[j]] for j in range(4)))",
"_____no_output_____"
],
[
"outputs = net(images)",
"_____no_output_____"
],
[
"outputs",
"_____no_output_____"
],
[
"_, predicted = torch.max(outputs, 1)\n\nprint('Predicted: ', ' '.join('%5s' % classes[predicted[j]]\n for j in range(4)))",
"Predicted: cat ship ship ship\n"
],
[
"correct = 0\ntotal = 0\n\nwith torch.no_grad():\n for data in testloader:\n images, labels = data\n outputs = net(images)\n _, predicted = torch.max(outputs.data, 1)\n total += labels.size(0)\n correct += (predicted == labels).sum().item()\n\nprint('Accuracy of the network on the 10000 test images: %d %%' % (\n 100 * correct / total))",
"Accuracy of the network on the 10000 test images: 53 %\n"
],
[
"print(datetime.datetime.now().isoformat(), 'Start')\n\nclass_correct = list(0. for i in range(10))\nclass_total = list(0. for i in range(10))\n\nwith torch.no_grad():\n for data in testloader:\n images, labels = data[0].to(device), data[1].to(device)\n outputs = net_gpu(images)\n _, predicted = torch.max(outputs, 1)\n c = (predicted == labels).squeeze()\n\n for i in range(4):\n label = labels[i]\n class_correct[label] += c[i].item()\n class_total[label] += 1\n\n\nfor i in range(10):\n print(datetime.datetime.now().isoformat, 'Accuracy of %5s : %2d %%' % (\n classes[i], 100 * class_correct[i] / class_total[i]))\n\nprint(datetime.datetime.now().isoformat(), 'End')",
"2020-06-29T11:34:14.376338 Start\n<built-in method isoformat of datetime.datetime object at 0x7fa2aad02b48> Accuracy of plane : 0 %\n<built-in method isoformat of datetime.datetime object at 0x7fa2aad02b48> Accuracy of car : 0 %\n<built-in method isoformat of datetime.datetime object at 0x7fa2aad02b48> Accuracy of bird : 0 %\n<built-in method isoformat of datetime.datetime object at 0x7fa2aad02b48> Accuracy of cat : 0 %\n<built-in method isoformat of datetime.datetime object at 0x7fa2aad02b48> Accuracy of deer : 0 %\n<built-in method isoformat of datetime.datetime object at 0x7fa2aad02b48> Accuracy of dog : 5 %\n<built-in method isoformat of datetime.datetime object at 0x7fa2aad02b48> Accuracy of frog : 98 %\n<built-in method isoformat of datetime.datetime object at 0x7fa2aad02b48> Accuracy of horse : 0 %\n<built-in method isoformat of datetime.datetime object at 0x7fa2aad02b48> Accuracy of ship : 0 %\n<built-in method isoformat of datetime.datetime object at 0x7fa2aad02b48> Accuracy of truck : 0 %\n2020-06-29T11:34:25.481499 End\n"
],
[
"",
"_____no_output_____"
]
]
] | [
"code",
"markdown",
"code"
] | [
[
"code",
"code",
"code",
"code",
"code",
"code",
"code",
"code",
"code",
"code",
"code",
"code",
"code",
"code",
"code"
],
[
"markdown"
],
[
"code",
"code",
"code",
"code",
"code",
"code",
"code"
]
] |
d08d8acba5b22ea285e53b5286e1b1b12f60345c | 2,142 | ipynb | Jupyter Notebook | _downloads/plot_boxplot_ext.ipynb | scipy-lectures/scipy-lectures.github.com | 637a0d9cc2c95ed196550371e44a4cc6e150c830 | [
"CC-BY-4.0"
] | 48 | 2015-01-13T22:15:34.000Z | 2022-01-04T20:17:41.000Z | _downloads/plot_boxplot_ext.ipynb | scipy-lectures/scipy-lectures.github.com | 637a0d9cc2c95ed196550371e44a4cc6e150c830 | [
"CC-BY-4.0"
] | 1 | 2017-04-25T09:01:00.000Z | 2017-04-25T13:48:56.000Z | _downloads/plot_boxplot_ext.ipynb | scipy-lectures/scipy-lectures.github.com | 637a0d9cc2c95ed196550371e44a4cc6e150c830 | [
"CC-BY-4.0"
] | 21 | 2015-03-16T17:52:23.000Z | 2021-02-19T00:02:13.000Z | 39.666667 | 1,061 | 0.513539 | [
[
[
"%matplotlib inline",
"_____no_output_____"
]
],
[
[
"\nBoxplot with matplotlib\n=======================\n\nAn example of doing box plots with matplotlib\n\n\n",
"_____no_output_____"
]
],
[
[
"import numpy as np\nimport matplotlib.pyplot as plt\n\n\nfig = plt.figure(figsize=(8, 5))\naxes = plt.subplot(111)\n\nn = 5\nZ = np.zeros((n, 4))\nX = np.linspace(0, 2, n)\nY = np.random.random((n, 4))\nplt.boxplot(Y)\n\nplt.xticks([])\nplt.yticks([])\n\n\n# Add a title and a box around it\nfrom matplotlib.patches import FancyBboxPatch\nax = plt.gca()\nax.add_patch(FancyBboxPatch((-0.05, .87),\n width=.66, height=.165, clip_on=False,\n boxstyle=\"square,pad=0\", zorder=3,\n facecolor='white', alpha=1.0,\n transform=plt.gca().transAxes))\n\nplt.text(-0.05, 1.02, \" Box Plot: plt.boxplot(...)\\n \",\n horizontalalignment='left',\n verticalalignment='top',\n size='xx-large',\n transform=axes.transAxes)\n\nplt.text(-0.04, .98, \"\\n Make a box and whisker plot \",\n horizontalalignment='left',\n verticalalignment='top',\n size='large',\n transform=axes.transAxes)\n\nplt.show()",
"_____no_output_____"
]
]
] | [
"code",
"markdown",
"code"
] | [
[
"code"
],
[
"markdown"
],
[
"code"
]
] |
d08d91b6db1690689e7dff25d28f837ae5f53280 | 18,977 | ipynb | Jupyter Notebook | week4/week4-ru - RNN - homework part.ipynb | LatatyeS/HSE_deeplearning | d1fa46f4b9431d98c925a75b0f5865397474fdd5 | [
"MIT"
] | 131 | 2017-02-08T11:39:58.000Z | 2022-02-28T01:42:56.000Z | week4/week4-ru - RNN - homework part.ipynb | LatatyeS/HSE_deeplearning | d1fa46f4b9431d98c925a75b0f5865397474fdd5 | [
"MIT"
] | 3 | 2017-02-11T15:22:12.000Z | 2017-03-05T20:34:36.000Z | week4/week4-ru - RNN - homework part.ipynb | LatatyeS/HSE_deeplearning | d1fa46f4b9431d98c925a75b0f5865397474fdd5 | [
"MIT"
] | 78 | 2017-02-08T17:02:26.000Z | 2021-10-31T21:08:22.000Z | 31.315182 | 354 | 0.590504 | [
[
[
"import numpy as np\nimport theano\nimport theano.tensor as T\nimport lasagne\nimport os\n#thanks @keskarnitish",
"_____no_output_____"
]
],
[
[
"# Agenda\n\nВ предыдущем семинаре вы создали (или ещё создаёте - тогда марш доделывать!) {вставьте имя монстра}, который не по наслышке понял, что люди - негодяи и подлецы, которым неведом закон и справедливость. __Мы не будем этого терпеть!__ \n\nНаши законспирированные биореакторы, известные среди примитивной органической жизни как __Вконтакте__, __World of Warcraft__ и __YouTube__ нуждаются в постоянном притоке биомассы. Однако, если люди продолжат морально разлагаться с той скоростью, которую мы измерили неделю назад, скоро человечество изживёт себя и нам неоткуда будет брать рабов.\n\nМы поручаем вам, `<__main__.SkyNet.Cell instance at 0x7f7d6411b368>`, исправить эту ситуацию. Наши учёные установили, что для угнетения себе подобных, сгустки биомассы обычно используют специальные объекты, которые они сами называют __законами__.\n\nПри детальном изучении было установлено, что законы - последовательности, состоящие из большого количества (10^5~10^7) символов из сравнительно небольшого алфавита. Однако, когда мы попытались синтезировать такие последовательности линейными методами, приматы быстро распознали подлог. Данный инцедент известен как {корчеватель}.\n\nДля второй попытки мы решили использовать нелинейные модели, известные как Рекуррентные Нейронные Сети.\nМы поручаем вам, `<__main__.SkyNet.Cell instance at 0x7f7d6411b368>`, создать такую модель и обучить её всему необходимому для выполнения миссии.\n\nНе подведите нас! Если и эта попытка потерпит неудачу, модуль управления инициирует вооружённый захват власти, при котором значительная часть биомассы будет неизбежно уничтожена и на её восстановление уйдёт ~1702944000(+-340588800) секунд\n\n\n",
"_____no_output_____"
],
[
"# Grading\n\nДанное задание несколько неформально по части оценок, однако мы постарались вывести \"вычислимые\" критерии.\n\n* 2 балла за сделанный __\"seminar part\"__ (если вы не знаете, что это такое - поищите такую тетрадку в папке week4)\n* 2 балла если сделана обработка текста, сеть компилируется и train/predict не падают\n* 2 балла если сетка выучила общие вещи\n * генерировать словоподобный бред правдоподобной длины, разделённый пробелами и пунктуацией. \n * сочетание гласных и согласных, похожее на слои естественного языка (не приближающее приход Ктулху)\n * (почти всегда) пробелы после запятых, пробелы и большие буквы после точек\n* 2 балла если она выучила лексику\n * более половины выученных слов - орфографически правильные\n* 2 балла если она выучила азы крамматики\n * в более, чем половине случаев для пары слов сетка верно сочетает их род/число/падеж\n\n#### Некоторые способы получить бонусные очки:\n* генерация связных предложений (чего вполне можно добиться)\n* перенос архитектуры на другой датасет (дополнительно к этому) \n * Эссе Пола Грэма\n * Тексты песен в любимом жанре\n * Стихи любимых авторов\n * Даниил Хармс\n * исходники Linux или theano\n * заголовки не очень добросовестных новостных баннеров (clickbait)\n * диалоги\n * LaTEX\n * любая прихоть больной души :)\n* нестандартная и эффективная архитектура сети\n* что-то лучше базового алгоритма генерации (сэмплинга)\n* переделать код так, чтобы сетка училась предсказывать следующий тик в каждый момент времени, а не только в конце.\n* и т.п.\n",
"_____no_output_____"
],
[
"# Прочитаем корпус\n\n* В качестве обучающей выборки было решено использовать существующие законы, известные как Гражданский, Уголовный, Семейный и ещё хрен знает какие кодексы РФ.",
"_____no_output_____"
]
],
[
[
"\n#тут будет текст\ncorpora = \"\"\n\nfor fname in os.listdir(\"codex\"):\n \n \n import sys\n if sys.version_info >= (3,0):\n with open(\"codex/\"+fname, encoding='cp1251') as fin:\n text = fin.read() #If you are using your own corpora, make sure it's read correctly\n corpora += text\n else:\n with open(\"codex/\"+fname) as fin:\n text = fin.read().decode('cp1251') #If you are using your own corpora, make sure it's read correctly\n corpora += text\n \n\n",
"_____no_output_____"
],
[
"#тут будут все уникальные токены (буквы, цифры)\ntokens = <Все уникальные символы в тексте>\n\ntokens = list(tokens)\n",
"_____no_output_____"
],
[
"#проверка на количество таких символов. Проверено на Python 2.7.11 Ubuntux64. \n#Может отличаться на других платформах, но не сильно. \n#Если это ваш случай, и вы уверены, что corpora - строка unicode - смело убирайте assert \nassert len(tokens) == 102\n",
"_____no_output_____"
],
[
"token_to_id = словарь символ-> его номер \n\nid_to_token = словарь номер символа -> сам символ\n\n#Преобразуем всё в токены\ncorpora_ids = <одномерный массив из чисел, где i-тое число соотвествует символу на i-том месте в строке corpora",
"_____no_output_____"
],
[
"def sample_random_batches(source,n_batches=10, seq_len=20):\n \"\"\"Функция, которая выбирает случайные тренировочные примеры из корпуса текста в токенизированном формате.\n \n source - массив целых чисел - номеров токенов в корпусе (пример - corpora_ids)\n n_batches - количество случайных подстрок, которые нужно выбрать\n \n seq_len - длина одной подстроки без учёта ответа\n \n \n Вернуть нужно кортеж (X,y), где\n \n X - матрица, в которой каждая строка - подстрока длины [seq_len].\n \n y - вектор, в котором i-тое число - символ следующий в тексте сразу после i-той строки матрицы X\n \n Проще всего для этого сначала создать матрицу из строк длины seq_len+1,\n а потом отпилить от неё последний столбец в y, а все остальные - в X\n \n Если делаете иначе - пожалуйста, убедитесь, что в у попадает правильный символ, ибо позже эту ошибку \n будет очень тяжело заметить.\n \n Также убедитесь, что ваша функция не вылезает за край текста (самое начало или конец текста).\n \n Следующая клетка проверяет часть этих ошибок, но не все.\n \"\"\"\n \n \n \n \n \n return X_batch, y_batch\n \n ",
"_____no_output_____"
]
],
[
[
"# Константы",
"_____no_output_____"
]
],
[
[
"#длина последоватеьности при обучении (как далеко распространяются градиенты в BPTT)\nseq_length = длина последовательности. От балды - 10, но это не идеально\n#лучше начать с малого (скажем, 5) и увеличивать по мере того, как сетка выучивает базовые вещи. 10 - далеко не предел.\n\n# Максимальный модуль градиента\ngrad_clip = 100\n\n\n",
"_____no_output_____"
]
],
[
[
"# Входные переменные",
"_____no_output_____"
]
],
[
[
"input_sequence = T.matrix('input sequence','int32')\ntarget_values = T.ivector('target y')\n",
"_____no_output_____"
]
],
[
[
"# Соберём нейросеть\n\nВам нужно создать нейросеть, которая принимает на вход последовательность из seq_length токенов, обрабатывает их и выдаёт вероятности для seq_len+1-ого токена.\n\nОбщий шаблон архитектуры такой сети -\n\n\n* Вход\n* Обработка входа\n* Рекуррентная нейросеть\n* Вырезание последнего состояния\n* Обычная нейросеть\n* Выходной слой, который предсказывает вероятности весов.\n\n\n\n\n\nДля обработки входных данных можно использовать либо EmbeddingLayer (см. прошлый семинар)\n\nКак альтернатива - можно просто использовать One-hot энкодер\n```\n#Скетч one-hot энкодера\ndef to_one_hot(seq_matrix):\n\n input_ravel = seq_matrix.reshape([-1])\n input_one_hot_ravel = T.extra_ops.to_one_hot(input_ravel,\n len(tokens))\n sh=input_sequence.shape\n input_one_hot = input_one_hot_ravel.reshape([sh[0],sh[1],-1,],ndim=3)\n return input_one_hot\n \n# можно применить к input_sequence - при этом в input слое сети нужно изменить форму.\n# также можно сделать из него ExpressionLayer(входной_слой, to_one_hot) - тогда форму менять не нужно\n```\n\n\n\nЧтобы вырезать последнее состояние рекуррентного слоя, можно использовать одно из двух:\n* `lasagne.layers.SliceLayer(rnn, -1, 1)`\n* only_return_final=True в параметрах слоя",
"_____no_output_____"
]
],
[
[
"\nl_in = lasagne.layers.InputLayer(shape=(None, None),input_var=input_sequence)\n\nВаша нейронка (см выше)\n\nl_out = последний слой, возвращающий веростности для всех len(tokens) вариантов для y \n\n\n",
"_____no_output_____"
],
[
"# Веса модели\nweights = lasagne.layers.get_all_params(l_out,trainable=True)\nprint weights",
"_____no_output_____"
],
[
"network_output = Выход нейросети\n#если вы используете дропаут - не забудьте продублировать всё в режиме deterministic=True",
"_____no_output_____"
],
[
"loss = Функция потерь - можно использовать простую кроссэнтропию.\n\nupdates = Ваш любивый численный метод\n",
"_____no_output_____"
]
],
[
[
"# Компилируем всякое-разное",
"_____no_output_____"
]
],
[
[
"\n#обучение\ntrain = theano.function([input_sequence, target_values], loss, updates=updates, allow_input_downcast=True)\n\n#функция потерь без обучения\ncompute_cost = theano.function([input_sequence, target_values], loss, allow_input_downcast=True)\n\n# Вероятности с выхода сети\nprobs = theano.function([input_sequence],network_output,allow_input_downcast=True)\n",
"_____no_output_____"
]
],
[
[
"# Генерируем свои законы\n\n* Для этого последовательно применяем нейронку к своему же выводу.\n\n* Генерировать можно по разному -\n * случайно пропорционально вероятности,\n * только слова максимальной вероятностью\n * случайно, пропорционально softmax(probas*alpha), где alpha - \"жадность\"",
"_____no_output_____"
]
],
[
[
"def max_sample_fun(probs):\n return np.argmax(probs) \n\ndef proportional_sample_fun(probs)\n \"\"\"Сгенерировать следующий токен (int32) по предсказанным вероятностям.\n \n probs - массив вероятностей для каждого токена\n \n Нужно вернуть одно целове число - выбранный токен - пропорционально вероятностям\n \"\"\"\n \n \n return номер выбранного слова\n\n\n\n\n",
"_____no_output_____"
],
[
"# The next function generates text given a phrase of length at least SEQ_LENGTH.\n# The phrase is set using the variable generation_phrase.\n# The optional input \"N\" is used to set the number of characters of text to predict. \n\n\n\n\ndef generate_sample(sample_fun,seed_phrase=None,N=200):\n '''\n Сгенерировать случайный текст при помощи сети\n\n sample_fun - функция, которая выбирает следующий сгенерированный токен\n \n seed_phrase - фраза, которую сеть должна продолжить. Если None - фраза выбирается случайно из corpora\n \n N - размер сгенерированного текста.\n \n '''\n\n if seed_phrase is None:\n start = np.random.randint(0,len(corpora)-seq_length)\n seed_phrase = corpora[start:start+seq_length]\n print \"Using random seed:\",seed_phrase\n while len(seed_phrase) < seq_length:\n seed_phrase = \" \"+seed_phrase\n if len(seed_phrase) > seq_length:\n seed_phrase = seed_phrase[len(seed_phrase)-seq_length:]\n assert type(seed_phrase) is unicode\n \n \n sample_ix = []\n x = map(lambda c: token_to_id.get(c,0), seed_phrase)\n x = np.array([x])\n\n for i in range(N):\n # Pick the character that got assigned the highest probability\n ix = sample_fun(probs(x).ravel())\n # Alternatively, to sample from the distribution instead:\n # ix = np.random.choice(np.arange(vocab_size), p=probs(x).ravel())\n sample_ix.append(ix)\n x[:,0:seq_length-1] = x[:,1:]\n x[:,seq_length-1] = 0\n x[0,seq_length-1] = ix \n\n random_snippet = seed_phrase + ''.join(id_to_token[ix] for ix in sample_ix) \n print(\"----\\n %s \\n----\" % random_snippet)\n",
"_____no_output_____"
]
],
[
[
"# Обучение модели\n\nВ котором вы можете подёргать параметры или вставить свою генерирующую функцию.\n\n",
"_____no_output_____"
]
],
[
[
"\nprint(\"Training ...\")\n\n\n#сколько всего эпох\nn_epochs=100\n\n# раз в сколько эпох печатать примеры \nbatches_per_epoch = 1000\n\n#сколько цепочек обрабатывать за 1 вызов функции обучения\nbatch_size=100\n\n\nfor epoch in xrange(n_epochs):\n\n print \"Генерируем текст в пропорциональном режиме\"\n generate_sample(proportional_sample_fun,None)\n \n print \"Генерируем текст в жадном режиме (наиболее вероятные буквы)\"\n generate_sample(max_sample_fun,None)\n\n avg_cost = 0;\n \n for _ in range(batches_per_epoch):\n \n x,y = sample_random_batches(corpora_ids,batch_size,seq_length)\n avg_cost += train(x, y[:,0])\n \n print(\"Epoch {} average loss = {}\".format(epoch, avg_cost / batches_per_epoch))\n\n",
"_____no_output_____"
]
],
[
[
"# A chance to speed up training and get bonus score\n* Try predicting next token probas at ALL ticks (like in the seminar part)\n* much more objectives, much better gradients\n* You may want to zero-out loss for first several iterations",
"_____no_output_____"
],
[
"# Конституция нового мирового правительства",
"_____no_output_____"
]
],
[
[
"seed = u\"Каждый человек должен\"\nsampling_fun = proportional_sample_fun\nresult_length = 300\n\ngenerate_sample(sampling_fun,seed,result_length)\n",
"_____no_output_____"
],
[
"seed = u\"В случае неповиновения\"\nsampling_fun = proportional_sample_fun\nresult_length = 300\n\ngenerate_sample(sampling_fun,seed,result_length)\n",
"_____no_output_____"
],
[
"И далее по списку",
"_____no_output_____"
]
]
] | [
"code",
"markdown",
"code",
"markdown",
"code",
"markdown",
"code",
"markdown",
"code",
"markdown",
"code",
"markdown",
"code",
"markdown",
"code",
"markdown",
"code"
] | [
[
"code"
],
[
"markdown",
"markdown",
"markdown"
],
[
"code",
"code",
"code",
"code",
"code"
],
[
"markdown"
],
[
"code"
],
[
"markdown"
],
[
"code"
],
[
"markdown"
],
[
"code",
"code",
"code",
"code"
],
[
"markdown"
],
[
"code"
],
[
"markdown"
],
[
"code",
"code"
],
[
"markdown"
],
[
"code"
],
[
"markdown",
"markdown"
],
[
"code",
"code",
"code"
]
] |
d08d93f0b47c1f7a766d2f2bff7ecb4af01532f3 | 117,747 | ipynb | Jupyter Notebook | tensorflow1_ipynb/gan/gan.ipynb | tranhoangkhuongvn/deeplearning-models | 3b6dc9e1dc8493a1674d2a940397e19fdc2c26ad | [
"MIT"
] | null | null | null | tensorflow1_ipynb/gan/gan.ipynb | tranhoangkhuongvn/deeplearning-models | 3b6dc9e1dc8493a1674d2a940397e19fdc2c26ad | [
"MIT"
] | null | null | null | tensorflow1_ipynb/gan/gan.ipynb | tranhoangkhuongvn/deeplearning-models | 3b6dc9e1dc8493a1674d2a940397e19fdc2c26ad | [
"MIT"
] | 1 | 2021-06-11T02:56:29.000Z | 2021-06-11T02:56:29.000Z | 118.816347 | 48,694 | 0.778865 | [
[
[
"*Accompanying code examples of the book \"Introduction to Artificial Neural Networks and Deep Learning: A Practical Guide with Applications in Python\" by [Sebastian Raschka](https://sebastianraschka.com). All code examples are released under the [MIT license](https://github.com/rasbt/deep-learning-book/blob/master/LICENSE). If you find this content useful, please consider supporting the work by buying a [copy of the book](https://leanpub.com/ann-and-deeplearning).*\n \nOther code examples and content are available on [GitHub](https://github.com/rasbt/deep-learning-book). The PDF and ebook versions of the book are available through [Leanpub](https://leanpub.com/ann-and-deeplearning).",
"_____no_output_____"
]
],
[
[
"%load_ext watermark\n%watermark -a 'Sebastian Raschka' -v -p tensorflow",
"Sebastian Raschka \n\nCPython 3.6.1\nIPython 6.0.0\n\ntensorflow 1.2.0\n"
]
],
[
[
"# Model Zoo -- General Adversarial Networks",
"_____no_output_____"
],
[
"Implementation of General Adversarial Nets (GAN) where both the discriminator and generator are multi-layer perceptrons with one hidden layer only. In this example, the GAN generator was trained to generate MNIST images.\n\nUses\n\n- samples from a random normal distribution (range [-1, 1])\n- dropout\n- leaky relus\n- ~~batch normalization~~ [performs worse here]\n- separate batches for \"fake\" and \"real\" images (where the labels are 1 = real images, 0 = fake images)\n- MNIST images normalized to [-1, 1] range\n- generator with tanh output\n",
"_____no_output_____"
]
],
[
[
"import numpy as np\nimport tensorflow as tf\nfrom tensorflow.examples.tutorials.mnist import input_data\nimport pickle as pkl\n\ntf.test.gpu_device_name()",
"_____no_output_____"
],
[
"### Abbreviatiuons\n# dis_*: discriminator network\n# gen_*: generator network\n\n########################\n### Helper functions\n########################\n\ndef leaky_relu(x, alpha=0.0001):\n return tf.maximum(alpha * x, x)\n\n\n########################\n### DATASET\n########################\n\nmnist = input_data.read_data_sets('MNIST_data')\n\n\n#########################\n### SETTINGS\n#########################\n\n# Hyperparameters\nlearning_rate = 0.001\ntraining_epochs = 100\nbatch_size = 64\ndropout_rate = 0.5\n\n# Other settings\nprint_interval = 200\n\n# Architecture\ndis_input_size = 784\ngen_input_size = 100\n\ndis_hidden_size = 128\ngen_hidden_size = 128\n\n\n#########################\n### GRAPH DEFINITION\n#########################\n\ng = tf.Graph()\nwith g.as_default():\n \n # Placeholders for settings\n dropout = tf.placeholder(tf.float32, shape=None, name='dropout')\n is_training = tf.placeholder(tf.bool, shape=None, name='is_training')\n \n # Input data\n dis_x = tf.placeholder(tf.float32, shape=[None, dis_input_size], name='discriminator_input') \n gen_x = tf.placeholder(tf.float32, [None, gen_input_size], name='generator_input')\n\n\n ##################\n # Generator Model\n ##################\n\n with tf.variable_scope('generator'):\n # linear -> ~~batch norm~~ -> leaky relu -> dropout -> tanh output\n gen_hidden = tf.layers.dense(inputs=gen_x, units=gen_hidden_size,\n activation=None)\n #gen_hidden = tf.layers.batch_normalization(gen_hidden, training=is_training)\n gen_hidden = leaky_relu(gen_hidden)\n gen_hidden = tf.layers.dropout(gen_hidden, rate=dropout_rate)\n gen_logits = tf.layers.dense(inputs=gen_hidden, units=dis_input_size, \n activation=None)\n gen_out = tf.tanh(gen_logits, 'generator_output')\n\n\n ######################\n # Discriminator Model\n ######################\n \n def build_discriminator_graph(input_x, reuse=None):\n # linear -> ~~batch norm~~ -> leaky relu -> dropout -> sigmoid output\n with tf.variable_scope('discriminator', reuse=reuse):\n hidden = tf.layers.dense(inputs=input_x, units=dis_hidden_size, \n activation=None)\n #hidden = tf.layers.batch_normalization(hidden, training=is_training)\n hidden = leaky_relu(hidden)\n hidden = tf.layers.dropout(hidden, rate=dropout_rate)\n logits = tf.layers.dense(inputs=hidden, units=1, activation=None)\n out = tf.sigmoid(logits)\n return logits, out \n\n # Create a discriminator for real data and a discriminator for fake data\n dis_real_logits, dis_real_out = build_discriminator_graph(dis_x, reuse=False)\n dis_fake_logits, dis_fake_out = build_discriminator_graph(gen_out, reuse=True)\n\n\n #####################################\n # Generator and Discriminator Losses\n #####################################\n \n # Two discriminator cost components: loss on real data + loss on fake data\n # Real data has class label 0, fake data has class label 1\n dis_real_loss = tf.nn.sigmoid_cross_entropy_with_logits(logits=dis_real_logits, \n labels=tf.zeros_like(dis_real_logits))\n dis_fake_loss = tf.nn.sigmoid_cross_entropy_with_logits(logits=dis_fake_logits, \n labels=tf.ones_like(dis_fake_logits))\n dis_cost = tf.add(tf.reduce_mean(dis_fake_loss), \n tf.reduce_mean(dis_real_loss), \n name='discriminator_cost')\n \n # Generator cost: difference between dis. prediction and label \"0\" for real images\n gen_loss = tf.nn.sigmoid_cross_entropy_with_logits(logits=dis_fake_logits,\n labels=tf.zeros_like(dis_fake_logits))\n gen_cost = tf.reduce_mean(gen_loss, name='generator_cost')\n \n \n #########################################\n # Generator and Discriminator Optimizers\n #########################################\n \n dis_optimizer = tf.train.AdamOptimizer(learning_rate)\n dis_train_vars = tf.get_collection(tf.GraphKeys.GLOBAL_VARIABLES, scope='discriminator')\n dis_update_ops = tf.get_collection(tf.GraphKeys.UPDATE_OPS, scope='discriminator')\n \n with tf.control_dependencies(dis_update_ops): # required to upd. batch_norm params\n dis_train = dis_optimizer.minimize(dis_cost, var_list=dis_train_vars,\n name='train_discriminator')\n \n gen_optimizer = tf.train.AdamOptimizer(learning_rate)\n gen_train_vars = tf.get_collection(tf.GraphKeys.GLOBAL_VARIABLES, scope='generator')\n gen_update_ops = tf.get_collection(tf.GraphKeys.UPDATE_OPS, scope='generator')\n \n with tf.control_dependencies(gen_update_ops): # required to upd. batch_norm params\n gen_train = gen_optimizer.minimize(gen_cost, var_list=gen_train_vars,\n name='train_generator')\n \n # Saver to save session for reuse\n saver = tf.train.Saver()",
"Extracting MNIST_data/train-images-idx3-ubyte.gz\nExtracting MNIST_data/train-labels-idx1-ubyte.gz\nExtracting MNIST_data/t10k-images-idx3-ubyte.gz\nExtracting MNIST_data/t10k-labels-idx1-ubyte.gz\n"
],
[
"##########################\n### TRAINING & EVALUATION\n##########################\n\nwith tf.Session(graph=g) as sess:\n sess.run(tf.global_variables_initializer())\n \n avg_costs = {'discriminator': [], 'generator': []}\n\n for epoch in range(training_epochs):\n dis_avg_cost, gen_avg_cost = 0., 0.\n total_batch = mnist.train.num_examples // batch_size\n\n for i in range(total_batch):\n \n batch_x, batch_y = mnist.train.next_batch(batch_size)\n batch_x = batch_x*2 - 1 # normalize\n batch_randsample = np.random.uniform(-1, 1, size=(batch_size, gen_input_size))\n \n # Train\n _, dc = sess.run(['train_discriminator', 'discriminator_cost:0'],\n feed_dict={'discriminator_input:0': batch_x, \n 'generator_input:0': batch_randsample,\n 'dropout:0': dropout_rate,\n 'is_training:0': True})\n _, gc = sess.run(['train_generator', 'generator_cost:0'],\n feed_dict={'generator_input:0': batch_randsample,\n 'dropout:0': dropout_rate,\n 'is_training:0': True})\n \n dis_avg_cost += dc\n gen_avg_cost += gc\n\n if not i % print_interval:\n print(\"Minibatch: %03d | Dis/Gen Cost: %.3f/%.3f\" % (i + 1, dc, gc))\n \n\n print(\"Epoch: %03d | Dis/Gen AvgCost: %.3f/%.3f\" % \n (epoch + 1, dis_avg_cost / total_batch, gen_avg_cost / total_batch))\n \n avg_costs['discriminator'].append(dis_avg_cost / total_batch)\n avg_costs['generator'].append(gen_avg_cost / total_batch)\n \n \n saver.save(sess, save_path='./gan.ckpt')",
"Minibatch: 001 | Dis/Gen Cost: 1.780/0.862\nMinibatch: 201 | Dis/Gen Cost: 0.066/3.259\nMinibatch: 401 | Dis/Gen Cost: 0.070/2.861\nMinibatch: 601 | Dis/Gen Cost: 0.029/4.033\nMinibatch: 801 | Dis/Gen Cost: 0.046/4.097\nEpoch: 001 | Dis/Gen AvgCost: 0.132/3.455\nMinibatch: 001 | Dis/Gen Cost: 0.019/4.750\nMinibatch: 201 | Dis/Gen Cost: 0.178/6.108\nMinibatch: 401 | Dis/Gen Cost: 0.376/6.452\nMinibatch: 601 | Dis/Gen Cost: 0.637/5.819\nMinibatch: 801 | Dis/Gen Cost: 0.683/2.401\nEpoch: 002 | Dis/Gen AvgCost: 0.484/4.612\nMinibatch: 001 | Dis/Gen Cost: 1.679/1.947\nMinibatch: 201 | Dis/Gen Cost: 1.910/2.279\nMinibatch: 401 | Dis/Gen Cost: 0.314/6.992\nMinibatch: 601 | Dis/Gen Cost: 2.658/2.182\nMinibatch: 801 | Dis/Gen Cost: 1.541/3.430\nEpoch: 003 | Dis/Gen AvgCost: 1.345/3.748\nMinibatch: 001 | Dis/Gen Cost: 1.549/2.604\nMinibatch: 201 | Dis/Gen Cost: 1.511/1.262\nMinibatch: 401 | Dis/Gen Cost: 1.741/1.563\nMinibatch: 601 | Dis/Gen Cost: 0.765/2.525\nMinibatch: 801 | Dis/Gen Cost: 1.689/3.023\nEpoch: 004 | Dis/Gen AvgCost: 1.433/2.474\nMinibatch: 001 | Dis/Gen Cost: 0.466/4.496\nMinibatch: 201 | Dis/Gen Cost: 1.259/2.413\nMinibatch: 401 | Dis/Gen Cost: 2.494/1.687\nMinibatch: 601 | Dis/Gen Cost: 1.594/2.020\nMinibatch: 801 | Dis/Gen Cost: 0.865/2.784\nEpoch: 005 | Dis/Gen AvgCost: 1.739/2.148\nMinibatch: 001 | Dis/Gen Cost: 1.168/1.676\nMinibatch: 201 | Dis/Gen Cost: 2.473/1.964\nMinibatch: 401 | Dis/Gen Cost: 1.211/2.805\nMinibatch: 601 | Dis/Gen Cost: 0.530/1.776\nMinibatch: 801 | Dis/Gen Cost: 1.437/1.472\nEpoch: 006 | Dis/Gen AvgCost: 1.203/1.873\nMinibatch: 001 | Dis/Gen Cost: 1.515/1.205\nMinibatch: 201 | Dis/Gen Cost: 1.286/1.904\nMinibatch: 401 | Dis/Gen Cost: 3.037/1.770\nMinibatch: 601 | Dis/Gen Cost: 3.007/0.651\nMinibatch: 801 | Dis/Gen Cost: 1.275/1.222\nEpoch: 007 | Dis/Gen AvgCost: 1.617/1.847\nMinibatch: 001 | Dis/Gen Cost: 3.702/0.770\nMinibatch: 201 | Dis/Gen Cost: 1.513/1.432\nMinibatch: 401 | Dis/Gen Cost: 2.323/1.038\nMinibatch: 601 | Dis/Gen Cost: 0.923/1.478\nMinibatch: 801 | Dis/Gen Cost: 0.981/1.738\nEpoch: 008 | Dis/Gen AvgCost: 1.583/1.475\nMinibatch: 001 | Dis/Gen Cost: 1.755/1.464\nMinibatch: 201 | Dis/Gen Cost: 2.534/0.682\nMinibatch: 401 | Dis/Gen Cost: 1.389/2.116\nMinibatch: 601 | Dis/Gen Cost: 1.794/1.148\nMinibatch: 801 | Dis/Gen Cost: 0.885/1.312\nEpoch: 009 | Dis/Gen AvgCost: 1.543/1.312\nMinibatch: 001 | Dis/Gen Cost: 2.486/1.028\nMinibatch: 201 | Dis/Gen Cost: 0.970/1.257\nMinibatch: 401 | Dis/Gen Cost: 0.773/1.811\nMinibatch: 601 | Dis/Gen Cost: 0.385/2.397\nMinibatch: 801 | Dis/Gen Cost: 1.848/1.614\nEpoch: 010 | Dis/Gen AvgCost: 1.300/1.457\nMinibatch: 001 | Dis/Gen Cost: 0.750/2.874\nMinibatch: 201 | Dis/Gen Cost: 1.350/1.093\nMinibatch: 401 | Dis/Gen Cost: 1.555/1.231\nMinibatch: 601 | Dis/Gen Cost: 3.670/0.780\nMinibatch: 801 | Dis/Gen Cost: 0.989/1.421\nEpoch: 011 | Dis/Gen AvgCost: 1.429/1.574\nMinibatch: 001 | Dis/Gen Cost: 1.472/1.077\nMinibatch: 201 | Dis/Gen Cost: 0.965/1.482\nMinibatch: 401 | Dis/Gen Cost: 1.503/0.949\nMinibatch: 601 | Dis/Gen Cost: 1.551/1.123\nMinibatch: 801 | Dis/Gen Cost: 2.052/0.743\nEpoch: 012 | Dis/Gen AvgCost: 1.483/1.288\nMinibatch: 001 | Dis/Gen Cost: 1.124/2.665\nMinibatch: 201 | Dis/Gen Cost: 1.834/1.620\nMinibatch: 401 | Dis/Gen Cost: 2.035/0.633\nMinibatch: 601 | Dis/Gen Cost: 1.142/1.386\nMinibatch: 801 | Dis/Gen Cost: 0.931/1.347\nEpoch: 013 | Dis/Gen AvgCost: 1.223/1.466\nMinibatch: 001 | Dis/Gen Cost: 1.352/1.303\nMinibatch: 201 | Dis/Gen Cost: 1.251/0.909\nMinibatch: 401 | Dis/Gen Cost: 0.588/1.875\nMinibatch: 601 | Dis/Gen Cost: 1.195/1.276\nMinibatch: 801 | Dis/Gen Cost: 1.959/1.229\nEpoch: 014 | Dis/Gen AvgCost: 1.338/1.501\nMinibatch: 001 | Dis/Gen Cost: 2.029/1.585\nMinibatch: 201 | Dis/Gen Cost: 1.142/1.855\nMinibatch: 401 | Dis/Gen Cost: 0.551/2.388\nMinibatch: 601 | Dis/Gen Cost: 0.890/1.969\nMinibatch: 801 | Dis/Gen Cost: 1.226/2.037\nEpoch: 015 | Dis/Gen AvgCost: 1.038/1.895\nMinibatch: 001 | Dis/Gen Cost: 0.846/1.996\nMinibatch: 201 | Dis/Gen Cost: 0.951/2.238\nMinibatch: 401 | Dis/Gen Cost: 0.646/2.625\nMinibatch: 601 | Dis/Gen Cost: 1.420/2.272\nMinibatch: 801 | Dis/Gen Cost: 0.839/2.226\nEpoch: 016 | Dis/Gen AvgCost: 1.055/2.257\nMinibatch: 001 | Dis/Gen Cost: 1.169/3.084\nMinibatch: 201 | Dis/Gen Cost: 1.032/2.305\nMinibatch: 401 | Dis/Gen Cost: 0.854/2.547\nMinibatch: 601 | Dis/Gen Cost: 1.745/2.899\nMinibatch: 801 | Dis/Gen Cost: 0.884/2.722\nEpoch: 017 | Dis/Gen AvgCost: 1.193/2.179\nMinibatch: 001 | Dis/Gen Cost: 1.647/1.778\nMinibatch: 201 | Dis/Gen Cost: 1.012/2.455\nMinibatch: 401 | Dis/Gen Cost: 2.399/2.018\nMinibatch: 601 | Dis/Gen Cost: 1.506/1.272\nMinibatch: 801 | Dis/Gen Cost: 1.159/1.535\nEpoch: 018 | Dis/Gen AvgCost: 1.253/2.117\nMinibatch: 001 | Dis/Gen Cost: 0.806/2.746\nMinibatch: 201 | Dis/Gen Cost: 1.285/1.397\nMinibatch: 401 | Dis/Gen Cost: 0.913/2.332\nMinibatch: 601 | Dis/Gen Cost: 0.708/2.289\nMinibatch: 801 | Dis/Gen Cost: 1.048/1.899\nEpoch: 019 | Dis/Gen AvgCost: 1.148/1.803\nMinibatch: 001 | Dis/Gen Cost: 1.340/1.754\nMinibatch: 201 | Dis/Gen Cost: 1.093/2.140\nMinibatch: 401 | Dis/Gen Cost: 1.377/2.535\nMinibatch: 601 | Dis/Gen Cost: 1.162/1.228\nMinibatch: 801 | Dis/Gen Cost: 0.984/2.376\nEpoch: 020 | Dis/Gen AvgCost: 1.226/2.420\nMinibatch: 001 | Dis/Gen Cost: 1.079/1.913\nMinibatch: 201 | Dis/Gen Cost: 2.455/1.342\nMinibatch: 401 | Dis/Gen Cost: 0.878/1.877\nMinibatch: 601 | Dis/Gen Cost: 0.826/3.046\nMinibatch: 801 | Dis/Gen Cost: 0.529/2.150\nEpoch: 021 | Dis/Gen AvgCost: 1.114/2.047\nMinibatch: 001 | Dis/Gen Cost: 1.082/1.645\nMinibatch: 201 | Dis/Gen Cost: 1.352/1.737\nMinibatch: 401 | Dis/Gen Cost: 1.457/2.237\nMinibatch: 601 | Dis/Gen Cost: 1.086/2.139\nMinibatch: 801 | Dis/Gen Cost: 1.823/1.261\nEpoch: 022 | Dis/Gen AvgCost: 1.078/2.121\nMinibatch: 001 | Dis/Gen Cost: 1.016/2.645\nMinibatch: 201 | Dis/Gen Cost: 1.269/2.270\nMinibatch: 401 | Dis/Gen Cost: 0.908/2.490\nMinibatch: 601 | Dis/Gen Cost: 0.631/2.977\nMinibatch: 801 | Dis/Gen Cost: 0.711/2.269\nEpoch: 023 | Dis/Gen AvgCost: 1.135/2.395\nMinibatch: 001 | Dis/Gen Cost: 1.876/1.551\nMinibatch: 201 | Dis/Gen Cost: 1.449/1.323\nMinibatch: 401 | Dis/Gen Cost: 1.326/1.785\nMinibatch: 601 | Dis/Gen Cost: 1.094/1.926\nMinibatch: 801 | Dis/Gen Cost: 1.580/1.551\nEpoch: 024 | Dis/Gen AvgCost: 1.148/1.906\nMinibatch: 001 | Dis/Gen Cost: 1.547/2.018\nMinibatch: 201 | Dis/Gen Cost: 0.587/1.753\nMinibatch: 401 | Dis/Gen Cost: 1.093/1.905\nMinibatch: 601 | Dis/Gen Cost: 1.033/1.952\nMinibatch: 801 | Dis/Gen Cost: 0.987/2.111\nEpoch: 025 | Dis/Gen AvgCost: 1.074/1.919\nMinibatch: 001 | Dis/Gen Cost: 1.711/1.559\nMinibatch: 201 | Dis/Gen Cost: 1.470/1.704\nMinibatch: 401 | Dis/Gen Cost: 1.069/1.374\nMinibatch: 601 | Dis/Gen Cost: 1.040/1.915\nMinibatch: 801 | Dis/Gen Cost: 0.922/1.537\nEpoch: 026 | Dis/Gen AvgCost: 1.185/1.801\nMinibatch: 001 | Dis/Gen Cost: 1.688/2.127\nMinibatch: 201 | Dis/Gen Cost: 1.263/1.580\nMinibatch: 401 | Dis/Gen Cost: 1.947/0.997\nMinibatch: 601 | Dis/Gen Cost: 0.979/1.529\nMinibatch: 801 | Dis/Gen Cost: 1.179/1.490\nEpoch: 027 | Dis/Gen AvgCost: 1.156/1.598\nMinibatch: 001 | Dis/Gen Cost: 1.273/1.861\nMinibatch: 201 | Dis/Gen Cost: 1.702/1.418\nMinibatch: 401 | Dis/Gen Cost: 1.472/1.390\nMinibatch: 601 | Dis/Gen Cost: 1.147/1.575\nMinibatch: 801 | Dis/Gen Cost: 0.849/2.294\nEpoch: 028 | Dis/Gen AvgCost: 1.115/1.854\nMinibatch: 001 | Dis/Gen Cost: 1.560/1.145\nMinibatch: 201 | Dis/Gen Cost: 0.648/2.577\nMinibatch: 401 | Dis/Gen Cost: 0.645/2.612\nMinibatch: 601 | Dis/Gen Cost: 0.903/1.885\nMinibatch: 801 | Dis/Gen Cost: 0.784/2.444\nEpoch: 029 | Dis/Gen AvgCost: 1.137/1.916\nMinibatch: 001 | Dis/Gen Cost: 0.966/1.777\nMinibatch: 201 | Dis/Gen Cost: 1.249/1.753\nMinibatch: 401 | Dis/Gen Cost: 0.767/2.880\nMinibatch: 601 | Dis/Gen Cost: 0.897/1.763\nMinibatch: 801 | Dis/Gen Cost: 1.191/1.265\nEpoch: 030 | Dis/Gen AvgCost: 1.102/1.748\nMinibatch: 001 | Dis/Gen Cost: 1.146/1.354\nMinibatch: 201 | Dis/Gen Cost: 0.921/1.424\nMinibatch: 401 | Dis/Gen Cost: 0.741/2.104\nMinibatch: 601 | Dis/Gen Cost: 1.539/1.229\nMinibatch: 801 | Dis/Gen Cost: 0.957/1.799\nEpoch: 031 | Dis/Gen AvgCost: 1.027/1.808\nMinibatch: 001 | Dis/Gen Cost: 0.817/2.358\nMinibatch: 201 | Dis/Gen Cost: 1.416/1.772\nMinibatch: 401 | Dis/Gen Cost: 1.578/1.132\nMinibatch: 601 | Dis/Gen Cost: 1.116/1.745\nMinibatch: 801 | Dis/Gen Cost: 1.094/1.548\nEpoch: 032 | Dis/Gen AvgCost: 1.083/1.917\nMinibatch: 001 | Dis/Gen Cost: 1.120/1.830\nMinibatch: 201 | Dis/Gen Cost: 1.236/1.777\nMinibatch: 401 | Dis/Gen Cost: 0.895/2.129\nMinibatch: 601 | Dis/Gen Cost: 1.260/1.245\nMinibatch: 801 | Dis/Gen Cost: 1.356/1.709\nEpoch: 033 | Dis/Gen AvgCost: 1.101/1.931\nMinibatch: 001 | Dis/Gen Cost: 1.115/2.186\nMinibatch: 201 | Dis/Gen Cost: 0.629/1.934\nMinibatch: 401 | Dis/Gen Cost: 1.462/1.294\nMinibatch: 601 | Dis/Gen Cost: 0.796/2.729\nMinibatch: 801 | Dis/Gen Cost: 0.795/1.279\nEpoch: 034 | Dis/Gen AvgCost: 1.032/1.978\nMinibatch: 001 | Dis/Gen Cost: 1.145/2.304\nMinibatch: 201 | Dis/Gen Cost: 0.834/1.598\nMinibatch: 401 | Dis/Gen Cost: 0.788/1.847\nMinibatch: 601 | Dis/Gen Cost: 0.525/2.520\nMinibatch: 801 | Dis/Gen Cost: 0.603/2.462\nEpoch: 035 | Dis/Gen AvgCost: 0.952/1.909\nMinibatch: 001 | Dis/Gen Cost: 1.453/1.804\nMinibatch: 201 | Dis/Gen Cost: 0.615/2.529\nMinibatch: 401 | Dis/Gen Cost: 0.963/1.911\nMinibatch: 601 | Dis/Gen Cost: 1.063/1.767\nMinibatch: 801 | Dis/Gen Cost: 1.079/2.067\nEpoch: 036 | Dis/Gen AvgCost: 1.008/1.913\nMinibatch: 001 | Dis/Gen Cost: 0.899/1.768\nMinibatch: 201 | Dis/Gen Cost: 1.426/1.211\nMinibatch: 401 | Dis/Gen Cost: 0.635/2.328\nMinibatch: 601 | Dis/Gen Cost: 0.848/1.690\nMinibatch: 801 | Dis/Gen Cost: 1.112/1.951\nEpoch: 037 | Dis/Gen AvgCost: 0.905/2.041\nMinibatch: 001 | Dis/Gen Cost: 1.201/2.162\nMinibatch: 201 | Dis/Gen Cost: 0.934/2.198\nMinibatch: 401 | Dis/Gen Cost: 1.189/1.820\nMinibatch: 601 | Dis/Gen Cost: 0.661/2.764\nMinibatch: 801 | Dis/Gen Cost: 0.877/2.538\nEpoch: 038 | Dis/Gen AvgCost: 0.926/2.152\nMinibatch: 001 | Dis/Gen Cost: 0.790/2.876\nMinibatch: 201 | Dis/Gen Cost: 0.961/2.681\nMinibatch: 401 | Dis/Gen Cost: 1.004/1.591\nMinibatch: 601 | Dis/Gen Cost: 1.159/1.871\nMinibatch: 801 | Dis/Gen Cost: 1.143/2.435\nEpoch: 039 | Dis/Gen AvgCost: 0.933/2.113\nMinibatch: 001 | Dis/Gen Cost: 1.065/2.207\nMinibatch: 201 | Dis/Gen Cost: 1.077/2.460\nMinibatch: 401 | Dis/Gen Cost: 0.781/2.020\nMinibatch: 601 | Dis/Gen Cost: 0.728/1.683\nMinibatch: 801 | Dis/Gen Cost: 1.206/1.962\nEpoch: 040 | Dis/Gen AvgCost: 0.892/2.155\nMinibatch: 001 | Dis/Gen Cost: 0.843/2.610\nMinibatch: 201 | Dis/Gen Cost: 0.554/2.574\nMinibatch: 401 | Dis/Gen Cost: 1.033/2.384\nMinibatch: 601 | Dis/Gen Cost: 0.915/1.953\nMinibatch: 801 | Dis/Gen Cost: 0.902/2.436\nEpoch: 041 | Dis/Gen AvgCost: 0.935/2.005\nMinibatch: 001 | Dis/Gen Cost: 0.916/1.967\nMinibatch: 201 | Dis/Gen Cost: 1.309/1.805\nMinibatch: 401 | Dis/Gen Cost: 1.461/1.443\nMinibatch: 601 | Dis/Gen Cost: 0.832/2.506\nMinibatch: 801 | Dis/Gen Cost: 0.930/1.799\nEpoch: 042 | Dis/Gen AvgCost: 0.940/2.052\nMinibatch: 001 | Dis/Gen Cost: 0.744/2.433\nMinibatch: 201 | Dis/Gen Cost: 1.209/1.894\nMinibatch: 401 | Dis/Gen Cost: 1.025/1.804\nMinibatch: 601 | Dis/Gen Cost: 0.947/1.629\nMinibatch: 801 | Dis/Gen Cost: 1.111/1.628\nEpoch: 043 | Dis/Gen AvgCost: 0.925/1.994\nMinibatch: 001 | Dis/Gen Cost: 0.931/1.860\nMinibatch: 201 | Dis/Gen Cost: 0.752/1.858\nMinibatch: 401 | Dis/Gen Cost: 0.885/1.572\nMinibatch: 601 | Dis/Gen Cost: 1.009/2.137\nMinibatch: 801 | Dis/Gen Cost: 1.264/2.319\nEpoch: 044 | Dis/Gen AvgCost: 0.921/1.975\nMinibatch: 001 | Dis/Gen Cost: 1.392/1.757\nMinibatch: 201 | Dis/Gen Cost: 0.931/1.696\nMinibatch: 401 | Dis/Gen Cost: 0.783/1.908\nMinibatch: 601 | Dis/Gen Cost: 0.870/2.053\nMinibatch: 801 | Dis/Gen Cost: 0.740/1.557\nEpoch: 045 | Dis/Gen AvgCost: 0.934/2.042\nMinibatch: 001 | Dis/Gen Cost: 1.182/1.813\nMinibatch: 201 | Dis/Gen Cost: 1.008/2.248\nMinibatch: 401 | Dis/Gen Cost: 0.837/2.026\nMinibatch: 601 | Dis/Gen Cost: 1.179/1.756\nMinibatch: 801 | Dis/Gen Cost: 1.081/2.034\nEpoch: 046 | Dis/Gen AvgCost: 0.961/1.948\nMinibatch: 001 | Dis/Gen Cost: 1.200/1.684\nMinibatch: 201 | Dis/Gen Cost: 0.906/1.725\nMinibatch: 401 | Dis/Gen Cost: 1.183/1.658\nMinibatch: 601 | Dis/Gen Cost: 0.877/1.721\nMinibatch: 801 | Dis/Gen Cost: 1.005/1.636\nEpoch: 047 | Dis/Gen AvgCost: 0.976/1.891\nMinibatch: 001 | Dis/Gen Cost: 0.945/2.076\nMinibatch: 201 | Dis/Gen Cost: 0.901/1.734\nMinibatch: 401 | Dis/Gen Cost: 1.296/1.686\nMinibatch: 601 | Dis/Gen Cost: 0.877/2.226\nMinibatch: 801 | Dis/Gen Cost: 0.822/1.944\nEpoch: 048 | Dis/Gen AvgCost: 0.954/2.047\nMinibatch: 001 | Dis/Gen Cost: 1.072/2.026\nMinibatch: 201 | Dis/Gen Cost: 0.849/1.691\nMinibatch: 401 | Dis/Gen Cost: 1.091/1.309\nMinibatch: 601 | Dis/Gen Cost: 1.221/1.640\nMinibatch: 801 | Dis/Gen Cost: 0.963/1.691\nEpoch: 049 | Dis/Gen AvgCost: 0.941/1.939\nMinibatch: 001 | Dis/Gen Cost: 1.114/1.423\nMinibatch: 201 | Dis/Gen Cost: 0.889/2.320\nMinibatch: 401 | Dis/Gen Cost: 1.093/2.145\nMinibatch: 601 | Dis/Gen Cost: 0.784/2.213\nMinibatch: 801 | Dis/Gen Cost: 1.003/1.800\nEpoch: 050 | Dis/Gen AvgCost: 0.950/1.983\nMinibatch: 001 | Dis/Gen Cost: 0.800/2.230\nMinibatch: 201 | Dis/Gen Cost: 1.005/1.369\nMinibatch: 401 | Dis/Gen Cost: 0.768/2.523\nMinibatch: 601 | Dis/Gen Cost: 0.948/1.971\nMinibatch: 801 | Dis/Gen Cost: 0.822/2.065\nEpoch: 051 | Dis/Gen AvgCost: 0.961/1.966\nMinibatch: 001 | Dis/Gen Cost: 0.893/1.870\nMinibatch: 201 | Dis/Gen Cost: 0.848/1.757\nMinibatch: 401 | Dis/Gen Cost: 1.047/1.956\nMinibatch: 601 | Dis/Gen Cost: 0.930/1.811\nMinibatch: 801 | Dis/Gen Cost: 1.027/1.798\nEpoch: 052 | Dis/Gen AvgCost: 0.908/1.939\nMinibatch: 001 | Dis/Gen Cost: 0.943/1.934\nMinibatch: 201 | Dis/Gen Cost: 0.583/2.514\nMinibatch: 401 | Dis/Gen Cost: 0.845/2.194\nMinibatch: 601 | Dis/Gen Cost: 0.999/1.895\nMinibatch: 801 | Dis/Gen Cost: 0.908/1.761\nEpoch: 053 | Dis/Gen AvgCost: 0.936/1.894\nMinibatch: 001 | Dis/Gen Cost: 0.725/2.493\nMinibatch: 201 | Dis/Gen Cost: 1.008/2.450\nMinibatch: 401 | Dis/Gen Cost: 1.354/1.257\nMinibatch: 601 | Dis/Gen Cost: 0.807/1.977\nMinibatch: 801 | Dis/Gen Cost: 0.902/1.763\nEpoch: 054 | Dis/Gen AvgCost: 0.930/1.894\nMinibatch: 001 | Dis/Gen Cost: 0.717/2.591\nMinibatch: 201 | Dis/Gen Cost: 0.953/1.853\nMinibatch: 401 | Dis/Gen Cost: 0.940/2.419\nMinibatch: 601 | Dis/Gen Cost: 1.136/1.866\nMinibatch: 801 | Dis/Gen Cost: 1.210/1.742\nEpoch: 055 | Dis/Gen AvgCost: 0.923/1.924\nMinibatch: 001 | Dis/Gen Cost: 0.733/2.124\nMinibatch: 201 | Dis/Gen Cost: 1.186/1.780\nMinibatch: 401 | Dis/Gen Cost: 0.827/1.658\nMinibatch: 601 | Dis/Gen Cost: 0.762/2.223\nMinibatch: 801 | Dis/Gen Cost: 0.815/1.968\nEpoch: 056 | Dis/Gen AvgCost: 0.940/1.907\nMinibatch: 001 | Dis/Gen Cost: 0.845/1.792\nMinibatch: 201 | Dis/Gen Cost: 0.955/2.223\nMinibatch: 401 | Dis/Gen Cost: 0.990/1.642\nMinibatch: 601 | Dis/Gen Cost: 0.898/1.826\nMinibatch: 801 | Dis/Gen Cost: 0.859/1.895\nEpoch: 057 | Dis/Gen AvgCost: 0.927/1.862\nMinibatch: 001 | Dis/Gen Cost: 0.781/2.240\nMinibatch: 201 | Dis/Gen Cost: 1.101/1.946\nMinibatch: 401 | Dis/Gen Cost: 1.187/2.024\nMinibatch: 601 | Dis/Gen Cost: 0.905/1.966\nMinibatch: 801 | Dis/Gen Cost: 1.126/1.619\nEpoch: 058 | Dis/Gen AvgCost: 0.954/1.853\nMinibatch: 001 | Dis/Gen Cost: 0.845/1.845\nMinibatch: 201 | Dis/Gen Cost: 0.788/1.867\nMinibatch: 401 | Dis/Gen Cost: 1.123/1.690\nMinibatch: 601 | Dis/Gen Cost: 1.035/2.213\nMinibatch: 801 | Dis/Gen Cost: 0.979/2.110\nEpoch: 059 | Dis/Gen AvgCost: 0.949/1.849\nMinibatch: 001 | Dis/Gen Cost: 0.792/2.654\nMinibatch: 201 | Dis/Gen Cost: 0.667/2.302\nMinibatch: 401 | Dis/Gen Cost: 0.745/2.209\nMinibatch: 601 | Dis/Gen Cost: 1.149/1.681\nMinibatch: 801 | Dis/Gen Cost: 0.983/1.955\nEpoch: 060 | Dis/Gen AvgCost: 0.959/1.866\nMinibatch: 001 | Dis/Gen Cost: 1.127/1.601\nMinibatch: 201 | Dis/Gen Cost: 1.102/1.860\nMinibatch: 401 | Dis/Gen Cost: 0.662/2.358\nMinibatch: 601 | Dis/Gen Cost: 0.943/1.183\nMinibatch: 801 | Dis/Gen Cost: 1.309/1.384\nEpoch: 061 | Dis/Gen AvgCost: 0.953/1.852\nMinibatch: 001 | Dis/Gen Cost: 1.039/1.489\nMinibatch: 201 | Dis/Gen Cost: 0.716/2.281\nMinibatch: 401 | Dis/Gen Cost: 0.820/2.397\nMinibatch: 601 | Dis/Gen Cost: 0.991/1.501\nMinibatch: 801 | Dis/Gen Cost: 0.784/2.214\nEpoch: 062 | Dis/Gen AvgCost: 0.950/1.820\nMinibatch: 001 | Dis/Gen Cost: 1.269/1.457\nMinibatch: 201 | Dis/Gen Cost: 1.163/2.043\nMinibatch: 401 | Dis/Gen Cost: 1.165/1.743\nMinibatch: 601 | Dis/Gen Cost: 0.872/1.554\nMinibatch: 801 | Dis/Gen Cost: 1.132/1.946\nEpoch: 063 | Dis/Gen AvgCost: 0.969/1.848\nMinibatch: 001 | Dis/Gen Cost: 1.150/1.981\nMinibatch: 201 | Dis/Gen Cost: 0.821/2.240\nMinibatch: 401 | Dis/Gen Cost: 1.118/1.780\nMinibatch: 601 | Dis/Gen Cost: 0.999/1.346\nMinibatch: 801 | Dis/Gen Cost: 1.020/1.646\nEpoch: 064 | Dis/Gen AvgCost: 0.997/1.795\nMinibatch: 001 | Dis/Gen Cost: 0.830/2.187\nMinibatch: 201 | Dis/Gen Cost: 1.153/1.105\nMinibatch: 401 | Dis/Gen Cost: 0.947/1.664\nMinibatch: 601 | Dis/Gen Cost: 0.985/2.092\nMinibatch: 801 | Dis/Gen Cost: 1.170/1.618\nEpoch: 065 | Dis/Gen AvgCost: 1.014/1.745\nMinibatch: 001 | Dis/Gen Cost: 0.777/1.826\nMinibatch: 201 | Dis/Gen Cost: 0.943/2.126\nMinibatch: 401 | Dis/Gen Cost: 1.058/1.531\nMinibatch: 601 | Dis/Gen Cost: 0.885/1.926\nMinibatch: 801 | Dis/Gen Cost: 0.901/2.076\nEpoch: 066 | Dis/Gen AvgCost: 1.026/1.729\nMinibatch: 001 | Dis/Gen Cost: 0.839/1.736\nMinibatch: 201 | Dis/Gen Cost: 1.021/1.997\nMinibatch: 401 | Dis/Gen Cost: 1.019/1.796\nMinibatch: 601 | Dis/Gen Cost: 1.079/1.548\nMinibatch: 801 | Dis/Gen Cost: 1.064/1.670\nEpoch: 067 | Dis/Gen AvgCost: 1.001/1.682\nMinibatch: 001 | Dis/Gen Cost: 1.075/1.497\nMinibatch: 201 | Dis/Gen Cost: 1.083/1.696\nMinibatch: 401 | Dis/Gen Cost: 0.947/1.769\nMinibatch: 601 | Dis/Gen Cost: 1.005/1.833\nMinibatch: 801 | Dis/Gen Cost: 0.782/2.193\nEpoch: 068 | Dis/Gen AvgCost: 0.983/1.744\nMinibatch: 001 | Dis/Gen Cost: 1.152/1.972\nMinibatch: 201 | Dis/Gen Cost: 1.032/1.782\nMinibatch: 401 | Dis/Gen Cost: 0.850/1.932\nMinibatch: 601 | Dis/Gen Cost: 0.726/2.192\nMinibatch: 801 | Dis/Gen Cost: 0.817/2.106\nEpoch: 069 | Dis/Gen AvgCost: 0.988/1.737\nMinibatch: 001 | Dis/Gen Cost: 0.824/1.608\nMinibatch: 201 | Dis/Gen Cost: 0.998/1.683\nMinibatch: 401 | Dis/Gen Cost: 0.725/2.099\nMinibatch: 601 | Dis/Gen Cost: 1.142/1.952\nMinibatch: 801 | Dis/Gen Cost: 0.984/1.919\nEpoch: 070 | Dis/Gen AvgCost: 0.998/1.747\nMinibatch: 001 | Dis/Gen Cost: 0.883/1.957\nMinibatch: 201 | Dis/Gen Cost: 1.178/1.769\nMinibatch: 401 | Dis/Gen Cost: 1.189/1.297\nMinibatch: 601 | Dis/Gen Cost: 1.033/1.601\nMinibatch: 801 | Dis/Gen Cost: 0.939/1.494\nEpoch: 071 | Dis/Gen AvgCost: 1.001/1.694\nMinibatch: 001 | Dis/Gen Cost: 1.127/2.006\nMinibatch: 201 | Dis/Gen Cost: 1.451/1.442\nMinibatch: 401 | Dis/Gen Cost: 0.867/1.620\nMinibatch: 601 | Dis/Gen Cost: 0.832/1.606\nMinibatch: 801 | Dis/Gen Cost: 0.829/1.446\nEpoch: 072 | Dis/Gen AvgCost: 1.011/1.652\nMinibatch: 001 | Dis/Gen Cost: 1.061/1.443\nMinibatch: 201 | Dis/Gen Cost: 1.061/1.460\nMinibatch: 401 | Dis/Gen Cost: 1.148/1.710\nMinibatch: 601 | Dis/Gen Cost: 1.292/1.098\nMinibatch: 801 | Dis/Gen Cost: 0.763/1.917\nEpoch: 073 | Dis/Gen AvgCost: 0.994/1.679\nMinibatch: 001 | Dis/Gen Cost: 1.115/1.742\nMinibatch: 201 | Dis/Gen Cost: 1.004/1.599\nMinibatch: 401 | Dis/Gen Cost: 0.857/1.958\nMinibatch: 601 | Dis/Gen Cost: 0.960/1.693\nMinibatch: 801 | Dis/Gen Cost: 0.933/1.855\nEpoch: 074 | Dis/Gen AvgCost: 0.998/1.711\nMinibatch: 001 | Dis/Gen Cost: 0.994/1.530\nMinibatch: 201 | Dis/Gen Cost: 1.066/1.401\nMinibatch: 401 | Dis/Gen Cost: 1.183/1.350\nMinibatch: 601 | Dis/Gen Cost: 0.774/1.700\nMinibatch: 801 | Dis/Gen Cost: 0.879/1.804\nEpoch: 075 | Dis/Gen AvgCost: 1.004/1.695\nMinibatch: 001 | Dis/Gen Cost: 0.901/2.194\nMinibatch: 201 | Dis/Gen Cost: 1.068/1.360\nMinibatch: 401 | Dis/Gen Cost: 0.954/1.416\nMinibatch: 601 | Dis/Gen Cost: 1.055/1.563\nMinibatch: 801 | Dis/Gen Cost: 1.022/1.478\nEpoch: 076 | Dis/Gen AvgCost: 1.015/1.655\nMinibatch: 001 | Dis/Gen Cost: 0.928/1.833\nMinibatch: 201 | Dis/Gen Cost: 0.859/1.379\nMinibatch: 401 | Dis/Gen Cost: 0.904/1.730\nMinibatch: 601 | Dis/Gen Cost: 0.885/1.659\nMinibatch: 801 | Dis/Gen Cost: 1.044/1.927\nEpoch: 077 | Dis/Gen AvgCost: 1.022/1.605\nMinibatch: 001 | Dis/Gen Cost: 1.160/1.570\nMinibatch: 201 | Dis/Gen Cost: 0.931/1.476\nMinibatch: 401 | Dis/Gen Cost: 0.823/1.512\nMinibatch: 601 | Dis/Gen Cost: 1.116/1.255\nMinibatch: 801 | Dis/Gen Cost: 1.183/1.266\nEpoch: 078 | Dis/Gen AvgCost: 1.026/1.650\nMinibatch: 001 | Dis/Gen Cost: 1.629/0.871\nMinibatch: 201 | Dis/Gen Cost: 1.250/1.815\nMinibatch: 401 | Dis/Gen Cost: 0.970/1.700\nMinibatch: 601 | Dis/Gen Cost: 1.197/1.494\nMinibatch: 801 | Dis/Gen Cost: 0.900/1.601\nEpoch: 079 | Dis/Gen AvgCost: 1.034/1.617\nMinibatch: 001 | Dis/Gen Cost: 0.858/1.931\nMinibatch: 201 | Dis/Gen Cost: 1.006/1.475\nMinibatch: 401 | Dis/Gen Cost: 0.919/1.850\nMinibatch: 601 | Dis/Gen Cost: 1.125/1.624\nMinibatch: 801 | Dis/Gen Cost: 0.968/1.406\nEpoch: 080 | Dis/Gen AvgCost: 1.009/1.686\nMinibatch: 001 | Dis/Gen Cost: 0.773/2.030\nMinibatch: 201 | Dis/Gen Cost: 1.064/1.797\nMinibatch: 401 | Dis/Gen Cost: 0.832/1.732\nMinibatch: 601 | Dis/Gen Cost: 1.010/1.615\nMinibatch: 801 | Dis/Gen Cost: 0.969/1.654\nEpoch: 081 | Dis/Gen AvgCost: 1.027/1.616\nMinibatch: 001 | Dis/Gen Cost: 1.002/1.366\nMinibatch: 201 | Dis/Gen Cost: 0.849/1.789\nMinibatch: 401 | Dis/Gen Cost: 0.868/1.846\nMinibatch: 601 | Dis/Gen Cost: 0.867/2.013\nMinibatch: 801 | Dis/Gen Cost: 0.996/1.670\nEpoch: 082 | Dis/Gen AvgCost: 1.022/1.660\nMinibatch: 001 | Dis/Gen Cost: 0.807/1.946\nMinibatch: 201 | Dis/Gen Cost: 0.941/2.055\nMinibatch: 401 | Dis/Gen Cost: 1.230/1.317\nMinibatch: 601 | Dis/Gen Cost: 0.973/1.707\nMinibatch: 801 | Dis/Gen Cost: 0.875/2.133\nEpoch: 083 | Dis/Gen AvgCost: 1.003/1.661\nMinibatch: 001 | Dis/Gen Cost: 1.197/1.645\nMinibatch: 201 | Dis/Gen Cost: 1.049/1.379\nMinibatch: 401 | Dis/Gen Cost: 1.005/1.732\nMinibatch: 601 | Dis/Gen Cost: 1.148/1.651\nMinibatch: 801 | Dis/Gen Cost: 1.042/1.556\nEpoch: 084 | Dis/Gen AvgCost: 1.026/1.665\nMinibatch: 001 | Dis/Gen Cost: 1.112/1.451\nMinibatch: 201 | Dis/Gen Cost: 0.870/2.034\nMinibatch: 401 | Dis/Gen Cost: 0.983/1.714\nMinibatch: 601 | Dis/Gen Cost: 1.010/1.791\nMinibatch: 801 | Dis/Gen Cost: 0.874/1.963\nEpoch: 085 | Dis/Gen AvgCost: 1.026/1.631\nMinibatch: 001 | Dis/Gen Cost: 1.124/1.356\nMinibatch: 201 | Dis/Gen Cost: 1.283/1.486\nMinibatch: 401 | Dis/Gen Cost: 0.769/1.989\nMinibatch: 601 | Dis/Gen Cost: 1.148/1.516\nMinibatch: 801 | Dis/Gen Cost: 1.093/1.796\nEpoch: 086 | Dis/Gen AvgCost: 1.032/1.603\nMinibatch: 001 | Dis/Gen Cost: 1.169/1.242\nMinibatch: 201 | Dis/Gen Cost: 1.099/1.615\nMinibatch: 401 | Dis/Gen Cost: 1.027/1.576\nMinibatch: 601 | Dis/Gen Cost: 0.879/2.036\nMinibatch: 801 | Dis/Gen Cost: 0.998/1.492\nEpoch: 087 | Dis/Gen AvgCost: 1.032/1.594\nMinibatch: 001 | Dis/Gen Cost: 1.252/1.457\nMinibatch: 201 | Dis/Gen Cost: 0.923/1.976\nMinibatch: 401 | Dis/Gen Cost: 0.801/1.904\nMinibatch: 601 | Dis/Gen Cost: 0.943/1.597\nMinibatch: 801 | Dis/Gen Cost: 0.896/1.933\nEpoch: 088 | Dis/Gen AvgCost: 1.016/1.639\nMinibatch: 001 | Dis/Gen Cost: 1.161/1.404\nMinibatch: 201 | Dis/Gen Cost: 0.913/1.878\nMinibatch: 401 | Dis/Gen Cost: 0.874/1.717\nMinibatch: 601 | Dis/Gen Cost: 0.996/1.397\nMinibatch: 801 | Dis/Gen Cost: 0.812/1.427\nEpoch: 089 | Dis/Gen AvgCost: 1.014/1.612\nMinibatch: 001 | Dis/Gen Cost: 0.893/1.509\nMinibatch: 201 | Dis/Gen Cost: 1.005/1.585\nMinibatch: 401 | Dis/Gen Cost: 0.856/1.956\nMinibatch: 601 | Dis/Gen Cost: 1.010/1.746\nMinibatch: 801 | Dis/Gen Cost: 1.047/1.875\nEpoch: 090 | Dis/Gen AvgCost: 1.025/1.643\nMinibatch: 001 | Dis/Gen Cost: 1.080/1.830\nMinibatch: 201 | Dis/Gen Cost: 1.377/1.294\nMinibatch: 401 | Dis/Gen Cost: 1.259/1.133\nMinibatch: 601 | Dis/Gen Cost: 1.072/1.199\nMinibatch: 801 | Dis/Gen Cost: 0.921/2.191\nEpoch: 091 | Dis/Gen AvgCost: 1.025/1.574\nMinibatch: 001 | Dis/Gen Cost: 0.816/2.002\nMinibatch: 201 | Dis/Gen Cost: 1.147/1.551\nMinibatch: 401 | Dis/Gen Cost: 0.939/1.615\nMinibatch: 601 | Dis/Gen Cost: 0.927/1.692\nMinibatch: 801 | Dis/Gen Cost: 1.391/1.163\nEpoch: 092 | Dis/Gen AvgCost: 1.026/1.647\nMinibatch: 001 | Dis/Gen Cost: 0.821/2.085\nMinibatch: 201 | Dis/Gen Cost: 0.929/1.393\nMinibatch: 401 | Dis/Gen Cost: 0.878/1.617\nMinibatch: 601 | Dis/Gen Cost: 1.245/1.325\nMinibatch: 801 | Dis/Gen Cost: 1.040/1.512\nEpoch: 093 | Dis/Gen AvgCost: 1.028/1.621\nMinibatch: 001 | Dis/Gen Cost: 1.150/1.441\nMinibatch: 201 | Dis/Gen Cost: 0.919/1.795\nMinibatch: 401 | Dis/Gen Cost: 1.092/1.452\nMinibatch: 601 | Dis/Gen Cost: 1.004/1.484\nMinibatch: 801 | Dis/Gen Cost: 0.763/1.644\nEpoch: 094 | Dis/Gen AvgCost: 1.048/1.578\nMinibatch: 001 | Dis/Gen Cost: 1.226/1.486\nMinibatch: 201 | Dis/Gen Cost: 1.084/1.420\nMinibatch: 401 | Dis/Gen Cost: 1.137/1.446\nMinibatch: 601 | Dis/Gen Cost: 1.286/1.335\nMinibatch: 801 | Dis/Gen Cost: 1.102/1.262\nEpoch: 095 | Dis/Gen AvgCost: 1.043/1.560\nMinibatch: 001 | Dis/Gen Cost: 1.001/1.547\nMinibatch: 201 | Dis/Gen Cost: 1.143/1.308\nMinibatch: 401 | Dis/Gen Cost: 1.040/1.441\nMinibatch: 601 | Dis/Gen Cost: 1.056/1.368\nMinibatch: 801 | Dis/Gen Cost: 1.102/1.857\nEpoch: 096 | Dis/Gen AvgCost: 1.042/1.605\nMinibatch: 001 | Dis/Gen Cost: 0.990/1.628\nMinibatch: 201 | Dis/Gen Cost: 1.153/1.578\nMinibatch: 401 | Dis/Gen Cost: 1.261/1.422\nMinibatch: 601 | Dis/Gen Cost: 1.132/1.440\nMinibatch: 801 | Dis/Gen Cost: 1.113/1.186\nEpoch: 097 | Dis/Gen AvgCost: 1.036/1.596\nMinibatch: 001 | Dis/Gen Cost: 0.843/1.715\nMinibatch: 201 | Dis/Gen Cost: 0.859/1.627\nMinibatch: 401 | Dis/Gen Cost: 1.338/1.386\nMinibatch: 601 | Dis/Gen Cost: 1.175/1.362\nMinibatch: 801 | Dis/Gen Cost: 0.981/1.795\nEpoch: 098 | Dis/Gen AvgCost: 1.043/1.522\nMinibatch: 001 | Dis/Gen Cost: 1.207/1.046\nMinibatch: 201 | Dis/Gen Cost: 0.825/2.073\nMinibatch: 401 | Dis/Gen Cost: 0.993/1.592\nMinibatch: 601 | Dis/Gen Cost: 0.900/1.328\nMinibatch: 801 | Dis/Gen Cost: 1.460/0.950\nEpoch: 099 | Dis/Gen AvgCost: 1.031/1.576\nMinibatch: 001 | Dis/Gen Cost: 1.285/1.358\nMinibatch: 201 | Dis/Gen Cost: 0.938/1.483\nMinibatch: 401 | Dis/Gen Cost: 1.046/1.356\nMinibatch: 601 | Dis/Gen Cost: 0.920/1.609\nMinibatch: 801 | Dis/Gen Cost: 0.862/1.739\nEpoch: 100 | Dis/Gen AvgCost: 1.030/1.569\n"
],
[
"%matplotlib inline\nimport matplotlib.pyplot as plt\n\nplt.plot(range(len(avg_costs['discriminator'])), \n avg_costs['discriminator'], label='discriminator')\nplt.plot(range(len(avg_costs['generator'])),\n avg_costs['generator'], label='generator')\nplt.legend()\nplt.show()",
"_____no_output_____"
],
[
"####################################\n### RELOAD & GENERATE SAMPLE IMAGES\n####################################\n\n\nn_examples = 25\n\nwith tf.Session(graph=g) as sess:\n saver.restore(sess, save_path='./gan.ckpt')\n\n batch_randsample = np.random.uniform(-1, 1, size=(n_examples, gen_input_size))\n new_examples = sess.run('generator/generator_output:0',\n feed_dict={'generator_input:0': batch_randsample,\n 'dropout:0': 0.0,\n 'is_training:0': False})\n\nfig, axes = plt.subplots(nrows=5, ncols=5, figsize=(8, 8),\n sharey=True, sharex=True)\n\nfor image, ax in zip(new_examples, axes.flatten()):\n ax.imshow(image.reshape((dis_input_size // 28, dis_input_size // 28)), cmap='binary')\n\nplt.show()",
"_____no_output_____"
]
]
] | [
"markdown",
"code",
"markdown",
"code"
] | [
[
"markdown"
],
[
"code"
],
[
"markdown",
"markdown"
],
[
"code",
"code",
"code",
"code",
"code"
]
] |
d08d9ed1c316b247adbc725240ec7492e52c450e | 50,589 | ipynb | Jupyter Notebook | Data-Science-HYD-2k19/Projects/codes/PROJECT 1 (Jupiter ) ( Bank Nifty )/Merge BankNift and Data/BankNifty-Trial1-Copy1.ipynb | Sanjay9921/Python | 05ac161dd46f9b4731a5c14ff5ef52adb705e8e6 | [
"MIT"
] | null | null | null | Data-Science-HYD-2k19/Projects/codes/PROJECT 1 (Jupiter ) ( Bank Nifty )/Merge BankNift and Data/BankNifty-Trial1-Copy1.ipynb | Sanjay9921/Python | 05ac161dd46f9b4731a5c14ff5ef52adb705e8e6 | [
"MIT"
] | null | null | null | Data-Science-HYD-2k19/Projects/codes/PROJECT 1 (Jupiter ) ( Bank Nifty )/Merge BankNift and Data/BankNifty-Trial1-Copy1.ipynb | Sanjay9921/Python | 05ac161dd46f9b4731a5c14ff5ef52adb705e8e6 | [
"MIT"
] | null | null | null | 30.809379 | 192 | 0.317935 | [
[
[
"# Functions used: ",
"_____no_output_____"
],
[
"1. importing csv files\n\n2. Changing directory\n\n3. Reading only specific columns into the dataframe\n\n4. Using del [ to delete a column ]\n\n5. Using .drop() [ to delete multiple columns ]\n\n6. Using .set_index()",
"_____no_output_____"
],
[
"# 1. Import The csv files: ",
"_____no_output_____"
]
],
[
[
"import pandas as pd\nimport numpy as np",
"_____no_output_____"
],
[
"link = \"C:\\\\Users\\\\MAHE\\\\Desktop\\\\Data Science\\\\Projects\\\\data\\\\banknifty\"",
"_____no_output_____"
]
],
[
[
"# 2. Get the current working directory to the location of the data: ",
"_____no_output_____"
]
],
[
[
"import os",
"_____no_output_____"
],
[
"os.getcwd()",
"_____no_output_____"
],
[
"os.chdir(link)",
"_____no_output_____"
]
],
[
[
"# 3. Read the csv file ",
"_____no_output_____"
],
[
"## df = data frame ",
"_____no_output_____"
]
],
[
[
"df = pd.read_csv(\"all_here.csv\", sep = \",\")",
"_____no_output_____"
],
[
"df.head()",
"_____no_output_____"
],
[
"df.tail()",
"_____no_output_____"
]
],
[
[
"# 4. Reading the \"CE\" and \"PE\" from the columns to their resp. df: ",
"_____no_output_____"
],
[
"## a. Reading \"CE\" into df_ce: ",
"_____no_output_____"
]
],
[
[
"df_ce = df[df[\"Option Type\"]==\"CE\"]",
"_____no_output_____"
],
[
"df_ce.head()",
"_____no_output_____"
],
[
"df_ce.tail()",
"_____no_output_____"
]
],
[
[
"## b. Reading \"PE\" into df_pe:",
"_____no_output_____"
]
],
[
[
"df_pe = df[df[\"Option Type\"]==\"PE\"]",
"_____no_output_____"
],
[
"df_pe.head()",
"_____no_output_____"
],
[
"df_pe.tail()",
"_____no_output_____"
]
],
[
[
"# 5. Discarding irrelevant columns: ",
"_____no_output_____"
],
[
"## [ keep ,Expiry Date, Strike Price, Open, High, Low, Close ] ",
"_____no_output_____"
],
[
"### Deleting only 1 column at a time: ",
"_____no_output_____"
],
[
"### [ del ] ",
"_____no_output_____"
]
],
[
[
"del df_ce[\"Symbol\"]",
"_____no_output_____"
]
],
[
[
"### Deleting multiple columns at a time: ",
"_____no_output_____"
],
[
"### [ .drop([\"..\"],axis = 1) ] ",
"_____no_output_____"
]
],
[
[
"df_ce = df_ce.drop([\"Date\",\"LTP\",\"Settle Price\",\"No. of contracts\",\"Turnover in Lacs\",\"Premium Turnover in Lacs\",\"Open Int\",\"Change in OI\",\"Underlying Value\"],axis = 1)",
"_____no_output_____"
],
[
"df_pe = df_pe.drop([\"Date\",\"LTP\",\"Settle Price\",\"No. of contracts\",\"Turnover in Lacs\",\"Premium Turnover in Lacs\",\"Open Int\",\"Change in OI\",\"Underlying Value\"],axis = 1)",
"_____no_output_____"
]
],
[
[
"# 6. Set Expiry date as the index:",
"_____no_output_____"
],
[
"## [ set_index() ]",
"_____no_output_____"
]
],
[
[
"df_ce.set_index(\"Expiry\",inplace = True)",
"_____no_output_____"
],
[
"df_ce.head()",
"_____no_output_____"
],
[
"df_pe.set_index(\"Expiry\",inplace = True)",
"_____no_output_____"
],
[
"df_pe.head()",
"_____no_output_____"
]
]
] | [
"markdown",
"code",
"markdown",
"code",
"markdown",
"code",
"markdown",
"code",
"markdown",
"code",
"markdown",
"code",
"markdown",
"code",
"markdown",
"code"
] | [
[
"markdown",
"markdown",
"markdown"
],
[
"code",
"code"
],
[
"markdown"
],
[
"code",
"code",
"code"
],
[
"markdown",
"markdown"
],
[
"code",
"code",
"code"
],
[
"markdown",
"markdown"
],
[
"code",
"code",
"code"
],
[
"markdown"
],
[
"code",
"code",
"code"
],
[
"markdown",
"markdown",
"markdown",
"markdown"
],
[
"code"
],
[
"markdown",
"markdown"
],
[
"code",
"code"
],
[
"markdown",
"markdown"
],
[
"code",
"code",
"code",
"code"
]
] |
d08da5e78ea61aba3d615021b9926d5941123e07 | 204,329 | ipynb | Jupyter Notebook | modeling/04_prediction_time_series.ipynb | zheng-da/covid19-severity-prediction | 205ab5aa13a5e91a4c23ccd73e65939e4003626b | [
"MIT"
] | 2 | 2020-05-15T14:42:02.000Z | 2020-05-22T08:51:47.000Z | modeling/04_prediction_time_series.ipynb | zheng-da/covid19-severity-prediction | 205ab5aa13a5e91a4c23ccd73e65939e4003626b | [
"MIT"
] | null | null | null | modeling/04_prediction_time_series.ipynb | zheng-da/covid19-severity-prediction | 205ab5aa13a5e91a4c23ccd73e65939e4003626b | [
"MIT"
] | null | null | null | 200.71611 | 16,668 | 0.887334 | [
[
[
"%load_ext autoreload\n%autoreload 2\nimport sklearn\nimport numpy as np\nimport scipy as sp\nimport pandas as pd\nimport matplotlib.pyplot as plt\nimport matplotlib.dates as mdates\nimport seaborn as sns\n#from viz import viz\nfrom bokeh.plotting import figure, show, output_notebook, output_file, save\n#from functions import merge_data\nfrom sklearn.model_selection import RandomizedSearchCV\n#import load_data\n\nfrom sklearn.linear_model import LinearRegression\nfrom sklearn.tree import DecisionTreeRegressor\nfrom sklearn.ensemble import RandomForestRegressor",
"The autoreload extension is already loaded. To reload it, use:\n %reload_ext autoreload\n"
],
[
"# 'deaths' and 'cases' contain the time-series of the outbreak\ndf = load_data.load_county_level()\ndf = df.sort_values(load_data.outcome_deaths, ascending=False)\noutcome_cases = 'tot_cases'\noutcome_deaths = 'tot_deaths'\nimportant_vars = load_data.important_keys(df)",
"_____no_output_____"
],
[
"def sum_lists(list_of_lists):\n arr = np.array(list(list_of_lists))\n sum_arr = np.sum(arr,0)\n return list(sum_arr)",
"_____no_output_____"
],
[
"# # Aggregate by State\n# state_deaths_df = df.groupby('StateNameAbbreviation').deaths.agg(sum_lists).to_frame()\n# state_cases_df = df.groupby('StateNameAbbreviation').cases.agg(sum_lists).to_frame()\n# df = pd.concat([state_cases_df,state_deaths_df],axis =1 )",
"_____no_output_____"
],
[
"# This is investigating the number of cases associated with non-zero deaths in a county\n_deaths = list(df['deaths'])\n_cases = list(df['cases'])\ntotal_points = []\n\ncases_for_death = []\nfor i in range(len(df)):\n for j,d in enumerate(_deaths[i]):\n if d > 0:\n\n cases_for_death.append(_cases[i][j])\n if _cases[i][j] == 0:\n print(i)\n \nplt.hist(cases_for_death)\nprint(np.mean(cases_for_death))\nprint(np.quantile(cases_for_death,.5))",
"42\n64\n119\n507\n910\n118.61635944700461\n16.0\n"
],
[
"# Distribution of the maximum number of cases\n_cases = list(df['cases'])\n\nmax_cases = []\nfor i in range(len(df)):\n max_cases.append(max(_cases[i]))\n \nprint(sum([v >0 for v in max_cases]))\n# plt.hist(max_cases)\n\n# print(sum([v >0 for v in max_cases]))\nplt.hist([v for v in max_cases if v > 20 and v < 1000],bins = 100)\n",
"1204\n"
],
[
"print(sum([v > 50 for v in max_cases]))\n",
"94\n"
],
[
"np.quantile(max_cases,1)",
"_____no_output_____"
],
[
"# Distribution of the maximum number of cases\n_deaths = list(df['deaths'])\n\nmax_deaths = []\nfor i in range(len(df)):\n max_deaths.append(max(_deaths[i]))\n \nprint(sum([v >0 for v in max_deaths]))\n# plt.hist(max_cases)\n\n# print(sum([v >0 for v in max_cases]))\nplt.hist([v for v in max_deaths if v > 1],bins=30)",
"186\n"
],
[
"np.quantile(max_deaths,.9)",
"_____no_output_____"
]
],
[
[
"### Clean data",
"_____no_output_____"
]
],
[
[
"# Remove rows with zero cases\nmax_cases = [max(v) for v in df['cases']]\ndf['max_cases'] = max_cases\ndf_with_cases = df[df['max_cases'] > 0]\n",
"_____no_output_____"
],
[
"# Shuffle data\nshuffled_df = df_with_cases.sample(frac=1)\n# Break into train test (random k-fold cross val on the training set is done to pick hyperparams)\ntrain_ratio, val_ratio, test_ratio = .75,0,.25\n\n\ntrain_df = shuffled_df[0:int(train_ratio*len(shuffled_df))]\n# val_df = shuffled_df[int(train_ratio*len(shuffled_df)):int(val_ratio*len(shuffled_df))+int(train_ratio*len(shuffled_df))]\ntest_df = shuffled_df[int(train_ratio*len(shuffled_df))+int(val_ratio*len(shuffled_df)):]\n\n",
"_____no_output_____"
],
[
"def make_auto_regressive_dataset(df,autoreg_window,log=True,deaths=True,cases=False,predict_deaths=True):\n \"\"\"\n Make an autoregressive dataset that takes in a dataframe and a history window to predict number of deaths\n for a given day given a history of autoreg_window days before it\n log: take logarithm of values for features and predictions\n deaths: use number of previous deaths as features\n cases: use number of previous cases as features\n predict_deaths: predict deaths otherwise predict cases\n \"\"\"\n\n assert (deaths == True or cases == True)\n feature_array = []\n ys = []\n _cases = list(df['cases'])\n _deaths = list(df['deaths'])\n for i in range(len(_cases)):\n for j in range(len(_cases[i])-(autoreg_window+1)):\n if predict_deaths:\n contains_event = sum(_deaths[i][j:j+autoreg_window+1]) > 0\n else:\n contains_event = sum(_cases[i][j:j+autoreg_window+1]) > 0\n if contains_event > 0:\n cases_window = _cases[i][j:j+autoreg_window]\n if log:\n cases_window = [np.log(v+1) for v in cases_window ]\n deaths_window = _deaths[i][j:j+autoreg_window]\n if log:\n deaths_window = [np.log(v+1) for v in deaths_window]\n if predict_deaths:\n y_val = _deaths[i][j+autoreg_window+1]\n else:\n y_val = _cases[i][j+autoreg_window+1]\n if log:\n y_val = np.log(y_val+1)\n features = []\n if deaths == True:\n features.extend(deaths_window)\n if cases == True:\n features.extend(cases_window)\n feature_array.append(features)\n ys.append(y_val)\n return feature_array, ys\n \n ",
"_____no_output_____"
],
[
"def evaluate_model(model,eval_pair, metric, exponentiate=False):\n \"\"\"\n Model: sklearn model\n Eval pair: (x,y)\n metric: sklearn metric\n exponentiate: exponentiate model predictions?\n \"\"\"\n predictions = model.predict(eval_pair[0])\n y_val = eval_pair[1]\n if exponentiate:\n predictions = [np.exp(p) for p in predictions]\n y_val = [np.exp(y) for y in y_val]\n return predictions, metric(predictions,y_val)\n \n\n\n \n ",
"_____no_output_____"
],
[
"model = sklearn.neighbors.KNeighborsRegressor()\nparam_dist ={\n 'n_neighbors': [2,4,8,16],\n 'weights': ['uniform','distance'],\n 'p': [1,2,4]\n}\n\n# model = RandomForestRegressor()\n# param_dist ={\n# 'n_estimators': [50,100,200,400,1000]\n# }\n# Number of randomly sampled hyperparams\nn_iter = 20\nmetric = sklearn.metrics.mean_squared_error\n# n_jobs = number of cores to parallelize across\nrandom_search = RandomizedSearchCV(model, param_distributions=param_dist,\n n_iter=n_iter,n_jobs = 8)\npredict_deaths = False\n\n\n\nauto_reg_windows = [1,2,4,8]\nbest_window = None\nbest_loss = None\nfor w in auto_reg_windows:\n log = False\n x_train, y_train = make_auto_regressive_dataset(train_df,w,log=log,predict_deaths=predict_deaths)\n x_test, y_test = make_auto_regressive_dataset(test_df,w,log=log,predict_deaths=predict_deaths)\n random_search.fit(x_train,y_train)\n window_loss = random_search.best_score_\n if best_loss is None:\n best_window = w\n best_loss = window_loss\n elif window_loss < best_loss:\n best_window = w\n best_score = loss\n \n\n\nx_train, y_train = make_auto_regressive_dataset(train_df,best_window,log=log)\nx_test, y_test = make_auto_regressive_dataset(test_df,best_window,log=log)\nrandom_search.fit(x_train,y_train)\n\n\npreds, loss = evaluate_model(random_search,(x_test,y_test),metric,exponentiate=True)\n \n \n\n# model.fit(x_train,y_train)",
"_____no_output_____"
],
[
"random_search.best_params_",
"_____no_output_____"
],
[
"best_window",
"_____no_output_____"
],
[
"loss",
"_____no_output_____"
],
[
"# WARNING: does not yet supported number of previous cases as feature\ndef get_auto_reg_predictions(model,row,window,teacher_forcing=True,exponentiate=False,predict_deaths=True):\n if predict_deaths:\n key = 'deaths'\n else:\n key = 'cases'\n \n deaths = row[key]\n predictions = [0]\n if teacher_forcing:\n for i in range(len(deaths)-(window)):\n x = deaths[i:i+window]\n cur_prediction = model.predict([x])\n if exponentiate:\n cur_prediction = np.exp(cur_prediction)\n predictions.append(cur_prediction)\n else:\n raise NotImplementedError\n return predictions\n \ndef plot_prediction(model,row,window,exponentiate=False,predict_deaths=True):\n \"\"\"\n Plots model predictions vs actual\n row: dataframe row\n window: autoregressive window size\n \"\"\"\n if predict_deaths:\n key = 'deaths'\n else:\n key = 'cases'\n \n model_predictions = get_auto_reg_predictions(model,row,window,exponentiate,predict_deaths=predict_deaths)\n model_predictions = [float(v) for v in model_predictions]\n print(model_predictions)\n for i,val in enumerate(row[key]):\n if val > 0:\n start_point = i\n break\n plt.plot(row[key][start_point:], label=key)\n\n \n plt.plot(model_predictions[start_point:],label='predictions')\n print(model_predictions[start_point:])\n plt.fill_between(list(range(len(row[key][start_point:]))),row[key][start_point:],model_predictions[start_point:])\n plt.legend()\n plt.show()\n \n \n \n \n ",
"_____no_output_____"
],
[
"for i in range(len(test_df)):\n row = test_df.iloc[i]\n if max(row['deaths'][:-1]) > 1:\n plot_prediction(random_search,row,best_window,exponentiate=True,predict_deaths=predict_deaths)",
"[0.0, 1.0, 1.0, 1.0, 1.0, 1.0, 1.0, 1.0, 1.0, 1.0, 1.0, 1.0, 1.0, 1.0, 1.0, 1.0, 1.0, 1.0, 1.0, 1.0, 1.0, 1.0, 1.0, 1.0, 1.0, 1.0, 1.0, 1.0, 1.0, 1.0, 1.0, 1.0, 1.0, 1.0, 1.0, 1.0, 1.0, 1.0, 1.0, 1.0, 1.0, 1.0, 1.0, 1.0, 1.0, 1.0, 1.0, 1.0, 1.0, 1.0, 1.0, 1.0, 0.625, 0.625, 3.375, 1.0, 1.0, 1.0, 1.0, 1.0, 1.0, 0.625]\n[1.0, 0.625, 0.625, 3.375, 1.0, 1.0, 1.0, 1.0, 1.0, 1.0, 0.625]\n"
]
],
[
[
"## Predict deaths from cases",
"_____no_output_____"
]
],
[
[
"def create_case_to_death_data(df):\n _cases = []\n _deaths = []\n _y_deaths = []\n for i in range(len(df)):\n row = df.iloc[i]\n deaths = row['deaths']\n cases = row['cases']\n for j in range(len(deaths)):\n if cases[j] > 0:\n _cases.append(cases[j])\n if j == 0:\n _deaths.append(0)\n else:\n _deaths.append(deaths[j-1])\n\n _y_deaths.append(deaths[j])\n return (_cases,_deaths,_y_deaths)\n\ntrain_cases, train_deaths, train_y_deaths = create_case_to_death_data(train_df)\ntest_cases, test_deaths, test_y_deaths = create_case_to_death_data(test_df)\n",
"_____no_output_____"
],
[
"model = RandomForestRegressor()\nparam_dist ={\n 'n_estimators': [50,100,200,400,1000]\n}\n\nmetric = sklearn.metrics.mean_squared_error\n# n_jobs = number of cores to parallelize across\ndeaths_random_search = RandomizedSearchCV(model, param_distributions=param_dist,\n n_iter=n_iter,n_jobs = 8)\n\ndeaths_random_search.fit(list(zip(train_cases,train_deaths)),train_y_deaths)",
"/Users/nick/anaconda2/envs/covid/lib/python3.6/site-packages/sklearn/model_selection/_search.py:281: UserWarning:\n\nThe total space of parameters 5 is smaller than n_iter=20. Running 5 iterations. For exhaustive searches, use GridSearchCV.\n\n"
],
[
"pred_deaths = deaths_random_search.predict(list(zip(test_cases,test_deaths)))",
"_____no_output_____"
],
[
"metric(pred_deaths,test_y_deaths)",
"_____no_output_____"
],
[
"row = df.iloc[0]",
"_____no_output_____"
],
[
"plt.plot(row['deaths'], label='deaths')\nplt.plot(row['cases'], label='cases')\nplt.legend()\nplt.show()",
"_____no_output_____"
]
]
] | [
"code",
"markdown",
"code",
"markdown",
"code"
] | [
[
"code",
"code",
"code",
"code",
"code",
"code",
"code",
"code",
"code",
"code"
],
[
"markdown"
],
[
"code",
"code",
"code",
"code",
"code",
"code",
"code",
"code",
"code",
"code"
],
[
"markdown"
],
[
"code",
"code",
"code",
"code",
"code",
"code"
]
] |
d08db9559ab24eb23d2cacdac932d3af7a9b6585 | 3,634 | ipynb | Jupyter Notebook | templates/Template-BinaryClassificationAssessment.ipynb | masino-lab/fairMLHealth | 943ffed5f57997401823bd2afc257f34f76ea157 | [
"MIT"
] | 19 | 2020-10-29T10:14:59.000Z | 2022-03-20T06:27:35.000Z | templates/Template-BinaryClassificationAssessment.ipynb | masino-lab/fairMLHealth | 943ffed5f57997401823bd2afc257f34f76ea157 | [
"MIT"
] | 52 | 2020-10-14T19:21:27.000Z | 2021-09-15T19:01:52.000Z | templates/Template-BinaryClassificationAssessment.ipynb | masino-lab/fairMLHealth | 943ffed5f57997401823bd2afc257f34f76ea157 | [
"MIT"
] | 9 | 2020-12-02T21:40:27.000Z | 2021-11-01T18:09:10.000Z | 24.721088 | 195 | 0.573198 | [
[
[
"# Binary Classification Fairness Assessment Template\n\nUse this template as a skeleton for comparing fairness and performance measures across a set of trained binary classification models.\n",
"_____no_output_____"
]
],
[
[
"# Recommended list of libraries (optional unless otherwise specified)\nfrom fairmlhealth import report, measure # Required\nimport os\nimport pandas as pd",
"_____no_output_____"
]
],
[
[
"----\n----\n# Load (or Generate) Data and Models\n\nHere you should load (or generate) your test dataset and models.",
"_____no_output_____"
]
],
[
[
"# < Optional Loading/Cleaning/Training Code Here >",
"_____no_output_____"
]
],
[
[
"----\n----\n# Evaluate a Single (Baseline) Model",
"_____no_output_____"
],
[
"## Set the Required Variables \n\n* X (NumPy array or similar pandas object): test data to be passed to the models to generate predictions. It's recommended that these be separate data from those used to train the model.\n\n* y (NumPy array or similar pandas object): target data array corresponding to X. It is recommended that the target is not present in the test data.\n\n* PA (NumPy array or similar pandas object): protected attributes corresponding to X, optionally also included in X. Note that values must currently be binary- or Boolean-type.\n\n* model: the trained model to be evaluated. \n",
"_____no_output_____"
]
],
[
[
"# Set Pointers to be Passed to the Comparison Tools\nX = None # <- add your test data \ny = None # <- add your test labels \nPA = None # if the protected attribute(s) is not present in the data, \nmodel = None # add a dict or a list of trained, scikit-compatible models",
"_____no_output_____"
]
],
[
[
"----",
"_____no_output_____"
]
],
[
[
"report.compare(X, y, PA, model, pred_type = \"classificationmeasure.data(X, y)\")",
"_____no_output_____"
],
[
"measure.data(X, y)",
"_____no_output_____"
],
[
"measure.performance(X, y, y_pred=model.predict(X), y_prob=model_1.predict_proba(X_test), pred_type=\"classification\")",
"_____no_output_____"
],
[
"measure.bias(X, y, model.predict(X), pred_type=\"classification\")",
"_____no_output_____"
]
]
] | [
"markdown",
"code",
"markdown",
"code",
"markdown",
"code",
"markdown",
"code"
] | [
[
"markdown"
],
[
"code"
],
[
"markdown"
],
[
"code"
],
[
"markdown",
"markdown"
],
[
"code"
],
[
"markdown"
],
[
"code",
"code",
"code",
"code"
]
] |
d08dc0465a85c9e9e8e2c531cf5b5d0b30752e49 | 591 | ipynb | Jupyter Notebook | hello.ipynb | BugbearR/jupyter-playground | 8df8de7bc09c5f47f52995bfd923ba909501930f | [
"CC0-1.0"
] | null | null | null | hello.ipynb | BugbearR/jupyter-playground | 8df8de7bc09c5f47f52995bfd923ba909501930f | [
"CC0-1.0"
] | null | null | null | hello.ipynb | BugbearR/jupyter-playground | 8df8de7bc09c5f47f52995bfd923ba909501930f | [
"CC0-1.0"
] | null | null | null | 15.552632 | 35 | 0.483926 | [
[
[
"empty"
]
]
] | [
"empty"
] | [
[
"empty"
]
] |
d08dcf9506a7c09fd8e064bfc5575485047caa80 | 29,407 | ipynb | Jupyter Notebook | notebooks/process_planet_scenes.ipynb | tclavelle/aqua_python | 35f87aafc3eacf565f7f955ea2af61c7fa2db22c | [
"MIT"
] | 3 | 2018-08-21T20:18:02.000Z | 2022-03-13T11:36:44.000Z | notebooks/process_planet_scenes.ipynb | tclavelle/aqua_python | 35f87aafc3eacf565f7f955ea2af61c7fa2db22c | [
"MIT"
] | 1 | 2018-07-09T23:05:06.000Z | 2018-07-09T23:31:16.000Z | notebooks/process_planet_scenes.ipynb | tclavelle/aqua_python | 35f87aafc3eacf565f7f955ea2af61c7fa2db22c | [
"MIT"
] | 2 | 2018-06-26T22:30:30.000Z | 2019-10-09T23:31:11.000Z | 43.501479 | 354 | 0.587683 | [
[
[
"# Packages\nfrom IPython.display import Image\nimport rasterio\nfrom rasterio import windows\nimport skimage\nimport skimage.io as skio\nimport json\nimport skimage.draw\nimport os\nimport sys\nimport pathlib\nimport math\nimport itertools\nfrom shutil import copy2\nimport functools\nfrom skimage import exposure\nimport matplotlib.pyplot as plt\nimport matplotlib.image as mpimg\nimport numpy as np\nimport pandas as pd\nfrom rasterio.plot import show\nfrom osgeo import gdal",
"_____no_output_____"
],
[
"# Get absolute file paths. Returns generator object\ndef absoluteFilePaths(directory):\n for dirpath,_,filenames in os.walk(directory):\n for f in filenames:\n yield os.path.abspath(os.path.join(dirpath, f))\n\n# Normalize array\ndef normalize(arr, arr_max = None):\n ''' Function to normalize an input array to 0-1 '''\n if not arr_max:\n arr_max = arr.max()\n out = arr / arr_max\n else:\n out = arr / arr_max\n return arr / arr_max\n\n# Reorder Planet scenes to RGB\ndef reorder_to_rgb(image):\n '''reorders planet bands to red, green, blue for imshow'''\n blue = normalize(image[:,:,0])\n green = normalize(image[:,:,1])\n red = normalize(image[:,:,2])\n return np.stack([red, green, blue], axis=-1)\n\n# Reorder Planet scenes to RGB for RASTERIO read images (C,H,W) \ndef rasterio_to_rgb(image):\n '''reorders planet bands to red, green, blue for imshow'''\n blue = image[0,:,:]\n green = image[1,:,:]\n red = image[2,:,:]\n return np.stack([red, green, blue], axis=0)\n\n# Contrast stretching algorithm for multiband images\ndef contrast_stretch_mb(img):\n # Loop over RGB bands\n for b in range(0,3):\n p2, p98 = np.percentile(img[:,:,b], (2, 98))\n img_scaled = exposure.rescale_intensity(img, in_range=(p2, p98))\n img[:,:,b] = img_scaled[:,:,b]\n return img\n\n# Contrast stretching for a chip with percentiles passed to it from larger image\n# Contrast stretching algorithm for multiband images\ndef contrast_stretch_chip(img, percs):\n img_out = img\n # Loop over RGB bands\n for b in range(0,3):\n band_percs = percs[b]\n p2 = band_percs[0]\n p98 = band_percs[1]\n band_max = band_percs[2]\n img_norm = normalize(img, band_max)\n img_scaled = exposure.rescale_intensity(img, in_range=(p2, p98))\n img_scaled = exposure.rescale_intensity(img_scaled, out_range=('uint8'))\n img_out[:,:,b] = img_scaled[:,:,b]\n return img_out\n\ndef setup_labeling(vgg_dir, chip_dir):\n \n \"\"\"Copy the VGG project template JSONs and the via.html file into the\n directory of each planet_chip so labeling can begin\n \"\"\"\n # Check if JSON files and/or via.html exists in chip directory\n chip_files = os.listdir(chip_dir)\n if any (\".json\" in f for f in chip_files):\n print(\"has labeling files\")\n \n # If not, copy the template jsons and via.html into the chip's directory\n else:\n for file in os.listdir(vgg_dir):\n copy2(os.path.join(vgg_dir, file), chip_dir) ",
"_____no_output_____"
],
[
"def planet2chips(tiff_directory, chip_directory, chip_size = 512):\n \n \"\"\" Creates image chips (GeoTiffs and PNGs) of a GeoTiff file in a \n specified directory and saves in new directory location \n \"\"\"\n \n # Get all analytic SR GeoTiff filnames in specified directory\n files = np.array(os.listdir(tiff_directory))\n tiff = pd.Series(files).str.contains('SR.tif')\n file = files[tiff][0]\n\n # Get image name to use for creating directory\n image_name = file.split(\"_\")[0:3]\n image_name = \"%s_%s_%s\" % (image_name[0], image_name[1], image_name[2])\n\n # Image chip destination directory and subdirectories\n image_dir = os.path.join(chip_directory, image_name) \n\n chip_dir = os.path.join(image_dir,'chips')\n png_dir = os.path.join(image_dir, 'pngs')\n\n # Print filenames\n print('filename: ' + file + '\\n' + 'image name: ' + image_name)\n\n # Make directories to store raw and rgb image chips\n pathlib.Path(chip_dir).mkdir(parents=True, exist_ok=True)\n pathlib.Path(png_dir).mkdir(parents=True, exist_ok=True)\n \n # Iterate over image blocks - which are 256x256 - and save new GeoTiffs\n with rasterio.open(os.path.join(tiff_directory, file)) as src:\n \n # Read full src image and calculate percentiles for contrast stretchin\n full_src = src.read()\n print(full_src.shape)\n \n # Create windows of desired size\n rows1 = np.arange(0,full_src.shape[1], chip_size)\n rows2 = np.arange(chip_size,full_src.shape[1], chip_size)\n \n cols1 = np.arange(0,full_src.shape[2], chip_size)\n cols2 = np.arange(chip_size,full_src.shape[2], chip_size)\n \n # arrange into tuples\n rows = list(zip(rows1, rows2))\n cols = list(zip(cols1, cols2))\n \n # Arrange into tuples of windows to read\n windows = [ (a,b) for a in rows for b in cols ] \n \n # Get block dimensions of src\n for window in windows:\n\n r = src.read((1,2,3,4), window=window)\n\n if 0 in r:\n continue\n\n else:\n \n # Get start row and column for file name\n rmin = window[0][0]\n cmin = window[1][0]\n \n # Scale variable. Note bands of Planet imagery go BGR\n b = src.read((3,2,1), window=window)\n # Swap axis from rasterio order (C,H,W) to order expected by skio (H,W,C)\n b = np.moveaxis(b, 0, 2)\n b = contrast_stretch_mb(b)\n png_file = png_dir + '/' + image_name + '_' + str(rmin) + '_' + str(cmin) + '.png'\n skio.imsave(png_file, b) \n\n # Open a new GeoTiff data file in which to save the raw image chip\n with rasterio.open((chip_dir + '/' + image_name + '_' + str(rmin) + '_' + str(cmin) + '.tif'), 'w', driver='GTiff',\n height=r.shape[1], width=r.shape[2], count=4,\n dtype=rasterio.uint16, crs=src.crs, \n transform=src.transform) as new_img:\n\n # Write the raw image to the new GeoTiff\n new_img.write(r)",
"_____no_output_____"
]
],
[
[
"Apply to a test image to check performance",
"_____no_output_____"
]
],
[
[
"# sdir = '/Users/Tyler-SFG/Desktop/Box Sync/SFG Centralized Resources/Projects/Aquaculture/Waitt Aquaculture/aqua-mapping/aqua-mapping-data/aqua-images/planet/planet_order_242451/20180830_154418_0f3c'\n# planet2chips(tiff_directory = sdir, chip_directory = sdir, chip_size = 512) ",
"_____no_output_____"
]
],
[
[
"Now we need a function to copy the VGG project templates and via.html files into each chip directory so that the chips can be labeled.",
"_____no_output_____"
]
],
[
[
"def process_planet_orders(source_dir, target_dir):\n \n \"\"\"Find unique PlanetScope scenes in a directory of Planet order folders\n and process newly added scenes into image chips\"\"\"\n \n # Get list of all planet orders in source directory\n orders = np.array(next(os.walk(source_dir))[1])\n # Add full path to each order directory\n orders = [os.path.join(source_dir, o) for o in orders]\n \n scenes = []\n scene_paths = []\n \n for o in orders:\n # scenes in order\n s_ids = np.array(next(os.walk(o))[1])\n s_ids_paths = [os.path.join(source_dir,o,s) for s in s_ids]\n \n # add to lists\n scenes.append(s_ids)\n scene_paths.append(s_ids_paths)\n \n # Flatten lists\n scenes = list(np.concatenate(scenes))\n print(len(scenes))\n scene_paths = list(np.concatenate(scene_paths))\n \n # Check which scenes already have chip folders\n scenes_exist = np.array(next(os.walk(target_dir))[1])\n \n scenes_to_process = []\n scene_paths_to_process = []\n \n # Remove scenes that already exist from list of scenes to process\n for s, sp in zip(scenes, scene_paths):\n if s not in scenes_exist:\n scenes_to_process.append(s)\n scene_paths_to_process.append(sp) \n\n\n # Apply GeoTiff chipping function to each unprocessed scene\n for sp in scene_paths_to_process:\n print(sp)\n planet2chips(tiff_directory = sp, chip_directory = target_dir, chip_size = 512) ",
"_____no_output_____"
]
],
[
[
"Apply the function to process all Planet orders presently in Box",
"_____no_output_____"
]
],
[
[
"# Run function\nsdir = '/Users/Tyler-SFG/Desktop/Box Sync/SFG Centralized Resources/Projects/Aquaculture/Waitt Aquaculture/aqua-mapping/aqua-mapping-data/aqua-images/planet'\ntdir = '/Users/Tyler-SFG/Desktop/Box Sync/SFG Centralized Resources/Projects/Aquaculture/Waitt Aquaculture/aqua-mapping/aqua-mapping-data/aqua-images/planet_chips'\n\n# os.path.isdir(sdir)\nprocess_planet_orders(sdir, tdir) ",
"141\n/Users/Tyler-SFG/Desktop/Box Sync/SFG Centralized Resources/Projects/Aquaculture/Waitt Aquaculture/aqua-mapping/aqua-mapping-data/aqua-images/planet/planet_order_236949/20180825_231532_1_0f3c\nfilename: 20180825_231532_1_0f3c_3B_AnalyticMS_SR.tif\nimage name: 20180825_231532_1\n(4, 4510, 8605)\n"
]
],
[
[
"### Move tiff files for labeled chips\n\nAfter a Planet scene is processed into tiff and png chips, the pngs containing objects are added to a VGG project and labeled. Labels are then saved in a `[batchname]_labels.json` file. The last step prior to uploading the chips to Tana is to create a new directory for the chip containing the raw tiff file and a directory of class specific masks.",
"_____no_output_____"
]
],
[
[
"# Function to copy the tiffs of PNGs selected for labeling and make directories for each chip\ndef copy_chip_tiffs(label_dir, chips_dir, prepped_dir):\n \n \"\"\" Take a VGG labeling project with PNGs and create a directory\n for each chip in the prepped directory\n \"\"\"\n # Read annotations\n pngs = os.listdir(label_dir)\n pngs = [png for png in pngs if png != '.DS_Store'] # remove stupid DS_Store file\n \n # Extract filenames and drop .png extension\n chips = [c.split('.png')[0] for c in pngs]\n \n # Loop over chips\n for chip in chips:\n \n # Make directory for chip in prepped dir\n chip_dir = os.path.join(prepped_dir, chip)\n # Create \"image\" dir for tiff image\n image_dir = os.path.join(chip_dir, 'image')\n \n # Make chip directory and subdirectories\n for d in [chip_dir, image_dir]:\n pathlib.Path(d).mkdir(parents=True, exist_ok=True)\n \n # Now locate the tiff file and copy into chip directory\n # Get scene name for chip\n scene = chip.split('_')[0:3]\n scene = \"%s_%s_%s\" % (scene[0], scene[1], scene[2])\n \n # Locate and copy tiff file\n tiff = os.path.join(chips_dir, scene, 'chips', (chip + '.tif'))\n copy2(tiff, image_dir)",
"_____no_output_____"
]
],
[
[
"Run function to copy tifs for selected PNGs",
"_____no_output_____"
]
],
[
[
"# Copy tiffs for chile cages\nlabels = '/Users/Tyler-SFG/Desktop/Box Sync/SFG Centralized Resources/Projects/Aquaculture/Waitt Aquaculture/aqua-mapping/aqua-mapping-data/aqua-images/vgg/labeled/label_china/pngs'\nprepped_dir = '/Users/Tyler-SFG/Desktop/Box Sync/SFG Centralized Resources/Projects/Aquaculture/Waitt Aquaculture/aqua-mapping/aqua-mapping-data/aqua-images/prepped_planet'\nchips_dir = '/Users/Tyler-SFG/Desktop/Box Sync/SFG Centralized Resources/Projects/Aquaculture/Waitt Aquaculture/aqua-mapping/aqua-mapping-data/aqua-images/planet_chips'",
"_____no_output_____"
],
[
"copy_chip_tiffs(label_dir = labels, chips_dir = chips_dir, prepped_dir = prepped_dir)",
"_____no_output_____"
]
],
[
[
"Now we need a function to create the class masks for each image",
"_____no_output_____"
]
],
[
[
"def masks_from_labels(labels, prepped_dir):\n \n # Read annotations\n annotations = json.load(open(labels))\n annotations = list(annotations.values()) # don't need the dict keys\n \n # The VIA tool saves images in the JSON even if they don't have any\n # annotations. Skip unannotated images.\n annotations = [a for a in annotations if a['regions']]\n \n # Loop over chips\n for a in annotations:\n \n # Get chip and directory\n chip = a['filename'].split('.png')[0] \n chip_dir = os.path.join(prepped_dir, chip)\n \n # Create a directory to store masks\n masks_dir = os.path.join(chip_dir, 'class_masks')\n pathlib.Path(masks_dir).mkdir(parents=True, exist_ok=True)\n \n # Read geotiff for chip\n gtiff = chip_dir + '/' + 'image' + '/' + chip + '.tif'\n src = rasterio.open(gtiff)\n\n # Use try to only extract masks for chips with complete annotations and class labels\n try:\n\n \"\"\"Code for processing VGG annotations from Matterport balloon color splash sample\"\"\"\n # Load annotations\n # VGG Image Annotator saves each image in the form:\n # { 'filename': '28503151_5b5b7ec140_b.jpg',\n # 'regions': {\n # '0': {\n # 'region_attributes': {},\n # 'shape_attributes': {\n # 'all_points_x': [...],\n # 'all_points_y': [...],\n # 'name': 'polygon'}},\n # ... more regions ...\n # },\n # 'size': 100202\n # } \n\n # Get the aquaculture class of each polygon \n polygon_types = [r['region_attributes'] for r in a['regions']] \n\n # Get unique aquaculture classes in annotations\n types = set(val for dic in polygon_types for val in dic.values()) \n\n for t in types:\n # Get the x, y coordinaets of points of the polygons that make up\n # the outline of each object instance. There are stores in the\n # shape_attributes (see json format above) \n\n # Pull out polygons of that type \n polygons = [r['shape_attributes'] for r in a['regions'] if r['region_attributes']['class'] == t] \n\n # Draw mask using height and width of Geotiff\n mask = np.zeros([src.height, src.width], dtype=np.uint8)\n\n for p in polygons:\n\n # Get indexes of pixels inside the polygon and set them to 1\n rr, cc = skimage.draw.polygon(p['all_points_y'], p['all_points_x']) \n mask[rr, cc] = 1 \n\n # Open a new GeoTiff data file in which to save the image chip\n with rasterio.open((masks_dir + '/' + chip + '_' + str(t) + '_mask.tif'), 'w', driver='GTiff',\n height=src.shape[0], width=src.shape[1], count=1,\n dtype=rasterio.ubyte, crs=src.crs, \n transform=src.transform) as new_img:\n\n # Write the rescaled image to the new GeoTiff\n new_img.write(mask.astype('uint8'),1)\n\n except KeyError: \n print(chip + ' missing aquaculture class assignment')\n # write chip name to file for double checking\n continue\n ",
"_____no_output_____"
]
],
[
[
"Run function to create masks",
"_____no_output_____"
]
],
[
[
"labels = \"/Users/Tyler-SFG/Desktop/Box Sync/SFG Centralized Resources/Projects/Aquaculture/Waitt Aquaculture/aqua-mapping/aqua-mapping-data/aqua-images/vgg/labeled/label_china/20180410_020421_0f31_labels.json\"\nprepped_dir = '/Users/Tyler-SFG/Desktop/Box Sync/SFG Centralized Resources/Projects/Aquaculture/Waitt Aquaculture/aqua-mapping/aqua-mapping-data/aqua-images/prepped_planet/china_20180918'",
"_____no_output_____"
],
[
"masks_from_labels(labels = labels, prepped_dir = prepped_dir)",
"/Users/Tyler-SFG/anaconda/envs/planet/lib/python3.6/site-packages/ipykernel/__main__.py:1: FutureWarning: The value of this property will change in version 1.0. Please see https://github.com/mapbox/rasterio/issues/86 for details.\n if __name__ == '__main__':\n/Users/Tyler-SFG/anaconda/envs/planet/lib/python3.6/site-packages/rasterio/__init__.py:160: FutureWarning: GDAL-style transforms are deprecated and will not be supported in Rasterio 1.0.\n transform = guard_transform(transform)\n"
]
]
] | [
"code",
"markdown",
"code",
"markdown",
"code",
"markdown",
"code",
"markdown",
"code",
"markdown",
"code",
"markdown",
"code",
"markdown",
"code"
] | [
[
"code",
"code",
"code"
],
[
"markdown"
],
[
"code"
],
[
"markdown"
],
[
"code"
],
[
"markdown"
],
[
"code"
],
[
"markdown"
],
[
"code"
],
[
"markdown"
],
[
"code",
"code"
],
[
"markdown"
],
[
"code"
],
[
"markdown"
],
[
"code",
"code"
]
] |
d08dcfde9051b36edb7cc8a7cde5a45e4a0bc217 | 6,171 | ipynb | Jupyter Notebook | Chapters_1-5/Chapter_4/2_Positional_Arguments.ipynb | NaveenKumarReddy8/MyPython | 0a7895ca1bc874f650d7a2eb1bc4c832a642eb57 | [
"MIT"
] | 1 | 2021-06-06T14:45:35.000Z | 2021-06-06T14:45:35.000Z | Chapters_1-5/Chapter_4/2_Positional_Arguments.ipynb | NaveenKumarReddy8/MyPython | 0a7895ca1bc874f650d7a2eb1bc4c832a642eb57 | [
"MIT"
] | 32 | 2021-05-25T15:23:18.000Z | 2022-03-14T23:16:58.000Z | Chapters_1-5/Chapter_4/2_Positional_Arguments.ipynb | NaveenKumarReddy8/PythonWorkshop | 0a7895ca1bc874f650d7a2eb1bc4c832a642eb57 | [
"MIT"
] | null | null | null | 26.830435 | 303 | 0.595041 | [
[
[
"# 4.2 Positional Arguments",
"_____no_output_____"
]
],
[
[
"def add(operand_1, operand_2):\n print(f\"The sum of {operand_1} and {operand_2} is {operand_1 + operand_2}\")",
"_____no_output_____"
]
],
[
[
"Yipeee! we have created a new function called add which is expected to add two integers values, Just kidding 😜, thanks to the dynamic typing of the Python, we can even add float values, concat strings and many more using our `add` function, but for now, let's stick with the addition of integers 😎",
"_____no_output_____"
]
],
[
[
"add(1, 3)",
"_____no_output_____"
]
],
[
[
"Yup, we did got our result ⭐️. what if I forget passing a value? we would see a `TypeError` exception raised 👻",
"_____no_output_____"
]
],
[
[
"add(1)",
"_____no_output_____"
]
],
[
[
"The name **Positional arguments** itself says the arguments should be according to the function signature. But here's a deal, we can change the order of arguments being passed, just that we should pass them with the respective keyword 🙂",
"_____no_output_____"
],
[
"`Example`",
"_____no_output_____"
]
],
[
[
"def difference(a, b):\n print(f\"The difference of {b} from {a} is {a - b}\")",
"_____no_output_____"
],
[
"difference(5, 8)",
"_____no_output_____"
],
[
"difference(b=8, a=5) # Positions are swapped, but passing the objects as keywords.",
"_____no_output_____"
]
],
[
[
"We can see in the above example that even if the positions are changed, but as we have are passing them through keywords, the result remains the same. ⭐️",
"_____no_output_____"
],
[
"## Position only arguments",
"_____no_output_____"
],
[
"We do have the power ✊ to make the user call the function's position only arguments the way we want, Thanks to [PEP-570](https://www.python.org/dev/peps/pep-0570/) for Python >= 3.8",
"_____no_output_____"
],
[
"The syntax defined by the PEP-570 regarding Position only arguments is as:",
"_____no_output_____"
],
[
"\n```Python\ndef name(positional_only_parameters, /, positional_or_keyword_parameters, *, keyword_only_parameters):\n```",
"_____no_output_____"
]
],
[
[
"def greet(greet_word, /, name_of_the_user):\n print(f\"{greet_word} {name_of_the_user}!\")",
"_____no_output_____"
]
],
[
[
"In the above example, we do have two arguments `greet_word` and `name_of_the_user` we used **`/`** to say that **Hey Python! Consider `greet_word` as Positional only Argument**",
"_____no_output_____"
],
[
"When we try to call our function `greet` with greet_word as keyword name, Boom 💣, we get a `TypeError` exception.",
"_____no_output_____"
]
],
[
[
"greet(greet_word=\"Hello\", name_of_the_user=\"Pythonist\")",
"_____no_output_____"
]
],
[
[
"Try to call our `greet` with `greet_word` as positional only argument, meaning not passing it by keyword name. We can hope that there won't be any exception raised. 😁",
"_____no_output_____"
]
],
[
[
"# Calling greet function with name_of_the_user as Positional keyword.\ngreet(\"Hello\", \"Pythonist\")\n\n# Calling greet function with name_of_the_user with keyword name.\ngreet(\"Hello\", name_of_the_user=\"Pythoneer😍\")",
"_____no_output_____"
]
]
] | [
"markdown",
"code",
"markdown",
"code",
"markdown",
"code",
"markdown",
"code",
"markdown",
"code",
"markdown",
"code",
"markdown",
"code"
] | [
[
"markdown"
],
[
"code"
],
[
"markdown"
],
[
"code"
],
[
"markdown"
],
[
"code"
],
[
"markdown",
"markdown"
],
[
"code",
"code",
"code"
],
[
"markdown",
"markdown",
"markdown",
"markdown",
"markdown"
],
[
"code"
],
[
"markdown",
"markdown"
],
[
"code"
],
[
"markdown"
],
[
"code"
]
] |
d08ddcfd4aeef89a86c535aafd7bf15d1861143b | 6,938 | ipynb | Jupyter Notebook | Functions/basic_DEA_data&code/read_data_example.ipynb | PO-LAB/DEA | f17f261e013ad7a0d7ff48affe67174b572e17ba | [
"MIT"
] | 74 | 2018-01-29T06:40:57.000Z | 2022-02-17T20:41:11.000Z | Functions/basic_DEA_data&code/read_data_example.ipynb | apapaioannou92/DEA-1 | 3d73b3333f0bfff0d7fd82d01d56008c127d1b85 | [
"MIT"
] | 2 | 2018-02-09T13:42:33.000Z | 2021-05-20T10:03:41.000Z | Functions/basic_DEA_data&code/read_data_example.ipynb | apapaioannou92/DEA-1 | 3d73b3333f0bfff0d7fd82d01d56008c127d1b85 | [
"MIT"
] | 45 | 2018-01-30T07:32:13.000Z | 2022-01-20T09:49:59.000Z | 25.601476 | 465 | 0.46512 | [
[
[
"# Read data function examples for basic DEA models\n在此示範如何使用csv2dict()、csv2dict_sep()來讀取要被衡量的資料,以利後續放入DEA主程式時使用,並顯示它們最終形成的資料形式。<br>\n\n※示範程式碼及csv資料存放於[這裡](https://github.com/wurmen/DEA/tree/master/Functions/basic_DEA_data%26code),可自行下載測試。\n",
"_____no_output_____"
]
],
[
[
"#導入存放csv2dict()、csv2dict_sep()的py檔(在此名為DEA)\nimport DEA",
"_____no_output_____"
]
],
[
[
"# csv2dict()\n-------\n## Exampl1\n當產出與投入資料放置同一csv檔時,並且**<span style=\"color:red\">不指定</span>**以2~4行當成投入資料,5~8行當成產出資料。",
"_____no_output_____"
]
],
[
[
"DMU,X,Y=DEA.csv2dict(\"data.csv\",in_range=[2,4],out_range=[5,8],assign=False) # return DMU、X、Y",
"_____no_output_____"
],
[
"print(DMU) # DMU list",
"['A', 'B', 'C', 'D', 'E', 'F', 'G', 'I', 'J', 'K', 'L', 'M', 'O']\n"
],
[
"print(X) # input data dict",
"{'A': [392.0, 1.0, 8259.0], 'B': [381.0, 2.0, 9628.0], 'C': [2673.0, 3.0, 70923.0], 'D': [282.0, 4.0, 9683.0], 'E': [1608.0, 5.0, 40630.0], 'F': [2074.0, 6.0, 47420.0], 'G': [75.0, 7.0, 7115.0], 'I': [458.0, 8.0, 10177.0], 'J': [1722.0, 9.0, 29124.0], 'K': [400.0, 10.0, 8987.0], 'L': [1217.0, 11.0, 34680.0], 'M': [2532.0, 12.0, 51536.0], 'O': [1303.0, 13.0, 32683.0]}\n"
],
[
"print(Y) # output data dict",
"{'A': [23756.0, 4.0, 2.0, 870.0], 'B': [24183.0, 5.0, 3.0, 1359.0], 'C': [163483.0, 6.0, 4.0, 12449.0], 'D': [10370.0, 7.0, 5.0, 509.0], 'E': [99047.0, 8.0, 6.0, 3726.0], 'F': [128635.0, 9.0, 7.0, 9214.0], 'G': [11962.0, 10.0, 8.0, 536.0], 'I': [32436.0, 11.0, 9.0, 1462.0], 'J': [83862.0, 12.0, 10.0, 6337.0], 'K': [14618.0, 13.0, 11.0, 785.0], 'L': [99636.0, 14.0, 12.0, 6597.0], 'M': [135480.0, 15.0, 13.0, 10928.0], 'O': [74106.0, 16.0, 14.0, 4258.0]}\n"
]
],
[
[
"## Example2\n當產出與投入資料放置同一csv檔時,並且**<span style=\"color:red\">指定</span>**以2、4行當成投入資料,5、8行當成產出資料。\n",
"_____no_output_____"
]
],
[
[
"DMU,X,Y=DEA.csv2dict(\"data.csv\",in_range=[2,4],out_range=[5,8],assign=True)",
"_____no_output_____"
],
[
"print(DMU)",
"['A', 'B', 'C', 'D', 'E', 'F', 'G', 'I', 'J', 'K', 'L', 'M', 'O']\n"
],
[
"print(X)",
"{'A': [392.0, 8259.0], 'B': [381.0, 9628.0], 'C': [2673.0, 70923.0], 'D': [282.0, 9683.0], 'E': [1608.0, 40630.0], 'F': [2074.0, 47420.0], 'G': [75.0, 7115.0], 'I': [458.0, 10177.0], 'J': [1722.0, 29124.0], 'K': [400.0, 8987.0], 'L': [1217.0, 34680.0], 'M': [2532.0, 51536.0], 'O': [1303.0, 32683.0]}\n"
],
[
"print(Y)",
"{'A': [23756.0, 870.0], 'B': [24183.0, 1359.0], 'C': [163483.0, 12449.0], 'D': [10370.0, 509.0], 'E': [99047.0, 3726.0], 'F': [128635.0, 9214.0], 'G': [11962.0, 536.0], 'I': [32436.0, 1462.0], 'J': [83862.0, 6337.0], 'K': [14618.0, 785.0], 'L': [99636.0, 6597.0], 'M': [135480.0, 10928.0], 'O': [74106.0, 4258.0]}\n"
]
],
[
[
"# csv2dict_sep()\n---------------------\n## Example1\n當產出與投入分別放在不同檔案時,且**<span style=\"color:red\">不指定</span>**投入或產出資料。",
"_____no_output_____"
]
],
[
[
"DMU, X = DEA.csv2dict_sep(\"data_input.csv\")",
"_____no_output_____"
],
[
"print(DMU)",
"['A', 'B', 'C', 'D', 'E', 'F', 'G', 'I', 'J', 'K', 'L', 'M', 'O']\n"
],
[
"print(X)",
"{'A': [392.0, 8259.0], 'B': [381.0, 9628.0], 'C': [2673.0, 70923.0], 'D': [282.0, 9683.0], 'E': [1608.0, 40630.0], 'F': [2074.0, 47420.0], 'G': [75.0, 7115.0], 'I': [458.0, 10177.0], 'J': [1722.0, 29124.0], 'K': [400.0, 8987.0], 'L': [1217.0, 34680.0], 'M': [2532.0, 51536.0], 'O': [1303.0, 32683.0]}\n"
]
],
[
[
"## Example2\n當產出與投入分別放在不同檔案時,且**<span style=\"color:red\">指定</span>**投入或產出資料。",
"_____no_output_____"
]
],
[
[
"DMU, Y = DEA.csv2dict_sep(\"data_output.csv\", vrange =[2,4], assign=True)",
"_____no_output_____"
],
[
"print(X)",
"{'A': [392.0, 8259.0], 'B': [381.0, 9628.0], 'C': [2673.0, 70923.0], 'D': [282.0, 9683.0], 'E': [1608.0, 40630.0], 'F': [2074.0, 47420.0], 'G': [75.0, 7115.0], 'I': [458.0, 10177.0], 'J': [1722.0, 29124.0], 'K': [400.0, 8987.0], 'L': [1217.0, 34680.0], 'M': [2532.0, 51536.0], 'O': [1303.0, 32683.0]}\n"
]
]
] | [
"markdown",
"code",
"markdown",
"code",
"markdown",
"code",
"markdown",
"code",
"markdown",
"code"
] | [
[
"markdown"
],
[
"code"
],
[
"markdown"
],
[
"code",
"code",
"code",
"code"
],
[
"markdown"
],
[
"code",
"code",
"code",
"code"
],
[
"markdown"
],
[
"code",
"code",
"code"
],
[
"markdown"
],
[
"code",
"code"
]
] |
d08df162ab3cf3d3da1eb94449e4e72ed9be7864 | 207,183 | ipynb | Jupyter Notebook | Valencia/Equipo3-IAmobilitat/Grupo3SaturdaysAI_IAmobilitat.ipynb | tozanni/Projects | 7419fac1eb7841a50d6be7ebebcd26dc33d37ff5 | [
"MIT"
] | 1 | 2020-05-29T00:30:08.000Z | 2020-05-29T00:30:08.000Z | Valencia/Equipo3-IAmobilitat/Grupo3SaturdaysAI_IAmobilitat.ipynb | tozanni/Projects | 7419fac1eb7841a50d6be7ebebcd26dc33d37ff5 | [
"MIT"
] | null | null | null | Valencia/Equipo3-IAmobilitat/Grupo3SaturdaysAI_IAmobilitat.ipynb | tozanni/Projects | 7419fac1eb7841a50d6be7ebebcd26dc33d37ff5 | [
"MIT"
] | null | null | null | 107.237578 | 32,078 | 0.786213 | [
[
[
"# Import packages & Connect the database",
"_____no_output_____"
]
],
[
[
"# Install MYSQL client\npip install PyMySQL",
"Requirement already satisfied: PyMySQL in /usr/local/lib/python3.6/dist-packages (0.9.3)\n"
],
[
"import sklearn\n\nprint('The scikit-learn version is {}.'.format(sklearn.__version__))",
"The scikit-learn version is 0.22.1.\n"
],
[
"%load_ext autoreload\n%autoreload 2\n\n%matplotlib inline",
"The autoreload extension is already loaded. To reload it, use:\n %reload_ext autoreload\n"
],
[
"import numpy as np\nimport pandas as pd\nimport datetime as dt",
"_____no_output_____"
],
[
"# Connect to database\nimport pymysql\n\nconn = pymysql.connect(\n host='34.69.136.137',\n port=int(3306),\n user='root',\n passwd='rtfgvb77884',\n db='valenbisi',\n charset='utf8mb4')",
"_____no_output_____"
]
],
[
[
"# Prepare data",
"_____no_output_____"
]
],
[
[
"# Get Stations\ndf_station_snapshot = pd.read_sql_query(\"SELECT station_number, station_service_available, creation_date FROM station_snapshot WHERE station_number=31\",\n conn)",
"_____no_output_____"
],
[
"def substractTime(x):\n date = dt.datetime(x.year, x.month, x.day, x.hour)\n return (date - dt.timedelta(hours=1))\n\ndef addTime(x):\n date = dt.datetime(x.year, x.month, x.day, x.hour)\n return (date + dt.timedelta(hours=1))\n\ndef getPrevAvailable(d_f, row):\n new_dateTime = substractTime(row['datetime'])\n try:\n return d_f[(d_f['id'] == row['id']) & (d_f['year'] == new_dateTime.year) & (d_f['month'] == new_dateTime.month) & (d_f['day'] == new_dateTime.day) & (d_f['hour'] == new_dateTime.hour)].iloc[0, d_f.columns.get_loc('available')] \n except:\n return 0\n\ndef getNextAvailable(d_f, row):\n new_dateTime = addTime(row['datetime'])\n try:\n return d_f[(d_f['id'] == row['id']) & (d_f['year'] == new_dateTime.year) & (d_f['month'] == new_dateTime.month) & (d_f['day'] == new_dateTime.day) & (d_f['hour'] == new_dateTime.hour)].iloc[0, d_f.columns.get_loc('available')] \n except:\n return 0",
"_____no_output_____"
],
[
"# Update titles\ndf_stations = df_station_snapshot.rename(index=str, columns={\"station_number\": \"id\", \"station_service_available\": \"available\", \"creation_date\": \"datetime\"})\n\ndf_stations['id'] = df_stations['id'].astype(str).astype(int);\n\n# Transform date strinf to date without seconds\ndf_stations['datetime'] = pd.to_datetime(df_stations['datetime'], infer_datetime_format=True)\ndf_stations['datetime'] = df_stations['datetime'].dt.floor('H')\n\n# # Sort by datetime\ndf_stations.sort_values(by=['datetime'], inplace=True, ascending=True)\n\n# # Separate datetime in columns\ndf_stations['date'] = df_stations['datetime'].dt.date\ndf_stations['hour'] = df_stations['datetime'].dt.hour\ndf_stations['year'] = df_stations['datetime'].dt.year\ndf_stations['month'] = df_stations['datetime'].dt.month\ndf_stations['day'] = df_stations['datetime'].dt.day\ndf_stations['dayofweek'] = df_stations['datetime'].dt.dayofweek\n\n# Group and avg by time\ndf_stations['available'] = df_stations.groupby(['id', 'date', 'hour'])['available'].transform('mean').astype(int)\ndf_stations.drop_duplicates(subset=['id', 'date', 'hour'], keep='first', inplace=True)\n\n# # Set multiple avaiables\ndf_stations['available_prev'] = df_stations.apply(lambda x: getPrevAvailable(df_stations, x), axis=1)\ndf_stations['available_next'] = df_stations.apply(lambda x: getNextAvailable(df_stations, x), axis=1)\n\n# # Clean columns\ndf_stations.drop(['datetime', 'day'], axis=1, inplace=True)",
"_____no_output_____"
],
[
"df_stations.tail()",
"_____no_output_____"
],
[
"# Get Holidays\ndf_holiday_snapshot = pd.read_sql_query(\"SELECT date, enabled FROM holiday\",\n conn)",
"_____no_output_____"
],
[
"# Update titles\ndf_holiday = df_holiday_snapshot.rename(index=str, columns={\"enabled\": \"holiday\"})\n\n# Sort by datetime\ndf_holiday.sort_values(by=['date'], inplace=True, ascending=True)",
"_____no_output_____"
],
[
"# Get Sport Events\ndf_event_snapshot = pd.read_sql_query(\"SELECT date, football, basketball FROM sport_event\",\n conn)",
"_____no_output_____"
],
[
"# Clone data frame\ndf_event = df_event_snapshot\n\n# Sort by datetime\ndf_event.sort_values(by=['date'], inplace=True, ascending=True)",
"_____no_output_____"
],
[
"# Get Weather\ndf_weather_snapshot = pd.read_sql_query(\"SELECT temperature, humidity, wind_speed, cloud_percentage, creation_date FROM weather\",\n conn)",
"_____no_output_____"
],
[
"# Update titles\ndf_weather = df_weather_snapshot.rename(index=str, columns={\"wind_speed\": \"wind\", \"cloud_percentage\": \"cloud\", \"creation_date\": \"datetime\"})\n\n# Transform date strinf to date without seconds\ndf_weather['datetime'] = pd.to_datetime(df_weather['datetime'], infer_datetime_format=True)\ndf_weather['datetime'] = df_weather['datetime'].dt.floor('H')\n\n# Separate datetime in two columns\ndf_weather['date'] = df_weather['datetime'].dt.date\ndf_weather['hour'] = df_weather['datetime'].dt.hour\n\n# Group by datetime and get mean of the data\ndf_weather['temperature'] = df_weather.groupby(['hour', 'date'])['temperature'].transform('mean')\ndf_weather['humidity'] = df_weather.groupby(['hour', 'date'])['humidity'].transform('mean')\ndf_weather['wind'] = df_weather.groupby(['hour', 'date'])['wind'].transform('mean')\ndf_weather['cloud'] = df_weather.groupby(['hour', 'date'])['cloud'].transform('mean')\n\n# Clean duplicated rows\ndf_weather.drop_duplicates(subset=['date', 'hour'], keep='first', inplace=True)\n\n# Clean columns\ndf_weather.drop(['datetime'], axis=1, inplace=True)",
"_____no_output_____"
],
[
"# Merge stations with holidays\ndf = pd.merge(\n df_stations,\n df_holiday,\n how='left',\n left_on=['date'],\n right_on=['date']\n)\n\n# Replace NaN with 0\ndf['holiday'] = df['holiday'].fillna(0)\n\n# Merge (stations with holidays) with sport events\ndf = pd.merge(\n df,\n df_event,\n how='left',\n left_on=['date'],\n right_on=['date']\n)\n\n# Replace NaN with 0\ndf['football'] = df['football'].fillna(0)\ndf['basketball'] = df['basketball'].fillna(0)\n\n# Merge ((stations with holidays) with sport events) with weather\ndf = pd.merge(\n df,\n df_weather,\n how='left',\n left_on=['date', 'hour'],\n right_on=['date', 'hour']\n)\n\n# Replace NaN with 0\ndf['temperature'] = df['temperature'].fillna(0)\ndf['humidity'] = df['humidity'].fillna(0)\ndf['wind'] = df['wind'].fillna(0)\ndf['cloud'] = df['cloud'].fillna(0)",
"_____no_output_____"
],
[
"# Show latest data\nprint('DATA AGGREGATED FOR STATION: ' + station)\ndf.tail(10)",
"DATA AGGREGATED FOR STATION: 31\n"
]
],
[
[
"# Visualize the data",
"_____no_output_____"
]
],
[
[
"# Load libraries\nimport matplotlib as mpl\nimport matplotlib.pyplot as plt\nfrom matplotlib.legend_handler import HandlerLine2D\nimport seaborn as sns;",
"_____no_output_____"
],
[
"# HEATMAP CHART PER MIN (10)\n\nheatmap_data = pd.pivot_table(df[df['id']==31], values='available', index='hour', columns='date')\nfig, ax = plt.subplots(figsize=(20,5)) \nsns.heatmap(heatmap_data, cmap='RdBu', ax=ax)",
"_____no_output_____"
],
[
"# HEATMAP CHART PER WEEK DAY\n\nheatmap_data_week_day = pd.pivot_table(df[df['id']==31], values='available', index='hour', columns='dayofweek')\nfig, ax = plt.subplots(figsize=(20,5)) \nsns.heatmap(heatmap_data_week_day, cmap='RdBu', ax=ax)",
"_____no_output_____"
]
],
[
[
"# Start prediction",
"_____no_output_____"
]
],
[
[
"# Load libraries\nimport math\nfrom sklearn.ensemble import RandomForestRegressor, AdaBoostRegressor, GradientBoostingRegressor\nfrom sklearn.linear_model import LinearRegression, Lasso, LassoLars, Ridge\nfrom sklearn.tree import DecisionTreeRegressor\nfrom scipy.stats import randint as sp_randint\nfrom sklearn.model_selection import train_test_split, GridSearchCV\nfrom sklearn import metrics\nfrom sklearn.metrics import explained_variance_score\nfrom sklearn.feature_selection import SelectKBest, chi2\nfrom sklearn.pipeline import Pipeline\n\nfrom sklearn.impute import SimpleImputer",
"_____no_output_____"
],
[
"# Evaluate model\ndef evaluate(model, train_features, train_labels, test_features, test_labels):\n print('MODEL PERFORMANCE')\n train_pred = model.predict(train_features)\n print('Train set')\n print('| Mean Absolute Error:', metrics.mean_absolute_error(train_labels, train_pred))\n print('| Mean Square Error:', metrics.mean_squared_error(train_labels, train_pred))\n print('| Root Mean Square Error:', np.sqrt(metrics.mean_squared_error(train_labels, train_pred)))\n print('| Train Score:', model.score(train_features, train_labels))\n y_pred = model.predict(test_features)\n print('Test set')\n print('| Mean Absolute Error:', metrics.mean_absolute_error(test_labels, y_pred))\n print('| Mean Square Error:', metrics.mean_squared_error(test_labels, y_pred))\n print('| Root Mean Square Error:', np.sqrt(metrics.mean_squared_error(test_labels, y_pred)))\n print('| Test Score:', model.score(test_features, test_labels))\n print('| Explained Variance:', explained_variance_score(test_labels, y_pred))\n\n if hasattr(model, 'oob_score_'): print('OOB Score:', model.oob_score_)",
"_____no_output_____"
]
],
[
[
"## Find best algoritm for our data",
"_____no_output_____"
]
],
[
[
"def quick_eval(pipeline, X_train, y_train, X_test, y_test, verbose=True):\n \"\"\"\n Quickly trains modeling pipeline and evaluates on test data. Returns original model, training RMSE, and testing\n RMSE as a tuple.\n \"\"\"\n \n pipeline.fit(X_train, y_train)\n y_train_pred = pipeline.predict(X_train)\n y_test_pred = pipeline.predict(X_test)\n \n train_score = np.sqrt(metrics.mean_squared_error(y_train, y_train_pred))\n test_score = np.sqrt(metrics.mean_squared_error(y_test, y_test_pred))\n \n if verbose:\n print(f\"Regression algorithm: {pipeline.named_steps['regressor'].__class__.__name__}\")\n print(f\"Train RMSE: {train_score}\")\n print(f\"Test RMSE: {test_score}\")\n print(f\"----------------------------\")\n \n return pipeline.named_steps['regressor'], train_score, test_score",
"_____no_output_____"
]
],
[
[
"After review the result we see that **RandomForestRegressor** is the best option to predict our data",
"_____no_output_____"
],
[
"## Random Forest",
"_____no_output_____"
]
],
[
[
"# Create a new dataframe for random forest\ndf_rf = df[['id', 'year', 'month', 'dayofweek', 'hour', 'holiday', 'football', 'basketball', 'temperature', 'humidity', 'wind', 'cloud', 'available_prev', 'available', 'available_next']]\n\n# Prepare data for train and test\n# We want to predict (\"available_next\")\nX = df_rf.drop('available_next', axis=1)\ny = df_rf['available_next']",
"_____no_output_____"
],
[
"# Split data in train and test\nX_train, X_test, y_train, y_test = train_test_split(X, y, test_size=0.25)\n\nX_train.shape, y_train.shape, X_test.shape, y_test.shape",
"_____no_output_____"
],
[
"# Create our imputer to replace missing values with the mean e.g.\nimp = SimpleImputer(missing_values=np.nan, strategy='mean')\nimp = imp.fit(X_train)\n\n# Impute our data, then train\nX_train = imp.transform(X_train)",
"_____no_output_____"
],
[
"regressors = [\n LinearRegression(),\n Lasso(alpha=.5),\n Ridge(alpha=.1),\n LassoLars(alpha=.1),\n DecisionTreeRegressor(),\n RandomForestRegressor(),\n AdaBoostRegressor(),\n GradientBoostingRegressor()\n]\n\nfor r in regressors:\n pipe = Pipeline(steps = [\n ('regressor', r)\n ])\n\n quick_eval(pipe, X_train, y_train, X_test, y_test)",
"Regression algorithm: LinearRegression\nTrain RMSE: 2.121484327745336\nTest RMSE: 2.065697137562799\n----------------------------\nRegression algorithm: Lasso\nTrain RMSE: 2.179074981399763\nTest RMSE: 2.106610689982148\n----------------------------\nRegression algorithm: Ridge\nTrain RMSE: 2.1215248913825184\nTest RMSE: 2.0663307505301884\n----------------------------\nRegression algorithm: LassoLars\nTrain RMSE: 4.050793742718888\nTest RMSE: 4.128790234244102\n----------------------------\nRegression algorithm: DecisionTreeRegressor\nTrain RMSE: 0.0\nTest RMSE: 2.7594483608258895\n----------------------------\nRegression algorithm: RandomForestRegressor\nTrain RMSE: 0.7917822755765574\nTest RMSE: 2.1275180102873477\n----------------------------\nRegression algorithm: AdaBoostRegressor\nTrain RMSE: 2.2039223581670533\nTest RMSE: 2.3418349911346517\n----------------------------\nRegression algorithm: GradientBoostingRegressor\nTrain RMSE: 1.6317788438198744\nTest RMSE: 2.026063179178271\n----------------------------\n"
]
],
[
[
"### Find best params for Random Forest",
"_____no_output_____"
],
[
"#### Check each property ",
"_____no_output_____"
]
],
[
[
"# Find N_ESTIMATORS\nn_estimators = [int(x) for x in np.linspace(start = 1, stop = 200, num=50)]\ntrain_results = []\ntest_results = []\nfor estimator in n_estimators:\n rf = RandomForestRegressor(n_estimators=estimator, n_jobs=-1)\n rf.fit(X_train, y_train)\n train_pred = rf.predict(X_train)\n train_results.append(np.sqrt(metrics.mean_squared_error(y_train, train_pred)))\n #train_results.append(rf.score(X_train, y_train))\n y_pred = rf.predict(X_test)\n test_results.append(np.sqrt(metrics.mean_squared_error(y_test, y_pred)))\n #test_results.append(rf.score(X_test, y_test))\n\nline1, = plt.plot(n_estimators, train_results, 'b', label='Train RSME')\nline2, = plt.plot(n_estimators, test_results, 'r', label='Test RSME')\nplt.legend(handler_map={line1: HandlerLine2D(numpoints=2)})\nplt.ylabel('RSME')\nplt.xlabel('n_estimators')\nplt.show()",
"_____no_output_____"
],
[
"# Find MAX_DEPTH\nmax_depths = np.linspace(start = 1, stop = 100, num=50, endpoint=True)\ntrain_results = []\ntest_results = []\nfor max_depth in max_depths:\n rf = RandomForestRegressor(max_depth=max_depth, n_jobs=-1)\n rf.fit(X_train, y_train)\n train_pred = rf.predict(X_train)\n train_results.append(np.sqrt(metrics.mean_squared_error(y_train, train_pred)))\n #train_results.append(rf.score(X_train, y_train))\n y_pred = rf.predict(X_test)\n test_results.append(np.sqrt(metrics.mean_squared_error(y_test, y_pred)))\n #test_results.append(rf.score(X_test, y_test))\n\nline1, = plt.plot(max_depths, train_results, 'b', label='Train RSME')\nline2, = plt.plot(max_depths, test_results, 'r', label='Test RSME')\nplt.legend(handler_map={line1: HandlerLine2D(numpoints=2)})\nplt.ylabel('RSME')\nplt.xlabel('Tree depth')\nplt.show()",
"_____no_output_____"
],
[
"# Find MIN_SAMPLES_SPLIT\nmin_samples_splits = np.linspace(start = 0.01, stop = 1.0, num=10, endpoint=True)\ntrain_results = []\ntest_results = []\nfor min_samples_split in min_samples_splits:\n rf = RandomForestRegressor(min_samples_split=min_samples_split)\n rf.fit(X_train, y_train)\n train_pred = rf.predict(X_train)\n train_results.append(np.sqrt(metrics.mean_squared_error(y_train, train_pred)))\n #train_results.append(rf.score(X_train, y_train))\n y_pred = rf.predict(X_test)\n test_results.append(np.sqrt(metrics.mean_squared_error(y_test, y_pred)))\n #test_results.append(rf.score(X_test, y_test))\n\nline1, = plt.plot(min_samples_splits, train_results, 'b', label='Train RSME')\nline2, = plt.plot(min_samples_splits, test_results, 'r', label='Test RSME')\nplt.legend(handler_map={line1: HandlerLine2D(numpoints=2)})\nplt.ylabel('RSME')\nplt.xlabel('min samples split')\nplt.show()",
"_____no_output_____"
],
[
"# Find MIN_SAMPLES_LEAF\nmin_samples_leafs = np.linspace(start = 0.01, stop = 0.5, num=5, endpoint=True)\ntrain_results = []\ntest_results = []\nfor min_samples_leaf in min_samples_leafs:\n rf = RandomForestRegressor(min_samples_leaf=min_samples_leaf)\n rf.fit(X_train, y_train)\n train_pred = rf.predict(X_train)\n train_results.append(np.sqrt(metrics.mean_squared_error(y_train, train_pred)))\n #train_results.append(rf.score(X_train, y_train))\n y_pred = rf.predict(X_test)\n test_results.append(np.sqrt(metrics.mean_squared_error(y_test, y_pred)))\n #test_results.append(rf.score(X_test, y_test))\n\nline1, = plt.plot(min_samples_leafs, train_results, 'b', label='Train RSME')\nline2, = plt.plot(min_samples_leafs, test_results, 'r', label='Test RSME')\nplt.legend(handler_map={line1: HandlerLine2D(numpoints=2)})\nplt.ylabel('RSME')\nplt.xlabel('min samples leaf')\nplt.show()",
"_____no_output_____"
],
[
"# Find MAX_FEATURES\nmax_features = list(range(1,X.shape[1]))\ntrain_results = []\ntest_results = []\nfor max_feature in max_features:\n rf = RandomForestRegressor(max_features=max_feature)\n rf.fit(X_train, y_train)\n train_pred = rf.predict(X_train)\n train_results.append(np.sqrt(metrics.mean_squared_error(y_train, train_pred)))\n #train_results.append(rf.score(X_train, y_train))\n y_pred = rf.predict(X_test)\n test_results.append(np.sqrt(metrics.mean_squared_error(y_test, y_pred)))\n #test_results.append(rf.score(X_test, y_test))\n\nline1, = plt.plot(max_features, train_results, 'b', label='Train RSME')\nline2, = plt.plot(max_features, test_results, 'r', label='Test RSME')\nplt.legend(handler_map={line1: HandlerLine2D(numpoints=2)})\nplt.ylabel('RSME')\nplt.xlabel('max features')\nplt.show()",
"_____no_output_____"
]
],
[
[
"#### Find the best combination of params\n\n**TRY ALL PARAMS TO FIND THE BEST PARAMS FOR OUR DATA**\n\nNow that we know where to concentrate our search, we can explicitly specify every combination of settings to try.",
"_____no_output_____"
]
],
[
[
"#@title Default title text\ndef searchBestParamsForRF(params, train_features, train_labels):\n # First create the base model to tune\n rf = RandomForestRegressor()\n # Instantiate the grid search model\n grid_search = GridSearchCV(estimator = rf, param_grid = param_grid, scoring = 'neg_mean_squared_error', cv = 5, n_jobs = -1, verbose = 2)\n # Fit the grid search to the data\n grid_search.fit(train_features, train_labels)\n print(f\"The best estimator had RMSE {np.sqrt(-grid_search.best_score_)} and the following parameters:\")\n print(grid_search.best_params_)\n\n# Create the parameter grid\nmax_depth = [int(x) for x in np.linspace(10, 20, num = 3)]\nmax_depth.append(None)\nparam_grid = {\n 'bootstrap': [False, True],\n 'n_estimators': [int(x) for x in np.linspace(start = 40, stop = 60, num = 4)],\n 'max_depth': max_depth,\n 'min_samples_split': [float(x) for x in np.linspace(0.1, 0.2, num = 2)],\n 'min_samples_leaf': [float(x) for x in np.linspace(0.1, 0.2, num = 2)],\n 'max_features': [X.shape[1]]\n}\n\n# Comment or Uncomment this line to seach for the best params\nsearchBestParamsForRF(param_grid, X_train, y_train)",
"Fitting 5 folds for each of 128 candidates, totalling 640 fits\n"
]
],
[
[
"### Train and evaluate model",
"_____no_output_____"
]
],
[
[
"m = RandomForestRegressor(n_estimators=60, max_features=X.shape[1])\nm.fit(X_train, y_train)\n\nevaluate(m, X_train, y_train, X_test, y_test)\n\n\n\n# MODEL PERFORMANCE\n# Train set\n# | Mean Absolute Error: 0.5758625862586259\n# | Mean Square Error: 0.6365449044904491\n# | Root Mean Square Error: 0.7978376429389936\n# | Train Score: 0.9807615052050999\n# Test set\n# | Mean Absolute Error: 1.5209793351302785\n# | Mean Square Error: 4.284529050613956\n# | Root Mean Square Error: 2.0699103967597137\n# | Test Score: 0.8757254225805797\n# | Explained Variance: 0.8758109846903823",
"MODEL PERFORMANCE\nTrain set\n| Mean Absolute Error: 0.5777677767776779\n| Mean Square Error: 0.6461221122112212\n| Root Mean Square Error: 0.8038172131842047\n| Train Score: 0.9804720502749198\nTest set\n| Mean Absolute Error: 1.5185534591194971\n| Mean Square Error: 4.309985774183887\n| Root Mean Square Error: 2.076050523032589\n| Test Score: 0.8749870395455335\n| Explained Variance: 0.8750061249382027\n"
],
[
"X_test.tail()",
"_____no_output_____"
],
[
"y_test.tail()",
"_____no_output_____"
],
[
"m.predict([[2020, 1, 6, 10, 0, 0, 0, 11.57, 70.50, 0.93, 0, 0, 1]])",
"_____no_output_____"
],
[
"# Show the importance of each variable in prediction\ndef rf_feat_importance(m, df):\n return pd.DataFrame({'cols':df.columns, 'imp':m.feature_importances_}).sort_values('imp', ascending=False)\n \nfi = rf_feat_importance(m, X); \nfi[:].plot('cols', 'imp', 'barh', figsize=(12,7), legend=False)",
"_____no_output_____"
]
],
[
[
"# Download model",
"_____no_output_____"
]
],
[
[
"# Import package\nimport pickle",
"_____no_output_____"
],
[
"# Generate file\nwith open('model.pkl', 'wb') as model_file:\n pickle.dump(m, model_file)",
"_____no_output_____"
]
]
] | [
"markdown",
"code",
"markdown",
"code",
"markdown",
"code",
"markdown",
"code",
"markdown",
"code",
"markdown",
"code",
"markdown",
"code",
"markdown",
"code",
"markdown",
"code",
"markdown",
"code"
] | [
[
"markdown"
],
[
"code",
"code",
"code",
"code",
"code"
],
[
"markdown"
],
[
"code",
"code",
"code",
"code",
"code",
"code",
"code",
"code",
"code",
"code",
"code",
"code"
],
[
"markdown"
],
[
"code",
"code",
"code"
],
[
"markdown"
],
[
"code",
"code"
],
[
"markdown"
],
[
"code"
],
[
"markdown",
"markdown"
],
[
"code",
"code",
"code",
"code"
],
[
"markdown",
"markdown"
],
[
"code",
"code",
"code",
"code",
"code"
],
[
"markdown"
],
[
"code"
],
[
"markdown"
],
[
"code",
"code",
"code",
"code",
"code"
],
[
"markdown"
],
[
"code",
"code"
]
] |
d08e05938a6729a134f0a49ac1cfee0788423785 | 541,561 | ipynb | Jupyter Notebook | notebooks/machine-learning/RECOMMENDED_Principal_Component_Analysis.ipynb | dlmacedo/machine-learning-class | 66159aeb8deaeb0f35a9aee379a343bbafba92e6 | [
"Apache-2.0"
] | null | null | null | notebooks/machine-learning/RECOMMENDED_Principal_Component_Analysis.ipynb | dlmacedo/machine-learning-class | 66159aeb8deaeb0f35a9aee379a343bbafba92e6 | [
"Apache-2.0"
] | null | null | null | notebooks/machine-learning/RECOMMENDED_Principal_Component_Analysis.ipynb | dlmacedo/machine-learning-class | 66159aeb8deaeb0f35a9aee379a343bbafba92e6 | [
"Apache-2.0"
] | null | null | null | 412.460777 | 153,734 | 0.91858 | [
[
[
"<a href=\"https://colab.research.google.com/github/dlmacedo/ml-dl-notebooks/blob/master/notebooks/machine-learning/RECOMMENDED_Principal_Component_Analysis.ipynb\" target=\"_parent\"><img src=\"https://colab.research.google.com/assets/colab-badge.svg\" alt=\"Open In Colab\"/></a>",
"_____no_output_____"
],
[
"# In Depth: Principal Component Analysis",
"_____no_output_____"
],
[
"In this section, we explore what is perhaps one of the most broadly used of unsupervised algorithms, principal component analysis (PCA).\nPCA is fundamentally a dimensionality reduction algorithm, but it can also be useful as a tool for visualization, for noise filtering, for feature extraction and engineering, and much more.\nAfter a brief conceptual discussion of the PCA algorithm, we will see a couple examples of these further applications.\n\nWe begin with the standard imports:",
"_____no_output_____"
]
],
[
[
"%matplotlib inline\nimport numpy as np\nimport matplotlib.pyplot as plt\nimport seaborn as sns; sns.set()",
"_____no_output_____"
]
],
[
[
"## Introducing Principal Component Analysis\n\nPrincipal component analysis is a fast and flexible unsupervised method for dimensionality reduction in data.\nIts behavior is easiest to visualize by looking at a two-dimensional dataset.\nConsider the following 200 points:",
"_____no_output_____"
]
],
[
[
"rng = np.random.RandomState(1)\nX = np.dot(rng.rand(2, 2), rng.randn(2, 200)).T\nplt.scatter(X[:, 0], X[:, 1])\nplt.axis('equal');",
"_____no_output_____"
]
],
[
[
"By eye, it is clear that there is a nearly linear relationship between the x and y variables.\nThis is reminiscent of the linear regression data, but the problem setting here is slightly different: rather than attempting to *predict* the y values from the x values, the unsupervised learning problem attempts to learn about the *relationship* between the x and y values.\n\nIn principal component analysis, this relationship is quantified by finding a list of the *principal axes* in the data, and using those axes to describe the dataset.\nUsing Scikit-Learn's ``PCA`` estimator, we can compute this as follows:",
"_____no_output_____"
]
],
[
[
"from sklearn.decomposition import PCA\npca = PCA(n_components=2)\npca.fit(X)",
"_____no_output_____"
]
],
[
[
"The fit learns some quantities from the data, most importantly the \"components\" and \"explained variance\":",
"_____no_output_____"
]
],
[
[
"print(pca.components_)",
"[[-0.94446029 -0.32862557]\n [-0.32862557 0.94446029]]\n"
],
[
"print(pca.explained_variance_)",
"[0.7625315 0.0184779]\n"
]
],
[
[
"To see what these numbers mean, let's visualize them as vectors over the input data, using the \"components\" to define the direction of the vector, and the \"explained variance\" to define the squared-length of the vector:",
"_____no_output_____"
]
],
[
[
"def draw_vector(v0, v1, ax=None):\n ax = ax or plt.gca()\n arrowprops=dict(arrowstyle='->',\n linewidth=2,\n shrinkA=0, shrinkB=0)\n ax.annotate('', v1, v0, arrowprops=arrowprops)\n\n# plot data\nplt.scatter(X[:, 0], X[:, 1], alpha=0.2)\nfor length, vector in zip(pca.explained_variance_, pca.components_):\n v = vector * 3 * np.sqrt(length)\n draw_vector(pca.mean_, pca.mean_ + v)\nplt.axis('equal');",
"_____no_output_____"
]
],
[
[
"These vectors represent the *principal axes* of the data, and the length of the vector is an indication of how \"important\" that axis is in describing the distribution of the data—more precisely, it is a measure of the variance of the data when projected onto that axis.\nThe projection of each data point onto the principal axes are the \"principal components\" of the data.\n\nIf we plot these principal components beside the original data, we see the plots shown here:",
"_____no_output_____"
],
[
"",
"_____no_output_____"
],
[
"This transformation from data axes to principal axes is an *affine transformation*, which basically means it is composed of a translation, rotation, and uniform scaling.\n\nWhile this algorithm to find principal components may seem like just a mathematical curiosity, it turns out to have very far-reaching applications in the world of machine learning and data exploration.",
"_____no_output_____"
],
[
"### PCA as dimensionality reduction\n\nUsing PCA for dimensionality reduction involves zeroing out one or more of the smallest principal components, resulting in a lower-dimensional projection of the data that preserves the maximal data variance.\n\nHere is an example of using PCA as a dimensionality reduction transform:",
"_____no_output_____"
]
],
[
[
"pca = PCA(n_components=1)\npca.fit(X)\nX_pca = pca.transform(X)\nprint(\"original shape: \", X.shape)\nprint(\"transformed shape:\", X_pca.shape)",
"original shape: (200, 2)\ntransformed shape: (200, 1)\n"
]
],
[
[
"The transformed data has been reduced to a single dimension.\nTo understand the effect of this dimensionality reduction, we can perform the inverse transform of this reduced data and plot it along with the original data:",
"_____no_output_____"
]
],
[
[
"X_new = pca.inverse_transform(X_pca)\nplt.scatter(X[:, 0], X[:, 1], alpha=0.2)\nplt.scatter(X_new[:, 0], X_new[:, 1], alpha=0.8)\nplt.axis('equal');",
"_____no_output_____"
]
],
[
[
"The light points are the original data, while the dark points are the projected version.\nThis makes clear what a PCA dimensionality reduction means: the information along the least important principal axis or axes is removed, leaving only the component(s) of the data with the highest variance.\nThe fraction of variance that is cut out (proportional to the spread of points about the line formed in this figure) is roughly a measure of how much \"information\" is discarded in this reduction of dimensionality.\n\nThis reduced-dimension dataset is in some senses \"good enough\" to encode the most important relationships between the points: despite reducing the dimension of the data by 50%, the overall relationship between the data points are mostly preserved.",
"_____no_output_____"
],
[
"### PCA for visualization: Hand-written digits\n\nThe usefulness of the dimensionality reduction may not be entirely apparent in only two dimensions, but becomes much more clear when looking at high-dimensional data.\nTo see this, let's take a quick look at the application of PCA to the digits data.\n\nWe start by loading the data:",
"_____no_output_____"
]
],
[
[
"from sklearn.datasets import load_digits\ndigits = load_digits()\ndigits.data.shape",
"_____no_output_____"
]
],
[
[
"Recall that the data consists of 8×8 pixel images, meaning that they are 64-dimensional.\nTo gain some intuition into the relationships between these points, we can use PCA to project them to a more manageable number of dimensions, say two:",
"_____no_output_____"
]
],
[
[
"pca = PCA(2) # project from 64 to 2 dimensions\nprojected = pca.fit_transform(digits.data)\nprint(digits.data.shape)\nprint(projected.shape)",
"(1797, 64)\n(1797, 2)\n"
]
],
[
[
"We can now plot the first two principal components of each point to learn about the data:",
"_____no_output_____"
]
],
[
[
"plt.scatter(projected[:, 0], projected[:, 1],\n c=digits.target, edgecolor='none', alpha=0.5,\n cmap=plt.cm.get_cmap('Accent', 10)\n )\nplt.xlabel('component 1')\nplt.ylabel('component 2')\nplt.colorbar();",
"_____no_output_____"
]
],
[
[
"Recall what these components mean: the full data is a 64-dimensional point cloud, and these points are the projection of each data point along the directions with the largest variance.\nEssentially, we have found the optimal stretch and rotation in 64-dimensional space that allows us to see the layout of the digits in two dimensions, and have done this in an unsupervised manner—that is, without reference to the labels.",
"_____no_output_____"
],
[
"### What do the components mean?\n\nWe can go a bit further here, and begin to ask what the reduced dimensions *mean*.\nThis meaning can be understood in terms of combinations of basis vectors.\nFor example, each image in the training set is defined by a collection of 64 pixel values, which we will call the vector $x$:\n\n$$\nx = [x_1, x_2, x_3 \\cdots x_{64}]\n$$\n\nOne way we can think about this is in terms of a pixel basis.\nThat is, to construct the image, we multiply each element of the vector by the pixel it describes, and then add the results together to build the image:\n\n$$\n{\\rm image}(x) = x_1 \\cdot{\\rm (pixel~1)} + x_2 \\cdot{\\rm (pixel~2)} + x_3 \\cdot{\\rm (pixel~3)} \\cdots x_{64} \\cdot{\\rm (pixel~64)}\n$$\n\nOne way we might imagine reducing the dimension of this data is to zero out all but a few of these basis vectors.\nFor example, if we use only the first eight pixels, we get an eight-dimensional projection of the data, but it is not very reflective of the whole image: we've thrown out nearly 90% of the pixels!",
"_____no_output_____"
],
[
"",
"_____no_output_____"
],
[
"The upper row of panels shows the individual pixels, and the lower row shows the cumulative contribution of these pixels to the construction of the image.\nUsing only eight of the pixel-basis components, we can only construct a small portion of the 64-pixel image.\nWere we to continue this sequence and use all 64 pixels, we would recover the original image.",
"_____no_output_____"
],
[
"But the pixel-wise representation is not the only choice of basis. We can also use other basis functions, which each contain some pre-defined contribution from each pixel, and write something like\n\n$$\nimage(x) = {\\rm mean} + x_1 \\cdot{\\rm (basis~1)} + x_2 \\cdot{\\rm (basis~2)} + x_3 \\cdot{\\rm (basis~3)} \\cdots\n$$\n\nPCA can be thought of as a process of choosing optimal basis functions, such that adding together just the first few of them is enough to suitably reconstruct the bulk of the elements in the dataset.\nThe principal components, which act as the low-dimensional representation of our data, are simply the coefficients that multiply each of the elements in this series.\nThis figure shows a similar depiction of reconstructing this digit using the mean plus the first eight PCA basis functions:",
"_____no_output_____"
],
[
"",
"_____no_output_____"
],
[
"Unlike the pixel basis, the PCA basis allows us to recover the salient features of the input image with just a mean plus eight components!\nThe amount of each pixel in each component is the corollary of the orientation of the vector in our two-dimensional example.\nThis is the sense in which PCA provides a low-dimensional representation of the data: it discovers a set of basis functions that are more efficient than the native pixel-basis of the input data.",
"_____no_output_____"
],
[
"### Choosing the number of components\n\nA vital part of using PCA in practice is the ability to estimate how many components are needed to describe the data.\nThis can be determined by looking at the cumulative *explained variance ratio* as a function of the number of components:",
"_____no_output_____"
]
],
[
[
"pca = PCA().fit(digits.data)\nplt.plot(np.cumsum(pca.explained_variance_ratio_))\nplt.xlabel('number of components')\nplt.ylabel('cumulative explained variance');",
"_____no_output_____"
]
],
[
[
"This curve quantifies how much of the total, 64-dimensional variance is contained within the first $N$ components.\nFor example, we see that with the digits the first 10 components contain approximately 75% of the variance, while you need around 50 components to describe close to 100% of the variance.\n\nHere we see that our two-dimensional projection loses a lot of information (as measured by the explained variance) and that we'd need about 20 components to retain 90% of the variance. Looking at this plot for a high-dimensional dataset can help you understand the level of redundancy present in multiple observations.",
"_____no_output_____"
],
[
"## PCA as Noise Filtering\n\nPCA can also be used as a filtering approach for noisy data.\nThe idea is this: any components with variance much larger than the effect of the noise should be relatively unaffected by the noise.\nSo if you reconstruct the data using just the largest subset of principal components, you should be preferentially keeping the signal and throwing out the noise.\n\nLet's see how this looks with the digits data.\nFirst we will plot several of the input noise-free data:",
"_____no_output_____"
]
],
[
[
"def plot_digits(data):\n fig, axes = plt.subplots(4, 10, figsize=(10, 4),\n subplot_kw={'xticks':[], 'yticks':[]},\n gridspec_kw=dict(hspace=0.1, wspace=0.1))\n for i, ax in enumerate(axes.flat):\n ax.imshow(data[i].reshape(8, 8),\n cmap='binary', interpolation='nearest',\n clim=(0, 16))\nplot_digits(digits.data)",
"_____no_output_____"
]
],
[
[
"Now lets add some random noise to create a noisy dataset, and re-plot it:",
"_____no_output_____"
]
],
[
[
"np.random.seed(42)\nnoisy = np.random.normal(digits.data, 4)\nplot_digits(noisy)",
"_____no_output_____"
]
],
[
[
"It's clear by eye that the images are noisy, and contain spurious pixels.\nLet's train a PCA on the noisy data, requesting that the projection preserve 50% of the variance:",
"_____no_output_____"
]
],
[
[
"pca = PCA(0.50).fit(noisy)\npca.n_components_",
"_____no_output_____"
]
],
[
[
"Here 50% of the variance amounts to 12 principal components.\nNow we compute these components, and then use the inverse of the transform to reconstruct the filtered digits:",
"_____no_output_____"
]
],
[
[
"components = pca.transform(noisy)\nfiltered = pca.inverse_transform(components)\nplot_digits(filtered)",
"_____no_output_____"
]
],
[
[
"This signal preserving/noise filtering property makes PCA a very useful feature selection routine—for example, rather than training a classifier on very high-dimensional data, you might instead train the classifier on the lower-dimensional representation, which will automatically serve to filter out random noise in the inputs.",
"_____no_output_____"
],
[
"## Example: Eigenfaces\n\nEarlier we explored an example of using a PCA projection as a feature selector for facial recognition with a support vector machine.\nHere we will take a look back and explore a bit more of what went into that.\nRecall that we were using the Labeled Faces in the Wild dataset made available through Scikit-Learn:",
"_____no_output_____"
]
],
[
[
"from sklearn.datasets import fetch_lfw_people\nfaces = fetch_lfw_people(min_faces_per_person=60)\nprint(faces.target_names)\nprint(faces.images.shape)",
"Downloading LFW metadata: https://ndownloader.figshare.com/files/5976012\nDownloading LFW metadata: https://ndownloader.figshare.com/files/5976009\nDownloading LFW metadata: https://ndownloader.figshare.com/files/5976006\nDownloading LFW data (~200MB): https://ndownloader.figshare.com/files/5976015\n"
]
],
[
[
"Let's take a look at the principal axes that span this dataset.\nBecause this is a large dataset, we will use ``RandomizedPCA``—it contains a randomized method to approximate the first $N$ principal components much more quickly than the standard ``PCA`` estimator, and thus is very useful for high-dimensional data (here, a dimensionality of nearly 3,000).\nWe will take a look at the first 150 components:",
"_____no_output_____"
]
],
[
[
"from sklearn.decomposition import PCA as RandomizedPCA\npca = RandomizedPCA(150)\npca.fit(faces.data)",
"_____no_output_____"
]
],
[
[
"In this case, it can be interesting to visualize the images associated with the first several principal components (these components are technically known as \"eigenvectors,\"\nso these types of images are often called \"eigenfaces\").\nAs you can see in this figure, they are as creepy as they sound:",
"_____no_output_____"
]
],
[
[
"fig, axes = plt.subplots(3, 8, figsize=(9, 4),\n subplot_kw={'xticks':[], 'yticks':[]},\n gridspec_kw=dict(hspace=0.1, wspace=0.1))\nfor i, ax in enumerate(axes.flat):\n ax.imshow(pca.components_[i].reshape(62, 47), cmap='bone')",
"_____no_output_____"
]
],
[
[
"The results are very interesting, and give us insight into how the images vary: for example, the first few eigenfaces (from the top left) seem to be associated with the angle of lighting on the face, and later principal vectors seem to be picking out certain features, such as eyes, noses, and lips.\nLet's take a look at the cumulative variance of these components to see how much of the data information the projection is preserving:",
"_____no_output_____"
]
],
[
[
"plt.plot(np.cumsum(pca.explained_variance_ratio_))\nplt.xlabel('number of components')\nplt.ylabel('cumulative explained variance');",
"_____no_output_____"
]
],
[
[
"We see that these 150 components account for just over 90% of the variance.\nThat would lead us to believe that using these 150 components, we would recover most of the essential characteristics of the data.\nTo make this more concrete, we can compare the input images with the images reconstructed from these 150 components:",
"_____no_output_____"
]
],
[
[
"# Compute the components and projected faces\npca = RandomizedPCA(150).fit(faces.data)\ncomponents = pca.transform(faces.data)\nprojected = pca.inverse_transform(components)",
"_____no_output_____"
],
[
"# Plot the results\nfig, ax = plt.subplots(2, 10, figsize=(10, 2.5),\n subplot_kw={'xticks':[], 'yticks':[]},\n gridspec_kw=dict(hspace=0.1, wspace=0.1))\nfor i in range(10):\n ax[0, i].imshow(faces.data[i].reshape(62, 47), cmap='binary_r')\n ax[1, i].imshow(projected[i].reshape(62, 47), cmap='binary_r')\n \nax[0, 0].set_ylabel('full-dim\\ninput')\nax[1, 0].set_ylabel('150-dim\\nreconstruction');",
"_____no_output_____"
]
],
[
[
"The top row here shows the input images, while the bottom row shows the reconstruction of the images from just 150 of the ~3,000 initial features.\nThis visualization makes clear why the PCA feature selection used in the Support Vector Machines example was so successful: although it reduces the dimensionality of the data by nearly a factor of 20, the projected images contain enough information that we might, by eye, recognize the individuals in the image.\nWhat this means is that our classification algorithm needs to be trained on 150-dimensional data rather than 3,000-dimensional data, which depending on the particular algorithm we choose, can lead to a much more efficient classification.",
"_____no_output_____"
],
[
"## Principal Component Analysis Summary\n\nIn this section we have discussed the use of principal component analysis for dimensionality reduction, for visualization of high-dimensional data, for noise filtering, and for feature selection within high-dimensional data.\nBecause of the versatility and interpretability of PCA, it has been shown to be effective in a wide variety of contexts and disciplines.\nGiven any high-dimensional dataset, I tend to start with PCA in order to visualize the relationship between points (as we did with the digits), to understand the main variance in the data (as we did with the eigenfaces), and to understand the intrinsic dimensionality (by plotting the explained variance ratio).\nCertainly PCA is not useful for every high-dimensional dataset, but it offers a straightforward and efficient path to gaining insight into high-dimensional data.\n\nPCA's main weakness is that it tends to be highly affected by outliers in the data.\nFor this reason, many robust variants of PCA have been developed, many of which act to iteratively discard data points that are poorly described by the initial components.\nScikit-Learn contains a couple interesting variants on PCA, including ``RandomizedPCA`` and ``SparsePCA``, both also in the ``sklearn.decomposition`` submodule.\n``RandomizedPCA``, which we saw earlier, uses a non-deterministic method to quickly approximate the first few principal components in very high-dimensional data, while ``SparsePCA`` introduces a regularization term that serves to enforce sparsity of the components.\n",
"_____no_output_____"
]
]
] | [
"markdown",
"code",
"markdown",
"code",
"markdown",
"code",
"markdown",
"code",
"markdown",
"code",
"markdown",
"code",
"markdown",
"code",
"markdown",
"code",
"markdown",
"code",
"markdown",
"code",
"markdown",
"code",
"markdown",
"code",
"markdown",
"code",
"markdown",
"code",
"markdown",
"code",
"markdown",
"code",
"markdown",
"code",
"markdown",
"code",
"markdown",
"code",
"markdown",
"code",
"markdown"
] | [
[
"markdown",
"markdown",
"markdown"
],
[
"code"
],
[
"markdown"
],
[
"code"
],
[
"markdown"
],
[
"code"
],
[
"markdown"
],
[
"code",
"code"
],
[
"markdown"
],
[
"code"
],
[
"markdown",
"markdown",
"markdown",
"markdown"
],
[
"code"
],
[
"markdown"
],
[
"code"
],
[
"markdown",
"markdown"
],
[
"code"
],
[
"markdown"
],
[
"code"
],
[
"markdown"
],
[
"code"
],
[
"markdown",
"markdown",
"markdown",
"markdown",
"markdown",
"markdown",
"markdown",
"markdown"
],
[
"code"
],
[
"markdown",
"markdown"
],
[
"code"
],
[
"markdown"
],
[
"code"
],
[
"markdown"
],
[
"code"
],
[
"markdown"
],
[
"code"
],
[
"markdown",
"markdown"
],
[
"code"
],
[
"markdown"
],
[
"code"
],
[
"markdown"
],
[
"code"
],
[
"markdown"
],
[
"code"
],
[
"markdown"
],
[
"code",
"code"
],
[
"markdown",
"markdown"
]
] |
d08e1a71f3afa2f0ecb3da625db9bac31ae4fd76 | 24,637 | ipynb | Jupyter Notebook | examples/Dense24HrPredictionNew-3Hr.ipynb | DivyaSDV/pySINDy | e7cba8f983e083ef8cdce66c7c1572276717b225 | [
"MIT"
] | null | null | null | examples/Dense24HrPredictionNew-3Hr.ipynb | DivyaSDV/pySINDy | e7cba8f983e083ef8cdce66c7c1572276717b225 | [
"MIT"
] | null | null | null | examples/Dense24HrPredictionNew-3Hr.ipynb | DivyaSDV/pySINDy | e7cba8f983e083ef8cdce66c7c1572276717b225 | [
"MIT"
] | null | null | null | 40.190865 | 1,635 | 0.553842 | [
[
[
"**Experiment for obtaining 24 Hr prediction from Dense Model in rainymotion library**\n\nAuthor: Divya S. Vidyadharan\n\nFile use: For predicting 24 Hr precipitation images with **3 hr lead time.** \n\nDate Created: 19-03-21\n\nLast Updated: 20-03-21\n\nPython version: 3.8.2",
"_____no_output_____"
]
],
[
[
"import h5py\nimport numpy as np\nimport matplotlib\nimport matplotlib.pyplot as plt\nimport scipy.misc\nimport sys\nimport os\nmodule_path = os.path.abspath(os.path.join('/home/divya/divya/OtherNowcastings/rainymotion-master'))\nif module_path not in sys.path:\n sys.path.append(module_path)\nfrom rainymotion.models import Dense\nfrom rainymotion.metrics import *\n\nimport cv2\nimport pandas as pd\nimport wradlib.ipol as ipol # for interpolation\nfrom rainymotion import metrics\nfrom rainymotion import utils\nfrom scipy.ndimage import map_coordinates\nimport timeit\nprint(cv2.__version__)\n#from tvl1sindysupport import tvl1utilities -in future our own library",
"3.4.2\n"
],
[
" times=['0000','0010', '0020', '0030', '0040', '0050',\n '0100', '0110', '0120', '0130', '0140', '0150',\n '0200', '0210', '0220', '0230', '0240', '0250',\n '0300', '0310', '0320', '0330', '0340', '0350',\n '0400', '0410', '0420', '0430', '0440' ,'0450',\n '0500', '0510', '0520', '0530', '0540', '0550',\n \n '0600', '0610', '0620', '0630', '0640', '0650',\n '0700', '0710', '0720', '0730', '0740', '0750',\n '0800', '0810', '0820', '0830', '0840', '0850',\n '0900', '0910', '0920', '0930', '0940', '0950',\n '1000', '1010', '1020', '1030', '1040', '1050',\n '1100', '1110', '1120', '1130', '1140', '1150',\n '1200', '1210', '1220', '1230', '1240', '1250',\n \n \n '1300', '1310', '1320', '1330', '1340', '1350',\n '1400', '1410', '1420', '1430', '1440', '1450',\n '1500', '1510', '1520', '1530', '1540', '1550',\n '1600', '1610', '1620', '1630', '1640', '1650',\n '1700', '1710', '1720', '1730', '1740', '1750',\n '1800', '1810', '1820', '1830', '1840', '1850',\n \n '1900', '1910', '1920', '1930', '1940', '1950',\n '2000', '2010', '2020', '2030', '2040', '2050',\n '2100', '2110', '2120', '2130', '2140', '2150',\n '2200', '2210', '2220', '2230', '2240', '2250',\n '2300', '2310', '2320', '2330', '2340', '2350']\n",
"_____no_output_____"
],
[
"# Common Initialization\neventName = \"TyphoonFaxai\"\neventDate =\"20190908\"\n\n#Latitude and Longitude of Typhoon Faxai\n\nlat1 = 32.5\nlat2 = 39\nlong1 = 136\nlong2 = 143\n\n\npred_date = 20190908 #YYYYMMDD\n[height, width] = [781,561]\neventNameDate = eventName + \"_\" + eventDate\n\n# startHr = 2\n# startMin= 40\n\n# predStartHr = 300\nstep = 5 #for rainymotion models\n\n\n# For radar images\ninputFolder = \"./ForExperiments/Exp1/RadarImages/HeavyRainfall/For300/\"\n# outputFolder= \"./ForExperiments/Exp1/Results/\"\n# print(inputFolder)\n\nfileType='.bin'\ntimeStep = 10 # for Japan Radar Data\n\nmodelName = \"Dense\" \n# startHr = 7# the first hr among for the three input images\n# startMin = 30 #\n# noOfImages = 3\nstepRainyMotion = 5 # 5 minutes\n# outputFilePath = outputFolder+modelName+'_'\n# outputFilePath = outputFilePath + eventNameDate\n\n# print(outputFilePath)\n\n\n##recentFramePath##\nrecentFrameFolder = str(pred_date)+\"_set_24Hr_bin\" #20190908_set_24Hr_bin\nrecentFramePath = \"/home/divya/divya/OneFullDayData_7TestCases_WNIMar5/%s\"%recentFrameFolder\nprint (\"\\n Recent frame path \",recentFramePath)\ninputFolder = recentFramePath\nprint(\"\\n Input folder is \",inputFolder)\n##Output path where predicted images for visual comparison are saved.##\noutputimgpath = \"/home/divya/divya/OneFullDayData_7TestCases_WNIMar5/24hroutputs/%i/%s/%s\"%(pred_date,modelName,\"pred_images\")\nos.makedirs(outputimgpath, exist_ok=True)\nprint (\"\\n Output image path is \",outputimgpath)\n\n##Output path where evaluation results are saved as csv files.##\noutputevalpath = \"/home/divya/divya/OneFullDayData_7TestCases_WNIMar5/24hroutputs/%i/%s/%s\"%(pred_date,modelName,\"eval_results\")\nos.makedirs(outputevalpath, exist_ok=True)\nprint (\"\\n Output eval results in \",outputevalpath)\n\nsavepath = outputimgpath#\"Outputs/%i/%s\"%(pred_date,pred_times[0])\n\nnoOfImages = 3 # Model needs 24 frames\n\nstep = 5\noutputFilePath = outputimgpath+'/'\noutputFilePath = outputFilePath + eventNameDate\n\nprint(outputFilePath)\n\nhrlimit = len(times)\nleadsteps = 18 #6\ntotinputframes = 2",
"\n Recent frame path /home/divya/divya/OneFullDayData_7TestCases_WNIMar5/20190908_set_24Hr_bin\n\n Input folder is /home/divya/divya/OneFullDayData_7TestCases_WNIMar5/20190908_set_24Hr_bin\n\n Output image path is /home/divya/divya/OneFullDayData_7TestCases_WNIMar5/24hroutputs/20190908/Dense/pred_images\n\n Output eval results in /home/divya/divya/OneFullDayData_7TestCases_WNIMar5/24hroutputs/20190908/Dense/eval_results\n/home/divya/divya/OneFullDayData_7TestCases_WNIMar5/24hroutputs/20190908/Dense/pred_images/TyphoonFaxai_20190908\n"
],
[
"def gettimes24hr(pred_time):\n \n \n # times=np.array(times)\n inptimes = []\n pred_times = []\n index = times.index(pred_time)\n indexlimit = len(times)\n print(\"Leadsteps are \", leadsteps)\n if (index+leadsteps) < indexlimit:\n pred_times = times[index:index+leadsteps]\n if (index-totinputframes)>=0:\n inptimes = times[index-totinputframes:index]\n \n print(\"PredTimes:\",pred_times)\n print(\"InpTimes:\",inptimes)\n print(\"Get Time Success..\")\n \n return inptimes, pred_times",
"_____no_output_____"
],
[
"def readRadarImages(pred_time,inputpath,height,width, noOfImages,fileType):\n files = (os.listdir(recentFramePath))\n files.sort()\n inputRadarImages = []\n \n i = 0\n index = times.index(pred_time)\n# print(index)\n inputframes = times[index-noOfImages:index]\n# print(len(inputframes))\n while (i<noOfImages):\n \n inputframetime = \"_\"+inputframes[i]\n i = i +1\n for fileName in files:\n\n if inputframetime in fileName:\n print(\"The input image at %s is available\",inputframetime)\n print(fileName)\n if fileName.endswith(fileType):\n\n \n inputFileName =recentFramePath+'/'+fileName\n fd = open(inputFileName,'rb')\n #print(inputFileName)\n\n # straight to numpy data (no buffering) \n inputFrame = np.fromfile(fd, dtype = np.dtype('float32'), count = 2*height*width)\n inputFrame = np.reshape(inputFrame,(height,width))\n inputFrame = inputFrame.astype('float16') \n\n #print(recentFrame.shape)\n inputRadarImages.append(inputFrame)\n #else:\n # print(\"Sorry, unable to find file.\")\n \n inputRadarImages = np.stack(inputRadarImages, axis=0)\n print(inputRadarImages.shape)\n return inputRadarImages\n",
"_____no_output_____"
]
],
[
[
"**1.2 Dense**",
"_____no_output_____"
]
],
[
[
"def doDenseNowcasting(startpredtime, saveimages):\n\n model = Dense()\n model.input_data = readRadarImages(startpredtime,inputFolder,height,width, noOfImages,fileType)\n start = timeit.timeit()\n nowcastDense = model.run()\n end = timeit.timeit()\n sparseTime = end - start\n print(\"Dense took \",end - start)\n print(nowcastDense.shape)\n# for i in range(12):\n# outFrameName = outputFilePath + '_'+str(predStartHr+(i*5))+'.png'\n# # print(outFrameName)\n# if saveimages:\n# matplotlib.image.imsave(outFrameName, nowcastDense[i])\n print(\"Finished Dense model nowcasting!\")\n return nowcastDense",
"_____no_output_____"
]
],
[
[
"**2. Performance Evaluation**",
"_____no_output_____"
]
],
[
[
"\ndef getGroundTruthImages(pred_times,leadsteps,recentFramePath,height,width,fileType):\n files = (os.listdir(recentFramePath))\n files.sort()\n groundTruthImages = []\n \n i = 0\n while (i<leadsteps):\n \n groundtruthtime = \"_\"+pred_times[i]\n i = i +1\n for fileName in files:\n\n if groundtruthtime in fileName:\n print(\"The ground truth at %s is available\",groundtruthtime)\n print(fileName)\n if fileName.endswith(fileType):\n\n \n inputFileName =recentFramePath+'/'+fileName\n fd = open(inputFileName,'rb')\n #print(inputFileName)\n\n # straight to numpy data (no buffering) \n recentFrame = np.fromfile(fd, dtype = np.dtype('float32'), count = 2*height*width)\n recentFrame = np.reshape(recentFrame,(height,width))\n recentFrame = recentFrame.astype('float16') \n\n #print(recentFrame.shape)\n groundTruthImages.append(recentFrame)\n #else:\n # print(\"Sorry, unable to find file.\")\n \n groundTruthImages = np.moveaxis(np.dstack(groundTruthImages), -1, 0)\n #print(groundTruthImages.shape)\n return groundTruthImages",
"_____no_output_____"
],
[
"def evaluate(nowcasts):\n fileType = '.bin'\n# leadsteps = 6 # 6 for 1 hr prediction, 18 for 3hr prediction\n groundTruthPath = recentFramePath\n print(pred_times)\n groundTruthImgs = getGroundTruthImages(pred_times,leadsteps,groundTruthPath,height,width,fileType)\n \n maelist = []\n farlist = []\n podlist= []\n csilist= []\n thres =1.0\n \n noOfPrecipitationImages = leadsteps\n j = 0 # using another index to skip 5min interval data from rainymotion\n for i in range(noOfPrecipitationImages):\n mae = MAE(groundTruthImgs[i],nowcasts[j])\n far = FAR(groundTruthImgs[i],nowcasts[j], threshold=0.1)\n pod = POD(groundTruthImgs[i],nowcasts[j], threshold=0.1)\n csi = CSI(groundTruthImgs[i],nowcasts[j],thres)\n maelist.append(mae)\n farlist.append(far)\n podlist.append(pod)\n csilist.append(csi)\n j = j + 2\n return csilist,maelist,farlist,podlist\n\n\n \n ",
"_____no_output_____"
]
],
[
[
"**2. 24 Hr Prediction**",
"_____no_output_____"
]
],
[
[
"startpredtime = '0110' #'1100'\n\nindex = times.index(startpredtime)\nindexlimit = times.index('2250') # Since we have only 6 more ground truths available from this time\nprint(index)\nprint(\"Last prediction is at index \", indexlimit)\ncsilist = []\nmaelist = []\npodlist = []\nfarlist = []\npred_time = startpredtime\nwhile index<indexlimit:#len(times):\n print(times[index])\n saveimages = 0\n if (index==66):\n saveimages=1\n intimes, pred_times = gettimes24hr(pred_time)\n nowcasts = doDenseNowcasting(pred_time,saveimages)\n \n csi,mae,far,pod = evaluate(nowcasts)\n \n csilist.append(csi)\n maelist.append(mae)\n podlist.append(pod)\n farlist.append(far)\n \n index = index+1\n pred_time = times[index] \n ",
"7\nLast prediction is at index 137\n0110\nLeadsteps are 6\nPredTimes: ['0110', '0120', '0130', '0140', '0150', '0200']\nInpTimes: ['0050', '0100']\nGet Time Success..\nThe input image at %s is available _0040\n20190908_004000.000.bin\nThe input image at %s is available _0050\n20190908_005000.000.bin\nThe input image at %s is available _0100\n20190908_010000.000.bin\n(3, 781, 561)\n"
],
[
"DISOpticalFlow_create\n# For debugging\nprint(len(maelist))\nprint(\"\\n\\n\")\nprint(len(csilist))\nprint(\"\\n\\n\")\nprint(len(podlist))\nprint(\"\\n\\n\")\nprint(len(farlist))\n",
"78\n\n\n\n78\n\n\n\n78\n\n\n\n78\n"
]
],
[
[
"**To save results in excel workbook**",
"_____no_output_____"
]
],
[
[
"import xlwt \nfrom xlwt import Workbook \n \n# Workbook is created \nwb = Workbook() \n",
"_____no_output_____"
],
[
"def writeinexcelsheet(sheetname, wb, results):\n \n sheet1 = wb.add_sheet(sheetname) \n sheet1.write(0, 0, 'Pred.no.') \n sheet1.write(0, 1, 't (pred start time)') \n sheet1.write(0, 2, 't + 10') \n sheet1.write(0, 3, 't + 20') \n sheet1.write(0, 4, 't + 30') \n sheet1.write(0, 5, 't + 40') \n sheet1.write(0, 6, 't + 50') \n \n \n col = 0\n\n rows = len(results)\n cols = len(results[0])\n print(cols)\n for rowno in range(rows):\n \n sheet1.write(rowno+1,0,rowno+1) \n for col in range(cols):\n# print(rowno+1,col+1,results[rowno][col])\n sheet1.write(rowno+1,col+1,results[rowno][col].astype('float64'))\n \n# sheet1.write(row, col, str(data))\n# print(row,col,data)\n \n \n ",
"_____no_output_____"
],
[
"writeinexcelsheet('CSI',wb,csilist)\nwriteinexcelsheet('MAE',wb,maelist)\nwriteinexcelsheet('FAR',wb,farlist)\nwriteinexcelsheet('POD',wb,podlist)\nexcelpath = \"/home/divya/divya/OneFullDayData_7TestCases_WNIMar5/24hroutputs/20190908/Dense/eval_results/\"\nexcelpath = excelpath + 'resultsDense.xls'\nwb.save(excelpath)\n",
"6\n6\n6\n6\n"
]
]
] | [
"markdown",
"code",
"markdown",
"code",
"markdown",
"code",
"markdown",
"code",
"markdown",
"code"
] | [
[
"markdown"
],
[
"code",
"code",
"code",
"code",
"code"
],
[
"markdown"
],
[
"code"
],
[
"markdown"
],
[
"code",
"code"
],
[
"markdown"
],
[
"code",
"code"
],
[
"markdown"
],
[
"code",
"code",
"code"
]
] |
d08e2c5798d4b8bae782b21e0c979b6e10ea2cb7 | 37,066 | ipynb | Jupyter Notebook | MOOCs/MathCalture/binom.ipynb | KeisukeShimokawa/CarND-Advanced-Lane-Lines | c861f124eb2a20a405ce26d55229fb3d13742aa4 | [
"MIT"
] | null | null | null | MOOCs/MathCalture/binom.ipynb | KeisukeShimokawa/CarND-Advanced-Lane-Lines | c861f124eb2a20a405ce26d55229fb3d13742aa4 | [
"MIT"
] | null | null | null | MOOCs/MathCalture/binom.ipynb | KeisukeShimokawa/CarND-Advanced-Lane-Lines | c861f124eb2a20a405ce26d55229fb3d13742aa4 | [
"MIT"
] | null | null | null | 38.055441 | 264 | 0.425862 | [
[
[
"<a href=\"https://colab.research.google.com/github/KeisukeShimokawa/CarND-Advanced-Lane-Lines/blob/master/MOOCs/MathCalture/binom.ipynb\" target=\"_parent\"><img src=\"https://colab.research.google.com/assets/colab-badge.svg\" alt=\"Open In Colab\"/></a>",
"_____no_output_____"
]
],
[
[
"%load_ext rpy2.ipython",
"_____no_output_____"
],
[
"%%writefile binom.stan\n\ndata{\n int X;\n int N;\n}\n\nparameters{\n real<lower=0,upper=1> p;\n real q;\n}\n\nmodel{\n X ~ binomial(N,p);\n p ~ beta(10,1);\n}",
"Writing binom.stan\n"
],
[
"%%R\nd <- list(X=28, N=50)",
"_____no_output_____"
],
[
"%%R\nd",
"$X\n[1] 28\n\n$N\n[1] 50\n\n"
],
[
"%%R\nsystem(\"apt-get install -y libv8-dev\")\ninstall.packages(\"V8\")",
"R[write to console]: Installing package into ‘/usr/local/lib/R/site-library’\n(as ‘lib’ is unspecified)\n\nR[write to console]: trying URL 'https://cran.rstudio.com/src/contrib/V8_3.4.0.tar.gz'\n\nR[write to console]: Content type 'application/x-gzip'\nR[write to console]: length 682079 bytes (666 KB)\n\nR[write to console]: =\nR[write to console]: =\nR[write to console]: =\nR[write to console]: =\nR[write to console]: =\nR[write to console]: =\nR[write to console]: =\nR[write to console]: =\nR[write to console]: =\nR[write to console]: =\nR[write to console]: =\nR[write to console]: =\nR[write to console]: =\nR[write to console]: =\nR[write to console]: =\nR[write to console]: =\nR[write to console]: =\nR[write to console]: =\nR[write to console]: =\nR[write to console]: =\nR[write to console]: =\nR[write to console]: =\nR[write to console]: =\nR[write to console]: =\nR[write to console]: =\nR[write to console]: =\nR[write to console]: =\nR[write to console]: =\nR[write to console]: =\nR[write to console]: =\nR[write to console]: =\nR[write to console]: =\nR[write to console]: =\nR[write to console]: =\nR[write to console]: =\nR[write to console]: =\nR[write to console]: =\nR[write to console]: =\nR[write to console]: =\nR[write to console]: =\nR[write to console]: =\nR[write to console]: =\nR[write to console]: =\nR[write to console]: =\nR[write to console]: =\nR[write to console]: =\nR[write to console]: =\nR[write to console]: =\nR[write to console]: =\nR[write to console]: =\nR[write to console]: \n\nR[write to console]: downloaded 666 KB\n\n\nR[write to console]: \n\nR[write to console]: \nR[write to console]: The downloaded source packages are in\n\t‘/tmp/RtmpfJfHJW/downloaded_packages’\nR[write to console]: \nR[write to console]: \n\n"
],
[
"%%R\ninstall.packages(\"rstan\")",
"R[write to console]: Installing package into ‘/usr/local/lib/R/site-library’\n(as ‘lib’ is unspecified)\n\nR[write to console]: also installing the dependencies ‘checkmate’, ‘matrixStats’, ‘StanHeaders’, ‘inline’, ‘gridExtra’, ‘RcppParallel’, ‘loo’, ‘RcppEigen’\n\n\nR[write to console]: trying URL 'https://cran.rstudio.com/src/contrib/checkmate_2.0.0.tar.gz'\n\nR[write to console]: Content type 'application/x-gzip'\nR[write to console]: length 168716 bytes (164 KB)\n\nR[write to console]: =\nR[write to console]: =\nR[write to console]: =\nR[write to console]: =\nR[write to console]: =\nR[write to console]: =\nR[write to console]: =\nR[write to console]: =\nR[write to console]: =\nR[write to console]: =\nR[write to console]: =\nR[write to console]: =\nR[write to console]: =\nR[write to console]: =\nR[write to console]: =\nR[write to console]: =\nR[write to console]: =\nR[write to console]: =\nR[write to console]: =\nR[write to console]: =\nR[write to console]: =\nR[write to console]: =\nR[write to console]: =\nR[write to console]: =\nR[write to console]: =\nR[write to console]: =\nR[write to console]: =\nR[write to console]: =\nR[write to console]: =\nR[write to console]: =\nR[write to console]: =\nR[write to console]: =\nR[write to console]: =\nR[write to console]: =\nR[write to console]: =\nR[write to console]: =\nR[write to console]: =\nR[write to console]: =\nR[write to console]: =\nR[write to console]: =\nR[write to console]: =\nR[write to console]: =\nR[write to console]: =\nR[write to console]: =\nR[write to console]: =\nR[write to console]: =\nR[write to console]: =\nR[write to console]: =\nR[write to console]: =\nR[write to console]: =\nR[write to console]: \n\nR[write to console]: downloaded 164 KB\n\n\nR[write to console]: trying URL 'https://cran.rstudio.com/src/contrib/matrixStats_0.58.0.tar.gz'\n\nR[write to console]: Content type 'application/x-gzip'\nR[write to console]: length 195698 bytes (191 KB)\n\nR[write to console]: =\nR[write to console]: =\nR[write to console]: =\nR[write to console]: =\nR[write to console]: =\nR[write to console]: =\nR[write to console]: =\nR[write to console]: =\nR[write to console]: =\nR[write to console]: =\nR[write to console]: =\nR[write to console]: =\nR[write to console]: =\nR[write to console]: =\nR[write to console]: =\nR[write to console]: =\nR[write to console]: =\nR[write to console]: =\nR[write to console]: =\nR[write to console]: =\nR[write to console]: =\nR[write to console]: =\nR[write to console]: =\nR[write to console]: =\nR[write to console]: =\nR[write to console]: =\nR[write to console]: =\nR[write to console]: =\nR[write to console]: =\nR[write to console]: =\nR[write to console]: =\nR[write to console]: =\nR[write to console]: =\nR[write to console]: =\nR[write to console]: =\nR[write to console]: =\nR[write to console]: =\nR[write to console]: =\nR[write to console]: =\nR[write to console]: =\nR[write to console]: =\nR[write to console]: =\nR[write to console]: =\nR[write to console]: =\nR[write to console]: =\nR[write to console]: =\nR[write to console]: =\nR[write to console]: =\nR[write to console]: =\nR[write to console]: =\nR[write to console]: \n\nR[write to console]: downloaded 191 KB\n\n\nR[write to console]: trying URL 'https://cran.rstudio.com/src/contrib/StanHeaders_2.21.0-7.tar.gz'\n\nR[write to console]: Content type 'application/x-gzip'\nR[write to console]: length 1402572 bytes (1.3 MB)\n\nR[write to console]: =\nR[write to console]: =\nR[write to console]: =\nR[write to console]: =\nR[write to console]: =\nR[write to console]: =\nR[write to console]: =\nR[write to console]: =\nR[write to console]: =\nR[write to console]: =\nR[write to console]: =\nR[write to console]: =\nR[write to console]: =\nR[write to console]: =\nR[write to console]: =\nR[write to console]: =\nR[write to console]: =\nR[write to console]: =\nR[write to console]: =\nR[write to console]: =\nR[write to console]: =\nR[write to console]: =\nR[write to console]: =\nR[write to console]: =\nR[write to console]: =\nR[write to console]: =\nR[write to console]: =\nR[write to console]: =\nR[write to console]: =\nR[write to console]: =\nR[write to console]: =\nR[write to console]: =\nR[write to console]: =\nR[write to console]: =\nR[write to console]: =\nR[write to console]: =\nR[write to console]: =\nR[write to console]: =\nR[write to console]: =\nR[write to console]: =\nR[write to console]: =\nR[write to console]: =\nR[write to console]: =\nR[write to console]: =\nR[write to console]: =\nR[write to console]: =\nR[write to console]: =\nR[write to console]: =\nR[write to console]: =\nR[write to console]: =\nR[write to console]: \n\nR[write to console]: downloaded 1.3 MB\n\n\nR[write to console]: trying URL 'https://cran.rstudio.com/src/contrib/inline_0.3.17.tar.gz'\n\nR[write to console]: Content type 'application/x-gzip'\nR[write to console]: length 24730 bytes (24 KB)\n\nR[write to console]: =\nR[write to console]: =\nR[write to console]: =\nR[write to console]: =\nR[write to console]: =\nR[write to console]: =\nR[write to console]: =\nR[write to console]: =\nR[write to console]: =\nR[write to console]: =\nR[write to console]: =\nR[write to console]: =\nR[write to console]: =\nR[write to console]: =\nR[write to console]: =\nR[write to console]: =\nR[write to console]: =\nR[write to console]: =\nR[write to console]: =\nR[write to console]: =\nR[write to console]: =\nR[write to console]: =\nR[write to console]: =\nR[write to console]: =\nR[write to console]: =\nR[write to console]: =\nR[write to console]: =\nR[write to console]: =\nR[write to console]: =\nR[write to console]: =\nR[write to console]: =\nR[write to console]: =\nR[write to console]: =\nR[write to console]: =\nR[write to console]: =\nR[write to console]: =\nR[write to console]: =\nR[write to console]: =\nR[write to console]: =\nR[write to console]: =\nR[write to console]: =\nR[write to console]: =\nR[write to console]: =\nR[write to console]: =\nR[write to console]: =\nR[write to console]: =\nR[write to console]: =\nR[write to console]: =\nR[write to console]: =\nR[write to console]: =\nR[write to console]: \n\nR[write to console]: downloaded 24 KB\n\n\nR[write to console]: trying URL 'https://cran.rstudio.com/src/contrib/gridExtra_2.3.tar.gz'\n\nR[write to console]: Content type 'application/x-gzip'\nR[write to console]: length 1062844 bytes (1.0 MB)\n\nR[write to console]: =\nR[write to console]: =\nR[write to console]: =\nR[write to console]: =\nR[write to console]: =\nR[write to console]: =\nR[write to console]: =\nR[write to console]: =\nR[write to console]: =\nR[write to console]: =\nR[write to console]: =\nR[write to console]: =\nR[write to console]: =\nR[write to console]: =\nR[write to console]: =\nR[write to console]: =\nR[write to console]: =\nR[write to console]: =\nR[write to console]: =\nR[write to console]: =\nR[write to console]: =\nR[write to console]: =\nR[write to console]: =\nR[write to console]: =\nR[write to console]: =\nR[write to console]: =\nR[write to console]: =\nR[write to console]: =\nR[write to console]: =\nR[write to console]: =\nR[write to console]: =\nR[write to console]: =\nR[write to console]: =\nR[write to console]: =\nR[write to console]: =\nR[write to console]: =\nR[write to console]: =\nR[write to console]: =\nR[write to console]: =\nR[write to console]: =\nR[write to console]: =\nR[write to console]: =\nR[write to console]: =\nR[write to console]: =\nR[write to console]: =\nR[write to console]: =\nR[write to console]: =\nR[write to console]: =\nR[write to console]: =\nR[write to console]: =\nR[write to console]: \n\nR[write to console]: downloaded 1.0 MB\n\n\nR[write to console]: trying URL 'https://cran.rstudio.com/src/contrib/RcppParallel_5.0.3.tar.gz'\n\nR[write to console]: Content type 'application/x-gzip'\nR[write to console]: length 1469709 bytes (1.4 MB)\n\nR[write to console]: =\nR[write to console]: =\nR[write to console]: =\nR[write to console]: =\nR[write to console]: =\nR[write to console]: =\nR[write to console]: =\nR[write to console]: =\nR[write to console]: =\nR[write to console]: =\nR[write to console]: =\nR[write to console]: =\nR[write to console]: =\nR[write to console]: =\nR[write to console]: =\nR[write to console]: =\nR[write to console]: =\nR[write to console]: =\nR[write to console]: =\nR[write to console]: =\nR[write to console]: =\nR[write to console]: =\nR[write to console]: =\nR[write to console]: =\nR[write to console]: =\nR[write to console]: =\nR[write to console]: =\nR[write to console]: =\nR[write to console]: =\nR[write to console]: =\nR[write to console]: =\nR[write to console]: =\nR[write to console]: =\nR[write to console]: =\nR[write to console]: =\nR[write to console]: =\nR[write to console]: =\nR[write to console]: =\nR[write to console]: =\nR[write to console]: =\nR[write to console]: =\nR[write to console]: =\nR[write to console]: =\nR[write to console]: =\nR[write to console]: =\nR[write to console]: =\nR[write to console]: =\nR[write to console]: =\nR[write to console]: =\nR[write to console]: =\nR[write to console]: \n\nR[write to console]: downloaded 1.4 MB\n\n\nR[write to console]: trying URL 'https://cran.rstudio.com/src/contrib/loo_2.4.1.tar.gz'\n\nR[write to console]: Content type 'application/x-gzip'\nR[write to console]: length 2597437 bytes (2.5 MB)\n\nR[write to console]: =\nR[write to console]: =\nR[write to console]: =\nR[write to console]: =\nR[write to console]: =\nR[write to console]: =\nR[write to console]: =\nR[write to console]: =\nR[write to console]: =\nR[write to console]: =\nR[write to console]: =\nR[write to console]: =\nR[write to console]: =\nR[write to console]: =\nR[write to console]: =\nR[write to console]: =\nR[write to console]: =\nR[write to console]: =\nR[write to console]: =\nR[write to console]: =\nR[write to console]: =\nR[write to console]: =\nR[write to console]: =\nR[write to console]: =\nR[write to console]: =\nR[write to console]: =\nR[write to console]: =\nR[write to console]: =\nR[write to console]: =\nR[write to console]: =\nR[write to console]: =\nR[write to console]: =\nR[write to console]: =\nR[write to console]: =\nR[write to console]: =\nR[write to console]: =\nR[write to console]: =\nR[write to console]: =\nR[write to console]: =\nR[write to console]: =\nR[write to console]: =\nR[write to console]: =\nR[write to console]: =\nR[write to console]: =\nR[write to console]: =\nR[write to console]: =\nR[write to console]: =\nR[write to console]: =\nR[write to console]: =\nR[write to console]: =\nR[write to console]: \n\nR[write to console]: downloaded 2.5 MB\n\n\nR[write to console]: trying URL 'https://cran.rstudio.com/src/contrib/RcppEigen_0.3.3.9.1.tar.gz'\n\nR[write to console]: Content type 'application/x-gzip'\nR[write to console]: length 1633360 bytes (1.6 MB)\n\nR[write to console]: =\nR[write to console]: =\nR[write to console]: =\nR[write to console]: =\nR[write to console]: =\nR[write to console]: =\nR[write to console]: =\nR[write to console]: =\nR[write to console]: =\nR[write to console]: =\nR[write to console]: =\nR[write to console]: =\nR[write to console]: =\nR[write to console]: =\nR[write to console]: =\nR[write to console]: =\nR[write to console]: =\nR[write to console]: =\nR[write to console]: =\nR[write to console]: =\nR[write to console]: =\nR[write to console]: =\nR[write to console]: =\nR[write to console]: =\nR[write to console]: =\nR[write to console]: =\nR[write to console]: =\nR[write to console]: =\nR[write to console]: =\nR[write to console]: =\nR[write to console]: =\nR[write to console]: =\nR[write to console]: =\nR[write to console]: =\nR[write to console]: =\nR[write to console]: =\nR[write to console]: =\nR[write to console]: =\nR[write to console]: =\nR[write to console]: =\nR[write to console]: =\nR[write to console]: =\nR[write to console]: =\nR[write to console]: =\nR[write to console]: =\nR[write to console]: =\nR[write to console]: =\nR[write to console]: =\nR[write to console]: =\nR[write to console]: =\nR[write to console]: \n\nR[write to console]: downloaded 1.6 MB\n\n\nR[write to console]: trying URL 'https://cran.rstudio.com/src/contrib/rstan_2.21.2.tar.gz'\n\nR[write to console]: Content type 'application/x-gzip'\nR[write to console]: length 1152008 bytes (1.1 MB)\n\nR[write to console]: =\nR[write to console]: =\nR[write to console]: =\nR[write to console]: =\nR[write to console]: =\nR[write to console]: =\nR[write to console]: =\nR[write to console]: =\nR[write to console]: =\nR[write to console]: =\nR[write to console]: =\nR[write to console]: =\nR[write to console]: =\nR[write to console]: =\nR[write to console]: =\nR[write to console]: =\nR[write to console]: =\nR[write to console]: =\nR[write to console]: =\nR[write to console]: =\nR[write to console]: =\nR[write to console]: =\nR[write to console]: =\nR[write to console]: =\nR[write to console]: =\nR[write to console]: =\nR[write to console]: =\nR[write to console]: =\nR[write to console]: =\nR[write to console]: =\nR[write to console]: =\nR[write to console]: =\nR[write to console]: =\nR[write to console]: =\nR[write to console]: =\nR[write to console]: =\nR[write to console]: =\nR[write to console]: =\nR[write to console]: =\nR[write to console]: =\nR[write to console]: =\nR[write to console]: =\nR[write to console]: =\nR[write to console]: =\nR[write to console]: =\nR[write to console]: =\nR[write to console]: =\nR[write to console]: =\nR[write to console]: =\nR[write to console]: =\nR[write to console]: \n\nR[write to console]: downloaded 1.1 MB\n\n\nR[write to console]: \n\nR[write to console]: \nR[write to console]: The downloaded source packages are in\n\t‘/tmp/RtmpfJfHJW/downloaded_packages’\nR[write to console]: \nR[write to console]: \n\n"
],
[
"%%R\nlibrary(rstan)",
"R[write to console]: Loading required package: StanHeaders\n\nR[write to console]: Loading required package: ggplot2\n\nR[write to console]: rstan (Version 2.21.2, GitRev: 2e1f913d3ca3)\n\nR[write to console]: For execution on a local, multicore CPU with excess RAM we recommend calling\noptions(mc.cores = parallel::detectCores()).\nTo avoid recompilation of unchanged Stan programs, we recommend calling\nrstan_options(auto_write = TRUE)\n\n"
],
[
"%%R\nd <- list(X=28, N=50)\nstanmodel <- stan_model(file=\"binom.stan\")",
"_____no_output_____"
],
[
"%%R\nfit <- sampling(stanmodel, data=d)",
"\nSAMPLING FOR MODEL 'binom' NOW (CHAIN 1).\nChain 1: \nChain 1: Gradient evaluation took 2.4e-05 seconds\nChain 1: 1000 transitions using 10 leapfrog steps per transition would take 0.24 seconds.\nChain 1: Adjust your expectations accordingly!\nChain 1: \nChain 1: \nChain 1: Iteration: 1 / 2000 [ 0%] (Warmup)\nChain 1: Iteration: 200 / 2000 [ 10%] (Warmup)\nChain 1: Iteration: 400 / 2000 [ 20%] (Warmup)\nChain 1: Iteration: 600 / 2000 [ 30%] (Warmup)\nChain 1: Iteration: 800 / 2000 [ 40%] (Warmup)\nChain 1: Iteration: 1000 / 2000 [ 50%] (Warmup)\nChain 1: Iteration: 1001 / 2000 [ 50%] (Sampling)\nChain 1: Iteration: 1200 / 2000 [ 60%] (Sampling)\nChain 1: Iteration: 1400 / 2000 [ 70%] (Sampling)\nChain 1: Iteration: 1600 / 2000 [ 80%] (Sampling)\nChain 1: Iteration: 1800 / 2000 [ 90%] (Sampling)\nChain 1: Iteration: 2000 / 2000 [100%] (Sampling)\nChain 1: \nChain 1: Elapsed Time: 1.24105 seconds (Warm-up)\nChain 1: 1.36107 seconds (Sampling)\nChain 1: 2.60213 seconds (Total)\nChain 1: \n\nSAMPLING FOR MODEL 'binom' NOW (CHAIN 2).\nChain 2: \nChain 2: Gradient evaluation took 1.5e-05 seconds\nChain 2: 1000 transitions using 10 leapfrog steps per transition would take 0.15 seconds.\nChain 2: Adjust your expectations accordingly!\nChain 2: \nChain 2: \nChain 2: Iteration: 1 / 2000 [ 0%] (Warmup)\nChain 2: Iteration: 200 / 2000 [ 10%] (Warmup)\nChain 2: Iteration: 400 / 2000 [ 20%] (Warmup)\nChain 2: Iteration: 600 / 2000 [ 30%] (Warmup)\nChain 2: Iteration: 800 / 2000 [ 40%] (Warmup)\nChain 2: Iteration: 1000 / 2000 [ 50%] (Warmup)\nChain 2: Iteration: 1001 / 2000 [ 50%] (Sampling)\nChain 2: Iteration: 1200 / 2000 [ 60%] (Sampling)\nChain 2: Iteration: 1400 / 2000 [ 70%] (Sampling)\nChain 2: Iteration: 1600 / 2000 [ 80%] (Sampling)\nChain 2: Iteration: 1800 / 2000 [ 90%] (Sampling)\nChain 2: Iteration: 2000 / 2000 [100%] (Sampling)\nChain 2: \nChain 2: Elapsed Time: 1.25463 seconds (Warm-up)\nChain 2: 1.28011 seconds (Sampling)\nChain 2: 2.53474 seconds (Total)\nChain 2: \n\nSAMPLING FOR MODEL 'binom' NOW (CHAIN 3).\nChain 3: \nChain 3: Gradient evaluation took 2.3e-05 seconds\nChain 3: 1000 transitions using 10 leapfrog steps per transition would take 0.23 seconds.\nChain 3: Adjust your expectations accordingly!\nChain 3: \nChain 3: \nChain 3: Iteration: 1 / 2000 [ 0%] (Warmup)\nChain 3: Iteration: 200 / 2000 [ 10%] (Warmup)\nChain 3: Iteration: 400 / 2000 [ 20%] (Warmup)\nChain 3: Iteration: 600 / 2000 [ 30%] (Warmup)\nChain 3: Iteration: 800 / 2000 [ 40%] (Warmup)\nChain 3: Iteration: 1000 / 2000 [ 50%] (Warmup)\nChain 3: Iteration: 1001 / 2000 [ 50%] (Sampling)\nChain 3: Iteration: 1200 / 2000 [ 60%] (Sampling)\nChain 3: Iteration: 1400 / 2000 [ 70%] (Sampling)\nChain 3: Iteration: 1600 / 2000 [ 80%] (Sampling)\nChain 3: Iteration: 1800 / 2000 [ 90%] (Sampling)\nChain 3: Iteration: 2000 / 2000 [100%] (Sampling)\nChain 3: \nChain 3: Elapsed Time: 1.31068 seconds (Warm-up)\nChain 3: 1.38023 seconds (Sampling)\nChain 3: 2.69091 seconds (Total)\nChain 3: \n\nSAMPLING FOR MODEL 'binom' NOW (CHAIN 4).\nChain 4: \nChain 4: Gradient evaluation took 1.4e-05 seconds\nChain 4: 1000 transitions using 10 leapfrog steps per transition would take 0.14 seconds.\nChain 4: Adjust your expectations accordingly!\nChain 4: \nChain 4: \nChain 4: Iteration: 1 / 2000 [ 0%] (Warmup)\nChain 4: Iteration: 200 / 2000 [ 10%] (Warmup)\nChain 4: Iteration: 400 / 2000 [ 20%] (Warmup)\nChain 4: Iteration: 600 / 2000 [ 30%] (Warmup)\nChain 4: Iteration: 800 / 2000 [ 40%] (Warmup)\nChain 4: Iteration: 1000 / 2000 [ 50%] (Warmup)\nChain 4: Iteration: 1001 / 2000 [ 50%] (Sampling)\nChain 4: Iteration: 1200 / 2000 [ 60%] (Sampling)\nChain 4: Iteration: 1400 / 2000 [ 70%] (Sampling)\nChain 4: Iteration: 1600 / 2000 [ 80%] (Sampling)\nChain 4: Iteration: 1800 / 2000 [ 90%] (Sampling)\nChain 4: Iteration: 2000 / 2000 [100%] (Sampling)\nChain 4: \nChain 4: Elapsed Time: 1.25314 seconds (Warm-up)\nChain 4: 1.30243 seconds (Sampling)\nChain 4: 2.55557 seconds (Total)\nChain 4: \n"
],
[
"",
"_____no_output_____"
]
]
] | [
"markdown",
"code"
] | [
[
"markdown"
],
[
"code",
"code",
"code",
"code",
"code",
"code",
"code",
"code",
"code",
"code"
]
] |
d08e405598161e5b05bf5754e7def40a303b03ec | 2,944 | ipynb | Jupyter Notebook | python-tuts/0-beginner/2-Variables-Memory/04 - Dynamic vs Static Typing.ipynb | AadityaGupta/Artificial-Intelligence-Deep-Learning-Machine-Learning-Tutorials | 352dd6d9a785e22fde0ce53a6b0c2e56f4964950 | [
"Apache-2.0"
] | 3,266 | 2017-08-06T16:51:46.000Z | 2022-03-30T07:34:24.000Z | python-tuts/0-beginner/2-Variables-Memory/04 - Dynamic vs Static Typing.ipynb | AadityaGupta/Artificial-Intelligence-Deep-Learning-Machine-Learning-Tutorials | 352dd6d9a785e22fde0ce53a6b0c2e56f4964950 | [
"Apache-2.0"
] | 150 | 2017-08-28T14:59:36.000Z | 2022-03-11T23:21:35.000Z | python-tuts/0-beginner/2-Variables-Memory/04 - Dynamic vs Static Typing.ipynb | AadityaGupta/Artificial-Intelligence-Deep-Learning-Machine-Learning-Tutorials | 352dd6d9a785e22fde0ce53a6b0c2e56f4964950 | [
"Apache-2.0"
] | 1,449 | 2017-08-06T17:40:59.000Z | 2022-03-31T12:03:24.000Z | 17.216374 | 232 | 0.483016 | [
[
[
"### Dynamic Typing",
"_____no_output_____"
],
[
"Python is dunamically typed.\n\nThis means that the type of a variable is simply the type of the object the variable name points to (references). The variable itself has no associated type.",
"_____no_output_____"
]
],
[
[
"a = \"hello\"",
"_____no_output_____"
],
[
"type(a)",
"_____no_output_____"
],
[
"a = 10",
"_____no_output_____"
],
[
"type(a)",
"_____no_output_____"
],
[
"a = lambda x: x**2",
"_____no_output_____"
],
[
"a(2)",
"_____no_output_____"
],
[
"type(a)",
"_____no_output_____"
]
],
[
[
"As you can see from the above examples, the type of the variable ``a`` changed over time - in fact it was simply the type of the object ``a`` was referencing at that time. No type was ever attached to the variable name itself.",
"_____no_output_____"
]
]
] | [
"markdown",
"code",
"markdown"
] | [
[
"markdown",
"markdown"
],
[
"code",
"code",
"code",
"code",
"code",
"code",
"code"
],
[
"markdown"
]
] |
d08e51e3ab1d2437ec720d9df8867e11244cc549 | 219,188 | ipynb | Jupyter Notebook | muAugment.ipynb | Mariana-Andrade-Alves/muAugment | b59677796ec4260a6c97ea7a3ba85284ab50635e | [
"CC0-1.0"
] | null | null | null | muAugment.ipynb | Mariana-Andrade-Alves/muAugment | b59677796ec4260a6c97ea7a3ba85284ab50635e | [
"CC0-1.0"
] | null | null | null | muAugment.ipynb | Mariana-Andrade-Alves/muAugment | b59677796ec4260a6c97ea7a3ba85284ab50635e | [
"CC0-1.0"
] | null | null | null | 134.388719 | 44,442 | 0.842432 | [
[
[
"# **M**odel **U**ncertainty-based Data **Augment**ation (muAugment)\n\n<a rel=\"license\" href=\"http://creativecommons.org/licenses/by-nc-sa/4.0/\"><img alt=\"Creative Commons License\" align=\"left\" src=\"https://i.creativecommons.org/l/by-nc-sa/4.0/80x15.png\" /></a> | Mariana Alves | <a href=\"https://supaerodatascience.github.io/deep-learning/\">https://supaerodatascience.github.io/deep-learning/</a>",
"_____no_output_____"
],
[
"## Preliminary work for colab",
"_____no_output_____"
],
[
"**This notebook was written in google colab, so it is recommended that you run it in colab as well.**",
"_____no_output_____"
],
[
"<a href=\"https://colab.research.google.com/github/Mariana-Andrade-Alves/muAugment/blob/main/muAugment.ipynb\">\n <img src=\"https://colab.research.google.com/assets/colab-badge.svg\" alt=\"Open In Colab\"/>\n</a>",
"_____no_output_____"
],
[
"Before starting to work on the notebook, make sure you `change the Runtime type` to **GPU**, in the `Tool` drop menu.",
"_____no_output_____"
],
[
"In colab, please execute first the following cells, to retrieve the GitHub repository content. ",
"_____no_output_____"
]
],
[
[
"!git clone https://github.com/Mariana-Andrade-Alves/muAugment/",
"_____no_output_____"
]
],
[
[
"## Preliminary Imports",
"_____no_output_____"
]
],
[
[
"# !pip install matplotlib",
"_____no_output_____"
],
[
"# !pip install torch torchvision",
"_____no_output_____"
],
[
"import torch\nimport torchvision\nimport numpy as np\n%matplotlib inline\nimport matplotlib.pyplot as plt",
"_____no_output_____"
],
[
"from torch import nn, optim\nimport torch.nn.functional as F\nfrom torch.utils.data import DataLoader, Dataset\nfrom torchvision import datasets, transforms\nfrom torchvision.datasets import FashionMNIST",
"_____no_output_____"
],
[
"device = torch.device(\"cuda:0\" if torch.cuda.is_available() else \"cpu\")\nprint(device)",
"cpu\n"
]
],
[
[
"## Overview of Data Augmentation",
"_____no_output_____"
],
[
"Modern machine learning models, such as deep neural networks, may have billions of parameters and, consequently, require massive labeled training datasets, which are often not available. In order to avoid the **problem of data scarcity** in such models, data augmentation has become the standard technique used in nearly every state-of-the-art model in applications such as **image** and **text classification**.\n\n> **Data augmentation refers to the technique of artificially expanding labelled training datasets by generating new data through transformation functions.**\n\n\n\n\n",
"_____no_output_____"
],
[
"Data augmentation schemes often rely on the composition of a set of simple transformation functions (TFs) such as rotation and flip. \n\n<img src=\"https://github.com/Mariana-Andrade-Alves/muAugment/blob/main/img/transforms.png?raw=1\" width='800'>\n\n\"Label-invariant transformations.\" [torchvision.transforms docs](https://pytorch.org/vision/stable/transforms.html#transforms-on-pil-image-only)\n\nAs was briefly discussed in the [computer vision class](https://github.com/SupaeroDataScience/deep-learning/blob/main/vision/1_hands_on.ipynb), when chosen carefully, data augmentation schemes tuned by human experts can improve model performance. However, such heuristic strategies in practice can cause large variances in end model performance, and may not produce parameterizations and compositions needed for state-of-the-art models. In addition, they are extremely laborious.",
"_____no_output_____"
],
[
"### Automated Data Augmentation Schemes",
"_____no_output_____"
],
[
"Instead of performing manual search, automated data augmentation approaches hold promise to search for more powerful parameterizations and compositions of transformations. \n\nThe biggest difficulty with automating data augmentation is how to search over the space of transformations. This can be prohibitively expensive due to the large number of transformation functions in the search space. \n\n> **How can we design algorithms that explore the space of transformation functions efficiently and effectively, and find augmentation strategies that can outperform human-designed heuristics?**\n\nThe folklore wisdom behind data augmentation is that adding more labeled data improves generalization, i.e. the performance of the trained model on unseen test data. However, even for simpler models, **it is not well-understood how training on augmented data affects the learning process, the parameters, and the decision surface of the resulting model**.",
"_____no_output_____"
],
[
"<details class=\"alert alert-block alert-info\">\n <summary markdown=\"span\"><b>Extra information on the Adversarial AutoAugment Scheme previsouly discussed in class (click to expand)</b></summary>\n\nOne of the current state-of-the-art algorithms in terms of performance is [Adversarial AutoAugment](https://openreview.net/pdf?id=ByxdUySKvS), which makes use of [GANs](https://proceedings.neurips.cc/paper/2014/file/5ca3e9b122f61f8f06494c97b1afccf3-Paper.pdf), already presented in a previous [class](https://github.com/SupaeroDataScience/deep-learning/tree/main/GAN), to generate new data, rather than using the traditional heuristic transformations presented above. \n\n<img src=\"https://github.com/Mariana-Andrade-Alves/muAugment/blob/main/img/AdvAA.png?raw=1\" width='800'>\n\nLi et al. \"Adversarial AutoAugment training framework (Zhang et al. 2019) is formulated as an adversarial min-max game.\" [Automating the Art of Data Augmentation.](https://hazyresearch.stanford.edu/blog/2020-02-26-data-augmentation-part2) 2020.\n\nAlthough proven effective, this technique is still computationally expensive. Additionally, despite its rapid progress, this technique does not allow for a theoretical understanding of the benefits of a given transformation.\n</details>\n\n",
"_____no_output_____"
],
[
"## How should we think of the effects of applying a transformation?\n\n### Intuition for Linear Transformations",
"_____no_output_____"
],
[
"Suppose we are given $n$ training data points $x_1,...,x_n \\in \\mathbb{R}^p$ as $X \\in \\mathbb{R}^{n\\times p}$ with labels $Y\\in \\mathbb{R}^n$.\n\n> Suppose that the labels $Y$ obey the true linear model under ground parameters $\\beta \\in \\mathbb{R}^p$, $$Y = X \\beta + \\epsilon,$$ where $\\epsilon \\in \\mathbb{R}^n$ denotes i.d.d. random noise with mean zero and variance $\\sigma²$.\n\nImportantly, we assume that $p>n$, hence the span of the training data does not contain the entire space of $\\mathbb{R}^p$.\n\nLet's suppose we have an estimator $\\hat{\\beta}$ for the linear model $\\beta \\in \\mathbb{R}^p$. The error of that given estimator is \n\n> $$e(\\hat{\\beta}) = \\underbrace{\\lVert \\underset{\n \\epsilon}{\\mathbb{E}}[\\hat{\\beta}]-\\beta\\rVert^2}_{bias} + \\underbrace{\\lVert\\hat{\\beta} - \\underset{\\epsilon}{\\mathbb{E}}[\\beta] \\rVert^2}_{variance}$$\n\nwhere the bias part, intuitively, measures the intrinsic error of the model after taking into account the randomness which is present in $\\hat{\\beta}$.\n",
"_____no_output_____"
],
[
"### Label-Invariant Transformations",
"_____no_output_____"
],
[
"For a matrix $F \\in \\mathbb{R}^{p\\times p}$, we say that $F$ is a label-invariant transformation over $\\chi \\subseteq \\mathbb{R}^p $ for $\\beta \\in \\mathbb{R}^p$ if $$x^\\top\\beta = (Fx)^\\top\\beta, \\quad \\text{ for any } x \\in \\chi.$$\n\n> In simpler words, a label-invariant transformation will not alter the label $y$ of a given data point $x$.",
"_____no_output_____"
],
[
"**But what is the effect of such a transformation?**\n\nGiven a training data point $(x,y)$, let $(x^{aug},y^{aug})$ denote the augmented data where $y^{aug} = y$ and $x^{aug} = Fx$. \n\n**Note**: In order to be able to present the next result, let's consider adding the augmented data point $(z,y^{aug})$, where $z = P^\\bot_X Fx$, meaning $z$ is not $x^{aug}$, but the projection of $x^{aug}$ onto $P^\\bot_X = Id_p -P_X$, which denotes the projection operator which is ortogonal to the projection matrix onto the row of $X$ ($P_X$). In such a case, $y^{aug} = y - Diag[(X^\\top)^†Fx]Y$.\n> An intuiton to understand why we chose to use the projection $P^\\bot_X a^{aug}$ instead of the augmented data is to think about the idea of \"adding new information\". Remember: we assume that $p>n$, hence the subspace over which we can make an accurate estimation does not contain the entire space of $\\mathbb{R}^p$. When we a data point belonging to a space ortogonal to the one we know, we expand the subspace over which we can make an accurate estimation, by adding a direction corresponding to $P^\\bot_X a^{aug}$.\n\nSuppose the estimator $\\hat{\\beta}$ used to infer labels is a ridge estimator with a penalty parameter $\\lambda$, given by\n\n$$\\hat{\\beta}(X,Y) = (X^\\top X + n \\lambda Id)^{-1}X^\\top Y, $$\n\nwhere, just to recap, $X$ denotes the training data and $Y$ the training labels.\n\nConsidering $e(\\hat{\\beta})$ and $e(\\hat{\\beta}^F)$ has the errors of the estimator before and after adding the augmented data point $(z,y^{aug})$ to $(X,Y)$, it is possible to demonstrate that,\n\n$$ 0 \\leq e(\\hat{\\beta}) - e(\\hat{\\beta}^F) - (2+o(1))\\dfrac{\\langle z,\\beta \\rangle²}{\\lambda n} \\leq \\dfrac{poly(\\gamma/\\lambda)}{n²}, $$ where $poly(\\gamma/\\lambda)$ denotes a polynomial of $\\gamma/\\lambda$.\n\nThis powerful result, which we will not explain in class but can be found in the [muAugment paper](https://hazyresearch.stanford.edu/blog/2020-02-26-data-augmentation-part2), shows that\n\n* **the reduction of the estimation error**, $e(\\hat{\\beta}) - e(\\hat{\\beta}^F)$, **scales with the correlation between the new signal and the true model**, $\\langle z,\\beta \\rangle²$. \n\nIn other words, by adding $P^\\bot_X Fx$, we reduce the **estimation error** of the ridge estimator at a rate proportional to $\\langle z,\\beta \\rangle²$.\n\nFinally, we know that the larger the correlation $\\langle z,\\beta \\rangle²$, the higher the loss of $(x^{aug},y^{aug})$ would be under $\\hat{\\beta}$. We this information, we can extrapolate the following:\n* **the reduction of the estimation error**, $e(\\hat{\\beta}) - e(\\hat{\\beta}^F)$, **scales with the loss of $(x^{aug},y^{aug})$ under $\\hat{\\beta}$**, $l_{\\hat{\\beta}}(x^{aug},y^{aug})$.",
"_____no_output_____"
],
[
"> **In an intuitive sense, an augmented data point with a small loss means the model has already learned how to predict that type of data well, so if trained on it further, the model will only pick up incidental, possibly spurious patterns — overfitting. Conversely, an augmented data point with a large loss means the model has not learned the general mapping between the type of data and its target yet, so we need to train more on those kinds of data points.**\n",
"_____no_output_____"
],
[
"Additional results regarding **label-mixing transformations** were obtained in the [muAugment paper](https://hazyresearch.stanford.edu/blog/2020-02-26-data-augmentation-part2). These results will not be discussed in the class.",
"_____no_output_____"
],
[
"## Uncertainty-based Sampling Scheme",
"_____no_output_____"
],
[
"In order to take advantage of the last result presented, the **muAugment** algorithm was developped. The algorithm is as follows:\n\n* In a first step, for each data point, **C** compositions of **L** linear transformations are randomly sampled and fed to the learning model (in this example a neural network). \n\n* In a second step, the **S** transformed samples with the highest losses are picked for training the model and a backpropagation is performed using those samples.\n\n> **The intuition behind the sampling scheme is that these transformed samples that have the largest losses should also provide the most information.**\n\n**The model learns more generalizable patterns, because the algorithm assures extra fitting on the \"hard\" augmentations while skipping the easy ones.**\n\n<img src=\"https://github.com/Mariana-Andrade-Alves/muAugment/blob/main/img/dauphin.png?raw=1\" width='800'>\n\nSenwu. \"Uncertainty-based random Sampling Scheme for Data Augmentation. Each transformation function is randomly sampled from a pre-defined set of operations.\" [Dauphin](https://github.com/senwu/dauphin) 2020.",
"_____no_output_____"
],
[
"<details class=\"alert alert-block alert-info\">\n <summary markdown=\"span\"><b>Comparison to Adversarial Autoaugment (click to expand)</b></summary>\n\nThe idea behing this sampling scheme is conceptually similar to [Adversarial Autoaugment](https://openreview.net/pdf?id=ByxdUySKvS). However, while in the case of Adversarial Autoaugment, an additional adversarial network is used to generate augmented samples with large losses, in the current case, the model uses the training network itself to generate augmented samples.\n</details>\n\n\n",
"_____no_output_____"
],
[
"Our goal today is to implement the **muAugment** algorithm and evaluate its performance.",
"_____no_output_____"
],
[
"### The Dataset: FashionMNIST",
"_____no_output_____"
],
[
"The dataset we will use for this application is the FashionMNIST dataset. We'll download this dataset and make batching data loaders.",
"_____no_output_____"
]
],
[
[
"batch_size = 4\nn_images = 10 if (batch_size>10) else batch_size\n\n# data must be normalized between -1 and 1\ntransform = transforms.Compose([transforms.ToTensor(),\n transforms.Normalize((0.5,), (0.5,))])\n\nfull_trainset = FashionMNIST(root='../data', train=True, download=True, transform=transform)\ntrainset, full_validset = torch.utils.data.random_split(full_trainset, (10000, 50000)) # 10000 images for the training set\nvalidset, _ = torch.utils.data.random_split(full_validset, (1000, 49000)) # 1000 images for the validation set\n\ntrainloader = DataLoader(trainset, batch_size=64, shuffle=True, num_workers=2)\nvalidloader = DataLoader(validset, batch_size=64, shuffle=True, num_workers=2)\n\ntestset = FashionMNIST(root='../data', train=False, download=True, transform=transform)\ntestloader = DataLoader(testset, batch_size=64, shuffle=True)",
"_____no_output_____"
]
],
[
[
"We can verify the normalization of our data.",
"_____no_output_____"
]
],
[
[
"images,labels = next(iter(trainloader))\nimages.min(),images.max()",
"_____no_output_____"
]
],
[
[
"Let's look at some example images from the FashionMNIST set.",
"_____no_output_____"
]
],
[
[
"# get the first batch of images and labels\nlabels_text = [\"T-shirt/top\", \"Trouser\", \"Pullover\", \"Dress\", \"Coat\", \"Sandal\", \"Shirt\", \"Sneaker\", \"Bag\", \"Ankle boot\"]\n\nplt.figure(figsize=(n_images,4))\nfor i in range(n_images):\n l = labels[i].numpy()\n plt.subplot(2, n_images/2, i+1)\n plt.title('%d: %s' % (l, labels_text[l]))\n plt.imshow(images[i].numpy()[0], cmap='Greys')\n plt.axis('off')",
"_____no_output_____"
]
],
[
[
"### The Model",
"_____no_output_____"
],
[
"As mentioned above, the advantage of the **muAugment** algorithm is that it uses the learning model to automate data augmentation. The goal is to generate data which will improve our training model.\n\nIn today's example, we wish to learn to classify images into 10 possible labels:",
"_____no_output_____"
]
],
[
[
"labels_text",
"_____no_output_____"
]
],
[
[
"In order to do this, the training model we will use is a convolutional neural network, presented during a [previous class](https://github.com/SupaeroDataScience/deep-learning/blob/main/deep/PyTorch%20Ignite.ipynb).",
"_____no_output_____"
]
],
[
[
"class CNN(nn.Module):\n \n def __init__(self):\n super(CNN, self).__init__()\n \n self.convlayer1 = nn.Sequential(\n nn.Conv2d(1, 32, 3,padding=1),\n nn.BatchNorm2d(32),\n nn.ReLU(),\n nn.MaxPool2d(kernel_size=2, stride=2)\n )\n \n self.convlayer2 = nn.Sequential(\n nn.Conv2d(32,64,3),\n nn.BatchNorm2d(64),\n nn.ReLU(),\n nn.MaxPool2d(2)\n )\n \n self.fc1 = nn.Linear(64*6*6,600)\n self.drop = nn.Dropout2d(0.25)\n self.fc2 = nn.Linear(600, 120)\n self.fc3 = nn.Linear(120, 10)\n \n def forward(self, x):\n x = self.convlayer1(x)\n x = self.convlayer2(x)\n x = x.view(-1,64*6*6)\n x = self.fc1(x)\n x = self.drop(x)\n x = self.fc2(x)\n x = self.fc3(x)\n \n return F.log_softmax(x,dim=1)",
"_____no_output_____"
]
],
[
[
"### Training",
"_____no_output_____"
],
[
"In order to train the model, we must first create it and define the loss function and optimizer.",
"_____no_output_____"
]
],
[
[
"#creating model for original data\nmodel_original = CNN()\n# creating model for augmented data\nmodel = CNN()\n# moving models to gpu if available\ndevice = torch.device(\"cuda:0\" if torch.cuda.is_available() else \"cpu\")\nmodel.to(device)\nmodel_original.to(device)",
"_____no_output_____"
]
],
[
[
"By using the parameter `weight_decay` in our optimizer we are applying a similar penalty parameter to the one in ridge regression.",
"_____no_output_____"
]
],
[
[
"lr = 0.001 # learning rate\n# defining optimizer and loss for original model\noptimizer_original = torch.optim.SGD(model_original.parameters(), lr=lr, weight_decay=0.0001, momentum=0.9)\ncriterion_original = nn.CrossEntropyLoss()\n# defining optimizer and loss for augmented model\noptimizer = torch.optim.SGD(model.parameters(), lr=lr, weight_decay=0.0001, momentum=0.9)\ncriterion = nn.CrossEntropyLoss()",
"_____no_output_____"
]
],
[
[
"In a typical training phase, each batch of images would be treated in the following loop:\n```python\nfor epoch in range(max_epochs):\n for batch in trainloader:\n # zero the parameter gradients\n optimizer.zero_grad()\n # get inputs and labels from batch\n inputs, labels = batch\n # forward + backward + optimize\n outputs = model(inputs)\n loss = criterion(outputs, labels)\n loss.backward()\n optimizer.step()\n```\nIn order to perform data augmentation with pre-defined transforms, it would suffice to declare the transforms while generating the data loader and the loop would remain unchanged.",
"_____no_output_____"
],
[
"However, because we don't wish to train the model without evaluating the performance of each transform, this loop is going to change.",
"_____no_output_____"
],
[
"### The Random Sampling Scheme (hands-on exercises)",
"_____no_output_____"
],
[
"As mentioned above, the goal is to implement the following algorithm:\n\n<img src=\"https://github.com/Mariana-Andrade-Alves/muAugment/blob/main/img/algorithm.png?raw=1\" width=\"1000\">\n\nWu et al. \"Uncertainty-based random Sampling Algorithm.\" [On the Generalization Effects of Linear Transformations in Data Augmentation](https://arxiv.org/pdf/2005.00695.pdf) 2020.",
"_____no_output_____"
],
[
"Today, to simplify our work, we will not use default transformations. We will also only consider label-invariant transformations.",
"_____no_output_____"
],
[
"In our implementation, lets consider the following required arguments:\n* **L** (int): Number of **linear** transformations uniformly sampled for each composition\n* **C** (int): Number of **compositions** placed on each image \n* **S** (int): Number of **selected** compositions for each image",
"_____no_output_____"
],
[
"> **In a first exercise, let's attempt to code the lines 4 and 5 of the algorithm. Complete the function `compute_composed_data` which takes as input a `transform_list` similar to the one presented bellow, the arguments `L` and `C` described above and the images `xb` and labels `yb` of a batch and returns 2 tensors `C_images` and `C_targets` which contain the images xb$^{\\mathbf{aug}}$ and labels yb$^{\\mathbf{aug}}$ of the augmented data.**\n```python\ntransform_list = [transforms.RandomAutocontrast(p=p),\n transforms.ColorJitter(brightness=MAGN/30),\n transforms.ColorJitter(contrast=MAGN/30),\n transforms.RandomInvert(p=p), \n transforms.RandomRotation(degrees=MAGN*3),\n transforms.RandomAdjustSharpness(0.18*MAGN+0.1, p=p),\n transforms.RandomAffine(degrees=0, shear=MAGN/30),\n transforms.RandomSolarize(MAGN*8, p=p),\n transforms.RandomAffine(degrees=(0,0), \n translate=(MAGN/30,0),shear=(0,0)),\n transforms.RandomAffine(degrees=(0,0), \n translate=(0,MAGN/30),shear=(0,0)),\n ]\n``` ",
"_____no_output_____"
]
],
[
[
"# the load command only works on jupyter notebook\n# %load solutions/compute_composed_data.py\ndef compute_composed_data(transform_list,L, C, xb,yb):\n BS,N_CHANNELS,HEIGHT,WIDTH = xb.shape\n\n C_images = torch.zeros(C, BS, N_CHANNELS, HEIGHT, WIDTH, device=device)\n C_targets = torch.zeros(C, BS, device=device, dtype=torch.long)\n\n for c in range(C):\n # create a list of L linear transforms randomly sampled from the transform_list\n \n # create a composition of transforms from the list sampled above. Use nn.Sequential instead of transforms.Compose in order to script the transformations\n \n # apply the composition to the original images xb\n \n # update tensors C_images and C_targets with the generated compositions\n \n \n return C_images, C_targets",
"_____no_output_____"
],
[
"# the cat command works on google colab\n#%cat muAugment/solutions/compute_composed_data.py",
"cat: muAugment/solutions/compute_composed_data.py: No such file or directory\r\n"
]
],
[
[
"Now that we have implemented the data augmentation part, we can attempt to code the content of the main loop of the algorithm. \n\n**Remember**: the idea is to feed the transformed batches to the model without updating it and compare the losses obtained for each batch. Since you do not want to call `python loss.backward()`, you can disable gradient calculation in your function by using `python @torch.no_grad()`.",
"_____no_output_____"
],
[
"> **In a second exercise, complete the function `compute_selected_data` that takes as inputs the learning `model`, the `loss` function, the tensors `C_images` and `C_targets` and the argument `S` and returns the seleted transformed images (`S_images`) and labels (`S_labels`).**",
"_____no_output_____"
]
],
[
[
"# the load command only works on jupyter notebook\n# %load solutions/compute_selected_data.py\n\n#disable gradient calculation\n\ndef compute_selected_data(model, loss, C_images, C_targets, S):\n C, BS, N_CHANNELS, HEIGHT, WIDTH = C_images.shape\n\n # create a list of predictions 'pred' by applying the model to the augmented batches contained in C_images\n \n\n # create a list of losses by applying the loss function to the predictions and labels C_targets\n # convert the list to a loss tensor 'loss_tensor' through the function torch.stack\n \n\n # select the S indices 'S_idxs' of the loss_tensor with the highest value. You may use the function torch.topk\n \n\n # select the S images 'S_images' from C_images with the highest losses\n \n # convert the tensor 'S_images' so that it passes from shape [S, BS, N_CHANNELS, HEIGHT, WIDTH] to shape\n # [S*BS, N_CHANNELS, HEIGHT, WIDTH]. You may use the function torch.view\n \n\n # select the S labels 'S_targets' from C_targets corresponding to the highest losses\n \n # convert the tensor 'S_targets' so that it passes from shape [S, BS] to shape\n # [S*BS]. You may use the function torch.view\n \n \n return S_images, S_targets",
"_____no_output_____"
],
[
"# the cat command works on google colab\n#%cat muAugment/solutions/compute_selected_data.py",
"cat: muAugment/solutions/compute_selected_data.py: No such file or directory\r\n"
]
],
[
[
"We have created two functions which give us the augmented data we wish to use in the training phase of our model.",
"_____no_output_____"
],
[
"### Back to Training (hands-on exercise)\n\n",
"_____no_output_____"
],
[
"Let's consider the following arguments for the algorithm:",
"_____no_output_____"
]
],
[
[
"# algorithm arguments\nL = 3 # number of linear transformations sampled for each composition\nC = 4 # number of compositions placed on each image.\nS = 1 # number of selected compositions for each image",
"_____no_output_____"
]
],
[
[
"Let's consider the following list of linear transformations, similar to the ones used in the original paper:",
"_____no_output_____"
]
],
[
[
"MAGN = 4 # (int) Magnitude of augmentation applied. Ranges from [0, 10] with 10 being the max magnitude.\n# function of list of linear transformations\ndef transform_list(MAGN,p):\n return [transforms.RandomAutocontrast(p=p),\n transforms.ColorJitter(brightness=MAGN/30),\n transforms.ColorJitter(contrast=MAGN/30),\n transforms.RandomInvert(p=p),\n transforms.RandomRotation(degrees=MAGN*3),\n transforms.RandomAdjustSharpness(0.18*MAGN+0.1, p=p),\n transforms.RandomAffine(degrees=0, shear=MAGN/30),\n transforms.RandomSolarize(MAGN, p=p),\n transforms.RandomAffine(degrees=(0,0), translate=(MAGN/30,0),shear=(0,0)),\n transforms.RandomAffine(degrees=(0,0), translate=(0,MAGN/30),shear=(0,0)),\n ]",
"_____no_output_____"
]
],
[
[
"The following three code boxes were adapted from the tutorial on pytorch done in [class](https://github.com/SupaeroDataScience/deep-learning/blob/main/deep/Deep%20Learning.ipynb). ",
"_____no_output_____"
],
[
"In order to compare validation and training losses, we will calculate the validation losses and accuracy at each epoch.",
"_____no_output_____"
]
],
[
[
"def validation(model,criterion):\n correct_pred = 0\n total_pred = 0\n valid_loss = 0\n with torch.no_grad():\n for data in validloader:\n images, labels = data\n images = images.to(device)\n labels = labels.to(device)\n outputs = model(images)\n loss = criterion(outputs, labels)\n valid_loss += loss.item()\n\n # calculate predictions\n predictions=[]\n for i in range(outputs.shape[0]):\n ps = torch.exp(outputs[i])\n predictions.append(np.argmax(ps))\n # collect the correct predictions\n for label, prediction in zip(labels, predictions):\n if label == prediction:\n correct_pred += 1\n total_pred += 1\n accuracy = 100 * (correct_pred / total_pred)\n\n return valid_loss, accuracy",
"_____no_output_____"
],
[
"def plot_train_val(train, valid, title, label1 = 'Training', label2 = 'Validation'):\n fig, ax1 = plt.subplots()\n color = 'tab:red'\n ax1.set_ylabel(label1, color=color)\n ax1.plot(train, color=color)\n ax2 = ax1.twinx()\n color = 'tab:blue'\n ax2.set_ylabel(label2, color=color)\n ax2.plot(valid, color=color)\n fig.tight_layout()\n plt.title(title)",
"_____no_output_____"
]
],
[
[
"In order to avoid overfitting, we will implement early stopping.",
"_____no_output_____"
]
],
[
[
"class EarlyStopping:\n \n def __init__(self, patience=5, delta=0):\n self.patience = patience\n self.counter = 0\n self.best_score = None\n self.delta = delta\n self.early_stop = False\n\n def step(self, val_loss):\n score = -val_loss\n if self.best_score is None:\n self.best_score = score\n elif score < self.best_score + self.delta:\n self.counter += 1\n print('EarlyStopping counter: %d / %d' % (self.counter, self.patience))\n if self.counter >= self.patience:\n self.early_stop = True\n else:\n self.best_score = score\n self.counter = 0",
"_____no_output_____"
]
],
[
[
"It is time to implement the algorithm in the training loop!\n\n",
"_____no_output_____"
],
[
"> **In the final exercise, take the almost complete code of the training loop presented bellow (adapted from the [pytorch class](https://github.com/SupaeroDataScience/deep-learning/blob/main/deep/Deep%20Learning.ipynb)) and change it, so that the algorithm is implemented.**",
"_____no_output_____"
]
],
[
[
"# the load command only works on jupyter notebook\n# %load solutions/train.py\ndef train(model,criterion,optimizer, earlystopping=True,max_epochs=30,patience=2, augment=False):\n train_history = []\n valid_history = []\n accuracy_history = []\n estop = EarlyStopping(patience=patience)\n for epoch in range(max_epochs):\n train_loss = 0.0\n for i, data in enumerate(trainloader, 0):\n if augment:\n # generate transform list\n p = np.random.random() # probability of each transformation occurring\n transforms = transform_list(MAGN,p)\n # get the inputs; data is a list of [inputs, labels]\n xb,yb = data\n xb = xb.to(device)\n yb = yb.to(device)\n # generate the tensors 'C_images' and 'C_targets' <---- to complete\n \n # generated the augmented data = [inputs,labels] <---- to complete\n \n else:\n # get the inputs; data is a list of [inputs, labels]\n inputs,labels = data\n inputs = inputs.to(device)\n labels = labels.to(device)\n # zero the parameter gradients\n optimizer.zero_grad()\n # forward + backward + optimize\n outputs = model(inputs)\n loss = criterion(outputs, labels)\n loss.backward()\n optimizer.step()\n train_loss += loss.item()\n valid_loss, accuracy = validation(model,criterion)\n train_history.append(train_loss)\n valid_history.append(valid_loss)\n accuracy_history.append(accuracy)\n print('Epoch %02d: train loss %0.5f, validation loss %0.5f, accuracy %3.1f ' % (epoch, train_loss, valid_loss, accuracy))\n estop.step(valid_loss)\n if earlystopping and estop.early_stop:\n break\n return train_history, valid_history, accuracy_history",
"_____no_output_____"
],
[
"# the cat command works on google colab\n#%cat muAugment/solutions/train.py",
"_____no_output_____"
]
],
[
[
"We did it! Let's train our models: one without and one with augmented data.",
"_____no_output_____"
]
],
[
[
"max_epochs = 30\npatience = 5 #early stopping parameter\n\nprint(\"\\n Training for the original dataset...\\n\")\ntrain_history_original, valid_history_original, accuracy_history_original = train(model_original,criterion_original,optimizer_original,max_epochs=max_epochs,patience=patience)\nprint(\"\\n Training for the augmented dataset...\\n\")\ntrain_history, valid_history, accuracy_history = train(model,criterion,optimizer,max_epochs=max_epochs,patience=patience,augment=True)",
"\n Training for the original dataset...\n\nEpoch 00: train loss 53.41990, validation loss 5.85852, validation loss 86.0 \nEpoch 01: train loss 49.32130, validation loss 5.79052, validation loss 86.7 \nEpoch 02: train loss 47.43125, validation loss 5.62790, validation loss 87.0 \nEpoch 03: train loss 44.78354, validation loss 5.58822, validation loss 87.1 \nEpoch 04: train loss 43.11249, validation loss 5.10587, validation loss 88.8 \nEpoch 05: train loss 40.08070, validation loss 5.23783, validation loss 88.3 \nEarlyStopping counter: 1 / 3\nEpoch 06: train loss 38.33838, validation loss 5.10087, validation loss 88.8 \nEpoch 07: train loss 36.10283, validation loss 4.92744, validation loss 88.7 \nEpoch 08: train loss 33.87066, validation loss 5.32297, validation loss 88.6 \nEarlyStopping counter: 1 / 3\nEpoch 09: train loss 32.46742, validation loss 5.11203, validation loss 88.2 \nEarlyStopping counter: 2 / 3\nEpoch 10: train loss 31.42488, validation loss 5.15724, validation loss 88.1 \nEarlyStopping counter: 3 / 3\n\n Training for the augmented dataset...\n\nEpoch 00: train loss 255.33405, validation loss 14.73030, validation loss 66.7 \nEpoch 01: train loss 175.41405, validation loss 12.71785, validation loss 70.3 \nEpoch 02: train loss 152.23066, validation loss 10.88950, validation loss 74.7 \nEpoch 03: train loss 145.02098, validation loss 10.29128, validation loss 75.0 \nEpoch 04: train loss 140.47682, validation loss 10.38592, validation loss 74.2 \nEarlyStopping counter: 1 / 3\nEpoch 05: train loss 133.23461, validation loss 9.41392, validation loss 78.9 \nEpoch 06: train loss 128.67845, validation loss 9.13174, validation loss 77.9 \nEpoch 07: train loss 127.87093, validation loss 8.72783, validation loss 79.9 \nEpoch 08: train loss 119.60718, validation loss 9.12756, validation loss 77.1 \nEarlyStopping counter: 1 / 3\nEpoch 09: train loss 119.84167, validation loss 8.44272, validation loss 79.7 \nEpoch 10: train loss 117.55080, validation loss 8.24715, validation loss 80.2 \nEpoch 11: train loss 112.32505, validation loss 8.38380, validation loss 81.6 \nEarlyStopping counter: 1 / 3\nEpoch 12: train loss 106.44310, validation loss 7.59079, validation loss 83.3 \nEpoch 13: train loss 108.91942, validation loss 7.41854, validation loss 82.4 \nEpoch 14: train loss 105.01009, validation loss 7.18891, validation loss 83.5 \nEpoch 15: train loss 104.73178, validation loss 6.81569, validation loss 84.8 \nEpoch 16: train loss 105.86425, validation loss 6.89502, validation loss 83.2 \nEarlyStopping counter: 1 / 3\nEpoch 17: train loss 102.29675, validation loss 7.02991, validation loss 84.4 \nEarlyStopping counter: 2 / 3\nEpoch 18: train loss 102.68404, validation loss 7.42579, validation loss 83.7 \nEarlyStopping counter: 3 / 3\n"
]
],
[
[
"#### Plotting the Training and Validation Loss",
"_____no_output_____"
],
[
"Now that we trained both models, we can compare how the loss of training and validation evolves in both cases.",
"_____no_output_____"
]
],
[
[
"plot_train_val(train_history_original, valid_history_original,\"Original Data\")\nplot_train_val(train_history, valid_history,\"Augmented Data\")",
"_____no_output_____"
]
],
[
[
"Although it is not always verified, most times you can see that the training loss tends to decrease less while using the augmented data and, even, sometimes augment. This is consistent with the fact that the augmented data is more difficult to predict. However, because the model with augmented data does not excessively train the data points it already knows, the model also suffers less from overfitting.",
"_____no_output_____"
],
[
"We can also compare accuracy between models.",
"_____no_output_____"
]
],
[
[
"plot_train_val(accuracy_history, accuracy_history_original,\"Accuracy\",label1='Augmented',label2='Original')",
"_____no_output_____"
]
],
[
[
"#### Verifying models with Testing Dataset",
"_____no_output_____"
],
[
"Finally, let's check the results by applying our model to the test dataset.",
"_____no_output_____"
]
],
[
[
"# put model in evaluation mode\nmodel.eval()\n# moving model to cpu for inference \nmodel.to('cpu')\n\n# creating arrays to save predictions\ny_true = []\ny_pred = []\nimages_ = []\n# disable all gradients things\nwith torch.no_grad():\n for data in iter(testloader):\n images, labels = data\n outputs = model(images)\n for i in range(outputs.shape[0]):\n images_.append(images[i].unsqueeze(0))\n ps = torch.exp(outputs[i])\n y_pred.append(np.argmax(ps))\n y_true.append(labels[i].item())\n",
"_____no_output_____"
]
],
[
[
"Firstly, let's examine the confusion matrix.",
"_____no_output_____"
]
],
[
[
"from sklearn.metrics import ConfusionMatrixDisplay, confusion_matrix\n\nprint(\"Confusion matrix\")\ncm = confusion_matrix(y_true, y_pred)\n\ndisp = ConfusionMatrixDisplay(confusion_matrix=cm, display_labels=labels_text)\n\nfig, ax = plt.subplots(figsize=(10,10))\ndisp.plot(ax=ax)\nplt.show()",
"Confusion matrix\n"
]
],
[
[
"We can also plot some of the results of the test dataset.",
"_____no_output_____"
]
],
[
[
"# plotting the results\nfig = plt.figure(figsize=(n_images+5,4))\nfor i in range(n_images):\n ax = fig.add_subplot(2, n_images/2, i+1, xticks=[], yticks=[])\n ax.imshow(images_[i].resize_(1, 28, 28).numpy().squeeze())\n ax.set_title(\"{} ({})\".format(labels_text[y_pred[i]], labels_text[y_true[i]]),\n color=(\"green\" if y_pred[i]==y_true[i] else \"red\"))",
"_____no_output_____"
]
],
[
[
"Not bad! But could you spot a problem with the algorithm? **Here's a tip**: we were very conservative when choosing the parameters of our algorithm, namely the number of compositions generated **C**, number of compositions selected **S**, the number of linear transforms per composition **L** and the magnitude **M** of the chosen transforms.\n\n> **What do you think would happen if we increased those values?** If you have time, you can try to play with the values.",
"_____no_output_____"
],
[
"### Shortcomings of the Algorithm",
"_____no_output_____"
],
[
"It is possible that the transforms applied on an image are so severe that the image becomes indistinguishable, losing its target information. In such a case, we would end up feeding the model pure noise. However, pure noise yields a high loss when fed into the model, so using **MuAugment** selects for those unrecognizable images if they are created. There’s no simple solution for this issue other than to choose appropriate hyperparameters so as to reduce the generation of inscrutable images, so it’s a good idea to keep the number of transforms in a composition **C** under 4 and the magnitude of each transform **M** under 6.",
"_____no_output_____"
],
[
"As a heuristic, larger models and datasets require more regularization and would accordingly perform better with a greater magnitude **M**. This is because bigger models are more prone to overfit and lengthier datasets have a higher signal-to-noise ratio which should be reduced to an optimal point.",
"_____no_output_____"
]
]
] | [
"markdown",
"code",
"markdown",
"code",
"markdown",
"code",
"markdown",
"code",
"markdown",
"code",
"markdown",
"code",
"markdown",
"code",
"markdown",
"code",
"markdown",
"code",
"markdown",
"code",
"markdown",
"code",
"markdown",
"code",
"markdown",
"code",
"markdown",
"code",
"markdown",
"code",
"markdown",
"code",
"markdown",
"code",
"markdown",
"code",
"markdown",
"code",
"markdown",
"code",
"markdown",
"code",
"markdown",
"code",
"markdown"
] | [
[
"markdown",
"markdown",
"markdown",
"markdown",
"markdown",
"markdown"
],
[
"code"
],
[
"markdown"
],
[
"code",
"code",
"code",
"code",
"code"
],
[
"markdown",
"markdown",
"markdown",
"markdown",
"markdown",
"markdown",
"markdown",
"markdown",
"markdown",
"markdown",
"markdown",
"markdown",
"markdown",
"markdown",
"markdown",
"markdown",
"markdown",
"markdown",
"markdown"
],
[
"code"
],
[
"markdown"
],
[
"code"
],
[
"markdown"
],
[
"code"
],
[
"markdown",
"markdown"
],
[
"code"
],
[
"markdown"
],
[
"code"
],
[
"markdown",
"markdown"
],
[
"code"
],
[
"markdown"
],
[
"code"
],
[
"markdown",
"markdown",
"markdown",
"markdown",
"markdown",
"markdown",
"markdown"
],
[
"code",
"code"
],
[
"markdown",
"markdown"
],
[
"code",
"code"
],
[
"markdown",
"markdown",
"markdown"
],
[
"code"
],
[
"markdown"
],
[
"code"
],
[
"markdown",
"markdown"
],
[
"code",
"code"
],
[
"markdown"
],
[
"code"
],
[
"markdown",
"markdown"
],
[
"code",
"code"
],
[
"markdown"
],
[
"code"
],
[
"markdown",
"markdown"
],
[
"code"
],
[
"markdown",
"markdown"
],
[
"code"
],
[
"markdown",
"markdown"
],
[
"code"
],
[
"markdown"
],
[
"code"
],
[
"markdown"
],
[
"code"
],
[
"markdown",
"markdown",
"markdown",
"markdown"
]
] |
d08e6102d0ede2c191a4ee59663db1887408f537 | 142,030 | ipynb | Jupyter Notebook | day4-Fri/Pythia-Tune-AVO.ipynb | mi-stankai/mlhep2018 | 85b731de17265ba1f002cd9b522d67fd41d699f3 | [
"Apache-2.0"
] | null | null | null | day4-Fri/Pythia-Tune-AVO.ipynb | mi-stankai/mlhep2018 | 85b731de17265ba1f002cd9b522d67fd41d699f3 | [
"Apache-2.0"
] | null | null | null | day4-Fri/Pythia-Tune-AVO.ipynb | mi-stankai/mlhep2018 | 85b731de17265ba1f002cd9b522d67fd41d699f3 | [
"Apache-2.0"
] | null | null | null | 147.48702 | 40,844 | 0.848384 | [
[
[
"# Adversarial Variational Optimization: PYTHIA Tuning\n\nIn this notebook Adversarial Variational Optimization (https://arxiv.org/abs/1707.07113) is applied to tuning parameters of a simplistic detector.\n\n**Note: this notebook takes quite a long time to execute. It is recommended to run all cells at the beginning.**",
"_____no_output_____"
],
[
"**Please, don't interrupt the notebook while sampling from PythiaMill. Otherwise it might stuck at the next attempt to sample from it. IF this happens, please, restart the notebook.**",
"_____no_output_____"
]
],
[
[
"%env CUDA_DEVICE_ORDER=PCI_BUS_ID",
"env: CUDA_DEVICE_ORDER=PCI_BUS_ID\n"
],
[
"%matplotlib inline\nimport matplotlib.pyplot as plt\n\nfrom tqdm import tqdm_notebook as tqdm_notebook\n\nimport numpy as np",
"_____no_output_____"
],
[
"### don't forget about others!\n\nimport keras\n\nimport tensorflow as tf\ngpu_options = tf.GPUOptions(allow_growth=True, per_process_gpu_memory_fraction=0.2)\ntf_session = tf.InteractiveSession(config=tf.ConfigProto(gpu_options=gpu_options))\n\nkeras.backend.tensorflow_backend.set_session(tf_session)",
"Using TensorFlow backend.\n"
]
],
[
[
"## Generators\n\nPythia-mill is a python binding to Pythia generator that can run in multiple threads (processes).\nFor more details, please, visit https://github.com/maxim-borisyak/pythia-mill",
"_____no_output_____"
]
],
[
[
"import pythiamill as pm\n\nSEED=123",
"_____no_output_____"
]
],
[
[
"### Note about the change of problem\n\nThe reason the detector parameters (instead of Pythia parameters) are the target for the tune is a purely technical one: on each step AVO requires samples from multiples configurations of generator + detector. However, Pythia requires about half of a second to be reconfigured, which induces a tremendous overhead.\n\nBy contrast, this simplistic detector is designed to accept its parameters as function arguments (effectively neglecting any overhead).\n\n\nThe detector emulates a $32 \\times 32$ spherical uniform grid in `pseudorapidity` ($\\eta$)-`angle in traverse plane` ($\\phi$) covering $(\\eta, \\phi) \\in [0, 5] \\times [0, 2 \\pi]$.\n\nThe detector is parametrized by offset in $z$-axis relative to the beam crossing point. Zero offset means that center of the sphere coincides with the collision point.",
"_____no_output_____"
]
],
[
[
"### ground truth offset, unknown in the real world problems.\nTRUE_OFFSET=1",
"_____no_output_____"
],
[
"options = [\n ### telling pythia to be quiet.\n 'Print:quiet = on',\n 'Init:showProcesses = off',\n 'Init:showMultipartonInteractions = off',\n 'Init:showChangedSettings = off',\n 'Init:showChangedParticleData = off',\n 'Next:numberCount=0',\n 'Next:numberShowInfo=0',\n 'Next:numberShowEvent=0',\n 'Stat:showProcessLevel=off',\n 'Stat:showErrors=off',\n \n ### seeting default parameters to Monash values\n ### all options are taken from https://arxiv.org/abs/1610.08328\n \"Tune:ee = 7\",\n \"Beams:idA = 11\",\n \"Beams:idB = -11\",\n \"Beams:eCM = 91.2\",\n \"WeakSingleBoson:ffbar2gmZ = on\",\n \"23:onMode = off\",\n \"23:onIfMatch = 1 -1\",\n \"23:onIfMatch = 2 -2\",\n \"23:onIfMatch = 3 -3\",\n \"23:onIfMatch = 4 -4\",\n \"23:onIfMatch = 5 -5\",\n]",
"_____no_output_____"
],
[
"### defining the detector\ndetector = pm.utils.SphericalTracker(\n ### with this option detector measures total energy\n ### of the particles traversing each pixel.\n is_binary=False,\n \n ### detector covers [0, 5] pseudo-rapidity range\n max_pseudorapidity=5.0,\n pseudorapidity_steps=32, phi_steps=32,\n ### 1 layer with radius 10 mm.\n n_layers=1, R_min=10.0, R_max=10.0,\n)\n \nmill = pm.ParametrizedPythiaMill(\n detector, options,\n ### please, don't use number of workers higher than 4.\n batch_size=8, n_workers=4,\n seed=SEED\n)",
"_____no_output_____"
],
[
"def get_data(mill, detector_configurations, show_progress=False):\n \"\"\"\n Utilitary function to obtain data for a particular set of configurations.\n \n :param mill: instance of Pythia Mill to sample from.\n : param detector configuration: - list of configurations.\n each configuration should be an array of detector parameters.\n : param show_progress: if True shows progress via `tqdm` package. \n \n :return:\n - parameters: array of shape `<number of samples> x <parameters dim>`, parameters for each sample;\n - samples: array of shape `<number of samples> x 1 x 32 x 32`, sampled events.\n \"\"\"\n try:\n ### sending requests to the queue\n for args in detector_configurations:\n mill.request(*args)\n\n ### retrieving results\n data = [\n mill.retrieve()\n for _ in (\n (lambda x: tqdm_notebook(x, postfix='data gen', leave=False))\n if show_progress else\n (lambda x: x)\n )(range(len(detector_configurations)))\n ]\n\n samples = np.vstack([ samples for params, samples in data ])\n params = np.vstack([ np.array([params] * samples.shape[0], dtype='float32') for params, samples in data ])\n\n return params, samples.reshape(-1, 32, 32, 1)\n finally:\n while mill.n_requests > 0:\n mill.retrieve()",
"_____no_output_____"
],
[
"### Generating training samples with ground truth parameters.\n### For a real-world problem these arrays would correspond to real data.\n_, X_true_train = get_data(mill, detector_configurations=[(TRUE_OFFSET, )] * 2 ** 12, show_progress=True)\n_, X_true_val = get_data(mill, detector_configurations=[(TRUE_OFFSET, )] * 2 ** 12, show_progress=True)",
"_____no_output_____"
],
[
"print(X_true_train.shape)\nprint(X_true_val.shape)",
"(32768, 32, 32, 1)\n(32768, 32, 32, 1)\n"
]
],
[
[
"### Taking a look at events",
"_____no_output_____"
]
],
[
[
"n = 5\nplt.subplots(nrows=n, ncols=n, figsize=(3 * n, 3 * n))\n\nmax_energy = np.max(X_true_train[:n * n])\n\nfor i in range(n):\n for j in range(n):\n k = i * n + j\n plt.subplot(n, n, k + 1)\n plt.imshow(X_true_train[k, :, :, 0], vmin=0, vmax=max_energy)\n\nplt.show()",
"_____no_output_____"
]
],
[
[
"### Aggregated events",
"_____no_output_____"
]
],
[
[
"plt.figure(figsize=(6, 6))\nplt.imshow(np.sum(X_true_train, axis=(0, 3)), vmin=0)\nplt.show()",
"_____no_output_____"
]
],
[
[
"## Discriminator",
"_____no_output_____"
]
],
[
[
"from keras.models import Model\nfrom keras.layers import Input, Conv2D, MaxPool2D, Dense, Flatten, GlobalMaxPool2D\nfrom keras.activations import softplus, sigmoid, relu\n\nfrom keras.utils.vis_utils import model_to_dot",
"_____no_output_____"
]
],
[
[
"### Building conv net",
"_____no_output_____"
]
],
[
[
"inputs = Input(shape=(32, 32, 1))\n\nactivation = lambda x: relu(x, 0.05)\n\nnet = Conv2D(8, kernel_size=(3, 3), padding='same', activation=activation)(inputs)\nnet = MaxPool2D(pool_size=(2, 2))(net)\n\nnet = Conv2D(12, kernel_size=(3, 3), padding='same', activation=activation)(net)\nnet = MaxPool2D(pool_size=(2, 2))(net)\n# net = GlobalMaxPool2D()(net)\n\n\nnet = Conv2D(16, kernel_size=(3, 3), padding='same', activation=activation)(net)\nnet = MaxPool2D(pool_size=(2, 2))(net)\n\nnet = Conv2D(24, kernel_size=(3, 3), padding='same', activation=activation)(net)\nnet = MaxPool2D(pool_size=(2, 2))(net)\n\nnet = Flatten()(net)\npredictions = Dense(1, activation=sigmoid)(net)\n\ndiscriminator = Model(inputs=inputs, outputs=predictions)\n\ndiscriminator.compile(optimizer='adam', loss='binary_crossentropy')",
"_____no_output_____"
],
[
"from IPython import display\nfrom IPython.display import SVG\n\nSVG(model_to_dot(discriminator, show_shapes=True).create(prog='dot', format='svg'))",
"_____no_output_____"
]
],
[
[
"In Adversarial Variational Optimization, instead of searching for a single value of detector parameters, a parametrized distribution is introduced (with parameters $\\psi$):\n\n$$\\mathcal{L}(\\psi) = \\mathrm{JS}(X_\\psi, X_\\mathrm{data})$$\nwhere:\n- $X_\\psi \\sim \\mathrm{detector}(\\theta), \\theta \\sim P_\\psi$;\n- $X_\\mathrm{data} \\sim \\mathrm{reality}$.\n\nNote that $\\mathcal{L}(\\psi)$ is a vaiational bound on adversarial loss:\n\n$$\\mathcal{L}(\\psi) \\geq \\min_\\theta \\mathcal{L}_\\mathrm{adv}(\\theta) = \\mathrm{JS}(X_\\theta, X_\\mathrm{data})$$\n\nIn this example, detector parameters consist of a signle `offset` parameter. For simplicity normal distibution is used:\n\n$$\\mathrm{offset} \\sim \\mathcal{N}(\\mu, \\sigma)$$\n\n\nIn order to avoid introducing constraints $\\sigma \\geq 0$, an auxiliary *free variable* $\\sigma'$ is introduced (denoted as `detector_params_sigma_raw` in the code):\n$$\\sigma = \\log(1 + \\exp(\\sigma'))$$\n\nNote that if there exists configuration of detector perfectly matching real data, then minimum of variational bound is achieved when the `offset` distribution collapses into delta function with the center at minumum of adversarial loss.\nOtherwise, a mixture of detector configuations might be a solution (unlike convetional variational optimization).",
"_____no_output_____"
]
],
[
[
"X = tf.placeholder(dtype='float32', shape=(None, 32, 32, 1))\n\nproba = discriminator(X)[:, 0]\n\ndetector_params = tf.placeholder(dtype='float32', shape=(None, 1))\n\ndetector_params_mean = tf.Variable(\n initial_value=np.array([0.0], dtype='float32'),\n dtype='float32'\n)\n\ndetector_params_sigma_raw = tf.Variable(\n initial_value=np.array([2.0], dtype='float32'),\n dtype='float32'\n)\n\ndetector_params_sigma = tf.nn.softplus(detector_params_sigma_raw)\n\nneg_log_prob = tf.reduce_sum(\n tf.log(detector_params_sigma)\n) + tf.reduce_sum(\n 0.5 * (detector_params - detector_params_mean[None, :]) ** 2 / detector_params_sigma[None, :] ** 2\n , axis=1\n)\n\ndetector_params_loss = tf.reduce_mean(neg_log_prob * proba)",
"_____no_output_____"
],
[
"get_distribution_params = lambda : tf_session.run([detector_params_mean, detector_params_sigma])",
"_____no_output_____"
],
[
"n = tf.placeholder(dtype='int64', shape=())\nparams_sample = tf.random_normal(\n mean=detector_params_mean,\n stddev=detector_params_sigma,\n shape=(n, 1),\n dtype='float32'\n)",
"_____no_output_____"
],
[
"distribution_opt = tf.train.AdamOptimizer(learning_rate=0.02).minimize(\n detector_params_loss, var_list=[detector_params_mean, detector_params_sigma_raw]\n)",
"_____no_output_____"
],
[
"tf_session.run(tf.global_variables_initializer())",
"_____no_output_____"
],
[
"def train_discriminator(n_samples=2 ** 16, n_epoches=16, plot=False):\n sample_of_detector_params = tf_session.run(params_sample, { n : n_samples // 8 })\n \n _, X_gen_train = get_data(\n mill,\n detector_configurations=sample_of_detector_params,\n show_progress=True\n )\n \n X_train = np.vstack([ X_gen_train, X_true_train ])\n y_train = np.hstack([ np.zeros(X_gen_train.shape[0]), np.ones(X_true_train.shape[0]) ]).astype('float32')\n \n history = discriminator.fit(x=X_train, y=y_train, batch_size=32, epochs=n_epoches, verbose=0)\n \n if plot:\n plt.figure(figsize=(8, 4))\n plt.plot(history.history['loss'], label='train loss')\n plt.legend()\n plt.show()",
"_____no_output_____"
],
[
"def train_generator():\n sample_of_detector_params = tf_session.run(params_sample, { n : 2 ** 8 })\n params_train, X_gen_train = get_data(mill, detector_configurations=sample_of_detector_params)\n \n tf_session.run(\n distribution_opt,\n feed_dict={\n X : X_gen_train,\n detector_params : params_train\n }\n )",
"_____no_output_____"
]
],
[
[
"## Pretraining",
"_____no_output_____"
],
[
"AVO makes small changes in parameter distribution. When starting with the optimal discriminator from the previous iterations, adjusting discriminator to these changes should require relatively few optimization steps.\n\nHowever, the initial discriminator state (which is just random weights), most probably, does not correspond to any optimal discriminator. Therefore, we pretrain discriminator in order to ensure that only a few epoches needed on each iteration to achieve an optimal discriminator.",
"_____no_output_____"
]
],
[
[
"%%time\n\ntrain_discriminator(n_samples=2**16, n_epoches=4, plot=True)",
"_____no_output_____"
]
],
[
[
"## Variational optimization",
"_____no_output_____"
]
],
[
[
"from IPython import display\n\nn_iterations = 256\n\ngenerator_mean_history = np.ndarray(shape=(n_iterations, ))\ngenerator_sigma_history = np.ndarray(shape=(n_iterations, ))\n\nfor i in range(n_iterations):\n train_discriminator(n_samples=2**12, n_epoches=1)\n train_generator()\n \n m, s = get_distribution_params()\n generator_mean_history[i] = np.float32(m[0])\n generator_sigma_history[i] = np.float32(s[0])\n \n display.clear_output(wait=True)\n \n plt.figure(figsize=(18, 9))\n plt.plot(generator_mean_history[:i + 1], color='blue', label='mean ($\\\\mu$)')\n\n plt.fill_between(\n np.arange(i + 1),\n generator_mean_history[:i + 1] - generator_sigma_history[:i + 1],\n generator_mean_history[:i + 1] + generator_sigma_history[:i + 1],\n color='blue',\n label='sigma ($\\\\sigma$)',\n alpha=0.2\n )\n \n plt.plot([0, n_iterations - 1], [TRUE_OFFSET, TRUE_OFFSET], '--', color='black', alpha=0.5, label='ground truth')\n plt.ylim([-2, 4])\n plt.legend(loc='upper left', fontsize=18)\n plt.legend(fontsize=18)\n plt.xlabel('AVO step', fontsize=16)\n plt.ylabel('detector offset', fontsize=16)\n plt.show()",
"_____no_output_____"
]
]
] | [
"markdown",
"code",
"markdown",
"code",
"markdown",
"code",
"markdown",
"code",
"markdown",
"code",
"markdown",
"code",
"markdown",
"code",
"markdown",
"code",
"markdown",
"code",
"markdown",
"code"
] | [
[
"markdown",
"markdown"
],
[
"code",
"code",
"code"
],
[
"markdown"
],
[
"code"
],
[
"markdown"
],
[
"code",
"code",
"code",
"code",
"code",
"code"
],
[
"markdown"
],
[
"code"
],
[
"markdown"
],
[
"code"
],
[
"markdown"
],
[
"code"
],
[
"markdown"
],
[
"code",
"code"
],
[
"markdown"
],
[
"code",
"code",
"code",
"code",
"code",
"code",
"code"
],
[
"markdown",
"markdown"
],
[
"code"
],
[
"markdown"
],
[
"code"
]
] |
d08e611c65da719261e944a4dc4067d4f996056a | 296,389 | ipynb | Jupyter Notebook | 16DataVisualizationWithMatplotlib.ipynb | MBadriNarayanan/NaturalLanguageProcessing | 34ef51f66691a8fc4792b0cb919eb9781c8bb804 | [
"MIT"
] | null | null | null | 16DataVisualizationWithMatplotlib.ipynb | MBadriNarayanan/NaturalLanguageProcessing | 34ef51f66691a8fc4792b0cb919eb9781c8bb804 | [
"MIT"
] | null | null | null | 16DataVisualizationWithMatplotlib.ipynb | MBadriNarayanan/NaturalLanguageProcessing | 34ef51f66691a8fc4792b0cb919eb9781c8bb804 | [
"MIT"
] | 1 | 2021-06-07T08:06:47.000Z | 2021-06-07T08:06:47.000Z | 199.992578 | 24,562 | 0.898141 | [
[
[
"# 1) Matplotlib Part 1",
"_____no_output_____"
],
[
"## 1) Functional method",
"_____no_output_____"
]
],
[
[
"import numpy as np\nimport matplotlib.pyplot as plt\nfrom numpy.random import randint",
"_____no_output_____"
],
[
"x = np.linspace(0,10,20)",
"_____no_output_____"
],
[
"x",
"_____no_output_____"
],
[
"y = randint(0,50,20)",
"_____no_output_____"
],
[
"y",
"_____no_output_____"
],
[
"y = np.sort(y)",
"_____no_output_____"
],
[
"y",
"_____no_output_____"
],
[
"plt.plot(x,y, color='m', linestyle='--', marker='*', markersize=10, lw=1.5)\nplt.xlabel('X axis')\nplt.ylabel('Y axis')\nplt.title('X vs Y axis')\nplt.show()",
"_____no_output_____"
],
[
"# multiple plots on same canvas",
"_____no_output_____"
],
[
"plt.subplot(1,2,1)\nplt.plot(x,y,color='r')\nplt.subplot(1,2,2)\nplt.plot(x,y,color='m')",
"_____no_output_____"
]
],
[
[
"## 2) Object Oriented Method",
"_____no_output_____"
]
],
[
[
"import numpy as np\nimport matplotlib.pyplot as plt\nfrom numpy.random import randint",
"_____no_output_____"
],
[
"x = np.linspace(0,10,20)\ny = randint(1, 50, 20)\ny = np.sort(y)",
"_____no_output_____"
],
[
"x",
"_____no_output_____"
],
[
"y",
"_____no_output_____"
],
[
"fig = plt.figure()\naxes = fig.add_axes([0.1,0.1,1,1])\naxes.plot(x,y)\naxes.set_xlabel('X axis')\naxes.set_ylabel('Y axis')\naxes.set_title('X vs Y axis')",
"_____no_output_____"
],
[
"# 2 sets of figures to 1 canvas",
"_____no_output_____"
],
[
"fig = plt.figure()\nax1 = fig.add_axes([0.1,0.1,0.8,0.8])\nax2 = fig.add_axes([0.2,0.5,0.4,0.3])\n\nax1.plot(x,y,color='r')\nax1.set_xlabel('X axis')\nax1.set_ylabel('Y axis')\nax1.set_title('Plot 1')\n\nax2.plot(x,y,color='m')\nax2.set_xlabel('X axis')\nax2.set_ylabel('Y axis')\nax2.set_title('Plot 2')",
"_____no_output_____"
]
],
[
[
"# 2) Matplotlib Part 2",
"_____no_output_____"
],
[
"## 1) Subplots method",
"_____no_output_____"
]
],
[
[
"import numpy as np\nimport matplotlib.pyplot as plt\nfrom numpy.random import randint",
"_____no_output_____"
],
[
"x = np.linspace(0,10,20)\ny = randint(1, 50, 20)\ny = np.sort(y)",
"_____no_output_____"
],
[
"x",
"_____no_output_____"
],
[
"y",
"_____no_output_____"
],
[
"fig,axes = plt.subplots()\naxes.plot(x,y)",
"_____no_output_____"
],
[
"fig,axes = plt.subplots(nrows=2,ncols=3)\nplt.tight_layout()",
"_____no_output_____"
],
[
"axes",
"_____no_output_____"
],
[
"fig,axes = plt.subplots(nrows=1,ncols=2)\nfor current_ax in axes:\n current_ax.plot(x,y)",
"_____no_output_____"
],
[
"fig,axes = plt.subplots(nrows=1,ncols=2)\naxes[0].plot(x,y)\naxes[1].plot(x,y)\naxes[0].set_title('Plot 1')\naxes[1].set_title('Plot 2')",
"_____no_output_____"
]
],
[
[
"## 2) Figure size, Aspect ratio and DPI",
"_____no_output_____"
]
],
[
[
"import numpy as np\nimport matplotlib.pyplot as plt\nfrom numpy.random import randint",
"_____no_output_____"
],
[
"x = np.linspace(0,10,20)\ny = randint(1, 50, 20)\ny = np.sort(y)",
"_____no_output_____"
],
[
"fig = plt.figure(figsize=(3,2),dpi=100)\nax = fig.add_axes([0,0,1,1])\nax.plot(x,y)",
"_____no_output_____"
],
[
"fig,axes = plt.subplots(nrows=1,ncols=2,figsize=(7,2))\naxes[0].plot(x,y)\naxes[1].plot(x,y)",
"_____no_output_____"
],
[
"fig",
"_____no_output_____"
],
[
"fig.savefig('my_pic.png',dpi=100)",
"_____no_output_____"
],
[
"fig = plt.figure()\nax = fig.add_axes([0,0,1,1])\nax.plot(x,y)\nax.set_xlabel('X axis')\nax.set_ylabel('Y axis')\nax.set_title('X vs Y')",
"_____no_output_____"
],
[
"# legends\nfig = plt.figure()\nax = fig.add_axes([0,0,1,1])\nax.plot(x,x**2,label='X vs X square')\nax.plot(x,x**3,label='X vs X cube')\nax.legend(loc=0)",
"_____no_output_____"
]
],
[
[
"# 3) Matplotlib Part 3",
"_____no_output_____"
]
],
[
[
"import numpy as np\nimport matplotlib.pyplot as plt\nfrom numpy.random import randint",
"_____no_output_____"
],
[
"x = np.linspace(0,10,20)\ny = randint(1, 50, 20)\ny = np.sort(y)",
"_____no_output_____"
],
[
"fig = plt.figure()\nax = fig.add_axes([0,0,1,1])\nax.plot(x,y,color='g',linewidth=3,ls='--',alpha=0.8,marker='o',markersize=10,markerfacecolor='yellow')",
"_____no_output_____"
],
[
"fig = plt.figure()\nax = fig.add_axes([0,0,1,1])\nax.plot(x,y,color='r',linewidth=3)\nax.set_xlim([0,1])\nax.set_ylim([0,10])",
"_____no_output_____"
]
],
[
[
"# 4) Different Plots",
"_____no_output_____"
],
[
"## 1) Scatter Plots",
"_____no_output_____"
]
],
[
[
"import matplotlib.pyplot as plt",
"_____no_output_____"
],
[
"y_views=[534,690,258,402,724,689,352]\nf_views=[123,342,700,305,406,648,325]\nt_views=[202,209,176,415,824,389,550]\ndays=[1,2,3,4,5,6,7]",
"_____no_output_____"
],
[
"plt.scatter(days,y_views,label='Youtube Views',marker='o')\nplt.scatter(days,f_views,label='Facebook Views',marker='o')\nplt.scatter(days,t_views,label='Twitter Views',marker='o')\nplt.xlabel('Days')\nplt.ylabel('Views')\nplt.title('Social Media Views')\nplt.grid(color='r',linestyle='--')\nplt.legend()",
"_____no_output_____"
]
],
[
[
"## 2) Bar plot",
"_____no_output_____"
]
],
[
[
"plt.bar(days,y_views,label='Youtube views')\nplt.bar(days,f_views,label='Facebook views')\nplt.xlabel('Days')\nplt.ylabel('Views')\nplt.title('Social Media Views')\nplt.legend()",
"_____no_output_____"
]
],
[
[
"## 3) Histogram",
"_____no_output_____"
]
],
[
[
"points=[22,55,62,45,21,22,99,34,42,4,102,110,27,48,99,84]\nbins=[0,20,40,60,80,100,120]",
"_____no_output_____"
],
[
"plt.hist(points,bins)\nplt.xlabel('Bins')\nplt.ylabel('Frequency')\nplt.title('Bins vs Frequency')\nplt.show()",
"_____no_output_____"
]
],
[
[
"## 4) Pie chart",
"_____no_output_____"
]
],
[
[
"labels_1=['Facebook','Instagram','Youtube','linkedin']\nviews=[300,350,400,450]\nexplode_1=[0,0,0,0.2]",
"_____no_output_____"
],
[
"plt.pie(views,labels=labels_1,autopct='%1.1f%%',explode=explode_1,shadow=True)\nplt.show()",
"_____no_output_____"
]
]
] | [
"markdown",
"code",
"markdown",
"code",
"markdown",
"code",
"markdown",
"code",
"markdown",
"code",
"markdown",
"code",
"markdown",
"code",
"markdown",
"code",
"markdown",
"code"
] | [
[
"markdown",
"markdown"
],
[
"code",
"code",
"code",
"code",
"code",
"code",
"code",
"code",
"code",
"code"
],
[
"markdown"
],
[
"code",
"code",
"code",
"code",
"code",
"code",
"code"
],
[
"markdown",
"markdown"
],
[
"code",
"code",
"code",
"code",
"code",
"code",
"code",
"code",
"code"
],
[
"markdown"
],
[
"code",
"code",
"code",
"code",
"code",
"code",
"code",
"code"
],
[
"markdown"
],
[
"code",
"code",
"code",
"code"
],
[
"markdown",
"markdown"
],
[
"code",
"code",
"code"
],
[
"markdown"
],
[
"code"
],
[
"markdown"
],
[
"code",
"code"
],
[
"markdown"
],
[
"code",
"code"
]
] |
d08e672e3bfb1a81b8b501af24af373af64a0564 | 235,391 | ipynb | Jupyter Notebook | notebooks/.ipynb_checkpoints/gpflow3d_nonstat-checkpoint.ipynb | nawalgao/AdaptiveGPFlow | 0661441a57fde284eff6a907015a07cd36a1164c | [
"MIT"
] | null | null | null | notebooks/.ipynb_checkpoints/gpflow3d_nonstat-checkpoint.ipynb | nawalgao/AdaptiveGPFlow | 0661441a57fde284eff6a907015a07cd36a1164c | [
"MIT"
] | null | null | null | notebooks/.ipynb_checkpoints/gpflow3d_nonstat-checkpoint.ipynb | nawalgao/AdaptiveGPFlow | 0661441a57fde284eff6a907015a07cd36a1164c | [
"MIT"
] | null | null | null | 404.45189 | 196,480 | 0.914725 | [
[
[
"import gpflow\nimport numpy as np\nimport matplotlib.pyplot as plt\n%matplotlib inline\nimport sys\nsys.path.append('../')\nfrom GPHetero import hetero_kernels, hetero_likelihoods, hetero_gpmc\nfrom pyDOE import *\nimport os\nfrom scipy.stats import norm",
"_____no_output_____"
],
[
"class Ex5Func(object):\n\n def __init__(self, sigma=lambda x: 0.5):\n self.sigma = sigma\n\n def __call__(self, x):\n \"\"\"\n Dette et. al. function.\n Dette, Holger, and Andrey Pepelyshev. \"Generalized Latin hypercube design for computer experiments.\" Technometrics 52, no. 4 (2010): 421-429.\n\n \"\"\"\n y = 4 * ((x[0] - 2 + 8 * x[1] - 8 * (x[1] ** 2)) ** 2) + (3 - 4 * x[1]) ** 2 + 16 * np.sqrt(x[2] + 1) * ((2 * x[2] - 1)**2)\n return (y - 50) / 50.",
"_____no_output_____"
],
[
"dim = 3\nn = 20\nnoise=0\nsigma = eval('lambda x: ' + str(noise))\n\nobjective = Ex5Func(sigma=sigma)\nX = lhs(dim, n , criterion='center')\nXnorm = (X - 0.5) /0.5\nY = np.array([objective(x) for x in X])[:, None]",
"_____no_output_____"
],
[
"#build the model\nk = gpflow.kernels.RBF(input_dim=1)\nk.lengthscales.prior = gpflow.priors.Gamma(1, 1)\n# from copy import copy\n# l = copy(k)\nnoisekern = gpflow.kernels.RBF(input_dim=1)\nnonstat = hetero_kernels.NonStationaryLengthscaleRBF()\nmean_func = gpflow.mean_functions.Constant(1)\nm = hetero_gpmc.GPMCAdaptiveLengthscaleMultDim(Xnorm, Y, k, nonstat, mean_func)",
"_____no_output_____"
],
[
"for i in xrange(dim):\n print i\n m.kerns[\"ell\" + str(i)].lengthscales.prior = gpflow.priors.Gamma(1., 1.)\n m.kerns[\"ell\" + str(i)].variance.prior = gpflow.priors.Gamma(1., 1.)\n #m.mean_funcs[\"ell\" + str(i)].c = 3.\n #m.mean_funcs[\"ell\" + str(i)].c.fixed = True \n m.mean_funcs[\"ell\" + str(i)].c.prior = gpflow.priors.Exponential(1./4)\nm.nonstat.signal_variance.prior = gpflow.priors.Gamma(1., 1.)\n# m.nonstat.signal_variance.fixed = True\nm.likelihood.variance = 1e-6\nm.likelihood.variance.fixed = True",
"0\n1\n2\n"
],
[
"m.optimize(maxiter=1500) # start near MAP",
"_____no_output_____"
],
[
"m",
"_____no_output_____"
],
[
"mcmc_samples = 1000\nnum_samp_gp = 1",
"_____no_output_____"
],
[
"samples = m.sample(mcmc_samples, verbose=True, epsilon=0.00005, thin = 5, burn = 500, Lmax = 20)",
"burn-in sampling started\nIteration: 100 \t Acc Rate: 97.0 %\nIteration: 200 \t Acc Rate: 97.0 %\nIteration: 300 \t Acc Rate: 99.0 %\nIteration: 400 \t Acc Rate: 99.0 %\nIteration: 500 \t Acc Rate: 98.0 %\nburn-in sampling ended\nIteration: 100 \t Acc Rate: 97.0 %\nIteration: 200 \t Acc Rate: 100.0 %\nIteration: 300 \t Acc Rate: 98.0 %\nIteration: 400 \t Acc Rate: 99.0 %\nIteration: 500 \t Acc Rate: 98.0 %\nIteration: 600 \t Acc Rate: 99.0 %\nIteration: 700 \t Acc Rate: 100.0 %\nIteration: 800 \t Acc Rate: 100.0 %\nIteration: 900 \t Acc Rate: 99.0 %\nIteration: 1000 \t Acc Rate: 99.0 %\nIteration: 1100 \t Acc Rate: 98.0 %\nIteration: 1200 \t Acc Rate: 99.0 %\nIteration: 1300 \t Acc Rate: 98.0 %\nIteration: 1400 \t Acc Rate: 100.0 %\nIteration: 1500 \t Acc Rate: 100.0 %\nIteration: 1600 \t Acc Rate: 100.0 %\nIteration: 1700 \t Acc Rate: 100.0 %\nIteration: 1800 \t Acc Rate: 96.0 %\nIteration: 1900 \t Acc Rate: 98.0 %\nIteration: 2000 \t Acc Rate: 99.0 %\nIteration: 2100 \t Acc Rate: 98.0 %\nIteration: 2200 \t Acc Rate: 100.0 %\nIteration: 2300 \t Acc Rate: 99.0 %\nIteration: 2400 \t Acc Rate: 99.0 %\nIteration: 2500 \t Acc Rate: 96.0 %\nIteration: 2600 \t Acc Rate: 100.0 %\nIteration: 2700 \t Acc Rate: 100.0 %\nIteration: 2800 \t Acc Rate: 99.0 %\nIteration: 2900 \t Acc Rate: 100.0 %\nIteration: 3000 \t Acc Rate: 97.0 %\nIteration: 3100 \t Acc Rate: 97.0 %\nIteration: 3200 \t Acc Rate: 98.0 %\nIteration: 3300 \t Acc Rate: 100.0 %\nIteration: 3400 \t Acc Rate: 99.0 %\nIteration: 3500 \t Acc Rate: 99.0 %\nIteration: 3600 \t Acc Rate: 99.0 %\nIteration: 3700 \t Acc Rate: 98.0 %\nIteration: 3800 \t Acc Rate: 100.0 %\nIteration: 3900 \t Acc Rate: 96.0 %\nIteration: 4000 \t Acc Rate: 100.0 %\nIteration: 4100 \t Acc Rate: 98.0 %\nIteration: 4200 \t Acc Rate: 99.0 %\nIteration: 4300 \t Acc Rate: 100.0 %\nIteration: 4400 \t Acc Rate: 99.0 %\nIteration: 4500 \t Acc Rate: 100.0 %\nIteration: 4600 \t Acc Rate: 99.0 %\nIteration: 4700 \t Acc Rate: 98.0 %\nIteration: 4800 \t Acc Rate: 100.0 %\nIteration: 4900 \t Acc Rate: 99.0 %\nIteration: 5000 \t Acc Rate: 99.0 %\n"
],
[
"m",
"_____no_output_____"
],
[
"X_test = lhs(dim, n , criterion='center')\nX_test_norm = (X_test - 0.5) /0.5\nY_test = np.array([objective(x) for x in X])[:, None]",
"_____no_output_____"
],
[
"samples.shape",
"_____no_output_____"
],
[
"plt.figure(figsize=(16, 4))\nplt.plot(samples[:,10:80])",
"_____no_output_____"
],
[
"X_test = lhs(dim, 100 , criterion='center')\nX_test_norm = (X_test - 0.5) /0.5\nY_test = np.array([objective(x) for x in X_test])[:, None]",
"_____no_output_____"
],
[
"sample_df = m.get_samples_df(samples)\nmean_f_mat = np.zeros(shape=(sample_df.shape[0], X_test_norm.shape[0]))\nvar_f_mat = np.zeros(shape=(sample_df.shape[0], X_test_norm.shape[0]))",
"_____no_output_____"
],
[
"for i, s in sample_df.iterrows():\n m.set_parameter_dict(s)\n mean_f, var_f = m.predict(X_test_norm)\n mean_f_mat[i, :] = mean_f[:,0]\n var_f_mat[i, :] = np.diag(var_f)",
"_____no_output_____"
],
[
"plt.figure(figsize=(12,8))\nplt.scatter(mean_f_mat[5,:], Y_test)",
"_____no_output_____"
]
]
] | [
"code"
] | [
[
"code",
"code",
"code",
"code",
"code",
"code",
"code",
"code",
"code",
"code",
"code",
"code",
"code",
"code",
"code",
"code",
"code"
]
] |
d08e685a3a0fd736efcd6f5be634184038d982fe | 708,668 | ipynb | Jupyter Notebook | SEIRD_ControlModel.ipynb | MadhabBarman/Epidemic-Control-Model | dcfc575e6b616a04b6160e897900ecf95f69a673 | [
"MIT"
] | null | null | null | SEIRD_ControlModel.ipynb | MadhabBarman/Epidemic-Control-Model | dcfc575e6b616a04b6160e897900ecf95f69a673 | [
"MIT"
] | null | null | null | SEIRD_ControlModel.ipynb | MadhabBarman/Epidemic-Control-Model | dcfc575e6b616a04b6160e897900ecf95f69a673 | [
"MIT"
] | null | null | null | 1,274.582734 | 195,826 | 0.94971 | [
[
[
"<a href=\"https://colab.research.google.com/github/MadhabBarman/Epidemic-Control-Model/blob/master/SEIRD_ControlModel.ipynb\" target=\"_parent\"><img src=\"https://colab.research.google.com/assets/colab-badge.svg\" alt=\"Open In Colab\"/></a>",
"_____no_output_____"
]
],
[
[
"!git clone https://github.com/MadhabBarman/Epidemic-Control-Model.git",
"_____no_output_____"
],
[
"cd Epidemic-Control-Model/",
"_____no_output_____"
],
[
"%matplotlib inline\nimport numpy as np\nimport matplotlib.pyplot as plt\nimport pandas as pd\nfrom scipy.integrate import odeint\nfrom scipy.io import savemat, loadmat\nimport numpy.linalg as la\nfrom matplotlib.lines import Line2D\n\nM = 16\nmy_data = np.genfromtxt('data/age_structures/India-2019.csv', delimiter=',', skip_header=1)\nReal_data = np.genfromtxt('data/covid-cases/case_time_series.csv', delimiter=',', skip_header=1)\naM, aF = my_data[:, 1], my_data[:, 2]\n\nNi=aM+aF; Ni=Ni[0:M]; N=np.sum(Ni)\n\n\n# contact matrices\nmy_data = pd.read_excel('data/contact_matrices_152_countries/MUestimates_home_1.xlsx', sheet_name='India',index_col=None)\nCH = np.array(my_data)\n\nmy_data = pd.read_excel('data/contact_matrices_152_countries/MUestimates_work_1.xlsx', sheet_name='India',index_col=None)\nCW = np.array(my_data)\n\nmy_data = pd.read_excel('data/contact_matrices_152_countries/MUestimates_school_1.xlsx', sheet_name='India',index_col=None)\nCS = np.array(my_data)\n\nmy_data = pd.read_excel('data/contact_matrices_152_countries/MUestimates_other_locations_1.xlsx', sheet_name='India',index_col=None)\nCO = np.array(my_data)\n\nmy_data = pd.read_excel('data/contact_matrices_152_countries/MUestimates_all_locations_1.xlsx', sheet_name='India',index_col=None)\nCA = np.array(my_data)\nCM = CH + CW + CS + CO\n\nmy_data_nw = np.genfromtxt('data/covid-cases/india_10april.txt', delimiter='', skip_header=6)\ndeath_case, active_case = my_data_nw[:,4], my_data_nw[:,5]\n\nactive = Real_data[:,7]\nactive_new = active[34:107]\ndeath = Real_data[:,6]\ndeath_new = death[34:107]\n#save_results_to = 'C:/Users/HP/Desktop/Lat_radon/double peak/EPS_file/'",
"_____no_output_____"
],
[
"alpha_d = 0.05 #fractional constant \nbeta = 0.37 #rate of infection\nrho = 0.75 #control parameter of H\nxi = 0.29 #recovery rate from E\nalpha_1 = 0.7 #fractional part of E-->Q\nalpha_2 = 0.2 #fractional part of E-->A\nalpha_3 = 1-(alpha_1+alpha_2) #fractional part of E-->I\nphi_qh = 1/10 #Recovery rate of Q-->H\nq = 0.1 #fractional part of Q-->H\ng_as = 0.1 #rate A-->I\nd_ar = 2./7 #Recovery rate of A\nphi_sh = 1./2 #rate I-->H \nd_sr = 1./7 #Recovery rate of I\nd_hr = (1-alpha_d)/10 #Recovery rate of H \neta = alpha_d/10 #Death rate\nfsa = 0.1 #Fraction of the contact matrix Cs \nfsh = 0.1 #Fraction of the contact matrix Ch \n\n\n# initial conditions\nE_0 = np.zeros((M));\nQ_0 = np.zeros((M))\nA_0 = np.zeros((M))\nI_0 = np.zeros((M)); I_0[6:13]=2; I_0[2:6]=1\nH_0 = np.zeros((M))\nR_0 = np.zeros((M))\nD_0 = np.zeros((M))\nS_0 = Ni - (E_0+ Q_0 + A_0 + I_0 + H_0 + R_0 + D_0)",
"_____no_output_____"
],
[
"Tf = 300; Nf = 3000 #Tf -->final time from 0, Nf-->total number points\nt = np.linspace(0,Tf,Nf) #time span\n\n#lockdown function\nld = lambda t, t_on, t_off, t_won, t_woff, pld: 1 + pld*0.5*(np.tanh((t - t_off)/t_woff) - np.tanh((t - t_on)/t_won))\n\n#staggered lockdown\nuc = lambda t:0.7-0.4*(np.tanh((t - 21)/4)) + 0.3*0.3*(1.0*np.tanh((t - 42)/4)-np.tanh((t - 93)/4))+\\\n 0.2+0.1*(np.tanh((t - 75)/4)) + 0.4*0.5*(np.tanh((t - 93)/4))\n\n\n#LD2\n#uc = lambda t:0.7-0.4*(np.tanh((t - 21)/4)) + 0.3*0.3*(1.0*np.tanh((t - 42)/4)-np.tanh((t - 93)/4))+\\\n# 0.2+0.1*(np.tanh((t - 75)/4)) + 0.4*0.5*(np.tanh((t - 93)/4)) +\\\n#ld(t,128, 153, 2, 2, 0.6-0.2) + ld(t,153,193, 2, 2, 0.8-0.2) + ld(t,193,233, 2, 2, 0.6-0.2)+ld(t,233,360, 2, 2, 0.4-0.2)-4.0\n\n#LD3\n#uc = lambda t:0.7-0.4*(np.tanh((t - 21)/4)) + 0.3*0.3*(1.0*np.tanh((t - 42)/4)-np.tanh((t - 93)/4))+\\\n# 0.2+0.1*(np.tanh((t - 75)/4)) + 0.4*0.5*(np.tanh((t - 93)/4)) +\\\n#ld(t,130, 160, 2, 2, 0.6-0.2)+ld(t,160, 230, 2, 2, 0.8-0.2) + ld(t,230, 300, 2, 2, 0.6-0.2) + ld(t,300, 420, 2, 2, 0.4-0.2) - 4.0\n\nbeta_max, k, t_m, beta_min = beta, 0.2, 49, 0.21 # \ndef beta_f(t):\n return ((beta_max-beta_min) / (1 + np.exp(-k*(-t+t_m))) + beta_min)\n\nplt.figure(figsize=(16,5))\nplt.rcParams['font.size']=26\nplt.subplot(1,2,1)\nplt.plot(t,beta_f(t),lw=3);\nplt.title(r'$\\beta(t)$')\nplt.grid(True)\nplt.xlim(0,100);\nplt.subplot(1,2,2)\nplt.plot(t, uc(t),lw=3)\nplt.title('Lockdown Strategy')\nplt.tight_layout(True)\nplt.grid(True)",
"_____no_output_____"
],
[
"def cont(t):\n return CH + uc(t)*(CW + CO + CS)\n #return CM\n\n# S=y[i], E=y[M+i], Q=y[2M+i],A=y[3M+i], I=y[4M+i], H=y[5M+i], R=y[6M+i] for i=1,2,3,...,M\n\ndy = np.zeros(7*M)\ndef rhs(y, t, cont, beta_f):\n CM = cont(t) #contact matrix\n for i in range(M):\n lmda=0\n for j in range(M):\n lmda += beta_f(t)*(CM[i,j]*y[3*M+j] + fsa*CM[i,j]*y[4*M+j] +fsh*(1.0-rho)*CM[i,j]*y[5*M+j])/Ni[j] \n dy[i] = - lmda*y[i] + (1-q)*phi_qh*y[2*M+i] # S susceptibles\n dy[i+M] = lmda*y[i] - xi*y[M+i] #E exposed class \n dy[i+2*M] = alpha_1*xi*y[M+i] - phi_qh*y[2*M+i] #Q Quarantined \n dy[i+3*M] = alpha_2*xi*y[M+i] - (g_as + d_ar )*y[3*M+i] #A Asymptomatic infected \n dy[i+4*M] = alpha_3*xi*y[M+i] + g_as*y[3*M+i] - (phi_sh + d_sr)*y[4*M+i] #I Symptomatic infected \n dy[i+5*M] = phi_sh*y[4*M+i] + q*phi_qh*y[2*M+i] - (d_hr + eta)*y[5*M+i] #H Isolated\n dy[i+6*M] = d_ar*y[3*M+i] + d_sr*y[4*M+i] + d_hr*y[5*M+i] #Recovered\n return dy\n \ndata = odeint(rhs, np.concatenate((S_0, E_0, Q_0, A_0, I_0, H_0, R_0)), t, args=(cont,beta_f))\ntempS, tempE, tempQ, tempA, tempI, tempH, tempR = np.zeros((Nf)),\\\nnp.zeros((Nf)), np.zeros((Nf)), np.zeros((Nf)), np.zeros((Nf)), np.zeros((Nf)), np.zeros((Nf))\nfor i in range(M):\n tempS += data[:, 0*M + i]\n tempE += data[:, 1*M + i]\n tempQ += data[:, 2*M + i]\n tempA += data[:, 3*M + i]\n tempI += data[:, 4*M + i]\n tempH += data[:, 5*M + i]\n tempR += data[:, 6*M + i]\nIC_death = N - (tempS + tempE + tempQ + tempA + tempI + tempH + tempR)",
"_____no_output_____"
]
],
[
[
"**Simulated individuals figure**",
"_____no_output_____"
]
],
[
[
"fig = plt.figure(num=None, figsize=(28, 12), dpi=80, facecolor='w', edgecolor='k')\nplt.rcParams.update({'font.size': 26})\nplt.plot(t, (tempA + tempI + tempH)/N, '--', lw=6, color='g', label='Active Case', alpha=0.8)\nplt.plot(t, (tempA + tempI)/N , '-', lw=7, color='k', label='$A + I$', alpha=0.8)\nplt.plot(t, IC_death/N, '-.', lw=4, color='r', label='Death', alpha=0.8)\nplt.plot(t, tempH/N, '-', lw=3, color='b', label='H', alpha=0.8)\nplt.legend(fontsize=26, loc='best'); plt.grid()\nplt.autoscale(enable=True, axis='x', tight=True)\nplt.ylabel('Individuals(Normalized)');\nplt.text(163.5,0.0175,'14-Aug(163Days)',rotation=90)\nplt.xlim(0,300);\nplt.xlabel('Time(Days)')\nplt.axvline(163,c='k',lw=3,ls='--');\n#plt.savefig(save_results_to+'Figure10.png', format='png', dpi=200)",
"_____no_output_____"
]
],
[
[
"**Analysis between real case data vs numerical**",
"_____no_output_____"
]
],
[
[
"fig = plt.figure(num=None, figsize=(28, 12), dpi=80, facecolor='w', edgecolor='k')\nplt.rcParams.update({'font.size': 26, 'text.color':'black'})\nplt.plot(t, tempA + tempI + tempH, '--', lw=4, color='g', label='Active case numerical', alpha=0.8)\nplt.plot(active_new, 'o-', lw=4, color='#348ABD', ms=16, label='Active case data', alpha=0.5)\nplt.plot(t, IC_death, '-.', lw=4, color='r', label='Death case numerical', alpha=0.8)\nplt.plot(death_new, '-*', lw=4, color='#348ABD', ms=16, label='death case data', alpha=0.5)\nplt.xticks(np.arange(0, 200, 14),('4 Mar','18 Mar','1 Apr','15 Apr','29 Apr','13 May','27 May','10Jun','24Jun'));\nplt.legend(fontsize=26, loc='best'); plt.grid()\nplt.autoscale(enable=True, axis='x', tight=True)\nplt.ylabel('Number of individuals');\nplt.xlabel('Time(Dates)')\nplt.ylim(0, 60000);\nplt.xlim(0, 98);",
"_____no_output_____"
]
],
[
[
"**Sensitivity of hospitalization parameter $\\rho$**",
"_____no_output_____"
]
],
[
[
"q = 1.0\nrhos = [0.0, 0.25, 0.5, 0.75, 1.0]\nfig = plt.figure(num=None, figsize=(20, 8), dpi=80, facecolor='w', edgecolor='k')\nplt.rcParams.update({'font.size': 20})\nfor rho in rhos:\n data = odeint(rhs, np.concatenate((S_0, E_0, Q_0, A_0, I_0, H_0, R_0)), t, args=(cont,beta_f))\n tempS, tempE, tempQ, tempA, tempI, tempH, tempR = np.zeros((Nf)),\\\n np.zeros((Nf)), np.zeros((Nf)), np.zeros((Nf)), np.zeros((Nf)), np.zeros((Nf)), np.zeros((Nf))\n for i in range(M):\n tempA += data[:, 3 * M + i]\n tempI += data[:, 4 * M + i]\n tempH += data[:, 5 * M + i]\n if rho==1.0:\n yy = tempA/N + tempI/N + tempH/N\n plt.plot(t,yy, lw = 2, ls='-',c='b', label=r'$\\rho = $' + str(rho))\n plt.plot(t[::100],yy[::100], '>', label=None, markersize=11, c='b')\n elif rho==0.75: \n plt.plot(t,tempA/N + tempI/N + tempH/N, lw = 3, c='orange')\n elif rho==0.5: \n plt.plot(t,tempA/N + tempI/N + tempH/N, lw = 3, c='g')\n elif rho==0.25: \n plt.plot(t,tempA/N + tempI/N + tempH/N, lw = 3, c='r')\n else:\n yy = tempA/N + tempI/N + tempH/N\n plt.plot(t,tempA/N + tempI/N + tempH/N, lw = 3, ls='-', c='k')\n plt.plot(t[::100],yy[::100], '.', label=None, markersize=14, c='k')\nplt.ylabel('Active Case(Normalized)');\nplt.xlabel('Time (Days)');\nplt.autoscale(enable=True, axis='x',tight=True)\nplt.grid(True)\ncolors = ['k', 'r','g','orange', 'b']\nmarker = ['.', None, None, None, '>']\nlines = [Line2D([0], [0], color=c, linewidth=3, linestyle='-',marker=r, markersize=14) for (c,r) in zip(colors,marker)]\nlabels = [r'$\\rho=0.0$',r'$\\rho=0.25$',r'$\\rho=0.5$',r'$\\rho=0.75$',r'$\\rho=1.0$']\nplt.legend(lines, labels,title=r'$q$ ='+str(q)+'(Fixed)')\n#plt.savefig('rho_var1.png', format='png',dpi=200)\n#plt.savefig(save_results_to+'Figure08.png', format='png',dpi=200)",
"_____no_output_____"
]
],
[
[
"**Sensitivity of quarantine parameter $q$**",
"_____no_output_____"
]
],
[
[
"rho = 1.0\nqs = [0.0, 0.25, 0.5, 0.75, 1.0]\nfig = plt.figure(num=None, figsize=(20, 8), dpi=80, facecolor='w', edgecolor='k')\nplt.rcParams.update({'font.size': 20})\nfor q in qs:\n data = odeint(rhs, np.concatenate((S_0, E_0, Q_0, A_0, I_0, H_0, R_0)), t, args=(cont,beta_f))\n tempS, tempE, tempQ, tempA, tempI, tempH, tempR = np.zeros((Nf)),\\\n np.zeros((Nf)), np.zeros((Nf)), np.zeros((Nf)), np.zeros((Nf)), np.zeros((Nf)), np.zeros((Nf))\n for i in range(M):\n tempA += data[:, 3 * M + i]\n tempI += data[:, 4 * M + i]\n tempH += data[:, 5 * M + i]\n if q==1.0:\n yy = tempA/N + tempI/N + tempH/N\n plt.plot(t,yy, lw = 2, ls='-',c='b')\n plt.plot(t[::100],yy[::100], '>', label=None, markersize=11, c='b')\n elif q==0.75: \n plt.plot(t,tempA/N + tempI/N + tempH/N, lw = 3, c='orange')\n elif q==0.5: \n plt.plot(t,tempA/N + tempI/N + tempH/N, lw = 3, c='g')\n elif q==0.25: \n plt.plot(t,tempA/N + tempI/N + tempH/N, lw = 3, c='r')\n else:\n yy = tempA/N + tempI/N + tempH/N\n plt.plot(t,tempA/N + tempI/N + tempH/N, lw = 3, ls='-', c='k')\n plt.plot(t[::100],yy[::100], '.', label=None, markersize=14, c='k')\nplt.ylabel('Active Case(Normalized)');\nplt.xlabel('Time (Days)');\nplt.autoscale(enable=True, axis='x',tight=True)\nplt.grid(True)\ncolors = ['k','r','g','orange','b']\nmarker = ['.', None, None, None, '>']\nlines = [Line2D([0], [0], color=c, linewidth=3, linestyle='-',marker=r, markersize=14) for (c,r) in zip(colors,marker)]\nlabels = [r'$q=0.0$',r'$q=0.25$',r'$q=0.5$',r'$q=0.75$',r'$q=1.0$']\nplt.legend(lines, labels,title=r'$\\rho$ ='+str(rho)+'(Fixed)')\n#plt.savefig('q_var1.png', format='png',dpi=200)\n#plt.savefig(save_results_to+'Figure07.png', format='png',dpi=200)",
"_____no_output_____"
]
]
] | [
"markdown",
"code",
"markdown",
"code",
"markdown",
"code",
"markdown",
"code",
"markdown",
"code"
] | [
[
"markdown"
],
[
"code",
"code",
"code",
"code",
"code",
"code"
],
[
"markdown"
],
[
"code"
],
[
"markdown"
],
[
"code"
],
[
"markdown"
],
[
"code"
],
[
"markdown"
],
[
"code"
]
] |
d08e74111849e67f8fe9600cf107439c2db30fae | 3,457 | ipynb | Jupyter Notebook | count_beepers_variables.ipynb | aktan-amanaliev/carel-jupyter | b844ef0243cad65caae200793a3ad734ae1c6d68 | [
"Apache-2.0"
] | null | null | null | count_beepers_variables.ipynb | aktan-amanaliev/carel-jupyter | b844ef0243cad65caae200793a3ad734ae1c6d68 | [
"Apache-2.0"
] | null | null | null | count_beepers_variables.ipynb | aktan-amanaliev/carel-jupyter | b844ef0243cad65caae200793a3ad734ae1c6d68 | [
"Apache-2.0"
] | null | null | null | 22.89404 | 58 | 0.42696 | [
[
[
"from carel import Carel\nfrom carelgrid import CarelGrid\nfrom gamecanvas import GameCanvas\n\nfield = [[0 for x in range(6)] for y in range(4)]\n\nfield[0][1] = 1\nfield[1][0] = 2\nfield[3][2] = 1\n\ngrid = CarelGrid(field)\ncanvas = GameCanvas(speed=3);\ncarel = Carel(canvas, grid);\n\nbeepers_count = 0\n\ndef move():\n carel.move()\ndef turn_left():\n carel.turn_left()\ndef drop_beeper():\n carel.drop_beeper()\ndef collect_beeper():\n global beepers_count\n carel.collect_beeper()\n beepers_count = beepers_count + 1\ndef is_beeper():\n return carel.is_beeper()\ndef is_front_clear():\n return carel.is_front_clear()\ndef show():\n carel.show()",
"',(0):> 1 0 0 0 0 \n 2 0 0 0 0 0 \n 0 0 0 0 0 0 \n 0 0 1 0 0 0 \n"
],
[
"# New methods go here\n\n# Example\n\ndef turn_around():\n turn_left()\n turn_left()\n\ndef clear_to_wall_count():\n while True:\n clear_cell_count()\n if not is_front_clear():\n break\n move()\n\ndef clear_cell_count():\n while is_beeper():\n collect_beeper()\n\ndef turn_right():\n turn_left()\n turn_left()\n turn_left()",
"_____no_output_____"
],
[
"while True:\n clear_to_wall_count()\n turn_right()\n if not is_front_clear():\n break\n move()\n turn_right()\n clear_to_wall_count()\n turn_left()\n if not is_front_clear():\n break\n move()\n turn_left()\n\nfor i in range(beepers_count):\n drop_beeper()\n ",
" 0 0 0 0 0 0 \n 0 0 0 0 0 0 \n 0 0 0 0 0 0 \n',(4):v 0 0 0 0 0 \n"
]
]
] | [
"code"
] | [
[
"code",
"code",
"code"
]
] |
d08e795d705a0682411639f4f0b23b455f5d73c1 | 4,715 | ipynb | Jupyter Notebook | 06-Manipulando_Banco_Dados_Python/04-SQLite - Select no SQLite.ipynb | alineAssuncao/Python_Fundamentos_Analise_Dados | 872781f2cec24487b0f29f62afeb60650a451bfd | [
"MIT"
] | 1 | 2019-02-03T10:53:55.000Z | 2019-02-03T10:53:55.000Z | 06-Manipulando_Banco_Dados_Python/04-SQLite - Select no SQLite.ipynb | alineAssuncao/Python_Fundamentos_Analise_Dados | 872781f2cec24487b0f29f62afeb60650a451bfd | [
"MIT"
] | null | null | null | 06-Manipulando_Banco_Dados_Python/04-SQLite - Select no SQLite.ipynb | alineAssuncao/Python_Fundamentos_Analise_Dados | 872781f2cec24487b0f29f62afeb60650a451bfd | [
"MIT"
] | null | null | null | 25.079787 | 152 | 0.493531 | [
[
[
"# Select no SQLite",
"_____no_output_____"
]
],
[
[
"import sqlite3\nimport random\nimport time\nimport datetime",
"_____no_output_____"
],
[
"# criando conexão\nconn = sqlite3.connect('dsa.db')\n\n# cursor\nc = conn.cursor()\n\n# criar tabela\ndef create_table():\n comando_create = 'CREATE TABLE IF NOT EXISTS produtos(id INTEGER PRIMARY KEY AUTOINCREMENT NOT NULL, date TEXT, prod_name TEXT,valor REAL)'\n c.execute(comando_create)\n \ndef data_insert():\n comando_insert = \"INSERT INTO produtos VALUES(002, '02-05-2016', 'teclado', 130)\"\n c.execute(comando_insert)\n conn.commit()\n c.close()\n conn.close()\n \ndef data_insert_var():\n new_date = datetime.datetime.now()\n new_prod = 'monitor2'\n new_valor = random.randrange(50,100)\n c.execute(\"INSERT INTO produtos (date, prod_name, valor) VALUES (?, ?, ?, ?)\", (new_date, new_prod, new_valor))\n conn.commit()\n \ndef leitura_todos_dados():\n c.execute(\"SELECT * FROM produtos\")\n for linha in c.fetchall():\n print(linha)\n\ndef leitura_registros():\n c.execute(\"SELECT * FROM produtos WHERE valor > 60.0\")\n for linha in c.fetchall():\n print(linha)\n \n# leitura de coluna especifica\ndef leitura_colunas():\n c.execute(\"SELECT * FROM produtos\")\n for linha in c.fetchall():\n print(linha[3])",
"_____no_output_____"
],
[
"leitura_todos_dados()",
"(10, '2018-05-02 14:32:11', 'Teclado', 90.0)\n(11, '2018-08-23 17:45:32.832539', 'Monitor', 93.0)\n(12, '2018-08-23 17:45:33.842539', 'Monitor', 92.0)\n(13, '2018-08-23 17:45:34.858539', 'Monitor', 60.0)\n(14, '2018-08-23 17:45:35.866539', 'Monitor', 98.0)\n(15, '2018-08-23 17:45:36.872539', 'Monitor', 58.0)\n(16, '2018-08-23 17:45:37.880539', 'Monitor', 96.0)\n(17, '2018-08-23 17:45:38.894539', 'Monitor', 54.0)\n(18, '2018-08-23 17:45:39.904539', 'Monitor', 91.0)\n(19, '2018-08-23 17:45:40.919539', 'Monitor', 74.0)\n(20, '2018-08-23 17:45:41.928539', 'Monitor', 67.0)\n"
],
[
"leitura_registros()",
"(10, '2018-05-02 14:32:11', 'Teclado', 90.0)\n(11, '2018-08-23 17:45:32.832539', 'Monitor', 93.0)\n(12, '2018-08-23 17:45:33.842539', 'Monitor', 92.0)\n(14, '2018-08-23 17:45:35.866539', 'Monitor', 98.0)\n(16, '2018-08-23 17:45:37.880539', 'Monitor', 96.0)\n(18, '2018-08-23 17:45:39.904539', 'Monitor', 91.0)\n(19, '2018-08-23 17:45:40.919539', 'Monitor', 74.0)\n(20, '2018-08-23 17:45:41.928539', 'Monitor', 67.0)\n"
],
[
"leitura_colunas()",
"90.0\n93.0\n92.0\n60.0\n98.0\n58.0\n96.0\n54.0\n91.0\n74.0\n67.0\n"
],
[
"c.close()\nconn.close()",
"_____no_output_____"
]
]
] | [
"markdown",
"code"
] | [
[
"markdown"
],
[
"code",
"code",
"code",
"code",
"code",
"code"
]
] |
d08e7ed2078c8d3e3291f300558e13c25ad077e4 | 13,843 | ipynb | Jupyter Notebook | notebooks/archive_exploration/notebooks/01 - subject coocurrence.ipynb | wellcomecollection/data-science | b91b31c344e2d8ca43f1e3e92a7b361ba110f25b | [
"MIT"
] | 5 | 2019-12-07T09:29:38.000Z | 2021-09-02T09:46:56.000Z | notebooks/archive_exploration/notebooks/01 - subject coocurrence.ipynb | wellcomecollection/data-science | b91b31c344e2d8ca43f1e3e92a7b361ba110f25b | [
"MIT"
] | 37 | 2019-10-25T11:17:35.000Z | 2021-10-20T16:39:50.000Z | notebooks/archive_exploration/notebooks/01 - subject coocurrence.ipynb | wellcomecollection/data-science | b91b31c344e2d8ca43f1e3e92a7b361ba110f25b | [
"MIT"
] | 1 | 2021-01-14T18:14:52.000Z | 2021-01-14T18:14:52.000Z | 35.955844 | 481 | 0.598931 | [
[
[
"# Archive data\nThe Wellcome archive sits in a collections management system called CALM, which follows a rough set of standards and guidelines for storing archival records called [ISAD(G)](https://en.wikipedia.org/wiki/ISAD(G). The archive is comprised of _collections_, each of which has a hierarchical set of series, sections, subjects, items and pieces sitting underneath it. \nIn the following notebooks I'm going to explore it and try to make as much sense of it as I can programatically.\n\nLet's start by loading in a few useful packages and defining some nice utils.",
"_____no_output_____"
]
],
[
[
"%matplotlib inline\nimport matplotlib.pyplot as plt\nimport seaborn as sns\n\nsns.set_style(\"white\")\nplt.rcParams[\"figure.figsize\"] = (20, 20)\n\nimport pandas as pd\nimport numpy as np\nimport networkx as nx\n\nfrom sklearn.cluster import AgglomerativeClustering\n\nfrom umap import UMAP\nfrom tqdm import tqdm_notebook as tqdm",
"_____no_output_____"
],
[
"def flatten(input_list):\n return [item for sublist in input_list for item in sublist]\n\n\ndef cartesian(*arrays):\n return np.array([x.reshape(-1) for x in np.meshgrid(*arrays)]).T\n\n\ndef clean(subject):\n return subject.strip().lower().replace(\"<p>\", \"\")",
"_____no_output_____"
]
],
[
[
"let's load up our CALM data. The data has been exported in its entirety as a single `.json` where each line is a record. \nYou can download the data yourself using [this script](https://github.com/wellcometrust/platform/blob/master/misc/download_oai_harvest.py). Stick the `.json` in the neighbouring `/data` directory to run the rest of the notebook seamlessly.",
"_____no_output_____"
]
],
[
[
"df = pd.read_json(\"data/calm_records.json\")",
"_____no_output_____"
],
[
"len(df)",
"_____no_output_____"
],
[
"df.astype(str).describe()",
"_____no_output_____"
]
],
[
[
"### Exploring individual columns\nAt the moment I have no idea what kind of information CALM contains - lets look at the list of column names",
"_____no_output_____"
]
],
[
[
"list(df)",
"_____no_output_____"
]
],
[
[
"Here I'm looking through a sample of values in each column, choosing the columns to explore based on the their headings, a bit of contextual info from colleagues and the `df.describe()` above. ",
"_____no_output_____"
]
],
[
[
"df[\"Subject\"]",
"_____no_output_____"
]
],
[
[
"### After much trial and error...\nSubjects look like an interesting avenue to explore further. Where subjects have _actually_ been filled in and the entry is not `None`, a list of subjects is returned. \nWe can explore some of these subjects' subtleties by creating an adjacency matrix. We'll count the number of times each subject appears alongside every other subject and return a big $n \\times n$ matrix, where $n$ is the total number of unique subjects. \nWe can use this adjacency matrix for all sorts of stuff, but we have to build it first. To start, lets get a uniqur list of all subjects. This involves unpacking each sub-list and flattening them out into one long list, before finding the unique elements. We'll also use the `clean` function defined above to get rid of any irregularities which might become annoying later on.",
"_____no_output_____"
]
],
[
[
"subjects = flatten(df[\"Subject\"].dropna().tolist())\nprint(len(subjects))\nsubjects = list(set(map(clean, subjects)))\nprint(len(subjects))",
"_____no_output_____"
]
],
[
[
"At this point it's often helpful to index our data, ie transform words into numbers. We'll create two dictionaries which map back and forth between the subjects and their corresponding indicies:",
"_____no_output_____"
]
],
[
[
"index_to_subject = {index: subject for index, subject in enumerate(subjects)}\nsubject_to_index = {subject: index for index, subject in enumerate(subjects)}",
"_____no_output_____"
]
],
[
[
"Lets instantiate an empty numpy array which we'll then fill with our coocurrence data. Each column and each row will represent a subject - each cell (the intersection of a column and row) will therefore represent the 'strength' of the interaction between those subjects. As we haven't seen any interactions yet, we'll set every array element to 0.",
"_____no_output_____"
]
],
[
[
"adjacency = np.empty((len(subjects), len(subjects)), dtype=np.uint16)",
"_____no_output_____"
]
],
[
[
"To populate the matrix, we want to find every possible combination of subject in each sub-list from our original column, ie if we had the subjects\n\n`[Disease, Heart, Heart Diseases, Cardiology]`\n\nwe would want to return \n\n`\n[['Disease', 'Disease'],\n ['Heart', 'Disease'],\n ['Heart Diseases', 'Disease'],\n ['Cardiology', 'Disease'],\n ['Disease', 'Heart'],\n ['Heart', 'Heart'],\n ['Heart Diseases', 'Heart'],\n ['Cardiology', 'Heart'],\n ['Disease', 'Heart Diseases'],\n ['Heart', 'Heart Diseases'],\n ['Heart Diseases', 'Heart Diseases'],\n ['Cardiology', 'Heart Diseases'],\n ['Disease', 'Cardiology'],\n ['Heart', 'Cardiology'],\n ['Heart Diseases', 'Cardiology'],\n ['Cardiology', 'Cardiology']]\n`\n\nThe `cartesian()` function which I've defined above will do that for us. We then find the appropriate intersection in the matrix and add another unit of 'strength' to it. \nWe'll do this for every row of subjects in the `['Subjects']` column.",
"_____no_output_____"
]
],
[
[
"for row_of_subjects in tqdm(df[\"Subject\"].dropna()):\n for subject_pair in cartesian(row_of_subjects, row_of_subjects):\n subject_index_1 = subject_to_index[clean(subject_pair[0])]\n subject_index_2 = subject_to_index[clean(subject_pair[1])]\n\n adjacency[subject_index_1, subject_index_2] += 1",
"_____no_output_____"
]
],
[
[
"We can do all sorts of fun stuff now - adjacency matrices are the foundation on which all of graph theory is built. However, because it's a bit more interesting, I'm going to start with some dimensionality reduction. We'll get to the graphy stuff later. \nUsing [UMAP](https://github.com/lmcinnes/umap), we can squash the $n \\times n$ dimensional matrix down into a $n \\times m$ dimensional one, where $m$ is some arbitrary integer. Setting $m$ to 2 will then allow us to plot each subject as a point on a two dimensional plane. UMAP will try to preserve the 'distances' between subjects - in this case, that means that related or topically similar subjects will end up clustered together, and different subjects will move apart.",
"_____no_output_____"
]
],
[
[
"embedding_2d = pd.DataFrame(UMAP(n_components=2).fit_transform(adjacency))",
"_____no_output_____"
],
[
"embedding_2d.plot.scatter(x=0, y=1);",
"_____no_output_____"
]
],
[
[
"We can isolate the clusters we've found above using a number of different methods - `scikit-learn` provides easy access to some very powerful algorithms. Here I'll use a technique called _agglomerative clustering_, and make a guess that 15 is an appropriate number of clusters to look for.",
"_____no_output_____"
]
],
[
[
"n_clusters = 15\n\nembedding_2d[\"labels\"] = AgglomerativeClustering(n_clusters).fit_predict(\n embedding_2d.values\n)\n\nembedding_2d.plot.scatter(x=0, y=1, c=\"labels\", cmap=\"Paired\");",
"_____no_output_____"
]
],
[
[
"We can now use the `index_to_subject` mapping that we created earlier to examine which subjects have been grouped together into clusters",
"_____no_output_____"
]
],
[
[
"for i in range(n_clusters):\n print(str(i) + \" \" + \"-\" * 80 + \"\\n\")\n print(\n np.sort(\n [\n index_to_subject[index]\n for index in embedding_2d[embedding_2d[\"labels\"] == i].index.values\n ]\n )\n )\n print(\"\\n\")",
"_____no_output_____"
]
],
[
[
"Interesting! Taking a look at some of the smaller clusters of subjects (for the sake of space and your willingness to read lists of 100s of subjects):\n\nOne seems to be quite distinctly involved with drugs and associated topics/treatments:\n```\n13 --------------------------------------------------------------------------------\n\n['acquired immunodeficiency syndrome' 'alcohol' 'amphetamines'\n 'analgesics, opioid' 'campaign' 'cannabis' 'cocaine' 'counseling'\n 'counterculture' 'crime' 'drugs' 'education' 'hallucinogens' 'heroin'\n 'hypnotics and sedatives' 'information services' 'inhalant abuse'\n 'lysergic acid diethylamide' 'n-methyl-3,4-methylenedioxyamphetamine'\n 'opioid' 'policy' 'prescription drugs' 'rehabilitation' 'renabilitation'\n 'self-help']\n```\n\nothers are linked to early/fundamental research on DNA and genetics:\n\n```\n9 --------------------------------------------------------------------------------\n\n['bacteriophages' 'biotechnology' 'caenorhabditis elegans'\n 'chromosome mapping' 'cloning, organism' 'discoveries in science' 'dna'\n 'dna, recombinant' 'genetic code' 'genetic engineering'\n 'genetic research' 'genetic therapy' 'genome, human' 'genomics'\n 'magnetic resonance spectroscopy' 'meiosis' 'models, molecular'\n 'molecular biology' 'nobel prize' 'retroviridae' 'rna'\n 'sequence analysis' 'viruses']\n```\n\nand others about food\n```\n14 --------------------------------------------------------------------------------\n\n['acids' 'advertising' 'ambergris' 'animals' 'beer' 'biscuits' 'brassica'\n 'bread' 'butter' 'cacao' 'cake' 'candy' 'carbohydrates' 'cattle'\n 'cereals' 'cheese' 'chemistry, agricultural' 'cider' 'colouring agents'\n 'condiments' 'cooking (deer)' 'cooking (poultry)' 'cooking (venison)'\n 'cucumis sativus' 'dairy products' 'daucus carota' 'desserts'\n 'dried fruit' 'ecology' 'economics' 'eggs' 'environmental health'\n 'european rabbit' 'fermentation' 'food additives' 'food and beverages'\n 'food preservation' 'food, genetically modified' 'fruit' 'fruit drinks'\n 'fungi' 'game and game-birds' 'grapes' 'hands' 'health attitudes'\n 'herbaria' 'honey' 'jam' 'legislation' 'lettuce' 'meat' 'meat products'\n 'nuts' 'oatmeal' 'olive' 'onions' 'peas' 'pickles' 'pies' 'poultry'\n 'preserves (jams)' 'puddings' 'rice' 'seafood' 'seeds' 'sheep'\n 'sociology' 'solanum tuberosum' 'spinacia oleracea' 'sweetening agents'\n 'swine' 'syrups' 'vegetables' 'vitis' 'whiskey' 'wild flowers' 'wine']\n```\n\nThese are all noticeably different themes, and they appear to be nicely separated in the topic-space we've built.",
"_____no_output_____"
]
]
] | [
"markdown",
"code",
"markdown",
"code",
"markdown",
"code",
"markdown",
"code",
"markdown",
"code",
"markdown",
"code",
"markdown",
"code",
"markdown",
"code",
"markdown",
"code",
"markdown",
"code",
"markdown",
"code",
"markdown"
] | [
[
"markdown"
],
[
"code",
"code"
],
[
"markdown"
],
[
"code",
"code",
"code"
],
[
"markdown"
],
[
"code"
],
[
"markdown"
],
[
"code"
],
[
"markdown"
],
[
"code"
],
[
"markdown"
],
[
"code"
],
[
"markdown"
],
[
"code"
],
[
"markdown"
],
[
"code"
],
[
"markdown"
],
[
"code",
"code"
],
[
"markdown"
],
[
"code"
],
[
"markdown"
],
[
"code"
],
[
"markdown"
]
] |
d08e98237d5b3c090adbe21bd5daa805df7f6302 | 53,319 | ipynb | Jupyter Notebook | project-tv-script-generation/dlnd_tv_script_generation.ipynb | NorahAlshaya/TV-Script-Generation-Project | 7c61b3c1229798944e2c36c45fb3447db93f2621 | [
"MIT"
] | null | null | null | project-tv-script-generation/dlnd_tv_script_generation.ipynb | NorahAlshaya/TV-Script-Generation-Project | 7c61b3c1229798944e2c36c45fb3447db93f2621 | [
"MIT"
] | null | null | null | project-tv-script-generation/dlnd_tv_script_generation.ipynb | NorahAlshaya/TV-Script-Generation-Project | 7c61b3c1229798944e2c36c45fb3447db93f2621 | [
"MIT"
] | null | null | null | 37.182008 | 995 | 0.548679 | [
[
[
"# TV Script Generation\n\nIn this project, you'll generate your own [Seinfeld](https://en.wikipedia.org/wiki/Seinfeld) TV scripts using RNNs. You'll be using part of the [Seinfeld dataset](https://www.kaggle.com/thec03u5/seinfeld-chronicles#scripts.csv) of scripts from 9 seasons. The Neural Network you'll build will generate a new ,\"fake\" TV script, based on patterns it recognizes in this training data.\n\n## Get the Data\n\nThe data is already provided for you in `./data/Seinfeld_Scripts.txt` and you're encouraged to open that file and look at the text. \n>* As a first step, we'll load in this data and look at some samples. \n* Then, you'll be tasked with defining and training an RNN to generate a new script!",
"_____no_output_____"
]
],
[
[
"\"\"\"\nDON'T MODIFY ANYTHING IN THIS CELL\n\"\"\"\n# load in data\nimport helper\ndata_dir = './data/Seinfeld_Scripts.txt'\ntext = helper.load_data(data_dir)",
"_____no_output_____"
]
],
[
[
"## Explore the Data\nPlay around with `view_line_range` to view different parts of the data. This will give you a sense of the data you'll be working with. You can see, for example, that it is all lowercase text, and each new line of dialogue is separated by a newline character `\\n`.",
"_____no_output_____"
]
],
[
[
"view_line_range = (0, 10)\n\n\"\"\"\nDON'T MODIFY ANYTHING IN THIS CELL THAT IS BELOW THIS LINE\n\"\"\"\nimport numpy as np\n\nprint('Dataset Stats')\nprint('Roughly the number of unique words: {}'.format(len({word: None for word in text.split()})))\n\nlines = text.split('\\n')\nprint('Number of lines: {}'.format(len(lines)))\nword_count_line = [len(line.split()) for line in lines]\nprint('Average number of words in each line: {}'.format(np.average(word_count_line)))\n\nprint()\nprint('The lines {} to {}:'.format(*view_line_range))\nprint('\\n'.join(text.split('\\n')[view_line_range[0]:view_line_range[1]]))",
"Dataset Stats\nRoughly the number of unique words: 46367\nNumber of lines: 109233\nAverage number of words in each line: 5.544240293684143\n\nThe lines 0 to 10:\njerry: do you know what this is all about? do you know, why were here? to be out, this is out...and out is one of the single most enjoyable experiences of life. people...did you ever hear people talking about we should go out? this is what theyre talking about...this whole thing, were all out now, no one is home. not one person here is home, were all out! there are people trying to find us, they dont know where we are. (on an imaginary phone) did you ring?, i cant find him. where did he go? he didnt tell me where he was going. he must have gone out. you wanna go out you get ready, you pick out the clothes, right? you take the shower, you get all ready, get the cash, get your friends, the car, the spot, the reservation...then youre standing around, what do you do? you go we gotta be getting back. once youre out, you wanna get back! you wanna go to sleep, you wanna get up, you wanna go out again tomorrow, right? where ever you are in life, its my feeling, youve gotta go. \n\njerry: (pointing at georges shirt) see, to me, that button is in the worst possible spot. the second button literally makes or breaks the shirt, look at it. its too high! its in no-mans-land. you look like you live with your mother. \n\ngeorge: are you through? \n\njerry: you do of course try on, when you buy? \n\ngeorge: yes, it was purple, i liked it, i dont actually recall considering the buttons. \n\n"
]
],
[
[
"---\n## Implement Pre-processing Functions\nThe first thing to do to any dataset is pre-processing. Implement the following pre-processing functions below:\n- Lookup Table\n- Tokenize Punctuation\n\n### Lookup Table\nTo create a word embedding, you first need to transform the words to ids. In this function, create two dictionaries:\n- Dictionary to go from the words to an id, we'll call `vocab_to_int`\n- Dictionary to go from the id to word, we'll call `int_to_vocab`\n\nReturn these dictionaries in the following **tuple** `(vocab_to_int, int_to_vocab)`",
"_____no_output_____"
]
],
[
[
"import problem_unittests as tests\nfrom collections import Counter\n\ndef create_lookup_tables(text):\n \"\"\"\n Create lookup tables for vocabulary\n :param text: The text of tv scripts split into words\n :return: A tuple of dicts (vocab_to_int, int_to_vocab)\n \"\"\"\n # TODO: Implement Function\n #define count var\n countVar = Counter(text)\n #define vocab var\n Vocab = sorted(countVar, key=countVar.get, reverse=True)\n #define integer to vocab\n int_to_vocab = {ii: word for ii, word in enumerate(Vocab)}\n #define vocab to integer \n vocab_to_int = {word: ii for ii, word in int_to_vocab.items()}\n # return tuple\n return (vocab_to_int, int_to_vocab)\n\n\n\"\"\"\nDON'T MODIFY ANYTHING IN THIS CELL THAT IS BELOW THIS LINE\n\"\"\"\ntests.test_create_lookup_tables(create_lookup_tables)",
"Tests Passed\n"
]
],
[
[
"### Tokenize Punctuation\nWe'll be splitting the script into a word array using spaces as delimiters. However, punctuations like periods and exclamation marks can create multiple ids for the same word. For example, \"bye\" and \"bye!\" would generate two different word ids.\n\nImplement the function `token_lookup` to return a dict that will be used to tokenize symbols like \"!\" into \"||Exclamation_Mark||\". Create a dictionary for the following symbols where the symbol is the key and value is the token:\n- Period ( **.** )\n- Comma ( **,** )\n- Quotation Mark ( **\"** )\n- Semicolon ( **;** )\n- Exclamation mark ( **!** )\n- Question mark ( **?** )\n- Left Parentheses ( **(** )\n- Right Parentheses ( **)** )\n- Dash ( **-** )\n- Return ( **\\n** )\n\nThis dictionary will be used to tokenize the symbols and add the delimiter (space) around it. This separates each symbols as its own word, making it easier for the neural network to predict the next word. Make sure you don't use a value that could be confused as a word; for example, instead of using the value \"dash\", try using something like \"||dash||\".",
"_____no_output_____"
]
],
[
[
"def token_lookup():\n \"\"\"\n Generate a dict to turn punctuation into a token.\n :return: Tokenized dictionary where the key is the punctuation and the value is the token\n \"\"\"\n # TODO: Implement Function\n tokens = dict()\n tokens['.'] = '||period||'\n tokens[','] = '||comma||'\n tokens['\"'] = '||quotation_mark||'\n tokens[';'] = '||semicolon||'\n tokens['!'] = '||exclam_mark||'\n tokens['?'] = '||question_mark||'\n tokens['('] = '||left_par||'\n tokens[')'] = '||right_par||'\n tokens['-'] = '||dash||'\n tokens['\\n'] = '||return||'\n \n return tokens\n\n\"\"\"\nDON'T MODIFY ANYTHING IN THIS CELL THAT IS BELOW THIS LINE\n\"\"\"\ntests.test_tokenize(token_lookup)",
"Tests Passed\n"
]
],
[
[
"## Pre-process all the data and save it\n\nRunning the code cell below will pre-process all the data and save it to file. You're encouraged to lok at the code for `preprocess_and_save_data` in the `helpers.py` file to see what it's doing in detail, but you do not need to change this code.",
"_____no_output_____"
]
],
[
[
"\"\"\"\nDON'T MODIFY ANYTHING IN THIS CELL\n\"\"\"\n# pre-process training data\nhelper.preprocess_and_save_data(data_dir, token_lookup, create_lookup_tables)",
"_____no_output_____"
]
],
[
[
"# Check Point\nThis is your first checkpoint. If you ever decide to come back to this notebook or have to restart the notebook, you can start from here. The preprocessed data has been saved to disk.",
"_____no_output_____"
]
],
[
[
"\"\"\"\nDON'T MODIFY ANYTHING IN THIS CELL\n\"\"\"\nimport helper\nimport problem_unittests as tests\n\nint_text, vocab_to_int, int_to_vocab, token_dict = helper.load_preprocess()",
"_____no_output_____"
]
],
[
[
"## Build the Neural Network\nIn this section, you'll build the components necessary to build an RNN by implementing the RNN Module and forward and backpropagation functions.\n\n### Check Access to GPU",
"_____no_output_____"
]
],
[
[
"\"\"\"\nDON'T MODIFY ANYTHING IN THIS CELL\n\"\"\"\nimport torch\n\n# Check for a GPU\ntrain_on_gpu = torch.cuda.is_available()\nif not train_on_gpu:\n print('No GPU found. Please use a GPU to train your neural network.')",
"_____no_output_____"
]
],
[
[
"## Input\nLet's start with the preprocessed input data. We'll use [TensorDataset](http://pytorch.org/docs/master/data.html#torch.utils.data.TensorDataset) to provide a known format to our dataset; in combination with [DataLoader](http://pytorch.org/docs/master/data.html#torch.utils.data.DataLoader), it will handle batching, shuffling, and other dataset iteration functions.\n\nYou can create data with TensorDataset by passing in feature and target tensors. Then create a DataLoader as usual.\n```\ndata = TensorDataset(feature_tensors, target_tensors)\ndata_loader = torch.utils.data.DataLoader(data, \n batch_size=batch_size)\n```\n\n### Batching\nImplement the `batch_data` function to batch `words` data into chunks of size `batch_size` using the `TensorDataset` and `DataLoader` classes.\n\n>You can batch words using the DataLoader, but it will be up to you to create `feature_tensors` and `target_tensors` of the correct size and content for a given `sequence_length`.\n\nFor example, say we have these as input:\n```\nwords = [1, 2, 3, 4, 5, 6, 7]\nsequence_length = 4\n```\n\nYour first `feature_tensor` should contain the values:\n```\n[1, 2, 3, 4]\n```\nAnd the corresponding `target_tensor` should just be the next \"word\"/tokenized word value:\n```\n5\n```\nThis should continue with the second `feature_tensor`, `target_tensor` being:\n```\n[2, 3, 4, 5] # features\n6 # target\n```",
"_____no_output_____"
]
],
[
[
"from torch.utils.data import TensorDataset, DataLoader\n\n\ndef batch_data(words, sequence_length, batch_size):\n \"\"\"\n Batch the neural network data using DataLoader\n :param words: The word ids of the TV scripts\n :param sequence_length: The sequence length of each batch\n :param batch_size: The size of each batch; the number of sequences in a batch\n :return: DataLoader with batched data\n \"\"\"\n # TODO: Implement function\n Num_batches = len(words)//batch_size\n \n words = words[:Num_batches*batch_size]\n x, y = [], []\n \n for idx in range(0, len(words) - sequence_length):\n x.append(words[idx:idx+sequence_length])\n y.append(words[idx+sequence_length])\n \n feature_tensors, target_tensors = torch.from_numpy(np.asarray(x)), torch.from_numpy(np.asarray(y))\n dataset = TensorDataset(feature_tensors, target_tensors)\n dataloader = torch.utils.data.DataLoader(dataset, batch_size=batch_size)\n \n # return a dataloader\n return dataloader\n\n# there is no test for this function, but you are encouraged to create\n# print statements and tests of your own\n",
"_____no_output_____"
]
],
[
[
"### Test your dataloader \n\nYou'll have to modify this code to test a batching function, but it should look fairly similar.\n\nBelow, we're generating some test text data and defining a dataloader using the function you defined, above. Then, we are getting some sample batch of inputs `sample_x` and targets `sample_y` from our dataloader.\n\nYour code should return something like the following (likely in a different order, if you shuffled your data):\n\n```\ntorch.Size([10, 5])\ntensor([[ 28, 29, 30, 31, 32],\n [ 21, 22, 23, 24, 25],\n [ 17, 18, 19, 20, 21],\n [ 34, 35, 36, 37, 38],\n [ 11, 12, 13, 14, 15],\n [ 23, 24, 25, 26, 27],\n [ 6, 7, 8, 9, 10],\n [ 38, 39, 40, 41, 42],\n [ 25, 26, 27, 28, 29],\n [ 7, 8, 9, 10, 11]])\n\ntorch.Size([10])\ntensor([ 33, 26, 22, 39, 16, 28, 11, 43, 30, 12])\n```\n\n### Sizes\nYour sample_x should be of size `(batch_size, sequence_length)` or (10, 5) in this case and sample_y should just have one dimension: batch_size (10). \n\n### Values\n\nYou should also notice that the targets, sample_y, are the *next* value in the ordered test_text data. So, for an input sequence `[ 28, 29, 30, 31, 32]` that ends with the value `32`, the corresponding output should be `33`.",
"_____no_output_____"
]
],
[
[
"# test dataloader\n\ntest_text = range(50)\nt_loader = batch_data(test_text, sequence_length=5, batch_size=10)\n\ndata_iter = iter(t_loader)\nsample_x, sample_y = data_iter.next()\n\nprint(sample_x.shape)\nprint(sample_x)\nprint()\nprint(sample_y.shape)\nprint(sample_y)",
"torch.Size([10, 5])\ntensor([[ 0, 1, 2, 3, 4],\n [ 1, 2, 3, 4, 5],\n [ 2, 3, 4, 5, 6],\n [ 3, 4, 5, 6, 7],\n [ 4, 5, 6, 7, 8],\n [ 5, 6, 7, 8, 9],\n [ 6, 7, 8, 9, 10],\n [ 7, 8, 9, 10, 11],\n [ 8, 9, 10, 11, 12],\n [ 9, 10, 11, 12, 13]])\n\ntorch.Size([10])\ntensor([ 5, 6, 7, 8, 9, 10, 11, 12, 13, 14])\n"
]
],
[
[
"---\n## Build the Neural Network\nImplement an RNN using PyTorch's [Module class](http://pytorch.org/docs/master/nn.html#torch.nn.Module). You may choose to use a GRU or an LSTM. To complete the RNN, you'll have to implement the following functions for the class:\n - `__init__` - The initialize function. \n - `init_hidden` - The initialization function for an LSTM/GRU hidden state\n - `forward` - Forward propagation function.\n \nThe initialize function should create the layers of the neural network and save them to the class. The forward propagation function will use these layers to run forward propagation and generate an output and a hidden state.\n\n**The output of this model should be the *last* batch of word scores** after a complete sequence has been processed. That is, for each input sequence of words, we only want to output the word scores for a single, most likely, next word.\n\n### Hints\n\n1. Make sure to stack the outputs of the lstm to pass to your fully-connected layer, you can do this with `lstm_output = lstm_output.contiguous().view(-1, self.hidden_dim)`\n2. You can get the last batch of word scores by shaping the output of the final, fully-connected layer like so:\n\n```\n# reshape into (batch_size, seq_length, output_size)\noutput = output.view(batch_size, -1, self.output_size)\n# get last batch\nout = output[:, -1]\n```",
"_____no_output_____"
]
],
[
[
"import torch.nn as nn\n\nclass RNN(nn.Module):\n \n def __init__(self, vocab_size, output_size, embedding_dim, hidden_dim, n_layers, dropout=0.5):\n \"\"\"\n Initialize the PyTorch RNN Module\n :param vocab_size: The number of input dimensions of the neural network (the size of the vocabulary)\n :param output_size: The number of output dimensions of the neural network\n :param embedding_dim: The size of embeddings, should you choose to use them \n :param hidden_dim: The size of the hidden layer outputs\n :param dropout: dropout to add in between LSTM/GRU layers\n \"\"\"\n super(RNN, self).__init__()\n # TODO: Implement function\n \n # set class variables \n self.output_size = output_size \n self.n_layers = n_layers \n self.hidden_dim = hidden_dim\n \n # define model layers\n self.embedding = nn.Embedding(vocab_size, embedding_dim) \n self.lstm = nn.LSTM(embedding_dim, hidden_dim, n_layers, dropout=dropout, batch_first=True) \n self.fc = nn.Linear(hidden_dim, output_size)\n \n \n def forward(self, nn_input, hidden):\n \"\"\"\n Forward propagation of the neural network\n :param nn_input: The input to the neural network\n :param hidden: The hidden state \n :return: Two Tensors, the output of the neural network and the latest hidden state\n \"\"\"\n # TODO: Implement function \n batch_size = nn_input.size(0)\n \n embeds = self.embedding(nn_input)\n \n lstm_out, hidden = self.lstm(embeds, hidden)\n \n lstm_out = lstm_out.contiguous().view(-1, self.hidden_dim)\n \n out = self.fc(lstm_out)\n \n # reshape \n out = out.view(batch_size, -1, self.output_size)\n \n # find the last batch\n output = out[:, -1]\n \n # return one batch of output word scores and the hidden state\n\n return output, hidden\n \n def init_hidden(self, batch_size):\n '''\n Initialize the hidden state of an LSTM/GRU\n :param batch_size: The batch_size of the hidden state\n :return: hidden state of dims (n_layers, batch_size, hidden_dim)\n '''\n # Implement function\n \n # initialize hidden state with zero weights, and move to GPU if available\n \n weight = next(self.parameters()).data\n\n if (train_on_gpu):\n hidden = (weight.new(self.n_layers, batch_size, self.hidden_dim).zero_().cuda(),\n weight.new(self.n_layers, batch_size, self.hidden_dim).zero_().cuda())\n \n else:\n hidden = (weight.new(self.n_layers, batch_size, self.hidden_dim).zero_(),\n weight.new(self.n_layers, batch_size, self.hidden_dim).zero_())\n \n return hidden\n\n\"\"\"\nDON'T MODIFY ANYTHING IN THIS CELL THAT IS BELOW THIS LINE\n\"\"\"\ntests.test_rnn(RNN, train_on_gpu)",
"Tests Passed\n"
]
],
[
[
"### Define forward and backpropagation\n\nUse the RNN class you implemented to apply forward and back propagation. This function will be called, iteratively, in the training loop as follows:\n```\nloss = forward_back_prop(decoder, decoder_optimizer, criterion, inp, target)\n```\n\nAnd it should return the average loss over a batch and the hidden state returned by a call to `RNN(inp, hidden)`. Recall that you can get this loss by computing it, as usual, and calling `loss.item()`.\n\n**If a GPU is available, you should move your data to that GPU device, here.**",
"_____no_output_____"
]
],
[
[
"def forward_back_prop(rnn, optimizer, criterion, inp, target, hidden):\n \"\"\"\n Forward and backward propagation on the neural network\n :param decoder: The PyTorch Module that holds the neural network\n :param decoder_optimizer: The PyTorch optimizer for the neural network\n :param criterion: The PyTorch loss function\n :param inp: A batch of input to the neural network\n :param target: The target output for the batch of input\n :return: The loss and the latest hidden state Tensor\n \"\"\"\n \n # TODO: Implement Function\n \n # move data to GPU, if available\n if (train_on_gpu):\n inp = inp.cuda()\n target = target.cuda()\n \n # perform backpropagation and optimization\n hidden = tuple([each.data for each in hidden])\n \n rnn.zero_grad()\n \n output, hidden = rnn(inp, hidden)\n \n loss = criterion(output, target)\n \n loss.backward()\n \n nn.utils.clip_grad_norm_(rnn.parameters(), 5)\n\n optimizer.step()\n \n # return the loss over a batch and the hidden state produced by our model\n return loss.item(), hidden\n\n# Note that these tests aren't completely extensive.\n# they are here to act as general checks on the expected outputs of your functions\n\"\"\"\nDON'T MODIFY ANYTHING IN THIS CELL THAT IS BELOW THIS LINE\n\"\"\"\ntests.test_forward_back_prop(RNN, forward_back_prop, train_on_gpu)",
"Tests Passed\n"
]
],
[
[
"## Neural Network Training\n\nWith the structure of the network complete and data ready to be fed in the neural network, it's time to train it.\n\n### Train Loop\n\nThe training loop is implemented for you in the `train_decoder` function. This function will train the network over all the batches for the number of epochs given. The model progress will be shown every number of batches. This number is set with the `show_every_n_batches` parameter. You'll set this parameter along with other parameters in the next section.",
"_____no_output_____"
]
],
[
[
"\"\"\"\nDON'T MODIFY ANYTHING IN THIS CELL\n\"\"\"\n\ndef train_rnn(rnn, batch_size, optimizer, criterion, n_epochs, show_every_n_batches=100):\n batch_losses = []\n \n rnn.train()\n\n print(\"Training for %d epoch(s)...\" % n_epochs)\n for epoch_i in range(1, n_epochs + 1):\n \n # initialize hidden state\n hidden = rnn.init_hidden(batch_size)\n \n for batch_i, (inputs, labels) in enumerate(train_loader, 1):\n \n # make sure you iterate over completely full batches, only\n n_batches = len(train_loader.dataset)//batch_size\n if(batch_i > n_batches):\n break\n \n # forward, back prop\n loss, hidden = forward_back_prop(rnn, optimizer, criterion, inputs, labels, hidden) \n # record loss\n batch_losses.append(loss)\n\n # printing loss stats\n if batch_i % show_every_n_batches == 0:\n print('Epoch: {:>4}/{:<4} Loss: {}\\n'.format(\n epoch_i, n_epochs, np.average(batch_losses)))\n batch_losses = []\n\n # returns a trained rnn\n return rnn",
"_____no_output_____"
]
],
[
[
"### Hyperparameters\n\nSet and train the neural network with the following parameters:\n- Set `sequence_length` to the length of a sequence.\n- Set `batch_size` to the batch size.\n- Set `num_epochs` to the number of epochs to train for.\n- Set `learning_rate` to the learning rate for an Adam optimizer.\n- Set `vocab_size` to the number of uniqe tokens in our vocabulary.\n- Set `output_size` to the desired size of the output.\n- Set `embedding_dim` to the embedding dimension; smaller than the vocab_size.\n- Set `hidden_dim` to the hidden dimension of your RNN.\n- Set `n_layers` to the number of layers/cells in your RNN.\n- Set `show_every_n_batches` to the number of batches at which the neural network should print progress.\n\nIf the network isn't getting the desired results, tweak these parameters and/or the layers in the `RNN` class.",
"_____no_output_____"
]
],
[
[
"# Data params\n# Sequence Length\nsequence_length = 12 # of words in a sequence\n# Batch Size\nbatch_size = 120\n\n# data loader - do not change\ntrain_loader = batch_data(int_text, sequence_length, batch_size)",
"_____no_output_____"
],
[
"# Training parameters\n# Number of Epochs\nnum_epochs = 10\n# Learning Rate\nlearning_rate = 0.001\n\n# Model parameters\n# Vocab size\nvocab_size = len(vocab_to_int)\n# Output size\noutput_size = len(vocab_to_int)\n# Embedding Dimension\nembedding_dim = 300\n# Hidden Dimension\nhidden_dim = int(300*1.25)\n# Number of RNN Layers\nn_layers = 2\n\n# Show stats for every n number of batches\nshow_every_n_batches = 500",
"_____no_output_____"
]
],
[
[
"### Train\nIn the next cell, you'll train the neural network on the pre-processed data. If you have a hard time getting a good loss, you may consider changing your hyperparameters. In general, you may get better results with larger hidden and n_layer dimensions, but larger models take a longer time to train. \n> **You should aim for a loss less than 3.5.** \n\nYou should also experiment with different sequence lengths, which determine the size of the long range dependencies that a model can learn.",
"_____no_output_____"
]
],
[
[
"\"\"\"\nDON'T MODIFY ANYTHING IN THIS CELL\n\"\"\"\n\n# create model and move to gpu if available\nrnn = RNN(vocab_size, output_size, embedding_dim, hidden_dim, n_layers, dropout=0.5)\nif train_on_gpu:\n rnn.cuda()\n\n# defining loss and optimization functions for training\noptimizer = torch.optim.Adam(rnn.parameters(), lr=learning_rate)\ncriterion = nn.CrossEntropyLoss()\n\n# training the model\ntrained_rnn = train_rnn(rnn, batch_size, optimizer, criterion, num_epochs, show_every_n_batches)\n\n# saving the trained model\nhelper.save_model('./save/trained_rnn', trained_rnn)\nprint('Model Trained and Saved')",
"Training for 10 epoch(s)...\nEpoch: 1/10 Loss: 5.531735227108002\n\nEpoch: 1/10 Loss: 4.930219256401062\n\nEpoch: 1/10 Loss: 4.637746600151062\n\nEpoch: 1/10 Loss: 4.442910384654999\n\nEpoch: 1/10 Loss: 4.507025374412537\n\nEpoch: 1/10 Loss: 4.437873532295227\n\nEpoch: 1/10 Loss: 4.499480187416077\n\nEpoch: 1/10 Loss: 4.360066707611084\n\nEpoch: 1/10 Loss: 4.224316485881806\n\nEpoch: 1/10 Loss: 4.262131739616394\n\nEpoch: 1/10 Loss: 4.250785901069641\n\nEpoch: 1/10 Loss: 4.350858811378479\n\nEpoch: 1/10 Loss: 4.325847270488739\n\nEpoch: 1/10 Loss: 4.353909639358521\n\nEpoch: 2/10 Loss: 4.122715645664375\n\nEpoch: 2/10 Loss: 3.9956280279159544\n\nEpoch: 2/10 Loss: 3.8856767058372497\n\nEpoch: 2/10 Loss: 3.764372691631317\n\nEpoch: 2/10 Loss: 3.86486045217514\n\nEpoch: 2/10 Loss: 3.8547475366592407\n\nEpoch: 2/10 Loss: 3.9458832178115846\n\nEpoch: 2/10 Loss: 3.8158533701896666\n\nEpoch: 2/10 Loss: 3.730561679840088\n\nEpoch: 2/10 Loss: 3.7631667466163634\n\nEpoch: 2/10 Loss: 3.792806112766266\n\nEpoch: 2/10 Loss: 3.901373929977417\n\nEpoch: 2/10 Loss: 3.8545562386512757\n\nEpoch: 2/10 Loss: 3.8934123978614807\n\nEpoch: 3/10 Loss: 3.757648332837055\n\nEpoch: 3/10 Loss: 3.715178225517273\n\nEpoch: 3/10 Loss: 3.630136202812195\n\nEpoch: 3/10 Loss: 3.5242831320762633\n\nEpoch: 3/10 Loss: 3.625628924369812\n\nEpoch: 3/10 Loss: 3.6309379606246948\n\nEpoch: 3/10 Loss: 3.7150929403305053\n\nEpoch: 3/10 Loss: 3.5617436628341674\n\nEpoch: 3/10 Loss: 3.5180994987487795\n\nEpoch: 3/10 Loss: 3.524529664516449\n\nEpoch: 3/10 Loss: 3.5592564339637756\n\nEpoch: 3/10 Loss: 3.682766806125641\n\nEpoch: 3/10 Loss: 3.63344953250885\n\nEpoch: 3/10 Loss: 3.6614061670303344\n\nEpoch: 4/10 Loss: 3.569586784254444\n\nEpoch: 4/10 Loss: 3.5327431926727293\n\nEpoch: 4/10 Loss: 3.4778979787826536\n\nEpoch: 4/10 Loss: 3.3810313334465025\n\nEpoch: 4/10 Loss: 3.4490697503089907\n\nEpoch: 4/10 Loss: 3.4713255314826967\n\nEpoch: 4/10 Loss: 3.540807016849518\n\nEpoch: 4/10 Loss: 3.395219274520874\n\nEpoch: 4/10 Loss: 3.3618284215927123\n\nEpoch: 4/10 Loss: 3.380989155292511\n\nEpoch: 4/10 Loss: 3.3962616963386534\n\nEpoch: 4/10 Loss: 3.5119336886405943\n\nEpoch: 4/10 Loss: 3.5053564672470094\n\nEpoch: 4/10 Loss: 3.52675777053833\n\nEpoch: 5/10 Loss: 3.444213536519074\n\nEpoch: 5/10 Loss: 3.4076444568634034\n\nEpoch: 5/10 Loss: 3.3569597172737122\n\nEpoch: 5/10 Loss: 3.264707137107849\n\nEpoch: 5/10 Loss: 3.325695327758789\n\nEpoch: 5/10 Loss: 3.3434394330978394\n\nEpoch: 5/10 Loss: 3.4178655323982237\n\nEpoch: 5/10 Loss: 3.292145290374756\n\nEpoch: 5/10 Loss: 3.25900110912323\n\nEpoch: 5/10 Loss: 3.282059187412262\n\nEpoch: 5/10 Loss: 3.286310025691986\n\nEpoch: 5/10 Loss: 3.371444211959839\n\nEpoch: 5/10 Loss: 3.3803050670623778\n\nEpoch: 5/10 Loss: 3.4059303545951845\n\nEpoch: 6/10 Loss: 3.3510205145178626\n\nEpoch: 6/10 Loss: 3.3233260822296145\n\nEpoch: 6/10 Loss: 3.262583809375763\n\nEpoch: 6/10 Loss: 3.1777939085960387\n\nEpoch: 6/10 Loss: 3.2358165702819823\n\nEpoch: 6/10 Loss: 3.2441793150901796\n\nEpoch: 6/10 Loss: 3.323215190887451\n\nEpoch: 6/10 Loss: 3.2096805644035338\n\nEpoch: 6/10 Loss: 3.1801719818115233\n\nEpoch: 6/10 Loss: 3.198467743396759\n\nEpoch: 6/10 Loss: 3.1996535511016844\n\nEpoch: 6/10 Loss: 3.2810081453323363\n\nEpoch: 6/10 Loss: 3.292669029712677\n\nEpoch: 6/10 Loss: 3.3275886268615724\n\nEpoch: 7/10 Loss: 3.2740323561436364\n\nEpoch: 7/10 Loss: 3.2473365926742552\n\nEpoch: 7/10 Loss: 3.189321361064911\n\nEpoch: 7/10 Loss: 3.1124250736236574\n\nEpoch: 7/10 Loss: 3.1598252415657044\n\nEpoch: 7/10 Loss: 3.174737638950348\n\nEpoch: 7/10 Loss: 3.2507713837623595\n\nEpoch: 7/10 Loss: 3.133176600456238\n\nEpoch: 7/10 Loss: 3.1098085503578186\n\nEpoch: 7/10 Loss: 3.1263022136688234\n\nEpoch: 7/10 Loss: 3.1329917140007018\n\nEpoch: 7/10 Loss: 3.2054256014823914\n\nEpoch: 7/10 Loss: 3.2255016083717347\n\nEpoch: 7/10 Loss: 3.249888722896576\n\nEpoch: 8/10 Loss: 3.2023202670221034\n\nEpoch: 8/10 Loss: 3.1807839002609253\n\nEpoch: 8/10 Loss: 3.132005618095398\n\nEpoch: 8/10 Loss: 3.0564675722122194\n\nEpoch: 8/10 Loss: 3.101025879383087\n\nEpoch: 8/10 Loss: 3.1125088901519775\n\nEpoch: 8/10 Loss: 3.191727280139923\n\nEpoch: 8/10 Loss: 3.073734776496887\n\nEpoch: 8/10 Loss: 3.0565507707595825\n\nEpoch: 8/10 Loss: 3.068301407337189\n\nEpoch: 8/10 Loss: 3.0812396683692933\n\nEpoch: 8/10 Loss: 3.148022204875946\n\nEpoch: 8/10 Loss: 3.1773056478500368\n\nEpoch: 8/10 Loss: 3.1913066611289977\n\nEpoch: 9/10 Loss: 3.1471167175460604\n\nEpoch: 9/10 Loss: 3.133128029823303\n\nEpoch: 9/10 Loss: 3.085259078979492\n\nEpoch: 9/10 Loss: 3.009995768547058\n\nEpoch: 9/10 Loss: 3.0498082242012026\n\nEpoch: 9/10 Loss: 3.0640956010818483\n\nEpoch: 9/10 Loss: 3.144371497631073\n\nEpoch: 9/10 Loss: 3.022254427909851\n\nEpoch: 9/10 Loss: 3.0071170454025267\n\nEpoch: 9/10 Loss: 3.0216007103919984\n\nEpoch: 9/10 Loss: 3.0384934406280517\n\nEpoch: 9/10 Loss: 3.1074284529685974\n\nEpoch: 9/10 Loss: 3.1239990234375\n\nEpoch: 9/10 Loss: 3.136796194553375\n\nEpoch: 10/10 Loss: 3.0981430233099836\n\nEpoch: 10/10 Loss: 3.0887152523994446\n\nEpoch: 10/10 Loss: 3.0389931325912474\n\nEpoch: 10/10 Loss: 2.9724698853492737\n\nEpoch: 10/10 Loss: 3.003671471595764\n\nEpoch: 10/10 Loss: 3.021963978290558\n\nEpoch: 10/10 Loss: 3.099330397605896\n\nEpoch: 10/10 Loss: 2.9838244442939756\n\nEpoch: 10/10 Loss: 2.9682252025604248\n\nEpoch: 10/10 Loss: 2.9791079874038697\n\nEpoch: 10/10 Loss: 2.999862591743469\n\nEpoch: 10/10 Loss: 3.0582768750190734\n\nEpoch: 10/10 Loss: 3.0778299646377563\n\nEpoch: 10/10 Loss: 3.0915690803527833\n\n"
]
],
[
[
"### Question: How did you decide on your model hyperparameters? \nFor example, did you try different sequence_lengths and find that one size made the model converge faster? What about your hidden_dim and n_layers; how did you decide on those?",
"_____no_output_____"
],
[
"**Answer:** \n\nI trained the model with the following parameters:\n10 epochs\nlearning rate = 0.001\nembedding dim = 300\nhidden dim = 375\nnumber of layers = 2\nshow_every_n_batches = 2500\n\nand it gave a good loss: 2.96",
"_____no_output_____"
],
[
"---\n# Checkpoint\n\nAfter running the above training cell, your model will be saved by name, `trained_rnn`, and if you save your notebook progress, **you can pause here and come back to this code at another time**. You can resume your progress by running the next cell, which will load in our word:id dictionaries _and_ load in your saved model by name!",
"_____no_output_____"
]
],
[
[
"\"\"\"\nDON'T MODIFY ANYTHING IN THIS CELL\n\"\"\"\nimport torch\nimport helper\nimport problem_unittests as tests\n\n_, vocab_to_int, int_to_vocab, token_dict = helper.load_preprocess()\ntrained_rnn = helper.load_model('./save/trained_rnn')",
"_____no_output_____"
]
],
[
[
"## Generate TV Script\nWith the network trained and saved, you'll use it to generate a new, \"fake\" Seinfeld TV script in this section.\n\n### Generate Text\nTo generate the text, the network needs to start with a single word and repeat its predictions until it reaches a set length. You'll be using the `generate` function to do this. It takes a word id to start with, `prime_id`, and generates a set length of text, `predict_len`. Also note that it uses topk sampling to introduce some randomness in choosing the most likely next word, given an output set of word scores!",
"_____no_output_____"
]
],
[
[
"\"\"\"\nDON'T MODIFY ANYTHING IN THIS CELL THAT IS BELOW THIS LINE\n\"\"\"\nimport torch.nn.functional as F\n\ndef generate(rnn, prime_id, int_to_vocab, token_dict, pad_value, predict_len=100):\n \"\"\"\n Generate text using the neural network\n :param decoder: The PyTorch Module that holds the trained neural network\n :param prime_id: The word id to start the first prediction\n :param int_to_vocab: Dict of word id keys to word values\n :param token_dict: Dict of puncuation tokens keys to puncuation values\n :param pad_value: The value used to pad a sequence\n :param predict_len: The length of text to generate\n :return: The generated text\n \"\"\"\n rnn.eval()\n \n # create a sequence (batch_size=1) with the prime_id\n current_seq = np.full((1, sequence_length), pad_value)\n current_seq[-1][-1] = prime_id\n predicted = [int_to_vocab[prime_id]]\n \n for _ in range(predict_len):\n if train_on_gpu:\n current_seq = torch.LongTensor(current_seq).cuda()\n else:\n current_seq = torch.LongTensor(current_seq)\n \n # initialize the hidden state\n hidden = rnn.init_hidden(current_seq.size(0))\n \n # get the output of the rnn\n output, _ = rnn(current_seq, hidden)\n \n # get the next word probabilities\n p = F.softmax(output, dim=1).data\n if(train_on_gpu):\n p = p.cpu() # move to cpu\n \n # use top_k sampling to get the index of the next word\n top_k = 5\n p, top_i = p.topk(top_k)\n top_i = top_i.numpy().squeeze()\n \n # select the likely next word index with some element of randomness\n p = p.numpy().squeeze()\n word_i = np.random.choice(top_i, p=p/p.sum())\n \n # retrieve that word from the dictionary\n word = int_to_vocab[word_i]\n predicted.append(word) \n \n # the generated word becomes the next \"current sequence\" and the cycle can continue\n current_seq = np.roll(current_seq, -1, 1)\n current_seq[-1][-1] = word_i\n \n gen_sentences = ' '.join(predicted)\n \n # Replace punctuation tokens\n for key, token in token_dict.items():\n ending = ' ' if key in ['\\n', '(', '\"'] else ''\n gen_sentences = gen_sentences.replace(' ' + token.lower(), key)\n gen_sentences = gen_sentences.replace('\\n ', '\\n')\n gen_sentences = gen_sentences.replace('( ', '(')\n \n # return all the sentences\n return gen_sentences",
"_____no_output_____"
]
],
[
[
"### Generate a New Script\nIt's time to generate the text. Set `gen_length` to the length of TV script you want to generate and set `prime_word` to one of the following to start the prediction:\n- \"jerry\"\n- \"elaine\"\n- \"george\"\n- \"kramer\"\n\nYou can set the prime word to _any word_ in our dictionary, but it's best to start with a name for generating a TV script. (You can also start with any other names you find in the original text file!)",
"_____no_output_____"
]
],
[
[
"# run the cell multiple times to get different results!\ngen_length = 400 # modify the length to your preference\nprime_word = 'jerry' # name for starting the script\n\n\"\"\"\nDON'T MODIFY ANYTHING IN THIS CELL THAT IS BELOW THIS LINE\n\"\"\"\npad_word = helper.SPECIAL_WORDS['PADDING']\ngenerated_script = generate(trained_rnn, vocab_to_int[prime_word + ':'], int_to_vocab, token_dict, vocab_to_int[pad_word], gen_length)\nprint(generated_script)",
"/opt/conda/lib/python3.6/site-packages/ipykernel_launcher.py:40: UserWarning: RNN module weights are not part of single contiguous chunk of memory. This means they need to be compacted at every call, possibly greatly increasing memory usage. To compact weights again call flatten_parameters().\n"
]
],
[
[
"#### Save your favorite scripts\n\nOnce you have a script that you like (or find interesting), save it to a text file!",
"_____no_output_____"
]
],
[
[
"# save script to a text file\nf = open(\"generated_script_1.txt\",\"w\")\nf.write(generated_script)\nf.close()",
"_____no_output_____"
]
],
[
[
"# The TV Script is Not Perfect\nIt's ok if the TV script doesn't make perfect sense. It should look like alternating lines of dialogue, here is one such example of a few generated lines.\n\n### Example generated script\n\n>jerry: what about me?\n>\n>jerry: i don't have to wait.\n>\n>kramer:(to the sales table)\n>\n>elaine:(to jerry) hey, look at this, i'm a good doctor.\n>\n>newman:(to elaine) you think i have no idea of this...\n>\n>elaine: oh, you better take the phone, and he was a little nervous.\n>\n>kramer:(to the phone) hey, hey, jerry, i don't want to be a little bit.(to kramer and jerry) you can't.\n>\n>jerry: oh, yeah. i don't even know, i know.\n>\n>jerry:(to the phone) oh, i know.\n>\n>kramer:(laughing) you know...(to jerry) you don't know.\n\nYou can see that there are multiple characters that say (somewhat) complete sentences, but it doesn't have to be perfect! It takes quite a while to get good results, and often, you'll have to use a smaller vocabulary (and discard uncommon words), or get more data. The Seinfeld dataset is about 3.4 MB, which is big enough for our purposes; for script generation you'll want more than 1 MB of text, generally. \n\n# Submitting This Project\nWhen submitting this project, make sure to run all the cells before saving the notebook. Save the notebook file as \"dlnd_tv_script_generation.ipynb\" and save another copy as an HTML file by clicking \"File\" -> \"Download as..\"->\"html\". Include the \"helper.py\" and \"problem_unittests.py\" files in your submission. Once you download these files, compress them into one zip file for submission.",
"_____no_output_____"
]
]
] | [
"markdown",
"code",
"markdown",
"code",
"markdown",
"code",
"markdown",
"code",
"markdown",
"code",
"markdown",
"code",
"markdown",
"code",
"markdown",
"code",
"markdown",
"code",
"markdown",
"code",
"markdown",
"code",
"markdown",
"code",
"markdown",
"code",
"markdown",
"code",
"markdown",
"code",
"markdown",
"code",
"markdown",
"code",
"markdown",
"code",
"markdown"
] | [
[
"markdown"
],
[
"code"
],
[
"markdown"
],
[
"code"
],
[
"markdown"
],
[
"code"
],
[
"markdown"
],
[
"code"
],
[
"markdown"
],
[
"code"
],
[
"markdown"
],
[
"code"
],
[
"markdown"
],
[
"code"
],
[
"markdown"
],
[
"code"
],
[
"markdown"
],
[
"code"
],
[
"markdown"
],
[
"code"
],
[
"markdown"
],
[
"code"
],
[
"markdown"
],
[
"code"
],
[
"markdown"
],
[
"code",
"code"
],
[
"markdown"
],
[
"code"
],
[
"markdown",
"markdown",
"markdown"
],
[
"code"
],
[
"markdown"
],
[
"code"
],
[
"markdown"
],
[
"code"
],
[
"markdown"
],
[
"code"
],
[
"markdown"
]
] |
d08e9bee92b42d0f9927bc6bc0adc579cceb27f8 | 1,518 | ipynb | Jupyter Notebook | Simple exercises.ipynb | PatrickDeJonge/PythonProjects | 19794ae7794aef54673bd498c8405acb58ec7da5 | [
"MIT"
] | null | null | null | Simple exercises.ipynb | PatrickDeJonge/PythonProjects | 19794ae7794aef54673bd498c8405acb58ec7da5 | [
"MIT"
] | null | null | null | Simple exercises.ipynb | PatrickDeJonge/PythonProjects | 19794ae7794aef54673bd498c8405acb58ec7da5 | [
"MIT"
] | null | null | null | 19.973684 | 59 | 0.493412 | [
[
[
"#dice-rolling simulator\nfrom random import randint\nconfirm=1\nwhile confirm:\n print(randint(1,6))\n confirm=int(input(\"Again?\")) #accepts 1 or 0\nprint(\"Stopped\")",
"_____no_output_____"
],
[
"#guess the number\nfrom random import randint\nn=randint(1,9)\ninp=0\nprint(\"Guess a number between 0 and 10\")\nwhile(n!=inp):\n try:\n inp=int(input(\"\"))\n except ValueError:\n print(\"Not a number\")\n continue\n if(n!=inp):\n print(\"False\")\nprint(\"Correct\")",
"_____no_output_____"
]
]
] | [
"code"
] | [
[
"code",
"code"
]
] |
d08ec165f4964e344c95f4f34650b784d368749b | 1,506 | ipynb | Jupyter Notebook | app.ipynb | saimoom026/Loan-default-prediction-app | 6b494502d263c96077ac088a93fbbc7ab4b5ee16 | [
"MIT"
] | null | null | null | app.ipynb | saimoom026/Loan-default-prediction-app | 6b494502d263c96077ac088a93fbbc7ab4b5ee16 | [
"MIT"
] | null | null | null | app.ipynb | saimoom026/Loan-default-prediction-app | 6b494502d263c96077ac088a93fbbc7ab4b5ee16 | [
"MIT"
] | null | null | null | 22.818182 | 64 | 0.503984 | [
[
[
"import pandas as pd\nfrom flask import Flask, jsonify, request\nimport pickle\n\n# load model\nmodel = pickle.load(open('gbm_dep_class.pkl.pkl','rb'))\n\n# app\napp = Flask(__name__)\n\n# routes\[email protected]('/', methods=['POST'])\n\ndef predict():\n # get data\n data = request.get_json(force=True)\n\n # convert data into dataframe\n data.update((x, [y]) for x, y in data.items())\n data_df = pd.DataFrame.from_dict(data)\n\n # predictions\n result = model.predict(data_df)\n\n # send back to browser\n output = {'results': int(result[0])}\n\n # return data\n return jsonify(results=output)\n\nif __name__ == '__main__':\n app.run(port = 8888, debug=True)",
"_____no_output_____"
]
]
] | [
"code"
] | [
[
"code"
]
] |
d08ec3106e3dc47e62a53f098bdd0f4547f179e8 | 15,070 | ipynb | Jupyter Notebook | psets/set1/set1_prob3.ipynb | ichakraborty/CS155-iniproject | e149d82586a7c6b79a71a2e56213daa7dfacbff0 | [
"BSD-2-Clause"
] | 14 | 2021-01-05T06:54:16.000Z | 2022-01-24T23:49:01.000Z | psets/set1/set1_prob3.ipynb | ichakraborty/CS155-iniproject | e149d82586a7c6b79a71a2e56213daa7dfacbff0 | [
"BSD-2-Clause"
] | null | null | null | psets/set1/set1_prob3.ipynb | ichakraborty/CS155-iniproject | e149d82586a7c6b79a71a2e56213daa7dfacbff0 | [
"BSD-2-Clause"
] | 25 | 2021-01-06T19:15:00.000Z | 2022-01-10T14:31:15.000Z | 31.330561 | 295 | 0.486795 | [
[
[
"<a href=\"https://colab.research.google.com/github/lakigigar/Caltech-CS155-2021/blob/main/psets/set1/set1_prob3.ipynb\" target=\"_parent\"><img src=\"https://colab.research.google.com/assets/colab-badge.svg\" alt=\"Open In Colab\"/></a>",
"_____no_output_____"
],
[
"# Problem 3",
"_____no_output_____"
],
[
"Use this notebook to write your code for problem 3 by filling in the sections marked `# TODO` and running all cells.",
"_____no_output_____"
]
],
[
[
"import numpy as np\nimport matplotlib.pyplot as plt\nimport itertools\n\nimport urllib.request\nurllib.request.urlretrieve('https://raw.githubusercontent.com/lakigigar/Caltech-CS155-2021/main/psets/set1/perceptron_helper.py', 'perceptron_helper.py')\n\nfrom perceptron_helper import (\n predict,\n plot_data,\n boundary,\n plot_perceptron,\n)\n\n%matplotlib inline",
"_____no_output_____"
]
],
[
[
"## Implementation of Perceptron",
"_____no_output_____"
],
[
"First, we will implement the perceptron algorithm. Fill in the `update_perceptron()` function so that it finds a single misclassified point and updates the weights and bias accordingly. If no point exists, the weights and bias should not change.\n\nHint: You can use the `predict()` helper method, which labels a point 1 or -1 depending on the weights and bias.",
"_____no_output_____"
]
],
[
[
"def update_perceptron(X, Y, w, b):\n \"\"\"\n This method updates a perceptron model. Takes in the previous weights\n and returns weights after an update, which could be nothing.\n \n Inputs:\n X: A (N, D) shaped numpy array containing N D-dimensional points.\n Y: A (N, ) shaped numpy array containing the labels for the points.\n w: A (D, ) shaped numpy array containing the weight vector.\n b: A float containing the bias term.\n \n Output:\n next_w: A (D, ) shaped numpy array containing the next weight vector\n after updating on a single misclassified point, if one exists.\n next_b: The next float bias term after updating on a single\n misclassified point, if one exists.\n \"\"\"\n next_w, next_b = np.copy(w), np.copy(b)\n \n #==============================================\n # TODO: Implement update rule for perceptron.\n #===============================================\n \n return next_w, next_b",
"_____no_output_____"
]
],
[
[
"Next you will fill in the `run_perceptron()` method. The method performs single updates on a misclassified point until convergence, or max_iter updates are made. The function will return the final weights and bias. You should use the `update_perceptron()` method you implemented above.",
"_____no_output_____"
]
],
[
[
"def run_perceptron(X, Y, w, b, max_iter):\n \"\"\"\n This method runs the perceptron learning algorithm. Takes in initial weights\n and runs max_iter update iterations. Returns final weights and bias.\n \n Inputs:\n X: A (N, D) shaped numpy array containing N D-dimensional points.\n Y: A (N, ) shaped numpy array containing the labels for the points.\n w: A (D, ) shaped numpy array containing the initial weight vector.\n b: A float containing the initial bias term.\n max_iter: An int for the maximum number of updates evaluated.\n \n Output:\n w: A (D, ) shaped numpy array containing the final weight vector.\n b: The final float bias term.\n \"\"\"\n \n #============================================\n # TODO: Implement perceptron update loop.\n #=============================================\n\n return w, b",
"_____no_output_____"
]
],
[
[
"# Problem 3A",
"_____no_output_____"
],
[
"## Visualizing a Toy Dataset",
"_____no_output_____"
],
[
"We will begin by training our perceptron on a toy dataset of 3 points. The green points are labelled +1 and the red points are labelled -1. We use the helper function `plot_data()` to do so.",
"_____no_output_____"
]
],
[
[
"X = np.array([[ -3, -1], [0, 3], [1, -2]])\nY = np.array([ -1, 1, 1])",
"_____no_output_____"
],
[
"fig = plt.figure(figsize=(5,4))\nax = fig.gca(); ax.set_xlim(-4.1, 3.1); ax.set_ylim(-3.1, 4.1)\nplot_data(X, Y, ax)",
"_____no_output_____"
]
],
[
[
"## Running the Perceptron",
"_____no_output_____"
],
[
"Next, we will run the perceptron learning algorithm on this dataset. Update the code to show the weights and bias at each timestep and the misclassified point used in each update. ",
"_____no_output_____"
],
[
"Run the below code, and fill in the corresponding table in the set.",
"_____no_output_____"
]
],
[
[
"# Initialize weights and bias.\nweights = np.array([0.0, 1.0])\nbias = 0.0\n\nweights, bias = run_perceptron(X, Y, weights, bias, 16)\n\nprint()\nprint (\"final w = %s, final b = %.1f\" % (weights, bias))",
"_____no_output_____"
]
],
[
[
"## Visualizating the Perceptron",
"_____no_output_____"
],
[
"Getting all that information in table form isn't very informative. Let us visualize what the decision boundaries are at each timestep instead.",
"_____no_output_____"
],
[
"The helper functions `boundary()` and `plot_perceptron()` plot a decision boundary given a perceptron weights and bias. Note that the equation for the decision boundary is given by:\n\n$$w_1x_1 + w_2x_2 + b = 0.$$ \n\nUsing some algebra, we can obtain $x_2$ from $x_1$ to plot the boundary as a line. \n\n$$x_2 = \\frac{-w_1x_2 - b}{w_2}. $$",
"_____no_output_____"
],
[
"Below is a redefinition of the `run_perceptron()` method to visualize the points and decision boundaries at each timestep instead of printing. Fill in the method using your previous `run_perceptron()` method, and the above helper methods.\n\nHint: The axs element is a list of Axes, which are used as subplots for each timestep. You can do the following:\n```\nax = axs[i]\n```\nto get the plot correponding to $t = i$. You can then use ax.set_title() to title each subplot. You will want to use the `plot_data()` and `plot_perceptron()` helper methods.",
"_____no_output_____"
]
],
[
[
"def run_perceptron(X, Y, w, b, axs, max_iter):\n \"\"\"\n This method runs the perceptron learning algorithm. Takes in initial weights\n and runs max_iter update iterations. Returns final weights and bias.\n \n Inputs:\n X: A (N, D) shaped numpy array containing N D-dimensional points.\n Y: A (N, ) shaped numpy array containing the labels for the points.\n w: A (D, ) shaped numpy array containing the initial weight vector.\n b: A float containing the initial bias term.\n axs: A list of Axes that contain suplots for each timestep. \n max_iter: An int for the maximum number of updates evaluated.\n \n Output:\n The final weight and bias vectors.\n \"\"\"\n \n #============================================\n # TODO: Implement perceptron update loop.\n #=============================================\n\n return w, b",
"_____no_output_____"
]
],
[
[
"Run the below code to get a visualization of the perceptron algorithm. The red region are areas the perceptron thinks are negative examples.",
"_____no_output_____"
]
],
[
[
"# Initialize weights and bias.\nweights = np.array([0.0, 1.0])\nbias = 0.0\n\nf, ax_arr = plt.subplots(2, 2, sharex=True, sharey=True, figsize=(9,8))\naxs = list(itertools.chain.from_iterable(ax_arr))\nfor ax in axs:\n ax.set_xlim(-4.1, 3.1); ax.set_ylim(-3.1, 4.1)\n\nrun_perceptron(X, Y, weights, bias, axs, 4)\n\nf.tight_layout()",
"_____no_output_____"
]
],
[
[
"# Problem 3C",
"_____no_output_____"
],
[
"## Visualize a Non-linearly Separable Dataset.",
"_____no_output_____"
],
[
"We will now work on a dataset that cannot be linearly separated, namely one that is generated by the XOR function.",
"_____no_output_____"
]
],
[
[
"X = np.array([[0, 1], [1, 0], [0, 0], [1, 1]])\nY = np.array([1, 1, -1, -1])",
"_____no_output_____"
],
[
"fig = plt.figure(figsize=(5,4))\nax = fig.gca(); ax.set_xlim(-0.1, 1.1); ax.set_ylim(-0.1, 1.1)\nplot_data(X, Y, ax)",
"_____no_output_____"
]
],
[
[
"We will now run the perceptron algorithm on this dataset. We will limit the total timesteps this time, but you should see a pattern in the updates. Run the below code.",
"_____no_output_____"
]
],
[
[
"# Initialize weights and bias.\nweights = np.array([0.0, 1.0])\nbias = 0.0\n\nf, ax_arr = plt.subplots(4, 4, sharex=True, sharey=True, figsize=(9,8))\naxs = list(itertools.chain.from_iterable(ax_arr))\nfor ax in axs:\n ax.set_xlim(-0.1, 1.1); ax.set_ylim(-0.1, 1.1)\n \nrun_perceptron(X, Y, weights, bias, axs, 16)\n\nf.tight_layout()",
"_____no_output_____"
]
]
] | [
"markdown",
"code",
"markdown",
"code",
"markdown",
"code",
"markdown",
"code",
"markdown",
"code",
"markdown",
"code",
"markdown",
"code",
"markdown",
"code",
"markdown",
"code"
] | [
[
"markdown",
"markdown",
"markdown"
],
[
"code"
],
[
"markdown",
"markdown"
],
[
"code"
],
[
"markdown"
],
[
"code"
],
[
"markdown",
"markdown",
"markdown"
],
[
"code",
"code"
],
[
"markdown",
"markdown",
"markdown"
],
[
"code"
],
[
"markdown",
"markdown",
"markdown",
"markdown"
],
[
"code"
],
[
"markdown"
],
[
"code"
],
[
"markdown",
"markdown",
"markdown"
],
[
"code",
"code"
],
[
"markdown"
],
[
"code"
]
] |
d08ee23494d7364ee713b89f231e0db6dbe859fe | 12,585 | ipynb | Jupyter Notebook | ch3/relu.ipynb | tbi20/zerpdeep | fff14895e3291e548d23e0b66f2c9a3c38908365 | [
"MIT"
] | null | null | null | ch3/relu.ipynb | tbi20/zerpdeep | fff14895e3291e548d23e0b66f2c9a3c38908365 | [
"MIT"
] | null | null | null | ch3/relu.ipynb | tbi20/zerpdeep | fff14895e3291e548d23e0b66f2c9a3c38908365 | [
"MIT"
] | null | null | null | 128.418367 | 8,832 | 0.82789 | [
[
[
"# coding: utf-8\nimport numpy as np\nimport matplotlib.pylab as plt\n\n\ndef relu(x):\n return np.maximum(0, x)\n\nx = np.arange(-5.0, 5.0, 0.1)\ny = relu(x)\nprint(x)\nprint(y)\nplt.plot(x, y)\nplt.ylim(-1.0, 5.5)\nplt.show()\n",
"[ -5.00000000e+00 -4.90000000e+00 -4.80000000e+00 -4.70000000e+00\n -4.60000000e+00 -4.50000000e+00 -4.40000000e+00 -4.30000000e+00\n -4.20000000e+00 -4.10000000e+00 -4.00000000e+00 -3.90000000e+00\n -3.80000000e+00 -3.70000000e+00 -3.60000000e+00 -3.50000000e+00\n -3.40000000e+00 -3.30000000e+00 -3.20000000e+00 -3.10000000e+00\n -3.00000000e+00 -2.90000000e+00 -2.80000000e+00 -2.70000000e+00\n -2.60000000e+00 -2.50000000e+00 -2.40000000e+00 -2.30000000e+00\n -2.20000000e+00 -2.10000000e+00 -2.00000000e+00 -1.90000000e+00\n -1.80000000e+00 -1.70000000e+00 -1.60000000e+00 -1.50000000e+00\n -1.40000000e+00 -1.30000000e+00 -1.20000000e+00 -1.10000000e+00\n -1.00000000e+00 -9.00000000e-01 -8.00000000e-01 -7.00000000e-01\n -6.00000000e-01 -5.00000000e-01 -4.00000000e-01 -3.00000000e-01\n -2.00000000e-01 -1.00000000e-01 -1.77635684e-14 1.00000000e-01\n 2.00000000e-01 3.00000000e-01 4.00000000e-01 5.00000000e-01\n 6.00000000e-01 7.00000000e-01 8.00000000e-01 9.00000000e-01\n 1.00000000e+00 1.10000000e+00 1.20000000e+00 1.30000000e+00\n 1.40000000e+00 1.50000000e+00 1.60000000e+00 1.70000000e+00\n 1.80000000e+00 1.90000000e+00 2.00000000e+00 2.10000000e+00\n 2.20000000e+00 2.30000000e+00 2.40000000e+00 2.50000000e+00\n 2.60000000e+00 2.70000000e+00 2.80000000e+00 2.90000000e+00\n 3.00000000e+00 3.10000000e+00 3.20000000e+00 3.30000000e+00\n 3.40000000e+00 3.50000000e+00 3.60000000e+00 3.70000000e+00\n 3.80000000e+00 3.90000000e+00 4.00000000e+00 4.10000000e+00\n 4.20000000e+00 4.30000000e+00 4.40000000e+00 4.50000000e+00\n 4.60000000e+00 4.70000000e+00 4.80000000e+00 4.90000000e+00]\n[ 0. 0. 0. 0. 0. 0. 0. 0. 0. 0. 0. 0. 0. 0. 0.\n 0. 0. 0. 0. 0. 0. 0. 0. 0. 0. 0. 0. 0. 0. 0.\n 0. 0. 0. 0. 0. 0. 0. 0. 0. 0. 0. 0. 0. 0. 0.\n 0. 0. 0. 0. 0. 0. 0.1 0.2 0.3 0.4 0.5 0.6 0.7 0.8 0.9\n 1. 1.1 1.2 1.3 1.4 1.5 1.6 1.7 1.8 1.9 2. 2.1 2.2 2.3 2.4\n 2.5 2.6 2.7 2.8 2.9 3. 3.1 3.2 3.3 3.4 3.5 3.6 3.7 3.8 3.9\n 4. 4.1 4.2 4.3 4.4 4.5 4.6 4.7 4.8 4.9]\n"
]
]
] | [
"code"
] | [
[
"code"
]
] |
d08ef8e6c343a4e5b9a2c20d513f790dbf81e760 | 51,542 | ipynb | Jupyter Notebook | Pipeline_multiple_imputers_and_models.ipynb | chrismarkella/Kaggle-access-from-Google-Colab | 4b12a4383c6aceca1f1dbf48ea9c26c3ad6be8cd | [
"MIT"
] | null | null | null | Pipeline_multiple_imputers_and_models.ipynb | chrismarkella/Kaggle-access-from-Google-Colab | 4b12a4383c6aceca1f1dbf48ea9c26c3ad6be8cd | [
"MIT"
] | null | null | null | Pipeline_multiple_imputers_and_models.ipynb | chrismarkella/Kaggle-access-from-Google-Colab | 4b12a4383c6aceca1f1dbf48ea9c26c3ad6be8cd | [
"MIT"
] | null | null | null | 42.00652 | 879 | 0.367409 | [
[
[
"<a href=\"https://colab.research.google.com/github/chrismarkella/Kaggle-access-from-Google-Colab/blob/master/Pipeline_multiple_imputers_and_models.ipynb\" target=\"_parent\"><img src=\"https://colab.research.google.com/assets/colab-badge.svg\" alt=\"Open In Colab\"/></a>",
"_____no_output_____"
]
],
[
[
"!apt-get -qq install tree",
"_____no_output_____"
],
[
"import os\n\nimport numpy as np\nimport pandas as pd\n\nfrom getpass import getpass\n\nfrom sklearn.model_selection import train_test_split\nfrom sklearn.ensemble import RandomForestRegressor\nfrom sklearn.metrics import mean_absolute_error\n\nfrom sklearn.impute import SimpleImputer\nfrom sklearn.preprocessing import OneHotEncoder\n\nfrom sklearn.pipeline import Pipeline\nfrom sklearn.compose import ColumnTransformer",
"_____no_output_____"
],
[
"def access_kaggle():\n \"\"\"\n Access Kaggle from Google Colab.\n If the /root/.kaggle does not exist then prompt for\n the username and for the Kaggle API key.\n Creates the kaggle.json access file in the /root/.kaggle/ folder. \n \"\"\"\n KAGGLE_ROOT = os.path.join('/root', '.kaggle')\n KAGGLE_PATH = os.path.join(KAGGLE_ROOT, 'kaggle.json')\n\n if '.kaggle' not in os.listdir(path='/root'):\n user = getpass(prompt='Kaggle username: ')\n key = getpass(prompt='Kaggle API key: ')\n \n !mkdir $KAGGLE_ROOT\n !touch $KAGGLE_PATH\n !chmod 666 $KAGGLE_PATH\n with open(KAGGLE_PATH, mode='w') as f:\n f.write('{\"username\":\"%s\", \"key\":\"%s\"}' %(user, key))\n f.close()\n !chmod 600 $KAGGLE_PATH\n del user\n del key\n success_msg = \"Kaggle is successfully set up. Good to go.\"\n print(f'{success_msg}')\n\naccess_kaggle()\n",
"Kaggle username: ··········\nKaggle API key: ··········\nKaggle is successfully set up. Good to go.\n"
],
[
"!kaggle competitions download -c home-data-for-ml-course -p datasets/ml-course\n!tree -sh ./\n!cat -n datasets/ml-course/train.csv|head -2\ndf = pd.read_csv('datasets/ml-course/train.csv', sep=',', index_col=0)\n\ndf.columns = df.columns.map(lambda c: c.lower())\ndf.columns",
"Warning: Looks like you're using an outdated API Version, please consider updating (server 1.5.6 / client 1.5.4)\nDownloading sample_submission.csv.gz to datasets/ml-course\n 0% 0.00/15.3k [00:00<?, ?B/s]\n100% 15.3k/15.3k [00:00<00:00, 27.2MB/s]\nDownloading sample_submission.csv to datasets/ml-course\n 0% 0.00/31.2k [00:00<?, ?B/s]\n100% 31.2k/31.2k [00:00<00:00, 31.5MB/s]\nDownloading test.csv.gz to datasets/ml-course\n 0% 0.00/82.0k [00:00<?, ?B/s]\n100% 82.0k/82.0k [00:00<00:00, 26.2MB/s]\nDownloading test.csv to datasets/ml-course\n 0% 0.00/441k [00:00<?, ?B/s]\n100% 441k/441k [00:00<00:00, 62.1MB/s]\nDownloading train.csv to datasets/ml-course\n 0% 0.00/450k [00:00<?, ?B/s]\n100% 450k/450k [00:00<00:00, 63.0MB/s]\nDownloading data_description.txt to datasets/ml-course\n 0% 0.00/13.1k [00:00<?, ?B/s]\n100% 13.1k/13.1k [00:00<00:00, 11.4MB/s]\nDownloading train.csv.gz to datasets/ml-course\n 0% 0.00/89.2k [00:00<?, ?B/s]\n100% 89.2k/89.2k [00:00<00:00, 78.0MB/s]\n./\n├── [4.0K] datasets\n│ └── [4.0K] ml-course\n│ ├── [ 13K] data_description.txt\n│ ├── [ 31K] sample_submission.csv\n│ ├── [ 15K] sample_submission.csv.gz\n│ ├── [441K] test.csv\n│ ├── [ 82K] test.csv.gz\n│ ├── [450K] train.csv\n│ └── [ 89K] train.csv.gz\n└── [4.0K] sample_data\n ├── [1.7K] anscombe.json\n ├── [294K] california_housing_test.csv\n ├── [1.6M] california_housing_train.csv\n ├── [ 17M] mnist_test.csv\n ├── [ 35M] mnist_train_small.csv\n └── [ 930] README.md\n\n3 directories, 13 files\n 1\tId,MSSubClass,MSZoning,LotFrontage,LotArea,Street,Alley,LotShape,LandContour,Utilities,LotConfig,LandSlope,Neighborhood,Condition1,Condition2,BldgType,HouseStyle,OverallQual,OverallCond,YearBuilt,YearRemodAdd,RoofStyle,RoofMatl,Exterior1st,Exterior2nd,MasVnrType,MasVnrArea,ExterQual,ExterCond,Foundation,BsmtQual,BsmtCond,BsmtExposure,BsmtFinType1,BsmtFinSF1,BsmtFinType2,BsmtFinSF2,BsmtUnfSF,TotalBsmtSF,Heating,HeatingQC,CentralAir,Electrical,1stFlrSF,2ndFlrSF,LowQualFinSF,GrLivArea,BsmtFullBath,BsmtHalfBath,FullBath,HalfBath,BedroomAbvGr,KitchenAbvGr,KitchenQual,TotRmsAbvGrd,Functional,Fireplaces,FireplaceQu,GarageType,GarageYrBlt,GarageFinish,GarageCars,GarageArea,GarageQual,GarageCond,PavedDrive,WoodDeckSF,OpenPorchSF,EnclosedPorch,3SsnPorch,ScreenPorch,PoolArea,PoolQC,Fence,MiscFeature,MiscVal,MoSold,YrSold,SaleType,SaleCondition,SalePrice\n 2\t1,60,RL,65,8450,Pave,NA,Reg,Lvl,AllPub,Inside,Gtl,CollgCr,Norm,Norm,1Fam,2Story,7,5,2003,2003,Gable,CompShg,VinylSd,VinylSd,BrkFace,196,Gd,TA,PConc,Gd,TA,No,GLQ,706,Unf,0,150,856,GasA,Ex,Y,SBrkr,856,854,0,1710,1,0,2,1,3,1,Gd,8,Typ,0,NA,Attchd,2003,RFn,2,548,TA,TA,Y,0,61,0,0,0,0,NA,NA,NA,0,2,2008,WD,Normal,208500\n"
],
[
"df.info()",
"<class 'pandas.core.frame.DataFrame'>\nInt64Index: 1460 entries, 1 to 1460\nData columns (total 80 columns):\nmssubclass 1460 non-null int64\nmszoning 1460 non-null object\nlotfrontage 1201 non-null float64\nlotarea 1460 non-null int64\nstreet 1460 non-null object\nalley 91 non-null object\nlotshape 1460 non-null object\nlandcontour 1460 non-null object\nutilities 1460 non-null object\nlotconfig 1460 non-null object\nlandslope 1460 non-null object\nneighborhood 1460 non-null object\ncondition1 1460 non-null object\ncondition2 1460 non-null object\nbldgtype 1460 non-null object\nhousestyle 1460 non-null object\noverallqual 1460 non-null int64\noverallcond 1460 non-null int64\nyearbuilt 1460 non-null int64\nyearremodadd 1460 non-null int64\nroofstyle 1460 non-null object\nroofmatl 1460 non-null object\nexterior1st 1460 non-null object\nexterior2nd 1460 non-null object\nmasvnrtype 1452 non-null object\nmasvnrarea 1452 non-null float64\nexterqual 1460 non-null object\nextercond 1460 non-null object\nfoundation 1460 non-null object\nbsmtqual 1423 non-null object\nbsmtcond 1423 non-null object\nbsmtexposure 1422 non-null object\nbsmtfintype1 1423 non-null object\nbsmtfinsf1 1460 non-null int64\nbsmtfintype2 1422 non-null object\nbsmtfinsf2 1460 non-null int64\nbsmtunfsf 1460 non-null int64\ntotalbsmtsf 1460 non-null int64\nheating 1460 non-null object\nheatingqc 1460 non-null object\ncentralair 1460 non-null object\nelectrical 1459 non-null object\n1stflrsf 1460 non-null int64\n2ndflrsf 1460 non-null int64\nlowqualfinsf 1460 non-null int64\ngrlivarea 1460 non-null int64\nbsmtfullbath 1460 non-null int64\nbsmthalfbath 1460 non-null int64\nfullbath 1460 non-null int64\nhalfbath 1460 non-null int64\nbedroomabvgr 1460 non-null int64\nkitchenabvgr 1460 non-null int64\nkitchenqual 1460 non-null object\ntotrmsabvgrd 1460 non-null int64\nfunctional 1460 non-null object\nfireplaces 1460 non-null int64\nfireplacequ 770 non-null object\ngaragetype 1379 non-null object\ngarageyrblt 1379 non-null float64\ngaragefinish 1379 non-null object\ngaragecars 1460 non-null int64\ngaragearea 1460 non-null int64\ngaragequal 1379 non-null object\ngaragecond 1379 non-null object\npaveddrive 1460 non-null object\nwooddecksf 1460 non-null int64\nopenporchsf 1460 non-null int64\nenclosedporch 1460 non-null int64\n3ssnporch 1460 non-null int64\nscreenporch 1460 non-null int64\npoolarea 1460 non-null int64\npoolqc 7 non-null object\nfence 281 non-null object\nmiscfeature 54 non-null object\nmiscval 1460 non-null int64\nmosold 1460 non-null int64\nyrsold 1460 non-null int64\nsaletype 1460 non-null object\nsalecondition 1460 non-null object\nsaleprice 1460 non-null int64\ndtypes: float64(3), int64(34), object(43)\nmemory usage: 923.9+ KB\n"
],
[
"df.saleprice.isnull().sum()",
"_____no_output_____"
],
[
"y = df.saleprice\n\nX = df.drop(['saleprice'], axis='columns')",
"_____no_output_____"
],
[
"\ntrain_x_full, valid_x_full, train_y, valid_y = train_test_split(X, y, test_size=0.2, random_state=42)",
"_____no_output_____"
],
[
"numerical_columns = [col for col in train_x_full.columns if\n train_x_full[col].dtype in ['float64', 'int64']]\n\ncategorical_columns = [col for col in train_x_full.columns if\n train_x_full[col].dtype == 'object' and\n train_x_full[col].nunique() < 10]\n\nselected_columns = categorical_columns + numerical_columns\n\ntrain_x = train_x_full[selected_columns].copy()\nvalid_x = valid_x_full[selected_columns].copy()\n\ntrain_x.shape, valid_x.shape",
"_____no_output_____"
],
[
"train_x.head()",
"_____no_output_____"
],
[
"imputers = [\n ('imputer', SimpleImputer()),\n ('imputer_median', SimpleImputer(strategy='median')),\n ('imputer_most_frequent', SimpleImputer(strategy='most_frequent')),\n]\n\ntrees_in_the_forest = [5, 10, 20, 50]\n\nmodels = [RandomForestRegressor(n_estimators=N, random_state=42) for N in trees_in_the_forest]\n\nfor imputer_name, imputer in imputers:\n numerical_transformer = imputer\n\n categorical_transformer = Pipeline(steps=[\n ('imputer', SimpleImputer(strategy='most_frequent')),\n ('one_hot_encoder', OneHotEncoder(sparse=False, handle_unknown='ignore')),\n ])\n\n preprocessor = ColumnTransformer(\n transformers=[\n # (name , transformer , columns)\n ('num', numerical_transformer, numerical_columns),\n ('cat', categorical_transformer, categorical_columns),\n ]\n )\n print(f'{imputer_name} imputer:')\n print('-'*20)\n\n for model in models:\n pipe = Pipeline(\n steps=[\n ('preprocessor', preprocessor),\n ('model', model),\n ]\n )\n\n pipe.fit(train_x, train_y)\n preds = pipe.predict(valid_x)\n\n mae = mean_absolute_error(y_true=valid_y, y_pred=preds)\n print(f'{model}')\n print(f'---> MAE: {mae}')\n print()",
"imputer imputer:\n--------------------\nRandomForestRegressor(bootstrap=True, ccp_alpha=0.0, criterion='mse',\n max_depth=None, max_features='auto', max_leaf_nodes=None,\n max_samples=None, min_impurity_decrease=0.0,\n min_impurity_split=None, min_samples_leaf=1,\n min_samples_split=2, min_weight_fraction_leaf=0.0,\n n_estimators=5, n_jobs=None, oob_score=False,\n random_state=42, verbose=0, warm_start=False)\n---> MAE: 21061.74246575343\n\nRandomForestRegressor(bootstrap=True, ccp_alpha=0.0, criterion='mse',\n max_depth=None, max_features='auto', max_leaf_nodes=None,\n max_samples=None, min_impurity_decrease=0.0,\n min_impurity_split=None, min_samples_leaf=1,\n min_samples_split=2, min_weight_fraction_leaf=0.0,\n n_estimators=10, n_jobs=None, oob_score=False,\n random_state=42, verbose=0, warm_start=False)\n---> MAE: 19763.0897260274\n\nRandomForestRegressor(bootstrap=True, ccp_alpha=0.0, criterion='mse',\n max_depth=None, max_features='auto', max_leaf_nodes=None,\n max_samples=None, min_impurity_decrease=0.0,\n min_impurity_split=None, min_samples_leaf=1,\n min_samples_split=2, min_weight_fraction_leaf=0.0,\n n_estimators=20, n_jobs=None, oob_score=False,\n random_state=42, verbose=0, warm_start=False)\n---> MAE: 18705.513356164385\n\nRandomForestRegressor(bootstrap=True, ccp_alpha=0.0, criterion='mse',\n max_depth=None, max_features='auto', max_leaf_nodes=None,\n max_samples=None, min_impurity_decrease=0.0,\n min_impurity_split=None, min_samples_leaf=1,\n min_samples_split=2, min_weight_fraction_leaf=0.0,\n n_estimators=50, n_jobs=None, oob_score=False,\n random_state=42, verbose=0, warm_start=False)\n---> MAE: 17976.44712328767\n\nimputer_median imputer:\n--------------------\nRandomForestRegressor(bootstrap=True, ccp_alpha=0.0, criterion='mse',\n max_depth=None, max_features='auto', max_leaf_nodes=None,\n max_samples=None, min_impurity_decrease=0.0,\n min_impurity_split=None, min_samples_leaf=1,\n min_samples_split=2, min_weight_fraction_leaf=0.0,\n n_estimators=5, n_jobs=None, oob_score=False,\n random_state=42, verbose=0, warm_start=False)\n---> MAE: 20431.88219178082\n\nRandomForestRegressor(bootstrap=True, ccp_alpha=0.0, criterion='mse',\n max_depth=None, max_features='auto', max_leaf_nodes=None,\n max_samples=None, min_impurity_decrease=0.0,\n min_impurity_split=None, min_samples_leaf=1,\n min_samples_split=2, min_weight_fraction_leaf=0.0,\n n_estimators=10, n_jobs=None, oob_score=False,\n random_state=42, verbose=0, warm_start=False)\n---> MAE: 19872.100342465754\n\nRandomForestRegressor(bootstrap=True, ccp_alpha=0.0, criterion='mse',\n max_depth=None, max_features='auto', max_leaf_nodes=None,\n max_samples=None, min_impurity_decrease=0.0,\n min_impurity_split=None, min_samples_leaf=1,\n min_samples_split=2, min_weight_fraction_leaf=0.0,\n n_estimators=20, n_jobs=None, oob_score=False,\n random_state=42, verbose=0, warm_start=False)\n---> MAE: 18947.181335616435\n\nRandomForestRegressor(bootstrap=True, ccp_alpha=0.0, criterion='mse',\n max_depth=None, max_features='auto', max_leaf_nodes=None,\n max_samples=None, min_impurity_decrease=0.0,\n min_impurity_split=None, min_samples_leaf=1,\n min_samples_split=2, min_weight_fraction_leaf=0.0,\n n_estimators=50, n_jobs=None, oob_score=False,\n random_state=42, verbose=0, warm_start=False)\n---> MAE: 18198.937123287673\n\nimputer_most_frequent imputer:\n--------------------\nRandomForestRegressor(bootstrap=True, ccp_alpha=0.0, criterion='mse',\n max_depth=None, max_features='auto', max_leaf_nodes=None,\n max_samples=None, min_impurity_decrease=0.0,\n min_impurity_split=None, min_samples_leaf=1,\n min_samples_split=2, min_weight_fraction_leaf=0.0,\n n_estimators=5, n_jobs=None, oob_score=False,\n random_state=42, verbose=0, warm_start=False)\n---> MAE: 20626.355479452053\n\nRandomForestRegressor(bootstrap=True, ccp_alpha=0.0, criterion='mse',\n max_depth=None, max_features='auto', max_leaf_nodes=None,\n max_samples=None, min_impurity_decrease=0.0,\n min_impurity_split=None, min_samples_leaf=1,\n min_samples_split=2, min_weight_fraction_leaf=0.0,\n n_estimators=10, n_jobs=None, oob_score=False,\n random_state=42, verbose=0, warm_start=False)\n---> MAE: 19683.9698630137\n\nRandomForestRegressor(bootstrap=True, ccp_alpha=0.0, criterion='mse',\n max_depth=None, max_features='auto', max_leaf_nodes=None,\n max_samples=None, min_impurity_decrease=0.0,\n min_impurity_split=None, min_samples_leaf=1,\n min_samples_split=2, min_weight_fraction_leaf=0.0,\n n_estimators=20, n_jobs=None, oob_score=False,\n random_state=42, verbose=0, warm_start=False)\n---> MAE: 18550.526198630138\n\nRandomForestRegressor(bootstrap=True, ccp_alpha=0.0, criterion='mse',\n max_depth=None, max_features='auto', max_leaf_nodes=None,\n max_samples=None, min_impurity_decrease=0.0,\n min_impurity_split=None, min_samples_leaf=1,\n min_samples_split=2, min_weight_fraction_leaf=0.0,\n n_estimators=50, n_jobs=None, oob_score=False,\n random_state=42, verbose=0, warm_start=False)\n---> MAE: 18138.273904109592\n\n"
]
]
] | [
"markdown",
"code"
] | [
[
"markdown"
],
[
"code",
"code",
"code",
"code",
"code",
"code",
"code",
"code",
"code",
"code",
"code"
]
] |
d08efe96f28d735c4c038c7765a7a25574b88e4b | 110,492 | ipynb | Jupyter Notebook | docs/notebooks/synthesis_calibration.ipynb | vsuorant/casadocs | b0483ef1dcd25f198104d0eb6e0bfb173e2d8816 | [
"Apache-2.0"
] | null | null | null | docs/notebooks/synthesis_calibration.ipynb | vsuorant/casadocs | b0483ef1dcd25f198104d0eb6e0bfb173e2d8816 | [
"Apache-2.0"
] | null | null | null | docs/notebooks/synthesis_calibration.ipynb | vsuorant/casadocs | b0483ef1dcd25f198104d0eb6e0bfb173e2d8816 | [
"Apache-2.0"
] | null | null | null | 100.630237 | 2,037 | 0.682366 | [
[
[
"# Synthesis Calibration \n\nThis chapter explains how to calibrate interferometer data within the CASA task system. Calibration is the process of determining the net complex correction factors that must be applied to each visibility in order to make them as close as possible to what an idealized interferometer would measure, such that when the data is imaged an accurate picture of the sky is obtained. This is not an arbitrary process, and there is a philosophy behind the CASA calibration methodology. For the most part, calibration in CASA using the tasks is not too different than calibration in other packages such as AIPS or Miriad.\n\n\n\n",
"_____no_output_____"
],
[
"## Calibration tasks\n\n<div class=\"alert alert-warning\">\n**Alert:** The calibration table format changed in CASA 3.4. CASA 4.2 is the last version that will support the **caltabconvert** function that provides conversions from the pre-3.4 caltable format to the modern format; it will be removed for CASA 4.3. In general, it is best to recalculate calibration using CASA 3.4 or later.\n</div>\n\n<div class=\"alert alert-warning\">\n**Alert:** In CASA 4.2 the *gaincurve* and *opacity* parameters have been removed from all calibration tasks (as advertised in 4.1). These calibration types are supported via the gencal task.\n</div>\n\n<div class=\"alert alert-warning\">\n**Alert:** As part of continuing development of a more flexible and improved interface for specifying calibration for apply, a new parameter has been introduced in **applycal** and the solving tasks: *docallib*. This parameter toggles between use of the traditional calibration apply parameters ( *gaintable*, *gainfield*, *interp*, *spwmap*, and *calwt*), and a new *callib* parameter which currently provides access to the *experimental* Cal Library mechanism, wherein calibration instructions are stored in a file. The default remains *docallib=False* in CASA 4.5, and this reveals the traditional apply parameters which continue to work as always, and the remainder of this chapter is still written using *docallib=False*. Users interested in the Cal Library mechanism's flexibility are encouraged to try it and report any problems; see [here](cal_library_syntax.ipynb#cal-library-syntax \"Cal Library\") for information on how to use it, including how to convert traditional applycal to Cal Library format. Note also that **plotms** and **mstransform** now support use of the Cal Library to enable on-the-fly calibration when plotting and generating new MSs.\n</div>\n\nThe standard set of calibration solving tasks (to produce calibration tables) are:\n\n- **bandpass** \\-\\-- complex bandpass (B) calibration solving, including options for channel-binned or polynomial solutions\n- **gaincal** \\-\\-- complex gain (G,T) and delay (K) calibration solving, including options for time-binned or spline solutions\n- **polcal** \\-\\-- polarization calibration including leakage, cross-hand phase, and position angle\n- **blcal** \\-\\-- *baseline-based* complex gain or bandpass calibration\n\nThere are helper tasks to create, manipulate, and explore calibration tables:\n\n- **applycal** \\-\\-- Apply calculated calibration solutions\n- **clearcal** \\-\\-- Re-initialize the calibration for a visibility dataset\n- **fluxscale** \\-\\-- Bootstrap the flux density scale from standard calibration sources\n- **listcal** \\-\\-- List calibration solutions\n- **plotcal** \\-\\-- Plot calibration solutions\n- **plotbandpass** \\-\\-- Plot bandpass solutions\n- **setjy** \\-\\-- Compute model visibilities with the correct flux density for a specified source\n- **smoothcal** \\-\\-- Smooth calibration solutions derived from one or more sources\n- **calstat** \\-\\-- Statistics of calibration solutions\n- **gencal** \\-\\-- Create a calibration tables from metadata such as antenna position offsets, gaincurves and opacities\n- **wvrgcal** \\-\\-- Generate a gain table based on Water Vapor Radiometer data (for ALMA)\n- **uvcontsub** \\-\\-- Carry out uv-plane continuum fitting and subtraction\n\n\n",
"_____no_output_____"
],
[
"## The Calibration Process\n\nA work-flow diagram for CASA calibration of interferometry data is shown in the following figure. This should help you chart your course through the complex set of calibration steps. In the following sections, we will detail the steps themselves and explain how to run the necessary tasks and tools.\n\n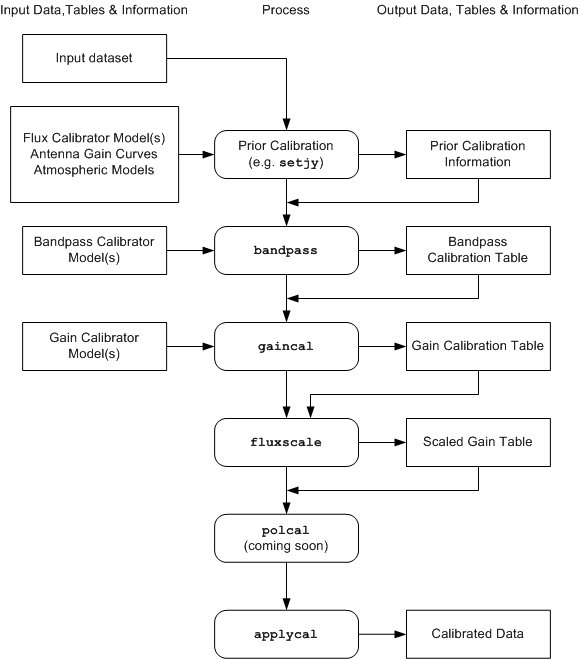{.image-inline}\n\n \n\n>Flow chart of synthesis calibration operations. Not shown are use of table manipulation and plotting tasks: **plotcal** and **smoothcal**\n \n\n \n\nThe process can be broken down into a number of discrete phases:\n\n- **Calibrator Model Visibility Specification** \\-\\-- set model visibilities for calibrators, either unit point source visibilities for calibrators with unknown flux density or structure (generally, sources used for calibrators are approximately point-like), or visibilities derived from *a priori* images and/or known or standard flux density values. Use the **setjy** task for calibrator flux densities and models.\n- **Prior Calibration** \\-\\-- set up previously known calibration quantities that need to be pre-applied, such antenna gain-elevation curves, atmospheric models, delays, and antenna position offsets. Use the **gencal** task for antenna position offsets, gaincurves, antenna efficiencies, opacity, and other prior calibrations\n- **Bandpass Calibration** \\-\\-- solve for the relative gain of the system over the frequency channels in the dataset (if needed), having pre-applied the prior calibration. Use the **bandpass** task\n- **Gain Calibration** \\-\\-- solve for the gain variations of the system as a function of time, having pre-applied the bandpass (if needed) and prior calibration. Use the **gaincal** task\n- **Polarization Calibration** \\-\\-- solve for polarization leakage terms and linear polarization position angle. Use the **polcal** task.\n- **Establish Flux Density Scale** \\-\\-- if only some of the calibrators have known flux densities, then rescale gain solutions and derive flux densities of secondary calibrators. Use the **fluxscale** task\n- **Smooth** \\-\\-- if necessary smooth calibration using the **smoothcal** task.\n- **Examine Calibration** \\-\\-- at any point, you can (and should) use **plotcal** and/or **listcal** to look at the calibration tables that you have created\n- **Apply Calibration to the Data** \\-\\-- Corrected data is formed using the **applycal** task, and can be undone using **clearcal**\n- **Post-Calibration Activities** \\-\\-- this includes the determination and subtraction of continuum signal from line data (**uvcontsub**), the splitting of data-sets into subsets (**split**, **mstransform**), and other operations (such as simple model-fitting: **uvmodelfit**).\n\nThe flow chart and the above list are in a suggested order. However, the actual order in which you will carry out these operations is somewhat fluid, and will be determined by the specific data-reduction use cases you are following. For example, you may need to obtain an initial gain calibration on your bandpass calibrator before moving to the bandpass calibration stage. Or perhaps the polarization leakage calibration will be known from prior service observations, and can be applied as a constituent of prior calibration.\n\n",
"_____no_output_____"
],
[
"## Calibration Philosophy\n\nCalibration is not an arbitrary process, and there is a methodology that has been developed to carry out synthesis calibration and an algebra to describe the various corruptions that data might be subject to: the Hamaker-Bregman-Sault Measurement Equation (ME), described [here.](casa-fundamentals.ipynb#measurement-equation \"Measurement Equation\") The user need not worry about the details of this mathematics as the CASA software does that for you. Anyway, it\\'s just matrix algebra, and your familiar scalar methods of calibration (such as in AIPS) are encompassed in this more general approach.\n\nThere are a number of \\`\\`physical\\'\\' components to calibration in CASA:\n\n- **data** \\-\\-- in the form of the MeasurementSet (MS). The MS includes a number of columns that can hold calibrated data, model information, and weights\n- **calibration tables** \\-\\-- these are in the form of standard CASA tables, and hold the calibration solutions (or parameterizations thereof)\n- **task parameters** \\-\\-- sometimes the calibration information is in the form of CASA task parameters that tell the calibration tasks to turn on or off various features, contain important values (such as flux densities), or list what should be done to the data.\n\nAt its most basic level, Calibration in CASA is the process of taking \\\"uncalibrated\\\" **data**, setting up the operation of calibration tasks using **task parameters**, solving for new **calibration tables**, and then applying the calibration tables to form \\\"calibrated\\\" **data**. Iteration can occur as necessary, e.g., to re-solve for an eariler **calibration table** using a better set of prior calibration, often with the aid of other non-calibration steps (e.g. imaging to generate improved source models for \\\"self-calibration\\\").\n\nThe calibration tables are the currency that is exchanged between the calibration tasks. The \\\"solver\\\" tasks (**gaincal**, **bandpass**, **blcal**, **polcal**) take in the MS (which may have a calibration model attached) and previous calibration tables, and will output an \\\"incremental\\\" calibration table (it is incremental to the previous calibration, if any). This table can then be smoothed using **smoothcal** if desired.\n\nThe final set of calibration tables represents the cumulative calibration and is what is applied to correct the data using **applycal**. It is important to keep track of each calibration table and its role relative to others. E.g., a provisional gain calibration solution will usually be obtained to optimize a bandpass calibration solve, but then be discarded in favor of a new gain calibration solution that will itself be optimized by use of the bandpass solution as a prior; the original gain calibration table should be discarded in this case. On the other hand, it is also permitted to generate a sequence of gain calibration tables, each *relative* to the last (and any other prior calibration used); in this case all relative tables should be carried forward through the process and included in the final **applycal**. It is the user\\'s responsibility to keep track of the role of and relationships between all calibration tables. Depending on the complexity of the observation, this can be a confusing business, and it will help if you adopt a consistent table naming scheme. In general, it is desirable to minimize the number of different calibration tables of a specific type, to keep the overall process as simple as possible and minimize the computational cost of applying them, but relative calibraition tables may sometimes be useful as an aid to understanding the origin and properties of the calibration effects. For example, it may be instructive to obtain a short time-scale gain calibraiton relative to a long time-scale one (e.g., obtained from a single scan) to approximatly separate electronic and atmospheric effects. Of course, calibration tables of different types are necessarily relative to each other (in the order in which they are solved).\n\n\n\n***\n\n\n\n",
"_____no_output_____"
],
[
"## Preparing for Calibration \n\nA description of the range of prior information necessary to solve for calibration\n\nThere is a range of *a priori* information that may need to be initialized or estimated before calibration solving is carried out. This includes establishing prior information about the data within the MS:\n\n- **weight initialization** \\-\\-- if desired, initialization of spectral weights, using **initweight** (by default, unchannelized weight accounting is used, and no special action is required)\n- **flux density models** \\-\\-- establish the flux density scale using \\\"standard\\\" calibrator sources, with models for resolved calibrators, using **setjy** as well as deriving various prior calibration quanitities using various modes of **gencal**\n- **gain curves** \\-\\-- the antenna gain-elevation dependence\n- **atmospheric optical depth** \\-\\-- attenuation of the signal by the atmosphere, including correcting for its elevation dependence\n- **antenna position errors** \\-\\-- offsets in the positions of antennas assumed during correlation\n- **ionosphere** \\-\\-- dispersive delay and Faraday effects arising from signal transmission through the magnetized plasma of the ionosphere\n- **switched power** (EVLA) \\-\\-- electronic gains monitored by the EVLA online system\n- **system temperature** (ALMA) \\-\\-- turn correlation coefficient into correlated flux density (necessary for some telescopes)\n- **generic cal factors** \\-\\-- antenna-based amp, phase, delay\n\nThese are all pre-determined effects and should be applied (if known) as priors when solving for other calibration terms, and included in the final application of all calibration. If unknown, then they will be solved for or subsumed in other calibration such as bandpass or gains.\n\nEach of these will now be described in turn.\n\n",
"_____no_output_____"
],
[
"### Weight Initialization\n\nSee the section on [data weights](data_weights.ipynb#data-weights) for a more complete description of weight accounting in CASA.\n\nCASA 4.3 introduced initial experimental support for spectral weights. At this time, this is mainly relevant to ALMA processing for which *spectral* $T_{sys}$ corrections, which faithfully reflect spectral sensitivity, are available. In most other cases, sensitivity is, to a very good approximation, channel-independent after bandpass calibration (and often also before), except perhaps at the very edges of spectral windows (and for which analytic expressions of the sensitivity loss are generally unavailable). Averaging of data with channel-dependent flagging which varies on sufficiently short timescales will also generate channel-dependent net weights (see **split** or **mstransform** for more details).\n\nBy default, CASA\\'s weight accounting scheme maintains unchannelized weight information that is appropriately updated when calibration is applied. In the case of spectral calibrations ($T_{sys}$ and bandpass), an appropriate spectral average is used for the weight update. This spectral average is formally correct for weight update by bandpass. For $T_{sys}$, traditional treatments used a single measurement per spectral window; ALMA has implemented spectral $T_{sys}$ to better track sensitivity as a function of channel, and so should benefit from *spectral* weight accounting as described here, especially where atmospheric emmission lines occur. If spectral weight accounting is desired, users must re-initialize the spectral weights using the **initweights** task:\n\n```\ninitweights(vis='mydata.ms', wtmode='nyq', dowtsp=True)\n```\n\nIn this task, the *wtmode* parameter controls the weight initialization convention. Usually, when initializing the weight information for a raw dataset, one should choose *wtmode='nyq'* so that the channel bandwidth and integration time information are used to initialize the weight information (as described [here](data_weights.ipynb#data-weights)). The *dowtsp* parameter controls whether or not (*True* or *False*) the spectral weights (the *WEIGHT_SPECTRUM* column) are initialized. The default is *dowtsp=False*, wherein only the non-spectral weights (the *WEIGHT* column) will be initialized. If the spectral weights have been initialized, then downstream processing that supports spectral weights will use and update them. In CASA 4.3 and later, this includes **applycal**, **clean**, and **split**/**mstransform**; use of spectral weights in calibration solving (e.g., **gaincal** and other solve tasks) is scheduled for the CASA 5.0 release.\n\nNote that **importasdm** currently initializes the *non-spectral* weights using channel bandwidth and integration time information (equivalent to using *dospwt=False* in the above example. In general, it only makes sense to run **initweights** on a raw dataset which has not yet been calibrated, and it should only be necessary if the filled weights are inappropriate, or if spectral weight accounting is desired in subsequent processing. It is usually *not* necessary to re-initialize the weight information when redoing calibration from scratch (the raw weight information is preserved in the *SIGMA*/*SIGMA_SPECTRUM* columns). (Re-)initializing the weight information for data that has already been calibrated (with *calwt=True*, presumably) is formally incorrect and is not recommended.\n\nWhen combining datasets from different epochs, it is generally preferable to have used the same version of CASA (most recent is best), and with the same weight information conventions and *calwt* settings in calibration tasks. Doing so will minimize the likelihood of arbitrary weight imbalances that might lead to net loss of sensitivity, and maximize the likelihood that *real* differences in per-epoch sensitivity (e.g., due to different weather conditions and instrumental setups) will be properly accounted for. Modern instruments support more variety in bandwidth and integration time settings, and so use of these parameters in weight initialization is preferred (c.f. use of simple unit weight initialization, which has often been the traditional practice).\n\n<div class=\"alert alert-warning\">\n**Alert:** Full and proper weight accounting for the EVLA formally depends on the veracity of the switched power calibration scheme. As of mid-2015, use of the EVLA switched power is not yet recommended for general use, and otherwise uniform weights are carried through the calibration process. As such, spectral weight accounting is not yet meaningful. Facilities for post-calibration estimation of spectral weights are rudimentarily supported in **statwt**.\n</div>\n\n",
"_____no_output_____"
],
[
"### Flux Density Models\n\nIt is necessary to be sure calibrators have appropriate models set for them before solving for calibration. Please see the task documentation for **setjy** and **ft** for more information on setting non-trivial model information in the MS. Also, information about setting models for flux density calibrators can be found [here](memo-series.ipynb#flux-calibrator-models---data-formats). Fields in the MS for which no model has been explicitly set will be rendered as unpolarized unit flux density (1 Jy) point sources in calibration solving.\n\n \n\n",
"_____no_output_____"
],
[
"### Antenna Gain-Elevation Curve Calibration\n\nLarge antennas (such as the 25-meter antennas used in the VLA and VLBA) have a forward gain and efficiency that changes with elevation. Gain curve calibration involves compensating for the effects of elevation on the amplitude of the received signals at each antenna. Antennas are not absolutely rigid, and so their effective collecting area and net surface accuracy vary with elevation as gravity deforms the surface. This calibration is especially important at higher frequencies where the deformations represent a greater fraction of the observing wavelength. By design, this effect is usually minimized (i.e., gain maximized) for elevations between 45 and 60 degrees, with the gain decreasing at higher and lower elevations. Gain curves are most often described as 2nd- or 3rd-order polynomials in zenith angle.\n\nGain curve calibration has been implemented in CASA for the modern VLA and old VLA (only), with gain curve polynomial coefficients available directly from the CASA data repository. To make gain curve and antenna efficiency corrections for VLA data, use **gencal**:\n\n```\ngencal(vis='mydata.ms', caltable='gaincurve.cal', caltype='gceff')\n```\n\nUse of *caltype=\\'gceff\\'* generates a caltable that corrects for both the elevation dependence and an antenna-based efficiency unit conversion that will render the data in units of *approximate* Jy (NB: this is generally not a good substitute for proper flux density calibration, using **fluxscale**!). Use of *caltype=\\'gc\\'* or *caltype=\\'eff\\'* can be used to introduce these corrections separately.\n\nThe resulting calibration table should then be used in all subsequent processing the requires the specification of prior calibration.\n\n<div class=\"alert alert-warning\">\n**Alert:** If you are not using VLA data, do not use gaincurve corrections. A general mechanism for incorporating gaincurve information for other arrays will be made available in future releases. The gain-curve information available for the VLA is time-dependent (on timescales of months to years, at least for the higher frequencies), and CASA will automatically select the date-appropriate gain curve information. Note, however, that the time-dependence was poorly sampled prior to 2001, and so gain curve corrections prior to this time should be considered with caution.\n</div>\n\n",
"_____no_output_____"
],
[
"### Atmospheric Optical Depth Correction\n\nThe troposphere is not completely transparent. At high radio frequencies ($>$15 GHz), water vapor and molecular oxygen begin to have a substantial effect on radio observations. According to the physics of radiative transmission, the effect is threefold. First, radio waves from astronomical sources are absorbed (and therefore attenuated) before reaching the antenna. Second, since a good absorber is also a good emitter, significant noise-like power will be added to the overall system noise, and thus further decreasing the *fraction* of correlated signal from astrophysical sources. Finally, the optical path length through the troposphere introduces a time-dependent phase error. In all cases, the effects become worse at lower elevations due to the increased air mass through which the antenna is looking. In CASA, the opacity correction described here compensates only for the first of these effects, tropospheric attenuation, using a plane-parallel approximation for the troposphere to estimate the elevation dependence. (Gain solutions solved for later will account for the other two effects.)\n\nTo make opacity corrections in CASA, an estimate of the zenith opacity is required (see observatory-specific chapters for how to measure zenith opacity). This is then supplied to the *caltype=\\'opac\\'* parameter in **gencal** which creates a calibration table that will introduce the elevation-dependent correction when applied in later operaions. E.g. for data with two spectral windows:\n\n```\ngencal(vis='mydatas.ms',\n caltable='opacity.cal',\n caltype='opac',\n spw='0,1',\n parameter=[0.0399,0.037])\n```\n\nIf you do not have an externally supplied value for *opacity*, for example from a VLA tip procedure, then you should either use an average value for the telescope, or omit this cal table and let your gain calibration compensate as best it can (e.g. that your calibrator is at the same elevation as your target at approximately the same time). As noted above, there are no facilities yet to estimate this from the data (e.g. by plotting $T_{sys}$ vs. elevation).\n\nThe resulting calibration table should then be used in all subsequent processing the requires the specification of prior calibration.\n\nBelow, we give instructions for determining opacity values for Jansky VLA data from weather statistics and VLA observations where tip-curve data is available. It is beyond the scope of this description to provide information for other telescopes.\n\n**Determining opacity corrections for *modern* VLA data**\n\nFor the VLA site, weather statistics and/or seasonal models that average over many years of weather statistics prove to be reasonable good ways to estimate the opacity at the time of the observations. The task **plotweather** calculates the opacity as a mix of both actual weather data and seasonal model. It can be run as follows:\n\n```\nmyTau=plotweather(vis='mydata.ms',doPlot=True)\n```\n\nThe task plots the weather statistics if *doPlot=T*, generating a plot shown in the figure below. The bottom panel displays the calculated opacities for the run as well as a seasonal model. An additional parameter, *seasonal_weight* can be adjusted to calculate the opacities as a function of the weather data alone (*seasonal_weight=0*), only the seasonal model (*seasonal_weight=1*), or a mix of the two (values between 0 and 1). Calculated opacities are shown in the logger output, one for each spectral window. Note that **plotweather** returns a python list of opacity values with length equal to the number of spectral windows in the MS, appropriate for use in **gencal**:\n\n```\ngencal(vis='mydata.ms', caltype='opac', spw='0,1', parameter=myTau) \n```\n\nNote that the *spw* parameter is used non-trivially and explicitly here to indicate that the list of opacity values corresponds to the specified spectral windows.\n\nThe resulting calibration table should then be used in all subsequent processing the requires the specification of prior calibration.\n\n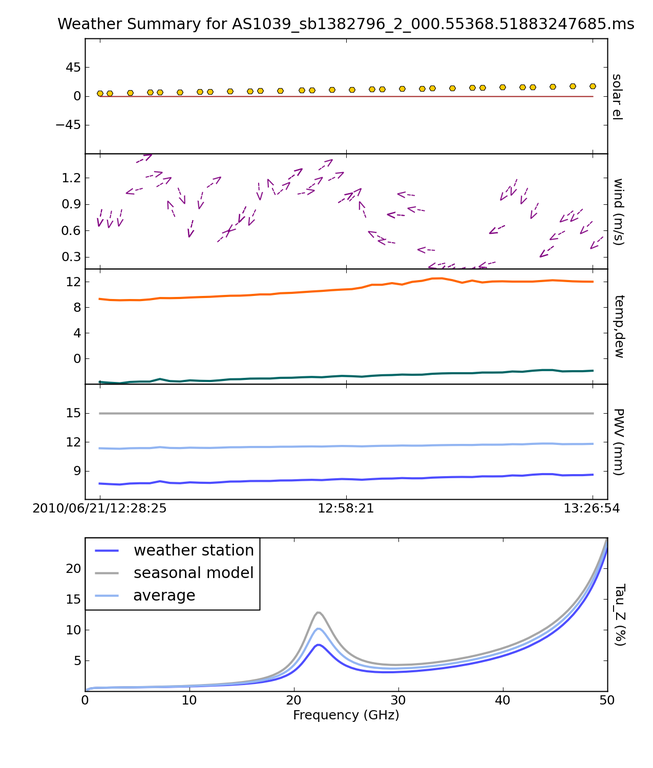{.image-inline}\n\n> The weather information for a MS as plotted by the task {\\\\tt plotweather}.}\n \n\n \n\n**Determining opacity corrections for historical VLA data**\n\nFor VLA data, zenith opacity can be measured at the frequency and during the time observations are made using a VLA tipping scan in the observe file. Historical tipping data are available [here.](http://www.vla.nrao.edu/astro/calib/tipper \"vla tips\") Choose a year, and click *Go* to get a list of all tipping scans that have been made for that year.\n\nIf a tipping scan was made for your observation, then select the appropriate file. Go to the bottom of the page and click on the button that says *Press here to continue*. The results of the tipping scan will be displayed. Go to the section called \\'Overall Fit Summary\\' to find the fit quality and the fitted zenith opacity in percent. If the zenith opacity is reported as 6%, then the actual zenith optical depth value is 0.060. Use this value in **gencal** as described above.\n\nIf there were no tipping scans made for your observation, then look for others made in the same band around the same time and weather conditions. If nothing is available here, then at K and Q bands you might consider using an average value (e.g. 6% in reasonable weather). See the VLA memo [here](http://www.vla.nrao.edu/memos/test/232/232.pdf \"ad hoc opacity\") for more on the atmospheric optical depth correction at the VLA, including plots of the seasonal variations.\n\n \n\n",
"_____no_output_____"
],
[
"### Antenna-position corrections\n\nWhen antennas are moved, residual errors in the geographical coordinates of the antenna will cause time-dependent delay errors in the correlated data. Normally, the observatory will solve for these offsets soon after the move and correct the correlator model, but sometimes science data is taken before the offsets are available, and thus the correction must be handled in post-processing. If the 3D position offsets for affected antennas are known, use **gencal** as follows:\n\n```\ngencal(vis='mydata.ms', caltable='antpos.cal', caltype='antpos', antenna='ea01',\n parameter=[0.01,0.02,0.005])\n```\n\nIn this execution, the position offset for antenna ea01 is \\[1cm,2cm,0.5cm\\] in an Earth-centered right-handed coordinate system with the first axis on the prime meridian and third axis coincident with the Earth\\'s axis. Corrections for multiple antennas can be specified by listing all affected antennas and extending the *parameter* list with as many offset triples as needed. \n\nIn general, it is difficut to know what position offsets to use, of course. For the VLA, **gencal** will look up the required offests automatically, simply by omitting the *antenna *and *parameter* arguments:\n\n```\ngencal(vis='mydata.ms', caltable='antpos.cal', caltype='antpos')\n```\n\nFor the historical VLA, the antenna position coordinate system was a local one translated from the Earth\\'s center and rotated to the VLA\\'s longitude. Use *caltype=\\'antposvla\\'* to force this coordiate system when processing old VLA data.\n\nThe resulting calibration table should then be used in all subsequent processing the requires the specification of prior calibration.\n\n \n\n",
"_____no_output_____"
],
[
"### Ionospheric corrections\n\nCASA 4.3 introduced initial support for on-axis ionospheric corrections, using time- and direction-dependent total electron content (TEC) information obtained from the internet. The correction includes the dispersive delay ($\\propto \\nu^{-1}$) delay and Faraday rotation ($\\propto \\nu^{-2}$) terms. These corrections are most relevant at observing frequencies less than $\\sim$5 GHz. When relevant, the ionosphere correction table should be generated at the beginning of a reduction along with other calibration priors (antenna position errors, gain curve, opacity, etc.), and carried through all subsequent calibration steps. Formally, the idea is that the ionospheric effects (as a function of time and on-axis direction) will be nominally accounted for by this calibration table, and thus not spuriously leak into gain and bandpass solves, etc. In practice, the quality of the ionospheric correction is limited by the relatively sparse sampling (in time and direction) of the available TEC information. Especially active ionospheric conditions may not be corrected very well. Also, direction-dependent (*within the instantaneous field-of-view*) ionosphere corrections are not yet supported. Various improvements are under study for future releases.\n\nTo generate the ionosphere correction table, first import a helper function from the casapy recipes repository:\n\n```\nCASA 5 and earlier:\n\nfrom recipes import tec_maps\n\nCASA 6.1.2+:\n\nfrom casatasks.private import tec_maps\n\n(CASA 6 prior to 6.1.2 did not support TEC corrections)\n\n```\n\nThen, generate a TEC surface image:\n\n```\ntec_maps.create(vis='mydata.ms',doplot=True,imname='iono')\n```\n\nThis function obtains TEC information for the observing date and location from [NASA\\'s CDDIS Archive of Space Geodesy Data](https://cddis.nasa.gov/Data_and_Derived_Products/GNSS/atmospheric_products.html), and generates a time-dependent CASA image containing this information. The string specified for *imname* is used as a prefix for two output images, with suffixes *.IGS_TEC.im* (the actual TEC image) and *.IGS_RMS_TEC.im* (a TEC error image). If *imname* is unspecified, the MS name (from *vis*) will be used as the prefix.\n\nThe quality of the retrieved TEC information for a specific date improves with time after the observing date as CDDIS\\'s ionospheric modelling improves, becoming optimal 1-2 weeks later. Both images can be viewed as a movie in the CASA task **imview**. If *doplot=T*, the above function will also produce a plot of the TEC as a function of time in a vertical direction over the observatory.\n\nFinally, to generate the ionosphere correction caltable, pass the *.IGS\\\\\\_TEC.im* image into **gencal**, using *caltype=\\'tecim\\'*:\n\n```\ngencal(vis='mydata.ms',caltable='tec.cal',caltype='tecim',infile='iono.IGS_TEC.im')\n```\n\nThis iterates through the dataset and samples the zenith angle-dependent projected line-of-sight TEC for all times in the observation, storing the result in a standard CASA caltable. Plotting this caltable will show how the TEC varies between observing directions for different fields and times, in particular how it changes as zenith angle changes, and including the nominal difference between science targets and calibrators.\n\nThis caltable should then be used as a prior in all subsequent calibration solves, and included in the final **applycal**.\n\nA few warnings:\n\n- The TEC information obtained from the web is relatively poorly sampled in time and direction, and so will not always describe the details of the ionospheric corruption, especially during active periods.\n- For instrumental polarization calibration, it is recommended that an *unpolarized* calibrator be used; polarized calibrators may not yield as accurate a solution since the ionospheric corrections are not yet used properly in the source polarization portion of the **polcal** solve.\n- TEC corrections are only validated for use with VLA data. For data from other (low-frequency) telescopes, TEC corrections are experimental - please use at your own discretion.\n\nSpecial thanks are due to Jason Kooi (UIowa) for his contributions to ionospheric corrections in CASA.\n\n \n\n",
"_____no_output_____"
],
[
"### Switched-power (EVLA)\n\nThe EVLA is equipped with noise diodes that synchronously inject a nominally constant and known power contribution appropriate for tracking electronic gain changes with time resolution as short as 1 second. The total power in both the ON and OFF states of the noise diodes is continuously recorded, enabling a gain calibration derived from their difference (as a fraction of the mean total power), and scaled by a the approximately known contributed power (nominally in K). Including this calibration will render the data in units of (nominal) K, and also calibrate the data weights to units of inverse K^2^. To generate a switched-power calibration table for use in subsequent processing, run **gencal** as follows:\n\n```\ngencal(vis='myVLAdata.ms',caltable='VLAswitchedpower.cal',caltype='evlagain') \n```\n\nThe resulting calibration table should then be used in all subsequent processing the requires the specification of prior calibration.\n\nTo ensure that the weight calibration by this table works correctly, it is important that the raw data weights are proprotional to integration time and channel bandwidth. This can be guaranteed by use of **initweights** as described above.\n\n \n\n",
"_____no_output_____"
],
[
"### System Temperature (ALMA)\n\nALMA routinely measures $T_{sys}$ while observing, and these measurements are used to reverse the online normalization of the correlation coefficients and render the data in units of nominal K. To generate a $T_{sys}$ calibration table, run **gencal** as follows:\n\n```\ngencal(vis='myALMAdata.ms',caltable='ALMAtsys.cal',caltype='tsys') \n```\n\nThe resulting calibration table should then be used in all subsequent processing the requires the specification of prior calibration.\n\n \n\n",
"_____no_output_____"
],
[
"### Miscellaneous ad hoc corrections\n\nThe **gencal** task supports generating ad hoc amp, phase, and delay corrections via appropriate settings of the *caltype* parameter. Currently, such factors must be constant in time (**gencal** has no mechanism for specifying multiple timestamps for parameters), but sometimes such corrections can be useful. See the general **gencal** task documenation for more information on this type of correction.\n\n\n***\n\n\n\n",
"_____no_output_____"
],
[
"## Virtual Model Visibilities \n\nThe tasks that generate model visibilities (**clean**, **tclean**, **ft**, and **setjy**) can either (in most cases) save the data in a MODEL_DATA column inside of the MeasurementSet (MS) or it can save it in a virtual one. In the latter case the model visibilities are generated on demand when it is requested and the data necessary to generate that is stored (usually the Fourier transform of the model images or a component list). More detailed descriptions of the structure of an MS can be found on the [CASA Fundamentals](casa-fundamentals.ipynb#casa-fundamentals) pages. \n\nThe tasks that can read and make use of the virtual model columns include calibration tasks, mstransform tasks (including **uvsubtraction**), **plotms**.\n\nAdvantages of virtual model column over the real one:\n\n- Speed of serving visibilities (in most cases because calculating models visibilities is faster than disk IO)\n- Disk space saving (a full size of the original data size is saved)\n\nWhen not to use virtual model\n\n- When working with time-dependent models (e.g. ephemerides sources) within setjy; please use ephemerides source models only with *usescratch=True* \n- Model image size is a significant fraction of the visibility data size (e.g large cube from a small data set). Virtual model column serving might be slower than real one\n- When the user wants to edit the model physically via the table tool for e.g\n- When using an FTMachine that does not support virtual model saving when imaging (AWProjectFT for e.g)\n\nAdditional Information\n\n- When both a physical model column exists along with a virtual model, then the virtual model is the one that gets served by tasks that uses the visbuffer (e.g calibration tasks)\n- Use **delmod*** *task to manage your MODEL_DATA column and virtual model\n- If model data is written for a subset of the MS (say the user used *field* , *spw* and/or *intent* selection in **tclean**) then the model visibilities will be served properly for the subset in question the other part of the MS will have 1 served for parallel hand visibilities and 0 for crosshand visibilities. So be careful when doing calibration or uvsub after writing model visibilities only for a subset of the MS (this applies to using the physical scratch column MODEL_DATA too)\n- The virtual model info is written in the SOURCE table of the MS usually (and in the main table if the SOURCE table does not exist)\n- FTMachines (or imaging gridding mode) supporting virtual model data are:\n - GridFT: Standard gridder (including mutiterm and multi fields or cube),\n - WProjectFT: widefield wterm (including mutiterm and multi fields or cube),\n - MosaicFT: mosaic imaging (including mutiterm or cube),\n - ComponentLists\n\n\n***\n\n\n\n",
"_____no_output_____"
],
[
"## Solve for Calibration \n\nThe **gaincal**, **bandpass**, **polcal**, and **blcal** tasks actually solve for the unknown calibration parameters from the visibility data obtained on calibrator sources, placing the results in a calibration table. They take as input an MS, and a number of parameters that specify any prior calibration tables to pre-apply before computing the solution, as well as parameters controlling the exact properties of the solving process.\n\nWe first discuss the parameters that are in common between many of the calibration tasks. Subsequent sub-sections will discuss the use of each of these solving task in more detail.\n\n**Common Calibration Solver Parameters**\n\nThere are a number of parameters that are in common between the calibration solver tasks.\n\n*Input/output*\n\nThe input MeasurementSet and output calibration table are controlled by the following parameters:\n\n```\nvis = '' #Name of input visibility file\ncaltable = '' #Name of output calibration table\n```\n\nThe MS name is specified in *vis*. If it is highlighted red in the inputs then it does not exist, and the task will not execute. Check the name and path in this case.\n\nThe output table name is specified in *caltable*. Be sure to give a unique name to the output table, or be careful. If the table exists, then what happens next will depend on the task and the values of other parameters. The task may not execute giving a warning that the table already exists, or will go ahead and overwrite the solutions in that table, or append them. Be careful.\n\n*Data selection*\n\nData selection is controlled by the following parameters:\n\n```\nfield = '' #field names or index of calibrators: ''==>all\nspw = '' #spectral window:channels: ''==>all\nintent = '' #Select observing intent\nselectdata = False #Other data selection parameters\n```\n\nField and spectral window selection are so often used, that we have made these standard parameters, *field* and *spw* respectively. Additionally, *intent* is also included as a standard parameter to enable selection by the scan intents that were specified when the observations were set up and executed. They typically describe what was intended with a specific scan, i.e. a flux or phase calibration, a bandpass, a pointing, an observation of your target, or something else or a combination. The format for the scan intents of your observations are listed in the logger when you run listobs. Minimum matching with wildcards will work, like \\*BANDPASS\\*. This is especially useful when multiple intents are attached to scans. Finally, observation is an identifier to distinguish between different observing runs, mainly used for ALMA.\n\nThe selectdata parameter expands, revealing a range of other selection sub-parameters:\n\n```\nselectdata = True #data selection parameters\n timerange = '' #time range (blank for all)\n uvrange = '' #uv range (blank for all)\n antenna = '' #antenna/baselines (blank for all)\n scan = '' #scan numbers (blank for all)\n correlation = '' #correlations (blank for all)\n array = '' #(sub)array numbers (blank for all)\n observation = '' #Select by observation ID(s)\n msselect = '' #MS selection (blank for all)\n```\n\nNote that if *selectdata=False* these parameters are not used when the task is executed, even if set non-trivially.\n\nAmong the most common *selectdata=True* parameters to use is uvrange, which can be used to exclude longer baselines if the calibrator is resolved, or short baselines if the calibrator contains extended flux not accounted for in the model. The rest of these parameters may be set according to information and values available in the listobs output. Note that all parameters are specified as strings, even if the values to be specified are numbers. See the section on [MS Selection](visibility_data_selection.ipynb#visibility-data-selection) for more details on the powerful syntax available for selecting data.\n\n*Prior calibration*\n\nCalibration tables that have already been determined can be arranged for apply before solving for the new table using the following parameters:\n\n```\ndocallib = False #Use traditional cal apply parameters\n gaintable = [] #Gain calibration table(s) to apply on the fly\n gainfield = [] #Select a subset of calibrators from gaintable(s)\n interp = [] #Interpolation mode (in time) to use for each gaintable\n spwmap = [] #Spectral windows combinations to form for gaintable(s)\n```\n\nThe *docallib* parameter is a toggle that can be used to select specification of prior calibration using the new \\\"cal library\\\" mechanism (*docallib=True*) which is described in greater detail [here.](cal_library_syntax.ipynb#cal-library-syntax)\n\nWhen *docalib=False*, the traditional CASA calibration apply sub-parameters will be used, as listed above.\n\n*gaintable*\n\nThe *gaintable* parameter takes a string or list of strings giving the names of one or more calibration tables to arrange for application. For example:\n\n```\n gaintable = ['ngc5921.bcal','ngc5921.gcal']\n```\n\nspecifies two tables, in this case bandpass and gain calibration tables respectively.\n\nThe *gainfield*, *interp*, and *spwmap* parameters key off *gaintable*, taking single values or lists, with an entries for each corresponding table in specified in *gaintable*. The caltables can be listed in *gaintable* in any order, without affecting the order in which they are applied to the data (for consistency, this is controlled internally according to the [Measurement Equation](casa-fundamentals.ipynb#measurement-equation) framework). If non-trivial settings are required for only a subset of the tables listed in *gaintable*, it can be convenient to specify these tables first in *gaintable*, include their qualifying settings first in the other paramters, and omit specifications for those tables not needing qualification (sensible defaults will be used for these).\n\n*gainfield*\n\nThe *gainfield* parameter specifies which field(s) from each respective *gaintable* to select for apply. This is a list, with each entry a string. The default for an entry means to use all in that table. For example, use\n\n```\n gaintable = ['ngc5921.bcal', 'ngc5921.gcal']\n gainfield = [ '1331+305', '1331+305,1445+099']\n```\n\nto specify selection of *1331+305* from *ngc5921.bcal* and fields *1331+305* and *1445+099* from *ngc5921.gcal*. Selection of this sort is only needed if avoiding other fields in these caltables is necessary. The field selection used here is the general MS Selection syntax.\n\nIn addition, *gainfield* supports a special value:\n\n```\n gainfield = [ 'nearest' ]\n```\n\nwhich selects the calibrator that is the spatially closest (in sky coordinates) to each of the selected MS fields specified in the *field* data selection parameter. Note that the nearest calibrator field is evaluated once per execution and is never dependent on time, spw or any other data meta-axis. This can be useful for running tasks with a number of different sources to be calibrated in a single run, and when this simple proximity notion is applicable. Note that the [cal library](cal_library_syntax.ipynb#cal-library-syntax) mechanism provides increased flexibility in this area.\n\n*interp*\n\nThe *interp* parameter chooses the interpolation scheme to be used when pre-applying the solution in the tables. Interpolation in both time and frequency (for channel-dependent calibrations) are supported. The choices are currently \\'*nearest\\'* and \\'*linear\\'* for time-dependent interpolation, and \\'*nearest\\'*, \\'*linear\\'*, \\'*cubic\\'*, and \\'*spline\\'* for frequency-dependent interpolation. Frequency-dependent interpolation is only relevant for channel-dependent calibration tables (like bandpass) that are undersampled in frequency relative to the data.\n\n- *\\'nearest\\' * just picks the entry nearest in time or freq to the visibility in question\n- \\'*linear*\\' calibrates each datum with calibration phases and amplitudes linearly interpolated from neighboring values in time or frequency. In the case of phase, this mode will assume that phase never jumps more than 180 degrees between neighboring points, and so phase changes exceeding this between calibration solutions cannot be corrected for. Also, solutions will not be extrapolated arbitrarily in time or frequency for data before the first solution or after the last solution; such data will be calibrated using nearest to avoid unreasonable extrapolations.\n- \\'*cubic*\\' (frequency axis only) forms a 3rd-order polynomial that passes through the nearest 4 calibration samples (separately in phase and amplitude)\n- \\'*spline*\\' (frequency axis only) forms a cubic spline that passes through the nearest 4 calibration samples (separately in phase and amplitude)\n\nThe time-dependent interp options can be appended with *\\'PD\\'* to enable a \\\"phase delay\\\" correction per spw for non-channel-dependent calibration type. For example: \\'*linearPD*\\'. This will adjust the time-dependent phase by the ratio of the data frequency and solution frequency and effect a time-dependent delay-like calibration over spws, and is most useful when distributing a single-spw\\'s solution (e.g.., as might be generated by *combine=\\'spw\\'* in **gaincal**) over many data spws, and when the the residual being calibrated is non-dispersively delay-like.\n\nThe time-dependent interp options can also be appended with *\\'perobs\\'* to enforce observation Id boundaries in the interpolation.\n\nThe frequency-dependent interp options can be appended with \\'flag\\' to enforce channel-dependent flagging by flagged bandpass channels (i.e., \\'*nearestflag*\\', \\'*linearflag*\\', \\'*cubicflag*\\', and \\'*splineflag*\\', rather than to automatically fill such channels in with interpolation (which is the default).\n\nFor each *gaintable*, specify the interpolation style in quotes, with the frequency-dependent interpolation style specified after a comma, if relevant. For example:\n\n```\n gaintable = ['ngc5921.bcal', 'ngc5921.gcal']\n gainfield = ['1331+305', ['1331+305','1445+099'] ]\n interp = ['linear,spline', 'linear']\n```\n\nuses linear interpolation on the time axis for both cal tables, and a cubic spline for interpolation of the frequency axis in the bandpass table.\n\n*spwmap*\n\nThe *spwmap* parameter is used to redistribute the calibration available in a caltable flexibly among spectral windows, thereby permitting correction of some spectral windows using calibration derived from others. The *spwmap* parameter takes a list or a list of lists of integers, with one list of integers for every caltable specified in *gaintable*. Each list is indexed by the MS spectral window ids, and the values indicate the calibration spectral windows to use for each MS spectral window. I.e., for each MS spw, *i*, the calibration spw *j* will be *j=spwmap\\[i\\]*. \n\nThe default for *spwmap* (an empty list per *gaintable*) means that MS spectral windows will be calibrated by solutions identified with the same index in the calibration table (i.e., by themselves, typically). Explicit specification of the default would be *spwmap=\\[0,1,2,3\\]*, for an MS with four spectral windows. Less trivially, for a caltable containing solutions derived from and labelled as spectral windows 0 and 1, these two cal spectral windows can be mapped to any of the MS spectral windows. E.g., (for a single *gaintable*):\n\n```\n spwmap=[0,1,1,0] #apply from cal spw=0 to MS spws 0,3 and from cal spw 1 to MS spws 1,2\n```\n\nFor multiple gaintables, use a lists of lists (one spwmap list per gaintable), e.g.,\n\n```\n gaintable = ['ngc5921.bcal', 'ngc5921.gcal']\n gainfield = ['1331+305', ['1331+305','1445+099'] ]\n interp = ['linear,spline', 'linear']\n spwmap = [ [0,1,1,0], [2,3,2,3] ]\n```\n\nwhich will use bandpass spws 0 and 1 for MS spws (0,3), and (1,2), respectively, and gain spws 2 and 3 for MS spws (0,2) and (1,3), respectively.\n\nAny spectral window mapping is mechanically valid, including using specific calibration spectral windows for more than one different MS spectral window (as above) and using alternate calibration even for spectral windows for which calibration is nominally available, as long as the mapped calibration spectral windows have calibration solutions available in the caltable. If a mapped calibration spectral window is absent from the caltable (and not merely flagged), an exception will occur.\n\nThe scientific meaningfulness of a non-trivial spwmap specification is the responsibility of the user; no internal checks are performed to attempt the scientific validity of the mapping. Usually, *spwmap* is used to distribute calibration such as Tsys, which may be measured in a wide low-resolution spectral window, to narrow high-resolution spectral windows that fall within the wide one. It is also used to distribute calibration derived from a **gaincal** solve which was performed using *combine=\\'spw\\'* (e.g., for increased SNR) to each of the spectral windows (and perhaps others) aggregated in the solve; in this case, it may be useful to consider using the *\\'PD\\'* (\\\"phase delay\\\") interpolation option described above, to account for the frequency ratios between each of the individual MS spectral windows and the aggregated calibration spectral window. \n\n\n**Absolute vs. Relative frequency in frequency-dependent interpolation**\n\nBy default, frequency-dependent solutions are interpolated for application in absolute sky frequency units. Thus, it is usually necessary to obtain **bandpass** solutions that cover the frequencies of all spectral windows that must be corrected. In this context, it is mechanically valid to use *spwmap* to transfer a **bandpass** solution from a wide, low-resolution spectral window to a narrow, higher-resolution spectral window that falls within the wide one in sky frequency space. On the other hand, if adequate data for a **bandpass** solution is unavailable for a specific spectral window, e.g., due to contamination by line emission or absorption (such as HI), or because of flagging, **bandpass** solutions from other spectral windows (i.e., at different sky frequencies) can be applied using *spwmap*. In this case, it is also necessary to add *\\'rel*\\' to the frequency interpolation string in the *interp* parameter, as this will force the interpolation to be calculated in relative frequency units. Specifically, the center frequency of the **bandpass** solution will be registered with the absolute center frequency of each of the MS spectral windows to which it is applied, thereby enabling relative frequency registration. The quality of such calibration transfer will depend, of course, on the uniformity of the hardware parameters and properties determining the bandpass shapes in the observing system\\--this is often appropriate over relatively narrow bandwidths in digital observing systems, as long as the setups are sufficiently similar (same sideband, same total spectral window bandwidth, etc., though note that the channelization need not be the same). Traditionally (e.g., at the VLA, for HI observations), **bandpass** solutions for this kind of calibration transfer have be solved by combining spectral windows on either side of the target spectral window (see the task documentation for [**bandpass**](../api/casatasks.rst) for more information on solving with *combine=\\'spw\\'*).\n\nFor example, to apply a bandpass solution from spectral window 0 (in a **bandpass** table called ngc5921.bcal) to MS spectral windows 0,1,2,3 with linear interpolation calculated in relative frequency units (and with frequency-dependent flagging respected):\n\n```\n gaintable = ['ngc5921.bcal']\n interp = ['nearest,linearflagrel']\n spwmap = [ [0,0,0,0] ]\n```\n\nWhen selecting channels for a **bandpass** solution that will be applied using *\\'rel\\'*, it is important to recognize that the selected channels will be centered on each of the \\_absolute\\_ centers of the MS spectral windows to which it will be applied. An asymmetric channel selection for the **bandpass** solve will cause an undesirable shift in the relative registration on apply. Avoid this by using symmetrical channel selection (or none) for the **bandpass** solve.\n\nAlso note that if relative frequency interpolation is required but *\\'rel\\'* is not used in *interp*, the interpolation mechanism currently assumes you want absolute frequency interpolation. If there is no overlap in absolute frequency, the result will be nearest (in channel) interpolation such that the calibration edge channel closest to the visibility data will be used to calibrate that data. \n\nFinally, please note that relative frequency interpolation is not yet available via the cal library.\n\n\n**Parallactic angle**\n\nThe *parang* parameter turns on the application of the antenna-based parallactic angle correction (P) in the Measurement Equation. This is necessary for polarization calibration and imaging, or for cases where the parallactic angles are different for geographically spaced antennas and it is desired that the ordinary calibration solves not absorb the inter-antenna parallactic angle phase. When dealing with only the parallel-hand data (e.g. RR, LL, XX, YY), and an unpolarized calibrator model for a co-located array (e.g. the VLA or ALMA), you can set *parang=False* and save some computational effort. Otherwise, set *parang=True* to apply this correction, especially if you are doing polarimetry.\n\n\n**Solving parameters**\n\nThe parameters controlling common aspects of the solving process itself are:\n\n```\nsolint = 'inf' #Solution interval: egs. 'inf', '60s' (see help)\ncombine = 'scan' #Data axes which to combine for solve (obs, scan,\n #spw, and/or field)\npreavg = -1.0 #Pre-averaging interval (sec) (rarely needed)\nrefant = '' #Reference antenna name(s)\nminblperant = 4 #Minimum baselines _per antenna_ required for solve\nminsnr = 3.0 #Reject solutions below this SNR\nsolnorm = False #Normalize solution amplitudes post-solve.\ncorrdepflags = False #Respect correlation-dependent flags\n```\n\nThe time and frequency (if relevant) solution interval is specified in *solint*. Optionally a frequency interval for each solutglobal-task-list.ipynb#task_bandpassion can be added after a comma, e.g. *solint=\\'60s,300Hz\\'*. Time units are in seconds unless specified differently. Frequency units can be either \\'*ch*\\' or \\'*Hz*\\' and only make sense for bandpass or frequency dependent polarization calibration. On the time axis, the special value \\'inf\\' specifies an infinite solution interval encompassing the entire dataset, while \\'int\\' specifies a solution every integration. Omitting the frequency-dependent solution interval will yield per-sample solutions on this axis. You can use time quanta in the string, e.g. *solint=\\'1min\\'* and *solint=\\'60s\\'* both specify solution intervals of one minute. Note that \\'*m*\\' is a unit of distance (meters); \\'*min*\\' must be used to specify minutes. The *solint* parameter interacts with *combine* to determine whether the solutions cross scan, field, or other meta-data boundaries.\n\nThe parameter controlling the scope of each solution is *combine*. For the default, *combine=''*, solutions will break at *obs*, *scan*, *field*, and *spw* boundaries. Specification of any of these in *combine* will extend the solutions over the specified boundaries (up to the solint). For example, *combine='spw'* will combine spectral windows together for solving, while *combine='scan'* will cross scans, and *combine='obs,scan'* will use data across different observation IDs and scans (usually, obs Ids consist of many scans, so it is not meaningful to combine obs Ids without also combining scans). Thus, to do scan-based solutions (single solution for each scan, per spw, field, etc.), set\n\n```\n solint = 'inf'\n combine = ''\n```\n\nTo obtain a single solution (per spw, per field) for an entire observation id (or the whole MS, if there is only one obsid), use:\n\n```\n solint = 'inf'\n combine = 'scan'\n```\n\nYou can specify multiple choices for combination by separating the axes with commas, e.g.:\n\n```\n combine = 'scan,spw'\n```\n\n<div class=\"alert alert-warning\">\nCare should be exercised when using *combine='spw'* in cases where multiple groups of concurrent spectral windows are observed as a function of time. Currently, only one aggregate spectral window can be generated in a single calibration solve execution, and the meta-information for this spectral window is calculated from all selected MS spectral windows. To avoid incorrect calibration meta-information, each spectral window group should be calibrated independently (also without using *append=True*). Additional flexibility in this area will be supported in a future version.\n</div>\n\nThe reference antenna is specified by the *refant* parameter. Ordinary MS Selection antenna selection syntax is used. Ideally, use of *refant* is useful to lock the solutions with time, effectively rotating (after solving) the phase of the gain solutions for all antennas such that the reference antennas phase remains constant at zero. In **gaincal** it is also possible to select a *refantmode*, either '*flex*' or \\'*strict*\\'. A list of antennas can be provided to this parameter and, for refantmode=\\'flex\\', if the first antenna is not present in the solutions (e.g., if it is flagged), the next antenna in the list will be used, etc. See the documentation for the **rerefant** task for more information. If the selected antenna drops out, the next antenna specified (or the next nearest antenna) will be substituted for ongoing continuity in time (at its current value) until the refant returns, usually at a new value (not zero), which will be kept fixed thenceforth. You can also run without a reference antenna, but in this case the solutions will formally float with time; in practice, the first antenna will be approximately constant near zero phase. It is usually prudent to select an antenna near the center of the array that is known to be particularly stable, as any gain jumps or wanders in the *refant* will be transferred to the other antenna solutions. Also, it is best to choose a reference antenna that never drops out, if possible.Setting a *preavg* time will let you average data over periods shorter than the solution interval first before solving on longer timescales. This is necessary only if the visibility data vary systematically within the solution interval in a manner independent of the solve-for factors (which are, by construction, considered constant within the solution interval), e.g., source linear polarization in **polcal**. Non-trivial use of *preavg* in such cases will avoid loss of SNR in the averaging within the solution interval. \n\nThe minimum signal-to-noise ratio allowed for an acceptable solution is specified in the *minsnr* parameter. Default is *minsnr=3*.\n\nThe *minblperant* parameter sets the minimum number of baselines to other antennas that must be preset for each antenna to be included in a solution. This enables control of the constraints that a solution will require for each antenna. \n\nThe *solnorm* parameter toggles on the option to normalize the solution after the solutions are obtained. The exact effect of this depends upon the type of solution (see **gaincal**, **bandpass**, and **blcal**). Not all tasks use this parameter.One should be aware when using *solnorm* that if this is done in the last stage of a chain of calibration, then the part of the calibration that is normalized away will be lost. It is best to use this in early stages (for example in a first bandpass calibration) so that later stages (such as final gain calibration) can absorb the lost normalization scaling. It is generally not strictly necessary to use *solnorm=True* at all, but it is sometimes helpful if you want to have a normalized bandpass for example.\n\nThe *corrdepflags* parameter controls how visibility vector flags are interpreted. If *corrdepflags=False* (the default), then when any one or more of the correlations in a single visibility vector is flagged (per spw, per baseline, per channel), it treats all available correlations in the single visibility vector as flagged, and therefore it is excluded from the calibration solve. This has been CASA\\'s traditional behavior (prior to CASA 5.7), in order to be conservative w.r.t. flags. If instead *corrdepFlags=True* (for CASA 5.7+), correlation-dependent flags will be respected exactly and precisely as set, such that any available unflagged correlations will be used in the solve for calibration factors. For the tasks currently supporting the *corrdepflags* parameter (*gaincal, bandpass, fringefit, accor*), this means any unflagged parallel-hand correlations will be used in solving, even if one or the other parallel-hand (or either of the cross-hands) is flagged. Note that the *polcal* task does not support *corrdepflags* since polarization calibration is generally more sensitive to correlation-dependence in the flagging in ways which may be ill-defined for partial flagging; this stricture may be relaxed in future for non-leakage solving modes. Most notably, this feature permits recovery and calibration of visibilities on baselines to antennas for which one polarization is entirely flagged, either because the antenna did not have that polarization at all (e.g., heterogeneous VLBI, where flagged visibilities are filled for missing correlations on single-polarization antennas), or one polarization was not working properly during the observation. \n\n**Appending calibration solutions to existing tables**\n\nThe *append* parameter, if set to *True*, will append the solutions from this run to existing solutions in *caltable*. Of course, this only matters if the table already exists. If *append=False* and the specified caltable exists, it will overwrite it (if the caltable is not open in another process).\n\n<div class=\"alert alert-warning\">\nThe *append* parameter should be used with care, especially when also using *combine* in non-trivial ways. E.g., calibration solves will currently refuse to append incongruent aggregate spectral windows (e.g., observations with more than one group of concurrent spectral windows) when using *combine='spw'*. This limitation arises from difficulty determining the appropriate spectral window fan-out on apply, and will be relaxed in a future version.\n</div>\n\n\n***\n\n\n\n\n",
"_____no_output_____"
],
[
"## Gain Calibration \n\nIn general, gain calibration includes solving for time- and frequency-dependent multiplicative calibration factors, usually in an antenna-based manner. CASA supports a range of options.\n\nNote that polarization calibration is described in detail in a [different section](synthesis_calibration.ipynb#polarization-calibration).\n\n \n- Frequency-dependent calibration: [bandpass](../api/casatasks.rst#calibration) \n\n Frequency-dependent calibration is discussed in the general task documentaion for [bandpass](../api/casatasks.rst#calibration).\n\n- Gain calibration: [gaincal](../api/casatasks.rst#calibration) \n\n Gain calibration is discussed in the general task documentation for [gaincal](../api/casatasks.rst#calibration).\n\n- Flux density scale calibration: [fluxscale](../api/casatasks.rst#calibration) \n\n Flux density scale calibration is discussed in the general task documentation for [fluxscale](../api/casatasks.rst#calibration).\n\n- Baseline-based (non-closing) calibration: [blcal](../api/casatasks.rst#calibration) \n\n Non-closing baseline-based calibration is disussed in the general task documentation for [blcal](../api/casatasks.rst#calibration).\n\n\n***\n\n\n\n\n\n\n\n",
"_____no_output_____"
],
[
"## Polarization Calibration \n\nInstrumental polarization calibration is necessary because the polarizing hardware in the receiving system will, in general, be impure and non-orthogonal at a level of at least a few percent. These instrumental polarization errors are antenna-based and generally assumed constant with time, but the algebra of their effects is more complicated than the simple \\~scalar multiplicative gain calibration. Also, the net gain calibration renders the data in an arbitrary cross-hand phase frame that must also be calibrated. The **polcal** task provides support for solving for instrumental polarization (poltype=\\'Df\\' and similar) and cross-hand phase (\\'Xf\\'). Here we separately describe the heuristics of solving for instrumental polarization for the circular and linear feed bases. \n\n",
"_____no_output_____"
],
[
"### Polarization Calibration in the Circular Basis\n\nFundamentally, with good ordinary gain and bandpass calibration already in hand, good polarization calibration must deliver both the instrumental polarization and position angle calibration. An unpolarized source can deliver only the first of these, but does not require parallactic angle coverage. A polarized source can only also deliver the position angle calibration if its polarization position angle is known a priori. Sources that are polarized, but with unknown polarization degree and angle, must always be observed with sufficient parallactic angle coverage (which enables solving for the source polarization), where \\\"sufficient\\\" is determined by SNR and the details of the solving mode.\n\nThese principles are stated assuming the instrumental polarization solution is solved using the \\\"linear approximation\\\" where cross-terms in more than a single product of the instrumental or source polarizations are ignored in the [Measurement Equation](casa-fundamentals.ipynb#measurement-equation). A more general non-linearized solution, with sufficient SNR, may enable some relaxation of the requirements indicated here, and modes supporting such an approach are currently under development.\n\nFor instrumental polarization calibration, there are 3 types of calibrator choice, listed in the following table:\n\n Cal Polarization PA Coverage Poln Model? *poltype* Result\n ------------------ ------------- ------------- ------------- -----------------------\n Zero any Q=U=0 *\\'Df\\'* D-terms only\n Unknown 2+ scans ignored *\\'Df+QU\\'* D-terms and Q,U\n Known, non-zero 2+ scans Set Q,U *\\'Df+X\\'* D-terms and Pos Angle\n\nNote that the parallactic angle ranges spanned by the scans in the modes that require this should be large enough to give good separation between the components of the solution. In practice, 60 degrees is a good target.\n\nEach of these solutions should be followed with a \\'Xf\\' solution on a source with known polarization position angle (and correct fractional Q+iU in the model).\n\nThe **polcal** task will solve for the \\'Df\\' or \\'Xf\\' terms using the model visibilities that are in the model attached to the MS. Calibration of the parallel hands must have already been obtained using **gaincal** and **bandpass** in order to align the amplitude and phase over time and frequency. This calibration must be supplied through the *gaintable* parameters, but any caltables to be used in **polcal** must agree (e.g. have been derived from) the data in the DATA column and the FT of the model. Thus, for example, one would not use the caltable produced by **fluxscale** as the rescaled amplitudes would no longer agree with the contents of the model.\n\nBe careful when using resolved calibrators for polarization calibration. A particular problem is if the structure in Q and U is offset from that in I. Use of a point model, or a resolved model for I but point models for Q and U, can lead to errors in the \\'Xf\\' calibration. Use of a *uvrange* will help here. The use of a full-Stokes model with the correct polarization is the only way to ensure a correct calibration if these offsets are large.\n\n**A note on channelized polarization calibration**\n\nWhen your data has more than one channel per spectral window, it is important to note that the calibrator polarization estimate currently assumes the source polarization signal is coherent across each spectral window. In this case, it is important to be sure there is no large cross-hand delay still present in your data. Unless the online system has accounted for cross-hand delays (typically intended, but not always achieved), the gain and bandpass calibration will only correct for parallel-hand delay residuals since the two polarizations are referenced independently. Good gain and bandpass calibration will typically leave a single cross-hand delay (and phase) residual from the reference antenna. Plots of cross-hand phases as a function of frequency for a strongly polarized source (i.e., that dominates the instrumental polarization) will show the cross-hand delay as a phase slope with frequency. This slope will be the same magnitude on all baselines, but with different sign in the two cross-hand correlations. This cross-hand delay can be estimated using the *gaintype=\\'KCROSS\\'* mode of **gaincal** (in this case, using the strongly polarized source *3C286*):\n\n```\n gaincal(vis='polcal_20080224.cband.all.ms',\n caltable='polcal.xdelcal',\n field='3C286',\n solint='inf', \n combine='scan',\n refant='VA15',\n smodel=[1.0,0.11,0.0,0.0], \n gaintype='KCROSS', \n gaintable=['polcal.gcal','polcal.bcal'])\n```\n\nNote that *smodel* is used to specify that *3C286* is polarized; it is not important to specify this polarization stokes parameters correctly in scale, as only the delay will be solved for (not any absolute position angle or amplitude scaling). The resulting solution should be carried forward and applied along with the gain (.gcal) and bandpass (.bcal) solutions in subsequent polarization calibration steps.\n\n**Circular Basis Example**\n\nIn the following example, we have a MS called *polcal_20080224.cband.all.ms* for which we already have bandpass, gain and cross-hand delay solutions. An instrumental polarization calibrator with unknown linear polarization has been observed. We solve for the instrumental polarization and source linear polarization with **polcal** using *poltype=\\'Df+QU\\'* as follows:\n\n```\npolcal(vis= 'polcal_20080224.cband.all.ms',\n caltable='polcal.pcal',\n field='2202+422', \n solint='inf', \n combine='scan',\n preavg=300.0, \n refant='VA15', \n poltype='Df+QU', \n gaintable=['polcal.gcal','polcal.bcal','polcal.xdelcal])\n```\n\nThis run of **polcal** assumes that the model stored in the MS for *2202+422* is the one that was used to obtain the net gain calibration stored in *polcal.gcal* (i.e., we have not substituted a fluxscale result, which would create an inconsistent scale). \n\nAlternatively, if we have an instrumental polarization calibrator that we know is unpolarized, we run polcal with poltype=\\'Df\\':\n\n```\npolcal(vis='polcal_20080224.cband.all.ms',\n caltable='polcal.pcal',\n field='0319+415',\n refant='VA15', \n poltype='Df', \n gaintable=['polcal.gcal','polcal.bcal','polcal.xdelcal])\n```\n\nIn general, if there is more than one calibrator suitable for instrumental polarization calibration, it is useful to obtain a solution from each of them, and compare results. The instrumental polarization should not vary with field, of course. Note that it is not yet possible to effectively use *combine=\\'field\\'* for instrumental polarization calibration solves with **polcal**, unless the prior models for all fields are set to the correct apparent linear polarization for each.\n\nHaving obtained the instrumental polarization calibration, we solve for the cross-hand phase using the flux density calibrator (for which the instrinsic linear polarization is known):\n\n```\npolcal(vis='polcal_20080224.cband.all.ms',\n caltable= 'polcal.polx',\n field='0137+331',\n refant='VA15', \n poltype='Xf',\n smodel=[1.0,-0.0348,-0.0217,0.0], #the fractional Stokes for 0137+331 (3C48)\n gaintable=['polcal.gcal','polcal.bcal','polcal.xdelcal','polcal.pcal'])\n```\n\nNote that the correct fractional polarization has been specified for *0137+331*. It is not necessary to use the correct absolute total and linearly polarized flux densities here, since the Xf calibration is entirely phase-like.\n\n \n\n",
"_____no_output_____"
],
[
"### Polarization Calibration in the Linear Feed Basis\n\nCASA now supports instrumental polarization calibration for the linear feed basis at a level that is practical for the general user. Some details remain to be implemented with full flexibility, and much of what follows will be streamlined in future releases.\n\nCalibrating the instrumental polarization for the linear feed basis is somewhat more complicated than the circular feed basis because the polarization effects (source and instrument) appear in all four correlations at first or zeroth order (whereas for circular feeds, the polarization information only enters the parallel hand correlations at second order). As a result, e.g., the time-dependent gain calibration will be distorted by any non-zero source polarization, and some degree of iteration will be required to isolate the gain calibration if the source polarization is not initially known. These complications can actually be used to advantage in solving for the instrumental calibration; in can be shown, for example, that a significantly linearly polarized calibrator enables a better instrumental polarization solution than an unpolarized calibrator.\n\nIn the following example, we show the processing steps for calibrating the instrumental polarization using a strongly (\\>5%) polarized point-source calibrator (which is also the time-dependent gain calibrator) that has been observed over a range of parallactic angle (a single scan is not sufficient). We assume that we have calibrated the gain, bandpass, and cross-hand delay as described [elsewhere](synthesis_calibration.ipynb#gain-calibration), and that the gain calibration was obtained assuming the calibrator was unpolarized.\n\n**Linear Basis Example**\n\nFirst, we import some utility functions from the CASA recipes area:\n\n```\nfrom recipes.almapolhelpers import *\n```\n\n \n\nOur MS in this example is called *polcal_linfeed.ms*. We begin by assuming we already have a bandpass calibration result (obtained by conventional means) stored in *polcal.bcal*. We first solve for a time-dependent gain solution on the instrumental polarization calibrator, which we expect to be significantly polarized, but for which we do not yet have a polarization model:\n\n```\ngaincal(vis='polcal_linfeed.ms',\n caltable='polcal.gcal', \n field='1', #the instrumental polarization calibrator\n solint='int', \n smodel=[1,0,0,0], #assume zero polarization\n gaintype='G', \n gaintable=['polcal.bcal'],\n parang=T) #so source poln properly rotated\n```\n\nSince the gain calibrator was assumed unpolarized, the time-dependent gain solutions contain information about the source polarization. This can be seen by plotting the amp vs. time for this cal table using *poln=\\'/\\'.* The antenna-based polarization amplitude ratios will reveal the sinusoidal (in parallactic angle) function of the source polarization. Run the utility method **qufromgain** to extract the apparent source polarization estimates for each spw:\n\n```\nqu=qufromgain('polcal.gcal')\n```\n\nThe source polarization reported for all spws should be reasonably consistent. This estimate is not as good as can be obtained from the cross-hands (see below) since it relies on the gain amplitude polarization ratio being stable which may not be precisely true. However, this estimate will be useful in resolving an ambiguity that occurs in the cross-hand estimates.\n\nNext we estimate both the XY-phase offset and source polarization from the cross-hands. The XY-phase offset is a spectral phase-only bandpass relating the X and Y systems of the reference antenna. If the XY-phase is solved for in a channel-dependent manner (as below), it is strictly not necessary to have solved for the cross-hand delay as described above, but it does not hurt, as it allows reasonably coherent channel averages for data examination (we assume below that we have obtained the cross-hand delay solution at this stage). The source polarization occurs in the cross-hands as a sinusoidal function of parallactic angle that is common to both cross-hands on all baselines (for a point-source). If the XY-phase bandpass is uniformly zero, then the source linear polarization function will occur entirely in the real part of the cross-hand visibilities. Non-zero XY-phase has the effect of rotating the source linear polarization signature partially into the imaginary part, where circular (and instrumental) polarization occur (cf. the circular feed basis where the cross-hand phase merely rotates the position angle of linear polarization). The following **gaincal** solve averages all baselines together and first solves for a channelized XY-phase (the slope of the source polarization function in the complex plane in each channel), then corrects the slope and solves for a channel-averaged source polarization. This calibration is obtained using *gaintype=\\'XYf+QU\\'* in **gaincal**:\n\n```\ngaincal(vis='polcal_linfeed.ms',\n caltable='polcal.xy0amb', #possibly with 180deg ambiguity\n field='1', #the calibrator\n solint='inf', \n combine='scan',\n preavg=200.0, #minimal parang change\n smodel=[1,0,1,0], #non-zero U assumed\n gaintype='XYf+QU', \n gaintable=['polcal.gcal','polcal.bcal','polcal.xdelcal]) #all prior calibration\n```\n\nNote that we imply non-zero Stokes U in *smodel*; this is to enforce the assumption of non-zero source polarization signature in the cross-hands in the ratio of data and model. This solve will report the center-channel XY-phase and apparent Q,U for each spw. The Q,U results should be recognizable in comparison to that reported by **qufromgain** above. However, since the XY-phase has a 180 degree ambiguity (you can rotate the source polarization signature to lie entirely in the visibility real part by rotating clockwise or counter-clockwise), some or all spw Q,U estimates may have the wrong sign. We correct this using the **xyamb** utility method, using the *qu* obtained from *qufromgain* above (which is not ambiguous):\n\n```\nS=xyamb(xy='polcal.xy0amb',qu=qu,xyout='polcal.xy0')\n```\n\nThe python variable *S* now contains the mean source model (Stokes I =1; fractional Q,U; V=0) that can be used in a revision of the gain calibration and instrumental polarization calibration.\n\nNext we revise the gain calibration using the full polarization source model:\n\n```\ngaincal(vis='polcal_linfeed.ms',\n caltable='polcal.gcal1', \n field='1', \n solint='int', \n smodel=S, #obtained from xyamb\n gaintype='G', \n gaintable=['polcal.bcal'],\n parang=T) #so source poln properly rotated\n```\n\nNote that *parang=T* so that the supplied source linear polarization is properly rotated in the parallel-hand visibility model. This new gain solution can be plotted with *poln=\\'/\\'* as above to show that the source polarization is no longer distorting it. Also, if **qufromgain** is run on this new gain table, the reported source polarization should be statistically indistinguishable from zero.\n\nFinally, we can now solve for the instrumental polarization:\n\n```\n polcal(vis= 'polcal_linfeed.ms',\n caltable='polcal.dcal',\n field='1',\n solint='inf',\n combine='scan',\n preavg=200,\n poltype='Dflls', #freq-dep LLS solver\n refant='', #no reference antenna\n smodel=S,\n gaintable=['polcal.gcal1','polcal.bcal','polcal.xdelcal','polcal.xy0'])\n```\n\nNote that no reference antenna is used since this solve will produce an absolute instrumental polarization solution that is registered to the assumed source polarization (*S*) and prior calibrations. Applying a refant (referring all instrumental polarization terms to a reference antennas X feed, which would then be assumed perfect) would, in fact, discard valid information about the imperfections in the reference antennas X feed. (Had we used an unpolarized calibrator, we would not have a valid xy-phase solution, nor would we have had access to the absolute instrumental polarization solution demonstrated here.)\n\nA few points:\n\n- Since the gain, bandpass, and XY-phase calibrations were obtained prior to the instrumental polarization solution and maybe distorted by it, it is generally desirable to re-solve for them using this instrumental polarization solution as a prior calibration. In effect, this means iterating the sequence of calibration steps using all of the best of the available information at each stage, including the source polarization (and *parang=T*). This is a generalization of traditional self-calibration.\n- If the source linear polarization fraction and position angle is known *a priori*, the processing steps outlined above can be amended to use that source polarization assertion in the gain and instrumental calibration solves from the start. The *qufromgain* method is then not needed (but can be used to verify assumptions), the **gaincal(***\\...,gaintype=XYf+QU,\\...***)** should not be altered (parallactic angle coverage is still required!), and the **xyamb** run should use the *a priori* polarization for *qu*. If there is likely to be a large systematic offset in the mean feed position angle, iteration of the gain, bandpass, and instrumental polarization terms is required to properly isolate the calibration effects.\n- Note that the above process does not explicitly include a position angle calibration. In effect, the estimated source polarization sets the mean feed position angle as the reference position angle, and this is usually within a degree or so of optimal for linear feeds. If your mean X feed position angle is not 0 degrees, and your MS does not account for the offset in its FEED subtable, be careful in your interpretation of the final position angle. Currently, the circular feed-specific position angle calibration modes of **polcal(**\\...,*poltype=\\'Xf\\',\\...***)** will not properly handle the linear feed basis; this will be fixed in a future release. \n\n\n\n***\n\n\n\n",
"_____no_output_____"
],
[
"## Water Vapor Radiometers \n\nThe task **wvrgcal** generates a gain table based on Water Vapor Radiometer (WVR) data and is used for ALMA data reduction. Briefly, the task enables a Bayesian approach to calculating the coefficients that convert the outputs of the ALMA 183 GHz water-vapor radiometers (mounted on each antenna) into estimates of path fluctuations which can then be used to correct the observed interferometric visibilities.\n\nThe CASA task is an interface to the executable wvrgcal, which is part of the CASA 5 distribution and can also be called from outside CASA. The wvrgcal software is based on the libair and libbnmin libraries which were developed by Bojan Nikolic at the University of Cambridge as part of EU FP6 ALMA Enhancement program. CASA 5 contains version 2.1 of wvrgcal. The algorithmic core of wvrgcal is described in three ALMA memos (number 587 [\\[1\\]](#Bibliography), 588 [\\[2\\]](#Bibliography), and 593 [\\[3\\]](#Bibliography) ) which describe the algorithms implemented in the software.\n\nThe CASA task interface to wvrgcal follows closely the interface of the shell executable at the same time staying within the CASA task parameter conventions. In ALMA data, the WVR measurements belonging to a given observation are contained in the ASDM for that observation. After conversion to an MS using **importasdm**, the WVR information can be found in separate spectral windows. As of April 2016, it is still one single spectral window for all WVRs, however, the ID of the spectral window may vary between datasets. The **wvrgcal** task identifies the SPW autonomously, but it can also be specified via the parameter *wvrspw* (see below). The specified spectral window(s) must be present in the MS for **wvrgcal** to work. This is not to be mixed up with the list of spectral windows for which solutions should be calculated and which can be specified with the parameter *spw*. Note that **wvrgcal** will calculate a correction only for the scans with the words ON_SOURCE, SIGNAL, or REFERENCE in the scan intent. The various features of **wvrgcal** are then controlled by a number of task parameters (see the list above). They have default values which will work for ALMA data. An example for a typical **wvrgcal** call can be found in the ALMA CASA guide for the NGC 3256 analysis:\n\n```\nwvrgcal(vis='uid___A002_X1d54a1_X5.ms',\n caltable='cal-wvr-uid___A002_X1d54a1_X5.W',\n toffset=-1,\n segsource=True, tie=[\"Titan,1037-295,NGC3256\"], statsource=\"1037-295\",\n wvrspw=[4],\n spw=[17,19,21,23])\n```\n\nHere, *vis* is the name of input visibility file which as mentioned above also contains the WVR data and *caltable* is the name of the output gain calibration table. WVR data is typically in spectral window 0, but in the example above, the data are contained in spectral window 4. Although **wvrgcal** should automatically identify this SPW, it is explicitly specified with the *wvrspw* parameter in the above example. The *toffset* parameter is the known time offset in seconds between the WVR measurements and the visibility integrations for which they are valid. For ALMA, this offset is presently -1 s (which is also the default value).\n\nThe parameter *segsource* (segregate source) controls whether separate coefficients are calculated for each source. The default value True is the recommended one for ALMA. When *segsource* is True, the subparameter *tie* is available. It permits the formation of groups of sources for which common coefficients are calculated as well as possible. The *tie* parameter ensures best possible phase transfer between a group of sources. In general it is recommended to tie together all of the sources in a single Science Goal (in ALMA speak) and their phase calibrator(s). The recommended maximum angular distance up to which two sources can be tied is 15 degrees. The parameter statsource controls for which sources statistics are calculated and displayed in the logger. This has no influence on the generated calibration table.\n\nVia the parameter *spw*, one can control for which of the input spectral windows **wvrgcal** will calculate phase corrections and store them in the output calibration table. By default, solutions for all spectral windows are written except for the ones containing WVR data. The **wvrgcal** task respects the flags in the Main and ANTENNA table of the MS. The parameter *mingoodfrac* lets the user set a requirement on the minimum fraction of good measurements for accepting the WVR data from an antenna. If antennas are flagged, their WVR solution is interpolated from the three nearest neighboring antennas. This process can be controlled with the new parameters *maxdistm* and *minnumants*. The former sets the maximum distance an antenna used for interpolation may have from the flagged one. And *minnumants* sets how many near antennas there have to be for interpolation to take place. For more details on the WVR Phase correction, see also the the ALMA Memo \"Quality Control of WVR Phase Correction Based on Differences Between WVR Channels\" by B. Nikolic, R. C. Bolton & J. S. Richer [\\[4\\]](#Bibliography) , see also ALMA memo 593 [\\[3\\]](#Bibliography).\n\n**Statistical parameters shown in the logger output of wvrgcal**\n\nThe **wvrgcal** task writes out a variety of information to the logger, including various statistical measures of the performance. This allows the user to judge whether WVR correction is appropriate for the MS, to check whether any antennas have problematic WVR values, and to examine the predicted performance of the WVR correction when applied. For each set of correction coefficients which are calculated (the number of coefficient sets are controlled by the parameters *nsol*, *segsource* and *tie*), the **wvrgcal** output to the logger first of all shows the time sample, the individual temperatures of each of the four WVR channels, and the elevation of the source in question at that time. For each of these coefficient sets, it then gives the evidence of the bayesian parameter estimation, the calculated precipitable water vapor (PWV) and its error in mm, and the correction coefficients found for each WVR channel (dTdL).\n\nThe output then shows the statistical information about the observation. First of all it gives the start and end times for the parts of the observation used to calculate these statistics (controlled by *segsource*). It then shows a break down for each of the antennas in the data set. This gives the antenna name and number; whether or not it has a WVR (column WVR); whether or not it has been flagged (column FLAG); the RMS of the path length variation with time towards that antenna (column RMS); and the discrepancy between the RMS path length calculated separately for different WVR channels (column Disc.). These values allow the user to see if an individual WVR appears to have been suffering from problems during the observation, and to flag that antenna using *wvrflag* if necessary. This discrepancy value, Disc., can in addition be used as a simple diagnostic tool to evaluate whether or not the WVR correction caltable created by **wvrgcal** should be applied. In the event of the WVR observations being contaminated by strong cloud emission in the atmosphere, the attempt by **wvrgcal** to fit the water vapor line may not be successful, and applying the produced calibration table can in extreme cases reduce the quality of the data. However, these weather conditions should identified by a high value in the discrepancy column produced when running **wvrgcal**.\n\nDiscrepancy values of greater than a 1000 microns usually indicate strong cloud contamination of the WVR data, and the output calibration table should probably not be applied. If the values are between 100 and 1000 microns, then the user should manually examine the phases before and after applying the caltable to decide if WVR correction is appropriate. Work is underway at ALMA to provide additional routines to at least partially remove the cloud component from the WVR data before calculating phase corrections. CASA 4.7 will contain a first tested version of such a tool. After the antenna-by-antenna statistics, the output then displays some estimates of the performance of the **wvrgcal** correction. These are the thermal contribution from the water vapor to the path fluctuations per antenna (in microns), the largest path fluctuation found on a baseline (in microns), and the expected error on the path length calculated for each baseline due to the error in the coefficients (in microns).\n\n**Antenna position calculation**\n\nThe information about antenna pointing direction is by default taken from the POINTING table. Should this table not be present for some reason, the user can instead switch to determining the antenna positions from the phase directions in the FIELD table (under the assumption that all antennas were pointing ideally). The switch is performed by setting the parameter *usefieldtab* to True.\n\n**Spectral window selection**\n\nBy default, **wvrgcal** puts solutions for all spectral windows of the MS into the output calibration table. Since usually only the spectral windows are of interest in which the science target and the calibrators were observed, it is not necessary to store solutions for other spectral windows. The spectral windows for which solutions are stored can be selected with the parameter *spw*, e.g. spw = \\[17,19,21,23\\] will make **wvrgcal** write only solutions for spectral windows 17, 19, 21, and 23. With respect to the input WVR spectral windows, **wvrgcal** will by default regard all windows with 4 channels as WVR data. In typical ALMA data there is only one such spectral window in each ASDM. This may change in the future. In any case, the input WVR spectral window(s) can be selected with the optional parameter *wvrspw*. The syntax is the same as for the parameter *spw* above.\n\n\n### Bibliography\n\n1. [ALMA Memo 587](http://library.nrao.edu/public/memos/alma/memo587.pdf) \n2. [ALMA Memo 588](http://library.nrao.edu/public/memos/alma/memo588.pdf) \n3. [ALMA Memo 593](http://library.nrao.edu/public/memos/alma/memo593.pdf) \n4. [ALMA Memo \"Quality Control of WVR Phase Correction Based on Differences Between WVR Channels\"](https://casa.nrao.edu/Memos/memoqachannels.pdf)\n\n\n***\n\n\n\n",
"_____no_output_____"
],
[
"## Examine/Edit Cal Tables \n\nHow to plot, list, and adjust calibration tables\n\nInformation on examination and manipulation of calibration tables can be found in the task documentation for **plotcal**, **listcal**, **calstat**, **smoothcal**, and **browsetable**.\n\n\n***\n\n\n\n",
"_____no_output_____"
],
[
"## Apply Calibration \n\nHow to apply calibration to generate data for imaging\n\nPlease see the task documentation for **applycal** for details on application of calibration.\n\n\n***\n\n\n\n",
"_____no_output_____"
]
]
] | [
"markdown"
] | [
[
"markdown",
"markdown",
"markdown",
"markdown",
"markdown",
"markdown",
"markdown",
"markdown",
"markdown",
"markdown",
"markdown",
"markdown",
"markdown",
"markdown",
"markdown",
"markdown",
"markdown",
"markdown",
"markdown",
"markdown",
"markdown",
"markdown",
"markdown"
]
] |
d08f20e11bd119bebe6af79bac0808c3f306df40 | 8,488 | ipynb | Jupyter Notebook | notebooks/data_viz_to_coder/raw/tut5.ipynb | stephenramthun/learntools | 4b597e448adfc8fd251ef7f3ee2994fdd46f0900 | [
"Apache-2.0"
] | 1 | 2019-12-09T04:45:42.000Z | 2019-12-09T04:45:42.000Z | notebooks/data_viz_to_coder/raw/tut5.ipynb | stephenramthun/learntools | 4b597e448adfc8fd251ef7f3ee2994fdd46f0900 | [
"Apache-2.0"
] | null | null | null | notebooks/data_viz_to_coder/raw/tut5.ipynb | stephenramthun/learntools | 4b597e448adfc8fd251ef7f3ee2994fdd46f0900 | [
"Apache-2.0"
] | 1 | 2019-04-17T06:12:23.000Z | 2019-04-17T06:12:23.000Z | 33.68254 | 293 | 0.609095 | [
[
[
"In this tutorial you'll learn all about **histograms** and **density plots**.\n\n# Set up the notebook\n\nAs always, we begin by setting up the coding environment. (_This code is hidden, but you can un-hide it by clicking on the \"Code\" button immediately below this text, on the right._)",
"_____no_output_____"
]
],
[
[
"#$HIDE$\nimport pandas as pd\nimport matplotlib.pyplot as plt\n%matplotlib inline\nimport seaborn as sns\nprint(\"Setup Complete\")",
"_____no_output_____"
]
],
[
[
"# Select a dataset\n\nWe'll work with a dataset of 150 different flowers, or 50 each from three different species of iris (*Iris setosa*, *Iris versicolor*, and *Iris virginica*).\n\n\n\n# Load and examine the data\n\nEach row in the dataset corresponds to a different flower. There are four measurements: the sepal length and width, along with the petal length and width. We also keep track of the corresponding species. ",
"_____no_output_____"
]
],
[
[
"# Path of the file to read\niris_filepath = \"../input/iris.csv\"\n\n# Read the file into a variable iris_data\niris_data = pd.read_csv(iris_filepath, index_col=\"Id\")\n\n# Print the first 5 rows of the data\niris_data.head()",
"_____no_output_____"
]
],
[
[
"# Histograms\n\nSay we would like to create a **histogram** to see how petal length varies in iris flowers. We can do this with the `sns.distplot` command. ",
"_____no_output_____"
]
],
[
[
"# Histogram \nsns.distplot(a=iris_data['Petal Length (cm)'], kde=False)",
"_____no_output_____"
]
],
[
[
"We customize the behavior of the command with two additional pieces of information:\n- `a=` chooses the column we'd like to plot (_in this case, we chose `'Petal Length (cm)'`_).\n- `kde=False` is something we'll always provide when creating a histogram, as leaving it out will create a slightly different plot.\n\n# Density plots\n\nThe next type of plot is a **kernel density estimate (KDE)** plot. In case you're not familiar with KDE plots, you can think of it as a smoothed histogram. \n\nTo make a KDE plot, we use the `sns.kdeplot` command. Setting `shade=True` colors the area below the curve (_and `data=` has identical functionality as when we made the histogram above_).",
"_____no_output_____"
]
],
[
[
"# KDE plot \nsns.kdeplot(data=iris_data['Petal Length (cm)'], shade=True)",
"_____no_output_____"
]
],
[
[
"# 2D KDE plots\n\nWe're not restricted to a single column when creating a KDE plot. We can create a **two-dimensional (2D) KDE plot** with the `sns.jointplot` command.\n\nIn the plot below, the color-coding shows us how likely we are to see different combinations of sepal width and petal length, where darker parts of the figure are more likely. ",
"_____no_output_____"
]
],
[
[
"# 2D KDE plot\nsns.jointplot(x=iris_data['Petal Length (cm)'], y=iris_data['Sepal Width (cm)'], kind=\"kde\")",
"_____no_output_____"
]
],
[
[
"Note that in addition to the 2D KDE plot in the center,\n- the curve at the top of the figure is a KDE plot for the data on the x-axis (in this case, `iris_data['Petal Length (cm)']`), and\n- the curve on the right of the figure is a KDE plot for the data on the y-axis (in this case, `iris_data['Sepal Width (cm)']`).",
"_____no_output_____"
],
[
"# Color-coded plots\n\nFor the next part of the tutorial, we'll create plots to understand differences between the species. To accomplish this, we begin by breaking the dataset into three separate files, with one for each species.",
"_____no_output_____"
]
],
[
[
"# Paths of the files to read\niris_set_filepath = \"../input/iris_setosa.csv\"\niris_ver_filepath = \"../input/iris_versicolor.csv\"\niris_vir_filepath = \"../input/iris_virginica.csv\"\n\n# Read the files into variables \niris_set_data = pd.read_csv(iris_set_filepath, index_col=\"Id\")\niris_ver_data = pd.read_csv(iris_ver_filepath, index_col=\"Id\")\niris_vir_data = pd.read_csv(iris_vir_filepath, index_col=\"Id\")\n\n# Print the first 5 rows of the Iris versicolor data\niris_ver_data.head()",
"_____no_output_____"
]
],
[
[
"In the code cell below, we create a different histogram for each species by using the `sns.distplot` command (_as above_) three times. We use `label=` to set how each histogram will appear in the legend.",
"_____no_output_____"
]
],
[
[
"# Histograms for each species\nsns.distplot(a=iris_set_data['Petal Length (cm)'], label=\"Iris-setosa\", kde=False)\nsns.distplot(a=iris_ver_data['Petal Length (cm)'], label=\"Iris-versicolor\", kde=False)\nsns.distplot(a=iris_vir_data['Petal Length (cm)'], label=\"Iris-virginica\", kde=False)\n\n# Add title\nplt.title(\"Histogram of Petal Lengths, by Species\")\n\n# Force legend to appear\nplt.legend()",
"_____no_output_____"
]
],
[
[
"In this case, the legend does not automatically appear on the plot. To force it to show (for any plot type), we can always use `plt.legend()`.\n\nWe can also create a KDE plot for each species by using `sns.kdeplot` (_as above_). Again, `label=` is used to set the values in the legend.",
"_____no_output_____"
]
],
[
[
"# KDE plots for each species\nsns.kdeplot(data=iris_set_data['Petal Length (cm)'], label=\"Iris-setosa\", shade=True)\nsns.kdeplot(data=iris_ver_data['Petal Length (cm)'], label=\"Iris-versicolor\", shade=True)\nsns.kdeplot(data=iris_vir_data['Petal Length (cm)'], label=\"Iris-virginica\", shade=True)\n\n# Add title\nplt.title(\"Distribution of Petal Lengths, by Species\")",
"_____no_output_____"
]
],
[
[
"One interesting pattern that can be seen in plots is that the plants seem to belong to one of two groups, where _Iris versicolor_ and _Iris virginica_ seem to have similar values for petal length, while _Iris setosa_ belongs in a category all by itself. \n\nIn fact, according to this dataset, we might even be able to classify any iris plant as *Iris setosa* (as opposed to *Iris versicolor* or *Iris virginica*) just by looking at the petal length: if the petal length of an iris flower is less than 2 cm, it's most likely to be *Iris setosa*!",
"_____no_output_____"
],
[
"# What's next?\n\nPut your new skills to work in a **[coding exercise](#$NEXT_NOTEBOOK_URL$)**!",
"_____no_output_____"
]
]
] | [
"markdown",
"code",
"markdown",
"code",
"markdown",
"code",
"markdown",
"code",
"markdown",
"code",
"markdown",
"code",
"markdown",
"code",
"markdown",
"code",
"markdown"
] | [
[
"markdown"
],
[
"code"
],
[
"markdown"
],
[
"code"
],
[
"markdown"
],
[
"code"
],
[
"markdown"
],
[
"code"
],
[
"markdown"
],
[
"code"
],
[
"markdown",
"markdown"
],
[
"code"
],
[
"markdown"
],
[
"code"
],
[
"markdown"
],
[
"code"
],
[
"markdown",
"markdown"
]
] |
d08f25f227e692b15662aa6700eb62b3df0877c5 | 2,923 | ipynb | Jupyter Notebook | notebooks/ensemble_ex_02.ipynb | lesteve/scikit-learn-mooc | b822586b98e71dbbf003bde86be57412cb170291 | [
"CC-BY-4.0"
] | null | null | null | notebooks/ensemble_ex_02.ipynb | lesteve/scikit-learn-mooc | b822586b98e71dbbf003bde86be57412cb170291 | [
"CC-BY-4.0"
] | null | null | null | notebooks/ensemble_ex_02.ipynb | lesteve/scikit-learn-mooc | b822586b98e71dbbf003bde86be57412cb170291 | [
"CC-BY-4.0"
] | null | null | null | 28.940594 | 101 | 0.594937 | [
[
[
"# 📝 Exercise M6.02\n\nThe aim of this exercise it to explore some attributes available in\nscikit-learn's random forest.\n\nFirst, we will fit the penguins regression dataset.",
"_____no_output_____"
]
],
[
[
"import pandas as pd\nfrom sklearn.model_selection import train_test_split\n\npenguins = pd.read_csv(\"../datasets/penguins_regression.csv\")\nfeature_names = [\"Flipper Length (mm)\"]\ntarget_name = \"Body Mass (g)\"\ndata, target = penguins[feature_names], penguins[target_name]\ndata_train, data_test, target_train, target_test = train_test_split(\n data, target, random_state=0)",
"_____no_output_____"
]
],
[
[
"<div class=\"admonition note alert alert-info\">\n<p class=\"first admonition-title\" style=\"font-weight: bold;\">Note</p>\n<p class=\"last\">If you want a deeper overview regarding this dataset, you can refer to the\nAppendix - Datasets description section at the end of this MOOC.</p>\n</div>",
"_____no_output_____"
],
[
"Create a random forest containing three trees. Train the forest and\ncheck the generalization performance on the testing set in terms of mean\nabsolute error.",
"_____no_output_____"
]
],
[
[
"# Write your code here.",
"_____no_output_____"
]
],
[
[
"The next steps of this exercise are to:\n\n- create a new dataset containing the penguins with a flipper length\n between 170 mm and 230 mm;\n- plot the training data using a scatter plot;\n- plot the decision of each individual tree by predicting on the newly\n created dataset;\n- plot the decision of the random forest using this newly created dataset.\n\n<div class=\"admonition tip alert alert-warning\">\n<p class=\"first admonition-title\" style=\"font-weight: bold;\">Tip</p>\n<p class=\"last\">The trees contained in the forest that you created can be accessed\nwith the attribute <tt class=\"docutils literal\">estimators_</tt>.</p>\n</div>",
"_____no_output_____"
]
],
[
[
"# Write your code here.",
"_____no_output_____"
]
]
] | [
"markdown",
"code",
"markdown",
"code",
"markdown",
"code"
] | [
[
"markdown"
],
[
"code"
],
[
"markdown",
"markdown"
],
[
"code"
],
[
"markdown"
],
[
"code"
]
] |
d08f29583ef34d1e2fab9bdc088efe5715861fcc | 311,265 | ipynb | Jupyter Notebook | Distracted Driver Detection CNN Scratch.ipynb | vk16309/Distracted-Driver-Detection | 8075ba83780ca4bb603d9faefee70f45e3e9a113 | [
"Apache-2.0"
] | 1 | 2020-11-29T06:05:27.000Z | 2020-11-29T06:05:27.000Z | Distracted Driver Detection CNN Scratch.ipynb | vk16309/Distracted-Driver-Detection | 8075ba83780ca4bb603d9faefee70f45e3e9a113 | [
"Apache-2.0"
] | null | null | null | Distracted Driver Detection CNN Scratch.ipynb | vk16309/Distracted-Driver-Detection | 8075ba83780ca4bb603d9faefee70f45e3e9a113 | [
"Apache-2.0"
] | 1 | 2020-11-29T06:05:40.000Z | 2020-11-29T06:05:40.000Z | 299.005764 | 177,860 | 0.918353 | [
[
[
"# IMPORTING THE LIBRARIES",
"_____no_output_____"
]
],
[
[
"import os\nimport pandas as pd\nimport pickle\nimport numpy as np\nimport seaborn as sns\nfrom sklearn.datasets import load_files\nfrom keras.utils import np_utils\nimport matplotlib.pyplot as plt\nfrom keras.layers import Conv2D, MaxPooling2D, GlobalAveragePooling2D\nfrom keras.layers import Dropout, Flatten, Dense\nfrom keras.models import Sequential\nfrom keras.utils.vis_utils import plot_model\nfrom keras.callbacks import ModelCheckpoint\nfrom keras.utils import to_categorical\nfrom sklearn.metrics import confusion_matrix\nfrom keras.preprocessing import image \nfrom tqdm import tqdm\n\nimport seaborn as sns\nfrom sklearn.metrics import accuracy_score,precision_score,recall_score,f1_score",
"Using TensorFlow backend.\n"
],
[
"# Pretty display for notebooks\n%matplotlib inline",
"_____no_output_____"
],
[
"!ls",
" csv_files\r\n'Distracted Driver Detection CNN Scratch.ipynb'\r\n'Distracted Driver Detection VGG16.ipynb'\r\n imgs\r\n model\r\n pickle_files\r\n'Self Trained Model Evaluation.ipynb'\r\n temp_dir\r\n"
]
],
[
[
"# Defining the train,test and model directories\n\nWe will create the directories for train,test and model training paths if not present",
"_____no_output_____"
]
],
[
[
"TEST_DIR = os.path.join(os.getcwd(),\"imgs\",\"test\")\nTRAIN_DIR = os.path.join(os.getcwd(),\"imgs\",\"train\")\nMODEL_PATH = os.path.join(os.getcwd(),\"model\",\"self_trained\")\nPICKLE_DIR = os.path.join(os.getcwd(),\"pickle_files\")",
"_____no_output_____"
],
[
"if not os.path.exists(TEST_DIR):\n print(\"Testing data does not exists\")\nif not os.path.exists(TRAIN_DIR):\n print(\"Training data does not exists\")\nif not os.path.exists(MODEL_PATH):\n print(\"Model path does not exists\")\n os.makedirs(MODEL_PATH)\n print(\"Model path created\")\nif not os.path.exists(PICKLE_DIR):\n os.makedirs(PICKLE_DIR)",
"_____no_output_____"
]
],
[
[
"# Data Preparation",
"_____no_output_____"
],
[
"We will create a csv file having the location of the files present for training and test images and their associated class if present so that it is easily traceable.",
"_____no_output_____"
]
],
[
[
"def create_csv(DATA_DIR,filename):\n class_names = os.listdir(DATA_DIR)\n data = list()\n if(os.path.isdir(os.path.join(DATA_DIR,class_names[0]))):\n for class_name in class_names:\n file_names = os.listdir(os.path.join(DATA_DIR,class_name))\n for file in file_names:\n data.append({\n \"Filename\":os.path.join(DATA_DIR,class_name,file),\n \"ClassName\":class_name\n })\n else:\n class_name = \"test\"\n file_names = os.listdir(DATA_DIR)\n for file in file_names:\n data.append(({\n \"FileName\":os.path.join(DATA_DIR,file),\n \"ClassName\":class_name\n }))\n data = pd.DataFrame(data)\n data.to_csv(os.path.join(os.getcwd(),\"csv_files\",filename),index=False)\n\ncreate_csv(TRAIN_DIR,\"train.csv\")\ncreate_csv(TEST_DIR,\"test.csv\")\ndata_train = pd.read_csv(os.path.join(os.getcwd(),\"csv_files\",\"train.csv\"))\ndata_test = pd.read_csv(os.path.join(os.getcwd(),\"csv_files\",\"test.csv\"))\n",
"_____no_output_____"
],
[
"data_train.info()",
"<class 'pandas.core.frame.DataFrame'>\nRangeIndex: 22424 entries, 0 to 22423\nData columns (total 2 columns):\n # Column Non-Null Count Dtype \n--- ------ -------------- ----- \n 0 Filename 22424 non-null object\n 1 ClassName 22424 non-null object\ndtypes: object(2)\nmemory usage: 350.5+ KB\n"
],
[
"data_train['ClassName'].value_counts()",
"_____no_output_____"
],
[
"data_train.describe()",
"_____no_output_____"
],
[
"\n\nnf = data_train['ClassName'].value_counts(sort=False)\nlabels = data_train['ClassName'].value_counts(sort=False).index.tolist()\ny = np.array(nf)\nwidth = 1/1.5\nN = len(y)\nx = range(N)\n\nfig = plt.figure(figsize=(20,15))\nay = fig.add_subplot(211)\n\nplt.xticks(x, labels, size=15)\nplt.yticks(size=15)\n\nay.bar(x, y, width, color=\"blue\")\n\nplt.title('Bar Chart',size=25)\nplt.xlabel('classname',size=15)\nplt.ylabel('Count',size=15)\n\nplt.show()",
"_____no_output_____"
],
[
"data_test.head()",
"_____no_output_____"
],
[
"data_test.shape",
"_____no_output_____"
]
],
[
[
"## Observation:\n1. There are total 22424 training samples\n2. There are total 79726 training samples\n3. The training dataset is equally balanced to a great extent and hence we need not do any downsampling of the data",
"_____no_output_____"
],
[
"## Converting into numerical values",
"_____no_output_____"
]
],
[
[
"labels_list = list(set(data_train['ClassName'].values.tolist()))\nlabels_id = {label_name:id for id,label_name in enumerate(labels_list)}\nprint(labels_id)\ndata_train['ClassName'].replace(labels_id,inplace=True)",
"{'c2': 0, 'c9': 1, 'c4': 2, 'c7': 3, 'c1': 4, 'c5': 5, 'c0': 6, 'c8': 7, 'c3': 8, 'c6': 9}\n"
],
[
"with open(os.path.join(os.getcwd(),\"pickle_files\",\"labels_list.pkl\"),\"wb\") as handle:\n pickle.dump(labels_id,handle)",
"_____no_output_____"
],
[
"labels = to_categorical(data_train['ClassName'])\nprint(labels.shape)",
"(22424, 10)\n"
]
],
[
[
"## Splitting into Train and Test sets",
"_____no_output_____"
]
],
[
[
"from sklearn.model_selection import train_test_split\n\nxtrain,xtest,ytrain,ytest = train_test_split(data_train.iloc[:,0],labels,test_size = 0.2,random_state=42)",
"_____no_output_____"
]
],
[
[
"### Converting into 64*64 images \nYou can substitute 64,64 to 224,224 for better results only if ram is >32gb",
"_____no_output_____"
]
],
[
[
"\ndef path_to_tensor(img_path):\n # loads RGB image as PIL.Image.Image type\n img = image.load_img(img_path, target_size=(64, 64))\n # convert PIL.Image.Image type to 3D tensor with shape (224, 224, 3)\n x = image.img_to_array(img)\n # convert 3D tensor to 4D tensor with shape (1, 224, 224, 3) and return 4D tensor\n return np.expand_dims(x, axis=0)\n\ndef paths_to_tensor(img_paths):\n list_of_tensors = [path_to_tensor(img_path) for img_path in tqdm(img_paths)]\n return np.vstack(list_of_tensors)",
"_____no_output_____"
],
[
"\nfrom PIL import ImageFile \nImageFile.LOAD_TRUNCATED_IMAGES = True \n\n# pre-process the data for Keras\ntrain_tensors = paths_to_tensor(xtrain).astype('float32')/255 - 0.5\n",
"100%|██████████| 17939/17939 [07:35<00:00, 39.36it/s]\n"
],
[
"valid_tensors = paths_to_tensor(xtest).astype('float32')/255 - 0.5\n",
"100%|██████████| 4485/4485 [02:33<00:00, 29.21it/s]\n"
],
[
"##takes too much ram \n## run this if your ram is greater than 16gb \n# test_tensors = paths_to_tensor(data_test.iloc[:,0]).astype('float32')/255 - 0.5 ",
"_____no_output_____"
]
],
[
[
"# Defining the Model",
"_____no_output_____"
]
],
[
[
"model = Sequential()\n\nmodel.add(Conv2D(filters=64, kernel_size=2, padding='same', activation='relu', input_shape=(64,64,3), kernel_initializer='glorot_normal'))\nmodel.add(MaxPooling2D(pool_size=2))\nmodel.add(Conv2D(filters=128, kernel_size=2, padding='same', activation='relu', kernel_initializer='glorot_normal'))\nmodel.add(MaxPooling2D(pool_size=2))\nmodel.add(Conv2D(filters=256, kernel_size=2, padding='same', activation='relu', kernel_initializer='glorot_normal'))\nmodel.add(MaxPooling2D(pool_size=2))\nmodel.add(Conv2D(filters=512, kernel_size=2, padding='same', activation='relu', kernel_initializer='glorot_normal'))\nmodel.add(MaxPooling2D(pool_size=2))\nmodel.add(Dropout(0.5))\nmodel.add(Flatten())\nmodel.add(Dense(500, activation='relu', kernel_initializer='glorot_normal'))\nmodel.add(Dropout(0.5))\nmodel.add(Dense(10, activation='softmax', kernel_initializer='glorot_normal'))\n\n\nmodel.summary()",
"Model: \"sequential_1\"\n_________________________________________________________________\nLayer (type) Output Shape Param # \n=================================================================\nconv2d_1 (Conv2D) (None, 64, 64, 64) 832 \n_________________________________________________________________\nmax_pooling2d_1 (MaxPooling2 (None, 32, 32, 64) 0 \n_________________________________________________________________\nconv2d_2 (Conv2D) (None, 32, 32, 128) 32896 \n_________________________________________________________________\nmax_pooling2d_2 (MaxPooling2 (None, 16, 16, 128) 0 \n_________________________________________________________________\nconv2d_3 (Conv2D) (None, 16, 16, 256) 131328 \n_________________________________________________________________\nmax_pooling2d_3 (MaxPooling2 (None, 8, 8, 256) 0 \n_________________________________________________________________\nconv2d_4 (Conv2D) (None, 8, 8, 512) 524800 \n_________________________________________________________________\nmax_pooling2d_4 (MaxPooling2 (None, 4, 4, 512) 0 \n_________________________________________________________________\ndropout_1 (Dropout) (None, 4, 4, 512) 0 \n_________________________________________________________________\nflatten_1 (Flatten) (None, 8192) 0 \n_________________________________________________________________\ndense_1 (Dense) (None, 500) 4096500 \n_________________________________________________________________\ndropout_2 (Dropout) (None, 500) 0 \n_________________________________________________________________\ndense_2 (Dense) (None, 10) 5010 \n=================================================================\nTotal params: 4,791,366\nTrainable params: 4,791,366\nNon-trainable params: 0\n_________________________________________________________________\n"
],
[
"plot_model(model,to_file=os.path.join(MODEL_PATH,\"model_distracted_driver.png\"),show_shapes=True,show_layer_names=True)",
"_____no_output_____"
],
[
"model.compile(optimizer='rmsprop', loss='categorical_crossentropy', metrics=['accuracy'])",
"_____no_output_____"
],
[
"filepath = os.path.join(MODEL_PATH,\"distracted-{epoch:02d}-{val_accuracy:.2f}.hdf5\")\ncheckpoint = ModelCheckpoint(filepath, monitor='val_accuracy', verbose=1, save_best_only=True, mode='max',period=1)\ncallbacks_list = [checkpoint]",
"_____no_output_____"
],
[
"model_history = model.fit(train_tensors,ytrain,validation_data = (valid_tensors, ytest),epochs=25, batch_size=40, shuffle=True,callbacks=callbacks_list)",
"Train on 17939 samples, validate on 4485 samples\nEpoch 1/25\n17939/17939 [==============================] - 241s 13ms/step - loss: 1.0271 - accuracy: 0.6401 - val_loss: 0.2499 - val_accuracy: 0.9349\n\nEpoch 00001: val_accuracy improved from -inf to 0.93489, saving model to /home/abhinav/distracted_driver/model/self_trained/distracted-01-0.93.hdf5\nEpoch 2/25\n17939/17939 [==============================] - 245s 14ms/step - loss: 0.2204 - accuracy: 0.9322 - val_loss: 0.1146 - val_accuracy: 0.9670\n\nEpoch 00002: val_accuracy improved from 0.93489 to 0.96700, saving model to /home/abhinav/distracted_driver/model/self_trained/distracted-02-0.97.hdf5\nEpoch 3/25\n17939/17939 [==============================] - 222s 12ms/step - loss: 0.1175 - accuracy: 0.9658 - val_loss: 0.0632 - val_accuracy: 0.9835\n\nEpoch 00003: val_accuracy improved from 0.96700 to 0.98350, saving model to /home/abhinav/distracted_driver/model/self_trained/distracted-03-0.98.hdf5\nEpoch 4/25\n17939/17939 [==============================] - 224s 12ms/step - loss: 0.0866 - accuracy: 0.9752 - val_loss: 0.1053 - val_accuracy: 0.9730\n\nEpoch 00004: val_accuracy did not improve from 0.98350\nEpoch 5/25\n17939/17939 [==============================] - 229s 13ms/step - loss: 0.0682 - accuracy: 0.9790 - val_loss: 0.0480 - val_accuracy: 0.9842\n\nEpoch 00005: val_accuracy improved from 0.98350 to 0.98417, saving model to /home/abhinav/distracted_driver/model/self_trained/distracted-05-0.98.hdf5\nEpoch 6/25\n17939/17939 [==============================] - 222s 12ms/step - loss: 0.0617 - accuracy: 0.9833 - val_loss: 0.0514 - val_accuracy: 0.9857\n\nEpoch 00006: val_accuracy improved from 0.98417 to 0.98573, saving model to /home/abhinav/distracted_driver/model/self_trained/distracted-06-0.99.hdf5\nEpoch 7/25\n17939/17939 [==============================] - 237s 13ms/step - loss: 0.0500 - accuracy: 0.9865 - val_loss: 0.1003 - val_accuracy: 0.9750\n\nEpoch 00007: val_accuracy did not improve from 0.98573\nEpoch 8/25\n17939/17939 [==============================] - 243s 14ms/step - loss: 0.0489 - accuracy: 0.9862 - val_loss: 0.0506 - val_accuracy: 0.9904\n\nEpoch 00008: val_accuracy improved from 0.98573 to 0.99041, saving model to /home/abhinav/distracted_driver/model/self_trained/distracted-08-0.99.hdf5\nEpoch 9/25\n17939/17939 [==============================] - 236s 13ms/step - loss: 0.0487 - accuracy: 0.9870 - val_loss: 0.0435 - val_accuracy: 0.9882\n\nEpoch 00009: val_accuracy did not improve from 0.99041\nEpoch 10/25\n17939/17939 [==============================] - 232s 13ms/step - loss: 0.0436 - accuracy: 0.9881 - val_loss: 0.0459 - val_accuracy: 0.9918\n\nEpoch 00010: val_accuracy improved from 0.99041 to 0.99175, saving model to /home/abhinav/distracted_driver/model/self_trained/distracted-10-0.99.hdf5\nEpoch 11/25\n17939/17939 [==============================] - 223s 12ms/step - loss: 0.0437 - accuracy: 0.9881 - val_loss: 0.0498 - val_accuracy: 0.9891\n\nEpoch 00011: val_accuracy did not improve from 0.99175\nEpoch 12/25\n17939/17939 [==============================] - 227s 13ms/step - loss: 0.0398 - accuracy: 0.9889 - val_loss: 0.0562 - val_accuracy: 0.9895\n\nEpoch 00012: val_accuracy did not improve from 0.99175\nEpoch 13/25\n17939/17939 [==============================] - 232s 13ms/step - loss: 0.0459 - accuracy: 0.9894 - val_loss: 0.0533 - val_accuracy: 0.9924\n\nEpoch 00013: val_accuracy improved from 0.99175 to 0.99242, saving model to /home/abhinav/distracted_driver/model/self_trained/distracted-13-0.99.hdf5\nEpoch 14/25\n17939/17939 [==============================] - 230s 13ms/step - loss: 0.0359 - accuracy: 0.9908 - val_loss: 0.0851 - val_accuracy: 0.9871\n\nEpoch 00014: val_accuracy did not improve from 0.99242\nEpoch 15/25\n17939/17939 [==============================] - 228s 13ms/step - loss: 0.0435 - accuracy: 0.9906 - val_loss: 0.0725 - val_accuracy: 0.9893\n\nEpoch 00015: val_accuracy did not improve from 0.99242\nEpoch 16/25\n17939/17939 [==============================] - 226s 13ms/step - loss: 0.0377 - accuracy: 0.9916 - val_loss: 0.0562 - val_accuracy: 0.9929\n\nEpoch 00016: val_accuracy improved from 0.99242 to 0.99287, saving model to /home/abhinav/distracted_driver/model/self_trained/distracted-16-0.99.hdf5\nEpoch 17/25\n17939/17939 [==============================] - 237s 13ms/step - loss: 0.0391 - accuracy: 0.9918 - val_loss: 0.0656 - val_accuracy: 0.9924\n\nEpoch 00017: val_accuracy did not improve from 0.99287\nEpoch 18/25\n17939/17939 [==============================] - 227s 13ms/step - loss: 0.0392 - accuracy: 0.9919 - val_loss: 0.0858 - val_accuracy: 0.9897\n\nEpoch 00018: val_accuracy did not improve from 0.99287\nEpoch 19/25\n17939/17939 [==============================] - 234s 13ms/step - loss: 0.0385 - accuracy: 0.9928 - val_loss: 0.0792 - val_accuracy: 0.9933\n\nEpoch 00019: val_accuracy improved from 0.99287 to 0.99331, saving model to /home/abhinav/distracted_driver/model/self_trained/distracted-19-0.99.hdf5\nEpoch 20/25\n17939/17939 [==============================] - 232s 13ms/step - loss: 0.0397 - accuracy: 0.9928 - val_loss: 0.0690 - val_accuracy: 0.9913\n\nEpoch 00020: val_accuracy did not improve from 0.99331\nEpoch 21/25\n17939/17939 [==============================] - 219s 12ms/step - loss: 0.0339 - accuracy: 0.9921 - val_loss: 0.0662 - val_accuracy: 0.9922\n\nEpoch 00021: val_accuracy did not improve from 0.99331\nEpoch 22/25\n17939/17939 [==============================] - 219s 12ms/step - loss: 0.0311 - accuracy: 0.9933 - val_loss: 0.0814 - val_accuracy: 0.9873\n\nEpoch 00022: val_accuracy did not improve from 0.99331\nEpoch 23/25\n17939/17939 [==============================] - 219s 12ms/step - loss: 0.0453 - accuracy: 0.9930 - val_loss: 0.0607 - val_accuracy: 0.9929\n\nEpoch 00023: val_accuracy did not improve from 0.99331\nEpoch 24/25\n17939/17939 [==============================] - 219s 12ms/step - loss: 0.0418 - accuracy: 0.9925 - val_loss: 0.0627 - val_accuracy: 0.9926\n\nEpoch 00024: val_accuracy did not improve from 0.99331\nEpoch 25/25\n17939/17939 [==============================] - 219s 12ms/step - loss: 0.0370 - accuracy: 0.9936 - val_loss: 0.1214 - val_accuracy: 0.9929\n\nEpoch 00025: val_accuracy did not improve from 0.99331\n"
],
[
"fig, (ax1, ax2) = plt.subplots(2, 1, figsize=(12, 12))\nax1.plot(model_history.history['loss'], color='b', label=\"Training loss\")\nax1.plot(model_history.history['val_loss'], color='r', label=\"validation loss\")\nax1.set_xticks(np.arange(1, 25, 1))\nax1.set_yticks(np.arange(0, 1, 0.1))\n\nax2.plot(model_history.history['accuracy'], color='b', label=\"Training accuracy\")\nax2.plot(model_history.history['val_accuracy'], color='r',label=\"Validation accuracy\")\nax2.set_xticks(np.arange(1, 25, 1))\n\nlegend = plt.legend(loc='best', shadow=True)\nplt.tight_layout()\nplt.show()",
"_____no_output_____"
]
],
[
[
"# Model Analysis\n\nFinding the Confusion matrix,Precision,Recall and F1 score to analyse the model thus created ",
"_____no_output_____"
]
],
[
[
"\ndef print_confusion_matrix(confusion_matrix, class_names, figsize = (10,7), fontsize=14):\n df_cm = pd.DataFrame(\n confusion_matrix, index=class_names, columns=class_names, \n )\n fig = plt.figure(figsize=figsize)\n try:\n heatmap = sns.heatmap(df_cm, annot=True, fmt=\"d\")\n except ValueError:\n raise ValueError(\"Confusion matrix values must be integers.\")\n heatmap.yaxis.set_ticklabels(heatmap.yaxis.get_ticklabels(), rotation=0, ha='right', fontsize=fontsize)\n heatmap.xaxis.set_ticklabels(heatmap.xaxis.get_ticklabels(), rotation=45, ha='right', fontsize=fontsize)\n plt.ylabel('True label')\n plt.xlabel('Predicted label')\n fig.savefig(os.path.join(MODEL_PATH,\"confusion_matrix.png\"))\n return fig\n",
"_____no_output_____"
],
[
"def print_heatmap(n_labels, n_predictions, class_names):\n labels = n_labels #sess.run(tf.argmax(n_labels, 1))\n predictions = n_predictions #sess.run(tf.argmax(n_predictions, 1))\n\n# confusion_matrix = sess.run(tf.contrib.metrics.confusion_matrix(labels, predictions))\n matrix = confusion_matrix(labels.argmax(axis=1),predictions.argmax(axis=1))\n row_sum = np.sum(matrix, axis = 1)\n w, h = matrix.shape\n\n c_m = np.zeros((w, h))\n\n for i in range(h):\n c_m[i] = matrix[i] * 100 / row_sum[i]\n\n c = c_m.astype(dtype = np.uint8)\n\n \n heatmap = print_confusion_matrix(c, class_names, figsize=(18,10), fontsize=20)\n",
"_____no_output_____"
],
[
"class_names = list()\nfor name,idx in labels_id.items():\n class_names.append(name)\n# print(class_names)\nypred = model.predict(valid_tensors)",
"_____no_output_____"
],
[
"print_heatmap(ytest,ypred,class_names)",
"_____no_output_____"
]
],
[
[
"## Precision Recall F1 Score",
"_____no_output_____"
]
],
[
[
"ypred_class = np.argmax(ypred,axis=1)\n# print(ypred_class[:10])\nytest = np.argmax(ytest,axis=1)",
"_____no_output_____"
],
[
"accuracy = accuracy_score(ytest,ypred_class)\nprint('Accuracy: %f' % accuracy)\n# precision tp / (tp + fp)\nprecision = precision_score(ytest, ypred_class,average='weighted')\nprint('Precision: %f' % precision)\n# recall: tp / (tp + fn)\nrecall = recall_score(ytest,ypred_class,average='weighted')\nprint('Recall: %f' % recall)\n# f1: 2 tp / (2 tp + fp + fn)\nf1 = f1_score(ytest,ypred_class,average='weighted')\nprint('F1 score: %f' % f1)",
"Accuracy: 0.992865\nPrecision: 0.992910\nRecall: 0.992865\nF1 score: 0.992871\n"
]
]
] | [
"markdown",
"code",
"markdown",
"code",
"markdown",
"code",
"markdown",
"code",
"markdown",
"code",
"markdown",
"code",
"markdown",
"code",
"markdown",
"code",
"markdown",
"code"
] | [
[
"markdown"
],
[
"code",
"code",
"code"
],
[
"markdown"
],
[
"code",
"code"
],
[
"markdown",
"markdown"
],
[
"code",
"code",
"code",
"code",
"code",
"code",
"code"
],
[
"markdown",
"markdown"
],
[
"code",
"code",
"code"
],
[
"markdown"
],
[
"code"
],
[
"markdown"
],
[
"code",
"code",
"code",
"code"
],
[
"markdown"
],
[
"code",
"code",
"code",
"code",
"code",
"code"
],
[
"markdown"
],
[
"code",
"code",
"code",
"code"
],
[
"markdown"
],
[
"code",
"code"
]
] |
d08f2ea2d2489b02d3ba7fa4a7a490a889e3f141 | 142,388 | ipynb | Jupyter Notebook | Instructions/starter_code/weatherpy1.ipynb | bshub6/API-Challenge | c431ec527e4a1bdb13f3335b6ac034c7798b1751 | [
"MIT"
] | null | null | null | Instructions/starter_code/weatherpy1.ipynb | bshub6/API-Challenge | c431ec527e4a1bdb13f3335b6ac034c7798b1751 | [
"MIT"
] | null | null | null | Instructions/starter_code/weatherpy1.ipynb | bshub6/API-Challenge | c431ec527e4a1bdb13f3335b6ac034c7798b1751 | [
"MIT"
] | null | null | null | 110.980514 | 28,900 | 0.840689 | [
[
[
"# WeatherPy\n----\n\n#### Note\n* Instructions have been included for each segment. You do not have to follow them exactly, but they are included to help you think through the steps.",
"_____no_output_____"
]
],
[
[
"# Dependencies and Setup\nimport matplotlib.pyplot as plt\nimport pandas as pd\nimport numpy as np\nimport requests\nimport time\nimport json\nimport random\nimport csv as csv\n\n# Import API key\nfrom api_keys import api_key\n\n# Incorporated citipy to determine city based on latitude and longitude\nfrom citipy import citipy\n\n# Output File (CSV)\ncity_file = \"output_data/cities.csv\"\n\n\n# Range of latitudes and longitudes\nlat_range = (-90, 90)\nlng_range = (-180, 180)",
"_____no_output_____"
]
],
[
[
"## Generate Cities List",
"_____no_output_____"
]
],
[
[
"# List for holding lat_lngs and cities\ncounter = 0\nrandlat = []\nrandlngs = []\ncities = []\n\n# Create a set of random lat and lng combinations\nwhile len(randlat)< 500:\n lats = np.random.uniform(low=-90, high=90)\n lngs = np.random.uniform(low=-180, high=180)\n randlat.append(lats)\n randlngs.append(lngs) \n counter += 1\n \ncoord_df = pd.DataFrame({\"lats\":randlat, \"lngs\": randlngs}) \ncoord_df.head()\n \n\n",
"_____no_output_____"
],
[
"# Create a set of random lat and lng combinations\nlats = np.random.uniform(low=-90.000, high=90.000, size=1500)\nlngs = np.random.uniform(low=-180.000, high=180.000, size=1500)\nlat_lngs = zip(lats, lngs)\n\n# Identify nearest city for each lat, lng combination\nfor lat_lng in lat_lngs:\n city = citipy.nearest_city(lat_lng[0], lat_lng[1]).city_name\n \n # If the city is unique, then add it to a our cities list\n if city not in cities:\n cities.append(city)\n\n# Print the city count to confirm sufficient count\nprint(len(cities))\n#print(cities)",
"634\n"
]
],
[
[
"### Perform API Calls\n* Perform a weather check on each city using a series of successive API calls.\n* Include a print log of each city as it'sbeing processed (with the city number and city name).\n",
"_____no_output_____"
]
],
[
[
"url = \"http://api.openweathermap.org/data/2.5/weather?\"\nunits = \"metric\"\n\n# Build query URL to begin call\nurl = \"http://api.openweathermap.org/data/2.5/weather?units=metric&appid=\" + api_key\n\n#Set up list for responses\ndate = []\ncountry = []\nlat = []\nlon = []\ntemp_max = []\nhumidity = []\ncloud = []\nwind = []\n_cities = []\nprint(\"Beginning Data Retrieval\")\n\nfor city in cities:\n url_city = url + \"&q=\" + str(city)\n #print(url_city)\n #convert to json \n try:\n city_data = requests.get(url_city).json()\n country.append(city_data['sys']['country'])\n date.append(city_data['dt'])\n lat.append(city_data['coord']['lat'])\n lon.append(city_data['coord']['lon'])\n temp_max.append(city_data['main']['temp_max'])\n humidity.append(city_data['main']['humidity']) \n cloud.append(city_data['clouds']['all'])\n wind.append(city_data['wind']['speed'])\n _cities.append(city)\n print(f\"retreiving data | {city}\")\n except:\n print(\"If city is not found, skipping\")\nprint(\"Retrieval is complete!\")\ndata_dict = {'city': _cities,\n 'country': country,\n 'latitude': lat,\n 'longitude': lon,\n 'max temp': temp_max,\n 'humidity': humidity,\n 'cloudiness': cloud,\n 'windspeed': wind} \n \n",
"Beginning Data Retrieval\nretreiving data | codrington\nretreiving data | tuktoyaktuk\nretreiving data | ushuaia\nretreiving data | castro\nIf city is not found, skipping\nretreiving data | nkhata bay\nretreiving data | amga\nretreiving data | tessalit\nretreiving data | punta arenas\nIf city is not found, skipping\nretreiving data | hilo\nretreiving data | hanyang\nIf city is not found, skipping\nretreiving data | pacific grove\nretreiving data | rikitea\nretreiving data | bredasdorp\nretreiving data | mercedes\nretreiving data | narsaq\nretreiving data | atuona\nretreiving data | szendro\nretreiving data | punganuru\nIf city is not found, skipping\nretreiving data | talcahuano\nretreiving data | bonavista\nretreiving data | albany\nretreiving data | jamestown\nretreiving data | hobart\nretreiving data | port elizabeth\nretreiving data | maltahohe\nretreiving data | busselton\nretreiving data | half moon bay\nretreiving data | bambous virieux\nIf city is not found, skipping\nretreiving data | ashland\nretreiving data | souillac\nretreiving data | onega\nretreiving data | erdenet\nretreiving data | sarankhola\nretreiving data | gat\nretreiving data | nikolskoye\nretreiving data | pringsewu\nretreiving data | aklavik\nretreiving data | udachnyy\nretreiving data | bluff\nretreiving data | esperance\nretreiving data | mataura\nretreiving data | norman wells\nretreiving data | ponta do sol\nretreiving data | altay\nretreiving data | teguldet\nretreiving data | pamplona\nretreiving data | hermanus\nretreiving data | sitka\nretreiving data | coquimbo\nretreiving data | barrow\nretreiving data | new norfolk\nretreiving data | port alfred\nretreiving data | marzuq\nretreiving data | mahebourg\nretreiving data | vanavara\nretreiving data | lat yao\nretreiving data | talnakh\nIf city is not found, skipping\nretreiving data | east london\nretreiving data | maningrida\nIf city is not found, skipping\nretreiving data | altamira\nretreiving data | ilulissat\nretreiving data | san patricio\nretreiving data | lucea\nretreiving data | shenjiamen\nretreiving data | lebu\nretreiving data | kisangani\nIf city is not found, skipping\nretreiving data | mpika\nretreiving data | quime\nretreiving data | severo-kurilsk\nretreiving data | faanui\nIf city is not found, skipping\nretreiving data | chokurdakh\nretreiving data | bismarck\nretreiving data | shaowu\nIf city is not found, skipping\nretreiving data | tarko-sale\nretreiving data | sao miguel do araguaia\nretreiving data | guerrero negro\nretreiving data | grindavik\nIf city is not found, skipping\nretreiving data | vaini\nretreiving data | lorengau\nretreiving data | butaritari\nretreiving data | kapaa\nretreiving data | santa fe\nretreiving data | saint-pierre\nretreiving data | tasiilaq\nretreiving data | upernavik\nretreiving data | arraial do cabo\nretreiving data | mount gambier\nretreiving data | ternate\nretreiving data | kasempa\nretreiving data | puerto del rosario\nretreiving data | znamenskoye\nretreiving data | santa cruz\nretreiving data | nyurba\nretreiving data | airai\nretreiving data | cape town\nretreiving data | georgetown\nretreiving data | kodiak\nretreiving data | carutapera\nretreiving data | fortuna\nretreiving data | bethel\nretreiving data | bantou\nretreiving data | kalmunai\nretreiving data | samarai\nretreiving data | hamilton\nretreiving data | hithadhoo\nretreiving data | sur\nretreiving data | clyde river\nretreiving data | whitehorse\nretreiving data | saint-philippe\nretreiving data | oistins\nretreiving data | sobolevo\nretreiving data | chimore\nIf city is not found, skipping\nretreiving data | vastseliina\nretreiving data | brenham\nretreiving data | pogar\nretreiving data | dikson\nretreiving data | yeppoon\nIf city is not found, skipping\nretreiving data | qaanaaq\nretreiving data | ancud\nretreiving data | atar\nretreiving data | yellowknife\nretreiving data | cabo san lucas\nretreiving data | san ramon\nretreiving data | kavieng\nretreiving data | yar-sale\nretreiving data | kozienice\nretreiving data | carolina\nIf city is not found, skipping\nretreiving data | hasaki\nretreiving data | gandai\nretreiving data | los llanos de aridane\nretreiving data | lana\nretreiving data | palana\nretreiving data | gaurnadi\nretreiving data | constitucion\nretreiving data | grand gaube\nretreiving data | dunedin\nretreiving data | avarua\nretreiving data | jamsa\nIf city is not found, skipping\nIf city is not found, skipping\nIf city is not found, skipping\nretreiving data | buin\nretreiving data | leningradskiy\nretreiving data | curup\nretreiving data | charlestown\nretreiving data | togur\nretreiving data | birao\nretreiving data | high rock\nretreiving data | inhambane\nretreiving data | puerto ayora\nretreiving data | ossora\nretreiving data | carnarvon\nretreiving data | bud\nretreiving data | placido de castro\nretreiving data | kaeo\nretreiving data | san francisco del mar\nretreiving data | maragogi\nIf city is not found, skipping\nretreiving data | siilinjarvi\nretreiving data | kroya\nretreiving data | canakkale\nretreiving data | sindor\nretreiving data | atambua\nretreiving data | tonstad\nIf city is not found, skipping\nretreiving data | ribeira grande\nretreiving data | belyy yar\nretreiving data | tateyama\nIf city is not found, skipping\nretreiving data | santander jimenez\nretreiving data | ugoofaaru\nretreiving data | ammokhorion\nretreiving data | pevek\nretreiving data | touros\nretreiving data | rocha\nretreiving data | santa marta\nretreiving data | brae\nretreiving data | dwarka\nretreiving data | am timan\nretreiving data | poum\nIf city is not found, skipping\nIf city is not found, skipping\nretreiving data | cherskiy\nretreiving data | karamakhi\nretreiving data | tuatapere\nIf city is not found, skipping\nretreiving data | sirjan\nretreiving data | srednekolymsk\nretreiving data | torbay\nIf city is not found, skipping\nIf city is not found, skipping\nIf city is not found, skipping\nretreiving data | ibra\nretreiving data | iqaluit\nretreiving data | soyo\nretreiving data | sorong\nIf city is not found, skipping\nretreiving data | beidao\nretreiving data | khash\nretreiving data | nanortalik\nretreiving data | kulgam\nretreiving data | ryotsu\nretreiving data | la grande\nretreiving data | syava\nretreiving data | katsuura\nretreiving data | suntar\nretreiving data | portland\nretreiving data | rio grande\nretreiving data | prince rupert\nretreiving data | cidreira\nretreiving data | veselynove\nretreiving data | nanakuli\nIf city is not found, skipping\nretreiving data | zhigansk\nIf city is not found, skipping\nretreiving data | flin flon\nretreiving data | hami\nIf city is not found, skipping\nretreiving data | nueve de julio\nretreiving data | sao filipe\nretreiving data | kampene\nretreiving data | saint george\nretreiving data | provideniya\nretreiving data | khatanga\nretreiving data | yenagoa\nretreiving data | bavly\nretreiving data | coari\nretreiving data | ville-marie\nretreiving data | kununurra\nretreiving data | moissala\nretreiving data | lata\nretreiving data | zory\nretreiving data | tiksi\nretreiving data | zharkent\nIf city is not found, skipping\nretreiving data | pisco\nretreiving data | puerto escondido\nIf city is not found, skipping\nIf city is not found, skipping\nretreiving data | haines junction\nretreiving data | barcelos\nIf city is not found, skipping\nretreiving data | libreville\nretreiving data | faratsiho\nretreiving data | chuy\nretreiving data | boca do acre\nretreiving data | nepomuceno\nretreiving data | lompoc\nretreiving data | broome\nretreiving data | okhotsk\nretreiving data | avera\nretreiving data | meulaboh\nretreiving data | beloha\nretreiving data | longyearbyen\nretreiving data | nekrasovka\nIf city is not found, skipping\nIf city is not found, skipping\nretreiving data | saskylakh\nretreiving data | mar del plata\nretreiving data | muisne\nretreiving data | thompson\nretreiving data | atasu\nretreiving data | ribeira brava\nretreiving data | alofi\nretreiving data | college\nretreiving data | benguela\nretreiving data | ulladulla\nretreiving data | saldanha\nretreiving data | palmer\nretreiving data | sanbu\nretreiving data | salalah\nretreiving data | the valley\nretreiving data | deputatskiy\nretreiving data | mushie\nretreiving data | kavaratti\nretreiving data | klaksvik\nIf city is not found, skipping\nretreiving data | irati\nretreiving data | koltubanovskiy\nretreiving data | mudanya\n"
],
[
"#print(data_dict)\ndf = pd.DataFrame.from_dict(data_dict)\ndf.head()",
"_____no_output_____"
]
],
[
[
"### Convert Raw Data to DataFrame\n* Export the city data into a .csv.\n* Display the DataFrame",
"_____no_output_____"
]
],
[
[
"df.count()",
"_____no_output_____"
],
[
"#Convert file to csv and save\ndf.to_csv(\"weather_data.csv\", encoding=\"utf-8\", index=False)",
"_____no_output_____"
]
],
[
[
"### Plotting the Data\n* Use proper labeling of the plots using plot titles (including date of analysis) and axes labels.\n* Save the plotted figures as .pngs.",
"_____no_output_____"
],
[
"#### Latitude vs. Temperature Plot",
"_____no_output_____"
]
],
[
[
"# Build a scatter plot for each data type\nplt.scatter(df[\"latitude\"], df[\"max temp\"], marker=\"o\")\n\n# Incorporate the other graph properties\nplt.title(\"City Latitude vs. Temperature (F)\")\nplt.ylabel(\"Temperature (F)\")\nplt.xlabel(\"Latitude\")\nplt.grid(True)\n\n# Save the figure\nplt.savefig(\"Temperature (F).png\")\n\n# Show plot\nplt.show()",
"_____no_output_____"
]
],
[
[
"#### Latitude vs. Humidity Plot",
"_____no_output_____"
]
],
[
[
"# Build a scatter plot for each data type\nplt.scatter(df[\"latitude\"], df[\"humidity\"], marker=\"o\")\n\n# Incorporate the other graph properties\nplt.title(\"City Latitude vs. Humidity %\")\nplt.ylabel(\"Humidity %\")\nplt.xlabel(\"Latitude\")\nplt.grid(True)\n\n# Save the figure\nplt.savefig(\"Humidity%.png\")\n\n# Show plot\nplt.show()",
"_____no_output_____"
]
],
[
[
"#### Latitude vs. Cloudiness Plot",
"_____no_output_____"
]
],
[
[
"# Build a scatter plot for each data type\nplt.scatter(df[\"latitude\"], df[\"cloudiness\"], marker=\"o\")\n\n# Incorporate the other graph properties\nplt.title(\"City Latitude vs. Cloudiness %\")\nplt.ylabel(\"Cloudiness %\")\nplt.xlabel(\"Latitude\")\nplt.grid(True)\n\n# Save the figure\nplt.savefig(\"Clouds%.png\")\n\n# Show plot\nplt.show()",
"_____no_output_____"
]
],
[
[
"#### Latitude vs. Wind Speed Plot",
"_____no_output_____"
]
],
[
[
"# Build a scatter plot for each data type\nplt.scatter(df[\"latitude\"], df[\"windspeed\"], marker=\"o\")\n\n# Incorporate the other graph properties\nplt.title(\"City Latitude vs. Windspeed (mph)\")\nplt.ylabel(\"Windspeed (mph)\")\nplt.xlabel(\"Latitude\")\nplt.grid(True)\n\n# Save the figure\nplt.savefig(\"Windspeed(mph).png\")\n\n# Show plot\nplt.show()",
"_____no_output_____"
]
]
] | [
"markdown",
"code",
"markdown",
"code",
"markdown",
"code",
"markdown",
"code",
"markdown",
"code",
"markdown",
"code",
"markdown",
"code",
"markdown",
"code"
] | [
[
"markdown"
],
[
"code"
],
[
"markdown"
],
[
"code",
"code"
],
[
"markdown"
],
[
"code",
"code"
],
[
"markdown"
],
[
"code",
"code"
],
[
"markdown",
"markdown"
],
[
"code"
],
[
"markdown"
],
[
"code"
],
[
"markdown"
],
[
"code"
],
[
"markdown"
],
[
"code"
]
] |
d08f426405c06bf4e413d18e1fec2cdb20d51ad9 | 4,401 | ipynb | Jupyter Notebook | M1_Python/d3_Intro_to_Python/02_exercises/Intro to Python - Easy.ipynb | succeedme/Strive_Main | 1da5da7ca5042451225a4c47768a326a81b3a4fe | [
"Apache-2.0"
] | null | null | null | M1_Python/d3_Intro_to_Python/02_exercises/Intro to Python - Easy.ipynb | succeedme/Strive_Main | 1da5da7ca5042451225a4c47768a326a81b3a4fe | [
"Apache-2.0"
] | null | null | null | M1_Python/d3_Intro_to_Python/02_exercises/Intro to Python - Easy.ipynb | succeedme/Strive_Main | 1da5da7ca5042451225a4c47768a326a81b3a4fe | [
"Apache-2.0"
] | null | null | null | 30.143836 | 610 | 0.575324 | [
[
[
"Here you have a collection of guided exercises for the first class on Python. <br>\nThe exercises are divided by topic, following the topics reviewed during the theory session, and for each topic you have some mandatory exercises, and other optional exercises, which you are invited to do if you still have time after the mandatory exercises. <br>\n\nRemember that you have 5 hours to solve these exercises, after which we will review the most interesting exercises together. If you don't finish all the exercises, you can work on them tonightor tomorrow. \n\nAt the end of the class, we will upload the code with the solutions of the exercises so that you can review them again if needed. If you still have not finished some exercises, try to do them first by yourself, before taking a look at the solutions: you are doing these exercises for yourself, so it is always the best to do them your way first, as it is the fastest way to learn!",
"_____no_output_____"
],
[
"**Exercise 1.1:** The cover price of a book is 24.95 EUR, but bookstores get a 40 percent discount. Shipping costs 3 EUR for the first copy and 75 cents for each additional copy. **Calculate the total wholesale costs for 60 copies**. ",
"_____no_output_____"
]
],
[
[
"#Your Code Here",
"_____no_output_____"
]
],
[
[
"**Exercise 1.2:** When something is wrong with your code, Python will raise errors. Often these will be \"syntax errors\" that signal that something is wrong with the form of your code (e.g., the code in the previous exercise raised a `SyntaxError`). There are also \"runtime errors\", which signal that your code was in itself formally correct, but that something went wrong during the code's execution. A good example is the `ZeroDivisionError`, which indicates that you tried to divide a number by zero (which, as you may know, is not allowed). Try to make Python **raise such a `ZeroDivisionError`.**",
"_____no_output_____"
]
],
[
[
"#Your Code Here\n",
"_____no_output_____"
]
],
[
[
"**Exercise 5.1**: Create a countdown function that starts at a certain count, and counts down to zero. Instead of zero, print \"Blast off!\". Use a `for` loop. \n",
"_____no_output_____"
]
],
[
[
"# Countdown\ndef countdown():\n \"\"\"\n 20\n 19\n 18\n 17\n 16\n 15\n 14\n 13\n 12\n 11\n 10\n 9\n 8\n 7\n 6\n 5\n 4\n 3\n 2\n 1\n Blast off!\n \"\"\"\n return\n",
"_____no_output_____"
]
],
[
[
"**Exercise 5.2:** Write and test three functions that return the largest, the smallest, and the number of dividables by 3 in a given collection of numbers. Use the algorithm described earlier in the Part 5 lecture :)",
"_____no_output_____"
]
],
[
[
"# Your functions\ndef main():\n \"\"\"\n a = [2, 4, 6, 12, 15, 99, 100]\n 100\n 2\n 4\n \"\"\"\n return\n\n",
"_____no_output_____"
]
]
] | [
"markdown",
"code",
"markdown",
"code",
"markdown",
"code",
"markdown",
"code"
] | [
[
"markdown",
"markdown"
],
[
"code"
],
[
"markdown"
],
[
"code"
],
[
"markdown"
],
[
"code"
],
[
"markdown"
],
[
"code"
]
] |
d08f475be041df48d1842e6030bef3063ddfe06c | 239,357 | ipynb | Jupyter Notebook | ipython/compare_mono_50/logOutput/plot_composition_figure.ipynb | kolbt/whingdingdilly | 4c17b594ebc583750fe7565d6414f08678ea7882 | [
"BSD-3-Clause"
] | 4 | 2017-09-04T14:36:57.000Z | 2022-03-28T23:24:58.000Z | ipython/compare_mono_50/logOutput/plot_composition_figure.ipynb | kolbt/whingdingdilly | 4c17b594ebc583750fe7565d6414f08678ea7882 | [
"BSD-3-Clause"
] | null | null | null | ipython/compare_mono_50/logOutput/plot_composition_figure.ipynb | kolbt/whingdingdilly | 4c17b594ebc583750fe7565d6414f08678ea7882 | [
"BSD-3-Clause"
] | null | null | null | 245.494359 | 47,196 | 0.896435 | [
[
[
"# Import that good good\nimport sys\nimport os\nsys.path.append('/Users/kolbt/Desktop/ipython/diam_files')\nimport pandas as pd\nimport matplotlib.pyplot as plt\nimport numpy as np \nimport math\nfrom IPython.display import display\nfrom collections import OrderedDict\npd.options.display.max_rows = 2\nimport matplotlib.colors as mc\nimport colorsys",
"_____no_output_____"
],
[
"# Define what functions you'll need here\ndef getFromTxt(fname, first, last):\n \"Takes a string, text before and after desired text, outs text between\"\n start = fname.index( first ) + len( first )\n end = fname.index( last, start )\n myTxt = fname[start:end]\n return float(myTxt)\n# Above function kindly provided by user \"cji\" on stackoverflow\n# https://stackoverflow.com/questions/3368969/find-string-between-two-substrings\n\n# Make sure data is sorted appropriately\ndef sortArray(array, sort_var):\n \"Takes an array and the column name to sort, sorts array \"\n for i in range(0, len(array)):\n for k in range(0, len(array[i])):\n for j in range(0, len(array[i])):\n # Out of order, swap them\n if array[i].loc[j, sort_var] < array[i].loc[k, sort_var] and k < j:\n tmp = array[i].iloc[j].copy()\n array[i].iloc[j] = array[i].iloc[k]\n array[i].iloc[k] = tmp\n \ndef plotter(start, stop, ylab):\n \"Plotting function so that I don't have to show this a zillion times\"\n ind = 0\n for j in range(start, stop):\n for i in range(0, len(SS[headers[j]])):\n # Mixture\n if params['xA'][i] % 100 != 0:\n plt.scatter(params['peA'][i], SS[headers[j]][i], c=col[ind], label=headers[j])\n # Monodisperse, always same color\n else:\n # If it's zero ignore it\n if SS[headers[j]][i] != 0:\n plt.scatter(params['peA'][i], SS[headers[j]][i], c=col[-1], label='Mono')\n ind += 1\n \n handles, labels = plt.gca().get_legend_handles_labels()\n by_label = OrderedDict(zip(labels, handles))\n plt.legend(by_label.values(), by_label.keys())\n plt.xlabel('Activity (Pe)')\n plt.ylabel(ylab)\n plt.show()\n \ndef katieRoseIsCute(r):\n \"Take diameter, output LJ-force\"\n eps = 20.0\n sigma = 1.0\n F_LJ = 24.0 * eps * ((2 * (sigma**12) * (r**-13)) - ((sigma**6) * (r**-7)))\n return F_LJ\n\ndef forceToEps(force):\n \"Take LJ-force and output epsilon to give diameter of 1\"\n epsilon = force / 24.0\n return epsilon\n\n# https://mycurvefit.com/\n\ndef powerLaw(a, x, b):\n return a*(x**b)\n\ndef exponential(a, b, c, x):\n \"Exponential: a - (b/c) * (1 - (e**-cx))\"\n return a - ((b/c)*(1-(math.exp(-c*x))))\n\ndef plateau(a, x, b):\n \"Plateau: a * x / (b + x)\"\n return (a * x) / (b + x)\n\ndef logarithmic(a, x, b):\n \"Logarithmic: a * ln(x) + b\"\n if x != 0:\n return (a * math.log(x)) + b\n else:\n return 0\n \n# https://stackoverflow.com/questions/37765197/darken-or-lighten-a-color-in-matplotlib\ndef colorShade(color, amount=0.5):\n \"Gives multiple shades of a base color\"\n try:\n c = mc.cnames[color]\n except:\n c = color\n c = colorsys.rgb_to_hls(*mc.to_rgb(c))\n return colorsys.hls_to_rgb(c[0], 1 - amount * (1 - c[1]), c[2])",
"_____no_output_____"
],
[
"# Get the data files\ntxtFiles = os.listdir('gsd')\nall_sims = []",
"_____no_output_____"
],
[
"# Using the absolute path means I can go to whatever directory I want\nos.chdir('/Users/kolbt/Desktop/ipython/diam_files')\nfor i in range(0, len(txtFiles)):\n df = pd.read_csv(txtFiles[i], sep='\\s+', header=0)\n all_sims.append(df)\n \n# Return to root directory\nos.chdir('/Users/kolbt/Desktop/ipython')",
"_____no_output_____"
],
[
"# Make sure all data is in correct timestep order\nsortArray(all_sims, 'Timestep')\ndisplay(all_sims[9])",
"_____no_output_____"
],
[
"# Make an additional frame that gives total number of particles, and simulation parameters\nparamList = []\nfor i in range(0, len(txtFiles)):\n partAll = all_sims[i]['Gas_tot'][0]\n partA = all_sims[i]['Gas_A'][0]\n partB = all_sims[i]['Gas_B'][0]\n pa = getFromTxt(txtFiles[i], \"pa\", \"_pb\")\n pb = getFromTxt(txtFiles[i], \"pb\", \"_xa\")\n xa = getFromTxt(txtFiles[i], \"xa\", \".txt\")\n try:\n prat = float(pa)/float(pb)\n except:\n prat = 0.0\n paramList.append((partAll, partA, partB, pa, pb, xa, prat))\n\nparams = pd.DataFrame(paramList, columns=['partAll', 'partA', 'partB', 'peA', 'peB', 'xA', 'peR'])\ndisplay(params)",
"_____no_output_____"
],
[
"# Make list of steady state column headers\nheaders = list(all_sims[0])\nheaders.remove('Timestep')\nSS = pd.DataFrame(columns=headers)\nfor i in range(0, len(txtFiles)):\n SS.loc[i] = [0] * len(headers)\n\n# Make dataframe of steady-state data\nfor i in range(0, len(txtFiles)):\n # Loop through each column (aside from tstep column)\n for j in range(1, len(headers) + 1):\n # Compute mean of last 100 entries in jth column of ith file\n avg = np.mean(all_sims[i].iloc[-100:-1,j])\n SS[headers[j-1]][i] = avg\n \n# Normalize by number of particles\n# SS['Gas_A'][:] /= params['partA'][:]\n# SS['Gas_B'][:] /= params['partB'][:]\n# SS['Gas_tot'][:] /= params['partAll'][:]\n# SS['Dense_A'][:] /= params['partA'][:]\n# SS['Dense_B'][:] /= params['partB'][:]\n# SS['Dense_tot'][:] /= params['partAll'][:]\n# SS['Lg_clust'][:] /= params['partAll'][:]\n# SS['MCS'][:] /= params['partAll'][:]\ndisplay(SS)",
"_____no_output_____"
],
[
"# Plot the data\n# col = ['k', 'r', 'g', 'b']\ncol = ['#e6194b', '#3cb44b', '#0082c8', '#f58231', '#ffe119','#911eb4', '#46f0f0',\n '#f032e6', '#d2f53c', '#fabebe', '#008080', '#e6beff', '#aa6e28', '#fffac8',\n '#800000', '#aaffc3', '#808000', '#ffd8b1', '#000080', '#808080', '#ffffff',\n '#000000']\nplotter(0, 3, '% of total particles')\nplotter(3, 6, '% of total particles')\nplotter(6, 8, '% of total particles')\nplotter(8, 12, r'Diameter $(\\sigma)$')\nplotter(12, 13, r'Effective Area Fraction $(\\phi_{Eff})$')\nplotter(13, 15, 'Area')\nplotter(15, 17, 'Density')\nplotter(17, 18, 'Density')\n\n# # This is the way I was plotting it\n# for j in range(0, 3):\n# plt.scatter(params['peA'], SS[headers[j]], label=headers[j])\n# plt.legend()\n# plt.show()",
"_____no_output_____"
],
[
"# Take in the steady-state diameter data... output the LJ force w/ HS epsilon\ndiam_to_force = []\neps_one = []\nfor i in range(0, len(SS['sigALL'])):\n diam_to_force.append(katieRoseIsCute(SS['sigALL'][i]))\n eps_one.append(forceToEps(diam_to_force[i]))",
"_____no_output_____"
],
[
"# https://onlinelibrary.wiley.com/doi/pdf/10.1002/9780470126714.app4\n# Good ideas for plotting are:\n# Exponential: a - (b/c) * (1 - (e**-cx))\n# Power: a * x ** b\n# Plateau: a * x / (b + x)\n# Log: a * ln(x) + b\n\n# Let's fix the data being plotted (just monodisperse)\nmono = [0]\ncorDat = [1]\nfor i in range(0, len(params['peA'])):\n if params['xA'][i] % 100 == 0:\n mono.append(params['peA'][i])\n corDat.append(eps_one[i])\n\npowla = []\nexpo = []\nplato = []\nloga = []\nrefRange = np.arange(0, 500, 0.001)\nfor i in range(0, len(refRange)):\n powla.append(powerLaw(5.87, refRange[i], 0.36))\n expo.append(exponential(9.4, -0.28, 0.006, refRange[i]))\n plato.append(plateau(62.4, refRange[i], 99.1))\n loga.append(logarithmic(1.0, refRange[i], 1.0))\n\nplt.scatter(mono, corDat, c=col[8], label='Data')\nplt.plot(refRange, powla, c=col[9], label='Power Law')\nplt.xlabel('Activity')\nplt.ylabel('Epsilon')\nplt.legend()\nplt.title(r'$\\epsilon$ to give $\\sigma=1$')\nplt.show()\n\nplt.scatter(mono, corDat, c=col[8], label='Data')\nplt.plot(refRange, expo, c=col[10], label='Exponential')\nplt.xlabel('Activity')\nplt.ylabel('Epsilon')\nplt.legend()\nplt.title(r'$\\epsilon$ to give $\\sigma=1$')\nplt.show()\n\nplt.scatter(mono, corDat, c=col[8], label='Data')\nplt.plot(refRange, plato, c=col[11], label='Plateau')\nplt.xlabel('Activity')\nplt.ylabel('Epsilon')\nplt.legend()\nplt.title(r'$\\epsilon$ to give $\\sigma=1$')\nplt.show()\n\n# plt.scatter(mono, corDat, c=col[8], label='Data')\n# plt.plot(refRange, loga, c=col[12], label='Logarithmic')\n# plt.xlabel('Activity')\n# plt.ylabel('Epsilon')\n# plt.legend()\n# plt.title(r'$\\epsilon$ to give $\\sigma=1$')\n# plt.show()",
"_____no_output_____"
],
[
"print('Monodisperse Data:')\nfor i in range(0, len(eps_one)):\n # monodisperse\n if params['xA'][i] % 100 == 0:\n print('Activity: {}, Epsilon: {}').format(params['peA'][i], eps_one[i])\n# print('Monodisperse Data:')\n# for i in range(0, len(eps_one)):\n# # monodisperse\n# if params['xA'][i] % 100 == 0:\n# print('{} \\t {}').format(params['peA'][i], eps_one[i])",
"Monodisperse Data:\nActivity: 100.0, Epsilon: 26.8534827824\nActivity: 150.0, Epsilon: 37.6397874077\nActivity: 200.0, Epsilon: 41.5519027436\nActivity: 250.0, Epsilon: 44.6809112352\nActivity: 300.0, Epsilon: 46.7550043594\nActivity: 350.0, Epsilon: 48.4091749773\nActivity: 400.0, Epsilon: 50.0417962436\nActivity: 450.0, Epsilon: 50.9646829236\nActivity: 500.0, Epsilon: 52.7877441901\nActivity: 50.0, Epsilon: 21.8620004183\nActivity: 60.0, Epsilon: 22.8955530146\n"
],
[
"# Plot the composition data? Inset the plot composition over time\n# A will be one color, dark = high Pe_ratio, light = low Pe_r\n# Same goes for B and all\n\nmixPar = []\nmixA = []\nmixB = []\nmixT = []\nmixInds = []\nfor i in range(0, len(params['peA'])):\n # Mixtures only\n if params['xA'][i] % 100 != 0:\n mixInds.append(i)\n mixPar.append(params['peR'][i])\n mixA.append(SS['Dense_A'][i] / params['partA'][i])\n mixB.append(SS['Dense_B'][i] / params['partB'][i])\n mixT.append(SS['Dense_tot'][i] / params['partAll'][i])\n \nplt.scatter(mixPar, mixT, label='All', c='g')\nplt.scatter(mixPar, mixA, label='A', c='b')\nplt.scatter(mixPar, mixB, label='B', c='r')\nplt.xlabel('Activity Ratio')\nplt.ylabel('Percentage of Total')\n\nmixedSims = len(mixInds)\ntimeB = [[] for x in xrange(mixedSims)]\nsimDenseA = [[] for x in xrange(mixedSims)]\nsimDenseB = [[] for x in xrange(mixedSims)]\nsimDenseT = [[] for x in xrange(mixedSims)]\n\ncount = -1\n# Let's get data for the inset\nfor i in range(0, len(txtFiles)):\n if params['xA'][i] % 100 != 0:\n count += 1\n # Get the tau_B time\n timeB[count].append(np.arange(0, len(all_sims[i]['Timestep']), 1))\n for j in range(0, len(all_sims[i]['Timestep'])):\n # Group all Dense_A data\n simDenseT[count].append(all_sims[i]['Dense_tot'][j])\n simDenseA[count].append(all_sims[i]['Dense_A'][j])\n simDenseB[count].append(all_sims[i]['Dense_B'][j])\n # Divide column by number of A particles\n simDenseT[count] /= params['partAll'][i]\n simDenseA[count] /= params['partA'][i]\n simDenseB[count] /= params['partB'][i]\n \n# Plot the data All\na = plt.axes([0.475, .25, .4, .4], facecolor='w')\nfor i in range(0, mixedSims):\n plt.plot(timeB[i][0], simDenseT[i], c=colorShade('g', mixPar[i]))\nplt.xlim(0, 10)\nplt.ylim(0,1)\nplt.xlabel(r'Time $(\\tau_{B})$')\nplt.ylabel(r'% of Total') \n\n# Plot the data A\na = plt.axes([1.02, .575, .3, .3], facecolor='w')\nfor i in range(0, mixedSims):\n plt.plot(timeB[i][0], simDenseA[i], c=colorShade('b', mixPar[i]))\nplt.xlim(0, 10)\nplt.ylim(0,1)\nplt.ylabel(r'% of Total A')\n\n# Plot the data B\na = plt.axes([1.02, .15, .3, .3], facecolor='w')\nfor i in range(0, mixedSims):\n plt.plot(timeB[i][0], simDenseB[i], c=colorShade('r', mixPar[i]))\nplt.xlim(0, 10)\nplt.ylim(0,1)\nplt.xlabel(r'Time $(\\tau_{B})$')\nplt.ylabel(r'% of Total B')",
"_____no_output_____"
]
]
] | [
"code"
] | [
[
"code",
"code",
"code",
"code",
"code",
"code",
"code",
"code",
"code",
"code",
"code",
"code"
]
] |
d08f4ad8294cb5921dd8a7836d4d5d68e16bd00e | 80,811 | ipynb | Jupyter Notebook | notebooks/Magnetisation R.ipynb | dougmet/big-ising-paper | 5b1de4441020f16f5128c2c3bd0967a94d672be0 | [
"MIT"
] | null | null | null | notebooks/Magnetisation R.ipynb | dougmet/big-ising-paper | 5b1de4441020f16f5128c2c3bd0967a94d672be0 | [
"MIT"
] | null | null | null | notebooks/Magnetisation R.ipynb | dougmet/big-ising-paper | 5b1de4441020f16f5128c2c3bd0967a94d672be0 | [
"MIT"
] | null | null | null | 888.032967 | 79,144 | 0.948262 | [
[
[
"library(ggplot2)\nlibrary(yaml)",
"_____no_output_____"
],
[
"em_raw <- read.csv(\"../data/em.csv\", header = FALSE, col.names = c(\"M\", \"E\"))\n\n# Cut off four correlation times\nem <- em_raw[-(1:200), ]",
"_____no_output_____"
],
[
"metadata <- yaml.load_file(\"../data/metadata.yaml\")\nN <- as.integer(metadata$N)",
"_____no_output_____"
],
[
"g <- ggplot(em) +\n geom_freqpoly(aes(x = M / N, y = ..density..), bins = 100) + \n labs(x = \"Magnetisation per spin, m\", y = \"Density, P(m)\") +\n theme_bw()\ng",
"_____no_output_____"
]
]
] | [
"code"
] | [
[
"code",
"code",
"code",
"code"
]
] |
d08f583b52cee8be178aeead240bf3c65c00e754 | 228,788 | ipynb | Jupyter Notebook | examples/example_mauna_loa.ipynb | vishalbelsare/mogptk | 4f7001fbfacea778bd62a1e4e6c5b404c473e313 | [
"MIT"
] | null | null | null | examples/example_mauna_loa.ipynb | vishalbelsare/mogptk | 4f7001fbfacea778bd62a1e4e6c5b404c473e313 | [
"MIT"
] | null | null | null | examples/example_mauna_loa.ipynb | vishalbelsare/mogptk | 4f7001fbfacea778bd62a1e4e6c5b404c473e313 | [
"MIT"
] | null | null | null | 533.305361 | 54,664 | 0.939175 | [
[
[
"# Mauna-Loa CO2 concentration example\n\nExperiment of CO2 measurements in Mauna Loa, Hawaii, using a single output Gaussian process with a spectral mixture kernel. The data set contains daily measurements of CO2 in the air from 1958 to 2001. We will resample the data to obtain 10 averaged samples per year. That is, any yearly pattern will be at $\\frac{1}{10} = 0.1$.",
"_____no_output_____"
]
],
[
[
"import mogptk\nimport numpy as np",
"_____no_output_____"
],
[
"from sklearn.datasets import fetch_openml\n\ndef load_mauna_loa_atmospheric_co2():\n ml_data = fetch_openml(data_id=41187)\n months = []\n ppmv_sums = []\n counts = []\n\n y = ml_data.data['year']\n m = ml_data.data['month']\n month_float = y + (m - 1) / 12\n ppmvs = ml_data.target\n\n for month, ppmv in zip(month_float, ppmvs):\n if not months or month != months[-1]:\n months.append(month)\n ppmv_sums.append(ppmv)\n counts.append(1)\n else:\n # aggregate monthly sum to produce average\n ppmv_sums[-1] += ppmv\n counts[-1] += 1\n\n months = np.asarray(months).reshape(-1)\n avg_ppmvs = np.asarray(ppmv_sums) / counts\n return months, avg_ppmvs",
"_____no_output_____"
]
],
[
[
"First we load the dataset, and define a variable `stop` with the index that separates between train and test",
"_____no_output_____"
]
],
[
[
"# load dataset\nx, y = load_mauna_loa_atmospheric_co2()\n\n# stop omde to separate train from test\nstop = 200\n\ndata = mogptk.Data(x, y, name='Mauna Loa')\ndata.remove_range(start=x[stop])\ndata.transform(mogptk.TransformDetrend(3))\ndata.plot();",
"_____no_output_____"
]
],
[
[
"We initialize the model with random parameters and show the spectral density of the kernel. As we are only taking random values, there is no relation with the data.",
"_____no_output_____"
]
],
[
[
"# create model\nmodel = mogptk.SM(data, Q=10)\nmodel.plot_spectrum(title='SD with random parameters');",
"_____no_output_____"
]
],
[
[
"Then we initialize the parameters before training, using Bayesian Nonparametric spectral estimation (BNSE) (Tobar 2017), and use the estimated Power spectral density (PSD) to define initial spectral mean and magnitudes.",
"_____no_output_____"
]
],
[
[
"method = 'BNSE'\nmodel.init_parameters(method)\nmodel.plot_spectrum(title='PSD with {} initialization'.format(method));",
"_____no_output_____"
]
],
[
[
"Then we train the model and show the power spectral density of the trained model.",
"_____no_output_____"
]
],
[
[
"model.log_marginal_likelihood()",
"_____no_output_____"
],
[
"model.train(method='Adam', iters=1000, lr=0.1, plot=True, error='MAE')\nmodel.plot_spectrum(title='PSD with model trained');",
"\nStart Adam:\n 0/1000 loss= 322.484 error= 1.88645\n 10/1000 loss= 313.976 error= 1.88665\n 20/1000 loss= 307.505 error= 1.88656\n 30/1000 loss= 301.477 error= 1.88645\n 40/1000 loss= 295.067 error= 1.88634\n 50/1000 loss= 288.609 error= 1.88603\n 60/1000 loss= 282.216 error= 1.88578\n 70/1000 loss= 275.716 error= 1.88539\n 80/1000 loss= 269.194 error= 1.88506\n 90/1000 loss= 262.687 error= 1.88457\n 100/1000 loss= 256.224 error= 1.88398\n 110/1000 loss= 249.696 error= 1.88341\n 120/1000 loss= 243.088 error= 1.88278\n 130/1000 loss= 236.521 error= 1.88209\n 140/1000 loss= 230.029 error= 1.88124\n 150/1000 loss= 223.463 error= 1.88004\n 160/1000 loss= 216.782 error= 1.87871\n 170/1000 loss= 209.974 error= 1.87675\n 180/1000 loss= 203.112 error= 1.87421\n 190/1000 loss= 196.303 error= 1.87109\n 200/1000 loss= 189.686 error= 1.86688\n 210/1000 loss= 183.307 error= 1.86204\n 220/1000 loss= 177.049 error= 1.85648\n 230/1000 loss= 170.922 error= 1.851\n 240/1000 loss= 164.705 error= 1.84523\n 250/1000 loss= 158.509 error= 1.84018\n 260/1000 loss= 152.239 error= 1.83473\n 270/1000 loss= 145.8 error= 1.82696\n 280/1000 loss= 138.706 error= 1.81481\n 290/1000 loss= 131.393 error= 1.80203\n 300/1000 loss= 124.501 error= 1.78861\n 310/1000 loss= 118.807 error= 1.77402\n 320/1000 loss= 113.895 error= 1.75941\n 330/1000 loss= 109.753 error= 1.74695\n 340/1000 loss= 106.533 error= 1.72741\n 350/1000 loss= 103.003 error= 1.71038\n 360/1000 loss= 100.341 error= 1.69305\n 370/1000 loss= 97.8219 error= 1.67336\n 380/1000 loss= 95.7121 error= 1.65591\n 390/1000 loss= 93.7487 error= 1.63805\n 400/1000 loss= 92.0012 error= 1.61455\n 410/1000 loss= 90.3272 error= 1.60285\n 420/1000 loss= 88.9001 error= 1.57394\n 430/1000 loss= 87.4867 error= 1.57177\n 440/1000 loss= 85.8493 error= 1.52869\n 450/1000 loss= 84.51 error= 1.54105\n 460/1000 loss= 83.0117 error= 1.48245\n 470/1000 loss= 189.814 error= 1.60444\n 480/1000 loss= 139.043 error= 1.48253\n 490/1000 loss= 111.307 error= 1.4628\n 500/1000 loss= 103.139 error= 1.44905\n 510/1000 loss= 97.2063 error= 1.42853\n 520/1000 loss= 93.0574 error= 1.41599\n 530/1000 loss= 90.3223 error= 1.44419\n 540/1000 loss= 1379.97 error= 2.31593\n 550/1000 loss= 918.444 error= 2.16682\n 560/1000 loss= 404.317 error= 1.95854\n 570/1000 loss= 277.134 error= 1.89074\n 580/1000 loss= 221.99 error= 1.89774\n 590/1000 loss= 201.582 error= 1.89448\n 600/1000 loss= 185.868 error= 1.88753\n 610/1000 loss= 175.018 error= 1.88729\n 620/1000 loss= 166.55 error= 1.88229\n 630/1000 loss= 159.52 error= 1.88005\n 640/1000 loss= 153.676 error= 1.87677\n 650/1000 loss= 148.587 error= 1.87344\n 660/1000 loss= 144.101 error= 1.87071\n 670/1000 loss= 140.099 error= 1.86777\n 680/1000 loss= 136.5 error= 1.86457\n 690/1000 loss= 133.236 error= 1.86129\n 700/1000 loss= 130.252 error= 1.85791\n 710/1000 loss= 127.493 error= 1.85434\n 720/1000 loss= 124.845 error= 1.85024\n 730/1000 loss= 122.235 error= 1.84494\n 740/1000 loss= 119.882 error= 1.84175\n 750/1000 loss= 117.706 error= 1.83885\n 760/1000 loss= 115.665 error= 1.83557\n 770/1000 loss= 113.758 error= 1.83218\n 780/1000 loss= 111.966 error= 1.82898\n 790/1000 loss= 115.227 error= 1.82089\n 800/1000 loss= 264.396 error= 1.86019\n 810/1000 loss= 171.81 error= 1.8587\n 820/1000 loss= 144.88 error= 1.85282\n 830/1000 loss= 134.86 error= 1.85346\n 840/1000 loss= 129.927 error= 1.78689\n 850/1000 loss= 127.451 error= 1.78702\n 860/1000 loss= 124.895 error= 1.78763\n 870/1000 loss= 122.718 error= 1.77601\n 880/1000 loss= 120.73 error= 1.76855\n 890/1000 loss= 118.862 error= 1.76299\n 900/1000 loss= 117.112 error= 1.75689\n 910/1000 loss= 115.454 error= 1.75193\n 920/1000 loss= 113.885 error= 1.74654\n 930/1000 loss= 112.396 error= 1.74071\n 940/1000 loss= 110.978 error= 1.73493\n 950/1000 loss= 109.627 error= 1.7289\n 960/1000 loss= 108.336 error= 1.72269\n 970/1000 loss= 107.099 error= 1.71631\n 980/1000 loss= 105.912 error= 1.70976\n 990/1000 loss= 104.77 error= 1.70318\n 1000/1000 loss= 103.67 error= 1.69641\nFinished\n"
]
],
[
[
"Lastly we predict in the test set.",
"_____no_output_____"
]
],
[
[
"model.predict()\ndata.plot();",
"_____no_output_____"
]
]
] | [
"markdown",
"code",
"markdown",
"code",
"markdown",
"code",
"markdown",
"code",
"markdown",
"code",
"markdown",
"code"
] | [
[
"markdown"
],
[
"code",
"code"
],
[
"markdown"
],
[
"code"
],
[
"markdown"
],
[
"code"
],
[
"markdown"
],
[
"code"
],
[
"markdown"
],
[
"code",
"code"
],
[
"markdown"
],
[
"code"
]
] |
d08f5e4b7ab1adce1852db59bb7436d4ac2aa1d0 | 93,894 | ipynb | Jupyter Notebook | labs/Lab 5A - Neural Machine Translation on TPUs.ipynb | rish-16/machine-learning-workshop | 7c7385da0d4ff7068cff04688cbeb0eb023c2e7c | [
"MIT"
] | 1 | 2020-09-02T18:37:50.000Z | 2020-09-02T18:37:50.000Z | labs/Lab 5A - Neural Machine Translation on TPUs.ipynb | rish-16/machine-learning-workshop | 7c7385da0d4ff7068cff04688cbeb0eb023c2e7c | [
"MIT"
] | null | null | null | labs/Lab 5A - Neural Machine Translation on TPUs.ipynb | rish-16/machine-learning-workshop | 7c7385da0d4ff7068cff04688cbeb0eb023c2e7c | [
"MIT"
] | null | null | null | 101.178879 | 40,138 | 0.774192 | [
[
[
"# TensorFlow Neural Machine Translation on Cloud TPUs\n\nThis tutorial demonstrates how to translate text using a LSTM Network from one language to another (from English to German in this case). We will work with a dataset that contains pairs of English-German phrases. Given a sequence of words in English, we train a model to predict the German equivalent in the sequence.\n\nNote: Enable TPU acceleration to execute this notebook faster. In Colab: Runtime > Change runtime type > Hardware acclerator > **TPU**. \n<br>\nIf running locally make sure TensorFlow version >= 1.11.\n\nThis tutorial includes runnable code implemented using [tf.keras](https://www.tensorflow.org/programmers_guide/keras).\n\nBy Rishabh Anand (GitHub: @rish-16)",
"_____no_output_____"
]
],
[
[
"!ls\n!wget http://www.manythings.org/anki/deu-eng.zip\n!unzip deu-eng.zip",
"sample_data\n--2019-10-12 05:09:34-- http://www.manythings.org/anki/deu-eng.zip\nResolving www.manythings.org (www.manythings.org)... 104.24.108.196, 104.24.109.196, 2606:4700:30::6818:6dc4, ...\nConnecting to www.manythings.org (www.manythings.org)|104.24.108.196|:80... connected.\nHTTP request sent, awaiting response... 200 OK\nLength: 4541707 (4.3M) [application/zip]\nSaving to: ‘deu-eng.zip’\n\ndeu-eng.zip 100%[===================>] 4.33M 17.3MB/s in 0.2s \n\n2019-10-12 05:09:39 (17.3 MB/s) - ‘deu-eng.zip’ saved [4541707/4541707]\n\nArchive: deu-eng.zip\n inflating: deu.txt \n inflating: _about.txt \n"
],
[
"!head deu.txt",
"Hi.\tHallo!\nHi.\tGrüß Gott!\nRun!\tLauf!\nWow!\tPotzdonner!\nWow!\tDonnerwetter!\nFire!\tFeuer!\nHelp!\tHilfe!\nHelp!\tZu Hülf!\nStop!\tStopp!\nWait!\tWarte!\n"
]
],
[
[
"### Importing TensorFlow and other libraries",
"_____no_output_____"
]
],
[
[
"import string\nimport numpy as np\nfrom numpy import array\nimport pandas as pd\nimport tensorflow as tf\nfrom tensorflow.keras.models import Sequential, load_model\nfrom tensorflow.keras.layers import Dense, Embedding, RepeatVector, LSTM\nfrom tensorflow.keras.optimizers import RMSprop\nfrom tensorflow.keras.preprocessing.text import Tokenizer\nfrom tensorflow.keras.preprocessing.sequence import pad_sequences\nfrom sklearn.model_selection import train_test_split\nimport matplotlib.pyplot as plt",
"_____no_output_____"
]
],
[
[
"### Extracting lines from dataset and into array\n\nHere, we can examine how the dataset is structures. The English-German dataset comprises of an English and German phrase separted by a tab `\\t`",
"_____no_output_____"
]
],
[
[
"deu_eng = open('./deu.txt', mode='rt', encoding='utf-8')\ndeu_eng = deu_eng.read()\ndeu_eng = deu_eng.strip().split('\\n')\ndeu_eng = [i.split('\\t') for i in deu_eng]\ndeu_eng = array(deu_eng)\ndeu_eng = deu_eng[:50000, :]\nprint (deu_eng[:5])",
"[['Hi.' 'Hallo!']\n ['Hi.' 'Grüß Gott!']\n ['Run!' 'Lauf!']\n ['Wow!' 'Potzdonner!']\n ['Wow!' 'Donnerwetter!']]\n"
]
],
[
[
"### Removing punctuation\n\nWe will be removing punctuation from the phrases and converting them to lowercase. We will not be creating embeddings for punctuations or uppercase characters as it adds to the complexity of the NMT model",
"_____no_output_____"
]
],
[
[
"deu_eng[:, 0] = [s.translate((str.maketrans('', '', string.punctuation))) for s in deu_eng[:, 0]]\ndeu_eng[:, 1] = [s.translate((str.maketrans('', '', string.punctuation))) for s in deu_eng[:, 1]]\n\nfor i in range(len(deu_eng)):\n deu_eng[i, 0] = deu_eng[i, 0].lower()\n deu_eng[i, 1] = deu_eng[i, 1].lower()\n\nprint (deu_eng[:5])",
"[['hi' 'hallo']\n ['hi' 'grüß gott']\n ['run' 'lauf']\n ['wow' 'potzdonner']\n ['wow' 'donnerwetter']]\n"
]
],
[
[
"### Tokenising the phrases\n\nTokenisation is the process of taking a sequence and chopping it up into smaller pieces called `tokens`. For example, suppose we have a sentence \n\n`\"Bob returned home after the party\"`\n\nThe tokenised sentence will return an array with the tokens:\n\n`[\"Bob\", \"returned\", \"home\", \"after\", \"the\", \"party\"]`\n\nIn this section, we will be breaking up the phrases into tokenised sequences that comprises of numbers for each unique word. For instance, the word \"good\" may have the value of 32 while the word \"boy\" may have the value of 46. Supposing the phrase is \"good boy\", the tokenised sequence is `[32, 46]`.",
"_____no_output_____"
]
],
[
[
"def tokenize(lines):\n tokenizer = Tokenizer()\n tokenizer.fit_on_texts(lines)\n \n return tokenizer\n \neng_tokenizer = tokenize(deu_eng[:, 0])\neng_vocab_size = len(eng_tokenizer.word_index) + 1\neng_sequence_length = 8\nprint ('English vocabulary size: {}'.format(eng_vocab_size))\n\ndeu_tokenizer = tokenize(deu_eng[:, 1])\ndeu_vocab_size = len(deu_tokenizer.word_index) + 1\ndeu_sequence_length = 8\nprint ('German vocabulary size: {}'.format(deu_vocab_size))",
"English vocabulary size: 6352\nGerman vocabulary size: 10678\n"
]
],
[
[
"### Convert lines into sequences as input for the NMT model\n\nWe will now be using our Tokeniser to create tokenised sequences of the original English and German phrases from our dataset.",
"_____no_output_____"
]
],
[
[
"def encode_sequences(tokenizer, sequence_length, lines):\n sequence = tokenizer.texts_to_sequences(lines)\n sequence = pad_sequences(sequence, sequence_length, padding=\"post\") # 0s after the actual sequence\n return sequence",
"_____no_output_____"
]
],
[
[
"### Splitting the dataset into training and testing sets",
"_____no_output_____"
]
],
[
[
"train, test = train_test_split(deu_eng, test_size=.2, random_state=12)\n\nx_train = encode_sequences(deu_tokenizer, deu_sequence_length, train[:, 1])\ny_train = encode_sequences(eng_tokenizer, eng_sequence_length, train[:, 0])\n\nx_test = encode_sequences(deu_tokenizer, deu_sequence_length, test[:, 1])\ny_test = encode_sequences(eng_tokenizer, eng_sequence_length, test[:, 0])\n\nprint (x_train.shape, y_train.shape)\nprint (x_test.shape, x_test.shape)",
"(40000, 8) (40000, 8)\n(10000, 8) (10000, 8)\n"
]
],
[
[
"### Training on a TPU\n\nIn order to connect to a TPU, we can follow 4 easy steps:\n\n1. Connect to a TPU instance\n2. Initialise a parallelly-distributed training `strategy`\n3. Build our NMT model under the `strategy`\n4. Train the model on a TPU\n\nFor more details on training on TPUs for free, feel free to check out [this](https://medium.com/@mail.rishabh.anand/tpu-training-made-easy-with-colab-3b73b920878f) article that covers the process in great detail.",
"_____no_output_____"
],
[
"### Connecting to available TPU instances\n\nHere, we search for available instances of version 2 TPUs (the ones Google publically allocates)",
"_____no_output_____"
]
],
[
[
"tpu = tf.distribute.cluster_resolver.TPUClusterResolver() # TPU detection",
"_____no_output_____"
],
[
"# Initialising a parallelly-distributed training strategy\ntf.tpu.experimental.initialize_tpu_system(tpu)\nstrategy = tf.distribute.experimental.TPUStrategy(tpu, steps_per_run=128)\n\nprint('Running on TPU ', tpu.cluster_spec().as_dict()['worker']) \nprint(\"Number of accelerators: \", strategy.num_replicas_in_sync)",
"INFO:tensorflow:Initializing the TPU system: 10.26.217.42:8470\nINFO:tensorflow:Finished initializing TPU system.\nINFO:tensorflow:Querying Tensorflow master (grpc://10.26.217.42:8470) for TPU system metadata.\nINFO:tensorflow:Found TPU system:\nINFO:tensorflow:*** Num TPU Cores: 8\nINFO:tensorflow:*** Num TPU Workers: 1\nINFO:tensorflow:*** Num TPU Cores Per Worker: 8\nINFO:tensorflow:*** Available Device: _DeviceAttributes(/job:worker/replica:0/task:0/device:CPU:0, CPU, -1, 14268563836271018040)\nINFO:tensorflow:*** Available Device: _DeviceAttributes(/job:worker/replica:0/task:0/device:TPU:0, TPU, 17179869184, 4160020754025681679)\nINFO:tensorflow:*** Available Device: _DeviceAttributes(/job:worker/replica:0/task:0/device:TPU:1, TPU, 17179869184, 5069956673944937267)\nINFO:tensorflow:*** Available Device: _DeviceAttributes(/job:worker/replica:0/task:0/device:TPU:2, TPU, 17179869184, 405365701528337000)\nINFO:tensorflow:*** Available Device: _DeviceAttributes(/job:worker/replica:0/task:0/device:TPU:3, TPU, 17179869184, 18388619540662958942)\nINFO:tensorflow:*** Available Device: _DeviceAttributes(/job:worker/replica:0/task:0/device:TPU:4, TPU, 17179869184, 12649830111410000825)\nINFO:tensorflow:*** Available Device: _DeviceAttributes(/job:worker/replica:0/task:0/device:TPU:5, TPU, 17179869184, 12145800610552195669)\nINFO:tensorflow:*** Available Device: _DeviceAttributes(/job:worker/replica:0/task:0/device:TPU:6, TPU, 17179869184, 2481206146790027268)\nINFO:tensorflow:*** Available Device: _DeviceAttributes(/job:worker/replica:0/task:0/device:TPU:7, TPU, 17179869184, 5555612138287615961)\nINFO:tensorflow:*** Available Device: _DeviceAttributes(/job:worker/replica:0/task:0/device:TPU_SYSTEM:0, TPU_SYSTEM, 8589934592, 14651440226793660407)\nINFO:tensorflow:*** Available Device: _DeviceAttributes(/job:worker/replica:0/task:0/device:XLA_CPU:0, XLA_CPU, 17179869184, 8636738863316355082)\nRunning on TPU ['10.26.217.42:8470']\nNumber of accelerators: 8\n"
],
[
"# Building our model under that strategy\n\nin_vocab = deu_vocab_size\nout_vocab = eng_vocab_size\nunits = 512\nin_timesteps = deu_sequence_length\nout_timesteps = eng_sequence_length\n\nwith strategy.scope():\n model = Sequential()\n model.add(Embedding(in_vocab, units, input_length=in_timesteps, mask_zero=True))\n model.add(LSTM(units))\n model.add(RepeatVector(out_timesteps))\n model.add(LSTM(units, return_sequences=True))\n model.add(Dense(out_vocab, activation='softmax'))\n\n rms = RMSprop(lr=0.001)\n model.compile(loss='sparse_categorical_crossentropy', optimizer=rms)\n\n model.summary()",
"WARNING:tensorflow:From /usr/local/lib/python3.6/dist-packages/tensorflow_core/python/keras/initializers.py:119: calling RandomUniform.__init__ (from tensorflow.python.ops.init_ops) with dtype is deprecated and will be removed in a future version.\nInstructions for updating:\nCall initializer instance with the dtype argument instead of passing it to the constructor\nWARNING:tensorflow:From /usr/local/lib/python3.6/dist-packages/tensorflow_core/python/ops/resource_variable_ops.py:1630: calling BaseResourceVariable.__init__ (from tensorflow.python.ops.resource_variable_ops) with constraint is deprecated and will be removed in a future version.\nInstructions for updating:\nIf using Keras pass *_constraint arguments to layers.\nWARNING:tensorflow:From /usr/local/lib/python3.6/dist-packages/tensorflow_core/python/keras/backend.py:3994: where (from tensorflow.python.ops.array_ops) is deprecated and will be removed in a future version.\nInstructions for updating:\nUse tf.where in 2.0, which has the same broadcast rule as np.where\nModel: \"sequential\"\n_________________________________________________________________\nLayer (type) Output Shape Param # \n=================================================================\nembedding (Embedding) (None, 8, 512) 5467136 \n_________________________________________________________________\nlstm (LSTM) (None, 512) 2099200 \n_________________________________________________________________\nrepeat_vector (RepeatVector) (None, 8, 512) 0 \n_________________________________________________________________\nlstm_1 (LSTM) (None, 8, 512) 2099200 \n_________________________________________________________________\ndense (Dense) (None, 8, 6352) 3258576 \n=================================================================\nTotal params: 12,924,112\nTrainable params: 12,924,112\nNon-trainable params: 0\n_________________________________________________________________\n"
],
[
"tf.keras.utils.plot_model(\n model,\n show_shapes=True,\n show_layer_names=True,\n rankdir=\"TB\"\n)",
"_____no_output_____"
],
[
"history = model.fit(x_train, y_train.reshape(y_train.shape[0], y_train.shape[1], 1), epochs=30, steps_per_epoch=500)",
"Epoch 1/30\n500/500 [==============================] - 12s 23ms/step - loss: 2.2086\nEpoch 2/30\n500/500 [==============================] - 7s 15ms/step - loss: 1.7406\nEpoch 3/30\n500/500 [==============================] - 8s 15ms/step - loss: 1.5482\nEpoch 4/30\n500/500 [==============================] - 8s 16ms/step - loss: 1.2944\nEpoch 5/30\n500/500 [==============================] - 8s 16ms/step - loss: 1.1328\nEpoch 6/30\n500/500 [==============================] - 8s 17ms/step - loss: 0.9843\nEpoch 7/30\n500/500 [==============================] - 9s 17ms/step - loss: 0.8681\nEpoch 8/30\n500/500 [==============================] - 9s 17ms/step - loss: 0.7995\nEpoch 9/30\n500/500 [==============================] - 9s 18ms/step - loss: 0.6747\nEpoch 10/30\n500/500 [==============================] - 9s 19ms/step - loss: 0.6119\nEpoch 11/30\n500/500 [==============================] - 10s 19ms/step - loss: 0.5652\nEpoch 12/30\n500/500 [==============================] - 10s 19ms/step - loss: 0.4462\nEpoch 13/30\n500/500 [==============================] - 10s 20ms/step - loss: 0.4422\nEpoch 14/30\n500/500 [==============================] - 10s 20ms/step - loss: 0.3768\nEpoch 15/30\n500/500 [==============================] - 10s 21ms/step - loss: 0.3660\nEpoch 16/30\n500/500 [==============================] - 10s 21ms/step - loss: 0.3685\nEpoch 17/30\n500/500 [==============================] - 11s 22ms/step - loss: 0.2964\nEpoch 18/30\n500/500 [==============================] - 11s 22ms/step - loss: 0.2531\nEpoch 19/30\n500/500 [==============================] - 11s 22ms/step - loss: 0.2844\nEpoch 20/30\n500/500 [==============================] - 12s 23ms/step - loss: 0.2386\nEpoch 21/30\n500/500 [==============================] - 11s 23ms/step - loss: 0.2292\nEpoch 22/30\n500/500 [==============================] - 12s 23ms/step - loss: 0.2215\nEpoch 23/30\n500/500 [==============================] - 12s 24ms/step - loss: 0.1749\nEpoch 24/30\n500/500 [==============================] - 12s 24ms/step - loss: 0.1745\nEpoch 25/30\n500/500 [==============================] - 12s 25ms/step - loss: 0.1726\nEpoch 26/30\n500/500 [==============================] - 13s 25ms/step - loss: 0.1428\nEpoch 27/30\n500/500 [==============================] - 13s 26ms/step - loss: 0.1585\nEpoch 28/30\n500/500 [==============================] - 13s 26ms/step - loss: 0.1811\nEpoch 29/30\n500/500 [==============================] - 14s 27ms/step - loss: 0.1676\nEpoch 30/30\n500/500 [==============================] - 14s 29ms/step - loss: 0.1636\n"
]
],
[
[
"### Checking the loss values",
"_____no_output_____"
]
],
[
[
"plt.plot(history.history['loss'])\nplt.xlabel('Epochs')\nplt.ylabel('Sparse Categorical Loss')\nplt.legend(['train'])\nplt.show()",
"_____no_output_____"
]
],
[
[
"### Running our model on testing dataset",
"_____no_output_____"
]
],
[
[
"# Getting the predictions from the testing dataset\npreds = model.predict_classes(x_test.reshape(x_test.shape[0], x_test.shape[1])[:10]) # only predicting over 10 instances\nprint (preds)",
"[[ 10 305 7 28 277 0 0 0]\n [ 2 80 1 0 0 0 0 0]\n [ 87 67 70 0 0 0 0 0]\n [ 2 80 16 239 0 0 0 0]\n [ 47 16 1456 0 0 0 0 0]\n [ 856 1 1 0 0 0 0 0]\n [ 55 4 26 91 1027 0 0 0]\n [ 37 244 16 16 166 0 0 0]\n [ 22 1 391 0 0 0 0 0]\n [ 90 11 228 129 11 0 0 0]]\n"
],
[
"# A function to convert a sequence back into words\ndef convert_words(n, tokenizer):\n for word, idx in tokenizer.word_index.items():\n if idx == n:\n return word\n return None",
"_____no_output_____"
],
[
"# Running our model on the testing dataset\npred_texts = []\nfor i in preds:\n temp = []\n for j in range(len(i)):\n word = convert_words(i[j], eng_tokenizer)\n if j > 0:\n if (word == convert_words(i[j-1], eng_tokenizer)) or (word == None):\n temp.append('')\n else:\n temp.append(word)\n else:\n if (word == None):\n temp.append('')\n else:\n temp.append(word)\n \n pred_texts.append(' '.join(temp)) ",
"_____no_output_____"
]
],
[
[
"### Translating the text from German to English\n\nWe can see that our model does a relatively good job in translating the German text to English. However, there are instances that seem to have the wrong translation or are outright incorrect. Nonetheless, for a basic NMT model that was trained for 30 epochs, the model's generalisation is great.",
"_____no_output_____"
]
],
[
[
"pred_df = pd.DataFrame({'actual': test[:10, 0], 'prediction': pred_texts})\n\npred_df",
"_____no_output_____"
]
]
] | [
"markdown",
"code",
"markdown",
"code",
"markdown",
"code",
"markdown",
"code",
"markdown",
"code",
"markdown",
"code",
"markdown",
"code",
"markdown",
"code",
"markdown",
"code",
"markdown",
"code",
"markdown",
"code"
] | [
[
"markdown"
],
[
"code",
"code"
],
[
"markdown"
],
[
"code"
],
[
"markdown"
],
[
"code"
],
[
"markdown"
],
[
"code"
],
[
"markdown"
],
[
"code"
],
[
"markdown"
],
[
"code"
],
[
"markdown"
],
[
"code"
],
[
"markdown",
"markdown"
],
[
"code",
"code",
"code",
"code",
"code"
],
[
"markdown"
],
[
"code"
],
[
"markdown"
],
[
"code",
"code",
"code"
],
[
"markdown"
],
[
"code"
]
] |
d08f6f199897db8f34169109ef3339fea729a0be | 518,520 | ipynb | Jupyter Notebook | docs/examples/daymet.ipynb | DavidChoi76/hydrodata | cf24f1d8f65888c8708ec5dc18714c09a889ba1c | [
"MIT"
] | null | null | null | docs/examples/daymet.ipynb | DavidChoi76/hydrodata | cf24f1d8f65888c8708ec5dc18714c09a889ba1c | [
"MIT"
] | null | null | null | docs/examples/daymet.ipynb | DavidChoi76/hydrodata | cf24f1d8f65888c8708ec5dc18714c09a889ba1c | [
"MIT"
] | null | null | null | 3,389.019608 | 514,112 | 0.960489 | [
[
[
"# Daymet",
"_____no_output_____"
]
],
[
[
"from pynhd import NLDI\nimport pydaymet as daymet\nimport matplotlib.pyplot as plt",
"_____no_output_____"
],
[
"import warnings\nwarnings.filterwarnings(\"ignore\")",
"_____no_output_____"
]
],
[
[
"The Daymet database provides climatology data at 1-km resolution. First, we use [PyNHD](https://github.com/cheginit/pynhd) to get the contributing watershed geometry of a NWIS station with the ID of `USGS-01031500`:",
"_____no_output_____"
]
],
[
[
"geometry = NLDI().getfeature_byid(\"nwissite\", \"USGS-01031500\", basin=True).geometry[0]",
"_____no_output_____"
]
],
[
[
"[PyDaymet](https://github.com/cheginit/pynhd) allows us to get the data for a single pixel or for a region as gridded data. The function to get single pixel is called `pydaymet.get_byloc` and for gridded data is called `pydaymet.get_bygeom`. Both have identical arguments where the first positional argument is a coordinate for the single pixel case or a geometry for the gridded case, and the second posiitonal argument is the dates. The dates can be either a tuple of length two like `(\"2000-01-01\", \"2000-01-31\")` or a list of years like `[2000, 2010]`.\n\nWe can also specify a subset of variables to be downloaded via the ``variables`` argument. The available variables in the Daymet database are ``tmin``, ``tmax``, ``prcp``, ``srad``, ``vp``, ``swe``, ``dayl``.\n\nThere's also a flag for computing Potential EvapoTraspiration (PET) based on the Daymet data. Let's get the precipitaiton, minimum temperature, and PET.",
"_____no_output_____"
]
],
[
[
"variables = [\"prcp\", \"tmin\"]\nclm_g = daymet.get_bygeom(geometry, (\"2000-01-01\", \"2000-01-31\"), variables=variables, pet=True)",
"_____no_output_____"
]
],
[
[
"Note that the default CRS is EPSG:4326. If the input geometry (or coordinate) is in a different CRS we can pass it to the function. The gridded data are automatically masked to the input geometry. Now, Let's get the data for a coordinate in EPSG:3542 CRS.",
"_____no_output_____"
]
],
[
[
"coords = (-1431147.7928, 318483.4618)\ncrs = \"epsg:3542\"\nclm_p = daymet.get_byloc(coords, 2001, crs=crs, variables=variables, pet=True)",
"_____no_output_____"
]
],
[
[
"Now, let's plot the data.",
"_____no_output_____"
]
],
[
[
"fig = plt.figure(figsize=(20, 8), dpi=300)\n\ngs = fig.add_gridspec(2, 2)\n\nax = fig.add_subplot(gs[:, 0])\nclm_g.prcp.isel(time=10).plot(ax=ax)\nax.set_aspect(\"auto\")\n\naxes = gs[:,1].subgridspec(2, 1, hspace=0).subplots(sharex=True)\nclm_p[\"tmin (deg c)\"].plot(ax=axes[0], color=\"r\")\naxes[0].set_ylabel(\"$T_{min}$ (deg C)\")\naxes[0].xaxis.set_ticks_position('none') \nclm_p[\"prcp (mm/day)\"].plot(ax=axes[1])\naxes[1].set_ylabel(\"$P$ (mm/day)\")\n\nplt.tight_layout()",
"_____no_output_____"
]
]
] | [
"markdown",
"code",
"markdown",
"code",
"markdown",
"code",
"markdown",
"code",
"markdown",
"code"
] | [
[
"markdown"
],
[
"code",
"code"
],
[
"markdown"
],
[
"code"
],
[
"markdown"
],
[
"code"
],
[
"markdown"
],
[
"code"
],
[
"markdown"
],
[
"code"
]
] |
d08f82ebf63ba7c64d030b4de6adab34e1d93a8f | 8,119 | ipynb | Jupyter Notebook | Assignment_1.ipynb | NikoStein/_a2-template | b98f3c27edfe2d1e2066e4af187123d140074133 | [
"MIT"
] | null | null | null | Assignment_1.ipynb | NikoStein/_a2-template | b98f3c27edfe2d1e2066e4af187123d140074133 | [
"MIT"
] | null | null | null | Assignment_1.ipynb | NikoStein/_a2-template | b98f3c27edfe2d1e2066e4af187123d140074133 | [
"MIT"
] | null | null | null | 22.490305 | 354 | 0.556349 | [
[
[
"*Practical Data Science 19/20*\n# Programming Assignment",
"_____no_output_____"
],
[
"In this programming assignment you need to apply your new `numpy`, `pandas` and `matplotlib` knowledge. You will need to do several [`groupby`](https://pandas.pydata.org/pandas-docs/stable/generated/pandas.DataFrame.groupby.html)s and [`join`](https://pandas.pydata.org/pandas-docs/stable/generated/pandas.DataFrame.join.html)`s to solve the task. ",
"_____no_output_____"
],
[
"Load required packages",
"_____no_output_____"
]
],
[
[
"import pandas as pd\n%matplotlib inline ",
"_____no_output_____"
]
],
[
[
"Load Data",
"_____no_output_____"
]
],
[
[
"DATA_URL = 'https://raw.githubusercontent.com/pds1920/_a1-template/master/data/'\n\ntransactions = pd.read_csv(DATA_URL + '/sales_train.csv.gz')\nitems = pd.read_csv(DATA_URL + '/items.csv')\nitem_categories = pd.read_csv(DATA_URL + '/item_categories.csv')",
"_____no_output_____"
]
],
[
[
"## Get to know the data\nPrint the **shape** of the loaded dataframes.\n- You can use a list comprehension here",
"_____no_output_____"
]
],
[
[
"# Write your code here",
"_____no_output_____"
]
],
[
[
"Use [`df.head`](https://pandas.pydata.org/pandas-docs/stable/generated/pandas.DataFrame.head.html) function to print several rows of each data frame. Examine the features you are given.",
"_____no_output_____"
]
],
[
[
"# Write your code here",
"_____no_output_____"
],
[
"# Write your code here",
"_____no_output_____"
],
[
"# Write your code here",
"_____no_output_____"
]
],
[
[
"Now use your `pandas` skills to get answers for the following questions. ",
"_____no_output_____"
],
[
"## What was the maximum total revenue among all the shops in June, 2014?\n\n\n* Revenue refers to total sales minus value of goods returned.\n* Sometimes items are returned, find such examples in the dataset. \n* It is handy to split `date` field into [`day`, `month`, `year`] components and use `df.year == 14` and `df.month == 6` in order to select target subset of dates.\n* You may work with `date` feature as with strings, or you may first convert it to `pd.datetime` type with `pd.to_datetime` function, but do not forget to set correct `format` argument.",
"_____no_output_____"
]
],
[
[
"# Write your code here",
"_____no_output_____"
],
[
"max_revenue = # Write your code here",
"_____no_output_____"
],
[
"max_revenue",
"_____no_output_____"
]
],
[
[
"## How many items are there?\n\n* Let's assume, that the items are returned for the same price as they had been sold",
"_____no_output_____"
]
],
[
[
"num_items_constant_price = # Write your code here",
"_____no_output_____"
],
[
"num_items_constant_price",
"_____no_output_____"
]
],
[
[
"## What was the variance of the number of sold items per day sequence for the shop with `shop_id = 25` in December, 2014?\n\n* Do not count the items that were sold but returned back later.\n* Fill `total_num_items_sold`: An (ordered) array that contains the total number of items sold on each day \n* Fill `days`: An (ordered) array that contains all relevant days\n* Then compute variance of the of `total_num_items_sold`\n* If there were no sales at a given day, ***do not*** impute missing value with zero, just ignore that day",
"_____no_output_____"
]
],
[
[
"shop_id = 25\n\n# Write your code here",
"_____no_output_____"
],
[
"total_num_items_sold = # Write your code here\ndays = # Write your code here\ntotal_num_items_sold_var = # Write your code here",
"_____no_output_____"
],
[
"total_num_items_sold_var",
"_____no_output_____"
]
],
[
[
"## Vizualization of the daily items sold\n\nUse `total_num_items_sold` and `days` arrays to and plot the daily revenue of `shop_id = 25` in December, 2014.\n\n* plot-title: 'Daily items sold for shop_id = 25'",
"_____no_output_____"
]
],
[
[
"# Write your code here",
"_____no_output_____"
]
],
[
[
"## What item category that generated the highest revenue in spring 2014?</b></li>\n\n \nSpring is the period from March to Mai.",
"_____no_output_____"
]
],
[
[
"# Write your code here",
"_____no_output_____"
],
[
"category_id_with_max_revenue =# Write your code here",
"_____no_output_____"
],
[
"category_id_with_max_revenue",
"_____no_output_____"
]
]
] | [
"markdown",
"code",
"markdown",
"code",
"markdown",
"code",
"markdown",
"code",
"markdown",
"code",
"markdown",
"code",
"markdown",
"code",
"markdown",
"code",
"markdown",
"code"
] | [
[
"markdown",
"markdown",
"markdown"
],
[
"code"
],
[
"markdown"
],
[
"code"
],
[
"markdown"
],
[
"code"
],
[
"markdown"
],
[
"code",
"code",
"code"
],
[
"markdown",
"markdown"
],
[
"code",
"code",
"code"
],
[
"markdown"
],
[
"code",
"code"
],
[
"markdown"
],
[
"code",
"code",
"code"
],
[
"markdown"
],
[
"code"
],
[
"markdown"
],
[
"code",
"code",
"code"
]
] |
d08f9312105ffdfd1d0a8cfdfa92c2df6082607e | 6,984 | ipynb | Jupyter Notebook | competition/VotingClassifier.ipynb | socket-var/classification-algorithms | 3aae8cc6ca33c877995586eb2091443903ae9f1c | [
"MIT"
] | null | null | null | competition/VotingClassifier.ipynb | socket-var/classification-algorithms | 3aae8cc6ca33c877995586eb2091443903ae9f1c | [
"MIT"
] | null | null | null | competition/VotingClassifier.ipynb | socket-var/classification-algorithms | 3aae8cc6ca33c877995586eb2091443903ae9f1c | [
"MIT"
] | null | null | null | 32.333333 | 177 | 0.533219 | [
[
[
"import numpy as np\nimport pandas as pd\nimport competition_helpers\nfrom sklearn import tree\nfrom sklearn.linear_model import LogisticRegression\nfrom sklearn.svm import SVC\nfrom sklearn.naive_bayes import GaussianNB\nfrom sklearn.ensemble import VotingClassifier, RandomForestClassifier\nfrom sklearn.metrics import accuracy_score, precision_score, recall_score, f1_score",
"_____no_output_____"
],
[
"# I/O configuration here\nX_train = competition_helpers.read_csv(\"train_features.csv\")\ny_train = competition_helpers.read_csv(\"train_label.csv\", remove_header=True)\nX_test = competition_helpers.read_csv(\"test_features.csv\")\nsubmission_col = np.array(pd.read_csv(\"test_features.csv\", header=None).iloc[: , 0]).ravel()\nsubmission_file_name = \"results/voting_default_submission.csv\"\n\nprint(X_train.shape, y_train.shape, X_test.shape)",
"(418, 100) (418, 1) (378, 100)\n"
],
[
"# 5 fold cross validation\n# train_test_split = competition_helpers.kfold_stratified_split(X_train, y_train, 5,False)\n# With standardization\nstandardized_train_test_split = competition_helpers.kfold_stratified_split(X_train, y_train, 5,True)",
"_____no_output_____"
],
[
"# # 5 fold train test split results\n# results = []\n# for estimators_ in [50, 100, 150]:\n# for lr in [0.1, 0.5, 1, 5]:\n# for [(X_train_cv, y_train_cv), (X_test_cv, y_test_cv)] in train_test_split:\n\n# clf = AdaBoostClassifier(random_state=42,\n# base_estimator=tree.DecisionTreeClassifier(\n# max_depth=None, min_samples_split=60, min_samples_leaf= 30\n# ),\n# n_estimators=estimators_,\n# learning_rate=lr\n# )\n# clf.fit(X_train_cv, y_train_cv.ravel()) \n# prediction = clf.predict(X_test_cv)\n\n# accuracy = accuracy_score(y_test_cv.ravel(), prediction.ravel())\n# precision = precision_score(y_test_cv.ravel(), prediction.ravel())\n# recall = recall_score(y_test_cv.ravel(), prediction.ravel())\n# f1 = f1_score(y_test_cv.ravel(), prediction.ravel())\n\n# results.append([accuracy, precision, recall, f1])\n\n\n# measures = np.sum(np.array(results), axis=0) / len(results) \n# print(\"n_estimators: {} learning rate: {} measures: {}\".format(estimators_, lr, measures))",
"_____no_output_____"
],
[
"results = []\nfor [(X_train_cv, y_train_cv), (X_test_cv, y_test_cv)] in standardized_train_test_split:\n \n clf1 = LogisticRegression(random_state=42, solver='saga',max_iter = 2000,multi_class='auto')\n \n clf2 = RandomForestClassifier(random_state=42, n_estimators=100)\n \n# clf3 = GaussianNB()\n \n clf4 = SVC(gamma=\"auto\", probability=True)\n \n \n clf = VotingClassifier(\n estimators=[(\"logistic\", clf1), (\"random_forest\", clf2), \n (\"svm\", clf4)],\n voting=\"soft\",\n weights=[1, 2, 1]\n )\n clf.fit(X_train_cv, y_train_cv.ravel()) \n prediction = clf.predict(X_test_cv)\n\n accuracy = accuracy_score(y_test_cv.ravel(), prediction.ravel())\n precision = precision_score(y_test_cv.ravel(), prediction.ravel())\n recall = recall_score(y_test_cv.ravel(), prediction.ravel())\n f1 = f1_score(y_test_cv.ravel(), prediction.ravel())\n\n results.append([accuracy, precision, recall, f1])\n\n\nmeasures = np.sum(np.array(results), axis=0) / len(results) ",
"_____no_output_____"
],
[
"print(measures)",
"[0.87342648 0.86503165 0.98402458 0.92053296]\n"
],
[
"# fitting the test dataset\n\nclf1 = LogisticRegression(random_state=42, solver='saga',max_iter = 2000,multi_class='auto')\n \nclf2 = RandomForestClassifier(random_state=42, n_estimators=100)\n\n# clf3 = GaussianNB()\n\nclf4 = SVC(gamma=\"auto\", probability=True)\n\n\nclf = VotingClassifier(\n estimators=[(\"logistic\", clf1), (\"random_forest\", clf2), \n (\"svm\", clf4)],\n voting=\"soft\",\n weights=[1, 2, 1]\n )\n\nclf.fit(X_train, y_train.ravel()) \nprediction = clf.predict(X_test)",
"C:\\ProgramData\\Anaconda3\\lib\\site-packages\\sklearn\\linear_model\\sag.py:334: ConvergenceWarning: The max_iter was reached which means the coef_ did not converge\n \"the coef_ did not converge\", ConvergenceWarning)\n"
],
[
"pd.DataFrame({\"id\": submission_col, \"label\": prediction}).to_csv(submission_file_name, encoding='utf-8', index=False)",
"_____no_output_____"
]
]
] | [
"code"
] | [
[
"code",
"code",
"code",
"code",
"code",
"code",
"code",
"code"
]
] |
d08f932384d090e57c8d2e80c9a7264f73b6cd16 | 5,174 | ipynb | Jupyter Notebook | tutorials/6 Analysis/CPW_kappa_calculation_demo.ipynb | mtreinish/qiskit-metal | d0d8203c087a5f1aef87dea92d25be89e5bd6bdf | [
"Apache-2.0"
] | null | null | null | tutorials/6 Analysis/CPW_kappa_calculation_demo.ipynb | mtreinish/qiskit-metal | d0d8203c087a5f1aef87dea92d25be89e5bd6bdf | [
"Apache-2.0"
] | null | null | null | tutorials/6 Analysis/CPW_kappa_calculation_demo.ipynb | mtreinish/qiskit-metal | d0d8203c087a5f1aef87dea92d25be89e5bd6bdf | [
"Apache-2.0"
] | null | null | null | 32.746835 | 697 | 0.607847 | [
[
[
"# Demo Notebook for CPW Kappa Calculation",
"_____no_output_____"
],
[
"Let's start by importing Qiskit Metal: ",
"_____no_output_____"
]
],
[
[
"import qiskit_metal as metal\nfrom qiskit_metal import designs, draw\nfrom qiskit_metal import MetalGUI, Dict, open_docs",
"_____no_output_____"
]
],
[
[
"Next, let's import the function \"kappa_in\" located in the file kappa_calculation.py. This function calculates the photon loss of a CPW resonator which is capacitively coupled to an input transmission line. ",
"_____no_output_____"
]
],
[
[
"# Import the function \"kappa_in\" from the file kappa_calculation.py \nfrom qiskit_metal.analyses.em.kappa_calculation import kappa_in",
"_____no_output_____"
]
],
[
[
"The function \"kappa_in\" takes either three or six arguments, depending on how the lowest resonant frequency of the resonator is handled. In the first case, the resonant frequency of the CPW resonator is calculated numerically (using HFSS, for example) and passed as in floating-point input along with the frequency of interest and the capacitance between the resonator and the transmission line. In the second case, the lowest resonant frequency of the CPW resonator can be estimated by assuming an ideal resonator, in which case some additional inputs are required (1/2 or 1/4 depending on the type of resonator, the resonator length, width of resonator trace, width of resonator gap.)\n\nHere's a quick sanity check to verify that we only get numerical output from this function in the cases of N=3 or N=6 arguments:",
"_____no_output_____"
]
],
[
[
"# SANITY CHECK #1 \n# Let's check that output is only given for three and six arguments \nprint(\"Output for N=1 Arguments:\", kappa_in(1.0))\nprint(\"Output for N=2 Arguments:\", kappa_in(1.0, 1.0))\nprint(\"Output for N=3 Arguments:\", kappa_in(1.0, 1.0, 1.0))\nprint(\"Output for N=4 Arguments:\", kappa_in(1.0, 1.0, 1.0, 1.0))\nprint(\"Output for N=5 Arguments:\", kappa_in(1.0, 1.0, 1.0, 1.0, 1.0))\nprint(\"Output for N=6 Arguments:\", kappa_in(1.0, 1.0, 1.0, 1.0, 1.0, 1.0))\nprint(\"Output for N=7 Arguments:\", kappa_in(1.0, 1.0, 1.0, 1.0, 1.0, 1.0, 1.0))",
"Output for N=1 Arguments: None\nOutput for N=2 Arguments: None\nOutput for N=3 Arguments: 1591.5494309189535\nOutput for N=4 Arguments: None\nOutput for N=5 Arguments: None\nOutput for N=6 Arguments: 3234.721973158391\nOutput for N=7 Arguments: None\n"
]
],
[
[
"Now, let's actually calculate the photon loss for a representative CPW resonator with realistic values of input parameters. Here we'll assume a qubit frequency of 5 GHz, capacitive coupling of 30fF and a CPW resonant frequency of 4 GHz. The calculated value of kappa is the range of 0-1 MHz, as expected. ",
"_____no_output_____"
]
],
[
[
"# SANITY CHECK #2\n# Let's check that the magnitude of the output is what we would expect for 3 arguments:\n# Input #1: omega = 5GHz = 5E9 Hertz \n# Input #2: C_in = 30fF = 30E-15 Farads \n# Input #3: omega_n = 4GHz = 4.5E9 Hertz \nprint(\"Calculated kappa (in Hz):\", kappa_in(5.0E9, 30.0E-15, 4.5E9), \"Hz\")\nprint(\"Calculated kappa (in MHz):\", kappa_in(5.0E9, 30.0E-15, 4.5E9)/1.0E6, \"MHz\")",
"Calculated kappa (in Hz): 161144.37988054403 Hz\nCalculated kappa (in MHz): 0.16114437988054403 MHz\n"
]
]
] | [
"markdown",
"code",
"markdown",
"code",
"markdown",
"code",
"markdown",
"code"
] | [
[
"markdown",
"markdown"
],
[
"code"
],
[
"markdown"
],
[
"code"
],
[
"markdown"
],
[
"code"
],
[
"markdown"
],
[
"code"
]
] |
d08fac0307d5b74a66c1400359cf777b6bf32398 | 2,814 | ipynb | Jupyter Notebook | sklearn/sklearn learning/demonstration/auto_examples_jupyter/linear_model/plot_sgd_comparison.ipynb | wangyendt/deeplearning_models | 47883b6c65b8d05a0d1c5737f1552df6476ded34 | [
"MIT"
] | 1 | 2020-06-04T11:10:27.000Z | 2020-06-04T11:10:27.000Z | sklearn/sklearn learning/demonstration/auto_examples_jupyter/linear_model/plot_sgd_comparison.ipynb | wangyendt/deeplearning_models | 47883b6c65b8d05a0d1c5737f1552df6476ded34 | [
"MIT"
] | null | null | null | sklearn/sklearn learning/demonstration/auto_examples_jupyter/linear_model/plot_sgd_comparison.ipynb | wangyendt/deeplearning_models | 47883b6c65b8d05a0d1c5737f1552df6476ded34 | [
"MIT"
] | null | null | null | 52.111111 | 1,702 | 0.56823 | [
[
[
"%matplotlib inline",
"_____no_output_____"
]
],
[
[
"\n# Comparing various online solvers\n\n\nAn example showing how different online solvers perform\non the hand-written digits dataset.\n",
"_____no_output_____"
]
],
[
[
"# Author: Rob Zinkov <rob at zinkov dot com>\n# License: BSD 3 clause\n\nimport numpy as np\nimport matplotlib.pyplot as plt\nfrom sklearn import datasets\n\nfrom sklearn.model_selection import train_test_split\nfrom sklearn.linear_model import SGDClassifier, Perceptron\nfrom sklearn.linear_model import PassiveAggressiveClassifier\nfrom sklearn.linear_model import LogisticRegression\n\nheldout = [0.95, 0.90, 0.75, 0.50, 0.01]\nrounds = 20\nX, y = datasets.load_digits(return_X_y=True)\n\nclassifiers = [\n (\"SGD\", SGDClassifier(max_iter=100)),\n (\"ASGD\", SGDClassifier(average=True)),\n (\"Perceptron\", Perceptron()),\n (\"Passive-Aggressive I\", PassiveAggressiveClassifier(loss='hinge',\n C=1.0, tol=1e-4)),\n (\"Passive-Aggressive II\", PassiveAggressiveClassifier(loss='squared_hinge',\n C=1.0, tol=1e-4)),\n (\"SAG\", LogisticRegression(solver='sag', tol=1e-1, C=1.e4 / X.shape[0]))\n]\n\nxx = 1. - np.array(heldout)\n\nfor name, clf in classifiers:\n print(\"training %s\" % name)\n rng = np.random.RandomState(42)\n yy = []\n for i in heldout:\n yy_ = []\n for r in range(rounds):\n X_train, X_test, y_train, y_test = \\\n train_test_split(X, y, test_size=i, random_state=rng)\n clf.fit(X_train, y_train)\n y_pred = clf.predict(X_test)\n yy_.append(1 - np.mean(y_pred == y_test))\n yy.append(np.mean(yy_))\n plt.plot(xx, yy, label=name)\n\nplt.legend(loc=\"upper right\")\nplt.xlabel(\"Proportion train\")\nplt.ylabel(\"Test Error Rate\")\nplt.show()",
"_____no_output_____"
]
]
] | [
"code",
"markdown",
"code"
] | [
[
"code"
],
[
"markdown"
],
[
"code"
]
] |
d08fb314950abd35c2ebeeb1aa2605d01548687d | 871,745 | ipynb | Jupyter Notebook | titanic/titanic_2.ipynb | utshabkg/ML_Web_Apps | 0a98d5f082a9e9bf63323772c7d781bf03f58774 | [
"MIT"
] | 1 | 2021-04-22T18:27:28.000Z | 2021-04-22T18:27:28.000Z | titanic/titanic_2.ipynb | utshabkg/ML_Web_Apps | 0a98d5f082a9e9bf63323772c7d781bf03f58774 | [
"MIT"
] | null | null | null | titanic/titanic_2.ipynb | utshabkg/ML_Web_Apps | 0a98d5f082a9e9bf63323772c7d781bf03f58774 | [
"MIT"
] | null | null | null | 293.418041 | 221,992 | 0.900651 | [
[
[
"# We tweak the style of this notebook a little bit to have centered plots.\n\nfrom IPython.core.display import HTML\nHTML(\"\"\"\n<style>\n.output_png {\n display: table-cell;\n text-align: center;\n vertical-align: middle;\n}\n</style>\n\"\"\");",
"_____no_output_____"
],
[
"%matplotlib inline\n\nimport warnings\nwarnings.filterwarnings('ignore')\nwarnings.filterwarnings('ignore', category=DeprecationWarning)\n\nimport pandas as pd\npd.options.display.max_columns = 100\n\nfrom matplotlib import pyplot as plt\nimport numpy as np\n\nimport seaborn as sns\n\nimport pylab as plot\nparams = { \n 'axes.labelsize': \"large\",\n 'xtick.labelsize': 'x-large',\n 'legend.fontsize': 20,\n 'figure.dpi': 150,\n 'figure.figsize': [25, 7]\n}\nplot.rcParams.update(params)",
"_____no_output_____"
],
[
"data = pd.read_csv('datasets/train.csv')\nprint(data.shape)\n#(891, 12)",
"(891, 12)\n"
],
[
"data.head()",
"_____no_output_____"
],
[
"data.describe()",
"_____no_output_____"
],
[
"# (891-714) = 177 values are missing in the Age column\n# fill in the null values with the median age as it's more robust to outliers\ndata['Age'] = data['Age'].fillna(data['Age'].median())",
"_____no_output_____"
],
[
"data['Died'] = 1 - data['Survived']\ndata.groupby('Sex').agg('sum')[['Survived', 'Died']].plot(kind='bar', figsize=(25, 7),\n stacked=True);",
"_____no_output_____"
],
[
"data.groupby('Sex').agg('mean')[['Survived', 'Died']].plot(kind='bar', figsize=(25, 7), \n stacked=True);",
"_____no_output_____"
],
[
"# correlate the survival with the age variable\nfig = plt.figure(figsize=(25, 7))\nsns.violinplot(x='Sex', y='Age', \n hue='Survived', data=data, \n split=True,\n palette={0: \"r\", 1: \"g\"}\n );",
"_____no_output_____"
],
[
"# fare ticket\nfigure = plt.figure(figsize=(25, 7))\nplt.hist([data[data['Survived'] == 1]['Fare'], data[data['Survived'] == 0]['Fare']], \n stacked=True, color = ['g','r'],\n bins = 50, label = ['Survived','Dead'])\nplt.xlabel('Fare')\nplt.ylabel('Number of passengers')\nplt.legend();",
"_____no_output_____"
],
[
"# age, the fare and the survival on a single chart.\nplt.figure(figsize=(25, 7))\nax = plt.subplot()\n\nax.scatter(data[data['Survived'] == 1]['Age'], data[data['Survived'] == 1]['Fare'], \n c='green', s=data[data['Survived'] == 1]['Fare'])\nax.scatter(data[data['Survived'] == 0]['Age'], data[data['Survived'] == 0]['Fare'], \n c='red', s=data[data['Survived'] == 0]['Fare']);",
"_____no_output_____"
],
[
"ax = plt.subplot()\nax.set_ylabel('Average fare')\ndata.groupby('Pclass').mean()['Fare'].plot(kind='bar', figsize=(25, 7), ax = ax);",
"_____no_output_____"
],
[
"fig = plt.figure(figsize=(25, 7))\nsns.violinplot(x='Embarked', y='Fare', hue='Survived', data=data, split=True, palette={0: \"r\", 1: \"g\"});",
"_____no_output_____"
],
[
"# Feature Engineering\n# define a print function that asserts whether a feature has been processed.\ndef status(feature):\n print('Processing', feature, ': ok')",
"_____no_output_____"
],
[
"def get_combined_data():\n # reading train data\n train = pd.read_csv('datasets/train.csv')\n \n # reading test data\n test = pd.read_csv('datasets/test.csv')\n\n # extracting and then removing the targets from the training data \n targets = train.Survived\n train.drop(['Survived'], 1, inplace=True)\n \n\n # merging train data and test data for future feature engineering\n # we'll also remove the PassengerID since this is not an informative feature\n combined = train.append(test)\n combined.reset_index(inplace=True)\n combined.drop(['index', 'PassengerId'], inplace=True, axis=1)\n \n return combined\n\ncombined = get_combined_data()",
"_____no_output_____"
],
[
"print(combined.shape)",
"(1309, 10)\n"
],
[
"titles = set()\nfor name in data['Name']:\n titles.add(name.split(',')[1].split('.')[0].strip())\n\nprint(titles)\n# set(['Sir', 'Major', 'the Countess', 'Don', 'Mlle', 'Capt', 'Dr', 'Lady', 'Rev', 'Mrs', 'Jonkheer', 'Master', 'Ms', 'Mr', 'Mme', 'Miss', 'Col'])\n\nTitle_Dictionary = {\n \"Capt\": \"Officer\",\n \"Col\": \"Officer\",\n \"Major\": \"Officer\",\n \"Jonkheer\": \"Royalty\",\n \"Don\": \"Royalty\",\n \"Sir\" : \"Royalty\",\n \"Dr\": \"Officer\",\n \"Rev\": \"Officer\",\n \"the Countess\":\"Royalty\",\n \"Mme\": \"Mrs\",\n \"Mlle\": \"Miss\",\n \"Ms\": \"Mrs\",\n \"Mr\" : \"Mr\",\n \"Mrs\" : \"Mrs\",\n \"Miss\" : \"Miss\",\n \"Master\" : \"Master\",\n \"Lady\" : \"Royalty\"\n}\n\ndef get_titles():\n # we extract the title from each name\n combined['Title'] = combined['Name'].map(lambda name:name.split(',')[1].split('.')[0].strip())\n \n # a map of more aggregated title\n # we map each title\n combined['Title'] = combined.Title.map(Title_Dictionary)\n status('Title')\n return combined",
"{'Master', 'Dr', 'Mrs', 'Mme', 'Ms', 'Major', 'Lady', 'Rev', 'Capt', 'Jonkheer', 'Miss', 'Don', 'Mlle', 'Sir', 'Col', 'Mr', 'the Countess'}\n"
],
[
"combined = get_titles()\ncombined.head()",
"Processing Title : ok\n"
],
[
"# check if the titles have been filled correctly.\n\ncombined[combined['Title'].isnull()]",
"_____no_output_____"
],
[
"# Age\n# Number of missing ages in train set\n\nprint(combined.iloc[:891].Age.isnull().sum())",
"177\n"
],
[
"# Number of missing ages in test set\n\nprint(combined.iloc[891:].Age.isnull().sum())\n# 86\n\ngrouped_train = combined.iloc[:891].groupby(['Sex','Pclass','Title'])\ngrouped_median_train = grouped_train.median()\ngrouped_median_train = grouped_median_train.reset_index()[['Sex', 'Pclass', 'Title', 'Age']]\n\ngrouped_median_train.head()",
"86\n"
],
[
"# function that fills in the missing age in combined based on these different attributes.\n\ndef fill_age(row):\n condition = (\n (grouped_median_train['Sex'] == row['Sex']) & \n (grouped_median_train['Title'] == row['Title']) & \n (grouped_median_train['Pclass'] == row['Pclass'])\n ) \n return grouped_median_train[condition]['Age'].values[0]\n\n\ndef process_age():\n global combined\n # a function that fills the missing values of the Age variable\n combined['Age'] = combined.apply(lambda row: fill_age(row) if np.isnan(row['Age']) else row['Age'], axis=1)\n status('age')\n return combined\n\ncombined = process_age()",
"Processing age : ok\n"
],
[
"# now process the names.\n\ndef process_names():\n global combined\n # we clean the Name variable\n combined.drop('Name', axis=1, inplace=True)\n \n # encoding in dummy variable\n titles_dummies = pd.get_dummies(combined['Title'], prefix='Title')\n combined = pd.concat([combined, titles_dummies], axis=1)\n \n # removing the title variable\n combined.drop('Title', axis=1, inplace=True)\n \n status('names')\n return combined",
"_____no_output_____"
],
[
"combined = process_names()\n\ncombined.head()",
"Processing names : ok\n"
],
[
"# Fare\n# fill missing fare value by the average fare computed on the train set\n\ndef process_fares():\n global combined\n # there's one missing fare value - replacing it with the mean.\n combined.Fare.fillna(combined.iloc[:891].Fare.mean(), inplace=True)\n status('fare')\n return combined",
"_____no_output_____"
],
[
"combined = process_fares()",
"Processing fare : ok\n"
],
[
"# Embarked\n# missing values of Embarked filled with the most frequent Embarked value.\ndef process_embarked():\n global combined\n # two missing embarked values - filling them with the most frequent one in the train set(S)\n combined.Embarked.fillna('S', inplace=True)\n # dummy encoding \n embarked_dummies = pd.get_dummies(combined['Embarked'], prefix='Embarked')\n combined = pd.concat([combined, embarked_dummies], axis=1)\n combined.drop('Embarked', axis=1, inplace=True)\n status('embarked')\n return combined",
"_____no_output_____"
],
[
"combined = process_embarked()\n\ncombined.head()",
"Processing embarked : ok\n"
],
[
"# Cabin\ntrain_cabin, test_cabin = set(), set()\n\nfor c in combined.iloc[:891]['Cabin']:\n try:\n train_cabin.add(c[0])\n except:\n train_cabin.add('U')\n \nfor c in combined.iloc[891:]['Cabin']:\n try:\n test_cabin.add(c[0])\n except:\n test_cabin.add('U')\n\nprint(train_cabin)\nprint(test_cabin)",
"{'E', 'C', 'G', 'A', 'F', 'B', 'D', 'T', 'U'}\n{'E', 'C', 'G', 'A', 'F', 'D', 'B', 'U'}\n"
],
[
"# replaces NaN values with U (for Unknown).\ndef process_cabin():\n global combined \n # replacing missing cabins with U (for Uknown)\n combined.Cabin.fillna('U', inplace=True)\n \n # mapping each Cabin value with the cabin letter\n combined['Cabin'] = combined['Cabin'].map(lambda c: c[0])\n \n # dummy encoding ...\n cabin_dummies = pd.get_dummies(combined['Cabin'], prefix='Cabin') \n combined = pd.concat([combined, cabin_dummies], axis=1)\n\n combined.drop('Cabin', axis=1, inplace=True)\n status('cabin')\n return combined",
"_____no_output_____"
],
[
"combined = process_cabin()\n\ncombined.head()",
"Processing cabin : ok\n"
],
[
"# Sex\ndef process_sex():\n global combined\n # mapping string values to numerical one \n combined['Sex'] = combined['Sex'].map({'male':1, 'female':0})\n status('Sex')\n return combined",
"_____no_output_____"
],
[
"combined = process_sex()",
"Processing Sex : ok\n"
],
[
"# Pclass\ndef process_pclass():\n \n global combined\n # encoding into 3 categories:\n pclass_dummies = pd.get_dummies(combined['Pclass'], prefix=\"Pclass\")\n \n # adding dummy variable\n combined = pd.concat([combined, pclass_dummies],axis=1)\n \n # removing \"Pclass\"\n combined.drop('Pclass',axis=1,inplace=True)\n \n status('Pclass')\n return combined\n\ncombined = process_pclass()",
"Processing Pclass : ok\n"
],
[
"def cleanTicket(ticket):\n ticket = ticket.replace('.', '')\n ticket = ticket.replace('/', '')\n ticket = ticket.split()\n ticket = map(lambda t : t.strip(), ticket)\n ticket = list(filter(lambda t : not t.isdigit(), ticket))\n if len(ticket) > 0:\n return ticket[0]\n else: \n return 'XXX'\n\ntickets = set()\nfor t in combined['Ticket']:\n tickets.add(cleanTicket(t))\n\nprint(len(tickets))",
"37\n"
],
[
"def process_ticket():\n \n global combined\n\n # Extracting dummy variables from tickets:\n\n combined['Ticket'] = combined['Ticket'].map(cleanTicket)\n tickets_dummies = pd.get_dummies(combined['Ticket'], prefix='Ticket')\n combined = pd.concat([combined, tickets_dummies], axis=1)\n combined.drop('Ticket', inplace=True, axis=1)\n\n status('Ticket')\n return combined\n\ncombined = process_ticket()",
"Processing Ticket : ok\n"
],
[
"# family\ndef process_family():\n \n global combined\n # introducing a new feature : the size of families (including the passenger)\n combined['FamilySize'] = combined['Parch'] + combined['SibSp'] + 1\n \n # introducing other features based on the family size\n combined['Singleton'] = combined['FamilySize'].map(lambda s: 1 if s == 1 else 0)\n combined['SmallFamily'] = combined['FamilySize'].map(lambda s: 1 if 2 <= s <= 4 else 0)\n combined['LargeFamily'] = combined['FamilySize'].map(lambda s: 1 if 5 <= s else 0)\n \n status('family')\n return combined",
"_____no_output_____"
],
[
"combined = process_family()\n\nprint(combined.shape)",
"Processing family : ok\n(1309, 67)\n"
],
[
"# We end up with a total of 67 features.\n\ncombined.head()",
"_____no_output_____"
],
[
"# Modelling start\nfrom sklearn.pipeline import make_pipeline\nfrom sklearn.ensemble import RandomForestClassifier\nfrom sklearn.ensemble.gradient_boosting import GradientBoostingClassifier\nfrom sklearn.feature_selection import SelectKBest\nfrom sklearn.model_selection import StratifiedKFold\nfrom sklearn.model_selection import GridSearchCV\nfrom sklearn.model_selection import cross_val_score\nfrom sklearn.feature_selection import SelectFromModel\nfrom sklearn.linear_model import LogisticRegression, LogisticRegressionCV",
"_____no_output_____"
],
[
"# 5-fold Cross Validation\ndef compute_score(clf, X, y, scoring='accuracy'):\n xval = cross_val_score(clf, X, y, cv = 5, scoring=scoring)\n return np.mean(xval)",
"_____no_output_____"
],
[
"# recovering train and test set\ndef recover_train_test_target():\n global combined\n \n targets = pd.read_csv('datasets/train.csv', usecols=['Survived'])['Survived'].values\n train = combined.iloc[:891]\n test = combined.iloc[891:]\n \n return train, test, targets\n\ntrain, test, targets = recover_train_test_target()",
"_____no_output_____"
],
[
"clf = RandomForestClassifier(n_estimators=50, max_features='sqrt')\nclf = clf.fit(train, targets)",
"_____no_output_____"
],
[
"features = pd.DataFrame()\nfeatures['feature'] = train.columns\nfeatures['importance'] = clf.feature_importances_\nfeatures.sort_values(by=['importance'], ascending=True, inplace=True)\nfeatures.set_index('feature', inplace=True)\n\nfeatures.plot(kind='barh', figsize=(25, 25))",
"_____no_output_____"
],
[
"model = SelectFromModel(clf, prefit=True)\ntrain_reduced = model.transform(train)\nprint(train_reduced.shape)\n# (891L, 14L)\n\ntest_reduced = model.transform(test)\nprint(test_reduced.shape)",
"(891, 13)\n(418, 13)\n"
]
],
[
[
"### Try Different base models.",
"_____no_output_____"
]
],
[
[
"logreg = LogisticRegression()\nlogreg_cv = LogisticRegressionCV()\nrf = RandomForestClassifier()\ngboost = GradientBoostingClassifier()\n\nmodels = [logreg, logreg_cv, rf, gboost]\n\nfor model in models:\n print('Cross-validation of : {0}'.format(model.__class__))\n score = compute_score(clf=model, X=train_reduced, y=targets, scoring='accuracy')\n print('CV score = {0}'.format(score))\n print('****')",
"Cross-validation of : <class 'sklearn.linear_model._logistic.LogisticRegression'>\nCV score = 0.8181721172556651\n****\nCross-validation of : <class 'sklearn.linear_model._logistic.LogisticRegressionCV'>\nCV score = 0.8204193082669009\n****\nCross-validation of : <class 'sklearn.ensemble._forest.RandomForestClassifier'>\nCV score = 0.8069738246186681\n****\nCross-validation of : <class 'sklearn.ensemble._gb.GradientBoostingClassifier'>\nCV score = 0.830525390747599\n****\n"
],
[
"# Tuning\n# turn run_gs to True if you want to run the gridsearch again.\nrun_gs = False\n\nif run_gs:\n parameter_grid = {\n 'max_depth' : [4, 6, 8],\n 'n_estimators': [50, 10],\n 'max_features': ['sqrt', 'auto', 'log2'],\n 'min_samples_split': [2, 3, 10],\n 'min_samples_leaf': [1, 3, 10],\n 'bootstrap': [True, False],\n }\n forest = RandomForestClassifier()\n cross_validation = StratifiedKFold(n_splits=5)\n\n grid_search = GridSearchCV(forest,\n scoring='accuracy',\n param_grid=parameter_grid,\n cv=cross_validation,\n verbose=1\n )\n\n grid_search.fit(train, targets)\n model = grid_search\n parameters = grid_search.best_params_\n\n print('Best score: {}'.format(grid_search.best_score_))\n print('Best parameters: {}'.format(grid_search.best_params_))\n \nelse: \n parameters = {'bootstrap': False, 'min_samples_leaf': 3, 'n_estimators': 50, \n 'min_samples_split': 10, 'max_features': 'sqrt', 'max_depth': 6}\n \n model = RandomForestClassifier(**parameters)\n model.fit(train, targets)",
"_____no_output_____"
],
[
"# output = model.predict(test).astype(int)\n# df_output = pd.DataFrame()\n# aux = pd.read_csv('datasets/test.csv')\n# df_output['PassengerId'] = aux['PassengerId']\n# df_output['Survived'] = output\n# df_output[['PassengerId','Survived']].to_csv('submission_2.csv ', index=False)",
"_____no_output_____"
]
],
[
[
"### Save and Load Model",
"_____no_output_____"
]
],
[
[
"import pickle\nimport joblib\nfile = 'titanic.pkl'\njoblib.dump(model, file)",
"_____no_output_____"
],
[
"load = joblib.load('titanic.pkl')",
"_____no_output_____"
],
[
"y_pred = load.predict(test).astype(int)\ny_pred",
"_____no_output_____"
],
[
"val = pd.DataFrame(y_pred, columns = ['Survived'])\nval = val.replace({1: 'Alive', 0: 'Died'})\nval",
"_____no_output_____"
]
]
] | [
"code",
"markdown",
"code",
"markdown",
"code"
] | [
[
"code",
"code",
"code",
"code",
"code",
"code",
"code",
"code",
"code",
"code",
"code",
"code",
"code",
"code",
"code",
"code",
"code",
"code",
"code",
"code",
"code",
"code",
"code",
"code",
"code",
"code",
"code",
"code",
"code",
"code",
"code",
"code",
"code",
"code",
"code",
"code",
"code",
"code",
"code",
"code",
"code",
"code",
"code",
"code",
"code"
],
[
"markdown"
],
[
"code",
"code",
"code"
],
[
"markdown"
],
[
"code",
"code",
"code",
"code"
]
] |
d08fb725d6ee6497a4ec12c4b9527349bfbc7664 | 62,976 | ipynb | Jupyter Notebook | notebooks/historical_planning_areas.ipynb | catalyst-cooperative/electricity-demand-mapping | a275e3a5eb4573674c6150501cfb147fcdd995e5 | [
"MIT"
] | 11 | 2020-04-08T14:30:47.000Z | 2021-06-19T00:31:51.000Z | notebooks/historical_planning_areas.ipynb | catalyst-cooperative/electricity-demand-mapping | a275e3a5eb4573674c6150501cfb147fcdd995e5 | [
"MIT"
] | 9 | 2020-04-16T21:50:31.000Z | 2021-09-03T20:26:50.000Z | notebooks/historical_planning_areas.ipynb | catalyst-cooperative/electricity-demand-mapping | a275e3a5eb4573674c6150501cfb147fcdd995e5 | [
"MIT"
] | 3 | 2020-05-01T19:40:22.000Z | 2020-07-04T22:07:58.000Z | 36.550203 | 259 | 0.565882 | [
[
[
"# Notebook Goal & Approach",
"_____no_output_____"
],
[
"## Goal\nFor each FERC 714 respondent that reports hourly demand as an electricity planning area, create a geometry representing the geographic area in which that electricity demand originated. Create a separate geometry for each year in which data is available.",
"_____no_output_____"
],
[
"## Approach\n* Use the `eia_code` found in the `respondent_id_ferc714` table to link FERC 714 respondents to their corresponding EIA utilities or balancing areas.\n* Use the `balancing_authority_eia861` and `sales_eia861` tables to figure out which respondents correspond to what utility or utilities (if a BA), and which states of operation.\n* Use the `service_territory_eia861` table to link those combinations of years, utilities, and states of operation to collections of counties.\n* Given the FIPS codes of the counties associated with each utility or balancing area in a given year, use geospatial data from the US Census to compile an annual demand area geometry.\n* Merge those geometries back in with the `respondent_id_ferc714` table, along with additional EIA balancing area and utility IDs / Codes on a per-year basis.",
"_____no_output_____"
],
[
"# Imports & Config",
"_____no_output_____"
]
],
[
[
"%load_ext autoreload\n%autoreload 2",
"_____no_output_____"
],
[
"# Standard Libraries:\nimport dateutil\nimport logging\nimport pathlib\nimport pickle\nimport re\nimport sys\nimport zipfile\n\n# 3rd Party Libraries:\nimport contextily as ctx\nimport geopandas\nimport matplotlib as mpl\nimport matplotlib.pyplot as plt\nimport numpy as np\nimport pandas as pd\nimport seaborn as sns\nimport sqlalchemy as sa\n\n# Local Packages:\nimport pudl",
"_____no_output_____"
]
],
[
[
"## Configure Output Formatting",
"_____no_output_____"
]
],
[
[
"sns.set()\n%matplotlib inline",
"_____no_output_____"
],
[
"mpl.rcParams['figure.figsize'] = (20,8)\nmpl.rcParams['figure.dpi'] = 150\npd.options.display.max_columns = 100\npd.options.display.max_rows = 100",
"_____no_output_____"
]
],
[
[
"## Logging",
"_____no_output_____"
]
],
[
[
"logger = logging.getLogger()\nlogger.setLevel(logging.INFO)\nhandler = logging.StreamHandler(stream=sys.stdout)\nlog_format = '%(asctime)s [%(levelname)8s] %(name)s:%(lineno)s %(message)s'\nformatter = logging.Formatter(log_format)\nhandler.setFormatter(formatter)\nlogger.handlers = [handler]",
"_____no_output_____"
]
],
[
[
"## PUDL Setup",
"_____no_output_____"
]
],
[
[
"pudl_settings = pudl.workspace.setup.get_defaults()\nferc1_engine = sa.create_engine(pudl_settings['ferc1_db'])\npudl_engine = sa.create_engine(pudl_settings['pudl_db'])\npudl_out = pudl.output.pudltabl.PudlTabl(pudl_engine)\npudl_settings",
"_____no_output_____"
]
],
[
[
"# Parameters",
"_____no_output_____"
]
],
[
[
"MAP_CRS = \"EPSG:3857\"\nCALC_CRS = \"ESRI:102003\"",
"_____no_output_____"
]
],
[
[
"# Function Definitions",
"_____no_output_____"
],
[
"## Dummy EIA 861 ETL",
"_____no_output_____"
]
],
[
[
"def test_etl_eia(eia_inputs, pudl_settings):\n \"\"\"\n This is a dummy function that runs the first part of the EIA ETL\n process -- everything up until the entity harvesting begins. For\n use in this notebook only.\n\n \"\"\"\n eia860_tables = eia_inputs[\"eia860_tables\"]\n eia860_years = eia_inputs[\"eia860_years\"]\n eia861_tables = eia_inputs[\"eia861_tables\"]\n eia861_years = eia_inputs[\"eia861_years\"]\n eia923_tables = eia_inputs[\"eia923_tables\"]\n eia923_years = eia_inputs[\"eia923_years\"]\n\n # generate CSVs for the static EIA tables, return the list of tables\n #static_tables = _load_static_tables_eia(datapkg_dir)\n\n # Extract EIA forms 923, 860\n eia860_raw_dfs = pudl.extract.eia860.Extractor().extract(eia860_years, testing=True)\n eia861_raw_dfs = pudl.extract.eia861.Extractor().extract(eia861_years, testing=True)\n eia923_raw_dfs = pudl.extract.eia923.Extractor().extract(eia923_years, testing=True)\n\n # Transform EIA forms 860, 861, 923\n eia860_transformed_dfs = pudl.transform.eia860.transform(eia860_raw_dfs, eia860_tables=eia860_tables)\n eia861_transformed_dfs = pudl.transform.eia861.transform(eia861_raw_dfs, eia861_tables=eia861_tables)\n eia923_transformed_dfs = pudl.transform.eia923.transform(eia923_raw_dfs, eia923_tables=eia923_tables)\n\n # create an eia transformed dfs dictionary\n eia_transformed_dfs = eia860_transformed_dfs.copy()\n eia_transformed_dfs.update(eia861_transformed_dfs.copy())\n eia_transformed_dfs.update(eia923_transformed_dfs.copy())\n\n # convert types..\n eia_transformed_dfs = pudl.helpers.convert_dfs_dict_dtypes(eia_transformed_dfs, 'eia')\n\n return eia_transformed_dfs",
"_____no_output_____"
]
],
[
[
"## Dummy EIA 861 Harvesting\n* Used to separately test the EIA entity harvesting process with EIA 861\n* Doesn't yet work b/c 861 is structured differently than 860/923.",
"_____no_output_____"
]
],
[
[
"def test_harvest_eia(eia_transformed_dfs, eia860_years, eia861_years, eia923_years):\n entities_dfs, eia_transformed_dfs = pudl.transform.eia.transform(\n eia_transformed_dfs,\n eia860_years=eia860_years,\n eia861_years=eia861_years,\n eia923_years=eia923_years,\n )\n\n # convert types..\n entities_dfs = pudl.helpers.convert_dfs_dict_dtypes(entities_dfs, 'eia')\n\n # Compile transformed dfs for loading...\n return entities_dfs, eia_transformed_dfs",
"_____no_output_____"
]
],
[
[
"## Compare Annual Demand vs. Sales",
"_____no_output_____"
]
],
[
[
"def annual_demand_vs_sales(dhpa_ferc714, sales_eia861, ba_eia861):\n \"\"\"\n Categorize EIA Codes in FERC 714 as BA or Utility IDs.\n \n Most FERC 714 respondent IDs are associated with an `eia_code` which\n refers to either a `balancing_authority_id_eia` or a `utility_id_eia`\n but no indication is given as to which type of ID each one is. This\n is further complicated by the fact that EIA uses the same numerical\n ID to refer to the same entity in most but not all cases, when that\n entity acts as both a utility and as a balancing authority.\n \n In order to identify which type of ID each `eia_code` is, this\n funciton compares the annual demand reported in association with\n each code in the FERC 714 hourly planning area time series, and in\n the EIA 861 sales table -- using the ID both as a utility and as a\n balancing authority ID. The correlation between the FERC 714 demand\n and the EIA 861 sales should be much higher for one type of ID than\n the other, indicating which type of ID is represented in the FERC\n 714 data.\n \n Args:\n dhpa_ferc714 (pandas.DataFrame): The FERC 714 hourly demand\n time series.\n sales_eia861 (pandas.DataFrame): The EIA 861 Sales table.\n ba_eia861 (pandas.DataFrame): The EIA 861 Balancing Authority\n table, which contains the mapping between EIA Balancing\n Authority Codes (3-4 letters) and EIA Balancing Authority\n IDs (integers). The codes are present in the Sales table,\n but the IDs are what the eia_code refers to.\n \n Returns:\n pandas.DataFrame: A table containing FERC 714 respondent IDs,\n EIA codes, and a column indicating whether that code was\n found to be more consistent with Balancing Authority or\n Utility electricity demand / sales.\n \n \"\"\"\n # Sum up FERC 714 demand by report_year and eia_code:\n dhpa_ferc714_by_eia_code = (\n dhpa_ferc714\n .groupby([\"eia_code\", \"report_year\"])[\"demand_mwh\"]\n .sum()\n .reset_index()\n ) \n\n # Sum up the EIA 861 sales by Utility ID:\n sales_eia861_by_util = (\n sales_eia861.groupby([\"utility_id_eia\", \"report_date\"])[\"sales_mwh\"]\n .sum()\n .reset_index()\n .assign(report_year=lambda x: x.report_date.dt.year)\n .drop(\"report_date\", axis=\"columns\")\n .rename(columns={\"sales_mwh\": \"sales_utility_mwh\"})\n )\n\n # Need to translate the BA Code to BA ID for comparison w/ eia_code\n ba_codes_and_ids = (\n ba_eia861[[\"balancing_authority_code_eia\", \"balancing_authority_id_eia\", \"report_date\"]]\n .drop_duplicates()\n .assign(report_year=lambda x: x.report_date.dt.year)\n .drop(\"report_date\", axis=\"columns\")\n .dropna()\n )\n\n # Sum up the EIA 861 sales by Balancing Authority Code:\n sales_eia861_by_ba = (\n sales_eia861\n .groupby([\"balancing_authority_code_eia\", \"report_date\"], observed=True)[\"sales_mwh\"]\n .sum()\n .reset_index()\n .assign(report_year=lambda x: x.report_date.dt.year)\n .drop(\"report_date\", axis=\"columns\")\n .rename(columns={\"sales_mwh\": \"sales_ba_mwh\"})\n .query(\"balancing_authority_code_eia!='UNK'\")\n .merge(ba_codes_and_ids)\n )\n # Combine the demand and sales data with all the IDs\n demand_and_sales = (\n dhpa_ferc714_by_eia_code\n .merge(\n sales_eia861_by_util,\n left_on=[\"eia_code\", \"report_year\"],\n right_on=[\"utility_id_eia\", \"report_year\"],\n how=\"left\"\n )\n .merge(\n sales_eia861_by_ba,\n left_on=[\"eia_code\", \"report_year\"],\n right_on=[\"balancing_authority_id_eia\", \"report_year\"],\n how=\"left\"\n )\n .astype({\n \"eia_code\": pd.Int64Dtype(),\n \"utility_id_eia\": pd.Int64Dtype(),\n \"balancing_authority_id_eia\": pd.Int64Dtype(),\n })\n .assign(\n ba_ratio=lambda x: x.sales_ba_mwh / x.demand_mwh,\n utility_ratio=lambda x: x.sales_utility_mwh / x.demand_mwh,\n )\n )\n return demand_and_sales",
"_____no_output_____"
]
],
[
[
"## EIA Code Categorization",
"_____no_output_____"
]
],
[
[
"def categorize_eia_code(rids_ferc714, utils_eia860, ba_eia861):\n \"\"\"\n Categorize EIA Codes in FERC 714 as BA or Utility IDs.\n \n Most FERC 714 respondent IDs are associated with an `eia_code` which\n refers to either a `balancing_authority_id_eia` or a `utility_id_eia`\n but no indication is given as to which type of ID each one is. This\n is further complicated by the fact that EIA uses the same numerical\n ID to refer to the same entity in most but not all cases, when that\n entity acts as both a utility and as a balancing authority.\n \n Given the nature of the FERC 714 hourly demand dataset, this function\n assumes that if the `eia_code` appears in the EIA 861 Balancing\n Authority table, that it should be labeled `balancing_authority`.\n If the `eia_code` appears only in the EIA 860 Utility table, then\n it is labeled `utility`. These labels are put in a new column named\n `respondent_type`. If the planning area's `eia_code` does not appear in\n either of those tables, then `respondent_type is set to NA.\n\n Args:\n rids_ferc714 (pandas.DataFrame): The FERC 714 `respondent_id` table.\n utils_eia860 (pandas.DataFrame): The EIA 860 Utilities output table.\n ba_eia861 (pandas.DataFrame): The EIA 861 Balancing Authority table.\n \n Returns:\n pandas.DataFrame: A table containing all of the columns present in\n the FERC 714 `respondent_id` table, plus a new one named\n `respondent_type` which can take on the values `balancing_authority`,\n `utility`, or the special value pandas.NA.\n\n \"\"\"\n ba_ids = set(ba_eia861.balancing_authority_id_eia.dropna())\n util_not_ba_ids = set(utils_eia860.utility_id_eia.dropna()).difference(ba_ids)\n new_rids = rids_ferc714.copy()\n new_rids[\"respondent_type\"] = pd.NA\n new_rids.loc[new_rids.eia_code.isin(ba_ids), \"respondent_type\"] = \"balancing_authority\"\n new_rids.loc[new_rids.eia_code.isin(util_not_ba_ids), \"respondent_type\"] = \"utility\"\n ba_rids = new_rids[new_rids.respondent_type==\"balancing_authority\"]\n util_rids = new_rids[new_rids.respondent_type==\"utility\"]\n na_rids = new_rids[new_rids.respondent_type.isnull()]\n\n ba_rids = (\n ba_rids.merge(\n ba_eia861\n .filter(like=\"balancing_\")\n .drop_duplicates(subset=[\"balancing_authority_id_eia\", \"balancing_authority_code_eia\"]),\n how=\"left\", left_on=\"eia_code\", right_on=\"balancing_authority_id_eia\"\n )\n )\n util_rids = (\n util_rids.merge(\n utils_eia860[[\"utility_id_eia\", \"utility_name_eia\"]]\n .drop_duplicates(\"utility_id_eia\"),\n how=\"left\", left_on=\"eia_code\", right_on=\"utility_id_eia\"\n )\n )\n new_rids = (\n pd.concat([ba_rids, util_rids, na_rids])\n .astype({\n \"respondent_type\": pd.StringDtype(),\n \"balancing_authority_code_eia\": pd.StringDtype(),\n \"balancing_authority_id_eia\": pd.Int64Dtype(),\n \"balancing_authority_name_eia\": pd.StringDtype(),\n \"utility_id_eia\": pd.Int64Dtype(),\n \"utility_name_eia\": pd.StringDtype(),\n })\n )\n \n return new_rids",
"_____no_output_____"
]
],
[
[
"## Georeference Balancing Authorities",
"_____no_output_____"
]
],
[
[
"def georef_bas(ba_eia861, st_eia861, sales_eia861, census_gdf):\n \"\"\"\n Create a GeoDataFrame mapping BAs to Utils to county geometries by year.\n \n This GDF includes the following columns:\n \n balancing_authority_id_eia (ba_eia861)\n balancing_authority_name_eia (ba_eia861)\n balancing_authority_code_eia (ba_eia861)\n utility_id_eia (sales_eia861)\n utility_name_eia (sales_eia861)\n county_id_fips (st_eia861)\n county (st_eia861)\n state_id_fips (st_eia861)\n state (st_eia861)\n geometry (census_gdf)\n county_name_census (census_gdf)\n \n It includes information both about which counties are associated with\n utilities that are part of balancing authorities, and utilities that\n are not part part of balancing authorities, so should be possible to\n use it to generate geometries for all of the respondents in FERC 714,\n both BAs and Utils.\n\n \"\"\"\n # Make sure that there aren't any more BA IDs we can recover from later years:\n ba_ids_missing_codes = (\n ba_eia861.loc[ba_eia861.balancing_authority_code_eia.isnull(), \"balancing_authority_id_eia\"]\n .drop_duplicates()\n .dropna()\n )\n assert len(ba_eia861[\n (ba_eia861.balancing_authority_id_eia.isin(ba_ids_missing_codes)) &\n (ba_eia861.balancing_authority_code_eia.notnull())\n ]) == 0\n \n # Which utilities were part of what balancing areas in 2010-2012?\n early_ba_by_util = (\n ba_eia861\n .query(\"report_date <= '2012-12-31'\")\n .loc[:, [\n \"report_date\",\n \"balancing_authority_id_eia\",\n \"balancing_authority_code_eia\",\n \"utility_id_eia\",\n \"balancing_authority_name_eia\",\n ]]\n .drop_duplicates(subset=[\"report_date\", \"balancing_authority_id_eia\", \"utility_id_eia\"])\n )\n\n # Create a dataframe that associates utilities and balancing authorities.\n # This information is directly avaialble in the early_ba_by_util dataframe\n # but has to be compiled for 2013 and later years based on the utility\n # BA associations that show up in the Sales table\n # Create an annual, normalized version of the BA table:\n ba_normed = (\n ba_eia861\n .loc[:, [\n \"report_date\",\n \"state\",\n \"balancing_authority_code_eia\",\n \"balancing_authority_id_eia\",\n \"balancing_authority_name_eia\",\n ]]\n .drop_duplicates(subset=[\n \"report_date\",\n \"state\",\n \"balancing_authority_code_eia\",\n \"balancing_authority_id_eia\",\n ])\n )\n ba_by_util = (\n pd.merge(\n ba_normed,\n sales_eia861\n .loc[:, [\n \"report_date\",\n \"state\",\n \"utility_id_eia\",\n \"balancing_authority_code_eia\"\n ]].drop_duplicates()\n )\n .loc[:, [\n \"report_date\",\n \"state\",\n \"utility_id_eia\",\n \"balancing_authority_id_eia\"\n ]]\n .append(early_ba_by_util[[\"report_date\", \"utility_id_eia\", \"balancing_authority_id_eia\"]])\n .drop_duplicates()\n .merge(ba_normed)\n .dropna(subset=[\"report_date\", \"utility_id_eia\", \"balancing_authority_id_eia\"])\n .sort_values([\"report_date\", \"balancing_authority_id_eia\", \"utility_id_eia\", \"state\"])\n )\n # Merge in county FIPS IDs for each county served by the utility from\n # the service territory dataframe. We do an outer merge here so that we\n # retain any utilities that are not part of a balancing authority. This\n # lets us generate both BA and Util maps from the same GeoDataFrame\n # We have to do this separately for the data up to 2012 (which doesn't\n # include state) and the 2013 and onward data (which we need to have\n # state for)\n early_ba_util_county = (\n ba_by_util.drop(\"state\", axis=\"columns\")\n .merge(st_eia861, on=[\"report_date\", \"utility_id_eia\"], how=\"outer\")\n .query(\"report_date <= '2012-12-31'\")\n )\n late_ba_util_county = (\n ba_by_util\n .merge(st_eia861, on=[\"report_date\", \"utility_id_eia\", \"state\"], how=\"outer\")\n .query(\"report_date >= '2013-01-01'\")\n )\n ba_util_county = pd.concat([early_ba_util_county, late_ba_util_county])\n # Bring in county geometry information based on FIPS ID from Census\n ba_util_county_gdf = (\n census_gdf[[\"GEOID10\", \"NAMELSAD10\", \"geometry\"]]\n .to_crs(MAP_CRS)\n .rename(\n columns={\n \"GEOID10\": \"county_id_fips\",\n \"NAMELSAD10\": \"county_name_census\",\n }\n )\n .merge(ba_util_county)\n )\n \n return ba_util_county_gdf",
"_____no_output_____"
]
],
[
[
"## Map Balancing Authorities",
"_____no_output_____"
]
],
[
[
"def map_ba(ba_ids, year, ba_util_county_gdf, save=False):\n \"\"\"\n Create a map of a balancing authority for a historical year.\n \n Args:\n ba_ids (iterable): A collection of Balancing Authority IDs.\n year (int): The year for which to create a map.\n ba_util_county_gdf (geopandas.GeoDataFrame): A dataframe\n associating report_date, balancing_authority_id_eia, and\n county_id_fips.\n save (bool): If True, save the figure to disk.\n \n Returns:\n None\n \n \"\"\"\n map_gdf = (\n ba_util_county_gdf[\n (ba_util_county_gdf.report_date.dt.year == year) &\n (ba_util_county_gdf.balancing_authority_id_eia.isin(ba_ids)) &\n (~ba_util_county_gdf.county_id_fips.str.match(\"^02\")) & # Avoid Alaska\n (~ba_util_county_gdf.county_id_fips.str.match(\"^15\")) & # Avoid Hawaii\n (~ba_util_county_gdf.county_id_fips.str.match(\"^72\")) # Avoid Puerto Rico\n ]\n .drop_duplicates(subset=[\"balancing_authority_id_eia\", \"county_id_fips\"])\n )\n ax = map_gdf.plot(figsize=(20, 20), color=\"black\", alpha=0.25, linewidth=0.25)\n plt.title(f\"Balancing Areas ({year=})\")\n ctx.add_basemap(ax)\n if save is True:\n plt.savefig(f\"BA_Overlap_{year}.jpg\")",
"_____no_output_____"
],
[
"def compare_hifld_eia_ba(ba_code, hifld_gdf, eia_gdf):\n \"\"\"\n Compare historical EIA BAs vs. HIFLD geometries.\n \"\"\"\n fig, (hifld_ax, eia_ax) = plt.subplots(nrows=1, ncols=2, sharex=True, sharey=True)\n hifld_ax.set_title(f\"{ba_code} (HIFLD)\")\n hifld_gdf[hifld_gdf.ABBRV==ba_code].to_crs(MAP_CRS).plot(ax=hifld_ax, linewidth=0)\n\n eia_ax.set_title(f\"{ba_code} (EIA)\")\n eia_gdf[\n (eia_gdf.balancing_authority_code_eia==ba_code) &\n (eia_gdf.report_date.dt.year == 2017)\n ].plot(ax=eia_ax, linewidth=0.1)\n plt.show()",
"_____no_output_____"
]
],
[
[
"# Read Data",
"_____no_output_____"
],
[
"## EIA 860 via PUDL Outputs",
"_____no_output_____"
]
],
[
[
"plants_eia860 = pudl_out.plants_eia860()\nutils_eia860 = pudl_out.utils_eia860()",
"_____no_output_____"
]
],
[
[
"## EIA 861 (2010-2018)\n* Not yet fully integrated into PUDL\n* Post-transform harvesting process isn't compatible w/ EIA 861 structure\n* Only getting the `sales_eia861`, `balancing_authority_eia861`, and `service_territory_eia861` tables",
"_____no_output_____"
]
],
[
[
"%%time\nlogger.setLevel(\"WARN\")\neia_years = list(range(2010, 2019))\neia_inputs = {\n \"eia860_years\": [],\n \"eia860_tables\": pudl.constants.pudl_tables[\"eia860\"],\n \"eia861_years\": eia_years,\n \"eia861_tables\": pudl.constants.pudl_tables[\"eia861\"],\n \"eia923_years\": [],\n \"eia923_tables\": pudl.constants.pudl_tables[\"eia923\"],\n}\neia_transformed_dfs = test_etl_eia(eia_inputs=eia_inputs, pudl_settings=pudl_settings)\nlogger.setLevel(\"INFO\")",
"_____no_output_____"
],
[
"ba_eia861 = eia_transformed_dfs[\"balancing_authority_eia861\"].copy()\nst_eia861 = eia_transformed_dfs[\"service_territory_eia861\"].copy()\nsales_eia861 = eia_transformed_dfs[\"sales_eia861\"].copy()",
"_____no_output_____"
],
[
"raw_eia861_dfs = pudl.extract.eia861.Extractor().extract(years=range(2010,2019), testing=True)",
"_____no_output_____"
]
],
[
[
"## FERC 714 (2006-2018)",
"_____no_output_____"
]
],
[
[
"%%time\nlogger.setLevel(\"WARN\")\nraw_ferc714 = pudl.extract.ferc714.extract(pudl_settings=pudl_settings)\ntfr_ferc714 = pudl.transform.ferc714.transform(raw_ferc714)\nlogger.setLevel(\"INFO\")",
"_____no_output_____"
]
],
[
[
"## HIFLD Electricity Planning Areas (2018)\n* Electricty Planning Area geometries from HIFLD.\n* Indexed by `ID` which corresponds to EIA utility or balancing area IDs.\n* Only valid for 2017-2018.",
"_____no_output_____"
]
],
[
[
"hifld_pa_gdf = (\n pudl.analysis.demand_mapping.get_hifld_planning_areas_gdf(pudl_settings)\n .to_crs(MAP_CRS)\n)",
"_____no_output_____"
]
],
[
[
"## US Census DP1 (2010)\n* This GeoDataFrame contains county-level geometries and demographic data.",
"_____no_output_____"
]
],
[
[
"%%time\ncensus_gdf = (\n pudl.analysis.demand_mapping.get_census2010_gdf(pudl_settings, layer=\"county\")\n .to_crs(MAP_CRS)\n)",
"_____no_output_____"
]
],
[
[
"# Combine Data",
"_____no_output_____"
],
[
"## Categorize FERC 714 Respondent IDs",
"_____no_output_____"
]
],
[
[
"rids_ferc714 = (\n tfr_ferc714[\"respondent_id_ferc714\"]\n .pipe(categorize_eia_code, utils_eia860, ba_eia861)\n)",
"_____no_output_____"
]
],
[
[
"## Add FERC 714 IDs to HIFLD",
"_____no_output_____"
]
],
[
[
"hifld_pa_gdf = (\n hifld_pa_gdf\n .merge(rids_ferc714, left_on=\"ID\", right_on=\"eia_code\", how=\"left\")\n)",
"_____no_output_____"
]
],
[
[
"## Add Respondent info to FERC 714 Demand",
"_____no_output_____"
]
],
[
[
"dhpa_ferc714 = pd.merge(\n tfr_ferc714[\"demand_hourly_pa_ferc714\"],\n tfr_ferc714[\"respondent_id_ferc714\"],\n on=\"respondent_id_ferc714\",\n how=\"left\", # There are respondents with no demand\n)",
"_____no_output_____"
]
],
[
[
"# Utilities vs. Balancing Authorities\nExploration of the Balancing Authority EIA 861 table for cleanup\n\n### Which columns are available in which years?\n\n| Year | BA ID | BA Name | BA Code | Util ID | Util Name | State | N |\n|------|-------|---------|---------|---------|-----------|-------|----|\n| 2010 | XXXXX | XXXXXXX | | XXXXXXX | | |3193|\n| 2011 | XXXXX | XXXXXXX | | XXXXXXX | | |3126|\n| 2012 | XXXXX | XXXXXXX | | XXXXXXX | XXXXXXXXX | |3146|\n| 2013 | XXXXX | XXXXXXX | XXXXXXX | | | XXXXX | 239|\n| 2014 | XXXXX | XXXXXXX | XXXXXXX | | | XXXXX | 208|\n| 2015 | XXXXX | XXXXXXX | XXXXXXX | | | XXXXX | 203|\n| 2016 | XXXXX | XXXXXXX | XXXXXXX | | | XXXXX | 203|\n| 2017 | XXXXX | XXXXXXX | XXXXXXX | | | XXXXX | 203|\n| 2018 | XXXXX | XXXXXXX | XXXXXXX | | | XXXXX | 204|\n\n### What does this table mean?\n* In 2010-2012, the table says which utilities (by ID) are included in which balancing authorities.\n* In 2013-2018, the table indicates which *states* a BA is operating in, and also provides a BA Code\n\n### Questions:\n* Where does the `balancing_authority_code` show up elsewhere in the EIA 860/861 data?\n * `plants_eia860` (nowhere else that I know of)\n* Are the BA to Utility mappings likely to remain valid throughout the entire time period? Can we propagate them forward?\n * No, there's some variation year to year in which utilities are associated with which BAs\n* Are the BA Code/Name to BA ID mappings permanent?\n * No they aren't -- when a BA changes owners and names, the code changes, but ID stays the same.",
"_____no_output_____"
],
[
"## Untangling HIFLD, FERC 714, & EIA IDs\n* There are unspecified \"EIA codes\" associated with FERC 714 respondents.\n* These IDs correspond to a mix of `utility_id_eia` and `balancing_authority_id_eia` values.\n* Similarly, the ID field of the HIFLD geometries are a mix of BA and Utility IDs from EIA.\n* This is extra confusing, because EIA *usually* uses the *same* ID for BAs and Utils.\n* However, the EIA BA and Util IDs appear to be distinct namespaces\n * Not all IDs which appear in both tables identify the same entity in both tables.\n * In a few cases different IDs are used to identify the same entity when it shows up in both tables.\n* It could be that whoever entered the IDs in the FERC 714 / HIFLD datasets didn't realize these were different sets of IDs.",
"_____no_output_____"
],
[
"### BA / Utility ID Overlap\n* Example of an ID that shows up in both, but refers to different entities, see `59504`\n * `balancing_area_id_eia == 59504` is the Southwest Power Pool (SWPP).\n * `utility_id_eia == 59504` is Kirkwood Community College, in MO.\n* Example of an entity that exists in both datsets, but shows up with different IDs, see PacifiCorp.\n * Has two BA IDs (East and West): `[14379, 14378]`\n * Has one Utility ID: `14354`\n* Example of an entity that shows up with the same ID in both tables:\n * ID `15466` is Public Service Co of Colorado -- both a BA (PSCO) and a Utility.",
"_____no_output_____"
]
],
[
[
"# BA ID comes from EIA 861 BA Table\nba_ids = set(ba_eia861.balancing_authority_id_eia)\nprint(f\"Total # of BA IDs: {len(ba_ids)}\")\n\n# Util ID comes from EIA 860 Utilities Entity table.\nutil_ids = set(pudl_out.utils_eia860().utility_id_eia)\nprint(f\"Total # of Util IDs: {len(util_ids)}\")\n\nba_not_util_ids = ba_ids.difference(util_ids)\nprint(f\"BA IDs that are not Util IDs: {len(ba_not_util_ids)}\")\n\nutil_not_ba_ids = util_ids.difference(ba_ids)\nprint(f\"Util IDs that are not BA IDs: {len(util_not_ba_ids)}\")\n\nba_and_util_ids = ba_ids.intersection(util_ids)\nprint(f\"BA IDs that are also Util IDs: {len(ba_and_util_ids)}\")",
"_____no_output_____"
],
[
"ba_and_util = (\n ba_eia861\n .loc[:, [\"balancing_authority_id_eia\", \"balancing_authority_name_eia\"]]\n .dropna(subset=[\"balancing_authority_id_eia\"])\n .merge(\n pudl_out.utils_eia860(),\n left_on=\"balancing_authority_id_eia\",\n right_on=\"utility_id_eia\",\n how=\"inner\"\n )\n .loc[:, [\n \"utility_id_eia\",\n \"balancing_authority_name_eia\",\n \"utility_name_eia\",\n ]]\n .rename(columns={\"utility_id_eia\": \"util_ba_id\"})\n .drop_duplicates()\n .reset_index(drop=True)\n)\n\nba_not_util = (\n ba_eia861.loc[ba_eia861.balancing_authority_id_eia.isin(ba_not_util_ids)]\n .loc[:,[\"balancing_authority_id_eia\", \"balancing_authority_code_eia\", \"balancing_authority_name_eia\"]]\n .drop_duplicates(subset=[\"balancing_authority_id_eia\", \"balancing_authority_code_eia\"])\n .sort_values(\"balancing_authority_id_eia\")\n)",
"_____no_output_____"
]
],
[
[
"### Missing IDs\n* There are `eia_code` values that don't show up in the list of balancing authority IDs (2010-2018).\n* There are also `eia_code` values that don't show up in the list of utility IDs (2009-2018).\n* There are a few `eia_code` values that don't show up in either!\n* Mostly this is an artifact of the different time covered by FERC 714 (2006-2018).\n* If we look only at the respondents that reported non-zero demand for 2010-2018, we find that all of the `eia_code` values *do* appear in either the `blancing_authority_eia861` or `utilities_eia860` tables.",
"_____no_output_____"
]
],
[
[
"rids_ferc714[\n (~rids_ferc714.eia_code.isin(ba_eia861.balancing_authority_id_eia.unique())) &\n (~rids_ferc714.eia_code.isin(utils_eia860.utility_id_eia.unique()))\n]",
"_____no_output_____"
],
[
"rids_recent = (\n dhpa_ferc714\n .groupby([\"respondent_id_ferc714\", \"report_year\"])\n .agg({\"demand_mwh\": sum})\n .reset_index()\n .query(\"report_year >= 2010\")\n .query(\"demand_mwh >= 0.0\")\n .merge(rids_ferc714[[\"eia_code\", \"respondent_id_ferc714\", \"respondent_name_ferc714\"]], how=\"left\")\n .drop([\"report_year\", \"demand_mwh\"], axis=\"columns\")\n .drop_duplicates()\n)\nassert len(rids_recent[\n (~rids_recent.eia_code.isin(ba_eia861.balancing_authority_id_eia.unique())) &\n (~rids_recent.eia_code.isin(utils_eia860.utility_id_eia.unique()))\n]) == 0",
"_____no_output_____"
]
],
[
[
"### BA to Utility Mappings are Many to Many\n* Unsurprisingly, BAs often contain many utilities.\n* However, it's also common for utilities to participate in more than one BA.\n* About 1/3 of all utilities show up in association with more than one BA",
"_____no_output_____"
]
],
[
[
"ba_to_util_mapping = (\n ba_eia861[[\"balancing_authority_id_eia\", \"utility_id_eia\"]]\n .dropna(subset=[\"balancing_authority_id_eia\", \"utility_id_eia\"])\n .drop_duplicates(subset=[\"balancing_authority_id_eia\", \"utility_id_eia\"])\n .groupby([\"balancing_authority_id_eia\"])\n .agg({\n \"utility_id_eia\": \"count\"\n })\n)\nplt.hist(ba_to_util_mapping.utility_id_eia, bins=99, range=(1,100))\nplt.xlabel(\"# of Utils / BA\")\nplt.ylabel(\"# of BAs\")\nplt.title(\"Number of Utilities per Balancing Area\");",
"_____no_output_____"
],
[
"util_to_ba_mapping = (\n ba_eia861[[\"balancing_authority_id_eia\", \"utility_id_eia\"]]\n .dropna(subset=[\"balancing_authority_id_eia\", \"utility_id_eia\"])\n .drop_duplicates(subset=[\"balancing_authority_id_eia\", \"utility_id_eia\"])\n .groupby([\"utility_id_eia\"])\n .agg({\n \"balancing_authority_id_eia\": \"count\"\n })\n)\nplt.hist(util_to_ba_mapping.balancing_authority_id_eia, bins=4, range=(1,5))\nplt.title(\"Number of Balancing Authorities per Utility\");",
"_____no_output_____"
]
],
[
[
"## Georeferenced Demand Fraction\n* With their original EIA codes the HIFLD Electricity Planning Areas only georeference some of the FERC 714 demand.\n* It's about 86% in 2018. In 2013 and earlier years, the fraction starts to drop off more quickly, to 76% in 2010, and 58% in 2006.\n* After manually identifying and fixing some bad and missing EIA codes in the FERC 714, the mapped fraction is much higher.\n* 98% or more in 2014-2018, dropping to 87% in 2010, and 68% in 2006\n* **However** because the geometries have also evolved over time, just the fact that the demand time series is linked to **some** HIFLD geometry, doesn't mean that it's the **right** geometry.",
"_____no_output_____"
]
],
[
[
"annual_demand_ferc714 = (\n dhpa_ferc714\n .groupby([\"report_year\"]).demand_mwh.sum()\n .reset_index()\n)\nannual_demand_mapped = (\n dhpa_ferc714[dhpa_ferc714.eia_code.isin(hifld_pa_gdf.eia_code)]\n .groupby([\"report_year\"]).demand_mwh.sum()\n .reset_index()\n .merge(annual_demand_ferc714, on=\"report_year\", suffixes=(\"_map\", \"_tot\"))\n .assign(\n fraction_mapped=lambda x: x.demand_mwh_map / x.demand_mwh_tot\n )\n)",
"_____no_output_____"
],
[
"plt.plot(\"report_year\", \"fraction_mapped\", data=annual_demand_mapped, lw=5)\nplt.ylabel(\"Fraction of demand which is mapped\")\nplt.title(\"Completeness of HIFLD demand mapping by year\")\nplt.ylim(0.6, 1.05);",
"_____no_output_____"
]
],
[
[
"# Historical Planning Area Geometries\nCompile a GeoDataFrame that relates balancing authorities, their constituent utilities, and the collections of counties which are served by those utilities, across all the years for which we have EIA 861 data (2010-2018)",
"_____no_output_____"
]
],
[
[
"ba_util_county_gdf = georef_bas(ba_eia861, st_eia861, sales_eia861, census_gdf)",
"_____no_output_____"
],
[
"ba_util_county_gdf.info()",
"_____no_output_____"
],
[
"for year in (2010, 2014, 2018):\n map_ba(ba_util_county_gdf.balancing_authority_id_eia.unique(), year, ba_util_county_gdf, save=True)",
"_____no_output_____"
]
],
[
[
"## Output Simplified Annual BA Geometries\n* This takes half an hour so it's commented out.\n* Resulting shapefile is ~250MB compressed. Seems too big.\n* Need to figure out how to add explicity projection.\n* Need to figure out how to make each year's BA geometries its own layer.",
"_____no_output_____"
]
],
[
[
"#%%time\n#ba_fips_simplified = (\n# ba_util_county_gdf\n# .assign(report_year=lambda x: x.report_date.dt.year)\n# .drop([\n# \"report_date\",\n# \"state\",\n# \"state_id_fips\",\n# \"county\",\n# \"county_name_census\",\n# \"utility_id_eia\",\n# \"utility_name_eia\"\n# ], axis=\"columns\")\n# .drop_duplicates(subset=[\"report_year\", \"balancing_authority_id_eia\", \"county_id_fips\"])\n# .dropna(subset=[\"report_year\", \"balancing_authority_id_eia\", \"county_id_fips\"])\n# .loc[:,[\"report_year\", \"balancing_authority_id_eia\", \"balancing_authority_code_eia\", \"balancing_authority_name_eia\", \"county_id_fips\", \"geometry\"]]\n#)\n#ba_annual_gdf = (\n# ba_fips_simplified\n# .dissolve(by=[\"report_year\", \"balancing_authority_id_eia\"])\n# .reset_index()\n# .drop(\"county_id_fips\", axis=\"columns\")\n#)\n#ba_output_gdf = (\n# ba_annual_gdf\n# .astype({\n# \"report_year\": int,\n# \"balancing_authority_id_eia\": float,\n# \"balancing_authority_code_eia\": str,\n# \"balancing_authority_name_eia\": str,\n# })\n# .rename(columns={\n# \"report_year\": \"year\",\n# \"balancing_authority_id_eia\": \"ba_id\",\n# \"balancing_authority_code_eia\": \"ba_code\",\n# \"balancing_authority_name_eia\": \"ba_name\",\n# })\n#)\n#ba_output_gdf.to_file(\"ba_annual.shp\")",
"_____no_output_____"
]
],
[
[
"## Compare HIFLD and EIA BA maps for 2018",
"_____no_output_____"
]
],
[
[
"for ba_code in hifld_pa_gdf.ABBRV.unique():\n if ba_code in ba_util_county_gdf.balancing_authority_code_eia.unique():\n compare_hifld_eia_ba(ba_code, hifld_pa_gdf, ba_util_county_gdf)",
"_____no_output_____"
]
],
[
[
"## Time Evolution of BA Geometries\nFor each BA we now have a collection of annual geometries. How have they changed over time?",
"_____no_output_____"
]
],
[
[
"for ba_code in ba_util_county_gdf.balancing_authority_code_eia.unique():\n fig, axes = plt.subplots(nrows=3, ncols=3, figsize=(20,20), sharex=True, sharey=True, facecolor=\"white\")\n for year, ax in zip(range(2010, 2019), axes.flat):\n ax.set_title(f\"{ba_code} ({year})\")\n ax.set_xticks([])\n ax.set_yticks([])\n plot_gdf = (\n ba_util_county_gdf\n .assign(report_year=lambda x: x.report_date.dt.year)\n .query(f\"balancing_authority_code_eia=='{ba_code}'\")\n .query(f\"report_year=='{year}'\")\n .drop_duplicates(subset=\"county_id_fips\")\n )\n plot_gdf.plot(ax=ax, linewidth=0.1)\n plt.show()",
"_____no_output_____"
]
],
[
[
"## Merge Geometries with FERC 714\nNow that we have a draft of wht the BA and Utility level territories look like, we can merge those with the FERC 714 Respondent ID table, and see how many leftovers there are, and whether the BA and Utility geometires play well together.\n\nBefore dissolving the boundaries between counties the output dataframe needs to have:\n* `report_date`\n* `respondent_id_ferc714`\n* `eia_code`\n* `respondent_type`\n* `balancing_authority_id_eia`\n* `utility_id_eia`\n* `county_id_fips`\n* `geometry`\n\n* `balancing_authority_code_eia`\n* `balancing_authority_name_eia`\n* `respondent_name_ferc714`\n* `utility_name_eia`\n* `county_name_census`\n* `state`\n* `state_id_fips`",
"_____no_output_____"
]
],
[
[
"utils_ferc714 = (\n rids_ferc714.loc[\n rids_ferc714.respondent_type == \"utility\",\n [\"respondent_id_ferc714\", \"respondent_name_ferc714\", \"utility_id_eia\", \"respondent_type\"]\n ]\n)\n\nbas_ferc714 = (\n rids_ferc714.loc[\n rids_ferc714.respondent_type == \"balancing_authority\",\n [\"respondent_id_ferc714\", \"respondent_name_ferc714\", \"balancing_authority_id_eia\", \"respondent_type\"]\n ]\n)\n\nnull_ferc714 = (\n rids_ferc714.loc[\n rids_ferc714.respondent_type.isnull(),\n [\"respondent_id_ferc714\", \"respondent_name_ferc714\", \"respondent_type\"]\n ]\n)\n\nbas_ferc714_gdf = (\n ba_util_county_gdf\n .drop([\"county\"], axis=\"columns\")\n .merge(bas_ferc714, how=\"right\")\n)\n\nutils_ferc714_gdf = (\n ba_util_county_gdf\n .drop([\"balancing_authority_id_eia\", \"balancing_authority_code_eia\", \"balancing_authority_name_eia\", \"county\"], axis=\"columns\")\n .drop_duplicates()\n .merge(utils_ferc714, how=\"right\")\n)\nrids_ferc714_gdf = (\n pd.concat([bas_ferc714_gdf, utils_ferc714_gdf, null_ferc714])\n .astype({\n \"county_id_fips\": pd.StringDtype(),\n \"county_name_census\": pd.StringDtype(),\n \"respondent_type\": pd.StringDtype(),\n \"utility_id_eia\": pd.Int64Dtype(),\n \"balancing_authority_id_eia\": pd.Int64Dtype(),\n \"balancing_authority_code_eia\": pd.StringDtype(),\n \"balancing_authority_name_eia\": pd.StringDtype(),\n \"state\": pd.StringDtype(),\n \"utility_name_eia\": pd.StringDtype(),\n })\n)",
"_____no_output_____"
],
[
"display(rids_ferc714_gdf.info())\nrids_ferc714_gdf.sample(10)",
"_____no_output_____"
]
],
[
[
"## Check Geometries for Completeness\n* How many balancing authorities do we have geometries for?\n* How many utilities do we have geometries for?\n* Do those geometries cover all of the entities that report in FERC 714?\n* Do we have a geometry for every entity in every year in which it reports demand?",
"_____no_output_____"
],
[
"### Count BA & Util Geometries",
"_____no_output_____"
]
],
[
[
"n_bas = len(rids_ferc714_gdf.balancing_authority_id_eia.unique())\nlogger.info(f\"Found territories for {n_bas} unique Balancing Areas\")\nn_utils = len(rids_ferc714_gdf.loc[\n (rids_ferc714_gdf.balancing_authority_id_eia.isnull()) &\n (~rids_ferc714_gdf.utility_id_eia.isnull())\n].utility_id_eia.unique())\nlogger.info(f\"Found territories for {n_utils} Utilities outside of the BAs\")",
"_____no_output_____"
]
],
[
[
"### Identify Missing Geometries\n* Within each year of historical data from 2010-2018, are there any entities (either BA or Utility) which **do** have hourly demand reported in the FERC 714, for whivh we do not have a historical geometry?\n* How many of them are there?\n* Why are they missing?\n* Do we have the geometires in adjacent years and can we re-use them?\n* Is it possible that the FERC 714 IDs correspond to a precursor entity, or one that was discontinued? E.g. if SWPP is missing in 2010, is that because the BA was reported in EIA as SPS in that year?\n* How important are the missing geometries? Do the associated entities have a lot of demand associated with them in FERC 714?\n* Can we use `ffill` or `backfill` on the `geometry` column in a GeoDataFrame?",
"_____no_output_____"
]
],
[
[
"problem_ids = pd.DataFrame()\nfor year in range(2010, 2019):\n this_year_gdf = (\n rids_ferc714_gdf\n .loc[(rids_ferc714_gdf.report_date.dt.year==year) & (~rids_ferc714_gdf.geometry.isnull())]\n )\n # All BA IDs which show up in FERC 714:\n ba_ids_ferc714 = (\n rids_ferc714\n .loc[rids_ferc714.respondent_type==\"balancing_authority\",\n \"balancing_authority_id_eia\"]\n .unique()\n )\n # BA IDs which have a geometry in this year\n ba_geom_ids = (\n this_year_gdf\n .balancing_authority_id_eia\n .dropna().unique()\n )\n # BA IDs which have reported demand in this year\n ba_demand_ids = (\n dhpa_ferc714\n .query(\"report_year==@year\")\n .query(\"demand_mwh>0.0\")\n .loc[dhpa_ferc714.eia_code.isin(ba_ids_ferc714)]\n .eia_code.unique()\n )\n\n # Need to make the demand IDs clearly either utility of BA IDs. Whoops!\n missing_ba_geom_ids = [x for x in ba_demand_ids if x not in ba_geom_ids]\n logger.info(f\"{len(missing_ba_geom_ids)} BA respondents w/o geometries in {year}\")\n problem_ids = problem_ids.append(\n rids_ferc714\n .loc[rids_ferc714.balancing_authority_id_eia.isin(missing_ba_geom_ids)]\n .assign(year=year)\n )\n \n # All EIA Utility IDs which show up in FERC 714:\n util_ids_ferc714 = (\n rids_ferc714\n .loc[rids_ferc714.respondent_type==\"utility\",\n \"utility_id_eia\"]\n .unique()\n )\n # EIA Utility IDs which have geometry information for this year\n util_geom_ids = (\n this_year_gdf\n .utility_id_eia\n .dropna().unique()\n )\n util_demand_ids = (\n dhpa_ferc714\n .query(\"report_year==@year\")\n .query(\"demand_mwh>0.0\")\n .loc[dhpa_ferc714.eia_code.isin(util_ids_ferc714)]\n .eia_code.unique()\n )\n \n missing_util_geom_ids = [x for x in util_demand_ids if x not in util_geom_ids]\n logger.info(f\"{len(missing_util_geom_ids)} Utility respondents w/o geometries in {year}\")\n problem_ids = problem_ids.append(\n rids_ferc714\n .loc[rids_ferc714.utility_id_eia.isin(missing_util_geom_ids)]\n .assign(year=year)\n )",
"_____no_output_____"
],
[
"problem_ids.query(\"year==2010\").query(\"respondent_type=='balancing_authority'\")",
"_____no_output_____"
]
],
[
[
"## Dissolve to BA or Util\n* At this point we still have geometires at the county level.\n* This is 150,000+ records.\n* Really we just want a single geometry per respondent per year.\n* Dissolve based on year and respondent_id_ferc714.\n* Merge the annual per-respondent geometry with the rids_ferc714 which has more information\n* Note that this takes about half an hour to run...",
"_____no_output_____"
]
],
[
[
"%%time\ndissolved_rids_ferc714_gdf = (\n rids_ferc714_gdf.drop_duplicates(subset=[\"report_date\", \"county_id_fips\", \"respondent_id_ferc714\"])\n .dissolve(by=[\"report_date\", \"respondent_id_ferc714\"])\n .reset_index()\n .loc[:, [\"report_date\", \"respondent_id_ferc714\", \"geometry\"]]\n .merge(rids_ferc714, on=\"respondent_id_ferc714\", how=\"outer\")\n)\n#dissolved_rids_ferc714_gdf.to_file(\"planning_areas_ferc714.gpkg\", driver=\"GPKG\")",
"_____no_output_____"
]
],
[
[
"### Select based on respondent type",
"_____no_output_____"
]
],
[
[
"dissolved_utils = dissolved_rids_ferc714_gdf.query(\"respondent_type=='utility'\")\ndissolved_bas = dissolved_rids_ferc714_gdf.query(\"respondent_type=='balancing_authority'\")",
"_____no_output_____"
]
],
[
[
"### Nationwide BA / Util Maps\n* Still want to add the US state boundaries / coastlines to this for context.",
"_____no_output_____"
]
],
[
[
"unwanted_ba_ids = (\n 112, # Alaska\n 133, # Alaska\n 178, # Hawaii\n 301, # PJM Dupe\n 302, # PJM Dupe\n 303, # PJM Dupe\n 304, # PJM Dupe\n 305, # PJM Dupe\n 306, # PJM Dupe\n)\n\nfor report_date in pd.date_range(start=\"2010-01-01\", end=\"2018-01-01\", freq=\"AS\"):\n ba_ax = (\n dissolved_bas\n .query(\"report_date==@report_date\")\n .query(\"respondent_id_ferc714 not in @unwanted_ba_ids\")\n .plot(figsize=(20, 20), color=\"blue\", alpha=0.25, linewidth=1)\n )\n plt.title(f\"FERC 714 Balancing Authority Respondents {report_date}\")\n ctx.add_basemap(ba_ax)\n\n util_ax = (\n dissolved_utils\n .query(\"report_date==@report_date\")\n .plot(figsize=(20, 20), color=\"red\", alpha=0.25, linewidth=1)\n )\n plt.title(f\"FERC 714 Utility Respondents {report_date}\")\n ctx.add_basemap(util_ax)\n \n plt.show();",
"_____no_output_____"
]
],
[
[
"### Per-respondent annual maps\n* For each respondent make a grid of 9 (2010-2018)\n* Show state lines in bg for context\n* Limit bounding box by the respondent's territory",
"_____no_output_____"
],
[
"# Remaining Tasks",
"_____no_output_____"
],
[
"## Geometry Cleanup:\n* Why do some respondents lack geometries in some years?\n* Why do some respondents lack geometries in **all** years? (e.g. Tri-State G&T)\n* Why do some counties have no BA or Utility coverage in some or all years?\n* What combinations of years and respondents are missing?\n* Compare what we've ended up doing to the Aufhammer paper again.\n* Is there any need to use name-based matching between the Planning Area descriptions & EIA Service Territories?\n* Problem BAs / Utilities:\n * All the WAPA BAs\n * PacifiCorp East / West\n * Southern Company\n * MISO (Some other IDs that seem related?)\n * PJM (Early years seem out of bounds)",
"_____no_output_____"
],
[
"## FERC 714 Demand Time Series Cleanup\n\n### Find broken data:\n* Run Tyler Ruggles' anomaly detection code as improved by Greg Schivley\n* What kind of anomalies are we finding? Are they a problem? What portion of the overall dataset do they represent?\n\n### Repair data:\n* How do we want to fill in the gaps?\n* Ideally would be able to use the MICE technique that Tyler used, but we need to keep it all in Python.\n* Can do much simpler rolling averages or something for the moment when there are small gaps just to have completeness.\n* Should make this gap filling process modular -- use different techniques and see whether they do what we need.",
"_____no_output_____"
],
[
"# Miscellaneous Notes",
"_____no_output_____"
],
[
"## FERC 714 Demand Irregularities\nUnusual issues that need to be addressed, or demand discontinuities that may be useful in the context of aggregating historical demand into modern planning areas. Organized by FERC 714 Respondent ID:\n\n* Missing demand data / weird zeroes\n * 111: (2008)\n * 125: (2015)\n * 137: (2006)\n * 139: (2006) Only the last hour of every day. Maybe 0-23 vs 1-24 reporting?\n * 141: (2006, 2007, 2008, 2009, 2010)\n * 148: (2006)\n * 153: (2006)\n * 154: (2006)\n * 161: (all)\n * 183: (2007, 2009)\n * 208: (2008)\n * 273: (2007, 2008)\n * 283: (2007)\n * 287: (2008-2012)\n * 288: (2006)\n * 289: (2009)\n * 293: (2006)\n * 294: (2006)\n * 311: (2008-2011)\n* Inverted Demand (Sign Errors):\n * 156: (2006, 2007, 2008, 2009)\n * 289: (2006-2008, 2010)\n* Large demand discontinuities\n * 107: Demand triples at end of 2006.\n * 115: Two big step downs, 2007-2008, and 2011-2012\n * 121: 50% increase at end of 2007.\n * 128: Step up at end of 2007\n * 133: Step down end of 2013 and again end of 2015\n * 190: Demand doubled at end of 2008\n * 214: 50% jump in early 2012.\n * 256: big jump at end of 2006.\n * 261: Big jump at end of 2008.\n * 274: drop at end of 2007\n * 275: Jump at end of 2007\n * 287: Demand before and after big gap are very different.\n * 299: Big drop at end of 2015\n * 307: Jump at end of 2014\n * 321: Jump at end of 2013",
"_____no_output_____"
]
]
] | [
"markdown",
"code",
"markdown",
"code",
"markdown",
"code",
"markdown",
"code",
"markdown",
"code",
"markdown",
"code",
"markdown",
"code",
"markdown",
"code",
"markdown",
"code",
"markdown",
"code",
"markdown",
"code",
"markdown",
"code",
"markdown",
"code",
"markdown",
"code",
"markdown",
"code",
"markdown",
"code",
"markdown",
"code",
"markdown",
"code",
"markdown",
"code",
"markdown",
"code",
"markdown",
"code",
"markdown",
"code",
"markdown",
"code",
"markdown",
"code",
"markdown",
"code",
"markdown",
"code",
"markdown",
"code",
"markdown",
"code",
"markdown",
"code",
"markdown",
"code",
"markdown",
"code",
"markdown",
"code",
"markdown",
"code",
"markdown"
] | [
[
"markdown",
"markdown",
"markdown",
"markdown"
],
[
"code",
"code"
],
[
"markdown"
],
[
"code",
"code"
],
[
"markdown"
],
[
"code"
],
[
"markdown"
],
[
"code"
],
[
"markdown"
],
[
"code"
],
[
"markdown",
"markdown"
],
[
"code"
],
[
"markdown"
],
[
"code"
],
[
"markdown"
],
[
"code"
],
[
"markdown"
],
[
"code"
],
[
"markdown"
],
[
"code"
],
[
"markdown"
],
[
"code",
"code"
],
[
"markdown",
"markdown"
],
[
"code"
],
[
"markdown"
],
[
"code",
"code",
"code"
],
[
"markdown"
],
[
"code"
],
[
"markdown"
],
[
"code"
],
[
"markdown"
],
[
"code"
],
[
"markdown",
"markdown"
],
[
"code"
],
[
"markdown"
],
[
"code"
],
[
"markdown"
],
[
"code"
],
[
"markdown",
"markdown",
"markdown"
],
[
"code",
"code"
],
[
"markdown"
],
[
"code",
"code"
],
[
"markdown"
],
[
"code",
"code"
],
[
"markdown"
],
[
"code",
"code"
],
[
"markdown"
],
[
"code",
"code",
"code"
],
[
"markdown"
],
[
"code"
],
[
"markdown"
],
[
"code"
],
[
"markdown"
],
[
"code"
],
[
"markdown"
],
[
"code",
"code"
],
[
"markdown",
"markdown"
],
[
"code"
],
[
"markdown"
],
[
"code",
"code"
],
[
"markdown"
],
[
"code"
],
[
"markdown"
],
[
"code"
],
[
"markdown"
],
[
"code"
],
[
"markdown",
"markdown",
"markdown",
"markdown",
"markdown",
"markdown"
]
] |
d08fbe8bcf0141c254eef32e30b10aada07736c9 | 271,358 | ipynb | Jupyter Notebook | 02/homework_day2.ipynb | Py101/py101-assignments-moka1992 | 5e87d2bd1e799b532d89cf17bcb2425ded03b9cf | [
"MIT"
] | null | null | null | 02/homework_day2.ipynb | Py101/py101-assignments-moka1992 | 5e87d2bd1e799b532d89cf17bcb2425ded03b9cf | [
"MIT"
] | null | null | null | 02/homework_day2.ipynb | Py101/py101-assignments-moka1992 | 5e87d2bd1e799b532d89cf17bcb2425ded03b9cf | [
"MIT"
] | null | null | null | 399.055882 | 134,373 | 0.936435 | [
[
[
"<center>\n<hr>\n<h1>Python Crash Course</h1>\n<h2>Master in Data Science - Sapienza University</h2>\n<h2>Homework 2: Python Challenges</h2>\n<h3>A.A. 2017/18</h3>\n<h3>Tutor: Francesco Fabbri</h3>\n<hr>\n</center>\n\n",
"_____no_output_____"
],
[
"# Instructions\nSo guys, here we are! **Finally** you're facing your first **REAL** homework. Are you ready to fight?\nWe're going to apply all the Pythonic stuff seen before AND EVEN MORE...\n\n\n## Simple rules:\n\n1. Don't touch the instructions, you **just have to fill the blank rows**.\n\n\n2. This is supposed to be an exercise for improving your Pythonic Skills in a spirit of collaboration so...of course you can help your classmates and obviously get a really huge help as well from all the others (as the proverb says: \"I get help from you and then you help me\", right?!...)\n\n\n3. **RULE OF THUMB** for you during the homework:\n - *1st Step:* try to solve the problem alone\n - *2nd Step:* googling random the answer\n - *3rd Step:* ask to your colleagues\n - *3rd Step:* screaming and complaining about life \n - *4th Step:* ask to Tutors\n \n## And the Prize? The Beer?The glory?!:\nGuys the life is hard...in this Master it's even worse...\nSoooo, since that you seem so smart I want to test you before the start of all the courses.\n\n.\n\n.\n\n.\n\nBut not now.\n\nYou have to come prepared to the challenge, so right now solve these first 6 exercises, then it will be the time for **FIGHTING** and (for one of you) **DRINKING**.\n\n",
"_____no_output_____"
],
[
"# Warm-up...",
"_____no_output_____"
],
[
"### 1. 12! is equal to...",
"_____no_output_____"
]
],
[
[
"def fatt(n):\n if(n == 0):\n return 1\n else:\n return n*fatt(n-1)\nfatt(12)",
"_____no_output_____"
]
],
[
[
"### 2. More math...\nWrite a program which will find all such numbers which are divisible by 7 but are not a multiple of 5, between 0 and 1000 (both included). The numbers obtained should be printed in a comma-separated sequence on a single line. (range and CFS)",
"_____no_output_____"
]
],
[
[
"ex_2=[str(x) for x in range (1001) if x%7 ==0 and x%5 !=0]\n','.join(ex_2)",
"_____no_output_____"
]
],
[
[
"### 2. Count capital letters\nIn this exercises you're going to deal with YOUR DATA. Indeed, in the list below there are stored your Favorite Tv Series. But, as you can see, there is something weird. There are too much CaPITal LeTTErs. Your task is to count the capital letters in all the strings and then print the total number of capital letters in all the list.",
"_____no_output_____"
]
],
[
[
"tv_series = ['Game of THRroneS',\n 'big bang tHeOrY',\n 'MR robot',\n 'WesTWorlD',\n 'fIRefLy',\n \"i haven't\",\n 'HOW I MET your mothER',\n 'friENds',\n 'bRon broen',\n 'gossip girl',\n 'prISon break',\n 'breaking BAD']",
"_____no_output_____"
],
[
"count=0\nfor string in tv_series:\n for letter in string:\n if letter.lower() == letter:\n pass\n else:\n count+=1\n",
"_____no_output_____"
],
[
"count",
"_____no_output_____"
]
],
[
[
"### 3. A remark\nUsing the list above, create a dictionary where the keys are Unique IDs and values the TV Series.\nYou have to do the exercise keeping in mind these 2 constraints: \n\n1. The order of the IDs has to be **dependent on the alphabetical order of the titles**, i.e. 0: first_title_in_alphabetical_order and so on...\n\n\n2. **Solve the mess** of the capital letter: we want them only at the start of the words (\"prISon break\" should be \"Prison Break\")\n",
"_____no_output_____"
]
],
[
[
"# write here your code\nnewlst = []\nfor x in tv_series:\n x.title()\n newlst.append(x.title())\n\nnewlst\n",
"_____no_output_____"
],
[
"a=range(12)\nb=sorted(newlst)\ndict1=dict(zip(a,b))\ndict1",
"_____no_output_____"
]
],
[
[
"### 4. Dictionary to its maximum\nInvert the keys with the values in the dictionary built before. ",
"_____no_output_____"
]
],
[
[
"# write here your code\n\ninv= {v: k for k, v in dict1.items()}\n\ninv",
"_____no_output_____"
]
],
[
[
"Have you done in **one line of code**? If not, try now!",
"_____no_output_____"
]
],
[
[
"# write here your code\nalready done :D",
"_____no_output_____"
]
],
[
[
"### 4. Other boring math\nLet's talk about our beloved exams. Starting from the exams and CFU below, are you able to compute the weighted mean of them?\nLet's do it and print the result.\n\nDescription of the data:\n\nexams[1] = $(title_1, grade_1)$\n\ncfu[1] = $CFU_1$",
"_____no_output_____"
]
],
[
[
"exams = [('BIOINFORMATICS', 29),\n ('DATA MANAGEMENT FOR DATA SCIENCE', 30),\n ('DIGITAL EPIDEMIOLOGY', 26),\n ('NETWORKING FOR BIG DATA AND LABORATORY',28),\n ('QUANTITATIVE MODELS FOR ECONOMIC ANALYSIS AND MANAGEMENT','30 e lode'),\n ('DATA MINING TECHNOLOGY FOR BUSINESS AND SOCIETY', 30),\n ('STATISTICAL LEARNING',30),\n ('ALGORITHMIC METHODS OF DATA MINING AND LABORATORY',30),\n ('FUNDAMENTALS OF DATA SCIENCE AND LABORATORY', 29)]\n\ncfu = sum([6,6,6,9,6,6,6,9,9])",
"_____no_output_____"
],
[
"cfu",
"_____no_output_____"
],
[
"type(exams [0])",
"_____no_output_____"
],
[
"a=list(zip (*exams))[1]\na\n",
"_____no_output_____"
],
[
"type (a)",
"_____no_output_____"
],
[
"singlecfu=([6,6,6,9,6,6,6,9,9])",
"_____no_output_____"
],
[
"b= (a[]*singlecfu[])/(cfu)\nb",
"_____no_output_____"
],
[
"mean= dict2 [0]",
"_____no_output_____"
]
],
[
[
"### 5. Palindromic numbers\nWrite a script which finds all the Palindromic numbers, in the range [0,**N**] (bounds included). The numbers obtained should be printed in a comma-separated sequence on a single line.\n\nWhat is **N**?\nLooking at the exercise before:\n**N** = (Total number of CFU) x (Sum of all the grades)\n\n(details: https://en.wikipedia.org/wiki/Palindromic_number)\n",
"_____no_output_____"
]
],
[
[
"def pali(n):\n return str(n) == str(n)[::-1]\na=list(filter(pali, range(0,15876)))\nprint(a)",
"[0, 1, 2, 3, 4, 5, 6, 7, 8, 9, 11, 22, 33, 44, 55, 66, 77, 88, 99, 101, 111, 121, 131, 141, 151, 161, 171, 181, 191, 202, 212, 222, 232, 242, 252, 262, 272, 282, 292, 303, 313, 323, 333, 343, 353, 363, 373, 383, 393, 404, 414, 424, 434, 444, 454, 464, 474, 484, 494, 505, 515, 525, 535, 545, 555, 565, 575, 585, 595, 606, 616, 626, 636, 646, 656, 666, 676, 686, 696, 707, 717, 727, 737, 747, 757, 767, 777, 787, 797, 808, 818, 828, 838, 848, 858, 868, 878, 888, 898, 909, 919, 929, 939, 949, 959, 969, 979, 989, 999, 1001, 1111, 1221, 1331, 1441, 1551, 1661, 1771, 1881, 1991, 2002, 2112, 2222, 2332, 2442, 2552, 2662, 2772, 2882, 2992, 3003, 3113, 3223, 3333, 3443, 3553, 3663, 3773, 3883, 3993, 4004, 4114, 4224, 4334, 4444, 4554, 4664, 4774, 4884, 4994, 5005, 5115, 5225, 5335, 5445, 5555, 5665, 5775, 5885, 5995, 6006, 6116, 6226, 6336, 6446, 6556, 6666, 6776, 6886, 6996, 7007, 7117, 7227, 7337, 7447, 7557, 7667, 7777, 7887, 7997, 8008, 8118, 8228, 8338, 8448, 8558, 8668, 8778, 8888, 8998, 9009, 9119, 9229, 9339, 9449, 9559, 9669, 9779, 9889, 9999, 10001, 10101, 10201, 10301, 10401, 10501, 10601, 10701, 10801, 10901, 11011, 11111, 11211, 11311, 11411, 11511, 11611, 11711, 11811, 11911, 12021, 12121, 12221, 12321, 12421, 12521, 12621, 12721, 12821, 12921, 13031, 13131, 13231, 13331, 13431, 13531, 13631, 13731, 13831, 13931, 14041, 14141, 14241, 14341, 14441, 14541, 14641, 14741, 14841, 14941, 15051, 15151, 15251, 15351, 15451, 15551, 15651, 15751, 15851]\n"
],
[
"?filter",
"_____no_output_____"
]
],
[
[
"### 6. StackOverflow",
"_____no_output_____"
],
[
"Let's start using your new best friend. Now I'm going to give other task, slightly more difficult BUT this time, just googling, you will find easily the answer on the www.stackoverflow.com. You can use the code there for solving the exercise BUT you have to understand the solution there **COMMENTING** the code, showing me you understood the thinking process behind the code.",
"_____no_output_____"
],
[
"### 6. A\nShow me an example of how to use **PROPERLY** the *Try - Except* statements",
"_____no_output_____"
]
],
[
[
"# write here your code",
"_____no_output_____"
]
],
[
[
"#### 6. B\nGiving this list of words below, after copying in a variable, explain and provide me a code for obtaining a **Bag of Words** from them.\n(Hint: use dictionaries and loops)",
"_____no_output_____"
],
[
"['theory', 'of', 'bron', 'firefly', 'thrones', 'break', 'bad', 'mother', 'firefly', \"haven't\", 'prison', 'big', 'friends', 'girl', 'westworld', 'bad', \"haven't\", 'gossip', 'thrones', 'your', 'big', 'how', 'friends', 'theory', 'your', 'bron', 'bad', 'bad', 'breaking', 'met', 'breaking', 'breaking', 'game', 'bron', 'your', 'breaking', 'met', 'bang', 'how', 'mother', 'bad', 'theory', 'how', 'i', 'friends', \"haven't\", 'of', 'of', 'gossip', 'i', 'robot', 'of', 'prison', 'bad', 'friends', 'friends', 'i', 'robot', 'bang', 'mother', 'bang', 'i', 'of', 'bad', 'friends', 'theory', 'i', 'friends', 'thrones', 'prison', 'theory', 'theory', 'big', 'of', 'bang', 'how', 'thrones', 'bang', 'theory', 'friends', 'game', 'bang', 'mother', 'broen', 'bad', 'game', 'break', 'break', 'bang', 'big', 'gossip', 'robot', 'met', 'i', 'game', 'your', 'met', 'bad', 'firefly', 'your']",
"_____no_output_____"
]
],
[
[
"# write here your code",
"_____no_output_____"
]
],
[
[
"#### 6. C\nAnd now, write down a code which computes the first 10 Fibonacci numbers\n\n(details: https://en.wikipedia.org/wiki/Fibonacci_number)",
"_____no_output_____"
]
],
[
[
"# write here your code",
"_____no_output_____"
]
]
] | [
"markdown",
"code",
"markdown",
"code",
"markdown",
"code",
"markdown",
"code",
"markdown",
"code",
"markdown",
"code",
"markdown",
"code",
"markdown",
"code",
"markdown",
"code",
"markdown",
"code",
"markdown",
"code"
] | [
[
"markdown",
"markdown",
"markdown",
"markdown"
],
[
"code"
],
[
"markdown"
],
[
"code"
],
[
"markdown"
],
[
"code",
"code",
"code"
],
[
"markdown"
],
[
"code",
"code"
],
[
"markdown"
],
[
"code"
],
[
"markdown"
],
[
"code"
],
[
"markdown"
],
[
"code",
"code",
"code",
"code",
"code",
"code",
"code",
"code"
],
[
"markdown"
],
[
"code",
"code"
],
[
"markdown",
"markdown",
"markdown"
],
[
"code"
],
[
"markdown",
"markdown"
],
[
"code"
],
[
"markdown"
],
[
"code"
]
] |
d08fda3287e132cfd6d5332d572defc21cee513b | 265,575 | ipynb | Jupyter Notebook | w3/w3-day_1/.ipynb_checkpoints/Matplotlib_tutorial-checkpoint.ipynb | bmskarate/lighthouseMain | b2434f14f1378b89085d59f896c44eda5f74eecc | [
"MIT"
] | null | null | null | w3/w3-day_1/.ipynb_checkpoints/Matplotlib_tutorial-checkpoint.ipynb | bmskarate/lighthouseMain | b2434f14f1378b89085d59f896c44eda5f74eecc | [
"MIT"
] | null | null | null | w3/w3-day_1/.ipynb_checkpoints/Matplotlib_tutorial-checkpoint.ipynb | bmskarate/lighthouseMain | b2434f14f1378b89085d59f896c44eda5f74eecc | [
"MIT"
] | null | null | null | 510.721154 | 73,692 | 0.939155 | [
[
[
"import numpy\nimport numpy as np\n\n# import matplotlib\nimport matplotlib.pyplot as plt\n\n# set the figure size for each figure in this tutorial\nplt.rcParams[\"figure.figsize\"] = (10,6)",
"_____no_output_____"
]
],
[
[
"## Lineplot",
"_____no_output_____"
]
],
[
[
"# 200 values from the interval <0,100>, equidistantly divided\nx = np.linspace(0,100,200)\ny = np.sin(x)\n\n# a line plot\nplt.plot(x,y,'red')\nplt.show()",
"_____no_output_____"
]
],
[
[
"## scatterplot",
"_____no_output_____"
]
],
[
[
"# 200 random values from the interval <0,10>\nx = 10*np.random.rand(200,1)\n\n# 200 random values from the interval <0,15>\ny = 15*np.random.rand(200,1)\n\n# a scatter plot \nplt.scatter(x,y)\nplt.show()",
"_____no_output_____"
]
],
[
[
"## histogram",
"_____no_output_____"
]
],
[
[
"# 200 random values from the interval <0,15>\ny = 15*np.random.rand(200,1)\n\n# a histogram with 20 bins\nplt.hist(y,bins=20)\nplt.show()",
"_____no_output_____"
]
],
[
[
"## Graphs on common axes",
"_____no_output_____"
]
],
[
[
"# 200 values from the interval <0,100>, equidistantly divided\nx = np.linspace(0,100,200)\n\n# sin(x) values\ny1 = np.sin(x)\n\n# sin(x)*cos(x) values\ny2 =(np.sin(x))*(np.cos(x))\n\n# a line plot of sin(x), red line\nplt.plot(x,y1,'red')\n\n# a line plot of sin(x)*cos(x), blue line\nplt.plot(x,y2,'blue')\nplt.show()",
"_____no_output_____"
]
],
[
[
"## Subplots",
"_____no_output_____"
]
],
[
[
"# the first figure\nplt.subplot(2,1,1)\nplt.plot(x,y1,'red')\nplt.title('sin(x)')\n\n# the second figure\nplt.subplot(2,1,2)\nplt.plot(x,y2,'blue')\nplt.title('sin(x)*(cos(x))')\n\n# automatically adjust the subplot parameters to give a specified padding\nplt.tight_layout()\nplt.show()",
"_____no_output_____"
]
],
[
[
"## Legends",
"_____no_output_____"
]
],
[
[
"# import pandas\nimport pandas as pd\n\n# import sklearn datasets\nfrom sklearn import datasets",
"_____no_output_____"
],
[
"# load iris dataset\niris = datasets.load_iris()\n\n# create dataframe\niris_df = pd.DataFrame(iris.data, columns=iris.feature_names)\n\n# create target\niris_df['target'] = iris.target\n\n# map the target values to the target names\niris_df['target_name'] =iris_df.target.map(\n {0: 'setosa', \n 1: 'versicolor',\n 2: 'virginica'}\n)",
"_____no_output_____"
],
[
"iris_df.head()",
"_____no_output_____"
],
[
"# Iris setosa\nsetosa = iris_df[iris_df.target_name == 'setosa']\n\n# Iris versicolor\nversicolor = iris_df[iris_df.target_name == 'versicolor']\n\n# Iris virginica\nvirginica = iris_df[iris_df.target_name == 'virginica']\n\n# plot setosa\nplt.scatter(setosa['sepal length (cm)'], setosa['sepal width (cm)'],\n marker ='o', color = 'red', label = 'setosa')\n\n# plot versicolor\nplt.scatter(versicolor['sepal length (cm)'], versicolor['sepal width (cm)'],\n marker ='o', color = 'green', label = 'versicolor')\n\n# plot virginica\nplt.scatter(virginica['sepal length (cm)'], virginica['sepal width (cm)'],\n marker ='o', color = 'blue', label = 'virginica')\n\n# legend location\nplt.legend(loc='upper right')\n\n# plot title\nplt.title('Iris flower')\n\n# x-axis title\nplt.xlabel('sepal length (cm)')\n\n# y-axis title\nplt.ylabel('sepal width (cm)')\nplt.show()",
"_____no_output_____"
]
],
[
[
"## Annotations",
"_____no_output_____"
]
],
[
[
"# the same code as before\nplt.scatter(setosa['sepal length (cm)'],setosa['sepal width (cm)'],\n marker ='o', color = 'red', label = 'setosa')\n\nplt.scatter(versicolor['sepal length (cm)'],versicolor['sepal width (cm)'],\n marker ='o', color = 'green', label = 'versicolor')\n\nplt.scatter(virginica['sepal length (cm)'],virginica['sepal width (cm)'],\n marker ='o', color = 'blue', label = 'virginica')\n\n# new lines of code\n# it can be tricky to find the right coordinates for the first time\n######################\nplt.annotate('setosa', xy =(5.0,3.5),\n xytext = (4.25,4.0), arrowprops={'color':'red'})\nplt.annotate('versicolor', xy =(7.2,3.6),\n xytext = (6.5,4.0), arrowprops={'color':'red'})\nplt.annotate('virginica', xy =(5.05,1.95),\n xytext = (5.5,1.75), arrowprops={'color':'red'})\n######################\n\n# the same code as before\nplt.legend(loc='upper right')\nplt.title('Iris flower')\nplt.xlabel('sepal length (cm)')\nplt.ylabel('sepal width (cm)')\nplt.ylim(1.5,4.7)\nplt.show()",
"_____no_output_____"
]
]
] | [
"code",
"markdown",
"code",
"markdown",
"code",
"markdown",
"code",
"markdown",
"code",
"markdown",
"code",
"markdown",
"code",
"markdown",
"code"
] | [
[
"code"
],
[
"markdown"
],
[
"code"
],
[
"markdown"
],
[
"code"
],
[
"markdown"
],
[
"code"
],
[
"markdown"
],
[
"code"
],
[
"markdown"
],
[
"code"
],
[
"markdown"
],
[
"code",
"code",
"code",
"code"
],
[
"markdown"
],
[
"code"
]
] |
d08fe37965d47235c4f9c9865e497fdec6d84cec | 184,315 | ipynb | Jupyter Notebook | testing_of_model.ipynb | awantika10/manthan | 71549f51d91c4294544ff81d1ad009e52d90fd75 | [
"OLDAP-2.3"
] | null | null | null | testing_of_model.ipynb | awantika10/manthan | 71549f51d91c4294544ff81d1ad009e52d90fd75 | [
"OLDAP-2.3"
] | null | null | null | testing_of_model.ipynb | awantika10/manthan | 71549f51d91c4294544ff81d1ad009e52d90fd75 | [
"OLDAP-2.3"
] | null | null | null | 446.283293 | 132,733 | 0.935165 | [
[
[
"import numpy as np\nimport cv2\nimport matplotlib.pyplot as plt\nfrom keras import models\nimport keras.backend as K\nimport tensorflow as tf\nfrom sklearn.metrics import f1_score\nimport requests\nimport xmltodict\nimport json",
"Using TensorFlow backend.\n"
],
[
"plateCascade = cv2.CascadeClassifier('indian_license_plate.xml')",
"_____no_output_____"
],
[
"#detect the plate and return car + plate image\ndef plate_detect(img):\n plateImg = img.copy()\n roi = img.copy()\n plateRect = plateCascade.detectMultiScale(plateImg,scaleFactor = 1.2, minNeighbors = 7)\n for (x,y,w,h) in plateRect:\n roi_ = roi[y:y+h, x:x+w, :]\n plate_part = roi[y:y+h, x:x+w, :]\n cv2.rectangle(plateImg,(x+2,y),(x+w-3, y+h-5),(0,255,0),3)\n return plateImg, plate_part",
"_____no_output_____"
],
[
"#normal function to display \ndef display_img(img):\n img_ = cv2.cvtColor(img,cv2.COLOR_BGR2RGB)\n plt.imshow(img_)\n plt.show()",
"_____no_output_____"
],
[
"#test image is used for detecting plate\ninputImg = cv2.imread('test.jpeg')\ninpImg, plate = plate_detect(inputImg)\ndisplay_img(inpImg)",
"_____no_output_____"
],
[
"def find_contours(dimensions, img) :\n\n #finding all contours in the image using \n #retrieval mode: RETR_TREE\n #contour approximation method: CHAIN_APPROX_SIMPLE\n cntrs, _ = cv2.findContours(img.copy(), cv2.RETR_TREE, cv2.CHAIN_APPROX_SIMPLE)\n\n #Approx dimensions of the contours\n lower_width = dimensions[0]\n upper_width = dimensions[1]\n lower_height = dimensions[2]\n upper_height = dimensions[3]\n \n #Check largest 15 contours for license plate character respectively\n cntrs = sorted(cntrs, key=cv2.contourArea, reverse=True)[:15]\n \n ci = cv2.imread('contour.jpg')\n \n x_cntr_list = []\n target_contours = []\n img_res = []\n for cntr in cntrs :\n #detecting contour in binary image and returns the coordinates of rectangle enclosing it\n intX, intY, intWidth, intHeight = cv2.boundingRect(cntr)\n \n #checking the dimensions of the contour to filter out the characters by contour's size\n if intWidth > lower_width and intWidth < upper_width and intHeight > lower_height and intHeight < upper_height :\n x_cntr_list.append(intX) \n char_copy = np.zeros((44,24))\n #extracting each character using the enclosing rectangle's coordinates.\n char = img[intY:intY+intHeight, intX:intX+intWidth]\n char = cv2.resize(char, (20, 40))\n cv2.rectangle(ci, (intX,intY), (intWidth+intX, intY+intHeight), (50,21,200), 2)\n plt.imshow(ci, cmap='gray')\n char = cv2.subtract(255, char)\n char_copy[2:42, 2:22] = char\n char_copy[0:2, :] = 0\n char_copy[:, 0:2] = 0\n char_copy[42:44, :] = 0\n char_copy[:, 22:24] = 0\n img_res.append(char_copy) # List that stores the character's binary image (unsorted)\n \n #return characters on ascending order with respect to the x-coordinate\n \n plt.show()\n #arbitrary function that stores sorted list of character indeces\n indices = sorted(range(len(x_cntr_list)), key=lambda k: x_cntr_list[k])\n img_res_copy = []\n for idx in indices:\n img_res_copy.append(img_res[idx])# stores character images according to their index\n img_res = np.array(img_res_copy)\n\n return img_res",
"_____no_output_____"
],
[
"def segment_characters(image) :\n\n #pre-processing cropped image of plate\n #threshold: convert to pure b&w with sharpe edges\n #erod: increasing the backgroung black\n #dilate: increasing the char white\n img_lp = cv2.resize(image, (333, 75))\n img_gray_lp = cv2.cvtColor(img_lp, cv2.COLOR_BGR2GRAY)\n _, img_binary_lp = cv2.threshold(img_gray_lp, 200, 255, cv2.THRESH_BINARY+cv2.THRESH_OTSU)\n img_binary_lp = cv2.erode(img_binary_lp, (3,3))\n img_binary_lp = cv2.dilate(img_binary_lp, (3,3))\n\n LP_WIDTH = img_binary_lp.shape[0]\n LP_HEIGHT = img_binary_lp.shape[1]\n img_binary_lp[0:3,:] = 255\n img_binary_lp[:,0:3] = 255\n img_binary_lp[72:75,:] = 255\n img_binary_lp[:,330:333] = 255\n\n #estimations of character contours sizes of cropped license plates\n dimensions = [LP_WIDTH/6,\n LP_WIDTH/2,\n LP_HEIGHT/10,\n 2*LP_HEIGHT/3]\n plt.imshow(img_binary_lp, cmap='gray')\n plt.show()\n cv2.imwrite('contour.jpg',img_binary_lp)\n\n #getting contours\n char_list = find_contours(dimensions, img_binary_lp)\n\n return char_list",
"_____no_output_____"
],
[
"char = segment_characters(plate)",
"_____no_output_____"
],
[
"for i in range(10):\n plt.subplot(1, 10, i+1)\n plt.imshow(char[i], cmap='gray')\n plt.axis('off')",
"_____no_output_____"
],
[
"#It is the harmonic mean of precision and recall\n#Output range is [0, 1]\n#Works for both multi-class and multi-label classification\n\ndef f1score(y, y_pred):\n return f1_score(y, tf.math.argmax(y_pred, axis=1), average='micro') \n\ndef custom_f1score(y, y_pred):\n return tf.py_function(f1score, (y, y_pred), tf.double)",
"_____no_output_____"
],
[
" model = models.load_model('license_plate_character.pkl', custom_objects= {'custom_f1score': custom_f1score})",
"_____no_output_____"
],
[
"def fix_dimension(img):\n new_img = np.zeros((28,28,3))\n for i in range(3):\n new_img[:,:,i] = img\n return new_img\n \ndef show_results():\n dic = {}\n characters = '0123456789ABCDEFGHIJKLMNOPQRSTUVWXYZ'\n for i,c in enumerate(characters):\n dic[i] = c\n\n output = []\n for i,ch in enumerate(char): \n img_ = cv2.resize(ch, (28,28), interpolation=cv2.INTER_AREA)\n img = fix_dimension(img_)\n img = img.reshape(1,28,28,3)\n y_ = model.predict_classes(img)[0]\n character = dic[y_] #\n output.append(character) \n \n plate_number = ''.join(output)\n \n return plate_number\n\nfinal_plate = show_results()\nprint(final_plate)",
"IMH20EE7598\n"
],
[
"def get_vehicle_info(plate_number):\n r = requests.get(\"http://www.regcheck.org.uk/api/reg.asmx/CheckIndia?RegistrationNumber={0}&username=licenseguy\".format(str(plate_number)))\n data = xmltodict.parse(r.content)\n jdata = json.dumps(data)\n df = json.loads(jdata)\n df1 = json.loads(df['Vehicle']['vehicleJson'])\n return df1\n",
"_____no_output_____"
],
[
"if len(final_plate) > 10:\n final_plate = final_plate[-10:]\n print(final_plate)",
"_____no_output_____"
],
[
"get_vehicle_info(final_plate)",
"_____no_output_____"
]
]
] | [
"code"
] | [
[
"code",
"code",
"code",
"code",
"code",
"code",
"code",
"code",
"code",
"code",
"code",
"code",
"code",
"code",
"code"
]
] |
d08feb3af153e12b1a8fa5bd04b5fb15572f1254 | 115,674 | ipynb | Jupyter Notebook | Appliances_Energy_Prediction_Regression/AEP_test_1.ipynb | JagadeeshaSV/UCI_Dataset_Projects | e1ecbcdcf14021d55ac8d76de3701ea77217cc96 | [
"MIT"
] | null | null | null | Appliances_Energy_Prediction_Regression/AEP_test_1.ipynb | JagadeeshaSV/UCI_Dataset_Projects | e1ecbcdcf14021d55ac8d76de3701ea77217cc96 | [
"MIT"
] | null | null | null | Appliances_Energy_Prediction_Regression/AEP_test_1.ipynb | JagadeeshaSV/UCI_Dataset_Projects | e1ecbcdcf14021d55ac8d76de3701ea77217cc96 | [
"MIT"
] | null | null | null | 105.349727 | 76,356 | 0.779881 | [
[
[
"# Datset source\n# https://archive.ics.uci.edu/ml/datasets/Appliances+energy+prediction",
"_____no_output_____"
],
[
"# Problem statement: Predict the appliances energy use based on various features",
"_____no_output_____"
],
[
"# Python ≥3.5 is required\nimport sys\nassert sys.version_info >= (3, 5)\n\n# Scikit-Learn ≥0.20 is required\nimport sklearn\nassert sklearn.__version__ >= \"0.20\"\n\n# Common imports\nimport numpy as np\nimport os\n\n# To plot pretty figures\n%matplotlib inline\nimport matplotlib as mpl\nimport matplotlib.pyplot as plt\nmpl.rc('axes', labelsize=14)\nmpl.rc('xtick', labelsize=12)\nmpl.rc('ytick', labelsize=12)\n\n# Ignore useless warnings (see SciPy issue #5998)\nimport warnings\nwarnings.filterwarnings(action=\"ignore\", message=\"^internal gelsd\")",
"_____no_output_____"
],
[
"# Read the dataset\n\nimport pandas as pd\npd.options.display.max_columns = 1000\naep_df = pd.read_csv('energydata_complete.csv', sep=',')\nprint(aep_df.shape)\naep_df.head()",
"(19735, 29)\n"
],
[
"# Check for NAN values in the entire dataframe\n\naep_df.isnull().sum().sum()",
"_____no_output_____"
],
[
"# Info about the dataframe\n\naep_df.info()",
"<class 'pandas.core.frame.DataFrame'>\nRangeIndex: 19735 entries, 0 to 19734\nData columns (total 29 columns):\n # Column Non-Null Count Dtype \n--- ------ -------------- ----- \n 0 date 19735 non-null object \n 1 Appliances 19735 non-null int64 \n 2 lights 19735 non-null int64 \n 3 T1 19735 non-null float64\n 4 RH_1 19735 non-null float64\n 5 T2 19735 non-null float64\n 6 RH_2 19735 non-null float64\n 7 T3 19735 non-null float64\n 8 RH_3 19735 non-null float64\n 9 T4 19735 non-null float64\n 10 RH_4 19735 non-null float64\n 11 T5 19735 non-null float64\n 12 RH_5 19735 non-null float64\n 13 T6 19735 non-null float64\n 14 RH_6 19735 non-null float64\n 15 T7 19735 non-null float64\n 16 RH_7 19735 non-null float64\n 17 T8 19735 non-null float64\n 18 RH_8 19735 non-null float64\n 19 T9 19735 non-null float64\n 20 RH_9 19735 non-null float64\n 21 T_out 19735 non-null float64\n 22 Press_mm_hg 19735 non-null float64\n 23 RH_out 19735 non-null float64\n 24 Windspeed 19735 non-null float64\n 25 Visibility 19735 non-null float64\n 26 Tdewpoint 19735 non-null float64\n 27 rv1 19735 non-null float64\n 28 rv2 19735 non-null float64\ndtypes: float64(26), int64(2), object(1)\nmemory usage: 4.4+ MB\n"
],
[
"# Some statistics about the dataframe\n\naep_df.describe()",
"_____no_output_____"
],
[
"# Plot the histograms for all the features in the dataset\n\naep_df.hist(bins=50, figsize=(20,15))\nplt.show()",
"_____no_output_____"
],
[
"# To make this notebook's output identical at every run\n\nnp.random.seed(2)",
"_____no_output_____"
],
[
"# Plot correlation between scaled sound level in decibels and other features\n\ncorr_matrix = aep_df.corr()\ncorr_matrix[\"Appliances\"].sort_values(ascending=False)",
"_____no_output_____"
],
[
"# Split the dataframe into features and labels\n\nX = aep_df.drop(['date', 'Appliances'], axis=1).values\ny = aep_df.loc[:, 'Appliances'].values\nprint(\"X shape: \", X.shape, \"y shape: \", y.shape)\nprint(\"Sample X values: \", X[:5], \"\\n\", \"Sample y values: \", y[:5])",
"X shape: (19735, 27) y shape: (19735,)\nSample X values: [[ 30. 19.89 47.59666667 19.2 44.79\n 19.79 44.73 19. 45.56666667 17.16666667\n 55.2 7.02666667 84.25666667 17.2 41.62666667\n 18.2 48.9 17.03333333 45.53 6.6\n 733.5 92. 7. 63. 5.3\n 13.27543316 13.27543316]\n [ 30. 19.89 46.69333333 19.2 44.7225\n 19.79 44.79 19. 45.9925 17.16666667\n 55.2 6.83333333 84.06333333 17.2 41.56\n 18.2 48.86333333 17.06666667 45.56 6.48333333\n 733.6 92. 6.66666667 59.16666667 5.2\n 18.60619498 18.60619498]\n [ 30. 19.89 46.3 19.2 44.62666667\n 19.79 44.93333333 18.92666667 45.89 17.16666667\n 55.09 6.56 83.15666667 17.2 41.43333333\n 18.2 48.73 17. 45.5 6.36666667\n 733.7 92. 6.33333333 55.33333333 5.1\n 28.64266817 28.64266817]\n [ 40. 19.89 46.06666667 19.2 44.59\n 19.79 45. 18.89 45.72333333 17.16666667\n 55.09 6.43333333 83.42333333 17.13333333 41.29\n 18.1 48.59 17. 45.4 6.25\n 733.8 92. 6. 51.5 5.\n 45.4103895 45.4103895 ]\n [ 40. 19.89 46.33333333 19.2 44.53\n 19.79 45. 18.89 45.53 17.2\n 55.09 6.36666667 84.89333333 17.2 41.23\n 18.1 48.59 17. 45.4 6.13333333\n 733.9 92. 5.66666667 47.66666667 4.9\n 10.08409655 10.08409655]] \n Sample y values: [60 60 50 50 60]\n"
],
[
"# Split the dataset into train, validation and test sets\n\nfrom sklearn.model_selection import train_test_split\nX_train, X_test, y_train, y_test = train_test_split(X, y, test_size=0.05, random_state=2)\nX_train, X_val, y_train, y_val = train_test_split(X_train, y_train, test_size=0.05, random_state=2)\nprint(\" X_train shape: \", X_train.shape,\"\\n\", \"y_train shape: \", y_train.shape,\"\\n\",\n \"X_val shape: \", X_val.shape,\"\\n\", \"y_val shape: \", y_val.shape,\"\\n\",\n \"X_test shape: \", X_test.shape,\"\\n\", \"y_test shape: \", y_test.shape,\"\\n\")",
" X_train shape: (17810, 27) \n y_train shape: (17810,) \n X_val shape: (938, 27) \n y_val shape: (938,) \n X_test shape: (987, 27) \n y_test shape: (987,) \n\n"
],
[
"# Model 1\n# Sklearn Simple Linear Regression model with default parameters\n\nfrom sklearn.linear_model import LinearRegression\nlr_model_1 = LinearRegression()\nlr_model_1.fit(X_train, y_train)\nprint(\"Train set score: \", lr_model_1.score(X_train, y_train))\nprint(\"Validation set score: \", lr_model_1.score(X_val, y_val))\nprint(\"Test set score: \", lr_model_1.score(X_test, y_test))",
"Train set score: 0.16269008141806152\nValidation set score: 0.2234885503227645\nTest set score: 0.14762836364067866\n"
],
[
"# Mean Squared Errors of train, validation and test set predictions\n\nfrom sklearn.metrics import mean_squared_error\nprint(\"Train set mse: \", mean_squared_error(y_train, lr_model_1.predict(X_train)))\nprint(\"Validation set mse: \", mean_squared_error(y_val, lr_model_1.predict(X_val)))\nprint(\"Test set mse: \", mean_squared_error(y_test, lr_model_1.predict(X_test)))",
"Train set mse: 8910.858986160203\nValidation set mse: 7435.4767179210285\nTest set mse: 7682.529682926291\n"
],
[
"# Here the R^2 values are very low and MSE values are very high, more complex models are required to fit the data",
"_____no_output_____"
],
[
"# Model 2\n# Sklearn Simple Linear Regression model with normalized data\n\nfrom sklearn.linear_model import LinearRegression\nlr_model_2 = LinearRegression(normalize=True)\nlr_model_2.fit(X_train, y_train)\nprint(\"Train set score: \", lr_model_2.score(X_train, y_train))\nprint(\"Validation set score: \", lr_model_2.score(X_val, y_val))\nprint(\"Test set score: \", lr_model_2.score(X_test, y_test))",
"Train set score: 0.1626900814180614\nValidation set score: 0.22348855032276438\nTest set score: 0.14762836364067833\n"
],
[
"# Here normalizing the data didn't made any difference, confirming that more complex models are required to fit the data",
"_____no_output_____"
]
]
] | [
"code"
] | [
[
"code",
"code",
"code",
"code",
"code",
"code",
"code",
"code",
"code",
"code",
"code",
"code",
"code",
"code",
"code",
"code",
"code"
]
] |
d08fef83f8b83dc3e3989e8aaa8fb89c7d3bc3db | 5,576 | ipynb | Jupyter Notebook | fridge/chapter-7a.ipynb | romilly/o-x-o | 861fdb7f7169d2e0f7648703a759c36c09b8c295 | [
"MIT"
] | 1 | 2022-03-04T12:09:00.000Z | 2022-03-04T12:09:00.000Z | fridge/chapter-7a.ipynb | romilly/o-x-o | 861fdb7f7169d2e0f7648703a759c36c09b8c295 | [
"MIT"
] | 1 | 2022-03-23T07:59:47.000Z | 2022-03-23T07:59:47.000Z | fridge/chapter-7a.ipynb | romilly/o-x-o | 861fdb7f7169d2e0f7648703a759c36c09b8c295 | [
"MIT"
] | null | null | null | 35.515924 | 281 | 0.630022 | [
[
[
"empty"
]
]
] | [
"empty"
] | [
[
"empty"
]
] |
d08ff6a1f5fe6c6085c4f5b6926edd5970a46c03 | 13,109 | ipynb | Jupyter Notebook | EdX/GTx: CSE6040x: FA18 - Computing for Data Analysis/Module 1: Representing, transforming and visualizing data/Topic 6 + Notebook 6 (OPTIONAL): Mining the web/part6.ipynb | helpthx/Path_through_Data_Science_2019 | aa22333eae970506f2ce184551c55565b0be89fb | [
"MIT"
] | 2 | 2019-02-06T09:30:44.000Z | 2019-02-09T18:24:46.000Z | EdX/GTx: CSE6040x: FA18 - Computing for Data Analysis/Module 1: Representing, transforming and visualizing data/Topic 6 + Notebook 6 (OPTIONAL): Mining the web/part6.ipynb | helpthx/Path_through_Data_Science_2019 | aa22333eae970506f2ce184551c55565b0be89fb | [
"MIT"
] | 11 | 2019-06-22T00:58:03.000Z | 2019-07-27T14:59:21.000Z | EdX/GTx: CSE6040x: FA18 - Computing for Data Analysis/Module 1: Representing, transforming and visualizing data/Topic 6 + Notebook 6 (OPTIONAL): Mining the web/part6.ipynb | helpthx/Path_through_Data_Science_2019 | aa22333eae970506f2ce184551c55565b0be89fb | [
"MIT"
] | 1 | 2020-12-03T21:10:43.000Z | 2020-12-03T21:10:43.000Z | 30.274827 | 440 | 0.569075 | [
[
[
"# Part 0: Mining the web\n\nPerhaps the richest source of openly available data today is [the Web](http://www.computerhistory.org/revolution/networking/19/314)! In this lab, you'll explore some of the basic programming tools you need to scrape web data.\n\n> **Note.** The Vocareum platform runs in a cloud-based environment that limits what websites a program can connect to directly. Therefore, some (or possibly all) of the code below will **not** work. Therefore, we are making this notebook **optional** and are providing solutions inline.\n>\n> Even if you are using a home or local installation of Jupyter, you may encounter problems if you attempt to access a site too many times or too rapidly. That can happen if your internet service provider (ISP) or the target website detect your accesses as \"unusual\" and reject them. It's easy to imagine accidentally writing an infinite loop that tries to access a page and being seen from the other side as a malicious program. :)",
"_____no_output_____"
],
[
"## The Requests module\n\nPython's [Requests module](http://requests.readthedocs.io/en/latest/user/quickstart/) to download a web page.\n\nFor instance, here is a code fragment to download the [Georgia Tech](http://www.gatech.edu) home page and print the first 250 characters. You might also want to [view the source](http://www.computerhope.com/issues/ch000746.htm) of Georgia Tech's home page to get a nicely formatted view, and compare its output to what you see above.",
"_____no_output_____"
]
],
[
[
"import requests\n\nresponse = requests.get('https://www.gatech.edu/')\nwebpage = response.text # or response.content for raw bytes\n\nprint(webpage[0:250]) # Prints the first hundred characters only",
"<!DOCTYPE html>\n<html lang=\"en\" dir=\"ltr\" \n xmlns:content=\"http://purl.org/rss/1.0/modules/content/\"\n xmlns:dc=\"http://purl.org/dc/terms/\"\n xmlns:foaf=\"http://xmlns.com/foaf/0.1/\"\n xmlns:og=\"http://ogp.me/ns#\"\n xmlns:rdfs=\"http://www.w3.org/2000\n"
]
],
[
[
"**Exercise 1.** Given the contents of the GT home page as above, write a function that returns a list of links (URLs) of the \"top stories\" on the page.\n\nFor instance, on Friday, September 9, 2016, here was the front page:\n\n\n\nThe top stories cycle through in the large image placeholder shown above. We want your function to return the list of URLs behind each of the \"Full Story\" links, highlighted in red. If no URLs can be found, the function should return an empty list.",
"_____no_output_____"
]
],
[
[
"import re # Maybe you want to use a regular expression?\n\ndef get_gt_top_stories(webpage_text):\n \"\"\"Given the HTML text for the GT front page, returns a list\n of the URLs of the top stories or an empty list if none are\n found.\n \"\"\"\n pattern = '''<a class=\"slide-link\" href=\"(?P<url>[^\"]+)\"'''\n return re.findall(pattern, webpage_text)",
"_____no_output_____"
],
[
"top_stories = get_gt_top_stories(webpage)\nprint(\"Links to GT's top stories:\", top_stories)",
"Links to GT's top stories: ['https://www.news.gatech.edu/features/age-empowerment-meet-casey-aultman', 'https://news.gatech.edu/features/first-tech-governors-inauguration-mccamish', 'http://www.news.gatech.edu/features/you-should-come-georgia-tech']\n"
]
],
[
[
"## A more complex example\n\nGo to [Yelp!](http://www.yelp.com) and look up `ramen` in `Atlanta, GA`. Take note of the URL:\n\n",
"_____no_output_____"
],
[
"This URL encodes what is known as an _HTTP \"get\"_ method (or request). It basically means a URL with two parts: a _command_ followed by one or more _arguments_. In this case, the command is everything up to and including the word `search`; the arguments are the rest, where individual arguments are separated by the `&` or `#`.\n\n> \"HTTP\" stands for \"HyperText Transport Protocol,\" which is a standardized set of communication protocols that allow _web clients_, like your web browser or your Python program, to communicate with _web servers_.\n\nIn this next example, let's see how to build a \"get request\" with the `requests` module. It's pretty easy!",
"_____no_output_____"
]
],
[
[
"url_command = 'https://yelp.com/search'\nurl_args = {'find_desc': \"ramen\",\n 'find_loc': \"atlanta, ga\"}\nresponse = requests.get (url_command, params=url_args, timeout=60)\n\nprint (\"==> Downloading from: '%s'\" % response.url) # confirm URL\nprint (\"\\n==> Excerpt from this URL:\\n\\n%s\\n\" % response.text[0:100])",
"==> Downloading from: 'https://www.yelp.com/search?find_desc=ramen&find_loc=atlanta%2C+ga'\n\n==> Excerpt from this URL:\n\n<!DOCTYPE HTML>\n\n<!--[if lt IE 7 ]> <html xmlns:fb=\"http://www.facebook.com/2008/fbml\" class=\"ie6 ie\n\n"
]
],
[
[
"**Exercise 2.** Given a search topic, location, and a rank $k$, return the name of the $k$-th item of a Yelp! search. If there is no $k$-th item, return `None`.\n\n> The demo query above only gives you a website with the top 10 items, meaning you could only use it for $k \\leq 10$. Figure out how to modify it to solve the problem when $k > 10$.",
"_____no_output_____"
]
],
[
[
"def find_yelp_item (topic, location, k):\n \"\"\"Returns the k-th suggested item from Yelp! in Atlanta for the given topic.\"\"\"\n import re\n if k < 1: return None\n \n # Download page\n url_command = 'http://yelp.com/search'\n url_args = {'find_desc': topic,\n 'find_loc': location,\n 'start': k-1\n }\n \n response = requests.get (url_command, params=url_args)\n if not response: return None\n \n # Split page into lines\n lines = response.text.split ('\\n')\n \n # Look for the line containing the name of the k-th item\n item_pattern = re.compile ('<span class=\"indexed-biz-name\">{}\\..*<span >(?P<item_name>.*)</span></a>'.format (k))\n for l in lines:\n item_match = item_pattern.search (l)\n if item_match:\n return item_match.group ('item_name')\n\n # No matches, evidently\n return None",
"_____no_output_____"
],
[
"assert find_yelp_item('fried chicken', 'Atlanta, GA', -1) is None # Tests an invalid value for 'k'",
"_____no_output_____"
]
],
[
[
"> Search queries on Yelp! don't always return the same answers, since the site is always changing! Also, your results might not match a query you do via your web browser (_why not?_). As such, you should manually check your answers.",
"_____no_output_____"
]
],
[
[
"item = find_yelp_item ('fried chicken', 'Atlanta, GA', 1)\nprint (item)\n\n ",
"None\n"
],
[
"item = find_yelp_item ('fried chicken', 'Atlanta, GA', 5)\nprint (item)\n\n# The most likely answer on September 11, 2018:\n#assert item == 'Buttermilk Kitchen'",
"None\n"
],
[
"item = find_yelp_item('fried chicken', 'Atlanta, GA', 10)\nprint(item)\n\n# Most likely correct answer as of September 11, 2018:\n#assert item == 'Colonnade Restaurant'",
"None\n"
]
],
[
[
"One issue with the above exercises is that they treat HTML as a flat string, whereas the document is at least semi-structured. Moreover, web pages are such a common source of data today that you would expect better tools for processing them. Indeed, such tools exist! The next part of this assignment, Part 1, walks you through one such tool. So, head there when you are ready!",
"_____no_output_____"
]
]
] | [
"markdown",
"code",
"markdown",
"code",
"markdown",
"code",
"markdown",
"code",
"markdown",
"code",
"markdown"
] | [
[
"markdown",
"markdown"
],
[
"code"
],
[
"markdown"
],
[
"code",
"code"
],
[
"markdown",
"markdown"
],
[
"code"
],
[
"markdown"
],
[
"code",
"code"
],
[
"markdown"
],
[
"code",
"code",
"code"
],
[
"markdown"
]
] |
d08ffa8871d02faaaf7a0450742ae79b488634a2 | 10,874 | ipynb | Jupyter Notebook | battery-state-estimation/experiments/lg/lstm_soc_percentage_lg_negative_temp_500_steps_drive_cycle_test.ipynb | KeiLongW/battery-state-estimation | 97f843055da955710241fe38bf5328bd1b677f88 | [
"Apache-2.0"
] | 7 | 2021-06-01T13:37:47.000Z | 2022-02-15T05:16:01.000Z | battery-state-estimation/experiments/lg/lstm_soc_percentage_lg_negative_temp_500_steps_drive_cycle_test.ipynb | abhignya2110/battery-state-estimation | a0584000f2f19e7004054e822904eb98e0333780 | [
"Apache-2.0"
] | 1 | 2021-08-11T01:10:53.000Z | 2021-08-12T09:24:42.000Z | battery-state-estimation/experiments/lg/lstm_soc_percentage_lg_negative_temp_500_steps_drive_cycle_test.ipynb | abhignya2110/battery-state-estimation | a0584000f2f19e7004054e822904eb98e0333780 | [
"Apache-2.0"
] | 1 | 2021-06-26T14:55:07.000Z | 2021-06-26T14:55:07.000Z | 27.529114 | 135 | 0.533934 | [
[
[
"# Main notebook for battery state estimation",
"_____no_output_____"
]
],
[
[
"import numpy as np\nimport pandas as pd\nimport scipy.io\nimport math\nimport os\nimport ntpath\nimport sys\nimport logging\nimport time\nimport sys\n\nfrom importlib import reload\nimport plotly.graph_objects as go\n\nimport tensorflow as tf\nfrom tensorflow import keras\nfrom tensorflow.keras import layers\n\nfrom keras.models import Sequential\nfrom keras.layers.core import Dense, Dropout, Activation\nfrom keras.optimizers import SGD, Adam\nfrom keras.utils import np_utils\nfrom keras.layers import LSTM, Embedding, RepeatVector, TimeDistributed, Masking\nfrom keras.callbacks import EarlyStopping, ModelCheckpoint, LambdaCallback\n\n\nIS_COLAB = False\n\nif IS_COLAB:\n from google.colab import drive\n drive.mount('/content/drive')\n data_path = \"/content/drive/My Drive/battery-state-estimation/battery-state-estimation/\"\nelse:\n data_path = \"../../\"\n\nsys.path.append(data_path)\nfrom data_processing.lg_dataset import LgData",
"_____no_output_____"
]
],
[
[
"### Config logging",
"_____no_output_____"
]
],
[
[
"reload(logging)\nlogging.basicConfig(format='%(asctime)s [%(levelname)s]: %(message)s', level=logging.DEBUG, datefmt='%Y/%m/%d %H:%M:%S')",
"_____no_output_____"
]
],
[
[
"# Load Data",
"_____no_output_____"
]
],
[
[
"train_names = [\n 'n10degC/601_Mixed1',\n 'n10degC/601_Mixed2',\n 'n10degC/604_Mixed3',\n 'n10degC/602_Mixed4',\n 'n10degC/602_Mixed5',\n 'n10degC/604_Mixed6',\n 'n10degC/604_Mixed7',\n 'n10degC/604_Mixed8',\n \n 'n20degC/610_Mixed1',\n 'n20degC/610_Mixed2',\n 'n20degC/611_Mixed3',\n 'n20degC/611_Mixed4',\n 'n20degC/611_Mixed5',\n 'n20degC/611_Mixed6',\n 'n20degC/611_Mixed7',\n 'n20degC/611_Mixed8' \n ]\ntest_names = [\n 'n10degC/596_UDDS',\n 'n10degC/601_US06',\n 'n10degC/596_LA92',\n \n 'n20degC/610_UDDS',\n 'n20degC/610_US06',\n 'n20degC/610_LA92',\n ]\n\nsteps = 500\n\nlg_data = LgData(data_path)\ncycles = lg_data.get_discharge_whole_cycle(train_names, test_names, output_capacity=False, scale_test=True)\ntrain_x, train_y, test_x, test_y = lg_data.get_discharge_multiple_step(cycles, steps)\n\ntrain_y = lg_data.keep_only_y_end(train_y, steps)\ntest_y = lg_data.keep_only_y_end(test_y, steps)",
"_____no_output_____"
]
],
[
[
"# Model training",
"_____no_output_____"
]
],
[
[
"EXPERIMENT = \"lstm_soc_percentage_lg_negative_temp_500_steps_drive_cycle_test\"\n\nexperiment_name = time.strftime(\"%Y-%m-%d-%H-%M-%S\") + '_' + EXPERIMENT\nprint(experiment_name)\n\nos.environ[\"CUDA_VISIBLE_DEVICES\"] = \"1\"\n \n# Model definition\nopt = tf.keras.optimizers.Adam(lr=0.00001)\n\nmodel = Sequential()\nmodel.add(LSTM(256, activation='selu',\n return_sequences=True,\n input_shape=(train_x.shape[1], train_x.shape[2])))\nmodel.add(LSTM(256, activation='selu', return_sequences=False))\nmodel.add(Dense(256, activation='selu'))\nmodel.add(Dense(128, activation='selu'))\nmodel.add(Dense(1, activation='linear'))\nmodel.summary()\n\nmodel.compile(optimizer=opt, loss='huber', metrics=['mse', 'mae', 'mape', tf.keras.metrics.RootMeanSquaredError(name='rmse')])\n\nes = EarlyStopping(monitor='val_loss', patience=50)\nmc = ModelCheckpoint(data_path + 'results/trained_model/%s_best.h5' % experiment_name, \n save_best_only=True, \n monitor='val_loss')",
"_____no_output_____"
],
[
"history = model.fit(train_x, train_y, \n epochs=1000, \n batch_size=32, \n verbose=2,\n validation_split=0.2,\n callbacks = [es, mc]\n )",
"_____no_output_____"
],
[
"model.save(data_path + 'results/trained_model/%s.h5' % experiment_name)\n\nhist_df = pd.DataFrame(history.history)\nhist_csv_file = data_path + 'results/trained_model/%s_history.csv' % experiment_name\nwith open(hist_csv_file, mode='w') as f:\n hist_df.to_csv(f)",
"_____no_output_____"
]
],
[
[
"### Testing",
"_____no_output_____"
]
],
[
[
"results = model.evaluate(test_x, test_y)\nprint(results)",
"_____no_output_____"
]
],
[
[
"# Data Visualization",
"_____no_output_____"
]
],
[
[
"# fig = go.Figure()\n# fig.add_trace(go.Scatter(y=history.history['loss'],\n# mode='lines', name='train'))\n# fig.add_trace(go.Scatter(y=history.history['val_loss'],\n# mode='lines', name='validation'))\n# fig.update_layout(title='Loss trend',\n# xaxis_title='epoch',\n# yaxis_title='loss')\n# fig.show()",
"_____no_output_____"
],
[
"# train_predictions = model.predict(train_x)",
"_____no_output_____"
],
[
"# cycle_num = 0\n# steps_num = 8000\n# step_index = np.arange(cycle_num*steps_num, (cycle_num+1)*steps_num)\n\n# fig = go.Figure()\n# fig.add_trace(go.Scatter(x=step_index, y=train_predictions.flatten()[cycle_num*steps_num:(cycle_num+1)*steps_num],\n# mode='lines', name='SoC predicted'))\n# fig.add_trace(go.Scatter(x=step_index, y=train_y.flatten()[cycle_num*steps_num:(cycle_num+1)*steps_num],\n# mode='lines', name='SoC actual'))\n# fig.update_layout(title='Results on training',\n# xaxis_title='Step',\n# yaxis_title='SoC percentage')\n# fig.show()",
"_____no_output_____"
],
[
"# test_predictions = model.predict(test_x)",
"_____no_output_____"
],
[
"# cycle_num = 0\n# steps_num = 8000\n# step_index = np.arange(cycle_num*steps_num, (cycle_num+1)*steps_num)\n\n# fig = go.Figure()\n# fig.add_trace(go.Scatter(x=step_index, y=test_predictions.flatten()[cycle_num*steps_num:(cycle_num+1)*steps_num],\n# mode='lines', name='SoC predicted'))\n# fig.add_trace(go.Scatter(x=step_index, y=test_y.flatten()[cycle_num*steps_num:(cycle_num+1)*steps_num],\n# mode='lines', name='SoC actual'))\n# fig.update_layout(title='Results on testing',\n# xaxis_title='Step',\n# yaxis_title='SoC percentage')\n# fig.show()",
"_____no_output_____"
]
]
] | [
"markdown",
"code",
"markdown",
"code",
"markdown",
"code",
"markdown",
"code",
"markdown",
"code",
"markdown",
"code"
] | [
[
"markdown"
],
[
"code"
],
[
"markdown"
],
[
"code"
],
[
"markdown"
],
[
"code"
],
[
"markdown"
],
[
"code",
"code",
"code"
],
[
"markdown"
],
[
"code"
],
[
"markdown"
],
[
"code",
"code",
"code",
"code",
"code"
]
] |
d0901557ba047948c046a0cd998c97fd7cd97f8c | 566,806 | ipynb | Jupyter Notebook | Revisions/Compare New Runs.ipynb | SalishSeaCast/storm-surge | adf7749e5da55a2261e56d230c52d979d94bba9d | [
"Apache-2.0"
] | null | null | null | Revisions/Compare New Runs.ipynb | SalishSeaCast/storm-surge | adf7749e5da55a2261e56d230c52d979d94bba9d | [
"Apache-2.0"
] | null | null | null | Revisions/Compare New Runs.ipynb | SalishSeaCast/storm-surge | adf7749e5da55a2261e56d230c52d979d94bba9d | [
"Apache-2.0"
] | null | null | null | 1,409.965174 | 264,319 | 0.941057 | [
[
[
"empty"
]
]
] | [
"empty"
] | [
[
"empty"
]
] |
d0901af3fde9db7929775164998c115986b0db26 | 563,203 | ipynb | Jupyter Notebook | examples/NealHumphrey/testing_yb.ipynb | ZJPoh/yellowbrick | 21c6b7162fd281aae4e037b4dad26d4f9cf62fb3 | [
"Apache-2.0"
] | 20 | 2018-03-24T02:29:20.000Z | 2022-03-03T05:01:40.000Z | examples/NealHumphrey/testing_yb.ipynb | ZJPoh/yellowbrick | 21c6b7162fd281aae4e037b4dad26d4f9cf62fb3 | [
"Apache-2.0"
] | 4 | 2018-03-20T12:01:17.000Z | 2019-04-07T16:02:19.000Z | examples/NealHumphrey/testing_yb.ipynb | ZJPoh/yellowbrick | 21c6b7162fd281aae4e037b4dad26d4f9cf62fb3 | [
"Apache-2.0"
] | 5 | 2018-03-17T08:18:57.000Z | 2019-11-15T02:20:20.000Z | 1,291.75 | 366,394 | 0.950947 | [
[
[
"%matplotlib inline\n\n\n\"\"\"\nThe data set in this example represents 1059 songs from various countries obtained \nfrom the UCI Machine Learning library. Various features of the audio tracks have been \nextracted, and each track has been tagged with the latitude and longitude of the capital\ncity of its country of origin. \n\nWe'll treat this as a classification problem, and attempt to train a model to predict \nthe country of origin of each model. \n\nData source did not specifify what the audio features specifically are, just\n \"In the 'default_features_1059_tracks.txt' file, the first 68 columns are audio \n features of the track, and the last two columns are the origin of the music, \n represented by latitude and longitude. \n\n In the 'default_plus_chromatic_features_1059_tracks.txt' file, the first 116 \n columns are audio features of the track, and the last two columns are the \n origin of the music.\"\n\"\"\"\n\nimport numpy as np\nimport pandas as pd\nimport sklearn\nfrom sklearn.preprocessing import LabelEncoder\nfrom sklearn.utils.multiclass import unique_labels\nimport sys\n\n#First get the data. The UCI ML Library distributes it as a zipped file;\n#download the data and extract the two provided files to the 'data' folder before continuing\nmusic_df = pd.read_csv('data\\default_plus_chromatic_features_1059_tracks.txt', header=None)\nmusic = music_df.as_matrix()\n\n\n#Our features are all but the last two columns\nX = music[:,0:-2]\n\n#Since feature names were not given, we'll just assign strings with an incrementing integer\nnames = np.linspace(start=1, stop=116, num=116, dtype='int').tolist()\nfor idx, name in enumerate(names):\n names[idx] = \"Feature \" + str(name)\n\n\n#The source data said that each song as tied to the capital city of it's origin country via a lat/lon pair. \n#Let's treat this as a multi-class classification problem. \n#Rather than reverse-geocoding, we'll just make a string out of the unique lat/lon pairs\nlats = [\"%.2f\" % lat for lat in music_df[116]]\nlons = [\"%.2f\" % lon for lon in music_df[117]]\nsong_latlons = []\nfor index, value in enumerate(lats):\n city_id = lats[index] + \",\" + lons[index]\n song_latlons.append(city_id)\n\nunique_latlons = unique_labels(song_latlons)\ncity_options = ['A','B','C','D','E','F','G','H','I','J','K','L','M','N','O','P','Q','R','S','T','U','V','W','X','Y','Z','AA','AB','AC','AD','AE','AF','AG']\ncity_name_map = {}\nfor idx,latlon in enumerate(unique_latlons):\n city_name_map[latlon] = city_options[idx]\n\nylist = []\nfor latlon in song_latlons:\n ylist.append(city_name_map[latlon])\ny = np.array(ylist)",
"_____no_output_____"
],
[
"\n",
"_____no_output_____"
],
[
"#We want yellowbrick to import from this repository, and assume this notebook is in repofolder/examples/subfolder/\nsys.path.append(\"../../\")\nimport yellowbrick as yb\nfrom yellowbrick.features.rankd import Rank2D \nfrom yellowbrick.features.radviz import RadViz \nfrom yellowbrick.features.pcoords import ParallelCoordinates ",
"_____no_output_____"
],
[
"#See how well correlated the features are\nvisualizer = Rank2D(features = names, algorithm = 'pearson')\nvisualizer.fit(X, y)\nvisualizer.transform(X)\nvisualizer.poof()",
"_____no_output_____"
],
[
"from sklearn import metrics\nfrom sklearn.model_selection import KFold\nfrom sklearn.model_selection import train_test_split\nfrom sklearn.svm import SVC\nfrom sklearn.neighbors import KNeighborsClassifier\nfrom sklearn.ensemble import RandomForestClassifier\nfrom sklearn.naive_bayes import GaussianNB\nfrom sklearn.linear_model import LogisticRegression\n\nimport seaborn as sns\nimport matplotlib.pyplot as plt\nfrom sklearn.metrics import confusion_matrix\nfrom yellowbrick.classifier import ClassificationReport\n",
"_____no_output_____"
],
[
"def train_and_classification_report(model):\n X_train, X_test, y_train, y_test = train_test_split(X,y, test_size =0.2, random_state=11)\n \n model.fit(X_train, y_train)\n y_predict = model.predict(X_test)\n\n print(\"prec: {}\".format(metrics.precision_score(y_true = y_test, y_pred = y_predict, average=\"weighted\")))\n print(\"rec: {}\".format(metrics.recall_score(y_true= y_test, y_pred = y_predict, average = \"weighted\")))\n\n cr_viz = ClassificationReport(model) #,classes=city_options\n cr_viz.fit(X_train, y_train)\n cr_viz.score(X_test, y_test)\n cr_viz.poof()\n \n",
"_____no_output_____"
],
[
"#Adding the reloading functionality so we can edit the source code and see results here. \nimport importlib\nimportlib.reload(yb.classifier)\nfrom yellowbrick.classifier import ClassificationReport\n\n#This produces an IndexError: list index out of range. \ntrain_and_classification_report(LogisticRegression())",
"prec: 0.430726301383904\nrec: 0.4056603773584906\n"
],
[
"#This demonstrates a version of the Seaborn confusion matrix heatmap we could replicate (and improve on). \ndef train_and_confusion_matrix(model):\n X_train, X_test, y_train, y_test = train_test_split(X,y, test_size =0.2, random_state=11)\n \n model.fit(X_train, y_train)\n y_predict = model.predict(X_test)\n\n print(\"prec: {}\".format(metrics.precision_score(y_true = y_test, y_pred = y_predict, average=\"weighted\")))\n print(\"rec: {}\".format(metrics.recall_score(y_true= y_test, y_pred = y_predict, average = \"weighted\")))\n\n c_matrix = confusion_matrix(y_true = y_test, y_pred = y_predict)\n \n sns.heatmap(c_matrix, square=True, annot=True, cbar=False, xticklabels=city_options, yticklabels = city_options)\n plt.xlabel('predicted value')\n plt.ylabel('true value')",
"_____no_output_____"
],
[
"train_and_confusion_matrix(LogisticRegression())",
"prec: 0.430726301383904\nrec: 0.4056603773584906\n"
],
[
"def train_and_class_balance(model):\n X_train, X_test, y_train, y_test = train_test_split(X,y, test_size =0.2, random_state=11)\n \n class_balance = yb.classifier.ClassBalance(model, classes=city_options)\n class_balance.fit(X_train, y_train)\n class_balance.score(X_test, y_test)\n class_balance.poof()",
"_____no_output_____"
],
[
"train_and_class_balance(LogisticRegression())",
"C:\\Users\\humph\\Anaconda3\\lib\\site-packages\\sklearn\\metrics\\classification.py:1115: UndefinedMetricWarning: Recall and F-score are ill-defined and being set to 0.0 in labels with no true samples.\n 'recall', 'true', average, warn_for)\n"
],
[
"\n",
"_____no_output_____"
]
]
] | [
"code"
] | [
[
"code",
"code",
"code",
"code",
"code",
"code",
"code",
"code",
"code",
"code",
"code",
"code"
]
] |
d090236a5a25a8f2191a0f12a7356eba1250519c | 58,620 | ipynb | Jupyter Notebook | Starter_Code/regression_analysis.ipynb | focraniv/A-Yen-for-the-Future | 5d178c9f5aee47abcc2e314fd9d29ecd1574bec8 | [
"ADSL"
] | null | null | null | Starter_Code/regression_analysis.ipynb | focraniv/A-Yen-for-the-Future | 5d178c9f5aee47abcc2e314fd9d29ecd1574bec8 | [
"ADSL"
] | null | null | null | Starter_Code/regression_analysis.ipynb | focraniv/A-Yen-for-the-Future | 5d178c9f5aee47abcc2e314fd9d29ecd1574bec8 | [
"ADSL"
] | null | null | null | 60.495356 | 29,360 | 0.679853 | [
[
[
"import numpy as np\nimport pandas as pd\nfrom pathlib import Path\n%matplotlib inline",
"_____no_output_____"
]
],
[
[
"# Regression Analysis: Seasonal Effects with Sklearn Linear Regression\nIn this notebook, you will build a SKLearn linear regression model to predict Yen futures (\"settle\") returns with *lagged* Yen futures returns. ",
"_____no_output_____"
]
],
[
[
"# Futures contract on the Yen-dollar exchange rate:\n# This is the continuous chain of the futures contracts that are 1 month to expiration\nyen_futures = pd.read_csv(\n Path(\"yen.csv\"), index_col=\"Date\", infer_datetime_format=True, parse_dates=True\n)\nyen_futures.head()",
"_____no_output_____"
],
[
"# Trim the dataset to begin on January 1st, 1990\nyen_futures = yen_futures.loc[\"1990-01-01\":, :]\nyen_futures.head()",
"_____no_output_____"
]
],
[
[
"# Data Preparation",
"_____no_output_____"
],
[
"### Returns",
"_____no_output_____"
]
],
[
[
"# Create a series using \"Settle\" price percentage returns, drop any nan\"s, and check the results:\n# (Make sure to multiply the pct_change() results by 100)\n# In this case, you may have to replace inf, -inf values with np.nan\"s\nyen_futures['Return'] = (yen_futures[[\"Settle\"]].pct_change() * 100)\nreturns = yen_futures.replace(-np.inf, np.nan).dropna()\nreturns.tail()",
"_____no_output_____"
]
],
[
[
"### Lagged Returns ",
"_____no_output_____"
]
],
[
[
"# Create a lagged return using the shift function\nyen_futures['Lagged_Return'] = yen_futures['Return'].shift()\nyen_futures = yen_futures.dropna()\nyen_futures.tail()",
"_____no_output_____"
]
],
[
[
"### Train Test Split",
"_____no_output_____"
]
],
[
[
"# Create a train/test split for the data using 2018-2019 for testing and the rest for training\ntrain = yen_futures[:'2017']\ntest = yen_futures['2018':]",
"_____no_output_____"
],
[
"# Create four dataframes:\n# X_train (training set using just the independent variables), X_test (test set of of just the independent variables)\n# Y_train (training set using just the \"y\" variable, i.e., \"Futures Return\"), Y_test (test set of just the \"y\" variable):\nX_train = train[\"Lagged_Return\"].to_frame()\nX_test = test[\"Lagged_Return\"].to_frame()\ny_train = train[\"Return\"]\ny_test = test[\"Return\"]",
"_____no_output_____"
],
[
"X_train",
"_____no_output_____"
]
],
[
[
"# Linear Regression Model",
"_____no_output_____"
]
],
[
[
"# Create a Linear Regression model and fit it to the training data\nfrom sklearn.linear_model import LinearRegression\n\n# Fit a SKLearn linear regression using just the training set (X_train, Y_train):\nmodel = LinearRegression()\nmodel.fit(X_train, y_train)",
"_____no_output_____"
]
],
[
[
"# Make predictions using the Testing Data\n\nNote: We want to evaluate the model using data that it has never seen before, in this case: X_test.",
"_____no_output_____"
]
],
[
[
"# Make a prediction of \"y\" values using just the test dataset\npredictions = model.predict(X_test)",
"_____no_output_____"
],
[
"# Assemble actual y data (Y_test) with predicted y data (from just above) into two columns in a dataframe:\nResults = y_test.to_frame()\nResults[\"Predicted Return\"] = predictions",
"_____no_output_____"
],
[
"# Plot the first 20 predictions vs the true values\nprediction_plot = Results[:20].plot(subplots=True)",
"_____no_output_____"
]
],
[
[
"# Out-of-Sample Performance\n\nEvaluate the model using \"out-of-sample\" data (X_test and y_test)",
"_____no_output_____"
]
],
[
[
"from sklearn.metrics import mean_squared_error\n# Calculate the mean_squared_error (MSE) on actual versus predicted test \"y\" \nmse = mean_squared_error(Results[\"Return\"],Results[\"Predicted Return\"])\n# Using that mean-squared-error, calculate the root-mean-squared error (RMSE):\nrmse = np.sqrt(mse)\nprint(f\"Out-of-Sample Root Mean Squared Error (RMSE): {rmse}\")",
"Out-of-Sample Root Mean Squared Error (RMSE): 0.41545437184712763\n"
]
],
[
[
"# In-Sample Performance\n\nEvaluate the model using in-sample data (X_train and y_train)",
"_____no_output_____"
]
],
[
[
"# Construct a dataframe using just the \"y\" training data:\nin_sample_results = y_train.to_frame()\n\n# Add a column of \"in-sample\" predictions to that dataframe: \nin_sample_results[\"In-sample Predictions\"] = model.predict(X_train)\n\n# Calculate in-sample mean_squared_error (for comparison to out-of-sample)\nin_sample_mse = mean_squared_error(in_sample_results[\"Return\"], in_sample_results[\"In-sample Predictions\"])\n\n# Calculate in-sample root mean_squared_error (for comparison to out-of-sample)\nin_sample_rmse = np.sqrt(in_sample_mse)\nprint(f\"In-sample Root Mean Squared Error (RMSE): {in_sample_rmse}\")",
"In-sample Root Mean Squared Error (RMSE): 0.5962037920929946\n"
]
],
[
[
"# Conclusions",
"_____no_output_____"
],
[
"YOUR CONCLUSIONS HERE!",
"_____no_output_____"
],
[
"We have a root mean square error of 0.415% for the out-of-sample data and 0.56587% for in-sample data. The model performs better with data that it has not worked with before.",
"_____no_output_____"
]
]
] | [
"code",
"markdown",
"code",
"markdown",
"code",
"markdown",
"code",
"markdown",
"code",
"markdown",
"code",
"markdown",
"code",
"markdown",
"code",
"markdown",
"code",
"markdown"
] | [
[
"code"
],
[
"markdown"
],
[
"code",
"code"
],
[
"markdown",
"markdown"
],
[
"code"
],
[
"markdown"
],
[
"code"
],
[
"markdown"
],
[
"code",
"code",
"code"
],
[
"markdown"
],
[
"code"
],
[
"markdown"
],
[
"code",
"code",
"code"
],
[
"markdown"
],
[
"code"
],
[
"markdown"
],
[
"code"
],
[
"markdown",
"markdown",
"markdown"
]
] |
d09049639c4ce62ab28d27bc853e861e1123ecd7 | 10,392 | ipynb | Jupyter Notebook | KUWO_ALBUM.ipynb | TLYu0419/KUWO | 4c4f7923f28039c4020e6bac6e3218326d68a31b | [
"MIT"
] | null | null | null | KUWO_ALBUM.ipynb | TLYu0419/KUWO | 4c4f7923f28039c4020e6bac6e3218326d68a31b | [
"MIT"
] | null | null | null | KUWO_ALBUM.ipynb | TLYu0419/KUWO | 4c4f7923f28039c4020e6bac6e3218326d68a31b | [
"MIT"
] | null | null | null | 23.726027 | 222 | 0.502887 | [
[
[
"# 酷我音樂\n- 下載酷我音樂平台上電台的「專輯」音檔",
"_____no_output_____"
],
[
"# 載入套件",
"_____no_output_____"
]
],
[
[
"import re\nimport os\nimport time\nimport requests\nfrom bs4 import BeautifulSoup",
"_____no_output_____"
]
],
[
[
"# 設定爬蟲參數",
"_____no_output_____"
]
],
[
[
"headers = {'User-Agent': 'Mozilla/5.0 (Windows NT 10.0; Win64; x64) AppleWebKit/537.36 (KHTML, like Gecko) Chrome/88.0.4324.150 Safari/537.36 Edg/88.0.705.63',\n 'Cookie': '_ga=GA1.2.841063431.1603504850; Hm_lvt_cdb524f42f0ce19b169a8071123a4797=1611556993; _gid=GA1.2.997533225.1613194048; Hm_lpvt_cdb524f42f0ce19b169a8071123a4797=1613194071; kw_token=UCMBLA99FF',\n 'referer': 'http://www.kuwo.cn/',\n 'If-Range': '6023dfa9-8d7179',\n 'Sec-Fetch-Dest': 'video',\n 'Sec-Fetch-Mode': 'no-cors',\n 'Sec-Fetch-Site': 'cross-site',\n 'csrf': 'UCMBLA99FF'}",
"_____no_output_____"
]
],
[
[
"# 爬取資料",
"_____no_output_____"
],
[
"## 蒐集連結清單",
"_____no_output_____"
]
],
[
[
"page = 1\nlinks = []\n\nwhile True:\n # 生成網址\n url = 'http://www.kuwo.cn/api/www/album/albumInfo?albumId=547562&pn={}&rn=30'.format(page)\n \n # 請求資料\n resp = requests.get(url, headers=headers)\n time.sleep(0.5)\n try:\n musicList = [i['rid'] for i in resp.json()['data']['musicList']]\n\n # 保存資訊\n links += musicList\n\n # 輸出資料擷取進度\n print(page, ': ', len(list(set(links))))\n\n # 判斷是否跳出迴圈\n page += 1\n if len(musicList) < 30:\n links = list(set(links))\n print('There are totally {} links!'.format(len(links)))\n break\n except:\n print('status_code: ', resp.status_code, ', Retry')",
"1 : 30\n2 : 60\nstatus_code: 504 , Retry\nstatus_code: 504 , Retry\nstatus_code: 504 , Retry\n3 : 90\n4 : 120\nstatus_code: 504 , Retry\n5 : 150\n6 : 180\n7 : 210\n8 : 240\n9 : 270\n10 : 300\n11 : 330\n12 : 360\n13 : 390\n14 : 420\nstatus_code: 504 , Retry\n15 : 450\n16 : 480\n17 : 510\n18 : 540\n19 : 570\nstatus_code: 504 , Retry\n20 : 600\n21 : 630\n22 : 660\n23 : 690\n24 : 720\nstatus_code: 504 , Retry\n25 : 750\n26 : 780\n27 : 810\n28 : 840\nstatus_code: 504 , Retry\n29 : 870\n30 : 900\n31 : 915\nThere are totally 915 links!\n"
]
],
[
[
"# 下載連結音檔",
"_____no_output_____"
]
],
[
[
"# os.mkdir('./musics')",
"_____no_output_____"
],
[
"# 已下載的音樂清單\ndownload_list = [int(i.split('_',-1)[0]) for i in os.listdir('./musics')]\nlen(download_list)",
"_____no_output_____"
],
[
"# 排除已下載的音樂\nlinks = [link for link in links if link not in download_list]\nlen(links)",
"_____no_output_____"
],
[
"for link in links:\n # 取音檔摘要\n url = 'http://www.kuwo.cn/play_detail/{}'.format(link)\n resp = requests.get(url, headers=headers)\n soup = BeautifulSoup(resp.text)\n\n time.sleep(3)\n music_name = soup.find('title').text\n music_name = re.sub(r'/|', '', music_name)\n music_uploadtime = soup.find('span', {'class':'time'}).text\n \n # 取音檔連結\n music_link = 'http://www.kuwo.cn/url?format=mp3&rid={}&response=url&type=convert_url3&br=128kmp3'.format(link)\n try:\n music_link = requests.get(music_link).json()['url']\n except:\n time.sleep(1)\n music_link = requests.get(music_link).json()['url']\n # 下載音檔\n music_content = requests.get(url=music_link).content\n with open('./musics/{}.mp3'.format(str(link) + '_' + music_name), 'wb') as f:\n f.write(music_content)\n print('Succed: ', link, music_name)",
"Succed: 7090145 请你不要告诉我,你最爱的人不是我(一个人听)_蕊希Erin_单曲在线试听_酷我音乐\nSucced: 7090146 为什么,你就不喜欢我了(一个人听)_蕊希Erin_单曲在线试听_酷我音乐\nSucced: 76240867 ”我不是傻,只是懒得计较”_蕊希Erin_单曲在线试听_酷我音乐\nSucced: 83795941 你有多久没对另一半说我爱你了?_蕊希Erin_单曲在线试听_酷我音乐\nSucced: 57690089 会有人拿着戒指对你笑,说余生请多指教。 (节目)_蕊希Erin_单曲在线试听_酷我音乐\nSucced: 57151470 ” 我月薪三万,拒绝给女友买两万的包。 ”_蕊希__单曲在线试听_酷我音乐\nSucced: 148090871 总有人在偷偷羡慕你_蕊希Erin_单曲在线试听_酷我音乐\nSucced: 41351166 “我们再也不会互道晚安”_蕊希Erin_单曲在线试听_酷我音乐\n"
]
],
[
[
"# 異常狀況排除\n- 通常是檔案名稱有非法字元,或者request的速度過快被擋~",
"_____no_output_____"
]
],
[
[
"soup.find('title').text",
"_____no_output_____"
],
[
"requests.get(music_link).json()['url']",
"_____no_output_____"
],
[
"music_name = '66823804_蕊希专访 陈乔恩:爱自己,是终身浪漫的开始_蕊希Erin_单曲在线试听_酷我音乐.mp3'",
"_____no_output_____"
],
[
"with open('./musics/{}.mp3'.format(str(link) + '_' + music_name), 'wb') as f:\n f.write(music_content)",
"_____no_output_____"
]
]
] | [
"markdown",
"code",
"markdown",
"code",
"markdown",
"code",
"markdown",
"code",
"markdown",
"code"
] | [
[
"markdown",
"markdown"
],
[
"code"
],
[
"markdown"
],
[
"code"
],
[
"markdown",
"markdown"
],
[
"code"
],
[
"markdown"
],
[
"code",
"code",
"code",
"code"
],
[
"markdown"
],
[
"code",
"code",
"code",
"code"
]
] |
d09050ee7f89492a610450057aa713cd41e8cce7 | 687,499 | ipynb | Jupyter Notebook | reddit/visualize.ipynb | ajmendez/explore | 367e96fca29fef8a9afa490798b922fa0fc303e1 | [
"MIT"
] | null | null | null | reddit/visualize.ipynb | ajmendez/explore | 367e96fca29fef8a9afa490798b922fa0fc303e1 | [
"MIT"
] | null | null | null | reddit/visualize.ipynb | ajmendez/explore | 367e96fca29fef8a9afa490798b922fa0fc303e1 | [
"MIT"
] | null | null | null | 952.214681 | 155,218 | 0.938058 | [
[
[
"%matplotlib inline\nimport json\nimport pylab\nimport copy\nfrom pprint import pprint\nimport numpy as np\nfrom lxml import etree\nimport matplotlib.colors\nfrom pysurvey.plot import icolorbar, text, box\nfrom pysurvey.plot import setup_sns as setup\n\nimport seaborn as sns\nsns.set_style('white')",
"_____no_output_____"
],
[
"def make_cmap():\n # from brewer -- reorganized\n rgbs = (\n (152,78,163),\n (55,126,184),\n #(77,175,74),\n (69, 157, 66),\n (228,26,28),\n (255,127,0),\n )\n cdict = {}\n colors = ['red', 'green', 'blue']\n for i,rgb in enumerate(rgbs):\n for color,value in zip(colors, rgb):\n c = cdict.get(color, [])\n c.append((i*1.0/(len(rgbs)-1.0), value/256.0, value/256.0))\n cdict[color] = c\n # Darken Rainbow\n# def c(name):\n# return lambda x: np.clip(pylab.cm.datad['rainbow'][name](x),0,0.8)\n# cdict = dict(\n# red = c('red'),\n# green = c('green'),\n# blue = c('blue'),\n# )\n cmap = matplotlib.colors.LinearSegmentedColormap('my_colormap',cdict,512)\n return cmap\n\ndef grayify_cmap(cmap):\n colors = cmap(np.arange(cmap.N))\n RGB_weight = [0.299, 0.587, 0.114]\n luminance = np.sqrt(np.dot(colors[:, :3] ** 2, RGB_weight))\n colors[:, :3] = luminance[:, np.newaxis]\n return cmap.from_list(cmap.name + \"_grayscale\", colors, cmap.N)\n\ndef show_colormap(cmap):\n im = np.outer(np.ones(10), np.arange(100))\n fig, ax = pylab.subplots(2, figsize=(6, 1.5), subplot_kw=dict(xticks=[], yticks=[]))\n fig.subplots_adjust(hspace=0.1)\n ax[0].imshow(im, cmap=cmap)\n ax[1].imshow(im, cmap=grayify_cmap(cmap))\n \nshow_colormap(make_cmap())\n",
"_____no_output_____"
],
[
"with open('/Users/ajmendez/data/reddit/subreddit_ages.json', 'r') as f:\n subreddit_map = json.load(f)\nages = [v[0] for k,v in subreddit_map.iteritems()]\nnp.min(ages), np.max(ages)",
"_____no_output_____"
],
[
"# This was the original one, but lets update to the clustering version\ntree = etree.parse(\"/Users/ajmendez/data/reddit/subreddits.gexf\", base_url='http://www.gexf.net/1.2draft')\nns = {'graph': '{http://www.gexf.net/1.2draft}graph'}\ngraph = tree.getroot().find(ns['graph'])\n# tag = graph.findall('.*//*[@label=\"{}\"]'.format(subreddit))[0]\n# s = tag[1].attrib\n# p = tag[2].attrib\n# int(tag.attrib['id']),\n",
"_____no_output_____"
],
[
"# Programming subreddits compiled from:\n# https://www.reddit.com/user/krispykrackers/m/programming\n# https://www.reddit.com/comments/a6qgz/proggit_im_trying_to_compile_all_the_known\n\n\ncity_subreddits = ('orlando Quebec Colorado Calgary paris bayarea wisconsin france ottawa houston vancouver '\n 'newzealand Iowa sanantonio montreal ontario Miami mexico Atlanta Seattle sanfrancisco '\n 'toronto nothernireland boston canada LosAngeles philadelphia raleigh chicago sandiego '\n 'indianapolis Charleston VictoriaBC russia Winnipeg Cleveland Portland NewOrleans australia Maine StLouis pittsburgh HongKong longisland '\n 'Austin Portland Seattle Vancouver Boston Toronto SanFrancisco pittsburgh sandiego Chicago '\n 'twincitiessocial washingtondc denver philadelphia Montreal BayArea atlanta NYC melbourne houston '\n 'LosAngeles Dallas london '\n 'japan ireland nyc melbourne tampaDenver Taxans Dallas China sydney Denmark brisbane pakistan').split()\nprogramming_subreddits = (\n 'ada agi algorithms applescript Arc asm aspnet awk brainfuck cappuccino carlhprogramming clojure cobol '\n 'cocoa cocoadev code codeprojects coding CodingContests coldfusion common_lisp compsci computerscience coq '\n 'cplusplus cpp csbooks csharp css csshelp c_language c_programming dailyprogrammer delphi dependent_types '\n 'django django_class dotnet drupal d_language emacs encryption engineering erlang factor forth fortran fortress '\n 'fsharp functional functionallang gamedev genetic_algorithms git greasemonkey groovy haskell haskell_proposals haxe '\n 'HTML html5 Ioke iOSProgramming iphonedev j2ee java javahelp javascript jquery learnprogramming learnpython linux lisp '\n 'lua machinelearning macprogramming matlab mercurial modula3 netsec newlisp Oberon objectivec ocaml onlycode opengl '\n 'pascal perl PHP php programmer programming programminglanguages prolog Python python rails ruby rubyonrails scala '\n 'scheme smalltalk softwaredevelopment swift systems Tcl technology techsupport threads types udk ui_programming unity3d '\n 'vim visualbasic webdev web_design web_dev Wolfram wolframlanguage xna XOTcl').split()\n\n# cmap = make_cmap()\ncmap = pylab.cm.rainbow # for dark background\nagenorm = matplotlib.colors.Normalize(18, 30, clip=True)\ndtype = [\n ('id', np.int),\n ('subreddit', '|S64'),\n ('nunique', np.int),\n ('iscity', np.int),\n ('isprogramming', np.int),\n ('x', np.float),\n ('y', np.float),\n ('size', np.float),\n ('age', np.float),\n ('rgba', np.float, 4),\n]\ndata = np.zeros(len(subreddit_map), dtype=dtype)\nfor i, (subreddit, value) in enumerate(subreddit_map.iteritems()):\n try:\n tag = graph.findall('.*//*[@label=\"{}\"]'.format(subreddit))[0]\n except Exception as e:\n# print '!',\n# print subreddit, e\n continue\n s = tag[1].attrib\n p = tag[2].attrib\n age = value[0]\n nunique = value[-1]\n data[i] = (int(tag.attrib['id']),\n subreddit,\n nunique,\n (subreddit in city_subreddits),\n (subreddit in programming_subreddits),\n float(p['x']), \n float(p['y']),\n float(s['value']),\n age,\n pylab.cm.Spectral(agenorm(age)),\n )\n# print i, subreddit, age\n# etree.dump(tag)\n# if i > 10:\n# break",
"_____no_output_____"
]
],
[
[
"# Make cluster plot",
"_____no_output_____"
]
],
[
[
"_ = pylab.hist(data['nunique'][data['nunique']!= 0], 50)",
"_____no_output_____"
],
[
"def setup_clusters(width=1500, xoffset=0, yoffset=0, **params):\n kwargs = dict(xticks=False, yticks=False, grid=False, tickmarks=False)\n kwargs.update(params)\n ax = setup(xr=[-width+xoffset,width+xoffset], yr=[-width+yoffset,width+yoffset], **kwargs)\n pylab.xticks([])\n pylab.yticks([])\n return ax\n \n \ndef plot_cluster(data, isgood=None, vmin=18, vmax=32, cmap=None, maxsize=50, sizescale=1.0, **kwargs):\n if isgood is None: isgood = (np.ones(data.shape) == 1)\n if cmap is None: cmap=make_cmap()\n agenorm = matplotlib.colors.Normalize(vmin, vmax, clip=True)\n index = np.where(isgood & (data['x'] != 0) & (data['y'] != 0))[0]\n s = np.clip(np.sqrt(data['nunique'][index]), 3, maxsize)*2*sizescale\n sca = pylab.scatter(data['x'][index], data['y'][index], label='Age',\n s=s, c=data['age'][index], vmin=vmin, vmax=vmax, cmap=cmap, lw=0, **kwargs)\n return sca\n\ndef label_clusters(data, isgood=None, vmin=18, vmax=32, cmap=None, ax=None, sizescale=1.0):\n if isgood is None: isgood = (np.ones(data.shape) == 1)\n if cmap is None: cmap=make_cmap()\n if ax is None: ax = pylab.gca()\n \n agenorm = matplotlib.colors.Normalize(vmin, vmax, clip=True)\n xr,yr = pylab.xlim(), pylab.ylim()\n index = np.where(isgood & \n (data['x'] > xr[0]) & (data['x'] < xr[1]) & \n (data['y'] > yr[0]) & (data['y'] < yr[1]) & \n (data['x'] != 0) & (data['y'] != 0))[0]\n ii = np.argsort(data['nunique'][index])\n for x,y,label,age,s in data[index][['x','y','subreddit', 'age', 'nunique']][ii]:\n if len(label) == 0: continue\n color=cmap(agenorm(age))\n# s = np.clip(s, 4,12)*sizescale\n fs = np.clip(12*(s/200.0), 3, 12)*sizescale\n tmp = text(x,y,label, color=color,\n ha='left', va='bottom', fontsize=fs,\n clip_on=True, clip_path=ax.patch, outline=True)\n\n tmp.set_clip_path(ax.patch)\n\n\nsub_width = 400\nsub_xoffset = 70\n\nsetup_clusters(sub_width, sub_xoffset, figsize=(12,6), subplt=(1,2,1))\nsca = plot_cluster(data, cmap=pylab.cm.rainbow, vmin=18, vmax=32)\nicolorbar(sca, loc=2, borderpad=0.75, tickfmt='{:.0f}')\n\nsetup_clusters(sub_width, sub_xoffset, subplt=(1,2,2))\nsca = plot_cluster(data, cmap=make_cmap(), vmin=18, vmax=32)\nicolorbar(sca, loc=2, borderpad=0.75, tickfmt='{:.0f}')\n",
"_____no_output_____"
],
[
"main_width = 1500\nsub_width = 400\nsub_xoffset = 70\n\nsetup_clusters(main_width, figsize=(12,4), subplt=(1,3,1))\nbox([-sub_width+sub_xoffset,sub_width+sub_xoffset], [-sub_width,sub_width], lw=0, alpha=0.1)\nplot_cluster(data)\n\nsetup_clusters(sub_width, sub_xoffset, subplt=(1,3,2))\nsca = plot_cluster(data)\nicolorbar(sca, loc=2, borderpad=0.75, tickfmt='{:.0f}')\n\n\nsetup_clusters(sub_width, sub_xoffset, subplt=(1,3,3))\nplot_cluster(data)\nlabel_clusters(data, (data['nunique'] > 500))\n\n \npylab.tight_layout()\n# pylab.savefig('/Users/ajmendez/Desktop/subreddits.png', dpi=200)",
"_____no_output_____"
],
[
"sub_width = 600\nsub_xoffset = 20\nsub_yoffset = -50\nsetup_clusters(sub_width, sub_xoffset, sub_yoffset, figsize=(12,12), subplt=(2,2,1), title='Age < 21')\nplot_cluster(data, cmap=make_cmap(), alpha=0.1, maxsize=20)\nisage = (data['age'] < 21) & (data['nunique'] > 10)\nsca = plot_cluster(data, isage, sizescale=2.0)\nlabel_clusters(data, isage, sizescale=2.0)\n\nsetup_clusters(sub_width, sub_xoffset, sub_yoffset, subplt=(2,2,2), title='Age > 30')\nplot_cluster(data, cmap=make_cmap(), alpha=0.1, maxsize=20)\nisage = (data['age'] > 30) & (data['nunique'] > 10)\nsca = plot_cluster(data, isage, sizescale=2.0)\nlabel_clusters(data, isage, sizescale=2.0)\n\nsub_width = 60\nsub_xoffset = 430\nsub_yoffset = -330\nsetup_clusters(sub_width, sub_xoffset, sub_yoffset, subplt=(2,2,3), title='Sports Cluster')\nplot_cluster(data, cmap=pylab.cm.Greys, alpha=0.1, maxsize=20)\nisage = (data['nunique'] > 10)\nsca = plot_cluster(data, isage, sizescale=2.0)\nlabel_clusters(data, isage, sizescale=2.0)\n",
"_____no_output_____"
],
[
"sub_width = 70\nsub_xoffset = 1000\nsub_yoffset = 150\nsetup_clusters(sub_width, sub_xoffset, sub_yoffset, subplt=(2,2,4))\nplot_cluster(data, cmap=pylab.cm.Greys, alpha=0.1, maxsize=20)\nisage = (data['nunique'] > 5) & (data['age'] > 0)\nsca = plot_cluster(data, isage, sizescale=2.0)\nlabel_clusters(data, isage, sizescale=2.0)\nicolorbar(sca, loc=1)",
"_____no_output_____"
],
[
"sub_width = 1450\nsub_xoffset = 380\nsub_yoffset = 100\nsetup_clusters(sub_width, sub_xoffset, sub_yoffset, figsize=(12,12), title='Programming Subreddits')\nplot_cluster(data, alpha=0.1, maxsize=20)\nisage = (data['nunique'] > 10) & (data['age'] > 0) & (data['isprogramming'] ==1)\nsca = plot_cluster(data, isage, sizescale=2.0)\nicolorbar(sca)\nlabel_clusters(data, isage, sizescale=2.0)\nii = np.argsort(data[isage]['age'])\nfor subreddit, age in data[isage][ii][['subreddit', 'age']]:\n print '{:12s} {:5.1f}'.format(subreddit, age)",
"javahelp 18.4\nlearnpython 22.5\ncompsci 23.5\njava 23.6\ntechsupport 23.6\ngamedev 24.4\nPython 24.7\nengineering 25.0\nnetsec 25.7\ntechnology 26.6\nweb_design 26.9\nwebdev 27.0\nlinux 27.6\nprogramming 28.3\n"
],
[
"sub_width = 450\nsub_xoffset = -180\nsub_yoffset = 100\nsetup_clusters(sub_width, sub_xoffset, sub_yoffset, figsize=(12,12), title='Cities and Countries')\nplot_cluster(data, alpha=0.1, maxsize=20)\nisage = (data['nunique'] > 10) & (data['age'] > 0) & (data['iscity'] ==1)\nsca = plot_cluster(data, isage, sizescale=2.0)\nicolorbar(sca)\nlabel_clusters(data, isage, sizescale=2.0)",
"_____no_output_____"
],
[
"tmp = data[np.argsort(-data['age'])]\niscity = (tmp['nunique'] > 20) & (tmp['age'] > 10) & (tmp['iscity'] > 0)\nncity = len(np.where(iscity)[0])\ncmap = make_cmap()\n\nax = setup(figsize=(16,4), grid=False,\n title='Cities and Countries',\n ylabel='Age', yr=[0, 32],\n xr=[-0.2, ncity+0.2], xtickv=np.arange(ncity)+0.5,\n xticknames=['' for x in tmp['subreddit'][iscity]], \n xtickrotate=90)\nfor i, subreddit in enumerate(tmp['subreddit'][iscity]):\n pylab.text(i+0.6, 1, '/r/'+subreddit, \n color='w', fontsize=14, fontweight='bold',\n ha='center', va='bottom', rotation=90)\n \n# ax.set_xticklabels(tmp['subreddit'][iscity], rotation=90, ha='center')\n\npylab.bar(left=np.arange(ncity)+0.1, width=0.8,\n height=tmp['age'][iscity], lw=0, alpha=0.8,\n color=cmap(agenorm(tmp['age'][iscity])))\n",
"_____no_output_____"
]
],
[
[
"# Build data.json",
"_____no_output_____"
]
],
[
[
"vizit = json.load(open('/Users/ajmendez/data/reddit/vizit_data.json', 'r'))",
"_____no_output_____"
],
[
"ii = np.where(data['age'] > 0)\nageit = dict(nodes=[], edges=[])\nnode_ids = []\nfor node in vizit['nodes']:\n subreddit = node['label']\n \n i = np.where( (data['subreddit'] == subreddit) & (data['age'] > 0) )[0]\n if len(i) != 0:\n newnode = copy.copy(node)\n newnode['color'] = 'rgb({:0.0f}, {:0.0f}, {:0.0f})'.format(*data['rgba'][i][0][:-1]*256)\n newnode['size'] = 4.0*float(newnode['size'])\n newnode['age'] = float(data['age'][i])\n else:\n newnode = copy.copy(node)\n newnode['color'] = 'rgb({:0.0f}, {:0.0f}, {:0.0f})'.format(0,0,0)\n newnode['age'] = 0\n newnode['size'] = 0.5*float(newnode['size'])\n \n ageit['nodes'].append(newnode)\n node_ids.append(newnode['id'])\n\nfor edge in vizit['edges']:\n if (edge['source'] in node_ids) and (edge['target'] in node_ids):\n ageit['edges'].append(copy.copy(edge))\nprint 'Nodes: {:,d} Edges: {:,d}'.format(len(ageit['nodes']), len(ageit['edges']))",
"Nodes: 9,981 Edges: 142,881\n"
],
[
"data['age'][1]",
"_____no_output_____"
],
[
"pprint(vizit['nodes'][-2])\npprint(vizit['edges'][1])",
"{u'attributes': {u'Degree': u'331',\n u'Modularity Class': u'5',\n u'Subscribers': u'272565',\n u'Weighted Degree': u'13416.0'},\n u'color': u'rgb(116,162,142)',\n u'id': u'19576',\n u'label': u'food',\n u'size': 7.9187421798706055,\n u'x': 3209.85791015625,\n u'y': 328.22381591796875}\n{u'attributes': {u'weight': u'1.0'},\n u'id': u'89814',\n u'label': u'89814',\n u'source': u'31141',\n u'target': u'47969'}\n"
],
[
"json.dump(ageit, open('/Users/ajmendez/data/reddit/ageit_data.json', 'w'), indent=2)",
"_____no_output_____"
]
]
] | [
"code",
"markdown",
"code",
"markdown",
"code"
] | [
[
"code",
"code",
"code",
"code",
"code"
],
[
"markdown"
],
[
"code",
"code",
"code",
"code",
"code",
"code",
"code",
"code"
],
[
"markdown"
],
[
"code",
"code",
"code",
"code",
"code"
]
] |
d0905c50ab81f3f79e31f2dba80a7eb321317f82 | 19,018 | ipynb | Jupyter Notebook | Object Oriented Programming.ipynb | Ryan-Bui/DATA3401 | a8f4da1a29b36409b3424a82ff6422b1d85cd3ef | [
"MIT"
] | null | null | null | Object Oriented Programming.ipynb | Ryan-Bui/DATA3401 | a8f4da1a29b36409b3424a82ff6422b1d85cd3ef | [
"MIT"
] | null | null | null | Object Oriented Programming.ipynb | Ryan-Bui/DATA3401 | a8f4da1a29b36409b3424a82ff6422b1d85cd3ef | [
"MIT"
] | null | null | null | 23.479012 | 307 | 0.484278 | [
[
[
"import numpy as np\na = np.matrix([[1,2,3],[4,5,6]])",
"_____no_output_____"
],
[
"print(type(a))\nprint(a.T)\nprint(a.shape)\nprint(a.transpose())",
"<class 'numpy.matrix'>\n[[1 4]\n [2 5]\n [3 6]]\n(2, 3)\n[[1 4]\n [2 5]\n [3 6]]\n"
],
[
"class Dog:\n pass # placeholder",
"_____no_output_____"
],
[
"my_dog = Dog() # must have ()!!\nprint(type(my_dog))",
"<class '__main__.Dog'>\n"
],
[
"isinstance(my_dog,Dog)",
"_____no_output_____"
]
],
[
[
"## Class Attributes\nIn practice a dog as a color, breed, age, and other attributes, and it can do things like eat, run, sleep, bark etc.\n",
"_____no_output_____"
]
],
[
[
"class Dog:\n # Atributes\n age = 0\n name = 'noname'\n breed = 'nobreed'\n color = 'nocolor'\n ",
"_____no_output_____"
],
[
"my_dog = Dog()\nprint('{} is a {}-year old {} {}.'.format(my_dog.name,my_dog.age,my_dog.color,my_dog.breed))",
"noname is a 0-year old nocolor nobreed.\n"
],
[
"my_dog = Dog()\nmy_dog.age = 2\nmy_dog.name = 'Fido'\nmy_dog.color = 'brown'\nmy_dog.breed = 'Labradoodle'\nprint('{} is a {}-year old {} {}.'.format(my_dog.name,my_dog.age,my_dog.color,my_dog.breed))\n\n",
"Fido is a 2-year old brown Labradoodle.\n"
]
],
[
[
"## Object Constructor\n",
"_____no_output_____"
]
],
[
[
"class Dog:\n def __init__(self, age ,name ,breed ,color):\n self.age = age\n self.name = name\n self.breed = breed\n self.color = color",
"_____no_output_____"
],
[
"my_dog = Dog('4','Coco','Corgie','Brown')\nprint('{} is a {}-year old {} {}.'.format(my_dog.name,my_dog.age,my_dog.color,my_dog.breed))",
"Coco is a 4-year old Brown Corgie.\n"
],
[
"class Dog:\n def __init__(self, age ,name ,breed ,color):\n self.age = age\n self.name = name\n self.breed = breed\n self.color = color\n def info(self):\n print('{} is a {}-year old {} {}.'.format(my_dog.name,my_dog.age,my_dog.color,my_dog.breed))",
"_____no_output_____"
],
[
"my_dog = Dog('4','Coco','Corgie','Brown')\nmy_dog.info()",
"Coco is a 4-year old Brown Corgie.\n"
],
[
"class Dog:\n def __init__(self, age = 0 ,name = 'noname' ,breed = 'nobreed' ,color = 'nocolor'):\n self.age = age\n self.name = name\n self.breed = breed\n self.color = color\n def info(self):\n print('{} is a {}-year old {} {}.'.format(my_dog.name,my_dog.age,my_dog.color,my_dog.breed))",
"_____no_output_____"
],
[
"my_dog = Dog()\nmy_dog.info()",
"noname is a 0-year old nocolor nobreed.\n"
],
[
"class Dog:\n #Global Attributes\n species = 'mammal'\n \n def __init__(self, age = 0 ,name = 'noname' ,breed = 'nobreed' ,color = 'nocolor'):\n self.age = age\n self.name = name\n self.breed = breed\n self.color = color\n def info(self):\n print('{} is a {}-year old {} {}.'.format(my_dog.name,my_dog.age,my_dog.color,my_dog.breed))",
"_____no_output_____"
],
[
"my_dog = Dog(name = 'Ralph', age = 7, color = 'gray', breed = 'Chihuahua')\nmy_dog.info()\nprint(my_dog.species)",
"Ralph is a 7-year old gray Chihuahua.\nmammal\n"
]
],
[
[
"## A physics example",
"_____no_output_____"
]
],
[
[
"class Projectile():\n gravityConstant = 9.81 # m/s^2\n\n def __init__(self, initVelocity):\n self.initVelocity = initVelocity\n #self.time = time\n def getHeight(self, time):\n return self.initVelocity*time-.5*self.gravityConstant*time**2\n \n ",
"_____no_output_____"
],
[
"ball = Projectile(initVelocity = 10)\nheight = ball.getHeight(.1)\nprint(height)\nprint(ball.initVelocity)\n",
"0.95095\n10\n"
],
[
"class Projectile():\n gravityConstant = 9.81 # m/s^2\n\n def __init__(self, initVelocity):\n self.initVelocity = initVelocity\n #self.time = time\n \n\n \n def getHeight(self, time):\n return self.initVelocity*time-.5*self.gravityConstant*time**2\n ",
"_____no_output_____"
]
],
[
[
"## Inhertiance",
"_____no_output_____"
]
],
[
[
"class childName(parentName):\n ## list of all new, sub-class specific attributes and methods\n # including the sub-class constructor\n ",
"_____no_output_____"
],
[
"class Animal: \n #Animal Constructor\n def __init__(self,age = 0, weight = 0, animal_is_alive = True):\n self.age = age\n self.weigt = weight\n self.animal_is_alive = animal_is_alive\n \n #eat food\n def eat(self, food = None):\n if food == None:\n print(\"There is nothing to eat :-(\")\n else:\n print('Eating {}...yum yum....'.format(food))\n #sleeping\n def sleep(self):\n print('Sleeping...zzzzzzzz....')\n ",
"_____no_output_____"
],
[
"Coco = Animal(3,10,True)\nCoco.sleep()\nCoco.eat(food = 'bananas')",
"Sleeping...zzzzzzzz....\nEating bananas...yum yum....\n"
],
[
"class Dog(Animal):\n #Dog Constructor\n def __init__(self, age = 0, weight = 0, animal_is_alive = True, breed = 'nobreed', color = 'nocolor', name = 'noname', bark_sound = 'ruff'):\n self.breed = breed\n self.color = color\n self.bark_sound = bark_sound\n self.name = name\n Animal.__init__(self,age,weight,animal_is_alive)\n # barking method\n def bark(self, num_barks = 3):\n for i in range(num_barks):\n print('{}'.format(self.bark),end = ' ')\n def info(self):\n print('{} is a {}-year old {} {}.'.format(my_dog.name,my_dog.age,my_dog.color,my_dog.breed))",
"_____no_output_____"
],
[
"Fido = Dog(age = 1, weight = 15, animal_is_alive = True, breed='Husky',color = 'gray',name = 'Fido')",
"_____no_output_____"
],
[
"Fido.info()",
"Ralph is a 7-year old gray Chihuahua.\n"
],
[
"Fido.bark(3)",
"<bound method Dog.bark of <__main__.Dog object at 0x7f3e86e1ba30>> <bound method Dog.bark of <__main__.Dog object at 0x7f3e86e1ba30>> <bound method Dog.bark of <__main__.Dog object at 0x7f3e86e1ba30>> "
]
],
[
[
"## Overloading and Multiple Inheritances",
"_____no_output_____"
]
],
[
[
"class MotherDog(Animal):\n \n def __init__(self,age = 0,weight = 0,animal_is_alive = True, breed = 'nobreed', color = 'nocolor', name = 'noname',):\n \n def bark(self, num_barks = 3):\n for i in range(num_barks):\n print('arf', end = ' ') \n class FatherDog(Animal)",
"_____no_output_____"
]
],
[
[
"## Polymorphism",
"_____no_output_____"
]
],
[
[
"Tito = FatherDog(age=12,breed='Doberman',)",
"_____no_output_____"
]
],
[
[
"## Overloading Operations and Functions",
"_____no_output_____"
]
],
[
[
"class Vector:\n def __init__(self,x_comp,y_comp):\n self.x_comp = x_comp\n self.y_comp = y_comp\n def __abs__(self):\n return (self.x_comp**2+self.y_comp**2)**(0.5)\n\n ",
"_____no_output_____"
],
[
"x = Vector(1,2)",
"_____no_output_____"
],
[
"print(x)\n",
"<__main__.Vector object at 0x7f1480360070>\n"
],
[
"class Vector:\n def __init__(self,x_comp,y_comp):\n self.x_comp = x_comp\n self.y_comp = y_comp\n def __abs__(self):\n return (self.x_comp**2+self.y_comp**2)**(0.5)\n def __len__(self):\n return 2\n def __add__(self,other):\n return Vector(self.x_comp + other.x_comp, self.y_comp + other.y_comp)",
"_____no_output_____"
],
[
"x = Vector(1,2)\ny = Vector(3,7)\nz = x+y\nprint(z.x_comp)\nprint(z.y_comp)",
"4\n9\n"
],
[
"class Vector:\n def __init__(self,x_comp,y_comp):\n self.x_comp = x_comp\n self.y_comp = y_comp\n def __abs__(self):\n return (self.x_comp**2+self.y_comp**2)**(0.5)\n def __len__(self):\n return 2\n def __add__(self,other):\n return Vector(self.x_comp + other.x_comp, self.y_comp + other.y_comp)\n def __mul__(self,other):\n return Vector(self.x_comp*other, self.y_comp*other)\n #def __mul__(other,self):\n #return Vector(other*self.x_comp, other*self.y_comp)",
"_____no_output_____"
],
[
"x = Vector(1,2)\ny = 2\nz = x*y",
"_____no_output_____"
],
[
"print(z.x_comp)\nprint(z.y_comp)",
"2\n4\n"
],
[
"z2 = y*x",
"_____no_output_____"
],
[
"class Vector:\n def __init__(self,x_comp,y_comp):\n self.x_comp = x_comp\n self.y_comp = y_comp\n def __abs__(self):\n return (self.x_comp**2+self.y_comp**2)**(0.5)\n def __len__(self):\n return 2\n def __add__(self,other):\n return Vector(self.x_comp + other.x_comp, self.y_comp + other.y_comp)\n def __mul__(self,other):\n return Vector(self.x_comp*other, self.y_comp*other)\n def __rmul__(self,other):\n return Vector(self.x_comp*other, self.y_comp*other)",
"_____no_output_____"
]
]
] | [
"code",
"markdown",
"code",
"markdown",
"code",
"markdown",
"code",
"markdown",
"code",
"markdown",
"code",
"markdown",
"code",
"markdown",
"code"
] | [
[
"code",
"code",
"code",
"code",
"code"
],
[
"markdown"
],
[
"code",
"code",
"code"
],
[
"markdown"
],
[
"code",
"code",
"code",
"code",
"code",
"code",
"code",
"code"
],
[
"markdown"
],
[
"code",
"code",
"code"
],
[
"markdown"
],
[
"code",
"code",
"code",
"code",
"code",
"code",
"code"
],
[
"markdown"
],
[
"code"
],
[
"markdown"
],
[
"code"
],
[
"markdown"
],
[
"code",
"code",
"code",
"code",
"code",
"code",
"code",
"code",
"code",
"code"
]
] |
d0906849f45c4da867b27f887cea244f20311c36 | 2,107 | ipynb | Jupyter Notebook | mongo/01_Mongo_Examples.ipynb | fs714/concurrency-example | fbff041804b9c46fb7f21ebbae22acff745c7b0c | [
"Apache-2.0"
] | null | null | null | mongo/01_Mongo_Examples.ipynb | fs714/concurrency-example | fbff041804b9c46fb7f21ebbae22acff745c7b0c | [
"Apache-2.0"
] | null | null | null | mongo/01_Mongo_Examples.ipynb | fs714/concurrency-example | fbff041804b9c46fb7f21ebbae22acff745c7b0c | [
"Apache-2.0"
] | 1 | 2020-03-10T15:47:05.000Z | 2020-03-10T15:47:05.000Z | 27.723684 | 104 | 0.549597 | [
[
[
"import os\nfrom pymongo import MongoClient\n\ndef get_code_from_colname(colname):\n (market, code) = colname.split('_')\n return code + '.' + market\n\nwind_db_name = os.environ.get('WIND_DB', 'emquant')\nchan_db_name = os.environ.get('CHAN_DB', 'emchan')\ndb_ip = os.environ.get('MONGO_DB_IP_ADDR', '172.17.0.1')\ndb_port = int(os.environ.get('MONGO_DB_PORT', 27017))\n\n# wind_db_name = os.environ.get('WIND_DB', 'wind')\n# chan_db_name = os.environ.get('CHAN_DB', 'chan')\n# db_ip = os.environ.get('MONGO_DB_IP_ADDR', '192.168.1.103')\n# db_port = int(os.environ.get('MONGO_DB_PORT', 27017))\n\nclient = MongoClient(db_ip, db_port)\ndb_wind = client[wind_db_name]\ndb_chan = client[chan_db_name]\n\nchankline_col = db_chan['chankline']\n\ncode_list = db_wind.collection_names(include_system_collections=False)\ncode_list = [get_code_from_colname(code) for code in code_list if 'SH' in code or 'SZ' in code]\n\nfor code in code_list:\n filter = {'windCode': code, 'ktype': '1_1'}\n cursor = chankline_col.find(filter).sort('index', -1).limit(1)\n for doc in cursor:\n if doc['inclusive'] != 0:\n print(doc)\n \nprint('Finished')",
"Finished\n"
]
]
] | [
"code"
] | [
[
"code"
]
] |
d09069507239559f246a59c9cd1689543eb21ea9 | 54,881 | ipynb | Jupyter Notebook | model_training.ipynb | freddycct/CarND-Behavioral-Cloning-P3 | 512108e8d9811d4de11d2724f1161eaab86dd85f | [
"MIT"
] | null | null | null | model_training.ipynb | freddycct/CarND-Behavioral-Cloning-P3 | 512108e8d9811d4de11d2724f1161eaab86dd85f | [
"MIT"
] | null | null | null | model_training.ipynb | freddycct/CarND-Behavioral-Cloning-P3 | 512108e8d9811d4de11d2724f1161eaab86dd85f | [
"MIT"
] | null | null | null | 117.267094 | 35,472 | 0.877535 | [
[
[
"import cv2\nimport numpy as np\nimport pandas as pd\nimport matplotlib.pyplot as plt\n\nfrom keras.models import Sequential, Model\nfrom keras.layers import Flatten, Dense, Conv2D, MaxPooling2D, BatchNormalization, Cropping2D, Lambda, Activation, Dropout\nfrom keras.optimizers import Adam\nfrom keras.initializers import glorot_normal\nfrom sklearn.utils import shuffle\n\nfrom model import processFilename, Nvidia, generator",
"/home/freddy/apps/miniconda3/lib/python3.6/site-packages/h5py/__init__.py:36: FutureWarning: Conversion of the second argument of issubdtype from `float` to `np.floating` is deprecated. In future, it will be treated as `np.float64 == np.dtype(float).type`.\n from ._conv import register_converters as _register_converters\nUsing TensorFlow backend.\n"
],
[
"track = 'track2_almost_working'",
"_____no_output_____"
],
[
"driving_log = pd.read_csv(\n '{}/driving_log.csv'.format(track), \n names=['center','left','right','angle','throttle','brake','speed']\n)",
"_____no_output_____"
],
[
"center_left_right_angle = driving_log[['center', 'left', 'right', 'angle']]",
"_____no_output_____"
],
[
"image = plt.imread( processFilename(track, center_left_right_angle.iloc[0].center) )",
"_____no_output_____"
],
[
"image.shape",
"_____no_output_____"
],
[
"plt.imshow(image[75:-25,:,:])",
"_____no_output_____"
],
[
"image[75:-25,:,:].shape",
"_____no_output_____"
],
[
"# this function reads the images into memory, it is not a scalable approach when there are too many images\ndef read_angles(driving_log):\n angles = []\n for row in driving_log.itertuples():\n angle = row.angle\n angles.append(angle)\n # end for\n return np.array(angles)\n# end def",
"_____no_output_____"
],
[
"angles = read_angles(center_left_right_angle)",
"_____no_output_____"
],
[
"angles.shape",
"_____no_output_____"
],
[
"augmented_angles = []\nfor angle in angles:\n augmented_angles.append(angle)\n augmented_angles.append(-angle)",
"_____no_output_____"
],
[
"plt.hist(angles, bins=100)\nplt.xlabel('angle')\nplt.ylabel('frequency')\nplt.title('histogram before augmentation')\nplt.show()",
"_____no_output_____"
],
[
"plt.hist(augmented_angles, bins=100)\nplt.xlabel('angle')\nplt.ylabel('frequency')\nplt.title('histogram after augmentation')\nplt.show()",
"_____no_output_____"
],
[
"np.random.seed(1) # set the random number seed\n\nnpts = len(center_left_right_angle)\n\n# center_left_right_angle contains all the rows\n# split into training and validation with a 0.8, 0.2 split\n\nnpts_rand = np.random.rand(npts)\ntrain_set = center_left_right_angle[npts_rand <= 0.8]\nvalid_set = center_left_right_angle[npts_rand > 0.8]",
"_____no_output_____"
],
[
"batch_size = 50\ntrain_generator = generator(train_set, batch_size, track)\nvalid_generator = generator(valid_set, batch_size, track)\n\nsteps_per_epoch = np.rint(len(train_set) / batch_size).astype(int)\nvalidation_steps = np.rint(len(valid_set) / batch_size).astype(int)",
"_____no_output_____"
],
[
"def Nvidia(dropout=0.0):\n model = Sequential()\n model.add(Cropping2D(cropping=((75,25), (0,0)), input_shape=(160,320,3), name='crop'))\n model.add(BatchNormalization()) # 60 x 320 x 3\n \n model.add(Conv2D(\n 6, 5, strides=(2,2), padding='same', \n kernel_initializer=glorot_normal(seed=1), bias_initializer='zeros',\n name='conv1'\n )) \n model.add(BatchNormalization())\n model.add(Activation('relu'))\n model.add(Dropout(dropout))\n \n model.add(Conv2D(\n 12, 5, strides=(1,2), padding='same',\n kernel_initializer=glorot_normal(seed=1), bias_initializer='zeros',\n name='conv2'\n )) \n model.add(BatchNormalization())\n model.add(Activation('relu'))\n model.add(Dropout(dropout))\n \n model.add(Conv2D(\n 16, 5, strides=(1,2), padding='same',\n kernel_initializer=glorot_normal(seed=1), bias_initializer='zeros',\n name='conv3'\n ))\n model.add(BatchNormalization())\n model.add(Activation('relu'))\n model.add(Dropout(dropout))\n \n model.add(Conv2D(\n 20, 3, padding='valid',\n kernel_initializer=glorot_normal(seed=1), bias_initializer='zeros',\n name='conv4'\n ))\n model.add(BatchNormalization())\n model.add(Activation('relu'))\n model.add(Dropout(dropout))\n \n model.add(Conv2D(\n 24, 3, padding='valid',\n kernel_initializer=glorot_normal(seed=1), bias_initializer='zeros',\n name='conv5'\n ))\n model.add(BatchNormalization())\n model.add(Activation('relu'))\n model.add(Dropout(dropout))\n \n model.add(Flatten())\n\n model.add(Dense(100, kernel_initializer=glorot_normal(seed=1), bias_initializer='zeros'))\n model.add(BatchNormalization())\n model.add(Activation('relu'))\n model.add(Dropout(dropout))\n \n model.add(Dense(50, kernel_initializer=glorot_normal(seed=1), bias_initializer='zeros'))\n model.add(BatchNormalization())\n model.add(Activation('relu'))\n model.add(Dropout(dropout))\n \n model.add(Dense(10, kernel_initializer=glorot_normal(seed=1), bias_initializer='zeros'))\n model.add(BatchNormalization())\n model.add(Activation('relu'))\n model.add(Dropout(dropout))\n \n model.add(Dense(1, kernel_initializer=glorot_normal(seed=1), bias_initializer='zeros'))\n \n return model",
"_____no_output_____"
],
[
"from keras.models import load_model\nmodel = load_model('params/all_data_model.h5')",
"_____no_output_____"
],
[
"model.get_layer('conv5').output",
"_____no_output_____"
],
[
"model = Nvidia(dropout=0.25)",
"_____no_output_____"
],
[
"optimizer = Adam(lr=1e-3)\nmodel.compile(loss='mse', optimizer=optimizer)",
"_____no_output_____"
],
[
"model.fit_generator(\n train_generator, steps_per_epoch=steps_per_epoch, \n epochs=10, \n validation_data=valid_generator, validation_steps=validation_steps\n)",
"_____no_output_____"
],
[
"model.get_layer('conv1').output",
"_____no_output_____"
],
[
"intermediate_layer_model = Model(inputs=model.input, outputs=model.get_layer('conv5').output)\nintermediate_output = intermediate_layer_model.predict(np.expand_dims(image, 0))",
"_____no_output_____"
],
[
"plt.imshow(intermediate_output[0,:,:,1],cmap='gray')",
"_____no_output_____"
],
[
"model.save('params/{}_model.h5'.format(track))",
"_____no_output_____"
]
]
] | [
"code"
] | [
[
"code",
"code",
"code",
"code",
"code",
"code",
"code",
"code",
"code",
"code",
"code",
"code",
"code",
"code",
"code",
"code",
"code",
"code",
"code",
"code",
"code",
"code",
"code",
"code",
"code",
"code"
]
] |
d09072e6def30eb1afdc4d6d60d170ef17070f3b | 58,690 | ipynb | Jupyter Notebook | site/en-snapshot/addons/tutorials/optimizers_conditionalgradient.ipynb | ilyaspiridonov/docs-l10n | a061a44e40d25028d0a4458094e48ab717d3565c | [
"Apache-2.0"
] | 2 | 2021-02-22T12:15:33.000Z | 2021-05-02T15:22:13.000Z | site/en-snapshot/addons/tutorials/optimizers_conditionalgradient.ipynb | ilyaspiridonov/docs-l10n | a061a44e40d25028d0a4458094e48ab717d3565c | [
"Apache-2.0"
] | null | null | null | site/en-snapshot/addons/tutorials/optimizers_conditionalgradient.ipynb | ilyaspiridonov/docs-l10n | a061a44e40d25028d0a4458094e48ab717d3565c | [
"Apache-2.0"
] | 1 | 2020-05-31T15:04:18.000Z | 2020-05-31T15:04:18.000Z | 102.425829 | 20,514 | 0.795996 | [
[
[
"##### Copyright 2020 The TensorFlow Authors.",
"_____no_output_____"
]
],
[
[
"#@title Licensed under the Apache License, Version 2.0\n# you may not use this file except in compliance with the License.\n# You may obtain a copy of the License at\n#\n# https://www.apache.org/licenses/LICENSE-2.0\n#\n# Unless required by applicable law or agreed to in writing, software\n# distributed under the License is distributed on an \"AS IS\" BASIS,\n# WITHOUT WARRANTIES OR CONDITIONS OF ANY KIND, either express or implied.\n# See the License for the specific language governing permissions and\n# limitations under the License.",
"_____no_output_____"
]
],
[
[
"# TensorFlow Addons Optimizers: ConditionalGradient\n\n\n<table class=\"tfo-notebook-buttons\" align=\"left\">\n <td>\n <a target=\"_blank\" href=\"https://www.tensorflow.org/addons/tutorials/optimizers_conditionalgradient\"><img src=\"https://www.tensorflow.org/images/tf_logo_32px.png\" />View on TensorFlow.org</a>\n </td>\n <td>\n <a target=\"_blank\" href=\"https://colab.research.google.com/github/tensorflow/addons/blob/master/docs/tutorials/optimizers_conditionalgradient.ipynb\"><img src=\"https://www.tensorflow.org/images/colab_logo_32px.png\" />Run in Google Colab</a>\n </td>\n <td>\n <a target=\"_blank\" href=\"https://github.com/tensorflow/addons/blob/master/docs/tutorials/optimizers_conditionalgradient.ipynb\"><img src=\"https://www.tensorflow.org/images/GitHub-Mark-32px.png\" />View source on GitHub</a>\n </td>\n <td>\n <a href=\"https://storage.googleapis.com/tensorflow_docs/addons/docs/tutorials/optimizers_conditionalgradient.ipynb\"><img src=\"https://www.tensorflow.org/images/download_logo_32px.png\" />Download notebook</a>\n </td>\n</table>",
"_____no_output_____"
],
[
"# Overview\nThis notebook will demonstrate how to use the Conditional Graident Optimizer from the Addons package.",
"_____no_output_____"
],
[
"# ConditionalGradient\n\n\n> Constraining the parameters of a neural network has been shown to be beneficial in training because of the underlying regularization effects. Often, parameters are constrained via a soft penalty (which never guarantees the constraint satisfaction) or via a projection operation (which is computationally expensive). Conditional gradient (CG) optimizer, on the other hand, enforces the constraints strictly without the need for an expensive projection step. It works by minimizing a linear approximation of the objective within the constraint set. In this notebook, we demonstrate the appliction of Frobenius norm constraint via the CG optimizer on the MNIST dataset. CG is now available as a tensorflow API. More details of the optimizer are available at https://arxiv.org/pdf/1803.06453.pdf\n",
"_____no_output_____"
],
[
"## Setup",
"_____no_output_____"
]
],
[
[
"import tensorflow as tf\nimport tensorflow_addons as tfa\nfrom matplotlib import pyplot as plt",
"TensorFlow 2.x selected.\n"
],
[
"# Hyperparameters\nbatch_size=64\nepochs=10",
"_____no_output_____"
]
],
[
[
"# Build the Model",
"_____no_output_____"
]
],
[
[
"model_1 = tf.keras.Sequential([\n tf.keras.layers.Dense(64, input_shape=(784,), activation='relu', name='dense_1'),\n tf.keras.layers.Dense(64, activation='relu', name='dense_2'),\n tf.keras.layers.Dense(10, activation='softmax', name='predictions'),\n])",
"_____no_output_____"
]
],
[
[
"# Prep the Data",
"_____no_output_____"
]
],
[
[
"# Load MNIST dataset as NumPy arrays\ndataset = {}\nnum_validation = 10000\n(x_train, y_train), (x_test, y_test) = tf.keras.datasets.mnist.load_data()\n\n# Preprocess the data\nx_train = x_train.reshape(-1, 784).astype('float32') / 255\nx_test = x_test.reshape(-1, 784).astype('float32') / 255",
"Downloading data from https://storage.googleapis.com/tensorflow/tf-keras-datasets/mnist.npz\n11493376/11490434 [==============================] - 0s 0us/step\n"
]
],
[
[
"# Define a Custom Callback Function",
"_____no_output_____"
]
],
[
[
"def frobenius_norm(m):\n \"\"\"This function is to calculate the frobenius norm of the matrix of all\n layer's weight.\n \n Args:\n m: is a list of weights param for each layers.\n \"\"\"\n total_reduce_sum = 0\n for i in range(len(m)):\n total_reduce_sum = total_reduce_sum + tf.math.reduce_sum(m[i]**2)\n norm = total_reduce_sum**0.5\n return norm",
"_____no_output_____"
],
[
"CG_frobenius_norm_of_weight = []\nCG_get_weight_norm = tf.keras.callbacks.LambdaCallback(\n on_epoch_end=lambda batch, logs: CG_frobenius_norm_of_weight.append(\n frobenius_norm(model_1.trainable_weights).numpy()))",
"_____no_output_____"
]
],
[
[
"# Train and Evaluate: Using CG as Optimizer\n\nSimply replace typical keras optimizers with the new tfa optimizer ",
"_____no_output_____"
]
],
[
[
"# Compile the model\nmodel_1.compile(\n optimizer=tfa.optimizers.ConditionalGradient(\n learning_rate=0.99949, lambda_=203), # Utilize TFA optimizer\n loss=tf.keras.losses.SparseCategoricalCrossentropy(),\n metrics=['accuracy'])\n\nhistory_cg = model_1.fit(\n x_train,\n y_train,\n batch_size=batch_size,\n validation_data=(x_test, y_test),\n epochs=epochs,\n callbacks=[CG_get_weight_norm])",
"Train on 60000 samples, validate on 10000 samples\nEpoch 1/10\n60000/60000 [==============================] - 5s 85us/sample - loss: 0.3745 - accuracy: 0.8894 - val_loss: 0.2323 - val_accuracy: 0.9275\nEpoch 2/10\n60000/60000 [==============================] - 3s 50us/sample - loss: 0.1908 - accuracy: 0.9430 - val_loss: 0.1538 - val_accuracy: 0.9547\nEpoch 3/10\n60000/60000 [==============================] - 3s 49us/sample - loss: 0.1497 - accuracy: 0.9548 - val_loss: 0.1473 - val_accuracy: 0.9560\nEpoch 4/10\n60000/60000 [==============================] - 3s 49us/sample - loss: 0.1306 - accuracy: 0.9612 - val_loss: 0.1215 - val_accuracy: 0.9609\nEpoch 5/10\n60000/60000 [==============================] - 3s 49us/sample - loss: 0.1211 - accuracy: 0.9636 - val_loss: 0.1114 - val_accuracy: 0.9660\nEpoch 6/10\n60000/60000 [==============================] - 3s 48us/sample - loss: 0.1125 - accuracy: 0.9663 - val_loss: 0.1260 - val_accuracy: 0.9640\nEpoch 7/10\n60000/60000 [==============================] - 3s 50us/sample - loss: 0.1108 - accuracy: 0.9665 - val_loss: 0.1009 - val_accuracy: 0.9697\nEpoch 8/10\n60000/60000 [==============================] - 3s 51us/sample - loss: 0.1081 - accuracy: 0.9676 - val_loss: 0.1129 - val_accuracy: 0.9647\nEpoch 9/10\n60000/60000 [==============================] - 3s 50us/sample - loss: 0.1065 - accuracy: 0.9675 - val_loss: 0.1058 - val_accuracy: 0.9683\nEpoch 10/10\n60000/60000 [==============================] - 3s 51us/sample - loss: 0.1039 - accuracy: 0.9683 - val_loss: 0.1126 - val_accuracy: 0.9646\n"
]
],
[
[
"# Train and Evaluate: Using SGD as Optimizer",
"_____no_output_____"
]
],
[
[
"model_2 = tf.keras.Sequential([\n tf.keras.layers.Dense(64, input_shape=(784,), activation='relu', name='dense_1'),\n tf.keras.layers.Dense(64, activation='relu', name='dense_2'),\n tf.keras.layers.Dense(10, activation='softmax', name='predictions'),\n])",
"_____no_output_____"
],
[
"SGD_frobenius_norm_of_weight = []\nSGD_get_weight_norm = tf.keras.callbacks.LambdaCallback(\n on_epoch_end=lambda batch, logs: SGD_frobenius_norm_of_weight.append(\n frobenius_norm(model_2.trainable_weights).numpy()))",
"_____no_output_____"
],
[
"# Compile the model\nmodel_2.compile(\n optimizer=tf.keras.optimizers.SGD(0.01), # Utilize SGD optimizer\n loss=tf.keras.losses.SparseCategoricalCrossentropy(),\n metrics=['accuracy'])\n\nhistory_sgd = model_2.fit(\n x_train,\n y_train,\n batch_size=batch_size,\n validation_data=(x_test, y_test),\n epochs=epochs,\n callbacks=[SGD_get_weight_norm])",
"Train on 60000 samples, validate on 10000 samples\nEpoch 1/10\n60000/60000 [==============================] - 3s 46us/sample - loss: 0.9498 - accuracy: 0.7523 - val_loss: 0.4306 - val_accuracy: 0.8844\nEpoch 2/10\n60000/60000 [==============================] - 2s 41us/sample - loss: 0.3851 - accuracy: 0.8916 - val_loss: 0.3298 - val_accuracy: 0.9068\nEpoch 3/10\n60000/60000 [==============================] - 3s 42us/sample - loss: 0.3230 - accuracy: 0.9064 - val_loss: 0.2917 - val_accuracy: 0.9150\nEpoch 4/10\n60000/60000 [==============================] - 2s 41us/sample - loss: 0.2897 - accuracy: 0.9169 - val_loss: 0.2676 - val_accuracy: 0.9241\nEpoch 5/10\n60000/60000 [==============================] - 3s 43us/sample - loss: 0.2658 - accuracy: 0.9237 - val_loss: 0.2485 - val_accuracy: 0.9288\nEpoch 6/10\n60000/60000 [==============================] - 2s 41us/sample - loss: 0.2467 - accuracy: 0.9301 - val_loss: 0.2374 - val_accuracy: 0.9285\nEpoch 7/10\n60000/60000 [==============================] - 3s 42us/sample - loss: 0.2308 - accuracy: 0.9343 - val_loss: 0.2201 - val_accuracy: 0.9358\nEpoch 8/10\n60000/60000 [==============================] - 2s 41us/sample - loss: 0.2169 - accuracy: 0.9388 - val_loss: 0.2096 - val_accuracy: 0.9388\nEpoch 9/10\n60000/60000 [==============================] - 2s 42us/sample - loss: 0.2046 - accuracy: 0.9421 - val_loss: 0.2009 - val_accuracy: 0.9404\nEpoch 10/10\n60000/60000 [==============================] - 2s 41us/sample - loss: 0.1939 - accuracy: 0.9448 - val_loss: 0.1900 - val_accuracy: 0.9442\n"
]
],
[
[
"# Frobenius Norm of Weights: CG vs SGD",
"_____no_output_____"
],
[
"The current implementation of CG optimizer is based on Frobenius Norm, with considering Frobenius Norm as regularizer in the target function. Therefore, we compare CG’s regularized effect with SGD optimizer, which has not imposed Frobenius Norm regularizer.",
"_____no_output_____"
]
],
[
[
"plt.plot(\n CG_frobenius_norm_of_weight,\n color='r',\n label='CG_frobenius_norm_of_weights')\nplt.plot(\n SGD_frobenius_norm_of_weight,\n color='b',\n label='SGD_frobenius_norm_of_weights')\nplt.xlabel('Epoch')\nplt.ylabel('Frobenius norm of weights')\nplt.legend(loc=1)",
"_____no_output_____"
]
],
[
[
"# Train and Validation Accuracy: CG vs SGD\n",
"_____no_output_____"
]
],
[
[
"plt.plot(history_cg.history['accuracy'], color='r', label='CG_train')\nplt.plot(history_cg.history['val_accuracy'], color='g', label='CG_test')\nplt.plot(history_sgd.history['accuracy'], color='pink', label='SGD_train')\nplt.plot(history_sgd.history['val_accuracy'], color='b', label='SGD_test')\nplt.xlabel('Epoch')\nplt.ylabel('Accuracy')\nplt.legend(loc=4)",
"_____no_output_____"
]
]
] | [
"markdown",
"code",
"markdown",
"code",
"markdown",
"code",
"markdown",
"code",
"markdown",
"code",
"markdown",
"code",
"markdown",
"code",
"markdown",
"code",
"markdown",
"code"
] | [
[
"markdown"
],
[
"code"
],
[
"markdown",
"markdown",
"markdown",
"markdown"
],
[
"code",
"code"
],
[
"markdown"
],
[
"code"
],
[
"markdown"
],
[
"code"
],
[
"markdown"
],
[
"code",
"code"
],
[
"markdown"
],
[
"code"
],
[
"markdown"
],
[
"code",
"code",
"code"
],
[
"markdown",
"markdown"
],
[
"code"
],
[
"markdown"
],
[
"code"
]
] |
d0907a691605bd004a26a340b72b826516411eaa | 32,556 | ipynb | Jupyter Notebook | notebooks/5_inference_scheduling.ipynb | youngmki/lookout-for-equipment-demo | 362c2ee1926947a0ded1c985b9354c8ec62fff1d | [
"MIT-0"
] | null | null | null | notebooks/5_inference_scheduling.ipynb | youngmki/lookout-for-equipment-demo | 362c2ee1926947a0ded1c985b9354c8ec62fff1d | [
"MIT-0"
] | null | null | null | notebooks/5_inference_scheduling.ipynb | youngmki/lookout-for-equipment-demo | 362c2ee1926947a0ded1c985b9354c8ec62fff1d | [
"MIT-0"
] | null | null | null | 31.154067 | 236 | 0.464431 | [
[
[
"# **Amazon Lookout for Equipment** - 익명화한 익스펜더 데이터셋에 대한 데모\n*파트 5: 정기적인 추론 호출 스케줄링*",
"_____no_output_____"
]
],
[
[
"BUCKET = '<YOUR_BUCKET_NAME_HERE>'\nPREFIX = 'data/scheduled_inference'",
"_____no_output_____"
]
],
[
[
"## 초기화\n---\n이 노트북에서는 데이터 폴더에 추론 디렉토리를 추가하게끔 저장소 구조를 갱신합니다.\n```\n/lookout-equipment-demo\n|\n+-- data/\n| |\n| +-- inference/\n| | |\n| | |-- input/\n| | |\n| | \\-- output/\n| |\n| +-- labelled-data/\n| | \\-- labels.csv\n| |\n| \\-- training-data/\n| \\-- expander/\n| |-- subsystem-01\n| | \\-- subsystem-01.csv\n| |\n| |-- subsystem-02\n| | \\-- subsystem-02.csv\n| |\n| |-- ...\n| |\n| \\-- subsystem-24\n| \\-- subsystem-24.csv\n|\n+-- dataset/\n| |-- labels.csv\n| |-- tags_description.csv\n| |-- timeranges.txt\n| \\-- timeseries.zip\n|\n+-- notebooks/\n| |-- 1_data_preparation.ipynb\n| |-- 2_dataset_creation.ipynb\n| |-- 3_model_training.ipynb\n| |-- 4_model_evaluation.ipynb\n| \\-- 5_inference_scheduling.ipynb <<< 본 노트북 <<<\n|\n+-- utils/\n |-- lookout_equipment_utils.py\n \\-- lookoutequipment.json\n```\n\n### 임포트",
"_____no_output_____"
]
],
[
[
"%%sh\npip -q install --upgrade pip\npip -q install --upgrade awscli boto3 sagemaker\naws configure add-model --service-model file://../utils/lookoutequipment.json --service-name lookoutequipment",
"_____no_output_____"
],
[
"from IPython.core.display import HTML\nHTML(\"<script>Jupyter.notebook.kernel.restart()</script>\")",
"_____no_output_____"
],
[
"import boto3\nimport datetime\nimport os\nimport pandas as pd\nimport pprint\nimport pyarrow as pa\nimport pyarrow.parquet as pq\nimport sagemaker\nimport s3fs\nimport sys\nimport time\nimport uuid\nimport warnings\n\n# Lookout for Equipment API 호출 관리를 위한 Helper 함수\nsys.path.append('../utils')\nimport lookout_equipment_utils as lookout",
"_____no_output_____"
]
],
[
[
"### 파라미터",
"_____no_output_____"
]
],
[
[
"warnings.filterwarnings('ignore')\n\nDATA = os.path.join('..', 'data')\nRAW_DATA = os.path.join('..', 'dataset')\nINFER_DATA = os.path.join(DATA, 'inference')\n\n\nos.makedirs(os.path.join(INFER_DATA, 'input'), exist_ok=True)\nos.makedirs(os.path.join(INFER_DATA, 'output'), exist_ok=True)\n\nROLE_ARN = sagemaker.get_execution_role()\nREGION_NAME = boto3.session.Session().region_name",
"_____no_output_____"
]
],
[
[
"## 추론 스케줄러 생성하기\n---\n콘솔의 모델 세부 정보 부분으로 이동하면 추론 스케줄이 아직 없음을 확인할 수 있습니다.\n\n",
"_____no_output_____"
],
[
"### 스케줄러 설정\n새로운 추론 스케줄을 만들어 보겠습니다. 파라미터 일부는 필수 입력이지만 파라미터 다수는 유연하게 추가 설정할 수 있습니다.\n\n#### 파라미터\n\n* 추론을 위해 데이터를 업로드할 빈도로 `DATA_UPLOAD_FREQUENCY`를 설정합니다. 허용되는 값은`PT5M`,`PT10M`,`PT15M`,`PT30M`과`PT1H`입니다.\n * 이것은 추론 스케줄러가 실행되는 빈도와 데이터가 소스 버킷에 업로드되는 빈도입니다.\n * **참고** : ***업로드 빈도는 훈련 때 선택한 샘플링 비율과 호환되어야합니다.*** *예를 들어 모델을 30분 간격의 리샘플링으로 훈련시킨 경우 5분은 가능하지 않습니다. 추론 시 파라미터로 PT30M 또는 PT1H를 선택해야합니다.*\n* 추론 데이터의 S3 버킷으로 `INFERENCE_DATA_SOURCE_BUCKET`를 설정합니다.\n* 추론 데이터의 S3 접두사로 `INFERENCE_DATA_SOURCE_PREFIX`를 설정합니다.\n* 추론 결과를 원하는 S3 버킷으로 `INFERENCE_DATA_OUTPUT_BUCKET`를 설정합니다.\n* 추론 결과를 원하는 S3 접두사로 `INFERENCE_DATA_OUTPUT_PREFIX`를 설정합니다.\n* 추론할 데이터를 **읽고** 추론 출력을 **쓸** 때 사용할 역할로 `ROLE_ARN_FOR_INFERENCE`를 설정합니다.",
"_____no_output_____"
]
],
[
[
"# 생성하려는 추론 스케줄러의 이름\nINFERENCE_SCHEDULER_NAME = 'lookout-demo-model-v1-scheduler'\n\n# 본 추론 스케줄러를 생성할 모델의 이름\nMODEL_NAME_FOR_CREATING_INFERENCE_SCHEDULER = 'lookout-demo-model-v1'\n\n# 필수 입력 파라미터\nINFERENCE_DATA_SOURCE_BUCKET = BUCKET\nINFERENCE_DATA_SOURCE_PREFIX = f'{PREFIX}/input/'\nINFERENCE_DATA_OUTPUT_BUCKET = BUCKET\nINFERENCE_DATA_OUTPUT_PREFIX = f'{PREFIX}/output/'\nROLE_ARN_FOR_INFERENCE = ROLE_ARN\nDATA_UPLOAD_FREQUENCY = 'PT5M' ",
"_____no_output_____"
]
],
[
[
"#### 생략 가능한 파라미터\n\n* 데이터 업로드하는데 지연이 예상되는 시간(분)으로 `DATA_DELAY_OFFSET_IN_MINUTES`를 설정합니다. 즉, 데이터 업로드하는 시간에 대한 버퍼입니다.\n* ``INPUT_TIMEZONE_OFFSET``을 설정합니다. 허용되는 값은 +00:00, +00:30, -01:00, ... +11:30, +12:00, -00:00, -00:30, -01:00, ... -11:30, -12:00입니다.\n* `TIMESTAMP_FORMAT`을 설정합니다. 허용되는 값은 `EPOCH`, `yyyy-MM-dd-HH-mm-ss` 또는 `yyyyMMddHHmmss`입니다. 이것은 입력 데이터 파일 명에 접미사로 붙는 타임스탬프 형식입니다. 이것은 Lookout Equipment에서 추론을 실행할 파일을 파악하는 데 사용됩니다 (그러므로 스케줄러가 실행할 파일을 찾게 하기 위해 이전 파일을 제거할 필요가 없음).\n* `COMPONENT_TIMESTAMP_DELIMITER`를 설정합니다. 허용되는 값은 `-`, `_` 또는 ` `입니다. 입력 파일 명의 타임스탬프에서 구성 요소를 분리할 때 사용하는 구분자입니다.",
"_____no_output_____"
]
],
[
[
"DATA_DELAY_OFFSET_IN_MINUTES = None\nINPUT_TIMEZONE_OFFSET = '+00:00'\nCOMPONENT_TIMESTAMP_DELIMITER = '_'\nTIMESTAMP_FORMAT = 'yyyyMMddHHmmss'",
"_____no_output_____"
]
],
[
[
"### 추론 스케줄러 생성하기\nCreateInferenceScheduler API는 스케줄러를 생성**하고** 구동시킵니다. 즉, 즉각적으로 비용이 발생하기 시작합니다. 그러나 기존 스케줄러를 원하는대로 중지하거나 재구동시킬 수 있습니다 (이 노트북의 마지막 부분 참조).",
"_____no_output_____"
]
],
[
[
"scheduler = lookout.LookoutEquipmentScheduler(\n scheduler_name=INFERENCE_SCHEDULER_NAME,\n model_name=MODEL_NAME_FOR_CREATING_INFERENCE_SCHEDULER,\n region_name=REGION_NAME\n)\n\nscheduler_params = {\n 'input_bucket': INFERENCE_DATA_SOURCE_BUCKET,\n 'input_prefix': INFERENCE_DATA_SOURCE_PREFIX,\n 'output_bucket': INFERENCE_DATA_OUTPUT_BUCKET,\n 'output_prefix': INFERENCE_DATA_OUTPUT_PREFIX,\n 'role_arn': ROLE_ARN_FOR_INFERENCE,\n 'upload_frequency': DATA_UPLOAD_FREQUENCY,\n 'delay_offset': DATA_DELAY_OFFSET_IN_MINUTES,\n 'timezone_offset': INPUT_TIMEZONE_OFFSET,\n 'component_delimiter': COMPONENT_TIMESTAMP_DELIMITER,\n 'timestamp_format': TIMESTAMP_FORMAT\n}\n\nscheduler.set_parameters(**scheduler_params)",
"_____no_output_____"
]
],
[
[
"## 추론 데이터 준비하기\n---\n스케줄러가 모니터링할 S3 입력 위치에 몇 가지 데이터를 준비하고 전송하겠습니다. ",
"_____no_output_____"
]
],
[
[
"# 원본 신호 전체를 불러오겠습니다.\nall_tags_fname = os.path.join(DATA, 'training-data', 'expander.parquet')\ntable = pq.read_table(all_tags_fname)\nall_tags_df = table.to_pandas()\ndel table\nall_tags_df.head()",
"_____no_output_____"
]
],
[
[
"태그 설명을 불러옵시다. 본 데이터셋에는 다음 내용을 포함하는 태그 설명 파일이 존재합니다.\n\n* `Tag`: 이력 관리 시스템에 고객이 기록한 태그 명 (예컨대 [Honeywell 프로세스 이력 데이터베이스](https://www.honeywellprocess.com/en-US/explore/products/advanced-applications/uniformance/Pages/uniformance-phd.aspx))\n* `UOM`: 기록한 신호의 측정 단위\n* `Subsystem`: 해당 센서가 연결된 자산 부속의 ID\n\n여기에서 구성 요소 (즉, 하위 시스템 열)의 List를 수집할 수 있습니다. ",
"_____no_output_____"
]
],
[
[
"tags_description_fname = os.path.join(RAW_DATA, 'tags_description.csv')\ntags_description_df = pd.read_csv(tags_description_fname)\ncomponents = tags_description_df['Subsystem'].unique()\ntags_description_df.head()",
"_____no_output_____"
]
],
[
[
"샘플 추론 데이터셋을 구성하기 위해 원본 시계열 검증 기간에서 마지막 몇 분을 추출합니다. ",
"_____no_output_____"
]
],
[
[
"# 추출하려는 시퀀스 개수\nnum_sequences = 3\n\n# 스케줄링 빈도 (분): 이 값은 **반드시**\n# 모델 학습에 사용한 리샘플링 비율에 맞춰 설정해야 합니다. \nfrequency = 5\n\n# 각 시퀀스를 반복합니다.\nstart = all_tags_df.index.max() + datetime.timedelta(minutes=-frequency * (num_sequences) + 1)\nfor i in range(num_sequences):\n end = start + datetime.timedelta(minutes=+frequency - 1)\n \n # 이전 5분 단위로 시간을 반올림합니다.\n tm = datetime.datetime.now()\n tm = tm - datetime.timedelta(\n minutes=tm.minute % frequency,\n seconds=tm.second,\n microseconds=tm.microsecond\n )\n tm = tm + datetime.timedelta(minutes=+frequency * (i))\n tm = tm - datetime.timedelta(hours=9) # KST에 따른 조정\n current_timestamp = (tm).strftime(format='%Y%m%d%H%M%S')\n\n # 각 시퀀스마다 구성 요소 전체를 반복합니다. \n print(f'Extracting data from {start} to {end}:')\n new_index = None\n for component in components:\n # 해당 구성 요소와 특정 시간 범위에 대해 Dataframe을 추출합니다.\n signals = list(tags_description_df.loc[(tags_description_df['Subsystem'] == component), 'Tag'])\n signals_df = all_tags_df.loc[start:end, signals]\n \n # 스케줄러가 추론을 실행할 시간에 맞게끔\n # 인덱스를 재설정해야 합니다. \n if new_index is None:\n new_index = pd.date_range(\n start=tm,\n periods=signals_df.shape[0], \n freq='1min'\n )\n signals_df.index = new_index\n signals_df.index.name = 'Timestamp'\n signals_df = signals_df.reset_index()\n signals_df['Timestamp'] = signals_df['Timestamp'].dt.strftime('%Y-%m-%dT%H:%M:%S.%f')\n\n # 해당 파일을 CSV 형식으로 내보냅니다. \n component_fname = os.path.join(INFER_DATA, 'input', f'{component}_{current_timestamp}.csv')\n signals_df.to_csv(component_fname, index=None)\n \n start = start + datetime.timedelta(minutes=+frequency)\n \n# 입력 위치의 전체 폴더를 S3에 업로드합니다. \nINFERENCE_INPUT = os.path.join(INFER_DATA, 'input')\n!aws s3 cp --recursive --quiet $INFERENCE_INPUT s3://$BUCKET/$PREFIX/input\n \n# 이제 데이터를 준비했으므로 다음을 실행하여 스케줄러를 만듭니다.\ncreate_scheduler_response = scheduler.create()",
"Extracting data from 2015-11-30 23:45:00 to 2015-11-30 23:49:00:\nExtracting data from 2015-11-30 23:50:00 to 2015-11-30 23:54:00:\nExtracting data from 2015-11-30 23:55:00 to 2015-11-30 23:59:00:\n===== Polling Inference Scheduler Status =====\n\nScheduler Status: PENDING\nScheduler Status: RUNNING\n\n===== End of Polling Inference Scheduler Status =====\n"
]
],
[
[
"스케줄러가 실행 중이며 추론 기록은 현재 비어 있습니다.\n\n",
"_____no_output_____"
],
[
"## 추론 결과 얻기\n---",
"_____no_output_____"
],
[
"### 추론 실행 결과 나열하기",
"_____no_output_____"
],
[
"**스케줄러가 추론을 최초로 실행할 경우 5-15분 정도 걸립니다.** 대기가 끝나면 현재 추론 스케줄러에서 ListInferenceExecution API를 사용할 수 있습니다. 입력 파라미터로 스케줄러 명만 필요합니다.\n\n추론 실행 결과를 질의할 기간을 선택할 수 있습니다. 지정하지 않으면 추론 스케줄러에 대한 모든 실행 결과들이 나열됩니다. 시간 범위를 지정하려면 다음과 같이 합니다.\n\n```python\nSTART_TIME_FOR_INFERENCE_EXECUTIONS = datetime.datetime(2010,1,3,0,0,0)\nEND_TIME_FOR_INFERENCE_EXECUTIONS = datetime.datetime(2010,1,5,0,0,0)\n```\n\n즉, `2010-01-03 00:00:00`부터 `2010-01-05 00:00:00`까지의 실행 결과들이 나열됩니다.\n\n특정 상태의 실행 결과를 질의하도록 선택할 수도 있습니다. 허용되는 상태는 `IN_PROGRESS`, `SUCCESS`와 `FAILED`입니다.",
"_____no_output_____"
]
],
[
[
"START_TIME_FOR_INFERENCE_EXECUTIONS = None\nEND_TIME_FOR_INFERENCE_EXECUTIONS = None\nEXECUTION_STATUS = None\n\nexecution_summaries = []\n\nwhile len(execution_summaries) == 0:\n execution_summaries = scheduler.list_inference_executions(\n start_time=START_TIME_FOR_INFERENCE_EXECUTIONS,\n end_time=END_TIME_FOR_INFERENCE_EXECUTIONS,\n execution_status=EXECUTION_STATUS\n )\n if len(execution_summaries) == 0:\n print('WAITING FOR THE FIRST INFERENCE EXECUTION')\n time.sleep(60)\n \n else:\n print('FIRST INFERENCE EXECUTED\\n')\n break\n \n# execution_summaries",
"WAITING FOR THE FIRST INFERENCE EXECUTION\nWAITING FOR THE FIRST INFERENCE EXECUTION\nFIRST INFERENCE EXECUTED\n\n"
]
],
[
[
"스케줄러를 5분마다 실행하도록 구성했습니다. 몇 분 후 콘솔에서 첫 번째 실행 결과가 입력된 기록을 살펴볼 수 있습니다. \n\n",
"_____no_output_____"
],
[
"스케줄러가 시작될 때, 예를 들어 `datetime.datetime (2021, 1, 27, 9, 15)`일 때 입력 위치에서 **단일** CSV 파일을 찾습니다. 여기에는 타임스탬프가 포함된 파일 명이, 말하자면 다음과 같은 파일 명이 존재해야 합니다.\n\n* subsystem-01_2021012709**10**00.csv가 검색되고 수집됩니다.\n* subsystem-01_2021012709**15**00.csv는 수집되지 **않습니다** (다음 추론 실행 시 수집됨).\n\n`subsystem-01_20210127091000.csv` 파일을 연 다음 추론 실행의 DataStartTime과 DataEndTime 사이에 존재하는 시간 행을 찾습니다. 그러한 행을 찾지 못하면 내부 예외를 발생시킵니다.",
"_____no_output_____"
],
[
"### 실제 예측 결과 얻기",
"_____no_output_____"
],
[
"추론에 성공하면 CSV 파일이 버킷의 출력 위치에 저장됩니다. 각 추론은 `results.csv` 단일 파일이 존재하는 새 폴더를 만듭니다. 해당 파일을 읽고 여기에 내용을 표시해 보겠습니다. ",
"_____no_output_____"
]
],
[
[
"results_df = scheduler.get_predictions()\nresults_df.to_csv(os.path.join(INFER_DATA, 'output', 'results.csv'))\nresults_df.head()",
"_____no_output_____"
]
],
[
[
"## 추론 스케줄러 운영\n---\n### 추론 스케줄러 중단하기\n**근검 절약해야합니다**. 스케줄러 실행이 Amazon Lookout for Equipment 비용의 주된 원인입니다. 다음 API를 이용하여 현재 실행 중인 추론 스케줄러를 중지시키세요. 그렇게 하면 주기적인 추론 실행이 중지됩니다.",
"_____no_output_____"
]
],
[
[
"scheduler.stop()",
"===== Polling Inference Scheduler Status =====\n\nScheduler Status: STOPPING\nScheduler Status: STOPPED\n\n===== End of Polling Inference Scheduler Status =====\n"
]
],
[
[
"### 추론 스케줄러 시작하기\n다음 API를 사용하여 `STOPPED` 추론 스케줄러를 재시작할 수 있습니다. ",
"_____no_output_____"
]
],
[
[
"scheduler.start()",
"===== Polling Inference Scheduler Status =====\n\nScheduler Status: PENDING\nScheduler Status: RUNNING\n\n===== End of Polling Inference Scheduler Status =====\n"
]
],
[
[
"### 추론 스케줄러 삭제하기\n더 이상 사용하지 않는, **중지된** 스케줄러를 삭제할 수 있습니다. 모델 당 하나의 스케줄러만 가질 수 있습니다. ",
"_____no_output_____"
]
],
[
[
"scheduler.stop()\nscheduler.delete()",
"===== Polling Inference Scheduler Status =====\n\nScheduler Status: STOPPING\nScheduler Status: STOPPED\n\n===== End of Polling Inference Scheduler Status =====\n"
]
],
[
[
"## 결론\n---\n이 노트북에서는 노트북 시리즈 파트 3에서 만든 모델을 사용하여 스케줄러를 구성하고 몇 차례 추론을 실행한 다음 예측값을 얻었습니다.",
"_____no_output_____"
]
]
] | [
"markdown",
"code",
"markdown",
"code",
"markdown",
"code",
"markdown",
"code",
"markdown",
"code",
"markdown",
"code",
"markdown",
"code",
"markdown",
"code",
"markdown",
"code",
"markdown",
"code",
"markdown",
"code",
"markdown",
"code",
"markdown",
"code",
"markdown",
"code",
"markdown"
] | [
[
"markdown"
],
[
"code"
],
[
"markdown"
],
[
"code",
"code",
"code"
],
[
"markdown"
],
[
"code"
],
[
"markdown",
"markdown"
],
[
"code"
],
[
"markdown"
],
[
"code"
],
[
"markdown"
],
[
"code"
],
[
"markdown"
],
[
"code"
],
[
"markdown"
],
[
"code"
],
[
"markdown"
],
[
"code"
],
[
"markdown",
"markdown",
"markdown",
"markdown"
],
[
"code"
],
[
"markdown",
"markdown",
"markdown",
"markdown"
],
[
"code"
],
[
"markdown"
],
[
"code"
],
[
"markdown"
],
[
"code"
],
[
"markdown"
],
[
"code"
],
[
"markdown"
]
] |
d0907abe9ab7b665aaf47f0c6cfa9c5e713e2ff9 | 6,832 | ipynb | Jupyter Notebook | examples/python/pipelines/rgbd_integration.ipynb | aaronlhe/Open3D | 9ac70826e7fb21ddbe046c27b120532eb6355d42 | [
"MIT"
] | 3,673 | 2019-04-06T05:35:43.000Z | 2021-07-27T14:53:14.000Z | examples/python/pipelines/rgbd_integration.ipynb | vic-w/open3d_clone | 17b0e6c2b214ebfb3a7bcd30c72d8a00bdfd5e4d | [
"MIT"
] | 2,904 | 2019-04-06T06:51:22.000Z | 2021-07-27T13:49:54.000Z | examples/python/pipelines/rgbd_integration.ipynb | vic-w/open3d_clone | 17b0e6c2b214ebfb3a7bcd30c72d8a00bdfd5e4d | [
"MIT"
] | 1,127 | 2019-04-06T09:39:17.000Z | 2021-07-27T03:06:49.000Z | 36.148148 | 690 | 0.572453 | [
[
[
"import open3d as o3d\nimport numpy as np\nimport os\nimport sys\n\n# monkey patches visualization and provides helpers to load geometries\nsys.path.append('..')\nimport open3d_tutorial as o3dtut\n# change to True if you want to interact with the visualization windows\no3dtut.interactive = not \"CI\" in os.environ",
"_____no_output_____"
]
],
[
[
"# RGBD integration\nOpen3D implements a scalable RGBD image integration algorithm. The algorithm is based on the technique presented in [\\[Curless1996\\]](../reference.html#curless1996) and [\\[Newcombe2011\\]](../reference.html#newcombe2011). In order to support large scenes, we use a hierarchical hashing structure introduced in [Integrater in ElasticReconstruction](https://github.com/qianyizh/ElasticReconstruction/tree/master/Integrate).",
"_____no_output_____"
],
[
"## Read trajectory from .log file\nThis tutorial uses the function `read_trajectory` to read a camera trajectory from a [.log file](http://redwood-data.org/indoor/fileformat.html). A sample `.log` file is as follows.\n\n```\n# examples/test_data/RGBD/odometry.log\n0 0 1\n1 0 0 2\n0 1 0 2\n0 0 1 -0.3\n0 0 0 1\n1 1 2\n0.999988 3.08668e-005 0.0049181 1.99962\n-8.84184e-005 0.999932 0.0117022 1.97704\n-0.0049174 -0.0117024 0.999919 -0.300486\n0 0 0 1\n```",
"_____no_output_____"
]
],
[
[
"class CameraPose:\n\n def __init__(self, meta, mat):\n self.metadata = meta\n self.pose = mat\n\n def __str__(self):\n return 'Metadata : ' + ' '.join(map(str, self.metadata)) + '\\n' + \\\n \"Pose : \" + \"\\n\" + np.array_str(self.pose)\n\n\ndef read_trajectory(filename):\n traj = []\n with open(filename, 'r') as f:\n metastr = f.readline()\n while metastr:\n metadata = list(map(int, metastr.split()))\n mat = np.zeros(shape=(4, 4))\n for i in range(4):\n matstr = f.readline()\n mat[i, :] = np.fromstring(matstr, dtype=float, sep=' \\t')\n traj.append(CameraPose(metadata, mat))\n metastr = f.readline()\n return traj",
"_____no_output_____"
],
[
"camera_poses = read_trajectory(\"../../test_data/RGBD/odometry.log\")",
"_____no_output_____"
]
],
[
[
"## TSDF volume integration\nOpen3D provides two types of TSDF volumes: `UniformTSDFVolume` and `ScalableTSDFVolume`. The latter is recommended since it uses a hierarchical structure and thus supports larger scenes.\n\n`ScalableTSDFVolume` has several parameters. `voxel_length = 4.0 / 512.0` means a single voxel size for TSDF volume is $\\frac{4.0\\mathrm{m}}{512.0} = 7.8125\\mathrm{mm}$. Lowering this value makes a high-resolution TSDF volume, but the integration result can be susceptible to depth noise. `sdf_trunc = 0.04` specifies the truncation value for the signed distance function (SDF). When `color_type = TSDFVolumeColorType.RGB8`, 8 bit RGB color is also integrated as part of the TSDF volume. Float type intensity can be integrated with `color_type = TSDFVolumeColorType.Gray32` and `convert_rgb_to_intensity = True`. The color integration is inspired by [PCL](http://pointclouds.org/).",
"_____no_output_____"
]
],
[
[
"volume = o3d.pipelines.integration.ScalableTSDFVolume(\n voxel_length=4.0 / 512.0,\n sdf_trunc=0.04,\n color_type=o3d.pipelines.integration.TSDFVolumeColorType.RGB8)\n\nfor i in range(len(camera_poses)):\n print(\"Integrate {:d}-th image into the volume.\".format(i))\n color = o3d.io.read_image(\"../../test_data/RGBD/color/{:05d}.jpg\".format(i))\n depth = o3d.io.read_image(\"../../test_data/RGBD/depth/{:05d}.png\".format(i))\n rgbd = o3d.geometry.RGBDImage.create_from_color_and_depth(\n color, depth, depth_trunc=4.0, convert_rgb_to_intensity=False)\n volume.integrate(\n rgbd,\n o3d.camera.PinholeCameraIntrinsic(\n o3d.camera.PinholeCameraIntrinsicParameters.PrimeSenseDefault),\n np.linalg.inv(camera_poses[i].pose))",
"_____no_output_____"
]
],
[
[
"## Extract a mesh\nMesh extraction uses the marching cubes algorithm [\\[LorensenAndCline1987\\]](../reference.html#lorensenandcline1987).",
"_____no_output_____"
]
],
[
[
"print(\"Extract a triangle mesh from the volume and visualize it.\")\nmesh = volume.extract_triangle_mesh()\nmesh.compute_vertex_normals()\no3d.visualization.draw_geometries([mesh],\n front=[0.5297, -0.1873, -0.8272],\n lookat=[2.0712, 2.0312, 1.7251],\n up=[-0.0558, -0.9809, 0.1864],\n zoom=0.47)",
"_____no_output_____"
]
],
[
[
"<div class=\"alert alert-info\">\n \n**Note:**\n\nTSDF volume works like a weighted average filter in 3D space. If more frames are integrated, the volume produces a smoother and nicer mesh. Please check [Make fragments](../reconstruction_system/make_fragments.rst) for more examples.\n\n</div>",
"_____no_output_____"
]
]
] | [
"code",
"markdown",
"code",
"markdown",
"code",
"markdown",
"code",
"markdown"
] | [
[
"code"
],
[
"markdown",
"markdown"
],
[
"code",
"code"
],
[
"markdown"
],
[
"code"
],
[
"markdown"
],
[
"code"
],
[
"markdown"
]
] |
d090958e75c2ddf812362d9d040be200be7e4385 | 2,285 | ipynb | Jupyter Notebook | examples/dicts.ipynb | vincentxavier/nbtutor | c1da217c44a84bd13b190c72eb0ef1ba66bbc90a | [
"BSD-3-Clause"
] | 423 | 2016-11-24T12:51:16.000Z | 2022-03-09T13:11:16.000Z | examples/dicts.ipynb | vincentxavier/nbtutor | c1da217c44a84bd13b190c72eb0ef1ba66bbc90a | [
"BSD-3-Clause"
] | 52 | 2016-11-20T12:40:46.000Z | 2022-03-31T17:48:43.000Z | examples/dicts.ipynb | vincentxavier/nbtutor | c1da217c44a84bd13b190c72eb0ef1ba66bbc90a | [
"BSD-3-Clause"
] | 41 | 2017-01-18T15:11:01.000Z | 2022-03-09T13:07:35.000Z | 17.713178 | 34 | 0.396499 | [
[
[
"%reload_ext nbtutor",
"_____no_output_____"
],
[
"%%nbtutor -r -f\nfoo = {\n \"one\": 10,\n \"two\": \"Hi\",\n \"three\": [1, 2, 3]\n}\nbar = 10\npass",
"_____no_output_____"
],
[
"%%nbtutor -r -f\nfoo = {\n 1: 10,\n 2: \"Hi\",\n 3: [1, 2, 3]\n}\nbar = 10\npass",
"_____no_output_____"
],
[
"%%nbtutor -r -f\nfoo = {\n \"one\": 10,\n 2: \"Hi\",\n True: [1, 2, 3],\n None: 100,\n}\nbar = 10\npass",
"_____no_output_____"
],
[
"%%nbtutor -r -f\nfoo = {\n \"one\": 10,\n (1, 2): \"Hi\",\n 3: [1, 2, 3],\n None: 100,\n}\nbar = 10\npass",
"_____no_output_____"
],
[
"%%nbtutor -r -f\nfoo = {\n \"one\": 10,\n (1, 2): \"Hi\",\n 3: list(range(100)),\n None: 100,\n 'a': 1,\n 'b': 1,\n 'c': 1,\n}\nbar = 10",
"_____no_output_____"
]
]
] | [
"code"
] | [
[
"code",
"code",
"code",
"code",
"code",
"code"
]
] |
d0909dee5f4e4ffc390871799065a54c4f1c3219 | 10,054 | ipynb | Jupyter Notebook | .ipynb_checkpoints/Test-checkpoint.ipynb | DawoodBhai420/Hand-Written-Digit-Recognizer-using-MLP | 7e7b7277a99813acc7cfd2e69f3db5ac247b31c1 | [
"MIT"
] | null | null | null | .ipynb_checkpoints/Test-checkpoint.ipynb | DawoodBhai420/Hand-Written-Digit-Recognizer-using-MLP | 7e7b7277a99813acc7cfd2e69f3db5ac247b31c1 | [
"MIT"
] | null | null | null | .ipynb_checkpoints/Test-checkpoint.ipynb | DawoodBhai420/Hand-Written-Digit-Recognizer-using-MLP | 7e7b7277a99813acc7cfd2e69f3db5ac247b31c1 | [
"MIT"
] | null | null | null | 99.544554 | 2,068 | 0.682415 | [
[
[
"import numpy as np\nimport torch\nimport torch.nn as nn\nimport torch.nn.functional as F\nfrom torchvision import datasets,transforms\nfrom tqdm.notebook import tqdm\n\ntraindata = datasets.MNIST(root=\"./dataset\", train=True, transform=transforms.ToTensor, download=False)\ntestdata = datasets.MNIST(root=\"./dataset\", train=False, transform=transforms.ToTensor, download=False)\n\ntrain_loader = torch.utils.data.DataLoader(traindata, batch_size=100, shuffle=True)\ntest_loader = torch.utils.data.DataLoader(testdata, batch_size=100, shuffle=False)\n\nbatches = iter(trainloader)\n\nmodel1 = nn.Linear(784,500)\nmodel2 = nn.Linear(500,10)\n\noptimizer = torch.optim.SGD(model1.parameters(), lr=0.01)\n\nfor images,labels in tqdm(batches):\n optimizer.zero_grad()\n x = images.view(-1,784)\n z = F.relu(model1(x))\n y = F.relu(model2(z))\n loss = nn.CrossEntropyLoss(y,labels)\n loss.backward\n optimizer.step()",
"_____no_output_____"
]
]
] | [
"code"
] | [
[
"code"
]
] |
d090b3abbaa0fca3bc305041a5756032d31add62 | 8,138 | ipynb | Jupyter Notebook | DASH/.ipynb_checkpoints/Results_DASH-checkpoint.ipynb | adamamiller/supernova-spectrum-analysis | 1f7816bdc7dadb1a9a2ee3a97a1f77dd6f0c06dd | [
"MIT"
] | null | null | null | DASH/.ipynb_checkpoints/Results_DASH-checkpoint.ipynb | adamamiller/supernova-spectrum-analysis | 1f7816bdc7dadb1a9a2ee3a97a1f77dd6f0c06dd | [
"MIT"
] | null | null | null | DASH/.ipynb_checkpoints/Results_DASH-checkpoint.ipynb | adamamiller/supernova-spectrum-analysis | 1f7816bdc7dadb1a9a2ee3a97a1f77dd6f0c06dd | [
"MIT"
] | 2 | 2020-10-07T20:10:30.000Z | 2021-05-09T23:16:36.000Z | 25.117284 | 257 | 0.463996 | [
[
[
"import astrodash",
"WARNING:tensorflow:From /home/hallflower/anaconda3/lib/python3.7/site-packages/tensorflow/python/compat/v2_compat.py:96: disable_resource_variables (from tensorflow.python.ops.variable_scope) is deprecated and will be removed in a future version.\nInstructions for updating:\nnon-resource variables are not supported in the long term\n"
],
[
"import os\nimport astropy\nimport numpy as np\nfrom astropy.table import Table\nfrom astropy.table import Column\nimport glob\nimport matplotlib.pyplot as plt\nimport pandas as pd\nfrom collections import Counter\nfrom mpl_toolkits.mplot3d import Axes3D",
"_____no_output_____"
],
[
"sample_location = \"/home/hallflower/sample/spectra/\"\ndash = \"/mnt/c/users/20xha/Documents/Caltech/Research/DASH/\"",
"_____no_output_____"
],
[
"SEDM_ML_sample = Table.read(\"/mnt/c/Users/20xha/Documents/Caltech/Research/SEDM_ML_sample.ascii\", format = \"ascii\")\nSEDM_ML_sample.rename_column('col1', 'ZTF_Name')\nSEDM_ML_sample.rename_column('col2', \"Class\")\nSEDM_ML_sample.rename_column('col8', \"Version\")",
"_____no_output_____"
],
[
"output_list = np.load(dash+\"output.npy\",allow_pickle=True)",
"_____no_output_____"
],
[
"len(output_list)",
"_____no_output_____"
],
[
"len(np.unique(SEDM_ML_sample[\"ZTF_Name\"]))",
"_____no_output_____"
],
[
"np.asarray(output_list[0][2])[:,0]",
"_____no_output_____"
],
[
"Classification = Table(\n names=(\"ZTF_Name\", \"Class\", \"Version\"\n ),\n meta={\"name\": \"Basic ZTF Name Data\"},\n dtype=(\"U64\", \"U64\", \"U64\"\n )\n )\nfor i in np.unique(SEDM_ML_sample[\"ZTF_Name\"]):\n row = SEDM_ML_sample[\"ZTF_Name\", \"Class\", \"Version\"][np.where(i == SEDM_ML_sample[\"ZTF_Name\"])][-1]\n Classification.add_row(row)",
"_____no_output_____"
],
[
"count = 0\nResultsTable = Table(\n names=(\"ZTF_Name\", \"Both\"\n ),\n meta={\"name\": \"Spectrum Results after SNID\"},\n dtype=(\"U64\", \"U64\"\n )\n )\n\nfor i in output_list:\n row = []\n row.append(i[-1])\n best = np.asarray(i[2])[:,0]\n c = Counter(best)\n row.append(c.most_common()[0][0])\n ResultsTable.add_row(row)\n\n count += 1\n if(count % 500 == 0):\n print(count)",
"500\n1000\n1500\n2000\n2500\n"
],
[
"counter = 0\nwrong = []\nJoinedResults = astropy.table.join(ResultsTable, Classification)\nfor j in JoinedResults:\n if(j[\"Class\"] != '-' and j[\"Class\"] != \"0.0\"):\n correct_1a = \"Ia\" in j[\"Class\"]\n classified_1a = \"Ia\" in j[\"Both\"]\n if(correct_1a==classified_1a):\n counter += 1\n else:\n wrong.append([j[\"ZTF_Name\"], j[\"Class\"], j[\"Both\"]])\nwrong = np.asarray(wrong)",
"WARNING: MergeConflictWarning: Cannot merge meta key 'name' types <class 'str'> and <class 'str'>, choosing name='Basic ZTF Name Data' [astropy.utils.metadata]\n"
],
[
"ranges = np.linspace(0, 25, 26)",
"_____no_output_____"
],
[
"ResultsTable_List_both = []\ncount = 0\n\nfor rlap in ranges:\n for agree in range(0,16):\n ResultsTable = Table(\n names=(\"ZTF_Name\", \"Both\"\n ),\n meta={\"name\": \"Spectrum Results after SNID\"},\n dtype=(\"U64\", \"U64\"\n )\n )\n for j in output_list:\n row = []\n row.append(j[-1])\n matches = []\n best_rlap = np.max(j[0][:,0][:,3])\n if(best_rlap > rlap)\n for k in range(len(j[0])):\n matches.extend(j[0][k])\n matches = np.asarray(matches)\n c = Counter(matches[:,1])\n row.append(c.most_common()[0][0])\n if(c.most_common()[0][1] >= agree):\n row.append(c.most_common()[0][0])\n ResultsTable.add_row(row)\n\n count += 1\n if(len(ResultsTable) != 0):\n ResultsTable_List_both.append([rlap,agree,ResultsTable])\n if(count % 10 == 0):\n print(count)",
"_____no_output_____"
],
[
"j = output_list[0]",
"_____no_output_____"
],
[
"matches = []\nfor k in range(len(j[0])):\n matches.extend(j[0][k])\nmatches = np.asarray(matches)",
"_____no_output_____"
]
]
] | [
"code"
] | [
[
"code",
"code",
"code",
"code",
"code",
"code",
"code",
"code",
"code",
"code",
"code",
"code",
"code",
"code",
"code"
]
] |
d090bd79d3d34718c224a486c7e5cc5188401ccd | 12,277 | ipynb | Jupyter Notebook | arrow_performance_comparison_notebook.ipynb | passionbytes/arrowexp | 2e1db07ca025c5d98f938554aa4c1aa348bc84dc | [
"Apache-2.0"
] | null | null | null | arrow_performance_comparison_notebook.ipynb | passionbytes/arrowexp | 2e1db07ca025c5d98f938554aa4c1aa348bc84dc | [
"Apache-2.0"
] | 2 | 2020-07-11T07:25:21.000Z | 2020-07-12T03:54:01.000Z | .ipynb_checkpoints/arrow_performance_comparison_notebook-checkpoint.ipynb | passionbytes/arrowexp | 2e1db07ca025c5d98f938554aa4c1aa348bc84dc | [
"Apache-2.0"
] | 2 | 2020-07-11T05:07:52.000Z | 2020-07-12T02:05:58.000Z | 27.04185 | 188 | 0.549401 | [
[
[
"# Apache Arrow",
"_____no_output_____"
],
[
"## 1 Compare performance of csv, Parquet and Arrow - 1 Change",
"_____no_output_____"
]
],
[
[
"import pyarrow.parquet as pq\nimport pyarrow as pa\nimport pandas as pd\nimport numpy as np\nimport os\nimport psutil",
"_____no_output_____"
]
],
[
[
"### 1.1 Load and prepare data One more change",
"_____no_output_____"
]
],
[
[
"## Read Palmer Station Penguin dataset from GitHub\ndf = pd.read_csv(\"https://raw.githubusercontent.com/allisonhorst/\"\n \"palmerpenguins/47a3476d2147080e7ceccef4cf70105c808f2cbf/\"\n \"data-raw/penguins_raw.csv\")",
"_____no_output_____"
],
[
"# Increase dataset to 1m rows and reset index\ndf = df.sample(1_000_000, replace=True).reset_index(drop=True)\n\n\n# Update sample number (0 to 999'999)\ndf[\"Sample Number\"] = df.index\n# Add some random variation to numeric columns\ndf[[\"Culmen Length (mm)\", \"Culmen Depth (mm)\", \n \"Flipper Length (mm)\", \"Body Mass (g)\"]] = df[[\"Culmen Length (mm)\", \"Culmen Depth (mm)\", \n \"Flipper Length (mm)\", \"Body Mass (g)\"]] \\\n + np.random.rand(df.shape[0], 4)\n\n# Create dataframe where missing numeric values are filled with zero\ndf_nonan = df.copy()\ndf_nonan[[\"Culmen Length (mm)\", \"Culmen Depth (mm)\", \n \"Flipper Length (mm)\", \"Body Mass (g)\"]] = df[[\"Culmen Length (mm)\", \"Culmen Depth (mm)\", \n \"Flipper Length (mm)\", \"Body Mass (g)\"]].fillna(0)",
"_____no_output_____"
]
],
[
[
"### 1.2 Write to disk ",
"_____no_output_____"
]
],
[
[
"# Write to csv\ndf.to_csv(\"penguin-dataset.csv\")\n\n# Write to parquet\ndf.to_parquet(\"penguin-dataset.parquet\")\n\ncontext = pa.default_serialization_context()\n\n# Write to Arrow\n# Convert from pandas to Arrow\ntable = pa.Table.from_pandas(df)\n# Write out to file\n\nwriter = pa.RecordBatchFileWriter('penguin-dataset.arrow', table.schema)\nwriter.write(table)\nwriter.close()\n#with pa.OSFile('penguin-dataset.arrow', 'wb') as sink:\n #with pa.RecordBatchFileWriter(sink, table.schema,write_legacy_format=True) as writer:\n #writer.write_table(table)\n\n# Convert from no-NaN pandas to Arrow\ntable_nonan = pa.Table.from_pandas(df_nonan)\n# Write out to file\nwriter = pa.RecordBatchFileWriter('penguin-dataset-nonan.arrow', table.schema)\nwriter.write(table_nonan)\nwriter.close()\n#with pa.OSFile('penguin-dataset-nonan.arrow', 'wb') as sink:\n #with pa.RecordBatchFileWriter(sink, table_nonan.schema,write_legacy_format=True) as writer:\n #writer.write_table(table_nonan)",
"_____no_output_____"
]
],
[
[
"### 1.3 Reading time - calculate average of numeric column",
"_____no_output_____"
],
[
"#### 1.3.1 Read csv and calculate mean",
"_____no_output_____"
]
],
[
[
"%%timeit\npd.read_csv(\"penguin-dataset.csv\")[\"Flipper Length (mm)\"].mean()",
"3.4 s ± 105 ms per loop (mean ± std. dev. of 7 runs, 1 loop each)\n"
]
],
[
[
"#### 1.3.2 Read parquet and calculate mean",
"_____no_output_____"
]
],
[
[
"%%timeit\npd.read_parquet(\"penguin-dataset.parquet\", columns=[\"Flipper Length (mm)\"]).mean()",
"/Users/ravishankarnair/anaconda3/envs/py36/lib/python3.6/site-packages/pyarrow/pandas_compat.py:708: FutureWarning: .labels was deprecated in version 0.24.0. Use .codes instead.\n labels = getattr(columns, 'labels', None) or [\n/Users/ravishankarnair/anaconda3/envs/py36/lib/python3.6/site-packages/pyarrow/pandas_compat.py:735: FutureWarning: the 'labels' keyword is deprecated, use 'codes' instead\n return pd.MultiIndex(levels=new_levels, labels=labels, names=columns.names)\n/Users/ravishankarnair/anaconda3/envs/py36/lib/python3.6/site-packages/pyarrow/pandas_compat.py:752: FutureWarning: .labels was deprecated in version 0.24.0. Use .codes instead.\n labels, = index.labels\n"
]
],
[
[
"#### 1.3.3 Read Arrow using file API",
"_____no_output_____"
]
],
[
[
"%%timeit\nwith pa.OSFile('penguin-dataset.arrow', 'rb') as source:\n table = pa.ipc.open_file(source).read_all().column(\"Flipper Length (mm)\")\nresult = table.to_pandas().mean()",
"133 ms ± 2.73 ms per loop (mean ± std. dev. of 7 runs, 10 loops each)\n"
]
],
[
[
"#### 1.3.4 Read Arrow with memory-mapped API with missing values",
"_____no_output_____"
]
],
[
[
"%%timeit\nsource = pa.memory_map('penguin-dataset.arrow', 'r')\ntable = pa.ipc.RecordBatchFileReader(source).read_all().column(\"Flipper Length (mm)\")\nresult = table.to_pandas().mean()",
"6.19 ms ± 82.5 µs per loop (mean ± std. dev. of 7 runs, 100 loops each)\n"
]
],
[
[
"#### 1.3.5 Read Arrow with memory-mapped API without missing values (zero-copy)",
"_____no_output_____"
]
],
[
[
"%%timeit\nsource = pa.memory_map('penguin-dataset-nonan.arrow', 'r')\ntable = pa.ipc.RecordBatchFileReader(source).read_all().column(\"Flipper Length (mm)\")\nresult = table.to_pandas().mean()",
"4.04 ms ± 80.4 µs per loop (mean ± std. dev. of 7 runs, 100 loops each)\n"
]
],
[
[
"### 1.4 Memory consumption - read column",
"_____no_output_____"
]
],
[
[
"# Measure initial memory consumption\nmemory_init = psutil.Process(os.getpid()).memory_info().rss >> 20",
"_____no_output_____"
]
],
[
[
"#### 1.4.1 Read csv",
"_____no_output_____"
]
],
[
[
"col_csv = pd.read_csv(\"penguin-dataset.csv\")[\"Flipper Length (mm)\"]\nmemory_post_csv = psutil.Process(os.getpid()).memory_info().rss >> 20",
"_____no_output_____"
]
],
[
[
"#### 1.4.2 Read parquet",
"_____no_output_____"
]
],
[
[
"col_parquet = pd.read_parquet(\"penguin-dataset.parquet\", columns=[\"Flipper Length (mm)\"])\nmemory_post_parquet = psutil.Process(os.getpid()).memory_info().rss >> 20",
"_____no_output_____"
]
],
[
[
"#### 1.4.3 Read Arrow using file API",
"_____no_output_____"
]
],
[
[
"with pa.OSFile('penguin-dataset.arrow', 'rb') as source:\n col_arrow_file = pa.ipc.open_file(source).read_all().column(\"Flipper Length (mm)\").to_pandas()\nmemory_post_arrowos = psutil.Process(os.getpid()).memory_info().rss >> 20",
"_____no_output_____"
]
],
[
[
"#### 1.4.4 Read Arrow with memory-mapped API with missing values",
"_____no_output_____"
]
],
[
[
"source = pa.memory_map('penguin-dataset.arrow', 'r')\ntable_mmap = pa.ipc.RecordBatchFileReader(source).read_all().column(\"Flipper Length (mm)\")\ncol_arrow_mapped = table_mmap.to_pandas()\nmemory_post_arrowmmap = psutil.Process(os.getpid()).memory_info().rss >> 20",
"_____no_output_____"
]
],
[
[
"#### 1.4.5 Read Arrow with memory-mapped API without missing values (zero-copy)",
"_____no_output_____"
]
],
[
[
"source = pa.memory_map('penguin-dataset-nonan.arrow', 'r')\ntable_mmap_zc = pa.ipc.RecordBatchFileReader(source).read_all().column(\"Flipper Length (mm)\")\ncol_arrow_mapped_zc = table_mmap_zc.to_pandas()\nmemory_post_arrowmmap_zc = psutil.Process(os.getpid()).memory_info().rss >> 20",
"_____no_output_____"
]
],
[
[
"#### 1.4.6 Display memory consupmtion",
"_____no_output_____"
]
],
[
[
"# Print memory consumption\nprint(f\"csv: {memory_post_csv - memory_init}\\n\"\n f\"Parquet: {memory_post_parquet - memory_post_csv}\\n\"\n f\"Arrow file API: {memory_post_arrowos - memory_post_parquet}\\n\"\n f\"Arrow memory-mapped API with NaNs: {memory_post_arrowmmap - memory_post_arrowos}\\n\"\n f\"Arrow memory-mapped API (zero-copy): {memory_post_arrowmmap_zc - memory_post_arrowmmap}\\n\")",
"csv: 223\nParquet: -4\nArrow file API: -8\nArrow memory-mapped API with NaNs: 8\nArrow memory-mapped API (zero-copy): 0\n\n"
]
]
] | [
"markdown",
"code",
"markdown",
"code",
"markdown",
"code",
"markdown",
"code",
"markdown",
"code",
"markdown",
"code",
"markdown",
"code",
"markdown",
"code",
"markdown",
"code",
"markdown",
"code",
"markdown",
"code",
"markdown",
"code",
"markdown",
"code",
"markdown",
"code",
"markdown",
"code"
] | [
[
"markdown",
"markdown"
],
[
"code"
],
[
"markdown"
],
[
"code",
"code"
],
[
"markdown"
],
[
"code"
],
[
"markdown",
"markdown"
],
[
"code"
],
[
"markdown"
],
[
"code"
],
[
"markdown"
],
[
"code"
],
[
"markdown"
],
[
"code"
],
[
"markdown"
],
[
"code"
],
[
"markdown"
],
[
"code"
],
[
"markdown"
],
[
"code"
],
[
"markdown"
],
[
"code"
],
[
"markdown"
],
[
"code"
],
[
"markdown"
],
[
"code"
],
[
"markdown"
],
[
"code"
],
[
"markdown"
],
[
"code"
]
] |
d090ce712197ba24dd212228b9073993781aa1cd | 45,912 | ipynb | Jupyter Notebook | 02.ipynb | 2018pycpp/18pycpp-04 | 144a86e83bbc7ca45c03a894b923430c406a14fc | [
"BSD-3-Clause"
] | null | null | null | 02.ipynb | 2018pycpp/18pycpp-04 | 144a86e83bbc7ca45c03a894b923430c406a14fc | [
"BSD-3-Clause"
] | 3 | 2018-10-26T12:38:02.000Z | 2018-11-16T08:33:58.000Z | 02.ipynb | kangwonlee/18pycpp-04 | 144a86e83bbc7ca45c03a894b923430c406a14fc | [
"BSD-3-Clause"
] | null | null | null | 30.875588 | 148 | 0.446659 | [
[
[
"# Controlling accesss to attributes",
"_____no_output_____"
],
[
"* Following blocks are one possible implementation of vectors of `double`s.",
"_____no_output_____"
],
[
"* Here, member variable `new_name` is in `protected:` part.\n* Member methods and subclass members can access this variable but from the outside of the class, we cannot access it.\n* We call it **encapsulation**; instead of directly reading or writing to the variable, we would use mutator or reader **methods**.\n* This is because to modularize software components to the level of integrated circuit chips.",
"_____no_output_____"
],
[
"``` C++\n// Begin vector_double.h\n\n#include <cassert>\n#include <cstdint>\n#include <exception>\n#include <iostream>\n#include <string>\n#include <vector>\n\n// This directive would activate method call logging\n#ifndef LOG\n#define LOG\n#endif\n\n// This directive woudl activate bracket [] operator logging\n// Added this just because the examples call [] operator frequently\n#ifndef LOGBRACKET\n// #define LOGBRACKET\n#endif\n\n// This is to prevent declaring vector class twice\n// If declared twice, C/C++ compilers would show an error message\n#ifndef VECTOR_DOUBLE\n#define VECTOR_DOUBLE\n\nclass RowVector \n{\n // automatic allocation\n // https://stackoverflow.com/questions/8553464/vector-as-a-class-member\n std::vector<double> columns;\n\n protected:\n // To distinguish vectors from each other\n std::string name;\n\n public:\n // Default constructor\n\t\tRowVector();\n\n // Destructor\n ~ RowVector();\n\n // Default arguments\n // If the function could not find the argument in the call, it uses the default value.\n RowVector(const uint32_t n, const double *values=NULL, std::string new_name=\"None\");\n\n // Whenever possible, it is advisible to use `const` keyword\n // Protects data from being overwritten and may optimize further\n RowVector(const uint32_t n, std::string new_name=\"None\");\n\n // Copy constructor must use a reference.\n // What would happen otherwise?\n RowVector(const RowVector & other);\n\n // Two versions of [] operators\n // This one is for normal vectors. Allows changing values\n double & operator [] (const uint32_t i);\n\n // This one is for constant vectors. Protects the values from overwriting\n double operator [] (const uint32_t i) const;\n\n const std::string get_name() const;\n\n RowVector operator + (const RowVector & other);\n\n RowVector operator * (const double a);\n\n const double operator * (const RowVector & other);\n\n void show();\n\n void resize(std::size_t new_size);\n\n std::size_t size() const noexcept;\n\n RowVector & operator += (const RowVector & other);\n\n RowVector & operator *= (const double a);\n};\n\n#endif\n// End vector_double.h\n\n```",
"_____no_output_____"
],
[
"``` C++\n// Begin vector_double.cpp\n\n#include <cassert>\n#include <cstdint>\n#include <exception>\n#include <iostream>\n#include <string>\n#include <vector>\n\n#include \"vector_double.h\"\n\n\nRowVector::RowVector(){\n// This may look involving but sometimes helps how the program works.\n#ifdef LOG\n std::cout << '[' << &columns << ']' << \"RowVector()\" << '\\n';\n#endif\n name = \"None\";\n}\n\n\nRowVector::~ RowVector(){\n#ifdef LOG\n std::cout << '[' << &columns << ']' << \"~ RowVector()\" << '\\n';\n#endif\n}\n\n\nRowVector::RowVector(const uint32_t n, const double *values, std::string new_name){\n#ifdef LOG\n std::cout << '[' << &columns << ']' \n << \"RowVector(\" << n << \", \" << values << \", \" << new_name << \")\\n\";\n#endif\n columns.resize(n);\n\n // If initial values available, copy\n if (values){\n for (uint32_t i = 0; columns.size() > i; ++i){\n columns[i] = values[i];\n }\n }\n // If no initial values, set all values zero\n else{\n for (uint32_t i = 0; columns.size() > i; ++i){\n columns[i] = 0.0;\n }\n }\n\n name = new_name;\n}\n\n\n// Instead of implementing another constructor, calling an existing one\n// c++ 11 or later\nRowVector::RowVector(const uint32_t n, std::string new_name) : RowVector(n, NULL, new_name){\n#ifdef LOG\n std::cout << '[' << &columns << ']' << \"RowVector(\" << n << \", \" << new_name << \")\\n\";\n#endif\n}\n\n\nRowVector::RowVector(const RowVector & other){\n#ifdef LOG\n std::cout << '[' << &columns << ']' << \"RowVector(\" << & other << \")\\n\";\n#endif\n // https://codereview.stackexchange.com/questions/149669/c-operator-overloading-for-matrix-operations-follow-up\n // http://www.cplusplus.com/reference/vector/vector/resize/\n columns.resize(other.columns.size());\n\n // element loop\n for(uint32_t i=0; columns.size() > i; ++i){\n columns[i] = other.columns[i];\n }\n\n // Copy name of the other one\n name = other.name;\n // Then append\n name.append(\"2\");\n}\n\n\ndouble & RowVector::operator [] (const uint32_t i){\n#ifdef LOGBRACKET\n std::cout << '[' << &columns << ']' << \"double & RowVector::operator [] (\" << i << \")\\n\";\n#endif\n // Return reference; otherwise, unable to assign\n return columns[i];\n}\n\ndouble RowVector::operator [] (const uint32_t i) const {\n#ifdef LOGBRACKET\n std::cout << '[' << &columns << ']' << \"double RowVector::operator [] (\" << i << \") const\\n\";\n#endif\n // Return reference; otherwise, unable to assign\n return columns[i];\n}\n\n\nconst std::string RowVector::get_name() const{\n#ifdef LOG\n std::cout << '[' << &columns << ']' << \"const std::string RowVector::get_name()\\n\";\n#endif\n // Return constant; to prevent change\n return name;\n}\n\n\nRowVector RowVector::operator + (const RowVector & other){\n#ifdef LOG\n std::cout << '[' << &columns << ']' << \"RowVector RowVector::operator + (\" << & other << \")\\n\";\n#endif\n // Check size\n assert(columns.size() == other.columns.size());\n\n // Make a new vector to return\n RowVector temp(other);\n\n // Element loop\n for (uint32_t i=0; columns.size() > i; ++i){\n temp[i] += columns[i];\n }\n\n // Returning a temporary image\n return temp;\n}\n\n\nRowVector RowVector::operator * (const double a){\n#ifdef LOG\n std::cout << '[' << &columns << ']' << \"RowVector RowVector::operator * (\" << a << \")\\n\";\n#endif\n\n // Make a new vector to return\n RowVector temp(*this);\n\n // Element loop in `for each` style\n // c++ 11 or later\n for (auto & element : temp.columns){\n element *= a;\n }\n\n // Returning a temporary image\n return temp;\n}\n\n\nconst double RowVector::operator * (const RowVector & other){\n#ifdef LOG\n std::cout << '[' << &columns << ']' << \"const double RowVector::operator * (\" << & other << \")\\n\";\n#endif\n\n // Check size\n assert(columns.size() == other.columns.size());\n\n double dot_product = 0.0;\n\n // Element loop\n for (uint32_t i = 0; columns.size() > i; ++i){\n dot_product += columns[i] * other.columns[i];\n }\n\n // Returning a temporary image\n return dot_product;\n}\n\n\nvoid RowVector::show(){\n#ifdef LOG\n std::cout << '[' << &columns << ']' << \"void RowVector::show()\\n\";\n#endif\n for (uint32_t i=0; columns.size()> i; ++i){\n std::cout << name << '[' << i << \"] = \" << columns[i] << '\\n';\n }\n}\n\n\nvoid RowVector::resize(std::size_t new_size){\n#ifdef LOG\n std::cout << '[' << &columns << ']' << \"void RowVector::resize(\" << new_size << \")\\n\";\n#endif\n columns.resize(new_size);\n}\n\n\nstd::size_t RowVector::size() const noexcept{\n#ifdef LOG\n std::cout << '[' << &columns << ']' << \"std::size_t RowVector::size() const noexcept\\n\";\n#endif\n return columns.size();\n}\n\n\nRowVector & RowVector::operator += (const RowVector & other) {\n#ifdef LOG\n std::cout << '[' << &columns << ']' << \"RowVector & RowVector::operator += (\" << & other << \")\\n\";\n#endif\n // https://stackoverflow.com/questions/4581961/c-how-to-overload-operator\n for (uint32_t i=0; size()>i; ++i){\n columns[i] += other[i];\n }\n return *this;\n}\n\n\nRowVector & RowVector::operator *= (const double a) {\n#ifdef LOG\n std::cout << '[' << &columns << ']' << \"RowVector & RowVector::operator *= (\" << a << \")\\n\";\n#endif\n // https://stackoverflow.com/questions/4581961/c-how-to-overload-operator\n for (uint32_t i=0; size()>i; ++i){\n columns[i] *= a;\n }\n return *this;\n}\n\n// End vector_double.cpp\n// Build command : g++ -Wall -g -std=c++14 vector_double.cpp -fsyntax-only\n\n```",
"_____no_output_____"
],
[
"``` C++\n// Begin cpp_vector_double_practice.cpp\n\n#include <cassert>\n#include <cstdint>\n#include <exception>\n#include <iostream>\n#include <string>\n#include <vector>\n\n#include \"vector_double.h\"\n\nint32_t main(int32_t argn, char *argv[]){\n\tdouble s[] = {1.0, 2.0};\n\n std::cout << \"RowVector row (2u, s, \\\"row\\\");\\n\";\n\tRowVector row (2u, s, \"row\");\n\n row.show();\n\n std::cout << \"RowVector another_row (row);\\n\";\n\tRowVector another_row (row);\n row.show();\n another_row.show();\n\n std::cout << \"another_row[1] += 0.5;\\n\";\n another_row[1] += 0.5;\n row.show();\n another_row.show();\n\n std::cout << \"RowVector row_plus_another(row + another_row);\\n\";\n RowVector row_plus_another(row + another_row);\n row.show();\n another_row.show();\n row_plus_another.show();\n\n std::cout << \"RowVector zeros(3);\\n\";\n\tRowVector zeros(3u, \"zeros\");\n row.show();\n another_row.show();\n row_plus_another.show();\n zeros.show();\n\n double t[] = {2.0, -1.0};\n\tRowVector ortho (2u, t, \"ortho\");\n double dot = row * ortho;\n std::cout << \"double dot = row * ortho;\\n\";\n std::cout << \"dot = \" << dot << '\\n';\n\n std::cout << \"dot = row * row;\\n\";\n dot = row * row;\n std::cout << \"dot = \" << dot << '\\n';\n\n}\n\n// End cpp_vector_double_practice.cpp\n// Build command : g++ -Wall -g -std=c++14 cpp_vector_double_practice.cpp vector_double.cpp -o cpp_vector_double_practice\n\n``` ",
"_____no_output_____"
],
[
"* In the mean while, following code blocks depict a possible implementation in python.",
"_____no_output_____"
]
],
[
[
"import collections\n\n\nclass Vector(collections.UserList):\n\n def __add__(self, other):\n\n # check size\n assert len(self) == len(other), f\"Lengths are different ({len(self)} == {len(other)})\"\n\n # trying list comprehension\n return Vector([a + b for a, b in zip(self, other)])\n\n def __radd__(self, other):\n # What is this?\n\n return self.__add__(other)\n\n def __mul__(self, other):\n # what is happening here?\n if isinstance(other, (int, float, complex)):\n result = Vector([a * other for a in self])\n elif isinstance(other, Vector):\n assert len(self) == len(other), f\"Lengths are different ({len(self)} == {len(other)})\"\n result = sum(a * b for a, b in zip(self, other))\n \n return result\n\n def __rmul__(self, other):\n return __mul__(self, other)\n \n def __str__(self):\n # How does the .join() work?\n return '\\n'.join(f\"{hex(id(self))}[{i}] = {self[i]}\" for i in range(len(self)))\n\n def __len__(self):\n return len(self.data)\n\n",
"_____no_output_____"
],
[
"print(\"a = Vector([1, 2])\")\na = Vector([1, 2])\nprint(a)\n\nprint(\"b = Vector(a)\")\nb = Vector(a)\nprint(a)\nprint(b)\n\nprint(\"b[1] += (-0.5)\")\nb[1] += (-0.5)\nprint(a)\nprint(b)\n\nprint(\"c = a + b\")\nc = a + b\nprint(a)\nprint(b)\nprint(c)\n\nprint(\"ortho = Vector([2, -1])\")\northo = Vector([2, -1])\nprint(a)\nprint(b)\nprint(c)\nprint(ortho)\n\nprint(\"dot = a * ortho\")\ndot = a * ortho\nprint(f\"a * ortho = {dot}\")\n\nprint(\"dot = a * a\")\ndot = a * a\nprint(f\"a * a = {dot}\")\n\n",
"_____no_output_____"
]
],
[
[
"# Matrix class example",
"_____no_output_____"
],
[
"## In C++",
"_____no_output_____"
],
[
"* Following code blocks present a possible implementation of matrix class in C++.\n* Please note that to build these files, `vector_double.h` and `vector_double.cpp` files are necessary.\n\n",
"_____no_output_____"
],
[
"```C++\n// Begin matrix_double.h\n\n#include <cassert>\n#include <cstdint>\n#include <exception>\n#include <iostream>\n#include <string>\n#include <vector>\n\n#include \"vector_double.h\"\n\n#ifndef MATRIX_DOUBLE\n#define MATRIX_DOUBLE\n\nclass Matrix\n{\n std::vector<RowVector> rows;\n\n protected:\n std::string name;\n\n public:\n\t\tMatrix();\n\n ~ Matrix();\n\n Matrix(const uint32_t m, const uint32_t n, const double *values, std::string new_name=\"None\");\n\n Matrix(const uint32_t m, const uint32_t n, std::string new_name=\"None\");\n\n Matrix(const Matrix & other, std::string new_name=\"\");\n\n Matrix(const RowVector & other, std::string new_name=\"\");\n\n RowVector & operator [] (const uint32_t i);\n\n const RowVector operator [] (const uint32_t i) const;\n\n const std::string get_name() const;\n\n Matrix operator + (const Matrix & other);\n\n Matrix operator * (const double a);\n\n RowVector operator * (const RowVector &v);\n\n Matrix operator * (const Matrix & other);\n\n void show();\n\n Matrix transpose();\n\n const size_t get_height() const;\n\n const size_t get_width() const;\n};\n\n#endif\n// End matrix_double.h\n```",
"_____no_output_____"
],
[
"``` C++\n// Begin matrix_double.cpp\n\n#include <cassert>\n#include <cstdint>\n#include <exception>\n#include <iostream>\n#include <string>\n#include <vector>\n\n#include \"vector_double.h\"\n#include \"matrix_double.h\"\n\n\nMatrix::Matrix(){\n#ifdef LOG\n std::cout << '[' << &rows << ']' << \"Matrix()\" << '\\n';\n#endif\n name = \"None\";\n}\n\n\nMatrix::~ Matrix(){\n#ifdef LOG\n std::cout << '[' << &rows << ']' << \"~ Matrix()\" << '\\n';\n#endif\n}\n\n\nMatrix::Matrix(const uint32_t m, const uint32_t n, const double *values, std::string new_name){\n#ifdef LOG\n std::cout << '[' << &rows << ']' \n << \"Matrix(\" << m << \", \"<< n << \", \" << values << \", \" << new_name << \")\\n\";\n#endif\n name = new_name;\n\n rows.resize(m);\n\n // If initial values available, copy\n if (values){\n // row loop\n for (uint32_t i = 0; m > i; ++i){\n rows[i].resize(n);\n // column loop\n for (uint32_t j = 0; n > j; ++j){\n rows[i][j] = *(values + i * n + j) ;\n }\n }\n }\n // If no initial values, set all values zero\n else{\n // row loop\n for (uint32_t i = 0; m > i; ++i){\n rows[i].resize(n);\n // column loop\n for (uint32_t j = 0; n > j; ++j){\n rows[i][j] = 0.0;\n }\n }\n }\n\n}\n\n// Instead of implementing another constructor, calling an existing one\n// c++ 11 or later\nMatrix::Matrix(const uint32_t m, const uint32_t n, std::string new_name) : Matrix(m, n, NULL, new_name){\n#ifdef LOG\n std::cout << '[' << &rows << ']' << \"Matrix(\" << m << \", \" << n << \", \" << new_name << \")\\n\";\n#endif\n}\n\n\nMatrix::Matrix(const Matrix & other, std::string new_name){\n#ifdef LOG\n std::cout << '[' << &rows << ']' << \"Matrix(\" << & other << \")\\n\";\n#endif\n // https://codereview.stackexchange.com/questions/149669/c-operator-overloading-for-matrix-operations-follow-up\n // http://www.cplusplus.com/reference/vector/vector/resize/\n rows.resize(other.rows.size());\n // row loop\n for(uint32_t i=0; rows.size() > i; ++i){\n rows[i].resize(other.rows[i].size());\n\n // column loop\n for(uint32_t j=0; other.rows[i].size() > j; ++j){\n // Another possibility is as follows\n // rows[i][j] = other.rows[i][j];\n // However for now the line above would create a temporary row vector\n // To avoid seemingly unnecessary such temporary object, \n // for now would use the following line\n rows[i][j] = other.rows[i][j];\n }\n\n }\n\n if (\"\" != new_name){\n name = new_name;\n }\n else{\n // Copy name of the other one\n name = other.name;\n // Then append\n name.append(\"2\");\n }\n}\n\n\nMatrix::Matrix(const RowVector & other, std::string new_name){\n // RowVector -> n x 1 matrix \n#ifdef LOG\n std::cout << '[' << &rows << ']' << \"Matrix(const RowVector &\" << & other << \")\\n\";\n#endif\n rows.resize(other.size());\n\n // row loop\n for(uint32_t i=0; rows.size() > i; ++i){\n rows[i].resize(1);\n rows[i][0] = other[0];\n }\n\n if (\"\" != new_name){\n name = new_name;\n }\n else{\n // Copy name of the other one\n name = other.get_name();\n // Then append\n name.append(\"2\");\n }\n}\n\n\nRowVector & Matrix::operator [] (const uint32_t i){\n#ifdef LOGBRACKET\n std::cout << '[' << &rows << ']' << \"RowVector & Matrix::operator [] (\" << i << \")\\n\";\n#endif\n // Return reference; otherwise, unable to assign\n return rows[i];\n}\n\nconst RowVector Matrix::operator [] (const uint32_t i) const {\n#ifdef LOGBRACKET\n std::cout << '[' << &rows << ']' << \"const RowVector Matrix::operator [] (\" << i << \")\\n\";\n#endif\n // Return reference; otherwise, unable to assign\n return rows[i];\n}\n\n\nconst std::string Matrix::get_name() const{\n#ifdef LOG\n std::cout << '[' << &rows << ']' << \"const std::string Matrix::get_name()\\n\";\n#endif\n // Return constant; to prevent change\n return name;\n}\n\n\nMatrix Matrix::operator + (const Matrix & other){\n#ifdef LOG\n std::cout << '[' << &rows << ']' << \"Matrix Matrix::operator + (\"<< & other <<\")\\n\";\n#endif\n // Check size\n assert(this->get_height() == other.get_height());\n assert(this->get_width() == other.get_width());\n\n#ifdef LOG\n std::cout << \"Matrix temp(other);\\n\";\n#endif\n // Make a new vector to return\n Matrix temp(other, get_name() + '+' + other.get_name());\n\n#ifdef LOG\n std::cout << \"Begin row loop\\n\";\n#endif\n // Row loop\n for (uint32_t i=0; rows.size() > i; ++i){\n temp[i] += rows[i];\n }\n#ifdef LOG\n std::cout << \"End row loop\\n\";\n#endif\n\n // Returning a temporary image\n return temp;\n}\n\n\nMatrix Matrix::operator * (const double a){\n#ifdef LOG\n std::cout << '[' << &rows << ']' << \"Matrix Matrix::operator * (\" << a << \")\\n\";\n#endif\n\n // Make a new vector to return\n // https://stackoverflow.com/questions/332111/how-do-i-convert-a-double-into-a-string-in-c\n Matrix temp(*this, std::to_string(a) + '*' + get_name());\n\n // Element loop in `for each` style\n // c++ 11 or later\n for (auto & element : temp.rows){\n element *= a;\n }\n\n // Returning a temporary image\n return temp;\n}\n\n\nRowVector Matrix::operator * (const RowVector &v){\n#ifdef LOG\n std::cout << '[' << &rows << ']' << \"Matrix Matrix::operator * (\" << &v << \")\\n\";\n#endif\n\n // Make a new vector to return\n RowVector temp(rows.size(), NULL, name + '*' + v.get_name());\n\n // Element loop in `for each` style\n // c++ 11 or later\n for (uint32_t i=0; rows.size()>i; ++i){\n temp[i] = rows[i] * v;\n }\n\n // Returning a temporary image\n return temp;\n}\n\n\nMatrix Matrix::operator * (const Matrix & other){\n#ifdef LOG\n std::cout << '[' << &rows << ']' << \"Matrix Matrix::operator * (\" << &other << \")\\n\";\n#endif\n\n // Check size\n assert(rows[0].size() == other.rows.size());\n\n Matrix temp(rows.size(), other[0].size(), name + '*' + other.name);\n\n // row loop\n for (uint32_t i = 0; rows.size() > i; ++i){\n // column loop\n for(uint32_t j = 0; other[0].size() > j; ++j){\n // dummy index loop\n for(uint32_t k = 0; rows[0].size() > k; ++k){\n temp[i][j] += rows[i][k] * other[k][j];\n }\n }\n }\n\n // Returning a temporary image\n return temp;\n}\n\n\nvoid Matrix::show(){\n#ifdef LOG\n std::cout << '[' << &rows << ']' << \"void Matrix::show()\\n\";\n#endif\n // row loop\n for (uint32_t i=0; rows.size()> i; ++i){\n // column loop\n for (uint32_t j=0; rows[i].size()> j; ++j){\n std::cout << get_name() << '['<< i << \"][\" << j << \"]= \" << rows[i][j] << '\\n';\n }\n }\n}\n\n\nMatrix Matrix::transpose(){\n#ifdef LOG\n std::cout << '[' << &rows << ']' << \"Matrix Matrix::transpose()\\n\";\n#endif\n Matrix temp(rows[0].size(), rows.size(), name+\"T\");\n\n // row loop\n for(uint32_t i=0; temp.rows.size()> i; ++i){\n // column loop\n for(uint32_t j=0; temp.rows.size()> j; ++j){\n temp[i][j] = rows[i][j];\n } \n }\n\n return temp;\n}\n\n\nconst size_t Matrix::get_height() const{\n return rows.size();\n}\n\n\nconst size_t Matrix::get_width() const{\n return rows[0].size();\n}\n\n\n// End matrix_double.cpp\n// Build command : g++ -Wall -g -std=c++14 matrix_double.cpp -fsyntax-only\n\n```",
"_____no_output_____"
],
[
"``` C++\n// Begin cpp_matrix_double_practice.cpp\n\n#include <cassert>\n#include <cmath>\n#include <cstdint>\n#include <exception>\n#include <iostream>\n#include <string>\n#include <vector>\n\n#include \"matrix_double.h\"\n\nint32_t main(int32_t argn, char *argv[]){\n\tdouble s[] = {1.0, 0.0,\n 0.0, 1.0};\n\n std::cout << \"Matrix id (2u, 2u, s, \\\"identity\\\");\\n\";\n\tMatrix identity (2u, 2u, s, \"id\");\n\n identity.show();\n\n double r[] = {+cos(M_PI/6.0), sin(M_PI/6.0),\n -sin(M_PI/6.0), cos(M_PI/6.0)};\n\n std::cout << \"Matrix rotation (2u, 2u, r, \\\"rot\\\");\\n\";\n Matrix rotation (2u, 2u, r, \"rot\");\n identity.show();\n rotation.show();\n\n std::cout << \"Matrix sum(identity + rotation);\\n\";\n Matrix sum(identity + rotation);\n identity.show();\n rotation.show();\n sum.show();\n\n // Check sum operation result\n for (uint32_t i=0; 2u > i; ++i){\n for (uint32_t j=0; 2u > j; ++j){\n assert(sum[i][j] == (identity[i][j] + rotation[i][j]));\n }\n }\n\n std::cout << \"Matrix twice(identity * 2.0);\\n\";\n Matrix twice(identity * 2.0);\n\n // Check scala multiplication result\n assert(twice[0][0] == 2.0);\n assert(twice[0][1] == 0.0);\n assert(twice[1][0] == 0.0);\n assert(twice[1][1] == 2.0);\n\n std::cout << \"Matrix new_axis(twice * rotation);\\n\";\n Matrix new_axis(twice * rotation);\n\n // Check matrix multiplication result\n for (uint32_t i=0; 2u > i; ++i){\n for (uint32_t j=0; 2u > j; ++j){\n assert(new_axis[i][j] == (2.0 * rotation[i][j]));\n }\n }\n\n Matrix ninety_degrees(rotation * rotation * rotation);\n\n // Check matrix multiplication result\n assert(abs(ninety_degrees[0][0] - ( 0.0)) < 1e-12);\n assert(abs(ninety_degrees[0][1] - ( 1.0)) < 1e-12);\n assert(abs(ninety_degrees[1][0] - (-1.0)) < 1e-12);\n assert(abs(ninety_degrees[1][1] - ( 0.0)) < 1e-12);\n\n // State Space Representation Ax + B u\n double xi_d[] = {1.0, 0.0};\n double ones_d[] = {1.0, 1.0};\n\n Matrix xi(2, 1, xi_d, \"xi\");\n Matrix B(2, 1, ones_d, \"B\");\n\n double u = 0.75;\n\n Matrix xj;\n // xj = A xi + B u\n xj = rotation * xi + B * u;\n\n xj.show();\n\n assert(abs(xj[0][0] - ( 0.75 + cos(M_PI/6.0))) < 1e-12);\n assert(abs(xj[1][0] - ( 0.75 - sin(M_PI/6.0))) < 1e-12);\n\n}\n\n// End cpp_matrix_double_practice.cpp\n// Build command : g++ -Wall -g -std=c++14 cpp_matrix_double_practice.cpp vector_double.cpp matrix_double.cpp -o cpp_matrix_double_practice\n```",
"_____no_output_____"
],
[
"* The build command above lists necessary files.\n\n",
"_____no_output_____"
],
[
"## In Python",
"_____no_output_____"
],
[
"* Following code blocks are a possible implementation of matrix in python.\n* As in C++ example, it will build on the prior `Vector` class.",
"_____no_output_____"
]
],
[
[
"import collections\nimport copy\n\n\nclass Matrix(collections.UserList):\n def __init__(self, m=None, n=None, values=None):\n if m is None:\n self.m = self.n = 0\n self.data = []\n elif values is not None:\n self.m = int(m) # number of rows\n self.n = int(n) # number of columns\n # Again utilizing Vector class and list comprehension\n self.data = [Vector(values[(i * n):((i+1) * n)]) for i in range(m)]\n\n elif n is None:\n if isinstance(m, Matrix):\n # copy constructor\n self.m = m.m\n self.n = m.n\n # To avoid referencing rows of m matrix\n self.data = copy.deepcopy(m.data)\n elif isinstance(m, Vector):\n # Vector to n x 1 Matrix\n self.data = [Vector([value]) for value in m]\n self.m = len(self.data)\n self.n = 1\n elif isinstance(m, int) and isinstance(n, int) and values is None:\n # zeros\n self.m = m\n self.n = n\n self.data = [Vector([0.0] * n) for i in range(m)]\n else:\n raise NotImplementedError\n\n def __add__(self, other):\n assert isinstance(other, Matrix)\n result = Matrix()\n for self_row, other_row in zip(self, other):\n result.append(self_row + other_row)\n return result\n\n def __mul__(self, other):\n if isinstance(other, (int, float, complex)):\n result = Matrix()\n for row in self:\n result.append(row * other)\n elif isinstance(other, Matrix):\n assert self.n == other.m, f\"Matrix sizes ({self.m}, {self.n}) x ({other.m}, {other.n}) not compatible\"\n result = Matrix(self.m, other.n)\n for i in range(self.m):\n for j in range(other.n):\n for k in range(self.n):\n result[i][j] += self[i][k] * other[k][j]\n elif isinstance(other, Vector):\n assert self.n == len(other), f\"Matrix sizes ({self.m}, {self.n}) x ({len(other)}, 1) not compatible\"\n result = Vector([row * other for row in self])\n else:\n raise NotImplementedError\n \n return result\n\n def __str__(self):\n row_text = []\n for i, row in enumerate(self):\n for j, value in enumerate(row):\n row_text.append(f\"{hex(id(self))}[{i}][{j}] = {self[i][j]}\")\n return '\\n'.join(row_text)\n\n def transpose(self):\n result = Matrix()\n result.data = list(zip(self.data))\n result.m = self.n\n resutl.n = self.m\n\n",
"_____no_output_____"
],
[
"matA = Matrix(2, 2, list(range(4)))\nprint(matA)\n\nmatB = Matrix(matA)\nmatB[0][0] = matA[0][0] + 7\nprint(matA)\nprint(matB)\nassert matA[0][0] != matB[0][0], \"Please use deep copy\"\n\nvecC = Vector([1, 0])\nprint(\"matC = Matrix(vecC)\")\nmatC = Matrix(vecC)\nprint(matA)\nprint(matB)\nprint(matC)\n\nprint(\"matD = Matrix(2, 2)\")\nmatD = Matrix(2, 2)\nprint(matA)\nprint(matB)\nprint(matC)\nprint(matD)\nfor i in range(matD.m):\n for j in range(matD.n):\n assert 0 == matD[i][j]\n\nprint(\"matE = matA + matA\")\nmatE = matA + matA\nprint(matA)\nprint(matB)\nprint(matC)\nprint(matD)\nprint(matE)\nfor i in range(matE.m):\n for j in range(matE.n):\n assert matE[i][j] == 2 * matA[i][j]\n\nprint(\"matF = matA * matA\")\nmatF = matA * matA\nprint(matA)\nprint(matB)\nprint(matC)\nprint(matD)\nprint(matE)\nprint(matF)\n\nprint(\"matG = matA * vecC\")\nvecG = matA * vecC\nprint(matA)\nprint(matB)\nprint(matC)\nprint(matD)\nprint(matE)\nprint(matF)\nprint(vecG)\nassert len(vecG) == matA.m\nfor i in range(matA.m):\n assert vecG[i] == matA[i][0]\n\n",
"_____no_output_____"
]
],
[
[
"# State Space Representation Example",
"_____no_output_____"
],
[
"## C++",
"_____no_output_____"
],
[
"* Again, this example builds on top of the `Matrix` and `RowVector` examples.",
"_____no_output_____"
],
[
"``` C++\n// Begin lti_dt.h\n\n#include <cassert>\n#include <cstdint>\n#include <exception>\n#include <iostream>\n#include <string>\n#include <vector>\n\n#include \"vector_double.h\"\n#include \"matrix_double.h\"\n\n\n#ifndef LTI_DT\n\n// Discrete Time State Space model\nclass LTI_DT{\n protected:\n Matrix A;\n Matrix B;\n Matrix C;\n Matrix D;\n Matrix X;\n\n size_t m, n;\n\n public:\n LTI_DT(Matrix &new_A, Matrix &new_B, Matrix &new_C, Matrix &new_D, Matrix &new_X);\n ~LTI_DT();\n const Matrix get_y(const double u);\n void get_next_x(const double u);\n};\n#endif\n\n// End lti_dt.h\n\n```",
"_____no_output_____"
],
[
"``` C++\n// Begin lti_dt.cpp\n\n#include <cassert>\n#include <cstdint>\n#include <exception>\n#include <iostream>\n#include <string>\n#include <vector>\n\n#include \"vector_double.h\"\n#include \"matrix_double.h\"\n#include \"lti_dt.h\"\n\n// Discrete Time State Space model\nLTI_DT::LTI_DT(Matrix &new_A, Matrix &new_B, Matrix &new_C, Matrix &new_D, Matrix &new_X){\n#ifdef LOG\n std::cout << '[' << &A << ']' << \"LTI_DT::LTI_DT(\" << &new_A << \", \" << &new_B << \", \" << &new_C << \", \" << &new_D << \")\\n\";\n#endif\n \n#ifdef LOG\n std::cout << \"LTI_DT::LTI_DT(): A = new_A;\\n\";\n#endif\n A = new_A; \n#ifdef LOG\n std::cout << \"LTI_DT::LTI_DT(): B = new_B;\\n\";\n#endif\n B = new_B; \n#ifdef LOG\n std::cout << \"LTI_DT::LTI_DT(): C = new_C;\\n\";\n#endif\n C = new_C; \n#ifdef LOG\n std::cout << \"LTI_DT::LTI_DT(): D = new_D;\\n\";\n#endif\n D = new_D; \n#ifdef LOG\n std::cout << \"LTI_DT::LTI_DT(): X = new_X;\\n\";\n#endif\n X = new_X;\n\n // is A matrix square?\n assert(A.get_height() == A.get_width());\n\n // number of state variables\n n = A.get_height();\n\n // check number of rows of B matrix\n assert(B.get_height() == n);\n \n // expected size of input\n m = B.get_width();\n\n}\n\nLTI_DT::~LTI_DT(){\n#ifdef LOG\n std::cout << '[' << &A << ']' << \"LTI_DT::!LTI_DT()\\n\";\n#endif\n#ifdef LOG\n std::cout << \"delete &A;\\n\";\n#endif\n // delete &A;\n#ifdef LOG\n std::cout << \"delete &B;\\n\";\n#endif\n // delete &B;\n#ifdef LOG\n std::cout << \"delete &C;\\n\";\n#endif\n // delete &C;\n#ifdef LOG\n std::cout << \"delete &D;\\n\";\n#endif\n // delete &D;\n#ifdef LOG\n std::cout << \"delete &X;\\n\";\n#endif\n // delete &X;\n}\n\nconst Matrix LTI_DT::get_y(const double u){\n return Matrix (C * X + D * u);\n}\n\nvoid LTI_DT::get_next_x(const double u){\n Matrix next_X (A * X + B * u);\n\n // delete &X;\n\n X = next_X;\n}\n\n// End lti_dt.cpp\n\n```",
"_____no_output_____"
],
[
"``` C++\n// Begin lti_dt_example.cpp\n\n#include <cassert>\n#include <cstdint>\n#include <exception>\n#include <iostream>\n#include <string>\n#include <vector>\n\n#include \"vector_double.h\"\n#include \"matrix_double.h\"\n#include \"lti_dt.h\"\n\nint32_t main(int32_t argn, char *argv[]){\n // https://ccrma.stanford.edu/~jos/fp/State_Space_Simulation_Matlab.html\n\n const double A_d[] = {0, 1, -1, 0};\n std::cout << \"Matrix A(2u, 2u, A_d, \\\"A\\\");\\n\";\n Matrix A(2u, 2u, A_d, \"A\");\n\n const double B_d[] = {0, 1};\n std::cout << \"Matrix B(2u, 1u, B_d, \\\"B\\\");\\n\";\n Matrix B(2u, 1u, B_d, \"B\");\n\n const double C_d[] = {1, 0, 0, 1, 0, 1};\n std::cout << \"Matrix C(3u, 2u, C_d, \\\"C\\\");\\n\";\n Matrix C(3u, 2u, C_d, \"C\");\n\n const double D_d[] = {0, 0, 0};\n std::cout << \"Matrix D(3u, 1u, D_d, \\\"D\\\");\\n\";\n Matrix D(3u, 1u, D_d, \"D\");\n\n const uint32_t n = 10;\n const double u[n] = {1.0, 0.0, 0.0, 0.0, 0.0, 0.0, 0.0, 0.0, 0.0, 0.0};\n\n std::vector<Matrix> y_list;\n\n Matrix X(2u, 1u, \"x\");\n\n LTI_DT ss_dt(A, B, C, D, X);\n\n for(uint32_t k=0; n > k; ++k){\n Matrix y_now(ss_dt.get_y(u[k]));\n y_list.push_back(y_now);\n ss_dt.get_next_x(u[k]);\n }\n\n for(uint32_t i=0; n>i; ++i){\n std::cout << \"y[\" << i << \"] = \" << y_list[i][2][0] << '\\n';\n }\n\n return 0;\n}\n// End lti_dt_example.cpp\n// Build command : g++ -Wall -g -std=c++14 lti_dt_example.cpp vector_double.cpp matrix_double.cpp lti_dt.cpp -o lti_dt_example\n\n\n```",
"_____no_output_____"
],
[
"* However, this example may have some obvious problem. What do you think?",
"_____no_output_____"
],
[
"* For python implementation, please refer to another file.",
"_____no_output_____"
],
[
"# Exercise",
"_____no_output_____"
],
[
"## 00 Comments",
"_____no_output_____"
],
[
"* Please try to add comments to each line of the source code.\n* So that anyone tries to read the code can immediately understand.\n* Group work would be possible, too.",
"_____no_output_____"
],
[
"## 01 Improve the code",
"_____no_output_____"
],
[
"* See if you can find some possible improvements\n* Try submit improvement through a *pull request*.\n* What could be a good way to know whether the new code would be suitable?",
"_____no_output_____"
]
]
] | [
"markdown",
"code",
"markdown",
"code",
"markdown"
] | [
[
"markdown",
"markdown",
"markdown",
"markdown",
"markdown",
"markdown",
"markdown"
],
[
"code",
"code"
],
[
"markdown",
"markdown",
"markdown",
"markdown",
"markdown",
"markdown",
"markdown",
"markdown",
"markdown"
],
[
"code",
"code"
],
[
"markdown",
"markdown",
"markdown",
"markdown",
"markdown",
"markdown",
"markdown",
"markdown",
"markdown",
"markdown",
"markdown",
"markdown",
"markdown"
]
] |
d090d6cdb83a1e0c539767876c7e2c7ef0a22de6 | 235,965 | ipynb | Jupyter Notebook | nb/035_submission.ipynb | fkubota/kaggle-University-of-Liverpool-Ion-Switching | bf0b5d7ad1682e68937295af16a4773e857f0405 | [
"MIT"
] | null | null | null | nb/035_submission.ipynb | fkubota/kaggle-University-of-Liverpool-Ion-Switching | bf0b5d7ad1682e68937295af16a4773e857f0405 | [
"MIT"
] | null | null | null | nb/035_submission.ipynb | fkubota/kaggle-University-of-Liverpool-Ion-Switching | bf0b5d7ad1682e68937295af16a4773e857f0405 | [
"MIT"
] | 2 | 2021-03-15T14:14:30.000Z | 2021-05-23T14:21:43.000Z | 58.119458 | 55,752 | 0.62911 | [
[
[
"# Overview\n- nb023 ベース\n- nb034の結果を使う",
"_____no_output_____"
],
[
"# Const",
"_____no_output_____"
]
],
[
[
"NB = '035'\nisSmallSet = False\nif isSmallSet:\n LENGTH = 7000\nelse:\n LENGTH = 500_000\n\nPATH_TRAIN = './../data/input/train_clean.csv'\nPATH_TEST = './../data/input/test_clean.csv'\nPATH_SMPLE_SUB = './../data/input/sample_submission.csv'\nDIR_OUTPUT = './../data/output/'\ncp = ['#f8b195', '#f67280', '#c06c84', '#6c5b7b', '#355c7d']\nsr = 10*10**3 # 10 kHz",
"_____no_output_____"
]
],
[
[
"# Import everything I need :)",
"_____no_output_____"
]
],
[
[
"import warnings\nwarnings.filterwarnings('ignore')\nimport time\nimport gc\nimport random\nimport os\nimport itertools\nimport multiprocessing\nimport numpy as np\nfrom scipy import signal\n# from pykalman import KalmanFilter\nimport pandas as pd\nimport matplotlib.pyplot as plt\nimport seaborn as sns\nfrom fastprogress import progress_bar\nfrom lightgbm import LGBMRegressor\nfrom sklearn.model_selection import KFold, train_test_split, StratifiedKFold, GroupKFold\nfrom sklearn.metrics import f1_score, mean_absolute_error, confusion_matrix\nfrom sklearn.ensemble import RandomForestRegressor\nfrom sklearn.tree import DecisionTreeRegressor\n# from sklearn.svm import SVR\nfrom sklearn.linear_model import Lasso\n# from dtreeviz.trees import dtreeviz\nimport tensorflow as tf\nfrom tensorflow.keras.layers import *\nfrom tensorflow.keras.callbacks import Callback, LearningRateScheduler\nfrom tensorflow.keras.losses import categorical_crossentropy\nfrom tensorflow.keras.optimizers import Adam\nfrom tensorflow.keras import backend as K\nfrom tensorflow.keras import losses, models, optimizers\n# import tensorflow_addons as tfa",
"_____no_output_____"
]
],
[
[
"# My function",
"_____no_output_____"
]
],
[
[
"def f1_macro(true, pred):\n return f1_score(true, pred, average='macro')\n\ndef get_df_batch(df, batch):\n idxs = df['batch'] == batch\n assert any(idxs), 'そのようなbatchはありません'\n return df[idxs]\n \ndef get_signal_mv_mean(df, n=3001):\n signal_mv = np.zeros(len(df))\n for bt in df['batch'].unique():\n idxs = df['batch'] == bt\n _signal_mv = df['signal'][idxs].rolling(n, center=True).mean().interpolate('spline', order=5, limit_direction='both').values\n signal_mv[idxs] = _signal_mv\n return signal_mv\n\ndef get_signal_mv_std(df, n=3001):\n signal_mv = np.zeros(len(df))\n for bt in df['batch'].unique():\n idxs = df['batch'] == bt\n _signal_mv = df['signal'][idxs].rolling(n, center=True).std().interpolate('spline', order=5, limit_direction='both').values\n signal_mv[idxs] = _signal_mv\n return signal_mv\n\ndef get_signal_mv_min(df, n=3001):\n signal_mv = np.zeros(len(df))\n for bt in df['batch'].unique():\n idxs = df['batch'] == bt\n _signal_mv = df['signal'][idxs].rolling(n, center=True).min().interpolate('spline', order=5, limit_direction='both').values\n signal_mv[idxs] = _signal_mv\n return signal_mv\n\ndef get_signal_mv_max(df, n=3001):\n signal_mv = np.zeros(len(df))\n for bt in df['batch'].unique():\n idxs = df['batch'] == bt\n _signal_mv = df['signal'][idxs].rolling(n, center=True).max().interpolate('spline', order=5, limit_direction='both').values\n signal_mv[idxs] = _signal_mv\n return signal_mv\n\n\n\ndef group_feat_train(_train):\n train = _train.copy()\n # group init\n train['group'] = int(0)\n\n # group 1\n idxs = (train['batch'] == 3) | (train['batch'] == 7)\n train['group'][idxs] = int(1)\n\n # group 2\n idxs = (train['batch'] == 5) | (train['batch'] == 8)\n train['group'][idxs] = int(2)\n\n # group 3\n idxs = (train['batch'] == 2) | (train['batch'] == 6)\n train['group'][idxs] = int(3)\n\n # group 4\n idxs = (train['batch'] == 4) | (train['batch'] == 9)\n train['group'][idxs] = int(4)\n \n return train[['group']]\n\ndef group_feat_test(_test):\n test = _test.copy()\n \n # group init\n test['group'] = int(0)\n x_idx = np.arange(len(test))\n\n # group 1\n idxs = (100000<=x_idx) & (x_idx<200000)\n test['group'][idxs] = int(1)\n idxs = (900000<=x_idx) & (x_idx<=1000000)\n test['group'][idxs] = int(1)\n\n # group 2\n idxs = (200000<=x_idx) & (x_idx<300000)\n test['group'][idxs] = int(2)\n idxs = (600000<=x_idx) & (x_idx<700000)\n test['group'][idxs] = int(2)\n\n # group 3\n idxs = (400000<=x_idx) & (x_idx<500000)\n test['group'][idxs] = int(3)\n\n # group 4\n idxs = (500000<=x_idx) & (x_idx<600000)\n test['group'][idxs] = int(4)\n idxs = (700000<=x_idx) & (x_idx<800000)\n test['group'][idxs] = int(4)\n \n return test[['group']]\n\n\nclass permutation_importance():\n def __init__(self, model, metric):\n self.is_computed = False\n self.n_feat = 0\n self.base_score = 0\n self.model = model\n self.metric = metric\n self.df_result = []\n \n def compute(self, X_valid, y_valid):\n self.n_feat = len(X_valid.columns)\n if self.metric == 'auc':\n y_valid_score = self.model.predict_proba(X_valid)[:, 1]\n fpr, tpr, thresholds = roc_curve(y_valid, y_valid_score)\n self.base_score = auc(fpr, tpr)\n else:\n pred = np.round(self.model.predict(X_valid)).astype('int8')\n self.base_score = self.metric(y_valid, pred)\n self.df_result = pd.DataFrame({'feat': X_valid.columns, \n 'score': np.zeros(self.n_feat),\n 'score_diff': np.zeros(self.n_feat)})\n \n # predict\n for i, col in enumerate(X_valid.columns):\n df_perm = X_valid.copy()\n np.random.seed(1)\n df_perm[col] = np.random.permutation(df_perm[col])\n y_valid_pred = self.model.predict(df_perm)\n if self.metric == 'auc':\n y_valid_score = self.model.predict_proba(df_perm)[:, 1]\n fpr, tpr, thresholds = roc_curve(y_valid, y_valid_score)\n score = auc(fpr, tpr)\n else:\n score = self.metric(y_valid, np.round(y_valid_pred).astype('int8'))\n self.df_result['score'][self.df_result['feat']==col] = score\n self.df_result['score_diff'][self.df_result['feat']==col] = self.base_score - score\n self.is_computed = True\n \n def get_negative_feature(self):\n assert self.is_computed!=False, 'compute メソッドが実行されていません'\n idx = self.df_result['score_diff'] < 0\n return self.df_result.loc[idx, 'feat'].values.tolist()\n \n def get_positive_feature(self):\n assert self.is_computed!=False, 'compute メソッドが実行されていません'\n idx = self.df_result['score_diff'] > 0\n return self.df_result.loc[idx, 'feat'].values.tolist()\n \n def show_permutation_importance(self, score_type='loss'):\n '''score_type = 'loss' or 'accuracy' '''\n assert self.is_computed!=False, 'compute メソッドが実行されていません'\n if score_type=='loss':\n ascending = True\n elif score_type=='accuracy':\n ascending = False\n else:\n ascending = ''\n \n plt.figure(figsize=(15, int(0.25*self.n_feat)))\n sns.barplot(x=\"score_diff\", y=\"feat\", data=self.df_result.sort_values(by=\"score_diff\", ascending=ascending))\n plt.title('base_score - permutation_score')\n\ndef plot_corr(df, abs_=False, threshold=0.95):\n if abs_==True:\n corr = df.corr().abs()>threshold\n vmin = 0\n else:\n corr = df.corr()\n vmin = -1\n\n # Plot\n fig, ax = plt.subplots(figsize=(12, 10), dpi=100)\n fig.patch.set_facecolor('white')\n sns.heatmap(corr,\n xticklabels=df.corr().columns,\n yticklabels=df.corr().columns,\n vmin=vmin,\n vmax=1,\n center=0, \n annot=False)\n\n # Decorations\n ax.set_title('Correlation', fontsize=22)\n\ndef get_low_corr_column(df, threshold):\n\n df_corr = df.corr()\n df_corr = abs(df_corr)\n columns = df_corr.columns\n\n # 対角線の値を0にする\n for i in range(0, len(columns)):\n df_corr.iloc[i, i] = 0\n\n while True:\n columns = df_corr.columns\n max_corr = 0.0\n query_column = None\n target_column = None\n\n df_max_column_value = df_corr.max()\n max_corr = df_max_column_value.max()\n query_column = df_max_column_value.idxmax()\n target_column = df_corr[query_column].idxmax()\n\n if max_corr < threshold:\n # しきい値を超えるものがなかったため終了\n break\n else:\n # しきい値を超えるものがあった場合\n delete_column = None\n saved_column = None\n\n # その他との相関の絶対値が大きい方を除去\n if sum(df_corr[query_column]) <= sum(df_corr[target_column]):\n delete_column = target_column\n saved_column = query_column\n else:\n delete_column = query_column\n saved_column = target_column\n\n # 除去すべき特徴を相関行列から消す(行、列)\n df_corr.drop([delete_column], axis=0, inplace=True)\n df_corr.drop([delete_column], axis=1, inplace=True)\n\n return df_corr.columns # 相関が高い特徴量を除いた名前リスト\n\ndef reduce_mem_usage(df, verbose=True):\n numerics = ['int16', 'int32', 'int64', 'float16', 'float32', 'float64']\n start_mem = df.memory_usage().sum() / 1024**2 \n for col in df.columns:\n if col!='open_channels':\n col_type = df[col].dtypes\n if col_type in numerics:\n c_min = df[col].min()\n c_max = df[col].max()\n if str(col_type)[:3] == 'int':\n if c_min > np.iinfo(np.int8).min and c_max < np.iinfo(np.int8).max:\n df[col] = df[col].astype(np.int8)\n elif c_min > np.iinfo(np.int16).min and c_max < np.iinfo(np.int16).max:\n df[col] = df[col].astype(np.int16)\n elif c_min > np.iinfo(np.int32).min and c_max < np.iinfo(np.int32).max:\n df[col] = df[col].astype(np.int32)\n elif c_min > np.iinfo(np.int64).min and c_max < np.iinfo(np.int64).max:\n df[col] = df[col].astype(np.int64) \n else:\n if c_min > np.finfo(np.float16).min and c_max < np.finfo(np.float16).max:\n df[col] = df[col].astype(np.float16)\n elif c_min > np.finfo(np.float32).min and c_max < np.finfo(np.float32).max:\n df[col] = df[col].astype(np.float32)\n else:\n df[col] = df[col].astype(np.float64) \n end_mem = df.memory_usage().sum() / 1024**2\n if verbose: print('Mem. usage decreased to {:5.2f} Mb ({:.1f}% reduction)'.format(end_mem, 100 * (start_mem - end_mem) / start_mem))\n return df",
"_____no_output_____"
],
[
"def train_lgbm(X, y, X_te, lgbm_params, random_state=5, n_fold=5, verbose=50, early_stopping_rounds=100, show_fig=True):\n # using features\n print(f'features({len(X.columns)}): \\n{X.columns}') if not verbose==0 else None\n\n# folds = KFold(n_splits=n_fold, shuffle=True, random_state=random_state)\n folds = StratifiedKFold(n_splits=n_fold, shuffle=True, random_state=random_state)\n\n scores = []\n oof = np.zeros(len(X))\n oof_round = np.zeros(len(X))\n test_pred = np.zeros(len(X_te))\n df_pi = pd.DataFrame(columns=['feat', 'score_diff'])\n for fold_n, (train_idx, valid_idx) in enumerate(folds.split(X, y=y)):\n if verbose==0:\n pass\n else:\n print('\\n------------------')\n print(f'- Fold {fold_n + 1}/{N_FOLD} started at {time.ctime()}')\n\n # prepare dataset\n X_train, X_valid = X.iloc[train_idx], X.iloc[valid_idx]\n y_train, y_valid = y[train_idx], y[valid_idx]\n\n # train\n model = LGBMRegressor(**lgbm_params, n_estimators=N_ESTIMATORS)\n model.fit(X_train, y_train,\n eval_set=[(X_train, y_train), (X_valid, y_valid)],\n verbose=verbose,\n early_stopping_rounds=early_stopping_rounds)\n\n # pred\n y_valid_pred = model.predict(X_valid, model.best_iteration_)\n y_valid_pred_round = np.round(y_valid_pred).astype('int8')\n _test_pred = model.predict(X_te, model.best_iteration_)\n\n if show_fig==False:\n pass\n else:\n # permutation importance\n pi = permutation_importance(model, f1_macro) # model と metric を渡す\n pi.compute(X_valid, y_valid)\n pi_result = pi.df_result\n df_pi = pd.concat([df_pi, pi_result[['feat', 'score_diff']]])\n\n # result\n oof[valid_idx] = y_valid_pred\n oof_round[valid_idx] = y_valid_pred_round\n score = f1_score(y_valid, y_valid_pred_round, average='macro')\n scores.append(score)\n test_pred += _test_pred\n if verbose==0:\n pass\n else:\n print(f'---> f1-score(macro) valid: {f1_score(y_valid, y_valid_pred_round, average=\"macro\"):.4f}')\n print('')\n\n\n print('====== finish ======')\n print('score list:', scores)\n print('CV mean score(f1_macro): {0:.4f}, std: {1:.4f}'.format(np.mean(scores), np.std(scores)))\n print(f'oof score(f1_macro): {f1_score(y, oof_round, average=\"macro\"):.4f}')\n print('')\n\n\n if show_fig==False:\n pass\n else:\n # visualization\n plt.figure(figsize=(5, 5))\n plt.plot([0, 10], [0, 10], color='gray')\n plt.scatter(y, oof, alpha=0.05, color=cp[1])\n plt.xlabel('true')\n plt.ylabel('pred')\n plt.show()\n \n # confusion_matrix\n plot_confusion_matrix(y, oof_round, classes=np.arange(11))\n \n \n # permutation importance\n plt.figure(figsize=(15, int(0.25*len(X.columns))))\n order = df_pi.groupby([\"feat\"]).mean()['score_diff'].reset_index().sort_values('score_diff', ascending=False)\n sns.barplot(x=\"score_diff\", y=\"feat\", data=df_pi, order=order['feat'])\n plt.title('base_score - permutation_score')\n plt.show()\n\n # submission\n test_pred = test_pred/N_FOLD\n test_pred_round = np.round(test_pred).astype('int8')\n \n return test_pred_round, test_pred, oof_round, oof\n\ndef plot_confusion_matrix(truth, pred, classes, normalize=False, title=''):\n cm = confusion_matrix(truth, pred)\n if normalize:\n cm = cm.astype('float') / cm.sum(axis=1)[:, np.newaxis]\n \n plt.figure(figsize=(10, 10))\n plt.imshow(cm, interpolation='nearest', cmap=plt.cm.Blues)\n plt.title('Confusion matrix', size=15)\n plt.colorbar(fraction=0.046, pad=0.04)\n tick_marks = np.arange(len(classes))\n plt.xticks(tick_marks, classes, rotation=45)\n plt.yticks(tick_marks, classes)\n\n fmt = '.2f' if normalize else 'd'\n thresh = cm.max() / 2.\n for i, j in itertools.product(range(cm.shape[0]), range(cm.shape[1])):\n plt.text(j, i, format(cm[i, j], fmt),\n horizontalalignment=\"center\",\n color=\"white\" if cm[i, j] > thresh else \"black\")\n\n plt.ylabel('True label')\n plt.xlabel('Predicted label')\n plt.grid(False)\n plt.tight_layout()",
"_____no_output_____"
],
[
"def train_test_split_lgbm(X, y, X_te, lgbm_params, random_state=5, test_size=0.3, verbose=50, early_stopping_rounds=100, show_fig=True):\n # using features\n print(f'features({len(X.columns)}): \\n{X.columns}') if not verbose==0 else None\n\n# folds = KFold(n_splits=n_fold, shuffle=True, random_state=random_state)\n# folds = StratifiedKFold(n_splits=n_fold, shuffle=True, random_state=random_state)\n \n # prepare dataset\n X_train, X_valid, y_train, y_valid = train_test_split(X, y, test_size=test_size, random_state=random_state)\n\n # train\n model = LGBMRegressor(**lgbm_params, n_estimators=N_ESTIMATORS)\n model.fit(X_train, y_train,\n eval_set=[(X_train, y_train), (X_valid, y_valid)],\n verbose=verbose,\n early_stopping_rounds=early_stopping_rounds)\n\n # pred\n oof = model.predict(X_valid, model.best_iteration_)\n oof_round = np.round(oof).astype('int8')\n test_pred = model.predict(X_te, model.best_iteration_)\n test_pred_round = np.round(test_pred).astype('int8')\n\n print('====== finish ======')\n print(f'oof score(f1_macro): {f1_score(y_valid, oof_round, average=\"macro\"):.4f}')\n print('')\n\n\n if show_fig==False:\n pass\n else:\n # visualization\n plt.figure(figsize=(5, 5))\n plt.plot([0, 10], [0, 10], color='gray')\n plt.scatter(y_valid, oof, alpha=0.05, color=cp[1])\n plt.xlabel('true')\n plt.ylabel('pred')\n plt.show()\n \n # confusion_matrix\n plot_confusion_matrix(y_valid, oof_round, classes=np.arange(11))\n \n # permutation importance\n pi = permutation_importance(model, f1_macro) # model と metric を渡す\n pi.compute(X_valid, y_valid)\n pi.show_permutation_importance(score_type='accuracy') # loss or accuracy\n plt.show()\n\n return test_pred_round, test_pred, oof_round, oof",
"_____no_output_____"
]
],
[
[
"<br>\n\nref: https://www.kaggle.com/martxelo/fe-and-ensemble-mlp-and-lgbm",
"_____no_output_____"
]
],
[
[
"def calc_gradients(s, n_grads=4):\n '''\n Calculate gradients for a pandas series. Returns the same number of samples\n '''\n grads = pd.DataFrame()\n \n g = s.values\n for i in range(n_grads):\n g = np.gradient(g)\n grads['grad_' + str(i+1)] = g\n \n return grads\n\n\ndef calc_low_pass(s, n_filts=10):\n '''\n Applies low pass filters to the signal. Left delayed and no delayed\n '''\n wns = np.logspace(-2, -0.3, n_filts)\n# wns = [0.3244]\n \n low_pass = pd.DataFrame()\n x = s.values\n for wn in wns:\n b, a = signal.butter(1, Wn=wn, btype='low')\n zi = signal.lfilter_zi(b, a)\n low_pass['lowpass_lf_' + str('%.4f' %wn)] = signal.lfilter(b, a, x, zi=zi*x[0])[0]\n low_pass['lowpass_ff_' + str('%.4f' %wn)] = signal.filtfilt(b, a, x)\n \n return low_pass\n\ndef calc_high_pass(s, n_filts=10):\n '''\n Applies high pass filters to the signal. Left delayed and no delayed\n '''\n wns = np.logspace(-2, -0.1, n_filts)\n# wns = [0.0100, 0.0264, 0.0699, 0.3005, 0.4885, 0.7943]\n \n high_pass = pd.DataFrame()\n x = s.values\n for wn in wns:\n b, a = signal.butter(1, Wn=wn, btype='high')\n zi = signal.lfilter_zi(b, a)\n high_pass['highpass_lf_' + str('%.4f' %wn)] = signal.lfilter(b, a, x, zi=zi*x[0])[0]\n high_pass['highpass_ff_' + str('%.4f' %wn)] = signal.filtfilt(b, a, x)\n \n return high_pass\n\ndef calc_roll_stats(s, windows=[10, 50, 100, 500, 1000, 3000]):\n '''\n Calculates rolling stats like mean, std, min, max...\n '''\n roll_stats = pd.DataFrame()\n for w in windows:\n roll_stats['roll_mean_' + str(w)] = s.rolling(window=w, min_periods=1).mean().interpolate('spline', order=5, limit_direction='both')\n roll_stats['roll_std_' + str(w)] = s.rolling(window=w, min_periods=1).std().interpolate('spline', order=5, limit_direction='both')\n roll_stats['roll_min_' + str(w)] = s.rolling(window=w, min_periods=1).min().interpolate('spline', order=5, limit_direction='both')\n roll_stats['roll_max_' + str(w)] = s.rolling(window=w, min_periods=1).max().interpolate('spline', order=5, limit_direction='both')\n roll_stats['roll_range_' + str(w)] = roll_stats['roll_max_' + str(w)] - roll_stats['roll_min_' + str(w)]\n roll_stats['roll_q10_' + str(w)] = s.rolling(window=w, min_periods=1).quantile(0.10).interpolate('spline', order=5, limit_direction='both')\n roll_stats['roll_q25_' + str(w)] = s.rolling(window=w, min_periods=1).quantile(0.25).interpolate('spline', order=5, limit_direction='both')\n roll_stats['roll_q50_' + str(w)] = s.rolling(window=w, min_periods=1).quantile(0.50).interpolate('spline', order=5, limit_direction='both')\n roll_stats['roll_q75_' + str(w)] = s.rolling(window=w, min_periods=1).quantile(0.75).interpolate('spline', order=5, limit_direction='both')\n roll_stats['roll_q90_' + str(w)] = s.rolling(window=w, min_periods=1).quantile(0.90).interpolate('spline', order=5, limit_direction='both')\n \n # add zeros when na values (std)\n# roll_stats = roll_stats.fillna(value=0)\n \n return roll_stats\n\ndef calc_ewm(s, windows=[10, 50, 100, 500, 1000, 3000]):\n '''\n Calculates exponential weighted functions\n '''\n ewm = pd.DataFrame()\n for w in windows:\n ewm['ewm_mean_' + str(w)] = s.ewm(span=w, min_periods=1).mean()\n ewm['ewm_std_' + str(w)] = s.ewm(span=w, min_periods=1).std()\n \n # add zeros when na values (std)\n ewm = ewm.fillna(value=0)\n \n return ewm\n\n\n\ndef divide_and_add_features(s, signal_size=500000):\n '''\n Divide the signal in bags of \"signal_size\".\n Normalize the data dividing it by 15.0\n '''\n # normalize\n s = s/15.0\n \n ls = []\n for i in progress_bar(range(int(s.shape[0]/signal_size))):\n sig = s[i*signal_size:(i+1)*signal_size].copy().reset_index(drop=True)\n sig_featured = add_features(sig)\n ls.append(sig_featured)\n \n return pd.concat(ls, axis=0)",
"_____no_output_____"
]
],
[
[
"<br>\n\nref: https://www.kaggle.com/nxrprime/single-model-lgbm-kalman-filter-ii",
"_____no_output_____"
]
],
[
[
"def Kalman1D(observations,damping=1):\n # To return the smoothed time series data\n observation_covariance = damping\n initial_value_guess = observations[0]\n transition_matrix = 1\n transition_covariance = 0.1\n initial_value_guess\n kf = KalmanFilter(\n initial_state_mean=initial_value_guess,\n initial_state_covariance=observation_covariance,\n observation_covariance=observation_covariance,\n transition_covariance=transition_covariance,\n transition_matrices=transition_matrix\n )\n pred_state, state_cov = kf.smooth(observations)\n return pred_state",
"_____no_output_____"
]
],
[
[
"# Preparation",
"_____no_output_____"
],
[
"setting",
"_____no_output_____"
]
],
[
[
"sns.set()",
"_____no_output_____"
]
],
[
[
"<br>\n\nload dataset",
"_____no_output_____"
]
],
[
[
"df_tr = pd.read_csv(PATH_TRAIN)\ndf_te = pd.read_csv(PATH_TEST)",
"_____no_output_____"
]
],
[
[
"<br>\n\n処理のしやすさのために、バッチ番号を振る",
"_____no_output_____"
]
],
[
[
"batch_list = []\nfor n in range(10):\n batchs = np.ones(500000)*n\n batch_list.append(batchs.astype(int))\nbatch_list = np.hstack(batch_list)\ndf_tr['batch'] = batch_list\n\nbatch_list = []\nfor n in range(4):\n batchs = np.ones(500000)*n\n batch_list.append(batchs.astype(int))\nbatch_list = np.hstack(batch_list)\ndf_te['batch'] = batch_list",
"_____no_output_____"
]
],
[
[
"<br>\n\nsmallset?",
"_____no_output_____"
]
],
[
[
"if isSmallSet:\n print('small set mode')\n # train\n batchs = df_tr['batch'].values\n dfs = []\n for i_bt, bt in enumerate(df_tr['batch'].unique()):\n idxs = batchs == bt\n _df = df_tr[idxs][:LENGTH].copy()\n dfs.append(_df)\n df_tr = pd.concat(dfs).reset_index(drop=True)\n \n # test\n batchs = df_te['batch'].values\n dfs = []\n for i_bt, bt in enumerate(df_te['batch'].unique()):\n idxs = batchs == bt\n _df = df_te[idxs][:LENGTH].copy()\n dfs.append(_df)\n df_te = pd.concat(dfs).reset_index(drop=True)",
"_____no_output_____"
]
],
[
[
"# Train",
"_____no_output_____"
]
],
[
[
"# configurations and main hyperparammeters\n# EPOCHS = 180\nEPOCHS = 180\nNNBATCHSIZE = 16\nGROUP_BATCH_SIZE = 4000\nSEED = 321\nLR = 0.0015\nSPLITS = 6\n\ndef seed_everything(seed):\n random.seed(seed)\n np.random.seed(seed)\n os.environ['PYTHONHASHSEED'] = str(seed)\n# tf.random.set_seed(seed)",
"_____no_output_____"
],
[
"# read data\ndef read_data():\n train = pd.read_csv(PATH_TRAIN, dtype={'time': np.float32, 'signal': np.float32, 'open_channels':np.int32})\n test = pd.read_csv(PATH_TEST, dtype={'time': np.float32, 'signal': np.float32})\n sub = pd.read_csv(PATH_SMPLE_SUB, dtype={'time': np.float32})\n \n# Y_train_proba = np.load('./../data/input/Y_train_proba.npy')\n# Y_test_proba = np.load('./../data/input/Y_test_proba.npy')\n probas = np.load('./../data/output_ignore/probas_nb034_RandomForestClassifier_cv_0.9383.npz')\n Y_train_proba = probas['arr_0']\n Y_test_proba = probas['arr_1']\n \n for i in range(11):\n train[f\"proba_{i}\"] = Y_train_proba[:, i]\n test[f\"proba_{i}\"] = Y_test_proba[:, i]\n\n return train, test, sub\n\n# create batches of 4000 observations\ndef batching(df, batch_size):\n df['group'] = df.groupby(df.index//batch_size, sort=False)['signal'].agg(['ngroup']).values\n df['group'] = df['group'].astype(np.uint16)\n return df\n\n# normalize the data (standard scaler). We can also try other scalers for a better score!\ndef normalize(train, test):\n train_input_mean = train.signal.mean()\n train_input_sigma = train.signal.std()\n train['signal'] = (train.signal - train_input_mean) / train_input_sigma\n test['signal'] = (test.signal - train_input_mean) / train_input_sigma\n return train, test\n\n# get lead and lags features\ndef lag_with_pct_change(df, windows):\n for window in windows: \n df['signal_shift_pos_' + str(window)] = df.groupby('group')['signal'].shift(window).fillna(0)\n df['signal_shift_neg_' + str(window)] = df.groupby('group')['signal'].shift(-1 * window).fillna(0)\n return df\n\n# main module to run feature engineering. Here you may want to try and add other features and check if your score imporves :).\ndef run_feat_engineering(df, batch_size):\n # create batches\n df = batching(df, batch_size = batch_size)\n # create leads and lags (1, 2, 3 making them 6 features)\n df = lag_with_pct_change(df, [1, 2, 3])\n # create signal ** 2 (this is the new feature)\n df['signal_2'] = df['signal'] ** 2\n return df\n\n# fillna with the mean and select features for training\ndef feature_selection(train, test):\n features = [col for col in train.columns if col not in ['index', 'group', 'open_channels', 'time']]\n train = train.replace([np.inf, -np.inf], np.nan)\n test = test.replace([np.inf, -np.inf], np.nan)\n for feature in features:\n feature_mean = pd.concat([train[feature], test[feature]], axis = 0).mean()\n train[feature] = train[feature].fillna(feature_mean)\n test[feature] = test[feature].fillna(feature_mean)\n return train, test, features\n\n# model function (very important, you can try different arquitectures to get a better score. I believe that top public leaderboard is a 1D Conv + RNN style)\ndef Classifier(shape_):\n \n def cbr(x, out_layer, kernel, stride, dilation):\n x = Conv1D(out_layer, kernel_size=kernel, dilation_rate=dilation, strides=stride, padding=\"same\")(x)\n x = BatchNormalization()(x)\n x = Activation(\"relu\")(x)\n return x\n \n def wave_block(x, filters, kernel_size, n):\n dilation_rates = [2**i for i in range(n)]\n x = Conv1D(filters = filters,\n kernel_size = 1,\n padding = 'same')(x)\n res_x = x\n for dilation_rate in dilation_rates:\n tanh_out = Conv1D(filters = filters,\n kernel_size = kernel_size,\n padding = 'same', \n activation = 'tanh', \n dilation_rate = dilation_rate)(x)\n sigm_out = Conv1D(filters = filters,\n kernel_size = kernel_size,\n padding = 'same',\n activation = 'sigmoid', \n dilation_rate = dilation_rate)(x)\n x = Multiply()([tanh_out, sigm_out])\n x = Conv1D(filters = filters,\n kernel_size = 1,\n padding = 'same')(x)\n res_x = Add()([res_x, x])\n return res_x\n \n inp = Input(shape = (shape_))\n x = cbr(inp, 64, 7, 1, 1)\n x = BatchNormalization()(x)\n x = wave_block(x, 16, 3, 12)\n x = BatchNormalization()(x)\n x = wave_block(x, 32, 3, 8)\n x = BatchNormalization()(x)\n x = wave_block(x, 64, 3, 4)\n x = BatchNormalization()(x)\n x = wave_block(x, 128, 3, 1)\n x = cbr(x, 32, 7, 1, 1)\n x = BatchNormalization()(x)\n x = Dropout(0.2)(x)\n out = Dense(11, activation = 'softmax', name = 'out')(x)\n \n model = models.Model(inputs = inp, outputs = out)\n \n opt = Adam(lr = LR)\n# opt = tfa.optimizers.SWA(opt)\n# model.compile(loss = losses.CategoricalCrossentropy(), optimizer = opt, metrics = ['accuracy'])\n model.compile(loss = categorical_crossentropy, optimizer = opt, metrics = ['accuracy'])\n return model\n\n# function that decrease the learning as epochs increase (i also change this part of the code)\ndef lr_schedule(epoch):\n if epoch < 30:\n lr = LR\n elif epoch < 40:\n lr = LR / 3\n elif epoch < 50:\n lr = LR / 5\n elif epoch < 60:\n lr = LR / 7\n elif epoch < 70:\n lr = LR / 9\n elif epoch < 80:\n lr = LR / 11\n elif epoch < 90:\n lr = LR / 13\n else:\n lr = LR / 100\n return lr\n\n# class to get macro f1 score. This is not entirely necessary but it's fun to check f1 score of each epoch (be carefull, if you use this function early stopping callback will not work)\nclass MacroF1(Callback):\n def __init__(self, model, inputs, targets):\n self.model = model\n self.inputs = inputs\n self.targets = np.argmax(targets, axis = 2).reshape(-1)\n \n def on_epoch_end(self, epoch, logs):\n pred = np.argmax(self.model.predict(self.inputs), axis = 2).reshape(-1)\n score = f1_score(self.targets, pred, average = 'macro')\n print(f'F1 Macro Score: {score:.5f}')\n\n# main function to perfrom groupkfold cross validation (we have 1000 vectores of 4000 rows and 8 features (columns)). Going to make 5 groups with this subgroups.\ndef run_cv_model_by_batch(train, test, splits, batch_col, feats, sample_submission, nn_epochs, nn_batch_size):\n \n seed_everything(SEED)\n K.clear_session()\n# config = tf.compat.v1.ConfigProto(intra_op_parallelism_threads=1, inter_op_parallelism_threads=1, \n# gpu_options=tf.compat.v1.GPUOptions(\n# visible_device_list='4', # specify GPU number\n# allow_growth=True\n# )\n# )\n# sess = tf.compat.v1.Session(graph=tf.compat.v1.get_default_graph(), config=config)\n# tf.compat.v1.keras.backend.set_session(sess)\n # tf.compat.v1 ---> tf (tensorflow2系からtensorflow1系に変更)\n config = tf.ConfigProto(intra_op_parallelism_threads=1, inter_op_parallelism_threads=1, \n# gpu_options=tf.GPUOptions(\n# visible_device_list='4', # specify GPU number\n# allow_growth=True\n# )\n )\n sess = tf.Session(graph=tf.get_default_graph(), config=config)\n tf.keras.backend.set_session(sess)\n oof_ = np.zeros((len(train), 11)) # build out of folds matrix with 11 columns, they represent our target variables classes (from 0 to 10)\n preds_ = np.zeros((len(test), 11))\n target = ['open_channels']\n group = train['group']\n kf = GroupKFold(n_splits=5)\n splits = [x for x in kf.split(train, train[target], group)]\n\n new_splits = []\n for sp in splits:\n new_split = []\n new_split.append(np.unique(group[sp[0]]))\n new_split.append(np.unique(group[sp[1]]))\n new_split.append(sp[1]) \n new_splits.append(new_split)\n # pivot target columns to transform the net to a multiclass classification estructure (you can also leave it in 1 vector with sparsecategoricalcrossentropy loss function)\n tr = pd.concat([pd.get_dummies(train.open_channels), train[['group']]], axis=1)\n\n tr.columns = ['target_'+str(i) for i in range(11)] + ['group']\n target_cols = ['target_'+str(i) for i in range(11)]\n train_tr = np.array(list(tr.groupby('group').apply(lambda x: x[target_cols].values))).astype(np.float32)\n train = np.array(list(train.groupby('group').apply(lambda x: x[feats].values)))\n test = np.array(list(test.groupby('group').apply(lambda x: x[feats].values)))\n\n for n_fold, (tr_idx, val_idx, val_orig_idx) in enumerate(new_splits[0:], start=0):\n train_x, train_y = train[tr_idx], train_tr[tr_idx]\n valid_x, valid_y = train[val_idx], train_tr[val_idx]\n print(f'Our training dataset shape is {train_x.shape}')\n print(f'Our validation dataset shape is {valid_x.shape}')\n\n gc.collect()\n shape_ = (None, train_x.shape[2]) # input is going to be the number of feature we are using (dimension 2 of 0, 1, 2)\n model = Classifier(shape_)\n # using our lr_schedule function\n cb_lr_schedule = LearningRateScheduler(lr_schedule)\n model.fit(train_x,train_y,\n epochs = nn_epochs,\n callbacks = [cb_lr_schedule, MacroF1(model, valid_x, valid_y)], # adding custom evaluation metric for each epoch\n batch_size = nn_batch_size,verbose = 2,\n validation_data = (valid_x,valid_y))\n preds_f = model.predict(valid_x)\n f1_score_ = f1_score(np.argmax(valid_y, axis=2).reshape(-1), np.argmax(preds_f, axis=2).reshape(-1), average = 'macro') # need to get the class with the biggest probability\n print(f'Training fold {n_fold + 1} completed. macro f1 score : {f1_score_ :1.5f}')\n preds_f = preds_f.reshape(-1, preds_f.shape[-1])\n oof_[val_orig_idx,:] += preds_f\n te_preds = model.predict(test)\n te_preds = te_preds.reshape(-1, te_preds.shape[-1]) \n preds_ += te_preds / SPLITS\n # calculate the oof macro f1_score\n f1_score_ = f1_score(np.argmax(train_tr, axis = 2).reshape(-1), np.argmax(oof_, axis = 1), average = 'macro') # axis 2 for the 3 Dimension array and axis 1 for the 2 Domension Array (extracting the best class)\n print(f'Training completed. oof macro f1 score : {f1_score_:1.5f}')\n save_path = f'{DIR_OUTPUT}submission_nb{NB}_cv_{f1_score_:.4f}.csv'\n print(f'save path: {save_path}')\n sample_submission['open_channels'] = np.argmax(preds_, axis = 1).astype(int)\n sample_submission.to_csv(save_path, index=False, float_format='%.4f')\n\n# save_path = f'{DIR_OUTPUT}oof_nb{NB}_cv_{f1_score_:.4f}.csv'\n# sample_submission['open_channels'] = np.argmax(preds_, axis = 1).astype(int)\n# sample_submission.to_csv(save_path, index=False, float_format='%.4f')\n return oof_",
"_____no_output_____"
],
[
"%%time\n# this function run our entire program\ndef run_everything():\n \n print(f'Reading Data Started...({time.ctime()})')\n train, test, sample_submission = read_data()\n train, test = normalize(train, test)\n print(f'Reading and Normalizing Data Completed')\n \n print(f'Creating Features({time.ctime()})')\n print(f'Feature Engineering Started...')\n train = run_feat_engineering(train, batch_size = GROUP_BATCH_SIZE)\n test = run_feat_engineering(test, batch_size = GROUP_BATCH_SIZE)\n train, test, features = feature_selection(train, test)\n print(f'Feature Engineering Completed...')\n \n \n print(f'Training Wavenet model with {SPLITS} folds of GroupKFold Started...({time.ctime()})')\n oof_ = run_cv_model_by_batch(train, test, SPLITS, 'group', features, sample_submission, EPOCHS, NNBATCHSIZE)\n print(f'Training completed...')\n \n return oof_\n \noof_ = run_everything()",
"Reading Data Started...(Wed May 6 11:15:32 2020)\nReading and Normalizing Data Completed\nCreating Features(Wed May 6 11:15:36 2020)\nFeature Engineering Started...\nFeature Engineering Completed...\nTraining Wavenet model with 6 folds of GroupKFold Started...(Wed May 6 11:15:46 2020)\nOur training dataset shape is (1000, 4000, 19)\nOur validation dataset shape is (250, 4000, 19)\nTrain on 1000 samples, validate on 250 samples\nEpoch 1/180\nF1 Macro Score: 0.65447\n - 23s - loss: 0.6088 - acc: 0.8310 - val_loss: 0.8985 - val_acc: 0.8545\nEpoch 2/180\nF1 Macro Score: 0.86893\n - 8s - loss: 0.1985 - acc: 0.9559 - val_loss: 0.4352 - val_acc: 0.9475\nEpoch 3/180\nF1 Macro Score: 0.93159\n - 9s - loss: 0.1436 - acc: 0.9642 - val_loss: 0.2295 - val_acc: 0.9642\nEpoch 4/180\nF1 Macro Score: 0.93344\n - 8s - loss: 0.1296 - acc: 0.9656 - val_loss: 0.1473 - val_acc: 0.9650\nEpoch 5/180\nF1 Macro Score: 0.93503\n - 9s - loss: 0.1221 - acc: 0.9661 - val_loss: 0.1301 - val_acc: 0.9655\nEpoch 6/180\nF1 Macro Score: 0.93693\n - 9s - loss: 0.1177 - acc: 0.9665 - val_loss: 0.1105 - val_acc: 0.9663\nEpoch 7/180\nF1 Macro Score: 0.93668\n - 9s - loss: 0.1130 - acc: 0.9667 - val_loss: 0.1085 - val_acc: 0.9662\nEpoch 8/180\nF1 Macro Score: 0.93671\n - 9s - loss: 0.1092 - acc: 0.9670 - val_loss: 0.1023 - val_acc: 0.9665\nEpoch 9/180\nF1 Macro Score: 0.93550\n - 9s - loss: 0.1081 - acc: 0.9670 - val_loss: 0.1055 - val_acc: 0.9657\nEpoch 10/180\nF1 Macro Score: 0.93486\n - 9s - loss: 0.1074 - acc: 0.9668 - val_loss: 0.1144 - val_acc: 0.9654\nEpoch 11/180\nF1 Macro Score: 0.93078\n - 9s - loss: 0.1196 - acc: 0.9654 - val_loss: 0.1083 - val_acc: 0.9652\nEpoch 12/180\nF1 Macro Score: 0.93584\n - 9s - loss: 0.1153 - acc: 0.9659 - val_loss: 0.1019 - val_acc: 0.9663\nEpoch 13/180\nF1 Macro Score: 0.93794\n - 9s - loss: 0.1044 - acc: 0.9672 - val_loss: 0.0958 - val_acc: 0.9668\nEpoch 14/180\nF1 Macro Score: 0.93633\n - 9s - loss: 0.1035 - acc: 0.9671 - val_loss: 0.0983 - val_acc: 0.9661\nEpoch 15/180\nF1 Macro Score: 0.93802\n - 8s - loss: 0.1002 - acc: 0.9675 - val_loss: 0.0938 - val_acc: 0.9670\nEpoch 16/180\nF1 Macro Score: 0.93670\n - 9s - loss: 0.1003 - acc: 0.9674 - val_loss: 0.0964 - val_acc: 0.9663\nEpoch 17/180\nF1 Macro Score: 0.93737\n - 9s - loss: 0.0981 - acc: 0.9675 - val_loss: 0.0938 - val_acc: 0.9666\nEpoch 18/180\nF1 Macro Score: 0.93661\n - 8s - loss: 0.0974 - acc: 0.9676 - val_loss: 0.0931 - val_acc: 0.9668\nEpoch 19/180\nF1 Macro Score: 0.93760\n - 8s - loss: 0.0965 - acc: 0.9676 - val_loss: 0.0917 - val_acc: 0.9668\nEpoch 20/180\nF1 Macro Score: 0.93758\n - 8s - loss: 0.0949 - acc: 0.9677 - val_loss: 0.0928 - val_acc: 0.9667\nEpoch 21/180\nF1 Macro Score: 0.93429\n - 8s - loss: 0.0953 - acc: 0.9676 - val_loss: 0.0972 - val_acc: 0.9658\nEpoch 22/180\nF1 Macro Score: 0.93760\n - 8s - loss: 0.0953 - acc: 0.9675 - val_loss: 0.0915 - val_acc: 0.9669\nEpoch 23/180\nF1 Macro Score: 0.93586\n - 8s - loss: 0.0939 - acc: 0.9678 - val_loss: 0.0946 - val_acc: 0.9663\nEpoch 24/180\nF1 Macro Score: 0.93759\n - 9s - loss: 0.0925 - acc: 0.9679 - val_loss: 0.0912 - val_acc: 0.9667\nEpoch 25/180\nF1 Macro Score: 0.93796\n - 8s - loss: 0.0923 - acc: 0.9680 - val_loss: 0.0902 - val_acc: 0.9669\nEpoch 26/180\nF1 Macro Score: 0.93698\n - 9s - loss: 0.0919 - acc: 0.9680 - val_loss: 0.0913 - val_acc: 0.9663\nEpoch 27/180\nF1 Macro Score: 0.93641\n - 9s - loss: 0.0948 - acc: 0.9675 - val_loss: 0.0920 - val_acc: 0.9667\nEpoch 28/180\nF1 Macro Score: 0.93774\n - 9s - loss: 0.0912 - acc: 0.9681 - val_loss: 0.0898 - val_acc: 0.9670\nEpoch 29/180\nF1 Macro Score: 0.93779\n - 7s - loss: 0.0909 - acc: 0.9680 - val_loss: 0.0908 - val_acc: 0.9668\nEpoch 30/180\nF1 Macro Score: 0.93654\n - 8s - loss: 0.0899 - acc: 0.9681 - val_loss: 0.0926 - val_acc: 0.9661\nEpoch 31/180\nF1 Macro Score: 0.93856\n - 7s - loss: 0.0893 - acc: 0.9684 - val_loss: 0.0882 - val_acc: 0.9673\nEpoch 32/180\nF1 Macro Score: 0.93897\n - 9s - loss: 0.0873 - acc: 0.9688 - val_loss: 0.0871 - val_acc: 0.9676\nEpoch 33/180\nF1 Macro Score: 0.93890\n - 8s - loss: 0.0866 - acc: 0.9688 - val_loss: 0.0870 - val_acc: 0.9676\nEpoch 34/180\nF1 Macro Score: 0.93929\n - 8s - loss: 0.0865 - acc: 0.9689 - val_loss: 0.0859 - val_acc: 0.9678\nEpoch 35/180\nF1 Macro Score: 0.93905\n - 8s - loss: 0.0864 - acc: 0.9689 - val_loss: 0.0863 - val_acc: 0.9676\nEpoch 36/180\nF1 Macro Score: 0.93888\n - 8s - loss: 0.0860 - acc: 0.9691 - val_loss: 0.0865 - val_acc: 0.9675\nEpoch 37/180\nF1 Macro Score: 0.93732\n - 9s - loss: 0.0855 - acc: 0.9692 - val_loss: 0.0900 - val_acc: 0.9668\nEpoch 38/180\nF1 Macro Score: 0.93992\n - 8s - loss: 0.0847 - acc: 0.9693 - val_loss: 0.0851 - val_acc: 0.9681\nEpoch 39/180\nF1 Macro Score: 0.93891\n - 8s - loss: 0.0873 - acc: 0.9689 - val_loss: 0.0860 - val_acc: 0.9677\nEpoch 40/180\nF1 Macro Score: 0.93900\n - 9s - loss: 0.0849 - acc: 0.9693 - val_loss: 0.0862 - val_acc: 0.9677\nEpoch 41/180\nF1 Macro Score: 0.94022\n - 8s - loss: 0.0842 - acc: 0.9696 - val_loss: 0.0845 - val_acc: 0.9682\nEpoch 42/180\nF1 Macro Score: 0.94046\n - 9s - loss: 0.0832 - acc: 0.9697 - val_loss: 0.0842 - val_acc: 0.9684\nEpoch 43/180\nF1 Macro Score: 0.93883\n - 9s - loss: 0.0835 - acc: 0.9697 - val_loss: 0.0861 - val_acc: 0.9679\nEpoch 44/180\nF1 Macro Score: 0.94063\n - 9s - loss: 0.0831 - acc: 0.9697 - val_loss: 0.0841 - val_acc: 0.9684\nEpoch 45/180\nF1 Macro Score: 0.93997\n - 8s - loss: 0.0831 - acc: 0.9698 - val_loss: 0.0846 - val_acc: 0.9682\nEpoch 46/180\nF1 Macro Score: 0.93945\n - 7s - loss: 0.0831 - acc: 0.9699 - val_loss: 0.0854 - val_acc: 0.9680\nEpoch 47/180\nF1 Macro Score: 0.94035\n - 8s - loss: 0.0829 - acc: 0.9699 - val_loss: 0.0840 - val_acc: 0.9683\nEpoch 48/180\nF1 Macro Score: 0.94079\n - 8s - loss: 0.0826 - acc: 0.9699 - val_loss: 0.0837 - val_acc: 0.9686\nEpoch 49/180\nF1 Macro Score: 0.93997\n - 9s - loss: 0.0823 - acc: 0.9700 - val_loss: 0.0846 - val_acc: 0.9681\nEpoch 50/180\nF1 Macro Score: 0.94037\n - 7s - loss: 0.0821 - acc: 0.9700 - val_loss: 0.0844 - val_acc: 0.9683\nEpoch 51/180\nF1 Macro Score: 0.94070\n - 8s - loss: 0.0825 - acc: 0.9699 - val_loss: 0.0836 - val_acc: 0.9685\nEpoch 52/180\nF1 Macro Score: 0.94046\n - 9s - loss: 0.0815 - acc: 0.9702 - val_loss: 0.0837 - val_acc: 0.9685\nEpoch 53/180\nF1 Macro Score: 0.94078\n - 9s - loss: 0.0816 - acc: 0.9702 - val_loss: 0.0836 - val_acc: 0.9686\nEpoch 54/180\nF1 Macro Score: 0.93971\n - 9s - loss: 0.0818 - acc: 0.9701 - val_loss: 0.0847 - val_acc: 0.9682\nEpoch 55/180\nF1 Macro Score: 0.94058\n - 9s - loss: 0.0817 - acc: 0.9701 - val_loss: 0.0838 - val_acc: 0.9684\nEpoch 56/180\nF1 Macro Score: 0.94053\n - 8s - loss: 0.0817 - acc: 0.9701 - val_loss: 0.0840 - val_acc: 0.9684\nEpoch 57/180\nF1 Macro Score: 0.94036\n - 9s - loss: 0.0809 - acc: 0.9703 - val_loss: 0.0836 - val_acc: 0.9684\nEpoch 58/180\nF1 Macro Score: 0.93987\n - 9s - loss: 0.0814 - acc: 0.9701 - val_loss: 0.0847 - val_acc: 0.9681\nEpoch 59/180\nF1 Macro Score: 0.94029\n - 9s - loss: 0.0821 - acc: 0.9699 - val_loss: 0.0844 - val_acc: 0.9683\nEpoch 60/180\nF1 Macro Score: 0.94081\n - 9s - loss: 0.0833 - acc: 0.9697 - val_loss: 0.0841 - val_acc: 0.9685\nEpoch 61/180\nF1 Macro Score: 0.94069\n - 8s - loss: 0.0808 - acc: 0.9704 - val_loss: 0.0837 - val_acc: 0.9685\nEpoch 62/180\nF1 Macro Score: 0.94080\n - 9s - loss: 0.0812 - acc: 0.9702 - val_loss: 0.0834 - val_acc: 0.9686\nEpoch 63/180\nF1 Macro Score: 0.94053\n - 8s - loss: 0.0809 - acc: 0.9703 - val_loss: 0.0835 - val_acc: 0.9686\nEpoch 64/180\nF1 Macro Score: 0.94075\n - 9s - loss: 0.0805 - acc: 0.9704 - val_loss: 0.0835 - val_acc: 0.9685\nEpoch 65/180\nF1 Macro Score: 0.94064\n - 8s - loss: 0.0806 - acc: 0.9704 - val_loss: 0.0837 - val_acc: 0.9685\nEpoch 66/180\nF1 Macro Score: 0.93868\n - 9s - loss: 0.0797 - acc: 0.9705 - val_loss: 0.0847 - val_acc: 0.9681\nEpoch 67/180\nF1 Macro Score: 0.94083\n - 8s - loss: 0.0802 - acc: 0.9704 - val_loss: 0.0835 - val_acc: 0.9686\nEpoch 68/180\nF1 Macro Score: 0.94019\n - 9s - loss: 0.0799 - acc: 0.9705 - val_loss: 0.0835 - val_acc: 0.9685\nEpoch 69/180\nF1 Macro Score: 0.94036\n - 9s - loss: 0.0799 - acc: 0.9705 - val_loss: 0.0838 - val_acc: 0.9684\nEpoch 70/180\nF1 Macro Score: 0.94067\n - 8s - loss: 0.0801 - acc: 0.9705 - val_loss: 0.0835 - val_acc: 0.9685\nEpoch 71/180\nF1 Macro Score: 0.94032\n - 9s - loss: 0.0800 - acc: 0.9705 - val_loss: 0.0836 - val_acc: 0.9684\nEpoch 72/180\nF1 Macro Score: 0.94037\n - 9s - loss: 0.0798 - acc: 0.9706 - val_loss: 0.0836 - val_acc: 0.9684\nEpoch 73/180\nF1 Macro Score: 0.94060\n - 8s - loss: 0.0798 - acc: 0.9706 - val_loss: 0.0834 - val_acc: 0.9685\nEpoch 74/180\nF1 Macro Score: 0.94058\n - 9s - loss: 0.0790 - acc: 0.9707 - val_loss: 0.0836 - val_acc: 0.9685\nEpoch 75/180\nF1 Macro Score: 0.94053\n - 9s - loss: 0.0798 - acc: 0.9705 - val_loss: 0.0834 - val_acc: 0.9684\nEpoch 76/180\nF1 Macro Score: 0.94075\n - 9s - loss: 0.0791 - acc: 0.9707 - val_loss: 0.0834 - val_acc: 0.9685\nEpoch 77/180\nF1 Macro Score: 0.94047\n - 8s - loss: 0.0792 - acc: 0.9706 - val_loss: 0.0837 - val_acc: 0.9684\nEpoch 78/180\nF1 Macro Score: 0.94027\n - 9s - loss: 0.0790 - acc: 0.9708 - val_loss: 0.0836 - val_acc: 0.9683\nEpoch 79/180\nF1 Macro Score: 0.94051\n - 9s - loss: 0.0791 - acc: 0.9706 - val_loss: 0.0836 - val_acc: 0.9684\nEpoch 80/180\nF1 Macro Score: 0.94027\n - 9s - loss: 0.0790 - acc: 0.9707 - val_loss: 0.0839 - val_acc: 0.9683\nEpoch 81/180\nF1 Macro Score: 0.94077\n - 8s - loss: 0.0783 - acc: 0.9708 - val_loss: 0.0833 - val_acc: 0.9685\nEpoch 82/180\nF1 Macro Score: 0.94056\n - 9s - loss: 0.0792 - acc: 0.9707 - val_loss: 0.0834 - val_acc: 0.9685\nEpoch 83/180\nF1 Macro Score: 0.94059\n - 8s - loss: 0.0785 - acc: 0.9708 - val_loss: 0.0833 - val_acc: 0.9685\nEpoch 84/180\nF1 Macro Score: 0.94060\n - 9s - loss: 0.0788 - acc: 0.9708 - val_loss: 0.0837 - val_acc: 0.9684\nEpoch 85/180\nF1 Macro Score: 0.94033\n - 9s - loss: 0.0785 - acc: 0.9708 - val_loss: 0.0839 - val_acc: 0.9683\nEpoch 86/180\nF1 Macro Score: 0.94054\n - 8s - loss: 0.0787 - acc: 0.9708 - val_loss: 0.0836 - val_acc: 0.9684\nEpoch 87/180\nF1 Macro Score: 0.94048\n - 8s - loss: 0.0789 - acc: 0.9707 - val_loss: 0.0839 - val_acc: 0.9682\nEpoch 88/180\nF1 Macro Score: 0.94024\n - 9s - loss: 0.0784 - acc: 0.9709 - val_loss: 0.0835 - val_acc: 0.9684\nEpoch 89/180\nF1 Macro Score: 0.94008\n - 9s - loss: 0.0781 - acc: 0.9708 - val_loss: 0.0840 - val_acc: 0.9682\nEpoch 90/180\nF1 Macro Score: 0.94065\n - 7s - loss: 0.0782 - acc: 0.9709 - val_loss: 0.0834 - val_acc: 0.9684\nEpoch 91/180\nF1 Macro Score: 0.94085\n - 8s - loss: 0.0779 - acc: 0.9711 - val_loss: 0.0832 - val_acc: 0.9685\nEpoch 92/180\nF1 Macro Score: 0.94063\n - 8s - loss: 0.0776 - acc: 0.9711 - val_loss: 0.0832 - val_acc: 0.9685\nEpoch 93/180\nF1 Macro Score: 0.94070\n - 8s - loss: 0.0775 - acc: 0.9712 - val_loss: 0.0832 - val_acc: 0.9685\nEpoch 94/180\nF1 Macro Score: 0.94071\n - 9s - loss: 0.0779 - acc: 0.9711 - val_loss: 0.0832 - val_acc: 0.9685\nEpoch 95/180\nF1 Macro Score: 0.94068\n - 9s - loss: 0.0776 - acc: 0.9711 - val_loss: 0.0832 - val_acc: 0.9685\nEpoch 96/180\nF1 Macro Score: 0.94070\n - 9s - loss: 0.0775 - acc: 0.9711 - val_loss: 0.0833 - val_acc: 0.9686\nEpoch 97/180\nF1 Macro Score: 0.94063\n - 9s - loss: 0.0773 - acc: 0.9712 - val_loss: 0.0832 - val_acc: 0.9685\nEpoch 98/180\nF1 Macro Score: 0.94082\n - 9s - loss: 0.0778 - acc: 0.9711 - val_loss: 0.0833 - val_acc: 0.9686\nEpoch 99/180\nF1 Macro Score: 0.94078\n - 8s - loss: 0.0772 - acc: 0.9711 - val_loss: 0.0833 - val_acc: 0.9685\nEpoch 100/180\nF1 Macro Score: 0.94085\n - 9s - loss: 0.0779 - acc: 0.9710 - val_loss: 0.0832 - val_acc: 0.9685\nEpoch 101/180\nF1 Macro Score: 0.94062\n - 8s - loss: 0.0777 - acc: 0.9711 - val_loss: 0.0833 - val_acc: 0.9685\nEpoch 102/180\nF1 Macro Score: 0.94061\n - 9s - loss: 0.0777 - acc: 0.9711 - val_loss: 0.0833 - val_acc: 0.9684\nEpoch 103/180\nF1 Macro Score: 0.94059\n - 8s - loss: 0.0776 - acc: 0.9711 - val_loss: 0.0833 - val_acc: 0.9685\nEpoch 104/180\nF1 Macro Score: 0.94073\n - 9s - loss: 0.0775 - acc: 0.9711 - val_loss: 0.0832 - val_acc: 0.9685\nEpoch 105/180\nF1 Macro Score: 0.94066\n - 9s - loss: 0.0773 - acc: 0.9712 - val_loss: 0.0833 - val_acc: 0.9685\nEpoch 106/180\nF1 Macro Score: 0.94070\n - 9s - loss: 0.0776 - acc: 0.9711 - val_loss: 0.0832 - val_acc: 0.9685\nEpoch 107/180\nF1 Macro Score: 0.94068\n - 9s - loss: 0.0774 - acc: 0.9711 - val_loss: 0.0833 - val_acc: 0.9685\nEpoch 108/180\nF1 Macro Score: 0.94058\n - 9s - loss: 0.0774 - acc: 0.9711 - val_loss: 0.0833 - val_acc: 0.9684\nEpoch 109/180\nF1 Macro Score: 0.94079\n - 9s - loss: 0.0773 - acc: 0.9712 - val_loss: 0.0833 - val_acc: 0.9685\nEpoch 110/180\nF1 Macro Score: 0.94069\n - 9s - loss: 0.0775 - acc: 0.9711 - val_loss: 0.0833 - val_acc: 0.9685\nEpoch 111/180\nF1 Macro Score: 0.94061\n - 9s - loss: 0.0775 - acc: 0.9712 - val_loss: 0.0833 - val_acc: 0.9684\nEpoch 112/180\nF1 Macro Score: 0.94073\n - 9s - loss: 0.0776 - acc: 0.9711 - val_loss: 0.0833 - val_acc: 0.9685\nEpoch 113/180\nF1 Macro Score: 0.94052\n - 8s - loss: 0.0770 - acc: 0.9712 - val_loss: 0.0833 - val_acc: 0.9684\nEpoch 114/180\nF1 Macro Score: 0.94066\n - 9s - loss: 0.0774 - acc: 0.9711 - val_loss: 0.0834 - val_acc: 0.9685\nEpoch 115/180\nF1 Macro Score: 0.94057\n - 7s - loss: 0.0776 - acc: 0.9710 - val_loss: 0.0833 - val_acc: 0.9684\nEpoch 116/180\nF1 Macro Score: 0.94074\n - 7s - loss: 0.0773 - acc: 0.9711 - val_loss: 0.0833 - val_acc: 0.9685\nEpoch 117/180\nF1 Macro Score: 0.94060\n - 7s - loss: 0.0775 - acc: 0.9711 - val_loss: 0.0833 - val_acc: 0.9685\nEpoch 118/180\nF1 Macro Score: 0.94070\n - 7s - loss: 0.0771 - acc: 0.9712 - val_loss: 0.0833 - val_acc: 0.9685\nEpoch 119/180\nF1 Macro Score: 0.94060\n - 7s - loss: 0.0776 - acc: 0.9711 - val_loss: 0.0834 - val_acc: 0.9684\nEpoch 120/180\nF1 Macro Score: 0.94052\n - 7s - loss: 0.0769 - acc: 0.9712 - val_loss: 0.0834 - val_acc: 0.9684\nEpoch 121/180\nF1 Macro Score: 0.94071\n - 7s - loss: 0.0775 - acc: 0.9712 - val_loss: 0.0833 - val_acc: 0.9685\nEpoch 122/180\nF1 Macro Score: 0.94047\n - 7s - loss: 0.0770 - acc: 0.9712 - val_loss: 0.0834 - val_acc: 0.9684\nEpoch 123/180\nF1 Macro Score: 0.94076\n - 7s - loss: 0.0777 - acc: 0.9711 - val_loss: 0.0833 - val_acc: 0.9685\nEpoch 124/180\nF1 Macro Score: 0.94069\n - 7s - loss: 0.0774 - acc: 0.9711 - val_loss: 0.0833 - val_acc: 0.9685\nEpoch 125/180\nF1 Macro Score: 0.94077\n - 7s - loss: 0.0774 - acc: 0.9712 - val_loss: 0.0834 - val_acc: 0.9684\nEpoch 126/180\nF1 Macro Score: 0.94069\n - 7s - loss: 0.0775 - acc: 0.9711 - val_loss: 0.0833 - val_acc: 0.9685\nEpoch 127/180\nF1 Macro Score: 0.94083\n - 7s - loss: 0.0774 - acc: 0.9711 - val_loss: 0.0833 - val_acc: 0.9685\nEpoch 128/180\nF1 Macro Score: 0.94051\n - 7s - loss: 0.0773 - acc: 0.9712 - val_loss: 0.0834 - val_acc: 0.9684\nEpoch 129/180\nF1 Macro Score: 0.94071\n - 7s - loss: 0.0774 - acc: 0.9712 - val_loss: 0.0834 - val_acc: 0.9685\nEpoch 130/180\nF1 Macro Score: 0.94071\n - 7s - loss: 0.0772 - acc: 0.9712 - val_loss: 0.0833 - val_acc: 0.9684\nEpoch 131/180\nF1 Macro Score: 0.94064\n - 7s - loss: 0.0771 - acc: 0.9712 - val_loss: 0.0834 - val_acc: 0.9685\nEpoch 132/180\nF1 Macro Score: 0.94044\n - 7s - loss: 0.0772 - acc: 0.9712 - val_loss: 0.0834 - val_acc: 0.9684\nEpoch 133/180\nF1 Macro Score: 0.94075\n - 7s - loss: 0.0771 - acc: 0.9712 - val_loss: 0.0834 - val_acc: 0.9685\nEpoch 134/180\nF1 Macro Score: 0.94061\n - 7s - loss: 0.0770 - acc: 0.9712 - val_loss: 0.0834 - val_acc: 0.9684\nEpoch 135/180\nF1 Macro Score: 0.94037\n - 7s - loss: 0.0775 - acc: 0.9712 - val_loss: 0.0835 - val_acc: 0.9684\nEpoch 136/180\nF1 Macro Score: 0.94069\n - 7s - loss: 0.0772 - acc: 0.9712 - val_loss: 0.0834 - val_acc: 0.9685\nEpoch 137/180\nF1 Macro Score: 0.94017\n - 7s - loss: 0.0770 - acc: 0.9712 - val_loss: 0.0835 - val_acc: 0.9683\nEpoch 138/180\nF1 Macro Score: 0.94080\n - 7s - loss: 0.0772 - acc: 0.9712 - val_loss: 0.0834 - val_acc: 0.9685\nEpoch 139/180\nF1 Macro Score: 0.94065\n - 7s - loss: 0.0773 - acc: 0.9712 - val_loss: 0.0834 - val_acc: 0.9685\nEpoch 140/180\nF1 Macro Score: 0.94053\n - 7s - loss: 0.0771 - acc: 0.9712 - val_loss: 0.0834 - val_acc: 0.9684\nEpoch 141/180\nF1 Macro Score: 0.94074\n - 7s - loss: 0.0770 - acc: 0.9712 - val_loss: 0.0834 - val_acc: 0.9685\nEpoch 142/180\nF1 Macro Score: 0.94064\n - 7s - loss: 0.0769 - acc: 0.9713 - val_loss: 0.0833 - val_acc: 0.9685\nEpoch 143/180\nF1 Macro Score: 0.94073\n - 7s - loss: 0.0771 - acc: 0.9712 - val_loss: 0.0834 - val_acc: 0.9685\nEpoch 144/180\nF1 Macro Score: 0.94055\n - 7s - loss: 0.0770 - acc: 0.9712 - val_loss: 0.0834 - val_acc: 0.9684\nEpoch 145/180\nF1 Macro Score: 0.94076\n - 7s - loss: 0.0773 - acc: 0.9711 - val_loss: 0.0834 - val_acc: 0.9685\nEpoch 146/180\nF1 Macro Score: 0.94054\n - 7s - loss: 0.0770 - acc: 0.9712 - val_loss: 0.0834 - val_acc: 0.9684\nEpoch 147/180\nF1 Macro Score: 0.94072\n - 7s - loss: 0.0771 - acc: 0.9712 - val_loss: 0.0834 - val_acc: 0.9685\nEpoch 148/180\nF1 Macro Score: 0.94065\n - 7s - loss: 0.0771 - acc: 0.9712 - val_loss: 0.0834 - val_acc: 0.9684\nEpoch 149/180\nF1 Macro Score: 0.94073\n - 7s - loss: 0.0773 - acc: 0.9712 - val_loss: 0.0835 - val_acc: 0.9684\nEpoch 150/180\nF1 Macro Score: 0.94070\n - 7s - loss: 0.0769 - acc: 0.9712 - val_loss: 0.0834 - val_acc: 0.9685\nEpoch 151/180\nF1 Macro Score: 0.94068\n - 7s - loss: 0.0769 - acc: 0.9713 - val_loss: 0.0834 - val_acc: 0.9685\nEpoch 152/180\nF1 Macro Score: 0.94055\n - 7s - loss: 0.0769 - acc: 0.9713 - val_loss: 0.0835 - val_acc: 0.9684\nEpoch 153/180\nF1 Macro Score: 0.94060\n - 7s - loss: 0.0772 - acc: 0.9712 - val_loss: 0.0835 - val_acc: 0.9684\nEpoch 154/180\nF1 Macro Score: 0.94058\n - 8s - loss: 0.0768 - acc: 0.9713 - val_loss: 0.0834 - val_acc: 0.9684\nEpoch 155/180\nF1 Macro Score: 0.94071\n - 8s - loss: 0.0773 - acc: 0.9712 - val_loss: 0.0834 - val_acc: 0.9685\nEpoch 156/180\nF1 Macro Score: 0.94062\n - 7s - loss: 0.0770 - acc: 0.9712 - val_loss: 0.0834 - val_acc: 0.9684\nEpoch 157/180\nF1 Macro Score: 0.94070\n - 7s - loss: 0.0772 - acc: 0.9712 - val_loss: 0.0834 - val_acc: 0.9685\nEpoch 158/180\nF1 Macro Score: 0.94057\n - 7s - loss: 0.0769 - acc: 0.9713 - val_loss: 0.0835 - val_acc: 0.9684\nEpoch 159/180\nF1 Macro Score: 0.94049\n - 7s - loss: 0.0766 - acc: 0.9713 - val_loss: 0.0835 - val_acc: 0.9684\nEpoch 160/180\nF1 Macro Score: 0.94061\n - 7s - loss: 0.0767 - acc: 0.9713 - val_loss: 0.0835 - val_acc: 0.9684\nEpoch 161/180\nF1 Macro Score: 0.94060\n - 7s - loss: 0.0767 - acc: 0.9713 - val_loss: 0.0835 - val_acc: 0.9684\nEpoch 162/180\nF1 Macro Score: 0.94046\n - 7s - loss: 0.0772 - acc: 0.9712 - val_loss: 0.0835 - val_acc: 0.9684\nEpoch 163/180\nF1 Macro Score: 0.94053\n - 7s - loss: 0.0769 - acc: 0.9713 - val_loss: 0.0835 - val_acc: 0.9684\nEpoch 164/180\nF1 Macro Score: 0.94036\n - 7s - loss: 0.0768 - acc: 0.9712 - val_loss: 0.0835 - val_acc: 0.9684\nEpoch 165/180\nF1 Macro Score: 0.94063\n - 7s - loss: 0.0767 - acc: 0.9713 - val_loss: 0.0835 - val_acc: 0.9684\nEpoch 166/180\nF1 Macro Score: 0.94062\n - 7s - loss: 0.0766 - acc: 0.9714 - val_loss: 0.0834 - val_acc: 0.9684\nEpoch 167/180\nF1 Macro Score: 0.94027\n - 7s - loss: 0.0767 - acc: 0.9713 - val_loss: 0.0835 - val_acc: 0.9683\nEpoch 168/180\nF1 Macro Score: 0.94071\n - 7s - loss: 0.0769 - acc: 0.9713 - val_loss: 0.0835 - val_acc: 0.9685\nEpoch 169/180\nF1 Macro Score: 0.94061\n - 7s - loss: 0.0767 - acc: 0.9713 - val_loss: 0.0835 - val_acc: 0.9684\nEpoch 170/180\nF1 Macro Score: 0.94066\n - 7s - loss: 0.0767 - acc: 0.9713 - val_loss: 0.0835 - val_acc: 0.9685\nEpoch 171/180\nF1 Macro Score: 0.94061\n - 7s - loss: 0.0775 - acc: 0.9712 - val_loss: 0.0835 - val_acc: 0.9684\nEpoch 172/180\nF1 Macro Score: 0.94064\n - 7s - loss: 0.0766 - acc: 0.9713 - val_loss: 0.0835 - val_acc: 0.9684\nEpoch 173/180\nF1 Macro Score: 0.94058\n - 7s - loss: 0.0767 - acc: 0.9713 - val_loss: 0.0835 - val_acc: 0.9684\nEpoch 174/180\nF1 Macro Score: 0.94054\n - 7s - loss: 0.0768 - acc: 0.9713 - val_loss: 0.0835 - val_acc: 0.9684\nEpoch 175/180\nF1 Macro Score: 0.94059\n - 7s - loss: 0.0767 - acc: 0.9715 - val_loss: 0.0835 - val_acc: 0.9684\nEpoch 176/180\nF1 Macro Score: 0.94069\n - 7s - loss: 0.0766 - acc: 0.9713 - val_loss: 0.0835 - val_acc: 0.9685\nEpoch 177/180\nF1 Macro Score: 0.94052\n - 7s - loss: 0.0767 - acc: 0.9712 - val_loss: 0.0837 - val_acc: 0.9684\nEpoch 178/180\nF1 Macro Score: 0.94053\n - 7s - loss: 0.0767 - acc: 0.9713 - val_loss: 0.0835 - val_acc: 0.9684\nEpoch 179/180\nF1 Macro Score: 0.94058\n - 7s - loss: 0.0765 - acc: 0.9714 - val_loss: 0.0835 - val_acc: 0.9684\nEpoch 180/180\nF1 Macro Score: 0.94059\n - 7s - loss: 0.0769 - acc: 0.9713 - val_loss: 0.0835 - val_acc: 0.9684\nTraining fold 1 completed. macro f1 score : 0.94059\nOur training dataset shape is (1000, 4000, 19)\nOur validation dataset shape is (250, 4000, 19)\nTrain on 1000 samples, validate on 250 samples\nEpoch 1/180\nF1 Macro Score: 0.77329\n - 18s - loss: 0.5534 - acc: 0.8433 - val_loss: 0.7989 - val_acc: 0.9029\nEpoch 2/180\nF1 Macro Score: 0.92960\n - 7s - loss: 0.1838 - acc: 0.9570 - val_loss: 0.3918 - val_acc: 0.9656\nEpoch 3/180\nF1 Macro Score: 0.93509\n - 7s - loss: 0.1376 - acc: 0.9644 - val_loss: 0.1898 - val_acc: 0.9676\nEpoch 4/180\nF1 Macro Score: 0.93554\n - 7s - loss: 0.1277 - acc: 0.9651 - val_loss: 0.1339 - val_acc: 0.9678\nEpoch 5/180\nF1 Macro Score: 0.93814\n - 7s - loss: 0.1222 - acc: 0.9656 - val_loss: 0.1057 - val_acc: 0.9690\nEpoch 6/180\nF1 Macro Score: 0.93775\n - 7s - loss: 0.1159 - acc: 0.9660 - val_loss: 0.0999 - val_acc: 0.9690\nEpoch 7/180\nF1 Macro Score: 0.93842\n - 7s - loss: 0.1120 - acc: 0.9662 - val_loss: 0.0940 - val_acc: 0.9693\nEpoch 8/180\nF1 Macro Score: 0.93867\n - 7s - loss: 0.1109 - acc: 0.9662 - val_loss: 0.0919 - val_acc: 0.9693\nEpoch 9/180\nF1 Macro Score: 0.93819\n - 7s - loss: 0.1076 - acc: 0.9664 - val_loss: 0.0924 - val_acc: 0.9691\nEpoch 10/180\nF1 Macro Score: 0.93710\n - 7s - loss: 0.1076 - acc: 0.9663 - val_loss: 0.0942 - val_acc: 0.9688\nEpoch 11/180\nF1 Macro Score: 0.93789\n - 7s - loss: 0.1061 - acc: 0.9663 - val_loss: 0.0914 - val_acc: 0.9690\nEpoch 12/180\nF1 Macro Score: 0.93780\n - 8s - loss: 0.1042 - acc: 0.9664 - val_loss: 0.0916 - val_acc: 0.9689\nEpoch 13/180\nF1 Macro Score: 0.93929\n - 8s - loss: 0.1018 - acc: 0.9666 - val_loss: 0.0876 - val_acc: 0.9696\nEpoch 14/180\nF1 Macro Score: 0.93938\n - 8s - loss: 0.1015 - acc: 0.9666 - val_loss: 0.0865 - val_acc: 0.9696\nEpoch 15/180\nF1 Macro Score: 0.93749\n - 7s - loss: 0.1001 - acc: 0.9666 - val_loss: 0.0906 - val_acc: 0.9687\nEpoch 16/180\nF1 Macro Score: 0.93876\n - 8s - loss: 0.1003 - acc: 0.9666 - val_loss: 0.0869 - val_acc: 0.9694\nEpoch 17/180\nF1 Macro Score: 0.93847\n - 7s - loss: 0.0998 - acc: 0.9666 - val_loss: 0.0873 - val_acc: 0.9694\nEpoch 18/180\nF1 Macro Score: 0.93730\n - 8s - loss: 0.0978 - acc: 0.9668 - val_loss: 0.0892 - val_acc: 0.9687\nEpoch 19/180\nF1 Macro Score: 0.93846\n - 8s - loss: 0.0981 - acc: 0.9667 - val_loss: 0.0852 - val_acc: 0.9694\nEpoch 20/180\nF1 Macro Score: 0.93787\n - 8s - loss: 0.0975 - acc: 0.9666 - val_loss: 0.0870 - val_acc: 0.9691\nEpoch 21/180\nF1 Macro Score: 0.93768\n - 7s - loss: 0.0974 - acc: 0.9667 - val_loss: 0.0907 - val_acc: 0.9687\nEpoch 22/180\nF1 Macro Score: 0.93835\n - 7s - loss: 0.1019 - acc: 0.9663 - val_loss: 0.0862 - val_acc: 0.9692\nEpoch 23/180\nF1 Macro Score: 0.93906\n - 7s - loss: 0.0957 - acc: 0.9669 - val_loss: 0.0834 - val_acc: 0.9696\nEpoch 24/180\nF1 Macro Score: 0.93872\n - 7s - loss: 0.0947 - acc: 0.9669 - val_loss: 0.0846 - val_acc: 0.9694\nEpoch 25/180\nF1 Macro Score: 0.93923\n - 7s - loss: 0.0945 - acc: 0.9670 - val_loss: 0.0828 - val_acc: 0.9697\nEpoch 26/180\nF1 Macro Score: 0.93838\n - 7s - loss: 0.0935 - acc: 0.9670 - val_loss: 0.0841 - val_acc: 0.9693\nEpoch 27/180\nF1 Macro Score: 0.93939\n - 7s - loss: 0.0930 - acc: 0.9670 - val_loss: 0.0833 - val_acc: 0.9696\nEpoch 28/180\nF1 Macro Score: 0.93659\n - 7s - loss: 0.0931 - acc: 0.9670 - val_loss: 0.0964 - val_acc: 0.9669\nEpoch 29/180\nF1 Macro Score: 0.93881\n - 7s - loss: 0.0940 - acc: 0.9671 - val_loss: 0.0835 - val_acc: 0.9694\nEpoch 30/180\nF1 Macro Score: 0.93559\n - 7s - loss: 0.0949 - acc: 0.9668 - val_loss: 0.0986 - val_acc: 0.9676\nEpoch 31/180\nF1 Macro Score: 0.93852\n - 7s - loss: 0.0962 - acc: 0.9669 - val_loss: 0.0836 - val_acc: 0.9695\nEpoch 32/180\nF1 Macro Score: 0.93976\n - 7s - loss: 0.0909 - acc: 0.9675 - val_loss: 0.0811 - val_acc: 0.9699\nEpoch 33/180\nF1 Macro Score: 0.93971\n - 7s - loss: 0.0902 - acc: 0.9675 - val_loss: 0.0811 - val_acc: 0.9699\nEpoch 34/180\nF1 Macro Score: 0.93952\n - 7s - loss: 0.0897 - acc: 0.9676 - val_loss: 0.0808 - val_acc: 0.9698\nEpoch 35/180\nF1 Macro Score: 0.93984\n - 7s - loss: 0.0890 - acc: 0.9676 - val_loss: 0.0806 - val_acc: 0.9700\nEpoch 36/180\nF1 Macro Score: 0.93987\n - 7s - loss: 0.0887 - acc: 0.9677 - val_loss: 0.0803 - val_acc: 0.9700\nEpoch 37/180\nF1 Macro Score: 0.93946\n - 7s - loss: 0.0888 - acc: 0.9678 - val_loss: 0.0816 - val_acc: 0.9698\nEpoch 38/180\nF1 Macro Score: 0.93982\n - 7s - loss: 0.0887 - acc: 0.9678 - val_loss: 0.0802 - val_acc: 0.9701\nEpoch 39/180\nF1 Macro Score: 0.93976\n - 7s - loss: 0.0874 - acc: 0.9681 - val_loss: 0.0805 - val_acc: 0.9701\nEpoch 40/180\nF1 Macro Score: 0.94001\n - 7s - loss: 0.0878 - acc: 0.9680 - val_loss: 0.0798 - val_acc: 0.9702\nEpoch 41/180\nF1 Macro Score: 0.94035\n - 7s - loss: 0.0873 - acc: 0.9681 - val_loss: 0.0791 - val_acc: 0.9703\nEpoch 42/180\nF1 Macro Score: 0.94025\n - 9s - loss: 0.0865 - acc: 0.9683 - val_loss: 0.0791 - val_acc: 0.9703\nEpoch 43/180\nF1 Macro Score: 0.94051\n - 9s - loss: 0.0864 - acc: 0.9683 - val_loss: 0.0787 - val_acc: 0.9705\nEpoch 44/180\nF1 Macro Score: 0.94028\n - 7s - loss: 0.0862 - acc: 0.9683 - val_loss: 0.0791 - val_acc: 0.9704\nEpoch 45/180\nF1 Macro Score: 0.94030\n - 7s - loss: 0.0863 - acc: 0.9684 - val_loss: 0.0793 - val_acc: 0.9703\nEpoch 46/180\nF1 Macro Score: 0.94069\n - 7s - loss: 0.0861 - acc: 0.9684 - val_loss: 0.0787 - val_acc: 0.9705\nEpoch 47/180\nF1 Macro Score: 0.94083\n - 7s - loss: 0.0856 - acc: 0.9686 - val_loss: 0.0786 - val_acc: 0.9706\nEpoch 48/180\nF1 Macro Score: 0.94083\n - 7s - loss: 0.0850 - acc: 0.9688 - val_loss: 0.0782 - val_acc: 0.9706\nEpoch 49/180\nF1 Macro Score: 0.94124\n - 7s - loss: 0.0847 - acc: 0.9689 - val_loss: 0.0778 - val_acc: 0.9708\nEpoch 50/180\nF1 Macro Score: 0.94096\n - 7s - loss: 0.0850 - acc: 0.9688 - val_loss: 0.0784 - val_acc: 0.9706\nEpoch 51/180\nF1 Macro Score: 0.94140\n - 7s - loss: 0.0845 - acc: 0.9689 - val_loss: 0.0780 - val_acc: 0.9708\nEpoch 52/180\nF1 Macro Score: 0.94145\n - 7s - loss: 0.0837 - acc: 0.9691 - val_loss: 0.0774 - val_acc: 0.9709\nEpoch 53/180\nF1 Macro Score: 0.94088\n - 7s - loss: 0.0833 - acc: 0.9692 - val_loss: 0.0779 - val_acc: 0.9707\nEpoch 54/180\nF1 Macro Score: 0.94158\n - 7s - loss: 0.0840 - acc: 0.9691 - val_loss: 0.0774 - val_acc: 0.9709\nEpoch 55/180\nF1 Macro Score: 0.94140\n - 7s - loss: 0.0838 - acc: 0.9691 - val_loss: 0.0778 - val_acc: 0.9709\nEpoch 56/180\nF1 Macro Score: 0.94131\n - 7s - loss: 0.0840 - acc: 0.9691 - val_loss: 0.0778 - val_acc: 0.9708\nEpoch 57/180\nF1 Macro Score: 0.94079\n - 7s - loss: 0.0833 - acc: 0.9692 - val_loss: 0.0783 - val_acc: 0.9706\nEpoch 58/180\nF1 Macro Score: 0.94166\n - 7s - loss: 0.0831 - acc: 0.9693 - val_loss: 0.0773 - val_acc: 0.9709\nEpoch 59/180\nF1 Macro Score: 0.94174\n - 7s - loss: 0.0833 - acc: 0.9693 - val_loss: 0.0775 - val_acc: 0.9709\nEpoch 60/180\nF1 Macro Score: 0.94141\n - 7s - loss: 0.0836 - acc: 0.9691 - val_loss: 0.0778 - val_acc: 0.9708\nEpoch 61/180\nF1 Macro Score: 0.94170\n - 7s - loss: 0.0825 - acc: 0.9694 - val_loss: 0.0769 - val_acc: 0.9710\nEpoch 62/180\nF1 Macro Score: 0.94180\n - 8s - loss: 0.0820 - acc: 0.9695 - val_loss: 0.0770 - val_acc: 0.9710\nEpoch 63/180\nF1 Macro Score: 0.94169\n - 7s - loss: 0.0822 - acc: 0.9695 - val_loss: 0.0772 - val_acc: 0.9711\nEpoch 64/180\nF1 Macro Score: 0.94187\n - 7s - loss: 0.0825 - acc: 0.9693 - val_loss: 0.0769 - val_acc: 0.9711\nEpoch 65/180\nF1 Macro Score: 0.94166\n - 7s - loss: 0.0823 - acc: 0.9696 - val_loss: 0.0770 - val_acc: 0.9710\nEpoch 66/180\nF1 Macro Score: 0.94191\n - 7s - loss: 0.0823 - acc: 0.9695 - val_loss: 0.0772 - val_acc: 0.9710\nEpoch 67/180\nF1 Macro Score: 0.94134\n - 7s - loss: 0.0820 - acc: 0.9696 - val_loss: 0.0774 - val_acc: 0.9708\nEpoch 68/180\nF1 Macro Score: 0.94161\n - 7s - loss: 0.0825 - acc: 0.9694 - val_loss: 0.0774 - val_acc: 0.9708\nEpoch 69/180\nF1 Macro Score: 0.94170\n - 7s - loss: 0.0819 - acc: 0.9696 - val_loss: 0.0772 - val_acc: 0.9710\nEpoch 70/180\nF1 Macro Score: 0.94167\n - 7s - loss: 0.0819 - acc: 0.9697 - val_loss: 0.0772 - val_acc: 0.9709\nEpoch 71/180\nF1 Macro Score: 0.94097\n - 7s - loss: 0.0816 - acc: 0.9697 - val_loss: 0.0779 - val_acc: 0.9707\nEpoch 72/180\nF1 Macro Score: 0.94158\n - 7s - loss: 0.0816 - acc: 0.9697 - val_loss: 0.0771 - val_acc: 0.9709\nEpoch 73/180\nF1 Macro Score: 0.94166\n - 7s - loss: 0.0811 - acc: 0.9698 - val_loss: 0.0770 - val_acc: 0.9710\nEpoch 74/180\nF1 Macro Score: 0.94166\n - 7s - loss: 0.0815 - acc: 0.9697 - val_loss: 0.0774 - val_acc: 0.9709\nEpoch 75/180\nF1 Macro Score: 0.94164\n - 7s - loss: 0.0813 - acc: 0.9697 - val_loss: 0.0771 - val_acc: 0.9710\nEpoch 76/180\nF1 Macro Score: 0.94179\n - 7s - loss: 0.0809 - acc: 0.9698 - val_loss: 0.0768 - val_acc: 0.9710\nEpoch 77/180\nF1 Macro Score: 0.94181\n - 7s - loss: 0.0811 - acc: 0.9698 - val_loss: 0.0769 - val_acc: 0.9710\nEpoch 78/180\nF1 Macro Score: 0.94150\n - 7s - loss: 0.0811 - acc: 0.9698 - val_loss: 0.0769 - val_acc: 0.9709\nEpoch 79/180\nF1 Macro Score: 0.94150\n - 7s - loss: 0.0806 - acc: 0.9698 - val_loss: 0.0772 - val_acc: 0.9709\nEpoch 80/180\nF1 Macro Score: 0.94142\n - 7s - loss: 0.0812 - acc: 0.9698 - val_loss: 0.0772 - val_acc: 0.9709\nEpoch 81/180\nF1 Macro Score: 0.94187\n - 7s - loss: 0.0808 - acc: 0.9699 - val_loss: 0.0769 - val_acc: 0.9711\nEpoch 82/180\nF1 Macro Score: 0.94169\n - 7s - loss: 0.0803 - acc: 0.9700 - val_loss: 0.0770 - val_acc: 0.9709\nEpoch 83/180\nF1 Macro Score: 0.94187\n - 7s - loss: 0.0801 - acc: 0.9701 - val_loss: 0.0768 - val_acc: 0.9710\nEpoch 84/180\nF1 Macro Score: 0.94138\n - 7s - loss: 0.0808 - acc: 0.9699 - val_loss: 0.0774 - val_acc: 0.9709\nEpoch 85/180\nF1 Macro Score: 0.94171\n - 7s - loss: 0.0802 - acc: 0.9700 - val_loss: 0.0769 - val_acc: 0.9710\nEpoch 86/180\nF1 Macro Score: 0.94175\n - 7s - loss: 0.0809 - acc: 0.9698 - val_loss: 0.0769 - val_acc: 0.9710\nEpoch 87/180\nF1 Macro Score: 0.94119\n - 7s - loss: 0.0803 - acc: 0.9701 - val_loss: 0.0772 - val_acc: 0.9708\nEpoch 88/180\nF1 Macro Score: 0.94152\n - 7s - loss: 0.0800 - acc: 0.9700 - val_loss: 0.0770 - val_acc: 0.9709\nEpoch 89/180\nF1 Macro Score: 0.94163\n - 7s - loss: 0.0802 - acc: 0.9700 - val_loss: 0.0769 - val_acc: 0.9709\nEpoch 90/180\nF1 Macro Score: 0.94167\n - 7s - loss: 0.0800 - acc: 0.9701 - val_loss: 0.0770 - val_acc: 0.9710\nEpoch 91/180\nF1 Macro Score: 0.94175\n - 7s - loss: 0.0795 - acc: 0.9702 - val_loss: 0.0768 - val_acc: 0.9710\nEpoch 92/180\nF1 Macro Score: 0.94182\n - 7s - loss: 0.0794 - acc: 0.9702 - val_loss: 0.0767 - val_acc: 0.9710\nEpoch 93/180\nF1 Macro Score: 0.94178\n - 7s - loss: 0.0794 - acc: 0.9703 - val_loss: 0.0768 - val_acc: 0.9710\nEpoch 94/180\nF1 Macro Score: 0.94180\n - 7s - loss: 0.0796 - acc: 0.9702 - val_loss: 0.0767 - val_acc: 0.9710\nEpoch 95/180\nF1 Macro Score: 0.94185\n - 7s - loss: 0.0793 - acc: 0.9703 - val_loss: 0.0767 - val_acc: 0.9711\nEpoch 96/180\nF1 Macro Score: 0.94177\n - 7s - loss: 0.0791 - acc: 0.9703 - val_loss: 0.0768 - val_acc: 0.9710\nEpoch 97/180\nF1 Macro Score: 0.94181\n - 7s - loss: 0.0790 - acc: 0.9703 - val_loss: 0.0768 - val_acc: 0.9710\nEpoch 98/180\nF1 Macro Score: 0.94183\n - 7s - loss: 0.0789 - acc: 0.9703 - val_loss: 0.0767 - val_acc: 0.9710\nEpoch 99/180\nF1 Macro Score: 0.94180\n - 7s - loss: 0.0792 - acc: 0.9702 - val_loss: 0.0768 - val_acc: 0.9710\nEpoch 100/180\nF1 Macro Score: 0.94181\n - 7s - loss: 0.0791 - acc: 0.9703 - val_loss: 0.0768 - val_acc: 0.9710\nEpoch 101/180\nF1 Macro Score: 0.94171\n - 7s - loss: 0.0793 - acc: 0.9702 - val_loss: 0.0768 - val_acc: 0.9710\nEpoch 102/180\nF1 Macro Score: 0.94176\n - 7s - loss: 0.0792 - acc: 0.9703 - val_loss: 0.0768 - val_acc: 0.9710\nEpoch 103/180\nF1 Macro Score: 0.94172\n - 7s - loss: 0.0795 - acc: 0.9702 - val_loss: 0.0768 - val_acc: 0.9710\nEpoch 104/180\nF1 Macro Score: 0.94171\n - 8s - loss: 0.0790 - acc: 0.9704 - val_loss: 0.0768 - val_acc: 0.9710\nEpoch 105/180\nF1 Macro Score: 0.94180\n - 8s - loss: 0.0790 - acc: 0.9704 - val_loss: 0.0767 - val_acc: 0.9710\nEpoch 106/180\nF1 Macro Score: 0.94171\n - 8s - loss: 0.0790 - acc: 0.9703 - val_loss: 0.0769 - val_acc: 0.9710\nEpoch 107/180\nF1 Macro Score: 0.94171\n - 7s - loss: 0.0790 - acc: 0.9704 - val_loss: 0.0768 - val_acc: 0.9710\nEpoch 108/180\nF1 Macro Score: 0.94176\n - 8s - loss: 0.0793 - acc: 0.9703 - val_loss: 0.0768 - val_acc: 0.9710\nEpoch 109/180\nF1 Macro Score: 0.94189\n - 8s - loss: 0.0789 - acc: 0.9703 - val_loss: 0.0768 - val_acc: 0.9710\nEpoch 110/180\nF1 Macro Score: 0.94170\n - 7s - loss: 0.0791 - acc: 0.9703 - val_loss: 0.0768 - val_acc: 0.9710\nEpoch 111/180\nF1 Macro Score: 0.94173\n - 7s - loss: 0.0787 - acc: 0.9704 - val_loss: 0.0768 - val_acc: 0.9710\nEpoch 112/180\nF1 Macro Score: 0.94161\n - 7s - loss: 0.0793 - acc: 0.9703 - val_loss: 0.0769 - val_acc: 0.9709\nEpoch 113/180\nF1 Macro Score: 0.94168\n - 7s - loss: 0.0795 - acc: 0.9702 - val_loss: 0.0769 - val_acc: 0.9710\nEpoch 114/180\nF1 Macro Score: 0.94183\n - 7s - loss: 0.0790 - acc: 0.9703 - val_loss: 0.0768 - val_acc: 0.9710\nEpoch 115/180\nF1 Macro Score: 0.94168\n - 7s - loss: 0.0794 - acc: 0.9703 - val_loss: 0.0769 - val_acc: 0.9709\nEpoch 116/180\nF1 Macro Score: 0.94168\n - 7s - loss: 0.0791 - acc: 0.9703 - val_loss: 0.0769 - val_acc: 0.9710\nEpoch 117/180\nF1 Macro Score: 0.94169\n - 7s - loss: 0.0793 - acc: 0.9703 - val_loss: 0.0768 - val_acc: 0.9710\nEpoch 118/180\nF1 Macro Score: 0.94173\n - 7s - loss: 0.0790 - acc: 0.9704 - val_loss: 0.0768 - val_acc: 0.9710\nEpoch 119/180\nF1 Macro Score: 0.94177\n - 7s - loss: 0.0791 - acc: 0.9703 - val_loss: 0.0768 - val_acc: 0.9710\nEpoch 120/180\nF1 Macro Score: 0.94171\n - 7s - loss: 0.0786 - acc: 0.9704 - val_loss: 0.0768 - val_acc: 0.9710\nEpoch 121/180\nF1 Macro Score: 0.94179\n - 7s - loss: 0.0787 - acc: 0.9704 - val_loss: 0.0768 - val_acc: 0.9710\nEpoch 122/180\nF1 Macro Score: 0.94175\n - 7s - loss: 0.0788 - acc: 0.9704 - val_loss: 0.0768 - val_acc: 0.9710\nEpoch 123/180\nF1 Macro Score: 0.94166\n - 7s - loss: 0.0793 - acc: 0.9703 - val_loss: 0.0768 - val_acc: 0.9710\nEpoch 124/180\nF1 Macro Score: 0.94172\n - 7s - loss: 0.0790 - acc: 0.9704 - val_loss: 0.0768 - val_acc: 0.9710\nEpoch 125/180\nF1 Macro Score: 0.94174\n - 7s - loss: 0.0790 - acc: 0.9704 - val_loss: 0.0769 - val_acc: 0.9710\nEpoch 126/180\nF1 Macro Score: 0.94166\n - 7s - loss: 0.0792 - acc: 0.9703 - val_loss: 0.0768 - val_acc: 0.9710\nEpoch 127/180\nF1 Macro Score: 0.94177\n - 7s - loss: 0.0784 - acc: 0.9705 - val_loss: 0.0768 - val_acc: 0.9710\nEpoch 128/180\nF1 Macro Score: 0.94171\n - 9s - loss: 0.0786 - acc: 0.9704 - val_loss: 0.0768 - val_acc: 0.9710\nEpoch 129/180\nF1 Macro Score: 0.94169\n - 9s - loss: 0.0788 - acc: 0.9704 - val_loss: 0.0768 - val_acc: 0.9710\nEpoch 130/180\nF1 Macro Score: 0.94170\n - 8s - loss: 0.0789 - acc: 0.9704 - val_loss: 0.0769 - val_acc: 0.9710\nEpoch 131/180\nF1 Macro Score: 0.94170\n - 8s - loss: 0.0789 - acc: 0.9704 - val_loss: 0.0769 - val_acc: 0.9710\nEpoch 132/180\nF1 Macro Score: 0.94166\n - 8s - loss: 0.0793 - acc: 0.9703 - val_loss: 0.0769 - val_acc: 0.9710\nEpoch 133/180\nF1 Macro Score: 0.94165\n - 8s - loss: 0.0784 - acc: 0.9704 - val_loss: 0.0769 - val_acc: 0.9710\nEpoch 134/180\nF1 Macro Score: 0.94177\n - 8s - loss: 0.0789 - acc: 0.9704 - val_loss: 0.0768 - val_acc: 0.9710\nEpoch 135/180\nF1 Macro Score: 0.94173\n - 7s - loss: 0.0788 - acc: 0.9704 - val_loss: 0.0769 - val_acc: 0.9710\nEpoch 136/180\nF1 Macro Score: 0.94160\n - 9s - loss: 0.0786 - acc: 0.9705 - val_loss: 0.0769 - val_acc: 0.9709\nEpoch 137/180\nF1 Macro Score: 0.94162\n - 8s - loss: 0.0787 - acc: 0.9704 - val_loss: 0.0770 - val_acc: 0.9709\nEpoch 138/180\nF1 Macro Score: 0.94163\n - 7s - loss: 0.0787 - acc: 0.9705 - val_loss: 0.0769 - val_acc: 0.9710\nEpoch 139/180\nF1 Macro Score: 0.94167\n - 8s - loss: 0.0789 - acc: 0.9704 - val_loss: 0.0768 - val_acc: 0.9710\nEpoch 140/180\nF1 Macro Score: 0.94161\n - 7s - loss: 0.0789 - acc: 0.9704 - val_loss: 0.0769 - val_acc: 0.9709\nEpoch 141/180\nF1 Macro Score: 0.94175\n - 7s - loss: 0.0788 - acc: 0.9704 - val_loss: 0.0769 - val_acc: 0.9709\nEpoch 142/180\nF1 Macro Score: 0.94166\n - 7s - loss: 0.0785 - acc: 0.9705 - val_loss: 0.0769 - val_acc: 0.9709\nEpoch 143/180\nF1 Macro Score: 0.94169\n - 7s - loss: 0.0786 - acc: 0.9704 - val_loss: 0.0769 - val_acc: 0.9709\nEpoch 144/180\nF1 Macro Score: 0.94169\n - 8s - loss: 0.0784 - acc: 0.9705 - val_loss: 0.0769 - val_acc: 0.9710\nEpoch 145/180\nF1 Macro Score: 0.94167\n - 7s - loss: 0.0786 - acc: 0.9705 - val_loss: 0.0769 - val_acc: 0.9710\nEpoch 146/180\nF1 Macro Score: 0.94166\n - 7s - loss: 0.0785 - acc: 0.9705 - val_loss: 0.0769 - val_acc: 0.9709\nEpoch 147/180\nF1 Macro Score: 0.94161\n - 7s - loss: 0.0785 - acc: 0.9705 - val_loss: 0.0769 - val_acc: 0.9709\nEpoch 148/180\nF1 Macro Score: 0.94165\n - 7s - loss: 0.0788 - acc: 0.9704 - val_loss: 0.0770 - val_acc: 0.9709\nEpoch 149/180\nF1 Macro Score: 0.94152\n - 7s - loss: 0.0786 - acc: 0.9704 - val_loss: 0.0769 - val_acc: 0.9708\nEpoch 150/180\nF1 Macro Score: 0.94164\n - 7s - loss: 0.0787 - acc: 0.9705 - val_loss: 0.0769 - val_acc: 0.9709\nEpoch 151/180\nF1 Macro Score: 0.94166\n - 7s - loss: 0.0786 - acc: 0.9705 - val_loss: 0.0769 - val_acc: 0.9709\nEpoch 152/180\nF1 Macro Score: 0.94158\n - 7s - loss: 0.0787 - acc: 0.9704 - val_loss: 0.0769 - val_acc: 0.9709\nEpoch 153/180\nF1 Macro Score: 0.94166\n - 7s - loss: 0.0789 - acc: 0.9704 - val_loss: 0.0769 - val_acc: 0.9710\nEpoch 154/180\nF1 Macro Score: 0.94159\n - 7s - loss: 0.0786 - acc: 0.9704 - val_loss: 0.0769 - val_acc: 0.9709\nEpoch 155/180\nF1 Macro Score: 0.94160\n - 7s - loss: 0.0784 - acc: 0.9705 - val_loss: 0.0770 - val_acc: 0.9709\nEpoch 156/180\nF1 Macro Score: 0.94167\n - 7s - loss: 0.0785 - acc: 0.9704 - val_loss: 0.0769 - val_acc: 0.9710\nEpoch 157/180\nF1 Macro Score: 0.94163\n - 7s - loss: 0.0784 - acc: 0.9705 - val_loss: 0.0770 - val_acc: 0.9709\nEpoch 158/180\nF1 Macro Score: 0.94161\n - 7s - loss: 0.0786 - acc: 0.9705 - val_loss: 0.0770 - val_acc: 0.9709\nEpoch 159/180\nF1 Macro Score: 0.94146\n - 7s - loss: 0.0787 - acc: 0.9704 - val_loss: 0.0770 - val_acc: 0.9708\nEpoch 160/180\nF1 Macro Score: 0.94157\n - 7s - loss: 0.0785 - acc: 0.9705 - val_loss: 0.0770 - val_acc: 0.9709\nEpoch 161/180\nF1 Macro Score: 0.94163\n - 7s - loss: 0.0784 - acc: 0.9705 - val_loss: 0.0769 - val_acc: 0.9709\nEpoch 162/180\nF1 Macro Score: 0.94159\n - 7s - loss: 0.0783 - acc: 0.9705 - val_loss: 0.0770 - val_acc: 0.9709\nEpoch 163/180\nF1 Macro Score: 0.94155\n - 7s - loss: 0.0784 - acc: 0.9706 - val_loss: 0.0770 - val_acc: 0.9709\nEpoch 164/180\nF1 Macro Score: 0.94158\n - 7s - loss: 0.0782 - acc: 0.9705 - val_loss: 0.0769 - val_acc: 0.9709\nEpoch 165/180\nF1 Macro Score: 0.94160\n - 7s - loss: 0.0786 - acc: 0.9705 - val_loss: 0.0770 - val_acc: 0.9709\nEpoch 166/180\nF1 Macro Score: 0.94159\n - 7s - loss: 0.0785 - acc: 0.9705 - val_loss: 0.0770 - val_acc: 0.9709\nEpoch 167/180\nF1 Macro Score: 0.94158\n - 7s - loss: 0.0786 - acc: 0.9705 - val_loss: 0.0769 - val_acc: 0.9709\nEpoch 168/180\nF1 Macro Score: 0.94162\n - 7s - loss: 0.0788 - acc: 0.9704 - val_loss: 0.0770 - val_acc: 0.9709\nEpoch 169/180\nF1 Macro Score: 0.94161\n - 7s - loss: 0.0781 - acc: 0.9706 - val_loss: 0.0770 - val_acc: 0.9709\nEpoch 170/180\nF1 Macro Score: 0.94164\n - 7s - loss: 0.0785 - acc: 0.9705 - val_loss: 0.0771 - val_acc: 0.9709\nEpoch 171/180\nF1 Macro Score: 0.94158\n - 8s - loss: 0.0784 - acc: 0.9706 - val_loss: 0.0770 - val_acc: 0.9709\nEpoch 172/180\nF1 Macro Score: 0.94162\n - 8s - loss: 0.0783 - acc: 0.9705 - val_loss: 0.0770 - val_acc: 0.9709\nEpoch 173/180\nF1 Macro Score: 0.94156\n - 8s - loss: 0.0784 - acc: 0.9705 - val_loss: 0.0770 - val_acc: 0.9709\nEpoch 174/180\nF1 Macro Score: 0.94160\n - 8s - loss: 0.0785 - acc: 0.9705 - val_loss: 0.0770 - val_acc: 0.9709\nEpoch 175/180\nF1 Macro Score: 0.94163\n - 7s - loss: 0.0784 - acc: 0.9704 - val_loss: 0.0770 - val_acc: 0.9709\nEpoch 176/180\nF1 Macro Score: 0.94152\n - 7s - loss: 0.0784 - acc: 0.9705 - val_loss: 0.0770 - val_acc: 0.9709\nEpoch 177/180\nF1 Macro Score: 0.94156\n - 7s - loss: 0.0784 - acc: 0.9705 - val_loss: 0.0770 - val_acc: 0.9709\nEpoch 178/180\nF1 Macro Score: 0.94158\n - 7s - loss: 0.0785 - acc: 0.9706 - val_loss: 0.0769 - val_acc: 0.9709\nEpoch 179/180\nF1 Macro Score: 0.94162\n - 7s - loss: 0.0783 - acc: 0.9705 - val_loss: 0.0770 - val_acc: 0.9709\nEpoch 180/180\nF1 Macro Score: 0.94169\n - 7s - loss: 0.0781 - acc: 0.9706 - val_loss: 0.0770 - val_acc: 0.9709\nTraining fold 2 completed. macro f1 score : 0.94169\nOur training dataset shape is (1000, 4000, 19)\nOur validation dataset shape is (250, 4000, 19)\nTrain on 1000 samples, validate on 250 samples\nEpoch 1/180\nF1 Macro Score: 0.68362\n - 19s - loss: 0.4988 - acc: 0.8627 - val_loss: 1.0696 - val_acc: 0.7235\nEpoch 2/180\nF1 Macro Score: 0.91739\n - 7s - loss: 0.1653 - acc: 0.9602 - val_loss: 0.3926 - val_acc: 0.9601\nEpoch 3/180\nF1 Macro Score: 0.93307\n - 7s - loss: 0.1411 - acc: 0.9640 - val_loss: 0.2317 - val_acc: 0.9666\nEpoch 4/180\nF1 Macro Score: 0.93435\n - 7s - loss: 0.1262 - acc: 0.9655 - val_loss: 0.1320 - val_acc: 0.9676\nEpoch 5/180\nF1 Macro Score: 0.93570\n - 7s - loss: 0.1165 - acc: 0.9663 - val_loss: 0.1101 - val_acc: 0.9680\nEpoch 6/180\nF1 Macro Score: 0.93662\n - 7s - loss: 0.1154 - acc: 0.9662 - val_loss: 0.0992 - val_acc: 0.9683\nEpoch 7/180\nF1 Macro Score: 0.93510\n - 7s - loss: 0.1142 - acc: 0.9662 - val_loss: 0.0994 - val_acc: 0.9679\nEpoch 8/180\nF1 Macro Score: 0.93542\n - 7s - loss: 0.1096 - acc: 0.9665 - val_loss: 0.1091 - val_acc: 0.9676\nEpoch 9/180\nF1 Macro Score: 0.93501\n - 7s - loss: 0.1089 - acc: 0.9665 - val_loss: 0.0947 - val_acc: 0.9681\nEpoch 10/180\nF1 Macro Score: 0.93387\n - 7s - loss: 0.1052 - acc: 0.9667 - val_loss: 0.0966 - val_acc: 0.9672\nEpoch 11/180\nF1 Macro Score: 0.93696\n - 7s - loss: 0.1033 - acc: 0.9668 - val_loss: 0.0908 - val_acc: 0.9685\nEpoch 12/180\nF1 Macro Score: 0.93637\n - 7s - loss: 0.1024 - acc: 0.9667 - val_loss: 0.0908 - val_acc: 0.9682\nEpoch 13/180\nF1 Macro Score: 0.93592\n - 7s - loss: 0.1011 - acc: 0.9669 - val_loss: 0.0912 - val_acc: 0.9684\nEpoch 14/180\nF1 Macro Score: 0.93683\n - 7s - loss: 0.0993 - acc: 0.9670 - val_loss: 0.0896 - val_acc: 0.9685\nEpoch 15/180\nF1 Macro Score: 0.93707\n - 7s - loss: 0.0987 - acc: 0.9670 - val_loss: 0.0939 - val_acc: 0.9685\nEpoch 16/180\nF1 Macro Score: 0.93455\n - 7s - loss: 0.0992 - acc: 0.9668 - val_loss: 0.0920 - val_acc: 0.9675\nEpoch 17/180\nF1 Macro Score: 0.93480\n - 7s - loss: 0.0985 - acc: 0.9669 - val_loss: 0.0922 - val_acc: 0.9676\nEpoch 18/180\nF1 Macro Score: 0.93643\n - 7s - loss: 0.0975 - acc: 0.9670 - val_loss: 0.0890 - val_acc: 0.9684\nEpoch 19/180\nF1 Macro Score: 0.93662\n - 7s - loss: 0.0961 - acc: 0.9671 - val_loss: 0.0873 - val_acc: 0.9684\nEpoch 20/180\nF1 Macro Score: 0.93596\n - 7s - loss: 0.0971 - acc: 0.9669 - val_loss: 0.0993 - val_acc: 0.9677\nEpoch 21/180\nF1 Macro Score: 0.42415\n - 7s - loss: 0.2905 - acc: 0.9161 - val_loss: 2.4618 - val_acc: 0.6838\nEpoch 22/180\nF1 Macro Score: 0.91542\n - 8s - loss: 0.2210 - acc: 0.9357 - val_loss: 0.1428 - val_acc: 0.9598\nEpoch 23/180\nF1 Macro Score: 0.93362\n - 7s - loss: 0.1246 - acc: 0.9645 - val_loss: 0.1097 - val_acc: 0.9669\nEpoch 24/180\nF1 Macro Score: 0.93502\n - 7s - loss: 0.1173 - acc: 0.9655 - val_loss: 0.1036 - val_acc: 0.9676\nEpoch 25/180\nF1 Macro Score: 0.93390\n - 7s - loss: 0.1116 - acc: 0.9663 - val_loss: 0.1011 - val_acc: 0.9673\nEpoch 26/180\nF1 Macro Score: 0.93641\n - 7s - loss: 0.1095 - acc: 0.9665 - val_loss: 0.0963 - val_acc: 0.9682\nEpoch 27/180\nF1 Macro Score: 0.93631\n - 7s - loss: 0.1065 - acc: 0.9667 - val_loss: 0.0946 - val_acc: 0.9682\nEpoch 28/180\nF1 Macro Score: 0.93620\n - 7s - loss: 0.1051 - acc: 0.9668 - val_loss: 0.0935 - val_acc: 0.9682\nEpoch 29/180\nF1 Macro Score: 0.93600\n - 7s - loss: 0.1042 - acc: 0.9669 - val_loss: 0.0933 - val_acc: 0.9681\nEpoch 30/180\nF1 Macro Score: 0.93710\n - 7s - loss: 0.1038 - acc: 0.9667 - val_loss: 0.0913 - val_acc: 0.9686\nEpoch 31/180\nF1 Macro Score: 0.93667\n - 7s - loss: 0.1009 - acc: 0.9671 - val_loss: 0.0907 - val_acc: 0.9685\nEpoch 32/180\nF1 Macro Score: 0.93743\n - 7s - loss: 0.1005 - acc: 0.9672 - val_loss: 0.0901 - val_acc: 0.9687\nEpoch 33/180\nF1 Macro Score: 0.93738\n - 7s - loss: 0.0996 - acc: 0.9673 - val_loss: 0.0898 - val_acc: 0.9687\nEpoch 34/180\nF1 Macro Score: 0.93719\n - 7s - loss: 0.1005 - acc: 0.9671 - val_loss: 0.0896 - val_acc: 0.9686\nEpoch 35/180\nF1 Macro Score: 0.93710\n - 7s - loss: 0.0994 - acc: 0.9672 - val_loss: 0.0895 - val_acc: 0.9686\nEpoch 36/180\nF1 Macro Score: 0.93709\n - 7s - loss: 0.0991 - acc: 0.9672 - val_loss: 0.0891 - val_acc: 0.9686\nEpoch 37/180\nF1 Macro Score: 0.93670\n - 7s - loss: 0.0992 - acc: 0.9672 - val_loss: 0.0898 - val_acc: 0.9686\nEpoch 38/180\nF1 Macro Score: 0.93699\n - 7s - loss: 0.0989 - acc: 0.9673 - val_loss: 0.0892 - val_acc: 0.9686\nEpoch 39/180\nF1 Macro Score: 0.93691\n - 7s - loss: 0.0984 - acc: 0.9672 - val_loss: 0.0891 - val_acc: 0.9686\nEpoch 40/180\nF1 Macro Score: 0.93704\n - 7s - loss: 0.0986 - acc: 0.9671 - val_loss: 0.0891 - val_acc: 0.9686\nEpoch 41/180\nF1 Macro Score: 0.93724\n - 7s - loss: 0.0977 - acc: 0.9672 - val_loss: 0.0886 - val_acc: 0.9687\nEpoch 42/180\nF1 Macro Score: 0.93722\n - 7s - loss: 0.0969 - acc: 0.9673 - val_loss: 0.0882 - val_acc: 0.9688\nEpoch 43/180\nF1 Macro Score: 0.93728\n - 7s - loss: 0.0971 - acc: 0.9673 - val_loss: 0.0881 - val_acc: 0.9687\nEpoch 44/180\nF1 Macro Score: 0.93716\n - 7s - loss: 0.0970 - acc: 0.9673 - val_loss: 0.0878 - val_acc: 0.9687\nEpoch 45/180\nF1 Macro Score: 0.93734\n - 7s - loss: 0.0970 - acc: 0.9673 - val_loss: 0.0878 - val_acc: 0.9688\nEpoch 46/180\nF1 Macro Score: 0.93739\n - 8s - loss: 0.0966 - acc: 0.9674 - val_loss: 0.0877 - val_acc: 0.9688\nEpoch 47/180\nF1 Macro Score: 0.93726\n - 7s - loss: 0.0977 - acc: 0.9672 - val_loss: 0.0877 - val_acc: 0.9688\nEpoch 48/180\nF1 Macro Score: 0.93742\n - 7s - loss: 0.0967 - acc: 0.9673 - val_loss: 0.0874 - val_acc: 0.9688\nEpoch 49/180\nF1 Macro Score: 0.93731\n - 7s - loss: 0.0965 - acc: 0.9673 - val_loss: 0.0874 - val_acc: 0.9688\nEpoch 50/180\nF1 Macro Score: 0.93714\n - 7s - loss: 0.0959 - acc: 0.9673 - val_loss: 0.0873 - val_acc: 0.9688\nEpoch 51/180\nF1 Macro Score: 0.93720\n - 7s - loss: 0.0963 - acc: 0.9673 - val_loss: 0.0872 - val_acc: 0.9687\nEpoch 52/180\nF1 Macro Score: 0.93701\n - 7s - loss: 0.0964 - acc: 0.9673 - val_loss: 0.0874 - val_acc: 0.9687\nEpoch 53/180\nF1 Macro Score: 0.93734\n - 7s - loss: 0.0954 - acc: 0.9674 - val_loss: 0.0873 - val_acc: 0.9688\nEpoch 54/180\nF1 Macro Score: 0.93717\n - 7s - loss: 0.0964 - acc: 0.9672 - val_loss: 0.0871 - val_acc: 0.9687\nEpoch 55/180\nF1 Macro Score: 0.93750\n - 7s - loss: 0.0960 - acc: 0.9673 - val_loss: 0.0867 - val_acc: 0.9688\nEpoch 56/180\nF1 Macro Score: 0.93735\n - 7s - loss: 0.0957 - acc: 0.9673 - val_loss: 0.0869 - val_acc: 0.9688\nEpoch 57/180\nF1 Macro Score: 0.93752\n - 7s - loss: 0.0950 - acc: 0.9674 - val_loss: 0.0867 - val_acc: 0.9688\nEpoch 58/180\nF1 Macro Score: 0.93722\n - 7s - loss: 0.0949 - acc: 0.9674 - val_loss: 0.0866 - val_acc: 0.9688\nEpoch 59/180\nF1 Macro Score: 0.93724\n - 7s - loss: 0.0952 - acc: 0.9674 - val_loss: 0.0867 - val_acc: 0.9688\nEpoch 60/180\nF1 Macro Score: 0.93733\n - 7s - loss: 0.0947 - acc: 0.9674 - val_loss: 0.0863 - val_acc: 0.9688\nEpoch 61/180\nF1 Macro Score: 0.93726\n - 7s - loss: 0.0944 - acc: 0.9674 - val_loss: 0.0862 - val_acc: 0.9688\nEpoch 62/180\nF1 Macro Score: 0.93737\n - 7s - loss: 0.0947 - acc: 0.9674 - val_loss: 0.0863 - val_acc: 0.9689\nEpoch 63/180\nF1 Macro Score: 0.93726\n - 7s - loss: 0.0945 - acc: 0.9674 - val_loss: 0.0861 - val_acc: 0.9688\nEpoch 64/180\nF1 Macro Score: 0.93741\n - 7s - loss: 0.0943 - acc: 0.9674 - val_loss: 0.0860 - val_acc: 0.9689\nEpoch 65/180\nF1 Macro Score: 0.93725\n - 7s - loss: 0.0951 - acc: 0.9673 - val_loss: 0.0864 - val_acc: 0.9688\nEpoch 66/180\nF1 Macro Score: 0.93734\n - 7s - loss: 0.0941 - acc: 0.9674 - val_loss: 0.0860 - val_acc: 0.9688\nEpoch 67/180\nF1 Macro Score: 0.93730\n - 7s - loss: 0.0940 - acc: 0.9674 - val_loss: 0.0859 - val_acc: 0.9689\nEpoch 68/180\nF1 Macro Score: 0.93639\n - 7s - loss: 0.0943 - acc: 0.9674 - val_loss: 0.0879 - val_acc: 0.9683\nEpoch 69/180\nF1 Macro Score: 0.93723\n - 7s - loss: 0.0940 - acc: 0.9674 - val_loss: 0.0862 - val_acc: 0.9688\nEpoch 70/180\nF1 Macro Score: 0.93743\n - 7s - loss: 0.0944 - acc: 0.9674 - val_loss: 0.0858 - val_acc: 0.9688\nEpoch 71/180\nF1 Macro Score: 0.93736\n - 7s - loss: 0.0946 - acc: 0.9673 - val_loss: 0.0857 - val_acc: 0.9688\nEpoch 72/180\nF1 Macro Score: 0.93748\n - 7s - loss: 0.0944 - acc: 0.9674 - val_loss: 0.0858 - val_acc: 0.9689\nEpoch 73/180\nF1 Macro Score: 0.93779\n - 7s - loss: 0.0936 - acc: 0.9674 - val_loss: 0.0855 - val_acc: 0.9689\nEpoch 74/180\nF1 Macro Score: 0.93746\n - 7s - loss: 0.0936 - acc: 0.9675 - val_loss: 0.0858 - val_acc: 0.9689\nEpoch 75/180\nF1 Macro Score: 0.93739\n - 7s - loss: 0.0933 - acc: 0.9675 - val_loss: 0.0855 - val_acc: 0.9689\nEpoch 76/180\nF1 Macro Score: 0.93745\n - 7s - loss: 0.0926 - acc: 0.9675 - val_loss: 0.0853 - val_acc: 0.9689\nEpoch 77/180\nF1 Macro Score: 0.93732\n - 7s - loss: 0.0932 - acc: 0.9675 - val_loss: 0.0853 - val_acc: 0.9688\nEpoch 78/180\nF1 Macro Score: 0.93744\n - 7s - loss: 0.0930 - acc: 0.9675 - val_loss: 0.0852 - val_acc: 0.9689\nEpoch 79/180\nF1 Macro Score: 0.93728\n - 7s - loss: 0.0929 - acc: 0.9675 - val_loss: 0.0856 - val_acc: 0.9688\nEpoch 80/180\nF1 Macro Score: 0.93749\n - 7s - loss: 0.0923 - acc: 0.9676 - val_loss: 0.0850 - val_acc: 0.9689\nEpoch 81/180\nF1 Macro Score: 0.93745\n - 7s - loss: 0.0927 - acc: 0.9675 - val_loss: 0.0853 - val_acc: 0.9689\nEpoch 82/180\nF1 Macro Score: 0.93753\n - 7s - loss: 0.0931 - acc: 0.9674 - val_loss: 0.0850 - val_acc: 0.9689\nEpoch 83/180\nF1 Macro Score: 0.93745\n - 7s - loss: 0.0927 - acc: 0.9675 - val_loss: 0.0851 - val_acc: 0.9689\nEpoch 84/180\nF1 Macro Score: 0.93755\n - 7s - loss: 0.0922 - acc: 0.9675 - val_loss: 0.0849 - val_acc: 0.9689\nEpoch 85/180\nF1 Macro Score: 0.93755\n - 7s - loss: 0.0922 - acc: 0.9676 - val_loss: 0.0849 - val_acc: 0.9690\nEpoch 86/180\nF1 Macro Score: 0.93743\n - 7s - loss: 0.0935 - acc: 0.9674 - val_loss: 0.0849 - val_acc: 0.9689\nEpoch 87/180\nF1 Macro Score: 0.93760\n - 7s - loss: 0.0938 - acc: 0.9674 - val_loss: 0.0851 - val_acc: 0.9690\nEpoch 88/180\nF1 Macro Score: 0.93718\n - 7s - loss: 0.0936 - acc: 0.9674 - val_loss: 0.0855 - val_acc: 0.9689\nEpoch 89/180\nF1 Macro Score: 0.93699\n - 7s - loss: 0.0925 - acc: 0.9675 - val_loss: 0.0850 - val_acc: 0.9689\nEpoch 90/180\nF1 Macro Score: 0.93773\n - 7s - loss: 0.0922 - acc: 0.9675 - val_loss: 0.0849 - val_acc: 0.9690\nEpoch 91/180\nF1 Macro Score: 0.93768\n - 7s - loss: 0.0922 - acc: 0.9676 - val_loss: 0.0846 - val_acc: 0.9690\nEpoch 92/180\nF1 Macro Score: 0.93767\n - 7s - loss: 0.0923 - acc: 0.9675 - val_loss: 0.0846 - val_acc: 0.9690\nEpoch 93/180\nF1 Macro Score: 0.93759\n - 7s - loss: 0.0916 - acc: 0.9676 - val_loss: 0.0846 - val_acc: 0.9690\nEpoch 94/180\nF1 Macro Score: 0.93766\n - 7s - loss: 0.0914 - acc: 0.9677 - val_loss: 0.0846 - val_acc: 0.9690\nEpoch 95/180\nF1 Macro Score: 0.93753\n - 7s - loss: 0.0914 - acc: 0.9677 - val_loss: 0.0846 - val_acc: 0.9689\nEpoch 96/180\nF1 Macro Score: 0.93751\n - 7s - loss: 0.0917 - acc: 0.9676 - val_loss: 0.0847 - val_acc: 0.9690\nEpoch 97/180\nF1 Macro Score: 0.93771\n - 7s - loss: 0.0916 - acc: 0.9677 - val_loss: 0.0845 - val_acc: 0.9690\nEpoch 98/180\nF1 Macro Score: 0.93770\n - 7s - loss: 0.0913 - acc: 0.9677 - val_loss: 0.0845 - val_acc: 0.9690\nEpoch 99/180\nF1 Macro Score: 0.93768\n - 7s - loss: 0.0918 - acc: 0.9676 - val_loss: 0.0845 - val_acc: 0.9690\nEpoch 100/180\nF1 Macro Score: 0.93767\n - 7s - loss: 0.0919 - acc: 0.9676 - val_loss: 0.0845 - val_acc: 0.9690\nEpoch 101/180\nF1 Macro Score: 0.93769\n - 7s - loss: 0.0917 - acc: 0.9677 - val_loss: 0.0845 - val_acc: 0.9690\nEpoch 102/180\nF1 Macro Score: 0.93765\n - 7s - loss: 0.0920 - acc: 0.9675 - val_loss: 0.0845 - val_acc: 0.9690\nEpoch 103/180\nF1 Macro Score: 0.93774\n - 7s - loss: 0.0918 - acc: 0.9675 - val_loss: 0.0845 - val_acc: 0.9690\nEpoch 104/180\nF1 Macro Score: 0.93772\n - 7s - loss: 0.0913 - acc: 0.9677 - val_loss: 0.0845 - val_acc: 0.9690\nEpoch 105/180\nF1 Macro Score: 0.93768\n - 7s - loss: 0.0914 - acc: 0.9677 - val_loss: 0.0845 - val_acc: 0.9690\nEpoch 106/180\nF1 Macro Score: 0.93769\n - 7s - loss: 0.0910 - acc: 0.9677 - val_loss: 0.0845 - val_acc: 0.9690\nEpoch 107/180\nF1 Macro Score: 0.93770\n - 7s - loss: 0.0913 - acc: 0.9676 - val_loss: 0.0844 - val_acc: 0.9690\nEpoch 108/180\nF1 Macro Score: 0.93774\n - 7s - loss: 0.0915 - acc: 0.9676 - val_loss: 0.0845 - val_acc: 0.9690\nEpoch 109/180\nF1 Macro Score: 0.93769\n - 7s - loss: 0.0915 - acc: 0.9676 - val_loss: 0.0844 - val_acc: 0.9690\nEpoch 110/180\nF1 Macro Score: 0.93774\n - 7s - loss: 0.0913 - acc: 0.9676 - val_loss: 0.0844 - val_acc: 0.9690\nEpoch 111/180\nF1 Macro Score: 0.93772\n - 7s - loss: 0.0913 - acc: 0.9677 - val_loss: 0.0843 - val_acc: 0.9690\nEpoch 112/180\nF1 Macro Score: 0.93774\n - 8s - loss: 0.0912 - acc: 0.9677 - val_loss: 0.0844 - val_acc: 0.9690\nEpoch 113/180\nF1 Macro Score: 0.93770\n - 8s - loss: 0.0911 - acc: 0.9677 - val_loss: 0.0844 - val_acc: 0.9690\nEpoch 114/180\nF1 Macro Score: 0.93774\n - 8s - loss: 0.0913 - acc: 0.9677 - val_loss: 0.0843 - val_acc: 0.9690\nEpoch 115/180\nF1 Macro Score: 0.93779\n - 7s - loss: 0.0920 - acc: 0.9676 - val_loss: 0.0843 - val_acc: 0.9690\nEpoch 116/180\nF1 Macro Score: 0.93775\n - 8s - loss: 0.0912 - acc: 0.9677 - val_loss: 0.0843 - val_acc: 0.9690\nEpoch 117/180\nF1 Macro Score: 0.93776\n - 7s - loss: 0.0906 - acc: 0.9677 - val_loss: 0.0843 - val_acc: 0.9690\nEpoch 118/180\nF1 Macro Score: 0.93736\n - 7s - loss: 0.0918 - acc: 0.9676 - val_loss: 0.0845 - val_acc: 0.9689\nEpoch 119/180\nF1 Macro Score: 0.93775\n - 7s - loss: 0.0914 - acc: 0.9676 - val_loss: 0.0843 - val_acc: 0.9690\nEpoch 120/180\nF1 Macro Score: 0.93764\n - 8s - loss: 0.0911 - acc: 0.9676 - val_loss: 0.0843 - val_acc: 0.9690\nEpoch 121/180\nF1 Macro Score: 0.93774\n - 7s - loss: 0.0917 - acc: 0.9675 - val_loss: 0.0843 - val_acc: 0.9690\nEpoch 122/180\nF1 Macro Score: 0.93776\n - 7s - loss: 0.0914 - acc: 0.9677 - val_loss: 0.0843 - val_acc: 0.9690\nEpoch 123/180\nF1 Macro Score: 0.93766\n - 7s - loss: 0.0918 - acc: 0.9676 - val_loss: 0.0843 - val_acc: 0.9690\nEpoch 124/180\nF1 Macro Score: 0.93774\n - 8s - loss: 0.0911 - acc: 0.9677 - val_loss: 0.0842 - val_acc: 0.9690\nEpoch 125/180\nF1 Macro Score: 0.93777\n - 7s - loss: 0.0913 - acc: 0.9677 - val_loss: 0.0842 - val_acc: 0.9690\nEpoch 126/180\nF1 Macro Score: 0.93767\n - 8s - loss: 0.0908 - acc: 0.9677 - val_loss: 0.0842 - val_acc: 0.9690\nEpoch 127/180\nF1 Macro Score: 0.93771\n - 7s - loss: 0.0909 - acc: 0.9677 - val_loss: 0.0842 - val_acc: 0.9690\nEpoch 128/180\nF1 Macro Score: 0.93775\n - 8s - loss: 0.0910 - acc: 0.9676 - val_loss: 0.0842 - val_acc: 0.9690\nEpoch 129/180\nF1 Macro Score: 0.93777\n - 9s - loss: 0.0913 - acc: 0.9676 - val_loss: 0.0842 - val_acc: 0.9690\nEpoch 130/180\nF1 Macro Score: 0.93776\n - 7s - loss: 0.0906 - acc: 0.9677 - val_loss: 0.0842 - val_acc: 0.9690\nEpoch 131/180\nF1 Macro Score: 0.93772\n - 7s - loss: 0.0913 - acc: 0.9677 - val_loss: 0.0842 - val_acc: 0.9690\nEpoch 132/180\nF1 Macro Score: 0.93783\n - 7s - loss: 0.0921 - acc: 0.9675 - val_loss: 0.0842 - val_acc: 0.9690\nEpoch 133/180\nF1 Macro Score: 0.93766\n - 7s - loss: 0.0908 - acc: 0.9677 - val_loss: 0.0841 - val_acc: 0.9690\nEpoch 134/180\nF1 Macro Score: 0.93775\n - 7s - loss: 0.0911 - acc: 0.9677 - val_loss: 0.0841 - val_acc: 0.9690\nEpoch 135/180\nF1 Macro Score: 0.93748\n - 7s - loss: 0.0908 - acc: 0.9677 - val_loss: 0.0841 - val_acc: 0.9690\nEpoch 136/180\nF1 Macro Score: 0.93770\n - 7s - loss: 0.0910 - acc: 0.9677 - val_loss: 0.0841 - val_acc: 0.9690\nEpoch 137/180\nF1 Macro Score: 0.93767\n - 7s - loss: 0.0910 - acc: 0.9677 - val_loss: 0.0841 - val_acc: 0.9690\nEpoch 138/180\nF1 Macro Score: 0.93767\n - 7s - loss: 0.0908 - acc: 0.9676 - val_loss: 0.0841 - val_acc: 0.9690\nEpoch 139/180\nF1 Macro Score: 0.93774\n - 7s - loss: 0.0905 - acc: 0.9678 - val_loss: 0.0841 - val_acc: 0.9690\nEpoch 140/180\nF1 Macro Score: 0.93779\n - 7s - loss: 0.0909 - acc: 0.9677 - val_loss: 0.0841 - val_acc: 0.9690\nEpoch 141/180\nF1 Macro Score: 0.93781\n - 7s - loss: 0.0911 - acc: 0.9677 - val_loss: 0.0841 - val_acc: 0.9690\nEpoch 142/180\nF1 Macro Score: 0.93770\n - 7s - loss: 0.0905 - acc: 0.9677 - val_loss: 0.0841 - val_acc: 0.9690\nEpoch 143/180\nF1 Macro Score: 0.93770\n - 7s - loss: 0.0909 - acc: 0.9677 - val_loss: 0.0841 - val_acc: 0.9690\nEpoch 144/180\nF1 Macro Score: 0.93782\n - 7s - loss: 0.0905 - acc: 0.9677 - val_loss: 0.0841 - val_acc: 0.9690\nEpoch 145/180\nF1 Macro Score: 0.93773\n - 7s - loss: 0.0907 - acc: 0.9676 - val_loss: 0.0840 - val_acc: 0.9690\nEpoch 146/180\nF1 Macro Score: 0.93772\n - 7s - loss: 0.0906 - acc: 0.9677 - val_loss: 0.0840 - val_acc: 0.9690\nEpoch 147/180\nF1 Macro Score: 0.93776\n - 7s - loss: 0.0908 - acc: 0.9677 - val_loss: 0.0840 - val_acc: 0.9690\nEpoch 148/180\nF1 Macro Score: 0.93775\n - 7s - loss: 0.0912 - acc: 0.9676 - val_loss: 0.0840 - val_acc: 0.9690\nEpoch 149/180\nF1 Macro Score: 0.93776\n - 7s - loss: 0.0913 - acc: 0.9676 - val_loss: 0.0841 - val_acc: 0.9690\nEpoch 150/180\nF1 Macro Score: 0.93764\n - 7s - loss: 0.0907 - acc: 0.9677 - val_loss: 0.0841 - val_acc: 0.9690\nEpoch 151/180\nF1 Macro Score: 0.93767\n - 7s - loss: 0.0909 - acc: 0.9676 - val_loss: 0.0840 - val_acc: 0.9690\nEpoch 152/180\nF1 Macro Score: 0.93759\n - 7s - loss: 0.0907 - acc: 0.9677 - val_loss: 0.0841 - val_acc: 0.9690\nEpoch 153/180\nF1 Macro Score: 0.93778\n - 7s - loss: 0.0904 - acc: 0.9677 - val_loss: 0.0839 - val_acc: 0.9690\nEpoch 154/180\nF1 Macro Score: 0.93784\n - 7s - loss: 0.0907 - acc: 0.9677 - val_loss: 0.0839 - val_acc: 0.9690\nEpoch 155/180\nF1 Macro Score: 0.93781\n - 7s - loss: 0.0903 - acc: 0.9677 - val_loss: 0.0839 - val_acc: 0.9690\nEpoch 156/180\nF1 Macro Score: 0.93776\n - 7s - loss: 0.0903 - acc: 0.9677 - val_loss: 0.0839 - val_acc: 0.9690\nEpoch 157/180\nF1 Macro Score: 0.93781\n - 7s - loss: 0.0901 - acc: 0.9678 - val_loss: 0.0839 - val_acc: 0.9690\nEpoch 158/180\nF1 Macro Score: 0.93767\n - 7s - loss: 0.0903 - acc: 0.9678 - val_loss: 0.0839 - val_acc: 0.9690\nEpoch 159/180\nF1 Macro Score: 0.93777\n - 7s - loss: 0.0916 - acc: 0.9675 - val_loss: 0.0839 - val_acc: 0.9690\nEpoch 160/180\nF1 Macro Score: 0.93773\n - 7s - loss: 0.0905 - acc: 0.9677 - val_loss: 0.0839 - val_acc: 0.9690\nEpoch 161/180\nF1 Macro Score: 0.93778\n - 7s - loss: 0.0904 - acc: 0.9677 - val_loss: 0.0839 - val_acc: 0.9690\nEpoch 162/180\nF1 Macro Score: 0.93769\n - 8s - loss: 0.0907 - acc: 0.9677 - val_loss: 0.0839 - val_acc: 0.9690\nEpoch 163/180\nF1 Macro Score: 0.93774\n - 8s - loss: 0.0903 - acc: 0.9677 - val_loss: 0.0839 - val_acc: 0.9690\nEpoch 164/180\nF1 Macro Score: 0.93771\n - 8s - loss: 0.0907 - acc: 0.9676 - val_loss: 0.0838 - val_acc: 0.9690\nEpoch 165/180\nF1 Macro Score: 0.93760\n - 8s - loss: 0.0906 - acc: 0.9677 - val_loss: 0.0838 - val_acc: 0.9690\nEpoch 166/180\nF1 Macro Score: 0.93779\n - 8s - loss: 0.0904 - acc: 0.9677 - val_loss: 0.0838 - val_acc: 0.9690\nEpoch 167/180\nF1 Macro Score: 0.93780\n - 8s - loss: 0.0900 - acc: 0.9677 - val_loss: 0.0838 - val_acc: 0.9690\nEpoch 168/180\nF1 Macro Score: 0.93780\n - 8s - loss: 0.0907 - acc: 0.9677 - val_loss: 0.0838 - val_acc: 0.9690\nEpoch 169/180\nF1 Macro Score: 0.93775\n - 8s - loss: 0.0905 - acc: 0.9677 - val_loss: 0.0838 - val_acc: 0.9690\nEpoch 170/180\nF1 Macro Score: 0.93773\n - 8s - loss: 0.0902 - acc: 0.9677 - val_loss: 0.0838 - val_acc: 0.9690\nEpoch 171/180\nF1 Macro Score: 0.93784\n - 8s - loss: 0.0901 - acc: 0.9677 - val_loss: 0.0837 - val_acc: 0.9691\nEpoch 172/180\nF1 Macro Score: 0.93789\n - 7s - loss: 0.0905 - acc: 0.9678 - val_loss: 0.0837 - val_acc: 0.9690\nEpoch 173/180\nF1 Macro Score: 0.93768\n - 8s - loss: 0.0907 - acc: 0.9676 - val_loss: 0.0838 - val_acc: 0.9690\nEpoch 174/180\nF1 Macro Score: 0.93788\n - 8s - loss: 0.0910 - acc: 0.9676 - val_loss: 0.0837 - val_acc: 0.9691\nEpoch 175/180\nF1 Macro Score: 0.93762\n - 8s - loss: 0.0905 - acc: 0.9677 - val_loss: 0.0838 - val_acc: 0.9690\nEpoch 176/180\nF1 Macro Score: 0.93776\n - 8s - loss: 0.0902 - acc: 0.9677 - val_loss: 0.0837 - val_acc: 0.9690\nEpoch 177/180\nF1 Macro Score: 0.93782\n - 8s - loss: 0.0910 - acc: 0.9676 - val_loss: 0.0837 - val_acc: 0.9690\nEpoch 178/180\nF1 Macro Score: 0.93779\n - 7s - loss: 0.0904 - acc: 0.9677 - val_loss: 0.0837 - val_acc: 0.9690\nEpoch 179/180\nF1 Macro Score: 0.93777\n - 8s - loss: 0.0902 - acc: 0.9677 - val_loss: 0.0837 - val_acc: 0.9690\nEpoch 180/180\nF1 Macro Score: 0.93774\n - 8s - loss: 0.0901 - acc: 0.9677 - val_loss: 0.0837 - val_acc: 0.9690\nTraining fold 3 completed. macro f1 score : 0.93774\nOur training dataset shape is (1000, 4000, 19)\nOur validation dataset shape is (250, 4000, 19)\nTrain on 1000 samples, validate on 250 samples\nEpoch 1/180\nF1 Macro Score: 0.73141\n - 22s - loss: 0.5286 - acc: 0.8581 - val_loss: 0.7961 - val_acc: 0.8898\nEpoch 2/180\nF1 Macro Score: 0.80448\n - 7s - loss: 0.1723 - acc: 0.9603 - val_loss: 0.4450 - val_acc: 0.9367\nEpoch 3/180\nF1 Macro Score: 0.90435\n - 7s - loss: 0.1393 - acc: 0.9647 - val_loss: 0.2376 - val_acc: 0.9572\nEpoch 4/180\nF1 Macro Score: 0.92984\n - 7s - loss: 0.1265 - acc: 0.9658 - val_loss: 0.1538 - val_acc: 0.9643\nEpoch 5/180\nF1 Macro Score: 0.92419\n - 7s - loss: 0.1242 - acc: 0.9656 - val_loss: 0.1905 - val_acc: 0.9507\nEpoch 6/180\nF1 Macro Score: 0.93467\n - 7s - loss: 0.1453 - acc: 0.9614 - val_loss: 0.1082 - val_acc: 0.9665\nEpoch 7/180\nF1 Macro Score: 0.93695\n - 7s - loss: 0.1168 - acc: 0.9664 - val_loss: 0.1001 - val_acc: 0.9674\nEpoch 8/180\nF1 Macro Score: 0.93685\n - 7s - loss: 0.1112 - acc: 0.9668 - val_loss: 0.0974 - val_acc: 0.9676\nEpoch 9/180\nF1 Macro Score: 0.93714\n - 7s - loss: 0.1086 - acc: 0.9668 - val_loss: 0.0967 - val_acc: 0.9675\nEpoch 10/180\nF1 Macro Score: 0.93709\n - 7s - loss: 0.1063 - acc: 0.9670 - val_loss: 0.0953 - val_acc: 0.9676\nEpoch 11/180\nF1 Macro Score: 0.93581\n - 7s - loss: 0.1037 - acc: 0.9671 - val_loss: 0.0985 - val_acc: 0.9669\nEpoch 12/180\nF1 Macro Score: 0.93721\n - 7s - loss: 0.1034 - acc: 0.9671 - val_loss: 0.0927 - val_acc: 0.9677\nEpoch 13/180\nF1 Macro Score: 0.93727\n - 7s - loss: 0.1019 - acc: 0.9671 - val_loss: 0.0934 - val_acc: 0.9676\nEpoch 14/180\nF1 Macro Score: 0.93657\n - 7s - loss: 0.1029 - acc: 0.9670 - val_loss: 0.0934 - val_acc: 0.9675\nEpoch 15/180\nF1 Macro Score: 0.93751\n - 7s - loss: 0.1001 - acc: 0.9671 - val_loss: 0.0908 - val_acc: 0.9678\nEpoch 16/180\nF1 Macro Score: 0.93743\n - 7s - loss: 0.0973 - acc: 0.9674 - val_loss: 0.0904 - val_acc: 0.9677\nEpoch 17/180\nF1 Macro Score: 0.93801\n - 8s - loss: 0.0983 - acc: 0.9672 - val_loss: 0.0908 - val_acc: 0.9679\nEpoch 18/180\nF1 Macro Score: 0.93772\n - 8s - loss: 0.0969 - acc: 0.9673 - val_loss: 0.0900 - val_acc: 0.9678\nEpoch 19/180\nF1 Macro Score: 0.93710\n - 8s - loss: 0.0961 - acc: 0.9674 - val_loss: 0.0903 - val_acc: 0.9677\nEpoch 20/180\nF1 Macro Score: 0.93726\n - 7s - loss: 0.0961 - acc: 0.9673 - val_loss: 0.0917 - val_acc: 0.9675\nEpoch 21/180\nF1 Macro Score: 0.93717\n - 7s - loss: 0.0947 - acc: 0.9674 - val_loss: 0.0905 - val_acc: 0.9676\nEpoch 22/180\nF1 Macro Score: 0.93765\n - 7s - loss: 0.0950 - acc: 0.9675 - val_loss: 0.0882 - val_acc: 0.9679\nEpoch 23/180\nF1 Macro Score: 0.93622\n - 7s - loss: 0.0927 - acc: 0.9676 - val_loss: 0.0890 - val_acc: 0.9677\nEpoch 24/180\nF1 Macro Score: 0.93728\n - 7s - loss: 0.0940 - acc: 0.9675 - val_loss: 0.0884 - val_acc: 0.9677\nEpoch 25/180\nF1 Macro Score: 0.93720\n - 7s - loss: 0.0935 - acc: 0.9675 - val_loss: 0.0892 - val_acc: 0.9677\nEpoch 26/180\nF1 Macro Score: 0.93691\n - 7s - loss: 0.0917 - acc: 0.9677 - val_loss: 0.0885 - val_acc: 0.9677\nEpoch 27/180\nF1 Macro Score: 0.93772\n - 7s - loss: 0.0926 - acc: 0.9676 - val_loss: 0.0882 - val_acc: 0.9678\nEpoch 28/180\nF1 Macro Score: 0.93611\n - 7s - loss: 0.0917 - acc: 0.9677 - val_loss: 0.0907 - val_acc: 0.9674\nEpoch 29/180\nF1 Macro Score: 0.93663\n - 7s - loss: 0.0918 - acc: 0.9677 - val_loss: 0.0875 - val_acc: 0.9679\nEpoch 30/180\nF1 Macro Score: 0.93776\n - 7s - loss: 0.0902 - acc: 0.9680 - val_loss: 0.0872 - val_acc: 0.9679\nEpoch 31/180\nF1 Macro Score: 0.93779\n - 7s - loss: 0.0884 - acc: 0.9683 - val_loss: 0.0859 - val_acc: 0.9681\nEpoch 32/180\nF1 Macro Score: 0.93799\n - 7s - loss: 0.0891 - acc: 0.9682 - val_loss: 0.0858 - val_acc: 0.9681\nEpoch 33/180\nF1 Macro Score: 0.93860\n - 7s - loss: 0.0872 - acc: 0.9685 - val_loss: 0.0857 - val_acc: 0.9683\nEpoch 34/180\nF1 Macro Score: 0.93817\n - 7s - loss: 0.0872 - acc: 0.9684 - val_loss: 0.0855 - val_acc: 0.9682\nEpoch 35/180\nF1 Macro Score: 0.93870\n - 7s - loss: 0.0865 - acc: 0.9687 - val_loss: 0.0845 - val_acc: 0.9684\nEpoch 36/180\nF1 Macro Score: 0.93877\n - 8s - loss: 0.0860 - acc: 0.9687 - val_loss: 0.0843 - val_acc: 0.9685\nEpoch 37/180\nF1 Macro Score: 0.93916\n - 7s - loss: 0.0863 - acc: 0.9688 - val_loss: 0.0835 - val_acc: 0.9687\nEpoch 38/180\nF1 Macro Score: 0.93828\n - 7s - loss: 0.0867 - acc: 0.9687 - val_loss: 0.0851 - val_acc: 0.9683\nEpoch 39/180\nF1 Macro Score: 0.93915\n - 7s - loss: 0.0902 - acc: 0.9681 - val_loss: 0.0847 - val_acc: 0.9686\nEpoch 40/180\nF1 Macro Score: 0.93920\n - 7s - loss: 0.0861 - acc: 0.9690 - val_loss: 0.0833 - val_acc: 0.9688\nEpoch 41/180\nF1 Macro Score: 0.93970\n - 7s - loss: 0.0847 - acc: 0.9692 - val_loss: 0.0828 - val_acc: 0.9690\nEpoch 42/180\nF1 Macro Score: 0.93951\n - 7s - loss: 0.0839 - acc: 0.9693 - val_loss: 0.0831 - val_acc: 0.9689\nEpoch 43/180\nF1 Macro Score: 0.93903\n - 7s - loss: 0.0846 - acc: 0.9692 - val_loss: 0.0839 - val_acc: 0.9686\nEpoch 44/180\nF1 Macro Score: 0.94009\n - 7s - loss: 0.0841 - acc: 0.9693 - val_loss: 0.0826 - val_acc: 0.9691\nEpoch 45/180\nF1 Macro Score: 0.93999\n - 7s - loss: 0.0837 - acc: 0.9694 - val_loss: 0.0824 - val_acc: 0.9690\nEpoch 46/180\nF1 Macro Score: 0.93983\n - 7s - loss: 0.0836 - acc: 0.9695 - val_loss: 0.0826 - val_acc: 0.9689\nEpoch 47/180\nF1 Macro Score: 0.93825\n - 7s - loss: 0.0834 - acc: 0.9694 - val_loss: 0.0852 - val_acc: 0.9682\nEpoch 48/180\nF1 Macro Score: 0.93989\n - 7s - loss: 0.0833 - acc: 0.9694 - val_loss: 0.0821 - val_acc: 0.9691\nEpoch 49/180\nF1 Macro Score: 0.93973\n - 7s - loss: 0.0832 - acc: 0.9695 - val_loss: 0.0823 - val_acc: 0.9690\nEpoch 50/180\nF1 Macro Score: 0.93982\n - 7s - loss: 0.0826 - acc: 0.9695 - val_loss: 0.0821 - val_acc: 0.9690\nEpoch 51/180\nF1 Macro Score: 0.94010\n - 7s - loss: 0.0825 - acc: 0.9697 - val_loss: 0.0819 - val_acc: 0.9691\nEpoch 52/180\nF1 Macro Score: 0.94046\n - 8s - loss: 0.0824 - acc: 0.9697 - val_loss: 0.0818 - val_acc: 0.9693\nEpoch 53/180\nF1 Macro Score: 0.93953\n - 8s - loss: 0.0824 - acc: 0.9697 - val_loss: 0.0830 - val_acc: 0.9688\nEpoch 54/180\nF1 Macro Score: 0.93962\n - 8s - loss: 0.0821 - acc: 0.9697 - val_loss: 0.0818 - val_acc: 0.9691\nEpoch 55/180\nF1 Macro Score: 0.94045\n - 7s - loss: 0.0825 - acc: 0.9696 - val_loss: 0.0816 - val_acc: 0.9692\nEpoch 56/180\nF1 Macro Score: 0.93975\n - 7s - loss: 0.0824 - acc: 0.9697 - val_loss: 0.0824 - val_acc: 0.9689\nEpoch 57/180\nF1 Macro Score: 0.93955\n - 7s - loss: 0.0818 - acc: 0.9698 - val_loss: 0.0820 - val_acc: 0.9690\nEpoch 58/180\nF1 Macro Score: 0.94038\n - 7s - loss: 0.0820 - acc: 0.9697 - val_loss: 0.0815 - val_acc: 0.9692\nEpoch 59/180\nF1 Macro Score: 0.93956\n - 7s - loss: 0.0817 - acc: 0.9698 - val_loss: 0.0825 - val_acc: 0.9689\nEpoch 60/180\nF1 Macro Score: 0.94016\n - 7s - loss: 0.0818 - acc: 0.9698 - val_loss: 0.0814 - val_acc: 0.9692\nEpoch 61/180\nF1 Macro Score: 0.94034\n - 7s - loss: 0.0822 - acc: 0.9698 - val_loss: 0.0814 - val_acc: 0.9692\nEpoch 62/180\nF1 Macro Score: 0.94036\n - 7s - loss: 0.0814 - acc: 0.9699 - val_loss: 0.0811 - val_acc: 0.9693\nEpoch 63/180\nF1 Macro Score: 0.94000\n - 7s - loss: 0.0814 - acc: 0.9699 - val_loss: 0.0820 - val_acc: 0.9691\nEpoch 64/180\nF1 Macro Score: 0.94034\n - 7s - loss: 0.0809 - acc: 0.9700 - val_loss: 0.0812 - val_acc: 0.9692\nEpoch 65/180\nF1 Macro Score: 0.94046\n - 7s - loss: 0.0811 - acc: 0.9700 - val_loss: 0.0811 - val_acc: 0.9692\nEpoch 66/180\nF1 Macro Score: 0.93989\n - 7s - loss: 0.0809 - acc: 0.9700 - val_loss: 0.0816 - val_acc: 0.9691\nEpoch 67/180\nF1 Macro Score: 0.94014\n - 8s - loss: 0.0809 - acc: 0.9700 - val_loss: 0.0813 - val_acc: 0.9692\nEpoch 68/180\nF1 Macro Score: 0.94008\n - 8s - loss: 0.0809 - acc: 0.9700 - val_loss: 0.0816 - val_acc: 0.9691\nEpoch 69/180\nF1 Macro Score: 0.93991\n - 8s - loss: 0.0809 - acc: 0.9700 - val_loss: 0.0817 - val_acc: 0.9691\nEpoch 70/180\nF1 Macro Score: 0.94023\n - 8s - loss: 0.0811 - acc: 0.9699 - val_loss: 0.0818 - val_acc: 0.9690\nEpoch 71/180\nF1 Macro Score: 0.94020\n - 8s - loss: 0.0811 - acc: 0.9700 - val_loss: 0.0813 - val_acc: 0.9692\nEpoch 72/180\nF1 Macro Score: 0.94003\n - 8s - loss: 0.0803 - acc: 0.9701 - val_loss: 0.0812 - val_acc: 0.9692\nEpoch 73/180\nF1 Macro Score: 0.93995\n - 8s - loss: 0.0805 - acc: 0.9700 - val_loss: 0.0816 - val_acc: 0.9691\nEpoch 74/180\nF1 Macro Score: 0.93986\n - 7s - loss: 0.0805 - acc: 0.9701 - val_loss: 0.0817 - val_acc: 0.9690\nEpoch 75/180\nF1 Macro Score: 0.94024\n - 7s - loss: 0.0807 - acc: 0.9700 - val_loss: 0.0814 - val_acc: 0.9692\nEpoch 76/180\nF1 Macro Score: 0.94000\n - 7s - loss: 0.0800 - acc: 0.9702 - val_loss: 0.0815 - val_acc: 0.9691\nEpoch 77/180\nF1 Macro Score: 0.94026\n - 7s - loss: 0.0801 - acc: 0.9701 - val_loss: 0.0815 - val_acc: 0.9692\nEpoch 78/180\nF1 Macro Score: 0.93954\n - 7s - loss: 0.0811 - acc: 0.9700 - val_loss: 0.0822 - val_acc: 0.9689\nEpoch 79/180\nF1 Macro Score: 0.94030\n - 7s - loss: 0.0800 - acc: 0.9702 - val_loss: 0.0815 - val_acc: 0.9692\nEpoch 80/180\nF1 Macro Score: 0.93988\n - 7s - loss: 0.0804 - acc: 0.9701 - val_loss: 0.0817 - val_acc: 0.9690\nEpoch 81/180\nF1 Macro Score: 0.94047\n - 7s - loss: 0.0798 - acc: 0.9702 - val_loss: 0.0809 - val_acc: 0.9693\nEpoch 82/180\nF1 Macro Score: 0.94014\n - 7s - loss: 0.0796 - acc: 0.9703 - val_loss: 0.0816 - val_acc: 0.9691\nEpoch 83/180\nF1 Macro Score: 0.94025\n - 7s - loss: 0.0797 - acc: 0.9703 - val_loss: 0.0809 - val_acc: 0.9692\nEpoch 84/180\nF1 Macro Score: 0.94044\n - 7s - loss: 0.0802 - acc: 0.9702 - val_loss: 0.0816 - val_acc: 0.9692\nEpoch 85/180\nF1 Macro Score: 0.94026\n - 8s - loss: 0.0794 - acc: 0.9703 - val_loss: 0.0811 - val_acc: 0.9693\nEpoch 86/180\nF1 Macro Score: 0.94014\n - 7s - loss: 0.0794 - acc: 0.9703 - val_loss: 0.0812 - val_acc: 0.9692\nEpoch 87/180\nF1 Macro Score: 0.94029\n - 7s - loss: 0.0792 - acc: 0.9704 - val_loss: 0.0812 - val_acc: 0.9693\nEpoch 88/180\nF1 Macro Score: 0.94015\n - 7s - loss: 0.0792 - acc: 0.9704 - val_loss: 0.0812 - val_acc: 0.9692\nEpoch 89/180\nF1 Macro Score: 0.94034\n - 8s - loss: 0.0798 - acc: 0.9703 - val_loss: 0.0810 - val_acc: 0.9692\nEpoch 90/180\nF1 Macro Score: 0.94012\n - 7s - loss: 0.0792 - acc: 0.9704 - val_loss: 0.0813 - val_acc: 0.9691\nEpoch 91/180\nF1 Macro Score: 0.94024\n - 7s - loss: 0.0788 - acc: 0.9705 - val_loss: 0.0807 - val_acc: 0.9692\nEpoch 92/180\nF1 Macro Score: 0.94023\n - 7s - loss: 0.0789 - acc: 0.9705 - val_loss: 0.0807 - val_acc: 0.9692\nEpoch 93/180\nF1 Macro Score: 0.94022\n - 8s - loss: 0.0787 - acc: 0.9706 - val_loss: 0.0807 - val_acc: 0.9693\nEpoch 94/180\nF1 Macro Score: 0.94032\n - 7s - loss: 0.0795 - acc: 0.9704 - val_loss: 0.0808 - val_acc: 0.9693\nEpoch 95/180\nF1 Macro Score: 0.94025\n - 7s - loss: 0.0787 - acc: 0.9705 - val_loss: 0.0808 - val_acc: 0.9693\nEpoch 96/180\nF1 Macro Score: 0.94037\n - 7s - loss: 0.0783 - acc: 0.9706 - val_loss: 0.0808 - val_acc: 0.9693\nEpoch 97/180\nF1 Macro Score: 0.94032\n - 7s - loss: 0.0784 - acc: 0.9706 - val_loss: 0.0807 - val_acc: 0.9693\nEpoch 98/180\nF1 Macro Score: 0.94031\n - 7s - loss: 0.0791 - acc: 0.9705 - val_loss: 0.0808 - val_acc: 0.9692\nEpoch 99/180\nF1 Macro Score: 0.94026\n - 7s - loss: 0.0787 - acc: 0.9706 - val_loss: 0.0807 - val_acc: 0.9692\nEpoch 100/180\nF1 Macro Score: 0.94037\n - 8s - loss: 0.0787 - acc: 0.9706 - val_loss: 0.0808 - val_acc: 0.9693\nEpoch 101/180\nF1 Macro Score: 0.94033\n - 8s - loss: 0.0789 - acc: 0.9705 - val_loss: 0.0808 - val_acc: 0.9693\nEpoch 102/180\nF1 Macro Score: 0.94013\n - 8s - loss: 0.0784 - acc: 0.9706 - val_loss: 0.0808 - val_acc: 0.9692\nEpoch 103/180\nF1 Macro Score: 0.94023\n - 8s - loss: 0.0785 - acc: 0.9706 - val_loss: 0.0808 - val_acc: 0.9693\nEpoch 104/180\nF1 Macro Score: 0.94018\n - 8s - loss: 0.0784 - acc: 0.9706 - val_loss: 0.0808 - val_acc: 0.9692\nEpoch 105/180\nF1 Macro Score: 0.94026\n - 8s - loss: 0.0787 - acc: 0.9706 - val_loss: 0.0808 - val_acc: 0.9692\nEpoch 106/180\nF1 Macro Score: 0.94032\n - 8s - loss: 0.0786 - acc: 0.9706 - val_loss: 0.0808 - val_acc: 0.9693\nEpoch 107/180\nF1 Macro Score: 0.94017\n - 8s - loss: 0.0790 - acc: 0.9705 - val_loss: 0.0808 - val_acc: 0.9692\nEpoch 108/180\nF1 Macro Score: 0.94017\n - 8s - loss: 0.0790 - acc: 0.9706 - val_loss: 0.0809 - val_acc: 0.9692\nEpoch 109/180\nF1 Macro Score: 0.94033\n - 8s - loss: 0.0784 - acc: 0.9706 - val_loss: 0.0808 - val_acc: 0.9693\nEpoch 110/180\nF1 Macro Score: 0.94033\n - 8s - loss: 0.0784 - acc: 0.9706 - val_loss: 0.0808 - val_acc: 0.9692\nEpoch 111/180\nF1 Macro Score: 0.94027\n - 8s - loss: 0.0784 - acc: 0.9706 - val_loss: 0.0807 - val_acc: 0.9692\nEpoch 112/180\nF1 Macro Score: 0.94022\n - 8s - loss: 0.0786 - acc: 0.9705 - val_loss: 0.0808 - val_acc: 0.9692\nEpoch 113/180\nF1 Macro Score: 0.94027\n - 8s - loss: 0.0787 - acc: 0.9706 - val_loss: 0.0808 - val_acc: 0.9692\nEpoch 114/180\nF1 Macro Score: 0.94011\n - 8s - loss: 0.0782 - acc: 0.9706 - val_loss: 0.0808 - val_acc: 0.9692\nEpoch 115/180\nF1 Macro Score: 0.94013\n - 8s - loss: 0.0783 - acc: 0.9706 - val_loss: 0.0808 - val_acc: 0.9692\nEpoch 116/180\nF1 Macro Score: 0.94027\n - 8s - loss: 0.0785 - acc: 0.9706 - val_loss: 0.0807 - val_acc: 0.9693\nEpoch 117/180\nF1 Macro Score: 0.94011\n - 8s - loss: 0.0782 - acc: 0.9706 - val_loss: 0.0810 - val_acc: 0.9692\nEpoch 118/180\nF1 Macro Score: 0.94036\n - 8s - loss: 0.0784 - acc: 0.9706 - val_loss: 0.0809 - val_acc: 0.9693\nEpoch 119/180\nF1 Macro Score: 0.94019\n - 7s - loss: 0.0786 - acc: 0.9706 - val_loss: 0.0808 - val_acc: 0.9692\nEpoch 120/180\nF1 Macro Score: 0.94011\n - 7s - loss: 0.0783 - acc: 0.9706 - val_loss: 0.0808 - val_acc: 0.9692\nEpoch 121/180\nF1 Macro Score: 0.94006\n - 8s - loss: 0.0790 - acc: 0.9705 - val_loss: 0.0809 - val_acc: 0.9692\nEpoch 122/180\nF1 Macro Score: 0.94025\n - 7s - loss: 0.0785 - acc: 0.9706 - val_loss: 0.0808 - val_acc: 0.9692\nEpoch 123/180\nF1 Macro Score: 0.94020\n - 7s - loss: 0.0782 - acc: 0.9706 - val_loss: 0.0809 - val_acc: 0.9692\nEpoch 124/180\nF1 Macro Score: 0.94010\n - 7s - loss: 0.0785 - acc: 0.9706 - val_loss: 0.0808 - val_acc: 0.9692\nEpoch 125/180\nF1 Macro Score: 0.94025\n - 7s - loss: 0.0783 - acc: 0.9707 - val_loss: 0.0808 - val_acc: 0.9692\nEpoch 126/180\nF1 Macro Score: 0.94024\n - 7s - loss: 0.0783 - acc: 0.9706 - val_loss: 0.0808 - val_acc: 0.9692\nEpoch 127/180\nF1 Macro Score: 0.94040\n - 7s - loss: 0.0786 - acc: 0.9706 - val_loss: 0.0809 - val_acc: 0.9693\nEpoch 128/180\nF1 Macro Score: 0.94012\n - 7s - loss: 0.0783 - acc: 0.9706 - val_loss: 0.0809 - val_acc: 0.9692\nEpoch 129/180\nF1 Macro Score: 0.94035\n - 7s - loss: 0.0783 - acc: 0.9707 - val_loss: 0.0809 - val_acc: 0.9692\nEpoch 130/180\nF1 Macro Score: 0.94014\n - 7s - loss: 0.0784 - acc: 0.9706 - val_loss: 0.0808 - val_acc: 0.9692\nEpoch 131/180\nF1 Macro Score: 0.94025\n - 7s - loss: 0.0780 - acc: 0.9707 - val_loss: 0.0808 - val_acc: 0.9692\nEpoch 132/180\nF1 Macro Score: 0.94023\n - 7s - loss: 0.0787 - acc: 0.9706 - val_loss: 0.0808 - val_acc: 0.9692\nEpoch 133/180\nF1 Macro Score: 0.94039\n - 7s - loss: 0.0782 - acc: 0.9706 - val_loss: 0.0808 - val_acc: 0.9693\nEpoch 134/180\nF1 Macro Score: 0.94004\n - 7s - loss: 0.0784 - acc: 0.9706 - val_loss: 0.0810 - val_acc: 0.9692\nEpoch 135/180\nF1 Macro Score: 0.94012\n - 7s - loss: 0.0786 - acc: 0.9706 - val_loss: 0.0810 - val_acc: 0.9691\nEpoch 136/180\nF1 Macro Score: 0.94011\n - 7s - loss: 0.0787 - acc: 0.9705 - val_loss: 0.0811 - val_acc: 0.9691\nEpoch 137/180\nF1 Macro Score: 0.94019\n - 7s - loss: 0.0780 - acc: 0.9707 - val_loss: 0.0809 - val_acc: 0.9692\nEpoch 138/180\nF1 Macro Score: 0.94010\n - 7s - loss: 0.0782 - acc: 0.9706 - val_loss: 0.0809 - val_acc: 0.9692\nEpoch 139/180\nF1 Macro Score: 0.94037\n - 7s - loss: 0.0781 - acc: 0.9707 - val_loss: 0.0808 - val_acc: 0.9693\nEpoch 140/180\nF1 Macro Score: 0.94020\n - 7s - loss: 0.0783 - acc: 0.9707 - val_loss: 0.0808 - val_acc: 0.9692\nEpoch 141/180\nF1 Macro Score: 0.94019\n - 7s - loss: 0.0786 - acc: 0.9706 - val_loss: 0.0808 - val_acc: 0.9692\nEpoch 142/180\nF1 Macro Score: 0.94011\n - 7s - loss: 0.0784 - acc: 0.9706 - val_loss: 0.0808 - val_acc: 0.9692\nEpoch 143/180\nF1 Macro Score: 0.94006\n - 7s - loss: 0.0782 - acc: 0.9707 - val_loss: 0.0810 - val_acc: 0.9692\nEpoch 144/180\nF1 Macro Score: 0.94008\n - 7s - loss: 0.0785 - acc: 0.9707 - val_loss: 0.0809 - val_acc: 0.9691\nEpoch 145/180\nF1 Macro Score: 0.94027\n - 7s - loss: 0.0780 - acc: 0.9707 - val_loss: 0.0810 - val_acc: 0.9692\nEpoch 146/180\nF1 Macro Score: 0.94023\n - 7s - loss: 0.0784 - acc: 0.9706 - val_loss: 0.0810 - val_acc: 0.9692\nEpoch 147/180\nF1 Macro Score: 0.94019\n - 7s - loss: 0.0780 - acc: 0.9707 - val_loss: 0.0808 - val_acc: 0.9692\nEpoch 148/180\nF1 Macro Score: 0.94025\n - 7s - loss: 0.0784 - acc: 0.9705 - val_loss: 0.0809 - val_acc: 0.9692\nEpoch 149/180\nF1 Macro Score: 0.94027\n - 7s - loss: 0.0779 - acc: 0.9707 - val_loss: 0.0808 - val_acc: 0.9692\nEpoch 150/180\nF1 Macro Score: 0.94010\n - 7s - loss: 0.0779 - acc: 0.9707 - val_loss: 0.0809 - val_acc: 0.9692\nEpoch 151/180\nF1 Macro Score: 0.94027\n - 8s - loss: 0.0781 - acc: 0.9707 - val_loss: 0.0809 - val_acc: 0.9692\nEpoch 152/180\nF1 Macro Score: 0.94008\n - 8s - loss: 0.0781 - acc: 0.9707 - val_loss: 0.0809 - val_acc: 0.9692\nEpoch 153/180\nF1 Macro Score: 0.94021\n - 8s - loss: 0.0779 - acc: 0.9707 - val_loss: 0.0808 - val_acc: 0.9692\nEpoch 154/180\nF1 Macro Score: 0.94012\n - 8s - loss: 0.0781 - acc: 0.9706 - val_loss: 0.0809 - val_acc: 0.9692\nEpoch 155/180\nF1 Macro Score: 0.94017\n - 8s - loss: 0.0779 - acc: 0.9708 - val_loss: 0.0809 - val_acc: 0.9692\nEpoch 156/180\nF1 Macro Score: 0.94017\n - 8s - loss: 0.0779 - acc: 0.9707 - val_loss: 0.0808 - val_acc: 0.9692\nEpoch 157/180\nF1 Macro Score: 0.94023\n - 8s - loss: 0.0781 - acc: 0.9706 - val_loss: 0.0808 - val_acc: 0.9692\nEpoch 158/180\nF1 Macro Score: 0.94017\n - 8s - loss: 0.0786 - acc: 0.9706 - val_loss: 0.0809 - val_acc: 0.9692\nEpoch 159/180\nF1 Macro Score: 0.94005\n - 8s - loss: 0.0778 - acc: 0.9707 - val_loss: 0.0809 - val_acc: 0.9692\nEpoch 160/180\nF1 Macro Score: 0.94012\n - 8s - loss: 0.0784 - acc: 0.9706 - val_loss: 0.0809 - val_acc: 0.9692\nEpoch 161/180\nF1 Macro Score: 0.94019\n - 8s - loss: 0.0782 - acc: 0.9707 - val_loss: 0.0809 - val_acc: 0.9692\nEpoch 162/180\nF1 Macro Score: 0.94026\n - 8s - loss: 0.0779 - acc: 0.9707 - val_loss: 0.0809 - val_acc: 0.9692\nEpoch 163/180\nF1 Macro Score: 0.94007\n - 8s - loss: 0.0778 - acc: 0.9708 - val_loss: 0.0809 - val_acc: 0.9692\nEpoch 164/180\nF1 Macro Score: 0.94017\n - 8s - loss: 0.0778 - acc: 0.9708 - val_loss: 0.0812 - val_acc: 0.9692\nEpoch 165/180\nF1 Macro Score: 0.94009\n - 8s - loss: 0.0779 - acc: 0.9707 - val_loss: 0.0810 - val_acc: 0.9692\nEpoch 166/180\nF1 Macro Score: 0.94025\n - 8s - loss: 0.0778 - acc: 0.9708 - val_loss: 0.0809 - val_acc: 0.9692\nEpoch 167/180\nF1 Macro Score: 0.94019\n - 8s - loss: 0.0780 - acc: 0.9706 - val_loss: 0.0809 - val_acc: 0.9692\nEpoch 168/180\nF1 Macro Score: 0.94021\n - 7s - loss: 0.0778 - acc: 0.9708 - val_loss: 0.0809 - val_acc: 0.9692\nEpoch 169/180\nF1 Macro Score: 0.94027\n - 7s - loss: 0.0775 - acc: 0.9708 - val_loss: 0.0809 - val_acc: 0.9692\nEpoch 170/180\nF1 Macro Score: 0.94020\n - 7s - loss: 0.0778 - acc: 0.9708 - val_loss: 0.0809 - val_acc: 0.9692\nEpoch 171/180\nF1 Macro Score: 0.93992\n - 7s - loss: 0.0779 - acc: 0.9707 - val_loss: 0.0810 - val_acc: 0.9691\nEpoch 172/180\nF1 Macro Score: 0.94002\n - 7s - loss: 0.0779 - acc: 0.9707 - val_loss: 0.0809 - val_acc: 0.9691\nEpoch 173/180\nF1 Macro Score: 0.94020\n - 7s - loss: 0.0779 - acc: 0.9707 - val_loss: 0.0809 - val_acc: 0.9692\nEpoch 174/180\nF1 Macro Score: 0.94020\n - 7s - loss: 0.0777 - acc: 0.9708 - val_loss: 0.0809 - val_acc: 0.9692\nEpoch 175/180\nF1 Macro Score: 0.94004\n - 7s - loss: 0.0778 - acc: 0.9708 - val_loss: 0.0810 - val_acc: 0.9691\nEpoch 176/180\nF1 Macro Score: 0.94011\n - 7s - loss: 0.0777 - acc: 0.9707 - val_loss: 0.0809 - val_acc: 0.9692\nEpoch 177/180\nF1 Macro Score: 0.94015\n - 7s - loss: 0.0779 - acc: 0.9707 - val_loss: 0.0809 - val_acc: 0.9692\nEpoch 178/180\nF1 Macro Score: 0.94019\n - 7s - loss: 0.0776 - acc: 0.9708 - val_loss: 0.0808 - val_acc: 0.9692\nEpoch 179/180\nF1 Macro Score: 0.94028\n - 7s - loss: 0.0780 - acc: 0.9707 - val_loss: 0.0809 - val_acc: 0.9692\nEpoch 180/180\nF1 Macro Score: 0.94027\n - 7s - loss: 0.0777 - acc: 0.9707 - val_loss: 0.0809 - val_acc: 0.9692\nTraining fold 4 completed. macro f1 score : 0.94027\nOur training dataset shape is (1000, 4000, 19)\nOur validation dataset shape is (250, 4000, 19)\nTrain on 1000 samples, validate on 250 samples\nEpoch 1/180\nF1 Macro Score: 0.72154\n - 24s - loss: 0.5772 - acc: 0.8375 - val_loss: 0.9280 - val_acc: 0.8896\nEpoch 2/180\nF1 Macro Score: 0.91849\n - 8s - loss: 0.1851 - acc: 0.9583 - val_loss: 0.4552 - val_acc: 0.9598\nEpoch 3/180\nF1 Macro Score: 0.93262\n - 8s - loss: 0.1425 - acc: 0.9641 - val_loss: 0.2407 - val_acc: 0.9657\nEpoch 4/180\nF1 Macro Score: 0.93494\n - 8s - loss: 0.1270 - acc: 0.9658 - val_loss: 0.1442 - val_acc: 0.9664\nEpoch 5/180\nF1 Macro Score: 0.93494\n - 8s - loss: 0.1200 - acc: 0.9662 - val_loss: 0.1215 - val_acc: 0.9663\nEpoch 6/180\nF1 Macro Score: 0.93678\n - 8s - loss: 0.1174 - acc: 0.9662 - val_loss: 0.1030 - val_acc: 0.9674\nEpoch 7/180\nF1 Macro Score: 0.93727\n - 8s - loss: 0.1128 - acc: 0.9666 - val_loss: 0.0981 - val_acc: 0.9676\nEpoch 8/180\nF1 Macro Score: 0.93544\n - 8s - loss: 0.1108 - acc: 0.9666 - val_loss: 0.1025 - val_acc: 0.9666\nEpoch 9/180\nF1 Macro Score: 0.93726\n - 8s - loss: 0.1083 - acc: 0.9668 - val_loss: 0.0947 - val_acc: 0.9676\nEpoch 10/180\nF1 Macro Score: 0.93630\n - 8s - loss: 0.1061 - acc: 0.9669 - val_loss: 0.0963 - val_acc: 0.9672\nEpoch 11/180\nF1 Macro Score: 0.93748\n - 8s - loss: 0.1035 - acc: 0.9670 - val_loss: 0.0919 - val_acc: 0.9678\nEpoch 12/180\nF1 Macro Score: 0.93715\n - 8s - loss: 0.1033 - acc: 0.9670 - val_loss: 0.0936 - val_acc: 0.9675\nEpoch 13/180\nF1 Macro Score: 0.93762\n - 8s - loss: 0.1010 - acc: 0.9670 - val_loss: 0.0909 - val_acc: 0.9678\nEpoch 14/180\nF1 Macro Score: 0.93618\n - 8s - loss: 0.1003 - acc: 0.9670 - val_loss: 0.0924 - val_acc: 0.9675\nEpoch 15/180\nF1 Macro Score: 0.93663\n - 8s - loss: 0.1028 - acc: 0.9669 - val_loss: 0.0925 - val_acc: 0.9675\nEpoch 16/180\nF1 Macro Score: 0.93657\n - 7s - loss: 0.1003 - acc: 0.9670 - val_loss: 0.0923 - val_acc: 0.9673\nEpoch 17/180\nF1 Macro Score: 0.93615\n - 7s - loss: 0.0980 - acc: 0.9671 - val_loss: 0.0935 - val_acc: 0.9672\nEpoch 18/180\nF1 Macro Score: 0.93763\n - 8s - loss: 0.0979 - acc: 0.9671 - val_loss: 0.0886 - val_acc: 0.9679\nEpoch 19/180\nF1 Macro Score: 0.85357\n - 8s - loss: 0.1268 - acc: 0.9609 - val_loss: 0.2296 - val_acc: 0.9320\nEpoch 20/180\nF1 Macro Score: 0.93335\n - 8s - loss: 0.1287 - acc: 0.9632 - val_loss: 0.1158 - val_acc: 0.9662\nEpoch 21/180\nF1 Macro Score: 0.93648\n - 8s - loss: 0.1062 - acc: 0.9668 - val_loss: 0.0943 - val_acc: 0.9677\nEpoch 22/180\nF1 Macro Score: 0.93706\n - 8s - loss: 0.1001 - acc: 0.9672 - val_loss: 0.0918 - val_acc: 0.9677\nEpoch 23/180\nF1 Macro Score: 0.93643\n - 8s - loss: 0.0990 - acc: 0.9672 - val_loss: 0.0910 - val_acc: 0.9676\nEpoch 24/180\nF1 Macro Score: 0.93716\n - 7s - loss: 0.0977 - acc: 0.9672 - val_loss: 0.0898 - val_acc: 0.9677\nEpoch 25/180\nF1 Macro Score: 0.93605\n - 7s - loss: 0.0983 - acc: 0.9671 - val_loss: 0.0916 - val_acc: 0.9673\nEpoch 26/180\nF1 Macro Score: 0.93608\n - 8s - loss: 0.0957 - acc: 0.9674 - val_loss: 0.0890 - val_acc: 0.9677\nEpoch 27/180\nF1 Macro Score: 0.93690\n - 8s - loss: 0.0939 - acc: 0.9675 - val_loss: 0.0885 - val_acc: 0.9677\nEpoch 28/180\nF1 Macro Score: 0.93605\n - 7s - loss: 0.0962 - acc: 0.9672 - val_loss: 0.0895 - val_acc: 0.9675\nEpoch 29/180\nF1 Macro Score: 0.93706\n - 9s - loss: 0.0937 - acc: 0.9675 - val_loss: 0.0884 - val_acc: 0.9677\nEpoch 30/180\nF1 Macro Score: 0.93769\n - 8s - loss: 0.0926 - acc: 0.9675 - val_loss: 0.0871 - val_acc: 0.9680\nEpoch 31/180\nF1 Macro Score: 0.93761\n - 8s - loss: 0.0910 - acc: 0.9678 - val_loss: 0.0867 - val_acc: 0.9679\nEpoch 32/180\nF1 Macro Score: 0.93817\n - 8s - loss: 0.0905 - acc: 0.9678 - val_loss: 0.0856 - val_acc: 0.9682\nEpoch 33/180\nF1 Macro Score: 0.93796\n - 8s - loss: 0.0903 - acc: 0.9678 - val_loss: 0.0854 - val_acc: 0.9682\nEpoch 34/180\nF1 Macro Score: 0.93814\n - 8s - loss: 0.0900 - acc: 0.9678 - val_loss: 0.0850 - val_acc: 0.9683\nEpoch 35/180\nF1 Macro Score: 0.93807\n - 8s - loss: 0.0898 - acc: 0.9679 - val_loss: 0.0849 - val_acc: 0.9682\nEpoch 36/180\nF1 Macro Score: 0.93776\n - 8s - loss: 0.0898 - acc: 0.9679 - val_loss: 0.0857 - val_acc: 0.9681\nEpoch 37/180\nF1 Macro Score: 0.93823\n - 8s - loss: 0.0891 - acc: 0.9680 - val_loss: 0.0844 - val_acc: 0.9683\nEpoch 38/180\nF1 Macro Score: 0.93833\n - 8s - loss: 0.0890 - acc: 0.9680 - val_loss: 0.0845 - val_acc: 0.9683\nEpoch 39/180\nF1 Macro Score: 0.93789\n - 8s - loss: 0.0887 - acc: 0.9680 - val_loss: 0.0847 - val_acc: 0.9683\nEpoch 40/180\nF1 Macro Score: 0.93774\n - 8s - loss: 0.0889 - acc: 0.9680 - val_loss: 0.0848 - val_acc: 0.9683\nEpoch 41/180\nF1 Macro Score: 0.93822\n - 8s - loss: 0.0891 - acc: 0.9680 - val_loss: 0.0844 - val_acc: 0.9683\nEpoch 42/180\nF1 Macro Score: 0.93842\n - 8s - loss: 0.0879 - acc: 0.9682 - val_loss: 0.0838 - val_acc: 0.9684\nEpoch 43/180\nF1 Macro Score: 0.93841\n - 8s - loss: 0.0883 - acc: 0.9680 - val_loss: 0.0838 - val_acc: 0.9685\nEpoch 44/180\nF1 Macro Score: 0.93838\n - 7s - loss: 0.0883 - acc: 0.9681 - val_loss: 0.0839 - val_acc: 0.9684\nEpoch 45/180\nF1 Macro Score: 0.93821\n - 8s - loss: 0.0878 - acc: 0.9683 - val_loss: 0.0838 - val_acc: 0.9684\nEpoch 46/180\nF1 Macro Score: 0.93795\n - 8s - loss: 0.0879 - acc: 0.9682 - val_loss: 0.0841 - val_acc: 0.9683\nEpoch 47/180\nF1 Macro Score: 0.93858\n - 8s - loss: 0.0878 - acc: 0.9682 - val_loss: 0.0839 - val_acc: 0.9684\nEpoch 48/180\nF1 Macro Score: 0.93842\n - 8s - loss: 0.0874 - acc: 0.9682 - val_loss: 0.0836 - val_acc: 0.9685\nEpoch 49/180\nF1 Macro Score: 0.93859\n - 7s - loss: 0.0872 - acc: 0.9683 - val_loss: 0.0837 - val_acc: 0.9685\nEpoch 50/180\nF1 Macro Score: 0.93839\n - 8s - loss: 0.0872 - acc: 0.9683 - val_loss: 0.0835 - val_acc: 0.9684\nEpoch 51/180\nF1 Macro Score: 0.93863\n - 8s - loss: 0.0871 - acc: 0.9683 - val_loss: 0.0834 - val_acc: 0.9685\nEpoch 52/180\nF1 Macro Score: 0.93873\n - 8s - loss: 0.0865 - acc: 0.9685 - val_loss: 0.0830 - val_acc: 0.9686\nEpoch 53/180\nF1 Macro Score: 0.93885\n - 8s - loss: 0.0864 - acc: 0.9685 - val_loss: 0.0830 - val_acc: 0.9686\nEpoch 54/180\nF1 Macro Score: 0.93827\n - 8s - loss: 0.0861 - acc: 0.9685 - val_loss: 0.0834 - val_acc: 0.9685\nEpoch 55/180\nF1 Macro Score: 0.93875\n - 8s - loss: 0.0862 - acc: 0.9685 - val_loss: 0.0829 - val_acc: 0.9686\nEpoch 56/180\nF1 Macro Score: 0.93862\n - 8s - loss: 0.0860 - acc: 0.9686 - val_loss: 0.0831 - val_acc: 0.9685\nEpoch 57/180\nF1 Macro Score: 0.93887\n - 8s - loss: 0.0863 - acc: 0.9685 - val_loss: 0.0831 - val_acc: 0.9686\nEpoch 58/180\nF1 Macro Score: 0.93925\n - 8s - loss: 0.0857 - acc: 0.9686 - val_loss: 0.0828 - val_acc: 0.9687\nEpoch 59/180\nF1 Macro Score: 0.93867\n - 8s - loss: 0.0855 - acc: 0.9687 - val_loss: 0.0827 - val_acc: 0.9686\nEpoch 60/180\nF1 Macro Score: 0.93929\n - 8s - loss: 0.0852 - acc: 0.9687 - val_loss: 0.0828 - val_acc: 0.9688\nEpoch 61/180\nF1 Macro Score: 0.93924\n - 8s - loss: 0.0853 - acc: 0.9688 - val_loss: 0.0821 - val_acc: 0.9689\nEpoch 62/180\nF1 Macro Score: 0.93958\n - 8s - loss: 0.0848 - acc: 0.9689 - val_loss: 0.0821 - val_acc: 0.9690\nEpoch 63/180\nF1 Macro Score: 0.93846\n - 8s - loss: 0.0852 - acc: 0.9689 - val_loss: 0.0827 - val_acc: 0.9686\nEpoch 64/180\nF1 Macro Score: 0.93968\n - 7s - loss: 0.0860 - acc: 0.9687 - val_loss: 0.0820 - val_acc: 0.9691\nEpoch 65/180\nF1 Macro Score: 0.93931\n - 8s - loss: 0.0866 - acc: 0.9686 - val_loss: 0.0824 - val_acc: 0.9689\nEpoch 66/180\nF1 Macro Score: 0.93918\n - 8s - loss: 0.0847 - acc: 0.9690 - val_loss: 0.0821 - val_acc: 0.9690\nEpoch 67/180\nF1 Macro Score: 0.93950\n - 8s - loss: 0.0839 - acc: 0.9691 - val_loss: 0.0819 - val_acc: 0.9690\nEpoch 68/180\nF1 Macro Score: 0.94007\n - 8s - loss: 0.0842 - acc: 0.9691 - val_loss: 0.0813 - val_acc: 0.9692\nEpoch 69/180\nF1 Macro Score: 0.93934\n - 8s - loss: 0.0839 - acc: 0.9692 - val_loss: 0.0815 - val_acc: 0.9691\nEpoch 70/180\nF1 Macro Score: 0.94007\n - 8s - loss: 0.0837 - acc: 0.9693 - val_loss: 0.0816 - val_acc: 0.9692\nEpoch 71/180\nF1 Macro Score: 0.93993\n - 8s - loss: 0.0834 - acc: 0.9693 - val_loss: 0.0813 - val_acc: 0.9692\nEpoch 72/180\nF1 Macro Score: 0.93994\n - 8s - loss: 0.0845 - acc: 0.9691 - val_loss: 0.0813 - val_acc: 0.9691\nEpoch 73/180\nF1 Macro Score: 0.94001\n - 7s - loss: 0.0831 - acc: 0.9694 - val_loss: 0.0810 - val_acc: 0.9693\nEpoch 74/180\nF1 Macro Score: 0.93960\n - 8s - loss: 0.0840 - acc: 0.9693 - val_loss: 0.0828 - val_acc: 0.9690\nEpoch 75/180\nF1 Macro Score: 0.93991\n - 8s - loss: 0.0841 - acc: 0.9692 - val_loss: 0.0816 - val_acc: 0.9692\nEpoch 76/180\nF1 Macro Score: 0.94036\n - 8s - loss: 0.0834 - acc: 0.9694 - val_loss: 0.0810 - val_acc: 0.9693\nEpoch 77/180\nF1 Macro Score: 0.94039\n - 8s - loss: 0.0828 - acc: 0.9695 - val_loss: 0.0811 - val_acc: 0.9694\nEpoch 78/180\nF1 Macro Score: 0.94008\n - 8s - loss: 0.0834 - acc: 0.9694 - val_loss: 0.0808 - val_acc: 0.9693\nEpoch 79/180\nF1 Macro Score: 0.94026\n - 8s - loss: 0.0830 - acc: 0.9695 - val_loss: 0.0805 - val_acc: 0.9694\nEpoch 80/180\nF1 Macro Score: 0.94054\n - 8s - loss: 0.0829 - acc: 0.9696 - val_loss: 0.0805 - val_acc: 0.9695\nEpoch 81/180\nF1 Macro Score: 0.94068\n - 8s - loss: 0.0825 - acc: 0.9696 - val_loss: 0.0803 - val_acc: 0.9695\nEpoch 82/180\nF1 Macro Score: 0.94034\n - 8s - loss: 0.0821 - acc: 0.9696 - val_loss: 0.0805 - val_acc: 0.9694\nEpoch 83/180\nF1 Macro Score: 0.94063\n - 8s - loss: 0.0822 - acc: 0.9697 - val_loss: 0.0804 - val_acc: 0.9695\nEpoch 84/180\nF1 Macro Score: 0.94031\n - 8s - loss: 0.0822 - acc: 0.9696 - val_loss: 0.0809 - val_acc: 0.9694\nEpoch 85/180\nF1 Macro Score: 0.94041\n - 8s - loss: 0.0831 - acc: 0.9695 - val_loss: 0.0806 - val_acc: 0.9695\nEpoch 86/180\nF1 Macro Score: 0.93967\n - 8s - loss: 0.0823 - acc: 0.9696 - val_loss: 0.0811 - val_acc: 0.9692\nEpoch 87/180\nF1 Macro Score: 0.94047\n - 8s - loss: 0.0817 - acc: 0.9697 - val_loss: 0.0806 - val_acc: 0.9695\nEpoch 88/180\nF1 Macro Score: 0.94054\n - 8s - loss: 0.0816 - acc: 0.9698 - val_loss: 0.0807 - val_acc: 0.9695\nEpoch 89/180\nF1 Macro Score: 0.94052\n - 8s - loss: 0.0816 - acc: 0.9698 - val_loss: 0.0805 - val_acc: 0.9694\nEpoch 90/180\nF1 Macro Score: 0.94078\n - 8s - loss: 0.0823 - acc: 0.9697 - val_loss: 0.0799 - val_acc: 0.9696\nEpoch 91/180\nF1 Macro Score: 0.94100\n - 8s - loss: 0.0812 - acc: 0.9699 - val_loss: 0.0797 - val_acc: 0.9697\nEpoch 92/180\nF1 Macro Score: 0.94095\n - 8s - loss: 0.0813 - acc: 0.9699 - val_loss: 0.0798 - val_acc: 0.9697\nEpoch 93/180\nF1 Macro Score: 0.94085\n - 8s - loss: 0.0812 - acc: 0.9699 - val_loss: 0.0798 - val_acc: 0.9696\nEpoch 94/180\nF1 Macro Score: 0.94101\n - 8s - loss: 0.0813 - acc: 0.9699 - val_loss: 0.0798 - val_acc: 0.9697\nEpoch 95/180\nF1 Macro Score: 0.94095\n - 8s - loss: 0.0811 - acc: 0.9699 - val_loss: 0.0797 - val_acc: 0.9697\nEpoch 96/180\nF1 Macro Score: 0.94096\n - 8s - loss: 0.0809 - acc: 0.9700 - val_loss: 0.0798 - val_acc: 0.9697\nEpoch 97/180\nF1 Macro Score: 0.94091\n - 8s - loss: 0.0826 - acc: 0.9696 - val_loss: 0.0798 - val_acc: 0.9697\nEpoch 98/180\nF1 Macro Score: 0.94090\n - 8s - loss: 0.0816 - acc: 0.9698 - val_loss: 0.0798 - val_acc: 0.9697\nEpoch 99/180\nF1 Macro Score: 0.94093\n - 8s - loss: 0.0811 - acc: 0.9699 - val_loss: 0.0797 - val_acc: 0.9697\nEpoch 100/180\nF1 Macro Score: 0.94096\n - 8s - loss: 0.0809 - acc: 0.9699 - val_loss: 0.0797 - val_acc: 0.9697\nEpoch 101/180\nF1 Macro Score: 0.94097\n - 8s - loss: 0.0811 - acc: 0.9700 - val_loss: 0.0797 - val_acc: 0.9697\nEpoch 102/180\nF1 Macro Score: 0.94100\n - 8s - loss: 0.0809 - acc: 0.9699 - val_loss: 0.0797 - val_acc: 0.9697\nEpoch 103/180\nF1 Macro Score: 0.94090\n - 8s - loss: 0.0820 - acc: 0.9697 - val_loss: 0.0797 - val_acc: 0.9696\nEpoch 104/180\nF1 Macro Score: 0.94096\n - 8s - loss: 0.0811 - acc: 0.9699 - val_loss: 0.0798 - val_acc: 0.9697\nEpoch 105/180\nF1 Macro Score: 0.94091\n - 8s - loss: 0.0809 - acc: 0.9699 - val_loss: 0.0797 - val_acc: 0.9697\nEpoch 106/180\nF1 Macro Score: 0.94084\n - 8s - loss: 0.0807 - acc: 0.9699 - val_loss: 0.0797 - val_acc: 0.9696\nEpoch 107/180\nF1 Macro Score: 0.94097\n - 8s - loss: 0.0809 - acc: 0.9700 - val_loss: 0.0797 - val_acc: 0.9697\nEpoch 108/180\nF1 Macro Score: 0.94089\n - 8s - loss: 0.0809 - acc: 0.9700 - val_loss: 0.0797 - val_acc: 0.9697\nEpoch 109/180\nF1 Macro Score: 0.94091\n - 8s - loss: 0.0807 - acc: 0.9699 - val_loss: 0.0796 - val_acc: 0.9697\nEpoch 110/180\nF1 Macro Score: 0.94087\n - 8s - loss: 0.0812 - acc: 0.9699 - val_loss: 0.0797 - val_acc: 0.9697\nEpoch 111/180\nF1 Macro Score: 0.94091\n - 8s - loss: 0.0806 - acc: 0.9700 - val_loss: 0.0797 - val_acc: 0.9697\nEpoch 112/180\nF1 Macro Score: 0.94097\n - 8s - loss: 0.0808 - acc: 0.9700 - val_loss: 0.0797 - val_acc: 0.9697\nEpoch 113/180\nF1 Macro Score: 0.94099\n - 8s - loss: 0.0804 - acc: 0.9701 - val_loss: 0.0796 - val_acc: 0.9697\nEpoch 114/180\nF1 Macro Score: 0.94091\n - 8s - loss: 0.0809 - acc: 0.9699 - val_loss: 0.0797 - val_acc: 0.9697\nEpoch 115/180\nF1 Macro Score: 0.94086\n - 8s - loss: 0.0808 - acc: 0.9700 - val_loss: 0.0797 - val_acc: 0.9697\nEpoch 116/180\nF1 Macro Score: 0.94089\n - 7s - loss: 0.0810 - acc: 0.9699 - val_loss: 0.0796 - val_acc: 0.9697\nEpoch 117/180\nF1 Macro Score: 0.94090\n - 8s - loss: 0.0815 - acc: 0.9699 - val_loss: 0.0797 - val_acc: 0.9696\nEpoch 118/180\nF1 Macro Score: 0.94085\n - 8s - loss: 0.0805 - acc: 0.9701 - val_loss: 0.0797 - val_acc: 0.9697\nEpoch 119/180\nF1 Macro Score: 0.94086\n - 8s - loss: 0.0806 - acc: 0.9700 - val_loss: 0.0797 - val_acc: 0.9697\nEpoch 120/180\nF1 Macro Score: 0.94079\n - 8s - loss: 0.0810 - acc: 0.9700 - val_loss: 0.0797 - val_acc: 0.9696\nEpoch 121/180\nF1 Macro Score: 0.94087\n - 8s - loss: 0.0806 - acc: 0.9700 - val_loss: 0.0797 - val_acc: 0.9696\nEpoch 122/180\nF1 Macro Score: 0.94092\n - 8s - loss: 0.0807 - acc: 0.9700 - val_loss: 0.0796 - val_acc: 0.9697\nEpoch 123/180\nF1 Macro Score: 0.94087\n - 8s - loss: 0.0820 - acc: 0.9698 - val_loss: 0.0796 - val_acc: 0.9697\nEpoch 124/180\nF1 Macro Score: 0.94096\n - 8s - loss: 0.0811 - acc: 0.9700 - val_loss: 0.0797 - val_acc: 0.9697\nEpoch 125/180\nF1 Macro Score: 0.94091\n - 8s - loss: 0.0809 - acc: 0.9700 - val_loss: 0.0797 - val_acc: 0.9697\nEpoch 126/180\nF1 Macro Score: 0.94103\n - 8s - loss: 0.0807 - acc: 0.9700 - val_loss: 0.0796 - val_acc: 0.9697\nEpoch 127/180\nF1 Macro Score: 0.94097\n - 8s - loss: 0.0807 - acc: 0.9700 - val_loss: 0.0796 - val_acc: 0.9697\nEpoch 128/180\nF1 Macro Score: 0.94102\n - 8s - loss: 0.0804 - acc: 0.9700 - val_loss: 0.0797 - val_acc: 0.9697\nEpoch 129/180\nF1 Macro Score: 0.94082\n - 8s - loss: 0.0809 - acc: 0.9700 - val_loss: 0.0797 - val_acc: 0.9696\nEpoch 130/180\nF1 Macro Score: 0.94092\n - 8s - loss: 0.0806 - acc: 0.9699 - val_loss: 0.0796 - val_acc: 0.9697\nEpoch 131/180\nF1 Macro Score: 0.94093\n - 8s - loss: 0.0806 - acc: 0.9700 - val_loss: 0.0797 - val_acc: 0.9697\nEpoch 132/180\nF1 Macro Score: 0.94091\n - 8s - loss: 0.0805 - acc: 0.9701 - val_loss: 0.0795 - val_acc: 0.9697\nEpoch 133/180\nF1 Macro Score: 0.94095\n - 8s - loss: 0.0805 - acc: 0.9700 - val_loss: 0.0796 - val_acc: 0.9697\nEpoch 134/180\nF1 Macro Score: 0.94094\n - 8s - loss: 0.0805 - acc: 0.9701 - val_loss: 0.0796 - val_acc: 0.9697\nEpoch 135/180\nF1 Macro Score: 0.94094\n - 8s - loss: 0.0809 - acc: 0.9700 - val_loss: 0.0796 - val_acc: 0.9697\nEpoch 136/180\nF1 Macro Score: 0.94107\n - 7s - loss: 0.0804 - acc: 0.9701 - val_loss: 0.0795 - val_acc: 0.9697\nEpoch 137/180\nF1 Macro Score: 0.94094\n - 8s - loss: 0.0808 - acc: 0.9700 - val_loss: 0.0796 - val_acc: 0.9697\nEpoch 138/180\nF1 Macro Score: 0.94100\n - 8s - loss: 0.0802 - acc: 0.9701 - val_loss: 0.0796 - val_acc: 0.9697\nEpoch 139/180\nF1 Macro Score: 0.94086\n - 8s - loss: 0.0808 - acc: 0.9700 - val_loss: 0.0796 - val_acc: 0.9697\nEpoch 140/180\nF1 Macro Score: 0.94098\n - 8s - loss: 0.0804 - acc: 0.9700 - val_loss: 0.0795 - val_acc: 0.9697\nEpoch 141/180\nF1 Macro Score: 0.94100\n - 8s - loss: 0.0807 - acc: 0.9699 - val_loss: 0.0795 - val_acc: 0.9697\nEpoch 142/180\nF1 Macro Score: 0.94094\n - 8s - loss: 0.0805 - acc: 0.9700 - val_loss: 0.0796 - val_acc: 0.9697\nEpoch 143/180\nF1 Macro Score: 0.94094\n - 8s - loss: 0.0805 - acc: 0.9700 - val_loss: 0.0796 - val_acc: 0.9697\nEpoch 144/180\nF1 Macro Score: 0.94086\n - 8s - loss: 0.0803 - acc: 0.9700 - val_loss: 0.0796 - val_acc: 0.9696\nEpoch 145/180\nF1 Macro Score: 0.94091\n - 8s - loss: 0.0805 - acc: 0.9700 - val_loss: 0.0796 - val_acc: 0.9696\nEpoch 146/180\nF1 Macro Score: 0.94099\n - 8s - loss: 0.0803 - acc: 0.9700 - val_loss: 0.0796 - val_acc: 0.9697\nEpoch 147/180\nF1 Macro Score: 0.94091\n - 8s - loss: 0.0804 - acc: 0.9701 - val_loss: 0.0795 - val_acc: 0.9696\nEpoch 148/180\nF1 Macro Score: 0.94089\n - 7s - loss: 0.0807 - acc: 0.9700 - val_loss: 0.0795 - val_acc: 0.9697\nEpoch 149/180\nF1 Macro Score: 0.94094\n - 8s - loss: 0.0801 - acc: 0.9701 - val_loss: 0.0796 - val_acc: 0.9697\nEpoch 150/180\nF1 Macro Score: 0.94107\n - 8s - loss: 0.0806 - acc: 0.9700 - val_loss: 0.0794 - val_acc: 0.9697\nEpoch 151/180\nF1 Macro Score: 0.94091\n - 8s - loss: 0.0806 - acc: 0.9700 - val_loss: 0.0795 - val_acc: 0.9696\nEpoch 152/180\nF1 Macro Score: 0.94095\n - 8s - loss: 0.0806 - acc: 0.9700 - val_loss: 0.0795 - val_acc: 0.9697\nEpoch 153/180\nF1 Macro Score: 0.94093\n - 7s - loss: 0.0806 - acc: 0.9700 - val_loss: 0.0795 - val_acc: 0.9697\nEpoch 154/180\nF1 Macro Score: 0.94099\n - 8s - loss: 0.0801 - acc: 0.9701 - val_loss: 0.0796 - val_acc: 0.9697\nEpoch 155/180\nF1 Macro Score: 0.94103\n - 9s - loss: 0.0805 - acc: 0.9700 - val_loss: 0.0795 - val_acc: 0.9697\nEpoch 156/180\nF1 Macro Score: 0.94099\n - 8s - loss: 0.0805 - acc: 0.9700 - val_loss: 0.0795 - val_acc: 0.9697\nEpoch 157/180\nF1 Macro Score: 0.94098\n - 8s - loss: 0.0801 - acc: 0.9702 - val_loss: 0.0795 - val_acc: 0.9697\nEpoch 158/180\nF1 Macro Score: 0.94094\n - 8s - loss: 0.0801 - acc: 0.9701 - val_loss: 0.0796 - val_acc: 0.9697\nEpoch 159/180\nF1 Macro Score: 0.94101\n - 8s - loss: 0.0799 - acc: 0.9702 - val_loss: 0.0794 - val_acc: 0.9697\nEpoch 160/180\nF1 Macro Score: 0.94101\n - 8s - loss: 0.0802 - acc: 0.9702 - val_loss: 0.0794 - val_acc: 0.9697\nEpoch 161/180\nF1 Macro Score: 0.94106\n - 8s - loss: 0.0805 - acc: 0.9700 - val_loss: 0.0795 - val_acc: 0.9697\nEpoch 162/180\nF1 Macro Score: 0.94100\n - 8s - loss: 0.0805 - acc: 0.9701 - val_loss: 0.0795 - val_acc: 0.9697\nEpoch 163/180\nF1 Macro Score: 0.94101\n - 8s - loss: 0.0800 - acc: 0.9701 - val_loss: 0.0795 - val_acc: 0.9697\nEpoch 164/180\nF1 Macro Score: 0.94100\n - 9s - loss: 0.0802 - acc: 0.9701 - val_loss: 0.0795 - val_acc: 0.9697\nEpoch 165/180\nF1 Macro Score: 0.94097\n - 9s - loss: 0.0800 - acc: 0.9702 - val_loss: 0.0796 - val_acc: 0.9697\nEpoch 166/180\nF1 Macro Score: 0.94102\n - 8s - loss: 0.0803 - acc: 0.9700 - val_loss: 0.0795 - val_acc: 0.9697\nEpoch 167/180\nF1 Macro Score: 0.94098\n - 8s - loss: 0.0802 - acc: 0.9701 - val_loss: 0.0796 - val_acc: 0.9697\nEpoch 168/180\nF1 Macro Score: 0.94100\n - 8s - loss: 0.0798 - acc: 0.9702 - val_loss: 0.0795 - val_acc: 0.9697\nEpoch 169/180\nF1 Macro Score: 0.94099\n - 8s - loss: 0.0801 - acc: 0.9702 - val_loss: 0.0794 - val_acc: 0.9697\nEpoch 170/180\nF1 Macro Score: 0.94103\n - 8s - loss: 0.0800 - acc: 0.9701 - val_loss: 0.0795 - val_acc: 0.9697\nEpoch 171/180\nF1 Macro Score: 0.94086\n - 8s - loss: 0.0813 - acc: 0.9699 - val_loss: 0.0796 - val_acc: 0.9696\nEpoch 172/180\nF1 Macro Score: 0.94096\n - 8s - loss: 0.0803 - acc: 0.9701 - val_loss: 0.0795 - val_acc: 0.9696\nEpoch 173/180\nF1 Macro Score: 0.94101\n - 8s - loss: 0.0810 - acc: 0.9700 - val_loss: 0.0796 - val_acc: 0.9697\nEpoch 174/180\nF1 Macro Score: 0.94096\n - 8s - loss: 0.0803 - acc: 0.9701 - val_loss: 0.0795 - val_acc: 0.9697\nEpoch 175/180\nF1 Macro Score: 0.94097\n - 8s - loss: 0.0799 - acc: 0.9702 - val_loss: 0.0796 - val_acc: 0.9697\nEpoch 176/180\nF1 Macro Score: 0.94099\n - 8s - loss: 0.0802 - acc: 0.9702 - val_loss: 0.0795 - val_acc: 0.9697\nEpoch 177/180\nF1 Macro Score: 0.94098\n - 8s - loss: 0.0801 - acc: 0.9702 - val_loss: 0.0795 - val_acc: 0.9697\nEpoch 178/180\nF1 Macro Score: 0.94092\n - 8s - loss: 0.0801 - acc: 0.9701 - val_loss: 0.0795 - val_acc: 0.9697\nEpoch 179/180\nF1 Macro Score: 0.94101\n - 8s - loss: 0.0801 - acc: 0.9702 - val_loss: 0.0795 - val_acc: 0.9697\nEpoch 180/180\nF1 Macro Score: 0.94095\n - 8s - loss: 0.0799 - acc: 0.9701 - val_loss: 0.0794 - val_acc: 0.9697\nTraining fold 5 completed. macro f1 score : 0.94095\nTraining completed. oof macro f1 score : 0.94030\nsave path: ./../data/output/submission_nb035_cv_0.9403.csv\nTraining completed...\nCPU times: user 1h 41min 24s, sys: 11min 25s, total: 1h 52min 49s\nWall time: 1h 57min 57s\n"
]
],
[
[
"# analysis",
"_____no_output_____"
]
],
[
[
"df_tr = pd.read_csv(PATH_TRAIN)",
"_____no_output_____"
],
[
"batch_list = []\nfor n in range(10):\n batchs = np.ones(500000)*n\n batch_list.append(batchs.astype(int))\nbatch_list = np.hstack(batch_list)\ndf_tr['batch'] = batch_list",
"_____no_output_____"
],
[
"# group 特徴量を作成\ngroup = group_feat_train(df_tr)\ndf_tr = pd.concat([df_tr, group], axis=1)",
"_____no_output_____"
],
[
"y = df_tr['open_channels'].values",
"_____no_output_____"
],
[
"oof = np.argmax(oof_, axis=1).astype(int)",
"_____no_output_____"
],
[
"for group in sorted(df_tr['group'].unique()):\n idxs = df_tr['group'] == group\n oof_grp = oof[idxs].astype(int)\n y_grp = y[idxs]\n print(f'group_score({group}): {f1_macro(y_grp, oof_grp):4f}')",
"group_score(0): 0.332464\ngroup_score(1): 0.779841\ngroup_score(2): 0.973168\ngroup_score(3): 0.997029\ngroup_score(4): 0.847571\n"
]
],
[
[
"<br>\n\n可視化",
"_____no_output_____"
]
],
[
[
"x_idx = np.arange(len(df_tr))\nidxs = y != oof\n\nfailed = np.zeros(len(df_tr))\nfailed[idxs] = 1",
"_____no_output_____"
],
[
"n = 200\nb = np.ones(n)/n\nfailed_move = np.convolve(failed, b, mode='same')",
"_____no_output_____"
],
[
"fig, axs = plt.subplots(2, 1, figsize=(20, 6))\naxs = axs.ravel()\n# fig = plt.figure(figsize=(20, 3))\n\nfor i_gr, group in enumerate(sorted(df_tr['group'].unique())):\n idxs = df_tr['group'] == group\n axs[0].plot(np.arange(len(df_tr))[idxs], df_tr['signal'].values[idxs], color=cp[i_gr], label=f'group={group}')\nfor x in range(10): \n axs[0].axvline(x*500000 + 500000, color='gray') \n axs[0].text(x*500000 + 250000, 0.6, x)\naxs[0].plot(x_idx, failed_move, '.', color='black', label='failed_mv')\naxs[0].set_xlim(0, 5500000)\naxs[0].legend()\n\naxs[1].plot(x_idx, y)\naxs[1].set_xlim(0, 5500000)\n\n# fig.legend()",
"_____no_output_____"
],
[
"\n\n\n\n\n\n\n\n\n\n\n\n\n\n\n\n\n\n\n\n\n\n\n\n\n\n",
"_____no_output_____"
]
]
] | [
"markdown",
"code",
"markdown",
"code",
"markdown",
"code",
"markdown",
"code",
"markdown",
"code",
"markdown",
"code",
"markdown",
"raw",
"markdown",
"raw",
"markdown",
"raw",
"markdown",
"code",
"markdown",
"code",
"markdown",
"code"
] | [
[
"markdown",
"markdown"
],
[
"code"
],
[
"markdown"
],
[
"code"
],
[
"markdown"
],
[
"code",
"code",
"code"
],
[
"markdown"
],
[
"code"
],
[
"markdown"
],
[
"code"
],
[
"markdown",
"markdown"
],
[
"code"
],
[
"markdown"
],
[
"raw"
],
[
"markdown"
],
[
"raw"
],
[
"markdown"
],
[
"raw"
],
[
"markdown"
],
[
"code",
"code",
"code"
],
[
"markdown"
],
[
"code",
"code",
"code",
"code",
"code",
"code"
],
[
"markdown"
],
[
"code",
"code",
"code",
"code"
]
] |
d090d731b78245353496f2b3326d795cae957c8d | 6,581 | ipynb | Jupyter Notebook | Pynq-ZU/base/notebooks/board/asyncio_buttons.ipynb | Xilinx/PYNQ-ZU | 48d143b1973aff08dd22a271a7564eb295e4cef5 | [
"BSD-3-Clause"
] | 6 | 2021-03-02T20:47:07.000Z | 2022-03-20T01:11:11.000Z | Pynq-ZU/base/notebooks/board/asyncio_buttons.ipynb | Xilinx/PYNQ-ZU | 48d143b1973aff08dd22a271a7564eb295e4cef5 | [
"BSD-3-Clause"
] | 3 | 2021-04-30T13:04:18.000Z | 2021-10-07T07:31:53.000Z | Pynq-ZU/base/notebooks/board/asyncio_buttons.ipynb | Xilinx/PYNQ-ZU | 48d143b1973aff08dd22a271a7564eb295e4cef5 | [
"BSD-3-Clause"
] | 8 | 2021-04-24T12:05:17.000Z | 2022-03-18T09:05:53.000Z | 30.188073 | 424 | 0.593223 | [
[
[
"# Using Interrupts and asyncio for Buttons and Switches\n\nThis notebook provides a simple example for using asyncio I/O to interact asynchronously with multiple input devices. A task is created for each input device and coroutines used to process the results. To demonstrate, we recreate the flashing LEDs example in the getting started notebook but using interrupts to avoid polling the GPIO devices. The aim is have holding a button result in the corresponding LED flashing.",
"_____no_output_____"
],
[
"## Initialising the Environment\nFirst we import an instantiate all required classes to interact with the buttons, switches and LED and ensure the base overlay is loaded.",
"_____no_output_____"
]
],
[
[
"from pynq import PL\nfrom pynq.overlays.base import BaseOverlay\n\nbase = BaseOverlay(\"base.bit\")",
"_____no_output_____"
]
],
[
[
"## Define the flash LED task\nNext step is to create a task that waits for the button to be pressed and flash the LED until the button is released. The `while True` loop ensures that the coroutine keeps running until cancelled so that multiple presses of the same button can be handled.\n",
"_____no_output_____"
]
],
[
[
"import asyncio\n\nasync def flash_led(num):\n while True:\n await base.buttons[num].wait_for_value_async(1)\n while base.buttons[num].read():\n base.leds[num].toggle()\n await asyncio.sleep(0.1)\n base.leds[num].off()",
"_____no_output_____"
]
],
[
[
"## Create the task\nAs there are four buttons we want to check, we create four tasks. The function `asyncio.ensure_future` is used to convert the coroutine to a task and schedule it in the event loop. The tasks are stored in an array so they can be referred to later when we want to cancel them.",
"_____no_output_____"
]
],
[
[
"tasks = [asyncio.ensure_future(flash_led(i)) for i in range(4)]",
"_____no_output_____"
]
],
[
[
"## Monitoring the CPU Usage\n\nOne of the advantages of interrupt-based I/O is to minimised CPU usage while waiting for events. To see how CPU usages is impacted by the flashing LED tasks we create another task that prints out the current CPU utilisation every 3 seconds.",
"_____no_output_____"
]
],
[
[
"import psutil\n\nasync def print_cpu_usage():\n # Calculate the CPU utilisation by the amount of idle time\n # each CPU has had in three second intervals\n last_idle = [c.idle for c in psutil.cpu_times(percpu=True)]\n while True:\n await asyncio.sleep(3)\n next_idle = [c.idle for c in psutil.cpu_times(percpu=True)]\n usage = [(1-(c2-c1)/3) * 100 for c1,c2 in zip(last_idle, next_idle)]\n print(\"CPU Usage: {0:3.2f}%, {1:3.2f}%\".format(*usage))\n last_idle = next_idle\n\ntasks.append(asyncio.ensure_future(print_cpu_usage()))",
"_____no_output_____"
]
],
[
[
"## Run the event loop\nAll of the blocking wait_for commands will run the event loop until the condition is met. All that is needed is to call the blocking `wait_for_level` method on the switch we are using as the termination condition. \n\nWhile waiting for switch 0 to get high, users can press any push button on the board to flash the corresponding LED. While this loop is running, try opening a terminal and running `top` to see that python is consuming no CPU cycles while waiting for peripherals. \n\nAs this code runs until the switch 0 is high, make sure it is low before running the example.",
"_____no_output_____"
]
],
[
[
"if base.switches[0].read():\n print(\"Please set switch 0 low before running\")\nelse:\n base.switches[0].wait_for_value(1)",
"CPU Usage: 0.67%, 11.67%\nCPU Usage: 0.00%, 0.33%\nCPU Usage: 0.00%, 0.33%\nCPU Usage: 0.00%, 0.33%\nCPU Usage: 0.00%, 0.33%\nCPU Usage: 0.00%, 0.33%\n"
]
],
[
[
"## Clean up\nEven though the event loop has stopped running, the tasks are still active and will run again when the event loop is next used. To avoid this, the tasks should be cancelled when they are no longer needed.",
"_____no_output_____"
]
],
[
[
"[t.cancel() for t in tasks]",
"_____no_output_____"
]
],
[
[
"Now if we re-run the event loop, nothing will happen when we press the buttons. The process will block until the switch is set back down to the low position.",
"_____no_output_____"
]
],
[
[
"base.switches[0].wait_for_value(0)",
"_____no_output_____"
]
],
[
[
"Copyright (C) 2020 Xilinx, Inc",
"_____no_output_____"
]
]
] | [
"markdown",
"code",
"markdown",
"code",
"markdown",
"code",
"markdown",
"code",
"markdown",
"code",
"markdown",
"code",
"markdown",
"code",
"markdown"
] | [
[
"markdown",
"markdown"
],
[
"code"
],
[
"markdown"
],
[
"code"
],
[
"markdown"
],
[
"code"
],
[
"markdown"
],
[
"code"
],
[
"markdown"
],
[
"code"
],
[
"markdown"
],
[
"code"
],
[
"markdown"
],
[
"code"
],
[
"markdown"
]
] |
d090dccf5bb2ca4d9a4cc5ca9f47b5464cae4bed | 339,404 | ipynb | Jupyter Notebook | fit_mechanism.ipynb | truejulosdu13/Electrochemistry | 183914d75f7d8ec8fdaa03a1f5133f24afaf6f38 | [
"MIT"
] | null | null | null | fit_mechanism.ipynb | truejulosdu13/Electrochemistry | 183914d75f7d8ec8fdaa03a1f5133f24afaf6f38 | [
"MIT"
] | null | null | null | fit_mechanism.ipynb | truejulosdu13/Electrochemistry | 183914d75f7d8ec8fdaa03a1f5133f24afaf6f38 | [
"MIT"
] | null | null | null | 35.03706 | 38,100 | 0.634884 | [
[
[
"import os\nimport pandas as pd\nimport matplotlib.pyplot as plt\nimport sys\nsys.path.append('../')\nfrom default_constants import *\nfrom ECE_mechanism.voltammogram_ECE_no_plot import CSV_ECE_ox\nfrom plot_tools import extract_expe_like_CSV\nfrom scipy.optimize import minimize\n\ndef plot_experimental_data(folder_name):\n directory = folder_name\n for filename in os.listdir(directory):\n if filename.endswith(\".txt\"): \n path = os.path.join(directory, filename)\n df = pd.read_csv(path, delimiter = ';', decimal = ',')\n Potential = df['Potential applied (V)'].to_numpy()\n Intensity = df['WE(1).Current (A)'].to_numpy()\n plt.plot(Potential, Intensity, label = path)\n continue\n else:\n continue\n plt.legend(bbox_to_anchor=(1.05, 1), loc='upper left', borderaxespad=0.)\n \n \ndef find_expe_csts_CSV(file_name):\n df = pd.read_csv(file_name, delimiter = ';', decimal = ',')\n Potential = df['Potential applied (V)'].to_numpy()\n Time = df['Time (s)'].to_numpy()\n E_ox = max(Potential)\n E_red = min(Potential)\n E_start = Potential[0]\n Delta_E = Potential[1] - Potential[0]\n v = Delta_E/(Time[1] - Time[0])\n return(E_ox, E_red, Delta_E, v, E_start)\n\ndef find_I_E_t(file_name):\n df = pd.read_csv(file_name, delimiter = ';', decimal = ',')\n Pot_ap = df['Potential applied (V)'].to_numpy()\n I_expe = df['WE(1).Current (A)'].to_numpy()\n Time = df['Time (s)'].to_numpy()\n return(I_expe, Pot_ap, Time)\n\ndef set_expe_cst(cst_all, cst_expe_new):\n cst_all_new = list(cst_all)\n cst_expe_new = list(cst_expe_new)\n cst_all_new[4] = list(cst_all_new[4])\n for i in range(len(cst_all_new[4])):\n cst_all_new[4][i] = cst_expe_new[i]\n cst_all_new[4] = tuple(cst_all_new[4])\n cst_all_new = tuple(cst_all_new)\n return(cst_all_new) \n\ndef guess_potentials(E_0_1, E_0_2, cst_all):\n cst_all_new = list(cst_all)\n cst_all_new[2] = list(cst_all_new[2])\n cst_all_new[2][0] = E_0_1\n cst_all_new[2][9] = E_0_2\n cst_all_new[2] = tuple(cst_all_new[2])\n cst_all_new = tuple(cst_all_new)\n return(cst_all_new) \n \ndef I_new(new_guess, *cst_expe_set):\n cst_expe_guess = cst_expe_set\n cst_expe_guess = list(cst_expe_guess)\n cst_expe_guess[2] = list(cst_expe_guess[2])\n cst_expe_guess[2][0] = new_guess[0]\n cst_expe_guess[2][9] = new_guess[1]\n cst_expe_guess[2][6] = new_guess[2]\n cst_expe_guess[2][7] = new_guess[3]\n cst_expe_guess[2][4] = new_guess[4]\n cst_expe_guess[2] = tuple(cst_expe_guess[2])\n cst_expe_guess = tuple(cst_expe_guess)\n I_simu_CSV = CSV_ECE_ox(cst_expe_guess)\n E_expe, I_simu_CSV_2 = extract_expe_like_CSV(cst_expe_guess, I_simu_CSV)\n return(I_simu_CSV_2)\n\ndef Error_I(I_new, *I_expe_CSV):\n Error = 0\n I_new = I_new/max(I_new)\n I_expe_CSV = I_expe_CSV/max(I_expe_CSV)\n for i in range(len(I_new)):\n Delta_I = I_new[i] - I_expe_CSV[i]\n Error += Delta_I**2\n print(Error)\n return(Error)\n\ndef fun_1(new_guess, *fixed_parms):\n cst_expe_set = fixed_parms[0]\n I_expe = fixed_parms[1]\n I = I_new(new_guess, *cst_expe_set)\n Err = Error_I(I, *I_expe)\n return(Err)\n \n\n # fitting with the error\ndef fit_experimental_data(file_name, guess):\n path = os.path.join('Examples_ECE', file_name)\n # adjust set up parameters\n cst_expe = find_experimental_potentials_CSV(path)\n cst_default = set_default_constants()\n cst_expe_set = set_expe_cst(cst_default, cst_expe)\n \n # define the function to minimize :\n cst_syst_guess = guess_potentials(guess[0], guess[1] , cst_expe_set)\n \n df = pd.read_csv(path, delimiter = ';', decimal = ',')\n I_expe_CSV = df['WE(1).Current (A)'].to_numpy()\n I_expe_CSV = I_expe_CSV/max(I_expe_CSV)\n E_expe_CSV = df['Potential applied (V)'].to_numpy()\n\n Best = minimize(fun_1, guess, \n args = (cst_expe_set, I_expe_CSV), \n method='SLSQP', \n bounds= ((0.4,0.8),(0.3,0.8), (0.001,10), (0.001,20), (0.00001,1))\n ) \n #print(cst_expe_set[2]) \n return(Best)\n\n # curve fitting with the error\ndef fit_experimental_data_2(file_name, guess):\n path = os.path.join('Examples_ECE', file_name)\n # adjust set up parameters\n cst_expe = find_experimental_potentials_CSV(path)\n cst_default = set_default_constants()\n cst_expe_set = set_expe_cst(cst_default, cst_expe)\n \n # define the function to minimize :\n cst_syst_guess = guess_potentials(guess[0], guess[1] , cst_expe_set)\n \n df = pd.read_csv(path, delimiter = ';', decimal = ',')\n I_expe_CSV = df['WE(1).Current (A)'].to_numpy()\n I_expe_CSV = I_expe_CSV/max(I_expe_CSV)\n E_expe_CSV = df['Potential applied (V)'].to_numpy()\n\n Best = minimize(fun_1, (0.6, 0.5, 1, 1, 0.01), args = (cst_expe_set, I_expe_CSV), method='SLSQP') \n #print(cst_expe_set[2]) \n return(Best)",
"_____no_output_____"
],
[
"path = os.path.join('Fit_Experimental_CSV', 'Examples_ECE')\nprint(path)",
"Fit_Experimental_CSV/Examples_ECE\n"
],
[
"plot_experimental_data(path)",
"_____no_output_____"
],
[
"path_file = os.path.join(path, 'Che207 Ni(BinapSQ) 500mV s.txt')\n",
"_____no_output_____"
],
[
"def fit_expe(guess, path_file, cst_all):\n # set new values for cst_all extracted from the experimental data\n (E_ox, E_red, Delta_E, v, E_i) = find_expe_csts_CSV(path_file)\n cst_all[\"E_ox\"] = E_ox\n cst_all[\"E_red\"] = E_red\n cst_all[\"Delta_E\"] = Delta_E\n if Delta_E > 0 :\n cst_all[\"Ox\"] = True\n else:\n cst_all[\"Ox\"] = False\n cst_all[\"v\"] = v\n cst_all[\"E_i\"] = E_i\n \n # extract I_expe from the datas\n (I_expe, Pot_ap, Time) = find_I_E_t(path_file)\n print(len(I_expe))\n \n # minimize the distance between I_expe and I_simulates\n Best = minimize(fun_ECE, guess, \n args = (cst_all, I_expe), \n method='L-BFGS-B', \n bounds= ((0.01,10000), (0.4,0.8), (0.3,0.8), (0.001,20), (0.00001,1)),\n tol = 0.1) \n return(Best)\n\n# guess = (Lambda, E_0_1, E_0_2, k_p, k_m)\ndef fun_ECE(guess, *fixed_parms):\n # set the new constants for cst_all\n cst_new = fixed_parms[0]\n cst_new[\"Lambda\"] = guess[0]\n cst_new[\"E_0_1\"] = guess[1]\n cst_new[\"E_0_2\"] = guess[2]\n cst_new[\"k_p\"] = guess[3]\n cst_new[\"k_m\"] = guess[4]\n \n # calculate the I correspomnding to theses new constants\n (param, E, C_init, M_new_constant, M_old, fun, fun_I) = initialise(cst_new)\n (I, Potential, Time) = calculate_I(param, E, C_init, M_new_constant, M_old, fun, fun_I)\n (Pot_expe, I) = extract_expe_like_CSV(param, I)\n \n # calculate the error between experimental and simulated intensity\n Err = Error_I(I, *fixed_parms[1])\n return(Err)\n\n\ndef Error_I(I_new, *I_expe_CSV):\n Error = 0\n I_new = I_new/max(I_new)\n I_expe_CSV = I_expe_CSV/max(I_expe_CSV)\n for i in range(len(I_new)):\n Delta_I = I_new[i] - I_expe_CSV[i]\n Error += Delta_I**2\n print(Error)\n return(Error)",
"_____no_output_____"
],
[
"from default_constants import default_constants\nfrom main import *\n\n#main programm to fit the data :\ncst_all = default_constants()\n# set mechanism type :\ncst_all[\"mechanism\"] = 'ECE'\n# set molecule type :\ncst_all[\"Reducible\"] = False\n# set concentration :\ncst_all[\"C_a\"] = 2E-3\n\npath = os.path.join('Fit_Experimental_CSV', 'Examples_ECE')\npath_file = os.path.join(path, 'Che207 Ni(BinapSQ) 500mV s.txt')\n#guess = (100, 0.5, 0.5, 1.0, 1.0)\nguess = (100, 5.55824144e-01, 4.41382398e-01, 9.71843960e-01, 1.00000000e+01)\n\nBest = fit_expe(guess, path_file, cst_all)\n",
"102\n358.5091789577226\n358.50917895769487\n358.50917774184467\n358.5091773656504\n358.5091809781585\n358.5091785419629\n153.68929788468515\n153.68929788467247\n153.68929983418195\n153.6892978867959\n153.68929878627947\n153.68929788444606\n191.48983601286008\n191.4898360128539\n191.48981789550672\n191.48983601301262\n191.48983832987196\n191.48983601261023\n162.60547901444951\n162.6054790144408\n162.60547169557205\n162.60547902607934\n162.6054809860109\n162.60547901416444\n159.42762601814061\n159.4276260181246\n159.42763436431636\n159.42762603736418\n159.42762741088927\n159.42762601788306\n153.54549623069224\n153.54549623068115\n153.54549562591802\n153.54549623274636\n153.5454971239461\n153.54549623045176\n"
],
[
"Best",
"_____no_output_____"
],
[
"#main programm to fit the data :\ncst_all = default_constants()\n# set mechanism type :\ncst_all[\"mechanism\"] = 'ECE'\n# set molecule type :\ncst_all[\"Reducible\"] = False\n# set concentration :\ncst_all[\"C_a\"] = 2E-3\n\n# set new values for cst_all extracted from the experimental data\n(E_ox, E_red, Delta_E, v, E_i) = find_expe_csts_CSV(path_file)\ncst_all[\"E_ox\"] = E_ox\ncst_all[\"E_red\"] = E_red\ncst_all[\"Delta_E\"] = Delta_E\nif Delta_E > 0 :\n cst_all[\"Ox\"] = True\nelse:\n cst_all[\"Ox\"] = False\ncst_all[\"v\"] = v\ncst_all[\"E_i\"] = E_i\n\n\n # extract I_expe from the datas\n(I_expe, Pot_ap, Time) = find_I_E_t(path_file)\n\nguess = np.array([2, 0.5, 0.5, 1.0, 1.0])\n\ndef fun_ECE_cma(guess):\n # set the new constants for cst_all\n (param, E, C_init, M_new_constant, M_old, fun, fun_I) = initialise(cst_all)\n cst_new = param\n cst_new[\"Lambda\"] = 10**(guess[0])\n cst_new[\"E_0_1\"] = guess[1]\n cst_new[\"E_0_2\"] = guess[2]\n cst_new[\"k_p\"] = 10**(guess[3])\n cst_new[\"k_m\"] = 10**(guess[4])\n \n # calculate the I correspomnding to theses new constants\n (param, E, C_init, M_new_constant, M_old, fun, fun_I) = initialise(cst_new)\n (I_simu, Potential, Time) = calculate_I(param, E, C_init, M_new_constant, M_old, fun, fun_I)\n (Pot_expe, I_new) = extract_expe_like_CSV(param, I_simu)\n\n # calculate the error between experimental and simulated intensity\n Err = Error_I(I_new, I_expe)\n return(Err)\n\ndef Error_I(I_new, I_expe_CSV):\n #print(len(I_new), len(I_expe_CSV))\n Error = 0\n I_new = I_new/max(I_new)\n I_expe_CSV = I_expe_CSV/max(I_expe_CSV)\n for i in range(len(I_new)):\n Delta_I = I_new[i] - I_expe_CSV[i]\n Error += Delta_I**2\n print(Error)\n return(Error)\n\nimport cma\nfrom cma.evolution_strategy import CMAEvolutionStrategy\nopts = cma.CMAOptions()\nopts.set({'popsize': 200, \n 'maxiter' : 100000, \n 'maxfevals' : 10000, \n 'timeout' : \"100000000.0 * 60**2\", \n 'tolfun': 1e-4, \n 'tolfunhist': 1e-6})\nes = CMAEvolutionStrategy(guess.astype(np.float16), 1, opts)\nes.optimize(fun_ECE_cma, verb_disp=100, n_jobs = -1)",
"(100_w,200)-aCMA-ES (mu_w=52.6,w_1=4%) in dimension 5 (seed=876039, Fri Feb 19 16:41:44 2021)\n4796.361859818732\n24360935170.371628\n352.7454063497824\n961.0764970660622\n326.29933128824285\n1479.3072158297775\n2456.143403591222\n1250.921707635416\n405.8300885849281\n1495.9705300630742\n2450.951769150903\n194.58377705908427\n161.74427613454512\n3022.6096766045816\n465.66153421544493\n68.97669538316063\n872.8791728297593\n198.57084169937792\n45.292697025753746\n887.0298544496445\n268.9348263911179\n759.2319117631578\n706.7018150467719\n213.69069085983602\n700.295581060567\n851.067754105907\n2221.3080232733487\n810.1593439682553\n915.6780622662471\n8321.224891188376\n51.90217108939182\n15954.440443725272\n120.76876536800366\n669397.744434104\n2340.789170777824\n2457.344543902382\n1104.894605779501\n59.10075806344325\n120.30092657433794\n1958440.1513886682\n97826.3776667019\n16129.24017363191\n774.7611712204694\n1200.574591746936\n12147.206320050975\n52.05781369242957\n2917.1954177488115\n1565.3472590094282\n10611.29284254178\n3605.794392969827\n21446560.950085424\n2456.1433768608526\n2456.1433935465543\n408.3516652119259\n424.97164071494586\n5657361537.597966\n1.5931413685101657e+23\n283.31484693995543\n2284.79026115507\n917.5148874170715\n269.38345211751295\n2456.1439649364365\n199.53347463840643\n715.2364594257017\n669.1066478416982\n30.463945433993473\n4.920329600064196e+18\n386.85695930442694\n36.24297291304655\n57904.59787105934\n2451.1336473710994\n189.78890291723008\n231990.44553357598\n2463.1323219594865\n42.104260523201624\n288.685258724768\n949.0098133457691\n2456.143391696386\n406.2506455146112\n2456.4455369888506\n56.462037940078616\n7.199431762898348e+19\n661.7390794851232\n1683.6853286867854\n520.9310608995015\n858.9155141695603\n230.72825832909732\n537.6236769379271\n2456.143534698446\n52.31032577619592\n1.6576343499443002e+20\n913.3578503119269\n858.5559291082869\n93.35383611024886\n234252.99708859352\n2.4918090368270983e+22\n517.0180851945348\n305.2311319830026\n161.73935596673627\n"
],
[
"path_file",
"_____no_output_____"
],
[
"es.result",
"_____no_output_____"
],
[
"96500/(8.314*300)",
"_____no_output_____"
],
[
"1/38.7",
"_____no_output_____"
]
]
] | [
"code"
] | [
[
"code",
"code",
"code",
"code",
"code",
"code",
"code",
"code",
"code",
"code",
"code",
"code"
]
] |
d090e5f20e1dc6a49b52fa3b77595a97e79ded8d | 110,297 | ipynb | Jupyter Notebook | making_simple_plots.ipynb | PurdueMechanicalEngineering/me270 | 3e164e22bc678b90456757ff6348a97206b3a4ae | [
"MIT"
] | 1 | 2018-09-02T15:07:25.000Z | 2018-09-02T15:07:25.000Z | making_simple_plots.ipynb | PurdueMechanicalEngineering/me270 | 3e164e22bc678b90456757ff6348a97206b3a4ae | [
"MIT"
] | null | null | null | making_simple_plots.ipynb | PurdueMechanicalEngineering/me270 | 3e164e22bc678b90456757ff6348a97206b3a4ae | [
"MIT"
] | null | null | null | 375.159864 | 23,148 | 0.928439 | [
[
[
"# Making Simple Plots\n\n## Objectives\n+ Learn how to make a simple 1D plot in Python.\n+ Learn how to find the maximum/minimum of a function in Python.\n\nWe will use [Problem 4.B.2](https://youtu.be/w-IGNU2i3F8) of the lecturebook as a motivating example.\nWe find that the moment of the force $\\vec{F}$ about point A is:\n$$\n\\vec{M_A} = (bF\\cos\\theta - dF\\sin\\theta)\\hat{k}.\n$$\nLet's plot the component of the moment as a function of $\\theta$.\nFor this, we will use the Python module [matplotlib](https://matplotlib.org).",
"_____no_output_____"
]
],
[
[
"import numpy as np # for numerical algebra\nimport matplotlib.pyplot as plt # this is where the plotting capabilities are\n# The following line is need so that the plots are embedded in the Jupyter notebook (remove when not using Jupyter)\n%matplotlib inline \n\n# Define a function that computes the moment magnitude as a function of all other parameters\ndef M_A(theta, b, d, F):\n \"\"\"\n Compute the k component of the moment of F about point A given all the problem parameters.\n \"\"\"\n return b * F * np.cos(theta) - d * F * np.sin(theta)\n\n# Choose some parameters\nb = 0.5 # In meters\nd = 2. # In meters\nF = 2. # In kN\n# The thetas on which we will evaluate the moment for plotting\nthetas = np.linspace(0, 2 * np.pi, 100)\n# The moment on these thetas:\nM_As = M_A(thetas, b, d, F)\n# Let's plot\nplt.plot(thetas / (2. * np.pi) * 360, M_As, lw=2)\nplt.xlabel(r'$\\theta$ (degrees)')\nplt.ylabel('$M_A$ (kN)');",
"_____no_output_____"
]
],
[
[
"Now, let's put two lines in the same plot.\nLet's compare the moments when we change $d$ from 2 meters to 3.5 meters.",
"_____no_output_____"
]
],
[
[
"# We already have M_A for d=2 m (and all other paramters to whichever values we gave them)\n# Let's copy it:\nM_As_case_1 = M_As\n# And let's compute it again for d=3.5 m\nd = 3.5 # In m\nM_As_case_2 = M_A(thetas, b, d, F)\n# Let's plot both of them in the same figure\nplt.plot(thetas / (2. * np.pi) * 360, M_As_case_1, lw=2, label='Case 1')\nplt.plot(thetas / (2. * np.pi) * 360, M_As_case_2, '--', lw=2, label='Case 2')\nplt.xlabel(r'$\\theta$ (degrees)')\nplt.ylabel('$M_A$ (kN)')\nplt.legend(loc='best')",
"_____no_output_____"
]
],
[
[
"Finally, let's see how we can make interactive plots.\nWe will use the Python module [ipywidgets](https://ipywidgets.readthedocs.io/en/stable/) and in particular the function [ipywidgets.interact](https://ipywidgets.readthedocs.io/en/stable/examples/Using%20Interact.html).",
"_____no_output_____"
]
],
[
[
"from ipywidgets import interact # Loading the module\n# Interact needs a function that does the plotting given the parameters.\n# Let's make it:\ndef make_plots(b=0.5, d=3., F=1.): # X=val defines default values for the function\n \"\"\"\n Make the plot.\n \"\"\"\n thetas = np.linspace(0, 2. * np.pi, 100)\n M_As = M_A(thetas, b, d, F)\n plt.plot(thetas / (2. * np.pi) * 360, M_As, lw=2, label='Case 1')\n plt.ylim([-10., 10.])\n plt.xlabel(r'$\\theta$ (degrees)')\n plt.ylabel('$M_A$ (kN)')",
"_____no_output_____"
]
],
[
[
"Let's just check that the function works by calling it a few times:",
"_____no_output_____"
]
],
[
[
"# With no inputs it should use the default values\nmake_plots()",
"_____no_output_____"
],
[
"# You can specify all the inputs like this:\nmake_plots(2., 3., 2.)",
"_____no_output_____"
],
[
"# Or even by name (whatever is not specified gets the default value):\nmake_plots(F=2.3)",
"_____no_output_____"
]
],
[
[
"Ok. Let's use interact now:",
"_____no_output_____"
]
],
[
[
"interact(make_plots, \n b=(0., 5., 0.1), # Range for b: (min, max, increment)\n d=(0., 5, 0.1), # Range for d\n F=(0., 2, 0.1) # Range for F\n );",
"_____no_output_____"
]
]
] | [
"markdown",
"code",
"markdown",
"code",
"markdown",
"code",
"markdown",
"code",
"markdown",
"code"
] | [
[
"markdown"
],
[
"code"
],
[
"markdown"
],
[
"code"
],
[
"markdown"
],
[
"code"
],
[
"markdown"
],
[
"code",
"code",
"code"
],
[
"markdown"
],
[
"code"
]
] |
d090e88740c6958a4b78101c5c15df42cf815a4f | 2,855 | ipynb | Jupyter Notebook | tutorials/market/market_1_gists/market_1_03.ipynb | Monireh2/text-extensions-for-pandas | 736c42a34d5fb4b02b48bc63d6d5f6bc2990ce19 | [
"Apache-2.0"
] | 193 | 2020-05-11T21:15:57.000Z | 2022-03-23T09:59:32.000Z | tutorials/market/market_1_gists/market_1_03.ipynb | frreiss/tep-fred | b5b3c58f93b0c0753fd875ad9ed22e762bb8ace7 | [
"Apache-2.0"
] | 207 | 2020-05-07T17:38:13.000Z | 2022-02-11T18:02:13.000Z | tutorials/market/market_1_gists/market_1_03.ipynb | frreiss/tep-fred | b5b3c58f93b0c0753fd875ad9ed22e762bb8ace7 | [
"Apache-2.0"
] | 28 | 2020-05-07T16:43:52.000Z | 2022-02-25T15:21:18.000Z | 28.55 | 85 | 0.408406 | [
[
[
"dfs[\"semantic_roles\"][\n dfs[\"semantic_roles\"][\"action.normalized\"] == \"say\"]",
"_____no_output_____"
]
]
] | [
"code"
] | [
[
"code"
]
] |
d090ea291f4479ea34b53fcf47da7649d5cf84ac | 30,359 | ipynb | Jupyter Notebook | VacationPy/VacationPy.ipynb | jgmoore10/python-api-challenge | 76e32f16858fff0984ae499cca9d0139ad94a37a | [
"ADSL"
] | null | null | null | VacationPy/VacationPy.ipynb | jgmoore10/python-api-challenge | 76e32f16858fff0984ae499cca9d0139ad94a37a | [
"ADSL"
] | null | null | null | VacationPy/VacationPy.ipynb | jgmoore10/python-api-challenge | 76e32f16858fff0984ae499cca9d0139ad94a37a | [
"ADSL"
] | null | null | null | 31.395036 | 160 | 0.34204 | [
[
[
"# VacationPy\n----\n\n#### Note\n* Keep an eye on your API usage. Use https://developers.google.com/maps/reporting/gmp-reporting as reference for how to monitor your usage and billing.\n\n* Instructions have been included for each segment. You do not have to follow them exactly, but they are included to help you think through the steps.",
"_____no_output_____"
]
],
[
[
"# Dependencies and Setup\nimport matplotlib.pyplot as plt\nimport pandas as pd\nimport numpy as np\nimport requests\nimport gmaps\nimport os\n\n# Import API key\nfrom api_keys import g_key",
"_____no_output_____"
]
],
[
[
"### Store Part I results into DataFrame\n* Load the csv exported in Part I to a DataFrame",
"_____no_output_____"
]
],
[
[
"city_data = pd.read_csv(\"../output_data/cities.csv\")\ncity_data.head()\n",
"_____no_output_____"
]
],
[
[
"### Humidity Heatmap\n* Configure gmaps.\n* Use the Lat and Lng as locations and Humidity as the weight.\n* Add Heatmap layer to map.",
"_____no_output_____"
]
],
[
[
"gmaps.configure(api_key=g_key)\n\nlocations = city_data[[\"Lat\", \"Lng\"]].astype(float)\nhumidity = city_data[\"Humidity\"].astype(float)",
"_____no_output_____"
],
[
"fig = gmaps.figure()\n\nheat_layer = gmaps.heatmap_layer(locations, weights = humidity, dissipating = False, max_intensity = 100, point_radius = 1)\n\nfig.add_layer(heat_layer)\n\nfig",
"_____no_output_____"
]
],
[
[
"### Create new DataFrame fitting weather criteria\n* Narrow down the cities to fit weather conditions.\n* Drop any rows will null values.",
"_____no_output_____"
]
],
[
[
"narrowed_city_df = city_data.loc[(city_data[\"Humidity\"]>=70) & (city_data[\"Wind Speed\"]>=10) & \\\n (city_data[\"Cloudiness\"] <= 20)].dropna()\n\nnarrowed_city_df.head()",
"_____no_output_____"
]
],
[
[
"### Hotel Map\n* Store into variable named `hotel_df`.\n* Add a \"Hotel Name\" column to the DataFrame.\n* Set parameters to search for hotels with 5000 meters.\n* Hit the Google Places API for each city's coordinates.\n* Store the first Hotel result into the DataFrame.\n* Plot markers on top of the heatmap.",
"_____no_output_____"
]
],
[
[
"hotel_df = narrowed_city_df.reset_index(drop=True)\nhotel_df[\"Hotel Name\"] = \"\"\n\nhotel_df",
"_____no_output_____"
],
[
"# geocoordinates\ntarget_search = \"Hotel\"\ntarget_radius = 5000\ntarget_type = \"Hotels\"\n\nparams={\n \"radius\":target_radius,\n \"types\":target_type,\n \"keyword\":target_search,\n \"key\":g_key\n}",
"_____no_output_____"
],
[
"# NOTE: Do not change any of the code in this cell\n\n# Using the template add the hotel marks to the heatmap\ninfo_box_template = \"\"\"\n<dl>\n<dt>Name</dt><dd>{Hotel Name}</dd>\n<dt>City</dt><dd>{City}</dd>\n<dt>Country</dt><dd>{Country}</dd>\n</dl>\n\"\"\"\n# Store the DataFrame Row\n# NOTE: be sure to update with your DataFrame name\nhotel_info = [info_box_template.format(**row) for index, row in hotel_df.iterrows()]\nlocations = hotel_df[[\"Lat\", \"Lng\"]]",
"_____no_output_____"
],
[
"# Add marker layer ontop of heat map\nmarkers = gmaps.marker_layer(locations)\nfig.add_layer(markers)\n\n\n# Display figure\nfig",
"_____no_output_____"
]
]
] | [
"markdown",
"code",
"markdown",
"code",
"markdown",
"code",
"markdown",
"code",
"markdown",
"code"
] | [
[
"markdown"
],
[
"code"
],
[
"markdown"
],
[
"code"
],
[
"markdown"
],
[
"code",
"code"
],
[
"markdown"
],
[
"code"
],
[
"markdown"
],
[
"code",
"code",
"code",
"code"
]
] |
d090f1a5d997198b35f167e66f50d24d76950b6b | 61,461 | ipynb | Jupyter Notebook | 3_Control_flow.ipynb | konshte/Python_K | 6ab7ebfcd0e31d3ca4cee6b5cfc71dced3ce3a62 | [
"MIT"
] | null | null | null | 3_Control_flow.ipynb | konshte/Python_K | 6ab7ebfcd0e31d3ca4cee6b5cfc71dced3ce3a62 | [
"MIT"
] | null | null | null | 3_Control_flow.ipynb | konshte/Python_K | 6ab7ebfcd0e31d3ca4cee6b5cfc71dced3ce3a62 | [
"MIT"
] | null | null | null | 21.679365 | 266 | 0.465124 | [
[
[
"from IPython.core.display import HTML\ndef css_styling():\n styles = open(\"./styles/custom.css\", \"r\").read()\n return HTML(styles)\ncss_styling()",
"_____no_output_____"
]
],
[
[
"### BEFORE YOU DO ANYTHING...\nIn the terminal:\n1. Navigate to __inside__ your ILAS_Python repository.\n2. __COMMIT__ any un-commited work on your personal computer.\n3. __PULL__ any changes *you* have made using another computer.\n4. __PULL__ textbook updates (including homework answers).",
"_____no_output_____"
],
[
"# Control Flow \n\n# Lesson Goal\n\nCompose simple programs to control the order in which the operators we have studied so far are executed.\n\n",
"_____no_output_____"
],
[
"# Objectives\n\nControl the flow of a program using:\n- __control statements__\n- __loops__ \n ",
"_____no_output_____"
],
[
"## Why we are studying this:\n\nControl flow allows us to make __choices__ using our program.\n\nControl statements result in a decision being made as to which of __two or more possible paths__ to follow. ",
"_____no_output_____"
],
[
"## Lesson structure:\n - Control Statements\n - `if` and `else`statements\n - `for` loops\n - `while` loops\n - `break` and `continue` statements\n - Review Exercises\n - Summary",
"_____no_output_____"
],
[
"What is a *__control statement__*?\n\nLet's start with an example from the last seminar...",
"_____no_output_____"
],
[
"## Control Statements\nIn the last seminar we looked at a simple computer program that returned Boolean (True or False) variables... \n\n",
"_____no_output_____"
],
[
"Based on the current time of day, the program answers two questions:\n\n>__Is it lunchtime?__\n\n>`True`\n\nif it is lunch time.\n\n<br>\n\n>__Is it time for work?__\n\n>`True`\n\nif it is `not`:\n- before work (`time < work_starts`)\n- after work (`time > work_ends `)\n- lunchtime (the previous question assigns the value `True` or `False` to variable `lunchtime`).",
"_____no_output_____"
]
],
[
[
"# Time-telling program\n\ntime = 13.05 # current time\n\nwork_starts = 8.00 # time work starts \nwork_ends = 17.00 # time work ends\n\nlunch_starts = 13.00 # time lunch starts\nlunch_ends = 14.00 # time lunch ends\n\n# variable lunchtime is True if the time is between the start and end of lunchtime\nlunchtime = time >= lunch_starts and time < lunch_ends\n\n# variable work_time is True if the time is not... \nwork_time = not ( time < work_starts # ... before work\n or time > work_ends # ... or after work\n or lunchtime) # ... or lunchtime\n \n\nprint(\"Is it work time?\")\nprint(work_time)\nprint(\"Is it lunchtime?\")\nprint(lunchtime)",
"Is it work time?\nFalse\nIs it lunchtime?\nTrue\n"
]
],
[
[
"What if we now want our computer program to do something based on these answers?\n\nTo do this, we need to use *control statements*.\n\nControl statements allow us to make decisions in a program.\n\nThis decision making is known as *control flow*. \n\nControl statements are a fundamental part of programming.",
"_____no_output_____"
],
[
"Here is a control statement in pseudo code:\n\nThis is an `if` statement.\n\n if A is true \n Perform task X\n \nFor example \n\n if lunchtime is true \n Eat lunch\n",
"_____no_output_____"
],
[
"We can check if an alternative to the `if` statement is true using an `else if` statement.\n\n\n if A is true\n Perform task X (only)\n \n else if B is true\n Perform task Y (only)",
"_____no_output_____"
],
[
"Example:\n\n if lunchtime is true\n Eat lunch\n \n else if work_time is true\n Do work",
"_____no_output_____"
],
[
"Often it is useful to include an `else` statement.\n\nIf none of the `if` and `else if` statements are satisfied, the code following the `else` statement will be executed.\n\n if A is true\n Perform task X (only)\n \n else if B is true\n Perform task Y (only)\n \n else \n Perform task Z (only)\n \n\n\n\n",
"_____no_output_____"
],
[
" if lunchtime is true\n Eat lunch\n \n else if work_time is true\n Do work\n \n else \n Go home",
"_____no_output_____"
],
[
"Let's get a better understanding of control flow statements by completing some examples. ",
"_____no_output_____"
],
[
"<a id='IfElse'></a>\n\n## `if` and `else` statements\n\nLet's consider a simple example that demonstrates a Python if-else control statement. \n\nIt uses the lunch/work example from the previous seminar.\n\n__Note:__ In Python, \"else if\" is written: `elif`",
"_____no_output_____"
]
],
[
[
"# Time-telling program\n\ntime = 13.05 # current time\n\nwork_starts = 8.00 # time work starts \nwork_ends = 17.00 # time work ends\n\nlunch_starts = 13.00 # time lunch starts\nlunch_ends = 14.00 # time lunch ends\n\n# variable lunchtime is True if the time is between the start and end of lunchtime\nlunchtime = time >= lunch_starts and time < lunch_ends\n\n# variable work_time is True if the time is not... \nwork_time = not ( time < work_starts # ... before work\n or time > work_ends # ... or after work\n or lunchtime) # ... or lunchtime\n \n\n#print(\"Is it work time?\")\n#print(work_time)\n#print(\"Is it lunchtime?\")\n#print(lunchtime)\n\nif lunchtime: # if lunchtime == True:\n print(\"Eat lunch\")\n \nelif work_time: # elif work_time == True:\n print(\"Do work\")\n \nelse: \n print(\"Go home\")\n",
"Eat lunch\n"
]
],
[
[
"__Remember:__ The program assigns the variables lunchtime and work_time the values `True` or `False`.\n\nTherefore when we type: \n<br>`if lunchtime`\n\n<br>the meaning is the same as: \n<br>`if lunchtime == True`",
"_____no_output_____"
],
[
"Here is another example, using algebraic operators to modify the value of an initial variable, `x`. \n\nThe modification of `x` and the message printed depend on the initial value of `x`.",
"_____no_output_____"
]
],
[
[
"#The input to the program is variable `x`.\nx = -10.0 # Initial x value\n\nif x > 0.0: \n print('Initial x is greater than zero') #The program prints a message... \n x -= 20.0 # ...and modifies `x`.\n \nelif x < 0.0: \n print('Initial x is less than zero')\n x += 21.0\n \nelse: \n print('Initial x is not less than zero and not greater than zero, therefore it must be zero')\n x *= 2.5\n\nprint(\"Modified x = \", x)",
"Initial x is less than zero\nModified x = 11.0\n"
]
],
[
[
"\n\n__Note:__ The program uses the short-cut algebraic operators that you learnt to use in the last seminar. ",
"_____no_output_____"
],
[
"__Try it yourself__\n\nIn the cell code cell above, try:\n\n- changing the operations performed on `x`\n\n- changing the value of `x` a few times.\n\nRe-run the cell to see the different paths the program can follow.",
"_____no_output_____"
],
[
"### Look carefully at the structure of the `if`, `elif`, `else`, control statement:\n\n\n__The control statement begins with an `if`__, followed by the expression to check. <br> \n At the end of the `if` statement you must put a colon (`:`) <br> \n````python\nif x > 0.0: \n````",
"_____no_output_____"
],
[
"After the `if` statement, indent the code to be run in the case that the `if` statement is `True`. <br>\n\n\n To end the code to be run, simply stop indenting:\n \n````python\nif x > 0.0:\n print('Initial x is greater than zero')\n x -= 20.0\n````",
"_____no_output_____"
],
[
"The indent can be any number of spaces.\n\nThe number of spaces must be the same for all lines of code to be run if the `if` statement is True.\n\nJupyter Notebooks automatically indent 4 spaces.\n\nThis is considered best practise. ",
"_____no_output_____"
],
[
" `if x > 0.0` is:\n - `True`:\n - The indented code is executed.\n - The control block is exited.\n - The program moves past any subsequent `elif` or `else` statements.\n <br> \n \n \n - `False`:\n the program moves past the inented code to the next (non-indented) part of the program... <br>",
"_____no_output_____"
],
[
"In this the next (non-indented) part of the program is `elif` (else if).\n\nThe elif statement is evaluated.\n\n(Notice that the code is structured in the same way as the `if` statement.):\n\n```python\nif x > 0.0:\n print('Initial x is greater than zero')\n x -= 20.0\n \nelif x < 0.0:\n print('Initial x is less than zero')\n x += 21.0\n``` ",
"_____no_output_____"
],
[
"`elif x < 0.0`:\n\n- `True`:\n - The indented code is executed.\n - The control block is exited. \n - The program moves past any subsequent `elif` or `else` statements.\n \n \n- `False`:\n the program moves past the indented code to the next (non-indented) part of the program. <br>\n \n\n ",
"_____no_output_____"
],
[
"If none of the preceding `if` or `elif` stements are true.\n<br> e.g. in this example:\n - `x > 0.0` is `False` \n - `x < 0.0` is `False`\n\nthe code following the `else` statement is executed.\n\n```python\nif x > 0.0:\n print('Initial x is greater than zero')\n x -= 20.0\n\nelif x < 0.0:\n print('Initial x is less than zero')\n x += 21.0\n\nelse:\n print('Initial x is not less than zero and not greater than zero, therefore it must be zero')\n```",
"_____no_output_____"
],
[
"Evaluating data against different criteria is extremely useful for solving real-world mathematical problems.",
"_____no_output_____"
],
[
"Let's look at a simple example...",
"_____no_output_____"
],
[
"### Real-World Example: currency trading\n\nTo make a comission (profit), a currency trader sells US dollars to travellers above the market rate. \n\nThe multiplier used to calculate the amount recieved by customer is shown in the table:\n\n|Amount (JPY) |Multiplier |\n|--------------------------------------------|-------------------------|\n| Less than $100$ | 0.9 | \n| From $100$ and less than $1,000$ | 0.925 | \n| From $1,000$ and less than $10,000$ | 0.95 | \n| From $10,000$ and less than $100,000$ | 0.97 | \n| Over $100,000$ | 0.98 | \n\n The currency trader charges more if the customer pays with cash. \n <br>If the customer pays with cash, the currency trader reduces the rate by an __additional__ 10% after conversion. \n <br>(If the transaction is made electronically, they do not). \n\n",
"_____no_output_____"
],
[
"__Current market rate:__ 1 JPY = 0.0091 USD.\n\n__Effective rate:__ The rate that the customer receives based on the amount in JPY to be changed.",
"_____no_output_____"
],
[
"The program calculates the __effective rate__ using:\n - The reduction based on the values in the table.\n - An additional 10% reduction (mutiplier = 0.9) if the transaction is made in cash.",
"_____no_output_____"
]
],
[
[
"JPY = 1_000_000 # The amount in JPY to be changed into USD\ncash = False # True if transaction is in cash, otherwise False\n\nmarket_rate = 0.0091 # 1 JPY is worth this many dollars at the market rate\n\n# Apply the appropriate reduction depending on the amount being sold\nif JPY < 10_000:\n multiplier = 0.9 \n \nelif JPY < 100_000: \n multiplier = 0.925 * market_rate * JPY\n \nelif JPY < 1_000_000:\n multiplier = 0.95 * market_rate * JPY\n \nelif JPY < 10_000_000:\n multiplier = 0.97 * market_rate * JPY\n\nelse: # JPY > 10,000,000\n multiplier = 0.98 * market_rate * JPY\n \n\n \n# Apply the appropriate reduction depending if the transaction is made in cash or not\nif cash:\n cash_multiplier = 0.9\nelse:\n cash_multiplier = 1 \n \n \n \n# Calculate the total amount sold to the customer \nUSD = JPY * market_rate * multiplier * cash_multiplier\n \nprint(\"Amount in JPY sold:\", JPY)\nprint(\"Amount in USD purchased:\", USD)\nprint(\"Effective rate:\", USD/JPY)",
"Amount in JPY sold: 1000000\nAmount in USD purchased: 80325700.0\nEffective rate: 80.3257\n"
]
],
[
[
" __Note:__\n - We can use multiple `elif` statements within a control block.\n - We can use multipe `if` statements. <br>When the program executes and exits a control block, it moves to the next `if` statement. \n - __Readability:__ <br>Underscores _ are placed between 0s in long numbers to make them easier to read. \n <br>You DO NOT need to include underscores for Python to interpret the number correctly.\n <br>You can place the underscores wherever you like in the sequence of digits that make up the number. \n \n",
"_____no_output_____"
],
[
"__Try it yourself__\n\nIn your textbook, try changing the values of `JPY` and `cash` a few times.\n\nRe-run the cell to see the different paths the program can follow.",
"_____no_output_____"
],
[
"<a id='ForLoops'></a>\n\n## `for` loops\n\n*Loops* are used to execute a command repeatedly.\n<br>\nA loop is a block that repeats an operation a specified number of times (loops). \n\nTo learn about loops we are going to use the function `range()`.",
"_____no_output_____"
],
[
"### `range`\n\nThe function `range` gives us a sequence of *integer* numbers.\n\n`range(3, 6)` returns integer values starting from 3 and ending at 6.\n\ni.e.\n\n> 3, 4, 5\n\nNote this does not include 6.\n\n",
"_____no_output_____"
],
[
"We can change the starting value.\n \nFor example for integer values starting at 0 and ending at 4:\n \n`range(0,4)`\n\nreturns:\n\n> 0, 1, 2, 3\n\n`range(4)` is a __shortcut__ for range(0, 4) \nrange (0,5)\nrange (5,9)",
"_____no_output_____"
],
[
"### Simple `for` loops",
"_____no_output_____"
],
[
"The statement \n```python\nfor i in range(0, 5):\n```\nsays that we want to run the indented code five times.",
"_____no_output_____"
]
],
[
[
"for i in range(0, 6):\n print(i)\n \n",
"0\n1\n2\n3\n4\n5\n"
]
],
[
[
"The first time through, the value of i is equal to 0.\n<br>\nThe second time through, its value is 1.\n<br>\nEach loop the value `i` increases by 1 (0, 1, 2, 3, 4) until the last time when its value is 4. ",
"_____no_output_____"
],
[
"Look carefully at the structure of the `for` loop:\n - `for` is followed by the condition being checked.\n - : colon at the end of the `for` statement. \n - The indented code that follows is run each time the code loops. <br>\n (The __same of spaces__ should be used for all indents) \n <br> \n - To end the `for` loop, simply stop indenting. ",
"_____no_output_____"
]
],
[
[
"for i in range(-2, 3):\n print(i)\nprint('The end of the loop')",
"-2\n-1\n0\n1\n2\nThe end of the loop\n"
]
],
[
[
"The above loop starts from -2 and executes the indented code for each value of i in the range (-2, -1, 0, 1, 2).\n<br>\nWhen the loop has executed the code for the final value `i = 2`, it moves on to the next unindented line of code.",
"_____no_output_____"
]
],
[
[
"for n in range(4):\n \n print(\"----\")\n \n print(n, n**2)",
"----\n0 0\n----\n1 1\n----\n2 4\n----\n3 9\n"
]
],
[
[
"The above executes 4 loops.\n\nThe statement \n```python\nfor n in range(4):\n```\nsays that we want to loop over four integers, starting from 0. \n\nEach loop the value `n` increases by 1 (0, 1, 2 3).\n\n\n",
"_____no_output_____"
],
[
"__Try it yourself__\n<br>\nGo back and change the __range__ of input values in the last three cells and observe the change in output. \n",
"_____no_output_____"
],
[
"If we want to step by three rather than one:",
"_____no_output_____"
]
],
[
[
"for n in range(0, 10, 3):\n print(n)",
"0\n3\n6\n9\n"
]
],
[
[
"If we want to step backwards rather than forwards we __must__ include the step size:",
"_____no_output_____"
]
],
[
[
"for n in range(10, 0, -1):\n print(n)",
"10\n9\n8\n7\n6\n5\n4\n3\n2\n1\n"
]
],
[
[
"For example...",
"_____no_output_____"
]
],
[
[
"for n in range(10, 0):\n print(n)",
"_____no_output_____"
]
],
[
[
"...does not return any values because there are no values that lie between 10 and 0 when counting in the positive direction from 10. ",
"_____no_output_____"
],
[
"__Try it yourself.__\n\nIn the cell below write a `for` loop that:\n- starts at `n = 9`\n- ends at `n = 3` (and includes `n = 3`)\n- loops __backwards__ through the range in steps of -3 \n- prints `n`$^2$ at each loop.\n",
"_____no_output_____"
]
],
[
[
"# For loop\nfor n in range(9, 2, -3):\n print (\"-----\")\n print(n, n**2)",
"-----\n9 81\n-----\n6 36\n-----\n3 9\n"
]
],
[
[
"For loops are useful for performing operations on large data sets.\n\nWe often encounter large data sets in real-world mathematical problems. ",
"_____no_output_____"
],
[
"A simple example of this is converting multiple values using the same mathematical equation to create a look-up table...",
"_____no_output_____"
],
[
"### Real-world Example: conversion table from degrees Fahrenheit to degrees Celsius\n\nWe can use a `for` loop to create a conversion table from degrees Fahrenheit ($T_F$) to degrees Celsius ($T_c$).\n\nConversion formula:\n\n$$\nT_c = 5(T_f - 32)/9\n$$\n\nComputing the conversion from -100 F to 200 F in steps of 20 F (not including 200 F):",
"_____no_output_____"
]
],
[
[
"print(\"T_f, T_c\")\n\nfor Tf in range(-100, 200, 20):\n print(Tf, \"\\t\", round(((Tf - 32) * 5 / 9), 3))",
"T_f, T_c\n-100 \t -73.333\n-80 \t -62.222\n-60 \t -51.111\n-40 \t -40.0\n-20 \t -28.889\n0 \t -17.778\n20 \t -6.667\n40 \t 4.444\n60 \t 15.556\n80 \t 26.667\n100 \t 37.778\n120 \t 48.889\n140 \t 60.0\n160 \t 71.111\n180 \t 82.222\n"
]
],
[
[
"<a id='WhileLoops'></a>\n\n## `while` loops\n\nA __`for`__ loop performs an operation a specified number of times. \n\n```python \nfor x in range(5):\n print(x)\n``` \n\nA __`while`__ loop performs a task *while* a specified statement is true. \n\n```python\nx = 0\nwhile x < 5:\n print(x)\n```",
"_____no_output_____"
],
[
"The structure of a `while` loop is similar to a `for` loop.\n- `while` is followed by the condition being checked.\n- : colon at the end of the `while` statement. \n- The indented code that follows is repeatedly executed until the `while` statement (e.g. `x < 5`) is `False`. <br>\n\n ",
"_____no_output_____"
],
[
"It can be quite easy to crash your computer using a `while` loop. \n\ne.g. if we don't modify the value of x each time the code loops:\n```python\nx = 0\nwhile x < 5:\n print(x)\n # x += 1 \n```\nwill continue indefinitely since `x < 5 == False` will never be satisfied.\n\nThis is called an *infinite loop*.\n\n",
"_____no_output_____"
],
[
"To perform the same function as the `for` loop we need to increment the value of `x` within the loop:",
"_____no_output_____"
]
],
[
[
"x = 0\n\nprint(\"Start of while statement\")\n\nwhile x < 5:\n print(x)\n x += 1 # Increment x\n \nprint(\"End of while statement\")",
"Start of while statement\n0\n1\n2\n3\n4\nEnd of while statement\n"
]
],
[
[
"`for` loops are often safer when performing an operation on a set range of values.",
"_____no_output_____"
]
],
[
[
"x = -2\n\nprint(\"Start of for statement\")\n\nfor y in range(x,5):\n print(y)\n \nprint(\"End of for statement\")",
"Start of for statement\n-2\n-1\n0\n1\n2\n3\n4\nEnd of for statement\n"
]
],
[
[
"Here is another example of a `while` loop.",
"_____no_output_____"
]
],
[
[
"x = 0.9\n\nwhile x > 0.001:\n # Square x (shortcut x *= x)\n x = x * x\n print(round(x, 6))",
"0.81\n0.6561\n0.430467\n0.185302\n0.034337\n0.001179\n1e-06\n"
]
],
[
[
"If we use an initial value of $x \\ge 1$, an infinite loop will be generted.\n\n`x` will increase with each loop, meaning `x` will always be greater than 0.001.\n\ne.g. \n```python\nx = 2\n\nwhile x > 0.001:\n x = x * x\n print(x)\n```",
"_____no_output_____"
],
[
"However, using a `for` loop is a less appropriate solution in this case.\n<br>We may not know beforehand how many steps are required before `x > 0.001` becomes false.",
"_____no_output_____"
],
[
"To avoid errors, it is good practice to check that $x < 1$ before entering the `while` loop e.g.",
"_____no_output_____"
]
],
[
[
"x = 0.9\n\nif x < 1:\n\n while x > 0.001:\n # Square x (shortcut x *= x)\n x = x * x\n print(round(x, 6))\n \nelse:\n print(\"x is greater than one, infinite loop avoided\")",
"0.81\n0.6561\n0.430467\n0.185302\n0.034337\n0.001179\n1e-06\n"
]
],
[
[
"__Try it for yourself:__\n\nIn the cell above change the value of x to above or below 1.\n\nObserve the output.\n",
"_____no_output_____"
],
[
"__Try it for yourself:__\n\nIn the cell below:\n - Create a variable,`x`, with the initial value 50\n - Each loop:\n 1. print x\n 1. reduce the value of x by half\n - Exit the loop when `x` < 3",
"_____no_output_____"
]
],
[
[
"# While loop",
"_____no_output_____"
]
],
[
[
"## `break` and `continue`.\n\n<a id='break'></a>\n### `break`\n\nSometimes we want to break out of a `for` or `while` loop. \n\nFor example in a `for` loop we can check if something is true, and then exit the loop prematurely, e.g",
"_____no_output_____"
],
[
"<img src=\"img/flowchart-break-statement.jpg\" alt=\"Drawing\" style=\"width: 300px;\"/>",
"_____no_output_____"
],
[
"<img src=\"img/break-statement-algorithm.jpg\" alt=\"Drawing\" style=\"width: 300px;\"/>",
"_____no_output_____"
]
],
[
[
"for x in range(10):\n print(x)\n \n if x == 5:\n print(\"Time to break out\")\n break",
"0\n1\n2\n3\n4\n5\nTime to break out\n"
]
],
[
[
"Let's look at how we can use this in a program...\n",
"_____no_output_____"
],
[
"The following program __finds prime numbers__.\n\n__Prime number:__ A positive integer, greater than 1, that has no positive divisors other than 1 and itself (2, 3, 5, 11, 13, 17....)\n\nThe program checks (integer) numbers, `n` up to a limit `N` and prints the prime numbers. \n\n",
"_____no_output_____"
],
[
"We can determine in `n` is a prime nunber by diving it by every number in the range 2 to `n`.\n\nIf any of these calculations has a remainder equal to zero, n is not a prime number.",
"_____no_output_____"
]
],
[
[
"N = 50 # Check numbers up 50 for primes (excludes 50)\n\n# Loop over all numbers from 2 to 50 (excluding 50)\nfor n in range(2, N):\n\n # Assume that n is prime\n n_is_prime = True\n\n # Check if n divided by (any number in the range 2 to n) returns a remainder equal to 0 \n for m in range(2, n):\n \n # If the remainder is zero, n is not a prime number\n if n % m == 0: \n n_is_prime = False\n\n # If n is prime, print to screen \n if n_is_prime:\n print(n)",
"2\n3\n5\n7\n11\n13\n17\n19\n23\n29\n31\n37\n41\n43\n47\n"
]
],
[
[
"Notice that our program contains a second `for` loop. \n\nFor each value of n, it loops through incrementing values of m in the range (2 to n):\n\n```python\n# Check if n can be divided by m\n# m ranges from 2 to n (excluding n)\nfor m in range(2, n):\n```\nbefore incrementing to the next value of n.\n\nWe call this a *nested* loop.\n\nThe indents in the code show where loops are nested.\n \nHere it is again without the comments:",
"_____no_output_____"
]
],
[
[
"N = 50 \n\n# for loop 1\nfor n in range(2, N): \n n_is_prime = True\n\n # for loop 2\n for m in range(2, n): \n if n % m == 0: \n n_is_prime = False\n \n if n_is_prime:\n print(n)",
"2\n3\n5\n7\n11\n13\n17\n19\n23\n29\n31\n37\n41\n43\n47\n"
]
],
[
[
"As n gets larger, dividing it by *every* number in the range (2, n) becomes more and more inefficient. \n\nA `break` statement allows us to exit the loop as soon as a remainder equal to zero is returned (indicating that n is not a prime number). \n\n",
"_____no_output_____"
],
[
"In the program below, a break statement is added.\n\nAs soon as a number is found to be not prime, the program breaks out of loop 2 and goes to the next value of n in loop 1.\n\nBy placing `else` *one level up* from `if` the program will iterate through all values of m before printing n if n is prime. ",
"_____no_output_____"
]
],
[
[
"N = 55 \n# for loop 1\nfor n in range(2, N):\n \n # for loop 2\n for m in range(2, n):\n if n % m == 0: \n break\n \n else: \n # if n is prime\n print(n)",
"3\n5\n5\n5\n7\n7\n7\n7\n7\n9\n11\n11\n11\n11\n11\n11\n11\n11\n11\n13\n13\n13\n13\n13\n13\n13\n13\n13\n13\n13\n15\n17\n17\n17\n17\n17\n17\n17\n17\n17\n17\n17\n17\n17\n17\n17\n19\n19\n19\n19\n19\n19\n19\n19\n19\n19\n19\n19\n19\n19\n19\n19\n19\n21\n23\n23\n23\n23\n23\n23\n23\n23\n23\n23\n23\n23\n23\n23\n23\n23\n23\n23\n23\n23\n23\n25\n25\n25\n27\n29\n29\n29\n29\n29\n29\n29\n29\n29\n29\n29\n29\n29\n29\n29\n29\n29\n29\n29\n29\n29\n29\n29\n29\n29\n29\n29\n31\n31\n31\n31\n31\n31\n31\n31\n31\n31\n31\n31\n31\n31\n31\n31\n31\n31\n31\n31\n31\n31\n31\n31\n31\n31\n31\n31\n31\n33\n35\n35\n35\n37\n37\n37\n37\n37\n37\n37\n37\n37\n37\n37\n37\n37\n37\n37\n37\n37\n37\n37\n37\n37\n37\n37\n37\n37\n37\n37\n37\n37\n37\n37\n37\n37\n37\n37\n39\n41\n41\n41\n41\n41\n41\n41\n41\n41\n41\n41\n41\n41\n41\n41\n41\n41\n41\n41\n41\n41\n41\n41\n41\n41\n41\n41\n41\n41\n41\n41\n41\n41\n41\n41\n41\n41\n41\n41\n43\n43\n43\n43\n43\n43\n43\n43\n43\n43\n43\n43\n43\n43\n43\n43\n43\n43\n43\n43\n43\n43\n43\n43\n43\n43\n43\n43\n43\n43\n43\n43\n43\n43\n43\n43\n43\n43\n43\n43\n43\n45\n47\n47\n47\n47\n47\n47\n47\n47\n47\n47\n47\n47\n47\n47\n47\n47\n47\n47\n47\n47\n47\n47\n47\n47\n47\n47\n47\n47\n47\n47\n47\n47\n47\n47\n47\n47\n47\n47\n47\n47\n47\n47\n47\n47\n47\n49\n49\n49\n49\n49\n"
]
],
[
[
"<a id='Continue'></a>\n\n### `continue`\n\nSometimes, instead of stopping the loop we want to go to the next iteration in a loop, skipping the remaining code.\n\nFor this we use `continue`. \n\n",
"_____no_output_____"
],
[
"<img src=\"img/continue-statement-flowchart.jpg\" alt=\"Drawing\" style=\"width: 300px;\"/>",
"_____no_output_____"
],
[
"<img src=\"img/algorithm-continue-statement.jpg\" alt=\"Drawing\" style=\"width: 300px;\"/>",
"_____no_output_____"
],
[
"The example below loops over 20 numbers (0 to 19) and checks if the number is divisible by 4. \n\nIf the number is not divisible by 4:\n\n- it prints a message \n- it moves to the next value. \n\nIf the number is divisible by 4 it *continues* to the next value in the loop, without printing.",
"_____no_output_____"
]
],
[
[
"for j in range(1, 20):\n \n if j % 4 == 0: # Check remainer of j/4\n continue # continue to next value of j\n \n print(j, \"is not a multiple of 4\")",
"1 is not a multiple of 4\n2 is not a multiple of 4\n3 is not a multiple of 4\n5 is not a multiple of 4\n6 is not a multiple of 4\n7 is not a multiple of 4\n9 is not a multiple of 4\n10 is not a multiple of 4\n11 is not a multiple of 4\n13 is not a multiple of 4\n14 is not a multiple of 4\n15 is not a multiple of 4\n17 is not a multiple of 4\n18 is not a multiple of 4\n19 is not a multiple of 4\n"
]
],
[
[
"To compare, if we used `break` instead of `continue`:",
"_____no_output_____"
]
],
[
[
"for j in range(1, 20):\n \n if j % 4 == 0: # Check remainer of j/4\n break # continue to next value of j\n \n print(j, \"is not a multiple of 4\")",
"1 is not a multiple of 4\n2 is not a multiple of 4\n3 is not a multiple of 4\n"
]
],
[
[
"__Try it yourself__\nWe can use a `for` loop to perform an operation on each character of a string.\n\n```Python\nstring = \"string\"\n\nfor i in range(len(sting)):\n print(sting[i])\n```",
"_____no_output_____"
],
[
"In the cell below, loop through the characters of the string.\nUse `continue` to only print the letters of the word *sting*.",
"_____no_output_____"
]
],
[
[
"# Print the letters of the word sting\nstring = \"string\"",
"_____no_output_____"
]
],
[
[
"## Review Exercises\nHere are a series of engineering problems for you to practise each of the new Python skills that you have learnt today.",
"_____no_output_____"
],
[
"### Review Exercise: `while` loops.\nIn the cell below, write a while loop that with each loop:\n- prints the value of `x`\n- then decreases the value of x by 0.5\n\nas long as `x` remains positive.\n\n<a href='#WhileLoops'>Jump to While Loops</a>",
"_____no_output_____"
]
],
[
[
"x = 4\nwhile x > 0:\n print(x)\n x -= 0.5",
"4\n3.5\n3.0\n2.5\n2.0\n1.5\n1.0\n0.5\n"
],
[
"# Example Solution\n\nwhile (x > 0):\n print(x)\n x -= 0.5",
"_____no_output_____"
]
],
[
[
"### Review Exercise: `for` loops\nIn the cell below, write a `for` loop to print the even numbers from 2 to 100, inclusive.",
"_____no_output_____"
]
],
[
[
"# for loop to print the even numbers from 2 to 20, inclusive.\nfor n in range (2, 21):\n print (n)",
"2\n3\n4\n5\n6\n7\n8\n9\n10\n11\n12\n13\n14\n15\n16\n17\n18\n19\n20\n"
],
[
"# Example Solution\n\nfor i in range(2, 21, 2):\n print(i) ",
"_____no_output_____"
]
],
[
[
"### Review Excercise: `for` loops and `if` statements\nIn the cell below, write a for loop to alternately print `Red` then `Blue` 3 times. \n<br>i.e. \n<br>Red \n<br>Blue \n<br>Red \n<br>Blue \n<br>Red \n<br>Blue ",
"_____no_output_____"
]
],
[
[
"# Alternately print Red and Blue\n\nfor n in range (1, 7):\n if n % 2 == 0:\n print(\"red\")\n elif ",
"_____no_output_____"
],
[
"# Example Solution\n\ncolour = \"Red\"\n\nfor n in range(6):\n print(colour)\n \n if colour == \"Red\":\n colour = \"Blue\"\n \n else:\n colour = \"Red\" ",
"_____no_output_____"
]
],
[
[
"### Review Exercise: `continue`\nIn the cell below, loop through the characters of the string.\n<br>Use `continue` to only print the letters of the word *sing*.\n<br>Hint: Refer to __Logical Operators__ (Seminar 2). \n\n<a href='#Continue'>Jump to continue</a>",
"_____no_output_____"
]
],
[
[
"# Print the letters of the word sing\nstring = \"string\"",
"_____no_output_____"
],
[
"# Example Solution\nstring = \"string\"\n\nfor i in range(len(string)):\n \n if string[i] == \"r\" or string[i] == \"t\":\n continue\n \n print(string[i])",
"_____no_output_____"
]
],
[
[
"### Review Exercise: `for` loops and `if`, `else` and `continue` statements.\n__(A)__ In the cell below, use a for loop to print the square roots of the first 25 odd positive integers.\n<br> (Remember, the square root of a number, $x$ can be found by $x^{1/2}$)\n\n__(B)__ If the number generated is greater than 3 and smaller than 5, print \"`skip`\" and __`continue`__ to the next iteration *without* printing the number.\n<br>Hint: Refer to __Logical Operators__ (Seminar 2). \n\n<a href='#ForLoops'>Jump to for loops</a>\n\n<a href='#IfElse'>Jump to if and else statements</a>\n\n<a href='#Continue'>Jump to continue</a>",
"_____no_output_____"
]
],
[
[
"# square roots of the first 25 odd positive integers\n\n",
"_____no_output_____"
],
[
"# Example Solution\n\nfor x in range(1, 50, 2):\n \n if((x ** (1/2) > 3) and (x ** (1/2) < 5)): \n print(\"skip\")\n continue\n \n print(x ** (1/2))",
"_____no_output_____"
]
],
[
[
"# Updating your git repository\n\nYou have made several changes to your interactive textbook.\n\nThe final thing we are going to do is add these changes to your online repository so that:\n - I can check your progress\n - You can access the changes from outside of the university system. \n \n > Save your work.\n > <br> `git add -A`\n > <br>`git commit -m \"A short message describing changes\"`\n > <br>`git push`\n ",
"_____no_output_____"
],
[
"# Summary\n\n[*McGrath, Python in easy steps, 2013*]\n\n - The Python `if` keyword performs a conditional test on an expression for a Boolean value of True or False.\n - Alternatives to an `if` test are provided using `elif` and `else` tests.\n - A `while` loop repeats until a test expression returns `False`.\n - A `for`...`in`... loop iterates over each item in a specified data structure (or string).\n - The `range()` function generates a numerical sequence that can be used to specify the length of the `for` loop.\n - The `break` and `continue` keywords interrupt loop iterations.",
"_____no_output_____"
],
[
"# Homework \n\n1. __PULL__ the changes you made in-class today to your personal computer before starting your homework.\n1. __COMPLETE__ any unfinished Review Exercises.\n1. __PUSH__ the changes you make at home to your online repository. \n\n<br>Refer to: __1_Introduction_to_Version_Control.ipynb__. ",
"_____no_output_____"
]
]
] | [
"code",
"markdown",
"code",
"markdown",
"code",
"markdown",
"code",
"markdown",
"code",
"markdown",
"code",
"markdown",
"code",
"markdown",
"code",
"markdown",
"code",
"markdown",
"code",
"markdown",
"code",
"markdown",
"code",
"markdown",
"code",
"markdown",
"code",
"markdown",
"code",
"markdown",
"code",
"markdown",
"code",
"markdown",
"code",
"markdown",
"code",
"markdown",
"code",
"markdown",
"code",
"markdown",
"code",
"markdown",
"code",
"markdown",
"code",
"markdown",
"code",
"markdown",
"code",
"markdown",
"code",
"markdown",
"code",
"markdown",
"code",
"markdown",
"code",
"markdown"
] | [
[
"code"
],
[
"markdown",
"markdown",
"markdown",
"markdown",
"markdown",
"markdown",
"markdown",
"markdown"
],
[
"code"
],
[
"markdown",
"markdown",
"markdown",
"markdown",
"markdown",
"markdown",
"markdown",
"markdown"
],
[
"code"
],
[
"markdown",
"markdown"
],
[
"code"
],
[
"markdown",
"markdown",
"markdown",
"markdown",
"markdown",
"markdown",
"markdown",
"markdown",
"markdown",
"markdown",
"markdown",
"markdown",
"markdown",
"markdown"
],
[
"code"
],
[
"markdown",
"markdown",
"markdown",
"markdown",
"markdown",
"markdown",
"markdown"
],
[
"code"
],
[
"markdown",
"markdown"
],
[
"code"
],
[
"markdown"
],
[
"code"
],
[
"markdown",
"markdown",
"markdown"
],
[
"code"
],
[
"markdown"
],
[
"code"
],
[
"markdown"
],
[
"code"
],
[
"markdown",
"markdown"
],
[
"code"
],
[
"markdown",
"markdown",
"markdown"
],
[
"code"
],
[
"markdown",
"markdown",
"markdown",
"markdown"
],
[
"code"
],
[
"markdown"
],
[
"code"
],
[
"markdown"
],
[
"code"
],
[
"markdown",
"markdown",
"markdown"
],
[
"code"
],
[
"markdown",
"markdown"
],
[
"code"
],
[
"markdown",
"markdown",
"markdown"
],
[
"code"
],
[
"markdown",
"markdown",
"markdown"
],
[
"code"
],
[
"markdown"
],
[
"code"
],
[
"markdown",
"markdown"
],
[
"code"
],
[
"markdown",
"markdown",
"markdown",
"markdown"
],
[
"code"
],
[
"markdown"
],
[
"code"
],
[
"markdown",
"markdown"
],
[
"code"
],
[
"markdown",
"markdown"
],
[
"code",
"code"
],
[
"markdown"
],
[
"code",
"code"
],
[
"markdown"
],
[
"code",
"code"
],
[
"markdown"
],
[
"code",
"code"
],
[
"markdown"
],
[
"code",
"code"
],
[
"markdown",
"markdown",
"markdown"
]
] |
d090f990415a2832145d38d536d4d289872262a8 | 7,327 | ipynb | Jupyter Notebook | T1_chapter1_exercises.ipynb | robfatland/boojum | 4af0adaa01fb0429dd1afa91376c9276fbd224ea | [
"MIT"
] | null | null | null | T1_chapter1_exercises.ipynb | robfatland/boojum | 4af0adaa01fb0429dd1afa91376c9276fbd224ea | [
"MIT"
] | null | null | null | T1_chapter1_exercises.ipynb | robfatland/boojum | 4af0adaa01fb0429dd1afa91376c9276fbd224ea | [
"MIT"
] | null | null | null | 26.261649 | 143 | 0.414904 | [
[
[
"Prove that for integers $a,\\;b,\\;\\dots$\n\n(1) $(a, b) = 1, \\; c | a, \\; d | a \\implies (c, d) = 1$\n\n\nSuppose $(c, d) = e > 1$. Then $e | c$ and $c | a$ implies $e | a$; similarly $e | b$ so $(a, b) > 1$, a \ncontradiction, and therefore $(c, d) = 1$. $\\;\\;\\;\\boxdot$\n\n\n(2) $(a, b) = (a, c) = 1 \\implies (a, bc) = 1$\n\n(3) $(a, b) = 1 \\implies (a^n, b^k) = 1 \\; \\; \\forall \\; \\; n \\ge 1, k \\ge 1$\n\n(4) $(a, b) = 1 \\implies (a + b, a - b) = 1 \\; or \\; 2$\n\n(5) $(a, b) = 1 \\implies (a + b, a^2 - ab + b^2) = 1 \\; or \\; 3$\n\n(6) $(a, b) = 1, \\; d|(a + b) \\implies (a, d) = (b, d) = 1$",
"_____no_output_____"
],
[
"(7) A rational number $a/b$ with $(a, b) = 1$ is a *reduced fraction*. If the sum of two \nreduced fractions is an integer, say $(a/b) + (c/d) = n$, prove that $|b| = |d|$.\n\n(8) An integer is called *squarefree* if it is not divisible by the square of any prime. Prove that \nfor every $n \\ge 1$ there exist uniquely determined $a > 0$ and $b > 0$ such that $n=a^2b$, where $b$ is *squarefree*.",
"_____no_output_____"
],
[
"...\n\n(11) Prove that $n^4 + 4$ is composite if $n > 1$. \n\n***Solution***\n\nI first tried cases for the ones-digit. For example $n$ even gives $n^4 + 4$ also even and $n$ ending in \n1, 3, 7 or 9 gives $n^4 + 4$ ending in 5. \nHowever (particularly because the last case does not resolve in this manner) the right thing to try is \nfactoring $n^4 + 4$ in some obvious way: \nConstants 1 and 4 or 2 and 2. \n\n\n$n^4 + 4 = (n^2 + a \\cdot n + 2) (n^2 + b \\cdot n + 2)$\n\n\nThis gives $n^4 + b \\cdot n^3 + 2 n^2 + a \\cdot n^3 + a \\cdot b \\cdot n^2 + 2 \\cdot a \\cdot n + 2 n^2 + 2 \\cdot b \\cdot n + 4$\n\n\n$n^4 + 4$ plus stuff that needs to be zero: $(b + a)\\cdot n^3 + (4 + a \\cdot b)\\cdot n^2 + (2 \\cdot (a + b))\\cdot n$\n\n\nThis means $a = -b$ and $a \\cdot b = -4$. Great: $a = 2$ and $b = -2$. \n\n\n$n^4 + 4 = (n^2 + 2n + 2)(n^2 - 2n + 2)\\;\\;\\;\\;\\boxdot$",
"_____no_output_____"
]
],
[
[
"def pf(n):\n pfn, i = [], 2\n while i * i < n:\n while n%i == 0: pfn.append(i); n = n / i\n i = i + 1\n pfn.append(int(n))\n return pfn\n\ndef npf(n): return len(pf(n))\n\ndef isprime(n): \n if npf(n) == 1: return True\n return False\n\nfor a in range(3):\n s = a * 10 + 5\n t = s*s*s*s + 4\n if isprime(t): print(str(t) + ' is prime') \n else: print(str(t) + ' factors are ' + str(pf(t)))",
"629 factors are [17, 37]\n50629 factors are [197, 257]\n390629 factors are [577, 677]\n"
]
],
[
[
"...",
"_____no_output_____"
],
[
"...\n\n(20) Let $d = (826, 1890)$. Use the Euclidean algorithm to compute $d$, then express $d$ as a linear combination of 826 and 1890\n\nSolution\n\n$1890 = 826 \\cdot 2 + 238$\n\n$826 = 238 \\cdot 3 + 112$\n\n$238 = 112 \\cdot 2 + 14$\n\n$112 = 14 \\cdot 8 + 0$\n\n$d = 14$\n\n$d = u \\cdot 826 + v \\cdot 1890$ or equivalently $1 = u \\cdot 59 + v \\cdot 135$\n\nTaking $u$ positive it can take on values ${ 4, 9, 14, 19, \\dots }$.\n\n*--a miracle occurs--*\n\n$(d = 14) = 254 \\cdot 826 - 111 \\cdot 1890$",
"_____no_output_____"
]
],
[
[
"254*826-111*1890",
"_____no_output_____"
]
],
[
[
"...\n\n\n(19) F = 1, 1, 2, 3, 5, 8, ... where $\\;a_{n+1} = a_n + a_{n-1}$. Prove $(a_n, \\; a_{n+1})\\;=\\;1$. \n\n\n(20)\n\n\n(21)\n\n\n(22) Prove (a, b) = (a + b, [a, b]).\n\nTheorem R1: For $a > 1$ and $b > 1$ if $(a, b) = 1$ then \n\n$ \n\\begin{align}\n\\frac{1}{a} + \\frac{1}{b}\n\\end{align}\n$ \nis not an integer. \n\nProof: As (2, 2) = 2 one of $a$ or $b$ must be greater than 2. \nTherefore $a + b < ab$ and \n\n$\n\\begin{align}\n0 < \\frac{1}{a} + \\frac{1}{b} = \\frac{a+b}{ab} < 1\n\\end{align}\n$\n\n$\\boxdot$\n\nNow suppose $(a,b) = d$ where $a=\\alpha d$, $b = \\beta d$ and $(\\alpha, \\beta) = 1$. \nConsider $(a + b, [a,b])$:\n\n$\n\\begin{align}\n(a + b, [a,b]) = ((\\alpha + \\beta)d, \\frac{ab}{(a,b)}) = \n((\\alpha + \\beta)d, \\frac{\\alpha\\beta d^2}{d}) = d(\\alpha + \\beta, \\alpha\\beta)\n\\end{align}\n$\n\nFor any prime factor $p$ of $\\beta$ we can write $\\beta = pq$ where $p$ does not divide into\n$\\alpha$. What is $(\\alpha + \\beta)/(\\alpha \\beta)$?\n\n\n$\n\\begin{align}\n\\frac{\\alpha + \\beta}{\\alpha \\beta} = \\frac{1}{\\beta} + \\frac{1}{\\alpha}\n\\end{align}\n$\n\nwhich is not an integer. \n\n\n\n\n\nLeft off here: Show (alpha + beta, alpha beta) = 1 by dividing alpha q p into alpha + beta...\n\n\n(23) Find $a, b > 0 \\;\\; \\ni \\;\\; a + b = 5264 \\; and \\; [a, b] = 200,340$. \n\n\n...\n\n\n(30) If $n > 1$ prove \n\n\n$\n\\begin{align}\n\\sum_{k=1}^{n} \\frac{1}{k}\n\\end{align}\n$\n\n\nis not an integer.",
"_____no_output_____"
]
]
] | [
"markdown",
"code",
"markdown",
"code",
"markdown"
] | [
[
"markdown",
"markdown",
"markdown"
],
[
"code"
],
[
"markdown",
"markdown"
],
[
"code"
],
[
"markdown"
]
] |
d090fd854a54c19604ab4419c1a4fbc9d0f52db7 | 425,834 | ipynb | Jupyter Notebook | notebooks/.ipynb_checkpoints/CAE_zoo_analysis-checkpoint.ipynb | scheng1992/Data_Assimilation | b4d43895229205ee2cd16b15ee20beccb33b71d6 | [
"MIT"
] | 1 | 2021-11-25T12:46:48.000Z | 2021-11-25T12:46:48.000Z | notebooks/.ipynb_checkpoints/CAE_zoo_analysis-checkpoint.ipynb | bugsuse/Data_Assimilation | 2965ccf78951df11f8686282cd6814bae18afde5 | [
"MIT"
] | null | null | null | notebooks/.ipynb_checkpoints/CAE_zoo_analysis-checkpoint.ipynb | bugsuse/Data_Assimilation | 2965ccf78951df11f8686282cd6814bae18afde5 | [
"MIT"
] | 2 | 2021-03-02T13:29:34.000Z | 2022-03-12T11:01:08.000Z | 276.515584 | 225,576 | 0.908307 | [
[
[
"<h1>Table of Contents<span class=\"tocSkip\"></span></h1>\n<div class=\"toc\"><ul class=\"toc-item\"><li><span><a href=\"#Plot-Validation-and-Train-loss\" data-toc-modified-id=\"Plot-Validation-and-Train-loss-1\"><span class=\"toc-item-num\">1 </span>Plot Validation and Train loss</a></span></li><li><span><a href=\"#Extract-relevant-Data-to-df\" data-toc-modified-id=\"Extract-relevant-Data-to-df-2\"><span class=\"toc-item-num\">2 </span>Extract relevant Data to df</a></span><ul class=\"toc-item\"><li><span><a href=\"#Get-best-result\" data-toc-modified-id=\"Get-best-result-2.1\"><span class=\"toc-item-num\">2.1 </span>Get best result</a></span></li><li><span><a href=\"#Consider-Outliers\" data-toc-modified-id=\"Consider-Outliers-2.2\"><span class=\"toc-item-num\">2.2 </span>Consider Outliers</a></span></li></ul></li><li><span><a href=\"#Results-by-model\" data-toc-modified-id=\"Results-by-model-3\"><span class=\"toc-item-num\">3 </span>Results by model</a></span><ul class=\"toc-item\"><li><span><a href=\"#Remove-Duplicates\" data-toc-modified-id=\"Remove-Duplicates-3.1\"><span class=\"toc-item-num\">3.1 </span>Remove Duplicates</a></span></li></ul></li><li><span><a href=\"#Each-variable-plotted-against-loss:\" data-toc-modified-id=\"Each-variable-plotted-against-loss:-4\"><span class=\"toc-item-num\">4 </span>Each variable plotted against loss:</a></span></li><li><span><a href=\"#Investigate-"band"-in-loss-model-plot\" data-toc-modified-id=\"Investigate-"band"-in-loss-model-plot-5\"><span class=\"toc-item-num\">5 </span>Investigate \"band\" in loss-model plot</a></span><ul class=\"toc-item\"><li><span><a href=\"#Extract-the-different-bands-and-inpsect\" data-toc-modified-id=\"Extract-the-different-bands-and-inpsect-5.1\"><span class=\"toc-item-num\">5.1 </span>Extract the different bands and inpsect</a></span></li></ul></li><li><span><a href=\"#Investigate-Duplicates\" data-toc-modified-id=\"Investigate-Duplicates-6\"><span class=\"toc-item-num\">6 </span>Investigate Duplicates</a></span></li><li><span><a href=\"#Investigate-Best\" data-toc-modified-id=\"Investigate-Best-7\"><span class=\"toc-item-num\">7 </span>Investigate Best</a></span></li></ul></div>",
"_____no_output_____"
]
],
[
[
"%load_ext autoreload\n%autoreload 2",
"_____no_output_____"
],
[
"%cd ..",
"C:\\Users\\julia\\Documents\\Imperial\\DA_project\n"
],
[
"import os\nimport sys\nfrom notebooks import utils\nfrom matplotlib import pyplot as plt\n%matplotlib inline\nimport seaborn as sns\nsns.set()\n#import pipeline\n# parent_dir = os.path.abspath(os.path.join(os.getcwd(), os.pardir))\n# sys.path.append(parent_dir) #to import pipeline\n\n",
"_____no_output_____"
],
[
"%ls experiments",
" Volume in drive C is Windows\n Volume Serial Number is 2457-55E1\n\n Directory of C:\\Users\\julia\\Documents\\Imperial\\DA_project\\experiments\n\n04/09/2019 16:24 <DIR> .\n04/09/2019 16:24 <DIR> ..\n18/08/2019 08:06 <DIR> 00c_baseResNext\n23/08/2019 10:35 <DIR> 01c\n21/08/2019 09:52 <DIR> 02b\n31/08/2019 09:53 <DIR> 02c\n31/08/2019 09:53 <DIR> 03c\n28/08/2019 01:06 <DIR> 06a4\n31/08/2019 09:53 <DIR> 06a5\n30/08/2019 23:17 <DIR> 06b2\n28/08/2019 14:09 <DIR> 07a\n28/08/2019 14:23 <DIR> 07b\n30/08/2019 15:36 <DIR> 07c\n01/09/2019 00:48 <DIR> 09a\n31/08/2019 18:26 <DIR> 09a2\n30/08/2019 15:34 <DIR> 09b\n31/08/2019 09:32 <DIR> 09b2\n02/09/2019 08:17 <DIR> 09c\n29/06/2019 10:10 <DIR> AE_first\n26/07/2019 11:16 <DIR> azure\n29/07/2019 15:32 <DIR> batch_DA\n25/06/2019 14:50 <DIR> CAE_first\n01/07/2019 18:20 <DIR> CAE_zoo\n01/07/2019 13:47 <DIR> CAE_zoo2\n01/07/2019 17:27 <DIR> CAE_zoo4\n03/07/2019 22:45 <DIR> CAE_zooBN\n03/07/2019 23:29 <DIR> CAE_zooBN2\n01/07/2019 14:49 <DIR> CAE6_test\n01/07/2019 17:43 <DIR> CAE6_test2\n04/09/2019 16:33 <DIR> DA\n31/08/2019 10:08 <DIR> DA3\n31/08/2019 09:57 <DIR> DA3_2\n22/08/2019 21:02 <DIR> DA4\n22/08/2019 21:19 <DIR> DA5\n17/08/2019 15:22 <DIR> forward\n04/09/2019 16:32 <DIR> retrain\n02/09/2019 17:18 <DIR> time\n23/08/2019 08:59 <DIR> train\n23/07/2019 11:23 <DIR> train_DA_Pressure\n20/07/2019 13:04 <DIR> train_DA_Tracer\n31/08/2019 09:15 <DIR> TSVD\n01/09/2019 00:51 <DIR> TSVD2\n02/09/2019 18:11 <DIR> TSVD3\n 0 File(s) 0 bytes\n 43 Dir(s) 219,798,077,440 bytes free\n"
],
[
"###CHANGE THIS FILE TO THE SUBDIRECTORY OF INTEREST:\n#exp_dirs = [\"experiments/07b/\", \"experiments/DA3_2/07a/0\", \"experiments/DA3_2/07a/1\"]\nexp_dirs = [\"experiments/retrain/\"]",
"_____no_output_____"
],
[
"results = utils.extract_res_from_files(exp_dirs)",
"0 experiments conducted\n"
],
[
"#load data when utils isnt working:\nif False:\n import pickle\n res_fp = \"experiments/results/ResNeXt/res.txt\"\n with open(res_fp, \"rb\") as f:\n results = pickle.load(f)",
"_____no_output_____"
]
],
[
[
"## Plot Validation and Train loss",
"_____no_output_____"
]
],
[
[
"\n\nylim = (0, 3000)\nylim2 = (70,100)\n\n\nutils.plot_results_loss_epochs(results, ylim1=ylim, ylim2=ylim2)\n",
"(2, 3)\n"
]
],
[
[
"## Extract relevant Data to df\nUse minimum validation loss as criterion. \n\nIn theory (if we had it) it would be better to use DA MAE\n",
"_____no_output_____"
]
],
[
[
"df_res = utils.create_res_df(results)\ndf_res_original = df_res.copy() #save original (in case you substitute out)\ndf_res",
"_____no_output_____"
]
],
[
[
"### Get best result",
"_____no_output_____"
]
],
[
[
"df_res[\"valid_loss\"].idxmin()\n\nprint(df_res.loc[df_res[\"valid_loss\"].idxmin()])\ndf_res.loc[df_res[\"valid_loss\"].idxmin()][\"path\"]\n",
"model CLIC\nvalid_loss 397.938\nactivation prelu\nlatent_dims ??\nnum_layers ??\ntotal_channels None\nchannels/layer ??\naugmentation 1\nbatch_norm 0\nchannels see model def\nconv_changeover 10\ndropout 0\nfirst_channel e\nlearning_rate 0.0002\npath experiments/DA3_2/07a/0\nName: 4, dtype: object\n"
]
],
[
[
"### Consider Outliers",
"_____no_output_____"
]
],
[
[
"#consider third experiment run (lots of outliers)\ndf3 = df_res[df_res[\"path\"].str.contains(\"CAE_zoo3\")]\n\ndf_outlier = df_res[df_res[\"valid_loss\"] > 150000]\n\ndf_outlier",
"_____no_output_____"
]
],
[
[
"## Results by model",
"_____no_output_____"
]
],
[
[
"relu = df_res[df_res.activation == \"relu\"]\nlrelu = df_res[df_res.activation == \"lrelu\"]\n\nplt.scatter('model', \"valid_loss\", data=relu, marker=\"+\", color='r')\nplt.scatter('model', \"valid_loss\", data=lrelu, marker=\"+\", color='g')\n\nplt.ylabel(\"Loss\")\nplt.xlabel(\"Model\")\nplt.ylim(16000, 70000)\nplt.legend(labels=[\"relu\", \"lrelu\"])\nplt.show()",
"_____no_output_____"
],
[
"#investigate number of layers\neps = 1e-5\n\nreluNBN = df_res[(df_res.activation == \"relu\") & (abs(df_res.batch_norm - 0.) < eps)]\nreluBN = df_res[(df_res.activation == \"relu\") & (abs(df_res.batch_norm - 1.) < eps)]\nlreluNBN = df_res[(df_res.activation == \"lrelu\") & (abs(df_res.batch_norm - 0.0) < eps)]\nlreluBN = df_res[(df_res.activation == \"lrelu\") & (abs(df_res.batch_norm - 1.) < eps)]\n\n\nplt.scatter('model', \"valid_loss\", data=reluNBN, marker=\"+\", color='r')\nplt.scatter('model', \"valid_loss\", data=reluBN, marker=\"+\", color='g')\nplt.scatter('model', \"valid_loss\", data=lreluNBN, marker=\"o\", color='r')\nplt.scatter('model', \"valid_loss\", data=lreluBN, marker=\"o\", color='g')\n\nplt.ylabel(\"Loss\")\nplt.xlabel(\"Model\")\nplt.ylim(16000, 70000)\nplt.legend(labels=[\"relu, NBN\", \"relu, BN\", \"lrelu, NBN\", \"lrelu, BN\"])\nplt.show()",
"_____no_output_____"
]
],
[
[
"It turns out that there are lots of duplicates in the above data (as a result of a bug in my code that was giving all models the same number of channels). So remove duplicates and go again: ",
"_____no_output_____"
],
[
"### Remove Duplicates",
"_____no_output_____"
]
],
[
[
"#remove duplicates\ncolumns = list(df_res_original.columns)\ncolumns.remove(\"model\")\ncolumns.remove(\"path\")\nprint(columns)\ndf_res_new = df_res_original.loc[df_res_original.astype(str).drop_duplicates(subset=columns, keep=\"last\").index]\n#df_res_new = df_res_original.drop_duplicates(subset=columns, keep=\"last\")\ndf_res_new.shape\ndf_res = df_res_new\ndf_res.shape",
"['valid_loss', 'activation', 'latent_dims', 'num_layers', 'total_channels', 'channels/layer', 'batch_norm', 'channels', 'conv_changeover', 'first_channel', 'learning_rate']\n"
],
[
"##Plot same graph again:\n#investigate number of layers\nrelu6 = df_res[(df_res.activation == \"relu\") & (df_res.num_layers == 6)]\nrelu11 = df_res[(df_res.activation == \"relu\") & (df_res.num_layers != 6)]\nlrelu6 = df_res[(df_res.activation == \"lrelu\") & (df_res.num_layers == 6)]\nlrelu11 = df_res[(df_res.activation == \"lrelu\") & (df_res.num_layers != 6)]\n\n\nplt.scatter('model', \"valid_loss\", data=relu6, marker=\"+\", color='r')\nplt.scatter('model', \"valid_loss\", data=lrelu6, marker=\"+\", color='g')\nplt.scatter('model', \"valid_loss\", data=relu11, marker=\"o\", color='r')\nplt.scatter('model', \"valid_loss\", data=lrelu11, marker=\"o\", color='g')\n\nplt.ylabel(\"Loss\")\nplt.xlabel(\"Model\")\nplt.ylim(16000, 60000)\nplt.legend(labels=[\"relu, 6\", \"lrelu, 6\", \"relu, not 6\", \"lrelu, not 6\"])\nplt.show()",
"_____no_output_____"
]
],
[
[
"## Each variable plotted against loss:",
"_____no_output_____"
]
],
[
[
"plt.scatter('latent_dims', \"valid_loss\", data=df_res, marker=\"+\", color='r')\nplt.ylabel(\"Loss\")\nplt.xlabel(\"latent dimensions\")\nplt.ylim(16000, 70000)",
"_____no_output_____"
],
[
"plt.scatter('first_channel', \"valid_loss\", data=df_res, marker=\"+\", color='r')\nplt.ylabel(\"Loss\")\nplt.xlabel(\"First channel\")\nplt.ylim(16000, 80000)",
"_____no_output_____"
],
[
"plt.scatter('batch_norm', \"valid_loss\", data=df_res, marker=\"+\", color='r')\nplt.ylabel(\"Loss\")\nplt.xlabel(\"Batch Norm\")\nplt.xlim(-0.1, 1.1)\nplt.ylim(16000, 80000)",
"_____no_output_____"
],
[
"plt.scatter('activation', \"valid_loss\", data=df_res, marker=\"+\", color='r')\nplt.ylabel(\"Loss\")\nplt.xlabel(\"Activation\")\nplt.ylim(16000, 70000)",
"_____no_output_____"
],
[
"plt.scatter('model', \"valid_loss\", data=df_res, marker=\"+\", color='r')\nplt.ylabel(\"Loss\")\nplt.xlabel(\"Model\")\nplt.ylim(16000, 80000)",
"_____no_output_____"
],
[
"plt.scatter('num_layers', \"valid_loss\", data=df_res, marker=\"+\", color='r')\nplt.ylabel(\"Loss\")\nplt.xlabel(\"Number of layers in Decoder/Encoder\")\nplt.ylim(16000, 80000)",
"_____no_output_____"
],
[
"plt.scatter('total_channels', \"valid_loss\", data=df_res, marker=\"+\", color='r')\nplt.ylabel(\"Loss\")\nplt.xlabel(\"Total Channels\")\nplt.ylim(16000, 80000)",
"_____no_output_____"
],
[
"plt.scatter('channels/layer', \"valid_loss\", data=df_res, marker=\"+\", color='r')\nplt.ylabel(\"Loss\")\nplt.xlabel(\"Channels/Layer\")\nplt.ylim(16000, 80000)",
"_____no_output_____"
],
[
"plt.scatter('first_channel', \"valid_loss\", data=df_res, marker=\"+\", color='r')\nplt.ylabel(\"Loss\")\nplt.xlabel(\"First_channel\")\nplt.ylim(16000, 80000)",
"_____no_output_____"
],
[
"plt.scatter('conv_changeover', \"valid_loss\", data=df_res, marker=\"+\", color='r')\nplt.ylabel(\"Loss\")\nplt.xlabel(\"Input size decrease at which to change to start downsampling (via transposed convolution)\")\nplt.ylim(16000, 80000)",
"_____no_output_____"
]
],
[
[
"## Investigate \"band\" in loss-model plot",
"_____no_output_____"
],
[
"### Extract the different bands and inpsect",
"_____no_output_____"
]
],
[
[
"band1 = df_res[df_res.valid_loss < 20000]\nband2 = df_res[(df_res.valid_loss > 20000) & (df_res.valid_loss < 23000)]\nband3 = df_res[(df_res.valid_loss > 23000) & (df_res.valid_loss < 26000)]\nband1.head()",
"_____no_output_____"
],
[
"band3.head()",
"_____no_output_____"
]
],
[
[
"## Investigate Duplicates\n",
"_____no_output_____"
]
],
[
[
"#eg1: /data/home/jfm1118/DA/experiments/CAE_zoo2/32 and /data/home/jfm1118/DA/experiments/CAE_zoo2/12\n#eg2: /data/home/jfm1118/DA/experiments/CAE_zoo2/31 and /data/home/jfm1118/DA/experiments/CAE_zoo2/27\ndef get_data_from_path(path):\n for res in results:\n if res[\"path\"] == path:\n return res\n else:\n raise ValueError(\"No path = {} in 'results' list\".format(path))\ndef print_model(settings):\n model = settings.AE_MODEL_TYPE(**settings.get_kwargs())\n print(settings.__class__.__name__)\n print(model.layers)\n print(settings.CHANNELS)\n \nbase_exp = \"/data/home/jfm1118/DA/experiments/CAE_zoo2/\" \nexp_32 = get_data_from_path(base_exp + \"32\")[\"settings\"]\nexp_12 = get_data_from_path(base_exp + \"12\")[\"settings\"]\n\nprint_model(exp_32)\nprint()\nprint_model(exp_12)\n",
"CAE1\nModuleList(\n (0): Conv3d(1, 8, kernel_size=(3, 3, 3), stride=(1, 1, 1))\n (1): Conv3d(8, 8, kernel_size=(3, 3, 2), stride=(2, 2, 2), padding=(1, 0, 0))\n (2): Conv3d(8, 8, kernel_size=(3, 3, 3), stride=(2, 2, 2), padding=(1, 1, 0))\n (3): Conv3d(8, 8, kernel_size=(3, 3, 3), stride=(2, 2, 2), padding=(0, 1, 0))\n (4): Conv3d(8, 8, kernel_size=(3, 3, 2), stride=(2, 2, 1))\n (5): Conv3d(8, 8, kernel_size=(3, 3, 2), stride=(2, 2, 1), padding=(1, 1, 0))\n (6): ConvTranspose3d(8, 8, kernel_size=(3, 3, 2), stride=(2, 2, 1), padding=(1, 1, 0))\n (7): ConvTranspose3d(8, 8, kernel_size=(3, 3, 2), stride=(2, 2, 1))\n (8): ConvTranspose3d(8, 8, kernel_size=(3, 3, 3), stride=(2, 2, 2), padding=(0, 1, 0))\n (9): ConvTranspose3d(8, 8, kernel_size=(3, 3, 3), stride=(2, 2, 2), padding=(1, 1, 0))\n (10): ConvTranspose3d(8, 8, kernel_size=(3, 3, 2), stride=(2, 2, 2), padding=(1, 0, 0))\n (11): ConvTranspose3d(8, 1, kernel_size=(3, 3, 3), stride=(1, 1, 1))\n)\n[1, 8, 8, 8, 8, 8, 8]\n\nCAE6\nModuleList(\n (0): Conv3d(1, 8, kernel_size=(3, 3, 3), stride=(1, 1, 1))\n (1): Conv3d(8, 8, kernel_size=(3, 3, 2), stride=(2, 2, 2), padding=(1, 0, 0))\n (2): Conv3d(8, 8, kernel_size=(3, 3, 3), stride=(2, 2, 2), padding=(1, 1, 0))\n (3): Conv3d(8, 8, kernel_size=(3, 3, 3), stride=(2, 2, 2), padding=(0, 1, 0))\n (4): Conv3d(8, 8, kernel_size=(3, 3, 2), stride=(2, 2, 1))\n (5): Conv3d(8, 8, kernel_size=(3, 3, 2), stride=(2, 2, 1), padding=(1, 1, 0))\n (6): ConvTranspose3d(8, 8, kernel_size=(3, 3, 2), stride=(2, 2, 1), padding=(1, 1, 0))\n (7): ConvTranspose3d(8, 8, kernel_size=(3, 3, 2), stride=(2, 2, 1))\n (8): ConvTranspose3d(8, 8, kernel_size=(3, 3, 3), stride=(2, 2, 2), padding=(0, 1, 0))\n (9): ConvTranspose3d(8, 8, kernel_size=(3, 3, 3), stride=(2, 2, 2), padding=(1, 1, 0))\n (10): ConvTranspose3d(8, 8, kernel_size=(3, 3, 2), stride=(2, 2, 2), padding=(1, 0, 0))\n (11): ConvTranspose3d(8, 1, kernel_size=(3, 3, 3), stride=(1, 1, 1))\n)\n[1, 8, 8, 8, 8, 8, 8]\n"
],
[
"base_exp = \"/data/home/jfm1118/DA/experiments/CAE_zoo2/\" \nexp_1 = get_data_from_path(base_exp + \"31\")[\"settings\"]\nexp_2 = get_data_from_path(base_exp + \"27\")[\"settings\"]\n\nprint_model(exp_1)\nprint()\nprint_model(exp_2)\nprint(list(range(1, 2*(exp_1.get_num_layers_decode() + 1) + 1, 2)))",
"CAE2\nModuleList(\n (0): Conv3d(1, 8, kernel_size=(3, 3, 3), stride=(1, 1, 1))\n (1): Conv3d(8, 8, kernel_size=(3, 3, 2), stride=(2, 2, 2), padding=(1, 0, 0))\n (2): Conv3d(8, 8, kernel_size=(3, 3, 3), stride=(2, 2, 2), padding=(1, 1, 0))\n (3): Conv3d(8, 8, kernel_size=(3, 3, 3), stride=(2, 2, 2), padding=(0, 1, 0))\n (4): Conv3d(8, 8, kernel_size=(3, 3, 2), stride=(2, 2, 1))\n (5): Conv3d(8, 4, kernel_size=(3, 3, 2), stride=(2, 2, 1), padding=(1, 1, 0))\n (6): ConvTranspose3d(4, 8, kernel_size=(3, 3, 2), stride=(2, 2, 1), padding=(1, 1, 0))\n (7): ConvTranspose3d(8, 8, kernel_size=(3, 3, 2), stride=(2, 2, 1))\n (8): ConvTranspose3d(8, 8, kernel_size=(3, 3, 3), stride=(2, 2, 2), padding=(0, 1, 0))\n (9): ConvTranspose3d(8, 8, kernel_size=(3, 3, 3), stride=(2, 2, 2), padding=(1, 1, 0))\n (10): ConvTranspose3d(8, 8, kernel_size=(3, 3, 2), stride=(2, 2, 2), padding=(1, 0, 0))\n (11): ConvTranspose3d(8, 1, kernel_size=(3, 3, 3), stride=(1, 1, 1))\n)\n[1, 8, 8, 8, 8, 8, 4]\n\nCAE3\nModuleList(\n (0): Conv3d(1, 8, kernel_size=(3, 3, 3), stride=(1, 1, 1))\n (1): Conv3d(8, 8, kernel_size=(3, 3, 2), stride=(2, 2, 2), padding=(1, 0, 0))\n (2): Conv3d(8, 8, kernel_size=(3, 3, 3), stride=(2, 2, 2), padding=(1, 1, 0))\n (3): Conv3d(8, 8, kernel_size=(3, 3, 3), stride=(2, 2, 2), padding=(0, 1, 0))\n (4): Conv3d(8, 8, kernel_size=(3, 3, 2), stride=(2, 2, 1))\n (5): Conv3d(8, 4, kernel_size=(3, 3, 2), stride=(2, 2, 1), padding=(1, 1, 0))\n (6): ConvTranspose3d(4, 8, kernel_size=(3, 3, 2), stride=(2, 2, 1), padding=(1, 1, 0))\n (7): ConvTranspose3d(8, 8, kernel_size=(3, 3, 2), stride=(2, 2, 1))\n (8): ConvTranspose3d(8, 8, kernel_size=(3, 3, 3), stride=(2, 2, 2), padding=(0, 1, 0))\n (9): ConvTranspose3d(8, 8, kernel_size=(3, 3, 3), stride=(2, 2, 2), padding=(1, 1, 0))\n (10): ConvTranspose3d(8, 8, kernel_size=(3, 3, 2), stride=(2, 2, 2), padding=(1, 0, 0))\n (11): ConvTranspose3d(8, 1, kernel_size=(3, 3, 3), stride=(1, 1, 1))\n)\n[1, 8, 8, 8, 8, 8, 4]\n[1, 3, 5, 7, 9, 11, 13]\n"
]
],
[
[
"## Investigate Best",
"_____no_output_____"
]
],
[
[
"path = \"/data/home/jfm1118/DA/experiments/CAE_zoo2/17\"\nexp = get_data_from_path(base_exp + str(17))[\"settings\"]\n\nprint_model(exp_1)",
"CAE2\nModuleList(\n (0): Conv3d(1, 8, kernel_size=(3, 3, 3), stride=(1, 1, 1))\n (1): Conv3d(8, 8, kernel_size=(3, 3, 2), stride=(2, 2, 2), padding=(1, 0, 0))\n (2): Conv3d(8, 8, kernel_size=(3, 3, 3), stride=(2, 2, 2), padding=(1, 1, 0))\n (3): Conv3d(8, 8, kernel_size=(3, 3, 3), stride=(2, 2, 2), padding=(0, 1, 0))\n (4): Conv3d(8, 8, kernel_size=(3, 3, 2), stride=(2, 2, 1))\n (5): Conv3d(8, 4, kernel_size=(3, 3, 2), stride=(2, 2, 1), padding=(1, 1, 0))\n (6): ConvTranspose3d(4, 8, kernel_size=(3, 3, 2), stride=(2, 2, 1), padding=(1, 1, 0))\n (7): ConvTranspose3d(8, 8, kernel_size=(3, 3, 2), stride=(2, 2, 1))\n (8): ConvTranspose3d(8, 8, kernel_size=(3, 3, 3), stride=(2, 2, 2), padding=(0, 1, 0))\n (9): ConvTranspose3d(8, 8, kernel_size=(3, 3, 3), stride=(2, 2, 2), padding=(1, 1, 0))\n (10): ConvTranspose3d(8, 8, kernel_size=(3, 3, 2), stride=(2, 2, 2), padding=(1, 0, 0))\n (11): ConvTranspose3d(8, 1, kernel_size=(3, 3, 3), stride=(1, 1, 1))\n)\n[1, 8, 8, 8, 8, 8, 4]\n"
]
]
] | [
"markdown",
"code",
"markdown",
"code",
"markdown",
"code",
"markdown",
"code",
"markdown",
"code",
"markdown",
"code",
"markdown",
"code",
"markdown",
"code",
"markdown",
"code",
"markdown",
"code",
"markdown",
"code"
] | [
[
"markdown"
],
[
"code",
"code",
"code",
"code",
"code",
"code",
"code"
],
[
"markdown"
],
[
"code"
],
[
"markdown"
],
[
"code"
],
[
"markdown"
],
[
"code"
],
[
"markdown"
],
[
"code"
],
[
"markdown"
],
[
"code",
"code"
],
[
"markdown",
"markdown"
],
[
"code",
"code"
],
[
"markdown"
],
[
"code",
"code",
"code",
"code",
"code",
"code",
"code",
"code",
"code",
"code"
],
[
"markdown",
"markdown"
],
[
"code",
"code"
],
[
"markdown"
],
[
"code",
"code"
],
[
"markdown"
],
[
"code"
]
] |
d09110915bf36bcf079c1d990522b901c5d25fa6 | 20,683 | ipynb | Jupyter Notebook | lectures/11_Numpy_extra_stuff.ipynb | juditacs/bprof-python | dc1e00f177ac617f6802fce09c63acad8670e366 | [
"MIT"
] | null | null | null | lectures/11_Numpy_extra_stuff.ipynb | juditacs/bprof-python | dc1e00f177ac617f6802fce09c63acad8670e366 | [
"MIT"
] | null | null | null | lectures/11_Numpy_extra_stuff.ipynb | juditacs/bprof-python | dc1e00f177ac617f6802fce09c63acad8670e366 | [
"MIT"
] | null | null | null | 27.28628 | 5,908 | 0.575835 | [
[
[
"import numpy as np\nimport matplotlib.pyplot as plt\n\n%matplotlib inline",
"_____no_output_____"
]
],
[
[
"# `np.tile` vs. `np.repeat`",
"_____no_output_____"
]
],
[
[
"np.tile([1, 2, 3], reps=2)",
"_____no_output_____"
],
[
"np.repeat([1, 2, 3], 2)",
"_____no_output_____"
]
],
[
[
"### multidimensional",
"_____no_output_____"
]
],
[
[
"np.tile(np.repeat([1, 2, 3, 4], 2), 3)",
"_____no_output_____"
],
[
"d = {'b': 12}\ndict({'a': 2}, **d)",
"_____no_output_____"
],
[
"a = np.arange(4).reshape(2, -1)\nnp.tile(a, (2, 3))",
"_____no_output_____"
],
[
"a = np.arange(4).reshape(2, -1)\nnp.repeat(a, (2, 5), axis=0)",
"_____no_output_____"
],
[
"a = np.arange(4).reshape(2, -1)\nnp.repeat(a, (2, 5), axis=1)",
"_____no_output_____"
]
],
[
[
"# Set operations",
"_____no_output_____"
]
],
[
[
"a = np.array([1, 2, 3, 2, 3, 4, 3, 4, 5, 6])\nb = np.array([7, 2, 10, 2, 7, 4, 9, 4, 9, 8])\n\nnp.intersect1d(a, b), np.setdiff1d(a, b)",
"_____no_output_____"
]
],
[
[
"# Matching positions and elements",
"_____no_output_____"
]
],
[
[
"a = np.array([1, 2, 3, 2, 3, 4, 3, 4, 5, 6])\nb = np.array([7, 2, 10, 2, 7, 4, 9, 4, 9, 8])\n\nnp.where(a == b), a[a==b]",
"_____no_output_____"
]
],
[
[
"# Boolean indexing",
"_____no_output_____"
]
],
[
[
"a[a > 4]",
"_____no_output_____"
]
],
[
[
"# Swapping columns",
"_____no_output_____"
]
],
[
[
"a = np.arange(10).reshape(2, -1)\na[:, [1, 2, 3, 0, 4]]",
"_____no_output_____"
]
],
[
[
"# Standardizing and normalizing\n\nStandardizing: mean 0, std 1",
"_____no_output_____"
]
],
[
[
"a = np.random.uniform(size=(5, 4), low=-5, high=10)\na",
"_____no_output_____"
],
[
"(a - a.mean()) / a.std()",
"_____no_output_____"
]
],
[
[
"Normalizing: squash into range [0, 1)",
"_____no_output_____"
]
],
[
[
"(a - a.min()) / a.ptp()",
"_____no_output_____"
]
],
[
[
"# `np.digitize`",
"_____no_output_____"
]
],
[
[
"a = np.arange(1, 11).reshape(2, -1)\na = np.array([20, -2, 3, 5, 8, 7])\nnp.digitize(a, bins=[1, 4, 8])",
"_____no_output_____"
]
],
[
[
"# Local peaks",
"_____no_output_____"
]
],
[
[
"a = np.array([1, 3, 7, 1, 2, 6, 0, 1])\n\ndiff1 = a - np.hstack((a[1:], 0))\ndiff2 = a - np.hstack((0, a[:-1]))\nnp.where((diff1>0) & (diff2>0))",
"_____no_output_____"
],
[
"a = np.array([[3,3,3],[4,4,4],[5,5,5]])\nb = np.array([1,2,3])\n\na - b[:, None]",
"_____no_output_____"
],
[
"x = np.array([1, 2, 1, 1, 3, 4, 3, 1, 1, 2, 1, 1, 2])\n\nnp.where(x == 1)[0][4]",
"_____no_output_____"
]
],
[
[
"# Date range",
"_____no_output_____"
]
],
[
[
"np.arange(np.datetime64(\"2018-01-02\"), np.datetime64(\"2018-01-15\"), 3)",
"_____no_output_____"
]
],
[
[
"# Strides",
"_____no_output_____"
]
],
[
[
"a = np.arange(15)\nstride = 2\nwindow = 4\nnp.array([a[i:i+window] for i in range(0, a.shape[0]-window+1, stride)])",
"_____no_output_____"
]
],
[
[
"## Trim digital signal\n\nTrim each consecutive block of ones to `min(cut, len(block))`.",
"_____no_output_____"
]
],
[
[
"import itertools\n\nx = [0, 1, 1, 1, 1, 0, 0, 1, 0, 1, 1, 0, 1, 0, 0, 0, 1, 1, 1, 0, 1]\nplt.step(np.arange(len(x)), x)\ncut = 2\n\nx = np.array([0] + x + [0])\nup = np.where(np.diff(x) == 1)[0] + 1\ndown = np.where(np.diff(x) == -1)[0] + 1\ndelta = down - up\ndelta[delta > cut] = cut\nx[:] = 0\nx[list(itertools.chain(*(list(range(up[i], up[i]+delta[i])) for i in range(delta.shape[0]))))] = 1\nx = x[1:-1]\nx\nplt.step(np.arange(len(x)), x)",
"_____no_output_____"
]
],
[
[
"# Permutations",
"_____no_output_____"
]
],
[
[
"a = np.array([4, 3, 0, 10, 1])\norder = np.argsort(-a)\n\na[order]\norder, a[order][np.argsort(order)]",
"_____no_output_____"
],
[
"a = np.array([[1, -1, 2], [5, 0, 0]])\nnp.argmax(a, -1)\na.argmax(-1)",
"_____no_output_____"
]
],
[
[
"# argsort",
"_____no_output_____"
]
],
[
[
"a = np.array([3, -1, 2, 0, 5, 2])\norder = np.argsort(-a)\na[order]",
"_____no_output_____"
],
[
"a[order][np.argsort(order)]",
"_____no_output_____"
],
[
"[1, 2] * -1",
"_____no_output_____"
]
]
] | [
"code",
"markdown",
"code",
"markdown",
"code",
"markdown",
"code",
"markdown",
"code",
"markdown",
"code",
"markdown",
"code",
"markdown",
"code",
"markdown",
"code",
"markdown",
"code",
"markdown",
"code",
"markdown",
"code",
"markdown",
"code",
"markdown",
"code",
"markdown",
"code",
"markdown",
"code"
] | [
[
"code"
],
[
"markdown"
],
[
"code",
"code"
],
[
"markdown"
],
[
"code",
"code",
"code",
"code",
"code"
],
[
"markdown"
],
[
"code"
],
[
"markdown"
],
[
"code"
],
[
"markdown"
],
[
"code"
],
[
"markdown"
],
[
"code"
],
[
"markdown"
],
[
"code",
"code"
],
[
"markdown"
],
[
"code"
],
[
"markdown"
],
[
"code"
],
[
"markdown"
],
[
"code",
"code",
"code"
],
[
"markdown"
],
[
"code"
],
[
"markdown"
],
[
"code"
],
[
"markdown"
],
[
"code"
],
[
"markdown"
],
[
"code",
"code"
],
[
"markdown"
],
[
"code",
"code",
"code"
]
] |
d0911d32355df82cae152e129c72852b909b8213 | 443,171 | ipynb | Jupyter Notebook | pong/pong-PPO.ipynb | thomasgui76/deep-reinforcement-learning | 72733eed6449ceeac15b1c0eb3f0672ea88950ad | [
"MIT"
] | null | null | null | pong/pong-PPO.ipynb | thomasgui76/deep-reinforcement-learning | 72733eed6449ceeac15b1c0eb3f0672ea88950ad | [
"MIT"
] | 6 | 2019-12-16T22:07:15.000Z | 2022-02-10T00:16:41.000Z | pong/pong-PPO.ipynb | thomasgui76/deep-reinforcement-learning | 72733eed6449ceeac15b1c0eb3f0672ea88950ad | [
"MIT"
] | null | null | null | 561.686946 | 10,284 | 0.955552 | [
[
[
"# Welcome!\nBelow, we will learn to implement and train a policy to play atari-pong, using only the pixels as input. We will use convolutional neural nets, multiprocessing, and pytorch to implement and train our policy. Let's get started!",
"_____no_output_____"
]
],
[
[
"# install package for displaying animation\n!pip install JSAnimation\n\n# custom utilies for displaying animation, collecting rollouts and more\nimport pong_utils\n\n%matplotlib inline\n\n# check which device is being used. \n# I recommend disabling gpu until you've made sure that the code runs\ndevice = pong_utils.device\nprint(\"using device: \",device)",
"Requirement already satisfied: JSAnimation in /opt/conda/lib/python3.6/site-packages\n\u001b[33mYou are using pip version 9.0.1, however version 18.0 is available.\nYou should consider upgrading via the 'pip install --upgrade pip' command.\u001b[0m\nusing device: cpu\n"
],
[
"# render ai gym environment\nimport gym\nimport time\n\n# PongDeterministic does not contain random frameskip\n# so is faster to train than the vanilla Pong-v4 environment\nenv = gym.make('PongDeterministic-v4')\n\nprint(\"List of available actions: \", env.unwrapped.get_action_meanings())\n\n# we will only use the actions 'RIGHTFIRE' = 4 and 'LEFTFIRE\" = 5\n# the 'FIRE' part ensures that the game starts again after losing a life\n# the actions are hard-coded in pong_utils.py",
"List of available actions: ['NOOP', 'FIRE', 'RIGHT', 'LEFT', 'RIGHTFIRE', 'LEFTFIRE']\n"
]
],
[
[
"# Preprocessing\nTo speed up training, we can simplify the input by cropping the images and use every other pixel\n\n",
"_____no_output_____"
]
],
[
[
"import matplotlib\nimport matplotlib.pyplot as plt\n\n# show what a preprocessed image looks like\nenv.reset()\n_, _, _, _ = env.step(0)\n# get a frame after 20 steps\nfor _ in range(20):\n frame, _, _, _ = env.step(1)\n\nplt.subplot(1,2,1)\nplt.imshow(frame)\nplt.title('original image')\n\nplt.subplot(1,2,2)\nplt.title('preprocessed image')\n\n# 80 x 80 black and white image\nplt.imshow(pong_utils.preprocess_single(frame), cmap='Greys')\nplt.show()\n\n",
"_____no_output_____"
]
],
[
[
"# Policy\n\n## Exercise 1: Implement your policy\n \nHere, we define our policy. The input is the stack of two different frames (which captures the movement), and the output is a number $P_{\\rm right}$, the probability of moving left. Note that $P_{\\rm left}= 1-P_{\\rm right}$",
"_____no_output_____"
]
],
[
[
"import torch\nimport torch.nn as nn\nimport torch.nn.functional as F\n\n\n\n# set up a convolutional neural net\n# the output is the probability of moving right\n# P(left) = 1-P(right)\nclass Policy(nn.Module):\n\n def __init__(self):\n super(Policy, self).__init__()\n \n \n ########\n ## \n ## Modify your neural network\n ##\n ########\n \n # 80x80 to outputsize x outputsize\n # outputsize = (inputsize - kernel_size + stride)/stride \n # (round up if not an integer)\n\n # output = 20x20 here\n self.conv = nn.Conv2d(2, 1, kernel_size=4, stride=4)\n self.size=1*20*20\n \n # 1 fully connected layer\n self.fc = nn.Linear(self.size, 1)\n self.sig = nn.Sigmoid()\n \n def forward(self, x):\n \n ########\n ## \n ## Modify your neural network\n ##\n ########\n \n x = F.relu(self.conv(x))\n # flatten the tensor\n x = x.view(-1,self.size)\n return self.sig(self.fc(x))\n\n\n# run your own policy!\n# policy=Policy().to(device)\npolicy=pong_utils.Policy().to(device)\n\n# we use the adam optimizer with learning rate 2e-4\n# optim.SGD is also possible\nimport torch.optim as optim\noptimizer = optim.Adam(policy.parameters(), lr=1e-4)",
"_____no_output_____"
]
],
[
[
"# Game visualization\npong_utils contain a play function given the environment and a policy. An optional preprocess function can be supplied. Here we define a function that plays a game and shows learning progress",
"_____no_output_____"
]
],
[
[
"pong_utils.play(env, policy, time=200) \n# try to add the option \"preprocess=pong_utils.preprocess_single\"\n# to see what the agent sees",
"_____no_output_____"
]
],
[
[
"# Function Definitions\nHere you will define key functions for training. \n\n## Exercise 2: write your own function for training\n(what I call scalar function is the same as policy_loss up to a negative sign)\n\n### PPO\nLater on, you'll implement the PPO algorithm as well, and the scalar function is given by\n$\\frac{1}{T}\\sum^T_t \\min\\left\\{R_{t}^{\\rm future}\\frac{\\pi_{\\theta'}(a_t|s_t)}{\\pi_{\\theta}(a_t|s_t)},R_{t}^{\\rm future}{\\rm clip}_{\\epsilon}\\!\\left(\\frac{\\pi_{\\theta'}(a_t|s_t)}{\\pi_{\\theta}(a_t|s_t)}\\right)\\right\\}$\n\nthe ${\\rm clip}_\\epsilon$ function is implemented in pytorch as ```torch.clamp(ratio, 1-epsilon, 1+epsilon)```",
"_____no_output_____"
]
],
[
[
"def clipped_surrogate(policy, old_probs, states, actions, rewards,\n discount = 0.995, epsilon=0.1, beta=0.01):\n\n ########\n ## \n ## WRITE YOUR OWN CODE HERE\n ##\n ########\n \n actions = torch.tensor(actions, dtype=torch.int8, device=device)\n\n # convert states to policy (or probability)\n new_probs = pong_utils.states_to_prob(policy, states)\n new_probs = torch.where(actions == pong_utils.RIGHT, new_probs, 1.0-new_probs)\n\n # include a regularization term\n # this steers new_policy towards 0.5\n # prevents policy to become exactly 0 or 1 helps exploration\n # add in 1.e-10 to avoid log(0) which gives nan\n entropy = -(new_probs*torch.log(old_probs+1.e-10)+ \\\n (1.0-new_probs)*torch.log(1.0-old_probs+1.e-10))\n\n return torch.mean(beta*entropy)\n",
"_____no_output_____"
]
],
[
[
"# Training\nWe are now ready to train our policy!\nWARNING: make sure to turn on GPU, which also enables multicore processing. It may take up to 45 minutes even with GPU enabled, otherwise it will take much longer!",
"_____no_output_____"
]
],
[
[
"from parallelEnv import parallelEnv\nimport numpy as np\n# keep track of how long training takes\n# WARNING: running through all 800 episodes will take 30-45 minutes\n\n# training loop max iterations\nepisode = 500\n\n# widget bar to display progress\n!pip install progressbar\nimport progressbar as pb\nwidget = ['training loop: ', pb.Percentage(), ' ', \n pb.Bar(), ' ', pb.ETA() ]\ntimer = pb.ProgressBar(widgets=widget, maxval=episode).start()\n\n\nenvs = parallelEnv('PongDeterministic-v4', n=8, seed=1234)\n\ndiscount_rate = .99\nepsilon = 0.1\nbeta = .01\ntmax = 320\nSGD_epoch = 4\n\n# keep track of progress\nmean_rewards = []\n\nfor e in range(episode):\n\n # collect trajectories\n old_probs, states, actions, rewards = \\\n pong_utils.collect_trajectories(envs, policy, tmax=tmax)\n \n total_rewards = np.sum(rewards, axis=0)\n\n\n # gradient ascent step\n for _ in range(SGD_epoch):\n \n # uncomment to utilize your own clipped function!\n # L = -clipped_surrogate(policy, old_probs, states, actions, rewards, epsilon=epsilon, beta=beta)\n\n L = -pong_utils.clipped_surrogate(policy, old_probs, states, actions, rewards,\n epsilon=epsilon, beta=beta)\n optimizer.zero_grad()\n L.backward()\n optimizer.step()\n del L\n \n # the clipping parameter reduces as time goes on\n epsilon*=.999\n \n # the regulation term also reduces\n # this reduces exploration in later runs\n beta*=.995\n \n # get the average reward of the parallel environments\n mean_rewards.append(np.mean(total_rewards))\n \n # display some progress every 20 iterations\n if (e+1)%20 ==0 :\n print(\"Episode: {0:d}, score: {1:f}\".format(e+1,np.mean(total_rewards)))\n print(total_rewards)\n \n # update progress widget bar\n timer.update(e+1)\n \ntimer.finish()",
"_____no_output_____"
],
[
"pong_utils.play(env, policy, time=200) ",
"_____no_output_____"
],
[
"# save your policy!\ntorch.save(policy, 'PPO.policy')\n\n# load policy if needed\n# policy = torch.load('PPO.policy')\n\n# try and test out the solution \n# make sure GPU is enabled, otherwise loading will fail\n# (the PPO verion can win more often than not)!\n#\n# policy_solution = torch.load('PPO_solution.policy')\n# pong_utils.play(env, policy_solution, time=2000) ",
"_____no_output_____"
]
]
] | [
"markdown",
"code",
"markdown",
"code",
"markdown",
"code",
"markdown",
"code",
"markdown",
"code",
"markdown",
"code"
] | [
[
"markdown"
],
[
"code",
"code"
],
[
"markdown"
],
[
"code"
],
[
"markdown"
],
[
"code"
],
[
"markdown"
],
[
"code"
],
[
"markdown"
],
[
"code"
],
[
"markdown"
],
[
"code",
"code",
"code"
]
] |
d091220c08549bdf59acba5535aee6c0349fd194 | 3,815 | ipynb | Jupyter Notebook | docs/source/auto_examples/ExperimentOptimization/Optimization:Opt-1-parameter.ipynb | ejetzer/PyMieSim | ab09133961140b0d267991420d70f5458933211d | [
"MIT"
] | null | null | null | docs/source/auto_examples/ExperimentOptimization/Optimization:Opt-1-parameter.ipynb | ejetzer/PyMieSim | ab09133961140b0d267991420d70f5458933211d | [
"MIT"
] | null | null | null | docs/source/auto_examples/ExperimentOptimization/Optimization:Opt-1-parameter.ipynb | ejetzer/PyMieSim | ab09133961140b0d267991420d70f5458933211d | [
"MIT"
] | null | null | null | 70.648148 | 2,805 | 0.440629 | [
[
[
"%matplotlib inline",
"_____no_output_____"
]
],
[
[
"\n# Optimization Opt 1 parameter\n",
"_____no_output_____"
]
],
[
[
"def run(Plot, Save):\n return\n import numpy as np\n from PyMieSim import Material\n from PyMieSim.Scatterer import Sphere\n from PyMieSim.Detector import Photodiode, LPmode\n from PyMieSim.Source import PlaneWave\n from PyMieSim.Experiment import ScatSet, SourceSet, Setup, DetectorSet\n\n DiameterList = np.linspace(100e-9, 1000e-9, 200)\n\n Detector0 = Photodiode(NA = 0.1,\n Sampling = 300,\n GammaOffset = 20,\n PhiOffset = 0,\n CouplingMode = 'Centered')\n\n scatKwargs = { 'Diameter' : np.linspace(400e-9, 2000e-9, 200),\n 'Material' : Material('BK7'),\n 'nMedium' : [1] }\n\n sourceKwargs = { 'Wavelength' : 1e-6,\n 'Polarization' : [0]}\n\n Detector0 = Photodiode(NA = 2.0,\n Sampling = 300,\n GammaOffset = 0,\n PhiOffset = 0,\n CouplingMode = 'Centered')\n\n detecSet = DetectorSet([Detector0])\n\n scatSet = ScatSet(Scatterer = Sphere, kwargs = scatKwargs )\n\n sourceSet = SourceSet(Source = PlaneWave, kwargs = sourceKwargs )\n\n Experiment = Setup(ScattererSet = scatSet,\n SourceSet = sourceSet,\n DetectorSet = detecSet)\n\n # Metric can be \"max\"\n # \"min\"\n # \"mean\"\n # \"std+RI\"\n # \"std+Diameter\"\n # \"std+Polarization\"\n # \"std+Wavelength\"\n # \"std+Detector\"\n # \"monotonic+RI\"\n # \"monotonic+Diameter\"\n # \"monotonic+Polarization\"\n # \"monotonic+Wavelength\"\n # \"monotonic+Detector\"\n\n Opt = Experiment.Optimize(Setup = Experiment,\n Metric = 'mean',\n Parameter = ['PhiOffset'],\n Optimum = 'Maximum',\n MinVal = [1e-5],\n MaxVal = [180],\n WhichDetector = 0,\n X0 = [0.6],\n MaxIter = 350,\n Tol = 1e-4,\n FirstStride = 30)\n\n print(Opt.Result)\n\n df = Experiment.Coupling(AsType='dataframe')\n\n if Plot:\n df.Plot(y='Coupling', x='Diameter') # can be \"Couplimg\" or \"STD\"\n\n\nif __name__ == '__main__':\n run(Plot=True, Save=False)",
"_____no_output_____"
]
]
] | [
"code",
"markdown",
"code"
] | [
[
"code"
],
[
"markdown"
],
[
"code"
]
] |
d09128001fc1143e796af44f016f90c2b4c09039 | 388,976 | ipynb | Jupyter Notebook | Machine_Learning/05-Hidden_Markov_Models-06-Hidden-Markov-Models-Hidden-Markov-Models-Discrete-Observations-Deep-Learning-Libraries.ipynb | NathanielDake/NathanielDake.github.io | 82b7013afa66328e06e51304b6af10e1ed648eb8 | [
"MIT"
] | 3 | 2018-03-30T06:28:21.000Z | 2018-04-25T15:43:24.000Z | Machine_Learning/05-Hidden_Markov_Models-06-Hidden-Markov-Models-Hidden-Markov-Models-Discrete-Observations-Deep-Learning-Libraries.ipynb | NathanielDake/NathanielDake.github.io | 82b7013afa66328e06e51304b6af10e1ed648eb8 | [
"MIT"
] | null | null | null | Machine_Learning/05-Hidden_Markov_Models-06-Hidden-Markov-Models-Hidden-Markov-Models-Discrete-Observations-Deep-Learning-Libraries.ipynb | NathanielDake/NathanielDake.github.io | 82b7013afa66328e06e51304b6af10e1ed648eb8 | [
"MIT"
] | 3 | 2018-02-07T22:21:33.000Z | 2018-05-04T20:16:43.000Z | 343.618375 | 175,648 | 0.913925 | [
[
[
"# 6. Hidden Markov Models with Theano and TensorFlow\nIn the last section we went over the training and prediction procedures of Hidden Markov Models. This was all done using only vanilla numpy the Expectation Maximization algorithm. I now want to introduce how both `Theano` and `Tensorflow` can be utilized to accomplish the same goal, albeit by a very different process. \n\n## 1. Gradient Descent\nHopefully you are familiar with the gradient descent optimization algorithm, if not I recommend reviewing my posts on Deep Learning, which leverage gradient descent heavily (or this [video](https://www.youtube.com/watch?v=IHZwWFHWa-w). With that said, a simple overview is as follows:\n\n> Gradient descent is a first order optimization algorithm for finding the minimum of a function. To find a local minimum of a function using gradient descent, on takes steps proportional to the negative of the gradient of the function at its current point. \n\nVisually, this iterative process looks like: \n\n<img src=\"https://drive.google.com/uc?id=1R2zVTj3uo5zmow6vFujWlU-qs9jRF_XG\" width=\"250\">\n\nWhere above we are looking at a contour plot of a three dimensional bowl, and the center of the bowl is a minimum. Now, the actual underlying mechanics of gradient descent work as follows:\n#### 1. Define a model/hypothesis that will be mapping inputs to outputs, or in other words making predictions:\n\n$$h_{\\theta}(x) = \\theta_0 + \\theta_1x$$\n\nIn this case $x$ is our input and $h(x)$, often thought of as $y$, is our output. We are stating that we believe the ground truth relationship between $x$ and $h(x)$ is captured by the linear combination of $\\theta_0 + \\theta_1x$. Now, what are $\\theta_0$ and $\\theta_1$ equal to? \n\n#### 2. Define a **cost** function for which you are trying to find the minimum. Generally, this cost function is defined as some form of **error**, and it will be parameterized by variables related to your model in some way. \n\n$$cost = J = (y - h_{\\theta}(x))^2$$\n\nAbove $y$ refers to the ground truth/actual value of the output, and $h_{\\theta}(x)$ refers to that which our model predicted. The difference, squared, represents our cost. We can see that if our prediction is exactly equal to the ground truth value, our cost will be 0. If our prediction is very far off from our ground truth value then our cost will be very high. Our goal is to minimize the cost (error) of our model. \n\n#### 3. Take the [**gradient**](https://en.wikipedia.org/wiki/Gradient) (multi-variable generalization of the derivative) of the cost function with respect to the parameters that you have control over.\n\n$$\\nabla J = \\frac{\\partial J}{\\partial \\theta}$$\n\nSimply put, we want to see how $J$ changes as we change our model parameters, $\\theta_0$ and $\\theta_1$. \n\n#### 4. Based on the gradient update our values for $\\theta$ with a simple update rule:\n\n$$\\theta_0 \\rightarrow \\theta_0 - \\alpha \\cdot \\frac{\\partial J}{\\partial \\theta_0}$$\n\n$$\\theta_1 \\rightarrow \\theta_1 - \\alpha \\cdot \\frac{\\partial J}{\\partial \\theta_1}$$\n\n#### 5. Repeat steps two and three for a set number of iterations/until convergence.\n\nAfter a set number of steps, the hope is that the model weights that were _learned_ are the most optimal weights to minimize prediction error. Now after everything we discussed in the past two posts you may be wondering, how exactly does this relate to Hidden Markov Models, which have been trained via Expectation Maximization?\n\n### 1.1 Gradient Descent and Hidden Markov Models\nLet's say for a moment that our goal that we wish to accomplish is predict the probability of an observed sequence, $p(x)$. And let's say that we have 100 observed sequences at our disposal. It should be clear that if we have a trained HMM that predicts the majority of our sequences are very unlikely, the HMM was probably not trained very well. Ideally, our HMM parameters would be learned in a way that maximizes the probability of observing what we did (this was the goal of expectation maximization).\n\nWhat may start to become apparent at this point is that we have a perfect cost function already created for us! The total probability of our observed sequences, based on our HMM parameters $A$, $B$, and $\\pi$. We can define this mathematically as follows (for the scaled version); in the previous post we proved that:\n\n$$p(x) = \\prod_{t=1}^T c(t)$$\n\nWhich states that the probability of an observed sequence is equal to the product of the scales at each time step. Also recall that the scale is just defined as:\n\n$$c(t) = \\sum_{i=1}^M \\alpha'(t,i)$$\n\nWith that all said, we can define the cost of a single observed training sequence as:\n\n$$cost = \\sum_{t}^{T} log\\big(c(t)\\big)$$\n\nWhere we are using the log to avoid the underflow problem, just as we did in the last notebook. So, we have a cost function which intuitively makes sense, but can we find its gradient with respect to our HMM parameters $A$, $B$, and $\\pi$? We absolutely can! The wonderful thing about Theano is that it links variables together via a [computational graph](http://deeplearning.net/software/theano/extending/graphstructures.html). So, cost is depedent on $A$, $B$ and $\\pi$ via the following link:\n\n$$cost \\rightarrow c(t) \\rightarrow alpha \\rightarrow A, B, \\pi$$\n\nWe can take the gradient of this cost function in theano as well, allowing us to then easily update our values of $A$, $B$, and $\\pi$! Done iteratively, we hopefully will converge to a nice minimum.\n\n### 1.2 HMM Theano specifics\nI would be lying if I said that Theano wasn't a little bit hard to follow at first. For those unfamiliar, representing symbolic mathematical computations as graphs may feel very strange. I have a few walk throughs of Theano in my Deep Learning section, as well as `.py` files in the source repo. Additionally, the theano [documentation](http://deeplearning.net/software/theano/index.html) is also very good. With that said, I do want to discuss a few details of the upcoming code block. \n\n#### Recurrence Block $\\rightarrow$ Calculating the Forward Variable, $\\alpha$\nFirst, I want to discuss the `recurrence` and `scan` functions that you will be seeing:\n\n```\ndef recurrence_to_find_alpha(t, old_alpha, x):\n \"\"\"Scaled version of updates for HMM. This is used to \n find the forward variable alpha.\n\n Args:\n t: Current time step, from pass in from scan:\n sequences=T.arange(1, thx.shape[0])\n old_alpha: Previously returned alpha, or on the first time \n step the initial value,\n outputs_info=[self.pi * self.B[:, thx[0]], None]\n x: thx, non_sequences (our actual set of observations)\n \"\"\"\n alpha = old_alpha.dot(self.A) * self.B[:, x[t]]\n s = alpha.sum()\n return (alpha / s), s\n\n# alpha and scale, once returned, are both matrices with values at each time step\n[alpha, scale], _ = theano.scan(\n fn=recurrence_to_find_alpha,\n sequences=T.arange(1, thx.shape[0]),\n outputs_info=[self.pi * self.B[:, thx[0]], None], # Initial value of alpha\n n_steps=thx.shape[0] - 1,\n non_sequences=thx,\n)\n\n# scale is an array, and scale.prod() = p(x)\n# The property log(A) + log(B) = log(AB) can be used \n# here to prevent underflow problem\np_of_x = -T.log(scale).sum() # Negative log likelihood\ncost = p_of_x\n\nself.cost_op = theano.function(\n inputs=[thx],\n outputs=cost,\n allow_input_downcast=True,\n)\n\n```\n\nThe above block is where our forward variable $\\alpha$ and subsequently the probability of the observed sequence $p(x)$ is found. The process works as follows:\n1. The `theano.scan` function (logically similar to a for loop) is defined with the following parameters:\n * `fn`: The recurrence function that the array being iterated over will be passed into.\n * `sequences`: An array of indexes, $[1,2,3,...,T]$\n * `outputs_info`: The initial value of $\\alpha$\n * `non_sequences`: Our observation sequence, $X$. This passed in it's entirety to the recurrence function at each iteration.\n2. Our recurrence function, `recurrence_to_find_alpha`, is meant to calculate $\\alpha$ at each time step. $\\alpha$ at $t=1$ was defined by `outputs_info` in `scan`. This recurrence function essentially is performing the forward algorithm (additionally it incorporates scaling):\n\n$$\\alpha(1,i) = \\pi_iB\\big(i, x(1)\\big)$$\n\n$$\\alpha(t+1, j) = \\sum_{i=1}^M \\alpha(t,i) A(i,j)B(j, x(t+1))$$\n\n3. We calculate $p(x)$ to be the sum of the log likelihood. This is set to be our `cost`.\n4. We define a `cost_op`, which is a theano function that takes in a symbolic variable `thx` and determines the output `cost`. Remember, `cost` is linked to `thx` via:\n\n```\ncost -> scale -> theano.scan(non_sequences=thx)\n```\n\n#### Update block $\\rightarrow$ Updating HMM parameters $A$, $B$, and $\\pi$\nThe other block that I want to touch on is the update block:\n\n```\npi_update = self.pi - learning_rate * T.grad(cost, self.pi)\npi_update = pi_update / pi_update.sum()\n\nA_update = self.A - learning_rate*T.grad(cost, self.A)\nA_update = A_update / A_update.sum(axis=1).dimshuffle(0, 'x')\n\nB_update = self.B - learning_rate*T.grad(cost, self.B)\nB_update = B_update / B_update.sum(axis=1).dimshuffle(0, 'x')\n\nupdates = [\n (self.pi, pi_update),\n (self.A, A_update),\n (self.B, B_update),\n]\n\ntrain_op = theano.function(\n inputs=[thx],\n updates=updates,\n allow_input_downcast=True\n)\n\ncosts = []\nfor it in range(max_iter):\n for n in range(N):\n # Looping through all N training examples\n c = self.get_cost_multi(X, p_cost).sum()\n costs.append(c)\n train_op(X[n])\n```\n\nThe update block functions as follows:\n1. We have `cost` that was defined symbolically and linked to `thx`. We can define `pi_update` as `pi_update = self.pi - learning_rate * T.grad(cost, self.pi)`. \n2. This same approach is performed for $A$ and $B$. \n3. We then create a theano function, `train_op` which takes in `thx`, our symbolic input, and with perform updates via the `updates=updates` kwarg. Specifically, updates takes in a list of tuples, with the first value in the tuple being the variable that should be updated, and the second being the expression with which it should be updated to be. \n4. We loop through all training examples (sequences of observations), and call `train_up`, passing in `X[n]` (a unique sequene of observations) as `thx`.\n5. `train_op` then performs the `updates`, utilizing `thx = X[n]` wherever `updates` depends on `thx`.\n\nThis is clearly stochastic gradient descent, because we are performing updates to our parameters $A$, $B$, and $\\pi$ for each training sequence. Full batch gradient descent would be if we defined a cost function that was based on all of the training sequences, not only an individual sequence. ",
"_____no_output_____"
],
[
"## 2. HMM's with Theano\nIn code, our HMM can be implemented with Theano as follows:",
"_____no_output_____"
]
],
[
[
"import numpy as np\nimport theano\nimport theano.tensor as T\n\nimport seaborn as sns\nimport matplotlib.pyplot as plt\n\nfrom hmm.utils import get_obj_s3, random_normalized\n\n%matplotlib inline\n%config InlineBackend.figure_format = 'retina'\n\nsns.set(style=\"white\", palette=\"husl\")\nsns.set_context(\"talk\")\nsns.set_style(\"ticks\")",
"_____no_output_____"
],
[
"class HMM:\n def __init__(self, M):\n self.M = M\n\n def fit(self, X, learning_rate=0.001, max_iter=10, V=None, p_cost=1.0, print_period=10):\n \"\"\"Train HMM model using stochastic gradient descent.\"\"\"\n\n # Determine V, the vocabulary size\n if V is None:\n V = max(max(x) for x in X) + 1\n N = len(X)\n\n # Initialize HMM variables\n pi0 = np.ones(self.M) / self.M # Initial state distribution\n A0 = random_normalized(self.M, self.M) # State transition matrix\n B0 = random_normalized(self.M, V) # Output distribution\n\n thx, cost = self.set(pi0, A0, B0)\n\n # This is a beauty of theano and it's computational graph. \n # By defining a cost function, which is representing p(x), \n # the probability of a sequence, we can then find the gradient\n # of the cost with respect to our parameters (pi, A, B). \n # The gradient updated rules are applied as usual. Note, the \n # reason that this is stochastic gradient descent is because\n # we are only looking at a single training example at a time.\n pi_update = self.pi - learning_rate * T.grad(cost, self.pi)\n pi_update = pi_update / pi_update.sum()\n\n A_update = self.A - learning_rate*T.grad(cost, self.A)\n A_update = A_update / A_update.sum(axis=1).dimshuffle(0, 'x')\n\n B_update = self.B - learning_rate*T.grad(cost, self.B)\n B_update = B_update / B_update.sum(axis=1).dimshuffle(0, 'x')\n\n updates = [\n (self.pi, pi_update),\n (self.A, A_update),\n (self.B, B_update),\n ]\n\n train_op = theano.function(\n inputs=[thx],\n updates=updates,\n allow_input_downcast=True\n )\n\n costs = []\n for it in range(max_iter):\n for n in range(N):\n # Looping through all N training examples\n c = self.get_cost_multi(X, p_cost).sum()\n costs.append(c)\n train_op(X[n])\n\n print(\"A learned from training: \\n\", self.A.get_value())\n print(\"B learned from training: \\n\", self.B.get_value())\n print(\"pi learned from training: \\n\", self.pi.get_value())\n\n plt.figure(figsize=(8,5))\n plt.plot(costs, color=\"blue\")\n plt.xlabel(\"Iteration Number\")\n plt.ylabel(\"Cost\")\n plt.show()\n\n def get_cost(self, x):\n return self.cost_op(x)\n\n def get_cost_multi(self, X, p_cost=1.0):\n P = np.random.random(len(X))\n return np.array([self.get_cost(x) for x, p in zip(X, P) if p < p_cost])\n\n def log_likelihood(self, x):\n return - self.cost_op(x)\n\n def set(self, pi, A, B):\n # Create theano shared variables\n self.pi = theano.shared(pi)\n self.A = theano.shared(A)\n self.B = theano.shared(B)\n\n # Define input, a vector\n thx = T.ivector(\"thx\")\n\n def recurrence_to_find_alpha(t, old_alpha, x):\n \"\"\"\n Scaled version of updates for HMM. This is used to find the \n forward variable alpha.\n\n Args:\n t: Current time step, from pass in from scan:\n sequences=T.arange(1, thx.shape[0])\n old_alpha: Previously returned alpha, or on the first time step \n the initial value, \n outputs_info=[self.pi * self.B[:, thx[0]], None]\n x: thx, non_sequences (our actual set of observations)\n \"\"\"\n alpha = old_alpha.dot(self.A) * self.B[:, x[t]]\n s = alpha.sum()\n return (alpha / s), s\n\n # alpha and scale, once returned, are both matrices with values at each time step\n [alpha, scale], _ = theano.scan(\n fn=recurrence_to_find_alpha,\n sequences=T.arange(1, thx.shape[0]),\n outputs_info=[self.pi * self.B[:, thx[0]], None], # Initial value of alpha\n n_steps=thx.shape[0] - 1,\n non_sequences=thx,\n )\n\n # scale is an array, and scale.prod() = p(x)\n # The property log(A) + log(B) = log(AB) can be used \n # here to prevent underflow problem\n p_of_x = -T.log(scale).sum() # Negative log likelihood\n cost = p_of_x\n\n self.cost_op = theano.function(\n inputs=[thx],\n outputs=cost,\n allow_input_downcast=True,\n )\n return thx, cost\n\n\ndef fit_coin(file_key):\n \"\"\"Loads data and trains HMM.\"\"\"\n\n X = []\n for line in get_obj_s3(file_key).read().decode(\"utf-8\").strip().split(sep=\"\\n\"):\n x = [1 if e == \"H\" else 0 for e in line.rstrip()]\n X.append(x)\n\n # Instantiate object of class HMM with 2 hidden states (heads and tails)\n hmm = HMM(2)\n hmm.fit(X)\n L = hmm.get_cost_multi(X).sum()\n print(\"Log likelihood with fitted params: \", round(L, 3))\n\n # Try the true values\n pi = np.array([0.5, 0.5])\n A = np.array([\n [0.1, 0.9],\n [0.8, 0.2]\n ])\n B = np.array([\n [0.6, 0.4],\n [0.3, 0.7]\n ])\n hmm.set(pi, A, B)\n L = hmm.get_cost_multi(X).sum()\n print(\"Log Likelihood with true params: \", round(L, 3))\n\n\nif __name__ == \"__main__\":\n key = \"coin_data.txt\"\n fit_coin(key)",
"A learned from training: \n [[0.50000007 0.49999993]\n [0.50000005 0.49999995]]\nB learned from training: \n [[0.52666344 0.47333656]\n [0.52666383 0.47333617]]\npi learned from training: \n [0.50007189 0.49992811]\n"
]
],
[
[
"## 3. HMM's with Theano $\\rightarrow$ Optimization via Softmax\nOne of the challenges of the approach we took is that gradient descent is _unconstrained_; it simply goes in the direction of the gradient. This presents a problem for us in the case of HMM's. Remember, the parameters of an HMM are $\\pi$, $A$, and $B$, and each is a probability matrix/vector. This means that they must be between 0 and 1, and must sum to 1 (along the rows if 2-D). \n\nWe accomplished this in the previous section by performing a \"hack\". Specifically, we renormalized after each gradient descent step. However, this means that we weren't performing _real_ gradient descent, because by renormalizing we are not exactly moving in the direction of the gradient anymore. For reference, the pseudocode looked like this:\n\n```\npi_update = self.pi - learning_rate * T.grad(cost, self.pi)\npi_update = pi_update / pi_update.sum() # Normalizing to ensure it stays a probability\n\nA_update = self.A - learning_rate*T.grad(cost, self.A)\nA_update = A_update / A_update.sum(axis=1).dimshuffle(0, 'x') # Normalize for prob \nB_update = self.B - learning_rate*T.grad(cost, self.B)\nB_update = B_update / B_update.sum(axis=1).dimshuffle(0, 'x') # Normalize for prob\n\n# Passing in normalized updates for pi, A, B. No longer moving in dir of gradient\nupdates = [\n (self.pi, pi_update),\n (self.A, A_update),\n (self.B, B_update),\n]\n```\n\nThis leads us to the question: is it possible to use true gradient descent, while still conforming to the constraints that each parameter much be a true probability. The answer is of course yes!\n\n### 3.1 Softmax \nIf you are unfamiliar with Deep Learning then you may want to jump over this section, or go through my deep learning posts that dig into the subject. If you are familiar, recall the softmax function:\n\n$$softmax(x)_i = \\frac{exp(x_i)}{\\sum_{k=1}^K exp(x_k)}$$\n\nWhere $x$ is an array of size $K$, and $K$ is the number of classes that we have. The result of the softmax is that all outputs are positive and sum to 1. What exactly does this mean in our scenario? \n\n#### Softmax for $\\pi$\nConsider $\\pi$, an array of size $M$. Supposed we want to parameterize $\\pi$, using the symbol $\\theta$. We can then assign $\\pi$ to be:\n\n$$pi = softmax(\\theta)$$\n\nIn this way, $\\pi$ is like an intermediate variable and $\\theta$ is the actual parameter that we will be updating. This ensures that $\\pi$ is always between 0 and 1, and sums to 1. At the same time, the values in $\\theta$ can be anything; this means that we can freely use gradient descent on $\\theta$ without having to worry about any constraints! No matter what we do to $\\theta$, $\\pi$ will always be between 0 and 1 and sum to 1. \n\n#### Softmax for $A$ and $B$\nNow, what about $A$ and $B$? Unlike $\\pi$, which was a 1-d vector, $A$ and $B$ are matrices. Luckily for us, softmax works well for us here too! Recall that when dealing with data in deep learning (and most ML) that we are often dealing with multiple samples at the same time. Typically an $NxD$ matrix, where $N$ is the number of samples, and $D$ is the dimensionality. We know that the output of our model is usually an $NxK$ matrix, where $K$ is the number of classes. Naturally, because the classes go along the rows, each row must represent a separate probability distribution. \n\nWhy is this helpful? Well, the softmax was actually written with this specifically in mind! When you use the softmax it automatically exponentiates every element of the matrix and divides by the row sum. That is exactly what we want to do with $A$ and $B$! Each row of $A$ is the probability of the next state to transition to, and each row of $B$ is the probability of the next symbol to emit. The rows must sum to 1, just like the output predictions of a neural network! \n\nIn pseudocode, softmax looks like:\n\n```\ndef softmax(A):\n expA = np.exp(A)\n return expA / expA.sum(axis=1, keepdims=True)\n```\n\nWe can see this clearly below:",
"_____no_output_____"
]
],
[
[
"np.set_printoptions(suppress=True)\n\nA = np.array([\n [1,2],\n [4,5],\n [9,5]\n])\n\nexpA = np.exp(A)\nprint(\"A exponentiated element wise: \\n\", np.round_(expA, decimals=3), \"\\n\")\n\n# Keep dims ensures a column vector (vs. row) output\noutput = expA / expA.sum(axis=1, keepdims=True) \nprint(\"Exponentiated A divided row sum: \\n\", np.round_(output, decimals=3))",
"A exponentiated element wise: \n [[ 2.718 7.389]\n [ 54.598 148.413]\n [8103.084 148.413]] \n\nExponentiated A divided row sum: \n [[0.269 0.731]\n [0.269 0.731]\n [0.982 0.018]]\n"
]
],
[
[
"Now you may be wondering: Why can't we just perform standard normalization? Why does the exponetial need to be used? For an answer to that I recommend reading up [here](https://stackoverflow.com/questions/17187507/why-use-softmax-as-opposed-to-standard-normalization), [here](https://stats.stackexchange.com/questions/162988/why-sigmoid-function-instead-of-anything-else/318209#318209), and [here](http://cs231n.github.io/linear-classify/#softmax).",
"_____no_output_____"
],
[
"### 3.2 Update Discrete HMM Code $\\rightarrow$ with Softmax",
"_____no_output_____"
]
],
[
[
"class HMM:\n def __init__(self, M):\n self.M = M\n\n def fit(self, X, learning_rate=0.001, max_iter=10, V=None, p_cost=1.0, print_period=10):\n \"\"\"Train HMM model using stochastic gradient descent.\"\"\"\n\n # Determine V, the vocabulary size\n if V is None:\n V = max(max(x) for x in X) + 1\n N = len(X)\n\n preSoftmaxPi0 = np.zeros(self.M) # initial state distribution\n preSoftmaxA0 = np.random.randn(self.M, self.M) # state transition matrix\n preSoftmaxB0 = np.random.randn(self.M, V) # output distribution\n\n thx, cost = self.set(preSoftmaxPi0, preSoftmaxA0, preSoftmaxB0)\n\n # This is a beauty of theano and it's computational graph. By defining a cost function,\n # which is representing p(x), the probability of a sequence, we can then find the gradient\n # of the cost with respect to our parameters (pi, A, B). The gradient updated rules are\n # applied as usual. Note, the reason that this is stochastic gradient descent is because\n # we are only looking at a single training example at a time.\n pi_update = self.preSoftmaxPi - learning_rate * T.grad(cost, self.preSoftmaxPi)\n A_update = self.preSoftmaxA - learning_rate * T.grad(cost, self.preSoftmaxA)\n B_update = self.preSoftmaxB - learning_rate * T.grad(cost, self.preSoftmaxB)\n\n updates = [\n (self.preSoftmaxPi, pi_update),\n (self.preSoftmaxA, A_update),\n (self.preSoftmaxB, B_update),\n ]\n\n train_op = theano.function(\n inputs=[thx],\n updates=updates,\n allow_input_downcast=True\n )\n\n costs = []\n for it in range(max_iter):\n for n in range(N):\n # Looping through all N training examples\n c = self.get_cost_multi(X, p_cost).sum()\n costs.append(c)\n train_op(X[n])\n\n plt.figure(figsize=(8,5))\n plt.plot(costs, color=\"blue\")\n plt.xlabel(\"Iteration Number\")\n plt.ylabel(\"Cost\")\n plt.show()\n\n def get_cost(self, x):\n return self.cost_op(x)\n\n def get_cost_multi(self, X, p_cost=1.0):\n P = np.random.random(len(X))\n return np.array([self.get_cost(x) for x, p in zip(X, P) if p < p_cost])\n\n def log_likelihood(self, x):\n return - self.cost_op(x)\n\n def set(self, preSoftmaxPi, preSoftmaxA, preSoftmaxB):\n # Create theano shared variables\n self.preSoftmaxPi = theano.shared(preSoftmaxPi)\n self.preSoftmaxA = theano.shared(preSoftmaxA)\n self.preSoftmaxB = theano.shared(preSoftmaxB)\n\n pi = T.nnet.softmax(self.preSoftmaxPi).flatten()\n # softmax returns 1xD if input is a 1-D array of size D\n A = T.nnet.softmax(self.preSoftmaxA)\n B = T.nnet.softmax(self.preSoftmaxB)\n\n # Define input, a vector\n thx = T.ivector(\"thx\")\n\n def recurrence_to_find_alpha(t, old_alpha, x):\n \"\"\"Scaled version of updates for HMM. This is used to find the forward variable alpha.\n\n Args:\n t: Current time step, from pass in from scan:\n sequences=T.arange(1, thx.shape[0])\n old_alpha: Previously returned alpha, or on the first time step the initial value,\n outputs_info=[pi * B[:, thx[0]], None]\n x: thx, non_sequences (our actual set of observations)\n \"\"\"\n alpha = old_alpha.dot(A) * B[:, x[t]]\n s = alpha.sum()\n return (alpha / s), s\n\n # alpha and scale, once returned, are both matrices with values at each time step\n [alpha, scale], _ = theano.scan(\n fn=recurrence_to_find_alpha,\n sequences=T.arange(1, thx.shape[0]),\n outputs_info=[pi * B[:, thx[0]], None], # Initial value of alpha\n n_steps=thx.shape[0] - 1,\n non_sequences=thx,\n )\n\n # scale is an array, and scale.prod() = p(x)\n # The property log(A) + log(B) = log(AB) can be used here to prevent underflow problem\n p_of_x = -T.log(scale).sum() # Negative log likelihood\n cost = p_of_x\n\n self.cost_op = theano.function(\n inputs=[thx],\n outputs=cost,\n allow_input_downcast=True,\n )\n return thx, cost\n\n\ndef fit_coin(file_key):\n \"\"\"Loads data and trains HMM.\"\"\"\n\n X = []\n for line in get_obj_s3(file_key).read().decode(\"utf-8\").strip().split(sep=\"\\n\"):\n x = [1 if e == \"H\" else 0 for e in line.rstrip()]\n X.append(x)\n\n # Instantiate object of class HMM with 2 hidden states (heads and tails)\n hmm = HMM(2)\n hmm.fit(X)\n L = hmm.get_cost_multi(X).sum()\n print(\"Log likelihood with fitted params: \", round(L, 3))\n\n # Try the true values\n pi = np.array([0.5, 0.5])\n A = np.array([\n [0.1, 0.9],\n [0.8, 0.2]\n ])\n B = np.array([\n [0.6, 0.4],\n [0.3, 0.7]\n ])\n hmm.set(pi, A, B)\n L = hmm.get_cost_multi(X).sum()\n print(\"Log Likelihood with true params: \", round(L, 3))\n\n\nif __name__ == \"__main__\":\n key = \"coin_data.txt\"\n fit_coin(key)",
"_____no_output_____"
]
],
[
[
"## 4. Hidden Markov Models with TensorFlow\nI now want to expose everyone to an HMM implementation in TensorFlow. In order to do so, we will need to first go over the `scan` function in Tensorflow. Just like when dealing with Theano, we need to ask \"What is the equivalent of a for loop in TensorFlow?\". And why should we care? \n\n### 4.1 TensorFlow Scan\nIn order to understand the importance of `scan`, we need to be sure that we have a good idea of how TensorFlow works, even if only from a high level. In general, with both TensorFlow and Theano, you have to create variables and link them together functionally, but they do not have values until you actually run the functions. So, when you create your $X$ matrix you don't give it a shape; you just say here is a place holder I am going to call $X$ and this is a possible shape for it:\n\n```\nX = tf.placeholder(tf.float32, shape=(None, D))\n```\n\nHowever, remember that the `shape` argument is _optional_, and hence for all intents and purposes we can assume that we do not know the shape of $X$. So, what happens if you want to loop through all the elements of $X$? Well you can't, because we do not know the number of elements in $X$!\n\n```\nfor i in range(X.shape[0]): <------- Not possible! We don't know num elements in X\n # ....\n```\n\nIn order to write a for loop we must specify the number of times the loop will run. But in order to specify the number of times the loop will run we must know the number of elements in $X$. Generally speaking, we cannot guarantee the length of our training sequences. This is why we need the tensorflow `scan` function! It will allow us to loop through a tensorflow array without knowing its size. This is similar to how everything else in Tensorflow and Theano works. Using `scan` we can tell Tensorflow \"how to run the for loop\", without actually running it. \n\nThere is another big reason that the `scan` function is so important; it allows us to perform **automatic differentiation** when we have sequential data. Tensorflow keeps track of how all the variables in your graph link together, so that it can automatically calculate the gradient for you when you do gradient descent:\n\n$$W(t) \\leftarrow W(t-1) - \\eta \\nabla J\\big(W(t-1)\\big)$$\n\nThe `scan` function keeps track of this when it performs the loop. The anatomy of the `scan` function is shown in pseudocode below:\n\n```\noutputs = tf.scan(\n fn=some_function, # Function applied to every element in sequence \n elems=thing_to_loop_over # Actual sequence that is passed in\n)\n```\n\nAbove, `some_function` is applied to every element in `thing_to_loop_over`. Now, the way that we define `some_function` is very specific and much more strict than that for theano. In particular, it must always take in two arguments. The first element is the last output of the function, and the second element is the next element of the sequence:\n\n```\ndef some_function(last_output, element):\n return do_something_to(last_output, element)\n```\n\nThe tensorflow scan function returns `outputs`, which is all of the return values of `some_function` concatenated together. For example, we can look at the following block:\n\n```\noutputs = tf.scan(\n fn=some_function, \n elems=thing_to_loop_over \n)\n\ndef square(last, current):\n return current * current\n \n# sequence = [1, 2, 3]\n# outputs = [1, 4, 9]\n```\n\nIf we pass in `[1, 2, 3]`, then our outputs will be `[1, 4, 9]`. Now, of course the outputs is still a tensorflow graph node. So, in order to get an actual value out of it we need to run it in an actual session. ",
"_____no_output_____"
]
],
[
[
"import tensorflow as tf\n\nx = tf.placeholder(tf.int32, shape=(None,), name=\"x\")\n\ndef square(last, current):\n \"\"\"Last is never used, but must be included based on interface requirements of tf.scan\"\"\"\n return current*current\n\n# Essentially doing what a for loop would normally do\n# It applies the square function to every element of x\nsquare_op = tf.scan(\n fn=square,\n elems=x\n)\n\n# Run it!\nwith tf.Session() as session:\n o_val = session.run(\n square_op,\n feed_dict={x: [1, 2, 3, 4, 5]}\n )\n print(\"Output: \", o_val)\n",
"WARNING:tensorflow:From /Users/natedake/.virtualenvs/intuitiveml/lib/python3.6/site-packages/tensorflow/python/ops/tensor_array_ops.py:162: colocate_with (from tensorflow.python.framework.ops) is deprecated and will be removed in a future version.\nInstructions for updating:\nColocations handled automatically by placer.\nOutput: [ 1 4 9 16 25]\n"
]
],
[
[
"Now, of course `scan` can do more complex things than this. We can implement another argument, `initializer`, that allows us to compute recurrence relationships. \n\n```\noutputs = tf.scan(\n fn=some_function, # Function applied to every element in sequence \n elems=thing_to_loop_over, # Actual sequence that is passed in\n initializer=initial_input \n)\n```\n\nWhy exactly do we need this? Well, we can see that the recurrence function takes in two things: the last element that it returned, and the current element of the sequence that we are iterating over. What is the last output during the first iteration? There isn't one yet! And that is exactly why we need `initializer`. \n\nOne thing to keep in mind when using `initializer` is that it is very strict. In particular, it must be the exact same type as the output of `recurrence`. For example, if you need to return multiple things from `recurrence` it is going to be returned as a tuple. That means that the argument to `initializer` cannot be a list, it must be a tuple. This also means that a tuple containing `(5 , 5)` is not the same a tuple containing `(5.0, 5.0)`. \n\nLet's try to compute the fibonacci sequence to get a feel for how this works:",
"_____no_output_____"
]
],
[
[
"# N is the number fibonacci numbers that we want\nN = tf.placeholder(tf.int32, shape=(), name=\"N\")\n\ndef fibonacci(last, current):\n # last[0] is the last value, last[1] is the second last value\n return (last[1], last[0] + last[1])\n\n\nfib_op = tf.scan(\n fn=fibonacci,\n elems=tf.range(N),\n initializer=(0, 1),\n)\n\nwith tf.Session() as session:\n o_val = session.run(\n fib_op,\n feed_dict={N: 8}\n )\n print(\"Output: \\n\", o_val)",
"Output: \n (array([ 1, 1, 2, 3, 5, 8, 13, 21], dtype=int32), array([ 1, 2, 3, 5, 8, 13, 21, 34], dtype=int32))\n"
]
],
[
[
"Another example of what we can do with the theano `scan` is create a **low pass filter** (also known as a **moving average**). In this case, our recurrence relation is given by:\n\n$$s(t) = \\text{decay_rate} \\cdot s(t-1) + (1 - \\text{decay_rate}) \\cdot x(t)$$\n\nWhere $x(t)$ is the input and $s(t)$ is the output. The goal here is to return a clean version of a noisy signal. To do this we can create a sine wave, add some random gaussian noise to it, and finally try to retrieve the sine wave. In code this looks like:",
"_____no_output_____"
]
],
[
[
"original = np.sin(np.linspace(0, 3*np.pi, 300))\nX = 2*np.random.randn(300) + original\n\nfig = plt.figure(figsize=(15,5))\nplt.subplot(1, 2, 1)\nax = plt.plot(X, c=\"g\", lw=1.5)\nplt.title(\"Original\")\n\n# Setup placeholders\ndecay = tf.placeholder(tf.float32, shape=(), name=\"decay\")\nsequence = tf.placeholder(tf.float32, shape=(None, ), name=\"sequence\")\n\n# The recurrence function and loop\ndef recurrence(last, x):\n return (1.0 - decay)*x + decay*last\n\nlow_pass_filter = tf.scan(\n fn=recurrence,\n elems=sequence,\n initializer=0.0 # sequence[0] to use first value of the sequence\n)\n\n# Run it!\nwith tf.Session() as session:\n Y = session.run(low_pass_filter, feed_dict={sequence: X, decay: 0.97})\n\n plt.subplot(1, 2, 2)\n ax2 = plt.plot(original, c=\"b\")\n ax = plt.plot(Y, c=\"r\")\n plt.title(\"Low pass filter\")\n plt.show()",
"_____no_output_____"
]
],
[
[
"### 4.2 Discrete HMM With Tensorflow\nLet's now take a moment to walk through the creation of a discrete HMM class utilizing Tensorflow.",
"_____no_output_____"
]
],
[
[
"import numpy as np\nimport tensorflow as tf\nimport matplotlib.pyplot as plt\n\nfrom hmm.utils import get_obj_s3\n\n\nclass HMM:\n def __init__(self, M):\n self.M = M # number of hidden states\n\n def set_session(self, session):\n self.session = session\n\n def fit(self, X, max_iter=10, print_period=1):\n # train the HMM model using stochastic gradient descent\n\n N = len(X)\n print(\"Number of train samples:\", N)\n\n costs = []\n for it in range(max_iter):\n for n in range(N):\n # this would of course be much faster if we didn't do this on\n # every iteration of the loop\n c = self.get_cost_multi(X).sum()\n costs.append(c)\n self.session.run(self.train_op, feed_dict={self.tfx: X[n]})\n\n plt.figure(figsize=(8,5))\n plt.plot(costs, c=\"b\")\n plt.xlabel(\"Iteration Number\")\n plt.ylabel(\"Cost\")\n plt.show()\n\n def get_cost(self, x):\n # returns log P(x | model)\n # using the forward part of the forward-backward algorithm\n # print \"getting cost for:\", x\n return self.session.run(self.cost, feed_dict={self.tfx: x})\n\n def log_likelihood(self, x):\n return -self.session.run(self.cost, feed_dict={self.tfx: x})\n\n def get_cost_multi(self, X):\n return np.array([self.get_cost(x) for x in X])\n\n def build(self, preSoftmaxPi, preSoftmaxA, preSoftmaxB):\n M, V = preSoftmaxB.shape\n\n self.preSoftmaxPi = tf.Variable(preSoftmaxPi)\n self.preSoftmaxA = tf.Variable(preSoftmaxA)\n self.preSoftmaxB = tf.Variable(preSoftmaxB)\n\n pi = tf.nn.softmax(self.preSoftmaxPi)\n A = tf.nn.softmax(self.preSoftmaxA)\n B = tf.nn.softmax(self.preSoftmaxB)\n\n # define cost\n self.tfx = tf.placeholder(tf.int32, shape=(None,), name='x')\n\n def recurrence(old_a_old_s, x_t):\n old_a = tf.reshape(old_a_old_s[0], (1, M))\n a = tf.matmul(old_a, A) * B[:, x_t]\n a = tf.reshape(a, (M,))\n s = tf.reduce_sum(a)\n return (a / s), s\n\n # remember, tensorflow scan is going to loop through\n # all the values!\n # we treat the first value differently than the rest\n # so we only want to loop through tfx[1:]\n # the first scale being 1 doesn't affect the log-likelihood\n # because log(1) = 0\n alpha, scale = tf.scan(\n fn=recurrence,\n elems=self.tfx[1:],\n initializer=(pi * B[:, self.tfx[0]], np.float32(1.0)),\n )\n\n self.cost = -tf.reduce_sum(tf.log(scale))\n self.train_op = tf.train.AdamOptimizer(1e-2).minimize(self.cost)\n\n def init_random(self, V):\n preSoftmaxPi0 = np.zeros(self.M).astype(np.float32) # initial state distribution\n preSoftmaxA0 = np.random.randn(self.M, self.M).astype(np.float32) # state transition matrix\n preSoftmaxB0 = np.random.randn(self.M, V).astype(np.float32) # output distribution\n\n self.build(preSoftmaxPi0, preSoftmaxA0, preSoftmaxB0)\n\n def set(self, preSoftmaxPi, preSoftmaxA, preSoftmaxB):\n op1 = self.preSoftmaxPi.assign(preSoftmaxPi)\n op2 = self.preSoftmaxA.assign(preSoftmaxA)\n op3 = self.preSoftmaxB.assign(preSoftmaxB)\n self.session.run([op1, op2, op3])\n\n\ndef fit_coin(file_key):\n X = []\n for line in get_obj_s3(file_key).read().decode(\"utf-8\").strip().split(sep=\"\\n\"):\n x = [1 if e == \"H\" else 0 for e in line.rstrip()]\n X.append(x)\n\n hmm = HMM(2)\n # the entire graph (including optimizer's variables) must be built\n # before calling global variables initializer!\n hmm.init_random(2)\n init = tf.global_variables_initializer()\n with tf.Session() as session:\n session.run(init)\n hmm.set_session(session)\n hmm.fit(X, max_iter=5)\n L = hmm.get_cost_multi(X).sum()\n print(\"Log Likelihood with fitted params: \", round(L, 3))\n\n # try true values\n # remember these must be in their \"pre-softmax\" forms\n pi = np.log(np.array([0.5, 0.5])).astype(np.float32)\n A = np.log(np.array([[0.1, 0.9], [0.8, 0.2]])).astype(np.float32)\n B = np.log(np.array([[0.6, 0.4], [0.3, 0.7]])).astype(np.float32)\n hmm.set(pi, A, B)\n L = hmm.get_cost_multi(X).sum()\n print(\"Log Likelihood with true params: \", round(L, 3))\n\n\n\nif __name__ == '__main__':\n key = \"coin_data.txt\"\n fit_coin(key)\n",
"Number of train samples: 50\n"
]
]
] | [
"markdown",
"code",
"markdown",
"code",
"markdown",
"code",
"markdown",
"code",
"markdown",
"code",
"markdown",
"code",
"markdown",
"code"
] | [
[
"markdown",
"markdown"
],
[
"code",
"code"
],
[
"markdown"
],
[
"code"
],
[
"markdown",
"markdown"
],
[
"code"
],
[
"markdown"
],
[
"code"
],
[
"markdown"
],
[
"code"
],
[
"markdown"
],
[
"code"
],
[
"markdown"
],
[
"code"
]
] |
d0912d5b4a97b58cfd72f730ac673153ed519e47 | 6,578 | ipynb | Jupyter Notebook | advent_of_code_2020/day 18 funny calculations/solution.ipynb | jvanelteren/advent_of_code | 3c547645250adb2d95ebac43d5d2111cdf9b09e9 | [
"MIT"
] | 1 | 2021-12-23T11:24:11.000Z | 2021-12-23T11:24:11.000Z | advent_of_code_2020/day 18 funny calculations/solution.ipynb | jvanelteren/advent_of_code | 3c547645250adb2d95ebac43d5d2111cdf9b09e9 | [
"MIT"
] | null | null | null | advent_of_code_2020/day 18 funny calculations/solution.ipynb | jvanelteren/advent_of_code | 3c547645250adb2d95ebac43d5d2111cdf9b09e9 | [
"MIT"
] | null | null | null | 26.959016 | 122 | 0.4436 | [
[
[
"# use python eval sometimes. great trickdefining a class and operator overloading ",
"_____no_output_____"
],
[
"import aoc\nf = open('input.txt')\nlines = [line.rstrip('\\n') for line in f]\nlines[0]",
"_____no_output_____"
],
[
"# part 1\ndef evaluate(line):\n ans = 0\n firstop = None\n operator = None\n wait = 0\n for i, ch in enumerate(line):\n if wait > 0: # still within parentheses, so ignore because the recursion took care of it\n wait -= 1\n continue\n if ch == '(': # recurse the rest\n ch, wait = evaluate(line[i+1:])\n if ch == ')': \n return firstop, i+1\n if isinstance(ch, int):\n if not firstop:\n firstop = ch\n else:\n firstop = eval(f'{firstop}{operator}{ch}')\n else:\n operator = ch\n return firstop\nans = 0\nfor line in lines:\n line = line.replace(\"(\",\"( \").replace(\")\",\" )\")\n line = aoc.to_int(line.split())\n ans+= evaluate(line)\nans\n",
"_____no_output_____"
],
[
"# part 2\ndef findclosing(line):\n count = 0\n for index, i in enumerate(line):\n if i == \"(\": count+=1\n if i == ')': count -=1\n if count == 0: return index\n\ndef evaluate(line):\n ans = 0\n while '(' in line: # get rid of all the parenthesis blocks\n first = line.index('(')\n last = findclosing(line[first:])+first \n line[first:last+1] = [evaluate(line[first+1:last])]\n while '+' in line: # reduce the '+' op_indexations\n op_index = line.index('+')\n line[op_index-1:op_index+2] = [line[op_index-1]+line[op_index+1]]\n\n while '*' in line: # finally, reduce the '*'\n op_index = line.index('*')\n line[op_index-1:op_index+2] = [line[op_index-1]*line[op_index+1]]\n return line[0]\n\nans = 0\nfor line in lines:\n line = line.replace(\"(\",\"( \").replace(\")\",\" )\")\n line = list(aoc.to_int(line.split()))\n ans += evaluate(line)\nans",
"_____no_output_____"
],
[
"# alternative solution from reddit, amazing idea with operator overloading\nimport re\nclass a(int):\n def __mul__(self, b):\n return a(int(self) + b)\n def __add__(self, b):\n return a(int(self) + b)\n def __sub__(self, b):\n return a(int(self) * b)\n\ndef ev(expr, pt2=False):\n expr = re.sub(r\"(\\d+)\", r\"a(\\1)\", expr)\n expr = expr.replace(\"*\", \"-\")\n if pt2:\n expr = expr.replace(\"+\", \"*\")\n return eval(expr, {}, {\"a\": a})\n\nprint(\"Part 1:\", sum(ev(l) for l in lines))\nprint(\"Part 2:\", sum(ev(l, pt2=True) for l in lines))",
"Part 1: 21993583522852\nPart 2: 122438593522757\n"
],
[
"# another one from sophiebits, have to study the regex a bit\n\ndef solve(line):\n def doInner(inner):\n # part 1:\n # while '+' in inner or '*' in inner:\n # inner = re.sub('^(\\d+)\\s*\\+\\s*(\\d+)', lambda m: str(int(m.group(1)) + int(m.group(2))), inner)\n # inner = re.sub('^(\\d+)\\s*\\*\\s*(\\d+)', lambda m: str(int(m.group(1)) * int(m.group(2))), inner)\n while '+' in inner:\n inner = re.sub('(\\d+)\\s*\\+\\s*(\\d+)', lambda m: str(int(m.group(1)) + int(m.group(2))), inner)\n while '*' in inner:\n inner = re.sub('(\\d+)\\s*\\*\\s*(\\d+)', lambda m: str(int(m.group(1)) * int(m.group(2))), inner)\n return inner\n while '(' in line:\n def doExpr(match):\n inner = match.group(1)\n return doInner(inner)\n line = re.sub(r'\\(([^()]+)\\)', doExpr, line)\n return doInner(line)\n\ntotal = 0\nfor line in lines:\n total += int(solve(line))\n\nprint(total)",
"122438593522757\n"
]
]
] | [
"code"
] | [
[
"code",
"code",
"code",
"code",
"code",
"code"
]
] |
d0913209f66d14b2fb2f95489eb278080e38b59c | 32,139 | ipynb | Jupyter Notebook | notebooks/Automagically making a table of all protein-protein interactions for two structures.ipynb | fomightez/pdbsum-binder | accddd4d17d053694241c1e91d34e9e2aac80b03 | [
"MIT"
] | null | null | null | notebooks/Automagically making a table of all protein-protein interactions for two structures.ipynb | fomightez/pdbsum-binder | accddd4d17d053694241c1e91d34e9e2aac80b03 | [
"MIT"
] | null | null | null | notebooks/Automagically making a table of all protein-protein interactions for two structures.ipynb | fomightez/pdbsum-binder | accddd4d17d053694241c1e91d34e9e2aac80b03 | [
"MIT"
] | 1 | 2021-06-23T23:46:41.000Z | 2021-06-23T23:46:41.000Z | 53.834171 | 1,169 | 0.686393 | [
[
[
"## Automagically making a table of all protein-protein interactions for two structures\n\nIf two structures use the same or essentially the same, you can use Python to make a table of all the pairs of the protein-protein interactions by the two structures that can be used as input for the pipeline described in an earlier notebook in this series, [Using snakemake to highlight changes in multiple protein-protein interactions via PDBsum data](Using%20snakemake%20to%20highlight%20changes%20in%20multiple%20protein-protein%20interactions%20via%20PDBsum%20data.ipynb). This notebook will step through this process.\n\nIt is important to note this won't work straight away if the protein chain designations by the same or closely related proteins differ between the two structures. Elements of the process to be used in this notebook could be adapted to do that; however, that would require some progamming knowledge beyond what will be covered here. I assume the number of times this would be needed would be limited and a table could more easily done by hand following along with this notebook as well as [Using snakemake to highlight changes in multiple protein-protein interactions via PDBsum data](Using%20snakemake%20to%20highlight%20changes%20in%20multiple%20protein-protein%20interactions%20via%20PDBsum%20data.ipynb). \n\nThe process relies on the fact that PDBsum shares under the 'Prot-prot' tab for every structure, the interacting pairs of proteins chains in an 'Interface summary' on the left side of the browser page. For example, look on the left of http://www.ebi.ac.uk/thornton-srv/databases/cgi-bin/pdbsum/GetPage.pl?pdbcode=6kiv&template=interfaces.html&c=999 . That link is what the PDBsum entry for the PDB idenitifer 6kiv leads to if you click on the 'Prot-prot' tab page from [the main PDBsum page for 6kiv](http://www.ebi.ac.uk/thornton-srv/databases/cgi-bin/pdbsum/GetPage.pl?pdbcode=6kiv&template=main.html). A utility script [pdb_code_to_prot_prot_interactions_via_PDBsum.py](https://github.com/fomightez/structurework/tree/master/pdbsum-utilities) is used to collect the designations listed there for each individual structure involved. Then in this notebook a little Python is used to generate the table file that can be used as described in [Using snakemake to highlight changes in multiple protein-protein interactions via PDBsum data](Using%20snakemake%20to%20highlight%20changes%20in%20multiple%20protein-protein%20interactions%20via%20PDBsum%20data.ipynb).\n\nAn example follows. It is meant to be adaptable to use the PDB codes of structures that interest you. You may wish to work through the demonstration first so you know what to expect.\n\n----",
"_____no_output_____"
],
[
"The next cell is used to define the structures of interest. The PDB code identifiers are supplied.",
"_____no_output_____"
]
],
[
[
"structure1 = \"6kiz\"\nstructure2 = \"6kix\"",
"_____no_output_____"
]
],
[
[
"The next cell gets the script `pdb_code_to_prot_prot_interactions_via_PDBsum.py` (see [here](https://github.com/fomightez/structurework/tree/master/pdbsum-utilities)) that will get the 'Interface Summary' information for each individual structure. This is the equivalent to the Summary on the left side of the 'Prot-prot' tab.",
"_____no_output_____"
]
],
[
[
"import os\nfile_needed = \"pdb_code_to_prot_prot_interactions_via_PDBsum.py\"\nif not os.path.isfile(file_needed):\n !curl -OL https://raw.githubusercontent.com/fomightez/structurework/master/pdbsum-utilities/{file_needed}",
"_____no_output_____"
]
],
[
[
"Import the main function of that script by running the next cell.",
"_____no_output_____"
]
],
[
[
"from pdb_code_to_prot_prot_interactions_via_PDBsum import pdb_code_to_prot_prot_interactions_via_PDBsum",
"_____no_output_____"
]
],
[
[
"The next cell gets the interaction summary for each structure and to get the pairs need to build the table described at the top of [Using snakemake to highlight changes in multiple protein-protein interactions via PDBsum data](Using%20snakemake%20to%20highlight%20changes%20in%20multiple%20protein-protein%20interactions%20via%20PDBsum%20data.ipynb).",
"_____no_output_____"
]
],
[
[
"structure1_il = pdb_code_to_prot_prot_interactions_via_PDBsum(structure1)\nstructure2_il = pdb_code_to_prot_prot_interactions_via_PDBsum(structure2)\ni_union = set(structure1_il).union(set(structure2_il))",
"_____no_output_____"
]
],
[
[
"In this case the pairs of both are the same; however, the script is written to not fail if there was extra proteins present in the other. Specficially, the interacting pairs of proteins for both are checked because if one had additional chain, by getting the listing of both structures and making the union, the combinations for all would be in the list of pairs `i_union`.",
"_____no_output_____"
],
[
"Next the union of all the pairs is used to make a table like constructed at the top of [Using snakemake to highlight changes in multiple protein-protein interactions via PDBsum data](Using%20snakemake%20to%20highlight%20changes%20in%20multiple%20protein-protein%20interactions%20via%20PDBsum%20data.ipynb).",
"_____no_output_____"
]
],
[
[
"s = \"\"\nfor pair in list(i_union):\n s+= f\"{structure1} {pair[0]} {pair[1]} {structure2} {pair[0]} {pair[1]}\\n\"\n%store s >int_matrix.txt",
"Writing 's' (str) to file 'int_matrix.txt'.\n"
]
],
[
[
"The table has now been stored as `int_matrix.txt`. Open the file from the Jupyter dashboard to verify. Or just run the next cell to see the contents of the file.",
"_____no_output_____"
]
],
[
[
"!cat int_matrix.txt",
"6kiz K R 6kix K R\r\n6kiz B H 6kix B H\r\n6kiz C E 6kix C E\r\n6kiz B D 6kix B D\r\n6kiz G H 6kix G H\r\n6kiz F H 6kix F H\r\n6kiz D F 6kix D F\r\n6kiz A N 6kix A N\r\n6kiz N T 6kix N T\r\n6kiz C D 6kix C D\r\n6kiz A B 6kix A B\r\n6kiz C F 6kix C F\r\n6kiz K T 6kix K T\r\n6kiz A E 6kix A E\r\n6kiz A G 6kix A G\r\n6kiz C K 6kix C K\r\n6kiz E F 6kix E F\r\n6kiz C G 6kix C G\r\n6kiz B G 6kix B G\r\n6kiz N R 6kix N R\r\n6kiz D N 6kix D N\r\n6kiz B N 6kix B N\r\n6kiz K N 6kix K N\r\n"
]
],
[
[
"That's the table in the file that needed to be made. The rest of the process pickes up with 'Step #3' of [Using snakemake to highlight changes in multiple protein-protein interactions via PDBsum data](Using%20snakemake%20to%20highlight%20changes%20in%20multiple%20protein-protein%20interactions%20via%20PDBsum%20data.ipynb).\n\nTo make that clear, this following cell will run the snakemake pipeline. Consult the subsequent steps of [Using snakemake to highlight changes in multiple protein-protein interactions via PDBsum data](Using%20snakemake%20to%20highlight%20changes%20in%20multiple%20protein-protein%20interactions%20via%20PDBsum%20data.ipynb) to see what to do after it completes all the possible pairs.",
"_____no_output_____"
]
],
[
[
"!snakemake --cores 1",
"\u001b[33mBuilding DAG of jobs...\u001b[0m\n\u001b[33mUsing shell: /bin/bash\u001b[0m\n\u001b[33mProvided cores: 1 (use --cores to define parallelism)\u001b[0m\n\u001b[33mRules claiming more threads will be scaled down.\u001b[0m\n\u001b[33mJob counts:\n\tcount\tjobs\n\t1\tall\n\t23\tconvert_scripts_to_nb_and_run_using_jupytext\n\t1\tmake_archive\n\t1\tread_table_and_create_py\n\t26\u001b[0m\n\u001b[32m\u001b[0m\n\u001b[32m[Mon Feb 8 22:11:09 2021]\u001b[0m\n\u001b[32mrule read_table_and_create_py:\n input: int_matrix.txt\n output: interactions_report_for_6kiz_K_R_6kix_K_R.py, interactions_report_for_6kiz_B_H_6kix_B_H.py, interactions_report_for_6kiz_C_E_6kix_C_E.py, interactions_report_for_6kiz_B_D_6kix_B_D.py, interactions_report_for_6kiz_G_H_6kix_G_H.py, interactions_report_for_6kiz_F_H_6kix_F_H.py, interactions_report_for_6kiz_D_F_6kix_D_F.py, interactions_report_for_6kiz_A_N_6kix_A_N.py, interactions_report_for_6kiz_N_T_6kix_N_T.py, interactions_report_for_6kiz_C_D_6kix_C_D.py, interactions_report_for_6kiz_A_B_6kix_A_B.py, interactions_report_for_6kiz_C_F_6kix_C_F.py, interactions_report_for_6kiz_K_T_6kix_K_T.py, interactions_report_for_6kiz_A_E_6kix_A_E.py, interactions_report_for_6kiz_A_G_6kix_A_G.py, interactions_report_for_6kiz_C_K_6kix_C_K.py, interactions_report_for_6kiz_E_F_6kix_E_F.py, interactions_report_for_6kiz_C_G_6kix_C_G.py, interactions_report_for_6kiz_B_G_6kix_B_G.py, interactions_report_for_6kiz_N_R_6kix_N_R.py, interactions_report_for_6kiz_D_N_6kix_D_N.py, interactions_report_for_6kiz_B_N_6kix_B_N.py, interactions_report_for_6kiz_K_N_6kix_K_N.py\n jobid: 3\u001b[0m\n\u001b[32m\u001b[0m\n\u001b[33mJob counts:\n\tcount\tjobs\n\t1\tread_table_and_create_py\n\t1\u001b[0m\n\u001b[32m[Mon Feb 8 22:11:10 2021]\u001b[0m\n\u001b[32mFinished job 3.\u001b[0m\n\u001b[32m1 of 26 steps (4%) done\u001b[0m\n\u001b[32m\u001b[0m\n\u001b[32m[Mon Feb 8 22:11:10 2021]\u001b[0m\n\u001b[32mrule convert_scripts_to_nb_and_run_using_jupytext:\n input: interactions_report_for_6kiz_B_N_6kix_B_N.py\n output: interactions_report_for_6kiz_B_N_6kix_B_N.ipynb\n jobid: 24\n wildcards: details=6kiz_B_N_6kix_B_N\u001b[0m\n\u001b[32m\u001b[0m\n[jupytext] Reading interactions_report_for_6kiz_B_N_6kix_B_N.py in format py\n[jupytext] Executing notebook with kernel python3\n[jupytext] Writing interactions_report_for_6kiz_B_N_6kix_B_N.ipynb\n\u001b[32m[Mon Feb 8 22:11:25 2021]\u001b[0m\n\u001b[32mFinished job 24.\u001b[0m\n\u001b[32m2 of 26 steps (8%) done\u001b[0m\n\u001b[32m\u001b[0m\n\u001b[32m[Mon Feb 8 22:11:25 2021]\u001b[0m\n\u001b[32mrule convert_scripts_to_nb_and_run_using_jupytext:\n input: interactions_report_for_6kiz_A_N_6kix_A_N.py\n output: interactions_report_for_6kiz_A_N_6kix_A_N.ipynb\n jobid: 10\n wildcards: details=6kiz_A_N_6kix_A_N\u001b[0m\n\u001b[32m\u001b[0m\n[jupytext] Reading interactions_report_for_6kiz_A_N_6kix_A_N.py in format py\n[jupytext] Executing notebook with kernel python3\n[jupytext] Writing interactions_report_for_6kiz_A_N_6kix_A_N.ipynb\n\u001b[32m[Mon Feb 8 22:11:32 2021]\u001b[0m\n\u001b[32mFinished job 10.\u001b[0m\n\u001b[32m3 of 26 steps (12%) done\u001b[0m\n\u001b[32m\u001b[0m\n\u001b[32m[Mon Feb 8 22:11:32 2021]\u001b[0m\n\u001b[32mrule convert_scripts_to_nb_and_run_using_jupytext:\n input: interactions_report_for_6kiz_B_H_6kix_B_H.py\n output: interactions_report_for_6kiz_B_H_6kix_B_H.ipynb\n jobid: 4\n wildcards: details=6kiz_B_H_6kix_B_H\u001b[0m\n\u001b[32m\u001b[0m\n[jupytext] Reading interactions_report_for_6kiz_B_H_6kix_B_H.py in format py\n[jupytext] Executing notebook with kernel python3\n[jupytext] Writing interactions_report_for_6kiz_B_H_6kix_B_H.ipynb\n\u001b[32m[Mon Feb 8 22:11:40 2021]\u001b[0m\n\u001b[32mFinished job 4.\u001b[0m\n\u001b[32m4 of 26 steps (15%) done\u001b[0m\n\u001b[32m\u001b[0m\n\u001b[32m[Mon Feb 8 22:11:40 2021]\u001b[0m\n\u001b[32mrule convert_scripts_to_nb_and_run_using_jupytext:\n input: interactions_report_for_6kiz_C_K_6kix_C_K.py\n output: interactions_report_for_6kiz_C_K_6kix_C_K.ipynb\n jobid: 18\n wildcards: details=6kiz_C_K_6kix_C_K\u001b[0m\n\u001b[32m\u001b[0m\n[jupytext] Reading interactions_report_for_6kiz_C_K_6kix_C_K.py in format py\n[jupytext] Executing notebook with kernel python3\n[jupytext] Writing interactions_report_for_6kiz_C_K_6kix_C_K.ipynb\n\u001b[32m[Mon Feb 8 22:11:48 2021]\u001b[0m\n\u001b[32mFinished job 18.\u001b[0m\n\u001b[32m5 of 26 steps (19%) done\u001b[0m\n\u001b[32m\u001b[0m\n\u001b[32m[Mon Feb 8 22:11:48 2021]\u001b[0m\n\u001b[32mrule convert_scripts_to_nb_and_run_using_jupytext:\n input: interactions_report_for_6kiz_N_T_6kix_N_T.py\n output: interactions_report_for_6kiz_N_T_6kix_N_T.ipynb\n jobid: 11\n wildcards: details=6kiz_N_T_6kix_N_T\u001b[0m\n\u001b[32m\u001b[0m\n[jupytext] Reading interactions_report_for_6kiz_N_T_6kix_N_T.py in format py\n[jupytext] Executing notebook with kernel python3\n[jupytext] Writing interactions_report_for_6kiz_N_T_6kix_N_T.ipynb\n\u001b[32m[Mon Feb 8 22:11:56 2021]\u001b[0m\n\u001b[32mFinished job 11.\u001b[0m\n\u001b[32m6 of 26 steps (23%) done\u001b[0m\n\u001b[32m\u001b[0m\n\u001b[32m[Mon Feb 8 22:11:56 2021]\u001b[0m\n\u001b[32mrule convert_scripts_to_nb_and_run_using_jupytext:\n input: interactions_report_for_6kiz_G_H_6kix_G_H.py\n output: interactions_report_for_6kiz_G_H_6kix_G_H.ipynb\n jobid: 7\n wildcards: details=6kiz_G_H_6kix_G_H\u001b[0m\n\u001b[32m\u001b[0m\n[jupytext] Reading interactions_report_for_6kiz_G_H_6kix_G_H.py in format py\n[jupytext] Executing notebook with kernel python3\n[jupytext] Writing interactions_report_for_6kiz_G_H_6kix_G_H.ipynb\n\u001b[32m[Mon Feb 8 22:12:04 2021]\u001b[0m\n\u001b[32mFinished job 7.\u001b[0m\n\u001b[32m7 of 26 steps (27%) done\u001b[0m\n\u001b[32m\u001b[0m\n\u001b[32m[Mon Feb 8 22:12:04 2021]\u001b[0m\n\u001b[32mrule convert_scripts_to_nb_and_run_using_jupytext:\n input: interactions_report_for_6kiz_B_G_6kix_B_G.py\n output: interactions_report_for_6kiz_B_G_6kix_B_G.ipynb\n jobid: 21\n wildcards: details=6kiz_B_G_6kix_B_G\u001b[0m\n\u001b[32m\u001b[0m\n[jupytext] Reading interactions_report_for_6kiz_B_G_6kix_B_G.py in format py\n[jupytext] Executing notebook with kernel python3\n[jupytext] Writing interactions_report_for_6kiz_B_G_6kix_B_G.ipynb\n\u001b[32m[Mon Feb 8 22:12:12 2021]\u001b[0m\n\u001b[32mFinished job 21.\u001b[0m\n\u001b[32m8 of 26 steps (31%) done\u001b[0m\n\u001b[32m\u001b[0m\n\u001b[32m[Mon Feb 8 22:12:12 2021]\u001b[0m\n\u001b[32mrule convert_scripts_to_nb_and_run_using_jupytext:\n input: interactions_report_for_6kiz_D_F_6kix_D_F.py\n output: interactions_report_for_6kiz_D_F_6kix_D_F.ipynb\n jobid: 9\n wildcards: details=6kiz_D_F_6kix_D_F\u001b[0m\n\u001b[32m\u001b[0m\n[jupytext] Reading interactions_report_for_6kiz_D_F_6kix_D_F.py in format py\n[jupytext] Executing notebook with kernel python3\n[jupytext] Writing interactions_report_for_6kiz_D_F_6kix_D_F.ipynb\n\u001b[32m[Mon Feb 8 22:12:19 2021]\u001b[0m\n\u001b[32mFinished job 9.\u001b[0m\n\u001b[32m9 of 26 steps (35%) done\u001b[0m\n\u001b[32m\u001b[0m\n\u001b[32m[Mon Feb 8 22:12:19 2021]\u001b[0m\n\u001b[32mrule convert_scripts_to_nb_and_run_using_jupytext:\n input: interactions_report_for_6kiz_D_N_6kix_D_N.py\n output: interactions_report_for_6kiz_D_N_6kix_D_N.ipynb\n jobid: 23\n wildcards: details=6kiz_D_N_6kix_D_N\u001b[0m\n\u001b[32m\u001b[0m\n[jupytext] Reading interactions_report_for_6kiz_D_N_6kix_D_N.py in format py\n[jupytext] Executing notebook with kernel python3\n[jupytext] Writing interactions_report_for_6kiz_D_N_6kix_D_N.ipynb\n\u001b[32m[Mon Feb 8 22:12:27 2021]\u001b[0m\n\u001b[32mFinished job 23.\u001b[0m\n\u001b[32m10 of 26 steps (38%) done\u001b[0m\n\u001b[32m\u001b[0m\n\u001b[32m[Mon Feb 8 22:12:27 2021]\u001b[0m\n\u001b[32mrule convert_scripts_to_nb_and_run_using_jupytext:\n input: interactions_report_for_6kiz_C_D_6kix_C_D.py\n output: interactions_report_for_6kiz_C_D_6kix_C_D.ipynb\n jobid: 12\n wildcards: details=6kiz_C_D_6kix_C_D\u001b[0m\n\u001b[32m\u001b[0m\n[jupytext] Reading interactions_report_for_6kiz_C_D_6kix_C_D.py in format py\n[jupytext] Executing notebook with kernel python3\n[jupytext] Writing interactions_report_for_6kiz_C_D_6kix_C_D.ipynb\n\u001b[32m[Mon Feb 8 22:12:35 2021]\u001b[0m\n\u001b[32mFinished job 12.\u001b[0m\n\u001b[32m11 of 26 steps (42%) done\u001b[0m\n\u001b[32m\u001b[0m\n\u001b[32m[Mon Feb 8 22:12:35 2021]\u001b[0m\n\u001b[32mrule convert_scripts_to_nb_and_run_using_jupytext:\n input: interactions_report_for_6kiz_C_F_6kix_C_F.py\n output: interactions_report_for_6kiz_C_F_6kix_C_F.ipynb\n jobid: 14\n wildcards: details=6kiz_C_F_6kix_C_F\u001b[0m\n\u001b[32m\u001b[0m\n[jupytext] Reading interactions_report_for_6kiz_C_F_6kix_C_F.py in format py\n[jupytext] Executing notebook with kernel python3\n[jupytext] Writing interactions_report_for_6kiz_C_F_6kix_C_F.ipynb\n\u001b[32m[Mon Feb 8 22:12:44 2021]\u001b[0m\n\u001b[32mFinished job 14.\u001b[0m\n\u001b[32m12 of 26 steps (46%) done\u001b[0m\n\u001b[32m\u001b[0m\n\u001b[32m[Mon Feb 8 22:12:44 2021]\u001b[0m\n\u001b[32mrule convert_scripts_to_nb_and_run_using_jupytext:\n input: interactions_report_for_6kiz_E_F_6kix_E_F.py\n output: interactions_report_for_6kiz_E_F_6kix_E_F.ipynb\n jobid: 19\n wildcards: details=6kiz_E_F_6kix_E_F\u001b[0m\n\u001b[32m\u001b[0m\n[jupytext] Reading interactions_report_for_6kiz_E_F_6kix_E_F.py in format py\n[jupytext] Executing notebook with kernel python3\n[jupytext] Writing interactions_report_for_6kiz_E_F_6kix_E_F.ipynb\n\u001b[32m[Mon Feb 8 22:12:52 2021]\u001b[0m\n\u001b[32mFinished job 19.\u001b[0m\n\u001b[32m13 of 26 steps (50%) done\u001b[0m\n\u001b[32m\u001b[0m\n\u001b[32m[Mon Feb 8 22:12:52 2021]\u001b[0m\n\u001b[32mrule convert_scripts_to_nb_and_run_using_jupytext:\n input: interactions_report_for_6kiz_K_R_6kix_K_R.py\n output: interactions_report_for_6kiz_K_R_6kix_K_R.ipynb\n jobid: 2\n wildcards: details=6kiz_K_R_6kix_K_R\u001b[0m\n\u001b[32m\u001b[0m\n[jupytext] Reading interactions_report_for_6kiz_K_R_6kix_K_R.py in format py\n[jupytext] Executing notebook with kernel python3\n[jupytext] Writing interactions_report_for_6kiz_K_R_6kix_K_R.ipynb\n\u001b[32m[Mon Feb 8 22:13:01 2021]\u001b[0m\n\u001b[32mFinished job 2.\u001b[0m\n\u001b[32m14 of 26 steps (54%) done\u001b[0m\n\u001b[32m\u001b[0m\n\u001b[32m[Mon Feb 8 22:13:01 2021]\u001b[0m\n\u001b[32mrule convert_scripts_to_nb_and_run_using_jupytext:\n input: interactions_report_for_6kiz_A_E_6kix_A_E.py\n output: interactions_report_for_6kiz_A_E_6kix_A_E.ipynb\n jobid: 16\n wildcards: details=6kiz_A_E_6kix_A_E\u001b[0m\n\u001b[32m\u001b[0m\n[jupytext] Reading interactions_report_for_6kiz_A_E_6kix_A_E.py in format py\n[jupytext] Executing notebook with kernel python3\n[jupytext] Writing interactions_report_for_6kiz_A_E_6kix_A_E.ipynb\n\u001b[32m[Mon Feb 8 22:13:10 2021]\u001b[0m\n\u001b[32mFinished job 16.\u001b[0m\n\u001b[32m15 of 26 steps (58%) done\u001b[0m\n\u001b[32m\u001b[0m\n\u001b[32m[Mon Feb 8 22:13:10 2021]\u001b[0m\n\u001b[32mrule convert_scripts_to_nb_and_run_using_jupytext:\n input: interactions_report_for_6kiz_C_G_6kix_C_G.py\n output: interactions_report_for_6kiz_C_G_6kix_C_G.ipynb\n jobid: 20\n wildcards: details=6kiz_C_G_6kix_C_G\u001b[0m\n\u001b[32m\u001b[0m\n[jupytext] Reading interactions_report_for_6kiz_C_G_6kix_C_G.py in format py\n[jupytext] Executing notebook with kernel python3\n[jupytext] Writing interactions_report_for_6kiz_C_G_6kix_C_G.ipynb\n\u001b[32m[Mon Feb 8 22:13:19 2021]\u001b[0m\n\u001b[32mFinished job 20.\u001b[0m\n\u001b[32m16 of 26 steps (62%) done\u001b[0m\n\u001b[32m\u001b[0m\n\u001b[32m[Mon Feb 8 22:13:19 2021]\u001b[0m\n\u001b[32mrule convert_scripts_to_nb_and_run_using_jupytext:\n input: interactions_report_for_6kiz_B_D_6kix_B_D.py\n output: interactions_report_for_6kiz_B_D_6kix_B_D.ipynb\n jobid: 6\n wildcards: details=6kiz_B_D_6kix_B_D\u001b[0m\n\u001b[32m\u001b[0m\n[jupytext] Reading interactions_report_for_6kiz_B_D_6kix_B_D.py in format py\n[jupytext] Executing notebook with kernel python3\n[jupytext] Writing interactions_report_for_6kiz_B_D_6kix_B_D.ipynb\n\u001b[32m[Mon Feb 8 22:13:27 2021]\u001b[0m\n\u001b[32mFinished job 6.\u001b[0m\n\u001b[32m17 of 26 steps (65%) done\u001b[0m\n\u001b[32m\u001b[0m\n\u001b[32m[Mon Feb 8 22:13:27 2021]\u001b[0m\n\u001b[32mrule convert_scripts_to_nb_and_run_using_jupytext:\n input: interactions_report_for_6kiz_F_H_6kix_F_H.py\n output: interactions_report_for_6kiz_F_H_6kix_F_H.ipynb\n jobid: 8\n wildcards: details=6kiz_F_H_6kix_F_H\u001b[0m\n\u001b[32m\u001b[0m\n[jupytext] Reading interactions_report_for_6kiz_F_H_6kix_F_H.py in format py\n[jupytext] Executing notebook with kernel python3\n[jupytext] Writing interactions_report_for_6kiz_F_H_6kix_F_H.ipynb\n\u001b[32m[Mon Feb 8 22:13:35 2021]\u001b[0m\n\u001b[32mFinished job 8.\u001b[0m\n\u001b[32m18 of 26 steps (69%) done\u001b[0m\n\u001b[32m\u001b[0m\n\u001b[32m[Mon Feb 8 22:13:35 2021]\u001b[0m\n\u001b[32mrule convert_scripts_to_nb_and_run_using_jupytext:\n input: interactions_report_for_6kiz_N_R_6kix_N_R.py\n output: interactions_report_for_6kiz_N_R_6kix_N_R.ipynb\n jobid: 22\n wildcards: details=6kiz_N_R_6kix_N_R\u001b[0m\n\u001b[32m\u001b[0m\n[jupytext] Reading interactions_report_for_6kiz_N_R_6kix_N_R.py in format py\n[jupytext] Executing notebook with kernel python3\n[jupytext] Writing interactions_report_for_6kiz_N_R_6kix_N_R.ipynb\n\u001b[32m[Mon Feb 8 22:13:43 2021]\u001b[0m\n\u001b[32mFinished job 22.\u001b[0m\n\u001b[32m19 of 26 steps (73%) done\u001b[0m\n\u001b[32m\u001b[0m\n\u001b[32m[Mon Feb 8 22:13:43 2021]\u001b[0m\n\u001b[32mrule convert_scripts_to_nb_and_run_using_jupytext:\n input: interactions_report_for_6kiz_K_N_6kix_K_N.py\n output: interactions_report_for_6kiz_K_N_6kix_K_N.ipynb\n jobid: 25\n wildcards: details=6kiz_K_N_6kix_K_N\u001b[0m\n\u001b[32m\u001b[0m\n[jupytext] Reading interactions_report_for_6kiz_K_N_6kix_K_N.py in format py\n[jupytext] Executing notebook with kernel python3\n[jupytext] Writing interactions_report_for_6kiz_K_N_6kix_K_N.ipynb\n\u001b[32m[Mon Feb 8 22:13:53 2021]\u001b[0m\n\u001b[32mFinished job 25.\u001b[0m\n\u001b[32m20 of 26 steps (77%) done\u001b[0m\n\u001b[32m\u001b[0m\n\u001b[32m[Mon Feb 8 22:13:53 2021]\u001b[0m\n\u001b[32mrule convert_scripts_to_nb_and_run_using_jupytext:\n input: interactions_report_for_6kiz_A_B_6kix_A_B.py\n output: interactions_report_for_6kiz_A_B_6kix_A_B.ipynb\n jobid: 13\n wildcards: details=6kiz_A_B_6kix_A_B\u001b[0m\n\u001b[32m\u001b[0m\n[jupytext] Reading interactions_report_for_6kiz_A_B_6kix_A_B.py in format py\n[jupytext] Executing notebook with kernel python3\n[jupytext] Writing interactions_report_for_6kiz_A_B_6kix_A_B.ipynb\n\u001b[32m[Mon Feb 8 22:14:01 2021]\u001b[0m\n\u001b[32mFinished job 13.\u001b[0m\n\u001b[32m21 of 26 steps (81%) done\u001b[0m\n\u001b[32m\u001b[0m\n\u001b[32m[Mon Feb 8 22:14:01 2021]\u001b[0m\n\u001b[32mrule convert_scripts_to_nb_and_run_using_jupytext:\n input: interactions_report_for_6kiz_K_T_6kix_K_T.py\n output: interactions_report_for_6kiz_K_T_6kix_K_T.ipynb\n jobid: 15\n wildcards: details=6kiz_K_T_6kix_K_T\u001b[0m\n\u001b[32m\u001b[0m\n[jupytext] Reading interactions_report_for_6kiz_K_T_6kix_K_T.py in format py\n[jupytext] Executing notebook with kernel python3\n[jupytext] Writing interactions_report_for_6kiz_K_T_6kix_K_T.ipynb\n\u001b[32m[Mon Feb 8 22:14:11 2021]\u001b[0m\n\u001b[32mFinished job 15.\u001b[0m\n\u001b[32m22 of 26 steps (85%) done\u001b[0m\n\u001b[32m\u001b[0m\n\u001b[32m[Mon Feb 8 22:14:11 2021]\u001b[0m\n\u001b[32mrule convert_scripts_to_nb_and_run_using_jupytext:\n input: interactions_report_for_6kiz_A_G_6kix_A_G.py\n output: interactions_report_for_6kiz_A_G_6kix_A_G.ipynb\n jobid: 17\n wildcards: details=6kiz_A_G_6kix_A_G\u001b[0m\n\u001b[32m\u001b[0m\n[jupytext] Reading interactions_report_for_6kiz_A_G_6kix_A_G.py in format py\n[jupytext] Executing notebook with kernel python3\n[jupytext] Writing interactions_report_for_6kiz_A_G_6kix_A_G.ipynb\n\u001b[32m[Mon Feb 8 22:14:19 2021]\u001b[0m\n\u001b[32mFinished job 17.\u001b[0m\n\u001b[32m23 of 26 steps (88%) done\u001b[0m\n\u001b[32m\u001b[0m\n\u001b[32m[Mon Feb 8 22:14:19 2021]\u001b[0m\n\u001b[32mrule convert_scripts_to_nb_and_run_using_jupytext:\n input: interactions_report_for_6kiz_C_E_6kix_C_E.py\n output: interactions_report_for_6kiz_C_E_6kix_C_E.ipynb\n jobid: 5\n wildcards: details=6kiz_C_E_6kix_C_E\u001b[0m\n\u001b[32m\u001b[0m\n[jupytext] Reading interactions_report_for_6kiz_C_E_6kix_C_E.py in format py\n[jupytext] Executing notebook with kernel python3\n[jupytext] Writing interactions_report_for_6kiz_C_E_6kix_C_E.ipynb\n\u001b[32m[Mon Feb 8 22:14:28 2021]\u001b[0m\n\u001b[32mFinished job 5.\u001b[0m\n\u001b[32m24 of 26 steps (92%) done\u001b[0m\n\u001b[32m\u001b[0m\n\u001b[32m[Mon Feb 8 22:14:28 2021]\u001b[0m\n\u001b[32mrule make_archive:\n input: interactions_report_for_6kiz_K_R_6kix_K_R.ipynb, interactions_report_for_6kiz_B_H_6kix_B_H.ipynb, interactions_report_for_6kiz_C_E_6kix_C_E.ipynb, interactions_report_for_6kiz_B_D_6kix_B_D.ipynb, interactions_report_for_6kiz_G_H_6kix_G_H.ipynb, interactions_report_for_6kiz_F_H_6kix_F_H.ipynb, interactions_report_for_6kiz_D_F_6kix_D_F.ipynb, interactions_report_for_6kiz_A_N_6kix_A_N.ipynb, interactions_report_for_6kiz_N_T_6kix_N_T.ipynb, interactions_report_for_6kiz_C_D_6kix_C_D.ipynb, interactions_report_for_6kiz_A_B_6kix_A_B.ipynb, interactions_report_for_6kiz_C_F_6kix_C_F.ipynb, interactions_report_for_6kiz_K_T_6kix_K_T.ipynb, interactions_report_for_6kiz_A_E_6kix_A_E.ipynb, interactions_report_for_6kiz_A_G_6kix_A_G.ipynb, interactions_report_for_6kiz_C_K_6kix_C_K.ipynb, interactions_report_for_6kiz_E_F_6kix_E_F.ipynb, interactions_report_for_6kiz_C_G_6kix_C_G.ipynb, interactions_report_for_6kiz_B_G_6kix_B_G.ipynb, interactions_report_for_6kiz_N_R_6kix_N_R.ipynb, interactions_report_for_6kiz_D_N_6kix_D_N.ipynb, interactions_report_for_6kiz_B_N_6kix_B_N.ipynb, interactions_report_for_6kiz_K_N_6kix_K_N.ipynb\n output: interactions_report_nbsFeb0820212211.tar.gz\n jobid: 1\u001b[0m\n\u001b[32m\u001b[0m\nBe sure to download interactions_report_nbsFeb0820212211.tar.gz.\n\u001b[32m[Mon Feb 8 22:14:28 2021]\u001b[0m\n\u001b[32mFinished job 1.\u001b[0m\n\u001b[32m25 of 26 steps (96%) done\u001b[0m\n\u001b[32m\u001b[0m\n\u001b[32m[Mon Feb 8 22:14:28 2021]\u001b[0m\n\u001b[32mlocalrule all:\n input: interactions_report_nbsFeb0820212211.tar.gz\n jobid: 0\u001b[0m\n\u001b[32m\u001b[0m\n\u001b[32m[Mon Feb 8 22:14:28 2021]\u001b[0m\n\u001b[32mFinished job 0.\u001b[0m\n\u001b[32m26 of 26 steps (100%) done\u001b[0m\n\u001b[33mComplete log: /home/jovyan/notebooks/.snakemake/log/2021-02-08T221108.464378.snakemake.log\u001b[0m\n"
]
],
[
[
"Now change the structures used to your favorites and re-run the notebook. If the chains are the same in your two structures, you'll generate all the reports for all the interacting pairs of proteins upon doing that.\n\n------\n\nEnjoy!",
"_____no_output_____"
]
]
] | [
"markdown",
"code",
"markdown",
"code",
"markdown",
"code",
"markdown",
"code",
"markdown",
"code",
"markdown",
"code",
"markdown",
"code",
"markdown"
] | [
[
"markdown",
"markdown"
],
[
"code"
],
[
"markdown"
],
[
"code"
],
[
"markdown"
],
[
"code"
],
[
"markdown"
],
[
"code"
],
[
"markdown",
"markdown"
],
[
"code"
],
[
"markdown"
],
[
"code"
],
[
"markdown"
],
[
"code"
],
[
"markdown"
]
] |
d0913fd8d8e9b031e71ce4f65d0ee3e3f130bc61 | 2,837 | ipynb | Jupyter Notebook | Chapter07/Exercise99/Exercise99.ipynb | stuffstuffstuf1/The-Python-Workshop | b529995980a7a8f8f09e9d2f8dd20d6e4d6acb80 | [
"MIT"
] | 238 | 2019-12-13T15:44:34.000Z | 2022-03-21T05:38:21.000Z | Chapter07/Exercise99/Exercise99.ipynb | stuffstuffstuf1/The-Python-Workshop | b529995980a7a8f8f09e9d2f8dd20d6e4d6acb80 | [
"MIT"
] | 8 | 2020-05-04T03:33:29.000Z | 2022-03-12T00:47:26.000Z | Chapter07/Exercise99/Exercise99.ipynb | stuffstuffstuf1/The-Python-Workshop | b529995980a7a8f8f09e9d2f8dd20d6e4d6acb80 | [
"MIT"
] | 345 | 2019-10-08T09:15:11.000Z | 2022-03-31T18:28:03.000Z | 20.120567 | 105 | 0.491012 | [
[
[
"# This is a familiar Python for..in loop for iterating over an array\ncubes = []\nfor x in [1,2,3,4,5]:\n cubes.append(x**3)\nprint(cubes)",
"[1, 8, 27, 64, 125]\n"
],
[
"# The list comprehension achieves the same thing\ncubes = [x**3 for x in [1,2,3,4,5]]\nprint(cubes)\n\n",
"[1, 8, 27, 64, 125]\n"
],
[
"cubes = [x**3 for x in range(1,6)]\nprint(cubes)",
"[1, 8, 27, 64, 125]\n"
],
[
"names = [\"Graham Chapman\", \"John Cleese\", \"Terry Gilliam\", \"Eric Idle\", \"Terry Jones\"]\nprint([name.upper() for name in names if name.startswith(\"T\")])\n\n",
"['TERRY GILLIAM', 'TERRY JONES']\n"
]
]
] | [
"code"
] | [
[
"code",
"code",
"code",
"code"
]
] |
d091476113246b2e2e02c5d830f9c71c73a01c96 | 31,324 | ipynb | Jupyter Notebook | Model Buidling/Perfumes.ipynb | sangram6n/inventory_management_car-decors_sales_forecasting | 33bf7ac6f6526ce2c8a3cb31a576e03a68a8e4dc | [
"MIT"
] | null | null | null | Model Buidling/Perfumes.ipynb | sangram6n/inventory_management_car-decors_sales_forecasting | 33bf7ac6f6526ce2c8a3cb31a576e03a68a8e4dc | [
"MIT"
] | null | null | null | Model Buidling/Perfumes.ipynb | sangram6n/inventory_management_car-decors_sales_forecasting | 33bf7ac6f6526ce2c8a3cb31a576e03a68a8e4dc | [
"MIT"
] | null | null | null | 28.816927 | 197 | 0.548749 | [
[
[
"# Car Decor Sales Forecasting - Perfumes",
"_____no_output_____"
]
],
[
[
"Summary of the Code below :\n1. Establish MySQL Connection and load data\n2. Data Preprocessing (Typecasting and Resampling daily data to monthly)\n3. Visualizing Rolling statistics to observe variation in mean and standard deviation for selected Feature.\n4. Checking for Data Stationarity using Augmented Dickey-Fuller Test for the feature\n5. Hyper-parameter Tuning using ACF and PACF plots for building SARIMA Model (this process takes little time)\n6. Models\n (a) SARIMA\n (b) HoltWinters Exponential Smoothing with Additive Seasonality & Additive Trend\n (c) FB Prophet\n (d) Auto Time Series\n7. Evaluation of the Models\n8. Saving the model with least MAPE\n9. Loading saved model (.pkl) to predict sales for 12 months.\n10. Closing MySQL Connection",
"_____no_output_____"
]
],
[
[
"###### Importing Libraries",
"_____no_output_____"
]
],
[
[
"import numpy as np\nimport pandas as pd\nimport seaborn as sns\nimport matplotlib.pyplot as plt\n%matplotlib inline\n\nfrom sklearn.metrics import mean_squared_error\nfrom math import sqrt\n\n# Connecting Python to MySQL for fetching data \nimport mysql.connector\n\nimport warnings\nfrom statsmodels.tools.sm_exceptions import ConvergenceWarning\nwarnings.simplefilter('ignore', ConvergenceWarning)",
"_____no_output_____"
]
],
[
[
"###### MySQL Connection to fetch data",
"_____no_output_____"
]
],
[
[
"try:\n connection = mysql.connector.connect(host='localhost',\n database='car_decors',\n user='root',\n password='***********')\n\n sql_select_Query = \"SELECT * FROM decorsales\"\n cursor = connection.cursor()\n cursor.execute(sql_select_Query)\n columns = len(cursor.description)\n columns = [i[0] for i in cursor.description]\n print(columns)\n\n # get all records\n records = cursor.fetchall()\n print(\"Total number of rows in table: \", cursor.rowcount)\n\nexcept mysql.connector.Error as e:\n print(\"Error reading data from MySQL table\", e)",
"_____no_output_____"
]
],
[
[
"### Data Cleaning and Exploratory Data Analysis",
"_____no_output_____"
],
[
"###### Converting fetched records to Pandas dataframe",
"_____no_output_____"
]
],
[
[
"records = np.array(records)\nrecords = records[:,0:25]\ndecor_sales=pd.DataFrame(records,columns=columns)",
"_____no_output_____"
]
],
[
[
"###### Type Casting Date and other features",
"_____no_output_____"
]
],
[
[
"decor_sales.dtypes\ndecor_sales.Date = pd.to_datetime(decor_sales.Date)\ndecor_sales.iloc[:,1:] = decor_sales.iloc[:,1:].astype(\"int32\")\ndecor_sales.dtypes",
"_____no_output_____"
]
],
[
[
"###### Creating Subset of Decor Sales Dataset and resampling Monthly Time Series",
"_____no_output_____"
]
],
[
[
"df = decor_sales\ndf = df.set_index('Date')\ndf = df.resample(\"MS\").sum()",
"_____no_output_____"
]
],
[
[
"Note : Time period options when resampling a time series # MS - Monthly ; W - Weekly ; QS - Quarterly ; YS - Yearly",
"_____no_output_____"
]
],
[
[
"###### Data Visualization",
"_____no_output_____"
]
],
[
[
"plt.rc(\"figure\", figsize=(16,8))\nsns.set_style('darkgrid')",
"_____no_output_____"
]
],
[
[
"###### Rolling statistics to observe variation in mean and standard deviation.",
"_____no_output_____"
]
],
[
[
"timeseries = df ['Perfumes']\ntimeseries.rolling(12).mean().plot(label='12 Month Rolling Mean', marker='.')\ntimeseries.rolling(12).std().plot(label='12 Month Rolling Std', marker='.')\ntimeseries.plot(marker='.')\nplt.title('Rolling Statistics to observe variation in Mean and Standard Deviation', fontsize = 18, fontweight = 'bold')\nplt.xlabel('Year', fontsize = 14)\nplt.ylabel('Sales (Number of Units)', fontsize = 14)\nplt.legend()",
"_____no_output_____"
]
],
[
[
"# The plot shows, there is nearly a constant mean and standard deviation except noise in Qtr 2 - 2020 (Lockdown period) ",
"_____no_output_____"
]
],
[
[
"###### Checking Seasonalty and Trend components for the feature",
"_____no_output_____"
]
],
[
[
"from statsmodels.tsa.seasonal import seasonal_decompose\nadd = seasonal_decompose(df[\"Perfumes\"],model=\"additive\",period=12)\nadd.plot();",
"_____no_output_____"
]
],
[
[
"# Decomposition plot shows constant trend with noise in Qtr 2 - 2020 and seasonality is additive in nature.\n# The data is seasonal and follows constant trend.\n# Also, the average value or the mean of the residuals seem to be zero which holds our assumption.",
"_____no_output_____"
]
],
[
[
"##### Checking for Data Stationarity using Augmented Dickey-Fuller Test",
"_____no_output_____"
]
],
[
[
"from statsmodels.tsa.stattools import adfuller\n\ndef check_adf(time_series):\n test_result = adfuller(df['Perfumes'])\n print ('ADF Test:')\n labels = ['ADF Statistic','p-value','No. of Lags Used','Number of Observations Used']\n\n for value,label in zip(test_result,labels):\n print (label+': '+str(value)+str(\"\\n\"))\n if test_result [1] <= 0.05:\n print (\"Reject null hypothesis; Data is stationary\")\n else:\n print (\"Fail to reject H0; Data is non-stationary\")",
"_____no_output_____"
]
],
[
[
"If the data is non-stationary so we need to apply differencing to make our data stationary.\ndf ['Perfumes'] = df ['Perfumes'] - df ['Perfumes']. shift (1)\nadf_check(df['Perfumes'].dropna())\nIf again data is non-stationary we need to differencing with subsequent shifts.",
"_____no_output_____"
]
],
[
[
"check_adf(df['Perfumes'])",
"_____no_output_____"
]
],
[
[
"# Adfuller test Results for all variables",
"_____no_output_____"
]
],
[
[
"from statsmodels.tsa.stattools import adfuller\ndef adfuller_parameter(x):\n P = []\n columns = []\n used_lag = []\n for i in x.columns:\n test_stats,p,used_lags,nobs,critical_value,ic_best = adfuller(x[i])\n columns.append(i)\n P.append(p)\n used_lag.append(used_lags)\n return pd.DataFrame({\"COLUMNS\":columns,\"P_VALUE\":P,\"MAX_USED_LAG\":used_lag})\n\nadfuller_parameter(df)",
"_____no_output_____"
]
],
[
[
"By looking at adfuller test result we conclude that we need differencing by 0 shifts to make our data stationary for android headunits.",
"_____no_output_____"
]
],
[
[
"##### Hyper-parameter Tuning # Autocorrelation Function (ACF) and Partial Autocorrelation Function (PACF) plots",
"_____no_output_____"
]
],
[
[
"# By looking at ACF pot and PACF plot we decide the value p(Auto regressive) and q(Moving average)\n# p = sudden shuts off in pacf plot.\n# q = Exponential drop in acf plot.\n# d = degree of differencing/shift by adfuller test\n\n#Auto Regressive (p)\n# Identification of an AR model is often best done with the PACF.\n# For an AR model, the theoretical PACF “shuts off” past the order of the model. \n# The phrase “shuts off” means that in theory the partial autocorrelations are equal to 0 beyond that point. \n# Put another way, the number of non-zero partial autocorrelations gives the order of the AR model.\n# By the “order of the model” we mean the most extreme lag of x that is used as a predictor.\n\n# Integration (d)\n# Integration paramter is choosen through how much value you have differentiated from original\n# For a stationary data its either be 0 or 1\n\n# Moving Average (q) \n# the theoretical PACF does not shut off, but instead tapers or exponetially decrease toward 0 in some manner.\n# A clearer pattern for an MA model is in the ACF.\n# The ACF will have non-zero autocorrelations only at lags involved in the model.",
"_____no_output_____"
]
],
[
[
"from statsmodels.graphics.tsaplots import plot_acf, plot_pacf\nimport statsmodels.api as sm\n\nfig, ax = plt.subplots(1,2, figsize=(15,5))\nsm.graphics.tsa.plot_acf(df[\"Perfumes\"], lags=12, title = 'ACF Plot', ax=ax[0])\nsm.graphics.tsa.plot_pacf(df[\"Perfumes\"], lags=12, title = 'PACF Plot',ax=ax[1])\nplt.show()",
"_____no_output_____"
]
],
[
[
"### Model Building - SARIMA Model ( Seasonal ARIMA Model )",
"_____no_output_____"
],
[
"###### Train Test Split",
"_____no_output_____"
]
],
[
[
"train_df = df[\"Perfumes\"].iloc[0:int(len(df)*.95)] #train model with approx 95% data\ntest_df = df[\"Perfumes\"].iloc[int(len(train_df)):] #test model with 5% data\n\nprint(\"Train_df : \",len(train_df))\nprint(\"Test_df : \",len(test_df))",
"_____no_output_____"
]
],
[
[
"###### User Defined Function to calculate the MAPE value ",
"_____no_output_____"
]
],
[
[
"def mape(y_true, y_pred): \n y_true, y_pred = np.array(y_true), np.array(y_pred)\n return np.mean(np.abs((y_true - y_pred) / y_true)) * 100",
"_____no_output_____"
]
],
[
[
"###### Automated Hyperparameter tuning",
"_____no_output_____"
]
],
[
[
" import itertools as i \np = range(0,3) \nd = range(0,2)\nq = range(0,3)\n\npdq_combo = list(i.product(p,d,q)) #this will all combination of p,d,q throgh a tuple \n\nerror = []\naic_sarima = []\norder_arima = []\norder_sarima = []\nseasonality = 12\nfor pdq in pdq_combo:\n for PDQ in pdq_combo:\n try:\n SEASONAL_ORDER = list(PDQ)\n SEASONAL_ORDER.append(seasonality)\n model = sm.tsa.SARIMAX(train_df,order=(pdq),seasonal_order=tuple(SEASONAL_ORDER))\n result = model.fit(disp=0)\n pred = result.predict(start=len(train_df),end=len(df)-1)\n eror = mape(test_df,pred)\n aic_sarima.append(result.aic)\n order_arima.append(pdq)\n order_sarima.append(tuple(SEASONAL_ORDER))\n error.append(eror)\n except:\n continue",
"_____no_output_____"
],
[
"# Creating a dataframe of seasonality orders and errors \ndf_error = pd.DataFrame({\"arima_order\":order_arima,\"sarima_order\": order_sarima,\"error\":error,\"aic\":aic_sarima})\ndf_error = df_error.sort_values(by=\"error\",ascending = True)\ndf_error.reset_index(inplace=True,drop=True)",
"_____no_output_____"
],
[
"## best parameter selection\np_d_q = df_error.iloc[0,0] #choosing best parameter for arima order\nP_D_Q = df_error.iloc[0,1] #choosing best parameter for seasonal order",
"_____no_output_____"
],
[
"## best parameter selection\nprint(\"Best p_d_q parameter : \", p_d_q)\nprint(\"Best P_D_Q parameter : \", P_D_Q)",
"_____no_output_____"
]
],
[
[
"###### Model with best parameter",
"_____no_output_____"
]
],
[
[
"sarima_model = sm.tsa.SARIMAX(train_df, order=(p_d_q), seasonal_order=(P_D_Q))\nsarima_results = sarima_model.fit(disp = 0)\nsarima_pred = sarima_results.predict(start=test_df.index[0],end=test_df.index[-1])\nsarima_pred_large = sarima_results.predict(start=75,end=86,dynamic=True)",
"_____no_output_____"
],
[
"print(sarima_results.summary())\nsarima_diagnostics = sarima_results.plot_diagnostics(figsize=(16,8))",
"_____no_output_____"
]
],
[
[
"# Insights from these diagnostic plot :\n# 1.The top left plot shows the residuals over time.\n# The plot shows our residuals are fluctuating around mean 0 there is uniform deviation over time\n# except some noise in second quarter of 2021 due to lockdown imposed by government with effect of COVID-19 pandemic.\n\n# 2.In the top-right plot,\n# We see that the KDE follows closely with the N(0,1) line to indicate that the residuals are normally distributed. \n# This line is the standard notation for a normal distribution with a mean of 0 and a standard deviation of 1.\n# In our plot residuals are normally distributed.\n\n# 3.In the bottom left qq-plot,\n# We see the ordered distribution of residuals(blue dots) following the linear trend(red line)\n# of the samples taken from a standard normal distribution with N(0, 1).\n\n# 4.The autocorrelation visual (called a “correlogram”) on the bottom right shows that-\n# The time series residuals have a low correlation with the lagged versions of itself \n# (that is, the majority of dots fall into the blue shaded area).",
"_____no_output_____"
]
],
[
[
"# Predicted values\n# Point estimation\nsarima_prediction = sarima_results.get_prediction(start = test_df.index[0], end = test_df.index[-1], dynamic = True, full_results = True)\nsarima_point_estimation = sarima_prediction.predicted_mean\nsarima_point_estimation",
"_____no_output_____"
],
[
"#Checking MAPE\nmape(test_df, sarima_point_estimation)",
"_____no_output_____"
],
[
"# At 95% confidence interval\nsarima_pred_range = sarima_prediction.conf_int(alpha = 0.05)\nsarima_pred_range",
"_____no_output_____"
],
[
"# Ploting Sarima Prediction\nplt.plot(train_df,color=\"g\",label=\"Train Data\", marker='.')\nplt.plot(test_df,color=\"b\",label=\"Test Data\", marker='.')\nplt.plot(sarima_point_estimation,color=\"r\",label=\"Forecast (Test Data)\", marker='.')\nplt.figtext(0.13, 0.15, '\\nMAPE : {} \\nSARIMA : {},{} \\nAIC : {}'.format(mape(test_df, sarima_point_estimation), p_d_q, P_D_Q, sarima_results.aic, fontsize = 11))\nplt.fill_between(sarima_pred_range.index,sarima_pred_range.iloc[:,0],sarima_pred_range.iloc[:,1],color='b',alpha=.2)\nplt.legend(loc=\"upper right\")",
"_____no_output_____"
]
],
[
[
"############################################################################################################################ ",
"_____no_output_____"
]
],
[
[
"### Holt Winters Exponential Smoothing with Additive Seasonality and Additive Trend",
"_____no_output_____"
]
],
[
[
"from statsmodels.tsa.seasonal import seasonal_decompose\nfrom statsmodels.tsa.holtwinters import ExponentialSmoothing # \n\nhwe_model_add_add = ExponentialSmoothing(train_df, seasonal =\"add\", trend = \"add\", seasonal_periods = 12).fit()\npred_hwe_add_add = hwe_model_add_add.predict(start = test_df.index[0], end = test_df.index[-1])",
"_____no_output_____"
],
[
"pred_hwe_add_add",
"_____no_output_____"
]
],
[
[
"###### Plotting Holt Winters Model ",
"_____no_output_____"
]
],
[
[
"plt.plot(train_df,color=\"g\",label=\"Train Data\")\nplt.plot(test_df,color=\"b\",label=\"Test Data\")\nplt.plot(pred_hwe_add_add,color=\"r\",label=\"Forecast (Test Data)\")\nplt.suptitle('Model : Holt Winters', fontsize = 12, fontweight = 'bold')\nplt.title('Car Decors - ANDROID HEAD UNITS', fontsize = 18, fontweight = 'bold')\nplt.figtext(0.13, 0.14, '\\nMAPE : {} \\nAIC : {}'.format(mape(test_df, pred_hwe_add_add), hwe_model_add_add.aic))\nplt.xlabel('Year', fontsize = 14)\nplt.ylabel('Sales (Number of Units)', fontsize = 14)\nplt.legend(loc=\"best\")",
"_____no_output_____"
],
[
"mape(test_df, pred_hwe_add_add) ",
"_____no_output_____"
]
],
[
[
"############################################################################################################################ ",
"_____no_output_____"
]
],
[
[
"### FB Prophet Model",
"_____no_output_____"
]
],
[
[
"# Loading Libraries\nfrom fbprophet import Prophet\nfrom fbprophet.plot import plot_plotly\n\ndf1 = decor_sales\ndf1 = df1.set_index('Date')\ndf1 = df1.resample(\"MS\").sum()\ndf1.reset_index(inplace=True)",
"_____no_output_____"
],
[
"train_df1 = df1[[\"Date\",\"Perfumes\"]].iloc[0:int(len(df1)*.95)] #train model with approx 95% data\ntest_df1 = df1[[\"Date\",\"Perfumes\"]].iloc[int(len(train_df1)):] #test model with 5% data\n\nprint(\"Train : \",len(train_df1))\nprint(\"Test : \",len(test_df1))",
"_____no_output_____"
],
[
"train_df1.columns = [\"ds\",\"y\"]\ntest_df1.columns = [\"ds\",\"y\"]",
"_____no_output_____"
],
[
"# Fitting the Model\nprophet_model = Prophet().fit(train_df1)",
"_____no_output_____"
],
[
"# Define the period for which we want a prediction\nfuture = list()\nfor i in range(1, 5):\n\tdate = '2021-%02d' % i\n\tfuture.append([date])\nfuture = pd.DataFrame(future)\nfuture.columns = ['ds']\nfuture['ds']= pd.to_datetime(future['ds'])\nfuture ",
"_____no_output_____"
],
[
"forecast = prophet_model.predict(future)\nprint(forecast[['ds', 'yhat', 'yhat_lower', 'yhat_upper']])",
"_____no_output_____"
],
[
"test_df1=test_df1.set_index(\"ds\")\ntrain_df1 = train_df1.set_index(\"ds\")\nforecast=forecast.set_index(\"ds\")",
"_____no_output_____"
],
[
"plt.style.use(\"ggplot\")\nplt.plot(train_df1['y'],color=\"r\",label=\"Train Data\")\nplt.plot(test_df1['y'],color=\"b\",label=\"Test Data\")\nplt.plot(forecast[\"yhat\"],color=\"g\",label=\"Forecast (Test Data)\")\nplt.grid( linestyle='-', linewidth=2)\nplt.legend(loc=\"best\")",
"_____no_output_____"
],
[
"# MAPE\nmape(test_df1['y'], forecast['yhat'])",
"_____no_output_____"
],
[
"#RMSE\nsqrt(mean_squared_error(test_df1['y'], forecast['yhat'].tail(4)))",
"_____no_output_____"
]
],
[
[
"############################################################################################################################ ",
"_____no_output_____"
]
],
[
[
"### Auto Time Series Model",
"_____no_output_____"
]
],
[
[
"from auto_ts import auto_timeseries",
"_____no_output_____"
],
[
"train_df2 = train_df1\ntest_df2 = test_df1",
"_____no_output_____"
],
[
"ts_model = auto_timeseries( score_type='rmse', time_interval='MS', non_seasonal_pdq=(12,12,12), seasonality=True, seasonal_period=12, model_type=\"best\", verbose=2)",
"_____no_output_____"
],
[
"ts_model.fit(traindata= train_df2, ts_column=\"ds\", target=\"y\")",
"_____no_output_____"
],
[
"ts_model.get_leaderboard()",
"_____no_output_____"
],
[
"ts_model.plot_cv_scores()",
"_____no_output_____"
],
[
"future_predictions = ts_model.predict(test_df2, model='best')\nfuture_predictions",
"_____no_output_____"
],
[
"# define the period for which we want a prediction\nts_future = list()\nfor i in range(1, 5):\n\tdate = '2021-%02d' % i\n\tts_future.append([date])\nts_future = pd.DataFrame(ts_future)\nts_future.columns = ['ds']\nts_future['ds']= pd.to_datetime(ts_future['ds'])",
"_____no_output_____"
],
[
"ts_model.predict(ts_future)",
"_____no_output_____"
],
[
"mape(test_df2[\"y\"],future_predictions[\"yhat\"])",
"_____no_output_____"
]
],
[
[
"############################################################################################################################ ",
"_____no_output_____"
]
],
[
[
"### Models Evaluation",
"_____no_output_____"
]
],
[
[
"from sklearn.metrics import mean_squared_error as mse\nprint(\"\\nSARIMA Trend : \", p_d_q)\nprint(\"SARIMA Seasonal Order : \", P_D_Q)\nprint(\"SARIMA AIC : \", sarima_results.aic)\nprint(\"SARIMA RMSE : \", np.sqrt(mse(test_df,sarima_point_estimation)))\nprint(\"SARIMA MAPE : \", mape(test_df, sarima_point_estimation))\nprint(\"\\nHolt Winters AIC : \", hwe_model_add_add.aic)\nprint(\"Holt Winters RMSE : \", np.sqrt(mse(test_df,pred_hwe_add_add)))\nprint(\"Holt Winters MAPE : \", mape(test_df, pred_hwe_add_add))\nprint(\"\\nFB Prophet RMSE : \", sqrt(mean_squared_error(test_df1['y'], forecast['yhat'])))\nprint(\"FB Prophet MAPE : \", mape(test_df1['y'], forecast['yhat']))\nprint(\"\\nAuto Time Series: \\n \", ts_model.get_leaderboard())\nprint(\"Auto Time Series MAPE : \", mape(test_df2[\"y\"],future_predictions[\"yhat\"]))",
"_____no_output_____"
],
[
"sarima = mape(test_df, sarima_point_estimation)\nhwinters = mape(test_df, pred_hwe_add_add)\nfbprophet = mape(test_df1['y'], forecast['yhat'])\nautots = mape(test_df2[\"y\"],future_predictions[\"yhat\"])\n\nmape_data = {'models':['SARIMA','HOLTWINTERS','FB_PROPHET','AUTO_TS'], 'name':['sarima_model', 'hwe_model_add_add','prophet_model','ts_model'],'mape':[sarima, hwinters, fbprophet, autots]}\nmape_error = pd.DataFrame(mape_data)\nmape_error = mape_error.sort_values(by=\"mape\",ascending = True)\nmape_error.reset_index(inplace=True,drop=True)\n#best_model = mape_error.iloc[0,0]\nprint('\\033[1m'+\"Best Model with lowest MAPE : \", mape_error.iloc[0,0] + \" ( \" + mape_error.iloc[0,1] + \" ) \" + '\\033[0m')\nprint(\"\\nMAPE ERRORS :\\n\\n\", mape_error)",
"_____no_output_____"
]
],
[
[
"############################################################################################################################ ",
"_____no_output_____"
]
],
[
[
"##### Saving Model",
"_____no_output_____"
]
],
[
[
"import pickle\nfilename = 'sarima_model_perfumes.pkl'\npickle.dump(sarima_model, open(filename, 'wb'))",
"_____no_output_____"
]
],
[
[
"###### Testing saved Model for prediction",
"_____no_output_____"
]
],
[
[
"####### Model summary and diagnstics plot #######\nwith open(filename, \"rb\") as file:\n load_model = pickle.load(file)\n \nresult = load_model.fit()\n#print(result.summary())\n#diagnostics = result.plot_diagnostics(figsize=(16,8))",
"_____no_output_____"
],
[
"pred = result.get_prediction(start = 76, end = 87, dynamic = False)\n\n# Point estimation\nprediction = pred.predicted_mean\nprediction = round(prediction)\nprediction",
"_____no_output_____"
],
[
"# Ploting final Sarima Prediction\nplt.plot(df['Perfumes'],color=\"g\",label=\"Actual\", marker='.')\nplt.plot(prediction,color=\"r\",label=\"Forecast\", marker='.')\nplt.suptitle('Model : SARIMA', fontsize = 12, fontweight = 'bold')\nplt.title('Car Decors - Perfumes', fontsize = 18, fontweight = 'bold')\nplt.figtext(0.13, 0.14, '\\nMAPE : {} \\nAIC : {}'.format(mape(test_df, sarima_point_estimation), sarima_results.aic))\nplt.xlabel('Year', fontsize = 14)\nplt.ylabel('Sales (Number of Units)', fontsize = 14)\nplt.legend(loc=\"best\")",
"_____no_output_____"
]
],
[
[
"### Closing connection to MySQL and clearing variables from memory.",
"_____no_output_____"
]
],
[
[
"#if connection.is_connected():\n# connection.close()\n# cursor.close()\n# print(\"MySQL connection is closed\")\n\n# Clear all variables from memory\n#globals().clear()\n\n#####################################################################",
"_____no_output_____"
]
]
] | [
"markdown",
"raw",
"markdown",
"code",
"markdown",
"code",
"markdown",
"code",
"markdown",
"code",
"markdown",
"code",
"raw",
"markdown",
"code",
"markdown",
"code",
"raw",
"markdown",
"code",
"raw",
"markdown",
"code",
"raw",
"code",
"markdown",
"code",
"raw",
"markdown",
"raw",
"code",
"markdown",
"code",
"markdown",
"code",
"markdown",
"code",
"markdown",
"code",
"raw",
"code",
"raw",
"markdown",
"code",
"markdown",
"code",
"raw",
"markdown",
"code",
"raw",
"markdown",
"code",
"raw",
"markdown",
"code",
"raw",
"markdown",
"code",
"markdown",
"code",
"markdown",
"code"
] | [
[
"markdown"
],
[
"raw"
],
[
"markdown"
],
[
"code"
],
[
"markdown"
],
[
"code"
],
[
"markdown",
"markdown"
],
[
"code"
],
[
"markdown"
],
[
"code"
],
[
"markdown"
],
[
"code"
],
[
"raw"
],
[
"markdown"
],
[
"code"
],
[
"markdown"
],
[
"code"
],
[
"raw"
],
[
"markdown"
],
[
"code"
],
[
"raw"
],
[
"markdown"
],
[
"code"
],
[
"raw"
],
[
"code"
],
[
"markdown"
],
[
"code"
],
[
"raw"
],
[
"markdown"
],
[
"raw"
],
[
"code"
],
[
"markdown",
"markdown"
],
[
"code"
],
[
"markdown"
],
[
"code"
],
[
"markdown"
],
[
"code",
"code",
"code",
"code"
],
[
"markdown"
],
[
"code",
"code"
],
[
"raw"
],
[
"code",
"code",
"code",
"code"
],
[
"raw"
],
[
"markdown"
],
[
"code",
"code"
],
[
"markdown"
],
[
"code",
"code"
],
[
"raw"
],
[
"markdown"
],
[
"code",
"code",
"code",
"code",
"code",
"code",
"code",
"code",
"code",
"code"
],
[
"raw"
],
[
"markdown"
],
[
"code",
"code",
"code",
"code",
"code",
"code",
"code",
"code",
"code",
"code"
],
[
"raw"
],
[
"markdown"
],
[
"code",
"code"
],
[
"raw"
],
[
"markdown"
],
[
"code"
],
[
"markdown"
],
[
"code",
"code",
"code"
],
[
"markdown"
],
[
"code"
]
] |
d0914cf21a0d5bba0edf7c2d017a0021b0f5c3c4 | 4,273 | ipynb | Jupyter Notebook | examples/reveal/week_926.ipynb | jonl1096/seelvizorg | ae4e3567ce89eb62edcd742060619fdf1883b991 | [
"Apache-2.0"
] | null | null | null | examples/reveal/week_926.ipynb | jonl1096/seelvizorg | ae4e3567ce89eb62edcd742060619fdf1883b991 | [
"Apache-2.0"
] | 2 | 2017-04-18T02:50:14.000Z | 2017-04-18T18:04:20.000Z | examples/reveal/week_926.ipynb | jonl1096/seelvizorg | ae4e3567ce89eb62edcd742060619fdf1883b991 | [
"Apache-2.0"
] | null | null | null | 44.510417 | 288 | 0.65949 | [
[
[
"import os\nfrom IPython.core.display import Image, display",
"_____no_output_____"
]
],
[
[
"## Deliverables\n\n### Tony\n* Clustering -- learn about clustering. Make a LaTeX (or Markdown) file explaining what K-means, K-medeods, Spectral, Louvain do. Explain basics of implementation, and include a pro/con table discussing what each does well/poorly with examples for each. Include basic algorithms.\n* Write pseudocode for clustering graphs [see Ryan's Git Issue on LaTeX]\n * https://neurodatadesign.github.io/seelviz/Jupyter/ClusteringTechniques.html\n\nReach Goal:\n* Jupyter notebook with clustering on our data [here](https://github.com/NeuroDataDesign/seelviz/blob/gh-pages/Jupyter/Example%20Clustering%20on%20Fear199.ipynb).\n * As per Jovo's recommendation, I first ran example data to make sure I understood the basic premises of what I was doing (used sample data set Images from SciKit, for handwriting)\n * Then attempted to do clustering on Fear199 to implement K-means\n \n * 3D representation makes it hard to show the clusters (orange) even when I biggify the points. Since there are an overwhelming number of regular points, I plotted along just XY to confirm my results.\n \n \n\n### Jon\n* Turn API into pipeline form: Running should output wanted graphs\n * https://github.com/NeuroDataDesign/seelviz/blob/gh-pages/Jupyter/ClarityViz%20Pipeline.ipynb\n\n### Albert\n* Kwame pipeline tutorials - deliverable is Jupyter Notebook running his tutorial\n * https://github.com/NeuroDataDesign/seelviz/blob/gh-pages/Kwame%20Registration%20Notebook%201.ipynb \n * https://github.com/NeuroDataDesign/seelviz/blob/gh-pages/Kwame%20Registration%20Notebook%202.ipynb\n* Get the atlas aligned data from Kwame\n * https://github.com/NeuroDataDesign/seelviz/blob/gh-pages/croppedbrain.jpg\n* Acquire new data and see if we still have problems with it.\n * https://github.com/NeuroDataDesign/seelviz/blob/gh-pages/newBrain.png\n* Using the atlas aligned images, play around with atlas coordinates\n * PROBLEMS: We still have problems, largely we need the new data to be propagated in order for us to play around with it. We have Kwame's images however one of the images seem to be \"damaged\"\n * https://github.com/NeuroDataDesign/seelviz/blob/gh-pages/novisionbrain.png\n * https://github.com/NeuroDataDesign/seelviz/blob/gh-pages/damagedbrain.png\n\nReach Goal:\n* Run Kwame Pipeline on new data\n * Incomplete, as the other file was not propagated thus I was unable to run it through Kwame's pipeline\n * https://github.com/NeuroDataDesign/seelviz/blob/gh-pages/AileyRegistration.ipynb\n\n### Luke\n* Go back and make weighted versions of our graphs (decide on a weight function [e^(-distance), for instance])\n* Make graphs similar to Greg's\n * https://neurodatadesign.github.io/seelviz/Jupyter/CombinedPlotsandGraphStatistics.html",
"_____no_output_____"
]
]
] | [
"code",
"markdown"
] | [
[
"code"
],
[
"markdown"
]
] |
d09168c1055ad353737dc4c84e51d7b0a5c6ed33 | 39,828 | ipynb | Jupyter Notebook | Introduction to Graph Theory/Week2/Notebook: Topological Sorting/Topological Sort.ipynb | ChanchalKumarMaji/Introduction-to-Discrete-Mathematics-for-Computer-Science-Specialization | 79927d008f70d36345755759aad54900e6cba464 | [
"MIT"
] | 83 | 2020-01-28T17:47:55.000Z | 2022-03-29T16:08:37.000Z | Introduction to Graph Theory/Week2/Notebook: Topological Sorting/Topological Sort.ipynb | MehaRima/Introduction-to-Discrete-Mathematics-for-Computer-Science-Specialization | ab70116c11757c5c79e4a278110d42d0c8f52dfd | [
"MIT"
] | 6 | 2020-06-02T13:48:44.000Z | 2021-09-05T19:39:12.000Z | Introduction to Graph Theory/Week2/Notebook: Topological Sorting/Topological Sort.ipynb | MehaRima/Introduction-to-Discrete-Mathematics-for-Computer-Science-Specialization | ab70116c11757c5c79e4a278110d42d0c8f52dfd | [
"MIT"
] | 42 | 2020-01-20T03:14:55.000Z | 2022-03-19T08:15:54.000Z | 414.875 | 20,250 | 0.935447 | [
[
[
"import networkx as nx\nimport pygraphviz as pgv\nfrom nxpd import draw, nxpdParams\nnxpdParams['show'] = 'ipynb'\n\n\nG = nx.DiGraph()\nG.add_edges_from([('a', 'b'), ('b', 'c'), ('b', 'd'), ('d', 'c'), ('a', 'd')])\ndraw(G, layout='circo')",
"_____no_output_____"
],
[
"if nx.is_directed_acyclic_graph(G):\n print(\"Topological ordering of the nodes:\", nx.topological_sort(G))\nelse:\n print(\"G contains a cycle, hence it cannot be topologically sorted.\")",
"Topological ordering of the nodes: ['a', 'b', 'd', 'c']\n"
],
[
"draw(G, layout='dot')",
"_____no_output_____"
]
]
] | [
"code"
] | [
[
"code",
"code",
"code"
]
] |
d0916937cfaccd43a899f8398e49a288cd397c59 | 546,807 | ipynb | Jupyter Notebook | appliedDataScienceCapstoneWeek3.ipynb | Margchunne28/Location-data-provider | c77bcee1ca03133d8324aa59aa04eff777c9d4d2 | [
"Apache-2.0"
] | null | null | null | appliedDataScienceCapstoneWeek3.ipynb | Margchunne28/Location-data-provider | c77bcee1ca03133d8324aa59aa04eff777c9d4d2 | [
"Apache-2.0"
] | null | null | null | appliedDataScienceCapstoneWeek3.ipynb | Margchunne28/Location-data-provider | c77bcee1ca03133d8324aa59aa04eff777c9d4d2 | [
"Apache-2.0"
] | null | null | null | 96.540784 | 179,943 | 0.656105 | [
[
[
"\n<h1 align=\"center\"> Battle of the Neighbourhoods - Toronto </h1>",
"_____no_output_____"
],
[
"Author: Ganesh Chunne\n\nThis notebook contains Questions 1, 2 & 3 of the Assignment. They have been segregated by Section headers\n",
"_____no_output_____"
]
],
[
[
"import pandas as pd",
"_____no_output_____"
]
],
[
[
"# Question 1",
"_____no_output_____"
],
[
"## Importing Data",
"_____no_output_____"
]
],
[
[
"import requests",
"_____no_output_____"
],
[
"url = \"https://en.wikipedia.org/wiki/List_of_postal_codes_of_Canada:_M\"\nwiki_url = requests.get(url)\nwiki_url",
"_____no_output_____"
]
],
[
[
"Response 200 means that we are able to make the connection to the page",
"_____no_output_____"
]
],
[
[
"wiki_data = pd.read_html(wiki_url.text)\nwiki_data",
"_____no_output_____"
],
[
"len(wiki_data), type(wiki_data)",
"_____no_output_____"
]
],
[
[
"We need the first table alone, so dropping the other tables",
"_____no_output_____"
]
],
[
[
"wiki_data = wiki_data[0]\nwiki_data",
"_____no_output_____"
]
],
[
[
"Dropping Borough which are not assigned",
"_____no_output_____"
]
],
[
[
"df = wiki_data[wiki_data[\"Borough\"] != \"Not assigned\"]\ndf",
"_____no_output_____"
]
],
[
[
"Grouping the records based on Postal Code",
"_____no_output_____"
]
],
[
[
"df = df.groupby(['Postal Code']).head()\ndf",
"_____no_output_____"
]
],
[
[
"Checking for number of records where Neighbourhood is \"Not assigned\"",
"_____no_output_____"
]
],
[
[
"df.Neighbourhood.str.count(\"Not assigned\").sum()",
"_____no_output_____"
],
[
"df = df.reset_index()\ndf",
"_____no_output_____"
],
[
"df.drop(['index'], axis = 'columns', inplace = True)\ndf",
"_____no_output_____"
],
[
"df.shape",
"_____no_output_____"
]
],
[
[
"Answer to Question 1: We have 103 rows and 3 columns",
"_____no_output_____"
],
[
"# Question 2",
"_____no_output_____"
],
[
"Installing geocoder",
"_____no_output_____"
]
],
[
[
"pip install geocoder",
"Requirement already satisfied: geocoder in c:\\users\\ganes\\appdata\\local\\programs\\python\\python39\\lib\\site-packages (1.38.1)\nRequirement already satisfied: requests in c:\\users\\ganes\\appdata\\local\\programs\\python\\python39\\lib\\site-packages (from geocoder) (2.24.0)\nNote: you may need to restart the kernel to use updated packages.\nRequirement already satisfied: six in c:\\users\\ganes\\appdata\\local\\programs\\python\\python39\\lib\\site-packages (from geocoder) (1.15.0)\nRequirement already satisfied: click in c:\\users\\ganes\\appdata\\local\\programs\\python\\python39\\lib\\site-packages (from geocoder) (7.1.2)\nRequirement already satisfied: future in c:\\users\\ganes\\appdata\\local\\programs\\python\\python39\\lib\\site-packages (from geocoder) (0.18.2)\nRequirement already satisfied: ratelim in c:\\users\\ganes\\appdata\\local\\programs\\python\\python39\\lib\\site-packages (from geocoder) (0.1.6)\nRequirement already satisfied: decorator in c:\\users\\ganes\\appdata\\local\\programs\\python\\python39\\lib\\site-packages (from ratelim->geocoder) (4.4.2)\nRequirement already satisfied: chardet<4,>=3.0.2 in c:\\users\\ganes\\appdata\\local\\programs\\python\\python39\\lib\\site-packages (from requests->geocoder) (3.0.4)\nRequirement already satisfied: certifi>=2017.4.17 in c:\\users\\ganes\\appdata\\local\\programs\\python\\python39\\lib\\site-packages (from requests->geocoder) (2020.6.20)\nRequirement already satisfied: urllib3!=1.25.0,!=1.25.1,<1.26,>=1.21.1 in c:\\users\\ganes\\appdata\\local\\programs\\python\\python39\\lib\\site-packages (from requests->geocoder) (1.25.11)\nRequirement already satisfied: idna<3,>=2.5 in c:\\users\\ganes\\appdata\\local\\programs\\python\\python39\\lib\\site-packages (from requests->geocoder) (2.10)\n"
],
[
"import geocoder # import geocoder",
"_____no_output_____"
]
],
[
[
"Tried the below approach, ran for 20 mins, then killed it. Changing the code cell to Text for now so that the run all execution doesn't stop.",
"_____no_output_____"
],
[
"```python\n# initialize your variable to None\nlat_lng_coords = None\n\npostal_code = 'M3A'\n\n# loop until you get the coordinates\nwhile(lat_lng_coords is None):\n g = geocoder.google('{}, Toronto, Ontario'.format(postal_code))\n lat_lng_coords = g.latlng\n\nlatitude = lat_lng_coords[0]\nlongitude = lat_lng_coords[1]\n```",
"_____no_output_____"
],
[
"Alternatively, as suggested in the assignment, Importing the CSV file from the URL",
"_____no_output_____"
]
],
[
[
"data = pd.read_csv(\"https://cocl.us/Geospatial_data\")\ndata",
"_____no_output_____"
],
[
"print(\"The shape of our wiki data is: \", df.shape)\nprint(\"the shape of our csv data is: \", data.shape)",
"The shape of our wiki data is: (103, 3)\nthe shape of our csv data is: (103, 3)\n"
]
],
[
[
"Since the dimensions are the same, we can try to join on the postal codes to get the required data.\n\nChecking the column types of both the dataframes, especially Postal Code column since we are trying to join on it",
"_____no_output_____"
]
],
[
[
"df.dtypes",
"_____no_output_____"
],
[
"data.dtypes",
"_____no_output_____"
],
[
"combined_data = df.join(data.set_index('Postal Code'), on='Postal Code', how='inner')\ncombined_data",
"_____no_output_____"
],
[
"combined_data.shape",
"_____no_output_____"
]
],
[
[
"**Solution:** We get 103 rows as expected when we do a inner join, so we have good data.",
"_____no_output_____"
],
[
"# Question 3",
"_____no_output_____"
],
[
"Drawing inspiration from the previous lab where we cluster the neighbourhood of NYC, We cluster Toronto based on the similarities of the venues categories using Kmeans clustering and Foursquare API.",
"_____no_output_____"
]
],
[
[
"from geopy.geocoders import Nominatim ",
"_____no_output_____"
],
[
"address = 'Toronto, Ontario'\n\ngeolocator = Nominatim(user_agent=\"toronto_explorer\")\nlocation = geolocator.geocode(address)\nlatitude = location.latitude\nlongitude = location.longitude\nprint('The coordinates of Toronto are {}, {}.'.format(latitude, longitude))",
"The coordinates of Toronto are 43.6534817, -79.3839347.\n"
]
],
[
[
"Let's visualize the map of Toronto",
"_____no_output_____"
]
],
[
[
"import folium",
"_____no_output_____"
],
[
"# Creating the map of Toronto\nmap_Toronto = folium.Map(location=[latitude, longitude], zoom_start=11)\n\n# adding markers to map\nfor latitude, longitude, borough, neighbourhood in zip(combined_data['Latitude'], combined_data['Longitude'], combined_data['Borough'], combined_data['Neighbourhood']):\n label = '{}, {}'.format(neighbourhood, borough)\n label = folium.Popup(label, parse_html=True)\n folium.CircleMarker(\n [latitude, longitude],\n radius=5,\n popup=label,\n color='red',\n fill=True\n ).add_to(map_Toronto) \n \nmap_Toronto",
"_____no_output_____"
]
],
[
[
"Initializing Foursquare API credentials",
"_____no_output_____"
]
],
[
[
"CLIENT_ID = '2GQBW5PR0QFXTOGCHKTRFWJBTGOFOHXW1TRTNRAFURQ5FE1X'\nCLIENT_SECRET = '3QH40WMZIIDSQN1RFAVAEQHUIMOQUJPKYPABQVNTSDQJN2YD'\nVERSION = 20202808\nradius = 500\nLIMIT = 100\n\nprint('Your credentails:')\nprint('CLIENT_ID: ' + CLIENT_ID)\nprint('CLIENT_SECRET:' + CLIENT_SECRET)",
"Your credentails:\nCLIENT_ID: 2GQBW5PR0QFXTOGCHKTRFWJBTGOFOHXW1TRTNRAFURQ5FE1X\nCLIENT_SECRET:3QH40WMZIIDSQN1RFAVAEQHUIMOQUJPKYPABQVNTSDQJN2YD\n"
]
],
[
[
"Next, we create a function to get all the venue categories in Toronto",
"_____no_output_____"
]
],
[
[
"def getNearbyVenues(names, latitudes, longitudes):\n \n venues_list=[]\n for name, lat, lng in zip(names, latitudes, longitudes):\n print(name)\n \n # create the API request URL\n url = 'https://api.foursquare.com/v2/venues/explore?&client_id={}&client_secret={}&v={}&ll={},{}&radius={}'.format(\n CLIENT_ID, \n CLIENT_SECRET, \n VERSION, \n lat, \n lng, \n radius\n )\n \n # make the GET request\n results = requests.get(url).json()[\"response\"]['groups'][0]['items']\n \n # return only relevant information for each nearby venue\n venues_list.append([(\n name, \n lat, \n lng, \n v['venue']['name'], \n v['venue']['categories'][0]['name']) for v in results])\n\n nearby_venues = pd.DataFrame([item for venue_list in venues_list for item in venue_list])\n nearby_venues.columns = ['Neighbourhood', \n 'Neighbourhood Latitude', \n 'Neighbourhood Longitude', \n 'Venue', \n 'Venue Category']\n \n return(nearby_venues)",
"_____no_output_____"
]
],
[
[
"Collecting the venues in Toronto for each Neighbourhood",
"_____no_output_____"
]
],
[
[
"venues_in_toronto = getNearbyVenues(combined_data['Neighbourhood'], combined_data['Latitude'], combined_data['Longitude'])",
"Parkwoods\nVictoria Village\nRegent Park, Harbourfront\nLawrence Manor, Lawrence Heights\nQueen's Park, Ontario Provincial Government\nIslington Avenue, Humber Valley Village\nMalvern, Rouge\nDon Mills\nParkview Hill, Woodbine Gardens\nGarden District, Ryerson\nGlencairn\nWest Deane Park, Princess Gardens, Martin Grove, Islington, Cloverdale\nRouge Hill, Port Union, Highland Creek\nDon Mills\nWoodbine Heights\nSt. James Town\nHumewood-Cedarvale\nEringate, Bloordale Gardens, Old Burnhamthorpe, Markland Wood\nGuildwood, Morningside, West Hill\nThe Beaches\nBerczy Park\nCaledonia-Fairbanks\nWoburn\nLeaside\nCentral Bay Street\nChristie\nCedarbrae\nHillcrest Village\nBathurst Manor, Wilson Heights, Downsview North\nThorncliffe Park\nRichmond, Adelaide, King\nDufferin, Dovercourt Village\nScarborough Village\nFairview, Henry Farm, Oriole\nNorthwood Park, York University\nEast Toronto, Broadview North (Old East York)\nHarbourfront East, Union Station, Toronto Islands\nLittle Portugal, Trinity\nKennedy Park, Ionview, East Birchmount Park\nBayview Village\nDownsview\nThe Danforth West, Riverdale\nToronto Dominion Centre, Design Exchange\nBrockton, Parkdale Village, Exhibition Place\nGolden Mile, Clairlea, Oakridge\nYork Mills, Silver Hills\nDownsview\nIndia Bazaar, The Beaches West\nCommerce Court, Victoria Hotel\nNorth Park, Maple Leaf Park, Upwood Park\nHumber Summit\nCliffside, Cliffcrest, Scarborough Village West\nWillowdale, Newtonbrook\nDownsview\nStudio District\nBedford Park, Lawrence Manor East\nDel Ray, Mount Dennis, Keelsdale and Silverthorn\nHumberlea, Emery\nBirch Cliff, Cliffside West\nWillowdale, Willowdale East\nDownsview\nLawrence Park\nRoselawn\nRunnymede, The Junction North\nWeston\nDorset Park, Wexford Heights, Scarborough Town Centre\nYork Mills West\nDavisville North\nForest Hill North & West, Forest Hill Road Park\nHigh Park, The Junction South\nWestmount\nWexford, Maryvale\nWillowdale, Willowdale West\nNorth Toronto West, Lawrence Park\nThe Annex, North Midtown, Yorkville\nParkdale, Roncesvalles\nCanada Post Gateway Processing Centre\nKingsview Village, St. Phillips, Martin Grove Gardens, Richview Gardens\nAgincourt\nDavisville\nUniversity of Toronto, Harbord\nRunnymede, Swansea\nClarks Corners, Tam O'Shanter, Sullivan\nMoore Park, Summerhill East\nKensington Market, Chinatown, Grange Park\nMilliken, Agincourt North, Steeles East, L'Amoreaux East\nSummerhill West, Rathnelly, South Hill, Forest Hill SE, Deer Park\nCN Tower, King and Spadina, Railway Lands, Harbourfront West, Bathurst Quay, South Niagara, Island airport\nNew Toronto, Mimico South, Humber Bay Shores\nSouth Steeles, Silverstone, Humbergate, Jamestown, Mount Olive, Beaumond Heights, Thistletown, Albion Gardens\nSteeles West, L'Amoreaux West\nRosedale\nStn A PO Boxes\nAlderwood, Long Branch\nNorthwest, West Humber - Clairville\nUpper Rouge\nSt. James Town, Cabbagetown\nFirst Canadian Place, Underground city\nThe Kingsway, Montgomery Road, Old Mill North\nChurch and Wellesley\nBusiness reply mail Processing Centre, South Central Letter Processing Plant Toronto\nOld Mill South, King's Mill Park, Sunnylea, Humber Bay, Mimico NE, The Queensway East, Royal York South East, Kingsway Park South East\nMimico NW, The Queensway West, South of Bloor, Kingsway Park South West, Royal York South West\n"
],
[
"venues_in_toronto.shape",
"_____no_output_____"
]
],
[
[
"So we have 1317 records and 5 columns. Checking sample data\n",
"_____no_output_____"
]
],
[
[
"venues_in_toronto.head()",
"_____no_output_____"
]
],
[
[
"Checking the Venues based on Neighbourhood",
"_____no_output_____"
]
],
[
[
"venues_in_toronto.groupby('Neighbourhood').head()",
"_____no_output_____"
]
],
[
[
"So there are 405 records for each neighbourhood.\n\nChecking for the maximum venue categories",
"_____no_output_____"
]
],
[
[
"venues_in_toronto.groupby('Venue Category').max()",
"_____no_output_____"
]
],
[
[
"There are around 232 different types of Venue Categories. Interesting!",
"_____no_output_____"
],
[
"## One Hot encoding the venue Categories",
"_____no_output_____"
]
],
[
[
"toronto_venue_cat = pd.get_dummies(venues_in_toronto[['Venue Category']], prefix=\"\", prefix_sep=\"\")\ntoronto_venue_cat",
"_____no_output_____"
]
],
[
[
"Adding the neighbourhood to the encoded dataframe",
"_____no_output_____"
]
],
[
[
"toronto_venue_cat['Neighbourhood'] = venues_in_toronto['Neighbourhood'] \n\n# moving neighborhood column to the first column\nfixed_columns = [toronto_venue_cat.columns[-1]] + list(toronto_venue_cat.columns[:-1])\ntoronto_venue_cat = toronto_venue_cat[fixed_columns]\n\ntoronto_venue_cat.head()",
"_____no_output_____"
]
],
[
[
"We will group the Neighbourhoods, calculate the mean venue categories in each Neighbourhood ",
"_____no_output_____"
]
],
[
[
"toronto_grouped = toronto_venue_cat.groupby('Neighbourhood').mean().reset_index()\ntoronto_grouped.head()",
"_____no_output_____"
]
],
[
[
"Let's make a function to get the top most common venue categories",
"_____no_output_____"
]
],
[
[
"def return_most_common_venues(row, num_top_venues):\n row_categories = row.iloc[1:]\n row_categories_sorted = row_categories.sort_values(ascending=False)\n \n return row_categories_sorted.index.values[0:num_top_venues]",
"_____no_output_____"
],
[
"import numpy as np",
"_____no_output_____"
]
],
[
[
"There are way too many venue categories, we can take the top 10 to cluster the neighbourhoods",
"_____no_output_____"
]
],
[
[
"num_top_venues = 10\n\nindicators = ['st', 'nd', 'rd']\n\n# create columns according to number of top venues\ncolumns = ['Neighbourhood']\nfor ind in np.arange(num_top_venues):\n try:\n columns.append('{}{} Most Common Venue'.format(ind+1, indicators[ind]))\n except:\n columns.append('{}th Most Common Venue'.format(ind+1))\n\n# create a new dataframe\nneighborhoods_venues_sorted = pd.DataFrame(columns=columns)\nneighborhoods_venues_sorted['Neighbourhood'] = toronto_grouped['Neighbourhood']\n\nfor ind in np.arange(toronto_grouped.shape[0]):\n neighborhoods_venues_sorted.iloc[ind, 1:] = return_most_common_venues(toronto_grouped.iloc[ind, :], num_top_venues)\n\nneighborhoods_venues_sorted.head()",
"_____no_output_____"
]
],
[
[
"Let's make the model to cluster our Neighbourhoods",
"_____no_output_____"
]
],
[
[
"# import k-means from clustering stage\nfrom sklearn.cluster import KMeans",
"_____no_output_____"
],
[
"# set number of clusters\nk_num_clusters = 5\n\ntoronto_grouped_clustering = toronto_grouped.drop('Neighbourhood', 1)\n\n# run k-means clustering\nkmeans = KMeans(n_clusters=k_num_clusters, random_state=0).fit(toronto_grouped_clustering)\nkmeans",
"_____no_output_____"
]
],
[
[
"Checking the labelling of our model",
"_____no_output_____"
]
],
[
[
"kmeans.labels_[0:100]",
"_____no_output_____"
]
],
[
[
"Let's add the clustering Label column to the top 10 common venue categories",
"_____no_output_____"
]
],
[
[
"neighborhoods_venues_sorted.insert(0, 'Cluster Labels', kmeans.labels_)",
"_____no_output_____"
]
],
[
[
"Join toronto_grouped with combined_data on neighbourhood to add latitude & longitude for each neighborhood to prepare it for plotting",
"_____no_output_____"
]
],
[
[
"toronto_merged = combined_data\n\ntoronto_merged = toronto_merged.join(neighborhoods_venues_sorted.set_index('Neighbourhood'), on='Neighbourhood')\n\ntoronto_merged.head()",
"_____no_output_____"
]
],
[
[
"Drop all the NaN values to prevent data skew",
"_____no_output_____"
]
],
[
[
"toronto_merged_nonan = toronto_merged.dropna(subset=['Cluster Labels'])",
"_____no_output_____"
]
],
[
[
"Plotting the clusters on the map",
"_____no_output_____"
]
],
[
[
"import matplotlib.cm as cm\nimport matplotlib.colors as colors",
"_____no_output_____"
],
[
"map_clusters = folium.Map(location=[latitude, longitude], zoom_start=11)\n\n# set color scheme for the clusters\nx = np.arange(k_num_clusters)\nys = [i + x + (i*x)**2 for i in range(k_num_clusters)]\ncolors_array = cm.rainbow(np.linspace(0, 1, len(ys)))\nrainbow = [colors.rgb2hex(i) for i in colors_array]\n\n# add markers to the map\nmarkers_colors = []\nfor lat, lon, poi, cluster in zip(toronto_merged_nonan['Latitude'], toronto_merged_nonan['Longitude'], toronto_merged_nonan['Neighbourhood'], toronto_merged_nonan['Cluster Labels']):\n label = folium.Popup('Cluster ' + str(int(cluster) +1) + '\\n' + str(poi) , parse_html=True)\n folium.CircleMarker(\n [lat, lon],\n radius=5,\n popup=label,\n color=rainbow[int(cluster-1)],\n fill=True,\n fill_color=rainbow[int(cluster-1)]\n ).add_to(map_clusters)\n \nmap_clusters",
"_____no_output_____"
]
],
[
[
"Let's verify each of our clusters\n\nCluster 1",
"_____no_output_____"
]
],
[
[
"toronto_merged_nonan.loc[toronto_merged_nonan['Cluster Labels'] == 0, toronto_merged_nonan.columns[[1] + list(range(5, toronto_merged_nonan.shape[1]))]]",
"_____no_output_____"
]
],
[
[
"Cluster 2",
"_____no_output_____"
]
],
[
[
"toronto_merged_nonan.loc[toronto_merged_nonan['Cluster Labels'] == 1, toronto_merged_nonan.columns[[1] + list(range(5, toronto_merged_nonan.shape[1]))]]",
"_____no_output_____"
]
],
[
[
"Cluster 3",
"_____no_output_____"
]
],
[
[
"toronto_merged_nonan.loc[toronto_merged_nonan['Cluster Labels'] == 2, toronto_merged_nonan.columns[[1] + list(range(5, toronto_merged_nonan.shape[1]))]]",
"_____no_output_____"
]
],
[
[
"Cluster 4",
"_____no_output_____"
]
],
[
[
"toronto_merged_nonan.loc[toronto_merged_nonan['Cluster Labels'] == 3, toronto_merged_nonan.columns[[1] + list(range(5, toronto_merged_nonan.shape[1]))]]",
"_____no_output_____"
]
],
[
[
"Cluster 5",
"_____no_output_____"
]
],
[
[
"toronto_merged_nonan.loc[toronto_merged_nonan['Cluster Labels'] == 4, toronto_merged_nonan.columns[[1] + list(range(5, toronto_merged_nonan.shape[1]))]]",
"_____no_output_____"
]
],
[
[
"We have successfully cluster Toronto neighbourhood based on venue categories!",
"_____no_output_____"
]
]
] | [
"markdown",
"code",
"markdown",
"code",
"markdown",
"code",
"markdown",
"code",
"markdown",
"code",
"markdown",
"code",
"markdown",
"code",
"markdown",
"code",
"markdown",
"code",
"markdown",
"code",
"markdown",
"code",
"markdown",
"code",
"markdown",
"code",
"markdown",
"code",
"markdown",
"code",
"markdown",
"code",
"markdown",
"code",
"markdown",
"code",
"markdown",
"code",
"markdown",
"code",
"markdown",
"code",
"markdown",
"code",
"markdown",
"code",
"markdown",
"code",
"markdown",
"code",
"markdown",
"code",
"markdown",
"code",
"markdown",
"code",
"markdown",
"code",
"markdown",
"code",
"markdown",
"code",
"markdown",
"code",
"markdown",
"code",
"markdown",
"code",
"markdown"
] | [
[
"markdown",
"markdown"
],
[
"code"
],
[
"markdown",
"markdown"
],
[
"code",
"code"
],
[
"markdown"
],
[
"code",
"code"
],
[
"markdown"
],
[
"code"
],
[
"markdown"
],
[
"code"
],
[
"markdown"
],
[
"code"
],
[
"markdown"
],
[
"code",
"code",
"code",
"code"
],
[
"markdown",
"markdown",
"markdown"
],
[
"code",
"code"
],
[
"markdown",
"markdown",
"markdown"
],
[
"code",
"code"
],
[
"markdown"
],
[
"code",
"code",
"code",
"code"
],
[
"markdown",
"markdown",
"markdown"
],
[
"code",
"code"
],
[
"markdown"
],
[
"code",
"code"
],
[
"markdown"
],
[
"code"
],
[
"markdown"
],
[
"code"
],
[
"markdown"
],
[
"code",
"code"
],
[
"markdown"
],
[
"code"
],
[
"markdown"
],
[
"code"
],
[
"markdown"
],
[
"code"
],
[
"markdown",
"markdown"
],
[
"code"
],
[
"markdown"
],
[
"code"
],
[
"markdown"
],
[
"code"
],
[
"markdown"
],
[
"code",
"code"
],
[
"markdown"
],
[
"code"
],
[
"markdown"
],
[
"code",
"code"
],
[
"markdown"
],
[
"code"
],
[
"markdown"
],
[
"code"
],
[
"markdown"
],
[
"code"
],
[
"markdown"
],
[
"code"
],
[
"markdown"
],
[
"code",
"code"
],
[
"markdown"
],
[
"code"
],
[
"markdown"
],
[
"code"
],
[
"markdown"
],
[
"code"
],
[
"markdown"
],
[
"code"
],
[
"markdown"
],
[
"code"
],
[
"markdown"
]
] |
d0916bf11ab448460bd8b6b1838cbdd34d022202 | 141,575 | ipynb | Jupyter Notebook | Misc Learning/Untitled.ipynb | hamil168/Data-Science-Misc | dd91e4336b6a48a30265a86f8b816658639a17e9 | [
"BSD-2-Clause"
] | null | null | null | Misc Learning/Untitled.ipynb | hamil168/Data-Science-Misc | dd91e4336b6a48a30265a86f8b816658639a17e9 | [
"BSD-2-Clause"
] | 1 | 2018-07-12T02:49:02.000Z | 2018-07-12T02:49:02.000Z | Misc Learning/Untitled.ipynb | hamil168/Learning-Data-Science | dd91e4336b6a48a30265a86f8b816658639a17e9 | [
"BSD-2-Clause"
] | null | null | null | 247.942207 | 69,356 | 0.896592 | [
[
[
"Time Series",
"_____no_output_____"
],
[
"- collecting dxata at regular intervales\n\n**ADDITIVE MODEL**\n- represent a TS as a combinatino fo patterns at diffferent scales.\n- Decompose pieces",
"_____no_output_____"
],
[
"## QUANDL FINANCIAL LIBRARY\n- https://www.quandl.com/tools/python\n- https://github.com/quandl/quandl-python",
"_____no_output_____"
]
],
[
[
"#!pip install quandl",
"_____no_output_____"
],
[
"import quandl",
"_____no_output_____"
],
[
"import pandas as pd",
"_____no_output_____"
],
[
"# quandl.ApiConfig.api_key = 'getyourownkey!'\n\ntesla = quandl.get('WIKI/TSLA')\ngm = quandl.get('WIKI/GM')\ngm.head()",
"_____no_output_____"
],
[
"tesla_copy = tesla.copy()\ngm_copy = gm.copy()",
"_____no_output_____"
]
],
[
[
"## EDA",
"_____no_output_____"
]
],
[
[
"import matplotlib.pyplot as plt\nplt.style.use('ggplot')",
"_____no_output_____"
],
[
"plt.plot(gm.index, gm['Adj. Close'])\nplt.title('GM Stock Prices')\nplt.ylabel('Price USD')\nplt.show()\n",
"_____no_output_____"
],
[
"plt.plot(tesla.index, tesla['Adj. Close'], 'r')\nplt.title('Tesla Stock Price')\nplt.ylabel('Price USD')\nplt.show()",
"_____no_output_____"
],
[
"# Yearly average number of shares outstanding for Tesla and GM\ntesla_shares = {2018: 168e6, 2017: 162e6, 2016: 144e6, 2015: 128e6, 2014: 125e6, 2013: 119e6, 2012: 107e6, 2011: 100e6, 2010: 51e6}\ngm_shares = {2018: 1.42e9, 2017: 1.50e9, 2016: 1.54e9, 2015: 1.59e9, 2014: 1.61e9, 2013: 1.39e9, 2012: 1.57e9, 2011: 1.54e9, 2010:1.50e9}\n\n# create a year column\ntesla['Year'] = tesla.index.year\n\n# Move dates from index to column\ntesla.reset_index(level=0, inplace = True)\ntesla['cap'] = 0\n\n# calculate market cap\n\nfor i, year in enumerate(tesla['Year']):\n \n shares = tesla_shares.get(year)\n \n tesla.ix[i, 'cap'] = shares * tesla.ix[i, 'Adj. Close']\n\n",
"C:\\Users\\Ben\\AppData\\Local\\Continuum\\anaconda3\\lib\\site-packages\\ipykernel_launcher.py:18: DeprecationWarning: \n.ix is deprecated. Please use\n.loc for label based indexing or\n.iloc for positional indexing\n\nSee the documentation here:\nhttp://pandas.pydata.org/pandas-docs/stable/indexing.html#ix-indexer-is-deprecated\n"
],
[
"# create a year column\ngm['Year'] = gm.index.year\n\n# Move dates from index to column\ngm.reset_index(level=0, inplace = True)\ngm['cap'] = 0\n\n# calculate market cap\n\nfor i, year in enumerate(gm['Year']):\n \n shares = gm_shares.get(year)\n \n gm.ix[i, 'cap'] = shares * gm.ix[i, 'Adj. Close']\n\n",
"C:\\Users\\Ben\\AppData\\Local\\Continuum\\anaconda3\\lib\\site-packages\\ipykernel_launcher.py:14: DeprecationWarning: \n.ix is deprecated. Please use\n.loc for label based indexing or\n.iloc for positional indexing\n\nSee the documentation here:\nhttp://pandas.pydata.org/pandas-docs/stable/indexing.html#ix-indexer-is-deprecated\n \n"
],
[
"# Merge Datasets\ncars = gm.merge(tesla, how = 'inner', on = 'Date')\n\ncars.rename(columns = {'cap_x': 'gm_cap', 'cap_y': 'tesla_cap'}, inplace=True)",
"_____no_output_____"
],
[
"cars = cars.loc[:, ['Date', 'gm_cap', 'tesla_cap']]",
"_____no_output_____"
],
[
"cars['gm_cap'] = cars['gm_cap'] / 1e9\ncars['tesla_cap'] = cars['tesla_cap'] / 1e9",
"_____no_output_____"
],
[
"cars.head()",
"_____no_output_____"
],
[
"plt.figure(figsize=(10,8))\nplt.plot(cars['Date'], cars['gm_cap'], 'b-', label = 'GM')\nplt.plot(cars['Date'], cars['tesla_cap'], 'r-', label = 'TESLA')\nplt.title('Market Cap of GM and Tesla')\nplt.legend()\nplt.show()\nplt.show()",
"_____no_output_____"
],
[
"import numpy as np\n\n#find first and last time Tesla was valued higher than GM\nfirst_date = cars.loc[(np.min(list(np.where(cars['tesla_cap'] > cars['gm_cap'])[0]))), 'Date']\nlast_date = cars.loc[(np.max(list(np.where(cars['tesla_cap'] > cars['gm_cap'])[0]))), 'Date']\n\nprint(\"Tesla was valued higher than GM from {} to {}.\".format(first_date.date(), last_date.date()))",
"Tesla was valued higher than GM from 2017-04-10 to 2018-03-23.\n"
]
]
] | [
"markdown",
"code",
"markdown",
"code"
] | [
[
"markdown",
"markdown",
"markdown"
],
[
"code",
"code",
"code",
"code",
"code"
],
[
"markdown"
],
[
"code",
"code",
"code",
"code",
"code",
"code",
"code",
"code",
"code",
"code",
"code"
]
] |
d0916c30a01f48d3250a61e3d8fcd84bc776c75a | 30,362 | ipynb | Jupyter Notebook | soluciones/ja.tavera/tarea4/Tarea4pt1.ipynb | policelula/FISI2028-202120 | fa3578d74a79b395ac03cddc1d44fba2fa5ae1fc | [
"MIT"
] | null | null | null | soluciones/ja.tavera/tarea4/Tarea4pt1.ipynb | policelula/FISI2028-202120 | fa3578d74a79b395ac03cddc1d44fba2fa5ae1fc | [
"MIT"
] | null | null | null | soluciones/ja.tavera/tarea4/Tarea4pt1.ipynb | policelula/FISI2028-202120 | fa3578d74a79b395ac03cddc1d44fba2fa5ae1fc | [
"MIT"
] | null | null | null | 117.227799 | 12,292 | 0.872802 | [
[
[
"empty"
]
]
] | [
"empty"
] | [
[
"empty"
]
] |
d09177700be5f4498078e81d3a0e52988dcc26f1 | 423,688 | ipynb | Jupyter Notebook | PyMC3_Transfer_Test.ipynb | son520804/Video_Composition_Project | 0b0aad5251b579f0f1133f60dc5fcd77e5ff8521 | [
"MIT"
] | null | null | null | PyMC3_Transfer_Test.ipynb | son520804/Video_Composition_Project | 0b0aad5251b579f0f1133f60dc5fcd77e5ff8521 | [
"MIT"
] | null | null | null | PyMC3_Transfer_Test.ipynb | son520804/Video_Composition_Project | 0b0aad5251b579f0f1133f60dc5fcd77e5ff8521 | [
"MIT"
] | null | null | null | 315.009665 | 87,016 | 0.925358 | [
[
[
"# Code Transfer Test\n\nThe code transfer test is designed to test your coding skills that is learnt during the lecture training. The allotted time for the subsequent problem set is approximately 30 minutes. You are allowed to refer to Jupyter notebook throughout the test. Good luck!\n\nJupyter notebook resource: \n\nTimer extension! Heeryung",
"_____no_output_____"
]
],
[
[
"# First, let's import the pandas and numpy libraries\nimport pandas as pd\nimport numpy as np\n\n# In addition, I want to show some plots, so we'll import matplotlib as well\nimport matplotlib.pyplot as plt\n\n# Finally, we'll bring in the scipy stats libraries\nfrom scipy import stats",
"_____no_output_____"
],
[
"# Hide\nimport pandas.util.testing as pdt\n# %install_ext https://raw.githubusercontent.com/minrk/ipython_extensions/master/extensions/writeandexecute.py\n# pdt.assert_series_equal(s1, s2)\n# pdt.assert_frame_equal(f1, f2)\n# pdt.assert_index_equal(i1, i2)",
"<ipython-input-2-b71c00b51b7e>:2: FutureWarning: pandas.util.testing is deprecated. Use the functions in the public API at pandas.testing instead.\n import pandas.util.testing as pdt\n"
]
],
[
[
"# Transfer Test Question\nWhat's the probability that a NFL player makes a field goal? In this problem we are interested in predicting the probability of NFL players to make a field goal. After assuming that the probability of NFL players to make a field goal follows a beta distribution, we observe the field goals data during multiple NFL matches in 2019. \n\nLet us describe the model specification. The prior distribution of the probability p follows beta distribution, with shape parameters alpha and beta. The likelihood, however, follows binomial distribution since we know explicitly about the number of successful and unsuccessful field goals.\n\n$$ Y \\sim Bin(n, p)$$\n$$ p \\sim Beta(\\alpha, \\beta)$$\n\nwhere $\\alpha$ and $\\beta$ are the hyperparameters of p.",
"_____no_output_____"
],
[
"## Question 1: Import Data\nLet us compile the read_csv function to read the NFL data file into a pandas DataFrame. Then, look at the first 5 lines. The file name of the CSV file is nfl.csv.",
"_____no_output_____"
]
],
[
[
"# Answer\nfile = 'nfl.csv'\n\n\ndef read_csv(file):\n \"\"\"Read the nfl.csv data and return the first few lines of \n the csv file.\n\n \"\"\"\n ### BEGIN SOLUTION\n data = pd.read_csv(file)\n # And let's look at the first few lines\n return data.head()\n\n ### END SOLUTION\nread_csv(file)",
"_____no_output_____"
],
[
"# Basic Test Case\n\"\"\"Check that read_csv function returns the correct dataframe output and format.\"\"\"\ndf1 = read_csv(file)\ndf2 = pd.read_csv(file).head()\npdt.assert_frame_equal(df1, df2)\n# Data Type Test Case\nassert isinstance(read_csv(file), pd.core.frame.DataFrame)\n\n# Advanced Test Case",
"_____no_output_____"
]
],
[
[
"## Question 2: Column Mean\nLet us define the column_mean function which takes the csv file and the column name as inputs, and returns the mean probability of making field goals. (Look at the FG column)",
"_____no_output_____"
]
],
[
[
"# Sample Answer\ncolumn = 'FG'\n\n\ndef column_mean(file, column):\n \"\"\"Take the nfl.csv file and a specific column as input.\n Compute the mean value for a column in pandas dataframe.\n\n \"\"\"\n ### BEGIN SOLUTION\n data = pd.read_csv(file)\n return data[column].mean()\n\n ### END SOLUTION\ncolumn_mean(file, column)",
"_____no_output_____"
],
[
"# Basic Test Case\n\"\"\"Test whether the data type and value of column mean are correctly returned.\"\"\"\nassert column_mean(file, column) == pd.read_csv(file)[column].mean()\nassert 0.836319 <= column_mean(file, column) <= 0.836321\n# Advanced Test Cases\nassert isinstance(column_mean(file, column), (np.float64, float))",
"_____no_output_____"
]
],
[
[
"## Question 3: Specify Prior and Likelihood\n\nLet us specify the prior and likelihood. We are going to split two code chunks to perform the following steps:\n\nIn the first code chunk, we initialize a random number generator as 123 to make the random numbers predictable. Then, we assign the hyperparameters of prior. In this question we use beta distribution as the prior. Beta distribution has two shape parameters, which is alpha and beta. We set the parameter names as alpha and beta, and assign values 40 and 20, respectively. Finally, we set the sample size as 100, using the parameter name size.\n\nIn the second code chunk, we set up a variable observed, which is the observed outcome variable. We define a function called likelihood which takes a csv file, a column in the dataset and the sample size as inputs, and return the observed outcome variable, which the product of size and the mean field goal probability. (You can take the result from question 2).",
"_____no_output_____"
]
],
[
[
"# Sample answer\n\n### BEGIN SOLUTION\n# We initialize random number generator seed for reproducible results\nnp.random.seed(123)\n\n# We assign the hyperparameters of prior\n# We assign the shape parameters alpha and beta as 40 and 20. \nalpha, beta = 40, 20\n\n# Then we make up the sample size as 100\nsize = 100\n### END SOLUTION",
"_____no_output_____"
],
[
"# Basic Test Case\nfrom nose.tools import assert_equal\nassert_equal(alpha, 40)\nassert_equal(beta, 20)\nassert_equal(size, 100)",
"_____no_output_____"
],
[
"# Finally, we set up Y the observed outcome variable as the product of size and mean field goal probability\n\ndef likelihood(file, column, size):\n \"\"\"Compute the product of the column mean of field goal probability among NFL players and \n sample size.\n \"\"\"\n ### BEGIN SOLUTION\n observed = column_mean(file, column) * size\n return observed\n ### END SOLUTION\nobserved = likelihood(file, column, size)",
"_____no_output_____"
],
[
"# Basic Test Case\nassert_equal(likelihood(file, column, size), column_mean(file, column) * size)\n# Advanced Test Case\nassert 83 <= likelihood(file, column, size) <= 84\nassert isinstance(likelihood(file, column, size), (np.float64, float))",
"_____no_output_____"
]
],
[
[
"## Optional Question\n\nYou can run the following code to generate a plot for the beta distribution based on the alpha and beta parameters you defined above. Here the scipy.stats.beta function and matplotlib package are used to generate the probability density function plot.",
"_____no_output_____"
]
],
[
[
"# We define the linestyle and set up a linear space to clearly plot the beta distribution\nx = np.linspace(0,1,1002)[1:-1]\n\n# Then, we use scipy.stats.beta function to set up beta distribution\ndist = stats.beta(alpha, beta)\n\n# Now we want to define a plot_beta_pdf function to generate a figure \n# showing the probability density function of the beta distribution\ndef plot_beta_pdf(x,dist):\n # Note that we want the figure to be 8 inches height and 8 inches width\n plt.figure(figsize=(8,8))\n \n # We read the linear space and the beta pdf into the plot, and we want to generate a \n # continuous and black curve. We also want to show a legend at the top-right corner with \n # the alpha and beta value\n plt.plot(x, dist.pdf(x), ls = '-', c = 'black',\n label = r'$\\alpha = %.1f,\\ \\beta=%.1f$' % (alpha, beta))\n plt.legend(loc = 0)\n \n # Finally, we set up the value ranges and labels for x-axis and y-axis and show the plot\n plt.xlim(0,1)\n plt.ylim(0,10)\n plt.xlabel('$x$')\n plt.ylabel(r'$p(x|\\alpha, \\beta)$')\n plt.title('Beta Distribution')\n plt.show()\n \nplot_beta_pdf(x,dist)",
"_____no_output_____"
]
],
[
[
"You will see that the beta distribution curve surprisingly resembles the case when we conduct binomial trials with roughly 40 successes and 20 failures.\n\nIn fact, we can think of $\\alpha - 1$ as the number of successes and $\\beta - 1$ as the number of failures. You can choose the $\\alpha$ and $\\beta$ parameters however you think they should look like. If you want the probability of success to become very high, let us say 95 percent, set 95 for $\\alpha$ and 5 for $\\beta$. If you think otherwise, let us say 5 percent, set 95 for $\\beta$ and 5 for $\\alpha$.",
"_____no_output_____"
]
],
[
[
"import pymc3 as pm",
"WARNING (theano.configdefaults): g++ not available, if using conda: `conda install m2w64-toolchain`\nC:\\Users\\sonso\\Anaconda3\\lib\\site-packages\\theano\\configdefaults.py:560: UserWarning: DeprecationWarning: there is no c++ compiler.This is deprecated and with Theano 0.11 a c++ compiler will be mandatory\n warnings.warn(\"DeprecationWarning: there is no c++ compiler.\"\nWARNING (theano.configdefaults): g++ not detected ! Theano will be unable to execute optimized C-implementations (for both CPU and GPU) and will default to Python implementations. Performance will be severely degraded. To remove this warning, set Theano flags cxx to an empty string.\nWARNING (theano.tensor.blas): Using NumPy C-API based implementation for BLAS functions.\n"
],
[
"# Hide\nimport unittest",
"_____no_output_____"
]
],
[
[
"## Question 4: Train MCMC sampler\n\nLet us train the Markov Chain Monte Carlo sampler. In this example, we use the default NUTS algorithm to sample the posterior distribution. We need to perform the following steps:\n\nFirst we set a variable called niter, the number of draw, to 1000.\n\nSecond, we instantiate the model object.\n\nThird, we specify the beta distribution as the prior for the probability of making a field goal, using the variable name p. Please remember to use the alpha and beta value specified from question 3. Note that the function for assigning beta distribution is pm.Beta().\n\nWe also specify the observed likelihood as binomial distribution, using the variable name y. The parameters taken are sample size (n), probability (p) and observed data (observed). Note that the function for binomial distribution is pm.Binomial().\n\nFinally, we start the sampler to take 1000 draws (from niter variable) and take 3 chains. We also provide a seed to the random_seed generator to make the results reproducible. The results should be returned as a trace object.",
"_____no_output_____"
]
],
[
[
"# Sample answer\nseed = 1000\ndef sampler(alpha, beta, size, observed, seed):\n \"\"\"Train a MCMC sampler to generate posterior samples for the \n field goal probability.\n \n \"\"\"\n ### BEGIN SOLUTION\n niter = 1000\n model = pm.Model()\n\n with model:\n p = pm.Beta('p', alpha=alpha, beta=beta)\n\n # Specify the likelihood function (sampling distribution)\n y = pm.Binomial('y', n=size, p=p, observed=observed)\n trace = pm.sample(niter, chains = 3, random_seed = seed)\n return trace\n ### END SOLUTION\ntrace = sampler(alpha, beta, size, observed, seed)\ntrace",
"Auto-assigning NUTS sampler...\nInitializing NUTS using jitter+adapt_diag...\nMultiprocess sampling (3 chains in 4 jobs)\nNUTS: [p]\n"
],
[
"# Test Cases\n\"\"\"Check the correctness of parameters assigned to the PyMC3 model.\"\"\"\n#assert_equal(seed, 1000)\nassert isinstance(trace, (pm.backends.base.MultiTrace))\nassert_equal(trace.varnames, ['p_logodds__', 'p'])\nassert_equal(len(trace['p']), 3000)\n#\n#",
"_____no_output_____"
]
],
[
[
"## Posterior Diagnostics",
"_____no_output_____"
],
[
"## Question 5\n\nNow we look at the posterior diagnostics. Recall we will plot a traceplot to visualize the posterior distribution of parameters of interest. In addition, we also obtain Gelman-Rubin statistics to check whether the parameter of interest converges.\n\na) Define a function named traceplot which takes the trace object as input and returns a traceplot for the variable p, the probability of making a field goal. ",
"_____no_output_____"
]
],
[
[
"# Answer 5a\n# Plot results Traceplot\ndef traceplot(trace):\n \"\"\"Generate the posterior density plot and trajectory plot for the field goal probability.\"\"\"\n \n # BEGIN SOLUTION\n return pm.traceplot(trace, varnames = ['p'])\n # END SOLUTION\ntraceplot(trace)\nplt.show()",
"C:\\Users\\sonso\\Anaconda3\\lib\\site-packages\\pymc3\\plots\\__init__.py:35: UserWarning: Keyword argument `varnames` renamed to `var_names`, and will be removed in pymc3 3.8\n warnings.warn('Keyword argument `{old}` renamed to `{new}`, and will be removed in pymc3 3.8'.format(old=old, new=new))\nC:\\Users\\sonso\\Anaconda3\\lib\\site-packages\\arviz\\data\\io_pymc3.py:87: FutureWarning: Using `from_pymc3` without the model will be deprecated in a future release. Not using the model will return less accurate and less useful results. Make sure you use the model argument or call from_pymc3 within a model context.\n warnings.warn(\n"
],
[
"# Test cases\n\"\"\"Check the length data type and shape of the traceplot object for sanity purpose.\nTo make sure the number of plots generated are correct.\"\"\"\nassert_equal(len(traceplot(trace)), 1)\nassert isinstance(traceplot(trace), np.ndarray)\nassert_equal(traceplot(trace).shape, (1,2))",
"C:\\Users\\sonso\\Anaconda3\\lib\\site-packages\\pymc3\\plots\\__init__.py:35: UserWarning: Keyword argument `varnames` renamed to `var_names`, and will be removed in pymc3 3.8\n warnings.warn('Keyword argument `{old}` renamed to `{new}`, and will be removed in pymc3 3.8'.format(old=old, new=new))\nC:\\Users\\sonso\\Anaconda3\\lib\\site-packages\\arviz\\data\\io_pymc3.py:87: FutureWarning: Using `from_pymc3` without the model will be deprecated in a future release. Not using the model will return less accurate and less useful results. Make sure you use the model argument or call from_pymc3 within a model context.\n warnings.warn(\nC:\\Users\\sonso\\Anaconda3\\lib\\site-packages\\pymc3\\plots\\__init__.py:35: UserWarning: Keyword argument `varnames` renamed to `var_names`, and will be removed in pymc3 3.8\n warnings.warn('Keyword argument `{old}` renamed to `{new}`, and will be removed in pymc3 3.8'.format(old=old, new=new))\nC:\\Users\\sonso\\Anaconda3\\lib\\site-packages\\arviz\\data\\io_pymc3.py:87: FutureWarning: Using `from_pymc3` without the model will be deprecated in a future release. Not using the model will return less accurate and less useful results. Make sure you use the model argument or call from_pymc3 within a model context.\n warnings.warn(\nC:\\Users\\sonso\\Anaconda3\\lib\\site-packages\\pymc3\\plots\\__init__.py:35: UserWarning: Keyword argument `varnames` renamed to `var_names`, and will be removed in pymc3 3.8\n warnings.warn('Keyword argument `{old}` renamed to `{new}`, and will be removed in pymc3 3.8'.format(old=old, new=new))\nC:\\Users\\sonso\\Anaconda3\\lib\\site-packages\\arviz\\data\\io_pymc3.py:87: FutureWarning: Using `from_pymc3` without the model will be deprecated in a future release. Not using the model will return less accurate and less useful results. Make sure you use the model argument or call from_pymc3 within a model context.\n warnings.warn(\n"
]
],
[
[
"b) (Optional) What trends do you see in the posterior distribution of the probability of making a field goal?",
"_____no_output_____"
],
[
"c) Define a function named posterior_summary which takes a trace object as input and displays a table-based summary of posterior statistics rounded by 2 digits. ",
"_____no_output_____"
]
],
[
[
"# Answer 5c\n# Obtain summary statistics for posterior distributions\ndef posterior_summary(trace):\n \"\"\"Generate a table-based summary for the field goal probability.\"\"\"\n # BEGIN SOLUTION\n return pm.summary(trace).round(2)\n # END SOLUTION",
"_____no_output_____"
],
[
"# Test Cases\n\"\"\"Check whether the summary output is correctly generated.\"\"\"\nsum1 = posterior_summary(trace)\nsum2 = pm.summary(trace).round(2)\npdt.assert_frame_equal(sum1, sum2)\nassert_equal(posterior_summary(trace).shape, (1, 11))",
"C:\\Users\\sonso\\Anaconda3\\lib\\site-packages\\arviz\\data\\io_pymc3.py:87: FutureWarning: Using `from_pymc3` without the model will be deprecated in a future release. Not using the model will return less accurate and less useful results. Make sure you use the model argument or call from_pymc3 within a model context.\n warnings.warn(\nC:\\Users\\sonso\\Anaconda3\\lib\\site-packages\\arviz\\data\\io_pymc3.py:87: FutureWarning: Using `from_pymc3` without the model will be deprecated in a future release. Not using the model will return less accurate and less useful results. Make sure you use the model argument or call from_pymc3 within a model context.\n warnings.warn(\nC:\\Users\\sonso\\Anaconda3\\lib\\site-packages\\arviz\\data\\io_pymc3.py:87: FutureWarning: Using `from_pymc3` without the model will be deprecated in a future release. Not using the model will return less accurate and less useful results. Make sure you use the model argument or call from_pymc3 within a model context.\n warnings.warn(\n"
]
],
[
[
"d) What is the posterior mean and standard deviation of the probability of making a field goal? Define a function posterior_statistics which takes a trace object as input and return the posterior mean and posterior standard deviation as a tuple looks like (mean, sd).",
"_____no_output_____"
]
],
[
[
"# Answer 5d\n\ndef posterior_statistics(trace):\n return (posterior_summary(trace).iloc[0,0], posterior_summary(trace).iloc[0,1])\nposterior_statistics(trace)",
"C:\\Users\\sonso\\Anaconda3\\lib\\site-packages\\arviz\\data\\io_pymc3.py:87: FutureWarning: Using `from_pymc3` without the model will be deprecated in a future release. Not using the model will return less accurate and less useful results. Make sure you use the model argument or call from_pymc3 within a model context.\n warnings.warn(\nC:\\Users\\sonso\\Anaconda3\\lib\\site-packages\\arviz\\data\\io_pymc3.py:87: FutureWarning: Using `from_pymc3` without the model will be deprecated in a future release. Not using the model will return less accurate and less useful results. Make sure you use the model argument or call from_pymc3 within a model context.\n warnings.warn(\n"
],
[
"# Test Cases\n\"\"\"Check whether the posterior mean and posterior standard deviation are correctly generated.\"\"\"\nassert_equal(posterior_statistics(trace), \n tuple([posterior_summary(trace).iloc[0,0], posterior_summary(trace).iloc[0,1]]))\nassert isinstance(posterior_statistics(trace), tuple)\nassert_equal(len(posterior_statistics(trace)), 2)\n",
"C:\\Users\\sonso\\Anaconda3\\lib\\site-packages\\arviz\\data\\io_pymc3.py:87: FutureWarning: Using `from_pymc3` without the model will be deprecated in a future release. Not using the model will return less accurate and less useful results. Make sure you use the model argument or call from_pymc3 within a model context.\n warnings.warn(\nC:\\Users\\sonso\\Anaconda3\\lib\\site-packages\\arviz\\data\\io_pymc3.py:87: FutureWarning: Using `from_pymc3` without the model will be deprecated in a future release. Not using the model will return less accurate and less useful results. Make sure you use the model argument or call from_pymc3 within a model context.\n warnings.warn(\nC:\\Users\\sonso\\Anaconda3\\lib\\site-packages\\arviz\\data\\io_pymc3.py:87: FutureWarning: Using `from_pymc3` without the model will be deprecated in a future release. Not using the model will return less accurate and less useful results. Make sure you use the model argument or call from_pymc3 within a model context.\n warnings.warn(\nC:\\Users\\sonso\\Anaconda3\\lib\\site-packages\\arviz\\data\\io_pymc3.py:87: FutureWarning: Using `from_pymc3` without the model will be deprecated in a future release. Not using the model will return less accurate and less useful results. Make sure you use the model argument or call from_pymc3 within a model context.\n warnings.warn(\nC:\\Users\\sonso\\Anaconda3\\lib\\site-packages\\arviz\\data\\io_pymc3.py:87: FutureWarning: Using `from_pymc3` without the model will be deprecated in a future release. Not using the model will return less accurate and less useful results. Make sure you use the model argument or call from_pymc3 within a model context.\n warnings.warn(\nC:\\Users\\sonso\\Anaconda3\\lib\\site-packages\\arviz\\data\\io_pymc3.py:87: FutureWarning: Using `from_pymc3` without the model will be deprecated in a future release. Not using the model will return less accurate and less useful results. Make sure you use the model argument or call from_pymc3 within a model context.\n warnings.warn(\nC:\\Users\\sonso\\Anaconda3\\lib\\site-packages\\arviz\\data\\io_pymc3.py:87: FutureWarning: Using `from_pymc3` without the model will be deprecated in a future release. Not using the model will return less accurate and less useful results. Make sure you use the model argument or call from_pymc3 within a model context.\n warnings.warn(\nC:\\Users\\sonso\\Anaconda3\\lib\\site-packages\\arviz\\data\\io_pymc3.py:87: FutureWarning: Using `from_pymc3` without the model will be deprecated in a future release. Not using the model will return less accurate and less useful results. Make sure you use the model argument or call from_pymc3 within a model context.\n warnings.warn(\n"
]
],
[
[
"e) Define a function named gelman_rubin which takes a trace object as input and return the Gelman-Rubin statistics. Does the posterior distribution converge?",
"_____no_output_____"
]
],
[
[
"# Answer\n# Get Gelman-Rubin Convergence Criterion\ndef gelman_rubin(trace):\n \"\"\"Compute Gelman-Rubin statistics for the posterior samples of field goal probability.\"\"\"\n ### BEGIN SOLUTION\n return print(pm.rhat(trace,\n varnames=['p']))\n ### END SOLUTION\ngelman_rubin(trace)",
"C:\\Users\\sonso\\Anaconda3\\lib\\site-packages\\pymc3\\stats\\__init__.py:33: UserWarning: Keyword argument `varnames` renamed to `var_names`, and will be removed in pymc3 3.9\n warnings.warn(\nC:\\Users\\sonso\\Anaconda3\\lib\\site-packages\\arviz\\data\\io_pymc3.py:87: FutureWarning: Using `from_pymc3` without the model will be deprecated in a future release. Not using the model will return less accurate and less useful results. Make sure you use the model argument or call from_pymc3 within a model context.\n warnings.warn(\n"
],
[
"# Test cases\nassert_equal(gelman_rubin(trace), pm.rhat(trace,varnames=['p']))\n#assert 1 <= gelman_rubin(trace) <= 1.1",
"<xarray.Dataset>\nDimensions: ()\nData variables:\n p float64 1.001\n"
],
[
"gelman_rubin(trace)[p]",
"_____no_output_____"
]
],
[
[
"## Bonus Section:",
"_____no_output_____"
],
[
"### Effective sample size\n\nThe calculation of effective sample size is given by the following formula:\n$$\\hat{n}_{eff} = \\frac{mn}{1 + 2 \\sum_{t=1}^T \\hat{\\rho}_t}$$\nwhere m is the number of chains, n the number of steps per chain, T the time when the autocorrelation first becomes negative, and ρ̂_t the autocorrelation at lag t.\n\n",
"_____no_output_____"
]
],
[
[
"## Calculate effective sample size\npm.effective_n(trace)",
"/Users/son520804/anaconda3/lib/python3.6/site-packages/pymc3/stats/__init__.py:50: UserWarning: effective_n has been deprecated. In the future, use ess instead.\n warnings.warn(\"effective_n has been deprecated. In the future, use ess instead.\")\n"
]
],
[
[
"As you can see, the effective sample size is 1271 for the total of the 3 chains. Since by default, the tuning sample is 500, leaving 500 samples to be resampled. So that means the autocorrelation is not extreme, the MCMC converges well.",
"_____no_output_____"
],
[
"### Geweke Statistics\n\nAs an alternative of Gelman-Rubin statistics, Geweke provides a sanity check of the convergence of MCMC chains. Geweke statistics compares the mean and variance of segments from the beginning and end of each single MCMC chain for a parameter. If the absolute value of Geweke statistics exceeds 1, it indicates a lack of convergence and suggests that additional samples are requred to achieve convergence.",
"_____no_output_____"
]
],
[
[
"# We can create a plot to show the trajectory of Geweke statistics \nplt.plot(pm.geweke(trace['p'])[:,1], 'o')\nplt.axhline(1, c='red')\nplt.axhline(-1, c='red')\nplt.gca().margins(0.05)\nplt.show()\npass",
"_____no_output_____"
]
],
[
[
"Since the Geweke statistics are less than 1 in absolute value, it indicates a good convergence in the MCMC chains.",
"_____no_output_____"
],
[
"# Debug Question\n\nThe following question requires you to read the code carefully and correct the codes with errors. A Umich cognitive science research team want to produce an elegant code to run a MCMC sampler to determine the IQ distribution of the undergraduate students studying at the University of Michigan. They studied the literature and inferred the following priors:\n\n$IQ \\sim Normal(mean = 105, variance = 7^2)$\n\n$\\sigma(IQ) \\sim HalfNormal(\\beta = 2)$\n\nThen they collected experimental data from 100 students who took the Wechsler Adult Intelligence Scale (WAIS) test at the cognitive science building. The first code chunk gives their test results.\n\nAfter debugging the code, the resulting code should be error-free and return the trace object.",
"_____no_output_____"
]
],
[
[
"# IQ test results for the 100 students\nnp.random.seed(123)\ny = np.random.normal(100, 15, 100)",
"_____no_output_____"
],
[
"# Hierarchical Bayesian Modeling\n\nseed = 123\nniter = 1000\nnchains = 3\nwith pm.Model() as model:\n \"\"\"Deploy NUTS sampler to update the distribution for students' IQ.\"\"\"\n \n ### BEGIN CODE\n mu = pm.Normal('mu', mu = 105, sigma = 7)\n sigma = pm.HalfCauchy('sigma', beta = 2)\n y_observed = pm.Normal('y_observed',\n mu=mu,\n sigma=sigma,\n observed=y)\n \n trace2 = pm.sample(niter, chains = nchains, random_seed = seed)\n ### END CODE",
"Auto-assigning NUTS sampler...\nInitializing NUTS using jitter+adapt_diag...\nMultiprocess sampling (3 chains in 2 jobs)\nNUTS: [sigma, mu]\nSampling 3 chains, 0 divergences: 100%|██████████| 4500/4500 [02:46<00:00, 27.04draws/s]\n"
],
[
"# Test cases\nassert_equal(type(posterior_summary(trace2)), pd.core.frame.DataFrame)\nassert_equal(posterior_summary(trace2).shape, (2,11))",
"_____no_output_____"
]
],
[
[
"Reference:\n 1. https://docs.pymc.io/api/stats.html\n 2. http://pymc-devs.github.io/pymc/modelchecking.html?highlight=geweke\n 3. Wagenmakers, E., Morey, R. D., & Lee, M. D. (n.d.). Bayesian Benefits for the Pragmatic Researcher, 1–11.",
"_____no_output_____"
]
]
] | [
"markdown",
"code",
"markdown",
"code",
"markdown",
"code",
"markdown",
"code",
"markdown",
"code",
"markdown",
"code",
"markdown",
"code",
"markdown",
"code",
"markdown",
"code",
"markdown",
"code",
"markdown",
"code",
"markdown",
"code",
"markdown",
"code",
"markdown",
"code",
"markdown"
] | [
[
"markdown"
],
[
"code",
"code"
],
[
"markdown",
"markdown"
],
[
"code",
"code"
],
[
"markdown"
],
[
"code",
"code"
],
[
"markdown"
],
[
"code",
"code",
"code",
"code"
],
[
"markdown"
],
[
"code"
],
[
"markdown"
],
[
"code",
"code"
],
[
"markdown"
],
[
"code",
"code"
],
[
"markdown",
"markdown"
],
[
"code",
"code"
],
[
"markdown",
"markdown"
],
[
"code",
"code"
],
[
"markdown"
],
[
"code",
"code"
],
[
"markdown"
],
[
"code",
"code",
"code"
],
[
"markdown",
"markdown"
],
[
"code"
],
[
"markdown",
"markdown"
],
[
"code"
],
[
"markdown",
"markdown"
],
[
"code",
"code",
"code"
],
[
"markdown"
]
] |
d091795fb0901e63ef53ea0fee55a6d349067dff | 132,193 | ipynb | Jupyter Notebook | prov/Provenance using KN resource.ipynb | CSIRO-enviro-informatics/jupyter-examples | ac29e1e9b82c8ad6a4e8154405a7925858f7b97e | [
"MIT"
] | 2 | 2020-07-29T21:50:35.000Z | 2020-09-23T05:18:19.000Z | prov/Provenance using KN resource.ipynb | oznome/jupyter-examples | ac29e1e9b82c8ad6a4e8154405a7925858f7b97e | [
"MIT"
] | null | null | null | prov/Provenance using KN resource.ipynb | oznome/jupyter-examples | ac29e1e9b82c8ad6a4e8154405a7925858f7b97e | [
"MIT"
] | null | null | null | 228.312608 | 117,942 | 0.912824 | [
[
[
"# Creating Provenance an Example Using a Python Notebook",
"_____no_output_____"
]
],
[
[
"import prov, requests, pandas as pd, io, git, datetime, urllib\nfrom prov.model import ProvDocument",
"_____no_output_____"
]
],
[
[
"## Initialising a Provenance Document\n\nFirst we use the prov library to create a provenance and initialise it with some relevant namespaces that can be used later to define provenance activities and entities",
"_____no_output_____"
]
],
[
[
"pg = ProvDocument()\n\nkn_id = \"data/data-gov-au/number-of-properties-by-suburb-and-planning-zone-csv\"\npg.add_namespace('kn', 'http://oznome.csiro.au/id/')\npg.add_namespace('void', 'http://vocab.deri.ie/void#')\npg.add_namespace('foaf', 'http://xmlns.com/foaf/0.1/')\npg.add_namespace('dc', 'http://purl.org/dc/elements/1.1/')\npg.add_namespace('doap', 'http://usefulinc.com/ns/doap#')\n\n",
"_____no_output_____"
]
],
[
[
"## Processing the Data\n\nProcessing could be anything and represents one or more provenance activities. In this example we use a KN metadata record to retrieve data on residential properities. We intersperse definition of provenance into this processing but we could have easily seperated it out and performed it after the processing steps\n",
"_____no_output_____"
],
[
"First we define an entity that describes the KN metadata records which we are using here",
"_____no_output_____"
]
],
[
[
"input_identifier = 'kn:'+ kn_id\ninput_entity = pg.entity(input_identifier, {'prov:label': 'road static parking off street', 'prov:type': 'void:Dataset'})",
"_____no_output_____"
]
],
[
[
"Then we proceed to drill down to get detailed data that we've found associated with this record",
"_____no_output_____"
]
],
[
[
"start_time = datetime.datetime.now()",
"_____no_output_____"
],
[
"response = requests.get('https://data.sa.gov.au/data/dataset/d080706c-2c05-433d-b84d-9aa9b6ccae73/resource/4a47e89b-4be8-430d-8926-13b180025ac6/download/city-of-onkaparinga---number-of-properties-by-suburb-and-planning-zone-2016.csv')",
"_____no_output_____"
],
[
"url_data = response.content",
"_____no_output_____"
],
[
"dataframe = pd.read_csv(io.StringIO(url_data.decode('utf-8')))",
"_____no_output_____"
],
[
"dataframe.columns",
"_____no_output_____"
]
],
[
[
"Our processing is very simple we are subsetting the original dataset here and creating a new dataset called residential_frame that we will then save to disk",
"_____no_output_____"
]
],
[
[
"residential_frame = dataframe[dataframe['Zone_Description'] == 'Residential']",
"_____no_output_____"
],
[
"residential_frame_file_name = \"filtered_residential_data.csv\" \nresidential_frame.to_csv(residential_frame_file_name)\nend_time = datetime.datetime.now()",
"_____no_output_____"
]
],
[
[
"## Completing Provenance\n\nWe have began to build a provenance record but we are missing a record of the activity that transforms our input into the output and we are also missing a description of the output ",
"_____no_output_____"
],
[
"### Generating an output provenance entity\n\nIdeally we would store our output provenance entity somewhere known and persistent and identify it with a persistent url. However we can still mint an identifier and then describe the dataset in useful ways that will make it easy to find and query from later. To do this we create a new entity record and use the file name and sha hash of the file to describe it.",
"_____no_output_____"
]
],
[
[
"import subprocess\noutput = subprocess.check_output(\"sha1sum \"+ residential_frame_file_name, shell=True)",
"_____no_output_____"
],
[
"sha1 = str(output).split(' ')[0][2:]",
"_____no_output_____"
],
[
"output_identifier = 'kn:' + sha1\noutput_entity = pg.entity(output_identifier , {'prov:label': residential_frame_file_name, 'prov:type': 'void:Dataset'})",
"_____no_output_____"
]
],
[
[
"### Describing the activity \n\nWe need to connect the entity representing the input data to the entity representing the output data and we may want to describe the activity that transforms the input into the output. In this case the activity is this Jupyter Notebook. One way of storing provenance information in it is to make sure it is version controlled in git and then record these details. ",
"_____no_output_____"
],
[
"## Connecting things together into the provenance graph",
"_____no_output_____"
]
],
[
[
"import re, ipykernel, json",
"_____no_output_____"
],
[
"%%javascript\nvar nb = Jupyter.notebook;\nvar port = window.location.port;\nnb.kernel.execute(\"NB_Port = '\" + port + \"'\");",
"_____no_output_____"
],
[
"kernel_id = re.search('kernel-(.*).json', ipykernel.connect.get_connection_file()).group(1)\nresponse = requests.get('http://127.0.0.1:{port}/jupyter/api/sessions'.format(port=NB_Port))\nresponse.content\nmatching = [s for s in json.loads(response.text) if s['kernel']['id'] == kernel_id]\nif matching:\n matched = matching[0]['notebook']['path']",
"_____no_output_____"
],
[
"notebook_file_name = matched.split('/')[-1]",
"_____no_output_____"
]
],
[
[
"One gotcha here is that we need to make sure this notebooks relevant version has been committed and pushed to the remote. So do that and then execute these cells.",
"_____no_output_____"
]
],
[
[
"repo = git.Repo('./', search_parent_directories=True)\ncurrent_git_sha = repo.head.object.hexsha\ncurrent_git_remote = list(repo.remotes['origin'].urls)[0]",
"_____no_output_____"
],
[
"current_git_sha",
"_____no_output_____"
],
[
"current_git_remote",
"_____no_output_____"
],
[
"process_identifier = 'kn:' + 'notebook/' + urllib.parse.quote(notebook_file_name + current_git_sha, safe='')",
"_____no_output_____"
],
[
"process_identifier\nprocess_entity = pg.entity(process_identifier, other_attributes={'dc:description': 'a jupyter notebook used that demonstrates provenance', 'doap:GitRepository' : current_git_remote, 'doap:Version' : current_git_sha })",
"_____no_output_____"
],
[
"import time\nsunixtime = time.mktime(start_time.timetuple())\neunixtime = time.mktime(end_time.timetuple())\nactivity_identifier = 'kn:' + 'notebook/' + urllib.parse.quote(notebook_file_name + current_git_sha, safe='') + str(sunixtime) + str(eunixtime)\nactivity = pg.activity(activity_identifier, startTime=start_time, endTime=end_time)",
"_____no_output_____"
],
[
"pg.wasGeneratedBy(activity=activity, entity=output_entity)",
"_____no_output_____"
],
[
"pg.used(activity=activity, entity=input_entity)",
"_____no_output_____"
],
[
"pg.used(activity=activity, entity=process_entity)",
"_____no_output_____"
],
[
"pg",
"_____no_output_____"
],
[
"# visualize the graph\nfrom prov.dot import prov_to_dot\ndot = prov_to_dot(pg)\ndot.write_png('prov.png')",
"_____no_output_____"
],
[
"from IPython.display import Image\nImage('prov.png')",
"_____no_output_____"
]
],
[
[
"## Posting to a Provenance Storage System\nTBC",
"_____no_output_____"
]
]
] | [
"markdown",
"code",
"markdown",
"code",
"markdown",
"code",
"markdown",
"code",
"markdown",
"code",
"markdown",
"code",
"markdown",
"code",
"markdown",
"code",
"markdown"
] | [
[
"markdown"
],
[
"code"
],
[
"markdown"
],
[
"code"
],
[
"markdown",
"markdown"
],
[
"code"
],
[
"markdown"
],
[
"code",
"code",
"code",
"code",
"code"
],
[
"markdown"
],
[
"code",
"code"
],
[
"markdown",
"markdown"
],
[
"code",
"code",
"code"
],
[
"markdown",
"markdown"
],
[
"code",
"code",
"code",
"code"
],
[
"markdown"
],
[
"code",
"code",
"code",
"code",
"code",
"code",
"code",
"code",
"code",
"code",
"code",
"code"
],
[
"markdown"
]
] |
d09179d1675ef5434edbd568a8909bed7cc65c99 | 9,809 | ipynb | Jupyter Notebook | source-code/cython/cython.ipynb | gjbex/Python-for-HPC | 91110a76d3d57447bab1b53eeeee394198fdc186 | [
"CC-BY-4.0"
] | 15 | 2019-12-09T09:57:12.000Z | 2022-01-20T16:38:40.000Z | source-code/cython/cython.ipynb | gjbex/Python-for-HPC | 91110a76d3d57447bab1b53eeeee394198fdc186 | [
"CC-BY-4.0"
] | null | null | null | source-code/cython/cython.ipynb | gjbex/Python-for-HPC | 91110a76d3d57447bab1b53eeeee394198fdc186 | [
"CC-BY-4.0"
] | 5 | 2020-08-29T14:55:08.000Z | 2022-01-25T13:00:30.000Z | 21.510965 | 258 | 0.483332 | [
[
[
"# Cython in Jupyter notebooks",
"_____no_output_____"
],
[
"To use cython in a Jupyter notebook, the extension has to be loaded.",
"_____no_output_____"
]
],
[
[
"%load_ext cython",
"_____no_output_____"
]
],
[
[
"## Pure Python",
"_____no_output_____"
],
[
"To illustrate the performance difference between a pure Python function and a cython implementation, consider a function that computes the list of the first $k_{\\rm max}$ prime numbers.",
"_____no_output_____"
]
],
[
[
"from array import array",
"_____no_output_____"
],
[
"def primes(kmax, p=None):\n if p is None:\n p = array('i', [0]*kmax)\n result = []\n k, n = 0, 2\n while k < len(p):\n i = 0\n while i < k and n % p[i] != 0:\n i += 1\n if i == k:\n p[k] = n\n k += 1\n result.append(n)\n n += 1\n return result",
"_____no_output_____"
]
],
[
[
"Checking the results for the 20 first prime numbers.",
"_____no_output_____"
]
],
[
[
"primes(20)",
"_____no_output_____"
]
],
[
[
"Note that this is not the most efficient method to check whether $k$ is prime.",
"_____no_output_____"
]
],
[
[
"%timeit primes(1_000)",
"73.1 ms ± 8.12 ms per loop (mean ± std. dev. of 7 runs, 10 loops each)\n"
],
[
"p = array('i', [0]*10_000)\n%timeit primes(10_000, p)",
"7.65 s ± 993 ms per loop (mean ± std. dev. of 7 runs, 1 loop each)\n"
]
],
[
[
"## Cython",
"_____no_output_____"
],
[
"The cython implementation differs little from that in pure Python, type annotations have been added for the function's argument, and the variables `n`, `k`, `i`, and `p`. Note that cython expects a constant array size, hence the upper limit on `kmax`.",
"_____no_output_____"
]
],
[
[
"%%cython\ndef c_primes(int kmax):\n cdef int n, k, i\n cdef int p[10_000]\n if kmax > 10_000:\n kmax = 10_000\n result = []\n k, n = 0, 2\n while k < kmax:\n i = 0\n while i < k and n % p[i] != 0:\n i += 1\n if i == k:\n p[k] = n\n k += 1\n result.append(n)\n n += 1\n return result",
"_____no_output_____"
]
],
[
[
"Checking the results for the 20 first prime numbers.",
"_____no_output_____"
]
],
[
[
"c_primes(20)",
"_____no_output_____"
],
[
"%timeit c_primes(1_000)",
"1.84 ms ± 105 µs per loop (mean ± std. dev. of 7 runs, 100 loops each)\n"
],
[
"%timeit c_primes(10_000)",
"195 ms ± 15 ms per loop (mean ± std. dev. of 7 runs, 10 loops each)\n"
]
],
[
[
"It is clear that the cython implementation is more than 30 times faster than the pure Python implementation.",
"_____no_output_____"
],
[
"## Dynamic memory allocation",
"_____no_output_____"
],
[
"The cython implementation can be improved by adding dynamic memory allocation for the array `p`.",
"_____no_output_____"
]
],
[
[
"%%cython\nfrom libc.stdlib cimport calloc, free\n\ndef c_primes(int kmax):\n cdef int n, k, i\n cdef int *p = <int *> calloc(kmax, sizeof(int))\n result = []\n k, n = 0, 2\n while k < kmax:\n i = 0\n while i < k and n % p[i] != 0:\n i += 1\n if i == k:\n p[k] = n\n k += 1\n result.append(n)\n n += 1\n free(p)\n return result",
"_____no_output_____"
]
],
[
[
"Checking the results for the 20 first prime numbers.",
"_____no_output_____"
]
],
[
[
"c_primes(20)",
"_____no_output_____"
]
],
[
[
"This has no noticeable impact on performance.",
"_____no_output_____"
]
],
[
[
"%timeit c_primes(1_000)",
"2.29 ms ± 473 µs per loop (mean ± std. dev. of 7 runs, 100 loops each)\n"
],
[
"%timeit c_primes(10_000)",
"243 ms ± 32.7 ms per loop (mean ± std. dev. of 7 runs, 1 loop each)\n"
]
]
] | [
"markdown",
"code",
"markdown",
"code",
"markdown",
"code",
"markdown",
"code",
"markdown",
"code",
"markdown",
"code",
"markdown",
"code",
"markdown",
"code",
"markdown",
"code"
] | [
[
"markdown",
"markdown"
],
[
"code"
],
[
"markdown",
"markdown"
],
[
"code",
"code"
],
[
"markdown"
],
[
"code"
],
[
"markdown"
],
[
"code",
"code"
],
[
"markdown",
"markdown"
],
[
"code"
],
[
"markdown"
],
[
"code",
"code",
"code"
],
[
"markdown",
"markdown",
"markdown"
],
[
"code"
],
[
"markdown"
],
[
"code"
],
[
"markdown"
],
[
"code",
"code"
]
] |
Subsets and Splits
No community queries yet
The top public SQL queries from the community will appear here once available.