Spaces:
Running
Running
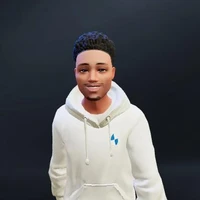
Integrate WhisperX for improved audio transcription and add real-time conversation support: update requirements to include WhisperX, refactor voice cloning to utilize WhisperX, implement WebSocket endpoints for real-time audio processing, and enhance audio transcription capabilities with alignment options.
be2a132
""" | |
Voice cloning module for CSM-1B TTS API. | |
This module provides functionality to clone voices from audio samples, | |
with advanced audio preprocessing and voice adaptation techniques. | |
""" | |
import os | |
import io | |
import time | |
import tempfile | |
import logging | |
import asyncio | |
import yt_dlp | |
# Replace standard whisper with WhisperX | |
# import whisper | |
import whisperx | |
from typing import Dict, List, Optional, Union, Tuple, BinaryIO | |
from pathlib import Path | |
import numpy as np | |
import torch | |
import torchaudio | |
from pydantic import BaseModel | |
from fastapi import UploadFile | |
from app.model import Segment | |
# Set up logging | |
logger = logging.getLogger(__name__) | |
# Directory for storing cloned voice data | |
CLONED_VOICES_DIR = "/app/cloned_voices" | |
os.makedirs(CLONED_VOICES_DIR, exist_ok=True) | |
# WhisperX model cache for performance | |
_whisperx_model = None | |
_whisperx_model_lock = asyncio.Lock() | |
class ClonedVoice(BaseModel): | |
"""Model representing a cloned voice.""" | |
id: str | |
name: str | |
created_at: float | |
speaker_id: int | |
description: Optional[str] = None | |
audio_duration: float | |
sample_count: int | |
class VoiceCloner: | |
"""Voice cloning utility for CSM-1B model.""" | |
def __init__(self, generator, device="cuda"): | |
"""Initialize the voice cloner with a generator instance.""" | |
self.generator = generator | |
self.device = device | |
self.sample_rate = generator.sample_rate | |
self.cloned_voices = self._load_existing_voices() | |
logger.info(f"Voice cloner initialized with {len(self.cloned_voices)} existing voices") | |
def _load_existing_voices(self) -> Dict[str, ClonedVoice]: | |
"""Load existing cloned voices from disk.""" | |
voices = {} | |
if not os.path.exists(CLONED_VOICES_DIR): | |
return voices | |
for voice_dir in os.listdir(CLONED_VOICES_DIR): | |
voice_path = os.path.join(CLONED_VOICES_DIR, voice_dir) | |
if not os.path.isdir(voice_path): | |
continue | |
info_path = os.path.join(voice_path, "info.json") | |
if os.path.exists(info_path): | |
try: | |
import json | |
with open(info_path, "r") as f: | |
voice_info = json.load(f) | |
voices[voice_dir] = ClonedVoice(**voice_info) | |
logger.info(f"Loaded cloned voice: {voice_dir}") | |
except Exception as e: | |
logger.error(f"Error loading voice {voice_dir}: {e}") | |
return voices | |
async def process_audio_file( | |
self, | |
file: Union[UploadFile, BinaryIO, str], | |
transcript: Optional[str] = None | |
) -> Tuple[torch.Tensor, Optional[str], float]: | |
""" | |
Process an audio file for voice cloning. | |
Args: | |
file: The audio file (UploadFile, file-like object, or path) | |
transcript: Optional transcript of the audio | |
Returns: | |
Tuple of (processed_audio, transcript, duration_seconds) | |
""" | |
temp_path = None | |
try: | |
# Handle different input types | |
if isinstance(file, str): | |
# It's a file path | |
audio_path = file | |
logger.info(f"Processing audio from file path: {audio_path}") | |
else: | |
# Create a temporary file | |
temp_fd, temp_path = tempfile.mkstemp(suffix=".wav") | |
os.close(temp_fd) # Close the file descriptor | |
if isinstance(file, UploadFile): | |
# It's a FastAPI UploadFile | |
logger.info("Processing audio from UploadFile") | |
contents = await file.read() | |
with open(temp_path, "wb") as f: | |
f.write(contents) | |
elif hasattr(file, 'read'): | |
# It's a file-like object - check if it's async | |
logger.info("Processing audio from file-like object") | |
if asyncio.iscoroutinefunction(file.read): | |
# It's an async read method | |
contents = await file.read() | |
else: | |
# It's a sync read method | |
contents = file.read() | |
with open(temp_path, "wb") as f: | |
f.write(contents) | |
else: | |
raise ValueError(f"Unsupported file type: {type(file)}") | |
audio_path = temp_path | |
logger.info(f"Saved uploaded audio to temporary file: {audio_path}") | |
# Load audio | |
logger.info(f"Loading audio from {audio_path}") | |
audio, sr = torchaudio.load(audio_path) | |
# Convert to mono if stereo | |
if audio.shape[0] > 1: | |
logger.info(f"Converting {audio.shape[0]} channels to mono") | |
audio = torch.mean(audio, dim=0, keepdim=True) | |
# Remove first dimension if it's 1 | |
if audio.shape[0] == 1: | |
audio = audio.squeeze(0) | |
# Resample if necessary | |
if sr != self.sample_rate: | |
logger.info(f"Resampling from {sr}Hz to {self.sample_rate}Hz") | |
audio = torchaudio.functional.resample( | |
audio, orig_freq=sr, new_freq=self.sample_rate | |
) | |
# Get audio duration | |
duration_seconds = len(audio) / self.sample_rate | |
# Process audio for better quality | |
logger.info(f"Preprocessing audio for quality enhancement") | |
processed_audio = self._preprocess_audio(audio) | |
processed_duration = len(processed_audio) / self.sample_rate | |
logger.info( | |
f"Processed audio: original duration={duration_seconds:.2f}s, " | |
f"processed duration={processed_duration:.2f}s" | |
) | |
return processed_audio, transcript, duration_seconds | |
except Exception as e: | |
logger.error(f"Error processing audio: {e}", exc_info=True) | |
raise RuntimeError(f"Failed to process audio file: {e}") | |
finally: | |
# Clean up temp file if we created one | |
if temp_path and os.path.exists(temp_path): | |
try: | |
os.unlink(temp_path) | |
logger.debug(f"Deleted temporary file {temp_path}") | |
except Exception as e: | |
logger.warning(f"Failed to delete temporary file {temp_path}: {e}") | |
def _preprocess_audio(self, audio: torch.Tensor) -> torch.Tensor: | |
""" | |
Preprocess audio for better voice cloning quality. | |
Args: | |
audio: Raw audio tensor | |
Returns: | |
Processed audio tensor | |
""" | |
# Normalize volume | |
if torch.max(torch.abs(audio)) > 0: | |
audio = audio / torch.max(torch.abs(audio)) | |
# Remove silence with dynamic threshold | |
audio = self._remove_silence(audio, threshold=0.02) # Slightly higher threshold to remove more noise | |
# Remove DC offset (very low frequency noise) | |
audio = audio - torch.mean(audio) | |
# Apply simple noise reduction | |
# This filters out very high frequencies that might contain noise | |
try: | |
audio_np = audio.cpu().numpy() | |
from scipy import signal | |
# Apply a bandpass filter to focus on speech frequencies (80Hz - 8000Hz) | |
sos = signal.butter(3, [80, 8000], 'bandpass', fs=self.sample_rate, output='sos') | |
filtered = signal.sosfilt(sos, audio_np) | |
# Normalize the filtered audio | |
filtered = filtered / (np.max(np.abs(filtered)) + 1e-8) | |
# Convert back to torch tensor | |
audio = torch.tensor(filtered, device=audio.device) | |
except Exception as e: | |
logger.warning(f"Advanced audio filtering failed, using basic processing: {e}") | |
# Ensure audio has correct amplitude | |
audio = audio * 0.9 # Slightly reduce volume to prevent clipping | |
return audio | |
def _remove_silence( | |
self, | |
audio: torch.Tensor, | |
threshold: float = 0.015, | |
min_silence_duration: float = 0.2 | |
) -> torch.Tensor: | |
""" | |
Remove silence from audio while preserving speech rhythm. | |
Args: | |
audio: Input audio tensor | |
threshold: Energy threshold for silence detection | |
min_silence_duration: Minimum silence duration in seconds | |
Returns: | |
Audio with silence removed | |
""" | |
# Convert to numpy for easier processing | |
audio_np = audio.cpu().numpy() | |
# Calculate energy | |
energy = np.abs(audio_np) | |
# Find regions above threshold (speech) | |
is_speech = energy > threshold | |
# Convert min_silence_duration to samples | |
min_silence_samples = int(min_silence_duration * self.sample_rate) | |
# Find speech segments | |
speech_segments = [] | |
in_speech = False | |
speech_start = 0 | |
for i in range(len(is_speech)): | |
if is_speech[i] and not in_speech: | |
# Start of speech segment | |
in_speech = True | |
speech_start = i | |
elif not is_speech[i] and in_speech: | |
# Potential end of speech segment | |
# Only end if silence is long enough | |
silence_count = 0 | |
for j in range(i, min(len(is_speech), i + min_silence_samples)): | |
if not is_speech[j]: | |
silence_count += 1 | |
else: | |
break | |
if silence_count >= min_silence_samples: | |
# End of speech segment | |
in_speech = False | |
speech_segments.append((speech_start, i)) | |
# Handle case where audio ends during speech | |
if in_speech: | |
speech_segments.append((speech_start, len(is_speech))) | |
# If no speech segments found, return original audio | |
if not speech_segments: | |
logger.warning("No speech segments detected, returning original audio") | |
return audio | |
# Add small buffer around segments | |
buffer_samples = int(0.05 * self.sample_rate) # 50ms buffer | |
processed_segments = [] | |
for start, end in speech_segments: | |
buffered_start = max(0, start - buffer_samples) | |
buffered_end = min(len(audio_np), end + buffer_samples) | |
processed_segments.append(audio_np[buffered_start:buffered_end]) | |
# Concatenate all segments with small pauses between them | |
small_pause = np.zeros(int(0.15 * self.sample_rate)) # 150ms pause | |
result = processed_segments[0] | |
for segment in processed_segments[1:]: | |
result = np.concatenate([result, small_pause, segment]) | |
return torch.tensor(result, device=audio.device) | |
def _enhance_speech(self, audio: torch.Tensor) -> torch.Tensor: | |
"""Enhance speech quality for better cloning results.""" | |
# This is a placeholder for more advanced speech enhancement | |
# In a production implementation, you could add: | |
# - Noise reduction | |
# - Equalization for speech frequencies | |
# - Gentle compression for better dynamics | |
return audio | |
async def clone_voice( | |
self, | |
audio_file: Union[UploadFile, BinaryIO, str], | |
voice_name: str, | |
transcript: Optional[str] = None, | |
description: Optional[str] = None, | |
speaker_id: Optional[int] = None # Make this optional | |
) -> ClonedVoice: | |
""" | |
Clone a voice from an audio file. | |
Args: | |
audio_file: Audio file with the voice to clone | |
voice_name: Name for the cloned voice | |
transcript: Transcript of the audio (optional) | |
description: Description of the voice (optional) | |
speaker_id: Speaker ID to use (default: auto-assigned) | |
Returns: | |
ClonedVoice object with voice information | |
""" | |
logger.info(f"Cloning new voice '{voice_name}' from audio file") | |
# Process the audio file | |
processed_audio, provided_transcript, duration = await self.process_audio_file( | |
audio_file, transcript | |
) | |
# Use a better speaker ID assignment - use a small number similar to the built-in voices | |
# This prevents issues with the speaker ID being interpreted as speech | |
if speaker_id is None: | |
# Use a number between 10-20 to avoid conflicts with built-in voices (0-5) | |
# but not too large like 999 which might cause issues | |
existing_ids = [v.speaker_id for v in self.cloned_voices.values()] | |
for potential_id in range(10, 20): | |
if potential_id not in existing_ids: | |
speaker_id = potential_id | |
break | |
else: | |
# If all IDs in range are taken, use a fallback | |
speaker_id = 10 | |
# Generate a unique ID for the voice | |
voice_id = f"{int(time.time())}_{voice_name.lower().replace(' ', '_')}" | |
# Create directory for the voice | |
voice_dir = os.path.join(CLONED_VOICES_DIR, voice_id) | |
os.makedirs(voice_dir, exist_ok=True) | |
# Save the processed audio | |
audio_path = os.path.join(voice_dir, "reference.wav") | |
torchaudio.save(audio_path, processed_audio.unsqueeze(0).cpu(), self.sample_rate) | |
# Save the transcript if provided | |
if provided_transcript: | |
transcript_path = os.path.join(voice_dir, "transcript.txt") | |
with open(transcript_path, "w") as f: | |
f.write(provided_transcript) | |
# Create and save voice info | |
voice_info = ClonedVoice( | |
id=voice_id, | |
name=voice_name, | |
created_at=time.time(), | |
speaker_id=speaker_id, | |
description=description, | |
audio_duration=duration, | |
sample_count=len(processed_audio) | |
) | |
# Save voice info as JSON | |
import json | |
with open(os.path.join(voice_dir, "info.json"), "w") as f: | |
f.write(json.dumps(voice_info.dict())) | |
# Add to cloned voices dictionary | |
self.cloned_voices[voice_id] = voice_info | |
logger.info(f"Voice '{voice_name}' cloned successfully with ID: {voice_id} and speaker_id: {speaker_id}") | |
return voice_info | |
def get_voice_context(self, voice_id: str) -> List[Segment]: | |
""" | |
Get context segments for a cloned voice. | |
Args: | |
voice_id: ID of the cloned voice | |
Returns: | |
List of context segments for the voice | |
""" | |
if voice_id not in self.cloned_voices: | |
logger.warning(f"Voice ID {voice_id} not found") | |
return [] | |
voice = self.cloned_voices[voice_id] | |
voice_dir = os.path.join(CLONED_VOICES_DIR, voice_id) | |
audio_path = os.path.join(voice_dir, "reference.wav") | |
if not os.path.exists(audio_path): | |
logger.error(f"Audio file for voice {voice_id} not found at {audio_path}") | |
return [] | |
try: | |
# Load the audio | |
audio, sr = torchaudio.load(audio_path) | |
audio = audio.squeeze(0) | |
# Resample if necessary | |
if sr != self.sample_rate: | |
audio = torchaudio.functional.resample( | |
audio, orig_freq=sr, new_freq=self.sample_rate | |
) | |
# Trim to a maximum of 5 seconds to avoid sequence length issues | |
# This is a balance between voice quality and model limitations | |
max_samples = 5 * self.sample_rate # 5 seconds | |
if audio.shape[0] > max_samples: | |
logger.info(f"Trimming voice sample from {audio.shape[0]} to {max_samples} samples") | |
# Take from beginning for better voice characteristics | |
audio = audio[:max_samples] | |
# Load transcript if available | |
transcript_path = os.path.join(voice_dir, "transcript.txt") | |
transcript = "" | |
if os.path.exists(transcript_path): | |
with open(transcript_path, "r") as f: | |
full_transcript = f.read() | |
# Take a portion of transcript that roughly matches our audio portion | |
words = full_transcript.split() | |
# Estimate 3 words per second as a rough average | |
word_count = min(len(words), int(5 * 3)) # 5 seconds * 3 words/second | |
transcript = " ".join(words[:word_count]) | |
else: | |
transcript = f"Voice sample for {voice.name}" | |
# Create context segment | |
segment = Segment( | |
text=transcript, | |
speaker=voice.speaker_id, | |
audio=audio.to(self.device) | |
) | |
logger.info(f"Created voice context segment with {audio.shape[0]/self.sample_rate:.1f}s audio") | |
return [segment] | |
except Exception as e: | |
logger.error(f"Error getting voice context for {voice_id}: {e}") | |
return [] | |
def list_voices(self) -> List[ClonedVoice]: | |
"""List all available cloned voices.""" | |
return list(self.cloned_voices.values()) | |
def delete_voice(self, voice_id: str) -> bool: | |
""" | |
Delete a cloned voice. | |
Args: | |
voice_id: ID of the voice to delete | |
Returns: | |
True if successful, False otherwise | |
""" | |
if voice_id not in self.cloned_voices: | |
return False | |
voice_dir = os.path.join(CLONED_VOICES_DIR, voice_id) | |
if os.path.exists(voice_dir): | |
try: | |
import shutil | |
shutil.rmtree(voice_dir) | |
del self.cloned_voices[voice_id] | |
return True | |
except Exception as e: | |
logger.error(f"Error deleting voice {voice_id}: {e}") | |
return False | |
return False | |
async def clone_voice_from_youtube( | |
self, # Don't forget the self parameter for class methods | |
youtube_url: str, | |
voice_name: str, | |
start_time: int = 0, | |
duration: int = 180, | |
description: str = None | |
) -> ClonedVoice: | |
""" | |
Clone a voice from a YouTube video. | |
Args: | |
youtube_url: URL of the YouTube video | |
voice_name: Name for the cloned voice | |
start_time: Start time in seconds | |
duration: Duration to extract in seconds | |
description: Optional description of the voice | |
Returns: | |
ClonedVoice object with voice information | |
""" | |
logger.info(f"Cloning voice '{voice_name}' from YouTube: {youtube_url}") | |
# Create temporary directory for processing | |
with tempfile.TemporaryDirectory() as temp_dir: | |
# Step 1: Download audio from YouTube | |
audio_path = await self._download_youtube_audio(youtube_url, temp_dir, start_time, duration) | |
# Step 2: Generate transcript using WhisperX | |
transcript = await self._generate_transcript(audio_path) | |
# Step 3: Clone the voice using the extracted audio and transcript | |
voice = await self.clone_voice( | |
audio_file=audio_path, | |
voice_name=voice_name, | |
transcript=transcript, | |
description=description or f"Voice cloned from YouTube: {youtube_url}" | |
) | |
return voice | |
async def _download_youtube_audio( | |
self, # Don't forget the self parameter | |
url: str, | |
output_dir: str, | |
start_time: int = 0, | |
duration: int = 180 | |
) -> str: | |
""" | |
Download audio from a YouTube video. | |
Args: | |
url: YouTube URL | |
output_dir: Directory to save the audio | |
start_time: Start time in seconds | |
duration: Duration to extract in seconds | |
Returns: | |
Path to the downloaded audio file | |
""" | |
output_path = os.path.join(output_dir, "youtube_audio.wav") | |
# Configure yt-dlp options | |
ydl_opts = { | |
'format': 'bestaudio/best', | |
'postprocessors': [{ | |
'key': 'FFmpegExtractAudio', | |
'preferredcodec': 'wav', | |
'preferredquality': '192', | |
}], | |
'outtmpl': output_path.replace(".wav", ""), | |
'quiet': True, | |
'no_warnings': True | |
} | |
# Download the video | |
with yt_dlp.YoutubeDL(ydl_opts) as ydl: | |
ydl.download([url]) | |
# Trim the audio to the specified segment | |
if start_time > 0 or duration < float('inf'): | |
import ffmpeg | |
trimmed_path = os.path.join(output_dir, "trimmed_audio.wav") | |
# Use ffmpeg to trim the audio | |
( | |
ffmpeg.input(output_path) | |
.audio | |
.filter('atrim', start=start_time, duration=duration) | |
.output(trimmed_path) | |
.run(quiet=True, overwrite_output=True) | |
) | |
return trimmed_path | |
return output_path | |
async def _generate_transcript(self, audio_path: str) -> str: | |
""" | |
Generate transcript from audio using WhisperX (faster than standard Whisper). | |
Args: | |
audio_path: Path to the audio file | |
Returns: | |
Transcript text | |
""" | |
global _whisperx_model | |
try: | |
# Use device with CUDA if available | |
device = "cuda" if torch.cuda.is_available() else "cpu" | |
# Use lock to ensure model loading is thread-safe | |
async with _whisperx_model_lock: | |
# Load WhisperX model if not already loaded | |
if _whisperx_model is None: | |
logger.info("Loading WhisperX model for transcription (one-time initialization)") | |
compute_type = "float16" if device == "cuda" else "float32" | |
_whisperx_model = whisperx.load_model( | |
"medium", # Can use "small" for faster processing, "medium" for better quality | |
device, | |
compute_type=compute_type, | |
asr_options={"beam_size": 5} | |
) | |
logger.info(f"WhisperX model loaded on {device}") | |
# Start processing timer | |
start_time = time.time() | |
# Process with WhisperX - much faster than standard whisper, | |
# especially for longer files | |
logger.info(f"Transcribing audio with WhisperX on {device}") | |
result = _whisperx_model.transcribe( | |
audio_path, | |
batch_size=16 if device == "cuda" else 1 # Larger batch size on GPU for faster processing | |
) | |
# Calculate and log processing time | |
processing_time = time.time() - start_time | |
logger.info(f"Transcription completed in {processing_time:.2f}s") | |
return result["text"] | |
except Exception as e: | |
logger.error(f"WhisperX transcription failed: {e}", exc_info=True) | |
# Fallback to conventional approach if WhisperX fails | |
logger.warning("Falling back to basic transcription method") | |
try: | |
model = whisperx.load_model("small", device, compute_type="float32") | |
result = model.transcribe(audio_path, batch_size=1) | |
return result["text"] | |
except Exception as fallback_error: | |
logger.error(f"Fallback transcription also failed: {fallback_error}") | |
return "Transcription failed. Please provide a transcript manually." | |
def generate_speech( | |
self, | |
text: str, | |
voice_id: str, | |
temperature: float = 0.65, | |
topk: int = 30, | |
max_audio_length_ms: int = 15000 | |
) -> torch.Tensor: | |
""" | |
Generate speech with a cloned voice. | |
Args: | |
text: Text to synthesize | |
voice_id: ID of the cloned voice to use | |
temperature: Sampling temperature (lower = more stable, higher = more varied) | |
topk: Top-k sampling parameter | |
max_audio_length_ms: Maximum audio length in milliseconds | |
Returns: | |
Generated audio tensor | |
""" | |
# Remove any async/await keywords - this is a synchronous function | |
if voice_id not in self.cloned_voices: | |
raise ValueError(f"Voice ID {voice_id} not found") | |
voice = self.cloned_voices[voice_id] | |
context = self.get_voice_context(voice_id) | |
if not context: | |
raise ValueError(f"Could not get context for voice {voice_id}") | |
# Preprocess text for better pronunciation | |
processed_text = self._preprocess_text(text) | |
logger.info(f"Generating speech with voice '{voice.name}' (ID: {voice_id}, speaker: {voice.speaker_id})") | |
try: | |
# Check if text is too long and should be split | |
if len(processed_text) > 200: | |
logger.info(f"Text is long ({len(processed_text)} chars), splitting for better quality") | |
from app.prompt_engineering import split_into_segments | |
# Split text into manageable segments | |
segments = split_into_segments(processed_text, max_chars=150) | |
logger.info(f"Split text into {len(segments)} segments") | |
all_audio_chunks = [] | |
# Process each segment | |
for i, segment_text in enumerate(segments): | |
logger.info(f"Generating segment {i+1}/{len(segments)}") | |
# Generate this segment - using plain text without formatting | |
segment_audio = self.generator.generate( | |
text=segment_text, # Use plain text, no formatting | |
speaker=voice.speaker_id, | |
context=context, | |
max_audio_length_ms=min(max_audio_length_ms, 10000), | |
temperature=temperature, | |
topk=topk, | |
) | |
all_audio_chunks.append(segment_audio) | |
# Use this segment as context for the next one for consistency | |
if i < len(segments) - 1: | |
context = [ | |
Segment( | |
text=segment_text, | |
speaker=voice.speaker_id, | |
audio=segment_audio | |
) | |
] | |
# Combine chunks with small silence between them | |
if len(all_audio_chunks) == 1: | |
audio = all_audio_chunks[0] | |
else: | |
silence_samples = int(0.1 * self.sample_rate) # 100ms silence | |
silence = torch.zeros(silence_samples, device=all_audio_chunks[0].device) | |
# Join segments with silence | |
audio_parts = [] | |
for i, chunk in enumerate(all_audio_chunks): | |
audio_parts.append(chunk) | |
if i < len(all_audio_chunks) - 1: # Don't add silence after the last chunk | |
audio_parts.append(silence) | |
# Concatenate all parts | |
audio = torch.cat(audio_parts) | |
return audio | |
else: | |
# For short text, generate directly - using plain text without formatting | |
audio = self.generator.generate( | |
text=processed_text, # Use plain text, no formatting | |
speaker=voice.speaker_id, | |
context=context, | |
max_audio_length_ms=max_audio_length_ms, | |
temperature=temperature, | |
topk=topk, | |
) | |
return audio | |
except Exception as e: | |
logger.error(f"Error generating speech with voice {voice_id}: {e}") | |
raise | |
def _preprocess_text(self, text: str) -> str: | |
"""Preprocess text for better pronunciation and voice cloning.""" | |
# Make sure text ends with punctuation for better phrasing | |
text = text.strip() | |
if not text.endswith(('.', '?', '!', ';')): | |
text = text + '.' | |
return text | |