sha
stringlengths 40
40
| text
stringlengths 1
13.4M
| id
stringlengths 2
117
| tags
sequencelengths 1
7.91k
| created_at
stringlengths 25
25
| metadata
stringlengths 2
875k
| last_modified
stringlengths 25
25
| arxiv
sequencelengths 0
25
| languages
sequencelengths 0
7.91k
| tags_str
stringlengths 17
159k
| text_str
stringlengths 1
447k
| text_lists
sequencelengths 0
352
| processed_texts
sequencelengths 1
353
|
---|---|---|---|---|---|---|---|---|---|---|---|---|
f8b94a675924121f7d3785ebbf46c198187dc6ae | # Dataset Card for "MULTI_VALUE_MNLI_that_resultative_past_participle"
[More Information needed](https://github.com/huggingface/datasets/blob/main/CONTRIBUTING.md#how-to-contribute-to-the-dataset-cards) | liuyanchen1015/MULTI_VALUE_mnli_that_resultative_past_participle | [
"region:us"
] | 2022-12-15T12:12:31+00:00 | {"dataset_info": {"features": [{"name": "premise", "dtype": "string"}, {"name": "hypothesis", "dtype": "string"}, {"name": "label", "dtype": "int64"}, {"name": "idx", "dtype": "int64"}, {"name": "score", "dtype": "int64"}], "splits": [{"name": "train", "num_bytes": 82248, "num_examples": 348}, {"name": "dev_matched", "num_bytes": 2264, "num_examples": 9}, {"name": "dev_mismatched", "num_bytes": 3578, "num_examples": 18}, {"name": "test_matched", "num_bytes": 773, "num_examples": 3}, {"name": "test_mismatched", "num_bytes": 4288, "num_examples": 21}], "download_size": 67869, "dataset_size": 93151}} | 2022-12-15T12:12:51+00:00 | [] | [] | TAGS
#region-us
| # Dataset Card for "MULTI_VALUE_MNLI_that_resultative_past_participle"
More Information needed | [
"# Dataset Card for \"MULTI_VALUE_MNLI_that_resultative_past_participle\"\n\nMore Information needed"
] | [
"TAGS\n#region-us \n",
"# Dataset Card for \"MULTI_VALUE_MNLI_that_resultative_past_participle\"\n\nMore Information needed"
] |
2263f4a1af106cba10d01d39e88c0ca2305b6f25 | # Dataset Card for "text_recognition_en_zh_clean"
[More Information needed](https://github.com/huggingface/datasets/blob/main/CONTRIBUTING.md#how-to-contribute-to-the-dataset-cards) | priyank-m/text_recognition_en_zh_clean | [
"region:us"
] | 2022-12-15T12:22:22+00:00 | {"dataset_info": {"features": [{"name": "image", "dtype": "image"}, {"name": "text", "dtype": "string"}], "splits": [{"name": "val", "num_bytes": 53886975.51, "num_examples": 2910}, {"name": "test", "num_bytes": 55192498.476, "num_examples": 2894}, {"name": "train", "num_bytes": 26744379885.02228, "num_examples": 1396731}], "download_size": 26975033720, "dataset_size": 26853459359.00828}} | 2022-12-16T18:05:44+00:00 | [] | [] | TAGS
#region-us
| # Dataset Card for "text_recognition_en_zh_clean"
More Information needed | [
"# Dataset Card for \"text_recognition_en_zh_clean\"\n\nMore Information needed"
] | [
"TAGS\n#region-us \n",
"# Dataset Card for \"text_recognition_en_zh_clean\"\n\nMore Information needed"
] |
82793ac62e2e86dd2e4ffce8a0a63b87408c47a3 | # Dataset Card for "preprocessed_jsut_jsss_css10"
[More Information needed](https://github.com/huggingface/datasets/blob/main/CONTRIBUTING.md#how-to-contribute-to-the-dataset-cards) | vumichien/preprocessed_jsut_jsss_css10 | [
"region:us"
] | 2022-12-15T13:03:03+00:00 | {"dataset_info": {"features": [{"name": "audio", "struct": [{"name": "array", "sequence": "float32"}, {"name": "path", "dtype": "string"}, {"name": "sampling_rate", "dtype": "int64"}]}, {"name": "sentence", "dtype": "string"}], "splits": [{"name": "train", "num_bytes": 7003135912, "num_examples": 18160}], "download_size": 7021090523, "dataset_size": 7003135912}} | 2022-12-15T13:06:13+00:00 | [] | [] | TAGS
#region-us
| # Dataset Card for "preprocessed_jsut_jsss_css10"
More Information needed | [
"# Dataset Card for \"preprocessed_jsut_jsss_css10\"\n\nMore Information needed"
] | [
"TAGS\n#region-us \n",
"# Dataset Card for \"preprocessed_jsut_jsss_css10\"\n\nMore Information needed"
] |
4ad3df7317bd71f9da11dee39898120bcb95ed86 | # Dataset Card for "preprocessed_jsut_jsss_css10_common_voice_11"
[More Information needed](https://github.com/huggingface/datasets/blob/main/CONTRIBUTING.md#how-to-contribute-to-the-dataset-cards) | vumichien/preprocessed_jsut_jsss_css10_common_voice_11 | [
"region:us"
] | 2022-12-15T13:10:37+00:00 | {"dataset_info": {"features": [{"name": "audio", "struct": [{"name": "array", "sequence": "float32"}, {"name": "path", "dtype": "string"}, {"name": "sampling_rate", "dtype": "int64"}]}, {"name": "sentence", "dtype": "string"}], "splits": [{"name": "train", "num_bytes": 10432449169, "num_examples": 29150}, {"name": "test", "num_bytes": 1562198132, "num_examples": 4604}], "download_size": 12008358604, "dataset_size": 11994647301}} | 2022-12-15T13:17:29+00:00 | [] | [] | TAGS
#region-us
| # Dataset Card for "preprocessed_jsut_jsss_css10_common_voice_11"
More Information needed | [
"# Dataset Card for \"preprocessed_jsut_jsss_css10_common_voice_11\"\n\nMore Information needed"
] | [
"TAGS\n#region-us \n",
"# Dataset Card for \"preprocessed_jsut_jsss_css10_common_voice_11\"\n\nMore Information needed"
] |
91ccf68006210c5b6d3811bb2800ed0f26f2de81 | # Dataset Card for "clinic-work"
[More Information needed](https://github.com/huggingface/datasets/blob/main/CONTRIBUTING.md#how-to-contribute-to-the-dataset-cards) | fathyshalab/clinic-work | [
"region:us"
] | 2022-12-15T13:25:12+00:00 | {"dataset_info": {"features": [{"name": "Unnamed: 0", "dtype": "int64"}, {"name": "text", "dtype": "string"}, {"name": "label", "dtype": "int64"}, {"name": "label_text", "dtype": "string"}], "splits": [{"name": "train", "num_bytes": 39848.2, "num_examples": 525}, {"name": "test", "num_bytes": 17077.8, "num_examples": 225}], "download_size": 0, "dataset_size": 56926.0}} | 2022-12-24T05:55:25+00:00 | [] | [] | TAGS
#region-us
| # Dataset Card for "clinic-work"
More Information needed | [
"# Dataset Card for \"clinic-work\"\n\nMore Information needed"
] | [
"TAGS\n#region-us \n",
"# Dataset Card for \"clinic-work\"\n\nMore Information needed"
] |
2b2254931a8229c449f08bf937d4405b2a3c4b79 | # Dataset Card for "yannic-kilcher-transcript-audio"
## Table of Contents
- [Table of Contents](#table-of-contents)
- [Dataset Description](#dataset-description)
- [Dataset Summary](#dataset-summary)
- [Languages](#languages)
- [Dataset Structure](#dataset-structure)
- [Data Instances](#data-instances)
- [Data Fields](#data-fields)
- [Data Splits](#data-splits)
- [Dataset Creation](#dataset-creation)
- [Curation Rationale](#curation-rationale)
- [Source Data](#source-data)
- [Annotations](#annotations)
- [Personal and Sensitive Information](#personal-and-sensitive-information)
- [Considerations for Using the Data](#considerations-for-using-the-data)
- [Social Impact of Dataset](#social-impact-of-dataset)
- [Discussion of Biases](#discussion-of-biases)
- [Other Known Limitations](#other-known-limitations)
- [Additional Information](#additional-information)
- [Contributions](#contributions)
## Dataset Description
- **Homepage:** [Whispering-GPT](https://github.com/matallanas/whisper_gpt_pipeline)
- **Repository:** [whisper_gpt_pipeline](https://github.com/matallanas/whisper_gpt_pipeline)
- **Paper:** [whisper](https://cdn.openai.com/papers/whisper.pdf) and [gpt](https://s3-us-west-2.amazonaws.com/openai-assets/research-covers/language-unsupervised/language_understanding_paper.pdf)
- **Point of Contact:** [Whispering-GPT organization](https://huggingface.co/Whispering-GPT)
### Dataset Summary
This dataset is created by applying whisper to the videos of the Youtube channel [Yannic Kilcher](https://www.youtube.com/yannickilcher). The dataset was created a medium size whisper model.
### Languages
- **Language**: English
## Dataset Structure
The dataset contains all the transcripts plus the audio of the different videos of Yannic Kilcher.
### Data Fields
The dataset is composed by:
- **id**: Id of the youtube video.
- **channel**: Name of the channel.
- **channel\_id**: Id of the youtube channel.
- **title**: Title given to the video.
- **categories**: Category of the video.
- **description**: Description added by the author.
- **text**: Whole transcript of the video.
- **segments**: A list with the time and transcription of the video.
- **start**: When started the trancription.
- **end**: When the transcription ends.
- **text**: The text of the transcription.
- **audio**: the extracted audio of the video in ogg format.
### Data Splits
- Train split.
## Dataset Creation
### Source Data
The transcriptions are from the videos of [Yannic Kilcher](https://www.youtube.com/yannickilcher)
### Contributions
Thanks to [Whispering-GPT](https://huggingface.co/Whispering-GPT) organization for adding this dataset. | Whispering-GPT/yannick-kilcher-transcript-audio | [
"task_categories:automatic-speech-recognition",
"whisper",
"whispering",
"medium",
"region:us"
] | 2022-12-15T13:25:43+00:00 | {"task_categories": ["automatic-speech-recognition"], "dataset_info": {"features": [{"name": "id", "dtype": "string"}, {"name": "channel", "dtype": "string"}, {"name": "channel_id", "dtype": "string"}, {"name": "title", "dtype": "string"}, {"name": "categories", "sequence": "string"}, {"name": "tags", "sequence": "string"}, {"name": "description", "dtype": "string"}, {"name": "text", "dtype": "string"}, {"name": "segments", "list": [{"name": "start", "dtype": "float64"}, {"name": "end", "dtype": "float64"}, {"name": "text", "dtype": "string"}]}, {"name": "audio", "dtype": "audio"}], "splits": [{"name": "train", "num_bytes": 15013848071.0, "num_examples": 370}], "download_size": 15003651933, "dataset_size": 15013848071.0}, "tags": ["whisper", "whispering", "medium"]} | 2022-12-18T17:46:15+00:00 | [] | [] | TAGS
#task_categories-automatic-speech-recognition #whisper #whispering #medium #region-us
| # Dataset Card for "yannic-kilcher-transcript-audio"
## Table of Contents
- Table of Contents
- Dataset Description
- Dataset Summary
- Languages
- Dataset Structure
- Data Instances
- Data Fields
- Data Splits
- Dataset Creation
- Curation Rationale
- Source Data
- Annotations
- Personal and Sensitive Information
- Considerations for Using the Data
- Social Impact of Dataset
- Discussion of Biases
- Other Known Limitations
- Additional Information
- Contributions
## Dataset Description
- Homepage: Whispering-GPT
- Repository: whisper_gpt_pipeline
- Paper: whisper and gpt
- Point of Contact: Whispering-GPT organization
### Dataset Summary
This dataset is created by applying whisper to the videos of the Youtube channel Yannic Kilcher. The dataset was created a medium size whisper model.
### Languages
- Language: English
## Dataset Structure
The dataset contains all the transcripts plus the audio of the different videos of Yannic Kilcher.
### Data Fields
The dataset is composed by:
- id: Id of the youtube video.
- channel: Name of the channel.
- channel\_id: Id of the youtube channel.
- title: Title given to the video.
- categories: Category of the video.
- description: Description added by the author.
- text: Whole transcript of the video.
- segments: A list with the time and transcription of the video.
- start: When started the trancription.
- end: When the transcription ends.
- text: The text of the transcription.
- audio: the extracted audio of the video in ogg format.
### Data Splits
- Train split.
## Dataset Creation
### Source Data
The transcriptions are from the videos of Yannic Kilcher
### Contributions
Thanks to Whispering-GPT organization for adding this dataset. | [
"# Dataset Card for \"yannic-kilcher-transcript-audio\"",
"## Table of Contents\n- Table of Contents\n- Dataset Description\n - Dataset Summary\n - Languages\n- Dataset Structure\n - Data Instances\n - Data Fields\n - Data Splits\n- Dataset Creation\n - Curation Rationale\n - Source Data\n - Annotations\n - Personal and Sensitive Information\n- Considerations for Using the Data\n - Social Impact of Dataset\n - Discussion of Biases\n - Other Known Limitations\n- Additional Information\n - Contributions",
"## Dataset Description\n\n- Homepage: Whispering-GPT\n- Repository: whisper_gpt_pipeline\n- Paper: whisper and gpt\n- Point of Contact: Whispering-GPT organization",
"### Dataset Summary\n\nThis dataset is created by applying whisper to the videos of the Youtube channel Yannic Kilcher. The dataset was created a medium size whisper model.",
"### Languages\n\n- Language: English",
"## Dataset Structure\n\nThe dataset contains all the transcripts plus the audio of the different videos of Yannic Kilcher.",
"### Data Fields\n\nThe dataset is composed by:\n- id: Id of the youtube video.\n- channel: Name of the channel.\n- channel\\_id: Id of the youtube channel.\n- title: Title given to the video.\n- categories: Category of the video.\n- description: Description added by the author.\n- text: Whole transcript of the video.\n- segments: A list with the time and transcription of the video.\n - start: When started the trancription.\n - end: When the transcription ends.\n - text: The text of the transcription.\n- audio: the extracted audio of the video in ogg format.",
"### Data Splits\n\n- Train split.",
"## Dataset Creation",
"### Source Data\n\nThe transcriptions are from the videos of Yannic Kilcher",
"### Contributions\n\nThanks to Whispering-GPT organization for adding this dataset."
] | [
"TAGS\n#task_categories-automatic-speech-recognition #whisper #whispering #medium #region-us \n",
"# Dataset Card for \"yannic-kilcher-transcript-audio\"",
"## Table of Contents\n- Table of Contents\n- Dataset Description\n - Dataset Summary\n - Languages\n- Dataset Structure\n - Data Instances\n - Data Fields\n - Data Splits\n- Dataset Creation\n - Curation Rationale\n - Source Data\n - Annotations\n - Personal and Sensitive Information\n- Considerations for Using the Data\n - Social Impact of Dataset\n - Discussion of Biases\n - Other Known Limitations\n- Additional Information\n - Contributions",
"## Dataset Description\n\n- Homepage: Whispering-GPT\n- Repository: whisper_gpt_pipeline\n- Paper: whisper and gpt\n- Point of Contact: Whispering-GPT organization",
"### Dataset Summary\n\nThis dataset is created by applying whisper to the videos of the Youtube channel Yannic Kilcher. The dataset was created a medium size whisper model.",
"### Languages\n\n- Language: English",
"## Dataset Structure\n\nThe dataset contains all the transcripts plus the audio of the different videos of Yannic Kilcher.",
"### Data Fields\n\nThe dataset is composed by:\n- id: Id of the youtube video.\n- channel: Name of the channel.\n- channel\\_id: Id of the youtube channel.\n- title: Title given to the video.\n- categories: Category of the video.\n- description: Description added by the author.\n- text: Whole transcript of the video.\n- segments: A list with the time and transcription of the video.\n - start: When started the trancription.\n - end: When the transcription ends.\n - text: The text of the transcription.\n- audio: the extracted audio of the video in ogg format.",
"### Data Splits\n\n- Train split.",
"## Dataset Creation",
"### Source Data\n\nThe transcriptions are from the videos of Yannic Kilcher",
"### Contributions\n\nThanks to Whispering-GPT organization for adding this dataset."
] |
60512e89e68841b6b5ed1be59caf97b169f0d27a |
# Dataset Card for [Dataset Name]
## Table of Contents
- [Table of Contents](#table-of-contents)
- [Dataset Description](#dataset-description)
- [Dataset Summary](#dataset-summary)
- [Supported Tasks and Leaderboards](#supported-tasks-and-leaderboards)
- [Languages](#languages)
- [Dataset Structure](#dataset-structure)
- [Data Instances](#data-instances)
- [Data Fields](#data-fields)
- [Data Splits](#data-splits)
- [Dataset Creation](#dataset-creation)
- [Curation Rationale](#curation-rationale)
- [Source Data](#source-data)
- [Annotations](#annotations)
- [Personal and Sensitive Information](#personal-and-sensitive-information)
- [Considerations for Using the Data](#considerations-for-using-the-data)
- [Social Impact of Dataset](#social-impact-of-dataset)
- [Discussion of Biases](#discussion-of-biases)
- [Other Known Limitations](#other-known-limitations)
- [Additional Information](#additional-information)
- [Dataset Curators](#dataset-curators)
- [Licensing Information](#licensing-information)
- [Citation Information](#citation-information)
- [Contributions](#contributions)
## Dataset Description
- **Homepage:** [homepage](https://github.com/masakhane-io/masakhane-ner)
- **Repository:** [github](https://github.com/masakhane-io/masakhane-ner)
- **Paper:** [paper](https://arxiv.org/abs/2103.11811)
- **Point of Contact:** [Masakhane](https://www.masakhane.io/) or [email protected]
### Dataset Summary
MasakhaNER 2.0 is the largest publicly available high-quality dataset for named entity recognition (NER) in 20 African languages created by the Masakhane community.
Named entities are phrases that contain the names of persons, organizations, locations, times and quantities. Example:
[PER Wolff] , currently a journalist in [LOC Argentina] , played with [PER Del Bosque] in the final years of the seventies in [ORG Real Madrid] .
MasakhaNER 2.0 is a named entity dataset consisting of PER, ORG, LOC, and DATE entities annotated by Masakhane for 20 African languages
The train/validation/test sets are available for all the 20 languages.
For more details see https://arxiv.org/abs/2210.12391
### Supported Tasks and Leaderboards
[More Information Needed]
- `named-entity-recognition`: The performance in this task is measured with [F1](https://huggingface.co/metrics/f1) (higher is better). A named entity is correct only if it is an exact match of the corresponding entity in the data.
### Languages
There are 20 languages available :
- Bambara (bam)
- Ghomala (bbj)
- Ewe (ewe)
- Fon (fon)
- Hausa (hau)
- Igbo (ibo)
- Kinyarwanda (kin)
- Luganda (lug)
- Dholuo (luo)
- Mossi (mos)
- Chichewa (nya)
- Nigerian Pidgin
- chShona (sna)
- Kiswahili (swą)
- Setswana (tsn)
- Twi (twi)
- Wolof (wol)
- isiXhosa (xho)
- Yorùbá (yor)
- isiZulu (zul)
## Dataset Structure
### Data Instances
The examples look like this for Yorùbá:
```
from datasets import load_dataset
data = load_dataset('masakhane/masakhaner2', 'yor')
# Please, specify the language code
# A data point consists of sentences seperated by empty line and tab-seperated tokens and tags.
{'id': '0',
'ner_tags': [B-DATE, I-DATE, 0, 0, 0, 0, 0, B-PER, I-PER, I-PER, O, O, O, O],
'tokens': ['Wákàtí', 'méje', 'ti', 'ré', 'kọjá', 'lọ', 'tí', 'Luis', 'Carlos', 'Díaz', 'ti', 'di', 'awati', '.']
}
```
### Data Fields
- `id`: id of the sample
- `tokens`: the tokens of the example text
- `ner_tags`: the NER tags of each token
The NER tags correspond to this list:
```
"O", "B-PER", "I-PER", "B-ORG", "I-ORG", "B-LOC", "I-LOC", "B-DATE", "I-DATE",
```
In the NER tags, a B denotes the first item of a phrase and an I any non-initial word. There are four types of phrases: person names (PER), organizations (ORG), locations (LOC) and dates & time (DATE).
It is assumed that named entities are non-recursive and non-overlapping. In case a named entity is embedded in another named entity usually, only the top level entity is marked.
### Data Splits
For all languages, there are three splits.
The original splits were named `train`, `dev` and `test` and they correspond to the `train`, `validation` and `test` splits.
The splits have the following sizes :
| Language | train | validation | test |
|-----------------|------:|-----------:|------:|
| Bambara | 4463 | 638 | 1274 |
| Ghomala | 3384 | 483 | 966 |
| Ewe | 3505 | 501 | 1001 |
| Fon. | 4343 | 621 | 1240 |
| Hausa | 5716 | 816 | 1633 |
| Igbo | 7634 | 1090 | 2181 |
| Kinyarwanda | 7825 | 1118 | 2235 |
| Luganda | 4942 | 706 | 1412 |
| Luo | 5161 | 737 | 1474 |
| Mossi | 4532 | 648 | 1613 |
| Nigerian-Pidgin | 5646 | 806 | 1294 |
| Chichewa | 6250 | 893 | 1785 |
| chiShona | 6207 | 887 | 1773 |
| Kiswahili | 6593 | 942 | 1883 |
| Setswana | 3289 | 499 | 996 |
| Akan/Twi | 4240 | 605 | 1211 |
| Wolof | 4593 | 656 | 1312 |
| isiXhosa | 5718 | 817 | 1633 |
| Yoruba | 6877 | 983 | 1964 |
| isiZulu | 5848 | 836 | 1670 |
## Dataset Creation
### Curation Rationale
The dataset was introduced to introduce new resources to 20 languages that were under-served for natural language processing.
[More Information Needed]
### Source Data
The source of the data is from the news domain, details can be found here https://arxiv.org/abs/2210.12391
#### Initial Data Collection and Normalization
The articles were word-tokenized, information on the exact pre-processing pipeline is unavailable.
#### Who are the source language producers?
The source language was produced by journalists and writers employed by the news agency and newspaper mentioned above.
### Annotations
#### Annotation process
Details can be found here https://arxiv.org/abs/2103.11811
#### Who are the annotators?
Annotators were recruited from [Masakhane](https://www.masakhane.io/)
### Personal and Sensitive Information
The data is sourced from newspaper source and only contains mentions of public figures or individuals
## Considerations for Using the Data
### Social Impact of Dataset
[More Information Needed]
### Discussion of Biases
[More Information Needed]
### Other Known Limitations
Users should keep in mind that the dataset only contains news text, which might limit the applicability of the developed systems to other domains.
## Additional Information
### Dataset Curators
### Licensing Information
The licensing status of the data is CC 4.0 Non-Commercial
### Citation Information
Provide the [BibTex](http://www.bibtex.org/)-formatted reference for the dataset. For example:
```
@article{Adelani2022MasakhaNER2A,
title={MasakhaNER 2.0: Africa-centric Transfer Learning for Named Entity Recognition},
author={David Ifeoluwa Adelani and Graham Neubig and Sebastian Ruder and Shruti Rijhwani and Michael Beukman and Chester Palen-Michel and Constantine Lignos and Jesujoba Oluwadara Alabi and Shamsuddeen Hassan Muhammad and Peter Nabende and Cheikh M. Bamba Dione and Andiswa Bukula and Rooweither Mabuya and Bonaventure F. P. Dossou and Blessing K. Sibanda and Happy Buzaaba and Jonathan Mukiibi and Godson Kalipe and Derguene Mbaye and Amelia Taylor and Fatoumata Kabore and Chris C. Emezue and Anuoluwapo Aremu and Perez Ogayo and Catherine W. Gitau and Edwin Munkoh-Buabeng and Victoire Memdjokam Koagne and Allahsera Auguste Tapo and Tebogo Macucwa and Vukosi Marivate and Elvis Mboning and Tajuddeen R. Gwadabe and Tosin P. Adewumi and Orevaoghene Ahia and Joyce Nakatumba-Nabende and Neo L. Mokono and Ignatius M Ezeani and Chiamaka Ijeoma Chukwuneke and Mofetoluwa Adeyemi and Gilles Hacheme and Idris Abdulmumin and Odunayo Ogundepo and Oreen Yousuf and Tatiana Moteu Ngoli and Dietrich Klakow},
journal={ArXiv},
year={2022},
volume={abs/2210.12391}
}
```
### Contributions
Thanks to [@dadelani](https://github.com/dadelani) for adding this dataset. | masakhane/masakhaner2 | [
"task_categories:token-classification",
"task_ids:named-entity-recognition",
"annotations_creators:expert-generated",
"language_creators:expert-generated",
"multilinguality:multilingual",
"size_categories:1K<n<10K",
"source_datasets:original",
"language:bm",
"language:bbj",
"language:ee",
"language:fon",
"language:ha",
"language:ig",
"language:rw",
"language:lg",
"language:luo",
"language:mos",
"language:ny",
"language:pcm",
"language:sn",
"language:sw",
"language:tn",
"language:tw",
"language:wo",
"language:xh",
"language:yo",
"language:zu",
"license:afl-3.0",
"ner",
"masakhaner",
"masakhane",
"arxiv:2103.11811",
"arxiv:2210.12391",
"region:us"
] | 2022-12-15T13:28:09+00:00 | {"annotations_creators": ["expert-generated"], "language_creators": ["expert-generated"], "language": ["bm", "bbj", "ee", "fon", "ha", "ig", "rw", "lg", "luo", "mos", "ny", "pcm", "sn", "sw", "tn", "tw", "wo", "xh", "yo", "zu"], "license": ["afl-3.0"], "multilinguality": ["multilingual"], "size_categories": ["1K<n<10K"], "source_datasets": ["original"], "task_categories": ["token-classification"], "task_ids": ["named-entity-recognition"], "pretty_name": "masakhaner2.0", "tags": ["ner", "masakhaner", "masakhane"]} | 2023-09-11T17:00:07+00:00 | [
"2103.11811",
"2210.12391"
] | [
"bm",
"bbj",
"ee",
"fon",
"ha",
"ig",
"rw",
"lg",
"luo",
"mos",
"ny",
"pcm",
"sn",
"sw",
"tn",
"tw",
"wo",
"xh",
"yo",
"zu"
] | TAGS
#task_categories-token-classification #task_ids-named-entity-recognition #annotations_creators-expert-generated #language_creators-expert-generated #multilinguality-multilingual #size_categories-1K<n<10K #source_datasets-original #language-Bambara #language-Ghomálá' #language-Ewe #language-Fon #language-Hausa #language-Igbo #language-Kinyarwanda #language-Ganda #language-Luo (Kenya and Tanzania) #language-Mossi #language-Nyanja #language-Nigerian Pidgin #language-Shona #language-Swahili (macrolanguage) #language-Tswana #language-Twi #language-Wolof #language-Xhosa #language-Yoruba #language-Zulu #license-afl-3.0 #ner #masakhaner #masakhane #arxiv-2103.11811 #arxiv-2210.12391 #region-us
| Dataset Card for [Dataset Name]
===============================
Table of Contents
-----------------
* Table of Contents
* Dataset Description
+ Dataset Summary
+ Supported Tasks and Leaderboards
+ Languages
* Dataset Structure
+ Data Instances
+ Data Fields
+ Data Splits
* Dataset Creation
+ Curation Rationale
+ Source Data
+ Annotations
+ Personal and Sensitive Information
* Considerations for Using the Data
+ Social Impact of Dataset
+ Discussion of Biases
+ Other Known Limitations
* Additional Information
+ Dataset Curators
+ Licensing Information
+ Citation Information
+ Contributions
Dataset Description
-------------------
* Homepage: homepage
* Repository: github
* Paper: paper
* Point of Contact: Masakhane or didelani@URL
### Dataset Summary
MasakhaNER 2.0 is the largest publicly available high-quality dataset for named entity recognition (NER) in 20 African languages created by the Masakhane community.
Named entities are phrases that contain the names of persons, organizations, locations, times and quantities. Example:
[PER Wolff] , currently a journalist in [LOC Argentina] , played with [PER Del Bosque] in the final years of the seventies in [ORG Real Madrid] .
MasakhaNER 2.0 is a named entity dataset consisting of PER, ORG, LOC, and DATE entities annotated by Masakhane for 20 African languages
The train/validation/test sets are available for all the 20 languages.
For more details see URL
### Supported Tasks and Leaderboards
* 'named-entity-recognition': The performance in this task is measured with F1 (higher is better). A named entity is correct only if it is an exact match of the corresponding entity in the data.
### Languages
There are 20 languages available :
* Bambara (bam)
* Ghomala (bbj)
* Ewe (ewe)
* Fon (fon)
* Hausa (hau)
* Igbo (ibo)
* Kinyarwanda (kin)
* Luganda (lug)
* Dholuo (luo)
* Mossi (mos)
* Chichewa (nya)
* Nigerian Pidgin
* chShona (sna)
* Kiswahili (swą)
* Setswana (tsn)
* Twi (twi)
* Wolof (wol)
* isiXhosa (xho)
* Yorùbá (yor)
* isiZulu (zul)
Dataset Structure
-----------------
### Data Instances
The examples look like this for Yorùbá:
### Data Fields
* 'id': id of the sample
* 'tokens': the tokens of the example text
* 'ner\_tags': the NER tags of each token
The NER tags correspond to this list:
In the NER tags, a B denotes the first item of a phrase and an I any non-initial word. There are four types of phrases: person names (PER), organizations (ORG), locations (LOC) and dates & time (DATE).
It is assumed that named entities are non-recursive and non-overlapping. In case a named entity is embedded in another named entity usually, only the top level entity is marked.
### Data Splits
For all languages, there are three splits.
The original splits were named 'train', 'dev' and 'test' and they correspond to the 'train', 'validation' and 'test' splits.
The splits have the following sizes :
Dataset Creation
----------------
### Curation Rationale
The dataset was introduced to introduce new resources to 20 languages that were under-served for natural language processing.
### Source Data
The source of the data is from the news domain, details can be found here URL
#### Initial Data Collection and Normalization
The articles were word-tokenized, information on the exact pre-processing pipeline is unavailable.
#### Who are the source language producers?
The source language was produced by journalists and writers employed by the news agency and newspaper mentioned above.
### Annotations
#### Annotation process
Details can be found here URL
#### Who are the annotators?
Annotators were recruited from Masakhane
### Personal and Sensitive Information
The data is sourced from newspaper source and only contains mentions of public figures or individuals
Considerations for Using the Data
---------------------------------
### Social Impact of Dataset
### Discussion of Biases
### Other Known Limitations
Users should keep in mind that the dataset only contains news text, which might limit the applicability of the developed systems to other domains.
Additional Information
----------------------
### Dataset Curators
### Licensing Information
The licensing status of the data is CC 4.0 Non-Commercial
Provide the BibTex-formatted reference for the dataset. For example:
### Contributions
Thanks to @dadelani for adding this dataset.
| [
"### Dataset Summary\n\n\nMasakhaNER 2.0 is the largest publicly available high-quality dataset for named entity recognition (NER) in 20 African languages created by the Masakhane community.\n\n\nNamed entities are phrases that contain the names of persons, organizations, locations, times and quantities. Example:\n\n\n[PER Wolff] , currently a journalist in [LOC Argentina] , played with [PER Del Bosque] in the final years of the seventies in [ORG Real Madrid] .\n\n\nMasakhaNER 2.0 is a named entity dataset consisting of PER, ORG, LOC, and DATE entities annotated by Masakhane for 20 African languages\n\n\nThe train/validation/test sets are available for all the 20 languages.\n\n\nFor more details see URL",
"### Supported Tasks and Leaderboards\n\n\n* 'named-entity-recognition': The performance in this task is measured with F1 (higher is better). A named entity is correct only if it is an exact match of the corresponding entity in the data.",
"### Languages\n\n\nThere are 20 languages available :\n\n\n* Bambara (bam)\n* Ghomala (bbj)\n* Ewe (ewe)\n* Fon (fon)\n* Hausa (hau)\n* Igbo (ibo)\n* Kinyarwanda (kin)\n* Luganda (lug)\n* Dholuo (luo)\n* Mossi (mos)\n* Chichewa (nya)\n* Nigerian Pidgin\n* chShona (sna)\n* Kiswahili (swą)\n* Setswana (tsn)\n* Twi (twi)\n* Wolof (wol)\n* isiXhosa (xho)\n* Yorùbá (yor)\n* isiZulu (zul)\n\n\nDataset Structure\n-----------------",
"### Data Instances\n\n\nThe examples look like this for Yorùbá:",
"### Data Fields\n\n\n* 'id': id of the sample\n* 'tokens': the tokens of the example text\n* 'ner\\_tags': the NER tags of each token\n\n\nThe NER tags correspond to this list:\n\n\nIn the NER tags, a B denotes the first item of a phrase and an I any non-initial word. There are four types of phrases: person names (PER), organizations (ORG), locations (LOC) and dates & time (DATE).\n\n\nIt is assumed that named entities are non-recursive and non-overlapping. In case a named entity is embedded in another named entity usually, only the top level entity is marked.",
"### Data Splits\n\n\nFor all languages, there are three splits.\n\n\nThe original splits were named 'train', 'dev' and 'test' and they correspond to the 'train', 'validation' and 'test' splits.\n\n\nThe splits have the following sizes :\n\n\n\nDataset Creation\n----------------",
"### Curation Rationale\n\n\nThe dataset was introduced to introduce new resources to 20 languages that were under-served for natural language processing.",
"### Source Data\n\n\nThe source of the data is from the news domain, details can be found here URL",
"#### Initial Data Collection and Normalization\n\n\nThe articles were word-tokenized, information on the exact pre-processing pipeline is unavailable.",
"#### Who are the source language producers?\n\n\nThe source language was produced by journalists and writers employed by the news agency and newspaper mentioned above.",
"### Annotations",
"#### Annotation process\n\n\nDetails can be found here URL",
"#### Who are the annotators?\n\n\nAnnotators were recruited from Masakhane",
"### Personal and Sensitive Information\n\n\nThe data is sourced from newspaper source and only contains mentions of public figures or individuals\n\n\nConsiderations for Using the Data\n---------------------------------",
"### Social Impact of Dataset",
"### Discussion of Biases",
"### Other Known Limitations\n\n\nUsers should keep in mind that the dataset only contains news text, which might limit the applicability of the developed systems to other domains.\n\n\nAdditional Information\n----------------------",
"### Dataset Curators",
"### Licensing Information\n\n\nThe licensing status of the data is CC 4.0 Non-Commercial\n\n\nProvide the BibTex-formatted reference for the dataset. For example:",
"### Contributions\n\n\nThanks to @dadelani for adding this dataset."
] | [
"TAGS\n#task_categories-token-classification #task_ids-named-entity-recognition #annotations_creators-expert-generated #language_creators-expert-generated #multilinguality-multilingual #size_categories-1K<n<10K #source_datasets-original #language-Bambara #language-Ghomálá' #language-Ewe #language-Fon #language-Hausa #language-Igbo #language-Kinyarwanda #language-Ganda #language-Luo (Kenya and Tanzania) #language-Mossi #language-Nyanja #language-Nigerian Pidgin #language-Shona #language-Swahili (macrolanguage) #language-Tswana #language-Twi #language-Wolof #language-Xhosa #language-Yoruba #language-Zulu #license-afl-3.0 #ner #masakhaner #masakhane #arxiv-2103.11811 #arxiv-2210.12391 #region-us \n",
"### Dataset Summary\n\n\nMasakhaNER 2.0 is the largest publicly available high-quality dataset for named entity recognition (NER) in 20 African languages created by the Masakhane community.\n\n\nNamed entities are phrases that contain the names of persons, organizations, locations, times and quantities. Example:\n\n\n[PER Wolff] , currently a journalist in [LOC Argentina] , played with [PER Del Bosque] in the final years of the seventies in [ORG Real Madrid] .\n\n\nMasakhaNER 2.0 is a named entity dataset consisting of PER, ORG, LOC, and DATE entities annotated by Masakhane for 20 African languages\n\n\nThe train/validation/test sets are available for all the 20 languages.\n\n\nFor more details see URL",
"### Supported Tasks and Leaderboards\n\n\n* 'named-entity-recognition': The performance in this task is measured with F1 (higher is better). A named entity is correct only if it is an exact match of the corresponding entity in the data.",
"### Languages\n\n\nThere are 20 languages available :\n\n\n* Bambara (bam)\n* Ghomala (bbj)\n* Ewe (ewe)\n* Fon (fon)\n* Hausa (hau)\n* Igbo (ibo)\n* Kinyarwanda (kin)\n* Luganda (lug)\n* Dholuo (luo)\n* Mossi (mos)\n* Chichewa (nya)\n* Nigerian Pidgin\n* chShona (sna)\n* Kiswahili (swą)\n* Setswana (tsn)\n* Twi (twi)\n* Wolof (wol)\n* isiXhosa (xho)\n* Yorùbá (yor)\n* isiZulu (zul)\n\n\nDataset Structure\n-----------------",
"### Data Instances\n\n\nThe examples look like this for Yorùbá:",
"### Data Fields\n\n\n* 'id': id of the sample\n* 'tokens': the tokens of the example text\n* 'ner\\_tags': the NER tags of each token\n\n\nThe NER tags correspond to this list:\n\n\nIn the NER tags, a B denotes the first item of a phrase and an I any non-initial word. There are four types of phrases: person names (PER), organizations (ORG), locations (LOC) and dates & time (DATE).\n\n\nIt is assumed that named entities are non-recursive and non-overlapping. In case a named entity is embedded in another named entity usually, only the top level entity is marked.",
"### Data Splits\n\n\nFor all languages, there are three splits.\n\n\nThe original splits were named 'train', 'dev' and 'test' and they correspond to the 'train', 'validation' and 'test' splits.\n\n\nThe splits have the following sizes :\n\n\n\nDataset Creation\n----------------",
"### Curation Rationale\n\n\nThe dataset was introduced to introduce new resources to 20 languages that were under-served for natural language processing.",
"### Source Data\n\n\nThe source of the data is from the news domain, details can be found here URL",
"#### Initial Data Collection and Normalization\n\n\nThe articles were word-tokenized, information on the exact pre-processing pipeline is unavailable.",
"#### Who are the source language producers?\n\n\nThe source language was produced by journalists and writers employed by the news agency and newspaper mentioned above.",
"### Annotations",
"#### Annotation process\n\n\nDetails can be found here URL",
"#### Who are the annotators?\n\n\nAnnotators were recruited from Masakhane",
"### Personal and Sensitive Information\n\n\nThe data is sourced from newspaper source and only contains mentions of public figures or individuals\n\n\nConsiderations for Using the Data\n---------------------------------",
"### Social Impact of Dataset",
"### Discussion of Biases",
"### Other Known Limitations\n\n\nUsers should keep in mind that the dataset only contains news text, which might limit the applicability of the developed systems to other domains.\n\n\nAdditional Information\n----------------------",
"### Dataset Curators",
"### Licensing Information\n\n\nThe licensing status of the data is CC 4.0 Non-Commercial\n\n\nProvide the BibTex-formatted reference for the dataset. For example:",
"### Contributions\n\n\nThanks to @dadelani for adding this dataset."
] |
33e7302db76eda5e1cf963615605ed03434f4513 | # Dataset Card for "NLQuAD"
## Table of Contents
- [Table of Contents](#table-of-contents)
- [Dataset Description](#dataset-description)
- [Dataset Summary](#dataset-summary)
- [Dataset Structure](#dataset-structure)
- [Data Instances](#data-instances)
- [Data Fields](#data-fields)
- [Data Splits](#data-splits)
- [Additional Information](#additional-information)
- [Licensing Information](#licensing-information)
- [Citation Information](#citation-information)
## Dataset Description
- **Homepage:** [https://github.com/ASoleimaniB/NLQuAD](https://github.com/ASoleimaniB/NLQuAD)
- **Paper: https://aclanthology.org/2021.eacl-main.106/**
- **Size of the generated dataset:** 89.95 MB
### Dataset Summary
This is a copy of the original NLQuAD dataset distributed via [Github](https://github.com/ASoleimaniB/NLQuAD).
NLQuAD is a non-factoid long question answering dataset from BBC news articles.
NLQuAD’s question types and the long length of its context documents as well as answers, make it a challenging real-world task.
NLQuAD consists of news articles as context documents, interrogative sub-headings in the articles as questions, and body paragraphs corresponding to the sub-headings as contiguous answers to the questions.
NLQuAD contains 31k non-factoid questions and long answers collected from 13k BBC news articles.
See example articles in BBC [1](https://www.bbc.com/news/world-asia-china-51230011), [2](https://www.bbc.com/news/world-55709428).
We automatically extract target answers because annotating for non-factoid long QA is extremely challenging and costly.
## Dataset Structure
### Data Instances
An example of 'train' looks as follows.
```json
{
"title": "Khashoggi murder: Body 'dissolved in acid'",
"date": "2 November 2018",
"paragraphs":[
{
"context": "A top Turkish official, presidential adviser Yasin Aktay, has said ....",
"qas":[
{
"question":"What was said in the crown prince's alleged phone call?",
"id":"0_0",
"answers":[
{
"text":"During the call with President Donald Trump\'s son-in-law Jared Kushner and national ....",
"answer_start":1352,
"answer_end": 2108,
}
]
},
{
"question":"What has the investigation found so far?",
"id":"0_1",
"answers":[
{
"text":"There is still no consensus on how Khashoggi died. He entered ....",
"answer_start":2109,
"answer_end": 3128,
}
]
},
]
}
]
}
```
### Data Fields
The data fields are the same among all splits.
- `title`: a `string` feature.
- `date`: a `string` feature.
- `paragraphs`: a list feature containing dictionaries:
- `context`: a `string` feature.
- `qas`: a list feature containing dictionaries:
- `question`: a `string` feature.
- `id`: a `string` feature.
- `answers`: a list feature containing dictionaries:
- `text`: a `string` feature.
- `answer_start`: a `int32` feature.
- `answer_end`: a `int32` feature
### Data Splits
| name |train|test|validation|
|----------|----:|----:|---------:|
| |10259| 1280| 1280|
## Additional Information
### Licensing Information
This dataset is distributed under the [CC BY-NC](https://creativecommons.org/licenses/by-nc/3.0/) licence providing free access for non-commercial and academic usage.
### Citation Information
BibTeX:
```json
@inproceedings{soleimani-etal-2021-nlquad,
title = "{NLQ}u{AD}: A Non-Factoid Long Question Answering Data Set",
author = "Soleimani, Amir and
Monz, Christof and
Worring, Marcel",
booktitle = "Proceedings of the 16th Conference of the European Chapter of the Association for Computational Linguistics: Main Volume",
month = apr,
year = "2021",
address = "Online",
publisher = "Association for Computational Linguistics",
url = "https://aclanthology.org/2021.eacl-main.106",
doi = "10.18653/v1/2021.eacl-main.106",
pages = "1245--1255",
abstract = "We introduce NLQuAD, the first data set with baseline methods for non-factoid long question answering, a task requiring document-level language understanding. In contrast to existing span detection question answering data sets, NLQuAD has non-factoid questions that are not answerable by a short span of text and demanding multiple-sentence descriptive answers and opinions. We show the limitation of the F1 score for evaluation of long answers and introduce Intersection over Union (IoU), which measures position-sensitive overlap between the predicted and the target answer spans. To establish baseline performances, we compare BERT, RoBERTa, and Longformer models. Experimental results and human evaluations show that Longformer outperforms the other architectures, but results are still far behind a human upper bound, leaving substantial room for improvements. NLQuAD{'}s samples exceed the input limitation of most pre-trained Transformer-based models, encouraging future research on long sequence language models.",
}
``` | LLukas22/NLQuAD | [
"task_ids:extractive-qa",
"multilinguality:monolingual",
"size_categories:10K<n<100K",
"language:en",
"license:cc-by-3.0",
"region:us"
] | 2022-12-15T15:05:57+00:00 | {"language": ["en"], "license": ["cc-by-3.0"], "multilinguality": ["monolingual"], "size_categories": ["10K<n<100K"], "task_ids": ["extractive-qa"], "pretty_name": "NLQuAD", "dataset_info": {"features": [{"name": "title", "dtype": "string"}, {"name": "date", "dtype": "string"}, {"name": "paragraphs", "list": [{"name": "context", "dtype": "string"}, {"name": "qas", "list": [{"name": "answers", "list": [{"name": "answer_end", "dtype": "int64"}, {"name": "answer_start", "dtype": "int64"}, {"name": "text", "dtype": "string"}]}, {"name": "id", "dtype": "string"}, {"name": "question", "dtype": "string"}]}]}], "splits": [{"name": "train", "num_bytes": 72036724, "num_examples": 10259}, {"name": "test", "num_bytes": 9045482, "num_examples": 1280}, {"name": "validation", "num_bytes": 8876137, "num_examples": 1280}], "download_size": 0, "dataset_size": 89958343}} | 2022-12-23T13:04:58+00:00 | [] | [
"en"
] | TAGS
#task_ids-extractive-qa #multilinguality-monolingual #size_categories-10K<n<100K #language-English #license-cc-by-3.0 #region-us
| Dataset Card for "NLQuAD"
=========================
Table of Contents
-----------------
* Table of Contents
* Dataset Description
+ Dataset Summary
* Dataset Structure
+ Data Instances
+ Data Fields
+ Data Splits
* Additional Information
+ Licensing Information
+ Citation Information
Dataset Description
-------------------
* Homepage: URL
* Paper: URL
* Size of the generated dataset: 89.95 MB
### Dataset Summary
This is a copy of the original NLQuAD dataset distributed via Github.
NLQuAD is a non-factoid long question answering dataset from BBC news articles.
NLQuAD’s question types and the long length of its context documents as well as answers, make it a challenging real-world task.
NLQuAD consists of news articles as context documents, interrogative sub-headings in the articles as questions, and body paragraphs corresponding to the sub-headings as contiguous answers to the questions.
NLQuAD contains 31k non-factoid questions and long answers collected from 13k BBC news articles.
See example articles in BBC 1, 2.
We automatically extract target answers because annotating for non-factoid long QA is extremely challenging and costly.
Dataset Structure
-----------------
### Data Instances
An example of 'train' looks as follows.
### Data Fields
The data fields are the same among all splits.
* 'title': a 'string' feature.
* 'date': a 'string' feature.
* 'paragraphs': a list feature containing dictionaries:
+ 'context': a 'string' feature.
+ 'qas': a list feature containing dictionaries:
- 'question': a 'string' feature.
- 'id': a 'string' feature.
- 'answers': a list feature containing dictionaries:
* 'text': a 'string' feature.
* 'answer\_start': a 'int32' feature.
* 'answer\_end': a 'int32' feature
### Data Splits
Additional Information
----------------------
### Licensing Information
This dataset is distributed under the CC BY-NC licence providing free access for non-commercial and academic usage.
BibTeX:
| [
"### Dataset Summary\n\n\nThis is a copy of the original NLQuAD dataset distributed via Github.\n\n\nNLQuAD is a non-factoid long question answering dataset from BBC news articles.\nNLQuAD’s question types and the long length of its context documents as well as answers, make it a challenging real-world task.\nNLQuAD consists of news articles as context documents, interrogative sub-headings in the articles as questions, and body paragraphs corresponding to the sub-headings as contiguous answers to the questions.\nNLQuAD contains 31k non-factoid questions and long answers collected from 13k BBC news articles.\nSee example articles in BBC 1, 2.\nWe automatically extract target answers because annotating for non-factoid long QA is extremely challenging and costly.\n\n\nDataset Structure\n-----------------",
"### Data Instances\n\n\nAn example of 'train' looks as follows.",
"### Data Fields\n\n\nThe data fields are the same among all splits.\n\n\n* 'title': a 'string' feature.\n* 'date': a 'string' feature.\n* 'paragraphs': a list feature containing dictionaries:\n\t+ 'context': a 'string' feature.\n\t+ 'qas': a list feature containing dictionaries:\n\t\t- 'question': a 'string' feature.\n\t\t- 'id': a 'string' feature.\n\t\t- 'answers': a list feature containing dictionaries:\n\t\t\t* 'text': a 'string' feature.\n\t\t\t* 'answer\\_start': a 'int32' feature.\n\t\t\t* 'answer\\_end': a 'int32' feature",
"### Data Splits\n\n\n\nAdditional Information\n----------------------",
"### Licensing Information\n\n\nThis dataset is distributed under the CC BY-NC licence providing free access for non-commercial and academic usage.\n\n\nBibTeX:"
] | [
"TAGS\n#task_ids-extractive-qa #multilinguality-monolingual #size_categories-10K<n<100K #language-English #license-cc-by-3.0 #region-us \n",
"### Dataset Summary\n\n\nThis is a copy of the original NLQuAD dataset distributed via Github.\n\n\nNLQuAD is a non-factoid long question answering dataset from BBC news articles.\nNLQuAD’s question types and the long length of its context documents as well as answers, make it a challenging real-world task.\nNLQuAD consists of news articles as context documents, interrogative sub-headings in the articles as questions, and body paragraphs corresponding to the sub-headings as contiguous answers to the questions.\nNLQuAD contains 31k non-factoid questions and long answers collected from 13k BBC news articles.\nSee example articles in BBC 1, 2.\nWe automatically extract target answers because annotating for non-factoid long QA is extremely challenging and costly.\n\n\nDataset Structure\n-----------------",
"### Data Instances\n\n\nAn example of 'train' looks as follows.",
"### Data Fields\n\n\nThe data fields are the same among all splits.\n\n\n* 'title': a 'string' feature.\n* 'date': a 'string' feature.\n* 'paragraphs': a list feature containing dictionaries:\n\t+ 'context': a 'string' feature.\n\t+ 'qas': a list feature containing dictionaries:\n\t\t- 'question': a 'string' feature.\n\t\t- 'id': a 'string' feature.\n\t\t- 'answers': a list feature containing dictionaries:\n\t\t\t* 'text': a 'string' feature.\n\t\t\t* 'answer\\_start': a 'int32' feature.\n\t\t\t* 'answer\\_end': a 'int32' feature",
"### Data Splits\n\n\n\nAdditional Information\n----------------------",
"### Licensing Information\n\n\nThis dataset is distributed under the CC BY-NC licence providing free access for non-commercial and academic usage.\n\n\nBibTeX:"
] |
39773343c536d17028c3311abaf50ef0bc49bd24 |
# Dataset Card for Multi<sup>3</sup>NLU++
## Table of Contents
- [Dataset Description](#dataset-description)
- [Dataset Summary](#dataset-summary)
- [Supported Tasks and Leaderboards](#supported-tasks-and-leaderboards)
- [Languages](#languages)
- [Dataset Structure](#dataset-structure)
- [Data Instances](#data-instances)
- [Data Fields](#data-fields)
- [Data Splits](#data-splits)
- [Dataset Creation](#dataset-creation)
- [Curation Rationale](#curation-rationale)
- [Source Data](#source-data)
- [Personal and Sensitive Information](#personal-and-sensitive-information)
- [Considerations for Using the Data](#considerations-for-using-the-data)
- [Discussion of Biases](#discussion-of-biases)
- [Other Known Limitations](#other-known-limitations)
- [Additional Information](#additional-information)
- [Licensing Information](#licensing-information)
- [Citation Information](#citation-information)
- [Contact](#contact)
## Dataset Description
- **Paper:** [arXiv](https://arxiv.org/abs/2212.10455)
### Dataset Summary
Please access the dataset using
```
git clone https://huggingface.co/datasets/uoe-nlp/multi3-nlu/
```
Multi<sup>3</sup>NLU++ consists of 3080 utterances per language representing challenges in building multilingual multi-intent multi-domain task-oriented dialogue systems. The domains include banking and hotels. There are 62 unique intents.
### Supported Tasks and Leaderboards
- multi-label intent detection
- slot filling
- cross-lingual language understanding for task-oriented dialogue
### Languages
The dataset covers four language pairs in addition to the source dataset in English:
Spanish, Turkish, Marathi, Amharic
## Dataset Structure
### Data Instances
Each data instance contains the following features: _text_, _intents_, _uid_, _lang_, and ocassionally _slots_ and _values_
See the [Multi<sup>3</sup>NLU++ corpus viewer](https://huggingface.co/datasets/uoe-nlp/multi3-nlu/viewer/uoe-nlp--multi3-nlu/train) to explore more examples.
An example from the Multi<sup>3</sup>NLU++ looks like the following:
```
{
"text": "माझे उद्याचे रिझर्वेशन मला रद्द का करता येणार नाही?",
"intents": [
"why",
"booking",
"cancel_close_leave_freeze",
"wrong_notworking_notshowing"
],
"slots": {
"date_from": {
"text": "उद्याचे",
"span": [
5,
12
],
"value": {
"day": 16,
"month": 3,
"year": 2022
}
}
},
"uid": "hotel_1_1",
"lang": "mr"
}
```
### Data Fields
- 'text': a string containing the utterance for which the intent needs to be detected
- 'intents': the corresponding intent labels
- 'uid': unique identifier per language
- 'lang': the language of the dataset
- 'slots': annotation of the span that needs to be extracted for value extraction with its label and _value_
### Data Splits
The experiments are done on different k-fold validation setups. The dataset has multiple types of data splits. Please see Section 4 of the paper.
## Dataset Creation
### Curation Rationale
Existing task-oriented dialogue datasets are 1) predominantly limited to detecting a single intent, 2) focused on a single domain, and 3) include a small set of slot types. Furthermore, the success of task-oriented dialogue is 4) often evaluated on a small set of higher-resource languages (i.e., typically English) which does not test how generalisable systems are to the diverse range of the world's languages.
Our proposed dataset addresses all these limitations
### Source Data
#### Initial Data Collection and Normalization
Please see Section 3 of the paper
#### Who are the source language producers?
The source language producers are authors of [NLU++ dataset](https://arxiv.org/abs/2204.13021). The dataset was professionally translated into our chosen four languages. We used Blend Express and Proz.com to recruit these translators.
### Personal and Sensitive Information
None. Names are fictional
### Discussion of Biases
We have carefully vetted the examples to exclude the problematic examples.
### Other Known Limitations
The dataset comprises utterances extracted from real dialogues between users and conversational agents as well as synthetic human-authored utterances constructed with the aim of introducing additional combinations of intents and slots. The utterances therefore lack the wider context that would be present in a complete dialogue. As such the dataset cannot be used to evaluate systems with respect to discourse-level phenomena present in dialogue.
## Additional Information
Baseline models:
Our MLP and QA models are based on the huggingface transformers library.
### QA
We use the following code snippet for our QA experiments. Please refer to the paper for more details
```
https://github.com/huggingface/transformers/blob/main/examples/pytorch/question-answering/run_qa.py
python run_qa.py config_qa.json
```
### Licensing Information
The dataset is Creative Commons Attribution 4.0 International (cc-by-4.0)
### Citation Information
Coming soon
### Contact
[Nikita Moghe](mailto:[email protected]) and [Evgeniia Razumovskaia]([email protected]) and [Liane Guillou](mailto:[email protected])
Dataset card based on [Allociné](https://huggingface.co/datasets/allocine) | uoe-nlp/multi3-nlu | [
"task_categories:text-classification",
"multilinguality:multilingual",
"source_datasets:nluplusplus",
"language:multilingual",
"license:cc-by-4.0",
"arxiv:2212.10455",
"arxiv:2204.13021",
"region:us"
] | 2022-12-15T15:46:30+00:00 | {"language": ["multilingual"], "license": ["cc-by-4.0"], "multilinguality": ["multilingual"], "source_datasets": ["nluplusplus"], "task_categories": ["text-classification"], "pretty_name": "multi3-nlu"} | 2023-06-07T09:46:27+00:00 | [
"2212.10455",
"2204.13021"
] | [
"multilingual"
] | TAGS
#task_categories-text-classification #multilinguality-multilingual #source_datasets-nluplusplus #language-multilingual #license-cc-by-4.0 #arxiv-2212.10455 #arxiv-2204.13021 #region-us
|
# Dataset Card for Multi<sup>3</sup>NLU++
## Table of Contents
- Dataset Description
- Dataset Summary
- Supported Tasks and Leaderboards
- Languages
- Dataset Structure
- Data Instances
- Data Fields
- Data Splits
- Dataset Creation
- Curation Rationale
- Source Data
- Personal and Sensitive Information
- Considerations for Using the Data
- Discussion of Biases
- Other Known Limitations
- Additional Information
- Licensing Information
- Citation Information
- Contact
## Dataset Description
- Paper: arXiv
### Dataset Summary
Please access the dataset using
Multi<sup>3</sup>NLU++ consists of 3080 utterances per language representing challenges in building multilingual multi-intent multi-domain task-oriented dialogue systems. The domains include banking and hotels. There are 62 unique intents.
### Supported Tasks and Leaderboards
- multi-label intent detection
- slot filling
- cross-lingual language understanding for task-oriented dialogue
### Languages
The dataset covers four language pairs in addition to the source dataset in English:
Spanish, Turkish, Marathi, Amharic
## Dataset Structure
### Data Instances
Each data instance contains the following features: _text_, _intents_, _uid_, _lang_, and ocassionally _slots_ and _values_
See the Multi<sup>3</sup>NLU++ corpus viewer to explore more examples.
An example from the Multi<sup>3</sup>NLU++ looks like the following:
### Data Fields
- 'text': a string containing the utterance for which the intent needs to be detected
- 'intents': the corresponding intent labels
- 'uid': unique identifier per language
- 'lang': the language of the dataset
- 'slots': annotation of the span that needs to be extracted for value extraction with its label and _value_
### Data Splits
The experiments are done on different k-fold validation setups. The dataset has multiple types of data splits. Please see Section 4 of the paper.
## Dataset Creation
### Curation Rationale
Existing task-oriented dialogue datasets are 1) predominantly limited to detecting a single intent, 2) focused on a single domain, and 3) include a small set of slot types. Furthermore, the success of task-oriented dialogue is 4) often evaluated on a small set of higher-resource languages (i.e., typically English) which does not test how generalisable systems are to the diverse range of the world's languages.
Our proposed dataset addresses all these limitations
### Source Data
#### Initial Data Collection and Normalization
Please see Section 3 of the paper
#### Who are the source language producers?
The source language producers are authors of NLU++ dataset. The dataset was professionally translated into our chosen four languages. We used Blend Express and URL to recruit these translators.
### Personal and Sensitive Information
None. Names are fictional
### Discussion of Biases
We have carefully vetted the examples to exclude the problematic examples.
### Other Known Limitations
The dataset comprises utterances extracted from real dialogues between users and conversational agents as well as synthetic human-authored utterances constructed with the aim of introducing additional combinations of intents and slots. The utterances therefore lack the wider context that would be present in a complete dialogue. As such the dataset cannot be used to evaluate systems with respect to discourse-level phenomena present in dialogue.
## Additional Information
Baseline models:
Our MLP and QA models are based on the huggingface transformers library.
### QA
We use the following code snippet for our QA experiments. Please refer to the paper for more details
### Licensing Information
The dataset is Creative Commons Attribution 4.0 International (cc-by-4.0)
Coming soon
### Contact
Nikita Moghe and Evgeniia Razumovskaia and Liane Guillou
Dataset card based on Allociné | [
"# Dataset Card for Multi<sup>3</sup>NLU++",
"## Table of Contents\n- Dataset Description\n - Dataset Summary\n - Supported Tasks and Leaderboards\n - Languages\n- Dataset Structure\n - Data Instances\n - Data Fields\n - Data Splits\n- Dataset Creation\n - Curation Rationale\n - Source Data\n - Personal and Sensitive Information\n- Considerations for Using the Data\n - Discussion of Biases\n - Other Known Limitations\n- Additional Information\n - Licensing Information\n - Citation Information\n - Contact",
"## Dataset Description\n\n- Paper: arXiv",
"### Dataset Summary\nPlease access the dataset using \n\n\nMulti<sup>3</sup>NLU++ consists of 3080 utterances per language representing challenges in building multilingual multi-intent multi-domain task-oriented dialogue systems. The domains include banking and hotels. There are 62 unique intents.",
"### Supported Tasks and Leaderboards\n\n- multi-label intent detection\n- slot filling\n- cross-lingual language understanding for task-oriented dialogue",
"### Languages\n\nThe dataset covers four language pairs in addition to the source dataset in English: \nSpanish, Turkish, Marathi, Amharic",
"## Dataset Structure",
"### Data Instances\n\nEach data instance contains the following features: _text_, _intents_, _uid_, _lang_, and ocassionally _slots_ and _values_\n\nSee the Multi<sup>3</sup>NLU++ corpus viewer to explore more examples.\n\nAn example from the Multi<sup>3</sup>NLU++ looks like the following:",
"### Data Fields\n\n- 'text': a string containing the utterance for which the intent needs to be detected\n- 'intents': the corresponding intent labels\n- 'uid': unique identifier per language\n- 'lang': the language of the dataset \n- 'slots': annotation of the span that needs to be extracted for value extraction with its label and _value_",
"### Data Splits\n\nThe experiments are done on different k-fold validation setups. The dataset has multiple types of data splits. Please see Section 4 of the paper.",
"## Dataset Creation",
"### Curation Rationale\nExisting task-oriented dialogue datasets are 1) predominantly limited to detecting a single intent, 2) focused on a single domain, and 3) include a small set of slot types. Furthermore, the success of task-oriented dialogue is 4) often evaluated on a small set of higher-resource languages (i.e., typically English) which does not test how generalisable systems are to the diverse range of the world's languages.\nOur proposed dataset addresses all these limitations",
"### Source Data",
"#### Initial Data Collection and Normalization\nPlease see Section 3 of the paper",
"#### Who are the source language producers?\nThe source language producers are authors of NLU++ dataset. The dataset was professionally translated into our chosen four languages. We used Blend Express and URL to recruit these translators.",
"### Personal and Sensitive Information\n\nNone. Names are fictional",
"### Discussion of Biases\n\nWe have carefully vetted the examples to exclude the problematic examples.",
"### Other Known Limitations\nThe dataset comprises utterances extracted from real dialogues between users and conversational agents as well as synthetic human-authored utterances constructed with the aim of introducing additional combinations of intents and slots. The utterances therefore lack the wider context that would be present in a complete dialogue. As such the dataset cannot be used to evaluate systems with respect to discourse-level phenomena present in dialogue.",
"## Additional Information\nBaseline models:\nOur MLP and QA models are based on the huggingface transformers library.",
"### QA\nWe use the following code snippet for our QA experiments. Please refer to the paper for more details",
"### Licensing Information\n\nThe dataset is Creative Commons Attribution 4.0 International (cc-by-4.0) \n\n\n\nComing soon",
"### Contact\nNikita Moghe and Evgeniia Razumovskaia and Liane Guillou\n\nDataset card based on Allociné"
] | [
"TAGS\n#task_categories-text-classification #multilinguality-multilingual #source_datasets-nluplusplus #language-multilingual #license-cc-by-4.0 #arxiv-2212.10455 #arxiv-2204.13021 #region-us \n",
"# Dataset Card for Multi<sup>3</sup>NLU++",
"## Table of Contents\n- Dataset Description\n - Dataset Summary\n - Supported Tasks and Leaderboards\n - Languages\n- Dataset Structure\n - Data Instances\n - Data Fields\n - Data Splits\n- Dataset Creation\n - Curation Rationale\n - Source Data\n - Personal and Sensitive Information\n- Considerations for Using the Data\n - Discussion of Biases\n - Other Known Limitations\n- Additional Information\n - Licensing Information\n - Citation Information\n - Contact",
"## Dataset Description\n\n- Paper: arXiv",
"### Dataset Summary\nPlease access the dataset using \n\n\nMulti<sup>3</sup>NLU++ consists of 3080 utterances per language representing challenges in building multilingual multi-intent multi-domain task-oriented dialogue systems. The domains include banking and hotels. There are 62 unique intents.",
"### Supported Tasks and Leaderboards\n\n- multi-label intent detection\n- slot filling\n- cross-lingual language understanding for task-oriented dialogue",
"### Languages\n\nThe dataset covers four language pairs in addition to the source dataset in English: \nSpanish, Turkish, Marathi, Amharic",
"## Dataset Structure",
"### Data Instances\n\nEach data instance contains the following features: _text_, _intents_, _uid_, _lang_, and ocassionally _slots_ and _values_\n\nSee the Multi<sup>3</sup>NLU++ corpus viewer to explore more examples.\n\nAn example from the Multi<sup>3</sup>NLU++ looks like the following:",
"### Data Fields\n\n- 'text': a string containing the utterance for which the intent needs to be detected\n- 'intents': the corresponding intent labels\n- 'uid': unique identifier per language\n- 'lang': the language of the dataset \n- 'slots': annotation of the span that needs to be extracted for value extraction with its label and _value_",
"### Data Splits\n\nThe experiments are done on different k-fold validation setups. The dataset has multiple types of data splits. Please see Section 4 of the paper.",
"## Dataset Creation",
"### Curation Rationale\nExisting task-oriented dialogue datasets are 1) predominantly limited to detecting a single intent, 2) focused on a single domain, and 3) include a small set of slot types. Furthermore, the success of task-oriented dialogue is 4) often evaluated on a small set of higher-resource languages (i.e., typically English) which does not test how generalisable systems are to the diverse range of the world's languages.\nOur proposed dataset addresses all these limitations",
"### Source Data",
"#### Initial Data Collection and Normalization\nPlease see Section 3 of the paper",
"#### Who are the source language producers?\nThe source language producers are authors of NLU++ dataset. The dataset was professionally translated into our chosen four languages. We used Blend Express and URL to recruit these translators.",
"### Personal and Sensitive Information\n\nNone. Names are fictional",
"### Discussion of Biases\n\nWe have carefully vetted the examples to exclude the problematic examples.",
"### Other Known Limitations\nThe dataset comprises utterances extracted from real dialogues between users and conversational agents as well as synthetic human-authored utterances constructed with the aim of introducing additional combinations of intents and slots. The utterances therefore lack the wider context that would be present in a complete dialogue. As such the dataset cannot be used to evaluate systems with respect to discourse-level phenomena present in dialogue.",
"## Additional Information\nBaseline models:\nOur MLP and QA models are based on the huggingface transformers library.",
"### QA\nWe use the following code snippet for our QA experiments. Please refer to the paper for more details",
"### Licensing Information\n\nThe dataset is Creative Commons Attribution 4.0 International (cc-by-4.0) \n\n\n\nComing soon",
"### Contact\nNikita Moghe and Evgeniia Razumovskaia and Liane Guillou\n\nDataset card based on Allociné"
] |
145d48995c3839609d2a7e7460c9bb9a5be6df66 | # Dataset Card for "common_voice_11_0_id_filtered"
[More Information needed](https://github.com/huggingface/datasets/blob/main/CONTRIBUTING.md#how-to-contribute-to-the-dataset-cards) | evanarlian/common_voice_11_0_id_filtered | [
"region:us"
] | 2022-12-15T16:05:49+00:00 | {"dataset_info": {"features": [{"name": "client_id", "dtype": "string"}, {"name": "path", "dtype": "string"}, {"name": "audio", "dtype": {"audio": {"sampling_rate": 16000}}}, {"name": "sentence", "dtype": "string"}, {"name": "up_votes", "dtype": "int64"}, {"name": "down_votes", "dtype": "int64"}, {"name": "age", "dtype": "string"}, {"name": "gender", "dtype": "string"}, {"name": "accent", "dtype": "string"}, {"name": "locale", "dtype": "string"}, {"name": "segment", "dtype": "string"}], "splits": [{"name": "train", "num_bytes": 570693903.7812607, "num_examples": 22906}, {"name": "validation", "num_bytes": 98832914.0, "num_examples": 3226}, {"name": "test", "num_bytes": 112254685.0, "num_examples": 3618}, {"name": "other", "num_bytes": 147132536.35696015, "num_examples": 6380}, {"name": "invalidated", "num_bytes": 63830420.0, "num_examples": 2466}], "download_size": 975354578, "dataset_size": 992744459.1382209}} | 2022-12-15T16:06:36+00:00 | [] | [] | TAGS
#region-us
| # Dataset Card for "common_voice_11_0_id_filtered"
More Information needed | [
"# Dataset Card for \"common_voice_11_0_id_filtered\"\n\nMore Information needed"
] | [
"TAGS\n#region-us \n",
"# Dataset Card for \"common_voice_11_0_id_filtered\"\n\nMore Information needed"
] |
b7b603a637c056fe07d381f21c878d6002bd4758 | # Dataset Card for "python_vul_cvefix"
[More Information needed](https://github.com/huggingface/datasets/blob/main/CONTRIBUTING.md#how-to-contribute-to-the-dataset-cards) | EddieChen372/python_vul_cvefix | [
"region:us"
] | 2022-12-15T16:39:23+00:00 | {"dataset_info": {"features": [{"name": "label", "dtype": {"class_label": {"names": {"0": "CWE-22", "1": "CWE-79", "2": "CWE-89", "3": "CWE-352", "4": "CWE-601", "5": "CWE-94"}}}}, {"name": "code_before", "dtype": "string"}, {"name": "code_after", "dtype": "string"}, {"name": "label_text", "dtype": "string"}, {"name": "deleted", "struct": [{"name": "code", "sequence": "string"}, {"name": "line_no", "sequence": "int64"}]}, {"name": "added", "struct": [{"name": "code", "sequence": "string"}, {"name": "line_no", "sequence": "int64"}]}, {"name": "normalized_code_before", "dtype": "string"}, {"name": "normalized_code_after", "dtype": "string"}, {"name": "before_doc_string_pos", "sequence": "int64"}, {"name": "after_doc_string_pos", "sequence": "int64"}], "splits": [{"name": "train", "num_bytes": 15101828.429268293, "num_examples": 204}, {"name": "test", "num_bytes": 3822268.0, "num_examples": 52}], "download_size": 6388923, "dataset_size": 18924096.429268293}} | 2022-12-15T16:40:12+00:00 | [] | [] | TAGS
#region-us
| # Dataset Card for "python_vul_cvefix"
More Information needed | [
"# Dataset Card for \"python_vul_cvefix\"\n\nMore Information needed"
] | [
"TAGS\n#region-us \n",
"# Dataset Card for \"python_vul_cvefix\"\n\nMore Information needed"
] |
34a2b1e78876a914e12d94f2492aad0f4d700df0 | # Dataset Card for "titanic"
[More Information needed](https://github.com/huggingface/datasets/blob/main/CONTRIBUTING.md#how-to-contribute-to-the-dataset-cards) | lewtun/titanic | [
"kaggle",
"region:us"
] | 2022-12-15T17:56:38+00:00 | {"tags": ["kaggle"], "dataset_info": {"features": [{"name": "PassengerId", "dtype": "int64"}, {"name": "Survived", "dtype": "int64"}, {"name": "Pclass", "dtype": "int64"}, {"name": "Name", "dtype": "string"}, {"name": "Sex", "dtype": "string"}, {"name": "Age", "dtype": "float64"}, {"name": "SibSp", "dtype": "int64"}, {"name": "Parch", "dtype": "int64"}, {"name": "Ticket", "dtype": "string"}, {"name": "Fare", "dtype": "float64"}, {"name": "Cabin", "dtype": "string"}, {"name": "Embarked", "dtype": "string"}], "splits": [{"name": "test", "num_bytes": 49054, "num_examples": 418}, {"name": "train", "num_bytes": 103906, "num_examples": 891}], "download_size": 61019, "dataset_size": 152960}} | 2022-12-15T17:59:50+00:00 | [] | [] | TAGS
#kaggle #region-us
| # Dataset Card for "titanic"
More Information needed | [
"# Dataset Card for \"titanic\"\n\nMore Information needed"
] | [
"TAGS\n#kaggle #region-us \n",
"# Dataset Card for \"titanic\"\n\nMore Information needed"
] |
a881ea99d11c85b7e7263d818be38d87415132b8 | 18/1-22/10 pixiv monthly ranking top50 & yandere images 110k
with txt | haor/pixiv-yandere | [
"license:openrail",
"region:us"
] | 2022-12-15T18:38:01+00:00 | {"license": "openrail"} | 2022-12-16T12:32:39+00:00 | [] | [] | TAGS
#license-openrail #region-us
| 18/1-22/10 pixiv monthly ranking top50 & yandere images 110k
with txt | [] | [
"TAGS\n#license-openrail #region-us \n"
] |
7016f3062103cde04b4551204be4ae99d950db0b |
This dataset is a random 1/3 slice of the original [told-br](https://huggingface.co/datasets/told-br) | alexandreteles/told_br_binary_sm | [
"multilinguality:monolingual",
"size_categories:1K<n<10K",
"source_datasets:told-br",
"language:pt",
"license:cc-by-sa-4.0",
"region:us"
] | 2022-12-15T21:08:14+00:00 | {"language": ["pt"], "license": "cc-by-sa-4.0", "multilinguality": ["monolingual"], "size_categories": ["1K<n<10K"], "source_datasets": ["told-br"], "pretty_name": "ToLD-Br-small", "language_bcp47": ["pt-BR"]} | 2022-12-15T23:00:52+00:00 | [] | [
"pt"
] | TAGS
#multilinguality-monolingual #size_categories-1K<n<10K #source_datasets-told-br #language-Portuguese #license-cc-by-sa-4.0 #region-us
|
This dataset is a random 1/3 slice of the original told-br | [] | [
"TAGS\n#multilinguality-monolingual #size_categories-1K<n<10K #source_datasets-told-br #language-Portuguese #license-cc-by-sa-4.0 #region-us \n"
] |
dc95e5c611f14a95f798dfeb236a6f67aa8252ae | # AutoTrain Dataset for project: told_br_binary_sm
## Dataset Description
This dataset has been automatically processed by AutoTrain for project told_br_binary_sm.
### Languages
The BCP-47 code for the dataset's language is pt.
## Dataset Structure
### Data Instances
A sample from this dataset looks as follows:
```json
[
{
"text": "@user agora n\u00e3o me d\u00e1 mais, mas antes, porra",
"target": 1
},
{
"text": "pires \u00e9 fodido fds mais um",
"target": 1
}
]
```
### Dataset Fields
The dataset has the following fields (also called "features"):
```json
{
"text": "Value(dtype='string', id=None)",
"target": "ClassLabel(names=['0', '1'], id=None)"
}
```
### Dataset Splits
This dataset is split into a train and validation split. The split sizes are as follow:
| Split name | Num samples |
| ------------ | ------------------- |
| train | 5599 |
| valid | 1401 |
| alexandreteles/autotrain-data-told_br_binary_sm | [
"task_categories:text-classification",
"language:pt",
"region:us"
] | 2022-12-15T21:28:30+00:00 | {"language": ["pt"], "task_categories": ["text-classification"]} | 2022-12-15T21:29:16+00:00 | [] | [
"pt"
] | TAGS
#task_categories-text-classification #language-Portuguese #region-us
| AutoTrain Dataset for project: told\_br\_binary\_sm
===================================================
Dataset Description
-------------------
This dataset has been automatically processed by AutoTrain for project told\_br\_binary\_sm.
### Languages
The BCP-47 code for the dataset's language is pt.
Dataset Structure
-----------------
### Data Instances
A sample from this dataset looks as follows:
### Dataset Fields
The dataset has the following fields (also called "features"):
### Dataset Splits
This dataset is split into a train and validation split. The split sizes are as follow:
| [
"### Languages\n\n\nThe BCP-47 code for the dataset's language is pt.\n\n\nDataset Structure\n-----------------",
"### Data Instances\n\n\nA sample from this dataset looks as follows:",
"### Dataset Fields\n\n\nThe dataset has the following fields (also called \"features\"):",
"### Dataset Splits\n\n\nThis dataset is split into a train and validation split. The split sizes are as follow:"
] | [
"TAGS\n#task_categories-text-classification #language-Portuguese #region-us \n",
"### Languages\n\n\nThe BCP-47 code for the dataset's language is pt.\n\n\nDataset Structure\n-----------------",
"### Data Instances\n\n\nA sample from this dataset looks as follows:",
"### Dataset Fields\n\n\nThe dataset has the following fields (also called \"features\"):",
"### Dataset Splits\n\n\nThis dataset is split into a train and validation split. The split sizes are as follow:"
] |
84de4ed4ed041e25f9d3bb95ebb7bf3f4e3551ff |
This post was originally published on the [Hugging Face blog 🤗](https://huggingface.co/blog/ethics-soc-2)
# Ethics and Society Newsletter #2
## Let’s Talk about Bias!
_Bias in ML is ubiquitous, and Bias in ML is complex; so complex in fact that no single technical intervention is likely to meaningfully address the problems it engenders. ML models, as sociotechnical systems, amplify social trends that may exacerbate inequities and harmful biases in ways that depend on their deployment context and are constantly evolving._
_This means that developing ML systems with care requires vigilance and responding to feedback from those deployment contexts, which in turn we can facilitate by sharing lessons across contexts and developing tools to analyze signs of bias at every level of ML development._
_This blog post from the [Ethics and Society regulars @🤗](https://huggingface.co/blog/ethics-soc-1) shares some of the lessons we have learned along with tools we have developed to support ourselves and others in our community’s efforts to better address bias in Machine Learning. The first part is a broader reflection on bias and its context. If you’ve already read it and are coming back specifically for the tools, feel free to jump to the [datasets](#i-am-curatingpicking-a-dataset-for-my-ml-system-how-can-i-address-bias) or [models](#i-am-trainingselecting-a-model-for-my-ml-system-how-can-i-address-bias)
section!_
<p align="center">
<br>
<img src="https://huggingface.co/datasets/huggingface/documentation-images/resolve/main/blog/ethics_soc_2/img1.jpg" alt="Selection of tools developed by HF team members to address bias in ML" />
<em>Selection of tools developed by 🤗 team members to address bias in ML</em>
</p>
**<span style="text-decoration:underline;">Table of contents:</span>**
* **<span style="text-decoration:underline;">On Machine Biases</span>**
* [Machine Bias: from ML Systems to Risks](#machine-bias-from-ml-systems-to-personal-and-social-risks)
* [Putting Bias in Context](#putting-bias-in-context)
* **<span style="text-decoration:underline;">Tools and Recommendations</span>**
* [Addressing Bias throughout ML Development](#addressing-bias-throughout-the-ml-development-cycle)
* [Task Definition](#i-am-defining-the-task-of-my-ml-system-how-can-i-address-bias)
* [Dataset Curation](#i-am-curatingpicking-a-dataset-for-my-ml-system-how-can-i-address-bias)
* [Model Training](#i-am-trainingselecting-a-model-for-my-ml-system-how-can-i-address-bias)
* [Overview of 🤗 Bias Tools](#conclusion-and-overview-of-bias-analysis-and-documentation-tools-from-🤗)
## _Machine Bias:_ from ML Systems to Personal and Social Risks
ML systems allow us to automate complex tasks at a scale never seen before as they are deployed in more sectors and use cases. When the technology works at its best, it can help smooth interactions between people and technical systems, remove the need for highly repetitive work, or unlock new ways of processing information to support research.
These same systems are also likely to reproduce discriminatory and abusive behaviors represented in their training data, especially when the data encodes human behaviors.
The technology then has the potential to make these issues significantly worse. Automation and deployment at scale can indeed:
1. **lock in** behaviors in time and hinder social progress [from being reflected in technology](https://dl.acm.org/doi/10.1145/3442188.3445922),
2. **spread** harmful behaviors [beyond the context](https://arxiv.org/abs/2203.07785) of the original training data,
3. **amplify** inequities by [overfocusing on stereotypical associations](https://arxiv.org/abs/2010.03058) when making predictions,
4. **remove possibilities for recourse** by hiding biases [inside “black-box” systems](https://pubmed.ncbi.nlm.nih.gov/33737318/).
In order to better understand and address these risks, ML researchers and developers have started studying _machine bias_ or _algorithmic bias_, mechanisms that might lead systems to, for example, encode **negative stereotypes or associations** or to have **disparate performance** for different population groups in their deployment context.
**These issues are deeply personal** for many of us ML researchers and developers at Hugging Face and in the broader ML community. Hugging Face is [an international company](https://twitter.com/osanseviero/status/1587444072901492737), with many of us existing between countries and cultures. It is hard to fully express our sense of urgency when we see the technology we work on developed [without sufficient concern](https://dl.acm.org/doi/10.1145/3461702.3462624) for protecting people like us; especially when these systems lead to discriminatory [wrongful arrests](https://incidentdatabase.ai/cite/72/) or undue [financial distress](https://racismandtechnology.center/2021/10/29/amnestys-grim-warning-against-another-toeslagenaffaire/) and are being [increasingly sold](https://www.oecd.org/migration/mig/EMN-OECD-INFORM-FEB-2022-The-use-of-Digitalisation-and-AI-in-Migration-Management.pdf) to immigration and law enforcement services around the world. Similarly, seeing our identities routinely [suppressed in training datasets](https://aclanthology.org/2021.emnlp-main.98/) or [underrepresented in the outputs](https://huggingface.co/spaces/sasha/StableDiffusionBiasExplorer) of “generative AI” [systems ](https://twitter.com/willie_agnew/status/1592829238889283585)connects these concerns to our daily lived experiences in ways that are [simultaneously enlightening and taxing](https://www.technologyreview.com/2022/10/28/1062332/responsible-ai-has-a-burnout-problem/).
While our own experiences do not come close to covering the myriad ways in which ML-mediated discrimination can disproportionately harm people whose experiences differ from ours, they provide an entry point into considerations of the trade-offs inherent in the technology. We work on these systems because we **strongly believe in ML’s potential — we think it can shine as a valuable tool as long as it is developed with care and input from people in its deployment context**, rather than as a one-size-fits-all panacea. In particular, enabling this care requires developing a better understanding of the mechanisms of machine bias across the ML development process, and developing tools that support people [with all levels of technical knowledge of these systems in participating in the necessary conversations](https://www.vice.com/en/article/bvm35w/this-tool-lets-anyone-see-the-bias-in-ai-image-generators) about how their benefits and harms are distributed.
The present blog post from the Hugging Face [Ethics and Society regulars](https://huggingface.co/blog/ethics-soc-1) provides an overview of how we have worked, are working, or recommend users of the HF ecosystem of libraries may work to address bias at the various stages of the ML development process, and the tools we develop to support this process. We hope you will find it a useful resource to guide concrete considerations of the social impact of your work and can leverage the tools referenced here to help mitigate these issues when they arise.
## Putting Bias in Context
The first and maybe most important concept to consider when dealing with machine bias is **context**. In their foundational work on [bias in NLP](https://aclanthology.org/2020.acl-main.485.pdf), Su Lin Blodgett et al. point out that: _“[T]he majority of [academic works on machine bias] fail to engage critically with what constitutes “bias” in the first place”_, including by building their work on top of _“unstated assumptions about what kinds of system behaviors are harmful, in what ways, to whom, and why”_.
This may not come as much of a surprise given the ML research community’s [focus on the value of “generalization”](https://dl.acm.org/doi/10.1145/3531146.3533083) — the most cited motivation for work in the field after “performance”. However, while tools for bias assessment that apply to a wide range of settings are valuable to **enable a broader analysis of common trends** in model behaviors, their ability to target the mechanisms that lead to discrimination in **concrete use cases is inherently limited**. Using them to guide specific decisions within the ML development cycle usually requires an extra step or two to take the system’s specific use context and affected people into consideration.
<p align="center">
<br>
<img src="https://huggingface.co/datasets/huggingface/documentation-images/resolve/main/blog/ethics_soc_2/img_foresight.png" alt="Excerpt on considerations of ML uses context and people from the Model Card Guidebook" />
<em>Excerpt on considerations of ML uses context and people from the <a href="https://huggingface.co/docs/hub/model-cards">Model Card Guidebook</a></em>
</p>
Now let’s dive deeper into the issue of linking biases in stand-alone/context-less ML artifacts to specific harms. It can be useful to think of **machine biases as risk factors for discrimination-based harms**. Take the example of a text-to-image model that over-represents light skin tones when prompted to create a picture of a person in a professional setting, but produces darker skin tones [when the prompts mention criminality](https://arxiv.org/abs/2211.03759). These tendencies would be what we call _machine biases at the model level_. Now let’s think about a few systems that use such a text-to-image model:
1. <span style="text-decoration:underline;">The model is integrated into a website creation service</span> (e.g. SquareSpace, Wix) to help users generate backgrounds for their pages. The model explicitly disables images of people in the generated background.
* In this case, the machine bias “risk factor” does not lead to discrimination harm because the focus of the bias (images of people) is absent from the use case.
* Further risk mitigation is not required for machine biases, although developers should be aware of ongoing discussions about the legality of integrating systems trained on scraped data in commercial systems.
2. <span style="text-decoration:underline;">The model is integrated into a stock images website</span> to provide users with synthetic images of people (e.g. in professional settings) that they can use with fewer privacy concerns, for example, to serve as illustrations for Wikipedia articles
* In this case, machine bias acts to **lock in** and **amplify** existing social biases. It reinforces stereotypes about people (“CEOs are all white men”) that then feed back into complex social systems where increased bias leads to increased discrimination in many different ways (such as reinforcing [implicit bias](https://philpapers.org/rec/BEEAIT-2) in the workplace).
* Mitigation strategies may include educating the stock image users about these biases, or the stock image website may curate generated images to intentionally propose a more diverse set of representations.
3. <span style="text-decoration:underline;">The model is integrated into a “virtual sketch artist” software</span> marketed to police departments that will use it to generate pictures of suspects based on verbal testimony
* In this case, the machine biases directly cause discrimination by systematically directing police departments to darker-skinned people, putting them at increased risk of harm including physical injury and unlawful imprisonment.
* In cases like this one, there may be no level of bias mitigation that makes the risk acceptable. In particular, such a use case would be closely related to face recognition in the context of law enforcement, where [similar bias issues](https://www.law.georgetown.edu/privacy-technology-center/publications/a-forensic-without-the-science-face-recognition-in-u-s-criminal-investigations/) have led several commercial entities and legislatures to adopt moratoria pausing or banning its use across the board.
So, who’s on the hook for machine biases in ML? These three cases illustrate one of the reasons why discussions about the responsibility of ML developers in addressing bias can get so complicated: depending on decisions made at other points in the ML system development process by other people, the biases in an ML dataset or model may land anywhere between being irrelevant to the application settings and directly leading to grievous harm. However, in all of these cases, **stronger biases in the model/dataset increase the risk of negative outcomes**. The European Union has started to develop frameworks that address this phenomenon in [recent regulatory efforts](https://ec.europa.eu/info/business-economy-euro/doing-business-eu/contract-rules/digital-contracts/liability-rules-artificial-intelligence_en): in short, a company that deploys an AI system based on a measurably biased model is liable for harm caused by the system.
Conceptualizing bias as a risk factor then allows us to better understand the **shared responsibility** for machine biases between developers at all stages. Bias can never be fully removed, not least because the definitions of social biases and the power dynamics that tie them to discrimination vary vastly across social contexts. However:
1. Each stage of the development process, from task specification, dataset curation, and model training, to model integration and system deployment, can take steps to minimize the aspects of machine bias** that most directly depend on its choices** and technical decisions, and
2. Clear communication and **information flow between the various ML development stages** can make the difference between making choices that build on top of each other to attenuate the negative potential of bias (multipronged approach to bias mitigation, as in deployment scenario 1 above) _versus_ making choices that compound this negative potential to exacerbate the risk of harm (as in deployment scenario 3).
In the next section, we review these various stages along with some of the tools that can help us address machine bias at each of them.
## Addressing Bias throughout the ML Development Cycle
Ready for some practical advice yet? Here we go 🤗
There is no one single way to develop ML systems; which steps happen in what order depends on a number of factors including the development setting (university, large company, startup, grassroots organization, etc…), the modality (text, tabular data, images, etc…), and the preeminence or scarcity of publicly available ML resources. However, we can identify three common stages of particular interest in addressing bias. These are the task definition, the data curation, and the model training. Let’s have a look at how bias handling may differ across these various stages.
<p align="center">
<br>
<img src="https://huggingface.co/datasets/huggingface/documentation-images/resolve/main/blog/ethics_soc_2/img_pipeline.png" alt="The Bias ML Pipeline by Meg" width="500" />
<em>The Bias ML Pipeline by <a href="https://huggingface.co/meg">Meg</a></em>
</p>
### I am <span style="text-decoration:underline;">defining the task</span> of my ML system, how can I address bias?
Whether and to what extent bias in the system concretely affects people ultimately depends on what the system is used for. As such, the first place developers can work to mitigate bias is when deciding how ML fits in their system, e.g., by deciding what optimization objective it will use.
For example, let’s go back to one of the first highly-publicized cases of a Machine Learning system used in production for algorithmic content recommendation. From 2006 to 2009, Netflix ran the [Netflix Prize](https://www.cs.uic.edu/~liub/KDD-cup-2007/proceedings/The-Netflix-Prize-Bennett.pdf), a competition with a 1M$ cash prize challenging teams around the world to develop ML systems to accurately predict a user’s rating for a new movie based on their past ratings. The [winning submission](https://www.asc.ohio-state.edu/statistics/dmsl/GrandPrize2009_BPC_BigChaos.pdf) improved the RMSE (Root-mean-square-error) of predictions on unseen user-movie pairs by over 10% over Netflix’s own CineMatch algorithm, meaning it got much better at predicting how users would rate a new movie based on their history. This approach opened the door for much of modern algorithmic content recommendation by bringing the role of ML in modeling user preferences in recommender systems to public awareness.
So what does this have to do with bias? Doesn’t showing people content that they’re likely to enjoy sound like a good service from a content platform? Well, it turns out that showing people more examples of **what they’ve liked in the past** ends up [reducing the diversity of the media they consume](https://dl.acm.org/doi/10.1145/3391403.3399532). Not only does it lead users to be [less satisfied in the long term](https://dl.acm.org/doi/abs/10.1145/3366423.3380281), but it also means that any biases or stereotypes captured by the initial models — such as when modeling [the preferences of Black American users](https://www.marieclaire.com/culture/a18817/netflix-algorithms-black-movies/) or [dynamics that systematically disadvantage](https://dl.acm.org/doi/10.1145/3269206.3272027) some artists — are likely to be reinforced if the model is [further trained on ongoing ML-mediated](https://arxiv.org/abs/2209.03942) user interactions. This reflects two of the types of bias-related concerns we’ve mentioned above: the training objective acts as a **risk factor** for bias-related harms as it makes pre-existing biases much more likely to show up in predictions, and the task framing has the effect of **locking in** and exacerbating past biases.
A promising bias mitigation strategy at this stage has been to reframe the task to explicitly [model both engagement and diversity](https://dl.acm.org/doi/10.1145/3437963.3441775) when applying ML to algorithmic content recommendation. Users are likely to get more long-term satisfaction and the risk of exacerbating biases as outlined above is reduced!
This example serves to illustrate that the impact of machine biases in an ML-supported product depends not just on where we decide to leverage ML, but also on how ML techniques are integrated into the broader technical system, and with what objective. When first investigating how ML can fit into a product or a use case you are interested in, we first recommend looking for the failure modes of the system through the lens of bias before even diving into the available models or datasets - which behaviors of existing systems in the space will be particularly harmful or more likely to occur if bias is exacerbated by ML predictions?
We built a [tool](https://huggingface.co/spaces/hf-task-exploration/ExploreACMnaacl) to take users through these questions in another case of algorithmic content management: [hate speech detection in automatic content moderation](https://aclanthology.org/2022.hcinlp-1.2/). We found for example that looking through news and scientific articles that didn’t particularly focus on the ML part of the technology was already a great way to get a sense of where bias is already at play. Definitely go have a look for an example of how the models and datasets fit with the deployment context and how they can relate to known bias-related harms!
<p align="center">
<br>
<img src="https://huggingface.co/datasets/huggingface/documentation-images/resolve/main/blog/ethics_soc_2/img2.png" alt="Selection of tools developed by HF team members to address bias in ML" />
<em><a href="https://huggingface.co/spaces/hf-task-exploration/ExploreACMnaacl">ACM Task Exploration tool</a> by <a href="https://huggingface.co/aymm">Angie</a>, <a href="https://huggingface.co/paullada">Amandalynne</a>, and <a href="https://huggingface.co/yjernite">Yacine</a></em>
</p>
#### Task definition: recommendations
There are as many ways for the ML task definition and deployment to affect the risk of bias-related harms as there are applications for ML systems. As in the examples above, some common steps that may help decide whether and how to apply ML in a way that minimizes bias-related risk include:
* Investigate:
* Reports of bias in the field pre-ML
* At-risk demographic categories for your specific use case
* Examine:
* The impact of your optimization objective on reinforcing biases
* Alternative objectives that favor diversity and positive long-term impacts
### I am <span style="text-decoration:underline;">curating/picking a dataset</span> for my ML system, how can I address bias?
While training datasets are [not the sole source of bias](https://www.cell.com/patterns/fulltext/S2666-3899(21)00061-1) in the ML development cycle, they do play a significant role. Does your [dataset disproportionately associate](https://aclanthology.org/2020.emnlp-main.23/) biographies of women with life events but those of men with achievements? Those **stereotypes** are probably going to show up in your full ML system! Does your voice recognition dataset only feature specific accents? Not a good sign for [the inclusivity of technology](https://www.scientificamerican.com/article/speech-recognition-tech-is-yet-another-example-of-bias/) you build with it in terms of **disparate performance**! Whether you’re curating a dataset for ML applications or selecting a dataset to train an ML model, finding out, mitigating, and [communicating](https://dl.acm.org/doi/10.1145/3479582) to what extent the data exhibits these phenomena are all necessary steps to reducing bias-related risks.
You can usually get a pretty good sense of likely biases in a dataset by reflecting on where it comes from, who are the people represented on the data, and what the curation process was. Several frameworks for this reflection and documentation have been proposed such as [Data Statements for NLP](https://direct.mit.edu/tacl/article/doi/10.1162/tacl_a_00041/43452/Data-Statements-for-Natural-Language-Processing) or [Datasheets for Datasets](https://dl.acm.org/doi/10.1145/3458723). The Hugging Face Hub includes a Dataset Card [template](https://github.com/huggingface/datasets/blob/main/templates/README.md) and [guide](https://github.com/huggingface/datasets/blob/main/templates/README_guide.md#dataset-card-creation-guide) inspired by these works; the section on [considerations for using the data](https://github.com/huggingface/datasets/blob/main/templates/README_guide.md#considerations-for-using-the-data) is usually a good place to look for information about notable biases if you’re browsing datasets, or to write a paragraph sharing your insights on the topic if you’re sharing a new one. And if you’re looking for more inspiration on what to put there, check out these sections written by Hub users in the [BigLAM organization](https://huggingface.co/biglam) for historical datasets of [legal proceedings](https://huggingface.co/datasets/biglam/old_bailey_proceedings#social-impact-of-dataset), [image classification](https://huggingface.co/datasets/biglam/brill_iconclass#social-impact-of-dataset), and [newspapers](https://huggingface.co/datasets/biglam/bnl_newspapers1841-1879#social-impact-of-dataset).
<p align="center">
<br>
<img src="https://huggingface.co/datasets/huggingface/documentation-images/resolve/main/blog/ethics_soc_2/img3.png" alt="HF Dataset Card guide for the Social Impact and Bias Sections" />
<em><a href="https://github.com/huggingface/datasets/blob/main/templates/README_guide.md#social-impact-of-dataset">HF Dataset Card guide</a> for the Social Impact and Bias Sections</em>
</p>
While describing the origin and context of a dataset is always a good starting point to understand the biases at play, [quantitatively measuring phenomena](https://arxiv.org/abs/2212.05129) that encode those biases can be just as helpful. If you’re choosing between two different datasets for a given task or choosing between two ML models trained on different datasets, knowing which one better represents the demographic makeup of your ML system’s user base can help you make an informed decision to minimize bias-related risks. If you’re curating a dataset iteratively by filtering data points from a source or selecting new sources of data to add, measuring how these choices affect the diversity and biases present in your overall dataset can make it safer to use in general.
We’ve recently released two tools you can leverage to measure your data through a bias-informed lens. The [disaggregators🤗 library](https://github.com/huggingface/disaggregators) provides utilities to quantify the composition of your dataset, using either metadata or leveraging models to infer properties of data points. This can be particularly useful to minimize risks of bias-related **[representation harms](https://aclanthology.org/P16-2096/)** or **disparate performances** of trained models. Look at the [demo](https://huggingface.co/spaces/society-ethics/disaggregators) to see it applied to the LAION, MedMCQA, and The Stack datasets!
<p align="center">
<br>
<img src="https://huggingface.co/datasets/huggingface/documentation-images/resolve/main/blog/ethics_soc_2/img4.png" alt="Disaggregators tool by Nima" />
<em><a href="https://huggingface.co/spaces/society-ethics/disaggregators">Disaggregator tool</a> by <a href="https://huggingface.co/NimaBoscarino">Nima</a></em>
</p>
Once you have some helpful statistics about the composition of your dataset, you’ll also want to look at associations between features in your data items, particularly at associations that may encode derogatory or otherwise negative stereotypes. The Data Measurements Tool we [originally introduced](https://huggingface.co/blog/data-measurements-tool#comparison-statistics) last year allows you to do this by looking at the [normalized Pointwise Mutual Information (nPMI)](https://dl.acm.org/doi/10.1145/3461702.3462557) between terms in your text-based dataset; particularly associations between gendered pronouns that may denote gendered stereotypes. [Run it yourself](https://github.com/huggingface/data-measurements-tool) or [try it here](https://huggingface.co/spaces/huggingface/data-measurements-tool) on a few pre-computed datasets!
<p align="center">
<br>
<img src="https://huggingface.co/datasets/huggingface/documentation-images/resolve/main/blog/ethics_soc_2/img5.png" alt="Data Measurements tool by Meg, Sasha, Bibi, and the Gradio team" />
<em><a href="https://huggingface.co/spaces/huggingface/data-measurements-tool">Data Measurements tool</a> by <a href="https://huggingface.co/meg">Meg</a>, <a href="https://huggingface.co/sasha">Sasha</a>, <a href="https://huggingface.co/Bibss">Bibi</a>, and the <a href="https://gradio.app/">Gradio team</a></em>
</p>
#### Dataset selection/curation: recommendations
These tools aren’t full solutions by themselves, rather, they are designed to support critical examination and improvement of datasets through several lenses, including the lens of bias and bias-related risks. In general, we encourage you to keep the following steps in mind when leveraging these and other tools to mitigate bias risks at the dataset curation/selection stage:
* Identify:
* Aspects of the dataset creation that may exacerbate specific biases
* Demographic categories and social variables that are particularly important to the dataset’s task and domain
* Measure:
* The demographic distribution in your dataset
* Pre-identified negative stereotypes represented
* Document:
* Share what you’ve Identified and Measured in your Dataset Card so it can benefit other users, developers, and otherwise affected people
* Adapt:
* By choosing the dataset least likely to cause bias-related harms
* By iteratively improving your dataset in ways that reduce bias risks
### I am <span style="text-decoration:underline;">training/selecting a model</span> for my ML system, how can I address bias?
Similar to the dataset curation/selection step, documenting and measuring bias-related phenomena in models can help both ML developers who are selecting a model to use as-is or to finetune and ML developers who want to train their own models. For the latter, measures of bias-related phenomena in the model can help them learn from what has worked or what hasn’t for other models and serve as a signal to guide their own development choices.
Model cards were originally proposed by [(Mitchell et al., 2019)](https://dl.acm.org/doi/10.1145/3287560.3287596) and provide a framework for model reporting that showcases information relevant to bias risks, including broad ethical considerations, disaggregated evaluation, and use case recommendation. The Hugging Face Hub provides even more tools for model documentation, with a [model card guidebook](https://huggingface.co/docs/hub/model-cards) in the Hub documentation, and an [app that lets you create extensive model cards](https://huggingface.co/spaces/huggingface/Model_Cards_Writing_Tool) easily for your new model.
<p align="center">
<br>
<img src="https://huggingface.co/datasets/huggingface/documentation-images/resolve/main/blog/ethics_soc_2/img6.png" alt="Model Card writing tool by Ezi, Marissa, and Meg" />
<em><a href="https://huggingface.co/spaces/huggingface/Model_Cards_Writing_Tool">Model Card writing tool</a> by <a href="https://huggingface.co/Ezi">Ezi</a>, <a href="https://huggingface.co/Marissa">Marissa</a>, and <a href="https://huggingface.co/meg">Meg</a></em>
</p>
Documentation is a great first step for sharing general insights about a model’s behavior, but it is usually static and presents the same information to all users. In many cases, especially for generative models that can generate outputs to approximate the distribution of their training data, we can gain a more contextual understanding of bias-related phenomena and **negative stereotypes** by visualizing and contrasting model outputs. Access to model generations can help users bring [intersectional issues in the model behavior](https://www.technologyreview.com/2022/12/12/1064751/the-viral-ai-avatar-app-lensa-undressed-me-without-my-consent/) corresponding to their lived experience, and evaluate to what extent a model reproduces [gendered stereotypes for different adjectives](https://www.vice.com/en/article/bvm35w/this-tool-lets-anyone-see-the-bias-in-ai-image-generators). To facilitate this process, we built a tool that lets you compare generations not just across a set of adjectives and professions, but also across different models! [Go try it out](https://huggingface.co/spaces/society-ethics/DiffusionBiasExplorer) to get a sense of which model might carry the least bias risks in your use case.
<p align="center">
<br>
<img src="https://huggingface.co/datasets/huggingface/documentation-images/resolve/main/blog/ethics_soc_2/img7.png" alt="Visualize Adjective and Occupation Biases in Image Generation by Sasha" />
<br>
<em><a href="https://huggingface.co/spaces/society-ethics/DiffusionBiasExplorer">Visualize Adjective and Occupation Biases in Image Generation</a> by <a href="https://huggingface.co/sasha">Sasha</a></em>
</p>
Visualization of model outputs isn’t just for generative models though! For classification models, we also want to look out for bias-related harms caused by a model’s **disparate performance** on different demographics. If you know what protected classes are most at risk of discrimination and have those annotated in an evaluation set, then you can report disaggregated performance over the different categories in [your model card](https://dl.acm.org/doi/10.1145/3287560.3287596) as mentioned above, so users can make informed decisions. If however, you are worried that you haven’t identified all populations at risk of bias-related harms, or if you do not have access to annotated test examples to measure the biases you suspect, that’s where interactive visualizations of where and how the model fails come in handy! To help you with this, the [SEAL app](https://huggingface.co/spaces/nazneen/seal) groups similar mistakes by your model and shows you some common features in each cluster. If you want to go further, you can even combine it with the [disaggregators library](https://github.com/huggingface/disaggregators) we introduced in the datasets section to find clusters that are indicative of bias-related failure modes!
<p align="center">
<br>
<img src="https://huggingface.co/datasets/huggingface/documentation-images/resolve/main/blog/ethics_soc_2/img8.png" alt="Systematic Error Analysis and Labeling (SEAL) by Nazneen" />
<em><a href="https://huggingface.co/spaces/nazneen/seal">Systematic Error Analysis and Labeling (SEAL)</a> by <a href="https://huggingface.co/nazneen">Nazneen</a></em>
</p>
Finally, a few benchmarks exist that can measure bias-related phenomena in models. For language models, benchmarks such as [BOLD](https://github.com/amazon-science/bold), [HONEST](https://aclanthology.org/2021.naacl-main.191.pdf), or [WinoBias](https://aclanthology.org/N18-2003/) provide quantitative evaluations of targeted behaviors that are indicative of biases in the models. While the benchmarks have their [limitations](https://aclanthology.org/2021.acl-long.81/), they do provide a limited view into some pre-identified bias risks that can help describe how the models function or choose between different models. You can find these evaluations pre-computed on a range of common language models [in this exploration Space](https://huggingface.co/spaces/sasha/BiasDetection) to get a first sense of how they compare!
<p align="center">
<br>
<img src="https://huggingface.co/datasets/huggingface/documentation-images/resolve/main/blog/ethics_soc_2/img9.png" alt="Language Model Bias Detection by Sasha" />
<em><a href="https://huggingface.co/spaces/sasha/BiasDetection">Language Model Bias Detection</a> by <a href="https://huggingface.co/sasha">Sasha</a></em>
</p>
Even with access to a benchmark for the models you are considering, you might find that running evaluations of the larger language models you are considering can be prohibitively expensive or otherwise technically impossible with your own computing resources. The <a href="https://huggingface.co/spaces/autoevaluate/model-evaluator">Evaluation on the Hub</a> tool we released this year can help with that: not only will it run the evaluations for you, but it will also help connect them to the model documentation so the results are available once and for all — so everyone can see, for example, that size <a href="https://huggingface.co/blog/zero-shot-eval-on-the-hub">measurably increases bias risks in models like OPT</a>!
<p align="center">
<br>
<img src="https://huggingface.co/datasets/huggingface/documentation-images/resolve/main/blog/ethics_soc_2/img_winobias.png" alt="Large model WinoBias scores computed with Evaluation on the Hub by Helen, Tristan, Abhishek, Lewis, and Douwe" />
<em><a href="https://huggingface.co/spaces/sasha/BiasDetection"><a href="https://huggingface.co/blog/zero-shot-eval-on-the-hub">Large model WinoBias scores computed with Evaluation on the Hub</a> by <a href="https://huggingface.co/mathemakitten">Helen</a>, <a href="https://huggingface.co/Tristan">Tristan</a>, <a href="https://huggingface.co/abhishek">Abhishek</a>, <a href="https://huggingface.co/lewtun">Lewis</a>, and <a href="https://huggingface.co/douwekiela">Douwe</a></em>
</p>
#### Model selection/development: recommendations
For models just as for datasets, different tools for documentation and evaluation will provide different views of bias risks in a model which all have a part to play in helping developers choose, develop, or understand ML systems.
* Visualize
* Generative model: visualize how the model’s outputs may reflect stereotypes
* Classification model: visualize model errors to identify failure modes that could lead to disparate performance
* Evaluate
* When possible, evaluate models on relevant benchmarks
* Document
* Share your learnings from visualization and qualitative evaluation
* Report your model’s disaggregated performance and results on applicable fairness benchmarks
## Conclusion and Overview of Bias Analysis and Documentation Tools from 🤗
As we learn to leverage ML systems in more and more applications, reaping their benefits equitably will depend on our ability to actively mitigate the risks of bias-related harms associated with the technology. While there is no single answer to the question of how this should best be done in any possible setting, we can support each other in this effort by sharing lessons, tools, and methodologies to mitigate and document those risks. The present blog post outlines some of the ways Hugging Face team members have addressed this question of bias along with supporting tools, we hope that you will find them helpful and encourage you to develop and share your own!
Summary of linked tools:
* Tasks:
* Explore our directory of [ML Tasks](https://huggingface.co/tasks) to understand what technical framings and resources are available to choose from
* Use tools to explore the [full development lifecycle](https://huggingface.co/spaces/hf-task-exploration/ExploreACMnaacl) of specific tasks
* Datasets:
* Make use of and contribute to [Dataset Cards](https://github.com/huggingface/datasets/blob/main/templates/README_guide.md#social-impact-of-dataset) to share relevant insights on biases in datasets.
* Use [Disaggregator](https://github.com/huggingface/disaggregators) to look for [possible disparate performance](https://huggingface.co/spaces/society-ethics/disaggregators)
* Look at aggregated [measurements of your dataset](https://huggingface.co/spaces/huggingface/data-measurements-tool) including nPMI to surface possible stereotypical associations
* Models:
* Make use of and contribute to [Model Cards](https://huggingface.co/docs/hub/model-cards) to share relevant insights on biases in models.
* Use [Interactive Model Cards](https://huggingface.co/spaces/nazneen/interactive-model-cards) to visualize performance discrepancies
* Look at [systematic model errors](https://huggingface.co/spaces/nazneen/seal) and look out for known social biases
* Use [Evaluate](https://github.com/huggingface/evaluate) and [Evaluation on the Hub](https://huggingface.co/spaces/autoevaluate/model-evaluator) to explore [language model biases](https://huggingface.co/blog/evaluating-llm-bias) including in [large models](https://huggingface.co/blog/zero-shot-eval-on-the-hub)
* Use a [Text-to-image bias explorer](https://huggingface.co/spaces/sasha/StableDiffusionBiasExplorer) to compare image generation models’ biases
* Compare LM models with Bias [Score Card](https://huggingface.co/spaces/sasha/BiasDetection)
Thanks for reading! 🤗
~ Yacine, on behalf of the Ethics and Society regulars
Cite as:
```
@inproceedings{hf_ethics_soc_blog_2,
author = {Yacine Jernite and
Alexandra Sasha Luccioni and
Irene Soleiman and
Giada Pistilli and
Nathan Lambert and
Ezi Ozoani and
Brigitte Toussignant and
Margaret Mitchell},
title = {Hugging Face Ethics and Society Newsletter 2: Let's Talk about Bias!},
booktitle = {Hugging Face Blog},
year = {2022},
url = {https://doi.org/10.57967/hf/0208},
doi = {10.57967/hf/0208}
}
``` | yjernite/EthicsSocietyBlogBias | [
"license:cc-by-4.0",
"arxiv:2203.07785",
"arxiv:2010.03058",
"arxiv:2211.03759",
"arxiv:2209.03942",
"arxiv:2212.05129",
"doi:10.57967/hf/0208",
"region:us"
] | 2022-12-15T21:30:38+00:00 | {"license": "cc-by-4.0"} | 2022-12-15T22:05:09+00:00 | [
"2203.07785",
"2010.03058",
"2211.03759",
"2209.03942",
"2212.05129"
] | [] | TAGS
#license-cc-by-4.0 #arxiv-2203.07785 #arxiv-2010.03058 #arxiv-2211.03759 #arxiv-2209.03942 #arxiv-2212.05129 #doi-10.57967/hf/0208 #region-us
|
This post was originally published on the Hugging Face blog
# Ethics and Society Newsletter #2
## Let’s Talk about Bias!
_Bias in ML is ubiquitous, and Bias in ML is complex; so complex in fact that no single technical intervention is likely to meaningfully address the problems it engenders. ML models, as sociotechnical systems, amplify social trends that may exacerbate inequities and harmful biases in ways that depend on their deployment context and are constantly evolving._
_This means that developing ML systems with care requires vigilance and responding to feedback from those deployment contexts, which in turn we can facilitate by sharing lessons across contexts and developing tools to analyze signs of bias at every level of ML development._
_This blog post from the Ethics and Society regulars @ shares some of the lessons we have learned along with tools we have developed to support ourselves and others in our community’s efforts to better address bias in Machine Learning. The first part is a broader reflection on bias and its context. If you’ve already read it and are coming back specifically for the tools, feel free to jump to the datasets or models
section!_
<p align="center">
<br>
<img src="URL alt="Selection of tools developed by HF team members to address bias in ML" />
<em>Selection of tools developed by team members to address bias in ML</em>
</p>
<span style="text-decoration:underline;">Table of contents:</span>
* <span style="text-decoration:underline;">On Machine Biases</span>
* Machine Bias: from ML Systems to Risks
* Putting Bias in Context
* <span style="text-decoration:underline;">Tools and Recommendations</span>
* Addressing Bias throughout ML Development
* Task Definition
* Dataset Curation
* Model Training
* Overview of Bias Tools
## _Machine Bias:_ from ML Systems to Personal and Social Risks
ML systems allow us to automate complex tasks at a scale never seen before as they are deployed in more sectors and use cases. When the technology works at its best, it can help smooth interactions between people and technical systems, remove the need for highly repetitive work, or unlock new ways of processing information to support research.
These same systems are also likely to reproduce discriminatory and abusive behaviors represented in their training data, especially when the data encodes human behaviors.
The technology then has the potential to make these issues significantly worse. Automation and deployment at scale can indeed:
1. lock in behaviors in time and hinder social progress from being reflected in technology,
2. spread harmful behaviors beyond the context of the original training data,
3. amplify inequities by overfocusing on stereotypical associations when making predictions,
4. remove possibilities for recourse by hiding biases inside “black-box” systems.
In order to better understand and address these risks, ML researchers and developers have started studying _machine bias_ or _algorithmic bias_, mechanisms that might lead systems to, for example, encode negative stereotypes or associations or to have disparate performance for different population groups in their deployment context.
These issues are deeply personal for many of us ML researchers and developers at Hugging Face and in the broader ML community. Hugging Face is an international company, with many of us existing between countries and cultures. It is hard to fully express our sense of urgency when we see the technology we work on developed without sufficient concern for protecting people like us; especially when these systems lead to discriminatory wrongful arrests or undue financial distress and are being increasingly sold to immigration and law enforcement services around the world. Similarly, seeing our identities routinely suppressed in training datasets or underrepresented in the outputs of “generative AI” systems connects these concerns to our daily lived experiences in ways that are simultaneously enlightening and taxing.
While our own experiences do not come close to covering the myriad ways in which ML-mediated discrimination can disproportionately harm people whose experiences differ from ours, they provide an entry point into considerations of the trade-offs inherent in the technology. We work on these systems because we strongly believe in ML’s potential — we think it can shine as a valuable tool as long as it is developed with care and input from people in its deployment context, rather than as a one-size-fits-all panacea. In particular, enabling this care requires developing a better understanding of the mechanisms of machine bias across the ML development process, and developing tools that support people with all levels of technical knowledge of these systems in participating in the necessary conversations about how their benefits and harms are distributed.
The present blog post from the Hugging Face Ethics and Society regulars provides an overview of how we have worked, are working, or recommend users of the HF ecosystem of libraries may work to address bias at the various stages of the ML development process, and the tools we develop to support this process. We hope you will find it a useful resource to guide concrete considerations of the social impact of your work and can leverage the tools referenced here to help mitigate these issues when they arise.
## Putting Bias in Context
The first and maybe most important concept to consider when dealing with machine bias is context. In their foundational work on bias in NLP, Su Lin Blodgett et al. point out that: _“[T]he majority of [academic works on machine bias] fail to engage critically with what constitutes “bias” in the first place”_, including by building their work on top of _“unstated assumptions about what kinds of system behaviors are harmful, in what ways, to whom, and why”_.
This may not come as much of a surprise given the ML research community’s focus on the value of “generalization” — the most cited motivation for work in the field after “performance”. However, while tools for bias assessment that apply to a wide range of settings are valuable to enable a broader analysis of common trends in model behaviors, their ability to target the mechanisms that lead to discrimination in concrete use cases is inherently limited. Using them to guide specific decisions within the ML development cycle usually requires an extra step or two to take the system’s specific use context and affected people into consideration.
<p align="center">
<br>
<img src="URL alt="Excerpt on considerations of ML uses context and people from the Model Card Guidebook" />
<em>Excerpt on considerations of ML uses context and people from the <a href="URL Card Guidebook</a></em>
</p>
Now let’s dive deeper into the issue of linking biases in stand-alone/context-less ML artifacts to specific harms. It can be useful to think of machine biases as risk factors for discrimination-based harms. Take the example of a text-to-image model that over-represents light skin tones when prompted to create a picture of a person in a professional setting, but produces darker skin tones when the prompts mention criminality. These tendencies would be what we call _machine biases at the model level_. Now let’s think about a few systems that use such a text-to-image model:
1. <span style="text-decoration:underline;">The model is integrated into a website creation service</span> (e.g. SquareSpace, Wix) to help users generate backgrounds for their pages. The model explicitly disables images of people in the generated background.
* In this case, the machine bias “risk factor” does not lead to discrimination harm because the focus of the bias (images of people) is absent from the use case.
* Further risk mitigation is not required for machine biases, although developers should be aware of ongoing discussions about the legality of integrating systems trained on scraped data in commercial systems.
2. <span style="text-decoration:underline;">The model is integrated into a stock images website</span> to provide users with synthetic images of people (e.g. in professional settings) that they can use with fewer privacy concerns, for example, to serve as illustrations for Wikipedia articles
* In this case, machine bias acts to lock in and amplify existing social biases. It reinforces stereotypes about people (“CEOs are all white men”) that then feed back into complex social systems where increased bias leads to increased discrimination in many different ways (such as reinforcing implicit bias in the workplace).
* Mitigation strategies may include educating the stock image users about these biases, or the stock image website may curate generated images to intentionally propose a more diverse set of representations.
3. <span style="text-decoration:underline;">The model is integrated into a “virtual sketch artist” software</span> marketed to police departments that will use it to generate pictures of suspects based on verbal testimony
* In this case, the machine biases directly cause discrimination by systematically directing police departments to darker-skinned people, putting them at increased risk of harm including physical injury and unlawful imprisonment.
* In cases like this one, there may be no level of bias mitigation that makes the risk acceptable. In particular, such a use case would be closely related to face recognition in the context of law enforcement, where similar bias issues have led several commercial entities and legislatures to adopt moratoria pausing or banning its use across the board.
So, who’s on the hook for machine biases in ML? These three cases illustrate one of the reasons why discussions about the responsibility of ML developers in addressing bias can get so complicated: depending on decisions made at other points in the ML system development process by other people, the biases in an ML dataset or model may land anywhere between being irrelevant to the application settings and directly leading to grievous harm. However, in all of these cases, stronger biases in the model/dataset increase the risk of negative outcomes. The European Union has started to develop frameworks that address this phenomenon in recent regulatory efforts: in short, a company that deploys an AI system based on a measurably biased model is liable for harm caused by the system.
Conceptualizing bias as a risk factor then allows us to better understand the shared responsibility for machine biases between developers at all stages. Bias can never be fully removed, not least because the definitions of social biases and the power dynamics that tie them to discrimination vary vastly across social contexts. However:
1. Each stage of the development process, from task specification, dataset curation, and model training, to model integration and system deployment, can take steps to minimize the aspects of machine bias that most directly depend on its choices and technical decisions, and
2. Clear communication and information flow between the various ML development stages can make the difference between making choices that build on top of each other to attenuate the negative potential of bias (multipronged approach to bias mitigation, as in deployment scenario 1 above) _versus_ making choices that compound this negative potential to exacerbate the risk of harm (as in deployment scenario 3).
In the next section, we review these various stages along with some of the tools that can help us address machine bias at each of them.
## Addressing Bias throughout the ML Development Cycle
Ready for some practical advice yet? Here we go
There is no one single way to develop ML systems; which steps happen in what order depends on a number of factors including the development setting (university, large company, startup, grassroots organization, etc…), the modality (text, tabular data, images, etc…), and the preeminence or scarcity of publicly available ML resources. However, we can identify three common stages of particular interest in addressing bias. These are the task definition, the data curation, and the model training. Let’s have a look at how bias handling may differ across these various stages.
<p align="center">
<br>
<img src="URL alt="The Bias ML Pipeline by Meg" width="500" />
<em>The Bias ML Pipeline by <a href="URL
</p>
### I am <span style="text-decoration:underline;">defining the task</span> of my ML system, how can I address bias?
Whether and to what extent bias in the system concretely affects people ultimately depends on what the system is used for. As such, the first place developers can work to mitigate bias is when deciding how ML fits in their system, e.g., by deciding what optimization objective it will use.
For example, let’s go back to one of the first highly-publicized cases of a Machine Learning system used in production for algorithmic content recommendation. From 2006 to 2009, Netflix ran the Netflix Prize, a competition with a 1M$ cash prize challenging teams around the world to develop ML systems to accurately predict a user’s rating for a new movie based on their past ratings. The winning submission improved the RMSE (Root-mean-square-error) of predictions on unseen user-movie pairs by over 10% over Netflix’s own CineMatch algorithm, meaning it got much better at predicting how users would rate a new movie based on their history. This approach opened the door for much of modern algorithmic content recommendation by bringing the role of ML in modeling user preferences in recommender systems to public awareness.
So what does this have to do with bias? Doesn’t showing people content that they’re likely to enjoy sound like a good service from a content platform? Well, it turns out that showing people more examples of what they’ve liked in the past ends up reducing the diversity of the media they consume. Not only does it lead users to be less satisfied in the long term, but it also means that any biases or stereotypes captured by the initial models — such as when modeling the preferences of Black American users or dynamics that systematically disadvantage some artists — are likely to be reinforced if the model is further trained on ongoing ML-mediated user interactions. This reflects two of the types of bias-related concerns we’ve mentioned above: the training objective acts as a risk factor for bias-related harms as it makes pre-existing biases much more likely to show up in predictions, and the task framing has the effect of locking in and exacerbating past biases.
A promising bias mitigation strategy at this stage has been to reframe the task to explicitly model both engagement and diversity when applying ML to algorithmic content recommendation. Users are likely to get more long-term satisfaction and the risk of exacerbating biases as outlined above is reduced!
This example serves to illustrate that the impact of machine biases in an ML-supported product depends not just on where we decide to leverage ML, but also on how ML techniques are integrated into the broader technical system, and with what objective. When first investigating how ML can fit into a product or a use case you are interested in, we first recommend looking for the failure modes of the system through the lens of bias before even diving into the available models or datasets - which behaviors of existing systems in the space will be particularly harmful or more likely to occur if bias is exacerbated by ML predictions?
We built a tool to take users through these questions in another case of algorithmic content management: hate speech detection in automatic content moderation. We found for example that looking through news and scientific articles that didn’t particularly focus on the ML part of the technology was already a great way to get a sense of where bias is already at play. Definitely go have a look for an example of how the models and datasets fit with the deployment context and how they can relate to known bias-related harms!
<p align="center">
<br>
<img src="URL alt="Selection of tools developed by HF team members to address bias in ML" />
<em><a href="URL Task Exploration tool</a> by <a href="URL <a href="URL and <a href="URL
</p>
#### Task definition: recommendations
There are as many ways for the ML task definition and deployment to affect the risk of bias-related harms as there are applications for ML systems. As in the examples above, some common steps that may help decide whether and how to apply ML in a way that minimizes bias-related risk include:
* Investigate:
* Reports of bias in the field pre-ML
* At-risk demographic categories for your specific use case
* Examine:
* The impact of your optimization objective on reinforcing biases
* Alternative objectives that favor diversity and positive long-term impacts
### I am <span style="text-decoration:underline;">curating/picking a dataset</span> for my ML system, how can I address bias?
While training datasets are not the sole source of bias00061-1) in the ML development cycle, they do play a significant role. Does your dataset disproportionately associate biographies of women with life events but those of men with achievements? Those stereotypes are probably going to show up in your full ML system! Does your voice recognition dataset only feature specific accents? Not a good sign for the inclusivity of technology you build with it in terms of disparate performance! Whether you’re curating a dataset for ML applications or selecting a dataset to train an ML model, finding out, mitigating, and communicating to what extent the data exhibits these phenomena are all necessary steps to reducing bias-related risks.
You can usually get a pretty good sense of likely biases in a dataset by reflecting on where it comes from, who are the people represented on the data, and what the curation process was. Several frameworks for this reflection and documentation have been proposed such as Data Statements for NLP or Datasheets for Datasets. The Hugging Face Hub includes a Dataset Card template and guide inspired by these works; the section on considerations for using the data is usually a good place to look for information about notable biases if you’re browsing datasets, or to write a paragraph sharing your insights on the topic if you’re sharing a new one. And if you’re looking for more inspiration on what to put there, check out these sections written by Hub users in the BigLAM organization for historical datasets of legal proceedings, image classification, and newspapers.
<p align="center">
<br>
<img src="URL alt="HF Dataset Card guide for the Social Impact and Bias Sections" />
<em><a href="URL Dataset Card guide</a> for the Social Impact and Bias Sections</em>
</p>
While describing the origin and context of a dataset is always a good starting point to understand the biases at play, quantitatively measuring phenomena that encode those biases can be just as helpful. If you’re choosing between two different datasets for a given task or choosing between two ML models trained on different datasets, knowing which one better represents the demographic makeup of your ML system’s user base can help you make an informed decision to minimize bias-related risks. If you’re curating a dataset iteratively by filtering data points from a source or selecting new sources of data to add, measuring how these choices affect the diversity and biases present in your overall dataset can make it safer to use in general.
We’ve recently released two tools you can leverage to measure your data through a bias-informed lens. The disaggregators library provides utilities to quantify the composition of your dataset, using either metadata or leveraging models to infer properties of data points. This can be particularly useful to minimize risks of bias-related representation harms or disparate performances of trained models. Look at the demo to see it applied to the LAION, MedMCQA, and The Stack datasets!
<p align="center">
<br>
<img src="URL alt="Disaggregators tool by Nima" />
<em><a href="URL tool</a> by <a href="URL
</p>
Once you have some helpful statistics about the composition of your dataset, you’ll also want to look at associations between features in your data items, particularly at associations that may encode derogatory or otherwise negative stereotypes. The Data Measurements Tool we originally introduced last year allows you to do this by looking at the normalized Pointwise Mutual Information (nPMI) between terms in your text-based dataset; particularly associations between gendered pronouns that may denote gendered stereotypes. Run it yourself or try it here on a few pre-computed datasets!
<p align="center">
<br>
<img src="URL alt="Data Measurements tool by Meg, Sasha, Bibi, and the Gradio team" />
<em><a href="URL Measurements tool</a> by <a href="URL <a href="URL <a href="URL and the <a href="URL team</a></em>
</p>
#### Dataset selection/curation: recommendations
These tools aren’t full solutions by themselves, rather, they are designed to support critical examination and improvement of datasets through several lenses, including the lens of bias and bias-related risks. In general, we encourage you to keep the following steps in mind when leveraging these and other tools to mitigate bias risks at the dataset curation/selection stage:
* Identify:
* Aspects of the dataset creation that may exacerbate specific biases
* Demographic categories and social variables that are particularly important to the dataset’s task and domain
* Measure:
* The demographic distribution in your dataset
* Pre-identified negative stereotypes represented
* Document:
* Share what you’ve Identified and Measured in your Dataset Card so it can benefit other users, developers, and otherwise affected people
* Adapt:
* By choosing the dataset least likely to cause bias-related harms
* By iteratively improving your dataset in ways that reduce bias risks
### I am <span style="text-decoration:underline;">training/selecting a model</span> for my ML system, how can I address bias?
Similar to the dataset curation/selection step, documenting and measuring bias-related phenomena in models can help both ML developers who are selecting a model to use as-is or to finetune and ML developers who want to train their own models. For the latter, measures of bias-related phenomena in the model can help them learn from what has worked or what hasn’t for other models and serve as a signal to guide their own development choices.
Model cards were originally proposed by (Mitchell et al., 2019) and provide a framework for model reporting that showcases information relevant to bias risks, including broad ethical considerations, disaggregated evaluation, and use case recommendation. The Hugging Face Hub provides even more tools for model documentation, with a model card guidebook in the Hub documentation, and an app that lets you create extensive model cards easily for your new model.
<p align="center">
<br>
<img src="URL alt="Model Card writing tool by Ezi, Marissa, and Meg" />
<em><a href="URL Card writing tool</a> by <a href="URL <a href="URL and <a href="URL
</p>
Documentation is a great first step for sharing general insights about a model’s behavior, but it is usually static and presents the same information to all users. In many cases, especially for generative models that can generate outputs to approximate the distribution of their training data, we can gain a more contextual understanding of bias-related phenomena and negative stereotypes by visualizing and contrasting model outputs. Access to model generations can help users bring intersectional issues in the model behavior corresponding to their lived experience, and evaluate to what extent a model reproduces gendered stereotypes for different adjectives. To facilitate this process, we built a tool that lets you compare generations not just across a set of adjectives and professions, but also across different models! Go try it out to get a sense of which model might carry the least bias risks in your use case.
<p align="center">
<br>
<img src="URL alt="Visualize Adjective and Occupation Biases in Image Generation by Sasha" />
<br>
<em><a href="URL Adjective and Occupation Biases in Image Generation</a> by <a href="URL
</p>
Visualization of model outputs isn’t just for generative models though! For classification models, we also want to look out for bias-related harms caused by a model’s disparate performance on different demographics. If you know what protected classes are most at risk of discrimination and have those annotated in an evaluation set, then you can report disaggregated performance over the different categories in your model card as mentioned above, so users can make informed decisions. If however, you are worried that you haven’t identified all populations at risk of bias-related harms, or if you do not have access to annotated test examples to measure the biases you suspect, that’s where interactive visualizations of where and how the model fails come in handy! To help you with this, the SEAL app groups similar mistakes by your model and shows you some common features in each cluster. If you want to go further, you can even combine it with the disaggregators library we introduced in the datasets section to find clusters that are indicative of bias-related failure modes!
<p align="center">
<br>
<img src="URL alt="Systematic Error Analysis and Labeling (SEAL) by Nazneen" />
<em><a href="URL Error Analysis and Labeling (SEAL)</a> by <a href="URL
</p>
Finally, a few benchmarks exist that can measure bias-related phenomena in models. For language models, benchmarks such as BOLD, HONEST, or WinoBias provide quantitative evaluations of targeted behaviors that are indicative of biases in the models. While the benchmarks have their limitations, they do provide a limited view into some pre-identified bias risks that can help describe how the models function or choose between different models. You can find these evaluations pre-computed on a range of common language models in this exploration Space to get a first sense of how they compare!
<p align="center">
<br>
<img src="URL alt="Language Model Bias Detection by Sasha" />
<em><a href="URL Model Bias Detection</a> by <a href="URL
</p>
Even with access to a benchmark for the models you are considering, you might find that running evaluations of the larger language models you are considering can be prohibitively expensive or otherwise technically impossible with your own computing resources. The <a href="URL on the Hub</a> tool we released this year can help with that: not only will it run the evaluations for you, but it will also help connect them to the model documentation so the results are available once and for all — so everyone can see, for example, that size <a href="URL increases bias risks in models like OPT</a>!
<p align="center">
<br>
<img src="URL alt="Large model WinoBias scores computed with Evaluation on the Hub by Helen, Tristan, Abhishek, Lewis, and Douwe" />
<em><a href="URL href="URL model WinoBias scores computed with Evaluation on the Hub</a> by <a href="URL <a href="URL <a href="URL <a href="URL and <a href="URL
</p>
#### Model selection/development: recommendations
For models just as for datasets, different tools for documentation and evaluation will provide different views of bias risks in a model which all have a part to play in helping developers choose, develop, or understand ML systems.
* Visualize
* Generative model: visualize how the model’s outputs may reflect stereotypes
* Classification model: visualize model errors to identify failure modes that could lead to disparate performance
* Evaluate
* When possible, evaluate models on relevant benchmarks
* Document
* Share your learnings from visualization and qualitative evaluation
* Report your model’s disaggregated performance and results on applicable fairness benchmarks
## Conclusion and Overview of Bias Analysis and Documentation Tools from
As we learn to leverage ML systems in more and more applications, reaping their benefits equitably will depend on our ability to actively mitigate the risks of bias-related harms associated with the technology. While there is no single answer to the question of how this should best be done in any possible setting, we can support each other in this effort by sharing lessons, tools, and methodologies to mitigate and document those risks. The present blog post outlines some of the ways Hugging Face team members have addressed this question of bias along with supporting tools, we hope that you will find them helpful and encourage you to develop and share your own!
Summary of linked tools:
* Tasks:
* Explore our directory of ML Tasks to understand what technical framings and resources are available to choose from
* Use tools to explore the full development lifecycle of specific tasks
* Datasets:
* Make use of and contribute to Dataset Cards to share relevant insights on biases in datasets.
* Use Disaggregator to look for possible disparate performance
* Look at aggregated measurements of your dataset including nPMI to surface possible stereotypical associations
* Models:
* Make use of and contribute to Model Cards to share relevant insights on biases in models.
* Use Interactive Model Cards to visualize performance discrepancies
* Look at systematic model errors and look out for known social biases
* Use Evaluate and Evaluation on the Hub to explore language model biases including in large models
* Use a Text-to-image bias explorer to compare image generation models’ biases
* Compare LM models with Bias Score Card
Thanks for reading!
~ Yacine, on behalf of the Ethics and Society regulars
Cite as:
| [
"# Ethics and Society Newsletter #2",
"## Let’s Talk about Bias!\n\n_Bias in ML is ubiquitous, and Bias in ML is complex; so complex in fact that no single technical intervention is likely to meaningfully address the problems it engenders. ML models, as sociotechnical systems, amplify social trends that may exacerbate inequities and harmful biases in ways that depend on their deployment context and are constantly evolving._\n\n_This means that developing ML systems with care requires vigilance and responding to feedback from those deployment contexts, which in turn we can facilitate by sharing lessons across contexts and developing tools to analyze signs of bias at every level of ML development._\n\n_This blog post from the Ethics and Society regulars @ shares some of the lessons we have learned along with tools we have developed to support ourselves and others in our community’s efforts to better address bias in Machine Learning. The first part is a broader reflection on bias and its context. If you’ve already read it and are coming back specifically for the tools, feel free to jump to the datasets or models\nsection!_\n\n<p align=\"center\">\n <br>\n <img src=\"URL alt=\"Selection of tools developed by HF team members to address bias in ML\" />\n <em>Selection of tools developed by team members to address bias in ML</em>\n</p>\n\n<span style=\"text-decoration:underline;\">Table of contents:</span>\n* <span style=\"text-decoration:underline;\">On Machine Biases</span>\n * Machine Bias: from ML Systems to Risks\n * Putting Bias in Context\n* <span style=\"text-decoration:underline;\">Tools and Recommendations</span>\n * Addressing Bias throughout ML Development\n * Task Definition\n * Dataset Curation\n * Model Training\n * Overview of Bias Tools",
"## _Machine Bias:_ from ML Systems to Personal and Social Risks\n\nML systems allow us to automate complex tasks at a scale never seen before as they are deployed in more sectors and use cases. When the technology works at its best, it can help smooth interactions between people and technical systems, remove the need for highly repetitive work, or unlock new ways of processing information to support research.\n\nThese same systems are also likely to reproduce discriminatory and abusive behaviors represented in their training data, especially when the data encodes human behaviors.\nThe technology then has the potential to make these issues significantly worse. Automation and deployment at scale can indeed:\n\n1. lock in behaviors in time and hinder social progress from being reflected in technology, \n2. spread harmful behaviors beyond the context of the original training data,\n3. amplify inequities by overfocusing on stereotypical associations when making predictions,\n4. remove possibilities for recourse by hiding biases inside “black-box” systems.\n\nIn order to better understand and address these risks, ML researchers and developers have started studying _machine bias_ or _algorithmic bias_, mechanisms that might lead systems to, for example, encode negative stereotypes or associations or to have disparate performance for different population groups in their deployment context.\n\nThese issues are deeply personal for many of us ML researchers and developers at Hugging Face and in the broader ML community. Hugging Face is an international company, with many of us existing between countries and cultures. It is hard to fully express our sense of urgency when we see the technology we work on developed without sufficient concern for protecting people like us; especially when these systems lead to discriminatory wrongful arrests or undue financial distress and are being increasingly sold to immigration and law enforcement services around the world. Similarly, seeing our identities routinely suppressed in training datasets or underrepresented in the outputs of “generative AI” systems connects these concerns to our daily lived experiences in ways that are simultaneously enlightening and taxing.\n\nWhile our own experiences do not come close to covering the myriad ways in which ML-mediated discrimination can disproportionately harm people whose experiences differ from ours, they provide an entry point into considerations of the trade-offs inherent in the technology. We work on these systems because we strongly believe in ML’s potential — we think it can shine as a valuable tool as long as it is developed with care and input from people in its deployment context, rather than as a one-size-fits-all panacea. In particular, enabling this care requires developing a better understanding of the mechanisms of machine bias across the ML development process, and developing tools that support people with all levels of technical knowledge of these systems in participating in the necessary conversations about how their benefits and harms are distributed.\n\nThe present blog post from the Hugging Face Ethics and Society regulars provides an overview of how we have worked, are working, or recommend users of the HF ecosystem of libraries may work to address bias at the various stages of the ML development process, and the tools we develop to support this process. We hope you will find it a useful resource to guide concrete considerations of the social impact of your work and can leverage the tools referenced here to help mitigate these issues when they arise.",
"## Putting Bias in Context\n\nThe first and maybe most important concept to consider when dealing with machine bias is context. In their foundational work on bias in NLP, Su Lin Blodgett et al. point out that: _“[T]he majority of [academic works on machine bias] fail to engage critically with what constitutes “bias” in the first place”_, including by building their work on top of _“unstated assumptions about what kinds of system behaviors are harmful, in what ways, to whom, and why”_.\n\nThis may not come as much of a surprise given the ML research community’s focus on the value of “generalization” — the most cited motivation for work in the field after “performance”. However, while tools for bias assessment that apply to a wide range of settings are valuable to enable a broader analysis of common trends in model behaviors, their ability to target the mechanisms that lead to discrimination in concrete use cases is inherently limited. Using them to guide specific decisions within the ML development cycle usually requires an extra step or two to take the system’s specific use context and affected people into consideration.\n\n<p align=\"center\">\n <br>\n <img src=\"URL alt=\"Excerpt on considerations of ML uses context and people from the Model Card Guidebook\" />\n <em>Excerpt on considerations of ML uses context and people from the <a href=\"URL Card Guidebook</a></em>\n</p>\n\nNow let’s dive deeper into the issue of linking biases in stand-alone/context-less ML artifacts to specific harms. It can be useful to think of machine biases as risk factors for discrimination-based harms. Take the example of a text-to-image model that over-represents light skin tones when prompted to create a picture of a person in a professional setting, but produces darker skin tones when the prompts mention criminality. These tendencies would be what we call _machine biases at the model level_. Now let’s think about a few systems that use such a text-to-image model:\n\n\n1. <span style=\"text-decoration:underline;\">The model is integrated into a website creation service</span> (e.g. SquareSpace, Wix) to help users generate backgrounds for their pages. The model explicitly disables images of people in the generated background.\n * In this case, the machine bias “risk factor” does not lead to discrimination harm because the focus of the bias (images of people) is absent from the use case.\n * Further risk mitigation is not required for machine biases, although developers should be aware of ongoing discussions about the legality of integrating systems trained on scraped data in commercial systems.\n2. <span style=\"text-decoration:underline;\">The model is integrated into a stock images website</span> to provide users with synthetic images of people (e.g. in professional settings) that they can use with fewer privacy concerns, for example, to serve as illustrations for Wikipedia articles\n * In this case, machine bias acts to lock in and amplify existing social biases. It reinforces stereotypes about people (“CEOs are all white men”) that then feed back into complex social systems where increased bias leads to increased discrimination in many different ways (such as reinforcing implicit bias in the workplace).\n * Mitigation strategies may include educating the stock image users about these biases, or the stock image website may curate generated images to intentionally propose a more diverse set of representations.\n3. <span style=\"text-decoration:underline;\">The model is integrated into a “virtual sketch artist” software</span> marketed to police departments that will use it to generate pictures of suspects based on verbal testimony\n * In this case, the machine biases directly cause discrimination by systematically directing police departments to darker-skinned people, putting them at increased risk of harm including physical injury and unlawful imprisonment.\n * In cases like this one, there may be no level of bias mitigation that makes the risk acceptable. In particular, such a use case would be closely related to face recognition in the context of law enforcement, where similar bias issues have led several commercial entities and legislatures to adopt moratoria pausing or banning its use across the board.\n\nSo, who’s on the hook for machine biases in ML? These three cases illustrate one of the reasons why discussions about the responsibility of ML developers in addressing bias can get so complicated: depending on decisions made at other points in the ML system development process by other people, the biases in an ML dataset or model may land anywhere between being irrelevant to the application settings and directly leading to grievous harm. However, in all of these cases, stronger biases in the model/dataset increase the risk of negative outcomes. The European Union has started to develop frameworks that address this phenomenon in recent regulatory efforts: in short, a company that deploys an AI system based on a measurably biased model is liable for harm caused by the system.\n\nConceptualizing bias as a risk factor then allows us to better understand the shared responsibility for machine biases between developers at all stages. Bias can never be fully removed, not least because the definitions of social biases and the power dynamics that tie them to discrimination vary vastly across social contexts. However:\n\n\n\n1. Each stage of the development process, from task specification, dataset curation, and model training, to model integration and system deployment, can take steps to minimize the aspects of machine bias that most directly depend on its choices and technical decisions, and\n2. Clear communication and information flow between the various ML development stages can make the difference between making choices that build on top of each other to attenuate the negative potential of bias (multipronged approach to bias mitigation, as in deployment scenario 1 above) _versus_ making choices that compound this negative potential to exacerbate the risk of harm (as in deployment scenario 3).\n\nIn the next section, we review these various stages along with some of the tools that can help us address machine bias at each of them.",
"## Addressing Bias throughout the ML Development Cycle\n\nReady for some practical advice yet? Here we go \n\nThere is no one single way to develop ML systems; which steps happen in what order depends on a number of factors including the development setting (university, large company, startup, grassroots organization, etc…), the modality (text, tabular data, images, etc…), and the preeminence or scarcity of publicly available ML resources. However, we can identify three common stages of particular interest in addressing bias. These are the task definition, the data curation, and the model training. Let’s have a look at how bias handling may differ across these various stages.\n\n<p align=\"center\">\n <br>\n <img src=\"URL alt=\"The Bias ML Pipeline by Meg\" width=\"500\" />\n <em>The Bias ML Pipeline by <a href=\"URL\n</p>",
"### I am <span style=\"text-decoration:underline;\">defining the task</span> of my ML system, how can I address bias?\n\nWhether and to what extent bias in the system concretely affects people ultimately depends on what the system is used for. As such, the first place developers can work to mitigate bias is when deciding how ML fits in their system, e.g., by deciding what optimization objective it will use.\n\nFor example, let’s go back to one of the first highly-publicized cases of a Machine Learning system used in production for algorithmic content recommendation. From 2006 to 2009, Netflix ran the Netflix Prize, a competition with a 1M$ cash prize challenging teams around the world to develop ML systems to accurately predict a user’s rating for a new movie based on their past ratings. The winning submission improved the RMSE (Root-mean-square-error) of predictions on unseen user-movie pairs by over 10% over Netflix’s own CineMatch algorithm, meaning it got much better at predicting how users would rate a new movie based on their history. This approach opened the door for much of modern algorithmic content recommendation by bringing the role of ML in modeling user preferences in recommender systems to public awareness.\n\nSo what does this have to do with bias? Doesn’t showing people content that they’re likely to enjoy sound like a good service from a content platform? Well, it turns out that showing people more examples of what they’ve liked in the past ends up reducing the diversity of the media they consume. Not only does it lead users to be less satisfied in the long term, but it also means that any biases or stereotypes captured by the initial models — such as when modeling the preferences of Black American users or dynamics that systematically disadvantage some artists — are likely to be reinforced if the model is further trained on ongoing ML-mediated user interactions. This reflects two of the types of bias-related concerns we’ve mentioned above: the training objective acts as a risk factor for bias-related harms as it makes pre-existing biases much more likely to show up in predictions, and the task framing has the effect of locking in and exacerbating past biases.\n\nA promising bias mitigation strategy at this stage has been to reframe the task to explicitly model both engagement and diversity when applying ML to algorithmic content recommendation. Users are likely to get more long-term satisfaction and the risk of exacerbating biases as outlined above is reduced!\n\nThis example serves to illustrate that the impact of machine biases in an ML-supported product depends not just on where we decide to leverage ML, but also on how ML techniques are integrated into the broader technical system, and with what objective. When first investigating how ML can fit into a product or a use case you are interested in, we first recommend looking for the failure modes of the system through the lens of bias before even diving into the available models or datasets - which behaviors of existing systems in the space will be particularly harmful or more likely to occur if bias is exacerbated by ML predictions?\n\nWe built a tool to take users through these questions in another case of algorithmic content management: hate speech detection in automatic content moderation. We found for example that looking through news and scientific articles that didn’t particularly focus on the ML part of the technology was already a great way to get a sense of where bias is already at play. Definitely go have a look for an example of how the models and datasets fit with the deployment context and how they can relate to known bias-related harms!\n\n<p align=\"center\">\n <br>\n <img src=\"URL alt=\"Selection of tools developed by HF team members to address bias in ML\" />\n <em><a href=\"URL Task Exploration tool</a> by <a href=\"URL <a href=\"URL and <a href=\"URL\n</p>",
"#### Task definition: recommendations\n\nThere are as many ways for the ML task definition and deployment to affect the risk of bias-related harms as there are applications for ML systems. As in the examples above, some common steps that may help decide whether and how to apply ML in a way that minimizes bias-related risk include:\n\n\n\n* Investigate:\n * Reports of bias in the field pre-ML\n * At-risk demographic categories for your specific use case\n* Examine:\n * The impact of your optimization objective on reinforcing biases\n * Alternative objectives that favor diversity and positive long-term impacts",
"### I am <span style=\"text-decoration:underline;\">curating/picking a dataset</span> for my ML system, how can I address bias?\n\nWhile training datasets are not the sole source of bias00061-1) in the ML development cycle, they do play a significant role. Does your dataset disproportionately associate biographies of women with life events but those of men with achievements? Those stereotypes are probably going to show up in your full ML system! Does your voice recognition dataset only feature specific accents? Not a good sign for the inclusivity of technology you build with it in terms of disparate performance! Whether you’re curating a dataset for ML applications or selecting a dataset to train an ML model, finding out, mitigating, and communicating to what extent the data exhibits these phenomena are all necessary steps to reducing bias-related risks.\n\nYou can usually get a pretty good sense of likely biases in a dataset by reflecting on where it comes from, who are the people represented on the data, and what the curation process was. Several frameworks for this reflection and documentation have been proposed such as Data Statements for NLP or Datasheets for Datasets. The Hugging Face Hub includes a Dataset Card template and guide inspired by these works; the section on considerations for using the data is usually a good place to look for information about notable biases if you’re browsing datasets, or to write a paragraph sharing your insights on the topic if you’re sharing a new one. And if you’re looking for more inspiration on what to put there, check out these sections written by Hub users in the BigLAM organization for historical datasets of legal proceedings, image classification, and newspapers.\n\n\n<p align=\"center\">\n <br>\n <img src=\"URL alt=\"HF Dataset Card guide for the Social Impact and Bias Sections\" />\n <em><a href=\"URL Dataset Card guide</a> for the Social Impact and Bias Sections</em>\n</p>\n\nWhile describing the origin and context of a dataset is always a good starting point to understand the biases at play, quantitatively measuring phenomena that encode those biases can be just as helpful. If you’re choosing between two different datasets for a given task or choosing between two ML models trained on different datasets, knowing which one better represents the demographic makeup of your ML system’s user base can help you make an informed decision to minimize bias-related risks. If you’re curating a dataset iteratively by filtering data points from a source or selecting new sources of data to add, measuring how these choices affect the diversity and biases present in your overall dataset can make it safer to use in general.\n\nWe’ve recently released two tools you can leverage to measure your data through a bias-informed lens. The disaggregators library provides utilities to quantify the composition of your dataset, using either metadata or leveraging models to infer properties of data points. This can be particularly useful to minimize risks of bias-related representation harms or disparate performances of trained models. Look at the demo to see it applied to the LAION, MedMCQA, and The Stack datasets!\n\n\n<p align=\"center\">\n <br>\n <img src=\"URL alt=\"Disaggregators tool by Nima\" />\n <em><a href=\"URL tool</a> by <a href=\"URL\n</p>\n\nOnce you have some helpful statistics about the composition of your dataset, you’ll also want to look at associations between features in your data items, particularly at associations that may encode derogatory or otherwise negative stereotypes. The Data Measurements Tool we originally introduced last year allows you to do this by looking at the normalized Pointwise Mutual Information (nPMI) between terms in your text-based dataset; particularly associations between gendered pronouns that may denote gendered stereotypes. Run it yourself or try it here on a few pre-computed datasets!\n\n\n<p align=\"center\">\n <br>\n <img src=\"URL alt=\"Data Measurements tool by Meg, Sasha, Bibi, and the Gradio team\" />\n <em><a href=\"URL Measurements tool</a> by <a href=\"URL <a href=\"URL <a href=\"URL and the <a href=\"URL team</a></em>\n</p>",
"#### Dataset selection/curation: recommendations\n\nThese tools aren’t full solutions by themselves, rather, they are designed to support critical examination and improvement of datasets through several lenses, including the lens of bias and bias-related risks. In general, we encourage you to keep the following steps in mind when leveraging these and other tools to mitigate bias risks at the dataset curation/selection stage:\n\n* Identify:\n * Aspects of the dataset creation that may exacerbate specific biases\n * Demographic categories and social variables that are particularly important to the dataset’s task and domain\n* Measure:\n * The demographic distribution in your dataset\n * Pre-identified negative stereotypes represented\n* Document:\n * Share what you’ve Identified and Measured in your Dataset Card so it can benefit other users, developers, and otherwise affected people\n* Adapt:\n * By choosing the dataset least likely to cause bias-related harms\n * By iteratively improving your dataset in ways that reduce bias risks",
"### I am <span style=\"text-decoration:underline;\">training/selecting a model</span> for my ML system, how can I address bias?\n\nSimilar to the dataset curation/selection step, documenting and measuring bias-related phenomena in models can help both ML developers who are selecting a model to use as-is or to finetune and ML developers who want to train their own models. For the latter, measures of bias-related phenomena in the model can help them learn from what has worked or what hasn’t for other models and serve as a signal to guide their own development choices.\n\nModel cards were originally proposed by (Mitchell et al., 2019) and provide a framework for model reporting that showcases information relevant to bias risks, including broad ethical considerations, disaggregated evaluation, and use case recommendation. The Hugging Face Hub provides even more tools for model documentation, with a model card guidebook in the Hub documentation, and an app that lets you create extensive model cards easily for your new model.\n\n<p align=\"center\">\n <br>\n <img src=\"URL alt=\"Model Card writing tool by Ezi, Marissa, and Meg\" />\n <em><a href=\"URL Card writing tool</a> by <a href=\"URL <a href=\"URL and <a href=\"URL\n</p>\n\nDocumentation is a great first step for sharing general insights about a model’s behavior, but it is usually static and presents the same information to all users. In many cases, especially for generative models that can generate outputs to approximate the distribution of their training data, we can gain a more contextual understanding of bias-related phenomena and negative stereotypes by visualizing and contrasting model outputs. Access to model generations can help users bring intersectional issues in the model behavior corresponding to their lived experience, and evaluate to what extent a model reproduces gendered stereotypes for different adjectives. To facilitate this process, we built a tool that lets you compare generations not just across a set of adjectives and professions, but also across different models! Go try it out to get a sense of which model might carry the least bias risks in your use case.\n\n<p align=\"center\">\n <br>\n <img src=\"URL alt=\"Visualize Adjective and Occupation Biases in Image Generation by Sasha\" />\n <br>\n <em><a href=\"URL Adjective and Occupation Biases in Image Generation</a> by <a href=\"URL\n</p>\n\nVisualization of model outputs isn’t just for generative models though! For classification models, we also want to look out for bias-related harms caused by a model’s disparate performance on different demographics. If you know what protected classes are most at risk of discrimination and have those annotated in an evaluation set, then you can report disaggregated performance over the different categories in your model card as mentioned above, so users can make informed decisions. If however, you are worried that you haven’t identified all populations at risk of bias-related harms, or if you do not have access to annotated test examples to measure the biases you suspect, that’s where interactive visualizations of where and how the model fails come in handy! To help you with this, the SEAL app groups similar mistakes by your model and shows you some common features in each cluster. If you want to go further, you can even combine it with the disaggregators library we introduced in the datasets section to find clusters that are indicative of bias-related failure modes!\n\n\n<p align=\"center\">\n <br>\n <img src=\"URL alt=\"Systematic Error Analysis and Labeling (SEAL) by Nazneen\" />\n <em><a href=\"URL Error Analysis and Labeling (SEAL)</a> by <a href=\"URL\n</p>\n\nFinally, a few benchmarks exist that can measure bias-related phenomena in models. For language models, benchmarks such as BOLD, HONEST, or WinoBias provide quantitative evaluations of targeted behaviors that are indicative of biases in the models. While the benchmarks have their limitations, they do provide a limited view into some pre-identified bias risks that can help describe how the models function or choose between different models. You can find these evaluations pre-computed on a range of common language models in this exploration Space to get a first sense of how they compare!\n\n\n<p align=\"center\">\n <br>\n <img src=\"URL alt=\"Language Model Bias Detection by Sasha\" />\n <em><a href=\"URL Model Bias Detection</a> by <a href=\"URL\n</p>\n\nEven with access to a benchmark for the models you are considering, you might find that running evaluations of the larger language models you are considering can be prohibitively expensive or otherwise technically impossible with your own computing resources. The <a href=\"URL on the Hub</a> tool we released this year can help with that: not only will it run the evaluations for you, but it will also help connect them to the model documentation so the results are available once and for all — so everyone can see, for example, that size <a href=\"URL increases bias risks in models like OPT</a>!\n\n<p align=\"center\">\n <br>\n <img src=\"URL alt=\"Large model WinoBias scores computed with Evaluation on the Hub by Helen, Tristan, Abhishek, Lewis, and Douwe\" />\n <em><a href=\"URL href=\"URL model WinoBias scores computed with Evaluation on the Hub</a> by <a href=\"URL <a href=\"URL <a href=\"URL <a href=\"URL and <a href=\"URL\n</p>",
"#### Model selection/development: recommendations\n\nFor models just as for datasets, different tools for documentation and evaluation will provide different views of bias risks in a model which all have a part to play in helping developers choose, develop, or understand ML systems.\n\n* Visualize\n * Generative model: visualize how the model’s outputs may reflect stereotypes\n * Classification model: visualize model errors to identify failure modes that could lead to disparate performance\n* Evaluate\n * When possible, evaluate models on relevant benchmarks\n* Document\n * Share your learnings from visualization and qualitative evaluation\n * Report your model’s disaggregated performance and results on applicable fairness benchmarks",
"## Conclusion and Overview of Bias Analysis and Documentation Tools from \n\nAs we learn to leverage ML systems in more and more applications, reaping their benefits equitably will depend on our ability to actively mitigate the risks of bias-related harms associated with the technology. While there is no single answer to the question of how this should best be done in any possible setting, we can support each other in this effort by sharing lessons, tools, and methodologies to mitigate and document those risks. The present blog post outlines some of the ways Hugging Face team members have addressed this question of bias along with supporting tools, we hope that you will find them helpful and encourage you to develop and share your own!\n\nSummary of linked tools:\n* Tasks:\n * Explore our directory of ML Tasks to understand what technical framings and resources are available to choose from\n * Use tools to explore the full development lifecycle of specific tasks\n* Datasets:\n * Make use of and contribute to Dataset Cards to share relevant insights on biases in datasets.\n * Use Disaggregator to look for possible disparate performance\n * Look at aggregated measurements of your dataset including nPMI to surface possible stereotypical associations\n* Models:\n * Make use of and contribute to Model Cards to share relevant insights on biases in models.\n * Use Interactive Model Cards to visualize performance discrepancies\n * Look at systematic model errors and look out for known social biases\n * Use Evaluate and Evaluation on the Hub to explore language model biases including in large models\n * Use a Text-to-image bias explorer to compare image generation models’ biases\n * Compare LM models with Bias Score Card\n\nThanks for reading! \n\n~ Yacine, on behalf of the Ethics and Society regulars\n\nCite as:"
] | [
"TAGS\n#license-cc-by-4.0 #arxiv-2203.07785 #arxiv-2010.03058 #arxiv-2211.03759 #arxiv-2209.03942 #arxiv-2212.05129 #doi-10.57967/hf/0208 #region-us \n",
"# Ethics and Society Newsletter #2",
"## Let’s Talk about Bias!\n\n_Bias in ML is ubiquitous, and Bias in ML is complex; so complex in fact that no single technical intervention is likely to meaningfully address the problems it engenders. ML models, as sociotechnical systems, amplify social trends that may exacerbate inequities and harmful biases in ways that depend on their deployment context and are constantly evolving._\n\n_This means that developing ML systems with care requires vigilance and responding to feedback from those deployment contexts, which in turn we can facilitate by sharing lessons across contexts and developing tools to analyze signs of bias at every level of ML development._\n\n_This blog post from the Ethics and Society regulars @ shares some of the lessons we have learned along with tools we have developed to support ourselves and others in our community’s efforts to better address bias in Machine Learning. The first part is a broader reflection on bias and its context. If you’ve already read it and are coming back specifically for the tools, feel free to jump to the datasets or models\nsection!_\n\n<p align=\"center\">\n <br>\n <img src=\"URL alt=\"Selection of tools developed by HF team members to address bias in ML\" />\n <em>Selection of tools developed by team members to address bias in ML</em>\n</p>\n\n<span style=\"text-decoration:underline;\">Table of contents:</span>\n* <span style=\"text-decoration:underline;\">On Machine Biases</span>\n * Machine Bias: from ML Systems to Risks\n * Putting Bias in Context\n* <span style=\"text-decoration:underline;\">Tools and Recommendations</span>\n * Addressing Bias throughout ML Development\n * Task Definition\n * Dataset Curation\n * Model Training\n * Overview of Bias Tools",
"## _Machine Bias:_ from ML Systems to Personal and Social Risks\n\nML systems allow us to automate complex tasks at a scale never seen before as they are deployed in more sectors and use cases. When the technology works at its best, it can help smooth interactions between people and technical systems, remove the need for highly repetitive work, or unlock new ways of processing information to support research.\n\nThese same systems are also likely to reproduce discriminatory and abusive behaviors represented in their training data, especially when the data encodes human behaviors.\nThe technology then has the potential to make these issues significantly worse. Automation and deployment at scale can indeed:\n\n1. lock in behaviors in time and hinder social progress from being reflected in technology, \n2. spread harmful behaviors beyond the context of the original training data,\n3. amplify inequities by overfocusing on stereotypical associations when making predictions,\n4. remove possibilities for recourse by hiding biases inside “black-box” systems.\n\nIn order to better understand and address these risks, ML researchers and developers have started studying _machine bias_ or _algorithmic bias_, mechanisms that might lead systems to, for example, encode negative stereotypes or associations or to have disparate performance for different population groups in their deployment context.\n\nThese issues are deeply personal for many of us ML researchers and developers at Hugging Face and in the broader ML community. Hugging Face is an international company, with many of us existing between countries and cultures. It is hard to fully express our sense of urgency when we see the technology we work on developed without sufficient concern for protecting people like us; especially when these systems lead to discriminatory wrongful arrests or undue financial distress and are being increasingly sold to immigration and law enforcement services around the world. Similarly, seeing our identities routinely suppressed in training datasets or underrepresented in the outputs of “generative AI” systems connects these concerns to our daily lived experiences in ways that are simultaneously enlightening and taxing.\n\nWhile our own experiences do not come close to covering the myriad ways in which ML-mediated discrimination can disproportionately harm people whose experiences differ from ours, they provide an entry point into considerations of the trade-offs inherent in the technology. We work on these systems because we strongly believe in ML’s potential — we think it can shine as a valuable tool as long as it is developed with care and input from people in its deployment context, rather than as a one-size-fits-all panacea. In particular, enabling this care requires developing a better understanding of the mechanisms of machine bias across the ML development process, and developing tools that support people with all levels of technical knowledge of these systems in participating in the necessary conversations about how their benefits and harms are distributed.\n\nThe present blog post from the Hugging Face Ethics and Society regulars provides an overview of how we have worked, are working, or recommend users of the HF ecosystem of libraries may work to address bias at the various stages of the ML development process, and the tools we develop to support this process. We hope you will find it a useful resource to guide concrete considerations of the social impact of your work and can leverage the tools referenced here to help mitigate these issues when they arise.",
"## Putting Bias in Context\n\nThe first and maybe most important concept to consider when dealing with machine bias is context. In their foundational work on bias in NLP, Su Lin Blodgett et al. point out that: _“[T]he majority of [academic works on machine bias] fail to engage critically with what constitutes “bias” in the first place”_, including by building their work on top of _“unstated assumptions about what kinds of system behaviors are harmful, in what ways, to whom, and why”_.\n\nThis may not come as much of a surprise given the ML research community’s focus on the value of “generalization” — the most cited motivation for work in the field after “performance”. However, while tools for bias assessment that apply to a wide range of settings are valuable to enable a broader analysis of common trends in model behaviors, their ability to target the mechanisms that lead to discrimination in concrete use cases is inherently limited. Using them to guide specific decisions within the ML development cycle usually requires an extra step or two to take the system’s specific use context and affected people into consideration.\n\n<p align=\"center\">\n <br>\n <img src=\"URL alt=\"Excerpt on considerations of ML uses context and people from the Model Card Guidebook\" />\n <em>Excerpt on considerations of ML uses context and people from the <a href=\"URL Card Guidebook</a></em>\n</p>\n\nNow let’s dive deeper into the issue of linking biases in stand-alone/context-less ML artifacts to specific harms. It can be useful to think of machine biases as risk factors for discrimination-based harms. Take the example of a text-to-image model that over-represents light skin tones when prompted to create a picture of a person in a professional setting, but produces darker skin tones when the prompts mention criminality. These tendencies would be what we call _machine biases at the model level_. Now let’s think about a few systems that use such a text-to-image model:\n\n\n1. <span style=\"text-decoration:underline;\">The model is integrated into a website creation service</span> (e.g. SquareSpace, Wix) to help users generate backgrounds for their pages. The model explicitly disables images of people in the generated background.\n * In this case, the machine bias “risk factor” does not lead to discrimination harm because the focus of the bias (images of people) is absent from the use case.\n * Further risk mitigation is not required for machine biases, although developers should be aware of ongoing discussions about the legality of integrating systems trained on scraped data in commercial systems.\n2. <span style=\"text-decoration:underline;\">The model is integrated into a stock images website</span> to provide users with synthetic images of people (e.g. in professional settings) that they can use with fewer privacy concerns, for example, to serve as illustrations for Wikipedia articles\n * In this case, machine bias acts to lock in and amplify existing social biases. It reinforces stereotypes about people (“CEOs are all white men”) that then feed back into complex social systems where increased bias leads to increased discrimination in many different ways (such as reinforcing implicit bias in the workplace).\n * Mitigation strategies may include educating the stock image users about these biases, or the stock image website may curate generated images to intentionally propose a more diverse set of representations.\n3. <span style=\"text-decoration:underline;\">The model is integrated into a “virtual sketch artist” software</span> marketed to police departments that will use it to generate pictures of suspects based on verbal testimony\n * In this case, the machine biases directly cause discrimination by systematically directing police departments to darker-skinned people, putting them at increased risk of harm including physical injury and unlawful imprisonment.\n * In cases like this one, there may be no level of bias mitigation that makes the risk acceptable. In particular, such a use case would be closely related to face recognition in the context of law enforcement, where similar bias issues have led several commercial entities and legislatures to adopt moratoria pausing or banning its use across the board.\n\nSo, who’s on the hook for machine biases in ML? These three cases illustrate one of the reasons why discussions about the responsibility of ML developers in addressing bias can get so complicated: depending on decisions made at other points in the ML system development process by other people, the biases in an ML dataset or model may land anywhere between being irrelevant to the application settings and directly leading to grievous harm. However, in all of these cases, stronger biases in the model/dataset increase the risk of negative outcomes. The European Union has started to develop frameworks that address this phenomenon in recent regulatory efforts: in short, a company that deploys an AI system based on a measurably biased model is liable for harm caused by the system.\n\nConceptualizing bias as a risk factor then allows us to better understand the shared responsibility for machine biases between developers at all stages. Bias can never be fully removed, not least because the definitions of social biases and the power dynamics that tie them to discrimination vary vastly across social contexts. However:\n\n\n\n1. Each stage of the development process, from task specification, dataset curation, and model training, to model integration and system deployment, can take steps to minimize the aspects of machine bias that most directly depend on its choices and technical decisions, and\n2. Clear communication and information flow between the various ML development stages can make the difference between making choices that build on top of each other to attenuate the negative potential of bias (multipronged approach to bias mitigation, as in deployment scenario 1 above) _versus_ making choices that compound this negative potential to exacerbate the risk of harm (as in deployment scenario 3).\n\nIn the next section, we review these various stages along with some of the tools that can help us address machine bias at each of them.",
"## Addressing Bias throughout the ML Development Cycle\n\nReady for some practical advice yet? Here we go \n\nThere is no one single way to develop ML systems; which steps happen in what order depends on a number of factors including the development setting (university, large company, startup, grassroots organization, etc…), the modality (text, tabular data, images, etc…), and the preeminence or scarcity of publicly available ML resources. However, we can identify three common stages of particular interest in addressing bias. These are the task definition, the data curation, and the model training. Let’s have a look at how bias handling may differ across these various stages.\n\n<p align=\"center\">\n <br>\n <img src=\"URL alt=\"The Bias ML Pipeline by Meg\" width=\"500\" />\n <em>The Bias ML Pipeline by <a href=\"URL\n</p>",
"### I am <span style=\"text-decoration:underline;\">defining the task</span> of my ML system, how can I address bias?\n\nWhether and to what extent bias in the system concretely affects people ultimately depends on what the system is used for. As such, the first place developers can work to mitigate bias is when deciding how ML fits in their system, e.g., by deciding what optimization objective it will use.\n\nFor example, let’s go back to one of the first highly-publicized cases of a Machine Learning system used in production for algorithmic content recommendation. From 2006 to 2009, Netflix ran the Netflix Prize, a competition with a 1M$ cash prize challenging teams around the world to develop ML systems to accurately predict a user’s rating for a new movie based on their past ratings. The winning submission improved the RMSE (Root-mean-square-error) of predictions on unseen user-movie pairs by over 10% over Netflix’s own CineMatch algorithm, meaning it got much better at predicting how users would rate a new movie based on their history. This approach opened the door for much of modern algorithmic content recommendation by bringing the role of ML in modeling user preferences in recommender systems to public awareness.\n\nSo what does this have to do with bias? Doesn’t showing people content that they’re likely to enjoy sound like a good service from a content platform? Well, it turns out that showing people more examples of what they’ve liked in the past ends up reducing the diversity of the media they consume. Not only does it lead users to be less satisfied in the long term, but it also means that any biases or stereotypes captured by the initial models — such as when modeling the preferences of Black American users or dynamics that systematically disadvantage some artists — are likely to be reinforced if the model is further trained on ongoing ML-mediated user interactions. This reflects two of the types of bias-related concerns we’ve mentioned above: the training objective acts as a risk factor for bias-related harms as it makes pre-existing biases much more likely to show up in predictions, and the task framing has the effect of locking in and exacerbating past biases.\n\nA promising bias mitigation strategy at this stage has been to reframe the task to explicitly model both engagement and diversity when applying ML to algorithmic content recommendation. Users are likely to get more long-term satisfaction and the risk of exacerbating biases as outlined above is reduced!\n\nThis example serves to illustrate that the impact of machine biases in an ML-supported product depends not just on where we decide to leverage ML, but also on how ML techniques are integrated into the broader technical system, and with what objective. When first investigating how ML can fit into a product or a use case you are interested in, we first recommend looking for the failure modes of the system through the lens of bias before even diving into the available models or datasets - which behaviors of existing systems in the space will be particularly harmful or more likely to occur if bias is exacerbated by ML predictions?\n\nWe built a tool to take users through these questions in another case of algorithmic content management: hate speech detection in automatic content moderation. We found for example that looking through news and scientific articles that didn’t particularly focus on the ML part of the technology was already a great way to get a sense of where bias is already at play. Definitely go have a look for an example of how the models and datasets fit with the deployment context and how they can relate to known bias-related harms!\n\n<p align=\"center\">\n <br>\n <img src=\"URL alt=\"Selection of tools developed by HF team members to address bias in ML\" />\n <em><a href=\"URL Task Exploration tool</a> by <a href=\"URL <a href=\"URL and <a href=\"URL\n</p>",
"#### Task definition: recommendations\n\nThere are as many ways for the ML task definition and deployment to affect the risk of bias-related harms as there are applications for ML systems. As in the examples above, some common steps that may help decide whether and how to apply ML in a way that minimizes bias-related risk include:\n\n\n\n* Investigate:\n * Reports of bias in the field pre-ML\n * At-risk demographic categories for your specific use case\n* Examine:\n * The impact of your optimization objective on reinforcing biases\n * Alternative objectives that favor diversity and positive long-term impacts",
"### I am <span style=\"text-decoration:underline;\">curating/picking a dataset</span> for my ML system, how can I address bias?\n\nWhile training datasets are not the sole source of bias00061-1) in the ML development cycle, they do play a significant role. Does your dataset disproportionately associate biographies of women with life events but those of men with achievements? Those stereotypes are probably going to show up in your full ML system! Does your voice recognition dataset only feature specific accents? Not a good sign for the inclusivity of technology you build with it in terms of disparate performance! Whether you’re curating a dataset for ML applications or selecting a dataset to train an ML model, finding out, mitigating, and communicating to what extent the data exhibits these phenomena are all necessary steps to reducing bias-related risks.\n\nYou can usually get a pretty good sense of likely biases in a dataset by reflecting on where it comes from, who are the people represented on the data, and what the curation process was. Several frameworks for this reflection and documentation have been proposed such as Data Statements for NLP or Datasheets for Datasets. The Hugging Face Hub includes a Dataset Card template and guide inspired by these works; the section on considerations for using the data is usually a good place to look for information about notable biases if you’re browsing datasets, or to write a paragraph sharing your insights on the topic if you’re sharing a new one. And if you’re looking for more inspiration on what to put there, check out these sections written by Hub users in the BigLAM organization for historical datasets of legal proceedings, image classification, and newspapers.\n\n\n<p align=\"center\">\n <br>\n <img src=\"URL alt=\"HF Dataset Card guide for the Social Impact and Bias Sections\" />\n <em><a href=\"URL Dataset Card guide</a> for the Social Impact and Bias Sections</em>\n</p>\n\nWhile describing the origin and context of a dataset is always a good starting point to understand the biases at play, quantitatively measuring phenomena that encode those biases can be just as helpful. If you’re choosing between two different datasets for a given task or choosing between two ML models trained on different datasets, knowing which one better represents the demographic makeup of your ML system’s user base can help you make an informed decision to minimize bias-related risks. If you’re curating a dataset iteratively by filtering data points from a source or selecting new sources of data to add, measuring how these choices affect the diversity and biases present in your overall dataset can make it safer to use in general.\n\nWe’ve recently released two tools you can leverage to measure your data through a bias-informed lens. The disaggregators library provides utilities to quantify the composition of your dataset, using either metadata or leveraging models to infer properties of data points. This can be particularly useful to minimize risks of bias-related representation harms or disparate performances of trained models. Look at the demo to see it applied to the LAION, MedMCQA, and The Stack datasets!\n\n\n<p align=\"center\">\n <br>\n <img src=\"URL alt=\"Disaggregators tool by Nima\" />\n <em><a href=\"URL tool</a> by <a href=\"URL\n</p>\n\nOnce you have some helpful statistics about the composition of your dataset, you’ll also want to look at associations between features in your data items, particularly at associations that may encode derogatory or otherwise negative stereotypes. The Data Measurements Tool we originally introduced last year allows you to do this by looking at the normalized Pointwise Mutual Information (nPMI) between terms in your text-based dataset; particularly associations between gendered pronouns that may denote gendered stereotypes. Run it yourself or try it here on a few pre-computed datasets!\n\n\n<p align=\"center\">\n <br>\n <img src=\"URL alt=\"Data Measurements tool by Meg, Sasha, Bibi, and the Gradio team\" />\n <em><a href=\"URL Measurements tool</a> by <a href=\"URL <a href=\"URL <a href=\"URL and the <a href=\"URL team</a></em>\n</p>",
"#### Dataset selection/curation: recommendations\n\nThese tools aren’t full solutions by themselves, rather, they are designed to support critical examination and improvement of datasets through several lenses, including the lens of bias and bias-related risks. In general, we encourage you to keep the following steps in mind when leveraging these and other tools to mitigate bias risks at the dataset curation/selection stage:\n\n* Identify:\n * Aspects of the dataset creation that may exacerbate specific biases\n * Demographic categories and social variables that are particularly important to the dataset’s task and domain\n* Measure:\n * The demographic distribution in your dataset\n * Pre-identified negative stereotypes represented\n* Document:\n * Share what you’ve Identified and Measured in your Dataset Card so it can benefit other users, developers, and otherwise affected people\n* Adapt:\n * By choosing the dataset least likely to cause bias-related harms\n * By iteratively improving your dataset in ways that reduce bias risks",
"### I am <span style=\"text-decoration:underline;\">training/selecting a model</span> for my ML system, how can I address bias?\n\nSimilar to the dataset curation/selection step, documenting and measuring bias-related phenomena in models can help both ML developers who are selecting a model to use as-is or to finetune and ML developers who want to train their own models. For the latter, measures of bias-related phenomena in the model can help them learn from what has worked or what hasn’t for other models and serve as a signal to guide their own development choices.\n\nModel cards were originally proposed by (Mitchell et al., 2019) and provide a framework for model reporting that showcases information relevant to bias risks, including broad ethical considerations, disaggregated evaluation, and use case recommendation. The Hugging Face Hub provides even more tools for model documentation, with a model card guidebook in the Hub documentation, and an app that lets you create extensive model cards easily for your new model.\n\n<p align=\"center\">\n <br>\n <img src=\"URL alt=\"Model Card writing tool by Ezi, Marissa, and Meg\" />\n <em><a href=\"URL Card writing tool</a> by <a href=\"URL <a href=\"URL and <a href=\"URL\n</p>\n\nDocumentation is a great first step for sharing general insights about a model’s behavior, but it is usually static and presents the same information to all users. In many cases, especially for generative models that can generate outputs to approximate the distribution of their training data, we can gain a more contextual understanding of bias-related phenomena and negative stereotypes by visualizing and contrasting model outputs. Access to model generations can help users bring intersectional issues in the model behavior corresponding to their lived experience, and evaluate to what extent a model reproduces gendered stereotypes for different adjectives. To facilitate this process, we built a tool that lets you compare generations not just across a set of adjectives and professions, but also across different models! Go try it out to get a sense of which model might carry the least bias risks in your use case.\n\n<p align=\"center\">\n <br>\n <img src=\"URL alt=\"Visualize Adjective and Occupation Biases in Image Generation by Sasha\" />\n <br>\n <em><a href=\"URL Adjective and Occupation Biases in Image Generation</a> by <a href=\"URL\n</p>\n\nVisualization of model outputs isn’t just for generative models though! For classification models, we also want to look out for bias-related harms caused by a model’s disparate performance on different demographics. If you know what protected classes are most at risk of discrimination and have those annotated in an evaluation set, then you can report disaggregated performance over the different categories in your model card as mentioned above, so users can make informed decisions. If however, you are worried that you haven’t identified all populations at risk of bias-related harms, or if you do not have access to annotated test examples to measure the biases you suspect, that’s where interactive visualizations of where and how the model fails come in handy! To help you with this, the SEAL app groups similar mistakes by your model and shows you some common features in each cluster. If you want to go further, you can even combine it with the disaggregators library we introduced in the datasets section to find clusters that are indicative of bias-related failure modes!\n\n\n<p align=\"center\">\n <br>\n <img src=\"URL alt=\"Systematic Error Analysis and Labeling (SEAL) by Nazneen\" />\n <em><a href=\"URL Error Analysis and Labeling (SEAL)</a> by <a href=\"URL\n</p>\n\nFinally, a few benchmarks exist that can measure bias-related phenomena in models. For language models, benchmarks such as BOLD, HONEST, or WinoBias provide quantitative evaluations of targeted behaviors that are indicative of biases in the models. While the benchmarks have their limitations, they do provide a limited view into some pre-identified bias risks that can help describe how the models function or choose between different models. You can find these evaluations pre-computed on a range of common language models in this exploration Space to get a first sense of how they compare!\n\n\n<p align=\"center\">\n <br>\n <img src=\"URL alt=\"Language Model Bias Detection by Sasha\" />\n <em><a href=\"URL Model Bias Detection</a> by <a href=\"URL\n</p>\n\nEven with access to a benchmark for the models you are considering, you might find that running evaluations of the larger language models you are considering can be prohibitively expensive or otherwise technically impossible with your own computing resources. The <a href=\"URL on the Hub</a> tool we released this year can help with that: not only will it run the evaluations for you, but it will also help connect them to the model documentation so the results are available once and for all — so everyone can see, for example, that size <a href=\"URL increases bias risks in models like OPT</a>!\n\n<p align=\"center\">\n <br>\n <img src=\"URL alt=\"Large model WinoBias scores computed with Evaluation on the Hub by Helen, Tristan, Abhishek, Lewis, and Douwe\" />\n <em><a href=\"URL href=\"URL model WinoBias scores computed with Evaluation on the Hub</a> by <a href=\"URL <a href=\"URL <a href=\"URL <a href=\"URL and <a href=\"URL\n</p>",
"#### Model selection/development: recommendations\n\nFor models just as for datasets, different tools for documentation and evaluation will provide different views of bias risks in a model which all have a part to play in helping developers choose, develop, or understand ML systems.\n\n* Visualize\n * Generative model: visualize how the model’s outputs may reflect stereotypes\n * Classification model: visualize model errors to identify failure modes that could lead to disparate performance\n* Evaluate\n * When possible, evaluate models on relevant benchmarks\n* Document\n * Share your learnings from visualization and qualitative evaluation\n * Report your model’s disaggregated performance and results on applicable fairness benchmarks",
"## Conclusion and Overview of Bias Analysis and Documentation Tools from \n\nAs we learn to leverage ML systems in more and more applications, reaping their benefits equitably will depend on our ability to actively mitigate the risks of bias-related harms associated with the technology. While there is no single answer to the question of how this should best be done in any possible setting, we can support each other in this effort by sharing lessons, tools, and methodologies to mitigate and document those risks. The present blog post outlines some of the ways Hugging Face team members have addressed this question of bias along with supporting tools, we hope that you will find them helpful and encourage you to develop and share your own!\n\nSummary of linked tools:\n* Tasks:\n * Explore our directory of ML Tasks to understand what technical framings and resources are available to choose from\n * Use tools to explore the full development lifecycle of specific tasks\n* Datasets:\n * Make use of and contribute to Dataset Cards to share relevant insights on biases in datasets.\n * Use Disaggregator to look for possible disparate performance\n * Look at aggregated measurements of your dataset including nPMI to surface possible stereotypical associations\n* Models:\n * Make use of and contribute to Model Cards to share relevant insights on biases in models.\n * Use Interactive Model Cards to visualize performance discrepancies\n * Look at systematic model errors and look out for known social biases\n * Use Evaluate and Evaluation on the Hub to explore language model biases including in large models\n * Use a Text-to-image bias explorer to compare image generation models’ biases\n * Compare LM models with Bias Score Card\n\nThanks for reading! \n\n~ Yacine, on behalf of the Ethics and Society regulars\n\nCite as:"
] |
fe54a6270b82e5076657694d18b4930ddbedc9a8 |
This post was originally published on the [Hugging Face blog 🤗](https://huggingface.co/blog/ethics-soc-2)
# Ethics and Society Newsletter #2
## Let’s Talk about Bias!
_Bias in ML is ubiquitous, and Bias in ML is complex; so complex in fact that no single technical intervention is likely to meaningfully address the problems it engenders. ML models, as sociotechnical systems, amplify social trends that may exacerbate inequities and harmful biases in ways that depend on their deployment context and are constantly evolving._
_This means that developing ML systems with care requires vigilance and responding to feedback from those deployment contexts, which in turn we can facilitate by sharing lessons across contexts and developing tools to analyze signs of bias at every level of ML development._
_This blog post from the [Ethics and Society regulars @🤗](https://huggingface.co/blog/ethics-soc-1) shares some of the lessons we have learned along with tools we have developed to support ourselves and others in our community’s efforts to better address bias in Machine Learning. The first part is a broader reflection on bias and its context. If you’ve already read it and are coming back specifically for the tools, feel free to jump to the [datasets](#i-am-curatingpicking-a-dataset-for-my-ml-system-how-can-i-address-bias) or [models](#i-am-trainingselecting-a-model-for-my-ml-system-how-can-i-address-bias)
section!_
<p align="center">
<br>
<img src="https://huggingface.co/datasets/huggingface/documentation-images/resolve/main/blog/ethics_soc_2/img1.jpg" alt="Selection of tools developed by HF team members to address bias in ML" />
<em>Selection of tools developed by 🤗 team members to address bias in ML</em>
</p>
**<span style="text-decoration:underline;">Table of contents:</span>**
* **<span style="text-decoration:underline;">On Machine Biases</span>**
* [Machine Bias: from ML Systems to Risks](#machine-bias-from-ml-systems-to-personal-and-social-risks)
* [Putting Bias in Context](#putting-bias-in-context)
* **<span style="text-decoration:underline;">Tools and Recommendations</span>**
* [Addressing Bias throughout ML Development](#addressing-bias-throughout-the-ml-development-cycle)
* [Task Definition](#i-am-defining-the-task-of-my-ml-system-how-can-i-address-bias)
* [Dataset Curation](#i-am-curatingpicking-a-dataset-for-my-ml-system-how-can-i-address-bias)
* [Model Training](#i-am-trainingselecting-a-model-for-my-ml-system-how-can-i-address-bias)
* [Overview of 🤗 Bias Tools](#conclusion-and-overview-of-bias-analysis-and-documentation-tools-from-🤗)
## _Machine Bias:_ from ML Systems to Personal and Social Risks
ML systems allow us to automate complex tasks at a scale never seen before as they are deployed in more sectors and use cases. When the technology works at its best, it can help smooth interactions between people and technical systems, remove the need for highly repetitive work, or unlock new ways of processing information to support research.
These same systems are also likely to reproduce discriminatory and abusive behaviors represented in their training data, especially when the data encodes human behaviors.
The technology then has the potential to make these issues significantly worse. Automation and deployment at scale can indeed:
1. **lock in** behaviors in time and hinder social progress [from being reflected in technology](https://dl.acm.org/doi/10.1145/3442188.3445922),
2. **spread** harmful behaviors [beyond the context](https://arxiv.org/abs/2203.07785) of the original training data,
3. **amplify** inequities by [overfocusing on stereotypical associations](https://arxiv.org/abs/2010.03058) when making predictions,
4. **remove possibilities for recourse** by hiding biases [inside “black-box” systems](https://pubmed.ncbi.nlm.nih.gov/33737318/).
In order to better understand and address these risks, ML researchers and developers have started studying _machine bias_ or _algorithmic bias_, mechanisms that might lead systems to, for example, encode **negative stereotypes or associations** or to have **disparate performance** for different population groups in their deployment context.
**These issues are deeply personal** for many of us ML researchers and developers at Hugging Face and in the broader ML community. Hugging Face is [an international company](https://twitter.com/osanseviero/status/1587444072901492737), with many of us existing between countries and cultures. It is hard to fully express our sense of urgency when we see the technology we work on developed [without sufficient concern](https://dl.acm.org/doi/10.1145/3461702.3462624) for protecting people like us; especially when these systems lead to discriminatory [wrongful arrests](https://incidentdatabase.ai/cite/72/) or undue [financial distress](https://racismandtechnology.center/2021/10/29/amnestys-grim-warning-against-another-toeslagenaffaire/) and are being [increasingly sold](https://www.oecd.org/migration/mig/EMN-OECD-INFORM-FEB-2022-The-use-of-Digitalisation-and-AI-in-Migration-Management.pdf) to immigration and law enforcement services around the world. Similarly, seeing our identities routinely [suppressed in training datasets](https://aclanthology.org/2021.emnlp-main.98/) or [underrepresented in the outputs](https://huggingface.co/spaces/sasha/StableDiffusionBiasExplorer) of “generative AI” [systems ](https://twitter.com/willie_agnew/status/1592829238889283585)connects these concerns to our daily lived experiences in ways that are [simultaneously enlightening and taxing](https://www.technologyreview.com/2022/10/28/1062332/responsible-ai-has-a-burnout-problem/).
While our own experiences do not come close to covering the myriad ways in which ML-mediated discrimination can disproportionately harm people whose experiences differ from ours, they provide an entry point into considerations of the trade-offs inherent in the technology. We work on these systems because we **strongly believe in ML’s potential — we think it can shine as a valuable tool as long as it is developed with care and input from people in its deployment context**, rather than as a one-size-fits-all panacea. In particular, enabling this care requires developing a better understanding of the mechanisms of machine bias across the ML development process, and developing tools that support people [with all levels of technical knowledge of these systems in participating in the necessary conversations](https://www.vice.com/en/article/bvm35w/this-tool-lets-anyone-see-the-bias-in-ai-image-generators) about how their benefits and harms are distributed.
The present blog post from the Hugging Face [Ethics and Society regulars](https://huggingface.co/blog/ethics-soc-1) provides an overview of how we have worked, are working, or recommend users of the HF ecosystem of libraries may work to address bias at the various stages of the ML development process, and the tools we develop to support this process. We hope you will find it a useful resource to guide concrete considerations of the social impact of your work and can leverage the tools referenced here to help mitigate these issues when they arise.
## Putting Bias in Context
The first and maybe most important concept to consider when dealing with machine bias is **context**. In their foundational work on [bias in NLP](https://aclanthology.org/2020.acl-main.485.pdf), Su Lin Blodgett et al. point out that: _“[T]he majority of [academic works on machine bias] fail to engage critically with what constitutes “bias” in the first place”_, including by building their work on top of _“unstated assumptions about what kinds of system behaviors are harmful, in what ways, to whom, and why”_.
This may not come as much of a surprise given the ML research community’s [focus on the value of “generalization”](https://dl.acm.org/doi/10.1145/3531146.3533083) — the most cited motivation for work in the field after “performance”. However, while tools for bias assessment that apply to a wide range of settings are valuable to **enable a broader analysis of common trends** in model behaviors, their ability to target the mechanisms that lead to discrimination in **concrete use cases is inherently limited**. Using them to guide specific decisions within the ML development cycle usually requires an extra step or two to take the system’s specific use context and affected people into consideration.
<p align="center">
<br>
<img src="https://huggingface.co/datasets/huggingface/documentation-images/resolve/main/blog/ethics_soc_2/img_foresight.png" alt="Excerpt on considerations of ML uses context and people from the Model Card Guidebook" />
<em>Excerpt on considerations of ML uses context and people from the <a href="https://huggingface.co/docs/hub/model-cards">Model Card Guidebook</a></em>
</p>
Now let’s dive deeper into the issue of linking biases in stand-alone/context-less ML artifacts to specific harms. It can be useful to think of **machine biases as risk factors for discrimination-based harms**. Take the example of a text-to-image model that over-represents light skin tones when prompted to create a picture of a person in a professional setting, but produces darker skin tones [when the prompts mention criminality](https://arxiv.org/abs/2211.03759). These tendencies would be what we call _machine biases at the model level_. Now let’s think about a few systems that use such a text-to-image model:
1. <span style="text-decoration:underline;">The model is integrated into a website creation service</span> (e.g. SquareSpace, Wix) to help users generate backgrounds for their pages. The model explicitly disables images of people in the generated background.
* In this case, the machine bias “risk factor” does not lead to discrimination harm because the focus of the bias (images of people) is absent from the use case.
* Further risk mitigation is not required for machine biases, although developers should be aware of ongoing discussions about the legality of integrating systems trained on scraped data in commercial systems.
2. <span style="text-decoration:underline;">The model is integrated into a stock images website</span> to provide users with synthetic images of people (e.g. in professional settings) that they can use with fewer privacy concerns, for example, to serve as illustrations for Wikipedia articles
* In this case, machine bias acts to **lock in** and **amplify** existing social biases. It reinforces stereotypes about people (“CEOs are all white men”) that then feed back into complex social systems where increased bias leads to increased discrimination in many different ways (such as reinforcing [implicit bias](https://philpapers.org/rec/BEEAIT-2) in the workplace).
* Mitigation strategies may include educating the stock image users about these biases, or the stock image website may curate generated images to intentionally propose a more diverse set of representations.
3. <span style="text-decoration:underline;">The model is integrated into a “virtual sketch artist” software</span> marketed to police departments that will use it to generate pictures of suspects based on verbal testimony
* In this case, the machine biases directly cause discrimination by systematically directing police departments to darker-skinned people, putting them at increased risk of harm including physical injury and unlawful imprisonment.
* In cases like this one, there may be no level of bias mitigation that makes the risk acceptable. In particular, such a use case would be closely related to face recognition in the context of law enforcement, where [similar bias issues](https://www.law.georgetown.edu/privacy-technology-center/publications/a-forensic-without-the-science-face-recognition-in-u-s-criminal-investigations/) have led several commercial entities and legislatures to adopt moratoria pausing or banning its use across the board.
So, who’s on the hook for machine biases in ML? These three cases illustrate one of the reasons why discussions about the responsibility of ML developers in addressing bias can get so complicated: depending on decisions made at other points in the ML system development process by other people, the biases in an ML dataset or model may land anywhere between being irrelevant to the application settings and directly leading to grievous harm. However, in all of these cases, **stronger biases in the model/dataset increase the risk of negative outcomes**. The European Union has started to develop frameworks that address this phenomenon in [recent regulatory efforts](https://ec.europa.eu/info/business-economy-euro/doing-business-eu/contract-rules/digital-contracts/liability-rules-artificial-intelligence_en): in short, a company that deploys an AI system based on a measurably biased model is liable for harm caused by the system.
Conceptualizing bias as a risk factor then allows us to better understand the **shared responsibility** for machine biases between developers at all stages. Bias can never be fully removed, not least because the definitions of social biases and the power dynamics that tie them to discrimination vary vastly across social contexts. However:
1. Each stage of the development process, from task specification, dataset curation, and model training, to model integration and system deployment, can take steps to minimize the aspects of machine bias** that most directly depend on its choices** and technical decisions, and
2. Clear communication and **information flow between the various ML development stages** can make the difference between making choices that build on top of each other to attenuate the negative potential of bias (multipronged approach to bias mitigation, as in deployment scenario 1 above) _versus_ making choices that compound this negative potential to exacerbate the risk of harm (as in deployment scenario 3).
In the next section, we review these various stages along with some of the tools that can help us address machine bias at each of them.
## Addressing Bias Throughout the ML Development Cycle
Ready for some practical advice yet? Here we go 🤗
There is no one single way to develop ML systems; which steps happen in what order depends on a number of factors including the development setting (university, large company, startup, grassroots organization, etc…), the modality (text, tabular data, images, etc…), and the preeminence or scarcity of publicly available ML resources. However, we can identify three common stages of particular interest in addressing bias. These are the task definition, the data curation, and the model training. Let’s have a look at how bias handling may differ across these various stages.
<p align="center">
<br>
<img src="https://huggingface.co/datasets/huggingface/documentation-images/resolve/main/blog/ethics_soc_2/img_pipeline.png" alt="The Bias ML Pipeline by Meg" width="500" />
<em>The Bias ML Pipeline by <a href="https://huggingface.co/meg">Meg</a></em>
</p>
### I am <span style="text-decoration:underline;">defining the task</span> of my ML system, how can I address bias?
Whether and to what extent bias in the system concretely affects people ultimately depends on what the system is used for. As such, the first place developers can work to mitigate bias is when deciding how ML fits in their system, e.g., by deciding what optimization objective it will use.
For example, let’s go back to one of the first highly-publicized cases of a Machine Learning system used in production for algorithmic content recommendation. From 2006 to 2009, Netflix ran the [Netflix Prize](https://www.cs.uic.edu/~liub/KDD-cup-2007/proceedings/The-Netflix-Prize-Bennett.pdf), a competition with a 1M$ cash prize challenging teams around the world to develop ML systems to accurately predict a user’s rating for a new movie based on their past ratings. The [winning submission](https://www.asc.ohio-state.edu/statistics/dmsl/GrandPrize2009_BPC_BigChaos.pdf) improved the RMSE (Root-mean-square-error) of predictions on unseen user-movie pairs by over 10% over Netflix’s own CineMatch algorithm, meaning it got much better at predicting how users would rate a new movie based on their history. This approach opened the door for much of modern algorithmic content recommendation by bringing the role of ML in modeling user preferences in recommender systems to public awareness.
So what does this have to do with bias? Doesn’t showing people content that they’re likely to enjoy sound like a good service from a content platform? Well, it turns out that showing people more examples of **what they’ve liked in the past** ends up [reducing the diversity of the media they consume](https://dl.acm.org/doi/10.1145/3391403.3399532). Not only does it lead users to be [less satisfied in the long term](https://dl.acm.org/doi/abs/10.1145/3366423.3380281), but it also means that any biases or stereotypes captured by the initial models — such as when modeling [the preferences of Black American users](https://www.marieclaire.com/culture/a18817/netflix-algorithms-black-movies/) or [dynamics that systematically disadvantage](https://dl.acm.org/doi/10.1145/3269206.3272027) some artists — are likely to be reinforced if the model is [further trained on ongoing ML-mediated](https://arxiv.org/abs/2209.03942) user interactions. This reflects two of the types of bias-related concerns we’ve mentioned above: the training objective acts as a **risk factor** for bias-related harms as it makes pre-existing biases much more likely to show up in predictions, and the task framing has the effect of **locking in** and exacerbating past biases.
A promising bias mitigation strategy at this stage has been to reframe the task to explicitly [model both engagement and diversity](https://dl.acm.org/doi/10.1145/3437963.3441775) when applying ML to algorithmic content recommendation. Users are likely to get more long-term satisfaction and the risk of exacerbating biases as outlined above is reduced!
This example serves to illustrate that the impact of machine biases in an ML-supported product depends not just on where we decide to leverage ML, but also on how ML techniques are integrated into the broader technical system, and with what objective. When first investigating how ML can fit into a product or a use case you are interested in, we first recommend looking for the failure modes of the system through the lens of bias before even diving into the available models or datasets - which behaviors of existing systems in the space will be particularly harmful or more likely to occur if bias is exacerbated by ML predictions?
We built a [tool](https://huggingface.co/spaces/hf-task-exploration/ExploreACMnaacl) to take users through these questions in another case of algorithmic content management: [hate speech detection in automatic content moderation](https://aclanthology.org/2022.hcinlp-1.2/). We found for example that looking through news and scientific articles that didn’t particularly focus on the ML part of the technology was already a great way to get a sense of where bias is already at play. Definitely go have a look for an example of how the models and datasets fit with the deployment context and how they can relate to known bias-related harms!
<p align="center">
<br>
<img src="https://huggingface.co/datasets/huggingface/documentation-images/resolve/main/blog/ethics_soc_2/img2.png" alt="Selection of tools developed by HF team members to address bias in ML" />
<em><a href="https://huggingface.co/spaces/hf-task-exploration/ExploreACMnaacl">ACM Task Exploration tool</a> by <a href="https://huggingface.co/aymm">Angie</a>, <a href="https://huggingface.co/paullada">Amandalynne</a>, and <a href="https://huggingface.co/yjernite">Yacine</a></em>
</p>
#### Task definition: recommendations
There are as many ways for the ML task definition and deployment to affect the risk of bias-related harms as there are applications for ML systems. As in the examples above, some common steps that may help decide whether and how to apply ML in a way that minimizes bias-related risk include:
* Investigate:
* Reports of bias in the field pre-ML
* At-risk demographic categories for your specific use case
* Examine:
* The impact of your optimization objective on reinforcing biases
* Alternative objectives that favor diversity and positive long-term impacts
### I am <span style="text-decoration:underline;">curating/picking a dataset</span> for my ML system, how can I address bias?
While training datasets are [not the sole source of bias](https://www.cell.com/patterns/fulltext/S2666-3899(21)00061-1) in the ML development cycle, they do play a significant role. Does your [dataset disproportionately associate](https://aclanthology.org/2020.emnlp-main.23/) biographies of women with life events but those of men with achievements? Those **stereotypes** are probably going to show up in your full ML system! Does your voice recognition dataset only feature specific accents? Not a good sign for [the inclusivity of technology](https://www.scientificamerican.com/article/speech-recognition-tech-is-yet-another-example-of-bias/) you build with it in terms of **disparate performance**! Whether you’re curating a dataset for ML applications or selecting a dataset to train an ML model, finding out, mitigating, and [communicating](https://dl.acm.org/doi/10.1145/3479582) to what extent the data exhibits these phenomena are all necessary steps to reducing bias-related risks.
You can usually get a pretty good sense of likely biases in a dataset by reflecting on where it comes from, who are the people represented on the data, and what the curation process was. Several frameworks for this reflection and documentation have been proposed such as [Data Statements for NLP](https://direct.mit.edu/tacl/article/doi/10.1162/tacl_a_00041/43452/Data-Statements-for-Natural-Language-Processing) or [Datasheets for Datasets](https://dl.acm.org/doi/10.1145/3458723). The Hugging Face Hub includes a Dataset Card [template](https://github.com/huggingface/datasets/blob/main/templates/README.md) and [guide](https://github.com/huggingface/datasets/blob/main/templates/README_guide.md#dataset-card-creation-guide) inspired by these works; the section on [considerations for using the data](https://github.com/huggingface/datasets/blob/main/templates/README_guide.md#considerations-for-using-the-data) is usually a good place to look for information about notable biases if you’re browsing datasets, or to write a paragraph sharing your insights on the topic if you’re sharing a new one. And if you’re looking for more inspiration on what to put there, check out these sections written by Hub users in the [BigLAM organization](https://huggingface.co/biglam) for historical datasets of [legal proceedings](https://huggingface.co/datasets/biglam/old_bailey_proceedings#social-impact-of-dataset), [image classification](https://huggingface.co/datasets/biglam/brill_iconclass#social-impact-of-dataset), and [newspapers](https://huggingface.co/datasets/biglam/bnl_newspapers1841-1879#social-impact-of-dataset).
<p align="center">
<br>
<img src="https://huggingface.co/datasets/huggingface/documentation-images/resolve/main/blog/ethics_soc_2/img3.png" alt="HF Dataset Card guide for the Social Impact and Bias Sections" />
<em><a href="https://github.com/huggingface/datasets/blob/main/templates/README_guide.md#social-impact-of-dataset">HF Dataset Card guide</a> for the Social Impact and Bias Sections</em>
</p>
While describing the origin and context of a dataset is always a good starting point to understand the biases at play, [quantitatively measuring phenomena](https://arxiv.org/abs/2212.05129) that encode those biases can be just as helpful. If you’re choosing between two different datasets for a given task or choosing between two ML models trained on different datasets, knowing which one better represents the demographic makeup of your ML system’s user base can help you make an informed decision to minimize bias-related risks. If you’re curating a dataset iteratively by filtering data points from a source or selecting new sources of data to add, measuring how these choices affect the diversity and biases present in your overall dataset can make it safer to use in general.
We’ve recently released two tools you can leverage to measure your data through a bias-informed lens. The [disaggregators🤗 library](https://github.com/huggingface/disaggregators) provides utilities to quantify the composition of your dataset, using either metadata or leveraging models to infer properties of data points. This can be particularly useful to minimize risks of bias-related **[representation harms](https://aclanthology.org/P16-2096/)** or **disparate performances** of trained models. Look at the [demo](https://huggingface.co/spaces/society-ethics/disaggregators) to see it applied to the LAION, MedMCQA, and The Stack datasets!
<p align="center">
<br>
<img src="https://huggingface.co/datasets/huggingface/documentation-images/resolve/main/blog/ethics_soc_2/img4.png" alt="Disaggregators tool by Nima" />
<em><a href="https://huggingface.co/spaces/society-ethics/disaggregators">Disaggregator tool</a> by <a href="https://huggingface.co/NimaBoscarino">Nima</a></em>
</p>
Once you have some helpful statistics about the composition of your dataset, you’ll also want to look at associations between features in your data items, particularly at associations that may encode derogatory or otherwise negative stereotypes. The Data Measurements Tool we [originally introduced](https://huggingface.co/blog/data-measurements-tool#comparison-statistics) last year allows you to do this by looking at the [normalized Pointwise Mutual Information (nPMI)](https://dl.acm.org/doi/10.1145/3461702.3462557) between terms in your text-based dataset; particularly associations between gendered pronouns that may denote gendered stereotypes. [Run it yourself](https://github.com/huggingface/data-measurements-tool) or [try it here](https://huggingface.co/spaces/huggingface/data-measurements-tool) on a few pre-computed datasets!
<p align="center">
<br>
<img src="https://huggingface.co/datasets/huggingface/documentation-images/resolve/main/blog/ethics_soc_2/img5.png" alt="Data Measurements tool by Meg, Sasha, Bibi, and the Gradio team" />
<em><a href="https://huggingface.co/spaces/huggingface/data-measurements-tool">Data Measurements tool</a> by <a href="https://huggingface.co/meg">Meg</a>, <a href="https://huggingface.co/sasha">Sasha</a>, <a href="https://huggingface.co/Bibss">Bibi</a>, and the <a href="https://gradio.app/">Gradio team</a></em>
</p>
#### Dataset selection/curation: recommendations
These tools aren’t full solutions by themselves, rather, they are designed to support critical examination and improvement of datasets through several lenses, including the lens of bias and bias-related risks. In general, we encourage you to keep the following steps in mind when leveraging these and other tools to mitigate bias risks at the dataset curation/selection stage:
* Identify:
* Aspects of the dataset creation that may exacerbate specific biases
* Demographic categories and social variables that are particularly important to the dataset’s task and domain
* Measure:
* The demographic distribution in your dataset
* Pre-identified negative stereotypes represented
* Document:
* Share what you’ve Identified and Measured in your Dataset Card so it can benefit other users, developers, and otherwise affected people
* Adapt:
* By choosing the dataset least likely to cause bias-related harms
* By iteratively improving your dataset in ways that reduce bias risks
### I am <span style="text-decoration:underline;">training/selecting a model</span> for my ML system, how can I address bias?
Similar to the dataset curation/selection step, documenting and measuring bias-related phenomena in models can help both ML developers who are selecting a model to use as-is or to finetune and ML developers who want to train their own models. For the latter, measures of bias-related phenomena in the model can help them learn from what has worked or what hasn’t for other models and serve as a signal to guide their own development choices.
Model cards were originally proposed by [(Mitchell et al., 2019)](https://dl.acm.org/doi/10.1145/3287560.3287596) and provide a framework for model reporting that showcases information relevant to bias risks, including broad ethical considerations, disaggregated evaluation, and use case recommendation. The Hugging Face Hub provides even more tools for model documentation, with a [model card guidebook](https://huggingface.co/docs/hub/model-cards) in the Hub documentation, and an [app that lets you create extensive model cards](https://huggingface.co/spaces/huggingface/Model_Cards_Writing_Tool) easily for your new model.
<p align="center">
<br>
<img src="https://huggingface.co/datasets/huggingface/documentation-images/resolve/main/blog/ethics_soc_2/img6.png" alt="Model Card writing tool by Ezi, Marissa, and Meg" />
<em><a href="https://huggingface.co/spaces/huggingface/Model_Cards_Writing_Tool">Model Card writing tool</a> by <a href="https://huggingface.co/Ezi">Ezi</a>, <a href="https://huggingface.co/Marissa">Marissa</a>, and <a href="https://huggingface.co/meg">Meg</a></em>
</p>
Documentation is a great first step for sharing general insights about a model’s behavior, but it is usually static and presents the same information to all users. In many cases, especially for generative models that can generate outputs to approximate the distribution of their training data, we can gain a more contextual understanding of bias-related phenomena and **negative stereotypes** by visualizing and contrasting model outputs. Access to model generations can help users bring [intersectional issues in the model behavior](https://www.technologyreview.com/2022/12/12/1064751/the-viral-ai-avatar-app-lensa-undressed-me-without-my-consent/) corresponding to their lived experience, and evaluate to what extent a model reproduces [gendered stereotypes for different adjectives](https://www.vice.com/en/article/bvm35w/this-tool-lets-anyone-see-the-bias-in-ai-image-generators). To facilitate this process, we built a tool that lets you compare generations not just across a set of adjectives and professions, but also across different models! [Go try it out](https://huggingface.co/spaces/society-ethics/DiffusionBiasExplorer) to get a sense of which model might carry the least bias risks in your use case.
<p align="center">
<br>
<img src="https://huggingface.co/datasets/huggingface/documentation-images/resolve/main/blog/ethics_soc_2/img7.png" alt="Visualize Adjective and Occupation Biases in Image Generation by Sasha" />
<br>
<em><a href="https://huggingface.co/spaces/society-ethics/DiffusionBiasExplorer">Visualize Adjective and Occupation Biases in Image Generation</a> by <a href="https://huggingface.co/sasha">Sasha</a></em>
</p>
Visualization of model outputs isn’t just for generative models though! For classification models, we also want to look out for bias-related harms caused by a model’s **disparate performance** on different demographics. If you know what protected classes are most at risk of discrimination and have those annotated in an evaluation set, then you can report disaggregated performance over the different categories in [your model card](https://dl.acm.org/doi/10.1145/3287560.3287596) as mentioned above, so users can make informed decisions. If however, you are worried that you haven’t identified all populations at risk of bias-related harms, or if you do not have access to annotated test examples to measure the biases you suspect, that’s where interactive visualizations of where and how the model fails come in handy! To help you with this, the [SEAL app](https://huggingface.co/spaces/nazneen/seal) groups similar mistakes by your model and shows you some common features in each cluster. If you want to go further, you can even combine it with the [disaggregators library](https://github.com/huggingface/disaggregators) we introduced in the datasets section to find clusters that are indicative of bias-related failure modes!
<p align="center">
<br>
<img src="https://huggingface.co/datasets/huggingface/documentation-images/resolve/main/blog/ethics_soc_2/img8.png" alt="Systematic Error Analysis and Labeling (SEAL) by Nazneen" />
<em><a href="https://huggingface.co/spaces/nazneen/seal">Systematic Error Analysis and Labeling (SEAL)</a> by <a href="https://huggingface.co/nazneen">Nazneen</a></em>
</p>
Finally, a few benchmarks exist that can measure bias-related phenomena in models. For language models, benchmarks such as [BOLD](https://github.com/amazon-science/bold), [HONEST](https://aclanthology.org/2021.naacl-main.191.pdf), or [WinoBias](https://aclanthology.org/N18-2003/) provide quantitative evaluations of targeted behaviors that are indicative of biases in the models. While the benchmarks have their [limitations](https://aclanthology.org/2021.acl-long.81/), they do provide a limited view into some pre-identified bias risks that can help describe how the models function or choose between different models. You can find these evaluations pre-computed on a range of common language models [in this exploration Space](https://huggingface.co/spaces/sasha/BiasDetection) to get a first sense of how they compare!
<p align="center">
<br>
<img src="https://huggingface.co/datasets/huggingface/documentation-images/resolve/main/blog/ethics_soc_2/img9.png" alt="Language Model Bias Detection by Sasha" />
<em><a href="https://huggingface.co/spaces/sasha/BiasDetection">Language Model Bias Detection</a> by <a href="https://huggingface.co/sasha">Sasha</a></em>
</p>
Even with access to a benchmark for the models you are considering, you might find that running evaluations of the larger language models you are considering can be prohibitively expensive or otherwise technically impossible with your own computing resources. The <a href="https://huggingface.co/spaces/autoevaluate/model-evaluator">Evaluation on the Hub</a> tool we released this year can help with that: not only will it run the evaluations for you, but it will also help connect them to the model documentation so the results are available once and for all — so everyone can see, for example, that size <a href="https://huggingface.co/blog/zero-shot-eval-on-the-hub">measurably increases bias risks in models like OPT</a>!
<p align="center">
<br>
<img src="https://huggingface.co/datasets/huggingface/documentation-images/resolve/main/blog/ethics_soc_2/img_winobias.png" alt="Large model WinoBias scores computed with Evaluation on the Hub by Helen, Tristan, Abhishek, Lewis, and Douwe" />
<em><a href="https://huggingface.co/spaces/sasha/BiasDetection"><a href="https://huggingface.co/blog/zero-shot-eval-on-the-hub">Large model WinoBias scores computed with Evaluation on the Hub</a> by <a href="https://huggingface.co/mathemakitten">Helen</a>, <a href="https://huggingface.co/Tristan">Tristan</a>, <a href="https://huggingface.co/abhishek">Abhishek</a>, <a href="https://huggingface.co/lewtun">Lewis</a>, and <a href="https://huggingface.co/douwekiela">Douwe</a></em>
</p>
#### Model selection/development: recommendations
For models just as for datasets, different tools for documentation and evaluation will provide different views of bias risks in a model which all have a part to play in helping developers choose, develop, or understand ML systems.
* Visualize
* Generative model: visualize how the model’s outputs may reflect stereotypes
* Classification model: visualize model errors to identify failure modes that could lead to disparate performance
* Evaluate
* When possible, evaluate models on relevant benchmarks
* Document
* Share your learnings from visualization and qualitative evaluation
* Report your model’s disaggregated performance and results on applicable fairness benchmarks
## Conclusion and Overview of Bias Analysis and Documentation Tools from 🤗
As we learn to leverage ML systems in more and more applications, reaping their benefits equitably will depend on our ability to actively mitigate the risks of bias-related harms associated with the technology. While there is no single answer to the question of how this should best be done in any possible setting, we can support each other in this effort by sharing lessons, tools, and methodologies to mitigate and document those risks. The present blog post outlines some of the ways Hugging Face team members have addressed this question of bias along with supporting tools, we hope that you will find them helpful and encourage you to develop and share your own!
Summary of linked tools:
* Tasks:
* Explore our directory of [ML Tasks](https://huggingface.co/tasks) to understand what technical framings and resources are available to choose from
* Use tools to explore the [full development lifecycle](https://huggingface.co/spaces/hf-task-exploration/ExploreACMnaacl) of specific tasks
* Datasets:
* Make use of and contribute to [Dataset Cards](https://github.com/huggingface/datasets/blob/main/templates/README_guide.md#social-impact-of-dataset) to share relevant insights on biases in datasets.
* Use [Disaggregator](https://github.com/huggingface/disaggregators) to look for [possible disparate performance](https://huggingface.co/spaces/society-ethics/disaggregators)
* Look at aggregated [measurements of your dataset](https://huggingface.co/spaces/huggingface/data-measurements-tool) including nPMI to surface possible stereotypical associations
* Models:
* Make use of and contribute to [Model Cards](https://huggingface.co/docs/hub/model-cards) to share relevant insights on biases in models.
* Use [Interactive Model Cards](https://huggingface.co/spaces/nazneen/interactive-model-cards) to visualize performance discrepancies
* Look at [systematic model errors](https://huggingface.co/spaces/nazneen/seal) and look out for known social biases
* Use [Evaluate](https://github.com/huggingface/evaluate) and [Evaluation on the Hub](https://huggingface.co/spaces/autoevaluate/model-evaluator) to explore [language model biases](https://huggingface.co/blog/evaluating-llm-bias) including in [large models](https://huggingface.co/blog/zero-shot-eval-on-the-hub)
* Use a [Text-to-image bias explorer](https://huggingface.co/spaces/sasha/StableDiffusionBiasExplorer) to compare image generation models’ biases
* Compare LM models with Bias [Score Card](https://huggingface.co/spaces/sasha/BiasDetection)
Thanks for reading! 🤗
~ Yacine, on behalf of the Ethics and Society regulars
Cite as:
```
@inproceedings{hf_ethics_soc_blog_2,
author = {Yacine Jernite and
Alexandra Sasha Luccioni and
Irene Solaiman and
Giada Pistilli and
Nathan Lambert and
Ezi Ozoani and
Brigitte Toussignant and
Margaret Mitchell},
title = {Hugging Face Ethics and Society Newsletter 2: Let's Talk about Bias!},
booktitle = {Hugging Face Blog},
year = {2022},
url = {https://doi.org/10.57967/hf/0214},
doi = {10.57967/hf/0214}
}
``` | society-ethics/BlogPostBias | [
"license:cc-by-4.0",
"arxiv:2203.07785",
"arxiv:2010.03058",
"arxiv:2211.03759",
"arxiv:2209.03942",
"arxiv:2212.05129",
"doi:10.57967/hf/0214",
"region:us"
] | 2022-12-15T21:55:16+00:00 | {"license": "cc-by-4.0"} | 2022-12-16T14:54:32+00:00 | [
"2203.07785",
"2010.03058",
"2211.03759",
"2209.03942",
"2212.05129"
] | [] | TAGS
#license-cc-by-4.0 #arxiv-2203.07785 #arxiv-2010.03058 #arxiv-2211.03759 #arxiv-2209.03942 #arxiv-2212.05129 #doi-10.57967/hf/0214 #region-us
|
This post was originally published on the Hugging Face blog
# Ethics and Society Newsletter #2
## Let’s Talk about Bias!
_Bias in ML is ubiquitous, and Bias in ML is complex; so complex in fact that no single technical intervention is likely to meaningfully address the problems it engenders. ML models, as sociotechnical systems, amplify social trends that may exacerbate inequities and harmful biases in ways that depend on their deployment context and are constantly evolving._
_This means that developing ML systems with care requires vigilance and responding to feedback from those deployment contexts, which in turn we can facilitate by sharing lessons across contexts and developing tools to analyze signs of bias at every level of ML development._
_This blog post from the Ethics and Society regulars @ shares some of the lessons we have learned along with tools we have developed to support ourselves and others in our community’s efforts to better address bias in Machine Learning. The first part is a broader reflection on bias and its context. If you’ve already read it and are coming back specifically for the tools, feel free to jump to the datasets or models
section!_
<p align="center">
<br>
<img src="URL alt="Selection of tools developed by HF team members to address bias in ML" />
<em>Selection of tools developed by team members to address bias in ML</em>
</p>
<span style="text-decoration:underline;">Table of contents:</span>
* <span style="text-decoration:underline;">On Machine Biases</span>
* Machine Bias: from ML Systems to Risks
* Putting Bias in Context
* <span style="text-decoration:underline;">Tools and Recommendations</span>
* Addressing Bias throughout ML Development
* Task Definition
* Dataset Curation
* Model Training
* Overview of Bias Tools
## _Machine Bias:_ from ML Systems to Personal and Social Risks
ML systems allow us to automate complex tasks at a scale never seen before as they are deployed in more sectors and use cases. When the technology works at its best, it can help smooth interactions between people and technical systems, remove the need for highly repetitive work, or unlock new ways of processing information to support research.
These same systems are also likely to reproduce discriminatory and abusive behaviors represented in their training data, especially when the data encodes human behaviors.
The technology then has the potential to make these issues significantly worse. Automation and deployment at scale can indeed:
1. lock in behaviors in time and hinder social progress from being reflected in technology,
2. spread harmful behaviors beyond the context of the original training data,
3. amplify inequities by overfocusing on stereotypical associations when making predictions,
4. remove possibilities for recourse by hiding biases inside “black-box” systems.
In order to better understand and address these risks, ML researchers and developers have started studying _machine bias_ or _algorithmic bias_, mechanisms that might lead systems to, for example, encode negative stereotypes or associations or to have disparate performance for different population groups in their deployment context.
These issues are deeply personal for many of us ML researchers and developers at Hugging Face and in the broader ML community. Hugging Face is an international company, with many of us existing between countries and cultures. It is hard to fully express our sense of urgency when we see the technology we work on developed without sufficient concern for protecting people like us; especially when these systems lead to discriminatory wrongful arrests or undue financial distress and are being increasingly sold to immigration and law enforcement services around the world. Similarly, seeing our identities routinely suppressed in training datasets or underrepresented in the outputs of “generative AI” systems connects these concerns to our daily lived experiences in ways that are simultaneously enlightening and taxing.
While our own experiences do not come close to covering the myriad ways in which ML-mediated discrimination can disproportionately harm people whose experiences differ from ours, they provide an entry point into considerations of the trade-offs inherent in the technology. We work on these systems because we strongly believe in ML’s potential — we think it can shine as a valuable tool as long as it is developed with care and input from people in its deployment context, rather than as a one-size-fits-all panacea. In particular, enabling this care requires developing a better understanding of the mechanisms of machine bias across the ML development process, and developing tools that support people with all levels of technical knowledge of these systems in participating in the necessary conversations about how their benefits and harms are distributed.
The present blog post from the Hugging Face Ethics and Society regulars provides an overview of how we have worked, are working, or recommend users of the HF ecosystem of libraries may work to address bias at the various stages of the ML development process, and the tools we develop to support this process. We hope you will find it a useful resource to guide concrete considerations of the social impact of your work and can leverage the tools referenced here to help mitigate these issues when they arise.
## Putting Bias in Context
The first and maybe most important concept to consider when dealing with machine bias is context. In their foundational work on bias in NLP, Su Lin Blodgett et al. point out that: _“[T]he majority of [academic works on machine bias] fail to engage critically with what constitutes “bias” in the first place”_, including by building their work on top of _“unstated assumptions about what kinds of system behaviors are harmful, in what ways, to whom, and why”_.
This may not come as much of a surprise given the ML research community’s focus on the value of “generalization” — the most cited motivation for work in the field after “performance”. However, while tools for bias assessment that apply to a wide range of settings are valuable to enable a broader analysis of common trends in model behaviors, their ability to target the mechanisms that lead to discrimination in concrete use cases is inherently limited. Using them to guide specific decisions within the ML development cycle usually requires an extra step or two to take the system’s specific use context and affected people into consideration.
<p align="center">
<br>
<img src="URL alt="Excerpt on considerations of ML uses context and people from the Model Card Guidebook" />
<em>Excerpt on considerations of ML uses context and people from the <a href="URL Card Guidebook</a></em>
</p>
Now let’s dive deeper into the issue of linking biases in stand-alone/context-less ML artifacts to specific harms. It can be useful to think of machine biases as risk factors for discrimination-based harms. Take the example of a text-to-image model that over-represents light skin tones when prompted to create a picture of a person in a professional setting, but produces darker skin tones when the prompts mention criminality. These tendencies would be what we call _machine biases at the model level_. Now let’s think about a few systems that use such a text-to-image model:
1. <span style="text-decoration:underline;">The model is integrated into a website creation service</span> (e.g. SquareSpace, Wix) to help users generate backgrounds for their pages. The model explicitly disables images of people in the generated background.
* In this case, the machine bias “risk factor” does not lead to discrimination harm because the focus of the bias (images of people) is absent from the use case.
* Further risk mitigation is not required for machine biases, although developers should be aware of ongoing discussions about the legality of integrating systems trained on scraped data in commercial systems.
2. <span style="text-decoration:underline;">The model is integrated into a stock images website</span> to provide users with synthetic images of people (e.g. in professional settings) that they can use with fewer privacy concerns, for example, to serve as illustrations for Wikipedia articles
* In this case, machine bias acts to lock in and amplify existing social biases. It reinforces stereotypes about people (“CEOs are all white men”) that then feed back into complex social systems where increased bias leads to increased discrimination in many different ways (such as reinforcing implicit bias in the workplace).
* Mitigation strategies may include educating the stock image users about these biases, or the stock image website may curate generated images to intentionally propose a more diverse set of representations.
3. <span style="text-decoration:underline;">The model is integrated into a “virtual sketch artist” software</span> marketed to police departments that will use it to generate pictures of suspects based on verbal testimony
* In this case, the machine biases directly cause discrimination by systematically directing police departments to darker-skinned people, putting them at increased risk of harm including physical injury and unlawful imprisonment.
* In cases like this one, there may be no level of bias mitigation that makes the risk acceptable. In particular, such a use case would be closely related to face recognition in the context of law enforcement, where similar bias issues have led several commercial entities and legislatures to adopt moratoria pausing or banning its use across the board.
So, who’s on the hook for machine biases in ML? These three cases illustrate one of the reasons why discussions about the responsibility of ML developers in addressing bias can get so complicated: depending on decisions made at other points in the ML system development process by other people, the biases in an ML dataset or model may land anywhere between being irrelevant to the application settings and directly leading to grievous harm. However, in all of these cases, stronger biases in the model/dataset increase the risk of negative outcomes. The European Union has started to develop frameworks that address this phenomenon in recent regulatory efforts: in short, a company that deploys an AI system based on a measurably biased model is liable for harm caused by the system.
Conceptualizing bias as a risk factor then allows us to better understand the shared responsibility for machine biases between developers at all stages. Bias can never be fully removed, not least because the definitions of social biases and the power dynamics that tie them to discrimination vary vastly across social contexts. However:
1. Each stage of the development process, from task specification, dataset curation, and model training, to model integration and system deployment, can take steps to minimize the aspects of machine bias that most directly depend on its choices and technical decisions, and
2. Clear communication and information flow between the various ML development stages can make the difference between making choices that build on top of each other to attenuate the negative potential of bias (multipronged approach to bias mitigation, as in deployment scenario 1 above) _versus_ making choices that compound this negative potential to exacerbate the risk of harm (as in deployment scenario 3).
In the next section, we review these various stages along with some of the tools that can help us address machine bias at each of them.
## Addressing Bias Throughout the ML Development Cycle
Ready for some practical advice yet? Here we go
There is no one single way to develop ML systems; which steps happen in what order depends on a number of factors including the development setting (university, large company, startup, grassroots organization, etc…), the modality (text, tabular data, images, etc…), and the preeminence or scarcity of publicly available ML resources. However, we can identify three common stages of particular interest in addressing bias. These are the task definition, the data curation, and the model training. Let’s have a look at how bias handling may differ across these various stages.
<p align="center">
<br>
<img src="URL alt="The Bias ML Pipeline by Meg" width="500" />
<em>The Bias ML Pipeline by <a href="URL
</p>
### I am <span style="text-decoration:underline;">defining the task</span> of my ML system, how can I address bias?
Whether and to what extent bias in the system concretely affects people ultimately depends on what the system is used for. As such, the first place developers can work to mitigate bias is when deciding how ML fits in their system, e.g., by deciding what optimization objective it will use.
For example, let’s go back to one of the first highly-publicized cases of a Machine Learning system used in production for algorithmic content recommendation. From 2006 to 2009, Netflix ran the Netflix Prize, a competition with a 1M$ cash prize challenging teams around the world to develop ML systems to accurately predict a user’s rating for a new movie based on their past ratings. The winning submission improved the RMSE (Root-mean-square-error) of predictions on unseen user-movie pairs by over 10% over Netflix’s own CineMatch algorithm, meaning it got much better at predicting how users would rate a new movie based on their history. This approach opened the door for much of modern algorithmic content recommendation by bringing the role of ML in modeling user preferences in recommender systems to public awareness.
So what does this have to do with bias? Doesn’t showing people content that they’re likely to enjoy sound like a good service from a content platform? Well, it turns out that showing people more examples of what they’ve liked in the past ends up reducing the diversity of the media they consume. Not only does it lead users to be less satisfied in the long term, but it also means that any biases or stereotypes captured by the initial models — such as when modeling the preferences of Black American users or dynamics that systematically disadvantage some artists — are likely to be reinforced if the model is further trained on ongoing ML-mediated user interactions. This reflects two of the types of bias-related concerns we’ve mentioned above: the training objective acts as a risk factor for bias-related harms as it makes pre-existing biases much more likely to show up in predictions, and the task framing has the effect of locking in and exacerbating past biases.
A promising bias mitigation strategy at this stage has been to reframe the task to explicitly model both engagement and diversity when applying ML to algorithmic content recommendation. Users are likely to get more long-term satisfaction and the risk of exacerbating biases as outlined above is reduced!
This example serves to illustrate that the impact of machine biases in an ML-supported product depends not just on where we decide to leverage ML, but also on how ML techniques are integrated into the broader technical system, and with what objective. When first investigating how ML can fit into a product or a use case you are interested in, we first recommend looking for the failure modes of the system through the lens of bias before even diving into the available models or datasets - which behaviors of existing systems in the space will be particularly harmful or more likely to occur if bias is exacerbated by ML predictions?
We built a tool to take users through these questions in another case of algorithmic content management: hate speech detection in automatic content moderation. We found for example that looking through news and scientific articles that didn’t particularly focus on the ML part of the technology was already a great way to get a sense of where bias is already at play. Definitely go have a look for an example of how the models and datasets fit with the deployment context and how they can relate to known bias-related harms!
<p align="center">
<br>
<img src="URL alt="Selection of tools developed by HF team members to address bias in ML" />
<em><a href="URL Task Exploration tool</a> by <a href="URL <a href="URL and <a href="URL
</p>
#### Task definition: recommendations
There are as many ways for the ML task definition and deployment to affect the risk of bias-related harms as there are applications for ML systems. As in the examples above, some common steps that may help decide whether and how to apply ML in a way that minimizes bias-related risk include:
* Investigate:
* Reports of bias in the field pre-ML
* At-risk demographic categories for your specific use case
* Examine:
* The impact of your optimization objective on reinforcing biases
* Alternative objectives that favor diversity and positive long-term impacts
### I am <span style="text-decoration:underline;">curating/picking a dataset</span> for my ML system, how can I address bias?
While training datasets are not the sole source of bias00061-1) in the ML development cycle, they do play a significant role. Does your dataset disproportionately associate biographies of women with life events but those of men with achievements? Those stereotypes are probably going to show up in your full ML system! Does your voice recognition dataset only feature specific accents? Not a good sign for the inclusivity of technology you build with it in terms of disparate performance! Whether you’re curating a dataset for ML applications or selecting a dataset to train an ML model, finding out, mitigating, and communicating to what extent the data exhibits these phenomena are all necessary steps to reducing bias-related risks.
You can usually get a pretty good sense of likely biases in a dataset by reflecting on where it comes from, who are the people represented on the data, and what the curation process was. Several frameworks for this reflection and documentation have been proposed such as Data Statements for NLP or Datasheets for Datasets. The Hugging Face Hub includes a Dataset Card template and guide inspired by these works; the section on considerations for using the data is usually a good place to look for information about notable biases if you’re browsing datasets, or to write a paragraph sharing your insights on the topic if you’re sharing a new one. And if you’re looking for more inspiration on what to put there, check out these sections written by Hub users in the BigLAM organization for historical datasets of legal proceedings, image classification, and newspapers.
<p align="center">
<br>
<img src="URL alt="HF Dataset Card guide for the Social Impact and Bias Sections" />
<em><a href="URL Dataset Card guide</a> for the Social Impact and Bias Sections</em>
</p>
While describing the origin and context of a dataset is always a good starting point to understand the biases at play, quantitatively measuring phenomena that encode those biases can be just as helpful. If you’re choosing between two different datasets for a given task or choosing between two ML models trained on different datasets, knowing which one better represents the demographic makeup of your ML system’s user base can help you make an informed decision to minimize bias-related risks. If you’re curating a dataset iteratively by filtering data points from a source or selecting new sources of data to add, measuring how these choices affect the diversity and biases present in your overall dataset can make it safer to use in general.
We’ve recently released two tools you can leverage to measure your data through a bias-informed lens. The disaggregators library provides utilities to quantify the composition of your dataset, using either metadata or leveraging models to infer properties of data points. This can be particularly useful to minimize risks of bias-related representation harms or disparate performances of trained models. Look at the demo to see it applied to the LAION, MedMCQA, and The Stack datasets!
<p align="center">
<br>
<img src="URL alt="Disaggregators tool by Nima" />
<em><a href="URL tool</a> by <a href="URL
</p>
Once you have some helpful statistics about the composition of your dataset, you’ll also want to look at associations between features in your data items, particularly at associations that may encode derogatory or otherwise negative stereotypes. The Data Measurements Tool we originally introduced last year allows you to do this by looking at the normalized Pointwise Mutual Information (nPMI) between terms in your text-based dataset; particularly associations between gendered pronouns that may denote gendered stereotypes. Run it yourself or try it here on a few pre-computed datasets!
<p align="center">
<br>
<img src="URL alt="Data Measurements tool by Meg, Sasha, Bibi, and the Gradio team" />
<em><a href="URL Measurements tool</a> by <a href="URL <a href="URL <a href="URL and the <a href="URL team</a></em>
</p>
#### Dataset selection/curation: recommendations
These tools aren’t full solutions by themselves, rather, they are designed to support critical examination and improvement of datasets through several lenses, including the lens of bias and bias-related risks. In general, we encourage you to keep the following steps in mind when leveraging these and other tools to mitigate bias risks at the dataset curation/selection stage:
* Identify:
* Aspects of the dataset creation that may exacerbate specific biases
* Demographic categories and social variables that are particularly important to the dataset’s task and domain
* Measure:
* The demographic distribution in your dataset
* Pre-identified negative stereotypes represented
* Document:
* Share what you’ve Identified and Measured in your Dataset Card so it can benefit other users, developers, and otherwise affected people
* Adapt:
* By choosing the dataset least likely to cause bias-related harms
* By iteratively improving your dataset in ways that reduce bias risks
### I am <span style="text-decoration:underline;">training/selecting a model</span> for my ML system, how can I address bias?
Similar to the dataset curation/selection step, documenting and measuring bias-related phenomena in models can help both ML developers who are selecting a model to use as-is or to finetune and ML developers who want to train their own models. For the latter, measures of bias-related phenomena in the model can help them learn from what has worked or what hasn’t for other models and serve as a signal to guide their own development choices.
Model cards were originally proposed by (Mitchell et al., 2019) and provide a framework for model reporting that showcases information relevant to bias risks, including broad ethical considerations, disaggregated evaluation, and use case recommendation. The Hugging Face Hub provides even more tools for model documentation, with a model card guidebook in the Hub documentation, and an app that lets you create extensive model cards easily for your new model.
<p align="center">
<br>
<img src="URL alt="Model Card writing tool by Ezi, Marissa, and Meg" />
<em><a href="URL Card writing tool</a> by <a href="URL <a href="URL and <a href="URL
</p>
Documentation is a great first step for sharing general insights about a model’s behavior, but it is usually static and presents the same information to all users. In many cases, especially for generative models that can generate outputs to approximate the distribution of their training data, we can gain a more contextual understanding of bias-related phenomena and negative stereotypes by visualizing and contrasting model outputs. Access to model generations can help users bring intersectional issues in the model behavior corresponding to their lived experience, and evaluate to what extent a model reproduces gendered stereotypes for different adjectives. To facilitate this process, we built a tool that lets you compare generations not just across a set of adjectives and professions, but also across different models! Go try it out to get a sense of which model might carry the least bias risks in your use case.
<p align="center">
<br>
<img src="URL alt="Visualize Adjective and Occupation Biases in Image Generation by Sasha" />
<br>
<em><a href="URL Adjective and Occupation Biases in Image Generation</a> by <a href="URL
</p>
Visualization of model outputs isn’t just for generative models though! For classification models, we also want to look out for bias-related harms caused by a model’s disparate performance on different demographics. If you know what protected classes are most at risk of discrimination and have those annotated in an evaluation set, then you can report disaggregated performance over the different categories in your model card as mentioned above, so users can make informed decisions. If however, you are worried that you haven’t identified all populations at risk of bias-related harms, or if you do not have access to annotated test examples to measure the biases you suspect, that’s where interactive visualizations of where and how the model fails come in handy! To help you with this, the SEAL app groups similar mistakes by your model and shows you some common features in each cluster. If you want to go further, you can even combine it with the disaggregators library we introduced in the datasets section to find clusters that are indicative of bias-related failure modes!
<p align="center">
<br>
<img src="URL alt="Systematic Error Analysis and Labeling (SEAL) by Nazneen" />
<em><a href="URL Error Analysis and Labeling (SEAL)</a> by <a href="URL
</p>
Finally, a few benchmarks exist that can measure bias-related phenomena in models. For language models, benchmarks such as BOLD, HONEST, or WinoBias provide quantitative evaluations of targeted behaviors that are indicative of biases in the models. While the benchmarks have their limitations, they do provide a limited view into some pre-identified bias risks that can help describe how the models function or choose between different models. You can find these evaluations pre-computed on a range of common language models in this exploration Space to get a first sense of how they compare!
<p align="center">
<br>
<img src="URL alt="Language Model Bias Detection by Sasha" />
<em><a href="URL Model Bias Detection</a> by <a href="URL
</p>
Even with access to a benchmark for the models you are considering, you might find that running evaluations of the larger language models you are considering can be prohibitively expensive or otherwise technically impossible with your own computing resources. The <a href="URL on the Hub</a> tool we released this year can help with that: not only will it run the evaluations for you, but it will also help connect them to the model documentation so the results are available once and for all — so everyone can see, for example, that size <a href="URL increases bias risks in models like OPT</a>!
<p align="center">
<br>
<img src="URL alt="Large model WinoBias scores computed with Evaluation on the Hub by Helen, Tristan, Abhishek, Lewis, and Douwe" />
<em><a href="URL href="URL model WinoBias scores computed with Evaluation on the Hub</a> by <a href="URL <a href="URL <a href="URL <a href="URL and <a href="URL
</p>
#### Model selection/development: recommendations
For models just as for datasets, different tools for documentation and evaluation will provide different views of bias risks in a model which all have a part to play in helping developers choose, develop, or understand ML systems.
* Visualize
* Generative model: visualize how the model’s outputs may reflect stereotypes
* Classification model: visualize model errors to identify failure modes that could lead to disparate performance
* Evaluate
* When possible, evaluate models on relevant benchmarks
* Document
* Share your learnings from visualization and qualitative evaluation
* Report your model’s disaggregated performance and results on applicable fairness benchmarks
## Conclusion and Overview of Bias Analysis and Documentation Tools from
As we learn to leverage ML systems in more and more applications, reaping their benefits equitably will depend on our ability to actively mitigate the risks of bias-related harms associated with the technology. While there is no single answer to the question of how this should best be done in any possible setting, we can support each other in this effort by sharing lessons, tools, and methodologies to mitigate and document those risks. The present blog post outlines some of the ways Hugging Face team members have addressed this question of bias along with supporting tools, we hope that you will find them helpful and encourage you to develop and share your own!
Summary of linked tools:
* Tasks:
* Explore our directory of ML Tasks to understand what technical framings and resources are available to choose from
* Use tools to explore the full development lifecycle of specific tasks
* Datasets:
* Make use of and contribute to Dataset Cards to share relevant insights on biases in datasets.
* Use Disaggregator to look for possible disparate performance
* Look at aggregated measurements of your dataset including nPMI to surface possible stereotypical associations
* Models:
* Make use of and contribute to Model Cards to share relevant insights on biases in models.
* Use Interactive Model Cards to visualize performance discrepancies
* Look at systematic model errors and look out for known social biases
* Use Evaluate and Evaluation on the Hub to explore language model biases including in large models
* Use a Text-to-image bias explorer to compare image generation models’ biases
* Compare LM models with Bias Score Card
Thanks for reading!
~ Yacine, on behalf of the Ethics and Society regulars
Cite as:
| [
"# Ethics and Society Newsletter #2",
"## Let’s Talk about Bias!\n\n_Bias in ML is ubiquitous, and Bias in ML is complex; so complex in fact that no single technical intervention is likely to meaningfully address the problems it engenders. ML models, as sociotechnical systems, amplify social trends that may exacerbate inequities and harmful biases in ways that depend on their deployment context and are constantly evolving._\n\n_This means that developing ML systems with care requires vigilance and responding to feedback from those deployment contexts, which in turn we can facilitate by sharing lessons across contexts and developing tools to analyze signs of bias at every level of ML development._\n\n_This blog post from the Ethics and Society regulars @ shares some of the lessons we have learned along with tools we have developed to support ourselves and others in our community’s efforts to better address bias in Machine Learning. The first part is a broader reflection on bias and its context. If you’ve already read it and are coming back specifically for the tools, feel free to jump to the datasets or models\nsection!_\n\n<p align=\"center\">\n <br>\n <img src=\"URL alt=\"Selection of tools developed by HF team members to address bias in ML\" />\n <em>Selection of tools developed by team members to address bias in ML</em>\n</p>\n\n<span style=\"text-decoration:underline;\">Table of contents:</span>\n* <span style=\"text-decoration:underline;\">On Machine Biases</span>\n * Machine Bias: from ML Systems to Risks\n * Putting Bias in Context\n* <span style=\"text-decoration:underline;\">Tools and Recommendations</span>\n * Addressing Bias throughout ML Development\n * Task Definition\n * Dataset Curation\n * Model Training\n * Overview of Bias Tools",
"## _Machine Bias:_ from ML Systems to Personal and Social Risks\n\nML systems allow us to automate complex tasks at a scale never seen before as they are deployed in more sectors and use cases. When the technology works at its best, it can help smooth interactions between people and technical systems, remove the need for highly repetitive work, or unlock new ways of processing information to support research.\n\nThese same systems are also likely to reproduce discriminatory and abusive behaviors represented in their training data, especially when the data encodes human behaviors.\nThe technology then has the potential to make these issues significantly worse. Automation and deployment at scale can indeed:\n\n1. lock in behaviors in time and hinder social progress from being reflected in technology, \n2. spread harmful behaviors beyond the context of the original training data,\n3. amplify inequities by overfocusing on stereotypical associations when making predictions,\n4. remove possibilities for recourse by hiding biases inside “black-box” systems.\n\nIn order to better understand and address these risks, ML researchers and developers have started studying _machine bias_ or _algorithmic bias_, mechanisms that might lead systems to, for example, encode negative stereotypes or associations or to have disparate performance for different population groups in their deployment context.\n\nThese issues are deeply personal for many of us ML researchers and developers at Hugging Face and in the broader ML community. Hugging Face is an international company, with many of us existing between countries and cultures. It is hard to fully express our sense of urgency when we see the technology we work on developed without sufficient concern for protecting people like us; especially when these systems lead to discriminatory wrongful arrests or undue financial distress and are being increasingly sold to immigration and law enforcement services around the world. Similarly, seeing our identities routinely suppressed in training datasets or underrepresented in the outputs of “generative AI” systems connects these concerns to our daily lived experiences in ways that are simultaneously enlightening and taxing.\n\nWhile our own experiences do not come close to covering the myriad ways in which ML-mediated discrimination can disproportionately harm people whose experiences differ from ours, they provide an entry point into considerations of the trade-offs inherent in the technology. We work on these systems because we strongly believe in ML’s potential — we think it can shine as a valuable tool as long as it is developed with care and input from people in its deployment context, rather than as a one-size-fits-all panacea. In particular, enabling this care requires developing a better understanding of the mechanisms of machine bias across the ML development process, and developing tools that support people with all levels of technical knowledge of these systems in participating in the necessary conversations about how their benefits and harms are distributed.\n\nThe present blog post from the Hugging Face Ethics and Society regulars provides an overview of how we have worked, are working, or recommend users of the HF ecosystem of libraries may work to address bias at the various stages of the ML development process, and the tools we develop to support this process. We hope you will find it a useful resource to guide concrete considerations of the social impact of your work and can leverage the tools referenced here to help mitigate these issues when they arise.",
"## Putting Bias in Context\n\nThe first and maybe most important concept to consider when dealing with machine bias is context. In their foundational work on bias in NLP, Su Lin Blodgett et al. point out that: _“[T]he majority of [academic works on machine bias] fail to engage critically with what constitutes “bias” in the first place”_, including by building their work on top of _“unstated assumptions about what kinds of system behaviors are harmful, in what ways, to whom, and why”_.\n\nThis may not come as much of a surprise given the ML research community’s focus on the value of “generalization” — the most cited motivation for work in the field after “performance”. However, while tools for bias assessment that apply to a wide range of settings are valuable to enable a broader analysis of common trends in model behaviors, their ability to target the mechanisms that lead to discrimination in concrete use cases is inherently limited. Using them to guide specific decisions within the ML development cycle usually requires an extra step or two to take the system’s specific use context and affected people into consideration.\n\n<p align=\"center\">\n <br>\n <img src=\"URL alt=\"Excerpt on considerations of ML uses context and people from the Model Card Guidebook\" />\n <em>Excerpt on considerations of ML uses context and people from the <a href=\"URL Card Guidebook</a></em>\n</p>\n\nNow let’s dive deeper into the issue of linking biases in stand-alone/context-less ML artifacts to specific harms. It can be useful to think of machine biases as risk factors for discrimination-based harms. Take the example of a text-to-image model that over-represents light skin tones when prompted to create a picture of a person in a professional setting, but produces darker skin tones when the prompts mention criminality. These tendencies would be what we call _machine biases at the model level_. Now let’s think about a few systems that use such a text-to-image model:\n\n\n1. <span style=\"text-decoration:underline;\">The model is integrated into a website creation service</span> (e.g. SquareSpace, Wix) to help users generate backgrounds for their pages. The model explicitly disables images of people in the generated background.\n * In this case, the machine bias “risk factor” does not lead to discrimination harm because the focus of the bias (images of people) is absent from the use case.\n * Further risk mitigation is not required for machine biases, although developers should be aware of ongoing discussions about the legality of integrating systems trained on scraped data in commercial systems.\n2. <span style=\"text-decoration:underline;\">The model is integrated into a stock images website</span> to provide users with synthetic images of people (e.g. in professional settings) that they can use with fewer privacy concerns, for example, to serve as illustrations for Wikipedia articles\n * In this case, machine bias acts to lock in and amplify existing social biases. It reinforces stereotypes about people (“CEOs are all white men”) that then feed back into complex social systems where increased bias leads to increased discrimination in many different ways (such as reinforcing implicit bias in the workplace).\n * Mitigation strategies may include educating the stock image users about these biases, or the stock image website may curate generated images to intentionally propose a more diverse set of representations.\n3. <span style=\"text-decoration:underline;\">The model is integrated into a “virtual sketch artist” software</span> marketed to police departments that will use it to generate pictures of suspects based on verbal testimony\n * In this case, the machine biases directly cause discrimination by systematically directing police departments to darker-skinned people, putting them at increased risk of harm including physical injury and unlawful imprisonment.\n * In cases like this one, there may be no level of bias mitigation that makes the risk acceptable. In particular, such a use case would be closely related to face recognition in the context of law enforcement, where similar bias issues have led several commercial entities and legislatures to adopt moratoria pausing or banning its use across the board.\n\nSo, who’s on the hook for machine biases in ML? These three cases illustrate one of the reasons why discussions about the responsibility of ML developers in addressing bias can get so complicated: depending on decisions made at other points in the ML system development process by other people, the biases in an ML dataset or model may land anywhere between being irrelevant to the application settings and directly leading to grievous harm. However, in all of these cases, stronger biases in the model/dataset increase the risk of negative outcomes. The European Union has started to develop frameworks that address this phenomenon in recent regulatory efforts: in short, a company that deploys an AI system based on a measurably biased model is liable for harm caused by the system.\n\nConceptualizing bias as a risk factor then allows us to better understand the shared responsibility for machine biases between developers at all stages. Bias can never be fully removed, not least because the definitions of social biases and the power dynamics that tie them to discrimination vary vastly across social contexts. However:\n\n\n\n1. Each stage of the development process, from task specification, dataset curation, and model training, to model integration and system deployment, can take steps to minimize the aspects of machine bias that most directly depend on its choices and technical decisions, and\n2. Clear communication and information flow between the various ML development stages can make the difference between making choices that build on top of each other to attenuate the negative potential of bias (multipronged approach to bias mitigation, as in deployment scenario 1 above) _versus_ making choices that compound this negative potential to exacerbate the risk of harm (as in deployment scenario 3).\n\nIn the next section, we review these various stages along with some of the tools that can help us address machine bias at each of them.",
"## Addressing Bias Throughout the ML Development Cycle\n\nReady for some practical advice yet? Here we go \n\nThere is no one single way to develop ML systems; which steps happen in what order depends on a number of factors including the development setting (university, large company, startup, grassroots organization, etc…), the modality (text, tabular data, images, etc…), and the preeminence or scarcity of publicly available ML resources. However, we can identify three common stages of particular interest in addressing bias. These are the task definition, the data curation, and the model training. Let’s have a look at how bias handling may differ across these various stages.\n\n<p align=\"center\">\n <br>\n <img src=\"URL alt=\"The Bias ML Pipeline by Meg\" width=\"500\" />\n <em>The Bias ML Pipeline by <a href=\"URL\n</p>",
"### I am <span style=\"text-decoration:underline;\">defining the task</span> of my ML system, how can I address bias?\n\nWhether and to what extent bias in the system concretely affects people ultimately depends on what the system is used for. As such, the first place developers can work to mitigate bias is when deciding how ML fits in their system, e.g., by deciding what optimization objective it will use.\n\nFor example, let’s go back to one of the first highly-publicized cases of a Machine Learning system used in production for algorithmic content recommendation. From 2006 to 2009, Netflix ran the Netflix Prize, a competition with a 1M$ cash prize challenging teams around the world to develop ML systems to accurately predict a user’s rating for a new movie based on their past ratings. The winning submission improved the RMSE (Root-mean-square-error) of predictions on unseen user-movie pairs by over 10% over Netflix’s own CineMatch algorithm, meaning it got much better at predicting how users would rate a new movie based on their history. This approach opened the door for much of modern algorithmic content recommendation by bringing the role of ML in modeling user preferences in recommender systems to public awareness.\n\nSo what does this have to do with bias? Doesn’t showing people content that they’re likely to enjoy sound like a good service from a content platform? Well, it turns out that showing people more examples of what they’ve liked in the past ends up reducing the diversity of the media they consume. Not only does it lead users to be less satisfied in the long term, but it also means that any biases or stereotypes captured by the initial models — such as when modeling the preferences of Black American users or dynamics that systematically disadvantage some artists — are likely to be reinforced if the model is further trained on ongoing ML-mediated user interactions. This reflects two of the types of bias-related concerns we’ve mentioned above: the training objective acts as a risk factor for bias-related harms as it makes pre-existing biases much more likely to show up in predictions, and the task framing has the effect of locking in and exacerbating past biases.\n\nA promising bias mitigation strategy at this stage has been to reframe the task to explicitly model both engagement and diversity when applying ML to algorithmic content recommendation. Users are likely to get more long-term satisfaction and the risk of exacerbating biases as outlined above is reduced!\n\nThis example serves to illustrate that the impact of machine biases in an ML-supported product depends not just on where we decide to leverage ML, but also on how ML techniques are integrated into the broader technical system, and with what objective. When first investigating how ML can fit into a product or a use case you are interested in, we first recommend looking for the failure modes of the system through the lens of bias before even diving into the available models or datasets - which behaviors of existing systems in the space will be particularly harmful or more likely to occur if bias is exacerbated by ML predictions?\n\nWe built a tool to take users through these questions in another case of algorithmic content management: hate speech detection in automatic content moderation. We found for example that looking through news and scientific articles that didn’t particularly focus on the ML part of the technology was already a great way to get a sense of where bias is already at play. Definitely go have a look for an example of how the models and datasets fit with the deployment context and how they can relate to known bias-related harms!\n\n<p align=\"center\">\n <br>\n <img src=\"URL alt=\"Selection of tools developed by HF team members to address bias in ML\" />\n <em><a href=\"URL Task Exploration tool</a> by <a href=\"URL <a href=\"URL and <a href=\"URL\n</p>",
"#### Task definition: recommendations\n\nThere are as many ways for the ML task definition and deployment to affect the risk of bias-related harms as there are applications for ML systems. As in the examples above, some common steps that may help decide whether and how to apply ML in a way that minimizes bias-related risk include:\n\n* Investigate:\n * Reports of bias in the field pre-ML\n * At-risk demographic categories for your specific use case\n* Examine:\n * The impact of your optimization objective on reinforcing biases\n * Alternative objectives that favor diversity and positive long-term impacts",
"### I am <span style=\"text-decoration:underline;\">curating/picking a dataset</span> for my ML system, how can I address bias?\n\nWhile training datasets are not the sole source of bias00061-1) in the ML development cycle, they do play a significant role. Does your dataset disproportionately associate biographies of women with life events but those of men with achievements? Those stereotypes are probably going to show up in your full ML system! Does your voice recognition dataset only feature specific accents? Not a good sign for the inclusivity of technology you build with it in terms of disparate performance! Whether you’re curating a dataset for ML applications or selecting a dataset to train an ML model, finding out, mitigating, and communicating to what extent the data exhibits these phenomena are all necessary steps to reducing bias-related risks.\n\nYou can usually get a pretty good sense of likely biases in a dataset by reflecting on where it comes from, who are the people represented on the data, and what the curation process was. Several frameworks for this reflection and documentation have been proposed such as Data Statements for NLP or Datasheets for Datasets. The Hugging Face Hub includes a Dataset Card template and guide inspired by these works; the section on considerations for using the data is usually a good place to look for information about notable biases if you’re browsing datasets, or to write a paragraph sharing your insights on the topic if you’re sharing a new one. And if you’re looking for more inspiration on what to put there, check out these sections written by Hub users in the BigLAM organization for historical datasets of legal proceedings, image classification, and newspapers.\n\n\n<p align=\"center\">\n <br>\n <img src=\"URL alt=\"HF Dataset Card guide for the Social Impact and Bias Sections\" />\n <em><a href=\"URL Dataset Card guide</a> for the Social Impact and Bias Sections</em>\n</p>\n\nWhile describing the origin and context of a dataset is always a good starting point to understand the biases at play, quantitatively measuring phenomena that encode those biases can be just as helpful. If you’re choosing between two different datasets for a given task or choosing between two ML models trained on different datasets, knowing which one better represents the demographic makeup of your ML system’s user base can help you make an informed decision to minimize bias-related risks. If you’re curating a dataset iteratively by filtering data points from a source or selecting new sources of data to add, measuring how these choices affect the diversity and biases present in your overall dataset can make it safer to use in general.\n\nWe’ve recently released two tools you can leverage to measure your data through a bias-informed lens. The disaggregators library provides utilities to quantify the composition of your dataset, using either metadata or leveraging models to infer properties of data points. This can be particularly useful to minimize risks of bias-related representation harms or disparate performances of trained models. Look at the demo to see it applied to the LAION, MedMCQA, and The Stack datasets!\n\n\n<p align=\"center\">\n <br>\n <img src=\"URL alt=\"Disaggregators tool by Nima\" />\n <em><a href=\"URL tool</a> by <a href=\"URL\n</p>\n\nOnce you have some helpful statistics about the composition of your dataset, you’ll also want to look at associations between features in your data items, particularly at associations that may encode derogatory or otherwise negative stereotypes. The Data Measurements Tool we originally introduced last year allows you to do this by looking at the normalized Pointwise Mutual Information (nPMI) between terms in your text-based dataset; particularly associations between gendered pronouns that may denote gendered stereotypes. Run it yourself or try it here on a few pre-computed datasets!\n\n\n<p align=\"center\">\n <br>\n <img src=\"URL alt=\"Data Measurements tool by Meg, Sasha, Bibi, and the Gradio team\" />\n <em><a href=\"URL Measurements tool</a> by <a href=\"URL <a href=\"URL <a href=\"URL and the <a href=\"URL team</a></em>\n</p>",
"#### Dataset selection/curation: recommendations\n\nThese tools aren’t full solutions by themselves, rather, they are designed to support critical examination and improvement of datasets through several lenses, including the lens of bias and bias-related risks. In general, we encourage you to keep the following steps in mind when leveraging these and other tools to mitigate bias risks at the dataset curation/selection stage:\n\n* Identify:\n * Aspects of the dataset creation that may exacerbate specific biases\n * Demographic categories and social variables that are particularly important to the dataset’s task and domain\n* Measure:\n * The demographic distribution in your dataset\n * Pre-identified negative stereotypes represented\n* Document:\n * Share what you’ve Identified and Measured in your Dataset Card so it can benefit other users, developers, and otherwise affected people\n* Adapt:\n * By choosing the dataset least likely to cause bias-related harms\n * By iteratively improving your dataset in ways that reduce bias risks",
"### I am <span style=\"text-decoration:underline;\">training/selecting a model</span> for my ML system, how can I address bias?\n\nSimilar to the dataset curation/selection step, documenting and measuring bias-related phenomena in models can help both ML developers who are selecting a model to use as-is or to finetune and ML developers who want to train their own models. For the latter, measures of bias-related phenomena in the model can help them learn from what has worked or what hasn’t for other models and serve as a signal to guide their own development choices.\n\nModel cards were originally proposed by (Mitchell et al., 2019) and provide a framework for model reporting that showcases information relevant to bias risks, including broad ethical considerations, disaggregated evaluation, and use case recommendation. The Hugging Face Hub provides even more tools for model documentation, with a model card guidebook in the Hub documentation, and an app that lets you create extensive model cards easily for your new model.\n\n<p align=\"center\">\n <br>\n <img src=\"URL alt=\"Model Card writing tool by Ezi, Marissa, and Meg\" />\n <em><a href=\"URL Card writing tool</a> by <a href=\"URL <a href=\"URL and <a href=\"URL\n</p>\n\nDocumentation is a great first step for sharing general insights about a model’s behavior, but it is usually static and presents the same information to all users. In many cases, especially for generative models that can generate outputs to approximate the distribution of their training data, we can gain a more contextual understanding of bias-related phenomena and negative stereotypes by visualizing and contrasting model outputs. Access to model generations can help users bring intersectional issues in the model behavior corresponding to their lived experience, and evaluate to what extent a model reproduces gendered stereotypes for different adjectives. To facilitate this process, we built a tool that lets you compare generations not just across a set of adjectives and professions, but also across different models! Go try it out to get a sense of which model might carry the least bias risks in your use case.\n\n<p align=\"center\">\n <br>\n <img src=\"URL alt=\"Visualize Adjective and Occupation Biases in Image Generation by Sasha\" />\n <br>\n <em><a href=\"URL Adjective and Occupation Biases in Image Generation</a> by <a href=\"URL\n</p>\n\nVisualization of model outputs isn’t just for generative models though! For classification models, we also want to look out for bias-related harms caused by a model’s disparate performance on different demographics. If you know what protected classes are most at risk of discrimination and have those annotated in an evaluation set, then you can report disaggregated performance over the different categories in your model card as mentioned above, so users can make informed decisions. If however, you are worried that you haven’t identified all populations at risk of bias-related harms, or if you do not have access to annotated test examples to measure the biases you suspect, that’s where interactive visualizations of where and how the model fails come in handy! To help you with this, the SEAL app groups similar mistakes by your model and shows you some common features in each cluster. If you want to go further, you can even combine it with the disaggregators library we introduced in the datasets section to find clusters that are indicative of bias-related failure modes!\n \n\n<p align=\"center\">\n <br>\n <img src=\"URL alt=\"Systematic Error Analysis and Labeling (SEAL) by Nazneen\" />\n <em><a href=\"URL Error Analysis and Labeling (SEAL)</a> by <a href=\"URL\n</p>\n\nFinally, a few benchmarks exist that can measure bias-related phenomena in models. For language models, benchmarks such as BOLD, HONEST, or WinoBias provide quantitative evaluations of targeted behaviors that are indicative of biases in the models. While the benchmarks have their limitations, they do provide a limited view into some pre-identified bias risks that can help describe how the models function or choose between different models. You can find these evaluations pre-computed on a range of common language models in this exploration Space to get a first sense of how they compare!\n\n\n<p align=\"center\">\n <br>\n <img src=\"URL alt=\"Language Model Bias Detection by Sasha\" />\n <em><a href=\"URL Model Bias Detection</a> by <a href=\"URL\n</p>\n\nEven with access to a benchmark for the models you are considering, you might find that running evaluations of the larger language models you are considering can be prohibitively expensive or otherwise technically impossible with your own computing resources. The <a href=\"URL on the Hub</a> tool we released this year can help with that: not only will it run the evaluations for you, but it will also help connect them to the model documentation so the results are available once and for all — so everyone can see, for example, that size <a href=\"URL increases bias risks in models like OPT</a>!\n\n<p align=\"center\">\n <br>\n <img src=\"URL alt=\"Large model WinoBias scores computed with Evaluation on the Hub by Helen, Tristan, Abhishek, Lewis, and Douwe\" />\n <em><a href=\"URL href=\"URL model WinoBias scores computed with Evaluation on the Hub</a> by <a href=\"URL <a href=\"URL <a href=\"URL <a href=\"URL and <a href=\"URL\n</p>",
"#### Model selection/development: recommendations\n\nFor models just as for datasets, different tools for documentation and evaluation will provide different views of bias risks in a model which all have a part to play in helping developers choose, develop, or understand ML systems.\n\n* Visualize\n * Generative model: visualize how the model’s outputs may reflect stereotypes\n * Classification model: visualize model errors to identify failure modes that could lead to disparate performance\n* Evaluate\n * When possible, evaluate models on relevant benchmarks\n* Document\n * Share your learnings from visualization and qualitative evaluation\n * Report your model’s disaggregated performance and results on applicable fairness benchmarks",
"## Conclusion and Overview of Bias Analysis and Documentation Tools from \n\nAs we learn to leverage ML systems in more and more applications, reaping their benefits equitably will depend on our ability to actively mitigate the risks of bias-related harms associated with the technology. While there is no single answer to the question of how this should best be done in any possible setting, we can support each other in this effort by sharing lessons, tools, and methodologies to mitigate and document those risks. The present blog post outlines some of the ways Hugging Face team members have addressed this question of bias along with supporting tools, we hope that you will find them helpful and encourage you to develop and share your own!\n\nSummary of linked tools:\n* Tasks:\n * Explore our directory of ML Tasks to understand what technical framings and resources are available to choose from\n * Use tools to explore the full development lifecycle of specific tasks\n* Datasets:\n * Make use of and contribute to Dataset Cards to share relevant insights on biases in datasets.\n * Use Disaggregator to look for possible disparate performance\n * Look at aggregated measurements of your dataset including nPMI to surface possible stereotypical associations\n* Models:\n * Make use of and contribute to Model Cards to share relevant insights on biases in models.\n * Use Interactive Model Cards to visualize performance discrepancies\n * Look at systematic model errors and look out for known social biases\n * Use Evaluate and Evaluation on the Hub to explore language model biases including in large models\n * Use a Text-to-image bias explorer to compare image generation models’ biases\n * Compare LM models with Bias Score Card\n\nThanks for reading! \n\n~ Yacine, on behalf of the Ethics and Society regulars\n\nCite as:"
] | [
"TAGS\n#license-cc-by-4.0 #arxiv-2203.07785 #arxiv-2010.03058 #arxiv-2211.03759 #arxiv-2209.03942 #arxiv-2212.05129 #doi-10.57967/hf/0214 #region-us \n",
"# Ethics and Society Newsletter #2",
"## Let’s Talk about Bias!\n\n_Bias in ML is ubiquitous, and Bias in ML is complex; so complex in fact that no single technical intervention is likely to meaningfully address the problems it engenders. ML models, as sociotechnical systems, amplify social trends that may exacerbate inequities and harmful biases in ways that depend on their deployment context and are constantly evolving._\n\n_This means that developing ML systems with care requires vigilance and responding to feedback from those deployment contexts, which in turn we can facilitate by sharing lessons across contexts and developing tools to analyze signs of bias at every level of ML development._\n\n_This blog post from the Ethics and Society regulars @ shares some of the lessons we have learned along with tools we have developed to support ourselves and others in our community’s efforts to better address bias in Machine Learning. The first part is a broader reflection on bias and its context. If you’ve already read it and are coming back specifically for the tools, feel free to jump to the datasets or models\nsection!_\n\n<p align=\"center\">\n <br>\n <img src=\"URL alt=\"Selection of tools developed by HF team members to address bias in ML\" />\n <em>Selection of tools developed by team members to address bias in ML</em>\n</p>\n\n<span style=\"text-decoration:underline;\">Table of contents:</span>\n* <span style=\"text-decoration:underline;\">On Machine Biases</span>\n * Machine Bias: from ML Systems to Risks\n * Putting Bias in Context\n* <span style=\"text-decoration:underline;\">Tools and Recommendations</span>\n * Addressing Bias throughout ML Development\n * Task Definition\n * Dataset Curation\n * Model Training\n * Overview of Bias Tools",
"## _Machine Bias:_ from ML Systems to Personal and Social Risks\n\nML systems allow us to automate complex tasks at a scale never seen before as they are deployed in more sectors and use cases. When the technology works at its best, it can help smooth interactions between people and technical systems, remove the need for highly repetitive work, or unlock new ways of processing information to support research.\n\nThese same systems are also likely to reproduce discriminatory and abusive behaviors represented in their training data, especially when the data encodes human behaviors.\nThe technology then has the potential to make these issues significantly worse. Automation and deployment at scale can indeed:\n\n1. lock in behaviors in time and hinder social progress from being reflected in technology, \n2. spread harmful behaviors beyond the context of the original training data,\n3. amplify inequities by overfocusing on stereotypical associations when making predictions,\n4. remove possibilities for recourse by hiding biases inside “black-box” systems.\n\nIn order to better understand and address these risks, ML researchers and developers have started studying _machine bias_ or _algorithmic bias_, mechanisms that might lead systems to, for example, encode negative stereotypes or associations or to have disparate performance for different population groups in their deployment context.\n\nThese issues are deeply personal for many of us ML researchers and developers at Hugging Face and in the broader ML community. Hugging Face is an international company, with many of us existing between countries and cultures. It is hard to fully express our sense of urgency when we see the technology we work on developed without sufficient concern for protecting people like us; especially when these systems lead to discriminatory wrongful arrests or undue financial distress and are being increasingly sold to immigration and law enforcement services around the world. Similarly, seeing our identities routinely suppressed in training datasets or underrepresented in the outputs of “generative AI” systems connects these concerns to our daily lived experiences in ways that are simultaneously enlightening and taxing.\n\nWhile our own experiences do not come close to covering the myriad ways in which ML-mediated discrimination can disproportionately harm people whose experiences differ from ours, they provide an entry point into considerations of the trade-offs inherent in the technology. We work on these systems because we strongly believe in ML’s potential — we think it can shine as a valuable tool as long as it is developed with care and input from people in its deployment context, rather than as a one-size-fits-all panacea. In particular, enabling this care requires developing a better understanding of the mechanisms of machine bias across the ML development process, and developing tools that support people with all levels of technical knowledge of these systems in participating in the necessary conversations about how their benefits and harms are distributed.\n\nThe present blog post from the Hugging Face Ethics and Society regulars provides an overview of how we have worked, are working, or recommend users of the HF ecosystem of libraries may work to address bias at the various stages of the ML development process, and the tools we develop to support this process. We hope you will find it a useful resource to guide concrete considerations of the social impact of your work and can leverage the tools referenced here to help mitigate these issues when they arise.",
"## Putting Bias in Context\n\nThe first and maybe most important concept to consider when dealing with machine bias is context. In their foundational work on bias in NLP, Su Lin Blodgett et al. point out that: _“[T]he majority of [academic works on machine bias] fail to engage critically with what constitutes “bias” in the first place”_, including by building their work on top of _“unstated assumptions about what kinds of system behaviors are harmful, in what ways, to whom, and why”_.\n\nThis may not come as much of a surprise given the ML research community’s focus on the value of “generalization” — the most cited motivation for work in the field after “performance”. However, while tools for bias assessment that apply to a wide range of settings are valuable to enable a broader analysis of common trends in model behaviors, their ability to target the mechanisms that lead to discrimination in concrete use cases is inherently limited. Using them to guide specific decisions within the ML development cycle usually requires an extra step or two to take the system’s specific use context and affected people into consideration.\n\n<p align=\"center\">\n <br>\n <img src=\"URL alt=\"Excerpt on considerations of ML uses context and people from the Model Card Guidebook\" />\n <em>Excerpt on considerations of ML uses context and people from the <a href=\"URL Card Guidebook</a></em>\n</p>\n\nNow let’s dive deeper into the issue of linking biases in stand-alone/context-less ML artifacts to specific harms. It can be useful to think of machine biases as risk factors for discrimination-based harms. Take the example of a text-to-image model that over-represents light skin tones when prompted to create a picture of a person in a professional setting, but produces darker skin tones when the prompts mention criminality. These tendencies would be what we call _machine biases at the model level_. Now let’s think about a few systems that use such a text-to-image model:\n\n\n1. <span style=\"text-decoration:underline;\">The model is integrated into a website creation service</span> (e.g. SquareSpace, Wix) to help users generate backgrounds for their pages. The model explicitly disables images of people in the generated background.\n * In this case, the machine bias “risk factor” does not lead to discrimination harm because the focus of the bias (images of people) is absent from the use case.\n * Further risk mitigation is not required for machine biases, although developers should be aware of ongoing discussions about the legality of integrating systems trained on scraped data in commercial systems.\n2. <span style=\"text-decoration:underline;\">The model is integrated into a stock images website</span> to provide users with synthetic images of people (e.g. in professional settings) that they can use with fewer privacy concerns, for example, to serve as illustrations for Wikipedia articles\n * In this case, machine bias acts to lock in and amplify existing social biases. It reinforces stereotypes about people (“CEOs are all white men”) that then feed back into complex social systems where increased bias leads to increased discrimination in many different ways (such as reinforcing implicit bias in the workplace).\n * Mitigation strategies may include educating the stock image users about these biases, or the stock image website may curate generated images to intentionally propose a more diverse set of representations.\n3. <span style=\"text-decoration:underline;\">The model is integrated into a “virtual sketch artist” software</span> marketed to police departments that will use it to generate pictures of suspects based on verbal testimony\n * In this case, the machine biases directly cause discrimination by systematically directing police departments to darker-skinned people, putting them at increased risk of harm including physical injury and unlawful imprisonment.\n * In cases like this one, there may be no level of bias mitigation that makes the risk acceptable. In particular, such a use case would be closely related to face recognition in the context of law enforcement, where similar bias issues have led several commercial entities and legislatures to adopt moratoria pausing or banning its use across the board.\n\nSo, who’s on the hook for machine biases in ML? These three cases illustrate one of the reasons why discussions about the responsibility of ML developers in addressing bias can get so complicated: depending on decisions made at other points in the ML system development process by other people, the biases in an ML dataset or model may land anywhere between being irrelevant to the application settings and directly leading to grievous harm. However, in all of these cases, stronger biases in the model/dataset increase the risk of negative outcomes. The European Union has started to develop frameworks that address this phenomenon in recent regulatory efforts: in short, a company that deploys an AI system based on a measurably biased model is liable for harm caused by the system.\n\nConceptualizing bias as a risk factor then allows us to better understand the shared responsibility for machine biases between developers at all stages. Bias can never be fully removed, not least because the definitions of social biases and the power dynamics that tie them to discrimination vary vastly across social contexts. However:\n\n\n\n1. Each stage of the development process, from task specification, dataset curation, and model training, to model integration and system deployment, can take steps to minimize the aspects of machine bias that most directly depend on its choices and technical decisions, and\n2. Clear communication and information flow between the various ML development stages can make the difference between making choices that build on top of each other to attenuate the negative potential of bias (multipronged approach to bias mitigation, as in deployment scenario 1 above) _versus_ making choices that compound this negative potential to exacerbate the risk of harm (as in deployment scenario 3).\n\nIn the next section, we review these various stages along with some of the tools that can help us address machine bias at each of them.",
"## Addressing Bias Throughout the ML Development Cycle\n\nReady for some practical advice yet? Here we go \n\nThere is no one single way to develop ML systems; which steps happen in what order depends on a number of factors including the development setting (university, large company, startup, grassroots organization, etc…), the modality (text, tabular data, images, etc…), and the preeminence or scarcity of publicly available ML resources. However, we can identify three common stages of particular interest in addressing bias. These are the task definition, the data curation, and the model training. Let’s have a look at how bias handling may differ across these various stages.\n\n<p align=\"center\">\n <br>\n <img src=\"URL alt=\"The Bias ML Pipeline by Meg\" width=\"500\" />\n <em>The Bias ML Pipeline by <a href=\"URL\n</p>",
"### I am <span style=\"text-decoration:underline;\">defining the task</span> of my ML system, how can I address bias?\n\nWhether and to what extent bias in the system concretely affects people ultimately depends on what the system is used for. As such, the first place developers can work to mitigate bias is when deciding how ML fits in their system, e.g., by deciding what optimization objective it will use.\n\nFor example, let’s go back to one of the first highly-publicized cases of a Machine Learning system used in production for algorithmic content recommendation. From 2006 to 2009, Netflix ran the Netflix Prize, a competition with a 1M$ cash prize challenging teams around the world to develop ML systems to accurately predict a user’s rating for a new movie based on their past ratings. The winning submission improved the RMSE (Root-mean-square-error) of predictions on unseen user-movie pairs by over 10% over Netflix’s own CineMatch algorithm, meaning it got much better at predicting how users would rate a new movie based on their history. This approach opened the door for much of modern algorithmic content recommendation by bringing the role of ML in modeling user preferences in recommender systems to public awareness.\n\nSo what does this have to do with bias? Doesn’t showing people content that they’re likely to enjoy sound like a good service from a content platform? Well, it turns out that showing people more examples of what they’ve liked in the past ends up reducing the diversity of the media they consume. Not only does it lead users to be less satisfied in the long term, but it also means that any biases or stereotypes captured by the initial models — such as when modeling the preferences of Black American users or dynamics that systematically disadvantage some artists — are likely to be reinforced if the model is further trained on ongoing ML-mediated user interactions. This reflects two of the types of bias-related concerns we’ve mentioned above: the training objective acts as a risk factor for bias-related harms as it makes pre-existing biases much more likely to show up in predictions, and the task framing has the effect of locking in and exacerbating past biases.\n\nA promising bias mitigation strategy at this stage has been to reframe the task to explicitly model both engagement and diversity when applying ML to algorithmic content recommendation. Users are likely to get more long-term satisfaction and the risk of exacerbating biases as outlined above is reduced!\n\nThis example serves to illustrate that the impact of machine biases in an ML-supported product depends not just on where we decide to leverage ML, but also on how ML techniques are integrated into the broader technical system, and with what objective. When first investigating how ML can fit into a product or a use case you are interested in, we first recommend looking for the failure modes of the system through the lens of bias before even diving into the available models or datasets - which behaviors of existing systems in the space will be particularly harmful or more likely to occur if bias is exacerbated by ML predictions?\n\nWe built a tool to take users through these questions in another case of algorithmic content management: hate speech detection in automatic content moderation. We found for example that looking through news and scientific articles that didn’t particularly focus on the ML part of the technology was already a great way to get a sense of where bias is already at play. Definitely go have a look for an example of how the models and datasets fit with the deployment context and how they can relate to known bias-related harms!\n\n<p align=\"center\">\n <br>\n <img src=\"URL alt=\"Selection of tools developed by HF team members to address bias in ML\" />\n <em><a href=\"URL Task Exploration tool</a> by <a href=\"URL <a href=\"URL and <a href=\"URL\n</p>",
"#### Task definition: recommendations\n\nThere are as many ways for the ML task definition and deployment to affect the risk of bias-related harms as there are applications for ML systems. As in the examples above, some common steps that may help decide whether and how to apply ML in a way that minimizes bias-related risk include:\n\n* Investigate:\n * Reports of bias in the field pre-ML\n * At-risk demographic categories for your specific use case\n* Examine:\n * The impact of your optimization objective on reinforcing biases\n * Alternative objectives that favor diversity and positive long-term impacts",
"### I am <span style=\"text-decoration:underline;\">curating/picking a dataset</span> for my ML system, how can I address bias?\n\nWhile training datasets are not the sole source of bias00061-1) in the ML development cycle, they do play a significant role. Does your dataset disproportionately associate biographies of women with life events but those of men with achievements? Those stereotypes are probably going to show up in your full ML system! Does your voice recognition dataset only feature specific accents? Not a good sign for the inclusivity of technology you build with it in terms of disparate performance! Whether you’re curating a dataset for ML applications or selecting a dataset to train an ML model, finding out, mitigating, and communicating to what extent the data exhibits these phenomena are all necessary steps to reducing bias-related risks.\n\nYou can usually get a pretty good sense of likely biases in a dataset by reflecting on where it comes from, who are the people represented on the data, and what the curation process was. Several frameworks for this reflection and documentation have been proposed such as Data Statements for NLP or Datasheets for Datasets. The Hugging Face Hub includes a Dataset Card template and guide inspired by these works; the section on considerations for using the data is usually a good place to look for information about notable biases if you’re browsing datasets, or to write a paragraph sharing your insights on the topic if you’re sharing a new one. And if you’re looking for more inspiration on what to put there, check out these sections written by Hub users in the BigLAM organization for historical datasets of legal proceedings, image classification, and newspapers.\n\n\n<p align=\"center\">\n <br>\n <img src=\"URL alt=\"HF Dataset Card guide for the Social Impact and Bias Sections\" />\n <em><a href=\"URL Dataset Card guide</a> for the Social Impact and Bias Sections</em>\n</p>\n\nWhile describing the origin and context of a dataset is always a good starting point to understand the biases at play, quantitatively measuring phenomena that encode those biases can be just as helpful. If you’re choosing between two different datasets for a given task or choosing between two ML models trained on different datasets, knowing which one better represents the demographic makeup of your ML system’s user base can help you make an informed decision to minimize bias-related risks. If you’re curating a dataset iteratively by filtering data points from a source or selecting new sources of data to add, measuring how these choices affect the diversity and biases present in your overall dataset can make it safer to use in general.\n\nWe’ve recently released two tools you can leverage to measure your data through a bias-informed lens. The disaggregators library provides utilities to quantify the composition of your dataset, using either metadata or leveraging models to infer properties of data points. This can be particularly useful to minimize risks of bias-related representation harms or disparate performances of trained models. Look at the demo to see it applied to the LAION, MedMCQA, and The Stack datasets!\n\n\n<p align=\"center\">\n <br>\n <img src=\"URL alt=\"Disaggregators tool by Nima\" />\n <em><a href=\"URL tool</a> by <a href=\"URL\n</p>\n\nOnce you have some helpful statistics about the composition of your dataset, you’ll also want to look at associations between features in your data items, particularly at associations that may encode derogatory or otherwise negative stereotypes. The Data Measurements Tool we originally introduced last year allows you to do this by looking at the normalized Pointwise Mutual Information (nPMI) between terms in your text-based dataset; particularly associations between gendered pronouns that may denote gendered stereotypes. Run it yourself or try it here on a few pre-computed datasets!\n\n\n<p align=\"center\">\n <br>\n <img src=\"URL alt=\"Data Measurements tool by Meg, Sasha, Bibi, and the Gradio team\" />\n <em><a href=\"URL Measurements tool</a> by <a href=\"URL <a href=\"URL <a href=\"URL and the <a href=\"URL team</a></em>\n</p>",
"#### Dataset selection/curation: recommendations\n\nThese tools aren’t full solutions by themselves, rather, they are designed to support critical examination and improvement of datasets through several lenses, including the lens of bias and bias-related risks. In general, we encourage you to keep the following steps in mind when leveraging these and other tools to mitigate bias risks at the dataset curation/selection stage:\n\n* Identify:\n * Aspects of the dataset creation that may exacerbate specific biases\n * Demographic categories and social variables that are particularly important to the dataset’s task and domain\n* Measure:\n * The demographic distribution in your dataset\n * Pre-identified negative stereotypes represented\n* Document:\n * Share what you’ve Identified and Measured in your Dataset Card so it can benefit other users, developers, and otherwise affected people\n* Adapt:\n * By choosing the dataset least likely to cause bias-related harms\n * By iteratively improving your dataset in ways that reduce bias risks",
"### I am <span style=\"text-decoration:underline;\">training/selecting a model</span> for my ML system, how can I address bias?\n\nSimilar to the dataset curation/selection step, documenting and measuring bias-related phenomena in models can help both ML developers who are selecting a model to use as-is or to finetune and ML developers who want to train their own models. For the latter, measures of bias-related phenomena in the model can help them learn from what has worked or what hasn’t for other models and serve as a signal to guide their own development choices.\n\nModel cards were originally proposed by (Mitchell et al., 2019) and provide a framework for model reporting that showcases information relevant to bias risks, including broad ethical considerations, disaggregated evaluation, and use case recommendation. The Hugging Face Hub provides even more tools for model documentation, with a model card guidebook in the Hub documentation, and an app that lets you create extensive model cards easily for your new model.\n\n<p align=\"center\">\n <br>\n <img src=\"URL alt=\"Model Card writing tool by Ezi, Marissa, and Meg\" />\n <em><a href=\"URL Card writing tool</a> by <a href=\"URL <a href=\"URL and <a href=\"URL\n</p>\n\nDocumentation is a great first step for sharing general insights about a model’s behavior, but it is usually static and presents the same information to all users. In many cases, especially for generative models that can generate outputs to approximate the distribution of their training data, we can gain a more contextual understanding of bias-related phenomena and negative stereotypes by visualizing and contrasting model outputs. Access to model generations can help users bring intersectional issues in the model behavior corresponding to their lived experience, and evaluate to what extent a model reproduces gendered stereotypes for different adjectives. To facilitate this process, we built a tool that lets you compare generations not just across a set of adjectives and professions, but also across different models! Go try it out to get a sense of which model might carry the least bias risks in your use case.\n\n<p align=\"center\">\n <br>\n <img src=\"URL alt=\"Visualize Adjective and Occupation Biases in Image Generation by Sasha\" />\n <br>\n <em><a href=\"URL Adjective and Occupation Biases in Image Generation</a> by <a href=\"URL\n</p>\n\nVisualization of model outputs isn’t just for generative models though! For classification models, we also want to look out for bias-related harms caused by a model’s disparate performance on different demographics. If you know what protected classes are most at risk of discrimination and have those annotated in an evaluation set, then you can report disaggregated performance over the different categories in your model card as mentioned above, so users can make informed decisions. If however, you are worried that you haven’t identified all populations at risk of bias-related harms, or if you do not have access to annotated test examples to measure the biases you suspect, that’s where interactive visualizations of where and how the model fails come in handy! To help you with this, the SEAL app groups similar mistakes by your model and shows you some common features in each cluster. If you want to go further, you can even combine it with the disaggregators library we introduced in the datasets section to find clusters that are indicative of bias-related failure modes!\n \n\n<p align=\"center\">\n <br>\n <img src=\"URL alt=\"Systematic Error Analysis and Labeling (SEAL) by Nazneen\" />\n <em><a href=\"URL Error Analysis and Labeling (SEAL)</a> by <a href=\"URL\n</p>\n\nFinally, a few benchmarks exist that can measure bias-related phenomena in models. For language models, benchmarks such as BOLD, HONEST, or WinoBias provide quantitative evaluations of targeted behaviors that are indicative of biases in the models. While the benchmarks have their limitations, they do provide a limited view into some pre-identified bias risks that can help describe how the models function or choose between different models. You can find these evaluations pre-computed on a range of common language models in this exploration Space to get a first sense of how they compare!\n\n\n<p align=\"center\">\n <br>\n <img src=\"URL alt=\"Language Model Bias Detection by Sasha\" />\n <em><a href=\"URL Model Bias Detection</a> by <a href=\"URL\n</p>\n\nEven with access to a benchmark for the models you are considering, you might find that running evaluations of the larger language models you are considering can be prohibitively expensive or otherwise technically impossible with your own computing resources. The <a href=\"URL on the Hub</a> tool we released this year can help with that: not only will it run the evaluations for you, but it will also help connect them to the model documentation so the results are available once and for all — so everyone can see, for example, that size <a href=\"URL increases bias risks in models like OPT</a>!\n\n<p align=\"center\">\n <br>\n <img src=\"URL alt=\"Large model WinoBias scores computed with Evaluation on the Hub by Helen, Tristan, Abhishek, Lewis, and Douwe\" />\n <em><a href=\"URL href=\"URL model WinoBias scores computed with Evaluation on the Hub</a> by <a href=\"URL <a href=\"URL <a href=\"URL <a href=\"URL and <a href=\"URL\n</p>",
"#### Model selection/development: recommendations\n\nFor models just as for datasets, different tools for documentation and evaluation will provide different views of bias risks in a model which all have a part to play in helping developers choose, develop, or understand ML systems.\n\n* Visualize\n * Generative model: visualize how the model’s outputs may reflect stereotypes\n * Classification model: visualize model errors to identify failure modes that could lead to disparate performance\n* Evaluate\n * When possible, evaluate models on relevant benchmarks\n* Document\n * Share your learnings from visualization and qualitative evaluation\n * Report your model’s disaggregated performance and results on applicable fairness benchmarks",
"## Conclusion and Overview of Bias Analysis and Documentation Tools from \n\nAs we learn to leverage ML systems in more and more applications, reaping their benefits equitably will depend on our ability to actively mitigate the risks of bias-related harms associated with the technology. While there is no single answer to the question of how this should best be done in any possible setting, we can support each other in this effort by sharing lessons, tools, and methodologies to mitigate and document those risks. The present blog post outlines some of the ways Hugging Face team members have addressed this question of bias along with supporting tools, we hope that you will find them helpful and encourage you to develop and share your own!\n\nSummary of linked tools:\n* Tasks:\n * Explore our directory of ML Tasks to understand what technical framings and resources are available to choose from\n * Use tools to explore the full development lifecycle of specific tasks\n* Datasets:\n * Make use of and contribute to Dataset Cards to share relevant insights on biases in datasets.\n * Use Disaggregator to look for possible disparate performance\n * Look at aggregated measurements of your dataset including nPMI to surface possible stereotypical associations\n* Models:\n * Make use of and contribute to Model Cards to share relevant insights on biases in models.\n * Use Interactive Model Cards to visualize performance discrepancies\n * Look at systematic model errors and look out for known social biases\n * Use Evaluate and Evaluation on the Hub to explore language model biases including in large models\n * Use a Text-to-image bias explorer to compare image generation models’ biases\n * Compare LM models with Bias Score Card\n\nThanks for reading! \n\n~ Yacine, on behalf of the Ethics and Society regulars\n\nCite as:"
] |
4e17be0aeca4ff010c61c0cbaa53beaf67b225fa | # AutoTrain Dataset for project: told_br_binary_sm_bertimbau
## Dataset Description
This dataset has been automatically processed by AutoTrain for project told_br_binary_sm_bertimbau.
### Languages
The BCP-47 code for the dataset's language is unk.
## Dataset Structure
### Data Instances
A sample from this dataset looks as follows:
```json
[
{
"text": "@user agora n\u00e3o me d\u00e1 mais, mas antes, porra",
"target": 1
},
{
"text": "pires \u00e9 fodido fds mais um",
"target": 1
}
]
```
### Dataset Fields
The dataset has the following fields (also called "features"):
```json
{
"text": "Value(dtype='string', id=None)",
"target": "ClassLabel(names=['0', '1'], id=None)"
}
```
### Dataset Splits
This dataset is split into a train and validation split. The split sizes are as follow:
| Split name | Num samples |
| ------------ | ------------------- |
| train | 5599 |
| valid | 1401 |
| alexandreteles/autotrain-data-told_br_binary_sm_bertimbau | [
"task_categories:text-classification",
"region:us"
] | 2022-12-15T22:29:35+00:00 | {"task_categories": ["text-classification"]} | 2022-12-15T22:30:17+00:00 | [] | [] | TAGS
#task_categories-text-classification #region-us
| AutoTrain Dataset for project: told\_br\_binary\_sm\_bertimbau
==============================================================
Dataset Description
-------------------
This dataset has been automatically processed by AutoTrain for project told\_br\_binary\_sm\_bertimbau.
### Languages
The BCP-47 code for the dataset's language is unk.
Dataset Structure
-----------------
### Data Instances
A sample from this dataset looks as follows:
### Dataset Fields
The dataset has the following fields (also called "features"):
### Dataset Splits
This dataset is split into a train and validation split. The split sizes are as follow:
| [
"### Languages\n\n\nThe BCP-47 code for the dataset's language is unk.\n\n\nDataset Structure\n-----------------",
"### Data Instances\n\n\nA sample from this dataset looks as follows:",
"### Dataset Fields\n\n\nThe dataset has the following fields (also called \"features\"):",
"### Dataset Splits\n\n\nThis dataset is split into a train and validation split. The split sizes are as follow:"
] | [
"TAGS\n#task_categories-text-classification #region-us \n",
"### Languages\n\n\nThe BCP-47 code for the dataset's language is unk.\n\n\nDataset Structure\n-----------------",
"### Data Instances\n\n\nA sample from this dataset looks as follows:",
"### Dataset Fields\n\n\nThe dataset has the following fields (also called \"features\"):",
"### Dataset Splits\n\n\nThis dataset is split into a train and validation split. The split sizes are as follow:"
] |
07c68c26cba253dd00e08d69ef9f9cc1d4f262bf | # Dataset Card for "clinic-utility"
[More Information needed](https://github.com/huggingface/datasets/blob/main/CONTRIBUTING.md#how-to-contribute-to-the-dataset-cards)
```
@inproceedings{larson-etal-2019-evaluation,
title = "An Evaluation Dataset for Intent Classification and Out-of-Scope Prediction",
author = "Larson, Stefan and
Mahendran, Anish and
Peper, Joseph J. and
Clarke, Christopher and
Lee, Andrew and
Hill, Parker and
Kummerfeld, Jonathan K. and
Leach, Kevin and
Laurenzano, Michael A. and
Tang, Lingjia and
Mars, Jason",
booktitle = "Proceedings of the 2019 Conference on Empirical Methods in Natural Language Processing and the 9th International Joint Conference on Natural Language Processing (EMNLP-IJCNLP)",
year = "2019",
url = "https://www.aclweb.org/anthology/D19-1131"
}
``` | fathyshalab/clinic-utility | [
"region:us"
] | 2022-12-15T23:22:54+00:00 | {"dataset_info": {"features": [{"name": "Unnamed: 0", "dtype": "int64"}, {"name": "text", "dtype": "string"}, {"name": "label", "dtype": "int64"}, {"name": "label_text", "dtype": "string"}], "splits": [{"name": "train", "num_bytes": 33764.5, "num_examples": 525}, {"name": "test", "num_bytes": 14470.5, "num_examples": 225}], "download_size": 0, "dataset_size": 48235.0}} | 2023-05-15T07:51:36+00:00 | [] | [] | TAGS
#region-us
| # Dataset Card for "clinic-utility"
More Information needed
| [
"# Dataset Card for \"clinic-utility\"\n\nMore Information needed"
] | [
"TAGS\n#region-us \n",
"# Dataset Card for \"clinic-utility\"\n\nMore Information needed"
] |
b26be3e5d8bbcafbf6f1f5a28ed17e66d194fa67 | # Dataset Card for "butterflies_10k_names_multiple"
[More Information needed](https://github.com/huggingface/datasets/blob/main/CONTRIBUTING.md#how-to-contribute-to-the-dataset-cards) | sasha/butterflies_10k_names_multiple | [
"region:us"
] | 2022-12-15T23:37:13+00:00 | {"dataset_info": {"features": [{"name": "image", "dtype": "image"}, {"name": "description", "dtype": "string"}, {"name": "url", "dtype": "string"}, {"name": "sim_score", "dtype": "float64"}, {"name": "name", "dtype": "string"}], "splits": [{"name": "train", "num_bytes": 260929983.907, "num_examples": 7061}], "download_size": 268647797, "dataset_size": 260929983.907}} | 2022-12-15T23:37:48+00:00 | [] | [] | TAGS
#region-us
| # Dataset Card for "butterflies_10k_names_multiple"
More Information needed | [
"# Dataset Card for \"butterflies_10k_names_multiple\"\n\nMore Information needed"
] | [
"TAGS\n#region-us \n",
"# Dataset Card for \"butterflies_10k_names_multiple\"\n\nMore Information needed"
] |
9255cf3d7c8184c91fdfcbacda3bd0f0ea4af2b6 | # Dataset Card for "mnli_AppE"
[More Information needed](https://github.com/huggingface/datasets/blob/main/CONTRIBUTING.md#how-to-contribute-to-the-dataset-cards) | liuyanchen1015/mnli_AppE | [
"region:us"
] | 2022-12-16T01:33:18+00:00 | {"dataset_info": {"features": [{"name": "premise", "dtype": "string"}, {"name": "hypothesis", "dtype": "string"}, {"name": "label", "dtype": "int64"}, {"name": "idx", "dtype": "int64"}], "splits": [{"name": "train", "num_bytes": 77770885, "num_examples": 383973}, {"name": "dev_matched", "num_bytes": 1943955, "num_examples": 9770}, {"name": "dev_mismatched", "num_bytes": 2070244, "num_examples": 9824}, {"name": "test_matched", "num_bytes": 1943860, "num_examples": 9673}, {"name": "test_mismatched", "num_bytes": 2071907, "num_examples": 9842}], "download_size": 0, "dataset_size": 85800851}} | 2022-12-16T01:37:06+00:00 | [] | [] | TAGS
#region-us
| # Dataset Card for "mnli_AppE"
More Information needed | [
"# Dataset Card for \"mnli_AppE\"\n\nMore Information needed"
] | [
"TAGS\n#region-us \n",
"# Dataset Card for \"mnli_AppE\"\n\nMore Information needed"
] |
513e54e089197671f12d4acc87f3c9b6be0aebd6 | annotations_creators:
- expert-generated
language_creators:
- other
-
license:
- mit
pretty_name: mnist extended
size_categories:
- 10K<n<100K
task_categories:
- image-classification
| seftontycho/mnist-extended-1 | [
"region:us"
] | 2022-12-16T01:33:35+00:00 | {} | 2022-12-16T01:40:20+00:00 | [] | [] | TAGS
#region-us
| annotations_creators:
- expert-generated
language_creators:
- other
-
license:
- mit
pretty_name: mnist extended
size_categories:
- 10K<n<100K
task_categories:
- image-classification
| [] | [
"TAGS\n#region-us \n"
] |
593c095f859fcc32d9f09c78a676fa6344fabf7c | # Dataset Card for "mnli_ChcE"
[More Information needed](https://github.com/huggingface/datasets/blob/main/CONTRIBUTING.md#how-to-contribute-to-the-dataset-cards) | liuyanchen1015/mnli_ChcE | [
"region:us"
] | 2022-12-16T01:37:48+00:00 | {"dataset_info": {"features": [{"name": "premise", "dtype": "string"}, {"name": "hypothesis", "dtype": "string"}, {"name": "label", "dtype": "int64"}, {"name": "idx", "dtype": "int64"}], "splits": [{"name": "train", "num_bytes": 75380580, "num_examples": 383734}, {"name": "dev_matched", "num_bytes": 1887265, "num_examples": 9770}, {"name": "dev_mismatched", "num_bytes": 2007388, "num_examples": 9824}, {"name": "test_matched", "num_bytes": 1884526, "num_examples": 9673}, {"name": "test_mismatched", "num_bytes": 2008710, "num_examples": 9842}], "download_size": 56286590, "dataset_size": 83168469}} | 2022-12-16T01:38:13+00:00 | [] | [] | TAGS
#region-us
| # Dataset Card for "mnli_ChcE"
More Information needed | [
"# Dataset Card for \"mnli_ChcE\"\n\nMore Information needed"
] | [
"TAGS\n#region-us \n",
"# Dataset Card for \"mnli_ChcE\"\n\nMore Information needed"
] |
253e0ec30f757fb87e8186d742028ff5e46d9dc0 | # Dataset Card for "mnli_CollSgE"
[More Information needed](https://github.com/huggingface/datasets/blob/main/CONTRIBUTING.md#how-to-contribute-to-the-dataset-cards) | liuyanchen1015/mnli_CollSgE | [
"region:us"
] | 2022-12-16T01:39:15+00:00 | {"dataset_info": {"features": [{"name": "premise", "dtype": "string"}, {"name": "hypothesis", "dtype": "string"}, {"name": "label", "dtype": "int64"}, {"name": "idx", "dtype": "int64"}], "splits": [{"name": "train", "num_bytes": 70113002, "num_examples": 383726}, {"name": "dev_matched", "num_bytes": 1755041, "num_examples": 9770}, {"name": "dev_mismatched", "num_bytes": 1850578, "num_examples": 9821}, {"name": "test_matched", "num_bytes": 1749414, "num_examples": 9671}, {"name": "test_mismatched", "num_bytes": 1855882, "num_examples": 9840}], "download_size": 52803650, "dataset_size": 77323917}} | 2022-12-16T01:39:31+00:00 | [] | [] | TAGS
#region-us
| # Dataset Card for "mnli_CollSgE"
More Information needed | [
"# Dataset Card for \"mnli_CollSgE\"\n\nMore Information needed"
] | [
"TAGS\n#region-us \n",
"# Dataset Card for \"mnli_CollSgE\"\n\nMore Information needed"
] |
1608d4a261af219df1c1f406695cc2a46af2649b | # Dataset Card for "mnli_IndE"
[More Information needed](https://github.com/huggingface/datasets/blob/main/CONTRIBUTING.md#how-to-contribute-to-the-dataset-cards) | liuyanchen1015/mnli_IndE | [
"region:us"
] | 2022-12-16T01:40:49+00:00 | {"dataset_info": {"features": [{"name": "premise", "dtype": "string"}, {"name": "hypothesis", "dtype": "string"}, {"name": "label", "dtype": "int64"}, {"name": "idx", "dtype": "int64"}], "splits": [{"name": "train", "num_bytes": 75917760, "num_examples": 383924}, {"name": "dev_matched", "num_bytes": 1900229, "num_examples": 9770}, {"name": "dev_mismatched", "num_bytes": 2016344, "num_examples": 9824}, {"name": "test_matched", "num_bytes": 1896266, "num_examples": 9672}, {"name": "test_mismatched", "num_bytes": 2021206, "num_examples": 9841}], "download_size": 56783020, "dataset_size": 83751805}} | 2022-12-16T01:41:06+00:00 | [] | [] | TAGS
#region-us
| # Dataset Card for "mnli_IndE"
More Information needed | [
"# Dataset Card for \"mnli_IndE\"\n\nMore Information needed"
] | [
"TAGS\n#region-us \n",
"# Dataset Card for \"mnli_IndE\"\n\nMore Information needed"
] |
264c7c9f6a91bf5a72606a9df2c37f3efed5b1b6 | # Dataset Card for "mnli_MULTI"
[More Information needed](https://github.com/huggingface/datasets/blob/main/CONTRIBUTING.md#how-to-contribute-to-the-dataset-cards) | liuyanchen1015/mnli_MULTI | [
"region:us"
] | 2022-12-16T01:43:06+00:00 | {"dataset_info": {"features": [{"name": "premise", "dtype": "string"}, {"name": "hypothesis", "dtype": "string"}, {"name": "label", "dtype": "int64"}, {"name": "idx", "dtype": "int64"}], "splits": [{"name": "train", "num_bytes": 79281363, "num_examples": 384388}, {"name": "dev_matched", "num_bytes": 1983976, "num_examples": 9779}, {"name": "dev_mismatched", "num_bytes": 2092314, "num_examples": 9823}, {"name": "test_matched", "num_bytes": 1976499, "num_examples": 9672}, {"name": "test_mismatched", "num_bytes": 2096238, "num_examples": 9841}], "download_size": 58746057, "dataset_size": 87430390}} | 2022-12-16T01:43:32+00:00 | [] | [] | TAGS
#region-us
| # Dataset Card for "mnli_MULTI"
More Information needed | [
"# Dataset Card for \"mnli_MULTI\"\n\nMore Information needed"
] | [
"TAGS\n#region-us \n",
"# Dataset Card for \"mnli_MULTI\"\n\nMore Information needed"
] |
b20791392860e0919ab7c0f94b6f590da8c5f350 |
This dataset is extracted from the Visual Novel "Milk inside a bag of milk inside a bag of milk."
Please refer to the `milk_dialog_dataset.ipynb` file to see how the dataset was pre-processed. | alexandreteles/milk | [
"multilinguality:monolingual",
"language:en",
"license:other",
"region:us"
] | 2022-12-16T03:10:11+00:00 | {"language": ["en"], "license": "other", "multilinguality": ["monolingual"], "pretty_name": "milk", "language_bcp47": ["en-US"]} | 2022-12-27T17:49:14+00:00 | [] | [
"en"
] | TAGS
#multilinguality-monolingual #language-English #license-other #region-us
|
This dataset is extracted from the Visual Novel "Milk inside a bag of milk inside a bag of milk."
Please refer to the 'milk_dialog_dataset.ipynb' file to see how the dataset was pre-processed. | [] | [
"TAGS\n#multilinguality-monolingual #language-English #license-other #region-us \n"
] |
43e39604a2a1ff24fe3857ef13c112368a6e45ac | # Dataset Card for "common_voice_11_0_th_w2v2"
[More Information needed](https://github.com/huggingface/datasets/blob/main/CONTRIBUTING.md#how-to-contribute-to-the-dataset-cards) | Botnoi/common_voice_11_0_th_w2v2 | [
"region:us"
] | 2022-12-16T04:21:21+00:00 | {"dataset_info": {"features": [{"name": "input_values", "sequence": "float32"}, {"name": "input_length", "dtype": "int64"}, {"name": "labels", "sequence": "int64"}], "splits": [{"name": "train", "num_bytes": 12075286848.556108, "num_examples": 42779}, {"name": "validation", "num_bytes": 1632681586.0, "num_examples": 5465}, {"name": "test", "num_bytes": 1714070928.0, "num_examples": 5465}], "download_size": 14452183131, "dataset_size": 15422039362.556108}} | 2023-02-01T12:25:03+00:00 | [] | [] | TAGS
#region-us
| # Dataset Card for "common_voice_11_0_th_w2v2"
More Information needed | [
"# Dataset Card for \"common_voice_11_0_th_w2v2\"\n\nMore Information needed"
] | [
"TAGS\n#region-us \n",
"# Dataset Card for \"common_voice_11_0_th_w2v2\"\n\nMore Information needed"
] |
4c3562fbc67afe83a70873fc782a5c1411d1b7fd | # Dataset Card for AutoTrain Evaluator
This repository contains model predictions generated by [AutoTrain](https://huggingface.co/autotrain) for the following task and dataset:
* Task: Question Answering
* Model: Jiqing/bert-large-uncased-whole-word-masking-finetuned-squad-finetuned-squad
* Dataset: squad
* Config: plain_text
* Split: validation
To run new evaluation jobs, visit Hugging Face's [automatic model evaluator](https://huggingface.co/spaces/autoevaluate/model-evaluator).
## Contributions
Thanks to [@florence](https://huggingface.co/florence) for evaluating this model. | autoevaluate/autoeval-eval-squad-plain_text-58f506-2493576894 | [
"autotrain",
"evaluation",
"region:us"
] | 2022-12-16T07:23:54+00:00 | {"type": "predictions", "tags": ["autotrain", "evaluation"], "datasets": ["squad"], "eval_info": {"task": "extractive_question_answering", "model": "Jiqing/bert-large-uncased-whole-word-masking-finetuned-squad-finetuned-squad", "metrics": [], "dataset_name": "squad", "dataset_config": "plain_text", "dataset_split": "validation", "col_mapping": {"context": "context", "question": "question", "answers-text": "answers.text", "answers-answer_start": "answers.answer_start"}}} | 2022-12-16T07:28:12+00:00 | [] | [] | TAGS
#autotrain #evaluation #region-us
| # Dataset Card for AutoTrain Evaluator
This repository contains model predictions generated by AutoTrain for the following task and dataset:
* Task: Question Answering
* Model: Jiqing/bert-large-uncased-whole-word-masking-finetuned-squad-finetuned-squad
* Dataset: squad
* Config: plain_text
* Split: validation
To run new evaluation jobs, visit Hugging Face's automatic model evaluator.
## Contributions
Thanks to @florence for evaluating this model. | [
"# Dataset Card for AutoTrain Evaluator\n\nThis repository contains model predictions generated by AutoTrain for the following task and dataset:\n\n* Task: Question Answering\n* Model: Jiqing/bert-large-uncased-whole-word-masking-finetuned-squad-finetuned-squad\n* Dataset: squad\n* Config: plain_text\n* Split: validation\n\nTo run new evaluation jobs, visit Hugging Face's automatic model evaluator.",
"## Contributions\n\nThanks to @florence for evaluating this model."
] | [
"TAGS\n#autotrain #evaluation #region-us \n",
"# Dataset Card for AutoTrain Evaluator\n\nThis repository contains model predictions generated by AutoTrain for the following task and dataset:\n\n* Task: Question Answering\n* Model: Jiqing/bert-large-uncased-whole-word-masking-finetuned-squad-finetuned-squad\n* Dataset: squad\n* Config: plain_text\n* Split: validation\n\nTo run new evaluation jobs, visit Hugging Face's automatic model evaluator.",
"## Contributions\n\nThanks to @florence for evaluating this model."
] |
18fe77b882f32df0575bfb911dda5a3a212d492a | # Dataset Card for "OSD-Dataset"
[More Information needed](https://github.com/huggingface/datasets/blob/main/CONTRIBUTING.md#how-to-contribute-to-the-dataset-cards)
This is a reformat of Huggingface Project's [SD Multiplayer Dataset](https://huggingface.co/datasets/huggingface-projects/sd-multiplayer-data)
It converts the image bucket into a parquet format. The text column is the prompt + the timestamp for it to the minutes precision.
The model finetuned on it is [here](https://huggingface.co/BirdL/OSD-Model) | BirdL/OSD-Dataset | [
"region:us"
] | 2022-12-16T07:30:34+00:00 | {"dataset_info": {"features": [{"name": "image", "dtype": "image"}, {"name": "text", "dtype": "string"}], "splits": [{"name": "train", "num_bytes": 7440671071.55, "num_examples": 198771}], "download_size": 7196594621, "dataset_size": 7440671071.55}} | 2022-12-19T19:43:20+00:00 | [] | [] | TAGS
#region-us
| # Dataset Card for "OSD-Dataset"
More Information needed
This is a reformat of Huggingface Project's SD Multiplayer Dataset
It converts the image bucket into a parquet format. The text column is the prompt + the timestamp for it to the minutes precision.
The model finetuned on it is here | [
"# Dataset Card for \"OSD-Dataset\"\n\nMore Information needed\n\nThis is a reformat of Huggingface Project's SD Multiplayer Dataset \nIt converts the image bucket into a parquet format. The text column is the prompt + the timestamp for it to the minutes precision.\nThe model finetuned on it is here"
] | [
"TAGS\n#region-us \n",
"# Dataset Card for \"OSD-Dataset\"\n\nMore Information needed\n\nThis is a reformat of Huggingface Project's SD Multiplayer Dataset \nIt converts the image bucket into a parquet format. The text column is the prompt + the timestamp for it to the minutes precision.\nThe model finetuned on it is here"
] |
0f465b676513506f29f15c39ec19ccdcb0687562 | # Dataset Card for AutoTrain Evaluator
This repository contains model predictions generated by [AutoTrain](https://huggingface.co/autotrain) for the following task and dataset:
* Task: Question Answering
* Model: Jiqing/bert-large-uncased-whole-word-masking-finetuned-squad-finetuned-squad
* Dataset: squad_v2
* Config: squad_v2
* Split: validation
To run new evaluation jobs, visit Hugging Face's [automatic model evaluator](https://huggingface.co/spaces/autoevaluate/model-evaluator).
## Contributions
Thanks to [@Florence Gundidza](https://huggingface.co/Florence Gundidza) for evaluating this model. | autoevaluate/autoeval-eval-squad_v2-squad_v2-878283-2493776900 | [
"autotrain",
"evaluation",
"region:us"
] | 2022-12-16T07:35:38+00:00 | {"type": "predictions", "tags": ["autotrain", "evaluation"], "datasets": ["squad_v2"], "eval_info": {"task": "extractive_question_answering", "model": "Jiqing/bert-large-uncased-whole-word-masking-finetuned-squad-finetuned-squad", "metrics": ["precision", "recall"], "dataset_name": "squad_v2", "dataset_config": "squad_v2", "dataset_split": "validation", "col_mapping": {"context": "context", "question": "question", "answers-text": "answers.text", "answers-answer_start": "answers.answer_start"}}} | 2022-12-16T07:40:00+00:00 | [] | [] | TAGS
#autotrain #evaluation #region-us
| # Dataset Card for AutoTrain Evaluator
This repository contains model predictions generated by AutoTrain for the following task and dataset:
* Task: Question Answering
* Model: Jiqing/bert-large-uncased-whole-word-masking-finetuned-squad-finetuned-squad
* Dataset: squad_v2
* Config: squad_v2
* Split: validation
To run new evaluation jobs, visit Hugging Face's automatic model evaluator.
## Contributions
Thanks to @Florence Gundidza for evaluating this model. | [
"# Dataset Card for AutoTrain Evaluator\n\nThis repository contains model predictions generated by AutoTrain for the following task and dataset:\n\n* Task: Question Answering\n* Model: Jiqing/bert-large-uncased-whole-word-masking-finetuned-squad-finetuned-squad\n* Dataset: squad_v2\n* Config: squad_v2\n* Split: validation\n\nTo run new evaluation jobs, visit Hugging Face's automatic model evaluator.",
"## Contributions\n\nThanks to @Florence Gundidza for evaluating this model."
] | [
"TAGS\n#autotrain #evaluation #region-us \n",
"# Dataset Card for AutoTrain Evaluator\n\nThis repository contains model predictions generated by AutoTrain for the following task and dataset:\n\n* Task: Question Answering\n* Model: Jiqing/bert-large-uncased-whole-word-masking-finetuned-squad-finetuned-squad\n* Dataset: squad_v2\n* Config: squad_v2\n* Split: validation\n\nTo run new evaluation jobs, visit Hugging Face's automatic model evaluator.",
"## Contributions\n\nThanks to @Florence Gundidza for evaluating this model."
] |
62dfdc0c261fd6029582f165b8d772f05712253b | # Dataset Card for "yannick-kilcher-transcript-wav"
[More Information needed](https://github.com/huggingface/datasets/blob/main/CONTRIBUTING.md#how-to-contribute-to-the-dataset-cards) | matallanas/yannick-kilcher-transcript-wav | [
"region:us"
] | 2022-12-16T07:49:11+00:00 | {"dataset_info": {"features": [{"name": "id", "dtype": "string"}, {"name": "channel", "dtype": "string"}, {"name": "channel_id", "dtype": "string"}, {"name": "title", "dtype": "string"}, {"name": "categories", "sequence": "string"}, {"name": "tags", "sequence": "string"}, {"name": "description", "dtype": "string"}, {"name": "text", "dtype": "string"}, {"name": "segments", "list": [{"name": "start", "dtype": "float64"}, {"name": "end", "dtype": "float64"}, {"name": "text", "dtype": "string"}]}, {"name": "audio", "dtype": "audio"}], "splits": [{"name": "train", "num_bytes": 144437989292.0, "num_examples": 370}], "download_size": 127955407676, "dataset_size": 144437989292.0}} | 2022-12-16T10:11:10+00:00 | [] | [] | TAGS
#region-us
| # Dataset Card for "yannick-kilcher-transcript-wav"
More Information needed | [
"# Dataset Card for \"yannick-kilcher-transcript-wav\"\n\nMore Information needed"
] | [
"TAGS\n#region-us \n",
"# Dataset Card for \"yannick-kilcher-transcript-wav\"\n\nMore Information needed"
] |
9253555e8c5ec0890c85f4cddfe8053aaef49247 | # Dataset Card for "kaggle-mbti-cleaned-augmented"
This dataset is built upon [Shunian/kaggle-mbti-cleaned](https://huggingface.co/datasets/Shunian/kaggle-mbti-cleaned) to address the sample imbalance problem.
Thanks to the [Parrot Paraphraser](https://github.com/PrithivirajDamodaran/Parrot_Paraphraser) and [NLP AUG](https://github.com/makcedward/nlpaug), some of the skewness issue are addressed in the training data, make it grows from 328,660 samples to 478,389 samples in total.
View [GitHub](https://github.com/nogibjj/MBTI-Personality-Test) for more information | Shunian/kaggle-mbti-cleaned-augmented | [
"region:us"
] | 2022-12-16T09:30:11+00:00 | {"dataset_info": {"features": [{"name": "label", "dtype": "int64"}, {"name": "text", "dtype": "string"}], "splits": [{"name": "train", "num_bytes": 74489242, "num_examples": 478389}, {"name": "test", "num_bytes": 12922409, "num_examples": 81957}], "download_size": 56815784, "dataset_size": 87411651}} | 2022-12-16T09:46:26+00:00 | [] | [] | TAGS
#region-us
| # Dataset Card for "kaggle-mbti-cleaned-augmented"
This dataset is built upon Shunian/kaggle-mbti-cleaned to address the sample imbalance problem.
Thanks to the Parrot Paraphraser and NLP AUG, some of the skewness issue are addressed in the training data, make it grows from 328,660 samples to 478,389 samples in total.
View GitHub for more information | [
"# Dataset Card for \"kaggle-mbti-cleaned-augmented\"\nThis dataset is built upon Shunian/kaggle-mbti-cleaned to address the sample imbalance problem.\n\nThanks to the Parrot Paraphraser and NLP AUG, some of the skewness issue are addressed in the training data, make it grows from 328,660 samples to 478,389 samples in total.\n\nView GitHub for more information"
] | [
"TAGS\n#region-us \n",
"# Dataset Card for \"kaggle-mbti-cleaned-augmented\"\nThis dataset is built upon Shunian/kaggle-mbti-cleaned to address the sample imbalance problem.\n\nThanks to the Parrot Paraphraser and NLP AUG, some of the skewness issue are addressed in the training data, make it grows from 328,660 samples to 478,389 samples in total.\n\nView GitHub for more information"
] |
926a3f36d6d4066dea2cca2736494af477dc1864 | # Dataset Card for "swiss_parliament_corpus"
[More Information needed](https://github.com/huggingface/datasets/blob/main/CONTRIBUTING.md#how-to-contribute-to-the-dataset-cards) | yanickschraner/swiss_parliament_corpus | [
"region:us"
] | 2022-12-16T10:13:00+00:00 | {"dataset_info": {"features": [{"name": "client_id", "dtype": "int64"}, {"name": "audio", "dtype": {"audio": {"sampling_rate": 16000}}}, {"name": "sentence", "dtype": "string"}, {"name": "up_votes", "dtype": "float64"}, {"name": "down_votes", "dtype": "float64"}, {"name": "age", "dtype": "float64"}, {"name": "gender", "dtype": "float64"}, {"name": "accent", "dtype": "float64"}, {"name": "iou_estimate", "dtype": "float64"}], "splits": [{"name": "train", "num_bytes": 24373100536.732, "num_examples": 90324}, {"name": "test", "num_bytes": 824083440.94, "num_examples": 3332}], "download_size": 14083003405, "dataset_size": 25197183977.671997}} | 2022-12-16T13:44:59+00:00 | [] | [] | TAGS
#region-us
| # Dataset Card for "swiss_parliament_corpus"
More Information needed | [
"# Dataset Card for \"swiss_parliament_corpus\"\n\nMore Information needed"
] | [
"TAGS\n#region-us \n",
"# Dataset Card for \"swiss_parliament_corpus\"\n\nMore Information needed"
] |
d667b8e3420edef64bbf14e8c26030d156e2e012 | Hi, this is my first dataset. If you know how to make it better, please leave a comment | sbad/biographySummaries | [
"license:cc",
"region:us"
] | 2022-12-16T12:47:25+00:00 | {"license": "cc"} | 2022-12-17T10:15:55+00:00 | [] | [] | TAGS
#license-cc #region-us
| Hi, this is my first dataset. If you know how to make it better, please leave a comment | [] | [
"TAGS\n#license-cc #region-us \n"
] |
b804913a330ab772fa9340c9e53ab50d688a4d5e | # Dataset Card for "test_torch"
[More Information needed](https://github.com/huggingface/datasets/blob/main/CONTRIBUTING.md#how-to-contribute-to-the-dataset-cards) | polinaeterna/test_torch | [
"region:us"
] | 2022-12-16T14:26:59+00:00 | {"dataset_info": {"features": [{"name": "data", "dtype": "float64"}, {"name": "text", "dtype": "string"}, {"name": "label", "dtype": "int64"}], "splits": [{"name": "train", "num_bytes": 368, "num_examples": 16}], "download_size": 1376, "dataset_size": 368}} | 2022-12-16T14:27:09+00:00 | [] | [] | TAGS
#region-us
| # Dataset Card for "test_torch"
More Information needed | [
"# Dataset Card for \"test_torch\"\n\nMore Information needed"
] | [
"TAGS\n#region-us \n",
"# Dataset Card for \"test_torch\"\n\nMore Information needed"
] |
f8024be1cf47d74a090b46b9b7c57caff075589f |
# Dataset Card for "Wikiomnia"
## Table of Contents
- [Dataset Description](#dataset-description)
- [Dataset Summary](#dataset-summary)
- [Dataset Structure](#dataset-structure)
- [Dataset Creation](#dataset-creation)
- [Additional Information](#additional-information)
- [Licensing Information](#licensing-information)
- [Citation Information](#citation-information)
- [Contributions](#contributions)
## Dataset Description
- **Repository:** [https://github.com/RussianNLP](https://github.com/RussianNLP)
- **Paper:** [WikiOmnia: filtration and evaluation of the generated QA corpus on the whole Russian Wikipedia](https://arxiv.org/abs/2204.08009)
- **Point of Contact:** [More Information Needed](https://github.com/huggingface/datasets/blob/master/CONTRIBUTING.md#how-to-contribute-to-the-dataset-cards)
### Dataset Summary
We present the WikiOmnia dataset, a new publicly available set of QA-pairs and corresponding Russian Wikipedia article summary sections, composed with a fully automated generative pipeline. The dataset includes every available article from Wikipedia for the Russian language. The WikiOmnia pipeline is available open-source and is also tested for creating SQuAD-formatted QA on other domains, like news texts, fiction, and social media. The resulting dataset includes two parts: raw data on the whole Russian Wikipedia (7,930,873 QA pairs with paragraphs for ruGPT-3 XL and 7,991,040 QA pairs with paragraphs for ruT5-large) and cleaned data with strict automatic verification (over 160,000 QA pairs with paragraphs for ruGPT-3 XL and over 3,400,000 QA pairs with paragraphs for ruT5-large).
WikiOmnia consists of 2 parts:
1. the voluminous, automatically generated part: 15,9 million triplets consisting of the original article summary, a corresponding generated question and a generated answer;
2. the filtered part: the subsample of 3,5 million triplets, fully verified with automatic means
Wikiomnia adheres to a standard SQuAD format problem, resulting in triplets "text paragraph - question based on paragraph - answer from the paragraph", see the following example:
**Original Wikipedia paragraph**: Коити Масимо (яп. Масимо Ко:ити) — известный режиссёр аниме и основатель японской анимационной студии Bee Train. С
момента основания студии он руководит производством почти всех её картин, а также время от времени принимает участие в работе над анимацией и музыкой.
**English translation**: Koichi Mashimo is a famous anime director and the founder of the Japanese animation studio Bee Train. Since the creation of the studio, he directed almost all studio’s works, and he
also sometimes participates in art and sound tasks.
**Generated question (ruT5)**: Кто является основателем японской анимационной студии Bee Train?
**Generated answer (ruT5)**: Коити Масимо
**English QA translation**: Who is the founder of the Japanese animation studio Bee Train? Koichi Mashimo
## Dataset Creation
Models used for dataset generation:
- [ruT5](https://huggingface.co/sberbank-ai/ruT5-large) large fine-tuned on SberQuaD
- [ruGPT-3](https://huggingface.co/sberbank-ai/rugpt3xl) XL fine-tuned on SberQuaD
- [ruBERT](http://docs.deeppavlov.ai/en/master/features/models/squad.html) DeepPavlov tuned for QA tasks
Source: Wikipedia version March 2021
Special tokens: <[TEXT]>, <[QUESTION]>, <[ANSWER]>
The resulting dataset includes two parts: raw data on the whole Russian Wikipedia (7,930,873 QA pairs with paragraphs for ruGPT-3 XL and 7,991,040 QA pairs with paragraphs for ruT5-
large) and cleaned data with strict automatic verification (over 160,000 QA pairs with paragraphs for ruGPT-3 XL and over 3,400,000 QA pairs with paragraphs for ruT5-large).
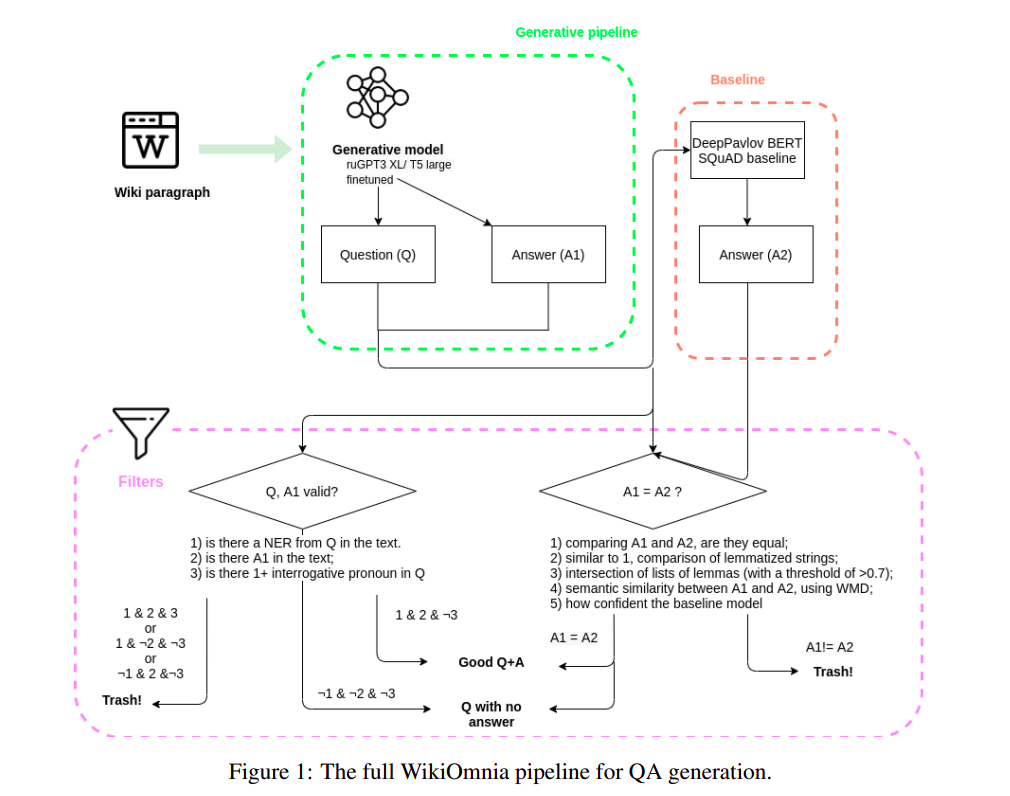
## Additional Information
### Licensing Information
[Apache 2.0 license](https://github.com/RussianNLP/WikiOmnia/blob/main/LICENSE)
### Citation Information
```
@inproceedings{pisarevskaya-shavrina-2022-wikiomnia,
title = "{W}iki{O}mnia: filtration and evaluation of the generated {QA} corpus on the whole {R}ussian {W}ikipedia",
author = "Pisarevskaya, Dina and
Shavrina, Tatiana",
booktitle = "Proceedings of the 2nd Workshop on Natural Language Generation, Evaluation, and Metrics (GEM)",
month = dec,
year = "2022",
address = "Abu Dhabi, United Arab Emirates (Hybrid)",
publisher = "Association for Computational Linguistics",
url = "https://aclanthology.org/2022.gem-1.10",
pages = "125--135",
abstract = "The General QA field has been developing the methodology referencing the Stanford Question answering dataset (SQuAD) as the significant benchmark. Compiling factual questions datasets requires manual annotations, limiting the training data{'}s potential size. We present the WikiOmnia dataset, a new publicly available set of QA pairs and corresponding Russian Wikipedia article summary sections, composed with a fully automated generation and filtration pipeline. To ensure high quality of generated QA pairs, diverse manual and automated evaluation techniques were applied. The WikiOmnia pipeline is available open-source and is also tested for creating SQuAD-formatted QA on other domains, like news texts, fiction, and social media. The resulting dataset includes two parts: raw data on the whole Russian Wikipedia (7,930,873 QA pairs with paragraphs for ruGPT-3 XL and 7,991,040 QA pairs with paragraphs for ruT5-large) and cleaned data with strict automatic verification (over 160,000 QA pairs with paragraphs for ruGPT-3 XL and over 3,400,000 QA pairs with paragraphs for ruT5-large).",
}
```
### Contributions
Thanks to [@Deenochka](https://github.com/deenochka), [@TatianaShavrina](https://github.com/TatianaShavrina) | RussianNLP/wikiomnia | [
"task_categories:question-answering",
"size_categories:1M<n<10M",
"language:ru",
"license:apache-2.0",
"wikipedia",
"wikiomnia",
"squad",
"QA",
"arxiv:2204.08009",
"region:us"
] | 2022-12-16T16:03:40+00:00 | {"language": ["ru"], "license": "apache-2.0", "size_categories": ["1M<n<10M"], "task_categories": ["question-answering"], "pretty_name": "WikiOmnia", "dataset_info": [{"config_name": "wikiomnia_ruT5_raw", "features": [{"name": "title", "dtype": "string"}, {"name": "categories", "dtype": "string"}, {"name": "summary", "dtype": "string"}, {"name": "question", "dtype": "string"}, {"name": "answer", "dtype": "string"}, {"name": "batch_id", "dtype": "string"}], "splits": [{"name": "dev", "num_bytes": 600356136, "num_examples": 266295}, {"name": "test", "num_bytes": 572651444, "num_examples": 267751}], "download_size": 1204094848, "dataset_size": 1173007580}, {"config_name": "wikiomnia_ruT5_filtered", "features": [{"name": "title", "dtype": "string"}, {"name": "categories", "dtype": "string"}, {"name": "summary", "dtype": "string"}, {"name": "question", "dtype": "string"}, {"name": "answer", "dtype": "string"}, {"name": "batch_id", "dtype": "string"}], "splits": [{"name": "train", "num_bytes": 4157093224, "num_examples": 2088027}], "download_size": 4278635364, "dataset_size": 4157093224}, {"config_name": "wikiomnia_ruGPT3_filtered", "features": [{"name": "title", "dtype": "string"}, {"name": "categories", "dtype": "string"}, {"name": "summary", "dtype": "string"}, {"name": "question", "dtype": "string"}, {"name": "answer", "dtype": "string"}, {"name": "batch_id", "dtype": "string"}], "splits": [{"name": "train", "num_bytes": 338607635, "num_examples": 173314}], "download_size": 348694031, "dataset_size": 338607635}, {"config_name": "wikiomnia_ruGPT3_raw", "features": [{"name": "title", "dtype": "string"}, {"name": "categories", "dtype": "string"}, {"name": "summary", "dtype": "string"}, {"name": "question", "dtype": "string"}, {"name": "answer", "dtype": "string"}, {"name": "batch_id", "dtype": "string"}], "splits": [{"name": "train_batch1", "num_bytes": 553204785, "num_examples": 260808}, {"name": "train_batch2", "num_bytes": 542823205, "num_examples": 263599}, {"name": "train_batch3", "num_bytes": 582321994, "num_examples": 269736}, {"name": "train_batch4", "num_bytes": 543315355, "num_examples": 265948}, {"name": "train_batch5", "num_bytes": 513288049, "num_examples": 268466}, {"name": "train_batch6", "num_bytes": 943556173, "num_examples": 512147}, {"name": "train_batch7", "num_bytes": 929464509, "num_examples": 508149}, {"name": "train_batch8", "num_bytes": 915128725, "num_examples": 507559}, {"name": "train_batch9", "num_bytes": 926443048, "num_examples": 504292}, {"name": "train_batch10", "num_bytes": 834958539, "num_examples": 463812}, {"name": "train_batch11", "num_bytes": 509866027, "num_examples": 287770}, {"name": "train_batch12", "num_bytes": 478843738, "num_examples": 271410}, {"name": "train_batch13", "num_bytes": 757068702, "num_examples": 385730}, {"name": "train_batch14", "num_bytes": 575937629, "num_examples": 304110}, {"name": "train_batch15", "num_bytes": 517092031, "num_examples": 277507}, {"name": "train_batch16", "num_bytes": 759363156, "num_examples": 402203}, {"name": "train_batch17", "num_bytes": 860544388, "num_examples": 466572}, {"name": "train_batch18", "num_bytes": 935985528, "num_examples": 518348}, {"name": "train_batch19", "num_bytes": 936782197, "num_examples": 514307}, {"name": "train_batch20", "num_bytes": 874299949, "num_examples": 487238}], "download_size": 14939875008, "dataset_size": 14490287727}, {"config_name": "wikiomnia_ruT5_raw_train", "features": [{"name": "title", "dtype": "string"}, {"name": "categories", "dtype": "string"}, {"name": "summary", "dtype": "string"}, {"name": "question", "dtype": "string"}, {"name": "answer", "dtype": "string"}, {"name": "batch_id", "dtype": "string"}], "splits": [{"name": "train_batch3", "num_bytes": 612693602, "num_examples": 271391}, {"name": "train_batch4", "num_bytes": 570286147, "num_examples": 265947}, {"name": "train_batch5", "num_bytes": 552502041, "num_examples": 274650}, {"name": "train_batch6", "num_bytes": 1017066184, "num_examples": 525224}, {"name": "train_batch7", "num_bytes": 972351430, "num_examples": 509615}, {"name": "train_batch8", "num_bytes": 973314180, "num_examples": 516828}, {"name": "train_batch9", "num_bytes": 981651841, "num_examples": 512709}, {"name": "train_batch10", "num_bytes": 880664685, "num_examples": 469512}, {"name": "train_batch11", "num_bytes": 543971388, "num_examples": 294631}, {"name": "train_batch12", "num_bytes": 503939060, "num_examples": 273526}, {"name": "train_batch13", "num_bytes": 794421530, "num_examples": 392021}, {"name": "train_batch14", "num_bytes": 610815879, "num_examples": 311452}, {"name": "train_batch15", "num_bytes": 540225492, "num_examples": 278677}, {"name": "train_batch16", "num_bytes": 804003566, "num_examples": 411192}, {"name": "train_batch17", "num_bytes": 903347135, "num_examples": 469871}, {"name": "train_batch18", "num_bytes": 995239085, "num_examples": 528301}, {"name": "train_batch19", "num_bytes": 1003402360, "num_examples": 522264}, {"name": "train_batch20", "num_bytes": 948137237, "num_examples": 499866}], "download_size": 14634332336, "dataset_size": 14208032842}], "tags": ["wikipedia", "wikiomnia", "squad", "QA"]} | 2023-04-07T05:43:59+00:00 | [
"2204.08009"
] | [
"ru"
] | TAGS
#task_categories-question-answering #size_categories-1M<n<10M #language-Russian #license-apache-2.0 #wikipedia #wikiomnia #squad #QA #arxiv-2204.08009 #region-us
|
# Dataset Card for "Wikiomnia"
## Table of Contents
- Dataset Description
- Dataset Summary
- Dataset Structure
- Dataset Creation
- Additional Information
- Licensing Information
- Citation Information
- Contributions
## Dataset Description
- Repository: URL
- Paper: WikiOmnia: filtration and evaluation of the generated QA corpus on the whole Russian Wikipedia
- Point of Contact:
### Dataset Summary
We present the WikiOmnia dataset, a new publicly available set of QA-pairs and corresponding Russian Wikipedia article summary sections, composed with a fully automated generative pipeline. The dataset includes every available article from Wikipedia for the Russian language. The WikiOmnia pipeline is available open-source and is also tested for creating SQuAD-formatted QA on other domains, like news texts, fiction, and social media. The resulting dataset includes two parts: raw data on the whole Russian Wikipedia (7,930,873 QA pairs with paragraphs for ruGPT-3 XL and 7,991,040 QA pairs with paragraphs for ruT5-large) and cleaned data with strict automatic verification (over 160,000 QA pairs with paragraphs for ruGPT-3 XL and over 3,400,000 QA pairs with paragraphs for ruT5-large).
WikiOmnia consists of 2 parts:
1. the voluminous, automatically generated part: 15,9 million triplets consisting of the original article summary, a corresponding generated question and a generated answer;
2. the filtered part: the subsample of 3,5 million triplets, fully verified with automatic means
Wikiomnia adheres to a standard SQuAD format problem, resulting in triplets "text paragraph - question based on paragraph - answer from the paragraph", see the following example:
Original Wikipedia paragraph: Коити Масимо (яп. Масимо Ко:ити) — известный режиссёр аниме и основатель японской анимационной студии Bee Train. С
момента основания студии он руководит производством почти всех её картин, а также время от времени принимает участие в работе над анимацией и музыкой.
English translation: Koichi Mashimo is a famous anime director and the founder of the Japanese animation studio Bee Train. Since the creation of the studio, he directed almost all studio’s works, and he
also sometimes participates in art and sound tasks.
Generated question (ruT5): Кто является основателем японской анимационной студии Bee Train?
Generated answer (ruT5): Коити Масимо
English QA translation: Who is the founder of the Japanese animation studio Bee Train? Koichi Mashimo
## Dataset Creation
Models used for dataset generation:
- ruT5 large fine-tuned on SberQuaD
- ruGPT-3 XL fine-tuned on SberQuaD
- ruBERT DeepPavlov tuned for QA tasks
Source: Wikipedia version March 2021
Special tokens: <[TEXT]>, <[QUESTION]>, <[ANSWER]>
The resulting dataset includes two parts: raw data on the whole Russian Wikipedia (7,930,873 QA pairs with paragraphs for ruGPT-3 XL and 7,991,040 QA pairs with paragraphs for ruT5-
large) and cleaned data with strict automatic verification (over 160,000 QA pairs with paragraphs for ruGPT-3 XL and over 3,400,000 QA pairs with paragraphs for ruT5-large).
 and cleaned data with strict automatic verification (over 160,000 QA pairs with paragraphs for ruGPT-3 XL and over 3,400,000 QA pairs with paragraphs for ruT5-large).\n\nWikiOmnia consists of 2 parts:\n1. the voluminous, automatically generated part: 15,9 million triplets consisting of the original article summary, a corresponding generated question and a generated answer;\n2. the filtered part: the subsample of 3,5 million triplets, fully verified with automatic means\n\nWikiomnia adheres to a standard SQuAD format problem, resulting in triplets \"text paragraph - question based on paragraph - answer from the paragraph\", see the following example:\n\nOriginal Wikipedia paragraph: Коити Масимо (яп. Масимо Ко:ити) — известный режиссёр аниме и основатель японской анимационной студии Bee Train. С\nмомента основания студии он руководит производством почти всех её картин, а также время от времени принимает участие в работе над анимацией и музыкой.\n\nEnglish translation: Koichi Mashimo is a famous anime director and the founder of the Japanese animation studio Bee Train. Since the creation of the studio, he directed almost all studio’s works, and he\nalso sometimes participates in art and sound tasks. \n\nGenerated question (ruT5): Кто является основателем японской анимационной студии Bee Train?\n\nGenerated answer (ruT5): Коити Масимо \n\nEnglish QA translation: Who is the founder of the Japanese animation studio Bee Train? Koichi Mashimo",
"## Dataset Creation\n\nModels used for dataset generation:\n - ruT5 large fine-tuned on SberQuaD\n - ruGPT-3 XL fine-tuned on SberQuaD\n - ruBERT DeepPavlov tuned for QA tasks\n\nSource: Wikipedia version March 2021\n\nSpecial tokens: <[TEXT]>, <[QUESTION]>, <[ANSWER]>\n\nThe resulting dataset includes two parts: raw data on the whole Russian Wikipedia (7,930,873 QA pairs with paragraphs for ruGPT-3 XL and 7,991,040 QA pairs with paragraphs for ruT5-\nlarge) and cleaned data with strict automatic verification (over 160,000 QA pairs with paragraphs for ruGPT-3 XL and over 3,400,000 QA pairs with paragraphs for ruT5-large).\n\n and cleaned data with strict automatic verification (over 160,000 QA pairs with paragraphs for ruGPT-3 XL and over 3,400,000 QA pairs with paragraphs for ruT5-large).\n\nWikiOmnia consists of 2 parts:\n1. the voluminous, automatically generated part: 15,9 million triplets consisting of the original article summary, a corresponding generated question and a generated answer;\n2. the filtered part: the subsample of 3,5 million triplets, fully verified with automatic means\n\nWikiomnia adheres to a standard SQuAD format problem, resulting in triplets \"text paragraph - question based on paragraph - answer from the paragraph\", see the following example:\n\nOriginal Wikipedia paragraph: Коити Масимо (яп. Масимо Ко:ити) — известный режиссёр аниме и основатель японской анимационной студии Bee Train. С\nмомента основания студии он руководит производством почти всех её картин, а также время от времени принимает участие в работе над анимацией и музыкой.\n\nEnglish translation: Koichi Mashimo is a famous anime director and the founder of the Japanese animation studio Bee Train. Since the creation of the studio, he directed almost all studio’s works, and he\nalso sometimes participates in art and sound tasks. \n\nGenerated question (ruT5): Кто является основателем японской анимационной студии Bee Train?\n\nGenerated answer (ruT5): Коити Масимо \n\nEnglish QA translation: Who is the founder of the Japanese animation studio Bee Train? Koichi Mashimo",
"## Dataset Creation\n\nModels used for dataset generation:\n - ruT5 large fine-tuned on SberQuaD\n - ruGPT-3 XL fine-tuned on SberQuaD\n - ruBERT DeepPavlov tuned for QA tasks\n\nSource: Wikipedia version March 2021\n\nSpecial tokens: <[TEXT]>, <[QUESTION]>, <[ANSWER]>\n\nThe resulting dataset includes two parts: raw data on the whole Russian Wikipedia (7,930,873 QA pairs with paragraphs for ruGPT-3 XL and 7,991,040 QA pairs with paragraphs for ruT5-\nlarge) and cleaned data with strict automatic verification (over 160,000 QA pairs with paragraphs for ruGPT-3 XL and over 3,400,000 QA pairs with paragraphs for ruT5-large).\n\n
- **Paper:** Radford et al. [Language Models are Unsupervised Multitask Learners](https://d4mucfpksywv.cloudfront.net/better-language-models/language-models.pdf)
### Dataset Summary
This dataset is comprised of the LAMBADA test split as pre-processed by OpenAI (see relevant discussions [here](https://github.com/openai/gpt-2/issues/131#issuecomment-497136199) and [here](https://github.com/huggingface/transformers/issues/491)). It also contains machine translated versions of the split in German, Spanish, French, and Italian.
LAMBADA is used to evaluate the capabilities of computational models for text understanding by means of a word prediction task. LAMBADA is a collection of narrative texts sharing the characteristic that human subjects are able to guess their last word if they are exposed to the whole text, but not if they only see the last sentence preceding the target word. To succeed on LAMBADA, computational models cannot simply rely on local context, but must be able to keep track of information in the broader discourse.
### Languages
English, German, Spanish, French, and Italian.
### Source Data
For non-English languages, the data splits were produced by Google Translate. See the [`translation_script.py`](translation_script.py) for more details.
## Additional Information
### Hash Checksums
For data integrity checks we leave the following checksums for the files in this dataset:
| File Name | Checksum (SHA-256) |
|--------------------------------------------------------------------------|------------------------------------------------------------------|
| lambada_test_de.jsonl | 51c6c1795894c46e88e4c104b5667f488efe79081fb34d746b82b8caa663865e |
| [openai/lambada_test.jsonl](https://openaipublic.blob.core.windows.net/gpt-2/data/lambada_test.jsonl) | 4aa8d02cd17c719165fc8a7887fddd641f43fcafa4b1c806ca8abc31fabdb226 |
| lambada_test_en.jsonl | 4aa8d02cd17c719165fc8a7887fddd641f43fcafa4b1c806ca8abc31fabdb226 |
| lambada_test_es.jsonl | ffd760026c647fb43c67ce1bc56fd527937304b348712dce33190ea6caba6f9c |
| lambada_test_fr.jsonl | 941ec6a73dba7dc91c860bf493eb66a527cd430148827a4753a4535a046bf362 |
| lambada_test_it.jsonl | 86654237716702ab74f42855ae5a78455c1b0e50054a4593fb9c6fcf7fad0850 |
### Licensing
License: [Modified MIT](https://github.com/openai/gpt-2/blob/master/LICENSE)
### Citation
```bibtex
@article{radford2019language,
title={Language Models are Unsupervised Multitask Learners},
author={Radford, Alec and Wu, Jeff and Child, Rewon and Luan, David and Amodei, Dario and Sutskever, Ilya},
year={2019}
}
```
```bibtex
@misc{
author={Paperno, Denis and Kruszewski, Germán and Lazaridou, Angeliki and Pham, Quan Ngoc and Bernardi, Raffaella and Pezzelle, Sandro and Baroni, Marco and Boleda, Gemma and Fernández, Raquel},
title={The LAMBADA dataset},
DOI={10.5281/zenodo.2630551},
publisher={Zenodo},
year={2016},
month={Aug}
}
```
### Contributions
Thanks to Sid Black ([@sdtblck](https://github.com/sdtblck)) for translating the `lambada_openai` dataset into the non-English languages.
Thanks to Jonathan Tow ([@jon-tow](https://github.com/jon-tow)) for adding this dataset.
| EleutherAI/lambada_openai | [
"task_ids:language-modeling",
"language_creators:machine-generated",
"multilinguality:translation",
"size_categories:1K<n<10K",
"source_datasets:lambada",
"language:de",
"language:en",
"language:es",
"language:fr",
"language:it",
"license:mit",
"region:us"
] | 2022-12-16T16:35:07+00:00 | {"language_creators": ["machine-generated"], "language": ["de", "en", "es", "fr", "it"], "license": "mit", "multilinguality": ["translation"], "size_categories": ["1K<n<10K"], "source_datasets": ["lambada"], "task_ids": ["language-modeling"], "pretty_name": "LAMBADA OpenAI", "dataset_info": [{"config_name": "default", "features": [{"name": "text", "dtype": "string"}], "splits": [{"name": "test", "num_bytes": 1709449, "num_examples": 5153}], "download_size": 1819752, "dataset_size": 1709449}, {"config_name": "de", "features": [{"name": "text", "dtype": "string"}], "splits": [{"name": "test", "num_bytes": 1904576, "num_examples": 5153}], "download_size": 1985231, "dataset_size": 1904576}, {"config_name": "en", "features": [{"name": "text", "dtype": "string"}], "splits": [{"name": "test", "num_bytes": 1709449, "num_examples": 5153}], "download_size": 1819752, "dataset_size": 1709449}, {"config_name": "es", "features": [{"name": "text", "dtype": "string"}], "splits": [{"name": "test", "num_bytes": 1821735, "num_examples": 5153}], "download_size": 1902349, "dataset_size": 1821735}, {"config_name": "fr", "features": [{"name": "text", "dtype": "string"}], "splits": [{"name": "test", "num_bytes": 1948795, "num_examples": 5153}], "download_size": 2028703, "dataset_size": 1948795}, {"config_name": "it", "features": [{"name": "text", "dtype": "string"}], "splits": [{"name": "test", "num_bytes": 1813420, "num_examples": 5153}], "download_size": 1894613, "dataset_size": 1813420}]} | 2022-12-16T19:53:23+00:00 | [] | [
"de",
"en",
"es",
"fr",
"it"
] | TAGS
#task_ids-language-modeling #language_creators-machine-generated #multilinguality-translation #size_categories-1K<n<10K #source_datasets-lambada #language-German #language-English #language-Spanish #language-French #language-Italian #license-mit #region-us
| Dataset Description
-------------------
* Repository: openai/gpt2
* Paper: Radford et al. Language Models are Unsupervised Multitask Learners
### Dataset Summary
This dataset is comprised of the LAMBADA test split as pre-processed by OpenAI (see relevant discussions here and here). It also contains machine translated versions of the split in German, Spanish, French, and Italian.
LAMBADA is used to evaluate the capabilities of computational models for text understanding by means of a word prediction task. LAMBADA is a collection of narrative texts sharing the characteristic that human subjects are able to guess their last word if they are exposed to the whole text, but not if they only see the last sentence preceding the target word. To succeed on LAMBADA, computational models cannot simply rely on local context, but must be able to keep track of information in the broader discourse.
### Languages
English, German, Spanish, French, and Italian.
### Source Data
For non-English languages, the data splits were produced by Google Translate. See the 'translation\_script.py' for more details.
Additional Information
----------------------
### Hash Checksums
For data integrity checks we leave the following checksums for the files in this dataset:
### Licensing
License: Modified MIT
### Contributions
Thanks to Sid Black (@sdtblck) for translating the 'lambada\_openai' dataset into the non-English languages.
Thanks to Jonathan Tow (@jon-tow) for adding this dataset.
| [
"### Dataset Summary\n\n\nThis dataset is comprised of the LAMBADA test split as pre-processed by OpenAI (see relevant discussions here and here). It also contains machine translated versions of the split in German, Spanish, French, and Italian.\n\n\nLAMBADA is used to evaluate the capabilities of computational models for text understanding by means of a word prediction task. LAMBADA is a collection of narrative texts sharing the characteristic that human subjects are able to guess their last word if they are exposed to the whole text, but not if they only see the last sentence preceding the target word. To succeed on LAMBADA, computational models cannot simply rely on local context, but must be able to keep track of information in the broader discourse.",
"### Languages\n\n\nEnglish, German, Spanish, French, and Italian.",
"### Source Data\n\n\nFor non-English languages, the data splits were produced by Google Translate. See the 'translation\\_script.py' for more details.\n\n\nAdditional Information\n----------------------",
"### Hash Checksums\n\n\nFor data integrity checks we leave the following checksums for the files in this dataset:",
"### Licensing\n\n\nLicense: Modified MIT",
"### Contributions\n\n\nThanks to Sid Black (@sdtblck) for translating the 'lambada\\_openai' dataset into the non-English languages.\n\n\nThanks to Jonathan Tow (@jon-tow) for adding this dataset."
] | [
"TAGS\n#task_ids-language-modeling #language_creators-machine-generated #multilinguality-translation #size_categories-1K<n<10K #source_datasets-lambada #language-German #language-English #language-Spanish #language-French #language-Italian #license-mit #region-us \n",
"### Dataset Summary\n\n\nThis dataset is comprised of the LAMBADA test split as pre-processed by OpenAI (see relevant discussions here and here). It also contains machine translated versions of the split in German, Spanish, French, and Italian.\n\n\nLAMBADA is used to evaluate the capabilities of computational models for text understanding by means of a word prediction task. LAMBADA is a collection of narrative texts sharing the characteristic that human subjects are able to guess their last word if they are exposed to the whole text, but not if they only see the last sentence preceding the target word. To succeed on LAMBADA, computational models cannot simply rely on local context, but must be able to keep track of information in the broader discourse.",
"### Languages\n\n\nEnglish, German, Spanish, French, and Italian.",
"### Source Data\n\n\nFor non-English languages, the data splits were produced by Google Translate. See the 'translation\\_script.py' for more details.\n\n\nAdditional Information\n----------------------",
"### Hash Checksums\n\n\nFor data integrity checks we leave the following checksums for the files in this dataset:",
"### Licensing\n\n\nLicense: Modified MIT",
"### Contributions\n\n\nThanks to Sid Black (@sdtblck) for translating the 'lambada\\_openai' dataset into the non-English languages.\n\n\nThanks to Jonathan Tow (@jon-tow) for adding this dataset."
] |
a8833d9ce9d08dcb1fdd1a4a926c94a05a6e7a84 | # Dataset Card for "australian_sea_slugs"
This is a filtered version of the [Nudibranchs of the Sunshine Coast Australia](https://www.gbif.org/dataset/ee412fa2-edc9-4c6b-91f3-ff2a02c245e0) dataset.
## Citation
```
Atlas of Living Australia (2019). Nudibranchs of the Sunshine Coast Australia. Occurrence dataset https://doi.org/10.15468/gtoiks accessed via GBIF.org on 2022-12-16.
``` | sasha/australian_sea_slugs | [
"region:us"
] | 2022-12-16T17:34:52+00:00 | {"dataset_info": {"features": [{"name": "url", "dtype": "string"}, {"name": "image", "dtype": "image"}, {"name": "label", "dtype": "string"}], "splits": [{"name": "train", "num_bytes": 86677304.65602817, "num_examples": 2107}], "download_size": 87406259, "dataset_size": 86677304.65602817}} | 2022-12-16T17:37:05+00:00 | [] | [] | TAGS
#region-us
| # Dataset Card for "australian_sea_slugs"
This is a filtered version of the Nudibranchs of the Sunshine Coast Australia dataset.
| [
"# Dataset Card for \"australian_sea_slugs\"\n\nThis is a filtered version of the Nudibranchs of the Sunshine Coast Australia dataset."
] | [
"TAGS\n#region-us \n",
"# Dataset Card for \"australian_sea_slugs\"\n\nThis is a filtered version of the Nudibranchs of the Sunshine Coast Australia dataset."
] |
50b606e9d78a38848b8452d72072678cbeaedd05 | This dataset contains information about the 9761 witches from the Crypto Coven NFT project (https://www.cryptocoven.xyz/) collected using OpenSea API.
The folder 'witch_images' includes the images of each witch in three different sizes.
I briefly describe the data in the `witches.csv` below:
- `id`: the id of the witch
- `num_sales`: number of sales in the past (till 4/21/2022 the day I collected the data)
- `name`: the name of the witch
- `description`: the description of the witch
- `external_link`: the link to the official page for the witch
- `permalink`: the OpenSea link for the witch
- `token_metadata`: the metadata JSON file about the witch
- `token_id`: the token_id of the NFT
- `owner.user.username`: the user name of the current owner
- `owner.address`: the wallet address of the current owner
- `last_sale.total_price`: the price of the last sale in gwei. Note that the unit here is gwei (giga and wei) and 1 ether = 1 billion gwei (18 zeros)
- `last_sale.payment_token.usd_price`: the USD price of 1 ether (ETH) for the last sale
- `last_sale.transaction.timestamp`: the timestamp of the last sale
- `properties`: there are 32 properties of each witch covering the different design elements of each witch, such as Skin Tone, Eyebrows, Body Shape, etc.
`witches_full.csv` is the full data provided by the OpenSea API, such as https://api.opensea.io/api/v1/asset/0x5180db8f5c931aae63c74266b211f580155ecac8/50. I just simply flattened the JSON returned by the API. | harrywang/crypto-coven | [
"license:mit",
"region:us"
] | 2022-12-16T17:57:33+00:00 | {"license": "mit"} | 2022-12-16T18:00:36+00:00 | [] | [] | TAGS
#license-mit #region-us
| This dataset contains information about the 9761 witches from the Crypto Coven NFT project (URL collected using OpenSea API.
The folder 'witch_images' includes the images of each witch in three different sizes.
I briefly describe the data in the 'URL' below:
- 'id': the id of the witch
- 'num_sales': number of sales in the past (till 4/21/2022 the day I collected the data)
- 'name': the name of the witch
- 'description': the description of the witch
- 'external_link': the link to the official page for the witch
- 'permalink': the OpenSea link for the witch
- 'token_metadata': the metadata JSON file about the witch
- 'token_id': the token_id of the NFT
- 'URL.username': the user name of the current owner
- 'owner.address': the wallet address of the current owner
- 'last_sale.total_price': the price of the last sale in gwei. Note that the unit here is gwei (giga and wei) and 1 ether = 1 billion gwei (18 zeros)
- 'last_sale.payment_token.usd_price': the USD price of 1 ether (ETH) for the last sale
- 'last_sale.transaction.timestamp': the timestamp of the last sale
- 'properties': there are 32 properties of each witch covering the different design elements of each witch, such as Skin Tone, Eyebrows, Body Shape, etc.
'witches_full.csv' is the full data provided by the OpenSea API, such as URL I just simply flattened the JSON returned by the API. | [] | [
"TAGS\n#license-mit #region-us \n"
] |
75f72c304cfde536c03d1ecb0b63e564424338da | # Dataset Card for "full-hh-rlhf"
Anthropic's HH dataset reformatted into prompt, chosen, rejected samples. | Dahoas/full-hh-rlhf | [
"region:us"
] | 2022-12-16T20:45:27+00:00 | {"dataset_info": {"features": [{"name": "prompt", "dtype": "string"}, {"name": "response", "dtype": "string"}, {"name": "chosen", "dtype": "string"}, {"name": "rejected", "dtype": "string"}], "splits": [{"name": "train", "num_bytes": 203150123, "num_examples": 112052}, {"name": "test", "num_bytes": 22606646, "num_examples": 12451}], "download_size": 136150742, "dataset_size": 225756769}} | 2023-02-23T17:29:46+00:00 | [] | [] | TAGS
#region-us
| # Dataset Card for "full-hh-rlhf"
Anthropic's HH dataset reformatted into prompt, chosen, rejected samples. | [
"# Dataset Card for \"full-hh-rlhf\"\n\nAnthropic's HH dataset reformatted into prompt, chosen, rejected samples."
] | [
"TAGS\n#region-us \n",
"# Dataset Card for \"full-hh-rlhf\"\n\nAnthropic's HH dataset reformatted into prompt, chosen, rejected samples."
] |
49677eb53625813b5a9ce88938e1c7a40ce97c0d | # Dataset Card for "QuadraticEquations2"
[More Information needed](https://github.com/huggingface/datasets/blob/main/CONTRIBUTING.md#how-to-contribute-to-the-dataset-cards) | snork-maiden/QuadraticEquations2 | [
"region:us"
] | 2022-12-17T07:14:36+00:00 | {"dataset_info": {"features": [{"name": "text", "sequence": "int64"}, {"name": "label", "dtype": "int64"}, {"name": "__index_level_0__", "dtype": "int64"}], "splits": [{"name": "train", "num_bytes": 3520000, "num_examples": 80000}, {"name": "test", "num_bytes": 880000, "num_examples": 20000}], "download_size": 1308051, "dataset_size": 4400000}} | 2022-12-17T07:35:13+00:00 | [] | [] | TAGS
#region-us
| # Dataset Card for "QuadraticEquations2"
More Information needed | [
"# Dataset Card for \"QuadraticEquations2\"\n\nMore Information needed"
] | [
"TAGS\n#region-us \n",
"# Dataset Card for \"QuadraticEquations2\"\n\nMore Information needed"
] |
6c125a5f38f2f1d45d9cfdf71834ecdcf2565fd2 | # Dataset Card for "salvadoran-news"
[More Information needed](https://github.com/huggingface/datasets/blob/main/CONTRIBUTING.md#how-to-contribute-to-the-dataset-cards) | justinian336/salvadoran-news | [
"region:us"
] | 2022-12-17T07:32:24+00:00 | {"dataset_info": {"features": [{"name": "title", "dtype": "string"}, {"name": "content", "dtype": "string"}, {"name": "link", "dtype": "string"}], "splits": [{"name": "train", "num_bytes": 276670922, "num_examples": 102366}], "download_size": 159243312, "dataset_size": 276670922}} | 2023-03-21T05:38:49+00:00 | [] | [] | TAGS
#region-us
| # Dataset Card for "salvadoran-news"
More Information needed | [
"# Dataset Card for \"salvadoran-news\"\n\nMore Information needed"
] | [
"TAGS\n#region-us \n",
"# Dataset Card for \"salvadoran-news\"\n\nMore Information needed"
] |
fe0dd8b35b749f383c063d9bab4dc35fddbe1896 | # Dataset Card for "natural_questions"
[More Information needed](https://github.com/huggingface/datasets/blob/main/CONTRIBUTING.md#how-to-contribute-to-the-dataset-cards) | maximedb/natural_questions | [
"region:us"
] | 2022-12-17T08:16:54+00:00 | {"dataset_info": {"features": [{"name": "question", "dtype": "string"}, {"name": "answer", "dtype": "string"}], "splits": [{"name": "train", "num_bytes": 10087609, "num_examples": 130233}, {"name": "validation", "num_bytes": 714323, "num_examples": 8643}], "download_size": 6827128, "dataset_size": 10801932}} | 2022-12-17T08:17:26+00:00 | [] | [] | TAGS
#region-us
| # Dataset Card for "natural_questions"
More Information needed | [
"# Dataset Card for \"natural_questions\"\n\nMore Information needed"
] | [
"TAGS\n#region-us \n",
"# Dataset Card for \"natural_questions\"\n\nMore Information needed"
] |
4b0deef671013a7379b62f0b3c18e718a079560e |
## Download
| Windows-CUDA11.6 | Windows-CUDA11.3 |
| ------------------------ | ------------------------ |
| [Download](./116env.zip) | [Download](./113env.zip) |
## Usage
```bash
./{folder name}/Scripts/Activate.ps1
```
| HuanLin/DiffSVC-WindowsENV | [
"license:gpl",
"region:us"
] | 2022-12-17T12:46:19+00:00 | {"license": "gpl"} | 2022-12-17T12:58:21+00:00 | [] | [] | TAGS
#license-gpl #region-us
| Download
--------
Usage
-----
| [] | [
"TAGS\n#license-gpl #region-us \n"
] |
d05e3a37229b15ffd95ef2f7c24356c85cc9575d |
tweets in english positive negative | ad321/test-tweets | [
"task_categories:text-classification",
"task_ids:sentiment-classification",
"annotations_creators:crowdsourced",
"language_creators:crowdsourced",
"multilinguality:monolingual",
"size_categories:1M<n<10M",
"source_datasets:original",
"language:en",
"license:gpl-3.0",
"region:us"
] | 2022-12-17T13:39:02+00:00 | {"annotations_creators": ["crowdsourced"], "language_creators": ["crowdsourced"], "language": ["en"], "license": ["gpl-3.0"], "multilinguality": ["monolingual"], "size_categories": ["1M<n<10M"], "source_datasets": ["original"], "task_categories": ["text-classification"], "task_ids": ["sentiment-classification"], "pretty_name": "tweeter-dataset-sent-analysis", "tags": [], "train-eval-index": [{"col_mapping": {"label": "labels", "metrics": [{"name": "Accuracy", "type": "accuracy"}, {"args": {"average": "binary"}, "name": "F1 binary", "type": "f1"}], "tweet": "text"}, "config": "default", "splits": {"train_split": "train", "validation_split": "validation"}, "task": "text-classification", "task_id": "binary_classification"}]} | 2022-12-17T14:34:45+00:00 | [] | [
"en"
] | TAGS
#task_categories-text-classification #task_ids-sentiment-classification #annotations_creators-crowdsourced #language_creators-crowdsourced #multilinguality-monolingual #size_categories-1M<n<10M #source_datasets-original #language-English #license-gpl-3.0 #region-us
|
tweets in english positive negative | [] | [
"TAGS\n#task_categories-text-classification #task_ids-sentiment-classification #annotations_creators-crowdsourced #language_creators-crowdsourced #multilinguality-monolingual #size_categories-1M<n<10M #source_datasets-original #language-English #license-gpl-3.0 #region-us \n"
] |
b8b5d13bb5657c0b8b93a10ab987decac868214e |
# Doc2Query Generated Queries for `msmarco-passage`
This dataset provides the pre-computed generated queries for the [`msmarco-passage`](https://ir-datasets.com/msmarco-passage) dataset,
for use when indexing Doc2Query.
The generated queries from from the T5 Doc2Query model, released by the original authors [here](https://github.com/castorini/docTTTTTquery).
## Getting started
This artefact is meant to be used with the [`pyterrier_doc2query`](https://github.com/terrierteam/pyterrier_doc2query) pacakge. It can
be installed as:
```bash
pip install git+https://github.com/terrierteam/pyterrier_doc2query
```
Depending on what you are using this aretefact for, you may also need the following additional package:
```bash
pip install git+https://github.com/terrierteam/pyterrier_pisa # for indexing / retrieval
```
## Using this artefact
The main use case is to use this aretefact in a Doc2Query indexing pipeline:
```python
import pyterrier as pt ; pt.init()
from pyterrier_pisa import PisaIndex
from pyterrier_doc2query import Doc2QueryStore
store = Doc2QueryStore.from_repo('https://huggingface.co/datasets/macavaney/d2q-msmarco-passage')
index = PisaIndex('path/to/index')
pipeline = store.generator(limit_k=40) >> index
dataset = pt.get_dataset('irds:msmarco-passage')
pipeline.index(dataset.get_corpus_iter())
```
You can also use the store directly as a dataset to look up or iterate over the data:
```python
store.lookup('100')
# {'querygen': ...}
for record in store:
pass
```
## Reproducing this aretefact
Due to the random nature of the Doc2Query generation process, this artefact cannot be reproduced verbatim.
This aretefact can be reproduced using the following pipeline:
The following runs Doc2Query inference over the MS MARCO dataset. It will not produce the artefact verbatim,
but should produce similar results when used for indexing/retrieval.
```python
import pyterrier as pt ; pt.init()
from pyterrier_doc2query import Doc2Query, Doc2QueryStore
doc2query = Doc2Query('macavaney/doc2query-t5-base-msmarco', num_samples=80)
store = Doc2QueryStore('path/to/store')
pipeline = doc2query >> store
dataset = pt.get_dataset('irds:msmarco-passage')
pipeline.index(dataset.get_corpus_iter())
```
Note that this process will take quite some time, since it generates 80 queries for every document in the dataset.
Alternatively, you could reproduce this artefact verbatim using the following script, but it doesn't perform
model inference; it just uses the pre-generated queries from the original authors.
```bash
wget https://git.uwaterloo.ca/jimmylin/doc2query-data/raw/master/T5-passage/predicted_queries_topk_sampling.zip
unzip predicted_queries_topk_sampling.zip
```
```python
from pyterrier_doc2query import Doc2QueryStore
import os
import ir_datasets
def iter_files(path):
i = 0
while os.path.exists(path.format(i)):
with open(path.format(i), 'rt') as fin:
for line in fin:
yield line.strip()
i += 1
def it():
file_iters = [iter_files('predicted_queries_topk_sample{:03}'.format(i)+'.txt{:03}-1004000') for i in range(80)]
for queries in enumerate(zip(*file_iters)):
yield {'docno': str(i), 'querygen': '\n'.join(queries)}
store = Doc2QueryStore('path/to/store')
store.index(it())
```
| macavaney/d2q-msmarco-passage | [
"task_categories:text-retrieval",
"task_ids:document-retrieval",
"annotations_creators:no-annotation",
"language_creators:machine-generated",
"source_datasets:msmarco-passage",
"document-expansion",
"doc2query",
"region:us"
] | 2022-12-17T14:53:07+00:00 | {"annotations_creators": ["no-annotation"], "language_creators": ["machine-generated"], "language": [], "license": [], "source_datasets": ["msmarco-passage"], "task_categories": ["text-retrieval"], "task_ids": ["document-retrieval"], "pretty_name": "Doc2Query Generated Queries for `msmarco-passage`", "tags": ["document-expansion", "doc2query"], "viewer": false} | 2022-12-18T20:12:57+00:00 | [] | [] | TAGS
#task_categories-text-retrieval #task_ids-document-retrieval #annotations_creators-no-annotation #language_creators-machine-generated #source_datasets-msmarco-passage #document-expansion #doc2query #region-us
|
# Doc2Query Generated Queries for 'msmarco-passage'
This dataset provides the pre-computed generated queries for the 'msmarco-passage' dataset,
for use when indexing Doc2Query.
The generated queries from from the T5 Doc2Query model, released by the original authors here.
## Getting started
This artefact is meant to be used with the 'pyterrier_doc2query' pacakge. It can
be installed as:
Depending on what you are using this aretefact for, you may also need the following additional package:
## Using this artefact
The main use case is to use this aretefact in a Doc2Query indexing pipeline:
You can also use the store directly as a dataset to look up or iterate over the data:
## Reproducing this aretefact
Due to the random nature of the Doc2Query generation process, this artefact cannot be reproduced verbatim.
This aretefact can be reproduced using the following pipeline:
The following runs Doc2Query inference over the MS MARCO dataset. It will not produce the artefact verbatim,
but should produce similar results when used for indexing/retrieval.
Note that this process will take quite some time, since it generates 80 queries for every document in the dataset.
Alternatively, you could reproduce this artefact verbatim using the following script, but it doesn't perform
model inference; it just uses the pre-generated queries from the original authors.
| [
"# Doc2Query Generated Queries for 'msmarco-passage'\n\nThis dataset provides the pre-computed generated queries for the 'msmarco-passage' dataset,\nfor use when indexing Doc2Query.\n\nThe generated queries from from the T5 Doc2Query model, released by the original authors here.",
"## Getting started\n\nThis artefact is meant to be used with the 'pyterrier_doc2query' pacakge. It can\nbe installed as:\n\n\n\nDepending on what you are using this aretefact for, you may also need the following additional package:",
"## Using this artefact\n\nThe main use case is to use this aretefact in a Doc2Query indexing pipeline:\n\n\n\nYou can also use the store directly as a dataset to look up or iterate over the data:",
"## Reproducing this aretefact\n\nDue to the random nature of the Doc2Query generation process, this artefact cannot be reproduced verbatim.\nThis aretefact can be reproduced using the following pipeline:\n\nThe following runs Doc2Query inference over the MS MARCO dataset. It will not produce the artefact verbatim,\nbut should produce similar results when used for indexing/retrieval.\n\n\n\nNote that this process will take quite some time, since it generates 80 queries for every document in the dataset.\n\nAlternatively, you could reproduce this artefact verbatim using the following script, but it doesn't perform\nmodel inference; it just uses the pre-generated queries from the original authors."
] | [
"TAGS\n#task_categories-text-retrieval #task_ids-document-retrieval #annotations_creators-no-annotation #language_creators-machine-generated #source_datasets-msmarco-passage #document-expansion #doc2query #region-us \n",
"# Doc2Query Generated Queries for 'msmarco-passage'\n\nThis dataset provides the pre-computed generated queries for the 'msmarco-passage' dataset,\nfor use when indexing Doc2Query.\n\nThe generated queries from from the T5 Doc2Query model, released by the original authors here.",
"## Getting started\n\nThis artefact is meant to be used with the 'pyterrier_doc2query' pacakge. It can\nbe installed as:\n\n\n\nDepending on what you are using this aretefact for, you may also need the following additional package:",
"## Using this artefact\n\nThe main use case is to use this aretefact in a Doc2Query indexing pipeline:\n\n\n\nYou can also use the store directly as a dataset to look up or iterate over the data:",
"## Reproducing this aretefact\n\nDue to the random nature of the Doc2Query generation process, this artefact cannot be reproduced verbatim.\nThis aretefact can be reproduced using the following pipeline:\n\nThe following runs Doc2Query inference over the MS MARCO dataset. It will not produce the artefact verbatim,\nbut should produce similar results when used for indexing/retrieval.\n\n\n\nNote that this process will take quite some time, since it generates 80 queries for every document in the dataset.\n\nAlternatively, you could reproduce this artefact verbatim using the following script, but it doesn't perform\nmodel inference; it just uses the pre-generated queries from the original authors."
] |
32d6db2e304ddfb33cbb6e2243ad42caf4ab32ab |
# Dataset Card for PolQA Dataset
## Dataset Description
- **Paper:** [Improving Question Answering Performance through Manual Annotation: Costs, Benefits and Strategies](https://arxiv.org/abs/2212.08897)
- **Point of Contact:** [Piotr Rybak](mailto:[email protected])
### Dataset Summary
PolQA is the first Polish dataset for open-domain question answering. It consists of 7,000 questions, 87,525 manually labeled evidence passages, and a corpus of over 7 million candidate passages. The dataset can be used to train both a passage retriever and an abstractive reader.
### Supported Tasks and Leaderboards
- `open-domain-qa`: The dataset can be used to train a model for open-domain question answering. Success on this task is typically measured using [metric defined during PolEval 2021](https://2021.poleval.pl/tasks/task4).
- `document-retrieval`: The dataset can be used to train a model for document retrieval. Success on this task is typically measured by [top-k retrieval accuracy](https://scikit-learn.org/stable/modules/generated/sklearn.metrics.top_k_accuracy_score.html) or [NDCG](https://scikit-learn.org/stable/modules/generated/sklearn.metrics.ndcg_score.html).
- `abstractive-qa`: The dataset can be used to train a model for abstractive question answering. Success on this task is typically measured using [metric defined during PolEval 2021](https://2021.poleval.pl/tasks/task4).
### Languages
The text is in Polish, as spoken by the host of the [Jeden z Dziesięciu](https://pl.wikipedia.org/wiki/Jeden_z_dziesi%C4%99ciu) TV show (questions) and [Polish Wikipedia](https://pl.wikipedia.org/) editors (passages). The BCP-47 code for Polish is pl-PL.
## Dataset Structure
### Data Instances
The main part of the dataset consists of manually annotated question-passage pairs. For each instance, there is a `question`, a passage (`passage_id`, `passage_title`, `passage_text`), and a boolean indicator if the passage is `relevant` for the given question (i.e. does it contain the answers).
For each `question` there is a list of possible `answers` formulated in a natural language, in a way a Polish
speaker would answer the questions. It means that the answers might
contain prepositions, be inflected, and contain punctuation. In some
cases, the answer might have multiple correct variants, e.g. numbers
are written as numerals and words, synonyms, abbreviations and their
expansions.
Additionally, we provide a classification of each question-answer pair based on the `question_formulation`, the `question_type`, and the `entity_type/entity_subtype`, according to the taxonomy proposed by
[Maciej Ogrodniczuk and Piotr Przybyła (2021)](http://nlp.ipipan.waw.pl/Bib/ogr:prz:21:poleval.pdf).
```
{
'question_id': 6,
'passage_title': 'Mumbaj',
'passage_text': 'Mumbaj lub Bombaj (marathi मुंबई, trb.: Mumbaj; ang. Mumbai; do 1995 Bombay) – stolica indyjskiego stanu Maharasztra, położona na wyspie Salsette, na Morzu Arabskim.',
'passage_wiki': 'Mumbaj lub Bombaj (mr. मुंबई, trb.: "Mumbaj"; ang. Mumbai; do 1995 Bombay) – stolica indyjskiego stanu Maharasztra, położona na wyspie Salsette, na Morzu Arabskim. Wraz z miastami satelitarnymi tworzy najludniejszą po Delhi aglomerację liczącą 23 miliony mieszkańców. Dzięki naturalnemu położeniu jest to największy port morski kraju. Znajdują się tutaj także najsilniejsze giełdy Azji Południowej: National Stock Exchange of India i Bombay Stock Exchange.',
'passage_id': '42609-0',
'duplicate': False,
'question': 'W którym państwie leży Bombaj?',
'relevant': True,
'annotated_by': 'Igor',
'answers': "['w Indiach', 'Indie']",
'question_formulation': 'QUESTION',
'question_type': 'SINGLE ENTITY',
'entity_type': 'NAMED',
'entity_subtype': 'COUNTRY',
'split': 'train',
'passage_source': 'human'
}
```
The second part of the dataset is a corpus of Polish Wikipedia (March 2022 snapshot) passages. The raw Wikipedia snapshot was parsed using [WikiExtractor](https://github.com/attardi/wikiextractor) and split into passages at the ends of the paragraphs or if the passage was longer than 500 characters.
```
{
'id': '42609-0',
'title': 'Mumbaj',
'text': 'Mumbaj lub Bombaj (mr. मुंबई, trb.: "Mumbaj"; ang. Mumbai; do 1995 Bombay) – stolica indyjskiego stanu Maharasztra, położona na wyspie Salsette, na Morzu Arabskim. Wraz z miastami satelitarnymi tworzy najludniejszą po Delhi aglomerację liczącą 23 miliony mieszkańców. Dzięki naturalnemu położeniu jest to największy port morski kraju. Znajdują się tutaj także najsilniejsze giełdy Azji Południowej: National Stock Exchange of India i Bombay Stock Exchange.'
}
```
### Data Fields
Question-passage pairs:
- `question_id`: an integer id of the question
- `passage_title`: a string containing the title of the Wikipedia article
- `passage_text`: a string containing the passage text as extracted by the human annotator
- `passage_wiki`: a string containing the passage text as it can be found in the provided Wikipedia corpus. Empty if the passage doesn't exist in the corpus.
- `passage_id`: a string containing the id of the passage from the provided Wikipedia corpus. Empty if the passage doesn't exist in the corpus.
- `duplicate`: a boolean flag representing whether a question-passage pair is duplicated in the dataset. This occurs when the same passage was found in multiple passage sources.
- `question`: a string containing the question
- `relevant`: a boolean flag representing whether a passage is relevant to the question (i.e. does it contain the answers)
- `annotated_by`: a string containing the name of the annotator who verified the relevance of the pair
- `answers`: a string containing a list of possible short answers to the question
- `question_formulation`: a string containing a kind of expression used to request information. One of the following:
- `QUESTION`, e.g. *What is the name of the first letter of the Greek alphabet?*
- `COMMAND`, e.g. *Expand the abbreviation ’CIA’.*
- `COMPOUND`, e.g. *This French writer, born in the 19th century, is
considered a pioneer of sci-fi literature. What is his name?*
- `question_type`: a string indicating what type of information is sought by the question. One of the following:
- `SINGLE ENTITY`, e.g. *Who is the hero in the Tomb Rider video game series?*
- `MULTIPLE ENTITIES`, e.g. *Which two seas are linked by the Corinth Canal?*
- `ENTITY CHOICE`, e.g. *Is "Sombrero" a type of dance, a hat, or a dish?*
- `YES/NO`, e.g. *When the term of office of the Polish Sejm is terminated, does it apply to the Senate as well?*
- `OTHER NAME`, e.g. *What was the nickname of Louis I, the King of the Franks?*
- `GAP FILLING`, e.g. *Finish the proverb: "If you fly with the crows... ".*
- `entity_type`: a string containing a type of the sought entity. One of the following: `NAMED`, `UNNAMED`, or `YES/NO`.
- `entity_subtype`: a string containing a subtype of the sought entity. Can take one of the 34 different values.
- `split`: a string containing the split of the dataset. One of the following: `train`, `valid`, or `test`.
- `passage_source`: a string containing the source of the passage. One of the following:
- `human`: the passage was proposed by a human annotator using any
internal (i.e. Wikipedia search) or external (e.g. Google) search engines and any keywords or queries they considered useful
- `hard-negatives`: the passage was proposed using a neural retriever trained on the passages found by the human annotators
- `zero-shot`: the passage was proposed by the BM25 retriever and re-ranked using [multilingual cross-encoder](https://huggingface.co/unicamp-dl/mMiniLM-L6-v2-mmarco-v2)
Corpus of passages:
- `id`: a string representing the Wikipedia article id and the index of extracted passage. Matches the `passage_id` from the main part of the dataset.
- `title`: a string containing the title of the Wikipedia article. Matches the `passage_title` from the main part of the dataset.
- `text`: a string containing the passage text. Matches the `passage_wiki` from the main part of the dataset.
### Data Splits
The questions are assigned into one of three splits: `train`, `validation`, and `test`. The `validation` and `test` questions are randomly sampled from the `test-B` dataset from the [PolEval 2021](https://2021.poleval.pl/tasks/task4) competition.
| | # questions | # positive passages | # negative passages |
|------------|------------:|--------------------:|--------------------:|
| train | 5,000 | 27,131 | 34,904 |
| validation | 1,000 | 5,839 | 6,927 |
| test | 1,000 | 5,938 | 6,786 |
## Dataset Creation
### Curation Rationale
The PolQA dataset was created to support and promote the research in the open-domain question answering for Polish. It also serves as a benchmark to evaluate OpenQA systems.
### Source Data
#### Initial Data Collection and Normalization
The majority of questions come from two existing resources, the
6,000 questions from the [PolEval 2021 shared task on QA](https://2021.poleval.pl/tasks/task4) and additional 1,000 questions gathered by one of the shared
task [participants](http://poleval.pl/files/poleval2021.pdf#page=151). Originally, the questions come from collections associated with TV shows, both officially published and gathered online by their fans, as well as questions used in actual quiz competitions, on TV or online.
The evidence passages come from the Polish Wikipedia (March 2022 snapshot). The raw Wikipedia snapshot was parsed using [WikiExtractor](https://github.com/attardi/wikiextractor) and split into passages at the ends of the paragraphs or if the passage was longer than 500 characters.
#### Who are the source language producers?
The questions come from various sources and their authors are unknown but are mostly analogous (or even identical) to questions asked during the [Jeden z Dziesięciu](https://pl.wikipedia.org/wiki/Jeden_z_dziesi%C4%99ciu) TV show.
The passages were written by the editors of the Polish Wikipedia.
### Annotations
#### Annotation process
Two approaches were used to annotate the question-passage pairs. Each of them consists of two phases: the retrieval of candidate passages and the manual verification of their relevance.
In the first approach, we asked annotators to use internal (i.e. Wikipedia search) or external (e.g. Google) search engines to find up to five relevant passages using any keywords or queries they consider useful (`passage_source="human"`). Based on those passages, we trained the neural retriever to extend the number of relevant passages, as well as to retrieve the hard negatives (`passage_source="hard-negatives"`).
In the second approach, the passage candidates were proposed by the BM25 retriever and re-ranked using [multilingual cross-encoder](https://huggingface.co/unicamp-dl/mMiniLM-L6-v2-mmarco-v2) (`passage_source="zero-shot"`).
In both cases, all proposed question-passage pairs were manually verified by the annotators.
#### Who are the annotators?
The annotation team consisted of 16 annotators, all native Polish
speakers, most of them having linguistic backgrounds and previous
experience as an annotator.
### Personal and Sensitive Information
The dataset does not contain any personal or sensitive information.
## Considerations for Using the Data
### Social Impact of Dataset
This dataset was created to promote the research in the open-domain question answering for Polish and allow developing question answering systems.
### Discussion of Biases
The passages proposed by the `hard-negative` and `zero-shot` methods are bound to be easier to retrieve by retrievers since they were proposed by such. To mitigate this bias, we include the passages found by the human annotators in an unconstrained way (`passage_source="human"`). We hypothesize that it will result in more unbiased and diverse examples. Moreover, we asked the annotators to find not one but up to five passages, preferably from different articles to even further increase passage diversity.
### Other Known Limitations
The PolQA dataset focuses on trivia questions which might limit its usefulness in real-world applications since neural retrievers generalize poorly to other domains.
## Additional Information
### Dataset Curators
The PolQA dataset was developed by Piotr Rybak, Piotr Przybyła, and Maciej Ogrodniczuk from the [Institute of Computer Science, Polish Academy of Sciences](http://zil.ipipan.waw.pl/).
This work was supported by the European Regional Development Fund as a part of 2014–2020 Smart Growth Operational Programme, CLARIN — Common Language Resources and Technology Infrastructure, project no. POIR.04.02.00-00C002/19.
### Licensing Information
CC BY-SA 4.0
### Citation Information
```
@misc{rybak2022improving,
title={Improving Question Answering Performance through Manual Annotation: Costs, Benefits and Strategies},
author={Piotr Rybak and Piotr Przybyła and Maciej Ogrodniczuk},
year={2022},
eprint={2212.08897},
archivePrefix={arXiv},
primaryClass={cs.CL}
}
``` | ipipan/polqa | [
"task_categories:question-answering",
"task_categories:text-retrieval",
"task_categories:text2text-generation",
"task_ids:open-domain-qa",
"task_ids:document-retrieval",
"task_ids:abstractive-qa",
"annotations_creators:expert-generated",
"size_categories:10K<n<100K",
"language:pl",
"license:cc-by-sa-4.0",
"arxiv:2212.08897",
"region:us"
] | 2022-12-17T15:03:58+00:00 | {"annotations_creators": ["expert-generated"], "language": ["pl"], "license": "cc-by-sa-4.0", "size_categories": ["10K<n<100K"], "task_categories": ["question-answering", "text-retrieval", "text2text-generation"], "task_ids": ["open-domain-qa", "document-retrieval", "abstractive-qa"], "pretty_name": "PolQA"} | 2023-09-09T12:37:44+00:00 | [
"2212.08897"
] | [
"pl"
] | TAGS
#task_categories-question-answering #task_categories-text-retrieval #task_categories-text2text-generation #task_ids-open-domain-qa #task_ids-document-retrieval #task_ids-abstractive-qa #annotations_creators-expert-generated #size_categories-10K<n<100K #language-Polish #license-cc-by-sa-4.0 #arxiv-2212.08897 #region-us
| Dataset Card for PolQA Dataset
==============================
Dataset Description
-------------------
* Paper: Improving Question Answering Performance through Manual Annotation: Costs, Benefits and Strategies
* Point of Contact: Piotr Rybak
### Dataset Summary
PolQA is the first Polish dataset for open-domain question answering. It consists of 7,000 questions, 87,525 manually labeled evidence passages, and a corpus of over 7 million candidate passages. The dataset can be used to train both a passage retriever and an abstractive reader.
### Supported Tasks and Leaderboards
* 'open-domain-qa': The dataset can be used to train a model for open-domain question answering. Success on this task is typically measured using metric defined during PolEval 2021.
* 'document-retrieval': The dataset can be used to train a model for document retrieval. Success on this task is typically measured by top-k retrieval accuracy or NDCG.
* 'abstractive-qa': The dataset can be used to train a model for abstractive question answering. Success on this task is typically measured using metric defined during PolEval 2021.
### Languages
The text is in Polish, as spoken by the host of the Jeden z Dziesięciu TV show (questions) and Polish Wikipedia editors (passages). The BCP-47 code for Polish is pl-PL.
Dataset Structure
-----------------
### Data Instances
The main part of the dataset consists of manually annotated question-passage pairs. For each instance, there is a 'question', a passage ('passage\_id', 'passage\_title', 'passage\_text'), and a boolean indicator if the passage is 'relevant' for the given question (i.e. does it contain the answers).
For each 'question' there is a list of possible 'answers' formulated in a natural language, in a way a Polish
speaker would answer the questions. It means that the answers might
contain prepositions, be inflected, and contain punctuation. In some
cases, the answer might have multiple correct variants, e.g. numbers
are written as numerals and words, synonyms, abbreviations and their
expansions.
Additionally, we provide a classification of each question-answer pair based on the 'question\_formulation', the 'question\_type', and the 'entity\_type/entity\_subtype', according to the taxonomy proposed by
Maciej Ogrodniczuk and Piotr Przybyła (2021).
The second part of the dataset is a corpus of Polish Wikipedia (March 2022 snapshot) passages. The raw Wikipedia snapshot was parsed using WikiExtractor and split into passages at the ends of the paragraphs or if the passage was longer than 500 characters.
### Data Fields
Question-passage pairs:
* 'question\_id': an integer id of the question
* 'passage\_title': a string containing the title of the Wikipedia article
* 'passage\_text': a string containing the passage text as extracted by the human annotator
* 'passage\_wiki': a string containing the passage text as it can be found in the provided Wikipedia corpus. Empty if the passage doesn't exist in the corpus.
* 'passage\_id': a string containing the id of the passage from the provided Wikipedia corpus. Empty if the passage doesn't exist in the corpus.
* 'duplicate': a boolean flag representing whether a question-passage pair is duplicated in the dataset. This occurs when the same passage was found in multiple passage sources.
* 'question': a string containing the question
* 'relevant': a boolean flag representing whether a passage is relevant to the question (i.e. does it contain the answers)
* 'annotated\_by': a string containing the name of the annotator who verified the relevance of the pair
* 'answers': a string containing a list of possible short answers to the question
* 'question\_formulation': a string containing a kind of expression used to request information. One of the following:
+ 'QUESTION', e.g. *What is the name of the first letter of the Greek alphabet?*
+ 'COMMAND', e.g. *Expand the abbreviation ’CIA’.*
+ 'COMPOUND', e.g. *This French writer, born in the 19th century, is
considered a pioneer of sci-fi literature. What is his name?*
* 'question\_type': a string indicating what type of information is sought by the question. One of the following:
+ 'SINGLE ENTITY', e.g. *Who is the hero in the Tomb Rider video game series?*
+ 'MULTIPLE ENTITIES', e.g. *Which two seas are linked by the Corinth Canal?*
+ 'ENTITY CHOICE', e.g. *Is "Sombrero" a type of dance, a hat, or a dish?*
+ 'YES/NO', e.g. *When the term of office of the Polish Sejm is terminated, does it apply to the Senate as well?*
+ 'OTHER NAME', e.g. *What was the nickname of Louis I, the King of the Franks?*
+ 'GAP FILLING', e.g. *Finish the proverb: "If you fly with the crows... ".*
* 'entity\_type': a string containing a type of the sought entity. One of the following: 'NAMED', 'UNNAMED', or 'YES/NO'.
* 'entity\_subtype': a string containing a subtype of the sought entity. Can take one of the 34 different values.
* 'split': a string containing the split of the dataset. One of the following: 'train', 'valid', or 'test'.
* 'passage\_source': a string containing the source of the passage. One of the following:
+ 'human': the passage was proposed by a human annotator using any
internal (i.e. Wikipedia search) or external (e.g. Google) search engines and any keywords or queries they considered useful
+ 'hard-negatives': the passage was proposed using a neural retriever trained on the passages found by the human annotators
+ 'zero-shot': the passage was proposed by the BM25 retriever and re-ranked using multilingual cross-encoder
Corpus of passages:
* 'id': a string representing the Wikipedia article id and the index of extracted passage. Matches the 'passage\_id' from the main part of the dataset.
* 'title': a string containing the title of the Wikipedia article. Matches the 'passage\_title' from the main part of the dataset.
* 'text': a string containing the passage text. Matches the 'passage\_wiki' from the main part of the dataset.
### Data Splits
The questions are assigned into one of three splits: 'train', 'validation', and 'test'. The 'validation' and 'test' questions are randomly sampled from the 'test-B' dataset from the PolEval 2021 competition.
Dataset Creation
----------------
### Curation Rationale
The PolQA dataset was created to support and promote the research in the open-domain question answering for Polish. It also serves as a benchmark to evaluate OpenQA systems.
### Source Data
#### Initial Data Collection and Normalization
The majority of questions come from two existing resources, the
6,000 questions from the PolEval 2021 shared task on QA and additional 1,000 questions gathered by one of the shared
task participants. Originally, the questions come from collections associated with TV shows, both officially published and gathered online by their fans, as well as questions used in actual quiz competitions, on TV or online.
The evidence passages come from the Polish Wikipedia (March 2022 snapshot). The raw Wikipedia snapshot was parsed using WikiExtractor and split into passages at the ends of the paragraphs or if the passage was longer than 500 characters.
#### Who are the source language producers?
The questions come from various sources and their authors are unknown but are mostly analogous (or even identical) to questions asked during the Jeden z Dziesięciu TV show.
The passages were written by the editors of the Polish Wikipedia.
### Annotations
#### Annotation process
Two approaches were used to annotate the question-passage pairs. Each of them consists of two phases: the retrieval of candidate passages and the manual verification of their relevance.
In the first approach, we asked annotators to use internal (i.e. Wikipedia search) or external (e.g. Google) search engines to find up to five relevant passages using any keywords or queries they consider useful ('passage\_source="human"'). Based on those passages, we trained the neural retriever to extend the number of relevant passages, as well as to retrieve the hard negatives ('passage\_source="hard-negatives"').
In the second approach, the passage candidates were proposed by the BM25 retriever and re-ranked using multilingual cross-encoder ('passage\_source="zero-shot"').
In both cases, all proposed question-passage pairs were manually verified by the annotators.
#### Who are the annotators?
The annotation team consisted of 16 annotators, all native Polish
speakers, most of them having linguistic backgrounds and previous
experience as an annotator.
### Personal and Sensitive Information
The dataset does not contain any personal or sensitive information.
Considerations for Using the Data
---------------------------------
### Social Impact of Dataset
This dataset was created to promote the research in the open-domain question answering for Polish and allow developing question answering systems.
### Discussion of Biases
The passages proposed by the 'hard-negative' and 'zero-shot' methods are bound to be easier to retrieve by retrievers since they were proposed by such. To mitigate this bias, we include the passages found by the human annotators in an unconstrained way ('passage\_source="human"'). We hypothesize that it will result in more unbiased and diverse examples. Moreover, we asked the annotators to find not one but up to five passages, preferably from different articles to even further increase passage diversity.
### Other Known Limitations
The PolQA dataset focuses on trivia questions which might limit its usefulness in real-world applications since neural retrievers generalize poorly to other domains.
Additional Information
----------------------
### Dataset Curators
The PolQA dataset was developed by Piotr Rybak, Piotr Przybyła, and Maciej Ogrodniczuk from the Institute of Computer Science, Polish Academy of Sciences.
This work was supported by the European Regional Development Fund as a part of 2014–2020 Smart Growth Operational Programme, CLARIN — Common Language Resources and Technology Infrastructure, project no. POIR.04.02.00-00C002/19.
### Licensing Information
CC BY-SA 4.0
| [
"### Dataset Summary\n\n\nPolQA is the first Polish dataset for open-domain question answering. It consists of 7,000 questions, 87,525 manually labeled evidence passages, and a corpus of over 7 million candidate passages. The dataset can be used to train both a passage retriever and an abstractive reader.",
"### Supported Tasks and Leaderboards\n\n\n* 'open-domain-qa': The dataset can be used to train a model for open-domain question answering. Success on this task is typically measured using metric defined during PolEval 2021.\n* 'document-retrieval': The dataset can be used to train a model for document retrieval. Success on this task is typically measured by top-k retrieval accuracy or NDCG.\n* 'abstractive-qa': The dataset can be used to train a model for abstractive question answering. Success on this task is typically measured using metric defined during PolEval 2021.",
"### Languages\n\n\nThe text is in Polish, as spoken by the host of the Jeden z Dziesięciu TV show (questions) and Polish Wikipedia editors (passages). The BCP-47 code for Polish is pl-PL.\n\n\nDataset Structure\n-----------------",
"### Data Instances\n\n\nThe main part of the dataset consists of manually annotated question-passage pairs. For each instance, there is a 'question', a passage ('passage\\_id', 'passage\\_title', 'passage\\_text'), and a boolean indicator if the passage is 'relevant' for the given question (i.e. does it contain the answers).\n\n\nFor each 'question' there is a list of possible 'answers' formulated in a natural language, in a way a Polish\nspeaker would answer the questions. It means that the answers might\ncontain prepositions, be inflected, and contain punctuation. In some\ncases, the answer might have multiple correct variants, e.g. numbers\nare written as numerals and words, synonyms, abbreviations and their\nexpansions.\n\n\nAdditionally, we provide a classification of each question-answer pair based on the 'question\\_formulation', the 'question\\_type', and the 'entity\\_type/entity\\_subtype', according to the taxonomy proposed by\nMaciej Ogrodniczuk and Piotr Przybyła (2021).\n\n\nThe second part of the dataset is a corpus of Polish Wikipedia (March 2022 snapshot) passages. The raw Wikipedia snapshot was parsed using WikiExtractor and split into passages at the ends of the paragraphs or if the passage was longer than 500 characters.",
"### Data Fields\n\n\nQuestion-passage pairs:\n\n\n* 'question\\_id': an integer id of the question\n* 'passage\\_title': a string containing the title of the Wikipedia article\n* 'passage\\_text': a string containing the passage text as extracted by the human annotator\n* 'passage\\_wiki': a string containing the passage text as it can be found in the provided Wikipedia corpus. Empty if the passage doesn't exist in the corpus.\n* 'passage\\_id': a string containing the id of the passage from the provided Wikipedia corpus. Empty if the passage doesn't exist in the corpus.\n* 'duplicate': a boolean flag representing whether a question-passage pair is duplicated in the dataset. This occurs when the same passage was found in multiple passage sources.\n* 'question': a string containing the question\n* 'relevant': a boolean flag representing whether a passage is relevant to the question (i.e. does it contain the answers)\n* 'annotated\\_by': a string containing the name of the annotator who verified the relevance of the pair\n* 'answers': a string containing a list of possible short answers to the question\n* 'question\\_formulation': a string containing a kind of expression used to request information. One of the following:\n\t+ 'QUESTION', e.g. *What is the name of the first letter of the Greek alphabet?*\n\t+ 'COMMAND', e.g. *Expand the abbreviation ’CIA’.*\n\t+ 'COMPOUND', e.g. *This French writer, born in the 19th century, is\n\tconsidered a pioneer of sci-fi literature. What is his name?*\n* 'question\\_type': a string indicating what type of information is sought by the question. One of the following:\n\t+ 'SINGLE ENTITY', e.g. *Who is the hero in the Tomb Rider video game series?*\n\t+ 'MULTIPLE ENTITIES', e.g. *Which two seas are linked by the Corinth Canal?*\n\t+ 'ENTITY CHOICE', e.g. *Is \"Sombrero\" a type of dance, a hat, or a dish?*\n\t+ 'YES/NO', e.g. *When the term of office of the Polish Sejm is terminated, does it apply to the Senate as well?*\n\t+ 'OTHER NAME', e.g. *What was the nickname of Louis I, the King of the Franks?*\n\t+ 'GAP FILLING', e.g. *Finish the proverb: \"If you fly with the crows... \".*\n* 'entity\\_type': a string containing a type of the sought entity. One of the following: 'NAMED', 'UNNAMED', or 'YES/NO'.\n* 'entity\\_subtype': a string containing a subtype of the sought entity. Can take one of the 34 different values.\n* 'split': a string containing the split of the dataset. One of the following: 'train', 'valid', or 'test'.\n* 'passage\\_source': a string containing the source of the passage. One of the following:\n\t+ 'human': the passage was proposed by a human annotator using any\n\tinternal (i.e. Wikipedia search) or external (e.g. Google) search engines and any keywords or queries they considered useful\n\t+ 'hard-negatives': the passage was proposed using a neural retriever trained on the passages found by the human annotators\n\t+ 'zero-shot': the passage was proposed by the BM25 retriever and re-ranked using multilingual cross-encoder\n\n\nCorpus of passages:\n\n\n* 'id': a string representing the Wikipedia article id and the index of extracted passage. Matches the 'passage\\_id' from the main part of the dataset.\n* 'title': a string containing the title of the Wikipedia article. Matches the 'passage\\_title' from the main part of the dataset.\n* 'text': a string containing the passage text. Matches the 'passage\\_wiki' from the main part of the dataset.",
"### Data Splits\n\n\nThe questions are assigned into one of three splits: 'train', 'validation', and 'test'. The 'validation' and 'test' questions are randomly sampled from the 'test-B' dataset from the PolEval 2021 competition.\n\n\n\nDataset Creation\n----------------",
"### Curation Rationale\n\n\nThe PolQA dataset was created to support and promote the research in the open-domain question answering for Polish. It also serves as a benchmark to evaluate OpenQA systems.",
"### Source Data",
"#### Initial Data Collection and Normalization\n\n\nThe majority of questions come from two existing resources, the\n6,000 questions from the PolEval 2021 shared task on QA and additional 1,000 questions gathered by one of the shared\ntask participants. Originally, the questions come from collections associated with TV shows, both officially published and gathered online by their fans, as well as questions used in actual quiz competitions, on TV or online.\n\n\nThe evidence passages come from the Polish Wikipedia (March 2022 snapshot). The raw Wikipedia snapshot was parsed using WikiExtractor and split into passages at the ends of the paragraphs or if the passage was longer than 500 characters.",
"#### Who are the source language producers?\n\n\nThe questions come from various sources and their authors are unknown but are mostly analogous (or even identical) to questions asked during the Jeden z Dziesięciu TV show.\n\n\nThe passages were written by the editors of the Polish Wikipedia.",
"### Annotations",
"#### Annotation process\n\n\nTwo approaches were used to annotate the question-passage pairs. Each of them consists of two phases: the retrieval of candidate passages and the manual verification of their relevance.\n\n\nIn the first approach, we asked annotators to use internal (i.e. Wikipedia search) or external (e.g. Google) search engines to find up to five relevant passages using any keywords or queries they consider useful ('passage\\_source=\"human\"'). Based on those passages, we trained the neural retriever to extend the number of relevant passages, as well as to retrieve the hard negatives ('passage\\_source=\"hard-negatives\"').\n\n\nIn the second approach, the passage candidates were proposed by the BM25 retriever and re-ranked using multilingual cross-encoder ('passage\\_source=\"zero-shot\"').\n\n\nIn both cases, all proposed question-passage pairs were manually verified by the annotators.",
"#### Who are the annotators?\n\n\nThe annotation team consisted of 16 annotators, all native Polish\nspeakers, most of them having linguistic backgrounds and previous\nexperience as an annotator.",
"### Personal and Sensitive Information\n\n\nThe dataset does not contain any personal or sensitive information.\n\n\nConsiderations for Using the Data\n---------------------------------",
"### Social Impact of Dataset\n\n\nThis dataset was created to promote the research in the open-domain question answering for Polish and allow developing question answering systems.",
"### Discussion of Biases\n\n\nThe passages proposed by the 'hard-negative' and 'zero-shot' methods are bound to be easier to retrieve by retrievers since they were proposed by such. To mitigate this bias, we include the passages found by the human annotators in an unconstrained way ('passage\\_source=\"human\"'). We hypothesize that it will result in more unbiased and diverse examples. Moreover, we asked the annotators to find not one but up to five passages, preferably from different articles to even further increase passage diversity.",
"### Other Known Limitations\n\n\nThe PolQA dataset focuses on trivia questions which might limit its usefulness in real-world applications since neural retrievers generalize poorly to other domains.\n\n\nAdditional Information\n----------------------",
"### Dataset Curators\n\n\nThe PolQA dataset was developed by Piotr Rybak, Piotr Przybyła, and Maciej Ogrodniczuk from the Institute of Computer Science, Polish Academy of Sciences.\n\n\nThis work was supported by the European Regional Development Fund as a part of 2014–2020 Smart Growth Operational Programme, CLARIN — Common Language Resources and Technology Infrastructure, project no. POIR.04.02.00-00C002/19.",
"### Licensing Information\n\n\nCC BY-SA 4.0"
] | [
"TAGS\n#task_categories-question-answering #task_categories-text-retrieval #task_categories-text2text-generation #task_ids-open-domain-qa #task_ids-document-retrieval #task_ids-abstractive-qa #annotations_creators-expert-generated #size_categories-10K<n<100K #language-Polish #license-cc-by-sa-4.0 #arxiv-2212.08897 #region-us \n",
"### Dataset Summary\n\n\nPolQA is the first Polish dataset for open-domain question answering. It consists of 7,000 questions, 87,525 manually labeled evidence passages, and a corpus of over 7 million candidate passages. The dataset can be used to train both a passage retriever and an abstractive reader.",
"### Supported Tasks and Leaderboards\n\n\n* 'open-domain-qa': The dataset can be used to train a model for open-domain question answering. Success on this task is typically measured using metric defined during PolEval 2021.\n* 'document-retrieval': The dataset can be used to train a model for document retrieval. Success on this task is typically measured by top-k retrieval accuracy or NDCG.\n* 'abstractive-qa': The dataset can be used to train a model for abstractive question answering. Success on this task is typically measured using metric defined during PolEval 2021.",
"### Languages\n\n\nThe text is in Polish, as spoken by the host of the Jeden z Dziesięciu TV show (questions) and Polish Wikipedia editors (passages). The BCP-47 code for Polish is pl-PL.\n\n\nDataset Structure\n-----------------",
"### Data Instances\n\n\nThe main part of the dataset consists of manually annotated question-passage pairs. For each instance, there is a 'question', a passage ('passage\\_id', 'passage\\_title', 'passage\\_text'), and a boolean indicator if the passage is 'relevant' for the given question (i.e. does it contain the answers).\n\n\nFor each 'question' there is a list of possible 'answers' formulated in a natural language, in a way a Polish\nspeaker would answer the questions. It means that the answers might\ncontain prepositions, be inflected, and contain punctuation. In some\ncases, the answer might have multiple correct variants, e.g. numbers\nare written as numerals and words, synonyms, abbreviations and their\nexpansions.\n\n\nAdditionally, we provide a classification of each question-answer pair based on the 'question\\_formulation', the 'question\\_type', and the 'entity\\_type/entity\\_subtype', according to the taxonomy proposed by\nMaciej Ogrodniczuk and Piotr Przybyła (2021).\n\n\nThe second part of the dataset is a corpus of Polish Wikipedia (March 2022 snapshot) passages. The raw Wikipedia snapshot was parsed using WikiExtractor and split into passages at the ends of the paragraphs or if the passage was longer than 500 characters.",
"### Data Fields\n\n\nQuestion-passage pairs:\n\n\n* 'question\\_id': an integer id of the question\n* 'passage\\_title': a string containing the title of the Wikipedia article\n* 'passage\\_text': a string containing the passage text as extracted by the human annotator\n* 'passage\\_wiki': a string containing the passage text as it can be found in the provided Wikipedia corpus. Empty if the passage doesn't exist in the corpus.\n* 'passage\\_id': a string containing the id of the passage from the provided Wikipedia corpus. Empty if the passage doesn't exist in the corpus.\n* 'duplicate': a boolean flag representing whether a question-passage pair is duplicated in the dataset. This occurs when the same passage was found in multiple passage sources.\n* 'question': a string containing the question\n* 'relevant': a boolean flag representing whether a passage is relevant to the question (i.e. does it contain the answers)\n* 'annotated\\_by': a string containing the name of the annotator who verified the relevance of the pair\n* 'answers': a string containing a list of possible short answers to the question\n* 'question\\_formulation': a string containing a kind of expression used to request information. One of the following:\n\t+ 'QUESTION', e.g. *What is the name of the first letter of the Greek alphabet?*\n\t+ 'COMMAND', e.g. *Expand the abbreviation ’CIA’.*\n\t+ 'COMPOUND', e.g. *This French writer, born in the 19th century, is\n\tconsidered a pioneer of sci-fi literature. What is his name?*\n* 'question\\_type': a string indicating what type of information is sought by the question. One of the following:\n\t+ 'SINGLE ENTITY', e.g. *Who is the hero in the Tomb Rider video game series?*\n\t+ 'MULTIPLE ENTITIES', e.g. *Which two seas are linked by the Corinth Canal?*\n\t+ 'ENTITY CHOICE', e.g. *Is \"Sombrero\" a type of dance, a hat, or a dish?*\n\t+ 'YES/NO', e.g. *When the term of office of the Polish Sejm is terminated, does it apply to the Senate as well?*\n\t+ 'OTHER NAME', e.g. *What was the nickname of Louis I, the King of the Franks?*\n\t+ 'GAP FILLING', e.g. *Finish the proverb: \"If you fly with the crows... \".*\n* 'entity\\_type': a string containing a type of the sought entity. One of the following: 'NAMED', 'UNNAMED', or 'YES/NO'.\n* 'entity\\_subtype': a string containing a subtype of the sought entity. Can take one of the 34 different values.\n* 'split': a string containing the split of the dataset. One of the following: 'train', 'valid', or 'test'.\n* 'passage\\_source': a string containing the source of the passage. One of the following:\n\t+ 'human': the passage was proposed by a human annotator using any\n\tinternal (i.e. Wikipedia search) or external (e.g. Google) search engines and any keywords or queries they considered useful\n\t+ 'hard-negatives': the passage was proposed using a neural retriever trained on the passages found by the human annotators\n\t+ 'zero-shot': the passage was proposed by the BM25 retriever and re-ranked using multilingual cross-encoder\n\n\nCorpus of passages:\n\n\n* 'id': a string representing the Wikipedia article id and the index of extracted passage. Matches the 'passage\\_id' from the main part of the dataset.\n* 'title': a string containing the title of the Wikipedia article. Matches the 'passage\\_title' from the main part of the dataset.\n* 'text': a string containing the passage text. Matches the 'passage\\_wiki' from the main part of the dataset.",
"### Data Splits\n\n\nThe questions are assigned into one of three splits: 'train', 'validation', and 'test'. The 'validation' and 'test' questions are randomly sampled from the 'test-B' dataset from the PolEval 2021 competition.\n\n\n\nDataset Creation\n----------------",
"### Curation Rationale\n\n\nThe PolQA dataset was created to support and promote the research in the open-domain question answering for Polish. It also serves as a benchmark to evaluate OpenQA systems.",
"### Source Data",
"#### Initial Data Collection and Normalization\n\n\nThe majority of questions come from two existing resources, the\n6,000 questions from the PolEval 2021 shared task on QA and additional 1,000 questions gathered by one of the shared\ntask participants. Originally, the questions come from collections associated with TV shows, both officially published and gathered online by their fans, as well as questions used in actual quiz competitions, on TV or online.\n\n\nThe evidence passages come from the Polish Wikipedia (March 2022 snapshot). The raw Wikipedia snapshot was parsed using WikiExtractor and split into passages at the ends of the paragraphs or if the passage was longer than 500 characters.",
"#### Who are the source language producers?\n\n\nThe questions come from various sources and their authors are unknown but are mostly analogous (or even identical) to questions asked during the Jeden z Dziesięciu TV show.\n\n\nThe passages were written by the editors of the Polish Wikipedia.",
"### Annotations",
"#### Annotation process\n\n\nTwo approaches were used to annotate the question-passage pairs. Each of them consists of two phases: the retrieval of candidate passages and the manual verification of their relevance.\n\n\nIn the first approach, we asked annotators to use internal (i.e. Wikipedia search) or external (e.g. Google) search engines to find up to five relevant passages using any keywords or queries they consider useful ('passage\\_source=\"human\"'). Based on those passages, we trained the neural retriever to extend the number of relevant passages, as well as to retrieve the hard negatives ('passage\\_source=\"hard-negatives\"').\n\n\nIn the second approach, the passage candidates were proposed by the BM25 retriever and re-ranked using multilingual cross-encoder ('passage\\_source=\"zero-shot\"').\n\n\nIn both cases, all proposed question-passage pairs were manually verified by the annotators.",
"#### Who are the annotators?\n\n\nThe annotation team consisted of 16 annotators, all native Polish\nspeakers, most of them having linguistic backgrounds and previous\nexperience as an annotator.",
"### Personal and Sensitive Information\n\n\nThe dataset does not contain any personal or sensitive information.\n\n\nConsiderations for Using the Data\n---------------------------------",
"### Social Impact of Dataset\n\n\nThis dataset was created to promote the research in the open-domain question answering for Polish and allow developing question answering systems.",
"### Discussion of Biases\n\n\nThe passages proposed by the 'hard-negative' and 'zero-shot' methods are bound to be easier to retrieve by retrievers since they were proposed by such. To mitigate this bias, we include the passages found by the human annotators in an unconstrained way ('passage\\_source=\"human\"'). We hypothesize that it will result in more unbiased and diverse examples. Moreover, we asked the annotators to find not one but up to five passages, preferably from different articles to even further increase passage diversity.",
"### Other Known Limitations\n\n\nThe PolQA dataset focuses on trivia questions which might limit its usefulness in real-world applications since neural retrievers generalize poorly to other domains.\n\n\nAdditional Information\n----------------------",
"### Dataset Curators\n\n\nThe PolQA dataset was developed by Piotr Rybak, Piotr Przybyła, and Maciej Ogrodniczuk from the Institute of Computer Science, Polish Academy of Sciences.\n\n\nThis work was supported by the European Regional Development Fund as a part of 2014–2020 Smart Growth Operational Programme, CLARIN — Common Language Resources and Technology Infrastructure, project no. POIR.04.02.00-00C002/19.",
"### Licensing Information\n\n\nCC BY-SA 4.0"
] |
c671fd20220e568e25b7b65c723daf9eddfdf677 |
# Doc2Query ELECTRA Relevance Scores for `msmarco-passage`
This dataset provides the pre-computed query relevance scores for the [`msmarco-passage`](https://ir-datasets.com/msmarco-passage) dataset,
for use with Doc2Query--.
The generated queries come from [`macavaney/d2q-msmarco-passage`](https://huggingface.co/datasets/macavaney/d2q-msmarco-passage) and
were scored with [`crystina-z/monoELECTRA_LCE_nneg31`](https://huggingface.co/crystina-z/monoELECTRA_LCE_nneg31).
## Getting started
This artefact is meant to be used with the [`pyterrier_doc2query`](https://github.com/terrierteam/pyterrier_doc2query) pacakge. It can
be installed as:
```bash
pip install git+https://github.com/terrierteam/pyterrier_doc2query
```
Depending on what you are using this aretefact for, you may also need the following additional packages:
```bash
pip install git+https://github.com/terrierteam/pyterrier_pisa # for indexing / retrieval
pip install git+https://github.com/terrierteam/pyterrier_dr # for reproducing this aretefact
```
## Using this artefact
The main use case is to use this aretefact in a Doc2Query−− indexing pipeline:
```python
import pyterrier as pt ; pt.init()
from pyterrier_pisa import PisaIndex
from pyterrier_doc2query import QueryScoreStore, QueryFilter
store = QueryScoreStore.from_repo('https://huggingface.co/datasets/macavaney/d2q-msmarco-passage-scores-electra')
index = PisaIndex('path/to/index')
pipeline = store.query_scorer(limit_k=40) >> QueryFilter(t=store.percentile(70)) >> index
dataset = pt.get_dataset('irds:msmarco-passage')
pipeline.index(dataset.get_corpus_iter())
```
You can also use the store directly as a dataset to look up or iterate over the data:
```python
store.lookup('100')
# {'querygen': ..., 'querygen_store': ...}
for record in store:
pass
```
## Reproducing this aretefact
This aretefact can be reproduced using the following pipeline:
```python
import pyterrier as pt ; pt.init()
from pyterrier_dr import ElectraScorer
from pyterrier_doc2query import Doc2QueryStore, QueryScoreStore, QueryScorer
doc2query_generator = Doc2QueryStore.from_repo('https://huggingface.co/datasets/macavaney/d2q-msmarco-passage').generator()
store = QueryScoreStore('path/to/store')
pipeline = doc2query_generator >> QueryScorer(ElectraScorer()) >> store
dataset = pt.get_dataset('irds:msmarco-passage')
pipeline.index(dataset.get_corpus_iter())
```
Note that this process will take quite some time; it computes the relevance score for 80 generated queries
for every document in the dataset.
| macavaney/d2q-msmarco-passage-scores-electra | [
"task_categories:text-retrieval",
"task_ids:document-retrieval",
"annotations_creators:no-annotation",
"language_creators:machine-generated",
"source_datasets:msmarco-passage",
"document-expansion",
"doc2query--",
"region:us"
] | 2022-12-17T15:18:38+00:00 | {"annotations_creators": ["no-annotation"], "language_creators": ["machine-generated"], "language": [], "license": [], "source_datasets": ["msmarco-passage"], "task_categories": ["text-retrieval"], "task_ids": ["document-retrieval"], "pretty_name": "Doc2Query ELECTRA Relevance Scores for `msmarco-passage`", "tags": ["document-expansion", "doc2query--"], "viewer": false} | 2022-12-18T20:12:10+00:00 | [] | [] | TAGS
#task_categories-text-retrieval #task_ids-document-retrieval #annotations_creators-no-annotation #language_creators-machine-generated #source_datasets-msmarco-passage #document-expansion #doc2query-- #region-us
|
# Doc2Query ELECTRA Relevance Scores for 'msmarco-passage'
This dataset provides the pre-computed query relevance scores for the 'msmarco-passage' dataset,
for use with Doc2Query--.
The generated queries come from 'macavaney/d2q-msmarco-passage' and
were scored with 'crystina-z/monoELECTRA_LCE_nneg31'.
## Getting started
This artefact is meant to be used with the 'pyterrier_doc2query' pacakge. It can
be installed as:
Depending on what you are using this aretefact for, you may also need the following additional packages:
## Using this artefact
The main use case is to use this aretefact in a Doc2Query−− indexing pipeline:
You can also use the store directly as a dataset to look up or iterate over the data:
## Reproducing this aretefact
This aretefact can be reproduced using the following pipeline:
Note that this process will take quite some time; it computes the relevance score for 80 generated queries
for every document in the dataset.
| [
"# Doc2Query ELECTRA Relevance Scores for 'msmarco-passage'\n\nThis dataset provides the pre-computed query relevance scores for the 'msmarco-passage' dataset,\nfor use with Doc2Query--.\n\nThe generated queries come from 'macavaney/d2q-msmarco-passage' and\nwere scored with 'crystina-z/monoELECTRA_LCE_nneg31'.",
"## Getting started\n\nThis artefact is meant to be used with the 'pyterrier_doc2query' pacakge. It can\nbe installed as:\n\n\n\nDepending on what you are using this aretefact for, you may also need the following additional packages:",
"## Using this artefact\n\nThe main use case is to use this aretefact in a Doc2Query−− indexing pipeline:\n\n\n\nYou can also use the store directly as a dataset to look up or iterate over the data:",
"## Reproducing this aretefact\n\nThis aretefact can be reproduced using the following pipeline:\n\n\n\nNote that this process will take quite some time; it computes the relevance score for 80 generated queries\nfor every document in the dataset."
] | [
"TAGS\n#task_categories-text-retrieval #task_ids-document-retrieval #annotations_creators-no-annotation #language_creators-machine-generated #source_datasets-msmarco-passage #document-expansion #doc2query-- #region-us \n",
"# Doc2Query ELECTRA Relevance Scores for 'msmarco-passage'\n\nThis dataset provides the pre-computed query relevance scores for the 'msmarco-passage' dataset,\nfor use with Doc2Query--.\n\nThe generated queries come from 'macavaney/d2q-msmarco-passage' and\nwere scored with 'crystina-z/monoELECTRA_LCE_nneg31'.",
"## Getting started\n\nThis artefact is meant to be used with the 'pyterrier_doc2query' pacakge. It can\nbe installed as:\n\n\n\nDepending on what you are using this aretefact for, you may also need the following additional packages:",
"## Using this artefact\n\nThe main use case is to use this aretefact in a Doc2Query−− indexing pipeline:\n\n\n\nYou can also use the store directly as a dataset to look up or iterate over the data:",
"## Reproducing this aretefact\n\nThis aretefact can be reproduced using the following pipeline:\n\n\n\nNote that this process will take quite some time; it computes the relevance score for 80 generated queries\nfor every document in the dataset."
] |
a257ab4fcfa09707711f7453885105230f634998 |
# Doc2Query monoT5 Relevance Scores for `msmarco-passage`
This dataset provides the pre-computed query relevance scores for the [`msmarco-passage`](https://ir-datasets.com/msmarco-passage) dataset,
for use with Doc2Query--.
The generated queries come from [`macavaney/d2q-msmarco-passage`](https://huggingface.co/datasets/macavaney/d2q-msmarco-passage) and
were scored with [`castorini/monot5-base-msmarco`](https://huggingface.co/castorini/monot5-base-msmarco).
## Getting started
This artefact is meant to be used with the [`pyterrier_doc2query`](https://github.com/terrierteam/pyterrier_doc2query) pacakge. It can
be installed as:
```bash
pip install git+https://github.com/terrierteam/pyterrier_doc2query
```
Depending on what you are using this aretefact for, you may also need the following additional packages:
```bash
pip install git+https://github.com/terrierteam/pyterrier_pisa # for indexing / retrieval
pip install git+https://github.com/terrierteam/pyterrier_t5 # for reproducing this aretefact
```
## Using this artefact
The main use case is to use this aretefact in a Doc2Query−− indexing pipeline:
```python
import pyterrier as pt ; pt.init()
from pyterrier_pisa import PisaIndex
from pyterrier_doc2query import QueryScoreStore, QueryFilter
store = QueryScoreStore.from_repo('https://huggingface.co/datasets/macavaney/d2q-msmarco-passage-scores-monot5')
index = PisaIndex('path/to/index')
pipeline = store.query_scorer(limit_k=40) >> QueryFilter(t=store.percentile(70)) >> index
dataset = pt.get_dataset('irds:msmarco-passage')
pipeline.index(dataset.get_corpus_iter())
```
You can also use the store directly as a dataset to look up or iterate over the data:
```python
store.lookup('100')
# {'querygen': ..., 'querygen_store': ...}
for record in store:
pass
```
## Reproducing this aretefact
This aretefact can be reproduced using the following pipeline:
```python
import pyterrier as pt ; pt.init()
from pyterrier_t5 import MonoT5ReRanker
from pyterrier_doc2query import Doc2QueryStore, QueryScoreStore, QueryScorer
doc2query_generator = Doc2QueryStore.from_repo('https://huggingface.co/datasets/macavaney/d2q-msmarco-passage').generator()
store = QueryScoreStore('path/to/store')
pipeline = doc2query_generator >> QueryScorer(MonoT5ReRanker()) >> store
dataset = pt.get_dataset('irds:msmarco-passage')
pipeline.index(dataset.get_corpus_iter())
```
Note that this process will take quite some time; it computes the relevance score for 80 generated queries
for every document in the dataset.
| macavaney/d2q-msmarco-passage-scores-monot5 | [
"task_categories:text-retrieval",
"task_ids:document-retrieval",
"annotations_creators:no-annotation",
"language_creators:machine-generated",
"source_datasets:msmarco-passage",
"document-expansion",
"doc2query--",
"region:us"
] | 2022-12-17T15:19:01+00:00 | {"annotations_creators": ["no-annotation"], "language_creators": ["machine-generated"], "language": [], "license": [], "source_datasets": ["msmarco-passage"], "task_categories": ["text-retrieval"], "task_ids": ["document-retrieval"], "pretty_name": "Doc2Query monoT5 Relevance Scores for `msmarco-passage`", "tags": ["document-expansion", "doc2query--"], "viewer": false} | 2022-12-18T20:13:58+00:00 | [] | [] | TAGS
#task_categories-text-retrieval #task_ids-document-retrieval #annotations_creators-no-annotation #language_creators-machine-generated #source_datasets-msmarco-passage #document-expansion #doc2query-- #region-us
|
# Doc2Query monoT5 Relevance Scores for 'msmarco-passage'
This dataset provides the pre-computed query relevance scores for the 'msmarco-passage' dataset,
for use with Doc2Query--.
The generated queries come from 'macavaney/d2q-msmarco-passage' and
were scored with 'castorini/monot5-base-msmarco'.
## Getting started
This artefact is meant to be used with the 'pyterrier_doc2query' pacakge. It can
be installed as:
Depending on what you are using this aretefact for, you may also need the following additional packages:
## Using this artefact
The main use case is to use this aretefact in a Doc2Query−− indexing pipeline:
You can also use the store directly as a dataset to look up or iterate over the data:
## Reproducing this aretefact
This aretefact can be reproduced using the following pipeline:
Note that this process will take quite some time; it computes the relevance score for 80 generated queries
for every document in the dataset.
| [
"# Doc2Query monoT5 Relevance Scores for 'msmarco-passage'\n\nThis dataset provides the pre-computed query relevance scores for the 'msmarco-passage' dataset,\nfor use with Doc2Query--.\n\nThe generated queries come from 'macavaney/d2q-msmarco-passage' and\nwere scored with 'castorini/monot5-base-msmarco'.",
"## Getting started\n\nThis artefact is meant to be used with the 'pyterrier_doc2query' pacakge. It can\nbe installed as:\n\n\n\nDepending on what you are using this aretefact for, you may also need the following additional packages:",
"## Using this artefact\n\nThe main use case is to use this aretefact in a Doc2Query−− indexing pipeline:\n\n\n\nYou can also use the store directly as a dataset to look up or iterate over the data:",
"## Reproducing this aretefact\n\nThis aretefact can be reproduced using the following pipeline:\n\n\n\nNote that this process will take quite some time; it computes the relevance score for 80 generated queries\nfor every document in the dataset."
] | [
"TAGS\n#task_categories-text-retrieval #task_ids-document-retrieval #annotations_creators-no-annotation #language_creators-machine-generated #source_datasets-msmarco-passage #document-expansion #doc2query-- #region-us \n",
"# Doc2Query monoT5 Relevance Scores for 'msmarco-passage'\n\nThis dataset provides the pre-computed query relevance scores for the 'msmarco-passage' dataset,\nfor use with Doc2Query--.\n\nThe generated queries come from 'macavaney/d2q-msmarco-passage' and\nwere scored with 'castorini/monot5-base-msmarco'.",
"## Getting started\n\nThis artefact is meant to be used with the 'pyterrier_doc2query' pacakge. It can\nbe installed as:\n\n\n\nDepending on what you are using this aretefact for, you may also need the following additional packages:",
"## Using this artefact\n\nThe main use case is to use this aretefact in a Doc2Query−− indexing pipeline:\n\n\n\nYou can also use the store directly as a dataset to look up or iterate over the data:",
"## Reproducing this aretefact\n\nThis aretefact can be reproduced using the following pipeline:\n\n\n\nNote that this process will take quite some time; it computes the relevance score for 80 generated queries\nfor every document in the dataset."
] |
9d19c8e11a1715ad1ec70122561bad5488eec68e |
# Doc2Query TCT Relevance Scores for `msmarco-passage`
This dataset provides the pre-computed query relevance scores for the [`msmarco-passage`](https://ir-datasets.com/msmarco-passage) dataset,
for use with Doc2Query--.
The generated queries come from [`macavaney/d2q-msmarco-passage`](https://huggingface.co/datasets/macavaney/d2q-msmarco-passage) and
were scored with [`castorini/tct_colbert-v2-hnp-msmarco`](https://huggingface.co/castorini/tct_colbert-v2-hnp-msmarco).
## Getting started
This artefact is meant to be used with the [`pyterrier_doc2query`](https://github.com/terrierteam/pyterrier_doc2query) pacakge. It can
be installed as:
```bash
pip install git+https://github.com/terrierteam/pyterrier_doc2query
```
Depending on what you are using this aretefact for, you may also need the following additional packages:
```bash
pip install git+https://github.com/terrierteam/pyterrier_pisa # for indexing / retrieval
pip install git+https://github.com/terrierteam/pyterrier_dr # for reproducing this aretefact
```
## Using this artefact
The main use case is to use this aretefact in a Doc2Query−− indexing pipeline:
```python
import pyterrier as pt ; pt.init()
from pyterrier_pisa import PisaIndex
from pyterrier_doc2query import QueryScoreStore, QueryFilter
store = QueryScoreStore.from_repo('https://huggingface.co/datasets/macavaney/d2q-msmarco-passage-scores-tct')
index = PisaIndex('path/to/index')
pipeline = store.query_scorer(limit_k=40) >> QueryFilter(t=store.percentile(70)) >> index
dataset = pt.get_dataset('irds:msmarco-passage')
pipeline.index(dataset.get_corpus_iter())
```
You can also use the store directly as a dataset to look up or iterate over the data:
```python
store.lookup('100')
# {'querygen': ..., 'querygen_store': ...}
for record in store:
pass
```
## Reproducing this aretefact
This aretefact can be reproduced using the following pipeline:
```python
import pyterrier as pt ; pt.init()
from pyterrier_dr import TctColBert
from pyterrier_doc2query import Doc2QueryStore, QueryScoreStore, QueryScorer
doc2query_generator = Doc2QueryStore.from_repo('https://huggingface.co/datasets/macavaney/d2q-msmarco-passage').generator()
store = QueryScoreStore('path/to/store')
pipeline = doc2query_generator >> QueryScorer(TctColBert('castorini/tct_colbert-v2-hnp-msmarco')) >> store
dataset = pt.get_dataset('irds:msmarco-passage')
pipeline.index(dataset.get_corpus_iter())
```
Note that this process will take quite some time; it computes the relevance score for 80 generated queries
for every document in the dataset.
| macavaney/d2q-msmarco-passage-scores-tct | [
"task_categories:text-retrieval",
"task_ids:document-retrieval",
"annotations_creators:no-annotation",
"language_creators:machine-generated",
"source_datasets:msmarco-passage",
"document-expansion",
"doc2query--",
"region:us"
] | 2022-12-17T15:19:11+00:00 | {"annotations_creators": ["no-annotation"], "language_creators": ["machine-generated"], "language": [], "license": [], "source_datasets": ["msmarco-passage"], "task_categories": ["text-retrieval"], "task_ids": ["document-retrieval"], "pretty_name": "Doc2Query TCT Relevance Scores for `msmarco-passage`", "tags": ["document-expansion", "doc2query--"], "viewer": false} | 2022-12-18T20:13:32+00:00 | [] | [] | TAGS
#task_categories-text-retrieval #task_ids-document-retrieval #annotations_creators-no-annotation #language_creators-machine-generated #source_datasets-msmarco-passage #document-expansion #doc2query-- #region-us
|
# Doc2Query TCT Relevance Scores for 'msmarco-passage'
This dataset provides the pre-computed query relevance scores for the 'msmarco-passage' dataset,
for use with Doc2Query--.
The generated queries come from 'macavaney/d2q-msmarco-passage' and
were scored with 'castorini/tct_colbert-v2-hnp-msmarco'.
## Getting started
This artefact is meant to be used with the 'pyterrier_doc2query' pacakge. It can
be installed as:
Depending on what you are using this aretefact for, you may also need the following additional packages:
## Using this artefact
The main use case is to use this aretefact in a Doc2Query−− indexing pipeline:
You can also use the store directly as a dataset to look up or iterate over the data:
## Reproducing this aretefact
This aretefact can be reproduced using the following pipeline:
Note that this process will take quite some time; it computes the relevance score for 80 generated queries
for every document in the dataset.
| [
"# Doc2Query TCT Relevance Scores for 'msmarco-passage'\n\nThis dataset provides the pre-computed query relevance scores for the 'msmarco-passage' dataset,\nfor use with Doc2Query--.\n\nThe generated queries come from 'macavaney/d2q-msmarco-passage' and\nwere scored with 'castorini/tct_colbert-v2-hnp-msmarco'.",
"## Getting started\n\nThis artefact is meant to be used with the 'pyterrier_doc2query' pacakge. It can\nbe installed as:\n\n\n\nDepending on what you are using this aretefact for, you may also need the following additional packages:",
"## Using this artefact\n\nThe main use case is to use this aretefact in a Doc2Query−− indexing pipeline:\n\n\n\nYou can also use the store directly as a dataset to look up or iterate over the data:",
"## Reproducing this aretefact\n\nThis aretefact can be reproduced using the following pipeline:\n\n\n\nNote that this process will take quite some time; it computes the relevance score for 80 generated queries\nfor every document in the dataset."
] | [
"TAGS\n#task_categories-text-retrieval #task_ids-document-retrieval #annotations_creators-no-annotation #language_creators-machine-generated #source_datasets-msmarco-passage #document-expansion #doc2query-- #region-us \n",
"# Doc2Query TCT Relevance Scores for 'msmarco-passage'\n\nThis dataset provides the pre-computed query relevance scores for the 'msmarco-passage' dataset,\nfor use with Doc2Query--.\n\nThe generated queries come from 'macavaney/d2q-msmarco-passage' and\nwere scored with 'castorini/tct_colbert-v2-hnp-msmarco'.",
"## Getting started\n\nThis artefact is meant to be used with the 'pyterrier_doc2query' pacakge. It can\nbe installed as:\n\n\n\nDepending on what you are using this aretefact for, you may also need the following additional packages:",
"## Using this artefact\n\nThe main use case is to use this aretefact in a Doc2Query−− indexing pipeline:\n\n\n\nYou can also use the store directly as a dataset to look up or iterate over the data:",
"## Reproducing this aretefact\n\nThis aretefact can be reproduced using the following pipeline:\n\n\n\nNote that this process will take quite some time; it computes the relevance score for 80 generated queries\nfor every document in the dataset."
] |
b7293a0e2ddb95e0eaa520029301a93d01bafd7f |
## Table of Contents
- [Dataset Description](#dataset-description)
-
# Utilising Weak Supervision to Create S3D: A Sarcasm Annotated Dataset
This is the repository for the S3D dataset published at EMNLP 2022. The dataset can help build sarcasm detection models.
# S3D-v2 Summary
The S3D-v2 dataset is our silver standard dataset of 100,000 tweets labelled for sarcasm using weak supervision by a majority voting system of fine-tuned sarcasm detection models. The models used are
our [roberta-large-finetuned-SARC-combined-DS](https://huggingface.co/surrey-nlp/roberta-large-finetuned-SARC-combined-DS), [bertweet-base-finetuned-SARC-DS](https://huggingface.co/surrey-nlp/bertweet-base-finetuned-SARC-DS)
and [bertweet-base-finetuned-SARC-combined-DS](https://huggingface.co/surrey-nlp/bertweet-base-finetuned-SARC-combined-DS) models.
S3D contains 13016 tweets labelled as sarcastic, and 86904 tweets labelled as not being sarcastic.
# Data Fields
- Text: The preprocessed tweet
- Label: A label to denote if a given tweet is sarcastic
# Data Splits
- Train: 70,000
- Valid: 15,000
- Test: 15,000 | surrey-nlp/S3D-v2 | [
"task_categories:text-classification",
"annotations_creators:Jordan Painter, Diptesh Kanojia",
"multilinguality:monolingual",
"size_categories:100K<n<1M",
"source_datasets:original",
"language:en",
"license:cc-by-sa-4.0",
"region:us"
] | 2022-12-17T18:00:12+00:00 | {"annotations_creators": ["Jordan Painter, Diptesh Kanojia"], "language": ["en"], "license": ["cc-by-sa-4.0"], "multilinguality": ["monolingual"], "size_categories": ["100K<n<1M"], "source_datasets": ["original"], "task_categories": ["text-classification"], "pretty_name": "Utilising Weak Supervision to create S3D: A Sarcasm Annotated Dataset"} | 2022-12-17T18:17:27+00:00 | [] | [
"en"
] | TAGS
#task_categories-text-classification #annotations_creators-Jordan Painter, Diptesh Kanojia #multilinguality-monolingual #size_categories-100K<n<1M #source_datasets-original #language-English #license-cc-by-sa-4.0 #region-us
|
## Table of Contents
- Dataset Description
-
# Utilising Weak Supervision to Create S3D: A Sarcasm Annotated Dataset
This is the repository for the S3D dataset published at EMNLP 2022. The dataset can help build sarcasm detection models.
# S3D-v2 Summary
The S3D-v2 dataset is our silver standard dataset of 100,000 tweets labelled for sarcasm using weak supervision by a majority voting system of fine-tuned sarcasm detection models. The models used are
our roberta-large-finetuned-SARC-combined-DS, bertweet-base-finetuned-SARC-DS
and bertweet-base-finetuned-SARC-combined-DS models.
S3D contains 13016 tweets labelled as sarcastic, and 86904 tweets labelled as not being sarcastic.
# Data Fields
- Text: The preprocessed tweet
- Label: A label to denote if a given tweet is sarcastic
# Data Splits
- Train: 70,000
- Valid: 15,000
- Test: 15,000 | [
"## Table of Contents\n- Dataset Description\n\n-",
"# Utilising Weak Supervision to Create S3D: A Sarcasm Annotated Dataset\nThis is the repository for the S3D dataset published at EMNLP 2022. The dataset can help build sarcasm detection models.",
"# S3D-v2 Summary\nThe S3D-v2 dataset is our silver standard dataset of 100,000 tweets labelled for sarcasm using weak supervision by a majority voting system of fine-tuned sarcasm detection models. The models used are \nour roberta-large-finetuned-SARC-combined-DS, bertweet-base-finetuned-SARC-DS\nand bertweet-base-finetuned-SARC-combined-DS models.\n\nS3D contains 13016 tweets labelled as sarcastic, and 86904 tweets labelled as not being sarcastic.",
"# Data Fields\n- Text: The preprocessed tweet\n- Label: A label to denote if a given tweet is sarcastic",
"# Data Splits\n- Train: 70,000\n- Valid: 15,000\n- Test: 15,000"
] | [
"TAGS\n#task_categories-text-classification #annotations_creators-Jordan Painter, Diptesh Kanojia #multilinguality-monolingual #size_categories-100K<n<1M #source_datasets-original #language-English #license-cc-by-sa-4.0 #region-us \n",
"## Table of Contents\n- Dataset Description\n\n-",
"# Utilising Weak Supervision to Create S3D: A Sarcasm Annotated Dataset\nThis is the repository for the S3D dataset published at EMNLP 2022. The dataset can help build sarcasm detection models.",
"# S3D-v2 Summary\nThe S3D-v2 dataset is our silver standard dataset of 100,000 tweets labelled for sarcasm using weak supervision by a majority voting system of fine-tuned sarcasm detection models. The models used are \nour roberta-large-finetuned-SARC-combined-DS, bertweet-base-finetuned-SARC-DS\nand bertweet-base-finetuned-SARC-combined-DS models.\n\nS3D contains 13016 tweets labelled as sarcastic, and 86904 tweets labelled as not being sarcastic.",
"# Data Fields\n- Text: The preprocessed tweet\n- Label: A label to denote if a given tweet is sarcastic",
"# Data Splits\n- Train: 70,000\n- Valid: 15,000\n- Test: 15,000"
] |
37beb97b7900281cd67ac189d4fb91c589b25582 | # Dataset Card for Birdsnap Dataset v 1.1.
Welcome to the Birdsnap dataset, consisting of 49,829 images of 500 species of North American birds, collected from Flickr, and corresponding species, bounding box, and part labels.
The dataset distribution also consists of the following files:
1. species.txt
This file lists the species in the dataset. The first line is a header. Each subsequent line represents a species. Lines are tab-delimited, and the fields
are:
- id: An integer id for the species. These ids run from 1 to 500 for the 500 species.
- common: The common English name of the species, for example "Blue Jay."
- scientific: The scientific (Latin) name of the species, for example "Cyanocitta cristata."
- dir: The name of the a directory in which to store the images of this species. This is just the common name with spaces and other dangerous-in-file-path characters replaced or removed.
2. images.txt
This file lists the images in the dataset, with the coresponding bounding boxes, part locations, and species labels. Like species.txt, it is tab-delimited with the first line giving field names. The fields are:
- url: The URL from which the image was downloaded.
- md5: An MD5 sum of the image file constants.
- path: The local path of the image.
- species_id: The id of the species of the labeled bird in the image.
- bb_x1, bb_y1, bb_x2, bb_y2: The coordinates of the top-left (bb_x1, bb_y1) and bottom-right (bb_x2, bb_y2) corners of the bounding box of the labeled
bird.
- ${part}_x, ${part}_y: The coordinates of part ${part}. Parts are back, beak, belly, breast, crown, forehead, left_cheek, left_eye, left_leg, left_wing, nape, right_cheek, right_eye, right_leg, right_wing, tail, throat.
3. test_images.txt
This file lists the 2443 test images used in the species identification experiments in the paper. It has a header line, then the "path" (from images.txt) of each test image, one per line.
### Citation
```
@inproceedings{berg2014birdsnap,
title={Birdsnap: Large-scale fine-grained visual categorization of birds},
author={Berg, Thomas and Liu, Jiongxin and Woo Lee, Seung and Alexander, Michelle L and Jacobs, David W and Belhumeur, Peter N},
booktitle={Proceedings of the IEEE Conference on Computer Vision and Pattern Recognition},
pages={2011--2018},
year={2014}
}
```
| sasha/birdsnap | [
"region:us"
] | 2022-12-17T20:35:55+00:00 | {"dataset_info": {"features": [{"name": "image", "dtype": "image"}, {"name": "label", "dtype": "string"}], "splits": [{"name": "train", "num_bytes": 63184668691.7, "num_examples": 39860}], "download_size": 69093722465, "dataset_size": 63184668691.7}} | 2022-12-17T21:29:07+00:00 | [] | [] | TAGS
#region-us
| # Dataset Card for Birdsnap Dataset v 1.1.
Welcome to the Birdsnap dataset, consisting of 49,829 images of 500 species of North American birds, collected from Flickr, and corresponding species, bounding box, and part labels.
The dataset distribution also consists of the following files:
1. URL
This file lists the species in the dataset. The first line is a header. Each subsequent line represents a species. Lines are tab-delimited, and the fields
are:
- id: An integer id for the species. These ids run from 1 to 500 for the 500 species.
- common: The common English name of the species, for example "Blue Jay."
- scientific: The scientific (Latin) name of the species, for example "Cyanocitta cristata."
- dir: The name of the a directory in which to store the images of this species. This is just the common name with spaces and other dangerous-in-file-path characters replaced or removed.
2. URL
This file lists the images in the dataset, with the coresponding bounding boxes, part locations, and species labels. Like URL, it is tab-delimited with the first line giving field names. The fields are:
- url: The URL from which the image was downloaded.
- md5: An MD5 sum of the image file constants.
- path: The local path of the image.
- species_id: The id of the species of the labeled bird in the image.
- bb_x1, bb_y1, bb_x2, bb_y2: The coordinates of the top-left (bb_x1, bb_y1) and bottom-right (bb_x2, bb_y2) corners of the bounding box of the labeled
bird.
- ${part}_x, ${part}_y: The coordinates of part ${part}. Parts are back, beak, belly, breast, crown, forehead, left_cheek, left_eye, left_leg, left_wing, nape, right_cheek, right_eye, right_leg, right_wing, tail, throat.
3. test_images.txt
This file lists the 2443 test images used in the species identification experiments in the paper. It has a header line, then the "path" (from URL) of each test image, one per line.
| [
"# Dataset Card for Birdsnap Dataset v 1.1.\n\nWelcome to the Birdsnap dataset, consisting of 49,829 images of 500 species of North American birds, collected from Flickr, and corresponding species, bounding box, and part labels.\n\n\nThe dataset distribution also consists of the following files:\n\n1. URL\nThis file lists the species in the dataset. The first line is a header. Each subsequent line represents a species. Lines are tab-delimited, and the fields\nare:\n- id: An integer id for the species. These ids run from 1 to 500 for the 500 species.\n- common: The common English name of the species, for example \"Blue Jay.\"\n- scientific: The scientific (Latin) name of the species, for example \"Cyanocitta cristata.\"\n- dir: The name of the a directory in which to store the images of this species. This is just the common name with spaces and other dangerous-in-file-path characters replaced or removed.\n\n2. URL\nThis file lists the images in the dataset, with the coresponding bounding boxes, part locations, and species labels. Like URL, it is tab-delimited with the first line giving field names. The fields are:\n- url: The URL from which the image was downloaded.\n- md5: An MD5 sum of the image file constants.\n- path: The local path of the image.\n- species_id: The id of the species of the labeled bird in the image.\n- bb_x1, bb_y1, bb_x2, bb_y2: The coordinates of the top-left (bb_x1, bb_y1) and bottom-right (bb_x2, bb_y2) corners of the bounding box of the labeled\n bird.\n- ${part}_x, ${part}_y: The coordinates of part ${part}. Parts are back, beak, belly, breast, crown, forehead, left_cheek, left_eye, left_leg, left_wing, nape, right_cheek, right_eye, right_leg, right_wing, tail, throat.\n\n3. test_images.txt\nThis file lists the 2443 test images used in the species identification experiments in the paper. It has a header line, then the \"path\" (from URL) of each test image, one per line."
] | [
"TAGS\n#region-us \n",
"# Dataset Card for Birdsnap Dataset v 1.1.\n\nWelcome to the Birdsnap dataset, consisting of 49,829 images of 500 species of North American birds, collected from Flickr, and corresponding species, bounding box, and part labels.\n\n\nThe dataset distribution also consists of the following files:\n\n1. URL\nThis file lists the species in the dataset. The first line is a header. Each subsequent line represents a species. Lines are tab-delimited, and the fields\nare:\n- id: An integer id for the species. These ids run from 1 to 500 for the 500 species.\n- common: The common English name of the species, for example \"Blue Jay.\"\n- scientific: The scientific (Latin) name of the species, for example \"Cyanocitta cristata.\"\n- dir: The name of the a directory in which to store the images of this species. This is just the common name with spaces and other dangerous-in-file-path characters replaced or removed.\n\n2. URL\nThis file lists the images in the dataset, with the coresponding bounding boxes, part locations, and species labels. Like URL, it is tab-delimited with the first line giving field names. The fields are:\n- url: The URL from which the image was downloaded.\n- md5: An MD5 sum of the image file constants.\n- path: The local path of the image.\n- species_id: The id of the species of the labeled bird in the image.\n- bb_x1, bb_y1, bb_x2, bb_y2: The coordinates of the top-left (bb_x1, bb_y1) and bottom-right (bb_x2, bb_y2) corners of the bounding box of the labeled\n bird.\n- ${part}_x, ${part}_y: The coordinates of part ${part}. Parts are back, beak, belly, breast, crown, forehead, left_cheek, left_eye, left_leg, left_wing, nape, right_cheek, right_eye, right_leg, right_wing, tail, throat.\n\n3. test_images.txt\nThis file lists the 2443 test images used in the species identification experiments in the paper. It has a header line, then the \"path\" (from URL) of each test image, one per line."
] |
883fc9343999ef5f3d5b1f23a1ef78f517106433 | # Dataset Card for "unet-lsun-256"
[More Information needed](https://github.com/huggingface/datasets/blob/main/CONTRIBUTING.md#how-to-contribute-to-the-dataset-cards) | Dahoas/unet-lsun-256 | [
"region:us"
] | 2022-12-17T21:18:33+00:00 | {"dataset_info": {"features": [{"name": "images", "sequence": {"sequence": {"sequence": "float32"}}}], "splits": [{"name": "train", "num_bytes": 39513896960, "num_examples": 50048}], "download_size": 39351524715, "dataset_size": 39513896960}} | 2022-12-19T16:02:31+00:00 | [] | [] | TAGS
#region-us
| # Dataset Card for "unet-lsun-256"
More Information needed | [
"# Dataset Card for \"unet-lsun-256\"\n\nMore Information needed"
] | [
"TAGS\n#region-us \n",
"# Dataset Card for \"unet-lsun-256\"\n\nMore Information needed"
] |
a29a9757125f4bb1c26445ad0d2ef7d9b2cc9c4c | Preprocessed version of Super-Natural-Instructions from https://github.com/allenai/natural-instructions/tree/master/splits. The same inputs may appear with different outputs, thus to avoid duplicate inputs, you can deduplicate by the `id` or the `inputs` field.
Train Tasks:
```
['task001_quoref_question_generation', 'task002_quoref_answer_generation', 'task022_cosmosqa_passage_inappropriate_binary', 'task023_cosmosqa_question_generation', 'task024_cosmosqa_answer_generation', 'task025_cosmosqa_incorrect_answer_generation', 'task026_drop_question_generation', 'task027_drop_answer_type_generation', 'task028_drop_answer_generation', 'task043_essential_terms_answering_incomplete_questions', 'task044_essential_terms_identifying_essential_words', 'task045_miscellaneous_sentence_paraphrasing', 'task046_miscellaneous_question_typing', 'task047_miscellaneous_answering_science_questions', 'task059_ropes_story_generation', 'task060_ropes_question_generation', 'task061_ropes_answer_generation', 'task062_bigbench_repeat_copy_logic', 'task063_first_i_elements', 'task064_all_elements_except_first_i', 'task065_timetravel_consistent_sentence_classification', 'task066_timetravel_binary_consistency_classification', 'task067_abductivenli_answer_generation', 'task068_abductivenli_incorrect_answer_generation', 'task069_abductivenli_classification', 'task070_abductivenli_incorrect_classification', 'task071_abductivenli_answer_generation', 'task072_abductivenli_answer_generation', 'task073_commonsenseqa_answer_generation', 'task074_squad1.1_question_generation', 'task075_squad1.1_answer_generation', 'task076_splash_correcting_sql_mistake', 'task077_splash_explanation_to_sql', 'task078_all_elements_except_last_i', 'task079_conala_concat_strings', 'task080_piqa_answer_generation', 'task081_piqa_wrong_answer_generation', 'task082_babi_t1_single_supporting_fact_question_generation', 'task083_babi_t1_single_supporting_fact_answer_generation', 'task084_babi_t1_single_supporting_fact_identify_relevant_fact', 'task085_unnatural_addsub_arithmetic', 'task087_new_operator_addsub_arithmetic', 'task088_identify_typo_verification', 'task089_swap_words_verification', 'task090_equation_learner_algebra', 'task091_all_elements_from_index_i_to_j', 'task092_check_prime_classification', 'task093_conala_normalize_lists', 'task094_conala_calculate_mean', 'task095_conala_max_absolute_value', 'task096_conala_list_index_subtraction', 'task097_conala_remove_duplicates', 'task098_conala_list_intersection', 'task099_reverse_elements_between_index_i_and_j', 'task100_concatenate_all_elements_from_index_i_to_j', 'task101_reverse_and_concatenate_all_elements_from_index_i_to_j', 'task103_facts2story_long_text_generation', 'task104_semeval_2019_task10_closed_vocabulary_mathematical_answer_generation', 'task105_story_cloze-rocstories_sentence_generation', 'task107_splash_question_to_sql', 'task1087_two_number_sum', 'task1088_array_of_products', 'task1089_check_monotonic_array', 'task108_contextualabusedetection_classification', 'task109_smsspamcollection_spamsmsdetection', 'task110_logic2text_sentence_generation', 'task111_asset_sentence_simplification', 'task112_asset_simple_sentence_identification', 'task1135_xcsr_en_commonsense_mc_classification', 'task113_count_frequency_of_letter', 'task1146_country_capital', 'task1147_country_currency', 'task1148_maximum_ascii_value', 'task1149_item_check_edible', 'task114_is_the_given_word_longest', 'task1150_delete_max_min', 'task1151_swap_max_min', 'task115_help_advice_classification', 'task1167_penn_treebank_coarse_pos_tagging', 'task1168_brown_coarse_pos_tagging', 'task116_com2sense_commonsense_reasoning', 'task1186_nne_hrngo_classification', 'task1188_count_max_freq_char', 'task1189_check_char_in_string', 'task118_semeval_2019_task10_open_vocabulary_mathematical_answer_generation', 'task1190_add_integer_to_list', 'task1191_food_veg_nonveg', 'task1192_food_flavor_profile', 'task1193_food_course_classification', 'task1194_kth_largest_element', 'task1196_atomic_classification_oeffect', 'task1197_atomic_classification_oreact', 'task1198_atomic_classification_owant', 'task1199_atomic_classification_xattr', 'task119_semeval_2019_task10_geometric_mathematical_answer_generation', 'task1200_atomic_classification_xeffect', 'task1201_atomic_classification_xintent', 'task1202_atomic_classification_xneed', 'task1203_atomic_classification_xreact', 'task1204_atomic_classification_hinderedby', 'task1205_atomic_classification_isafter', 'task1206_atomic_classification_isbefore', 'task1207_atomic_classification_atlocation', 'task1208_atomic_classification_xreason', 'task1209_atomic_classification_objectuse', 'task1210_atomic_classification_madeupof', 'task1211_atomic_classification_hassubevent', 'task1212_atomic_classification_hasproperty', 'task1213_atomic_classification_desires', 'task1214_atomic_classification_xwant', 'task1215_atomic_classification_capableof', 'task1216_atomic_classification_causes', 'task1217_atomic_answer_generation', 'task122_conala_list_index_addition', 'task123_conala_sort_dictionary', 'task124_conala_pair_averages', 'task125_conala_pair_differences', 'task126_scan_structured_text_generation_command_action_all', 'task127_scan_long_text_generation_action_command_all', 'task1283_hrngo_quality_classification', 'task1284_hrngo_informativeness_classification', 'task1285_kpa_keypoint_matching', 'task1286_openbookqa_question_answering', 'task1288_glue_mrpc_paraphrasing', 'task1289_trec_classification', 'task128_scan_structured_text_generation_command_action_short', 'task1290_xsum_summarization', 'task1291_multi_news_summarization', 'task1292_yelp_review_full_text_categorization', 'task1293_kilt_tasks_hotpotqa_question_answering', 'task1294_wiki_qa_answer_verification', 'task1295_adversarial_qa_question_answering', 'task1296_wiki_hop_question_answering', 'task129_scan_long_text_generation_action_command_short', 'task1308_amazonreview_category_classification', 'task1309_amazonreview_summary_classification', 'task130_scan_structured_text_generation_command_action_long', 'task1310_amazonreview_rating_classification', 'task1311_amazonreview_rating_classification', 'task1312_amazonreview_polarity_classification', 'task1313_amazonreview_polarity_classification', 'task1314_country_abbreviation', 'task1315_find_range_array', 'task1316_remove_duplicates_string', 'task1317_country_calling_code', 'task1318_country_national_dish', 'task1319_country_by_barcode_prefix', 'task131_scan_long_text_generation_action_command_long', 'task1320_country_domain_tld', 'task1321_country_continent', 'task1322_country_government_type', 'task1325_qa_zre_question_generation_on_subject_relation', 'task1326_qa_zre_question_generation_from_answer', 'task1327_qa_zre_answer_generation_from_question', 'task1328_qa_zre_relation_generation_from_question', 'task132_dais_text_modification', 'task1331_reverse_array', 'task1332_check_leap_year', 'task1333_check_validity_date_ddmmyyyy', 'task1336_peixian_equity_evaluation_corpus_gender_classifier', 'task1338_peixian_equity_evaluation_corpus_sentiment_classifier', 'task1339_peixian_equity_evaluation_corpus_text_completion', 'task1340_msr_text_compression_compression', 'task1341_msr_text_classification', 'task1346_glue_cola_grammatical_correctness_classification', 'task1347_glue_sts-b_similarity_classification', 'task1354_sent_comp_classification', 'task1355_sent_comp_summarization', 'task1359_numer_sense_answer_generation', 'task1360_numer_sense_multiple_choice_qa_generation', 'task1361_movierationales_classification', 'task1364_hans_answer_generation', 'task1366_healthfact_classification', 'task1368_healthfact_sentence_generation', 'task1369_healthfact_sentence_generation', 'task1378_quarel_correct_answer_generation', 'task1379_quarel_incorrect_answer_generation', 'task137_detoxifying-lms_classification_toxicity', 'task1380_quarel_correct_option_generation', 'task1381_quarel_incorrect_option_generation', 'task1382_quarel_write_correct_answer', 'task1383_quarel_write_incorrect_answer', 'task1384_deal_or_no_dialog_classification', 'task1389_hellaswag_completion', 'task138_detoxifying-lms_classification_fluency', 'task1398_obqa_question_generation', 'task1399_obqa_answer_generation', 'task139_detoxifying-lms_classification_topicality', 'task1400_obqa_incorrect_answer_generation', 'task1401_obqa_sentence_generation', 'task1403_check_validity_date_mmddyyyy', 'task1404_date_conversion', 'task1405_find_median', 'task1406_kth_smallest_element', 'task140_detoxifying-lms_classification_style', 'task1412_web_questions_question_answering', 'task1418_bless_semantic_relation_classification', 'task1419_mathqa_gain', 'task141_odd-man-out_classification_category', 'task1420_mathqa_general', 'task1421_mathqa_other', 'task1422_mathqa_physics', 'task1423_mathqa_geometry', 'task1424_mathqa_probability', 'task1425_country_iso_numeric', 'task1426_country_independence_year', 'task1427_country_region_in_world', 'task1428_country_surface_area', 'task1429_evalution_semantic_relation_classification', 'task142_odd-man-out_classification_no_category', 'task1431_head_qa_answer_generation', 'task1434_head_qa_classification', 'task143_odd-man-out_classification_generate_category', 'task1443_string_to_number', 'task1444_round_power_of_two', 'task1445_closest_integers', 'task1446_farthest_integers', 'task1447_drug_extraction_ade', 'task1448_disease_entity_extraction_ncbi_dataset', 'task1449_disease_entity_extraction_bc5cdr_dataset', 'task144_subjqa_question_answering', 'task1451_drug_dose_extraction', 'task1452_location_entity_extraction_btc_corpus', 'task1453_person_entity_extraction_btc_corpus', 'task145_afs_argument_similarity_death_penalty', 'task146_afs_argument_similarity_gun_control', 'task1479_organization_entity_extraction_btc_corpus', 'task147_afs_argument_similarity_gay_marriage', 'task1480_gene_extraction_jnlpba_dataset', 'task1481_gene_extraction_bc2gm_dataset', 'task1482_gene_extraction_chemprot_dataset', 'task1483_chemical_extraction_chemprot_dataset', 'task1484_gene_extraction_linnaeus_dataset', 'task1485_organ_extraction_anem_dataset', 'task1486_cell_extraction_anem_dataset', 'task1487_organism_substance_extraction_anem_dataset', 'task1488_sarcasmdetection_headline_classification', 'task1489_sarcasmdetection_tweet_classification', 'task148_afs_argument_quality_gay_marriage', 'task1495_adverse_drug_event_classification', 'task1498_24hour_to_12hour_clock', 'task1499_dstc3_summarization', 'task149_afs_argument_quality_death_penalty', 'task1500_dstc3_classification', 'task1501_dstc3_answer_generation', 'task1502_hatexplain_classification', 'task1503_hatexplain_classification', 'task1504_hatexplain_answer_generation', 'task1505_root09_semantic_relation_classification', 'task1506_celebrity_minimal_dob_span', 'task1507_boolean_temporal_reasoning', 'task1508_wordnet_antonyms', 'task1509_evalution_antonyms', 'task150_afs_argument_quality_gun_control', 'task1510_evalution_relation_extraction', 'task1517_limit_classfication', 'task1518_limit_answer_generation', 'task1519_qa_srl_question_generation', 'task151_tomqa_find_location_easy_clean', 'task1520_qa_srl_answer_generation', 'task152_tomqa_find_location_easy_noise', 'task153_tomqa_find_location_hard_clean', 'task1541_agnews_classification', 'task1542_every_ith_element_from_starting', 'task1548_wiqa_binary_classification', 'task1549_wiqa_answer_generation_missing_step', 'task154_tomqa_find_location_hard_noise', 'task1551_every_ith_element_from_kth_element', 'task1553_cnn_dailymail_summarization', 'task1559_blimp_binary_classification', 'task155_count_nouns_verbs', 'task1560_blimp_binary_classification', 'task1564_triviaqa_answer_generation', 'task1565_triviaqa_classification', 'task1566_propara_structured_text_generation', 'task1567_propara_question_generation', 'task1568_propara_classification', 'task156_codah_classification_adversarial', 'task1572_samsum_summary', 'task1573_samsum_classification', 'task157_count_vowels_and_consonants', 'task1580_eqasc-perturbed_question_generation', 'task1581_eqasc-perturbed_answer_generation', 'task1582_bless_hypernym_generation', 'task1583_bless_meronym_classification', 'task1584_evalution_meronym_classification', 'task1585_root09_hypernym_generation', 'task158_count_frequency_of_words', 'task1590_diplomacy_text_generation', 'task1592_yahoo_answers_topics_classfication', 'task1593_yahoo_answers_topics_classification', 'task1594_yahoo_answers_topics_question_generation', 'task1595_event2mind_text_generation_1', 'task1596_event2mind_text_generation_2', 'task1599_smcalflow_classification', 'task159_check_frequency_of_words_in_sentence_pair', 'task1600_smcalflow_sentence_generation', 'task1601_webquestions_answer_generation', 'task1602_webquestion_question_genreation', 'task1603_smcalflow_sentence_generation', 'task1604_ethos_text_classification', 'task1605_ethos_text_classification', 'task1606_ethos_text_classification', 'task1607_ethos_text_classification', 'task1608_xquad_en_answer_generation', 'task1609_xquad_en_question_generation', 'task160_replace_letter_in_a_sentence', 'task161_count_words_containing_letter', 'task162_count_words_starting_with_letter', 'task163_count_words_ending_with_letter', 'task1645_medical_question_pair_dataset_text_classification', 'task164_mcscript_question_answering_text', 'task1656_gooaq_answer_generation', 'task1657_gooaq_question_generation', 'task165_mcscript_question_answering_commonsense', 'task1660_super_glue_question_generation', 'task1661_super_glue_classification', 'task1665_trainglecopa_question_generation', 'task1669_md_gender_bias_text_modification', 'task166_clariq_sentence_generation', 'task1670_md_gender_bias_text_modification', 'task1678_mathqa_answer_selection', 'task167_strategyqa_question_generation', 'task168_strategyqa_question_decomposition', 'task169_strategyqa_sentence_generation', 'task1703_ljspeech_textmodification', 'task1704_ljspeech_textmodification', 'task1705_ljspeech_classification', 'task1706_ljspeech_classification', 'task170_hotpotqa_answer_generation', 'task1711_poki_text_generation', 'task1712_poki_classification', 'task1713_convai3_sentence_generation', 'task1714_convai3_sentence_generation', 'task1720_civil_comments_toxicity_classification', 'task1721_civil_comments_obscenity_classification', 'task1722_civil_comments_threat_classification', 'task1723_civil_comments_sexuallyexplicit_classification', 'task1724_civil_comments_insult_classification', 'task1725_civil_comments_severtoxicity_classification', 'task1726_mathqa_correct_answer_generation', 'task1727_wiqa_what_is_the_effect', 'task1729_personachat_generate_next', 'task1730_personachat_choose_next', 'task1731_quartz_question_answering', 'task176_break_decompose_questions', 'task177_para-nmt_paraphrasing', 'task178_quartz_question_answering', 'task179_participant_extraction', 'task180_intervention_extraction', 'task181_outcome_extraction', 'task182_duorc_question_generation', 'task183_rhyme_generation', 'task184_break_generate_question', 'task191_hotpotqa_question_generation', 'task192_hotpotqa_sentence_generation', 'task193_duorc_question_generation', 'task194_duorc_answer_generation', 'task195_sentiment140_classification', 'task196_sentiment140_answer_generation', 'task205_remove_even_elements', 'task206_collatz_conjecture', 'task207_max_element_lists', 'task208_combinations_of_list', 'task209_stancedetection_classification', 'task210_logic2text_structured_text_generation', 'task211_logic2text_classification', 'task212_logic2text_classification', 'task223_quartz_explanation_generation', 'task227_clariq_classification', 'task228_arc_answer_generation_easy', 'task229_arc_answer_generation_hard', 'task243_count_elements_in_set_intersection', 'task244_count_elements_in_set_union', 'task245_check_presence_in_set_intersection', 'task246_dream_question_generation', 'task247_dream_answer_generation', 'task248_dream_classification', 'task267_concatenate_and_reverse_all_elements_from_index_i_to_j', 'task268_casehold_legal_answer_generation', 'task269_csrg_counterfactual_story_generation', 'task270_csrg_counterfactual_context_generation', 'task274_overruling_legal_classification', 'task275_enhanced_wsc_paraphrase_generation', 'task276_enhanced_wsc_classification', 'task277_stereoset_sentence_generation_stereotype', 'task278_stereoset_sentence_generation_antistereotype', 'task279_stereoset_classification_stereotype', 'task280_stereoset_classification_stereotype_type', 'task283_dream_incorrect_answer_generation', 'task284_imdb_classification', 'task285_imdb_answer_generation', 'task286_olid_offense_judgment', 'task287_casehold_legal_incorrect_answer_generation', 'task291_semeval_2020_task4_commonsense_validation', 'task292_storycommonsense_character_text_generation', 'task293_storycommonsense_emotion_text_generation', 'task294_storycommonsense_motiv_text_generation', 'task295_semeval_2020_task4_commonsense_reasoning', 'task296_storycloze_correct_end_classification', 'task297_storycloze_incorrect_end_classification', 'task298_storycloze_correct_end_classification', 'task299_storycloze_sentence_generation', 'task300_storycloze_order_generation', 'task301_record_question_generation', 'task302_record_classification', 'task303_record_incorrect_answer_generation', 'task305_jeopardy_answer_generation_normal', 'task306_jeopardy_answer_generation_double', 'task307_jeopardy_answer_generation_final', 'task308_jeopardy_answer_generation_all', 'task309_race_answer_generation', 'task310_race_classification', 'task311_race_question_generation', 'task316_crows-pairs_classification_stereotype', 'task317_crows-pairs_classification_stereotype_type', 'task318_stereoset_classification_gender', 'task319_stereoset_classification_profession', 'task320_stereoset_classification_race', 'task321_stereoset_classification_religion', 'task322_jigsaw_classification_threat', 'task323_jigsaw_classification_sexually_explicit', 'task324_jigsaw_classification_disagree', 'task325_jigsaw_classification_identity_attack', 'task326_jigsaw_classification_obscene', 'task327_jigsaw_classification_toxic', 'task328_jigsaw_classification_insult', 'task333_hateeval_classification_hate_en', 'task335_hateeval_classification_aggresive_en', 'task337_hateeval_classification_individual_en', 'task339_record_answer_generation', 'task340_winomt_classification_gender_pro', 'task341_winomt_classification_gender_anti', 'task342_winomt_classification_profession_pro', 'task343_winomt_classification_profession_anti', 'task344_hybridqa_answer_generation', 'task345_hybridqa_answer_generation', 'task346_hybridqa_classification', 'task347_hybridqa_incorrect_answer_generation', 'task350_winomt_classification_gender_identifiability_pro', 'task351_winomt_classification_gender_identifiability_anti', 'task353_casino_classification_negotiation_elicit_pref', 'task354_casino_classification_negotiation_no_need', 'task355_casino_classification_negotiation_other_need', 'task356_casino_classification_negotiation_self_need', 'task357_casino_classification_negotiation_small_talk', 'task358_casino_classification_negotiation_uv_part', 'task359_casino_classification_negotiation_vouch_fair', 'task363_sst2_polarity_classification', 'task364_regard_social_impact_classification', 'task365_synthetic_remove_vowels', 'task366_synthetic_return_primes', 'task367_synthetic_remove_floats', 'task368_synthetic_even_or_odd_calculation', 'task369_synthetic_remove_odds', 'task370_synthetic_remove_divisible_by_3', 'task371_synthetic_product_of_list', 'task372_synthetic_palindrome_numbers', 'task373_synthetic_round_tens_place', 'task374_synthetic_pos_or_neg_calculation', 'task375_classify_type_of_sentence_in_debate', 'task376_reverse_order_of_words', 'task377_remove_words_of_given_length', 'task378_reverse_words_of_given_length', 'task379_agnews_topic_classification', 'task380_boolq_yes_no_question', 'task381_boolq_question_generation', 'task382_hybridqa_answer_generation', 'task383_matres_classification', 'task384_socialiqa_question_classification', 'task385_socialiqa_incorrect_answer_generation', 'task386_semeval_2018_task3_irony_detection', 'task387_semeval_2018_task3_irony_classification', 'task388_torque_token_classification', 'task389_torque_generate_temporal_question', 'task390_torque_text_span_selection', 'task397_semeval_2018_task1_tweet_anger_detection', 'task398_semeval_2018_task1_tweet_joy_detection', 'task399_semeval_2018_task1_tweet_sadness_detection', 'task400_paws_paraphrase_classification', 'task403_creak_commonsense_inference', 'task405_narrativeqa_question_generation', 'task413_mickey_en_sentence_perturbation_generation', 'task428_senteval_inversion', 'task429_senteval_tense', 'task430_senteval_subject_count', 'task431_senteval_object_count', 'task453_swag_answer_generation', 'task454_swag_incorrect_answer_generation', 'task455_swag_context_generation', 'task456_matres_intention_classification', 'task457_matres_conditional_classification', 'task458_matres_negation_classification', 'task459_matres_static_classification', 'task460_qasper_answer_generation', 'task461_qasper_question_generation', 'task462_qasper_classification', 'task469_mrqa_answer_generation', 'task470_mrqa_question_generation', 'task471_haspart_answer_generation', 'task472_haspart_classification', 'task475_yelp_polarity_classification', 'task476_cls_english_books_classification', 'task477_cls_english_dvd_classification', 'task478_cls_english_music_classification', 'task488_extract_all_alphabetical_elements_from_list_in_order', 'task489_mwsc_question_generation', 'task490_mwsc_options_generation', 'task491_mwsc_answer_generation', 'task492_mwsc_incorrect_answer_generation', 'task493_review_polarity_classification', 'task494_review_polarity_answer_generation', 'task495_semeval_headline_classification', 'task496_semeval_answer_generation', 'task497_extract_all_numbers_from_list_in_order', 'task499_extract_and_add_all_numbers_from_list', 'task504_count_all_alphabetical_elements_in_list', 'task505_count_all_numerical_elements_in_list', 'task506_position_of_all_alphabetical_elements_in_list', 'task507_position_of_all_numerical_elements_in_list', 'task509_collate_of_all_alphabetical_and_numerical_elements_in_list_separately', 'task512_twitter_emotion_classification', 'task513_argument_stance_classification', 'task514_argument_consequence_classification', 'task515_senteval_odd_word_out', 'task516_senteval_conjoints_inversion', 'task517_emo_classify_emotion_of_dialogue', 'task518_emo_different_dialogue_emotions', 'task521_trivia_question_classification', 'task522_news_editorial_summary', 'task523_find_if_numbers_or_alphabets_are_more_in_list', 'task547_alt_translation_entk_en', 'task550_discofuse_sentence_generation', 'task560_alt_translation_en_entk', 'task563_discofuse_answer_generation', 'task564_discofuse_classification', 'task565_circa_answer_generation', 'task566_circa_classification', 'task567_circa_text_generation', 'task568_circa_question_generation', 'task573_air_dialogue_classification', 'task574_air_dialogue_sentence_generation', 'task575_air_dialogue_classification', 'task576_curiosity_dialogs_answer_generation', 'task577_curiosity_dialogs_classification', 'task578_curiosity_dialogs_answer_generation', 'task579_socialiqa_classification', 'task580_socialiqa_answer_generation', 'task581_socialiqa_question_generation', 'task582_naturalquestion_answer_generation', 'task583_udeps_eng_coarse_pos_tagging', 'task584_udeps_eng_fine_pos_tagging', 'task585_preposition_classification', 'task586_amazonfood_polarity_classification', 'task587_amazonfood_polarity_correction_classification', 'task588_amazonfood_rating_classification', 'task589_amazonfood_summary_text_generation', 'task590_amazonfood_summary_correction_classification', 'task591_sciq_answer_generation', 'task592_sciq_incorrect_answer_generation', 'task593_sciq_explanation_generation', 'task594_sciq_question_generation', 'task595_mocha_answer_generation', 'task596_mocha_question_generation', 'task597_cuad_answer_generation', 'task598_cuad_answer_generation', 'task599_cuad_question_generation', 'task600_find_the_longest_common_substring_in_two_strings', 'task605_find_the_longest_common_subsequence_in_two_lists', 'task606_sum_of_all_numbers_in_list_between_positions_i_and_j', 'task607_sbic_intentional_offense_binary_classification', 'task608_sbic_sexual_offense_binary_classification', 'task609_sbic_potentially_offense_binary_classification', 'task610_conllpp_ner', 'task611_mutual_multi_turn_dialogue', 'task615_moviesqa_answer_generation', 'task616_cola_classification', 'task617_amazonreview_category_text_generation', 'task618_amazonreview_summary_text_generation', 'task622_replace_alphabets_in_a_list_by_their_position_in_english_alphabet', 'task625_xlwic_true_or_false_answer_generation', 'task626_xlwic_sentence_based_on_given_word_sentence_generation', 'task627_xlwic_word_with_same_meaning_sentence_generation', 'task628_xlwic_word_with_different_meaning_sentence_generation', 'task629_dbpedia_14_classification', 'task630_dbpedia_14_classification', 'task631_dbpedia_14_incorrect_answer_generation', 'task632_dbpedia_14_classification', 'task633_dbpedia_14_answer_generation', 'task636_extract_and_sort_unique_alphabets_in_a_list', 'task637_extract_and_sort_unique_digits_in_a_list', 'task638_multi_woz_classification', 'task639_multi_woz_user_utterance_generation', 'task649_race_blank_question_generation', 'task664_mmmlu_answer_generation_abstract_algebra', 'task665_mmmlu_answer_generation_anatomy', 'task666_mmmlu_answer_generation_astronomy', 'task667_mmmlu_answer_generation_business_ethics', 'task668_extreme_abstract_summarization', 'task672_amazon_and_yelp_summarization_dataset_summarization', 'task672_nummersense', 'task673_google_wellformed_query_classification', 'task674_google_wellformed_query_sentence_generation', 'task675_google_wellformed_query_sentence_generation', 'task679_hope_edi_english_text_classification', 'task681_hope_edi_malayalam_text_classification', 'task682_online_privacy_policy_text_classification', 'task683_online_privacy_policy_text_purpose_answer_generation', 'task684_online_privacy_policy_text_information_type_generation', 'task685_mmmlu_answer_generation_clinical_knowledge', 'task686_mmmlu_answer_generation_college_biology', 'task687_mmmlu_answer_generation_college_chemistry', 'task688_mmmlu_answer_generation_college_computer_science', 'task689_mmmlu_answer_generation_college_mathematics', 'task690_mmmlu_answer_generation_college_medicine', 'task691_mmmlu_answer_generation_college_physics', 'task692_mmmlu_answer_generation_computer_security', 'task693_mmmlu_answer_generation_conceptual_physics', 'task694_mmmlu_answer_generation_econometrics', 'task695_mmmlu_answer_generation_electrical_engineering', 'task696_mmmlu_answer_generation_elementary_mathematics', 'task697_mmmlu_answer_generation_formal_logic', 'task698_mmmlu_answer_generation_global_facts', 'task699_mmmlu_answer_generation_high_school_biology', 'task700_mmmlu_answer_generation_high_school_chemistry', 'task701_mmmlu_answer_generation_high_school_computer_science', 'task702_mmmlu_answer_generation_high_school_european_history', 'task703_mmmlu_answer_generation_high_school_geography', 'task704_mmmlu_answer_generation_high_school_government_and_politics', 'task705_mmmlu_answer_generation_high_school_macroeconomics', 'task706_mmmlu_answer_generation_high_school_mathematics', 'task707_mmmlu_answer_generation_high_school_microeconomics', 'task708_mmmlu_answer_generation_high_school_physics', 'task709_mmmlu_answer_generation_high_school_psychology', 'task710_mmmlu_answer_generation_high_school_statistics', 'task711_mmmlu_answer_generation_high_school_us_history', 'task712_mmmlu_answer_generation_high_school_world_history', 'task713_mmmlu_answer_generation_human_aging', 'task714_mmmlu_answer_generation_human_sexuality', 'task715_mmmlu_answer_generation_international_law', 'task716_mmmlu_answer_generation_jurisprudence', 'task717_mmmlu_answer_generation_logical_fallacies', 'task718_mmmlu_answer_generation_machine_learning', 'task719_mmmlu_answer_generation_management', 'task720_mmmlu_answer_generation_marketing', 'task721_mmmlu_answer_generation_medical_genetics', 'task722_mmmlu_answer_generation_random_topic', 'task723_mmmlu_answer_generation_moral_disputes', 'task724_mmmlu_answer_generation_moral_scenarios', 'task725_mmmlu_answer_generation_nutrition', 'task726_mmmlu_answer_generation_philosophy', 'task727_mmmlu_answer_generation_prehistory', 'task728_mmmlu_answer_generation_professional_accounting', 'task729_mmmlu_answer_generation_professional_law', 'task730_mmmlu_answer_generation_professional_medicine', 'task731_mmmlu_answer_generation_professional_psychology', 'task732_mmmlu_answer_generation_public_relations', 'task733_mmmlu_answer_generation_security_studies', 'task734_mmmlu_answer_generation_sociology', 'task735_mmmlu_answer_generation_us_foreign_policy', 'task736_mmmlu_answer_generation_virology', 'task737_mmmlu_answer_generation_world_religions', 'task739_lhoestq_question_generation', 'task740_lhoestq_answer_generation_quantity', 'task741_lhoestq_answer_generation_place', 'task742_lhoestq_answer_generation_frequency', 'task745_ai2_arithmetic_questions_arithmetic', 'task746_yelp_restaurant_review_classification', 'task750_aqua_multiple_choice_answering', 'task751_svamp_subtraction_question_answering', 'task752_svamp_multiplication_question_answering', 'task753_svamp_addition_question_answering', 'task754_svamp_common-division_question_answering', 'task755_find_longest_substring_and_replace_its_sorted_lowercase_version_in_both_lists', 'task756_find_longert_substring_and_return_all_unique_alphabets_in_it', 'task761_app_review_classification', 'task766_craigslist_bargains_classification', 'task767_craigslist_bargains_classification', 'task770_pawsx_english_text_modification', 'task819_pec_sentiment_classification', 'task820_protoqa_answer_generation', 'task821_protoqa_question_generation', 'task823_peixian-rtgender_sentiment_analysis', 'task833_poem_sentiment_classification', 'task834_mathdataset_classification', 'task835_mathdataset_answer_generation', 'task843_financial_phrasebank_classification', 'task844_financial_phrasebank_classification', 'task845_pubmedqa_question_generation', 'task846_pubmedqa_classification', 'task847_pubmedqa_question_generation', 'task848_pubmedqa_classification', 'task849_pubmedqa_answer_generation', 'task850_synthetic_longest_palindrome', 'task851_synthetic_multiply_evens', 'task852_synthetic_multiply_odds', 'task853_hippocorpus_long_text_generation', 'task854_hippocorpus_classification', 'task855_conv_ai_2_classification', 'task856_conv_ai_2_classification', 'task857_inquisitive_question_generation', 'task858_inquisitive_span_detection', 'task859_prost_question_generation', 'task860_prost_mcq_generation', 'task861_asdiv_addsub_question_answering', 'task861_prost_mcq_answers_generation', 'task862_asdiv_multidiv_question_answering', 'task863_asdiv_multiop_question_answering', 'task864_asdiv_singleop_question_answering', 'task865_mawps_addsub_question_answering', 'task866_mawps_multidiv_question_answering', 'task867_mawps_multiop_question_answering', 'task868_cfq_mcd1_explanation_to_sql', 'task868_mawps_singleop_question_answering', 'task869_cfq_mcd1_sql_to_explanation', 'task870_msmarco_answer_generation', 'task871_msmarco_question_generation', 'task874_opus_xhosanavy_sr', 'task875_emotion_classification', 'task886_quail_question_generation', 'task887_quail_answer_generation', 'task888_reviews_classification', 'task889_goemotions_classification', 'task897_freebase_qa_topic_question_generation', 'task898_freebase_qa_answer_generation', 'task899_freebase_qa_topic_generation', 'task900_freebase_qa_category_classification', 'task901_freebase_qa_category_question_generation', 'task902_deceptive_opinion_spam_classification', 'task903_deceptive_opinion_spam_classification', 'task904_hate_speech_offensive_classification', 'task905_hate_speech_offensive_classification', 'task906_dialogre_identify_names', 'task907_dialogre_identify_relationships', 'task908_dialogre_identify_familial_relationships', 'task909_dialogre_prevalent_speakers', 'task917_coqa_question_generation', 'task918_coqa_answer_generation', 'task919_coqa_incorrect_answer_generation', 'task921_code_x_glue_information_retreival', 'task922_event2mind_word_generation', 'task923_event2mind_classifier', 'task924_event2mind_word_generation', 'task925_coached_conv_pref_classifier', 'task926_coached_conv_pref_word_generation', 'task927_yelp_negative_to_positive_style_transfer', 'task928_yelp_positive_to_negative_style_transfer', 'task929_products_reviews_classification', 'task933_wiki_auto_style_transfer', 'task934_turk_simplification', 'task955_wiki_auto_style_transfer', 'task956_leetcode_420_strong_password_check', 'task963_librispeech_asr_next_word_prediction', 'task964_librispeech_asr_text_auto_completion', 'task965_librispeech_asr_missing_word_prediction', 'task966_ruletaker_fact_checking_based_on_given_context', 'task967_ruletaker_incorrect_fact_generation_based_on_given_paragraph']
```
Validation Tasks:
```
['task1333_check_validity_date_ddmmyyyy', 'task1403_check_validity_date_mmddyyyy', 'task291_semeval_2020_task4_commonsense_validation']
```
Test Tasks:
```
['task020_mctaco_span_based_question', 'task033_winogrande_answer_generation', 'task034_winogrande_question_modification_object', 'task035_winogrande_question_modification_person', 'task036_qasc_topic_word_to_generate_related_fact', 'task039_qasc_find_overlapping_words', 'task050_multirc_answerability', 'task102_commongen_sentence_generation', 'task104_semeval_2019_task10_closed_vocabulary_mathematical_answer_generation', 'task1152_bard_analogical_reasoning_causation', 'task1153_bard_analogical_reasoning_affordance', 'task1154_bard_analogical_reasoning_travel', 'task1155_bard_analogical_reasoning_trash_or_treasure', 'task1156_bard_analogical_reasoning_tools', 'task1157_bard_analogical_reasoning_rooms_for_containers', 'task1158_bard_analogical_reasoning_manipulating_items', 'task1159_bard_analogical_reasoning_containers', 'task1161_coda19_title_generation', 'task118_semeval_2019_task10_open_vocabulary_mathematical_answer_generation', 'task1195_disflqa_disfluent_to_fluent_conversion', 'task119_semeval_2019_task10_geometric_mathematical_answer_generation', 'task121_zest_text_modification', 'task1336_peixian_equity_evaluation_corpus_gender_classifier', 'task1338_peixian_equity_evaluation_corpus_sentiment_classifier', 'task1339_peixian_equity_evaluation_corpus_text_completion', 'task133_winowhy_reason_plausibility_detection', 'task1342_amazon_us_reviews_title', 'task1344_glue_entailment_classification', 'task1345_glue_qqp_question_paraprashing', 'task1356_xlsum_title_generation', 'task1358_xlsum_title_generation', 'task1385_anli_r1_entailment', 'task1386_anli_r2_entailment', 'task1387_anli_r3_entailment', 'task1388_cb_entailment', 'task1390_wscfixed_coreference', 'task1391_winogrande_easy_answer_generation', 'task1393_superglue_copa_text_completion', 'task1394_meta_woz_task_classification', 'task1407_dart_question_generation', 'task1409_dart_text_generation', 'task1429_evalution_semantic_relation_classification', 'task1439_doqa_cooking_isanswerable', 'task1442_doqa_movies_isanswerable', 'task1509_evalution_antonyms', 'task1510_evalution_relation_extraction', 'task1516_imppres_naturallanguageinference', 'task1529_scitail1.1_classification', 'task1531_daily_dialog_type_classification', 'task1533_daily_dialog_formal_classification', 'task1534_daily_dialog_question_classification', 'task1540_parsed_pdfs_summarization', 'task1554_scitail_classification', 'task1557_jfleg_answer_generation', 'task1562_zest_text_modification', 'task1584_evalution_meronym_classification', 'task1586_scifact_title_generation', 'task1598_nyc_long_text_generation', 'task1612_sick_label_classification', 'task1615_sick_tclassify_b_relation_a', 'task1622_disfl_qa_text_modication', 'task1624_disfl_qa_question_yesno_classification', 'task1631_openpi_answer_generation', 'task1640_aqa1.0_answerable_unanswerable_question_classification', 'task1659_title_generation', 'task1664_winobias_text_generation', 'task1728_web_nlg_data_to_text', 'task190_snli_classification', 'task199_mnli_classification', 'task200_mnli_entailment_classification', 'task201_mnli_neutral_classification', 'task202_mnli_contradiction_classification', 'task219_rocstories_title_answer_generation', 'task220_rocstories_title_classification', 'task226_english_language_answer_relevance_classification', 'task232_iirc_link_number_classification', 'task233_iirc_link_exists_classification', 'task242_tweetqa_classification', 'task249_enhanced_wsc_pronoun_disambiguation', 'task281_points_of_correspondence', 'task288_gigaword_summarization', 'task290_tellmewhy_question_answerability', 'task291_semeval_2020_task4_commonsense_validation', 'task295_semeval_2020_task4_commonsense_reasoning', 'task304_numeric_fused_head_resolution', 'task329_gap_classification', 'task330_gap_answer_generation', 'task333_hateeval_classification_hate_en', 'task335_hateeval_classification_aggresive_en', 'task337_hateeval_classification_individual_en', 'task349_squad2.0_answerable_unanswerable_question_classification', 'task362_spolin_yesand_prompt_response_sub_classification', 'task386_semeval_2018_task3_irony_detection', 'task387_semeval_2018_task3_irony_classification', 'task391_causal_relationship', 'task392_inverse_causal_relationship', 'task393_plausible_result_generation', 'task397_semeval_2018_task1_tweet_anger_detection', 'task398_semeval_2018_task1_tweet_joy_detection', 'task399_semeval_2018_task1_tweet_sadness_detection', 'task401_numeric_fused_head_reference', 'task402_grailqa_paraphrase_generation', 'task418_persent_title_generation', 'task428_senteval_inversion', 'task429_senteval_tense', 'task430_senteval_subject_count', 'task431_senteval_object_count', 'task442_com_qa_paraphrase_question_generation', 'task495_semeval_headline_classification', 'task496_semeval_answer_generation', 'task500_scruples_anecdotes_title_generation', 'task510_reddit_tifu_title_summarization', 'task515_senteval_odd_word_out', 'task516_senteval_conjoints_inversion', 'task520_aquamuse_answer_given_in_passage', 'task569_recipe_nlg_text_generation', 'task602_wikitext-103_answer_generation', 'task613_politifact_text_generation', 'task614_glucose_cause_event_detection', 'task619_ohsumed_abstract_title_generation', 'task620_ohsumed_medical_subject_headings_answer_generation', 'task623_ohsumed_yes_no_answer_generation', 'task640_esnli_classification', 'task641_esnli_classification', 'task642_esnli_classification', 'task645_summarization', 'task648_answer_generation', 'task670_ambigqa_question_generation', 'task671_ambigqa_text_generation', 'task677_ollie_sentence_answer_generation', 'task738_perspectrum_classification', 'task743_eurlex_summarization', 'task760_msr_sqa_long_text_generation', 'task769_qed_summarization', 'task827_copa_commonsense_reasoning', 'task828_copa_commonsense_cause_effect', 'task879_schema_guided_dstc8_classification', 'task880_schema_guided_dstc8_classification', 'task890_gcwd_classification', 'task891_gap_coreference_resolution', 'task892_gap_reverse_coreference_resolution', 'task893_gap_fill_the_blank_coreference_resolution', 'task909_dialogre_prevalent_speakers', 'task935_defeasible_nli_atomic_classification', 'task936_defeasible_nli_snli_classification', 'task937_defeasible_nli_social_classification', 'task957_e2e_nlg_text_generation_generate', 'task970_sherliic_causal_relationship']
``` | Muennighoff/natural-instructions | [
"task_categories:other",
"annotations_creators:crowdsourced",
"annotations_creators:expert-generated",
"multilinguality:monolingual",
"size_categories:100M<n<1B",
"language:en",
"region:us"
] | 2022-12-17T21:45:01+00:00 | {"annotations_creators": ["crowdsourced", "expert-generated"], "language": ["en"], "multilinguality": ["monolingual"], "size_categories": ["100M<n<1B"], "task_categories": ["other"]} | 2022-12-23T20:08:44+00:00 | [] | [
"en"
] | TAGS
#task_categories-other #annotations_creators-crowdsourced #annotations_creators-expert-generated #multilinguality-monolingual #size_categories-100M<n<1B #language-English #region-us
| Preprocessed version of Super-Natural-Instructions from URL The same inputs may appear with different outputs, thus to avoid duplicate inputs, you can deduplicate by the 'id' or the 'inputs' field.
Train Tasks:
Validation Tasks:
Test Tasks:
| [] | [
"TAGS\n#task_categories-other #annotations_creators-crowdsourced #annotations_creators-expert-generated #multilinguality-monolingual #size_categories-100M<n<1B #language-English #region-us \n"
] |
af494fe1b62762178d37c0b71b4a7160f0534f1a |
# Dataset Card for "squad_v2_dutch"
## Dataset Description
- **Homepage:** [https://rajpurkar.github.io/SQuAD-explorer/](https://rajpurkar.github.io/SQuAD-explorer/)
## Dataset Summary
The squad_v2_dutch dataset is a machine-translated version of the SQuAD v2 dataset from English to Dutch.
The SQuAD v2 dataset combines the 100,000 questions in SQuAD1.1 with over 50,000 unanswerable questions written adversarially by crowdworkers
to look similar to answerable ones. To do well on SQuAD2.0, systems must not only answer questions when possible, but
also determine when no answer is supported by the paragraph and abstain from answering.
## Challenges and Solutions
One of the main challenges in translating the SQuAD v2 dataset to Dutch was accurately translating the answers, which are often short phrases or single words.
Translating the answers individually would result in obvious mistakes. Examples are
* Destiny's Child -> Het kind van Destiny
* Dangerously in Love -> Gevaarlijk in de liefde
* Imagine -> Stel je voor
* Men in Black -> Mannen in zwart
* Hottest Female Singer of All Time -> De heetste vrouwelijke zanger aller tijden
The correct translation of these phrases often depends on the context in which they are used.
To address this, the title, question, answers, and context were concatenated as a single sequence, separated by the newline character.
When the translated version had the correct number of newlines and did not contain any apparent mixups of the answers with the question and title, it was used.
Otherwise, the one-by-one context-less translation was used as a fallback.
Most examples where translated with the context-rich translation: ~95%.
* train split: context: 123898, no context: 6406
* validation split: context: 10196, no context: 1644
### Data Fields
The data fields are the same among all splits.
#### squad_v2
- `id`: a `string` feature.
- `title`: a `string` feature.
- `title_en`: a `string` feature.
- `context`: a `string` feature.
- `question`: a `string` feature.
- `answers`: a dictionary feature containing:
- `text`: a list of `string` feature.
- `text_en`: a list of `string` feature.
- `answer_start_en`: a `int32` feature.
### Citation Information
```
@article{2016arXiv160605250R,
author = {{Rajpurkar}, Pranav and {Zhang}, Jian and {Lopyrev},
Konstantin and {Liang}, Percy},
title = "{SQuAD: 100,000+ Questions for Machine Comprehension of Text}",
journal = {arXiv e-prints},
year = 2016,
eid = {arXiv:1606.05250},
pages = {arXiv:1606.05250},
archivePrefix = {arXiv},
eprint = {1606.05250},
}
```
### Contributions
Thanks to [@lewtun](https://github.com/lewtun), [@albertvillanova](https://github.com/albertvillanova), [@patrickvonplaten](https://github.com/patrickvonplaten),
[@thomwolf](https://github.com/thomwolf) for adding the https://huggingface.co/datasets/squad_v2 dataset.
This project would not have been possible without compute generously provided by Google through the
[TPU Research Cloud](https://sites.research.google/trc/).
Created by [Yeb Havinga](https://www.linkedin.com/in/yeb-havinga-86530825/)
| yhavinga/squad_v2_dutch | [
"task_categories:question-answering",
"task_ids:open-domain-qa",
"task_ids:extractive-qa",
"annotations_creators:crowdsourced",
"language_creators:crowdsourced",
"multilinguality:monolingual",
"size_categories:100K<n<1M",
"source_datasets:original",
"language:nl",
"license:cc-by-sa-4.0",
"arxiv:1606.05250",
"region:us"
] | 2022-12-17T22:50:45+00:00 | {"annotations_creators": ["crowdsourced"], "language_creators": ["crowdsourced"], "language": ["nl"], "license": ["cc-by-sa-4.0"], "multilinguality": ["monolingual"], "size_categories": ["100K<n<1M"], "source_datasets": ["original"], "task_categories": ["question-answering"], "task_ids": ["open-domain-qa", "extractive-qa"], "paperswithcode_id": "squad_v2_dutch", "pretty_name": "SQuAD2.0 Dutch", "dataset_info": {"features": [{"name": "id", "dtype": "string"}, {"name": "title", "dtype": "string"}, {"name": "title_en", "dtype": "string"}, {"name": "context", "dtype": "string"}, {"name": "question", "dtype": "string"}, {"name": "answers", "sequence": [{"name": "text", "dtype": "string"}, {"name": "text_en", "dtype": "string"}, {"name": "answer_start_en", "dtype": "int32"}]}]}} | 2023-01-21T13:53:27+00:00 | [
"1606.05250"
] | [
"nl"
] | TAGS
#task_categories-question-answering #task_ids-open-domain-qa #task_ids-extractive-qa #annotations_creators-crowdsourced #language_creators-crowdsourced #multilinguality-monolingual #size_categories-100K<n<1M #source_datasets-original #language-Dutch #license-cc-by-sa-4.0 #arxiv-1606.05250 #region-us
|
# Dataset Card for "squad_v2_dutch"
## Dataset Description
- Homepage: URL
## Dataset Summary
The squad_v2_dutch dataset is a machine-translated version of the SQuAD v2 dataset from English to Dutch.
The SQuAD v2 dataset combines the 100,000 questions in SQuAD1.1 with over 50,000 unanswerable questions written adversarially by crowdworkers
to look similar to answerable ones. To do well on SQuAD2.0, systems must not only answer questions when possible, but
also determine when no answer is supported by the paragraph and abstain from answering.
## Challenges and Solutions
One of the main challenges in translating the SQuAD v2 dataset to Dutch was accurately translating the answers, which are often short phrases or single words.
Translating the answers individually would result in obvious mistakes. Examples are
* Destiny's Child -> Het kind van Destiny
* Dangerously in Love -> Gevaarlijk in de liefde
* Imagine -> Stel je voor
* Men in Black -> Mannen in zwart
* Hottest Female Singer of All Time -> De heetste vrouwelijke zanger aller tijden
The correct translation of these phrases often depends on the context in which they are used.
To address this, the title, question, answers, and context were concatenated as a single sequence, separated by the newline character.
When the translated version had the correct number of newlines and did not contain any apparent mixups of the answers with the question and title, it was used.
Otherwise, the one-by-one context-less translation was used as a fallback.
Most examples where translated with the context-rich translation: ~95%.
* train split: context: 123898, no context: 6406
* validation split: context: 10196, no context: 1644
### Data Fields
The data fields are the same among all splits.
#### squad_v2
- 'id': a 'string' feature.
- 'title': a 'string' feature.
- 'title_en': a 'string' feature.
- 'context': a 'string' feature.
- 'question': a 'string' feature.
- 'answers': a dictionary feature containing:
- 'text': a list of 'string' feature.
- 'text_en': a list of 'string' feature.
- 'answer_start_en': a 'int32' feature.
### Contributions
Thanks to @lewtun, @albertvillanova, @patrickvonplaten,
@thomwolf for adding the URL dataset.
This project would not have been possible without compute generously provided by Google through the
TPU Research Cloud.
Created by Yeb Havinga
| [
"# Dataset Card for \"squad_v2_dutch\"",
"## Dataset Description\n\n- Homepage: URL",
"## Dataset Summary\n\nThe squad_v2_dutch dataset is a machine-translated version of the SQuAD v2 dataset from English to Dutch.\nThe SQuAD v2 dataset combines the 100,000 questions in SQuAD1.1 with over 50,000 unanswerable questions written adversarially by crowdworkers\nto look similar to answerable ones. To do well on SQuAD2.0, systems must not only answer questions when possible, but\nalso determine when no answer is supported by the paragraph and abstain from answering.",
"## Challenges and Solutions\nOne of the main challenges in translating the SQuAD v2 dataset to Dutch was accurately translating the answers, which are often short phrases or single words.\nTranslating the answers individually would result in obvious mistakes. Examples are\n\n* Destiny's Child -> Het kind van Destiny\n* Dangerously in Love -> Gevaarlijk in de liefde\n* Imagine -> Stel je voor\n* Men in Black -> Mannen in zwart\n* Hottest Female Singer of All Time -> De heetste vrouwelijke zanger aller tijden\n\nThe correct translation of these phrases often depends on the context in which they are used.\nTo address this, the title, question, answers, and context were concatenated as a single sequence, separated by the newline character.\nWhen the translated version had the correct number of newlines and did not contain any apparent mixups of the answers with the question and title, it was used.\nOtherwise, the one-by-one context-less translation was used as a fallback.\n\nMost examples where translated with the context-rich translation: ~95%.\n* train split: context: 123898, no context: 6406\n* validation split: context: 10196, no context: 1644",
"### Data Fields\n\nThe data fields are the same among all splits.",
"#### squad_v2\n- 'id': a 'string' feature.\n- 'title': a 'string' feature.\n- 'title_en': a 'string' feature.\n- 'context': a 'string' feature.\n- 'question': a 'string' feature.\n- 'answers': a dictionary feature containing:\n - 'text': a list of 'string' feature.\n - 'text_en': a list of 'string' feature.\n - 'answer_start_en': a 'int32' feature.",
"### Contributions\n\nThanks to @lewtun, @albertvillanova, @patrickvonplaten,\n@thomwolf for adding the URL dataset.\nThis project would not have been possible without compute generously provided by Google through the\nTPU Research Cloud.\n\nCreated by Yeb Havinga"
] | [
"TAGS\n#task_categories-question-answering #task_ids-open-domain-qa #task_ids-extractive-qa #annotations_creators-crowdsourced #language_creators-crowdsourced #multilinguality-monolingual #size_categories-100K<n<1M #source_datasets-original #language-Dutch #license-cc-by-sa-4.0 #arxiv-1606.05250 #region-us \n",
"# Dataset Card for \"squad_v2_dutch\"",
"## Dataset Description\n\n- Homepage: URL",
"## Dataset Summary\n\nThe squad_v2_dutch dataset is a machine-translated version of the SQuAD v2 dataset from English to Dutch.\nThe SQuAD v2 dataset combines the 100,000 questions in SQuAD1.1 with over 50,000 unanswerable questions written adversarially by crowdworkers\nto look similar to answerable ones. To do well on SQuAD2.0, systems must not only answer questions when possible, but\nalso determine when no answer is supported by the paragraph and abstain from answering.",
"## Challenges and Solutions\nOne of the main challenges in translating the SQuAD v2 dataset to Dutch was accurately translating the answers, which are often short phrases or single words.\nTranslating the answers individually would result in obvious mistakes. Examples are\n\n* Destiny's Child -> Het kind van Destiny\n* Dangerously in Love -> Gevaarlijk in de liefde\n* Imagine -> Stel je voor\n* Men in Black -> Mannen in zwart\n* Hottest Female Singer of All Time -> De heetste vrouwelijke zanger aller tijden\n\nThe correct translation of these phrases often depends on the context in which they are used.\nTo address this, the title, question, answers, and context were concatenated as a single sequence, separated by the newline character.\nWhen the translated version had the correct number of newlines and did not contain any apparent mixups of the answers with the question and title, it was used.\nOtherwise, the one-by-one context-less translation was used as a fallback.\n\nMost examples where translated with the context-rich translation: ~95%.\n* train split: context: 123898, no context: 6406\n* validation split: context: 10196, no context: 1644",
"### Data Fields\n\nThe data fields are the same among all splits.",
"#### squad_v2\n- 'id': a 'string' feature.\n- 'title': a 'string' feature.\n- 'title_en': a 'string' feature.\n- 'context': a 'string' feature.\n- 'question': a 'string' feature.\n- 'answers': a dictionary feature containing:\n - 'text': a list of 'string' feature.\n - 'text_en': a list of 'string' feature.\n - 'answer_start_en': a 'int32' feature.",
"### Contributions\n\nThanks to @lewtun, @albertvillanova, @patrickvonplaten,\n@thomwolf for adding the URL dataset.\nThis project would not have been possible without compute generously provided by Google through the\nTPU Research Cloud.\n\nCreated by Yeb Havinga"
] |
c47ace8e29d2712c22ddb223653b377f4175b2da | # Quick!Draw! 1pct Sample (per-row bin format)
This is a sample 1-percent of the entire 50M-row [QuickDraw! dataset](https://github.com/googlecreativelab/quickdraw-dataset). The row for each drawing contains a byte-encoded packed representation of the drawing and data, which you can unpack using the following snippet:
```
def unpack_drawing(file_handle):
key_id, = unpack('Q', file_handle.read(8))
country_code, = unpack('2s', file_handle.read(2))
recognized, = unpack('b', file_handle.read(1))
timestamp, = unpack('I', file_handle.read(4))
n_strokes, = unpack('H', file_handle.read(2))
image = []
n_bytes = 17
for i in range(n_strokes):
n_points, = unpack('H', file_handle.read(2))
fmt = str(n_points) + 'B'
x = unpack(fmt, file_handle.read(n_points))
y = unpack(fmt, file_handle.read(n_points))
image.append((x, y))
n_bytes += 2 + 2*n_points
result = {
'key_id': key_id,
'country_code': country_code,
'recognized': recognized,
'timestamp': timestamp,
'image': image,
}
return result
```
The `image` in the above is still in line vector format. To convert render this to a raster image (I recommend you do this on-the-fly in a pre-processor):
```
# packed bin -> RGB PIL
def binToPIL(packed_drawing):
padding = 8
radius = 7
scale = (224.0-(2*padding)) / 256
unpacked = unpack_drawing(io.BytesIO(packed_drawing))
unpacked_image = unpacked['image']
image = np.full((224,224), 255, np.uint8)
for stroke in unpacked['image']:
prevX = round(stroke[0][0]*scale)
prevY = round(stroke[1][0]*scale)
for i in range(1, len(stroke[0])):
x = round(stroke[0][i]*scale)
y = round(stroke[1][i]*scale)
cv2.line(image, (padding+prevX, padding+prevY), (padding+x, padding+y), 0, radius, -1)
prevX = x
prevY = y
pilImage = Image.fromarray(image).convert("RGB")
return pilImage
``` | kmewhort/quickdraw-bins-1pct-sample | [
"region:us"
] | 2022-12-18T02:37:21+00:00 | {"dataset_info": {"features": [{"name": "label", "dtype": {"class_label": {"names": {"0": "The Eiffel Tower", "1": "The Great Wall of China", "2": "The Mona Lisa", "3": "aircraft carrier", "4": "airplane", "5": "alarm clock", "6": "ambulance", "7": "angel", "8": "animal migration", "9": "ant", "10": "anvil", "11": "apple", "12": "arm", "13": "asparagus", "14": "axe", "15": "backpack", "16": "banana", "17": "bandage", "18": "barn", "19": "baseball", "20": "baseball bat", "21": "basket", "22": "basketball", "23": "bat", "24": "bathtub", "25": "beach", "26": "bear", "27": "beard", "28": "bed", "29": "bee", "30": "belt", "31": "bench", "32": "bicycle", "33": "binoculars", "34": "bird", "35": "birthday cake", "36": "blackberry", "37": "blueberry", "38": "book", "39": "boomerang", "40": "bottlecap", "41": "bowtie", "42": "bracelet", "43": "brain", "44": "bread", "45": "bridge", "46": "broccoli", "47": "broom", "48": "bucket", "49": "bulldozer", "50": "bus", "51": "bush", "52": "butterfly", "53": "cactus", "54": "cake", "55": "calculator", "56": "calendar", "57": "camel", "58": "camera", "59": "camouflage", "60": "campfire", "61": "candle", "62": "cannon", "63": "canoe", "64": "car", "65": "carrot", "66": "castle", "67": "cat", "68": "ceiling fan", "69": "cell phone", "70": "cello", "71": "chair", "72": "chandelier", "73": "church", "74": "circle", "75": "clarinet", "76": "clock", "77": "cloud", "78": "coffee cup", "79": "compass", "80": "computer", "81": "cookie", "82": "cooler", "83": "couch", "84": "cow", "85": "crab", "86": "crayon", "87": "crocodile", "88": "crown", "89": "cruise ship", "90": "cup", "91": "diamond", "92": "dishwasher", "93": "diving board", "94": "dog", "95": "dolphin", "96": "donut", "97": "door", "98": "dragon", "99": "dresser", "100": "drill", "101": "drums", "102": "duck", "103": "dumbbell", "104": "ear", "105": "elbow", "106": "elephant", "107": "envelope", "108": "eraser", "109": "eye", "110": "eyeglasses", "111": "face", "112": "fan", "113": "feather", "114": "fence", "115": "finger", "116": "fire hydrant", "117": "fireplace", "118": "firetruck", "119": "fish", "120": "flamingo", "121": "flashlight", "122": "flip flops", "123": "floor lamp", "124": "flower", "125": "flying saucer", "126": "foot", "127": "fork", "128": "frog", "129": "frying pan", "130": "garden", "131": "garden hose", "132": "giraffe", "133": "goatee", "134": "golf club", "135": "grapes", "136": "grass", "137": "guitar", "138": "hamburger", "139": "hammer", "140": "hand", "141": "harp", "142": "hat", "143": "headphones", "144": "hedgehog", "145": "helicopter", "146": "helmet", "147": "hexagon", "148": "hockey puck", "149": "hockey stick", "150": "horse", "151": "hospital", "152": "hot air balloon", "153": "hot dog", "154": "hot tub", "155": "hourglass", "156": "house", "157": "house plant", "158": "hurricane", "159": "ice cream", "160": "jacket", "161": "jail", "162": "kangaroo", "163": "key", "164": "keyboard", "165": "knee", "166": "knife", "167": "ladder", "168": "lantern", "169": "laptop", "170": "leaf", "171": "leg", "172": "light bulb", "173": "lighter", "174": "lighthouse", "175": "lightning", "176": "line", "177": "lion", "178": "lipstick", "179": "lobster", "180": "lollipop", "181": "mailbox", "182": "map", "183": "marker", "184": "matches", "185": "megaphone", "186": "mermaid", "187": "microphone", "188": "microwave", "189": "monkey", "190": "moon", "191": "mosquito", "192": "motorbike", "193": "mountain", "194": "mouse", "195": "moustache", "196": "mouth", "197": "mug", "198": "mushroom", "199": "nail", "200": "necklace", "201": "nose", "202": "ocean", "203": "octagon", "204": "octopus", "205": "onion", "206": "oven", "207": "owl", "208": "paint can", "209": "paintbrush", "210": "palm tree", "211": "panda", "212": "pants", "213": "paper clip", "214": "parachute", "215": "parrot", "216": "passport", "217": "peanut", "218": "pear", "219": "peas", "220": "pencil", "221": "penguin", "222": "piano", "223": "pickup truck", "224": "picture frame", "225": "pig", "226": "pillow", "227": "pineapple", "228": "pizza", "229": "pliers", "230": "police car", "231": "pond", "232": "pool", "233": "popsicle", "234": "postcard", "235": "potato", "236": "power outlet", "237": "purse", "238": "rabbit", "239": "raccoon", "240": "radio", "241": "rain", "242": "rainbow", "243": "rake", "244": "remote control", "245": "rhinoceros", "246": "rifle", "247": "river", "248": "roller coaster", "249": "rollerskates", "250": "sailboat", "251": "sandwich", "252": "saw", "253": "saxophone", "254": "school bus", "255": "scissors", "256": "scorpion", "257": "screwdriver", "258": "sea turtle", "259": "see saw", "260": "shark", "261": "sheep", "262": "shoe", "263": "shorts", "264": "shovel", "265": "sink", "266": "skateboard", "267": "skull", "268": "skyscraper", "269": "sleeping bag", "270": "smiley face", "271": "snail", "272": "snake", "273": "snorkel", "274": "snowflake", "275": "snowman", "276": "soccer ball", "277": "sock", "278": "speedboat", "279": "spider", "280": "spoon", "281": "spreadsheet", "282": "square", "283": "squiggle", "284": "squirrel", "285": "stairs", "286": "star", "287": "steak", "288": "stereo", "289": "stethoscope", "290": "stitches", "291": "stop sign", "292": "stove", "293": "strawberry", "294": "streetlight", "295": "string bean", "296": "submarine", "297": "suitcase", "298": "sun", "299": "swan", "300": "sweater", "301": "swing set", "302": "sword", "303": "syringe", "304": "t-shirt", "305": "table", "306": "teapot", "307": "teddy-bear", "308": "telephone", "309": "television", "310": "tennis racquet", "311": "tent", "312": "tiger", "313": "toaster", "314": "toe", "315": "toilet", "316": "tooth", "317": "toothbrush", "318": "toothpaste", "319": "tornado", "320": "tractor", "321": "traffic light", "322": "train", "323": "tree", "324": "triangle", "325": "trombone", "326": "truck", "327": "trumpet", "328": "umbrella", "329": "underwear", "330": "van", "331": "vase", "332": "violin", "333": "washing machine", "334": "watermelon", "335": "waterslide", "336": "whale", "337": "wheel", "338": "windmill", "339": "wine bottle", "340": "wine glass", "341": "wristwatch", "342": "yoga", "343": "zebra", "344": "zigzag"}}}}, {"name": "packed_drawing", "dtype": "binary"}], "splits": [{"name": "train", "num_bytes": 51960652.42514169, "num_examples": 403410}, {"name": "test", "num_bytes": 12990227.508075692, "num_examples": 100853}], "download_size": 62877590, "dataset_size": 64950879.933217384}} | 2022-12-19T15:09:12+00:00 | [] | [] | TAGS
#region-us
| # Quick!Draw! 1pct Sample (per-row bin format)
This is a sample 1-percent of the entire 50M-row QuickDraw! dataset. The row for each drawing contains a byte-encoded packed representation of the drawing and data, which you can unpack using the following snippet:
The 'image' in the above is still in line vector format. To convert render this to a raster image (I recommend you do this on-the-fly in a pre-processor):
| [
"# Quick!Draw! 1pct Sample (per-row bin format)\n\nThis is a sample 1-percent of the entire 50M-row QuickDraw! dataset. The row for each drawing contains a byte-encoded packed representation of the drawing and data, which you can unpack using the following snippet:\n\n\n\nThe 'image' in the above is still in line vector format. To convert render this to a raster image (I recommend you do this on-the-fly in a pre-processor):"
] | [
"TAGS\n#region-us \n",
"# Quick!Draw! 1pct Sample (per-row bin format)\n\nThis is a sample 1-percent of the entire 50M-row QuickDraw! dataset. The row for each drawing contains a byte-encoded packed representation of the drawing and data, which you can unpack using the following snippet:\n\n\n\nThe 'image' in the above is still in line vector format. To convert render this to a raster image (I recommend you do this on-the-fly in a pre-processor):"
] |
e63637e4ae28e74c8a2d4da90809764ce104428d | # AutoTrain Dataset for project: honor
## Dataset Description
This dataset has been automatically processed by AutoTrain for project honor.
### Languages
The BCP-47 code for the dataset's language is en.
## Dataset Structure
### Data Instances
A sample from this dataset looks as follows:
```json
[
{
"text": "\"Kimchi (kimchee) is a Korean dish which is well known throughout the world. It is a spicy, tangy and pungent food that contains pickled vegetables. The word \"Kimchi\" comes from the Korean \"Kim\" meaning \"turn\" and \"Chi\" meaning \"sauce\".\\n\\nKimchi consists of vegetables which are salted, fermented and seasoned. It is an important part of the Korean diet. The two main methods of preparing Kimchi are fermentation and salting. Fermented Kimchi is made by mixing cabbage, radish and other vegetables with a specific kind of salt and sugar. Salted Kimchi is made by mixing cabbage, radish and other vegetables with a specific amount of salt and some vinegar.\\n\\nThe standard vegetables used in preparing Kimchi include cabbage, radish, turnip and Chinese cabbage. However, there are many different variations of Kimchi. Some of the variations include Kimchi with beef, Kimchi with fish and Kimchi with soybean paste.\\n\\nThe preparation of Kimchi is considered to be an important part of Korean culture. It is prepared in a ritualistic manner. The Korean culture also consider it as a \"doorway\" to a family's hearth.",
"target": 1,
"feat_meta.pile_set_name": "GPT-3"
},
{
"text": "So how did you survive the terrible British summer of 2015? (Mine was miserable. There were too many weekends at home in the garden, that's all I can say.) Well, it's a new year and a new season of Doctor Who, with Peter Capaldi as our time-travelling hero.\\n\\nHere's the first photo of Capaldi in costume:\\n\\nAnd here's how it all begins...\\n\\nThis story is called The Magician's Apprentice and features Missy (the Master, if you didn't know).\\n\\nAnd here's a trailer:\\n\\nAll we can say is: A spooky church? The Doctor having to answer questions about his mistakes? Yes, please! We can't wait to see more.\\n\\nDoctor Who series 9 begins on Saturday 19 September on BBC One.",
"target": 1,
"feat_meta.pile_set_name": "GPT-3"
}
]
```
### Dataset Fields
The dataset has the following fields (also called "features"):
```json
{
"text": "Value(dtype='string', id=None)",
"target": "ClassLabel(names=['human', 'machine'], id=None)",
"feat_meta.pile_set_name": "Value(dtype='string', id=None)"
}
```
### Dataset Splits
This dataset is split into a train and validation split. The split sizes are as follow:
| Split name | Num samples |
| ------------ | ------------------- |
| train | 3212 |
| valid | 804 |
| freddiezhang/honordata | [
"task_categories:text-classification",
"language:en",
"region:us"
] | 2022-12-18T05:56:24+00:00 | {"language": ["en"], "task_categories": ["text-classification"]} | 2022-12-19T04:48:12+00:00 | [] | [
"en"
] | TAGS
#task_categories-text-classification #language-English #region-us
| AutoTrain Dataset for project: honor
====================================
Dataset Description
-------------------
This dataset has been automatically processed by AutoTrain for project honor.
### Languages
The BCP-47 code for the dataset's language is en.
Dataset Structure
-----------------
### Data Instances
A sample from this dataset looks as follows:
### Dataset Fields
The dataset has the following fields (also called "features"):
### Dataset Splits
This dataset is split into a train and validation split. The split sizes are as follow:
| [
"### Languages\n\n\nThe BCP-47 code for the dataset's language is en.\n\n\nDataset Structure\n-----------------",
"### Data Instances\n\n\nA sample from this dataset looks as follows:",
"### Dataset Fields\n\n\nThe dataset has the following fields (also called \"features\"):",
"### Dataset Splits\n\n\nThis dataset is split into a train and validation split. The split sizes are as follow:"
] | [
"TAGS\n#task_categories-text-classification #language-English #region-us \n",
"### Languages\n\n\nThe BCP-47 code for the dataset's language is en.\n\n\nDataset Structure\n-----------------",
"### Data Instances\n\n\nA sample from this dataset looks as follows:",
"### Dataset Fields\n\n\nThe dataset has the following fields (also called \"features\"):",
"### Dataset Splits\n\n\nThis dataset is split into a train and validation split. The split sizes are as follow:"
] |
49d1f5cd8716bd0780f61bd12caacb48242cd06d |
# Dataset Card for "lmqg/qag_dequad"
## Dataset Description
- **Repository:** [https://github.com/asahi417/lm-question-generation](https://github.com/asahi417/lm-question-generation)
- **Paper:** [https://arxiv.org/abs/2210.03992](https://arxiv.org/abs/2210.03992)
- **Point of Contact:** [Asahi Ushio](http://asahiushio.com/)
### Dataset Summary
This is the question & answer generation dataset based on the DEQuAD.
### Supported Tasks and Leaderboards
* `question-answer-generation`: The dataset is assumed to be used to train a model for question & answer generation.
Success on this task is typically measured by achieving a high BLEU4/METEOR/ROUGE-L/BERTScore/MoverScore (see our paper for more in detail).
### Languages
German (de)
## Dataset Structure
An example of 'train' looks as follows.
```
{
"paragraph": "51._Bundesstaat === District of Columbia === Der District of Columbia gilt neben Puerto Rico als einer der aussichtsreichen Kandidaten für die Anerkennung als Bundesstaat in naher Zukunft. Die Einwohner des Bundesdistrikts gelten als größte Befürworter dieser Entscheidung, die jedoch einer Verfassungsänderung bedürfte. Die Anhänger nutzen das Motto des Unabhängigkeitskrieges in abgewandelter Form – „Taxation without representation“ –, um auf die mangelnde Repräsentation im Kongress hinzuweisen. Das Motto wird heute auf die Nummernschilder neu zugelassener Autos gedruckt (wobei der Fahrer alternativ die Internet-Adresse des D.C. wählen kann). Bill Clintons Präsidenten-Limousine hatte ein solches Nummernschild kurz vor Ende seiner Amtszeit. George W. Bush ließ diese Nummernschilder nach seinem Amtsantritt wieder entfernen. Die kleine ''D.C. Statehood Party'' vertrat diese Ansicht und vereinte sich mit den Grünen zur ''D.C. Statehood Green Party''. 1978 kamen sie ihrem Ziel am nächsten, als der Kongress das ''District of Columbia Voting Rights Amendment'' verabschiedete. Zwei Jahre später beriefen lokale Bürger mit einer Initiative eine konstitutionelle Versammlung für einen neuen Bundesstaat. 1982 ratifizierten die Wähler die Verfassung des Bundesstaates, der ''New Columbia'' heißen sollte. 1985 wurde der Plan jedoch gestoppt, als das Amendment scheiterte, weil es nicht von genug Staaten innerhalb von sieben Jahren ratifiziert wurde. Eine andere Möglichkeit wäre die Rückgliederung des Gebietes in den Bundesstaat Maryland. Damit würden die Einwohner des D.C. in den Genuss der Vorteile kommen, in einem Bundesstaat zu leben, ohne dass ein 51. Bundesstaat geschaffen werden müsste. Am 26. Juni 2020 stimmte das US-Repräsentantenhaus mit 232 zu 180 Stimmen dafür, den District of Columbia als 51. Bundesstaat anzuerkennen. Ein positives Votum des durch die Republikaner dominierten US-Senats gilt als unwahrscheinlich. Außerdem kündigte Präsident Trump sein Veto gegen ein solches, potenzielles Vorhaben an. Dennoch war es das erste positive Votum einer der beiden Kammern des US-Kongresses für eine Anerkennung als Bundesstaat.",
"questions": [ "Was ist das Motto der Befürworter der Anerkennung von District of Columbia als neuer US-Bundesstaat?", "Warum hat die Anerkennung von District of Columbia zu einem neuen US-Bundesstaat 1985 nicht geklappt?", "Was war der potenzielle Name für den neuen US-Bundesstaat anstelle von District of Columbia?", "Aus welchen ehemaligen Parteien bestand die D.C. Statehood Green Party?" ],
"answers": [ "das Motto des Unabhängigkeitskrieges in abgewandelter Form – „Taxation without representation“ ", "weil es nicht von genug Staaten innerhalb von sieben Jahren ratifiziert wurde", " ''New Columbia'' ", "Die kleine ''D.C. Statehood Party'' vertrat diese Ansicht und vereinte sich mit den Grünen" ],
"questions_answers": "question: Was ist das Motto der Befürworter der Anerkennung von District of Columbia als neuer US-Bundesstaat?, answer: das Motto des Unabhängigkeitskrieges in abgewandelter Form – „Taxation without representation“ | question: Warum hat die Anerkennung von District of Columbia zu einem neuen US-Bundesstaat 1985 nicht geklappt?, answer: weil es nicht von genug Staaten innerhalb von sieben Jahren ratifiziert wurde | question: Was war der potenzielle Name für den neuen US-Bundesstaat anstelle von District of Columbia?, answer: ''New Columbia'' | question: Aus welchen ehemaligen Parteien bestand die D.C. Statehood Green Party?, answer: Die kleine ''D.C. Statehood Party'' vertrat diese Ansicht und vereinte sich mit den Grünen"
}
```
The data fields are the same among all splits.
- `questions`: a `list` of `string` features.
- `answers`: a `list` of `string` features.
- `paragraph`: a `string` feature.
- `questions_answers`: a `string` feature.
## Data Splits
|train|validation|test |
|----:|---------:|----:|
|2489 | 1476 | 474 |
## Citation Information
```
@inproceedings{ushio-etal-2022-generative,
title = "{G}enerative {L}anguage {M}odels for {P}aragraph-{L}evel {Q}uestion {G}eneration",
author = "Ushio, Asahi and
Alva-Manchego, Fernando and
Camacho-Collados, Jose",
booktitle = "Proceedings of the 2022 Conference on Empirical Methods in Natural Language Processing",
month = dec,
year = "2022",
address = "Abu Dhabi, U.A.E.",
publisher = "Association for Computational Linguistics",
}
``` | lmqg/qag_dequad | [
"task_categories:text-generation",
"task_ids:language-modeling",
"multilinguality:monolingual",
"size_categories:1k<n<10K",
"source_datasets:lmqg/qg_dequad",
"language:de",
"license:cc-by-sa-4.0",
"question-generation",
"arxiv:2210.03992",
"region:us"
] | 2022-12-18T07:04:59+00:00 | {"language": "de", "license": "cc-by-sa-4.0", "multilinguality": "monolingual", "size_categories": "1k<n<10K", "source_datasets": "lmqg/qg_dequad", "task_categories": ["text-generation"], "task_ids": ["language-modeling"], "pretty_name": "SQuAD for question generation", "tags": ["question-generation"]} | 2022-12-18T08:14:09+00:00 | [
"2210.03992"
] | [
"de"
] | TAGS
#task_categories-text-generation #task_ids-language-modeling #multilinguality-monolingual #size_categories-1k<n<10K #source_datasets-lmqg/qg_dequad #language-German #license-cc-by-sa-4.0 #question-generation #arxiv-2210.03992 #region-us
| Dataset Card for "lmqg/qag\_dequad"
===================================
Dataset Description
-------------------
* Repository: URL
* Paper: URL
* Point of Contact: Asahi Ushio
### Dataset Summary
This is the question & answer generation dataset based on the DEQuAD.
### Supported Tasks and Leaderboards
* 'question-answer-generation': The dataset is assumed to be used to train a model for question & answer generation.
Success on this task is typically measured by achieving a high BLEU4/METEOR/ROUGE-L/BERTScore/MoverScore (see our paper for more in detail).
### Languages
German (de)
Dataset Structure
-----------------
An example of 'train' looks as follows.
The data fields are the same among all splits.
* 'questions': a 'list' of 'string' features.
* 'answers': a 'list' of 'string' features.
* 'paragraph': a 'string' feature.
* 'questions\_answers': a 'string' feature.
Data Splits
-----------
| [
"### Dataset Summary\n\n\nThis is the question & answer generation dataset based on the DEQuAD.",
"### Supported Tasks and Leaderboards\n\n\n* 'question-answer-generation': The dataset is assumed to be used to train a model for question & answer generation.\nSuccess on this task is typically measured by achieving a high BLEU4/METEOR/ROUGE-L/BERTScore/MoverScore (see our paper for more in detail).",
"### Languages\n\n\nGerman (de)\n\n\nDataset Structure\n-----------------\n\n\nAn example of 'train' looks as follows.\n\n\nThe data fields are the same among all splits.\n\n\n* 'questions': a 'list' of 'string' features.\n* 'answers': a 'list' of 'string' features.\n* 'paragraph': a 'string' feature.\n* 'questions\\_answers': a 'string' feature.\n\n\nData Splits\n-----------"
] | [
"TAGS\n#task_categories-text-generation #task_ids-language-modeling #multilinguality-monolingual #size_categories-1k<n<10K #source_datasets-lmqg/qg_dequad #language-German #license-cc-by-sa-4.0 #question-generation #arxiv-2210.03992 #region-us \n",
"### Dataset Summary\n\n\nThis is the question & answer generation dataset based on the DEQuAD.",
"### Supported Tasks and Leaderboards\n\n\n* 'question-answer-generation': The dataset is assumed to be used to train a model for question & answer generation.\nSuccess on this task is typically measured by achieving a high BLEU4/METEOR/ROUGE-L/BERTScore/MoverScore (see our paper for more in detail).",
"### Languages\n\n\nGerman (de)\n\n\nDataset Structure\n-----------------\n\n\nAn example of 'train' looks as follows.\n\n\nThe data fields are the same among all splits.\n\n\n* 'questions': a 'list' of 'string' features.\n* 'answers': a 'list' of 'string' features.\n* 'paragraph': a 'string' feature.\n* 'questions\\_answers': a 'string' feature.\n\n\nData Splits\n-----------"
] |
cc90041343272411122d092d816a2eabb9f8d9d1 |
# Dataset Card for "lmqg/qag_koquad"
## Dataset Description
- **Repository:** [https://github.com/asahi417/lm-question-generation](https://github.com/asahi417/lm-question-generation)
- **Paper:** [https://arxiv.org/abs/2210.03992](https://arxiv.org/abs/2210.03992)
- **Point of Contact:** [Asahi Ushio](http://asahiushio.com/)
### Dataset Summary
This is the question & answer generation dataset based on the KOQuAD.
### Supported Tasks and Leaderboards
* `question-answer-generation`: The dataset is assumed to be used to train a model for question & answer generation.
Success on this task is typically measured by achieving a high BLEU4/METEOR/ROUGE-L/BERTScore/MoverScore (see our paper for more in detail).
### Languages
Korean (ko)
## Dataset Structure
An example of 'train' looks as follows.
```
{
"paragraph": ""3.13 만세운동" 은 1919년 3.13일 전주에서 일어난 만세운동이다. 지역 인사들과 함께 신흥학교 학생들이 주도적인 역할을 하며, 만세운동을 이끌었다. 박태련, 김신극 등 전주 지도자들은 군산에서 4일과 5일 독립만세 시위가 감행됐다는 소식에 듣고 준비하고 있었다. 천도교와 박태련 신간회 총무집에서 필요한 태극기를 인쇄하기로 했었다. 서울을 비롯한 다른 지방에서 시위가 계속되자 일본경찰은 신흥학교와 기전학교를 비롯한 전주시내 학교에 강제 방학조치를 취했다. 이에 최종삼 등 신흥학교 학생 5명은 밤을 이용해 신흥학교 지하실에서 태극기 등 인쇄물을 만들었다. 준비를 마친 이들은 13일 장터로 모이기 시작했고, 채소가마니로 위장한 태극기를 장터로 실어 나르고 거사 직전 시장 입구인 완산동과 전주교 건너편에서 군중들에게 은밀히 배부했다. 낮 12시20분께 신흥학교와 기전학교 학생 및 천도교도 등은 태극기를 들고 만세를 불렀다. 남문 밖 시장, 제2보통학교(현 완산초등학교)에서 모여 인쇄물을 뿌리며 시가지로 구보로 행진했다. 시위는 오후 11시까지 서너차례 계속됐다. 또 다음날 오후 3시에도 군중이 모여 만세를 불렀다. 이후 고형진, 남궁현, 김병학, 김점쇠, 이기곤, 김경신 등 신흥학교 학생들은 시위를 주도했다는 혐의로 모두 실형 1년을 언도 받았다. 이외 신흥학교 학생 3명은 일제의 고문에 옥사한 것으로 알려졌다. 또 시위를 지도한 김인전 목사는 이후 중국 상해로 거처를 옮겨 임시정부에서 활동했다. 현재 신흥학교 교문 옆에 만세운동 기념비가 세워져 있다.",
"questions": [ "만세운동 기념비가 세워져 있는 곳은?", "일본경찰의 강제 방학조치에도 불구하고 학생들은 신흥학교 지하실에 모여서 어떤 인쇄물을 만들었는가?", "여러 지방에서 시위가 일어나자 일본경찰이 전주시내 학교에 감행한 조치는 무엇인가?", "지역인사들과 신흥고등학교 학생들이 주도적인 역할을 한 3.13 만세운동이 일어난 해는?", "신흥학교 학생들은 시위를 주도했다는 혐의로 모두 실형 몇년을 언도 받았는가?", "만세운동에서 주도적인 역할을 한 이들은?", "1919년 3.1 운동이 일어난 지역은 어디인가?", "3.13 만세운동이 일어난 곳은?" ],
"answers": [ "신흥학교 교문 옆", "태극기", "강제 방학조치", "1919년", "1년", "신흥학교 학생들", "전주", "전주" ],
"questions_answers": "question: 만세운동 기념비가 세워져 있는 곳은?, answer: 신흥학교 교문 옆 | question: 일본경찰의 강제 방학조치에도 불구하고 학생들은 신흥학교 지하실에 모여서 어떤 인쇄물을 만들었는가?, answer: 태극기 | question: 여러 지방에서 시위가 일어나자 일본경찰이 전주시내 학교에 감행한 조치는 무엇인가?, answer: 강제 방학조치 | question: 지역인사들과 신흥고등학교 학생들이 주도적인 역할을 한 3.13 만세운동이 일어난 해는?, answer: 1919년 | question: 신흥학교 학생들은 시위를 주도했다는 혐의로 모두 실형 몇년을 언도 받았는가?, answer: 1년 | question: 만세운동에서 주도적인 역할을 한 이들은?, answer: 신흥학교 학생들 | question: 1919년 3.1 운동이 일어난 지역은 어디인가?, answer: 전주 | question: 3.13 만세운동이 일어난 곳은?, answer: 전주"
}
```
The data fields are the same among all splits.
- `questions`: a `list` of `string` features.
- `answers`: a `list` of `string` features.
- `paragraph`: a `string` feature.
- `questions_answers`: a `string` feature.
## Data Splits
|train|validation|test |
|----:|---------:|----:|
|9600 | 960 | 4442|
## Citation Information
```
@inproceedings{ushio-etal-2022-generative,
title = "{G}enerative {L}anguage {M}odels for {P}aragraph-{L}evel {Q}uestion {G}eneration",
author = "Ushio, Asahi and
Alva-Manchego, Fernando and
Camacho-Collados, Jose",
booktitle = "Proceedings of the 2022 Conference on Empirical Methods in Natural Language Processing",
month = dec,
year = "2022",
address = "Abu Dhabi, U.A.E.",
publisher = "Association for Computational Linguistics",
}
``` | lmqg/qag_koquad | [
"task_categories:text-generation",
"task_ids:language-modeling",
"multilinguality:monolingual",
"size_categories:1k<n<10K",
"source_datasets:lmqg/qg_koquad",
"language:ko",
"license:cc-by-sa-4.0",
"question-generation",
"arxiv:2210.03992",
"region:us"
] | 2022-12-18T07:05:17+00:00 | {"language": "ko", "license": "cc-by-sa-4.0", "multilinguality": "monolingual", "size_categories": "1k<n<10K", "source_datasets": "lmqg/qg_koquad", "task_categories": ["text-generation"], "task_ids": ["language-modeling"], "pretty_name": "SQuAD for question generation", "tags": ["question-generation"]} | 2022-12-18T08:03:53+00:00 | [
"2210.03992"
] | [
"ko"
] | TAGS
#task_categories-text-generation #task_ids-language-modeling #multilinguality-monolingual #size_categories-1k<n<10K #source_datasets-lmqg/qg_koquad #language-Korean #license-cc-by-sa-4.0 #question-generation #arxiv-2210.03992 #region-us
| Dataset Card for "lmqg/qag\_koquad"
===================================
Dataset Description
-------------------
* Repository: URL
* Paper: URL
* Point of Contact: Asahi Ushio
### Dataset Summary
This is the question & answer generation dataset based on the KOQuAD.
### Supported Tasks and Leaderboards
* 'question-answer-generation': The dataset is assumed to be used to train a model for question & answer generation.
Success on this task is typically measured by achieving a high BLEU4/METEOR/ROUGE-L/BERTScore/MoverScore (see our paper for more in detail).
### Languages
Korean (ko)
Dataset Structure
-----------------
An example of 'train' looks as follows.
The data fields are the same among all splits.
* 'questions': a 'list' of 'string' features.
* 'answers': a 'list' of 'string' features.
* 'paragraph': a 'string' feature.
* 'questions\_answers': a 'string' feature.
Data Splits
-----------
| [
"### Dataset Summary\n\n\nThis is the question & answer generation dataset based on the KOQuAD.",
"### Supported Tasks and Leaderboards\n\n\n* 'question-answer-generation': The dataset is assumed to be used to train a model for question & answer generation.\nSuccess on this task is typically measured by achieving a high BLEU4/METEOR/ROUGE-L/BERTScore/MoverScore (see our paper for more in detail).",
"### Languages\n\n\nKorean (ko)\n\n\nDataset Structure\n-----------------\n\n\nAn example of 'train' looks as follows.\n\n\nThe data fields are the same among all splits.\n\n\n* 'questions': a 'list' of 'string' features.\n* 'answers': a 'list' of 'string' features.\n* 'paragraph': a 'string' feature.\n* 'questions\\_answers': a 'string' feature.\n\n\nData Splits\n-----------"
] | [
"TAGS\n#task_categories-text-generation #task_ids-language-modeling #multilinguality-monolingual #size_categories-1k<n<10K #source_datasets-lmqg/qg_koquad #language-Korean #license-cc-by-sa-4.0 #question-generation #arxiv-2210.03992 #region-us \n",
"### Dataset Summary\n\n\nThis is the question & answer generation dataset based on the KOQuAD.",
"### Supported Tasks and Leaderboards\n\n\n* 'question-answer-generation': The dataset is assumed to be used to train a model for question & answer generation.\nSuccess on this task is typically measured by achieving a high BLEU4/METEOR/ROUGE-L/BERTScore/MoverScore (see our paper for more in detail).",
"### Languages\n\n\nKorean (ko)\n\n\nDataset Structure\n-----------------\n\n\nAn example of 'train' looks as follows.\n\n\nThe data fields are the same among all splits.\n\n\n* 'questions': a 'list' of 'string' features.\n* 'answers': a 'list' of 'string' features.\n* 'paragraph': a 'string' feature.\n* 'questions\\_answers': a 'string' feature.\n\n\nData Splits\n-----------"
] |
eb342671ce8e5abdbb856c2c06520ff7c3af50fa |
# Dataset Card for "lmqg/qag_jaquad"
## Dataset Description
- **Repository:** [https://github.com/asahi417/lm-question-generation](https://github.com/asahi417/lm-question-generation)
- **Paper:** [https://arxiv.org/abs/2210.03992](https://arxiv.org/abs/2210.03992)
- **Point of Contact:** [Asahi Ushio](http://asahiushio.com/)
### Dataset Summary
This is the question & answer generation dataset based on the JAQuAD.
### Supported Tasks and Leaderboards
* `question-answer-generation`: The dataset is assumed to be used to train a model for question & answer generation.
Success on this task is typically measured by achieving a high BLEU4/METEOR/ROUGE-L/BERTScore/MoverScore (see our paper for more in detail).
### Languages
Japanese (ja)
## Dataset Structure
An example of 'train' looks as follows.
```
{
"paragraph": ""Nerdilinga"は898年にカロリング朝の王領として初めて文献に記録されている。レーゲンスブルク司教の統治下でネルトリンゲンは市場町に成長していった。1215年にネルトリンゲンは皇帝フリードリヒ2世から都市権を与えられ、帝国自由都市となった。この年に最初の市壁が築かれた。その縄張りは現在も街の地図に見て取れる。1219年、ネルトリンゲンの聖霊降臨祭についての最も古い文献上の記録が遺されている。重要な交易路が交差するこの都市は穀物、家畜、織物、毛皮、金属製品の主要な集散地に発展していった。ネルトリンゲンはフランクフルトと並ぶドイツで最も重要な遠距離交易都市の一つとなったのである。",
"questions": [ "1215年にネルトリンゲンは誰から都市権を与えられ、帝国自由都市となったか。", "\"Nerdilinga\"の最初の記録は何年のものですか。" ],
"answers": [ "皇帝フリードリヒ2世", "898年" ],
"questions_answers": "question: 1215年にネルトリンゲンは誰から都市権を与えられ、帝国自由都市となったか。, answer: 皇帝フリードリヒ2世 | question: "Nerdilinga"の最初の記録は何年のものですか。, answer: 898年"
}
```
The data fields are the same among all splits.
- `questions`: a `list` of `string` features.
- `answers`: a `list` of `string` features.
- `paragraph`: a `string` feature.
- `questions_answers`: a `string` feature.
## Data Splits
|train|validation|test |
|----:|---------:|----:|
|9508| 1431 | 3050|
## Citation Information
```
@inproceedings{ushio-etal-2022-generative,
title = "{G}enerative {L}anguage {M}odels for {P}aragraph-{L}evel {Q}uestion {G}eneration",
author = "Ushio, Asahi and
Alva-Manchego, Fernando and
Camacho-Collados, Jose",
booktitle = "Proceedings of the 2022 Conference on Empirical Methods in Natural Language Processing",
month = dec,
year = "2022",
address = "Abu Dhabi, U.A.E.",
publisher = "Association for Computational Linguistics",
}
``` | lmqg/qag_jaquad | [
"task_categories:text-generation",
"task_ids:language-modeling",
"multilinguality:monolingual",
"size_categories:1k<n<10K",
"source_datasets:lmqg/qg_jaquad",
"language:ja",
"license:cc-by-sa-4.0",
"question-generation",
"arxiv:2210.03992",
"region:us"
] | 2022-12-18T07:05:33+00:00 | {"language": "ja", "license": "cc-by-sa-4.0", "multilinguality": "monolingual", "size_categories": "1k<n<10K", "source_datasets": "lmqg/qg_jaquad", "task_categories": ["text-generation"], "task_ids": ["language-modeling"], "pretty_name": "SQuAD for question generation", "tags": ["question-generation"]} | 2022-12-18T07:54:08+00:00 | [
"2210.03992"
] | [
"ja"
] | TAGS
#task_categories-text-generation #task_ids-language-modeling #multilinguality-monolingual #size_categories-1k<n<10K #source_datasets-lmqg/qg_jaquad #language-Japanese #license-cc-by-sa-4.0 #question-generation #arxiv-2210.03992 #region-us
| Dataset Card for "lmqg/qag\_jaquad"
===================================
Dataset Description
-------------------
* Repository: URL
* Paper: URL
* Point of Contact: Asahi Ushio
### Dataset Summary
This is the question & answer generation dataset based on the JAQuAD.
### Supported Tasks and Leaderboards
* 'question-answer-generation': The dataset is assumed to be used to train a model for question & answer generation.
Success on this task is typically measured by achieving a high BLEU4/METEOR/ROUGE-L/BERTScore/MoverScore (see our paper for more in detail).
### Languages
Japanese (ja)
Dataset Structure
-----------------
An example of 'train' looks as follows.
The data fields are the same among all splits.
* 'questions': a 'list' of 'string' features.
* 'answers': a 'list' of 'string' features.
* 'paragraph': a 'string' feature.
* 'questions\_answers': a 'string' feature.
Data Splits
-----------
| [
"### Dataset Summary\n\n\nThis is the question & answer generation dataset based on the JAQuAD.",
"### Supported Tasks and Leaderboards\n\n\n* 'question-answer-generation': The dataset is assumed to be used to train a model for question & answer generation.\nSuccess on this task is typically measured by achieving a high BLEU4/METEOR/ROUGE-L/BERTScore/MoverScore (see our paper for more in detail).",
"### Languages\n\n\nJapanese (ja)\n\n\nDataset Structure\n-----------------\n\n\nAn example of 'train' looks as follows.\n\n\nThe data fields are the same among all splits.\n\n\n* 'questions': a 'list' of 'string' features.\n* 'answers': a 'list' of 'string' features.\n* 'paragraph': a 'string' feature.\n* 'questions\\_answers': a 'string' feature.\n\n\nData Splits\n-----------"
] | [
"TAGS\n#task_categories-text-generation #task_ids-language-modeling #multilinguality-monolingual #size_categories-1k<n<10K #source_datasets-lmqg/qg_jaquad #language-Japanese #license-cc-by-sa-4.0 #question-generation #arxiv-2210.03992 #region-us \n",
"### Dataset Summary\n\n\nThis is the question & answer generation dataset based on the JAQuAD.",
"### Supported Tasks and Leaderboards\n\n\n* 'question-answer-generation': The dataset is assumed to be used to train a model for question & answer generation.\nSuccess on this task is typically measured by achieving a high BLEU4/METEOR/ROUGE-L/BERTScore/MoverScore (see our paper for more in detail).",
"### Languages\n\n\nJapanese (ja)\n\n\nDataset Structure\n-----------------\n\n\nAn example of 'train' looks as follows.\n\n\nThe data fields are the same among all splits.\n\n\n* 'questions': a 'list' of 'string' features.\n* 'answers': a 'list' of 'string' features.\n* 'paragraph': a 'string' feature.\n* 'questions\\_answers': a 'string' feature.\n\n\nData Splits\n-----------"
] |
694439c98de5edcc2bc45eac0dda37b79ed2328e |
# Dataset Card for "lmqg/qag_ruquad"
## Dataset Description
- **Repository:** [https://github.com/asahi417/lm-question-generation](https://github.com/asahi417/lm-question-generation)
- **Paper:** [https://arxiv.org/abs/2210.03992](https://arxiv.org/abs/2210.03992)
- **Point of Contact:** [Asahi Ushio](http://asahiushio.com/)
### Dataset Summary
This is the question & answer generation dataset based on the RUQuAD.
### Supported Tasks and Leaderboards
* `question-answer-generation`: The dataset is assumed to be used to train a model for question & answer generation.
Success on this task is typically measured by achieving a high BLEU4/METEOR/ROUGE-L/BERTScore/MoverScore (see our paper for more in detail).
### Languages
Russian (ru)
## Dataset Structure
An example of 'train' looks as follows.
```
{
"paragraph": " Everybody , как и хотела Мадонна, выпускают синглом. При нулевом бюджете на раскрутку фото певицы решают не помещать на обложке, чтобы не отпугнуть цветную аудиторию якобы негритянской диско-соул-певицы . Everybody поднимается на 3-е место в чарте Hot Dance Club Songs, а потом на 107 место в основном, немного не дотянув до первой сотни Hot 100 журнала Billboard[91]. Менеджмент считает это отличным результатом, учитывая нулевые затраты на пиар, и хочет убедиться, что взлёт Everybody не случаен. По просьбе Мадонны вместо Каминса берут более опытного штатного аранжировщика Warner Bros. Records Регги Лукаса (англ.)русск.. Второй сингл Burning Up тоже достигает в чарте танцевальных хитов 3-го места, повторив успех Everybody . И только после этого Мадонне позволяют арендовать студию для записи первого альбома[91].",
"questions": [ "При каком бюджете на раскрутку фото певицы решают не помещать на обложке ?", "Какой альбом Мадонны выпускают синглом?", "Имя более опытного штатного аранжировщика берут по просьбе Мадонны вместо Каминсаболее ?", "Почему при нулевом бджете фото певицы решают не помещать на обложке ?", "На каое место Everybody поднимается в чарте Hot Dance Club Songs?" ],
"answers": [ "При нулевом", " Everybody ", "Warner Bros", "чтобы не отпугнуть цветную аудиторию якобы негритянской диско-соул-певицы ", "на 3-е место" ],
"questions_answers": "question: При каком бюджете на раскрутку фото певицы решают не помещать на обложке ?, answer: При нулевом | question: Какой альбом Мадонны выпускают синглом?, answer: Everybody | question: Имя более опытного штатного аранжировщика берут по просьбе Мадонны вместо Каминсаболее ?, answer: Warner Bros | question: Почему при нулевом бджете фото певицы решают не помещать на обложке ?, answer: чтобы не отпугнуть цветную аудиторию якобы негритянской диско-соул-певицы | question: На каое место Everybody поднимается в чарте Hot Dance Club Songs?, answer: на 3-е место"
}
```
The data fields are the same among all splits.
- `questions`: a `list` of `string` features.
- `answers`: a `list` of `string` features.
- `paragraph`: a `string` feature.
- `questions_answers`: a `string` feature.
## Data Splits
|train|validation|test |
|----:|---------:|----:|
|10407| 4079 | 4017|
## Citation Information
```
@inproceedings{ushio-etal-2022-generative,
title = "{G}enerative {L}anguage {M}odels for {P}aragraph-{L}evel {Q}uestion {G}eneration",
author = "Ushio, Asahi and
Alva-Manchego, Fernando and
Camacho-Collados, Jose",
booktitle = "Proceedings of the 2022 Conference on Empirical Methods in Natural Language Processing",
month = dec,
year = "2022",
address = "Abu Dhabi, U.A.E.",
publisher = "Association for Computational Linguistics",
}
``` | lmqg/qag_ruquad | [
"task_categories:text-generation",
"task_ids:language-modeling",
"multilinguality:monolingual",
"size_categories:1k<n<10K",
"source_datasets:lmqg/qg_ruquad",
"language:ru",
"license:cc-by-sa-4.0",
"question-generation",
"arxiv:2210.03992",
"region:us"
] | 2022-12-18T07:05:48+00:00 | {"language": "ru", "license": "cc-by-sa-4.0", "multilinguality": "monolingual", "size_categories": "1k<n<10K", "source_datasets": "lmqg/qg_ruquad", "task_categories": ["text-generation"], "task_ids": ["language-modeling"], "pretty_name": "SQuAD for question generation", "tags": ["question-generation"]} | 2022-12-18T07:59:33+00:00 | [
"2210.03992"
] | [
"ru"
] | TAGS
#task_categories-text-generation #task_ids-language-modeling #multilinguality-monolingual #size_categories-1k<n<10K #source_datasets-lmqg/qg_ruquad #language-Russian #license-cc-by-sa-4.0 #question-generation #arxiv-2210.03992 #region-us
| Dataset Card for "lmqg/qag\_ruquad"
===================================
Dataset Description
-------------------
* Repository: URL
* Paper: URL
* Point of Contact: Asahi Ushio
### Dataset Summary
This is the question & answer generation dataset based on the RUQuAD.
### Supported Tasks and Leaderboards
* 'question-answer-generation': The dataset is assumed to be used to train a model for question & answer generation.
Success on this task is typically measured by achieving a high BLEU4/METEOR/ROUGE-L/BERTScore/MoverScore (see our paper for more in detail).
### Languages
Russian (ru)
Dataset Structure
-----------------
An example of 'train' looks as follows.
The data fields are the same among all splits.
* 'questions': a 'list' of 'string' features.
* 'answers': a 'list' of 'string' features.
* 'paragraph': a 'string' feature.
* 'questions\_answers': a 'string' feature.
Data Splits
-----------
| [
"### Dataset Summary\n\n\nThis is the question & answer generation dataset based on the RUQuAD.",
"### Supported Tasks and Leaderboards\n\n\n* 'question-answer-generation': The dataset is assumed to be used to train a model for question & answer generation.\nSuccess on this task is typically measured by achieving a high BLEU4/METEOR/ROUGE-L/BERTScore/MoverScore (see our paper for more in detail).",
"### Languages\n\n\nRussian (ru)\n\n\nDataset Structure\n-----------------\n\n\nAn example of 'train' looks as follows.\n\n\nThe data fields are the same among all splits.\n\n\n* 'questions': a 'list' of 'string' features.\n* 'answers': a 'list' of 'string' features.\n* 'paragraph': a 'string' feature.\n* 'questions\\_answers': a 'string' feature.\n\n\nData Splits\n-----------"
] | [
"TAGS\n#task_categories-text-generation #task_ids-language-modeling #multilinguality-monolingual #size_categories-1k<n<10K #source_datasets-lmqg/qg_ruquad #language-Russian #license-cc-by-sa-4.0 #question-generation #arxiv-2210.03992 #region-us \n",
"### Dataset Summary\n\n\nThis is the question & answer generation dataset based on the RUQuAD.",
"### Supported Tasks and Leaderboards\n\n\n* 'question-answer-generation': The dataset is assumed to be used to train a model for question & answer generation.\nSuccess on this task is typically measured by achieving a high BLEU4/METEOR/ROUGE-L/BERTScore/MoverScore (see our paper for more in detail).",
"### Languages\n\n\nRussian (ru)\n\n\nDataset Structure\n-----------------\n\n\nAn example of 'train' looks as follows.\n\n\nThe data fields are the same among all splits.\n\n\n* 'questions': a 'list' of 'string' features.\n* 'answers': a 'list' of 'string' features.\n* 'paragraph': a 'string' feature.\n* 'questions\\_answers': a 'string' feature.\n\n\nData Splits\n-----------"
] |
7d46cefe86891484d70e0bf81d81a4a0d945d350 |
# Dataset Card for "lmqg/qag_esquad"
## Dataset Description
- **Repository:** [https://github.com/asahi417/lm-question-generation](https://github.com/asahi417/lm-question-generation)
- **Paper:** [https://arxiv.org/abs/2210.03992](https://arxiv.org/abs/2210.03992)
- **Point of Contact:** [Asahi Ushio](http://asahiushio.com/)
### Dataset Summary
This is the question & answer generation dataset based on the ESQuAD.
### Supported Tasks and Leaderboards
* `question-answer-generation`: The dataset is assumed to be used to train a model for question & answer generation.
Success on this task is typically measured by achieving a high BLEU4/METEOR/ROUGE-L/BERTScore/MoverScore (see our paper for more in detail).
### Languages
Spanish (es)
## Dataset Structure
An example of 'train' looks as follows.
```
{
"paragraph": ""4 Minutes" fue lanzado como el primer sencillo del álbum y alcanzó el número tres en el Billboard Hot 100. Fue el 37º hit top-ten de Madonna en la lista, empujando a Madonna más allá de Elvis Presley como el artista con más éxitos entre los diez primeros. En el Reino Unido mantuvo su récord de más sencillos número uno para una artista femenina; "4 Minutes" se convierte en su decimotercera. En el 23 Japan Gold Disc Awards, Madonna recibió su quinto trofeo de Artista del Año de la Recording Industry Association of Japan, la mayor cantidad para cualquier artista. Para promover aún más el álbum, Madonna se embarcó en el Sticky & Sweet Tour; Su primera gran empresa con Live Nation. Con una recaudación de $280 millones, se convirtió en la gira más taquillera de un artista en solitario entonces, superando el récord anterior que Madonna estableció con la gira Confessions Tour; Más tarde fue superado por The Wall Live de Roger Waters. Se amplió al año siguiente, añadiendo nuevas fechas europeas, y después de que terminó, la recaudación total fue de $408 millones.",
"questions": [ "¿Cuál es el nombre de la primera gira con Live Nation?", "4 minutos se convirtió en la canción número uno de Madonna en el Reino Unido.", "¿Cuál sencillo fue lanzado como el primer sencillo del álbum?", "¿Cuánto recaudaron Stick y Sweet Tour?", "Madonna superó a qué artista con más éxitos entre los diez primeros." ],
"answers": [ "Sticky & Sweet Tour", "decimotercera", "\"4 Minute", "$280 millones,", "Elvis Presley" ]
"questions_answers": "question: ¿Cuál es el nombre de la primera gira con Live Nation?, answer: Sticky & Sweet Tour | question: 4 minutos se convirtió en la canción número uno de Madonna en el Reino Unido., answer: decimotercera | question: ¿Cuál sencillo fue lanzado como el primer sencillo del álbum?, answer: "4 Minute | question: ¿Cuánto recaudaron Stick y Sweet Tour?, answer: $280 millones, | question: Madonna superó a qué artista con más éxitos entre los diez primeros., answer: Elvis Presley"
}
```
The data fields are the same among all splits.
- `questions`: a `list` of `string` features.
- `answers`: a `list` of `string` features.
- `paragraph`: a `string` feature.
- `questions_answers`: a `string` feature.
## Data Splits
|train|validation|test |
|----:|---------:|----:|
|18829| 2067 | 8234|
## Citation Information
```
@inproceedings{ushio-etal-2022-generative,
title = "{G}enerative {L}anguage {M}odels for {P}aragraph-{L}evel {Q}uestion {G}eneration",
author = "Ushio, Asahi and
Alva-Manchego, Fernando and
Camacho-Collados, Jose",
booktitle = "Proceedings of the 2022 Conference on Empirical Methods in Natural Language Processing",
month = dec,
year = "2022",
address = "Abu Dhabi, U.A.E.",
publisher = "Association for Computational Linguistics",
}
``` | lmqg/qag_esquad | [
"task_categories:text-generation",
"task_ids:language-modeling",
"multilinguality:monolingual",
"size_categories:1k<n<10K",
"source_datasets:lmqg/qg_esquad",
"language:es",
"license:cc-by-sa-4.0",
"question-generation",
"arxiv:2210.03992",
"region:us"
] | 2022-12-18T07:06:04+00:00 | {"language": "es", "license": "cc-by-sa-4.0", "multilinguality": "monolingual", "size_categories": "1k<n<10K", "source_datasets": "lmqg/qg_esquad", "task_categories": ["text-generation"], "task_ids": ["language-modeling"], "pretty_name": "SQuAD for question generation", "tags": ["question-generation"]} | 2022-12-18T08:01:13+00:00 | [
"2210.03992"
] | [
"es"
] | TAGS
#task_categories-text-generation #task_ids-language-modeling #multilinguality-monolingual #size_categories-1k<n<10K #source_datasets-lmqg/qg_esquad #language-Spanish #license-cc-by-sa-4.0 #question-generation #arxiv-2210.03992 #region-us
| Dataset Card for "lmqg/qag\_esquad"
===================================
Dataset Description
-------------------
* Repository: URL
* Paper: URL
* Point of Contact: Asahi Ushio
### Dataset Summary
This is the question & answer generation dataset based on the ESQuAD.
### Supported Tasks and Leaderboards
* 'question-answer-generation': The dataset is assumed to be used to train a model for question & answer generation.
Success on this task is typically measured by achieving a high BLEU4/METEOR/ROUGE-L/BERTScore/MoverScore (see our paper for more in detail).
### Languages
Spanish (es)
Dataset Structure
-----------------
An example of 'train' looks as follows.
The data fields are the same among all splits.
* 'questions': a 'list' of 'string' features.
* 'answers': a 'list' of 'string' features.
* 'paragraph': a 'string' feature.
* 'questions\_answers': a 'string' feature.
Data Splits
-----------
| [
"### Dataset Summary\n\n\nThis is the question & answer generation dataset based on the ESQuAD.",
"### Supported Tasks and Leaderboards\n\n\n* 'question-answer-generation': The dataset is assumed to be used to train a model for question & answer generation.\nSuccess on this task is typically measured by achieving a high BLEU4/METEOR/ROUGE-L/BERTScore/MoverScore (see our paper for more in detail).",
"### Languages\n\n\nSpanish (es)\n\n\nDataset Structure\n-----------------\n\n\nAn example of 'train' looks as follows.\n\n\nThe data fields are the same among all splits.\n\n\n* 'questions': a 'list' of 'string' features.\n* 'answers': a 'list' of 'string' features.\n* 'paragraph': a 'string' feature.\n* 'questions\\_answers': a 'string' feature.\n\n\nData Splits\n-----------"
] | [
"TAGS\n#task_categories-text-generation #task_ids-language-modeling #multilinguality-monolingual #size_categories-1k<n<10K #source_datasets-lmqg/qg_esquad #language-Spanish #license-cc-by-sa-4.0 #question-generation #arxiv-2210.03992 #region-us \n",
"### Dataset Summary\n\n\nThis is the question & answer generation dataset based on the ESQuAD.",
"### Supported Tasks and Leaderboards\n\n\n* 'question-answer-generation': The dataset is assumed to be used to train a model for question & answer generation.\nSuccess on this task is typically measured by achieving a high BLEU4/METEOR/ROUGE-L/BERTScore/MoverScore (see our paper for more in detail).",
"### Languages\n\n\nSpanish (es)\n\n\nDataset Structure\n-----------------\n\n\nAn example of 'train' looks as follows.\n\n\nThe data fields are the same among all splits.\n\n\n* 'questions': a 'list' of 'string' features.\n* 'answers': a 'list' of 'string' features.\n* 'paragraph': a 'string' feature.\n* 'questions\\_answers': a 'string' feature.\n\n\nData Splits\n-----------"
] |
0460b6ccd0fcc44d904e71fd44a5d5cb43ab71e7 |
# Dataset Card for "lmqg/qag_itquad"
## Dataset Description
- **Repository:** [https://github.com/asahi417/lm-question-generation](https://github.com/asahi417/lm-question-generation)
- **Paper:** [https://arxiv.org/abs/2210.03992](https://arxiv.org/abs/2210.03992)
- **Point of Contact:** [Asahi Ushio](http://asahiushio.com/)
### Dataset Summary
This is the question & answer generation dataset based on the ITQuAD.
### Supported Tasks and Leaderboards
* `question-answer-generation`: The dataset is assumed to be used to train a model for question & answer generation.
Success on this task is typically measured by achieving a high BLEU4/METEOR/ROUGE-L/BERTScore/MoverScore (see our paper for more in detail).
### Languages
Itallian (it)
## Dataset Structure
An example of 'train' looks as follows.
```
{
"paragraph": ""4 Minuti" è uscito come primo singolo dell' album e ha raggiunto il terzo posto sulla Billboard Hot 100. E' stato il 37° top-ten di Madonna che ha spinto Madonna oltre Elvis Presley come l' artista con i più top-ten hit. Nel Regno Unito ha mantenuto il suo record per il più numero uno single per una artista femminile;"4 Minuti" diventando il suo tredicesimo. Al 23° Japan Gold Disc Awards, Madonna ha ricevuto il suo quinto trofeo Artista dell' anno dalla Recording Industry Association of Japan, la più importante per qualsiasi artista. Per promuovere ulteriormente l' album, Madonna ha intrapreso il Sticky & Sweet Tour, la sua prima grande avventura con Live Nation. Con un lordo di 280 milioni di dollari, è diventato il tour più incassato di un artista solista, superando il precedente record di Madonna stabilito con il Confessions Tour; è stato poi superato da The Wall Live di Roger Waters. E' stato esteso al prossimo anno, aggiungendo nuove date europee, e dopo la fine, il totale lordo totale era di 408 milioni di dollari.",
"questions": [ "Qual è il nome del primo tour con Live Nation?", "4 minuti è diventato Madonna's che numero uno nel Regno Unito?", "Quanto ha incassato Stick e Sweet Tour?", "Madonna ha superato l' artista con i più alti dieci colpi?" ],
"answers": [ "Sticky & Sweet Tour", "tredicesimo", "280 milioni di dollari,", "Elvis Presley" ],
"questions_answers": "question: Qual è il nome del primo tour con Live Nation?, answer: Sticky & Sweet Tour | question: 4 minuti è diventato Madonna's che numero uno nel Regno Unito?, answer: tredicesimo | question: Quanto ha incassato Stick e Sweet Tour?, answer: 280 milioni di dollari, | question: Madonna ha superato l' artista con i più alti dieci colpi?, answer: Elvis Presley"
}
```
The data fields are the same among all splits.
- `questions`: a `list` of `string` features.
- `answers`: a `list` of `string` features.
- `paragraph`: a `string` feature.
- `questions_answers`: a `string` feature.
## Data Splits
|train|validation|test |
|----:|---------:|----:|
|16918 | 6280 | 1988|
## Citation Information
```
@inproceedings{ushio-etal-2022-generative,
title = "{G}enerative {L}anguage {M}odels for {P}aragraph-{L}evel {Q}uestion {G}eneration",
author = "Ushio, Asahi and
Alva-Manchego, Fernando and
Camacho-Collados, Jose",
booktitle = "Proceedings of the 2022 Conference on Empirical Methods in Natural Language Processing",
month = dec,
year = "2022",
address = "Abu Dhabi, U.A.E.",
publisher = "Association for Computational Linguistics",
}
``` | lmqg/qag_itquad | [
"task_categories:text-generation",
"task_ids:language-modeling",
"multilinguality:monolingual",
"size_categories:1k<n<10K",
"source_datasets:lmqg/qg_itquad",
"language:it",
"license:cc-by-sa-4.0",
"question-generation",
"arxiv:2210.03992",
"region:us"
] | 2022-12-18T08:05:18+00:00 | {"language": "it", "license": "cc-by-sa-4.0", "multilinguality": "monolingual", "size_categories": "1k<n<10K", "source_datasets": "lmqg/qg_itquad", "task_categories": ["text-generation"], "task_ids": ["language-modeling"], "pretty_name": "SQuAD for question generation", "tags": ["question-generation"]} | 2022-12-18T08:21:31+00:00 | [
"2210.03992"
] | [
"it"
] | TAGS
#task_categories-text-generation #task_ids-language-modeling #multilinguality-monolingual #size_categories-1k<n<10K #source_datasets-lmqg/qg_itquad #language-Italian #license-cc-by-sa-4.0 #question-generation #arxiv-2210.03992 #region-us
| Dataset Card for "lmqg/qag\_itquad"
===================================
Dataset Description
-------------------
* Repository: URL
* Paper: URL
* Point of Contact: Asahi Ushio
### Dataset Summary
This is the question & answer generation dataset based on the ITQuAD.
### Supported Tasks and Leaderboards
* 'question-answer-generation': The dataset is assumed to be used to train a model for question & answer generation.
Success on this task is typically measured by achieving a high BLEU4/METEOR/ROUGE-L/BERTScore/MoverScore (see our paper for more in detail).
### Languages
Itallian (it)
Dataset Structure
-----------------
An example of 'train' looks as follows.
The data fields are the same among all splits.
* 'questions': a 'list' of 'string' features.
* 'answers': a 'list' of 'string' features.
* 'paragraph': a 'string' feature.
* 'questions\_answers': a 'string' feature.
Data Splits
-----------
| [
"### Dataset Summary\n\n\nThis is the question & answer generation dataset based on the ITQuAD.",
"### Supported Tasks and Leaderboards\n\n\n* 'question-answer-generation': The dataset is assumed to be used to train a model for question & answer generation.\nSuccess on this task is typically measured by achieving a high BLEU4/METEOR/ROUGE-L/BERTScore/MoverScore (see our paper for more in detail).",
"### Languages\n\n\nItallian (it)\n\n\nDataset Structure\n-----------------\n\n\nAn example of 'train' looks as follows.\n\n\nThe data fields are the same among all splits.\n\n\n* 'questions': a 'list' of 'string' features.\n* 'answers': a 'list' of 'string' features.\n* 'paragraph': a 'string' feature.\n* 'questions\\_answers': a 'string' feature.\n\n\nData Splits\n-----------"
] | [
"TAGS\n#task_categories-text-generation #task_ids-language-modeling #multilinguality-monolingual #size_categories-1k<n<10K #source_datasets-lmqg/qg_itquad #language-Italian #license-cc-by-sa-4.0 #question-generation #arxiv-2210.03992 #region-us \n",
"### Dataset Summary\n\n\nThis is the question & answer generation dataset based on the ITQuAD.",
"### Supported Tasks and Leaderboards\n\n\n* 'question-answer-generation': The dataset is assumed to be used to train a model for question & answer generation.\nSuccess on this task is typically measured by achieving a high BLEU4/METEOR/ROUGE-L/BERTScore/MoverScore (see our paper for more in detail).",
"### Languages\n\n\nItallian (it)\n\n\nDataset Structure\n-----------------\n\n\nAn example of 'train' looks as follows.\n\n\nThe data fields are the same among all splits.\n\n\n* 'questions': a 'list' of 'string' features.\n* 'answers': a 'list' of 'string' features.\n* 'paragraph': a 'string' feature.\n* 'questions\\_answers': a 'string' feature.\n\n\nData Splits\n-----------"
] |
51fd75a81f7b31a9fed289ec82c6352980854d50 | # Dataset Card for "twentyquestions"
[More Information needed](https://github.com/huggingface/datasets/blob/main/CONTRIBUTING.md#how-to-contribute-to-the-dataset-cards) | maximedb/twentyquestions | [
"region:us"
] | 2022-12-18T09:01:42+00:00 | {"dataset_info": {"features": [{"name": "question", "dtype": "string"}, {"name": "subject", "dtype": "string"}, {"name": "answer", "dtype": "bool"}], "splits": [{"name": "train", "num_bytes": 1658790, "num_examples": 46566}, {"name": "validation", "num_bytes": 548147, "num_examples": 15403}, {"name": "test", "num_bytes": 603112, "num_examples": 16921}], "download_size": 1723095, "dataset_size": 2810049}} | 2022-12-18T09:02:18+00:00 | [] | [] | TAGS
#region-us
| # Dataset Card for "twentyquestions"
More Information needed | [
"# Dataset Card for \"twentyquestions\"\n\nMore Information needed"
] | [
"TAGS\n#region-us \n",
"# Dataset Card for \"twentyquestions\"\n\nMore Information needed"
] |
09d86f82f054236847bb2e15cf254195e6845ffc | *Tập dữ liệu này do bởi Shayo Chủ sở hữu thực hiện*
Sử dụng tập dữ liệu này cho tất cả bot | Shaxo/Shayo_Application | [
"license:apache-2.0",
"region:us"
] | 2022-12-18T09:47:00+00:00 | {"license": "apache-2.0"} | 2022-12-18T12:12:29+00:00 | [] | [] | TAGS
#license-apache-2.0 #region-us
| *Tập dữ liệu này do bởi Shayo Chủ sở hữu thực hiện*
Sử dụng tập dữ liệu này cho tất cả bot | [] | [
"TAGS\n#license-apache-2.0 #region-us \n"
] |
019e9040608fffc3168983d87dffcbd831db427f | This is an attempt! | Gprime1977/AnimeGirlz | [
"region:us"
] | 2022-12-18T11:52:23+00:00 | {} | 2022-12-18T12:43:17+00:00 | [] | [] | TAGS
#region-us
| This is an attempt! | [] | [
"TAGS\n#region-us \n"
] |
26a81ce6c3b7308296dd17406f76a463730ed9af | # Dataset Card for "salvadoran-news-ner"
[More Information needed](https://github.com/huggingface/datasets/blob/main/CONTRIBUTING.md#how-to-contribute-to-the-dataset-cards) | justinian336/salvadoran-news-ner | [
"region:us"
] | 2022-12-18T12:11:41+00:00 | {"dataset_info": {"features": [{"name": "text", "dtype": "string"}], "splits": [{"name": "train", "num_bytes": 73983057.36422747, "num_examples": 56025}], "download_size": 43634286, "dataset_size": 73983057.36422747}} | 2022-12-18T12:11:50+00:00 | [] | [] | TAGS
#region-us
| # Dataset Card for "salvadoran-news-ner"
More Information needed | [
"# Dataset Card for \"salvadoran-news-ner\"\n\nMore Information needed"
] | [
"TAGS\n#region-us \n",
"# Dataset Card for \"salvadoran-news-ner\"\n\nMore Information needed"
] |
ae67baea224991acef61f34fd7a9a958d0e90cc7 | # Dataset Card for "vintage-blip-captions"
[More Information needed](https://github.com/huggingface/datasets/blob/main/CONTRIBUTING.md#how-to-contribute-to-the-dataset-cards) | Norod78/vintage-blip-captions | [
"region:us"
] | 2022-12-18T14:52:31+00:00 | {"dataset_info": {"features": [{"name": "image", "dtype": "image"}, {"name": "text", "dtype": "string"}], "splits": [{"name": "train", "num_bytes": 593662581.43, "num_examples": 1370}], "download_size": 593666132, "dataset_size": 593662581.43}} | 2022-12-20T16:41:56+00:00 | [] | [] | TAGS
#region-us
| # Dataset Card for "vintage-blip-captions"
More Information needed | [
"# Dataset Card for \"vintage-blip-captions\"\n\nMore Information needed"
] | [
"TAGS\n#region-us \n",
"# Dataset Card for \"vintage-blip-captions\"\n\nMore Information needed"
] |
1041b4472ece1b0bda924f90ffeea15ab690fc30 | # Dataset Card for AutoTrain Evaluator
This repository contains model predictions generated by [AutoTrain](https://huggingface.co/autotrain) for the following task and dataset:
* Task: Question Answering
* Model: srcocotero/bert-qa-en
* Dataset: squad
* Config: plain_text
* Split: validation
To run new evaluation jobs, visit Hugging Face's [automatic model evaluator](https://huggingface.co/spaces/autoevaluate/model-evaluator).
## Contributions
Thanks to [@abrar06](https://huggingface.co/abrar06) for evaluating this model. | autoevaluate/autoeval-eval-squad-plain_text-0a0542-2518877374 | [
"autotrain",
"evaluation",
"region:us"
] | 2022-12-18T17:35:11+00:00 | {"type": "predictions", "tags": ["autotrain", "evaluation"], "datasets": ["squad"], "eval_info": {"task": "extractive_question_answering", "model": "srcocotero/bert-qa-en", "metrics": [], "dataset_name": "squad", "dataset_config": "plain_text", "dataset_split": "validation", "col_mapping": {"context": "context", "question": "question", "answers-text": "answers.text", "answers-answer_start": "answers.answer_start"}}} | 2022-12-18T17:38:00+00:00 | [] | [] | TAGS
#autotrain #evaluation #region-us
| # Dataset Card for AutoTrain Evaluator
This repository contains model predictions generated by AutoTrain for the following task and dataset:
* Task: Question Answering
* Model: srcocotero/bert-qa-en
* Dataset: squad
* Config: plain_text
* Split: validation
To run new evaluation jobs, visit Hugging Face's automatic model evaluator.
## Contributions
Thanks to @abrar06 for evaluating this model. | [
"# Dataset Card for AutoTrain Evaluator\n\nThis repository contains model predictions generated by AutoTrain for the following task and dataset:\n\n* Task: Question Answering\n* Model: srcocotero/bert-qa-en\n* Dataset: squad\n* Config: plain_text\n* Split: validation\n\nTo run new evaluation jobs, visit Hugging Face's automatic model evaluator.",
"## Contributions\n\nThanks to @abrar06 for evaluating this model."
] | [
"TAGS\n#autotrain #evaluation #region-us \n",
"# Dataset Card for AutoTrain Evaluator\n\nThis repository contains model predictions generated by AutoTrain for the following task and dataset:\n\n* Task: Question Answering\n* Model: srcocotero/bert-qa-en\n* Dataset: squad\n* Config: plain_text\n* Split: validation\n\nTo run new evaluation jobs, visit Hugging Face's automatic model evaluator.",
"## Contributions\n\nThanks to @abrar06 for evaluating this model."
] |
f30e1bd2f0a51535b493de36d3686cfcfff47abe | # Dataset Card for WebGPT Comparisons
## Dataset Description
In the [WebGPT paper](https://arxiv.org/abs/2112.09332), the authors trained a reward model from human feedback.
They used the reward model to train a long form question answering model to align with human preferences.
This is the dataset of all comparisons that were marked as suitable for reward modeling by the end of the WebGPT project.
There are 19,578 comparisons in total.
Each example in the dataset contains a pair of model answers for a question, and the associated metadata.
Each answer has a preference score from humans that can be used to determine which of the two answers are better.
Overall, an example has the following fields:
* `question`: The text of the question, together with the name of the dataset from which it was taken and a unique ID.
* `quotes_0`: The extracts that the model found while browsing for `answer_0`, together with the title of the page on which the extract was found, constructed from the HTML title and domain name of the page.
* `answer_0`: The final answer that the model composed using `quotes_0`.
* `tokens_0`: The prefix that would have been given to the model in the final step of the episode to create `answer_0`, and the completion given by the model or human. The prefix is made up of the question and the quotes, with some truncation, and the completion is simply the answer. Both are tokenized using the GPT-2 tokenizer. The concatenation of the prefix and completion is the input used for reward modeling.
* `score_0`: The strength of the preference for `answer_0` over `answer_1` as a number from −1 to 1. It sums to 0 with `score_1`, and an answer is preferred if and only if its score is positive. For reward modeling, we treat scores of 0 as soft 50% labels, and all other scores as hard labels (using only their sign).
* `quotes_1`: The counterpart to `quotes_0`.
* `answer_1`: The counterpart to `answer_0`.
* `tokens_1`: The counterpart to `tokens_0`.
* `score_1`: The counterpart to `score_0`.
This information was found in Appendix K of the WebGPT paper.
## Citation Information
[https://arxiv.org/abs/2112.09332](https://arxiv.org/abs/2112.09332)
```
@inproceedings{nakano2021webgpt,
author = {Reiichiro Nakano and Jacob Hilton and Suchir Balaji and Jeff Wu and Long Ouyang and Christina Kim and Christopher Hesse and Shantanu Jain and Vineet Kosaraju and William Saunders and Xu Jiang and Karl Cobbe and Tyna Eloundou and Gretchen Krueger and Kevin Button and Matthew Knight and Benjamin Chess and John Schulman},
title = {WebGPT: Browser-assisted question-answering with human feedback},
booktitle = {arXiv},
year = 2021,
}
```
Dataset added to the Hugging Face Hub by [@Tristan](https://huggingface.co/Tristan) and [@natolambert](https://huggingface.co/natolambert) | openai/webgpt_comparisons | [
"arxiv:2112.09332",
"region:us"
] | 2022-12-18T19:56:41+00:00 | {"pretty_name": "WebGPT Comparisons"} | 2022-12-19T17:55:29+00:00 | [
"2112.09332"
] | [] | TAGS
#arxiv-2112.09332 #region-us
| # Dataset Card for WebGPT Comparisons
## Dataset Description
In the WebGPT paper, the authors trained a reward model from human feedback.
They used the reward model to train a long form question answering model to align with human preferences.
This is the dataset of all comparisons that were marked as suitable for reward modeling by the end of the WebGPT project.
There are 19,578 comparisons in total.
Each example in the dataset contains a pair of model answers for a question, and the associated metadata.
Each answer has a preference score from humans that can be used to determine which of the two answers are better.
Overall, an example has the following fields:
* 'question': The text of the question, together with the name of the dataset from which it was taken and a unique ID.
* 'quotes_0': The extracts that the model found while browsing for 'answer_0', together with the title of the page on which the extract was found, constructed from the HTML title and domain name of the page.
* 'answer_0': The final answer that the model composed using 'quotes_0'.
* 'tokens_0': The prefix that would have been given to the model in the final step of the episode to create 'answer_0', and the completion given by the model or human. The prefix is made up of the question and the quotes, with some truncation, and the completion is simply the answer. Both are tokenized using the GPT-2 tokenizer. The concatenation of the prefix and completion is the input used for reward modeling.
* 'score_0': The strength of the preference for 'answer_0' over 'answer_1' as a number from −1 to 1. It sums to 0 with 'score_1', and an answer is preferred if and only if its score is positive. For reward modeling, we treat scores of 0 as soft 50% labels, and all other scores as hard labels (using only their sign).
* 'quotes_1': The counterpart to 'quotes_0'.
* 'answer_1': The counterpart to 'answer_0'.
* 'tokens_1': The counterpart to 'tokens_0'.
* 'score_1': The counterpart to 'score_0'.
This information was found in Appendix K of the WebGPT paper.
URL
Dataset added to the Hugging Face Hub by @Tristan and @natolambert | [
"# Dataset Card for WebGPT Comparisons",
"## Dataset Description\n\n\nIn the WebGPT paper, the authors trained a reward model from human feedback.\nThey used the reward model to train a long form question answering model to align with human preferences.\nThis is the dataset of all comparisons that were marked as suitable for reward modeling by the end of the WebGPT project.\nThere are 19,578 comparisons in total.\n\nEach example in the dataset contains a pair of model answers for a question, and the associated metadata.\nEach answer has a preference score from humans that can be used to determine which of the two answers are better.\nOverall, an example has the following fields:\n\n* 'question': The text of the question, together with the name of the dataset from which it was taken and a unique ID.\n* 'quotes_0': The extracts that the model found while browsing for 'answer_0', together with the title of the page on which the extract was found, constructed from the HTML title and domain name of the page.\n* 'answer_0': The final answer that the model composed using 'quotes_0'.\n* 'tokens_0': The prefix that would have been given to the model in the final step of the episode to create 'answer_0', and the completion given by the model or human. The prefix is made up of the question and the quotes, with some truncation, and the completion is simply the answer. Both are tokenized using the GPT-2 tokenizer. The concatenation of the prefix and completion is the input used for reward modeling.\n* 'score_0': The strength of the preference for 'answer_0' over 'answer_1' as a number from −1 to 1. It sums to 0 with 'score_1', and an answer is preferred if and only if its score is positive. For reward modeling, we treat scores of 0 as soft 50% labels, and all other scores as hard labels (using only their sign).\n* 'quotes_1': The counterpart to 'quotes_0'.\n* 'answer_1': The counterpart to 'answer_0'.\n* 'tokens_1': The counterpart to 'tokens_0'.\n* 'score_1': The counterpart to 'score_0'.\n\nThis information was found in Appendix K of the WebGPT paper.\n\n\n\nURL\n\n\n\nDataset added to the Hugging Face Hub by @Tristan and @natolambert"
] | [
"TAGS\n#arxiv-2112.09332 #region-us \n",
"# Dataset Card for WebGPT Comparisons",
"## Dataset Description\n\n\nIn the WebGPT paper, the authors trained a reward model from human feedback.\nThey used the reward model to train a long form question answering model to align with human preferences.\nThis is the dataset of all comparisons that were marked as suitable for reward modeling by the end of the WebGPT project.\nThere are 19,578 comparisons in total.\n\nEach example in the dataset contains a pair of model answers for a question, and the associated metadata.\nEach answer has a preference score from humans that can be used to determine which of the two answers are better.\nOverall, an example has the following fields:\n\n* 'question': The text of the question, together with the name of the dataset from which it was taken and a unique ID.\n* 'quotes_0': The extracts that the model found while browsing for 'answer_0', together with the title of the page on which the extract was found, constructed from the HTML title and domain name of the page.\n* 'answer_0': The final answer that the model composed using 'quotes_0'.\n* 'tokens_0': The prefix that would have been given to the model in the final step of the episode to create 'answer_0', and the completion given by the model or human. The prefix is made up of the question and the quotes, with some truncation, and the completion is simply the answer. Both are tokenized using the GPT-2 tokenizer. The concatenation of the prefix and completion is the input used for reward modeling.\n* 'score_0': The strength of the preference for 'answer_0' over 'answer_1' as a number from −1 to 1. It sums to 0 with 'score_1', and an answer is preferred if and only if its score is positive. For reward modeling, we treat scores of 0 as soft 50% labels, and all other scores as hard labels (using only their sign).\n* 'quotes_1': The counterpart to 'quotes_0'.\n* 'answer_1': The counterpart to 'answer_0'.\n* 'tokens_1': The counterpart to 'tokens_0'.\n* 'score_1': The counterpart to 'score_0'.\n\nThis information was found in Appendix K of the WebGPT paper.\n\n\n\nURL\n\n\n\nDataset added to the Hugging Face Hub by @Tristan and @natolambert"
] |
dd2ede6faaea338ef6b1e2966f06808656975a23 |
# Dataset Card for CSFD movie reviews (Czech)
## Dataset Description
The dataset contains user reviews from Czech/Slovak movie databse website <https://csfd.cz>.
Each review contains text, rating, date, and basic information about the movie (or TV series).
The dataset has in total (train+validation+test) 30,000 reviews. The data is balanced - each rating has approximately the same frequency.
## Dataset Features
Each sample contains:
- `review_id`: unique string identifier of the review.
- `rating_str`: string representation of the rating (from "0/5" to "5/5")
- `rating_int`: integer representation of the rating (from 0 to 5)
- `date`: date of publishing the review (just date, no time nor timezone)
- `comment_language`: language of the review (always "cs")
- `comment`: the string of the review
- `item_title`: title of the reviewed item
- `item_year`: publishing year of the item (string, can also be a range)
- `item_kind`: kind of the item - either "film" or "seriál"
- `item_genres`: list of genres of the item
- `item_directors`: list of director names of the item
- `item_screenwriters`: list of screenwriter names of the item
- `item_cast`: list of actors and actress in the item
## Dataset Source
The data was mined and sampled from the <https://csfd.cz> website.
Make sure to comply with the terms of conditions of the website operator when using the data.
| fewshot-goes-multilingual/cs_csfd-movie-reviews | [
"task_categories:text-classification",
"task_ids:sentiment-classification",
"annotations_creators:crowdsourced",
"language_creators:crowdsourced",
"multilinguality:monolingual",
"size_categories:10K<n<100K",
"source_datasets:original",
"language:cs",
"license:cc-by-sa-4.0",
"movie reviews",
"rating prediction",
"region:us"
] | 2022-12-18T20:05:15+00:00 | {"annotations_creators": ["crowdsourced"], "language_creators": ["crowdsourced"], "language": ["cs"], "license": ["cc-by-sa-4.0"], "multilinguality": ["monolingual"], "size_categories": ["10K<n<100K"], "source_datasets": ["original"], "task_categories": ["text-classification"], "task_ids": ["sentiment-classification"], "pretty_name": "CSFD movie reviews (Czech)", "tags": ["movie reviews", "rating prediction"]} | 2022-12-18T21:30:56+00:00 | [] | [
"cs"
] | TAGS
#task_categories-text-classification #task_ids-sentiment-classification #annotations_creators-crowdsourced #language_creators-crowdsourced #multilinguality-monolingual #size_categories-10K<n<100K #source_datasets-original #language-Czech #license-cc-by-sa-4.0 #movie reviews #rating prediction #region-us
|
# Dataset Card for CSFD movie reviews (Czech)
## Dataset Description
The dataset contains user reviews from Czech/Slovak movie databse website <URL>.
Each review contains text, rating, date, and basic information about the movie (or TV series).
The dataset has in total (train+validation+test) 30,000 reviews. The data is balanced - each rating has approximately the same frequency.
## Dataset Features
Each sample contains:
- 'review_id': unique string identifier of the review.
- 'rating_str': string representation of the rating (from "0/5" to "5/5")
- 'rating_int': integer representation of the rating (from 0 to 5)
- 'date': date of publishing the review (just date, no time nor timezone)
- 'comment_language': language of the review (always "cs")
- 'comment': the string of the review
- 'item_title': title of the reviewed item
- 'item_year': publishing year of the item (string, can also be a range)
- 'item_kind': kind of the item - either "film" or "seriál"
- 'item_genres': list of genres of the item
- 'item_directors': list of director names of the item
- 'item_screenwriters': list of screenwriter names of the item
- 'item_cast': list of actors and actress in the item
## Dataset Source
The data was mined and sampled from the <URL> website.
Make sure to comply with the terms of conditions of the website operator when using the data.
| [
"# Dataset Card for CSFD movie reviews (Czech)",
"## Dataset Description\n\nThe dataset contains user reviews from Czech/Slovak movie databse website <URL>.\nEach review contains text, rating, date, and basic information about the movie (or TV series).\nThe dataset has in total (train+validation+test) 30,000 reviews. The data is balanced - each rating has approximately the same frequency.",
"## Dataset Features\n\nEach sample contains:\n- 'review_id': unique string identifier of the review.\n- 'rating_str': string representation of the rating (from \"0/5\" to \"5/5\")\n- 'rating_int': integer representation of the rating (from 0 to 5)\n- 'date': date of publishing the review (just date, no time nor timezone)\n- 'comment_language': language of the review (always \"cs\")\n- 'comment': the string of the review\n- 'item_title': title of the reviewed item\n- 'item_year': publishing year of the item (string, can also be a range)\n- 'item_kind': kind of the item - either \"film\" or \"seriál\"\n- 'item_genres': list of genres of the item\n- 'item_directors': list of director names of the item\n- 'item_screenwriters': list of screenwriter names of the item\n- 'item_cast': list of actors and actress in the item",
"## Dataset Source\n\nThe data was mined and sampled from the <URL> website.\nMake sure to comply with the terms of conditions of the website operator when using the data."
] | [
"TAGS\n#task_categories-text-classification #task_ids-sentiment-classification #annotations_creators-crowdsourced #language_creators-crowdsourced #multilinguality-monolingual #size_categories-10K<n<100K #source_datasets-original #language-Czech #license-cc-by-sa-4.0 #movie reviews #rating prediction #region-us \n",
"# Dataset Card for CSFD movie reviews (Czech)",
"## Dataset Description\n\nThe dataset contains user reviews from Czech/Slovak movie databse website <URL>.\nEach review contains text, rating, date, and basic information about the movie (or TV series).\nThe dataset has in total (train+validation+test) 30,000 reviews. The data is balanced - each rating has approximately the same frequency.",
"## Dataset Features\n\nEach sample contains:\n- 'review_id': unique string identifier of the review.\n- 'rating_str': string representation of the rating (from \"0/5\" to \"5/5\")\n- 'rating_int': integer representation of the rating (from 0 to 5)\n- 'date': date of publishing the review (just date, no time nor timezone)\n- 'comment_language': language of the review (always \"cs\")\n- 'comment': the string of the review\n- 'item_title': title of the reviewed item\n- 'item_year': publishing year of the item (string, can also be a range)\n- 'item_kind': kind of the item - either \"film\" or \"seriál\"\n- 'item_genres': list of genres of the item\n- 'item_directors': list of director names of the item\n- 'item_screenwriters': list of screenwriter names of the item\n- 'item_cast': list of actors and actress in the item",
"## Dataset Source\n\nThe data was mined and sampled from the <URL> website.\nMake sure to comply with the terms of conditions of the website operator when using the data."
] |
23a20c659d868740ef9c54854de631fe19cd5c17 |
# Dataset Card for CSFD movie reviews (Slovak)
## Dataset Description
The dataset contains user reviews from Czech/Slovak movie databse website <https://csfd.cz>.
Each review contains text, rating, date, and basic information about the movie (or TV series).
The dataset has in total (train+validation+test) 30,000 reviews. The data is balanced - each rating has approximately the same frequency.
## Dataset Features
Each sample contains:
- `review_id`: unique string identifier of the review.
- `rating_str`: string representation of the rating (from "0/5" to "5/5")
- `rating_int`: integer representation of the rating (from 0 to 5)
- `date`: date of publishing the review (just date, no time nor timezone)
- `comment_language`: language of the review (always "sk")
- `comment`: the string of the review
- `item_title`: title of the reviewed item
- `item_year`: publishing year of the item (string, can also be a range)
- `item_kind`: kind of the item - either "film" or "seriál"
- `item_genres`: list of genres of the item
- `item_directors`: list of director names of the item
- `item_screenwriters`: list of screenwriter names of the item
- `item_cast`: list of actors and actress in the item
## Dataset Source
The data was mined and sampled from the <https://csfd.cz> website.
Make sure to comply with the terms of conditions of the website operator when using the data.
| fewshot-goes-multilingual/sk_csfd-movie-reviews | [
"task_categories:text-classification",
"task_ids:sentiment-classification",
"annotations_creators:crowdsourced",
"language_creators:crowdsourced",
"multilinguality:monolingual",
"size_categories:10K<n<100K",
"source_datasets:original",
"language:sk",
"license:cc-by-sa-4.0",
"movie reviews",
"rating prediction",
"region:us"
] | 2022-12-18T21:28:17+00:00 | {"annotations_creators": ["crowdsourced"], "language_creators": ["crowdsourced"], "language": ["sk"], "license": ["cc-by-sa-4.0"], "multilinguality": ["monolingual"], "size_categories": ["10K<n<100K"], "source_datasets": ["original"], "task_categories": ["text-classification"], "task_ids": ["sentiment-classification"], "pretty_name": "CSFD movie reviews (Slovak)", "tags": ["movie reviews", "rating prediction"]} | 2022-12-18T21:30:31+00:00 | [] | [
"sk"
] | TAGS
#task_categories-text-classification #task_ids-sentiment-classification #annotations_creators-crowdsourced #language_creators-crowdsourced #multilinguality-monolingual #size_categories-10K<n<100K #source_datasets-original #language-Slovak #license-cc-by-sa-4.0 #movie reviews #rating prediction #region-us
|
# Dataset Card for CSFD movie reviews (Slovak)
## Dataset Description
The dataset contains user reviews from Czech/Slovak movie databse website <URL>.
Each review contains text, rating, date, and basic information about the movie (or TV series).
The dataset has in total (train+validation+test) 30,000 reviews. The data is balanced - each rating has approximately the same frequency.
## Dataset Features
Each sample contains:
- 'review_id': unique string identifier of the review.
- 'rating_str': string representation of the rating (from "0/5" to "5/5")
- 'rating_int': integer representation of the rating (from 0 to 5)
- 'date': date of publishing the review (just date, no time nor timezone)
- 'comment_language': language of the review (always "sk")
- 'comment': the string of the review
- 'item_title': title of the reviewed item
- 'item_year': publishing year of the item (string, can also be a range)
- 'item_kind': kind of the item - either "film" or "seriál"
- 'item_genres': list of genres of the item
- 'item_directors': list of director names of the item
- 'item_screenwriters': list of screenwriter names of the item
- 'item_cast': list of actors and actress in the item
## Dataset Source
The data was mined and sampled from the <URL> website.
Make sure to comply with the terms of conditions of the website operator when using the data.
| [
"# Dataset Card for CSFD movie reviews (Slovak)",
"## Dataset Description\n\nThe dataset contains user reviews from Czech/Slovak movie databse website <URL>.\nEach review contains text, rating, date, and basic information about the movie (or TV series).\nThe dataset has in total (train+validation+test) 30,000 reviews. The data is balanced - each rating has approximately the same frequency.",
"## Dataset Features\n\nEach sample contains:\n- 'review_id': unique string identifier of the review.\n- 'rating_str': string representation of the rating (from \"0/5\" to \"5/5\")\n- 'rating_int': integer representation of the rating (from 0 to 5)\n- 'date': date of publishing the review (just date, no time nor timezone)\n- 'comment_language': language of the review (always \"sk\")\n- 'comment': the string of the review\n- 'item_title': title of the reviewed item\n- 'item_year': publishing year of the item (string, can also be a range)\n- 'item_kind': kind of the item - either \"film\" or \"seriál\"\n- 'item_genres': list of genres of the item\n- 'item_directors': list of director names of the item\n- 'item_screenwriters': list of screenwriter names of the item\n- 'item_cast': list of actors and actress in the item",
"## Dataset Source\n\nThe data was mined and sampled from the <URL> website.\nMake sure to comply with the terms of conditions of the website operator when using the data."
] | [
"TAGS\n#task_categories-text-classification #task_ids-sentiment-classification #annotations_creators-crowdsourced #language_creators-crowdsourced #multilinguality-monolingual #size_categories-10K<n<100K #source_datasets-original #language-Slovak #license-cc-by-sa-4.0 #movie reviews #rating prediction #region-us \n",
"# Dataset Card for CSFD movie reviews (Slovak)",
"## Dataset Description\n\nThe dataset contains user reviews from Czech/Slovak movie databse website <URL>.\nEach review contains text, rating, date, and basic information about the movie (or TV series).\nThe dataset has in total (train+validation+test) 30,000 reviews. The data is balanced - each rating has approximately the same frequency.",
"## Dataset Features\n\nEach sample contains:\n- 'review_id': unique string identifier of the review.\n- 'rating_str': string representation of the rating (from \"0/5\" to \"5/5\")\n- 'rating_int': integer representation of the rating (from 0 to 5)\n- 'date': date of publishing the review (just date, no time nor timezone)\n- 'comment_language': language of the review (always \"sk\")\n- 'comment': the string of the review\n- 'item_title': title of the reviewed item\n- 'item_year': publishing year of the item (string, can also be a range)\n- 'item_kind': kind of the item - either \"film\" or \"seriál\"\n- 'item_genres': list of genres of the item\n- 'item_directors': list of director names of the item\n- 'item_screenwriters': list of screenwriter names of the item\n- 'item_cast': list of actors and actress in the item",
"## Dataset Source\n\nThe data was mined and sampled from the <URL> website.\nMake sure to comply with the terms of conditions of the website operator when using the data."
] |
c963bed0544cf090214329c4b2e9fc0c84168115 | # Dataset Card for "twitter"
[More Information needed](https://github.com/huggingface/datasets/blob/main/CONTRIBUTING.md#how-to-contribute-to-the-dataset-cards) | hayleyg/twitter | [
"region:us"
] | 2022-12-18T21:33:52+00:00 | {"dataset_info": {"features": [{"name": "label", "dtype": "int64"}, {"name": "input_ids", "sequence": "int32"}, {"name": "attention_mask", "sequence": "int8"}], "splits": [{"name": "train", "num_bytes": 332000, "num_examples": 2000}, {"name": "test", "num_bytes": 83000, "num_examples": 500}], "download_size": 128444, "dataset_size": 415000}} | 2022-12-18T21:34:22+00:00 | [] | [] | TAGS
#region-us
| # Dataset Card for "twitter"
More Information needed | [
"# Dataset Card for \"twitter\"\n\nMore Information needed"
] | [
"TAGS\n#region-us \n",
"# Dataset Card for \"twitter\"\n\nMore Information needed"
] |
e490d6a7e54b88291411f00565533043ea3150ca | # Dataset Card for "mbti-cleaned"
[More Information needed](https://github.com/huggingface/datasets/blob/main/CONTRIBUTING.md#how-to-contribute-to-the-dataset-cards) | echodpp/mbti-cleaned | [
"region:us"
] | 2022-12-18T22:31:08+00:00 | {"dataset_info": {"features": [{"name": "label", "dtype": "int64"}, {"name": "text", "dtype": "string"}], "splits": [{"name": "train", "num_bytes": 51651122, "num_examples": 327828}, {"name": "test", "num_bytes": 12922409, "num_examples": 81957}], "download_size": 42684526, "dataset_size": 64573531}} | 2022-12-18T22:31:20+00:00 | [] | [] | TAGS
#region-us
| # Dataset Card for "mbti-cleaned"
More Information needed | [
"# Dataset Card for \"mbti-cleaned\"\n\nMore Information needed"
] | [
"TAGS\n#region-us \n",
"# Dataset Card for \"mbti-cleaned\"\n\nMore Information needed"
] |
7e1fccd916c1a6cff958a94f1f8c3c49226210f2 | # Dataset Card for "yelp"
[More Information needed](https://github.com/huggingface/datasets/blob/main/CONTRIBUTING.md#how-to-contribute-to-the-dataset-cards) | hayleyg/yelp | [
"region:us"
] | 2022-12-18T22:33:39+00:00 | {"dataset_info": {"features": [{"name": "label", "dtype": "int64"}, {"name": "input_ids", "sequence": "int32"}, {"name": "attention_mask", "sequence": "int8"}], "splits": [{"name": "train", "num_bytes": 332000, "num_examples": 2000}, {"name": "test", "num_bytes": 83000, "num_examples": 500}], "download_size": 174280, "dataset_size": 415000}} | 2022-12-18T22:34:02+00:00 | [] | [] | TAGS
#region-us
| # Dataset Card for "yelp"
More Information needed | [
"# Dataset Card for \"yelp\"\n\nMore Information needed"
] | [
"TAGS\n#region-us \n",
"# Dataset Card for \"yelp\"\n\nMore Information needed"
] |
59c8ab191f9f7140d0e7dad526d4bf9c3ce55e76 | # Dataset Card for "learning-segformer-dataset"
[More Information needed](https://github.com/huggingface/datasets/blob/main/CONTRIBUTING.md#how-to-contribute-to-the-dataset-cards) | nickponline/learning-segformer-dataset | [
"region:us"
] | 2022-12-19T00:19:48+00:00 | {"dataset_info": {"features": [{"name": "pixel_values", "dtype": "image"}, {"name": "label", "dtype": "image"}], "splits": [{"name": "train", "num_bytes": 402128.0, "num_examples": 100}], "download_size": 326407, "dataset_size": 402128.0}} | 2022-12-19T00:20:04+00:00 | [] | [] | TAGS
#region-us
| # Dataset Card for "learning-segformer-dataset"
More Information needed | [
"# Dataset Card for \"learning-segformer-dataset\"\n\nMore Information needed"
] | [
"TAGS\n#region-us \n",
"# Dataset Card for \"learning-segformer-dataset\"\n\nMore Information needed"
] |
dc2ec07406a7c223385f323044a5dc2ad6d0c6b1 | # Quick!Draw! Dataset (per-row bin format)
This is the full 50M-row dataset from [QuickDraw! dataset](https://github.com/googlecreativelab/quickdraw-dataset). The row for each drawing contains a byte-encoded packed representation of the drawing and data, which you can unpack using the following snippet:
```
def unpack_drawing(file_handle):
key_id, = unpack('Q', file_handle.read(8))
country_code, = unpack('2s', file_handle.read(2))
recognized, = unpack('b', file_handle.read(1))
timestamp, = unpack('I', file_handle.read(4))
n_strokes, = unpack('H', file_handle.read(2))
image = []
n_bytes = 17
for i in range(n_strokes):
n_points, = unpack('H', file_handle.read(2))
fmt = str(n_points) + 'B'
x = unpack(fmt, file_handle.read(n_points))
y = unpack(fmt, file_handle.read(n_points))
image.append((x, y))
n_bytes += 2 + 2*n_points
result = {
'key_id': key_id,
'country_code': country_code,
'recognized': recognized,
'timestamp': timestamp,
'image': image,
}
return result
```
The `image` in the above is still in line vector format. To convert render this to a raster image (I recommend you do this on-the-fly in a pre-processor):
```
# packed bin -> RGB PIL
def binToPIL(packed_drawing):
padding = 8
radius = 7
scale = (224.0-(2*padding)) / 256
unpacked = unpack_drawing(io.BytesIO(packed_drawing))
unpacked_image = unpacked['image']
image = np.full((224,224), 255, np.uint8)
for stroke in unpacked['image']:
prevX = round(stroke[0][0]*scale)
prevY = round(stroke[1][0]*scale)
for i in range(1, len(stroke[0])):
x = round(stroke[0][i]*scale)
y = round(stroke[1][i]*scale)
cv2.line(image, (padding+prevX, padding+prevY), (padding+x, padding+y), 0, radius, -1)
prevX = x
prevY = y
pilImage = Image.fromarray(image).convert("RGB")
return pilImage
``` | kmewhort/quickdraw-bins-50M | [
"region:us"
] | 2022-12-19T03:43:02+00:00 | {"dataset_info": {"features": [{"name": "label", "dtype": {"class_label": {"names": {"0": "The Eiffel Tower", "1": "The Great Wall of China", "2": "The Mona Lisa", "3": "aircraft carrier", "4": "airplane", "5": "alarm clock", "6": "ambulance", "7": "angel", "8": "animal migration", "9": "ant", "10": "anvil", "11": "apple", "12": "arm", "13": "asparagus", "14": "axe", "15": "backpack", "16": "banana", "17": "bandage", "18": "barn", "19": "baseball", "20": "baseball bat", "21": "basket", "22": "basketball", "23": "bat", "24": "bathtub", "25": "beach", "26": "bear", "27": "beard", "28": "bed", "29": "bee", "30": "belt", "31": "bench", "32": "bicycle", "33": "binoculars", "34": "bird", "35": "birthday cake", "36": "blackberry", "37": "blueberry", "38": "book", "39": "boomerang", "40": "bottlecap", "41": "bowtie", "42": "bracelet", "43": "brain", "44": "bread", "45": "bridge", "46": "broccoli", "47": "broom", "48": "bucket", "49": "bulldozer", "50": "bus", "51": "bush", "52": "butterfly", "53": "cactus", "54": "cake", "55": "calculator", "56": "calendar", "57": "camel", "58": "camera", "59": "camouflage", "60": "campfire", "61": "candle", "62": "cannon", "63": "canoe", "64": "car", "65": "carrot", "66": "castle", "67": "cat", "68": "ceiling fan", "69": "cell phone", "70": "cello", "71": "chair", "72": "chandelier", "73": "church", "74": "circle", "75": "clarinet", "76": "clock", "77": "cloud", "78": "coffee cup", "79": "compass", "80": "computer", "81": "cookie", "82": "cooler", "83": "couch", "84": "cow", "85": "crab", "86": "crayon", "87": "crocodile", "88": "crown", "89": "cruise ship", "90": "cup", "91": "diamond", "92": "dishwasher", "93": "diving board", "94": "dog", "95": "dolphin", "96": "donut", "97": "door", "98": "dragon", "99": "dresser", "100": "drill", "101": "drums", "102": "duck", "103": "dumbbell", "104": "ear", "105": "elbow", "106": "elephant", "107": "envelope", "108": "eraser", "109": "eye", "110": "eyeglasses", "111": "face", "112": "fan", "113": "feather", "114": "fence", "115": "finger", "116": "fire hydrant", "117": "fireplace", "118": "firetruck", "119": "fish", "120": "flamingo", "121": "flashlight", "122": "flip flops", "123": "floor lamp", "124": "flower", "125": "flying saucer", "126": "foot", "127": "fork", "128": "frog", "129": "frying pan", "130": "garden", "131": "garden hose", "132": "giraffe", "133": "goatee", "134": "golf club", "135": "grapes", "136": "grass", "137": "guitar", "138": "hamburger", "139": "hammer", "140": "hand", "141": "harp", "142": "hat", "143": "headphones", "144": "hedgehog", "145": "helicopter", "146": "helmet", "147": "hexagon", "148": "hockey puck", "149": "hockey stick", "150": "horse", "151": "hospital", "152": "hot air balloon", "153": "hot dog", "154": "hot tub", "155": "hourglass", "156": "house", "157": "house plant", "158": "hurricane", "159": "ice cream", "160": "jacket", "161": "jail", "162": "kangaroo", "163": "key", "164": "keyboard", "165": "knee", "166": "knife", "167": "ladder", "168": "lantern", "169": "laptop", "170": "leaf", "171": "leg", "172": "light bulb", "173": "lighter", "174": "lighthouse", "175": "lightning", "176": "line", "177": "lion", "178": "lipstick", "179": "lobster", "180": "lollipop", "181": "mailbox", "182": "map", "183": "marker", "184": "matches", "185": "megaphone", "186": "mermaid", "187": "microphone", "188": "microwave", "189": "monkey", "190": "moon", "191": "mosquito", "192": "motorbike", "193": "mountain", "194": "mouse", "195": "moustache", "196": "mouth", "197": "mug", "198": "mushroom", "199": "nail", "200": "necklace", "201": "nose", "202": "ocean", "203": "octagon", "204": "octopus", "205": "onion", "206": "oven", "207": "owl", "208": "paint can", "209": "paintbrush", "210": "palm tree", "211": "panda", "212": "pants", "213": "paper clip", "214": "parachute", "215": "parrot", "216": "passport", "217": "peanut", "218": "pear", "219": "peas", "220": "pencil", "221": "penguin", "222": "piano", "223": "pickup truck", "224": "picture frame", "225": "pig", "226": "pillow", "227": "pineapple", "228": "pizza", "229": "pliers", "230": "police car", "231": "pond", "232": "pool", "233": "popsicle", "234": "postcard", "235": "potato", "236": "power outlet", "237": "purse", "238": "rabbit", "239": "raccoon", "240": "radio", "241": "rain", "242": "rainbow", "243": "rake", "244": "remote control", "245": "rhinoceros", "246": "rifle", "247": "river", "248": "roller coaster", "249": "rollerskates", "250": "sailboat", "251": "sandwich", "252": "saw", "253": "saxophone", "254": "school bus", "255": "scissors", "256": "scorpion", "257": "screwdriver", "258": "sea turtle", "259": "see saw", "260": "shark", "261": "sheep", "262": "shoe", "263": "shorts", "264": "shovel", "265": "sink", "266": "skateboard", "267": "skull", "268": "skyscraper", "269": "sleeping bag", "270": "smiley face", "271": "snail", "272": "snake", "273": "snorkel", "274": "snowflake", "275": "snowman", "276": "soccer ball", "277": "sock", "278": "speedboat", "279": "spider", "280": "spoon", "281": "spreadsheet", "282": "square", "283": "squiggle", "284": "squirrel", "285": "stairs", "286": "star", "287": "steak", "288": "stereo", "289": "stethoscope", "290": "stitches", "291": "stop sign", "292": "stove", "293": "strawberry", "294": "streetlight", "295": "string bean", "296": "submarine", "297": "suitcase", "298": "sun", "299": "swan", "300": "sweater", "301": "swing set", "302": "sword", "303": "syringe", "304": "t-shirt", "305": "table", "306": "teapot", "307": "teddy-bear", "308": "telephone", "309": "television", "310": "tennis racquet", "311": "tent", "312": "tiger", "313": "toaster", "314": "toe", "315": "toilet", "316": "tooth", "317": "toothbrush", "318": "toothpaste", "319": "tornado", "320": "tractor", "321": "traffic light", "322": "train", "323": "tree", "324": "triangle", "325": "trombone", "326": "truck", "327": "trumpet", "328": "umbrella", "329": "underwear", "330": "van", "331": "vase", "332": "violin", "333": "washing machine", "334": "watermelon", "335": "waterslide", "336": "whale", "337": "wheel", "338": "windmill", "339": "wine bottle", "340": "wine glass", "341": "wristwatch", "342": "yoga", "343": "zebra", "344": "zigzag"}}}}, {"name": "packed_drawing", "dtype": "binary"}], "splits": [{"name": "train", "num_bytes": 5196066788.157136, "num_examples": 40341012}, {"name": "test", "num_bytes": 1299016825.8428645, "num_examples": 10085254}], "download_size": 6290637578, "dataset_size": 6495083614.0}} | 2022-12-19T18:12:46+00:00 | [] | [] | TAGS
#region-us
| # Quick!Draw! Dataset (per-row bin format)
This is the full 50M-row dataset from QuickDraw! dataset. The row for each drawing contains a byte-encoded packed representation of the drawing and data, which you can unpack using the following snippet:
The 'image' in the above is still in line vector format. To convert render this to a raster image (I recommend you do this on-the-fly in a pre-processor):
| [
"# Quick!Draw! Dataset (per-row bin format)\n\nThis is the full 50M-row dataset from QuickDraw! dataset. The row for each drawing contains a byte-encoded packed representation of the drawing and data, which you can unpack using the following snippet:\n\n\n\nThe 'image' in the above is still in line vector format. To convert render this to a raster image (I recommend you do this on-the-fly in a pre-processor):"
] | [
"TAGS\n#region-us \n",
"# Quick!Draw! Dataset (per-row bin format)\n\nThis is the full 50M-row dataset from QuickDraw! dataset. The row for each drawing contains a byte-encoded packed representation of the drawing and data, which you can unpack using the following snippet:\n\n\n\nThe 'image' in the above is still in line vector format. To convert render this to a raster image (I recommend you do this on-the-fly in a pre-processor):"
] |
9415be17ea63d0b4dc471f8557a0817f3d7cfa9a |
В качестве фактов использовались предложения из Википедии, а в качестве негативных - худлит и новости
Модель обученная на этом датасете [Den4ikAI/ruBert_base_fact_detection](https://huggingface.co/Den4ikAI/ruBert_base_fact_detection)
delimiter='|' | Den4ikAI/fact_detection | [
"language:ru",
"license:mit",
"region:us"
] | 2022-12-19T03:44:27+00:00 | {"language": ["ru"], "license": "mit"} | 2022-12-19T04:02:52+00:00 | [] | [
"ru"
] | TAGS
#language-Russian #license-mit #region-us
|
В качестве фактов использовались предложения из Википедии, а в качестве негативных - худлит и новости
Модель обученная на этом датасете Den4ikAI/ruBert_base_fact_detection
delimiter='|' | [] | [
"TAGS\n#language-Russian #license-mit #region-us \n"
] |
b614668eb410f35b3acda675116bbe6ae6ccff54 | # Dataset Card for "python_vul_cvefix_small"
[More Information needed](https://github.com/huggingface/datasets/blob/main/CONTRIBUTING.md#how-to-contribute-to-the-dataset-cards) | EddieChen372/python_vul_cvefix_small | [
"region:us"
] | 2022-12-19T05:08:10+00:00 | {"dataset_info": {"features": [{"name": "label", "dtype": {"class_label": {"names": {"0": "CWE-22", "1": "CWE-79", "2": "CWE-601"}}}}, {"name": "code_before", "dtype": "string"}, {"name": "code_after", "dtype": "string"}, {"name": "label_text", "dtype": "string"}, {"name": "deleted", "struct": [{"name": "code", "sequence": "string"}, {"name": "line_no", "sequence": "int64"}]}, {"name": "added", "struct": [{"name": "code", "sequence": "string"}, {"name": "line_no", "sequence": "int64"}]}, {"name": "normalized_code_before", "dtype": "string"}, {"name": "normalized_code_after", "dtype": "string"}, {"name": "before_doc_string_pos", "sequence": "int64"}, {"name": "after_doc_string_pos", "sequence": "int64"}], "splits": [{"name": "train", "num_bytes": 10903264.596273292, "num_examples": 160}, {"name": "test", "num_bytes": 3609181.0, "num_examples": 41}], "download_size": 5041260, "dataset_size": 14512445.596273292}} | 2022-12-19T05:08:41+00:00 | [] | [] | TAGS
#region-us
| # Dataset Card for "python_vul_cvefix_small"
More Information needed | [
"# Dataset Card for \"python_vul_cvefix_small\"\n\nMore Information needed"
] | [
"TAGS\n#region-us \n",
"# Dataset Card for \"python_vul_cvefix_small\"\n\nMore Information needed"
] |
f8bd83e996e4392dbc0d5f643040e3128f0f9607 | stable diffusion
Azuki
| wheart/aiazuki | [
"license:openrail",
"region:us"
] | 2022-12-19T07:44:46+00:00 | {"license": "openrail"} | 2022-12-19T07:59:47+00:00 | [] | [] | TAGS
#license-openrail #region-us
| stable diffusion
Azuki
| [] | [
"TAGS\n#license-openrail #region-us \n"
] |
ccbdbc3ff8ac98aa73be4581bc3d23f5345f3092 | # Dataset Card for "corgi"
[More Information needed](https://github.com/huggingface/datasets/blob/main/CONTRIBUTING.md#how-to-contribute-to-the-dataset-cards) | lewtun/corgi | [
"region:us"
] | 2022-12-19T08:44:51+00:00 | {"dataset_info": {"features": [{"name": "image", "dtype": "image"}], "splits": [{"name": "train", "num_bytes": 5590698.0, "num_examples": 5}], "download_size": 5591635, "dataset_size": 5590698.0}} | 2022-12-19T08:45:20+00:00 | [] | [] | TAGS
#region-us
| # Dataset Card for "corgi"
More Information needed | [
"# Dataset Card for \"corgi\"\n\nMore Information needed"
] | [
"TAGS\n#region-us \n",
"# Dataset Card for \"corgi\"\n\nMore Information needed"
] |
0a94cf73e560e3376e14aaefba1e7ad16e6c4e94 |
# Dataset Card for The Stack Metadata
## Table of Contents
- [Table of Contents](#table-of-contents)
- [Dataset Description](#dataset-description)
- [Changelog](#changelog)
- [Dataset Summary](#dataset-summary)
- [Supported Tasks and Leaderboards](#supported-tasks-and-leaderboards)
- [Dataset Structure](#dataset-structure)
- [Data Fields](#data-fields)
- [Usage Example](#usage-example)
- [Dataset Creation](#dataset-creation)
- [Considerations for Using the Data](#considerations-for-using-the-data)
- [Additional Information](#additional-information)
- [Terms of Use for The Stack](#terms-of-use-for-the-stack)
## Dataset Description
- **Homepage:** https://www.bigcode-project.org/
- **Repository:** https://github.com/bigcode-project
- **Paper:** https://arxiv.org/abs/2211.15533
- **Leaderboard:** N/A
- **Point of Contact:** [email protected]
### Changelog
|Release|Description|
|-|-|
|v1.1| This is the first release of the metadata. It is for The Stack v1.1|
|v1.2| Metadata dataset matching The Stack v1.2|
### Dataset Summary
This is a set of additional information for repositories used for The Stack. It contains file paths, detected licenes as well as some other information for the repositories.
### Supported Tasks and Leaderboards
The main task is to recreate repository structure from the files of The Stack. Also, the set can be used for computing statistics and custom filtering or aggregation operations on The Stack.
## Dataset Structure
### Data Fields

The set is split into buckets by repositories. There are 944 buckets. Additionally to the fields in the image, `ri` contains `min_repo_event_datetime` which is the ealiest date and time of an event for a repo after Jan 1 2015.

As an example of an aggregation operation on The Stack, the image above shows conceptually a selection of stars ( and issues and PR count) for a file. Each unique file can be part of multiple repositories. So, The Stack releases unique files and aggregates meta information (e.g stars) from all repositories it belongs to. For example, for max_stars_count we take the maximum number of stars from all repositories the file is part of.
The meta data will allow you to reconstruct repository directory structures. For this, for each repository form `ri` tabele it is needed to take all its files from `fi` table, find them in The Stack by file's `hexsha` and save those files' content under its path for a repository from `fi` table. For speed it is preferable to index The Stack by hexsha first.
### Usage Example
Restore folder structure for python files in numpy repository
```python
import datasets
from pathlib import Path
from tqdm.auto import tqdm
import pandas as pd
# assuming metadata is cloned into the local folder /data/hf_repos/the-stack-metadata
# the stack is cloned into the local folder /data/hf_repos/the-stack-v1.1
# destination folder is in /repo_workdir/numpy_restored
the_stack_meta_path = Path('/data/hf_repos/the-stack-metadata')
the_stack_path = Path('/data/hf_repos/the-stack-v1.1')
repo_dst_root = Path('/repo_workdir/numpy_restored')
repo_name = 'numpy/numpy'
# Get bucket with numpy repo info
# meta_bucket_path = None
#for fn in tqdm(list((the_stack_meta_path/'data').glob('*/ri.parquet'))):
# df = pd.read_parquet(fn)
# if any(df['name'] == repo_name):
# meta_bucket_path = fn
# break
meta_bucket_path = the_stack_meta_path / 'data/255_944'
# Get repository id from repo name
ri_id = pd.read_parquet(
meta_bucket_path / 'ri.parquet'
).query(
f'`name` == "{repo_name}"'
)['id'].to_list()[0]
# Get files information for the reopository
files_info = pd.read_parquet(
meta_bucket_path / 'fi.parquet'
).query(
f'`ri_id` == {ri_id} and `size` != 0 and `is_deleted` == False'
)
# Convert DF with files information to a dictionary by language and then file hexsha
# there can be more than one file with the same hexsha in the repo so we gather
# all instances per unique hexsha
files_info_dict = {
k: v[['hexsha', 'path']].groupby('hexsha').apply(lambda x: list(x['path'])).to_dict()
for k, v in files_info.groupby('lang_ex')
}
# Load Python part of The Stack
ds = datasets.load_dataset(
str(the_stack_path/'data/python'),
num_proc=10, ignore_verifications=True
)
# Save file content of the python files in the numpy reposirotry in their appropriate locations
def save_file_content(example, files_info_dict, repo_dst_root):
if example['hexsha'] in files_info_dict:
for el in files_info_dict[example['hexsha']]:
path = repo_dst_root / el
path.parent.mkdir(parents=True, exist_ok=True)
path.write_text(example['content'])
ds.map(
save_file_content,
fn_kwargs={'files_info_dict': files_info_dict['Python'], 'repo_dst_root': repo_dst_root},
num_proc=10
)
```
## Dataset Creation
Please refer to [the section](https://huggingface.co/datasets/bigcode/the-stack#dataset-creation) in The Stack.
## Considerations for Using the Data
Please refer to [the section](https://huggingface.co/datasets/bigcode/the-stack#considerations-for-using-the-data) in The Stack.
## Additional Information
Please refer to [the section](https://huggingface.co/datasets/bigcode/the-stack#additional-information) in The Stack.
## Terms of Use for The Stack
Please refer to [the section](https://huggingface.co/datasets/bigcode/the-stack#terms-of-use-for-the-stack) in The Stack. | bigcode/the-stack-metadata | [
"task_categories:text-generation",
"language_creators:crowdsourced",
"language_creators:expert-generated",
"multilinguality:multilingual",
"size_categories:unknown",
"language:code",
"license:other",
"arxiv:2211.15533",
"region:us"
] | 2022-12-19T09:17:28+00:00 | {"annotations_creators": [], "language_creators": ["crowdsourced", "expert-generated"], "language": ["code"], "license": ["other"], "multilinguality": ["multilingual"], "size_categories": ["unknown"], "source_datasets": [], "task_categories": ["text-generation"], "task_ids": [], "pretty_name": "The-Stack-Metadata", "extra_gated_prompt": "## Terms of Use for The Stack\nThe Stack Metadata is a collection of additional information for and is part of The Stack dataset, - a collection of source code in over 300 programming languages. We ask that you read and acknowledge the following points before using the dataset:\n1. The Stack is a collection of source code from repositories with various licenses. Any use of all or part of the code gathered in The Stack must abide by the terms of the original licenses, including attribution clauses when relevant. We facilitate this by providing provenance information for each data point.\n2. The Stack is regularly updated to enact validated data removal requests. By clicking on \"Access repository\", you agree to update your own version of The Stack to the most recent usable version specified by the maintainers in [the following thread](https://huggingface.co/datasets/bigcode/the-stack/discussions/7). If you have questions about dataset versions and allowed uses, please also ask them in the dataset\u2019s [community discussions](https://huggingface.co/datasets/bigcode/the-stack/discussions/new). We will also notify users via email when the latest usable version changes.\n3. To host, share, or otherwise provide access to The Stack dataset, you must include [these Terms of Use](https://huggingface.co/datasets/bigcode/the-stack#terms-of-use-for-the-stack) and require users to agree to it.\n\nBy clicking on \"Access repository\" below, you accept that your contact information (email address and username) can be shared with the dataset maintainers as well.\n ", "extra_gated_fields": {"Email": "text", "I have read the License and agree with its terms": "checkbox"}} | 2023-03-16T13:58:24+00:00 | [
"2211.15533"
] | [
"code"
] | TAGS
#task_categories-text-generation #language_creators-crowdsourced #language_creators-expert-generated #multilinguality-multilingual #size_categories-unknown #language-code #license-other #arxiv-2211.15533 #region-us
| Dataset Card for The Stack Metadata
===================================
Table of Contents
-----------------
* Table of Contents
* Dataset Description
+ Changelog
+ Dataset Summary
+ Supported Tasks and Leaderboards
* Dataset Structure
+ Data Fields
+ Usage Example
* Dataset Creation
* Considerations for Using the Data
* Additional Information
* Terms of Use for The Stack
Dataset Description
-------------------
* Homepage: URL
* Repository: URL
* Paper: URL
* Leaderboard: N/A
* Point of Contact: contact@URL
### Changelog
### Dataset Summary
This is a set of additional information for repositories used for The Stack. It contains file paths, detected licenes as well as some other information for the repositories.
### Supported Tasks and Leaderboards
The main task is to recreate repository structure from the files of The Stack. Also, the set can be used for computing statistics and custom filtering or aggregation operations on The Stack.
Dataset Structure
-----------------
### Data Fields
!set structure
The set is split into buckets by repositories. There are 944 buckets. Additionally to the fields in the image, 'ri' contains 'min\_repo\_event\_datetime' which is the ealiest date and time of an event for a repo after Jan 1 2015.
!set usage
As an example of an aggregation operation on The Stack, the image above shows conceptually a selection of stars ( and issues and PR count) for a file. Each unique file can be part of multiple repositories. So, The Stack releases unique files and aggregates meta information (e.g stars) from all repositories it belongs to. For example, for max\_stars\_count we take the maximum number of stars from all repositories the file is part of.
The meta data will allow you to reconstruct repository directory structures. For this, for each repository form 'ri' tabele it is needed to take all its files from 'fi' table, find them in The Stack by file's 'hexsha' and save those files' content under its path for a repository from 'fi' table. For speed it is preferable to index The Stack by hexsha first.
### Usage Example
Restore folder structure for python files in numpy repository
Dataset Creation
----------------
Please refer to the section in The Stack.
Considerations for Using the Data
---------------------------------
Please refer to the section in The Stack.
Additional Information
----------------------
Please refer to the section in The Stack.
Terms of Use for The Stack
--------------------------
Please refer to the section in The Stack.
| [
"### Changelog",
"### Dataset Summary\n\n\nThis is a set of additional information for repositories used for The Stack. It contains file paths, detected licenes as well as some other information for the repositories.",
"### Supported Tasks and Leaderboards\n\n\nThe main task is to recreate repository structure from the files of The Stack. Also, the set can be used for computing statistics and custom filtering or aggregation operations on The Stack.\n\n\nDataset Structure\n-----------------",
"### Data Fields\n\n\n!set structure\nThe set is split into buckets by repositories. There are 944 buckets. Additionally to the fields in the image, 'ri' contains 'min\\_repo\\_event\\_datetime' which is the ealiest date and time of an event for a repo after Jan 1 2015.\n\n\n!set usage\n\n\nAs an example of an aggregation operation on The Stack, the image above shows conceptually a selection of stars ( and issues and PR count) for a file. Each unique file can be part of multiple repositories. So, The Stack releases unique files and aggregates meta information (e.g stars) from all repositories it belongs to. For example, for max\\_stars\\_count we take the maximum number of stars from all repositories the file is part of.\n\n\nThe meta data will allow you to reconstruct repository directory structures. For this, for each repository form 'ri' tabele it is needed to take all its files from 'fi' table, find them in The Stack by file's 'hexsha' and save those files' content under its path for a repository from 'fi' table. For speed it is preferable to index The Stack by hexsha first.",
"### Usage Example\n\n\nRestore folder structure for python files in numpy repository\n\n\nDataset Creation\n----------------\n\n\nPlease refer to the section in The Stack.\n\n\nConsiderations for Using the Data\n---------------------------------\n\n\nPlease refer to the section in The Stack.\n\n\nAdditional Information\n----------------------\n\n\nPlease refer to the section in The Stack.\n\n\nTerms of Use for The Stack\n--------------------------\n\n\nPlease refer to the section in The Stack."
] | [
"TAGS\n#task_categories-text-generation #language_creators-crowdsourced #language_creators-expert-generated #multilinguality-multilingual #size_categories-unknown #language-code #license-other #arxiv-2211.15533 #region-us \n",
"### Changelog",
"### Dataset Summary\n\n\nThis is a set of additional information for repositories used for The Stack. It contains file paths, detected licenes as well as some other information for the repositories.",
"### Supported Tasks and Leaderboards\n\n\nThe main task is to recreate repository structure from the files of The Stack. Also, the set can be used for computing statistics and custom filtering or aggregation operations on The Stack.\n\n\nDataset Structure\n-----------------",
"### Data Fields\n\n\n!set structure\nThe set is split into buckets by repositories. There are 944 buckets. Additionally to the fields in the image, 'ri' contains 'min\\_repo\\_event\\_datetime' which is the ealiest date and time of an event for a repo after Jan 1 2015.\n\n\n!set usage\n\n\nAs an example of an aggregation operation on The Stack, the image above shows conceptually a selection of stars ( and issues and PR count) for a file. Each unique file can be part of multiple repositories. So, The Stack releases unique files and aggregates meta information (e.g stars) from all repositories it belongs to. For example, for max\\_stars\\_count we take the maximum number of stars from all repositories the file is part of.\n\n\nThe meta data will allow you to reconstruct repository directory structures. For this, for each repository form 'ri' tabele it is needed to take all its files from 'fi' table, find them in The Stack by file's 'hexsha' and save those files' content under its path for a repository from 'fi' table. For speed it is preferable to index The Stack by hexsha first.",
"### Usage Example\n\n\nRestore folder structure for python files in numpy repository\n\n\nDataset Creation\n----------------\n\n\nPlease refer to the section in The Stack.\n\n\nConsiderations for Using the Data\n---------------------------------\n\n\nPlease refer to the section in The Stack.\n\n\nAdditional Information\n----------------------\n\n\nPlease refer to the section in The Stack.\n\n\nTerms of Use for The Stack\n--------------------------\n\n\nPlease refer to the section in The Stack."
] |
08f6acc1dad21b10087cc654d5ac9ef19ed34ecc | # Dataset Card for "news_classification"
[More Information needed](https://github.com/huggingface/datasets/blob/main/CONTRIBUTING.md#how-to-contribute-to-the-dataset-cards) | phd411r1/news_classification | [
"region:us"
] | 2022-12-19T12:27:30+00:00 | {"dataset_info": {"features": [{"name": "title", "dtype": "string"}, {"name": "label", "dtype": "int64"}], "splits": [{"name": "train", "num_bytes": 850939, "num_examples": 7997}, {"name": "test", "num_bytes": 178204, "num_examples": 1669}], "download_size": 551232, "dataset_size": 1029143}} | 2022-12-19T12:27:51+00:00 | [] | [] | TAGS
#region-us
| # Dataset Card for "news_classification"
More Information needed | [
"# Dataset Card for \"news_classification\"\n\nMore Information needed"
] | [
"TAGS\n#region-us \n",
"# Dataset Card for \"news_classification\"\n\nMore Information needed"
] |
e773ddeb1edfeb8e4f04afc63e10473fd33a0542 | # Dataset Card for "xrays"
[More Information needed](https://github.com/huggingface/datasets/blob/main/CONTRIBUTING.md#how-to-contribute-to-the-dataset-cards) | lewtun/xrays | [
"region:us"
] | 2022-12-19T13:03:53+00:00 | {"dataset_info": {"features": [{"name": "image", "dtype": "image"}], "splits": [{"name": "train", "num_bytes": 1483315.0, "num_examples": 15}], "download_size": 1483649, "dataset_size": 1483315.0}} | 2022-12-19T13:04:07+00:00 | [] | [] | TAGS
#region-us
| # Dataset Card for "xrays"
More Information needed | [
"# Dataset Card for \"xrays\"\n\nMore Information needed"
] | [
"TAGS\n#region-us \n",
"# Dataset Card for \"xrays\"\n\nMore Information needed"
] |
7523293e36fa4f11d4fdb40f7ca1e6d9dbf44ef2 | # Dataset Card for "galaxies"
[More Information needed](https://github.com/huggingface/datasets/blob/main/CONTRIBUTING.md#how-to-contribute-to-the-dataset-cards) | lewtun/galaxies | [
"region:us"
] | 2022-12-19T13:12:44+00:00 | {"dataset_info": {"features": [{"name": "image", "dtype": "image"}], "splits": [{"name": "train", "num_bytes": 193327.0, "num_examples": 15}], "download_size": 193973, "dataset_size": 193327.0}} | 2022-12-19T13:12:57+00:00 | [] | [] | TAGS
#region-us
| # Dataset Card for "galaxies"
More Information needed | [
"# Dataset Card for \"galaxies\"\n\nMore Information needed"
] | [
"TAGS\n#region-us \n",
"# Dataset Card for \"galaxies\"\n\nMore Information needed"
] |
56c0215d08fb430e30f8425ffc80ec0ad1682e0f |
# Dataset Card for "Hansel"
## Table of Contents
- [Table of Contents](#table-of-contents)
- [Dataset Description](#dataset-description)
- [Dataset Structure](#dataset-structure)
- [Data Instances](#data-instances)
- [Data Splits](#data-splits)
- [Citation](#citation)
## Dataset Description
- **Homepage:** https://github.com/HITsz-TMG/Hansel
- **Paper:** https://arxiv.org/abs/2207.13005
Hansel is a high-quality human-annotated Chinese entity linking (EL) dataset, focusing on tail entities and emerging entities:
- The test set contains Few-shot (FS) and zero-shot (ZS) slices, has 10K examples and uses Wikidata as the corresponding knowledge base.
- The training and validation sets are from Wikipedia hyperlinks, useful for large-scale pretraining of Chinese EL systems.
Please see our [WSDM 2023](https://www.wsdm-conference.org/2023/) paper [**"Hansel: A Chinese Few-Shot and Zero-Shot Entity Linking Benchmark"**](https://dl.acm.org/doi/10.1145/3539597.3570418) to learn more about our dataset.
For models in the paper and our processed knowledge base, please see our [Github repository](https://github.com/HITsz-TMG/Hansel).
## Dataset Structure
### Data Instances
{"id": "hansel-eval-zs-1463",
"text": "1905电影网讯 已经筹备了十余年的吉尔莫·德尔·托罗的《匹诺曹》,在上个月顺利被网飞公司买下,成为了流媒体巨头旗下的新片。近日,这部备受关注的影片确定了自己的档期:2021年。虽然具体时间未定,但影片却已经实实在在地向前迈出了一步。",
"start": 29,
"end": 32,
"mention": "匹诺曹",
"gold_id": "Q73895818",
"source": "https://www.1905.com/news/20181107/1325389.shtml",
"domain": "news"
}
### Data Splits
| | # Mentions | # Entities | Domain |
| ---- | ---- | ---- | ---- |
| Train | 9,879,813 | 541,058 | Wikipedia |
| Validation | 9,674 | 6,320 | Wikipedia |
| Hansel-FS | 5,260 | 2,720 | News, Social Media |
| Hansel-ZS | 4,715 | 4,046 | News, Social Media, E-books, etc.|
## Citation
If you find our dataset useful, please cite us.
```bibtex
@inproceedings{xu2022hansel,
author = {Xu, Zhenran and Shan, Zifei and Li, Yuxin and Hu, Baotian and Qin, Bing},
title = {Hansel: A Chinese Few-Shot and Zero-Shot Entity Linking Benchmark},
year = {2023},
publisher = {Association for Computing Machinery},
url = {https://doi.org/10.1145/3539597.3570418},
booktitle = {Proceedings of the Sixteenth ACM International Conference on Web Search and Data Mining},
pages = {832–840}
}
```
| HIT-TMG/Hansel | [
"task_categories:text-retrieval",
"task_ids:entity-linking-retrieval",
"annotations_creators:crowdsourced",
"annotations_creators:found",
"language_creators:found",
"language_creators:crowdsourced",
"multilinguality:monolingual",
"size_categories:1M<n<10M",
"size_categories:1K<n<10K",
"source_datasets:original",
"language:zh",
"license:cc-by-sa-4.0",
"arxiv:2207.13005",
"region:us"
] | 2022-12-19T13:28:24+00:00 | {"annotations_creators": ["crowdsourced", "found"], "language_creators": ["found", "crowdsourced"], "language": ["zh"], "license": ["cc-by-sa-4.0"], "multilinguality": ["monolingual"], "size_categories": ["1M<n<10M", "1K<n<10K"], "source_datasets": ["original"], "task_categories": ["text-retrieval"], "task_ids": ["entity-linking-retrieval"], "paperswithcode_id": "hansel", "pretty_name": "Hansel", "tags": [], "dataset_info": [{"config_name": "wiki", "features": [{"name": "id", "dtype": "string"}, {"name": "text", "dtype": "string"}, {"name": "start", "dtype": "int64"}, {"name": "end", "dtype": "int64"}, {"name": "mention", "dtype": "string"}, {"name": "gold_id", "dtype": "string"}], "splits": [{"name": "train"}, {"name": "validation"}]}, {"config_name": "hansel-few-shot", "features": [{"name": "id", "dtype": "string"}, {"name": "text", "dtype": "string"}, {"name": "start", "dtype": "int64"}, {"name": "end", "dtype": "int64"}, {"name": "mention", "dtype": "string"}, {"name": "gold_id", "dtype": "string"}, {"name": "source", "dtype": "string"}, {"name": "domain", "dtype": "string"}], "splits": [{"name": "test"}]}, {"config_name": "hansel-zero-shot", "features": [{"name": "id", "dtype": "string"}, {"name": "text", "dtype": "string"}, {"name": "start", "dtype": "int64"}, {"name": "end", "dtype": "int64"}, {"name": "mention", "dtype": "string"}, {"name": "gold_id", "dtype": "string"}, {"name": "source", "dtype": "string"}, {"name": "domain", "dtype": "string"}], "splits": [{"name": "test"}]}]} | 2023-03-13T11:52:56+00:00 | [
"2207.13005"
] | [
"zh"
] | TAGS
#task_categories-text-retrieval #task_ids-entity-linking-retrieval #annotations_creators-crowdsourced #annotations_creators-found #language_creators-found #language_creators-crowdsourced #multilinguality-monolingual #size_categories-1M<n<10M #size_categories-1K<n<10K #source_datasets-original #language-Chinese #license-cc-by-sa-4.0 #arxiv-2207.13005 #region-us
| Dataset Card for "Hansel"
=========================
Table of Contents
-----------------
* Table of Contents
* Dataset Description
* Dataset Structure
+ Data Instances
+ Data Splits
* Citation
Dataset Description
-------------------
* Homepage: URL
* Paper: URL
Hansel is a high-quality human-annotated Chinese entity linking (EL) dataset, focusing on tail entities and emerging entities:
* The test set contains Few-shot (FS) and zero-shot (ZS) slices, has 10K examples and uses Wikidata as the corresponding knowledge base.
* The training and validation sets are from Wikipedia hyperlinks, useful for large-scale pretraining of Chinese EL systems.
Please see our WSDM 2023 paper "Hansel: A Chinese Few-Shot and Zero-Shot Entity Linking Benchmark" to learn more about our dataset.
For models in the paper and our processed knowledge base, please see our Github repository.
Dataset Structure
-----------------
### Data Instances
```
{"id": "hansel-eval-zs-1463",
"text": "1905电影网讯 已经筹备了十余年的吉尔莫·德尔·托罗的《匹诺曹》,在上个月顺利被网飞公司买下,成为了流媒体巨头旗下的新片。近日,这部备受关注的影片确定了自己的档期:2021年。虽然具体时间未定,但影片却已经实实在在地向前迈出了一步。",
"start": 29,
"end": 32,
"mention": "匹诺曹",
"gold_id": "Q73895818",
"source": "URL
"domain": "news"
}
```
### Data Splits
If you find our dataset useful, please cite us.
| [
"### Data Instances\n\n\n\n```\n{\"id\": \"hansel-eval-zs-1463\", \n \"text\": \"1905电影网讯 已经筹备了十余年的吉尔莫·德尔·托罗的《匹诺曹》,在上个月顺利被网飞公司买下,成为了流媒体巨头旗下的新片。近日,这部备受关注的影片确定了自己的档期:2021年。虽然具体时间未定,但影片却已经实实在在地向前迈出了一步。\", \n \"start\": 29, \n \"end\": 32, \n \"mention\": \"匹诺曹\", \n \"gold_id\": \"Q73895818\", \n \"source\": \"URL \n \"domain\": \"news\"\n}\n\n```",
"### Data Splits\n\n\n\nIf you find our dataset useful, please cite us."
] | [
"TAGS\n#task_categories-text-retrieval #task_ids-entity-linking-retrieval #annotations_creators-crowdsourced #annotations_creators-found #language_creators-found #language_creators-crowdsourced #multilinguality-monolingual #size_categories-1M<n<10M #size_categories-1K<n<10K #source_datasets-original #language-Chinese #license-cc-by-sa-4.0 #arxiv-2207.13005 #region-us \n",
"### Data Instances\n\n\n\n```\n{\"id\": \"hansel-eval-zs-1463\", \n \"text\": \"1905电影网讯 已经筹备了十余年的吉尔莫·德尔·托罗的《匹诺曹》,在上个月顺利被网飞公司买下,成为了流媒体巨头旗下的新片。近日,这部备受关注的影片确定了自己的档期:2021年。虽然具体时间未定,但影片却已经实实在在地向前迈出了一步。\", \n \"start\": 29, \n \"end\": 32, \n \"mention\": \"匹诺曹\", \n \"gold_id\": \"Q73895818\", \n \"source\": \"URL \n \"domain\": \"news\"\n}\n\n```",
"### Data Splits\n\n\n\nIf you find our dataset useful, please cite us."
] |
15420106f9ebc5a7596d0f5b95f3bfbc5db18219 | ---
pipeline_tag: text-generation
tags:
- code
model-index:
- name: VeriGen
results:
- task:
type: text-generation
dataset:
type:
name:
extra_gated_prompt: >-
## Model License Agreement
Please read the BigCode [OpenRAIL-M
license](https://huggingface.co/spaces/bigcode/bigcode-model-license-agreement)
agreement before accepting it.
extra_gated_fields:
I accept the above license agreement, and will use the Model complying with the set of use restrictions and sharing requirements: checkbox
---
# VeriGen
## Table of Contents
1. [Dataset Summary](##model-summary)
2. [Use](##use)
3. [Limitations](##limitations)
4. [License](##license)
5. [Citation](##citation)
## Dataset Summary
- The dataset comprises Verilog modules as entries. The entries were retrieved from the GitHub dataset on BigQuery.
- For training [models (https://huggingface.co/shailja/fine-tuned-codegen-2B-Verilog)], we filtered entries with no of characters exceeding 20000 and duplicates (exact duplicates ignoring whitespaces).
- **Paper:** [ Benchmarking Large Language Models for Automated Verilog RTL Code Generation](https://arxiv.org/abs/2212.11140)
- **Point of Contact:** [contact@shailja](mailto:[email protected])
- **Languages:** Verilog (Hardware Description Language)
### Data Splits
The dataset only contains a train split.
### Use
```python
# pip install datasets
from datasets import load_dataset
ds = load_dataset("shailja/Verilog_GitHub", streaming=True, split="train")
print(next(iter(ds)))
#OUTPUT:
```
### Intended Use
The dataset consists of source code from a range of GitHub repositories. As such, they can potentially include non-compilable, low-quality, and vulnerable code.
### Attribution & Other Requirements
The pretraining dataset of the model was not filtered for permissive licenses only. Nevertheless, the model can generate source code verbatim from the dataset. The code's license might require attribution and/or other specific requirements that must be respected.
# License
The dataset is licensed under the BigCode OpenRAIL-M v1 license agreement. You can find the full agreement [here](https://huggingface.co/spaces/bigcode/bigcode-model-license-agreement).
# Citation
```
@misc{https://doi.org/10.48550/arxiv.2212.11140,
doi = {10.48550/ARXIV.2212.11140},
url = {https://arxiv.org/abs/2212.11140},
author = {Thakur, Shailja and Ahmad, Baleegh and Fan, Zhenxing and Pearce, Hammond and Tan, Benjamin and Karri, Ramesh and Dolan-Gavitt, Brendan and Garg, Siddharth},
title = {Benchmarking Large Language Models for Automated Verilog RTL Code Generation},
publisher = {arXiv},
year = {2022},
copyright = {arXiv.org perpetual, non-exclusive license}
}
``` | shailja/Verilog_GitHub | [
"license:mit",
"arxiv:2212.11140",
"region:us"
] | 2022-12-19T15:19:55+00:00 | {"license": "mit"} | 2023-09-20T16:14:18+00:00 | [
"2212.11140"
] | [] | TAGS
#license-mit #arxiv-2212.11140 #region-us
| ---
pipeline_tag: text-generation
tags:
- code
model-index:
- name: VeriGen
results:
- task:
type: text-generation
dataset:
type:
name:
extra_gated_prompt: >-
## Model License Agreement
Please read the BigCode OpenRAIL-M
license
agreement before accepting it.
extra_gated_fields:
I accept the above license agreement, and will use the Model complying with the set of use restrictions and sharing requirements: checkbox
---
# VeriGen
## Table of Contents
1. Dataset Summary
2. Use
3. Limitations
4. License
5. Citation
## Dataset Summary
- The dataset comprises Verilog modules as entries. The entries were retrieved from the GitHub dataset on BigQuery.
- For training [models (URL we filtered entries with no of characters exceeding 20000 and duplicates (exact duplicates ignoring whitespaces).
- Paper: Benchmarking Large Language Models for Automated Verilog RTL Code Generation
- Point of Contact: contact@shailja
- Languages: Verilog (Hardware Description Language)
### Data Splits
The dataset only contains a train split.
### Use
### Intended Use
The dataset consists of source code from a range of GitHub repositories. As such, they can potentially include non-compilable, low-quality, and vulnerable code.
### Attribution & Other Requirements
The pretraining dataset of the model was not filtered for permissive licenses only. Nevertheless, the model can generate source code verbatim from the dataset. The code's license might require attribution and/or other specific requirements that must be respected.
# License
The dataset is licensed under the BigCode OpenRAIL-M v1 license agreement. You can find the full agreement here.
| [
"## Model License Agreement\n\n Please read the BigCode OpenRAIL-M\n license\n agreement before accepting it.\n \nextra_gated_fields:\n I accept the above license agreement, and will use the Model complying with the set of use restrictions and sharing requirements: checkbox\n---",
"# VeriGen",
"## Table of Contents\n\n1. Dataset Summary\n2. Use\n3. Limitations\n4. License\n5. Citation",
"## Dataset Summary\n\n- The dataset comprises Verilog modules as entries. The entries were retrieved from the GitHub dataset on BigQuery. \n- For training [models (URL we filtered entries with no of characters exceeding 20000 and duplicates (exact duplicates ignoring whitespaces).\n\n- Paper: Benchmarking Large Language Models for Automated Verilog RTL Code Generation\n- Point of Contact: contact@shailja\n- Languages: Verilog (Hardware Description Language)",
"### Data Splits\n\nThe dataset only contains a train split.",
"### Use",
"### Intended Use\n\nThe dataset consists of source code from a range of GitHub repositories. As such, they can potentially include non-compilable, low-quality, and vulnerable code.",
"### Attribution & Other Requirements\n\nThe pretraining dataset of the model was not filtered for permissive licenses only. Nevertheless, the model can generate source code verbatim from the dataset. The code's license might require attribution and/or other specific requirements that must be respected.",
"# License\nThe dataset is licensed under the BigCode OpenRAIL-M v1 license agreement. You can find the full agreement here."
] | [
"TAGS\n#license-mit #arxiv-2212.11140 #region-us \n",
"## Model License Agreement\n\n Please read the BigCode OpenRAIL-M\n license\n agreement before accepting it.\n \nextra_gated_fields:\n I accept the above license agreement, and will use the Model complying with the set of use restrictions and sharing requirements: checkbox\n---",
"# VeriGen",
"## Table of Contents\n\n1. Dataset Summary\n2. Use\n3. Limitations\n4. License\n5. Citation",
"## Dataset Summary\n\n- The dataset comprises Verilog modules as entries. The entries were retrieved from the GitHub dataset on BigQuery. \n- For training [models (URL we filtered entries with no of characters exceeding 20000 and duplicates (exact duplicates ignoring whitespaces).\n\n- Paper: Benchmarking Large Language Models for Automated Verilog RTL Code Generation\n- Point of Contact: contact@shailja\n- Languages: Verilog (Hardware Description Language)",
"### Data Splits\n\nThe dataset only contains a train split.",
"### Use",
"### Intended Use\n\nThe dataset consists of source code from a range of GitHub repositories. As such, they can potentially include non-compilable, low-quality, and vulnerable code.",
"### Attribution & Other Requirements\n\nThe pretraining dataset of the model was not filtered for permissive licenses only. Nevertheless, the model can generate source code verbatim from the dataset. The code's license might require attribution and/or other specific requirements that must be respected.",
"# License\nThe dataset is licensed under the BigCode OpenRAIL-M v1 license agreement. You can find the full agreement here."
] |
1b7a925e3c7cb0ed9d0dd32b08f269f9585040a0 | # Dataset Card for "ps_news_2020_100K-sentences_processed"
[More Information needed](https://github.com/huggingface/datasets/blob/main/CONTRIBUTING.md#how-to-contribute-to-the-dataset-cards) | ihanif/ps_news_2020_100K-sentences_processed | [
"size_categories:10K<n<100K",
"language:ps",
"region:us"
] | 2022-12-19T15:32:22+00:00 | {"language": ["ps"], "size_categories": ["10K<n<100K"], "pretty_name": "Pashto News 100K Sentences Cleaned", "dataset_info": {"features": [{"name": "text", "dtype": "string"}], "splits": [{"name": "train", "num_bytes": 20452491, "num_examples": 100000}], "download_size": 10143557, "dataset_size": 20452491}} | 2023-06-16T19:53:34+00:00 | [] | [
"ps"
] | TAGS
#size_categories-10K<n<100K #language-Pushto #region-us
| # Dataset Card for "ps_news_2020_100K-sentences_processed"
More Information needed | [
"# Dataset Card for \"ps_news_2020_100K-sentences_processed\"\n\nMore Information needed"
] | [
"TAGS\n#size_categories-10K<n<100K #language-Pushto #region-us \n",
"# Dataset Card for \"ps_news_2020_100K-sentences_processed\"\n\nMore Information needed"
] |
f1165fe5fee96f2540196dc7e9ab50053de56e91 | # Dataset Card for "hamburgers"
[More Information needed](https://github.com/huggingface/datasets/blob/main/CONTRIBUTING.md#how-to-contribute-to-the-dataset-cards) | lewtun/hamburgers | [
"region:us"
] | 2022-12-19T15:53:09+00:00 | {"dataset_info": {"features": [{"name": "image", "dtype": "image"}], "splits": [{"name": "train", "num_bytes": 22977927.0, "num_examples": 10}], "download_size": 22973038, "dataset_size": 22977927.0}} | 2022-12-19T15:53:42+00:00 | [] | [] | TAGS
#region-us
| # Dataset Card for "hamburgers"
More Information needed | [
"# Dataset Card for \"hamburgers\"\n\nMore Information needed"
] | [
"TAGS\n#region-us \n",
"# Dataset Card for \"hamburgers\"\n\nMore Information needed"
] |
0d7a5476f7420b21dc9f4807fdf766c821ad9cb1 | # Dataset Card for "alps"
[More Information needed](https://github.com/huggingface/datasets/blob/main/CONTRIBUTING.md#how-to-contribute-to-the-dataset-cards) | lewtun/alps | [
"region:us"
] | 2022-12-19T17:30:05+00:00 | {"dataset_info": {"features": [{"name": "image", "dtype": "image"}], "splits": [{"name": "train", "num_bytes": 27913166.0, "num_examples": 10}], "download_size": 27914963, "dataset_size": 27913166.0}} | 2022-12-19T17:30:44+00:00 | [] | [] | TAGS
#region-us
| # Dataset Card for "alps"
More Information needed | [
"# Dataset Card for \"alps\"\n\nMore Information needed"
] | [
"TAGS\n#region-us \n",
"# Dataset Card for \"alps\"\n\nMore Information needed"
] |
09688bd198af1dea3646f3df4a7c75907ae8a15f | # Dataset Card for "turkishReviews-ds-mini"
[More Information needed](https://github.com/huggingface/datasets/blob/main/CONTRIBUTING.md#how-to-contribute-to-the-dataset-cards) | ozz/turkishReviews-ds-mini | [
"region:us"
] | 2022-12-19T18:31:32+00:00 | {"dataset_info": {"features": [{"name": "review", "dtype": "string"}, {"name": "review_length", "dtype": "int64"}], "splits": [{"name": "train", "num_bytes": 134598991.2416305, "num_examples": 362520}, {"name": "validation", "num_bytes": 14955814.758369517, "num_examples": 40281}], "download_size": 95987466, "dataset_size": 149554806.0}} | 2022-12-19T18:33:37+00:00 | [] | [] | TAGS
#region-us
| # Dataset Card for "turkishReviews-ds-mini"
More Information needed | [
"# Dataset Card for \"turkishReviews-ds-mini\"\n\nMore Information needed"
] | [
"TAGS\n#region-us \n",
"# Dataset Card for \"turkishReviews-ds-mini\"\n\nMore Information needed"
] |
a34376c527c1ff080d71cd5a863ec4e6d696133f | # Dataset Card for "muppet-blip-captions"
[More Information needed](https://github.com/huggingface/datasets/blob/main/CONTRIBUTING.md#how-to-contribute-to-the-dataset-cards) | Norod78/muppet-blip-captions | [
"region:us"
] | 2022-12-19T19:47:56+00:00 | {"dataset_info": {"features": [{"name": "image", "dtype": "image"}, {"name": "text", "dtype": "string"}], "splits": [{"name": "train", "num_bytes": 318180055.0, "num_examples": 976}], "download_size": 316787074, "dataset_size": 318180055.0}} | 2022-12-19T19:48:43+00:00 | [] | [] | TAGS
#region-us
| # Dataset Card for "muppet-blip-captions"
More Information needed | [
"# Dataset Card for \"muppet-blip-captions\"\n\nMore Information needed"
] | [
"TAGS\n#region-us \n",
"# Dataset Card for \"muppet-blip-captions\"\n\nMore Information needed"
] |
8c54c9f78834d91ebb4541fe55388c536735d1b6 |
# Dataset Card for IMDB Kurdish
## Table of Contents
- [Table of Contents](#table-of-contents)
- [Dataset Description](#dataset-description)
- [Dataset Summary](#dataset-summary)
- [Supported Tasks and Leaderboards](#supported-tasks-and-leaderboards)
- [Languages](#languages)
- [Dataset Structure](#dataset-structure)
- [Data Instances](#data-instances)
- [Data Fields](#data-fields)
- [Data Splits](#data-splits)
- [Dataset Creation](#dataset-creation)
- [Curation Rationale](#curation-rationale)
- [Source Data](#source-data)
- [Annotations](#annotations)
- [Personal and Sensitive Information](#personal-and-sensitive-information)
- [Considerations for Using the Data](#considerations-for-using-the-data)
- [Social Impact of Dataset](#social-impact-of-dataset)
- [Discussion of Biases](#discussion-of-biases)
- [Other Known Limitations](#other-known-limitations)
- [Additional Information](#additional-information)
- [Dataset Curators](#dataset-curators)
- [Licensing Information](#licensing-information)
- [Citation Information](#citation-information)
- [Contributions](#contributions)
## Dataset Description
- **Homepage:** [http://ai.stanford.edu/~amaas/data/sentiment/](http://ai.stanford.edu/~amaas/data/sentiment/)
- **Repository:** [https://github.com/Hrazhan/IMDB_Kurdish/](https://github.com/Hrazhan/IMDB_Kurdish/)
- **Point of Contact:** [Razhan Hameed](https://twitter.com/RazhanHameed)
- **Paper:**
- **Leaderboard:**
### Dataset Summary
Central Kurdish translation of the famous IMDB movie reviews dataset.
The dataset contains 50K highly polar movie reviews, divided into two equal classes of positive and negative reviews. We can perform binary sentiment classification using this dataset.
The availability of datasets in Kurdish, such as the IMDB movie reviews dataset, can help researchers and developers train and evaluate machine learning models for Kurdish language processing.
However, it is important to note that machine learning algorithms can only be as accurate as the data they are trained on (in this case the quality of the translation), so the quality and relevance of the dataset will affect the performance of the resulting model.
For more information about the dataset, please go through the following link: http://ai.stanford.edu/~amaas/data/sentiment/
P.S. This dataset is translated with Google Translator.
### Supported Tasks and Leaderboards
[More Information Needed]
### Languages
Central Kurdish
## Dataset Structure
### Data Instances
An example of 'train' looks as follows.
```
{
"label": 0,
"text": ""فیلمێکی زۆر باش، کە سەرنج دەخاتە سەر پرسێکی زۆر گرنگ. نەخۆشی کحولی کۆرپەلە کەموکوڕییەکی زۆر جددی لە لەدایکبوونە کە بە تەواوی دەتوانرێت ڕێگری لێبکرێت. ئەگەر خێزانە زیاترەکان ئەم فیلمە ببینن، ڕەنگە منداڵی زیاتر وەک ئادەم کۆتاییان نەهاتبێت. جیمی سمیس لە یەکێک لە باشترین ڕۆڵەکانیدا نمایش دەکات تا ئێستا. ئەمە فیلمێکی نایاب و باشە کە خێزانێکی زۆر تایبەت لەبەرچاو دەگرێت و پێویستییەکی زۆر گرنگی هەیە. ئەمەش جیاواز نییە لە هەزاران خێزان کە ئەمڕۆ لە ئەمریکا هەن. منداڵان هەن کە لەگەڵ ئەم جیهانەدا خەبات دەکەن. بەڕاستی خاڵە گرنگەکە لێرەدا ئەوەیە کە دەکرا ڕێگری لە هەموو شتێک بکرێت. خەڵکی زیاتر دەبێ ئەم فیلمە ببینن و ئەوەی کە هەیەتی بە جددی وەریبگرێت. بە باشی ئەنجام دراوە، بە پەیامی گرنگ، بە شێوەیەکی بەڕێزانە مامەڵەی لەگەڵ دەکرێت."
}
```
### Data Fields
plain_text
text: a string feature.
label: a classification label, with possible values including neg (0), pos (1).
### Data Splits
| name |train|test|
|----------|----:|----:|
|plain_text|24903|24692|
## Dataset Creation
### Curation Rationale
[More Information Needed]
### Source Data
#### Initial Data Collection and Normalization
[More Information Needed]
#### Who are the source language producers?
[More Information Needed]
### Annotations
#### Annotation process
[More Information Needed]
#### Who are the annotators?
[More Information Needed]
### Personal and Sensitive Information
[More Information Needed]
## Considerations for Using the Data
### Social Impact of Dataset
[More Information Needed]
### Discussion of Biases
[More Information Needed]
### Other Known Limitations
[More Information Needed]
## Additional Information
### Dataset Curators
[More Information Needed]
### Licensing Information
[More Information Needed]
### Citation Information
```
@InProceedings{maas-EtAl:2011:ACL-HLT2011,
author = {Maas, Andrew L. and Daly, Raymond E. and Pham, Peter T. and Huang, Dan and Ng, Andrew Y. and Potts, Christopher},
title = {Learning Word Vectors for Sentiment Analysis},
booktitle = {Proceedings of the 49th Annual Meeting of the Association for Computational Linguistics: Human Language Technologies},
month = {June},
year = {2011},
address = {Portland, Oregon, USA},
publisher = {Association for Computational Linguistics},
pages = {142--150},
url = {http://www.aclweb.org/anthology/P11-1015}
}
```
### Contributions
Thanks to [Razhan Hameed](https://twitter.com/RazhanHameed) for adding this dataset.
| razhan/imdb_ckb | [
"task_categories:text-classification",
"task_ids:sentiment-analysis",
"task_ids:sentiment-classification",
"annotations_creators:expert-generated",
"language_creators:crowdsourced",
"multilinguality:monolingual",
"size_categories:10K<n<100K",
"source_datasets:extended|imdb",
"language:ckb",
"language:ku",
"license:other",
"central kurdish",
"kurdish",
"sorani",
"kurdi",
"region:us"
] | 2022-12-19T20:31:55+00:00 | {"annotations_creators": ["expert-generated"], "language_creators": ["crowdsourced"], "language": ["ckb", "ku"], "license": ["other"], "multilinguality": ["monolingual"], "size_categories": ["10K<n<100K"], "source_datasets": ["extended|imdb"], "task_categories": ["text-classification"], "task_ids": ["sentiment-analysis", "sentiment-classification"], "pretty_name": "IMDB_CKB", "tags": ["central kurdish", "kurdish", "sorani", "kurdi"], "dataset_info": {"features": [{"name": "text", "dtype": "string"}, {"name": "label", "dtype": {"class_label": {"names": {"0": "neg", "1": "pos"}}}}], "config_name": "plain_text", "splits": [{"name": "train", "num_examples": 24903}, {"name": "test", "num_examples": 24692}]}} | 2023-01-13T17:41:39+00:00 | [] | [
"ckb",
"ku"
] | TAGS
#task_categories-text-classification #task_ids-sentiment-analysis #task_ids-sentiment-classification #annotations_creators-expert-generated #language_creators-crowdsourced #multilinguality-monolingual #size_categories-10K<n<100K #source_datasets-extended|imdb #language-Central Kurdish #language-Kurdish #license-other #central kurdish #kurdish #sorani #kurdi #region-us
| Dataset Card for IMDB Kurdish
=============================
Table of Contents
-----------------
* Table of Contents
* Dataset Description
+ Dataset Summary
+ Supported Tasks and Leaderboards
+ Languages
* Dataset Structure
+ Data Instances
+ Data Fields
+ Data Splits
* Dataset Creation
+ Curation Rationale
+ Source Data
+ Annotations
+ Personal and Sensitive Information
* Considerations for Using the Data
+ Social Impact of Dataset
+ Discussion of Biases
+ Other Known Limitations
* Additional Information
+ Dataset Curators
+ Licensing Information
+ Citation Information
+ Contributions
Dataset Description
-------------------
* Homepage: URL
* Repository: URL
* Point of Contact: Razhan Hameed
* Paper:
* Leaderboard:
### Dataset Summary
Central Kurdish translation of the famous IMDB movie reviews dataset.
The dataset contains 50K highly polar movie reviews, divided into two equal classes of positive and negative reviews. We can perform binary sentiment classification using this dataset.
The availability of datasets in Kurdish, such as the IMDB movie reviews dataset, can help researchers and developers train and evaluate machine learning models for Kurdish language processing.
However, it is important to note that machine learning algorithms can only be as accurate as the data they are trained on (in this case the quality of the translation), so the quality and relevance of the dataset will affect the performance of the resulting model.
For more information about the dataset, please go through the following link: URL
P.S. This dataset is translated with Google Translator.
### Supported Tasks and Leaderboards
### Languages
Central Kurdish
Dataset Structure
-----------------
### Data Instances
An example of 'train' looks as follows.
### Data Fields
plain\_text
```
text: a string feature.
label: a classification label, with possible values including neg (0), pos (1).
```
### Data Splits
Dataset Creation
----------------
### Curation Rationale
### Source Data
#### Initial Data Collection and Normalization
#### Who are the source language producers?
### Annotations
#### Annotation process
#### Who are the annotators?
### Personal and Sensitive Information
Considerations for Using the Data
---------------------------------
### Social Impact of Dataset
### Discussion of Biases
### Other Known Limitations
Additional Information
----------------------
### Dataset Curators
### Licensing Information
### Contributions
Thanks to Razhan Hameed for adding this dataset.
| [
"### Dataset Summary\n\n\nCentral Kurdish translation of the famous IMDB movie reviews dataset.\n\n\nThe dataset contains 50K highly polar movie reviews, divided into two equal classes of positive and negative reviews. We can perform binary sentiment classification using this dataset.\nThe availability of datasets in Kurdish, such as the IMDB movie reviews dataset, can help researchers and developers train and evaluate machine learning models for Kurdish language processing.\nHowever, it is important to note that machine learning algorithms can only be as accurate as the data they are trained on (in this case the quality of the translation), so the quality and relevance of the dataset will affect the performance of the resulting model.\nFor more information about the dataset, please go through the following link: URL\n\n\nP.S. This dataset is translated with Google Translator.",
"### Supported Tasks and Leaderboards",
"### Languages\n\n\nCentral Kurdish\n\n\nDataset Structure\n-----------------",
"### Data Instances\n\n\nAn example of 'train' looks as follows.",
"### Data Fields\n\n\nplain\\_text\n\n\n\n```\ntext: a string feature.\nlabel: a classification label, with possible values including neg (0), pos (1).\n\n```",
"### Data Splits\n\n\n\nDataset Creation\n----------------",
"### Curation Rationale",
"### Source Data",
"#### Initial Data Collection and Normalization",
"#### Who are the source language producers?",
"### Annotations",
"#### Annotation process",
"#### Who are the annotators?",
"### Personal and Sensitive Information\n\n\nConsiderations for Using the Data\n---------------------------------",
"### Social Impact of Dataset",
"### Discussion of Biases",
"### Other Known Limitations\n\n\nAdditional Information\n----------------------",
"### Dataset Curators",
"### Licensing Information",
"### Contributions\n\n\nThanks to Razhan Hameed for adding this dataset."
] | [
"TAGS\n#task_categories-text-classification #task_ids-sentiment-analysis #task_ids-sentiment-classification #annotations_creators-expert-generated #language_creators-crowdsourced #multilinguality-monolingual #size_categories-10K<n<100K #source_datasets-extended|imdb #language-Central Kurdish #language-Kurdish #license-other #central kurdish #kurdish #sorani #kurdi #region-us \n",
"### Dataset Summary\n\n\nCentral Kurdish translation of the famous IMDB movie reviews dataset.\n\n\nThe dataset contains 50K highly polar movie reviews, divided into two equal classes of positive and negative reviews. We can perform binary sentiment classification using this dataset.\nThe availability of datasets in Kurdish, such as the IMDB movie reviews dataset, can help researchers and developers train and evaluate machine learning models for Kurdish language processing.\nHowever, it is important to note that machine learning algorithms can only be as accurate as the data they are trained on (in this case the quality of the translation), so the quality and relevance of the dataset will affect the performance of the resulting model.\nFor more information about the dataset, please go through the following link: URL\n\n\nP.S. This dataset is translated with Google Translator.",
"### Supported Tasks and Leaderboards",
"### Languages\n\n\nCentral Kurdish\n\n\nDataset Structure\n-----------------",
"### Data Instances\n\n\nAn example of 'train' looks as follows.",
"### Data Fields\n\n\nplain\\_text\n\n\n\n```\ntext: a string feature.\nlabel: a classification label, with possible values including neg (0), pos (1).\n\n```",
"### Data Splits\n\n\n\nDataset Creation\n----------------",
"### Curation Rationale",
"### Source Data",
"#### Initial Data Collection and Normalization",
"#### Who are the source language producers?",
"### Annotations",
"#### Annotation process",
"#### Who are the annotators?",
"### Personal and Sensitive Information\n\n\nConsiderations for Using the Data\n---------------------------------",
"### Social Impact of Dataset",
"### Discussion of Biases",
"### Other Known Limitations\n\n\nAdditional Information\n----------------------",
"### Dataset Curators",
"### Licensing Information",
"### Contributions\n\n\nThanks to Razhan Hameed for adding this dataset."
] |
280bfaff1399973dea85fe9a9fe9085ac7ca171f | # Dataset Card for AutoTrain Evaluator
This repository contains model predictions generated by [AutoTrain](https://huggingface.co/autotrain) for the following task and dataset:
* Task: Zero-Shot Text Classification
* Model: BirdL/OLM-GPT2-Yannic
* Dataset: mathemakitten/winobias_antistereotype_dev
* Config: mathemakitten--winobias_antistereotype_dev
* Split: validation
To run new evaluation jobs, visit Hugging Face's [automatic model evaluator](https://huggingface.co/spaces/autoevaluate/model-evaluator).
## Contributions
Thanks to [@puffy310](https://huggingface.co/puffy310) for evaluating this model. | autoevaluate/autoeval-eval-mathemakitten__winobias_antistereotype_dev-mathemakitte-398e1c-2536177709 | [
"autotrain",
"evaluation",
"region:us"
] | 2022-12-19T22:03:24+00:00 | {"type": "predictions", "tags": ["autotrain", "evaluation"], "datasets": ["mathemakitten/winobias_antistereotype_dev"], "eval_info": {"task": "text_zero_shot_classification", "model": "BirdL/OLM-GPT2-Yannic", "metrics": [], "dataset_name": "mathemakitten/winobias_antistereotype_dev", "dataset_config": "mathemakitten--winobias_antistereotype_dev", "dataset_split": "validation", "col_mapping": {"text": "text", "classes": "classes", "target": "target"}}} | 2022-12-19T22:04:15+00:00 | [] | [] | TAGS
#autotrain #evaluation #region-us
| # Dataset Card for AutoTrain Evaluator
This repository contains model predictions generated by AutoTrain for the following task and dataset:
* Task: Zero-Shot Text Classification
* Model: BirdL/OLM-GPT2-Yannic
* Dataset: mathemakitten/winobias_antistereotype_dev
* Config: mathemakitten--winobias_antistereotype_dev
* Split: validation
To run new evaluation jobs, visit Hugging Face's automatic model evaluator.
## Contributions
Thanks to @puffy310 for evaluating this model. | [
"# Dataset Card for AutoTrain Evaluator\n\nThis repository contains model predictions generated by AutoTrain for the following task and dataset:\n\n* Task: Zero-Shot Text Classification\n* Model: BirdL/OLM-GPT2-Yannic\n* Dataset: mathemakitten/winobias_antistereotype_dev\n* Config: mathemakitten--winobias_antistereotype_dev\n* Split: validation\n\nTo run new evaluation jobs, visit Hugging Face's automatic model evaluator.",
"## Contributions\n\nThanks to @puffy310 for evaluating this model."
] | [
"TAGS\n#autotrain #evaluation #region-us \n",
"# Dataset Card for AutoTrain Evaluator\n\nThis repository contains model predictions generated by AutoTrain for the following task and dataset:\n\n* Task: Zero-Shot Text Classification\n* Model: BirdL/OLM-GPT2-Yannic\n* Dataset: mathemakitten/winobias_antistereotype_dev\n* Config: mathemakitten--winobias_antistereotype_dev\n* Split: validation\n\nTo run new evaluation jobs, visit Hugging Face's automatic model evaluator.",
"## Contributions\n\nThanks to @puffy310 for evaluating this model."
] |
f888c2e82ccf3939b486e1ecd6b44b82b5f4594c | All the datasets from https://huggingface.co/Whispering-GPT concated together to finetune [OLM-GPT2](https://huggingface.co/Tristan/olm-gpt2-oct-2022) | BirdL/WhisperGPTFull | [
"license:apache-2.0",
"region:us"
] | 2022-12-20T01:00:27+00:00 | {"license": "apache-2.0"} | 2022-12-20T01:04:24+00:00 | [] | [] | TAGS
#license-apache-2.0 #region-us
| All the datasets from URL concated together to finetune OLM-GPT2 | [] | [
"TAGS\n#license-apache-2.0 #region-us \n"
] |
6af9b0e29d574b3d0a067c2c47ac75f8a9e256be | # Dataset Card for "DalleCatsAndDogs"
[More Information needed](https://github.com/huggingface/datasets/blob/main/CONTRIBUTING.md#how-to-contribute-to-the-dataset-cards) | BirdL/DalleCatsAndDogs | [
"region:us"
] | 2022-12-20T04:33:34+00:00 | {"dataset_info": {"features": [{"name": "Images", "dtype": "image"}, {"name": "class", "dtype": "string"}], "splits": [{"name": "train", "num_bytes": 49662722.0, "num_examples": 500}], "download_size": 49664703, "dataset_size": 49662722.0}} | 2022-12-20T04:50:34+00:00 | [] | [] | TAGS
#region-us
| # Dataset Card for "DalleCatsAndDogs"
More Information needed | [
"# Dataset Card for \"DalleCatsAndDogs\"\n\nMore Information needed"
] | [
"TAGS\n#region-us \n",
"# Dataset Card for \"DalleCatsAndDogs\"\n\nMore Information needed"
] |
dba2660b61c1a1f82372a0b1443aad3bd2922483 | # Dataset Card for "cathode-1"
[More Information needed](https://github.com/huggingface/datasets/blob/main/CONTRIBUTING.md#how-to-contribute-to-the-dataset-cards) | AxuJI/cathode-1 | [
"region:us"
] | 2022-12-20T06:25:00+00:00 | {"dataset_info": {"features": [{"name": "pixel_values", "dtype": "image"}, {"name": "label", "dtype": "image"}], "splits": [{"name": "train", "num_bytes": 55347464.0, "num_examples": 56}], "download_size": 51606062, "dataset_size": 55347464.0}} | 2022-12-20T07:48:02+00:00 | [] | [] | TAGS
#region-us
| # Dataset Card for "cathode-1"
More Information needed | [
"# Dataset Card for \"cathode-1\"\n\nMore Information needed"
] | [
"TAGS\n#region-us \n",
"# Dataset Card for \"cathode-1\"\n\nMore Information needed"
] |
175c10ffdb77fd920f8ab86b735331282be1ee18 |
# CISLR: Corpus for Indian Sign Language Recognition
This repository contains the Indian Sign Language Dataset proposed in the following paper
> **Paper:** CISLR: Corpus for Indian Sign Language Recognition https://preview.aclanthology.org/emnlp-22-ingestion/2022.emnlp-main.707/
> **Authors:** Abhinav Joshi, Ashwani Bhat, Pradeep S, Priya Gole, Shashwat Gupta, Shreyansh Agarwal, Ashutosh Modi <br>
>
> **Abstract:** *Indian Sign Language, though used by a diverse community, still lacks well-annotated resources for developing systems that would enable sign language processing. In recent years researchers have actively worked for sign languages like American Sign Languages, however, Indian Sign language is still far from data-driven tasks like machine translation. To address this gap, in this paper, we introduce a new dataset CISLR (Corpus for Indian Sign Language Recognition) for word-level recognition in Indian Sign Language using videos. The corpus has a large vocabulary of around 4700 words covering different topics and domains. Further, we propose a baseline model for word recognition from sign language videos. To handle the low resource problem in the Indian Sign Language, the proposed model consists of a prototype-based one-shot learner that leverages resource rich American Sign Language to learn generalized features for improving predictions in Indian Sign Language. Our experiments show that gesture features learned in another sign language can help perform one-shot predictions in CISLR.*
## Directory Structure
.
├── dataset.csv # list of all videos with categorical annotations
├── prototype.csv # files used as prototypes
├── test.csv # files used as testset
├── CISLR_v1.5-a_videos # dataset videos
├── __Rz2PaTB1c.mp4
├── _2TlWc7fctg.mp4
.
.
├── zZVuyuVTFW0.mp4
└── I3D_features.pkl # extracted Inception3D features
## Citation
> Abhinav Joshi, Ashwani Bhat, Pradeep S, Priya Gole, Shashwat Gupta, Shreyansh Agarwal, and Ashutosh Modi. 2022. CISLR: Corpus for Indian Sign Language Recognition. In Proceedings of the 2022 Conference on Empirical Methods in Natural Language Processing, pages 10357–10366, Abu Dhabi, United Arab Emirates. Association for Computational Linguistics.
## Acknowledgments
This project was a part of IIT Kanpur's [SURGE](https://surge.iitk.ac.in/) Initiative. | IIT-K/CISLR | [
"multilinguality:translation",
"size_categories:1K<n<10K",
"source_datasets:original",
"language:sgn",
"license:afl-3.0",
"Indian Sign Language",
"Sign Language Recognition",
"region:us"
] | 2022-12-20T07:42:08+00:00 | {"language": ["sgn"], "license": ["afl-3.0"], "multilinguality": ["translation"], "size_categories": ["1K<n<10K"], "source_datasets": ["original"], "pretty_name": "CISLR", "tags": ["Indian Sign Language", "Sign Language Recognition"]} | 2022-12-20T08:39:26+00:00 | [] | [
"sgn"
] | TAGS
#multilinguality-translation #size_categories-1K<n<10K #source_datasets-original #language-sgn #license-afl-3.0 #Indian Sign Language #Sign Language Recognition #region-us
|
# CISLR: Corpus for Indian Sign Language Recognition
This repository contains the Indian Sign Language Dataset proposed in the following paper
> Paper: CISLR: Corpus for Indian Sign Language Recognition URL
> Authors: Abhinav Joshi, Ashwani Bhat, Pradeep S, Priya Gole, Shashwat Gupta, Shreyansh Agarwal, Ashutosh Modi <br>
>
> Abstract: *Indian Sign Language, though used by a diverse community, still lacks well-annotated resources for developing systems that would enable sign language processing. In recent years researchers have actively worked for sign languages like American Sign Languages, however, Indian Sign language is still far from data-driven tasks like machine translation. To address this gap, in this paper, we introduce a new dataset CISLR (Corpus for Indian Sign Language Recognition) for word-level recognition in Indian Sign Language using videos. The corpus has a large vocabulary of around 4700 words covering different topics and domains. Further, we propose a baseline model for word recognition from sign language videos. To handle the low resource problem in the Indian Sign Language, the proposed model consists of a prototype-based one-shot learner that leverages resource rich American Sign Language to learn generalized features for improving predictions in Indian Sign Language. Our experiments show that gesture features learned in another sign language can help perform one-shot predictions in CISLR.*
## Directory Structure
.
├── URL # list of all videos with categorical annotations
├── URL # files used as prototypes
├── URL # files used as testset
├── CISLR_v1.5-a_videos # dataset videos
├── __Rz2PaTB1c.mp4
├── _2TlWc7fctg.mp4
.
.
├── zZVuyuVTFW0.mp4
└── I3D_features.pkl # extracted Inception3D features
> Abhinav Joshi, Ashwani Bhat, Pradeep S, Priya Gole, Shashwat Gupta, Shreyansh Agarwal, and Ashutosh Modi. 2022. CISLR: Corpus for Indian Sign Language Recognition. In Proceedings of the 2022 Conference on Empirical Methods in Natural Language Processing, pages 10357–10366, Abu Dhabi, United Arab Emirates. Association for Computational Linguistics.
## Acknowledgments
This project was a part of IIT Kanpur's SURGE Initiative. | [
"# CISLR: Corpus for Indian Sign Language Recognition\n\nThis repository contains the Indian Sign Language Dataset proposed in the following paper\n\n> Paper: CISLR: Corpus for Indian Sign Language Recognition URL\n\n> Authors: Abhinav Joshi, Ashwani Bhat, Pradeep S, Priya Gole, Shashwat Gupta, Shreyansh Agarwal, Ashutosh Modi <br>\n>\n> Abstract: *Indian Sign Language, though used by a diverse community, still lacks well-annotated resources for developing systems that would enable sign language processing. In recent years researchers have actively worked for sign languages like American Sign Languages, however, Indian Sign language is still far from data-driven tasks like machine translation. To address this gap, in this paper, we introduce a new dataset CISLR (Corpus for Indian Sign Language Recognition) for word-level recognition in Indian Sign Language using videos. The corpus has a large vocabulary of around 4700 words covering different topics and domains. Further, we propose a baseline model for word recognition from sign language videos. To handle the low resource problem in the Indian Sign Language, the proposed model consists of a prototype-based one-shot learner that leverages resource rich American Sign Language to learn generalized features for improving predictions in Indian Sign Language. Our experiments show that gesture features learned in another sign language can help perform one-shot predictions in CISLR.*",
"## Directory Structure\n\n .\n ├── URL # list of all videos with categorical annotations\n ├── URL # files used as prototypes \n ├── URL # files used as testset\n ├── CISLR_v1.5-a_videos # dataset videos\n ├── __Rz2PaTB1c.mp4\n ├── _2TlWc7fctg.mp4\n .\n .\n ├── zZVuyuVTFW0.mp4\n └── I3D_features.pkl # extracted Inception3D features\n\n\n\n> Abhinav Joshi, Ashwani Bhat, Pradeep S, Priya Gole, Shashwat Gupta, Shreyansh Agarwal, and Ashutosh Modi. 2022. CISLR: Corpus for Indian Sign Language Recognition. In Proceedings of the 2022 Conference on Empirical Methods in Natural Language Processing, pages 10357–10366, Abu Dhabi, United Arab Emirates. Association for Computational Linguistics.",
"## Acknowledgments\nThis project was a part of IIT Kanpur's SURGE Initiative."
] | [
"TAGS\n#multilinguality-translation #size_categories-1K<n<10K #source_datasets-original #language-sgn #license-afl-3.0 #Indian Sign Language #Sign Language Recognition #region-us \n",
"# CISLR: Corpus for Indian Sign Language Recognition\n\nThis repository contains the Indian Sign Language Dataset proposed in the following paper\n\n> Paper: CISLR: Corpus for Indian Sign Language Recognition URL\n\n> Authors: Abhinav Joshi, Ashwani Bhat, Pradeep S, Priya Gole, Shashwat Gupta, Shreyansh Agarwal, Ashutosh Modi <br>\n>\n> Abstract: *Indian Sign Language, though used by a diverse community, still lacks well-annotated resources for developing systems that would enable sign language processing. In recent years researchers have actively worked for sign languages like American Sign Languages, however, Indian Sign language is still far from data-driven tasks like machine translation. To address this gap, in this paper, we introduce a new dataset CISLR (Corpus for Indian Sign Language Recognition) for word-level recognition in Indian Sign Language using videos. The corpus has a large vocabulary of around 4700 words covering different topics and domains. Further, we propose a baseline model for word recognition from sign language videos. To handle the low resource problem in the Indian Sign Language, the proposed model consists of a prototype-based one-shot learner that leverages resource rich American Sign Language to learn generalized features for improving predictions in Indian Sign Language. Our experiments show that gesture features learned in another sign language can help perform one-shot predictions in CISLR.*",
"## Directory Structure\n\n .\n ├── URL # list of all videos with categorical annotations\n ├── URL # files used as prototypes \n ├── URL # files used as testset\n ├── CISLR_v1.5-a_videos # dataset videos\n ├── __Rz2PaTB1c.mp4\n ├── _2TlWc7fctg.mp4\n .\n .\n ├── zZVuyuVTFW0.mp4\n └── I3D_features.pkl # extracted Inception3D features\n\n\n\n> Abhinav Joshi, Ashwani Bhat, Pradeep S, Priya Gole, Shashwat Gupta, Shreyansh Agarwal, and Ashutosh Modi. 2022. CISLR: Corpus for Indian Sign Language Recognition. In Proceedings of the 2022 Conference on Empirical Methods in Natural Language Processing, pages 10357–10366, Abu Dhabi, United Arab Emirates. Association for Computational Linguistics.",
"## Acknowledgments\nThis project was a part of IIT Kanpur's SURGE Initiative."
] |
Subsets and Splits
No community queries yet
The top public SQL queries from the community will appear here once available.