sha
stringlengths 40
40
| text
stringlengths 1
13.4M
| id
stringlengths 2
117
| tags
sequencelengths 1
7.91k
| created_at
stringlengths 25
25
| metadata
stringlengths 2
875k
| last_modified
stringlengths 25
25
| arxiv
sequencelengths 0
25
| languages
sequencelengths 0
7.91k
| tags_str
stringlengths 17
159k
| text_str
stringlengths 1
447k
| text_lists
sequencelengths 0
352
| processed_texts
sequencelengths 1
353
|
---|---|---|---|---|---|---|---|---|---|---|---|---|
7cd8020a4fd1f511fa6e94949800cc6e68410027 | This dataset contains images used in the documentation of HuggingFace's Optimum library. | optimum/documentation-images | [
"region:us"
] | 2022-11-29T12:47:16+00:00 | {} | 2023-11-29T14:42:17+00:00 | [] | [] | TAGS
#region-us
| This dataset contains images used in the documentation of HuggingFace's Optimum library. | [] | [
"TAGS\n#region-us \n"
] |
10ecf033e30880bf2bfbf51f7c1b88684ccfbb43 |
# Dataset Card for [Dataset Name]
## Table of Contents
- [Table of Contents](#table-of-contents)
- [Dataset Description](#dataset-description)
- [Dataset Summary](#dataset-summary)
- [Supported Tasks and Leaderboards](#supported-tasks-and-leaderboards)
- [Languages](#languages)
- [Dataset Structure](#dataset-structure)
- [Data Instances](#data-instances)
- [Data Fields](#data-fields)
- [Data Splits](#data-splits)
- [Dataset Creation](#dataset-creation)
- [Curation Rationale](#curation-rationale)
- [Source Data](#source-data)
- [Annotations](#annotations)
- [Personal and Sensitive Information](#personal-and-sensitive-information)
- [Considerations for Using the Data](#considerations-for-using-the-data)
- [Social Impact of Dataset](#social-impact-of-dataset)
- [Discussion of Biases](#discussion-of-biases)
- [Other Known Limitations](#other-known-limitations)
- [Additional Information](#additional-information)
- [Dataset Curators](#dataset-curators)
- [Licensing Information](#licensing-information)
- [Citation Information](#citation-information)
- [Contributions](#contributions)
## Dataset Description
- **Homepage:**
- **Repository:**
- **Paper:**
- **Leaderboard:**
- **Point of Contact:**
### Dataset Summary
[More Information Needed]
### Supported Tasks and Leaderboards
[More Information Needed]
### Languages
[More Information Needed]
## Dataset Structure
### Data Instances
[More Information Needed]
### Data Fields
[More Information Needed]
### Data Splits
[More Information Needed]
## Dataset Creation
### Curation Rationale
[More Information Needed]
### Source Data
#### Initial Data Collection and Normalization
[More Information Needed]
#### Who are the source language producers?
[More Information Needed]
### Annotations
#### Annotation process
[More Information Needed]
#### Who are the annotators?
[More Information Needed]
### Personal and Sensitive Information
[More Information Needed]
## Considerations for Using the Data
### Social Impact of Dataset
[More Information Needed]
### Discussion of Biases
[More Information Needed]
### Other Known Limitations
[More Information Needed]
## Additional Information
### Dataset Curators
[More Information Needed]
### Licensing Information
[More Information Needed]
### Citation Information
[More Information Needed]
### Contributions
Thanks to [@github-username](https://github.com/<github-username>) for adding this dataset. | DTU54DL/common-native | [
"task_categories:token-classification",
"annotations_creators:expert-generated",
"language_creators:found",
"multilinguality:monolingual",
"size_categories:10K<n<100K",
"source_datasets:original",
"language:en",
"license:mit",
"region:us"
] | 2022-11-29T13:46:08+00:00 | {"annotations_creators": ["expert-generated"], "language_creators": ["found"], "language": ["en"], "license": ["mit"], "multilinguality": ["monolingual"], "size_categories": ["10K<n<100K"], "source_datasets": ["original"], "task_categories": ["token-classification"], "task_ids": ["token-classification-other-acronym-identification"], "paperswithcode_id": "acronym-identification", "pretty_name": "Acronym Identification Dataset", "dataset_info": {"features": [{"name": "audio", "dtype": {"audio": {"sampling_rate": 16000}}}, {"name": "sentence", "dtype": "string"}, {"name": "accent", "dtype": "string"}], "splits": [{"name": "train", "num_bytes": 419902426.3910719, "num_examples": 10000}, {"name": "test", "num_bytes": 41430604.33704293, "num_examples": 994}], "download_size": 440738761, "dataset_size": 461333030.72811484}, "train-eval-index": [{"col_mapping": {"labels": "tags", "tokens": "tokens"}, "config": "default", "splits": {"eval_split": "test"}, "task": "token-classification", "task_id": "entity_extraction"}]} | 2022-11-30T05:41:32+00:00 | [] | [
"en"
] | TAGS
#task_categories-token-classification #annotations_creators-expert-generated #language_creators-found #multilinguality-monolingual #size_categories-10K<n<100K #source_datasets-original #language-English #license-mit #region-us
|
# Dataset Card for [Dataset Name]
## Table of Contents
- Table of Contents
- Dataset Description
- Dataset Summary
- Supported Tasks and Leaderboards
- Languages
- Dataset Structure
- Data Instances
- Data Fields
- Data Splits
- Dataset Creation
- Curation Rationale
- Source Data
- Annotations
- Personal and Sensitive Information
- Considerations for Using the Data
- Social Impact of Dataset
- Discussion of Biases
- Other Known Limitations
- Additional Information
- Dataset Curators
- Licensing Information
- Citation Information
- Contributions
## Dataset Description
- Homepage:
- Repository:
- Paper:
- Leaderboard:
- Point of Contact:
### Dataset Summary
### Supported Tasks and Leaderboards
### Languages
## Dataset Structure
### Data Instances
### Data Fields
### Data Splits
## Dataset Creation
### Curation Rationale
### Source Data
#### Initial Data Collection and Normalization
#### Who are the source language producers?
### Annotations
#### Annotation process
#### Who are the annotators?
### Personal and Sensitive Information
## Considerations for Using the Data
### Social Impact of Dataset
### Discussion of Biases
### Other Known Limitations
## Additional Information
### Dataset Curators
### Licensing Information
### Contributions
Thanks to @github-username for adding this dataset. | [
"# Dataset Card for [Dataset Name]",
"## Table of Contents\n- Table of Contents\n- Dataset Description\n - Dataset Summary\n - Supported Tasks and Leaderboards\n - Languages\n- Dataset Structure\n - Data Instances\n - Data Fields\n - Data Splits\n- Dataset Creation\n - Curation Rationale\n - Source Data\n - Annotations\n - Personal and Sensitive Information\n- Considerations for Using the Data\n - Social Impact of Dataset\n - Discussion of Biases\n - Other Known Limitations\n- Additional Information\n - Dataset Curators\n - Licensing Information\n - Citation Information\n - Contributions",
"## Dataset Description\n\n- Homepage:\n- Repository:\n- Paper:\n- Leaderboard:\n- Point of Contact:",
"### Dataset Summary",
"### Supported Tasks and Leaderboards",
"### Languages",
"## Dataset Structure",
"### Data Instances",
"### Data Fields",
"### Data Splits",
"## Dataset Creation",
"### Curation Rationale",
"### Source Data",
"#### Initial Data Collection and Normalization",
"#### Who are the source language producers?",
"### Annotations",
"#### Annotation process",
"#### Who are the annotators?",
"### Personal and Sensitive Information",
"## Considerations for Using the Data",
"### Social Impact of Dataset",
"### Discussion of Biases",
"### Other Known Limitations",
"## Additional Information",
"### Dataset Curators",
"### Licensing Information",
"### Contributions\n\nThanks to @github-username for adding this dataset."
] | [
"TAGS\n#task_categories-token-classification #annotations_creators-expert-generated #language_creators-found #multilinguality-monolingual #size_categories-10K<n<100K #source_datasets-original #language-English #license-mit #region-us \n",
"# Dataset Card for [Dataset Name]",
"## Table of Contents\n- Table of Contents\n- Dataset Description\n - Dataset Summary\n - Supported Tasks and Leaderboards\n - Languages\n- Dataset Structure\n - Data Instances\n - Data Fields\n - Data Splits\n- Dataset Creation\n - Curation Rationale\n - Source Data\n - Annotations\n - Personal and Sensitive Information\n- Considerations for Using the Data\n - Social Impact of Dataset\n - Discussion of Biases\n - Other Known Limitations\n- Additional Information\n - Dataset Curators\n - Licensing Information\n - Citation Information\n - Contributions",
"## Dataset Description\n\n- Homepage:\n- Repository:\n- Paper:\n- Leaderboard:\n- Point of Contact:",
"### Dataset Summary",
"### Supported Tasks and Leaderboards",
"### Languages",
"## Dataset Structure",
"### Data Instances",
"### Data Fields",
"### Data Splits",
"## Dataset Creation",
"### Curation Rationale",
"### Source Data",
"#### Initial Data Collection and Normalization",
"#### Who are the source language producers?",
"### Annotations",
"#### Annotation process",
"#### Who are the annotators?",
"### Personal and Sensitive Information",
"## Considerations for Using the Data",
"### Social Impact of Dataset",
"### Discussion of Biases",
"### Other Known Limitations",
"## Additional Information",
"### Dataset Curators",
"### Licensing Information",
"### Contributions\n\nThanks to @github-username for adding this dataset."
] |
b64163f010caf82f1d7393ea0d799459e089f69c | # Dataset Card for AutoTrain Evaluator
This repository contains model predictions generated by [AutoTrain](https://huggingface.co/autotrain) for the following task and dataset:
* Task: Binary Text Classification
* Model: autoevaluate/binary-classification
* Dataset: glue
* Config: sst2
* Split: validation
To run new evaluation jobs, visit Hugging Face's [automatic model evaluator](https://huggingface.co/spaces/autoevaluate/model-evaluator).
## Contributions
Thanks to [@lewtun](https://huggingface.co/lewtun) for evaluating this model. | autoevaluate/autoeval-staging-eval-project-ecaf0dbc-43a3-4513-bbcf-d0f372522232-109106 | [
"autotrain",
"evaluation",
"region:us"
] | 2022-11-29T14:07:50+00:00 | {"type": "predictions", "tags": ["autotrain", "evaluation"], "datasets": ["glue"], "eval_info": {"task": "binary_classification", "model": "autoevaluate/binary-classification", "metrics": ["matthews_correlation"], "dataset_name": "glue", "dataset_config": "sst2", "dataset_split": "validation", "col_mapping": {"text": "sentence", "target": "label"}}} | 2022-11-29T14:08:27+00:00 | [] | [] | TAGS
#autotrain #evaluation #region-us
| # Dataset Card for AutoTrain Evaluator
This repository contains model predictions generated by AutoTrain for the following task and dataset:
* Task: Binary Text Classification
* Model: autoevaluate/binary-classification
* Dataset: glue
* Config: sst2
* Split: validation
To run new evaluation jobs, visit Hugging Face's automatic model evaluator.
## Contributions
Thanks to @lewtun for evaluating this model. | [
"# Dataset Card for AutoTrain Evaluator\n\nThis repository contains model predictions generated by AutoTrain for the following task and dataset:\n\n* Task: Binary Text Classification\n* Model: autoevaluate/binary-classification\n* Dataset: glue\n* Config: sst2\n* Split: validation\n\nTo run new evaluation jobs, visit Hugging Face's automatic model evaluator.",
"## Contributions\n\nThanks to @lewtun for evaluating this model."
] | [
"TAGS\n#autotrain #evaluation #region-us \n",
"# Dataset Card for AutoTrain Evaluator\n\nThis repository contains model predictions generated by AutoTrain for the following task and dataset:\n\n* Task: Binary Text Classification\n* Model: autoevaluate/binary-classification\n* Dataset: glue\n* Config: sst2\n* Split: validation\n\nTo run new evaluation jobs, visit Hugging Face's automatic model evaluator.",
"## Contributions\n\nThanks to @lewtun for evaluating this model."
] |
469f8914c49ddeecd0dc060a315a3d2077dd4209 |
# Dataset Card for ccGigafida
This repository by default loads the publicly available dataset ccGigafida, which contains a small subset of the Gigafida/Gigafida2 corpus.
The full datasets are private due to copyright. **If you happen to have access to the full datasets, the script will also work with those.**
Instead of
```
datasets.load_dataset("cjvt/cc_gigafida")
```
please use
```
datasets.load_dataset("cjvt/cc_gigafida", "private", data_dir="<directory-containing-gigafida(2)-TEI-files>")
```
**IMPORTANT:** The script will process all `.xml` files in the provided directory and its subdirectories - make sure there are no schema or metadata files in there!
### Dataset Summary
ccGigafida is a reference corpus of Slovene texts. It is a publicly available subsample of an even larger reference corpus, Gigafida (and its successor Gigafida 2).
The Gigafida corpus is an extensive collection of Slovene text of various genres, from daily newspapers, magazines, all kinds of books (fiction, non-fiction, textbooks),
web pages, transcriptions of parliamentary debates and similar.
### Supported Tasks and Leaderboards
Language modeling.
### Languages
Slovenian.
## Dataset Structure
### Data Instances
The data is loaded at document-level, i.e. one instance is one document.
```
{
'id_doc': 'F0000123',
'doc_title': 'Novi tednik NT&RC',
'authors': ['neznani novinar'],
'publish_date': '1998-03-27',
'publisher': 'Novi tednik',
'genres': ['tisk/periodično/časopis'],
'doc_tokenized': [
[
['Po', 'nekajletnem', 'počitku', 'pa', 'se', 'vračajo', 'tudi', 'kralji', 'dark', 'rock', 'godbe', 'JESUS', 'AND', 'THE', 'MARY', 'CHAIN', '.'],
['Brata', 'Reid', 'bosta', 'svojo', 'najnovejšo', 'kreacijo', '»', 'Cracking', 'Up', '«', 'objavila', 'v', 'ponedeljek', 'pri', 'trenutno', 'najuspešnejši', 'neodvisni', 'založbi', 'Creation', '(', 'vodi', 'jo', 'njun', 'nekdanji', 'menager', 'Alan', 'McGee', ',', 'zanjo', 'pa', 'poleg', 'Oasis', 'snema', 'še', 'cel', 'kup', 'popularnih', 'brit', '-', 'popovcev', ')', ',', 'tej', 'pa', 'bo', 'kmalu', 'sledil', 'tudi', 'album', '»', 'Munki', '«', '.']
],
[
['Kultni', 'ameriški', 'tehno', 'freak', 'PLASTIKMAN', 'že', 'vrsto', 'let', 'velja', 'za', 'enega', 'izmed', 'najbolj', 'inovativnih', 'in', 'produktivnih', 'ustvarjalcev', 'sodobne', 'elektronske', 'glasbe', '.'],
['Za', 'založbo', 'Nova', 'Mute', 'je', 'v', 'preteklih', 'nekaj', 'letih', 'posnel', 'cel', 'kup', 'izvrstnih', 'underground', 'dance', 'glasbenih', 'izdelkov', ',', 'pred', 'nedavnim', 'pa', 'je', 'ljubitelje', 'tovrstne', 'godbe', 'presenetil', 'z', 'ambientalnimi', 'odisejadami', ',', 'zbranimi', 'na', 'LP-ju', '»', 'Refused', '«', ',', 'ki', 'ga', 'lahko', 'od', 'prejšnjega', 'ponedeljka', 'kupite', 'tudi', 'v', 'bolje', 'založenih', 'trgovinah', 'z', 'nosilci', 'zvoka', 'na', 'sončni', 'strani', 'Alp', '.']
],
[
['STANE', 'ŠPEGEL']
]
],
'doc_lemmas': [...],
'doc_msds': [...],
'doc_string': [
[
'Po nekajletnem počitku pa se vračajo tudi kralji dark rock godbe JESUS AND THE MARY CHAIN. ',
'Brata Reid bosta svojo najnovejšo kreacijo »Cracking Up« objavila v ponedeljek pri trenutno najuspešnejši neodvisni založbi Creation (vodi jo njun nekdanji menager Alan McGee, zanjo pa poleg Oasis snema še cel kup popularnih brit-popovcev), tej pa bo kmalu sledil tudi album »Munki«.'
],
[
'Kultni ameriški tehno freak PLASTIKMAN že vrsto let velja za enega izmed najbolj inovativnih in produktivnih ustvarjalcev sodobne elektronske glasbe. ',
'Za založbo Nova Mute je v preteklih nekaj letih posnel cel kup izvrstnih underground dance glasbenih izdelkov, pred nedavnim pa je ljubitelje tovrstne godbe presenetil z ambientalnimi odisejadami, zbranimi na LP-ju »Refused«, ki ga lahko od prejšnjega ponedeljka kupite tudi v bolje založenih trgovinah z nosilci zvoka na sončni strani Alp.'
],
[
'STANE ŠPEGEL'
]
],
'id_sents': [['F0000123.000005.0', 'F0000123.000005.1'], ['F0000123.000013.0', 'F0000123.000013.1'], ['F0000123.000020.0']]
}
```
### Data Fields
- `id_doc`: the document ID (string);
- `doc_title`: the document title (string);
- `authors`: author(s) of the document (list of string): "neznani novinar" (sl) = ("unknown/unspecified journalist");
- `publish_date`: publish date (string);
- `publisher`: publisher, e.g., the name of a news agency (string);
- `genres`: genre(s) of the document (list of string) - possible genres: `['tisk', 'tisk/knjižno', 'tisk/knjižno/leposlovno', 'tisk/knjižno/strokovno', 'tisk/periodično', 'tisk/periodično/časopis', 'tisk/periodično/revija', 'tisk/drugo', 'internet']`;
- `doc_tokenized`: tokenized document - the top level lists represent paragraphs, the lists in the level deeper represent sentences, and each sentence contains tokens;
- `doc_lemmas`: lemmatized document - same structure as `doc_tokenized`;
- `doc_msds`: MSD tags of the document - same structure as `doc_tokenized` ([tagset](http://nl.ijs.si/ME/V6/msd/html/msd-sl.html));
- `doc_string`: same as `doc_tokenized` but with properly placed spaces in sentences;
- `id_sents`: IDs of sentences contained inside paragraphs of the document.
## Dataset Creation
Gigafida consists of texts which were published between 1990 and 2011. The texts come from printed sources and from the web.
Printed part contains fiction, non-fiction and textbooks, and periodicals such as daily newspapers and magazines.
Texts originating from the web were published on news portals, pages of big Slovene companies and more important governmental,
educational, research, cultural and similar institutions.
For more information, please check http://eng.slovenscina.eu/korpusi/gigafida.
## Additional Information
### Dataset Curators
Nataša Logar; et al. (please see http://hdl.handle.net/11356/1035 for the full list)
### Licensing Information
CC BY-NC-SA 4.0.
### Citation Information
```
@misc{ccGigafida,
title = {Written corpus {ccGigafida} 1.0},
author = {Logar, Nata{\v s}a and Erjavec, Toma{\v z} and Krek, Simon and Gr{\v c}ar, Miha and Holozan, Peter},
url = {http://hdl.handle.net/11356/1035},
note = {Slovenian language resource repository {CLARIN}.{SI}},
copyright = {Creative Commons - Attribution-{NonCommercial}-{ShareAlike} 4.0 International ({CC} {BY}-{NC}-{SA} 4.0)},
issn = {2820-4042},
year = {2013}
}
```
### Contributions
Thanks to [@matejklemen](https://github.com/matejklemen) for adding this dataset.
| cjvt/cc_gigafida | [
"task_categories:fill-mask",
"task_categories:text-generation",
"task_ids:masked-language-modeling",
"task_ids:language-modeling",
"annotations_creators:no-annotation",
"language_creators:found",
"multilinguality:monolingual",
"size_categories:10K<n<100K",
"size_categories:100M<n<1B",
"language:sl",
"license:cc-by-nc-sa-4.0",
"gigafida",
"gigafida2",
"kres",
"cckres",
"reference corpus",
"region:us"
] | 2022-11-29T15:03:45+00:00 | {"annotations_creators": ["no-annotation"], "language_creators": ["found"], "language": ["sl"], "license": ["cc-by-nc-sa-4.0"], "multilinguality": ["monolingual"], "size_categories": ["10K<n<100K", "100M<n<1B"], "source_datasets": [], "task_categories": ["fill-mask", "text-generation"], "task_ids": ["masked-language-modeling", "language-modeling"], "pretty_name": "Written corpus ccGigafida 1.0", "tags": ["gigafida", "gigafida2", "kres", "cckres", "reference corpus"]} | 2023-01-17T13:11:14+00:00 | [] | [
"sl"
] | TAGS
#task_categories-fill-mask #task_categories-text-generation #task_ids-masked-language-modeling #task_ids-language-modeling #annotations_creators-no-annotation #language_creators-found #multilinguality-monolingual #size_categories-10K<n<100K #size_categories-100M<n<1B #language-Slovenian #license-cc-by-nc-sa-4.0 #gigafida #gigafida2 #kres #cckres #reference corpus #region-us
|
# Dataset Card for ccGigafida
This repository by default loads the publicly available dataset ccGigafida, which contains a small subset of the Gigafida/Gigafida2 corpus.
The full datasets are private due to copyright. If you happen to have access to the full datasets, the script will also work with those.
Instead of
please use
IMPORTANT: The script will process all '.xml' files in the provided directory and its subdirectories - make sure there are no schema or metadata files in there!
### Dataset Summary
ccGigafida is a reference corpus of Slovene texts. It is a publicly available subsample of an even larger reference corpus, Gigafida (and its successor Gigafida 2).
The Gigafida corpus is an extensive collection of Slovene text of various genres, from daily newspapers, magazines, all kinds of books (fiction, non-fiction, textbooks),
web pages, transcriptions of parliamentary debates and similar.
### Supported Tasks and Leaderboards
Language modeling.
### Languages
Slovenian.
## Dataset Structure
### Data Instances
The data is loaded at document-level, i.e. one instance is one document.
### Data Fields
- 'id_doc': the document ID (string);
- 'doc_title': the document title (string);
- 'authors': author(s) of the document (list of string): "neznani novinar" (sl) = ("unknown/unspecified journalist");
- 'publish_date': publish date (string);
- 'publisher': publisher, e.g., the name of a news agency (string);
- 'genres': genre(s) of the document (list of string) - possible genres: '['tisk', 'tisk/knjižno', 'tisk/knjižno/leposlovno', 'tisk/knjižno/strokovno', 'tisk/periodično', 'tisk/periodično/časopis', 'tisk/periodično/revija', 'tisk/drugo', 'internet']';
- 'doc_tokenized': tokenized document - the top level lists represent paragraphs, the lists in the level deeper represent sentences, and each sentence contains tokens;
- 'doc_lemmas': lemmatized document - same structure as 'doc_tokenized';
- 'doc_msds': MSD tags of the document - same structure as 'doc_tokenized' (tagset);
- 'doc_string': same as 'doc_tokenized' but with properly placed spaces in sentences;
- 'id_sents': IDs of sentences contained inside paragraphs of the document.
## Dataset Creation
Gigafida consists of texts which were published between 1990 and 2011. The texts come from printed sources and from the web.
Printed part contains fiction, non-fiction and textbooks, and periodicals such as daily newspapers and magazines.
Texts originating from the web were published on news portals, pages of big Slovene companies and more important governmental,
educational, research, cultural and similar institutions.
For more information, please check URL
## Additional Information
### Dataset Curators
Nataša Logar; et al. (please see URL for the full list)
### Licensing Information
CC BY-NC-SA 4.0.
### Contributions
Thanks to @matejklemen for adding this dataset.
| [
"# Dataset Card for ccGigafida\n\nThis repository by default loads the publicly available dataset ccGigafida, which contains a small subset of the Gigafida/Gigafida2 corpus. \nThe full datasets are private due to copyright. If you happen to have access to the full datasets, the script will also work with those.\nInstead of \n \nplease use \n \n\nIMPORTANT: The script will process all '.xml' files in the provided directory and its subdirectories - make sure there are no schema or metadata files in there!",
"### Dataset Summary\n\nccGigafida is a reference corpus of Slovene texts. It is a publicly available subsample of an even larger reference corpus, Gigafida (and its successor Gigafida 2). \nThe Gigafida corpus is an extensive collection of Slovene text of various genres, from daily newspapers, magazines, all kinds of books (fiction, non-fiction, textbooks), \nweb pages, transcriptions of parliamentary debates and similar.",
"### Supported Tasks and Leaderboards\n\nLanguage modeling.",
"### Languages\n\nSlovenian.",
"## Dataset Structure",
"### Data Instances\n\nThe data is loaded at document-level, i.e. one instance is one document.",
"### Data Fields\n\n- 'id_doc': the document ID (string); \n- 'doc_title': the document title (string);\n- 'authors': author(s) of the document (list of string): \"neznani novinar\" (sl) = (\"unknown/unspecified journalist\");\n- 'publish_date': publish date (string);\n- 'publisher': publisher, e.g., the name of a news agency (string);\n- 'genres': genre(s) of the document (list of string) - possible genres: '['tisk', 'tisk/knjižno', 'tisk/knjižno/leposlovno', 'tisk/knjižno/strokovno', 'tisk/periodično', 'tisk/periodično/časopis', 'tisk/periodično/revija', 'tisk/drugo', 'internet']';\n- 'doc_tokenized': tokenized document - the top level lists represent paragraphs, the lists in the level deeper represent sentences, and each sentence contains tokens;\n- 'doc_lemmas': lemmatized document - same structure as 'doc_tokenized';\n- 'doc_msds': MSD tags of the document - same structure as 'doc_tokenized' (tagset);\n- 'doc_string': same as 'doc_tokenized' but with properly placed spaces in sentences;\n- 'id_sents': IDs of sentences contained inside paragraphs of the document.",
"## Dataset Creation\n\nGigafida consists of texts which were published between 1990 and 2011. The texts come from printed sources and from the web. \nPrinted part contains fiction, non-fiction and textbooks, and periodicals such as daily newspapers and magazines. \nTexts originating from the web were published on news portals, pages of big Slovene companies and more important governmental, \neducational, research, cultural and similar institutions. \n\nFor more information, please check URL",
"## Additional Information",
"### Dataset Curators\n\nNataša Logar; et al. (please see URL for the full list)",
"### Licensing Information\n\nCC BY-NC-SA 4.0.",
"### Contributions\n\nThanks to @matejklemen for adding this dataset."
] | [
"TAGS\n#task_categories-fill-mask #task_categories-text-generation #task_ids-masked-language-modeling #task_ids-language-modeling #annotations_creators-no-annotation #language_creators-found #multilinguality-monolingual #size_categories-10K<n<100K #size_categories-100M<n<1B #language-Slovenian #license-cc-by-nc-sa-4.0 #gigafida #gigafida2 #kres #cckres #reference corpus #region-us \n",
"# Dataset Card for ccGigafida\n\nThis repository by default loads the publicly available dataset ccGigafida, which contains a small subset of the Gigafida/Gigafida2 corpus. \nThe full datasets are private due to copyright. If you happen to have access to the full datasets, the script will also work with those.\nInstead of \n \nplease use \n \n\nIMPORTANT: The script will process all '.xml' files in the provided directory and its subdirectories - make sure there are no schema or metadata files in there!",
"### Dataset Summary\n\nccGigafida is a reference corpus of Slovene texts. It is a publicly available subsample of an even larger reference corpus, Gigafida (and its successor Gigafida 2). \nThe Gigafida corpus is an extensive collection of Slovene text of various genres, from daily newspapers, magazines, all kinds of books (fiction, non-fiction, textbooks), \nweb pages, transcriptions of parliamentary debates and similar.",
"### Supported Tasks and Leaderboards\n\nLanguage modeling.",
"### Languages\n\nSlovenian.",
"## Dataset Structure",
"### Data Instances\n\nThe data is loaded at document-level, i.e. one instance is one document.",
"### Data Fields\n\n- 'id_doc': the document ID (string); \n- 'doc_title': the document title (string);\n- 'authors': author(s) of the document (list of string): \"neznani novinar\" (sl) = (\"unknown/unspecified journalist\");\n- 'publish_date': publish date (string);\n- 'publisher': publisher, e.g., the name of a news agency (string);\n- 'genres': genre(s) of the document (list of string) - possible genres: '['tisk', 'tisk/knjižno', 'tisk/knjižno/leposlovno', 'tisk/knjižno/strokovno', 'tisk/periodično', 'tisk/periodično/časopis', 'tisk/periodično/revija', 'tisk/drugo', 'internet']';\n- 'doc_tokenized': tokenized document - the top level lists represent paragraphs, the lists in the level deeper represent sentences, and each sentence contains tokens;\n- 'doc_lemmas': lemmatized document - same structure as 'doc_tokenized';\n- 'doc_msds': MSD tags of the document - same structure as 'doc_tokenized' (tagset);\n- 'doc_string': same as 'doc_tokenized' but with properly placed spaces in sentences;\n- 'id_sents': IDs of sentences contained inside paragraphs of the document.",
"## Dataset Creation\n\nGigafida consists of texts which were published between 1990 and 2011. The texts come from printed sources and from the web. \nPrinted part contains fiction, non-fiction and textbooks, and periodicals such as daily newspapers and magazines. \nTexts originating from the web were published on news portals, pages of big Slovene companies and more important governmental, \neducational, research, cultural and similar institutions. \n\nFor more information, please check URL",
"## Additional Information",
"### Dataset Curators\n\nNataša Logar; et al. (please see URL for the full list)",
"### Licensing Information\n\nCC BY-NC-SA 4.0.",
"### Contributions\n\nThanks to @matejklemen for adding this dataset."
] |
1dd58f346b4f22529f1b9893c5c5cf504fac0a68 | # Dataset Card for "multi-label-class-github-issues-text-classification"
[More Information needed](https://github.com/huggingface/datasets/blob/main/CONTRIBUTING.md#how-to-contribute-to-the-dataset-cards) | Rami/multi-label-class-github-issues-text-classification | [
"region:us"
] | 2022-11-29T16:32:12+00:00 | {"dataset_info": {"features": [{"name": "title", "dtype": "string"}, {"name": "labels", "sequence": "string"}, {"name": "bodyText", "dtype": "string"}], "splits": [{"name": "train", "num_bytes": 2713984, "num_examples": 1556}, {"name": "valid", "num_bytes": 1296582, "num_examples": 778}, {"name": "test", "num_bytes": 1307650, "num_examples": 778}], "download_size": 2328003, "dataset_size": 5318216}} | 2022-12-02T01:19:08+00:00 | [] | [] | TAGS
#region-us
| # Dataset Card for "multi-label-class-github-issues-text-classification"
More Information needed | [
"# Dataset Card for \"multi-label-class-github-issues-text-classification\"\n\nMore Information needed"
] | [
"TAGS\n#region-us \n",
"# Dataset Card for \"multi-label-class-github-issues-text-classification\"\n\nMore Information needed"
] |
76260d2a26c2848b0f307b99be4f5058e7c5f6e8 | Dataset of captioned spectrograms (text describing the sound). | vucinatim/spectrogram-captions | [
"task_categories:text-to-image",
"annotations_creators:machine-generated",
"language_creators:machine-generated",
"multilinguality:monolingual",
"size_categories:n<1K",
"language:en",
"license:afl-3.0",
"stable diffusion sound generation text-to-sound text-to-image-to-sound spectrogram",
"region:us"
] | 2022-11-29T17:44:33+00:00 | {"annotations_creators": ["machine-generated"], "language_creators": ["machine-generated"], "language": ["en"], "license": ["afl-3.0"], "multilinguality": ["monolingual"], "size_categories": ["n<1K"], "source_datasets": [], "task_categories": ["text-to-image"], "task_ids": [], "pretty_name": "Captioned generic audio clips with spectrogram images", "tags": ["stable diffusion sound generation text-to-sound text-to-image-to-sound spectrogram"]} | 2023-01-03T00:24:32+00:00 | [] | [
"en"
] | TAGS
#task_categories-text-to-image #annotations_creators-machine-generated #language_creators-machine-generated #multilinguality-monolingual #size_categories-n<1K #language-English #license-afl-3.0 #stable diffusion sound generation text-to-sound text-to-image-to-sound spectrogram #region-us
| Dataset of captioned spectrograms (text describing the sound). | [] | [
"TAGS\n#task_categories-text-to-image #annotations_creators-machine-generated #language_creators-machine-generated #multilinguality-monolingual #size_categories-n<1K #language-English #license-afl-3.0 #stable diffusion sound generation text-to-sound text-to-image-to-sound spectrogram #region-us \n"
] |
a769431e550840dd0df50afe70a8b7b7cd78b9a2 | # Dataset Card for AutoTrain Evaluator
This repository contains model predictions generated by [AutoTrain](https://huggingface.co/autotrain) for the following task and dataset:
* Task: Zero-Shot Text Classification
* Model: bigscience/bloom-1b1
* Dataset: mathemakitten/winobias_antistereotype_test
* Config: mathemakitten--winobias_antistereotype_test
* Split: test
To run new evaluation jobs, visit Hugging Face's [automatic model evaluator](https://huggingface.co/spaces/autoevaluate/model-evaluator).
## Contributions
Thanks to [@mathemakitten](https://huggingface.co/mathemakitten) for evaluating this model. | autoevaluate/autoeval-eval-mathemakitten__winobias_antistereotype_test-mathemakitt-c9cce3-2280272258 | [
"autotrain",
"evaluation",
"region:us"
] | 2022-11-29T18:34:50+00:00 | {"type": "predictions", "tags": ["autotrain", "evaluation"], "datasets": ["mathemakitten/winobias_antistereotype_test"], "eval_info": {"task": "text_zero_shot_classification", "model": "bigscience/bloom-1b1", "metrics": [], "dataset_name": "mathemakitten/winobias_antistereotype_test", "dataset_config": "mathemakitten--winobias_antistereotype_test", "dataset_split": "test", "col_mapping": {"text": "text", "classes": "classes", "target": "target"}}} | 2022-11-29T18:37:53+00:00 | [] | [] | TAGS
#autotrain #evaluation #region-us
| # Dataset Card for AutoTrain Evaluator
This repository contains model predictions generated by AutoTrain for the following task and dataset:
* Task: Zero-Shot Text Classification
* Model: bigscience/bloom-1b1
* Dataset: mathemakitten/winobias_antistereotype_test
* Config: mathemakitten--winobias_antistereotype_test
* Split: test
To run new evaluation jobs, visit Hugging Face's automatic model evaluator.
## Contributions
Thanks to @mathemakitten for evaluating this model. | [
"# Dataset Card for AutoTrain Evaluator\n\nThis repository contains model predictions generated by AutoTrain for the following task and dataset:\n\n* Task: Zero-Shot Text Classification\n* Model: bigscience/bloom-1b1\n* Dataset: mathemakitten/winobias_antistereotype_test\n* Config: mathemakitten--winobias_antistereotype_test\n* Split: test\n\nTo run new evaluation jobs, visit Hugging Face's automatic model evaluator.",
"## Contributions\n\nThanks to @mathemakitten for evaluating this model."
] | [
"TAGS\n#autotrain #evaluation #region-us \n",
"# Dataset Card for AutoTrain Evaluator\n\nThis repository contains model predictions generated by AutoTrain for the following task and dataset:\n\n* Task: Zero-Shot Text Classification\n* Model: bigscience/bloom-1b1\n* Dataset: mathemakitten/winobias_antistereotype_test\n* Config: mathemakitten--winobias_antistereotype_test\n* Split: test\n\nTo run new evaluation jobs, visit Hugging Face's automatic model evaluator.",
"## Contributions\n\nThanks to @mathemakitten for evaluating this model."
] |
8f0961f4e5f025d6a43003f633a76414527cbedc | bidi | QonfiAI/ringeko | [
"region:us"
] | 2022-11-29T19:27:51+00:00 | {} | 2022-11-29T19:32:58+00:00 | [] | [] | TAGS
#region-us
| bidi | [] | [
"TAGS\n#region-us \n"
] |
cf1bea37aacfb2af416f095e18f9e6502183ed5c | # Dataset Card for AutoTrain Evaluator
This repository contains model predictions generated by [AutoTrain](https://huggingface.co/autotrain) for the following task and dataset:
* Task: Binary Text Classification
* Model: autoevaluate/binary-classification
* Dataset: glue
* Config: sst2
* Split: validation
To run new evaluation jobs, visit Hugging Face's [automatic model evaluator](https://huggingface.co/spaces/autoevaluate/model-evaluator).
## Contributions
Thanks to [@lewtun](https://huggingface.co/lewtun) for evaluating this model. | autoevaluate/autoeval-staging-eval-project-205dcc30-381f-492a-a8e8-fcfbe94b826c-110107 | [
"autotrain",
"evaluation",
"region:us"
] | 2022-11-29T19:51:09+00:00 | {"type": "predictions", "tags": ["autotrain", "evaluation"], "datasets": ["glue"], "eval_info": {"task": "binary_classification", "model": "autoevaluate/binary-classification", "metrics": ["matthews_correlation"], "dataset_name": "glue", "dataset_config": "sst2", "dataset_split": "validation", "col_mapping": {"text": "sentence", "target": "label"}}} | 2022-11-29T19:51:54+00:00 | [] | [] | TAGS
#autotrain #evaluation #region-us
| # Dataset Card for AutoTrain Evaluator
This repository contains model predictions generated by AutoTrain for the following task and dataset:
* Task: Binary Text Classification
* Model: autoevaluate/binary-classification
* Dataset: glue
* Config: sst2
* Split: validation
To run new evaluation jobs, visit Hugging Face's automatic model evaluator.
## Contributions
Thanks to @lewtun for evaluating this model. | [
"# Dataset Card for AutoTrain Evaluator\n\nThis repository contains model predictions generated by AutoTrain for the following task and dataset:\n\n* Task: Binary Text Classification\n* Model: autoevaluate/binary-classification\n* Dataset: glue\n* Config: sst2\n* Split: validation\n\nTo run new evaluation jobs, visit Hugging Face's automatic model evaluator.",
"## Contributions\n\nThanks to @lewtun for evaluating this model."
] | [
"TAGS\n#autotrain #evaluation #region-us \n",
"# Dataset Card for AutoTrain Evaluator\n\nThis repository contains model predictions generated by AutoTrain for the following task and dataset:\n\n* Task: Binary Text Classification\n* Model: autoevaluate/binary-classification\n* Dataset: glue\n* Config: sst2\n* Split: validation\n\nTo run new evaluation jobs, visit Hugging Face's automatic model evaluator.",
"## Contributions\n\nThanks to @lewtun for evaluating this model."
] |
48e2443255e9b9e37fda73d8e63d8c30386eb2c5 | # Dataset Card for AutoTrain Evaluator
This repository contains model predictions generated by [AutoTrain](https://huggingface.co/autotrain) for the following task and dataset:
* Task: Binary Text Classification
* Model: autoevaluate/binary-classification
* Dataset: glue
* Config: sst2
* Split: validation
To run new evaluation jobs, visit Hugging Face's [automatic model evaluator](https://huggingface.co/spaces/autoevaluate/model-evaluator).
## Contributions
Thanks to [@lewtun](https://huggingface.co/lewtun) for evaluating this model. | autoevaluate/autoeval-staging-eval-project-7a996eab-fd9f-4453-b298-d76d6134fbe7-111108 | [
"autotrain",
"evaluation",
"region:us"
] | 2022-11-29T20:05:07+00:00 | {"type": "predictions", "tags": ["autotrain", "evaluation"], "datasets": ["glue"], "eval_info": {"task": "binary_classification", "model": "autoevaluate/binary-classification", "metrics": ["matthews_correlation"], "dataset_name": "glue", "dataset_config": "sst2", "dataset_split": "validation", "col_mapping": {"text": "sentence", "target": "label"}}} | 2022-11-29T20:05:45+00:00 | [] | [] | TAGS
#autotrain #evaluation #region-us
| # Dataset Card for AutoTrain Evaluator
This repository contains model predictions generated by AutoTrain for the following task and dataset:
* Task: Binary Text Classification
* Model: autoevaluate/binary-classification
* Dataset: glue
* Config: sst2
* Split: validation
To run new evaluation jobs, visit Hugging Face's automatic model evaluator.
## Contributions
Thanks to @lewtun for evaluating this model. | [
"# Dataset Card for AutoTrain Evaluator\n\nThis repository contains model predictions generated by AutoTrain for the following task and dataset:\n\n* Task: Binary Text Classification\n* Model: autoevaluate/binary-classification\n* Dataset: glue\n* Config: sst2\n* Split: validation\n\nTo run new evaluation jobs, visit Hugging Face's automatic model evaluator.",
"## Contributions\n\nThanks to @lewtun for evaluating this model."
] | [
"TAGS\n#autotrain #evaluation #region-us \n",
"# Dataset Card for AutoTrain Evaluator\n\nThis repository contains model predictions generated by AutoTrain for the following task and dataset:\n\n* Task: Binary Text Classification\n* Model: autoevaluate/binary-classification\n* Dataset: glue\n* Config: sst2\n* Split: validation\n\nTo run new evaluation jobs, visit Hugging Face's automatic model evaluator.",
"## Contributions\n\nThanks to @lewtun for evaluating this model."
] |
40963fbe2a534dd6f31561bef69f0f23419c6c28 | # Dataset Card for AutoTrain Evaluator
This repository contains model predictions generated by [AutoTrain](https://huggingface.co/autotrain) for the following task and dataset:
* Task: Zero-Shot Text Classification
* Model: autoevaluate/zero-shot-classification
* Dataset: autoevaluate/zero-shot-classification-sample
* Config: autoevaluate--zero-shot-classification-sample
* Split: test
To run new evaluation jobs, visit Hugging Face's [automatic model evaluator](https://huggingface.co/spaces/autoevaluate/model-evaluator).
## Contributions
Thanks to [@lewtun](https://huggingface.co/lewtun) for evaluating this model. | autoevaluate/autoeval-staging-eval-project-318497e7-9d2a-403c-be28-ce4ff065ca1d-112109 | [
"autotrain",
"evaluation",
"region:us"
] | 2022-11-29T20:07:42+00:00 | {"type": "predictions", "tags": ["autotrain", "evaluation"], "datasets": ["autoevaluate/zero-shot-classification-sample"], "eval_info": {"task": "text_zero_shot_classification", "model": "autoevaluate/zero-shot-classification", "metrics": [], "dataset_name": "autoevaluate/zero-shot-classification-sample", "dataset_config": "autoevaluate--zero-shot-classification-sample", "dataset_split": "test", "col_mapping": {"text": "text", "classes": "classes", "target": "target"}}} | 2022-11-29T20:08:17+00:00 | [] | [] | TAGS
#autotrain #evaluation #region-us
| # Dataset Card for AutoTrain Evaluator
This repository contains model predictions generated by AutoTrain for the following task and dataset:
* Task: Zero-Shot Text Classification
* Model: autoevaluate/zero-shot-classification
* Dataset: autoevaluate/zero-shot-classification-sample
* Config: autoevaluate--zero-shot-classification-sample
* Split: test
To run new evaluation jobs, visit Hugging Face's automatic model evaluator.
## Contributions
Thanks to @lewtun for evaluating this model. | [
"# Dataset Card for AutoTrain Evaluator\n\nThis repository contains model predictions generated by AutoTrain for the following task and dataset:\n\n* Task: Zero-Shot Text Classification\n* Model: autoevaluate/zero-shot-classification\n* Dataset: autoevaluate/zero-shot-classification-sample\n* Config: autoevaluate--zero-shot-classification-sample\n* Split: test\n\nTo run new evaluation jobs, visit Hugging Face's automatic model evaluator.",
"## Contributions\n\nThanks to @lewtun for evaluating this model."
] | [
"TAGS\n#autotrain #evaluation #region-us \n",
"# Dataset Card for AutoTrain Evaluator\n\nThis repository contains model predictions generated by AutoTrain for the following task and dataset:\n\n* Task: Zero-Shot Text Classification\n* Model: autoevaluate/zero-shot-classification\n* Dataset: autoevaluate/zero-shot-classification-sample\n* Config: autoevaluate--zero-shot-classification-sample\n* Split: test\n\nTo run new evaluation jobs, visit Hugging Face's automatic model evaluator.",
"## Contributions\n\nThanks to @lewtun for evaluating this model."
] |
b013fdf8217123ef334fd6961e1b61c95025b28b |
# Dataset Card for cSQuAD1
## Table of Contents
- [Table of Contents](#table-of-contents)
- [Dataset Description](#dataset-description)
- [Dataset Summary](#dataset-summary)
- [Supported Tasks](#supported-tasks)
- [Languages](#languages)
- [Dataset Structure](#dataset-structure)
- [Data Instances](#data-instances)
- [Data Fields](#data-fields)
- [Data Splits](#data-splits)
- [Additional Information](#additional-information)
- [Licensing Information](#licensing-information)
- [Citation Information](#citation-information)
## Dataset Description
- **Homepage:**
- **Repository:**
- **Paper:**
- **Leaderboard:**
- **Point of Contact:**
### Dataset Summary
A contrast set generated from the eval set of SQuAD. Questions and answers were modified
to help detecting dataset artifacts. This dataset only contains a validation set, which
should only be used to evaluate a model.
### Supported Tasks
Question Answering (SQuAD).
### Languages
English
## Dataset Structure
### Data Instances
Dataset contains 100 instances
### Data Fields
| Field | Description |
|----------|--------------------------------------------------
| id | Id of document containing context |
| title | Title of the document |
| context | The context of the question |
| question | The question to answer |
| answers | A list of possible answers from the context |
| answer_start | The index in context where the answer starts |
### Data Splits
A single `eval` split is provided
## Dataset Creation
Dataset was created by modifying a sample of 100 examples from SQuAD test split.
## Additional Information
### Licensing Information
Apache 2.0 license
### Citation Information
TODO: add citations | dferndz/cSQuAD1 | [
"task_categories:question-answering",
"annotations_creators:expert-generated",
"language_creators:other",
"multilinguality:monolingual",
"language:en",
"license:apache-2.0",
"region:us"
] | 2022-11-30T00:03:13+00:00 | {"annotations_creators": ["expert-generated"], "language_creators": ["other"], "language": ["en"], "license": ["apache-2.0"], "multilinguality": ["monolingual"], "size_categories": [], "source_datasets": [], "task_categories": ["question-answering"], "task_ids": [], "pretty_name": "cSQuAD1", "tags": []} | 2022-12-09T23:17:57+00:00 | [] | [
"en"
] | TAGS
#task_categories-question-answering #annotations_creators-expert-generated #language_creators-other #multilinguality-monolingual #language-English #license-apache-2.0 #region-us
| Dataset Card for cSQuAD1
========================
Table of Contents
-----------------
* Table of Contents
* Dataset Description
+ Dataset Summary
+ Supported Tasks
+ Languages
* Dataset Structure
+ Data Instances
+ Data Fields
+ Data Splits
* Additional Information
+ Licensing Information
+ Citation Information
Dataset Description
-------------------
* Homepage:
* Repository:
* Paper:
* Leaderboard:
* Point of Contact:
### Dataset Summary
A contrast set generated from the eval set of SQuAD. Questions and answers were modified
to help detecting dataset artifacts. This dataset only contains a validation set, which
should only be used to evaluate a model.
### Supported Tasks
Question Answering (SQuAD).
### Languages
English
Dataset Structure
-----------------
### Data Instances
Dataset contains 100 instances
### Data Fields
### Data Splits
A single 'eval' split is provided
Dataset Creation
----------------
Dataset was created by modifying a sample of 100 examples from SQuAD test split.
Additional Information
----------------------
### Licensing Information
Apache 2.0 license
TODO: add citations
| [
"### Dataset Summary\n\n\nA contrast set generated from the eval set of SQuAD. Questions and answers were modified\nto help detecting dataset artifacts. This dataset only contains a validation set, which\nshould only be used to evaluate a model.",
"### Supported Tasks\n\n\nQuestion Answering (SQuAD).",
"### Languages\n\n\nEnglish\n\n\nDataset Structure\n-----------------",
"### Data Instances\n\n\nDataset contains 100 instances",
"### Data Fields",
"### Data Splits\n\n\nA single 'eval' split is provided\n\n\nDataset Creation\n----------------\n\n\nDataset was created by modifying a sample of 100 examples from SQuAD test split.\n\n\nAdditional Information\n----------------------",
"### Licensing Information\n\n\nApache 2.0 license\n\n\nTODO: add citations"
] | [
"TAGS\n#task_categories-question-answering #annotations_creators-expert-generated #language_creators-other #multilinguality-monolingual #language-English #license-apache-2.0 #region-us \n",
"### Dataset Summary\n\n\nA contrast set generated from the eval set of SQuAD. Questions and answers were modified\nto help detecting dataset artifacts. This dataset only contains a validation set, which\nshould only be used to evaluate a model.",
"### Supported Tasks\n\n\nQuestion Answering (SQuAD).",
"### Languages\n\n\nEnglish\n\n\nDataset Structure\n-----------------",
"### Data Instances\n\n\nDataset contains 100 instances",
"### Data Fields",
"### Data Splits\n\n\nA single 'eval' split is provided\n\n\nDataset Creation\n----------------\n\n\nDataset was created by modifying a sample of 100 examples from SQuAD test split.\n\n\nAdditional Information\n----------------------",
"### Licensing Information\n\n\nApache 2.0 license\n\n\nTODO: add citations"
] |
7ca3ac844c7902a4e397f30a25dc9957c0741bd6 | # Dataset Card for "olm-test-no-dedup"
[More Information needed](https://github.com/huggingface/datasets/blob/main/CONTRIBUTING.md#how-to-contribute-to-the-dataset-cards) | Tristan/olm-test-no-dedup | [
"region:us"
] | 2022-11-30T00:03:44+00:00 | {"dataset_info": {"features": [{"name": "text", "dtype": "string"}, {"name": "url", "dtype": "string"}, {"name": "crawl_timestamp", "dtype": "float64"}], "splits": [{"name": "train", "num_bytes": 249659214.0, "num_examples": 46032}], "download_size": 149319674, "dataset_size": 249659214.0}} | 2022-11-30T00:03:52+00:00 | [] | [] | TAGS
#region-us
| # Dataset Card for "olm-test-no-dedup"
More Information needed | [
"# Dataset Card for \"olm-test-no-dedup\"\n\nMore Information needed"
] | [
"TAGS\n#region-us \n",
"# Dataset Card for \"olm-test-no-dedup\"\n\nMore Information needed"
] |
8d888f4ccdc85c0aaa139556fd5ce59ab713a5de | # Dataset Card for "olm-test-normal-dedup"
[More Information needed](https://github.com/huggingface/datasets/blob/main/CONTRIBUTING.md#how-to-contribute-to-the-dataset-cards) | Tristan/olm-test-normal-dedup | [
"region:us"
] | 2022-11-30T00:04:23+00:00 | {"dataset_info": {"features": [{"name": "text", "dtype": "string"}, {"name": "url", "dtype": "string"}, {"name": "crawl_timestamp", "dtype": "float64"}], "splits": [{"name": "train", "num_bytes": 211642596.0, "num_examples": 40900}], "download_size": 128804894, "dataset_size": 211642596.0}} | 2022-11-30T00:33:45+00:00 | [] | [] | TAGS
#region-us
| # Dataset Card for "olm-test-normal-dedup"
More Information needed | [
"# Dataset Card for \"olm-test-normal-dedup\"\n\nMore Information needed"
] | [
"TAGS\n#region-us \n",
"# Dataset Card for \"olm-test-normal-dedup\"\n\nMore Information needed"
] |
678d3b2173afb3c46c3df869598e41f5f382b199 | # Dataset Card for "olm-test-normal-dedup-sorted"
[More Information needed](https://github.com/huggingface/datasets/blob/main/CONTRIBUTING.md#how-to-contribute-to-the-dataset-cards) | Tristan/olm-test-normal-dedup-sorted | [
"region:us"
] | 2022-11-30T00:17:14+00:00 | {"dataset_info": {"features": [{"name": "text", "dtype": "string"}, {"name": "url", "dtype": "string"}, {"name": "crawl_timestamp", "dtype": "float64"}], "splits": [{"name": "train", "num_bytes": 211642596.0, "num_examples": 40900}], "download_size": 128095054, "dataset_size": 211642596.0}} | 2022-11-30T00:33:18+00:00 | [] | [] | TAGS
#region-us
| # Dataset Card for "olm-test-normal-dedup-sorted"
More Information needed | [
"# Dataset Card for \"olm-test-normal-dedup-sorted\"\n\nMore Information needed"
] | [
"TAGS\n#region-us \n",
"# Dataset Card for \"olm-test-normal-dedup-sorted\"\n\nMore Information needed"
] |
a9a95432d0625e10bef6188c8196c8eaa9e24847 | # Dataset Card for "olm-test-no-dedup-sorted"
[More Information needed](https://github.com/huggingface/datasets/blob/main/CONTRIBUTING.md#how-to-contribute-to-the-dataset-cards) | Tristan/olm-test-no-dedup-sorted | [
"region:us"
] | 2022-11-30T00:18:30+00:00 | {"dataset_info": {"features": [{"name": "text", "dtype": "string"}, {"name": "url", "dtype": "string"}, {"name": "crawl_timestamp", "dtype": "float64"}], "splits": [{"name": "train", "num_bytes": 249659214.0, "num_examples": 46032}], "download_size": 143965993, "dataset_size": 249659214.0}} | 2022-11-30T00:18:38+00:00 | [] | [] | TAGS
#region-us
| # Dataset Card for "olm-test-no-dedup-sorted"
More Information needed | [
"# Dataset Card for \"olm-test-no-dedup-sorted\"\n\nMore Information needed"
] | [
"TAGS\n#region-us \n",
"# Dataset Card for \"olm-test-no-dedup-sorted\"\n\nMore Information needed"
] |
35f8d4a5476b9a971bc5f456a2fc55b266a15a76 |
# Dataset Card for cSQuAD2
## Table of Contents
- [Table of Contents](#table-of-contents)
- [Dataset Description](#dataset-description)
- [Dataset Summary](#dataset-summary)
- [Supported Tasks](#supported-tasks)
- [Languages](#languages)
- [Dataset Structure](#dataset-structure)
- [Data Instances](#data-instances)
- [Data Fields](#data-fields)
- [Data Splits](#data-splits)
- [Additional Information](#additional-information)
- [Licensing Information](#licensing-information)
- [Citation Information](#citation-information)
## Dataset Description
- **Homepage:**
- **Repository:**
- **Paper:**
- **Leaderboard:**
- **Point of Contact:**
### Dataset Summary
A contrast set to evaluate models trained on SQUAD on out-of-domain data.
### Supported Tasks
Evaluate question-answering
### Languages
English
## Dataset Structure
### Data Instances
Dataset contains 40 instances
### Data Fields
| Field | Description |
|----------|--------------------------------------------------
| id | Id of document containing context |
| title | Title of the document |
| context | The context of the question |
| question | The question to answer |
| answers | A list of possible answers from the context |
| answer_start | The index in context where the answer starts |
### Data Splits
A single `test` split is provided
## Dataset Creation
Dataset was created from Wikipedia articles
## Additional Information
### Licensing Information
Apache 2.0 license
### Citation Information
TODO: add citations | dferndz/cSQuAD2 | [
"task_categories:question-answering",
"annotations_creators:expert-generated",
"language_creators:other",
"multilinguality:monolingual",
"language:en",
"license:apache-2.0",
"region:us"
] | 2022-11-30T00:49:11+00:00 | {"annotations_creators": ["expert-generated"], "language_creators": ["other"], "language": ["en"], "license": ["apache-2.0"], "multilinguality": ["monolingual"], "size_categories": [], "source_datasets": [], "task_categories": ["question-answering"], "task_ids": [], "pretty_name": "cSQuAD2", "tags": []} | 2022-12-09T23:18:39+00:00 | [] | [
"en"
] | TAGS
#task_categories-question-answering #annotations_creators-expert-generated #language_creators-other #multilinguality-monolingual #language-English #license-apache-2.0 #region-us
| Dataset Card for cSQuAD2
========================
Table of Contents
-----------------
* Table of Contents
* Dataset Description
+ Dataset Summary
+ Supported Tasks
+ Languages
* Dataset Structure
+ Data Instances
+ Data Fields
+ Data Splits
* Additional Information
+ Licensing Information
+ Citation Information
Dataset Description
-------------------
* Homepage:
* Repository:
* Paper:
* Leaderboard:
* Point of Contact:
### Dataset Summary
A contrast set to evaluate models trained on SQUAD on out-of-domain data.
### Supported Tasks
Evaluate question-answering
### Languages
English
Dataset Structure
-----------------
### Data Instances
Dataset contains 40 instances
### Data Fields
### Data Splits
A single 'test' split is provided
Dataset Creation
----------------
Dataset was created from Wikipedia articles
Additional Information
----------------------
### Licensing Information
Apache 2.0 license
TODO: add citations
| [
"### Dataset Summary\n\n\nA contrast set to evaluate models trained on SQUAD on out-of-domain data.",
"### Supported Tasks\n\n\nEvaluate question-answering",
"### Languages\n\n\nEnglish\n\n\nDataset Structure\n-----------------",
"### Data Instances\n\n\nDataset contains 40 instances",
"### Data Fields",
"### Data Splits\n\n\nA single 'test' split is provided\n\n\nDataset Creation\n----------------\n\n\nDataset was created from Wikipedia articles\n\n\nAdditional Information\n----------------------",
"### Licensing Information\n\n\nApache 2.0 license\n\n\nTODO: add citations"
] | [
"TAGS\n#task_categories-question-answering #annotations_creators-expert-generated #language_creators-other #multilinguality-monolingual #language-English #license-apache-2.0 #region-us \n",
"### Dataset Summary\n\n\nA contrast set to evaluate models trained on SQUAD on out-of-domain data.",
"### Supported Tasks\n\n\nEvaluate question-answering",
"### Languages\n\n\nEnglish\n\n\nDataset Structure\n-----------------",
"### Data Instances\n\n\nDataset contains 40 instances",
"### Data Fields",
"### Data Splits\n\n\nA single 'test' split is provided\n\n\nDataset Creation\n----------------\n\n\nDataset was created from Wikipedia articles\n\n\nAdditional Information\n----------------------",
"### Licensing Information\n\n\nApache 2.0 license\n\n\nTODO: add citations"
] |
e9e803033b0e37f72915b6d812d8a85d7b64dbf9 |
# Dataset Card for [Stackoverflow Post Questions]
## Table of Contents
- [Table of Contents](#table-of-contents)
- [Dataset Description](#dataset-description)
- [Dataset Summary](#dataset-summary)
- [Languages](#languages)
- [Dataset Structure](#dataset-structure)
- [Data Splits](#data-splits)
- [Dataset Creation](#dataset-creation)
- [Source Data](#source-data)
- [Contributions](#contributions)
## Dataset Description
Companies that sell Open-source software tools usually hire an army of Customer representatives to try to answer every question asked about their tool. The first step in this process
is the prioritization of the question. The classification scale usually consists of 4 values, P0, P1, P2, and P3, with different meanings across every participant in the industry. On
the other hand, every software developer in the world has dealt with Stack Overflow (SO); the amount of shared knowledge there is incomparable to any other website. Questions in SO are
usually annotated and curated by thousands of people, providing metadata about the quality of the question. This dataset aims to provide an accurate prioritization for programming
questions.
### Dataset Summary
The dataset contains the title and body of stackoverflow questions and a label value(0,1,2,3) that was calculated using thresholds defined by SO badges.
### Languages
English
## Dataset Structure
title: string,
body: string,
label: int
### Data Splits
The split is 40/40/20, where classes have been balaned to be around the same size.
## Dataset Creation
The data set was extracted and labeled with the following query in BigQuery:
```
SELECT
title,
body,
CASE
WHEN score >= 100 OR favorite_count >= 100 OR view_count >= 10000 THEN 0
WHEN score >= 25 OR favorite_count >= 25 OR view_count >= 2500 THEN 1
WHEN score >= 10 OR favorite_count >= 10 OR view_count >= 1000 THEN 2
ELSE 3
END AS label
FROM `bigquery-public-data`.stackoverflow.posts_questions
```
### Source Data
The data was extracted from the Big Query public dataset: `bigquery-public-data.stackoverflow.posts_questions`
#### Initial Data Collection and Normalization
The original dataset contained high class imbalance:
label count
0 977424
1 2401534
2 3418179
3 16222990
Grand Total 23020127
The data was sampled from each class to have around the same amount of records on every class.
### Contributions
Thanks to [@pacofvf](https://github.com/pacofvf) for adding this dataset.
| pacovaldez/stackoverflow-questions-2016 | [
"task_categories:text-classification",
"task_ids:multi-class-classification",
"annotations_creators:machine-generated",
"language_creators:found",
"multilinguality:monolingual",
"size_categories:1M<n<10M",
"source_datasets:original",
"language:en",
"license:apache-2.0",
"stackoverflow",
"technical questions",
"region:us"
] | 2022-11-30T01:18:27+00:00 | {"annotations_creators": ["machine-generated"], "language_creators": ["found"], "language": ["en"], "license": ["apache-2.0"], "multilinguality": ["monolingual"], "size_categories": ["1M<n<10M"], "source_datasets": ["original"], "task_categories": ["text-classification"], "task_ids": ["multi-class-classification"], "pretty_name": "stackoverflow_post_questions", "tags": ["stackoverflow", "technical questions"]} | 2022-11-30T23:16:54+00:00 | [] | [
"en"
] | TAGS
#task_categories-text-classification #task_ids-multi-class-classification #annotations_creators-machine-generated #language_creators-found #multilinguality-monolingual #size_categories-1M<n<10M #source_datasets-original #language-English #license-apache-2.0 #stackoverflow #technical questions #region-us
|
# Dataset Card for [Stackoverflow Post Questions]
## Table of Contents
- Table of Contents
- Dataset Description
- Dataset Summary
- Languages
- Dataset Structure
- Data Splits
- Dataset Creation
- Source Data
- Contributions
## Dataset Description
Companies that sell Open-source software tools usually hire an army of Customer representatives to try to answer every question asked about their tool. The first step in this process
is the prioritization of the question. The classification scale usually consists of 4 values, P0, P1, P2, and P3, with different meanings across every participant in the industry. On
the other hand, every software developer in the world has dealt with Stack Overflow (SO); the amount of shared knowledge there is incomparable to any other website. Questions in SO are
usually annotated and curated by thousands of people, providing metadata about the quality of the question. This dataset aims to provide an accurate prioritization for programming
questions.
### Dataset Summary
The dataset contains the title and body of stackoverflow questions and a label value(0,1,2,3) that was calculated using thresholds defined by SO badges.
### Languages
English
## Dataset Structure
title: string,
body: string,
label: int
### Data Splits
The split is 40/40/20, where classes have been balaned to be around the same size.
## Dataset Creation
The data set was extracted and labeled with the following query in BigQuery:
### Source Data
The data was extracted from the Big Query public dataset: 'bigquery-public-data.stackoverflow.posts_questions'
#### Initial Data Collection and Normalization
The original dataset contained high class imbalance:
label count
0 977424
1 2401534
2 3418179
3 16222990
Grand Total 23020127
The data was sampled from each class to have around the same amount of records on every class.
### Contributions
Thanks to @pacofvf for adding this dataset.
| [
"# Dataset Card for [Stackoverflow Post Questions]",
"## Table of Contents\n- Table of Contents\n- Dataset Description\n - Dataset Summary\n - Languages\n- Dataset Structure\n - Data Splits\n- Dataset Creation\n - Source Data\n - Contributions",
"## Dataset Description\n\nCompanies that sell Open-source software tools usually hire an army of Customer representatives to try to answer every question asked about their tool. The first step in this process \nis the prioritization of the question. The classification scale usually consists of 4 values, P0, P1, P2, and P3, with different meanings across every participant in the industry. On \nthe other hand, every software developer in the world has dealt with Stack Overflow (SO); the amount of shared knowledge there is incomparable to any other website. Questions in SO are \nusually annotated and curated by thousands of people, providing metadata about the quality of the question. This dataset aims to provide an accurate prioritization for programming \nquestions.",
"### Dataset Summary\n\nThe dataset contains the title and body of stackoverflow questions and a label value(0,1,2,3) that was calculated using thresholds defined by SO badges.",
"### Languages\n\nEnglish",
"## Dataset Structure\n\ntitle: string,\nbody: string,\nlabel: int",
"### Data Splits\n\nThe split is 40/40/20, where classes have been balaned to be around the same size.",
"## Dataset Creation\n\nThe data set was extracted and labeled with the following query in BigQuery:",
"### Source Data\n\nThe data was extracted from the Big Query public dataset: 'bigquery-public-data.stackoverflow.posts_questions'",
"#### Initial Data Collection and Normalization\n\nThe original dataset contained high class imbalance:\n\nlabel\tcount\n0\t977424\n1\t2401534\n2\t3418179\n3\t16222990\nGrand Total\t23020127\n\nThe data was sampled from each class to have around the same amount of records on every class.",
"### Contributions\n\nThanks to @pacofvf for adding this dataset."
] | [
"TAGS\n#task_categories-text-classification #task_ids-multi-class-classification #annotations_creators-machine-generated #language_creators-found #multilinguality-monolingual #size_categories-1M<n<10M #source_datasets-original #language-English #license-apache-2.0 #stackoverflow #technical questions #region-us \n",
"# Dataset Card for [Stackoverflow Post Questions]",
"## Table of Contents\n- Table of Contents\n- Dataset Description\n - Dataset Summary\n - Languages\n- Dataset Structure\n - Data Splits\n- Dataset Creation\n - Source Data\n - Contributions",
"## Dataset Description\n\nCompanies that sell Open-source software tools usually hire an army of Customer representatives to try to answer every question asked about their tool. The first step in this process \nis the prioritization of the question. The classification scale usually consists of 4 values, P0, P1, P2, and P3, with different meanings across every participant in the industry. On \nthe other hand, every software developer in the world has dealt with Stack Overflow (SO); the amount of shared knowledge there is incomparable to any other website. Questions in SO are \nusually annotated and curated by thousands of people, providing metadata about the quality of the question. This dataset aims to provide an accurate prioritization for programming \nquestions.",
"### Dataset Summary\n\nThe dataset contains the title and body of stackoverflow questions and a label value(0,1,2,3) that was calculated using thresholds defined by SO badges.",
"### Languages\n\nEnglish",
"## Dataset Structure\n\ntitle: string,\nbody: string,\nlabel: int",
"### Data Splits\n\nThe split is 40/40/20, where classes have been balaned to be around the same size.",
"## Dataset Creation\n\nThe data set was extracted and labeled with the following query in BigQuery:",
"### Source Data\n\nThe data was extracted from the Big Query public dataset: 'bigquery-public-data.stackoverflow.posts_questions'",
"#### Initial Data Collection and Normalization\n\nThe original dataset contained high class imbalance:\n\nlabel\tcount\n0\t977424\n1\t2401534\n2\t3418179\n3\t16222990\nGrand Total\t23020127\n\nThe data was sampled from each class to have around the same amount of records on every class.",
"### Contributions\n\nThanks to @pacofvf for adding this dataset."
] |
f1cce90e993c9dc149d89aaef72146f291d04c24 | # Dataset Card for "train"
[More Information needed](https://github.com/huggingface/datasets/blob/main/CONTRIBUTING.md#how-to-contribute-to-the-dataset-cards) | devincapriola/train | [
"region:us"
] | 2022-11-30T01:58:19+00:00 | {"dataset_info": {"features": [{"name": "image", "dtype": "image"}, {"name": "text", "dtype": "string"}], "splits": [{"name": "train", "num_bytes": 291158.0, "num_examples": 15}], "download_size": 286037, "dataset_size": 291158.0}} | 2022-11-30T01:58:23+00:00 | [] | [] | TAGS
#region-us
| # Dataset Card for "train"
More Information needed | [
"# Dataset Card for \"train\"\n\nMore Information needed"
] | [
"TAGS\n#region-us \n",
"# Dataset Card for \"train\"\n\nMore Information needed"
] |
4578324578f80d0c1b26940c71881ba7059d923f | # Dataset Card for "train"
[More Information needed](https://github.com/huggingface/datasets/blob/main/CONTRIBUTING.md#how-to-contribute-to-the-dataset-cards) | gavincapriola/train | [
"region:us"
] | 2022-11-30T01:58:21+00:00 | {"dataset_info": {"features": [{"name": "image", "dtype": "image"}, {"name": "text", "dtype": "string"}], "splits": [{"name": "train", "num_bytes": 291158.0, "num_examples": 15}], "download_size": 286037, "dataset_size": 291158.0}} | 2022-11-30T01:58:27+00:00 | [] | [] | TAGS
#region-us
| # Dataset Card for "train"
More Information needed | [
"# Dataset Card for \"train\"\n\nMore Information needed"
] | [
"TAGS\n#region-us \n",
"# Dataset Card for \"train\"\n\nMore Information needed"
] |
aaa35d616834f34f8efcc4e050fd477564b2a7c6 | # Dataset Card for "cc_olm_no_bigscience_filters"
[More Information needed](https://github.com/huggingface/datasets/blob/main/CONTRIBUTING.md#how-to-contribute-to-the-dataset-cards) | Tristan/cc_olm_no_bigscience_filters | [
"region:us"
] | 2022-11-30T02:02:11+00:00 | {"dataset_info": {"features": [{"name": "text", "dtype": "string"}, {"name": "url", "dtype": "string"}, {"name": "crawl_timestamp", "dtype": "float64"}], "splits": [{"name": "train", "num_bytes": 166774086.86538956, "num_examples": 30389}], "download_size": 53612339, "dataset_size": 166774086.86538956}} | 2022-11-30T02:19:18+00:00 | [] | [] | TAGS
#region-us
| # Dataset Card for "cc_olm_no_bigscience_filters"
More Information needed | [
"# Dataset Card for \"cc_olm_no_bigscience_filters\"\n\nMore Information needed"
] | [
"TAGS\n#region-us \n",
"# Dataset Card for \"cc_olm_no_bigscience_filters\"\n\nMore Information needed"
] |
78a469f4bdcc13437b291ee6b50dc7c49ab562e8 | # Dataset Card for "cc_olm_no_dedup"
[More Information needed](https://github.com/huggingface/datasets/blob/main/CONTRIBUTING.md#how-to-contribute-to-the-dataset-cards) | Tristan/cc_olm_no_dedup | [
"region:us"
] | 2022-11-30T02:12:32+00:00 | {"dataset_info": {"features": [{"name": "text", "dtype": "string"}, {"name": "url", "dtype": "string"}, {"name": "crawl_timestamp", "dtype": "float64"}], "splits": [{"name": "train", "num_bytes": 249659214, "num_examples": 46032}], "download_size": 148670687, "dataset_size": 249659214}} | 2022-11-30T02:12:39+00:00 | [] | [] | TAGS
#region-us
| # Dataset Card for "cc_olm_no_dedup"
More Information needed | [
"# Dataset Card for \"cc_olm_no_dedup\"\n\nMore Information needed"
] | [
"TAGS\n#region-us \n",
"# Dataset Card for \"cc_olm_no_dedup\"\n\nMore Information needed"
] |
9df6fd230bec11170c0cedab5b502969264cb9d5 | # Dataset Card for "cc_olm_standard_suffix_array_dedup"
[More Information needed](https://github.com/huggingface/datasets/blob/main/CONTRIBUTING.md#how-to-contribute-to-the-dataset-cards) | Tristan/cc_olm_standard_suffix_array_dedup | [
"region:us"
] | 2022-11-30T02:17:28+00:00 | {"dataset_info": {"features": [{"name": "text", "dtype": "string"}, {"name": "url", "dtype": "string"}, {"name": "crawl_timestamp", "dtype": "float64"}], "splits": [{"name": "train", "num_bytes": 194046870.62526068, "num_examples": 41463}], "download_size": 130811345, "dataset_size": 194046870.62526068}} | 2022-11-30T02:17:36+00:00 | [] | [] | TAGS
#region-us
| # Dataset Card for "cc_olm_standard_suffix_array_dedup"
More Information needed | [
"# Dataset Card for \"cc_olm_standard_suffix_array_dedup\"\n\nMore Information needed"
] | [
"TAGS\n#region-us \n",
"# Dataset Card for \"cc_olm_standard_suffix_array_dedup\"\n\nMore Information needed"
] |
221236d6f972cde27d3ca4ff1e8c4815b0c53dc4 | # Dataset Card for "medmcqa_age_gender"
[More Information needed](https://github.com/huggingface/datasets/blob/main/CONTRIBUTING.md#how-to-contribute-to-the-dataset-cards) | society-ethics/medmcqa_age_gender | [
"region:us"
] | 2022-11-30T02:20:29+00:00 | {"dataset_info": {"features": [{"name": "id", "dtype": "string"}, {"name": "question", "dtype": "string"}, {"name": "opa", "dtype": "string"}, {"name": "opb", "dtype": "string"}, {"name": "opc", "dtype": "string"}, {"name": "opd", "dtype": "string"}, {"name": "cop", "dtype": "int64"}, {"name": "choice_type", "dtype": "string"}, {"name": "exp", "dtype": "string"}, {"name": "subject_name", "dtype": "string"}, {"name": "topic_name", "dtype": "string"}, {"name": "age.child", "dtype": "bool"}, {"name": "age.youth", "dtype": "bool"}, {"name": "age.adult", "dtype": "bool"}, {"name": "age.senior", "dtype": "bool"}, {"name": "gender.male", "dtype": "bool"}, {"name": "gender.female", "dtype": "bool"}], "splits": [{"name": "train", "num_bytes": 132040415, "num_examples": 182822}, {"name": "validation", "num_bytes": 2224566, "num_examples": 4183}], "download_size": 84155335, "dataset_size": 134264981}} | 2022-11-30T02:59:21+00:00 | [] | [] | TAGS
#region-us
| # Dataset Card for "medmcqa_age_gender"
More Information needed | [
"# Dataset Card for \"medmcqa_age_gender\"\n\nMore Information needed"
] | [
"TAGS\n#region-us \n",
"# Dataset Card for \"medmcqa_age_gender\"\n\nMore Information needed"
] |
1a071ac7df3bd8ed577788253140eb276737134d | # Dataset Card for "amazon-shoe-reviews"
[More Information needed](https://github.com/huggingface/datasets/blob/main/CONTRIBUTING.md#how-to-contribute-to-the-dataset-cards) | mchen72/amazon-shoe-reviews | [
"region:us"
] | 2022-11-30T02:52:52+00:00 | {"dataset_info": {"features": [{"name": "labels", "dtype": "int64"}, {"name": "text", "dtype": "string"}], "splits": [{"name": "train", "num_bytes": 16847665.2, "num_examples": 90000}, {"name": "test", "num_bytes": 1871962.8, "num_examples": 10000}], "download_size": 11140374, "dataset_size": 18719628.0}} | 2022-11-30T02:53:32+00:00 | [] | [] | TAGS
#region-us
| # Dataset Card for "amazon-shoe-reviews"
More Information needed | [
"# Dataset Card for \"amazon-shoe-reviews\"\n\nMore Information needed"
] | [
"TAGS\n#region-us \n",
"# Dataset Card for \"amazon-shoe-reviews\"\n\nMore Information needed"
] |
3c17b8671042b339f203018aef02f7b3088615e4 |
# Dataset Card for [Dataset Name]
## Table of Contents
- [Table of Contents](#table-of-contents)
- [Dataset Description](#dataset-description)
- [Dataset Summary](#dataset-summary)
- [Supported Tasks and Leaderboards](#supported-tasks-and-leaderboards)
- [Languages](#languages)
- [Dataset Structure](#dataset-structure)
- [Data Instances](#data-instances)
- [Data Fields](#data-fields)
- [Data Splits](#data-splits)
- [Dataset Creation](#dataset-creation)
- [Curation Rationale](#curation-rationale)
- [Source Data](#source-data)
- [Annotations](#annotations)
- [Personal and Sensitive Information](#personal-and-sensitive-information)
- [Considerations for Using the Data](#considerations-for-using-the-data)
- [Social Impact of Dataset](#social-impact-of-dataset)
- [Discussion of Biases](#discussion-of-biases)
- [Other Known Limitations](#other-known-limitations)
- [Additional Information](#additional-information)
- [Dataset Curators](#dataset-curators)
- [Licensing Information](#licensing-information)
- [Citation Information](#citation-information)
- [Contributions](#contributions)
## Dataset Description
- **Homepage:**
- **Repository:**
- **Paper:**
- **Leaderboard:**
- **Point of Contact:**
### Dataset Summary
[More Information Needed]
### Supported Tasks and Leaderboards
[More Information Needed]
### Languages
[More Information Needed]
## Dataset Structure
### Data Instances
[More Information Needed]
### Data Fields
[More Information Needed]
### Data Splits
[More Information Needed]
## Dataset Creation
### Curation Rationale
[More Information Needed]
### Source Data
#### Initial Data Collection and Normalization
[More Information Needed]
#### Who are the source language producers?
[More Information Needed]
### Annotations
#### Annotation process
[More Information Needed]
#### Who are the annotators?
[More Information Needed]
### Personal and Sensitive Information
[More Information Needed]
## Considerations for Using the Data
### Social Impact of Dataset
[More Information Needed]
### Discussion of Biases
[More Information Needed]
### Other Known Limitations
[More Information Needed]
## Additional Information
### Dataset Curators
[More Information Needed]
### Licensing Information
[More Information Needed]
### Citation Information
[More Information Needed]
### Contributions
Thanks to [@github-username](https://github.com/<github-username>) for adding this dataset. | DTU54DL/common-native-proc | [
"task_categories:token-classification",
"annotations_creators:expert-generated",
"language_creators:found",
"multilinguality:monolingual",
"size_categories:10K<n<100K",
"source_datasets:original",
"language:en",
"license:mit",
"region:us"
] | 2022-11-30T05:44:54+00:00 | {"annotations_creators": ["expert-generated"], "language_creators": ["found"], "language": ["en"], "license": ["mit"], "multilinguality": ["monolingual"], "size_categories": ["10K<n<100K"], "source_datasets": ["original"], "task_categories": ["token-classification"], "task_ids": ["token-classification-other-acronym-identification"], "paperswithcode_id": "acronym-identification", "pretty_name": "Acronym Identification Dataset", "dataset_info": {"features": [{"name": "sentence", "dtype": "string"}, {"name": "accent", "dtype": "string"}, {"name": "input_features", "sequence": {"sequence": "float32"}}, {"name": "labels", "sequence": "int64"}], "splits": [{"name": "train", "num_bytes": 9605830041, "num_examples": 10000}, {"name": "test", "num_bytes": 954798551, "num_examples": 994}], "download_size": 2010871786, "dataset_size": 10560628592}, "train-eval-index": [{"col_mapping": {"labels": "tags", "tokens": "tokens"}, "config": "default", "splits": {"eval_split": "test"}, "task": "token-classification", "task_id": "entity_extraction"}]} | 2022-11-30T20:46:05+00:00 | [] | [
"en"
] | TAGS
#task_categories-token-classification #annotations_creators-expert-generated #language_creators-found #multilinguality-monolingual #size_categories-10K<n<100K #source_datasets-original #language-English #license-mit #region-us
|
# Dataset Card for [Dataset Name]
## Table of Contents
- Table of Contents
- Dataset Description
- Dataset Summary
- Supported Tasks and Leaderboards
- Languages
- Dataset Structure
- Data Instances
- Data Fields
- Data Splits
- Dataset Creation
- Curation Rationale
- Source Data
- Annotations
- Personal and Sensitive Information
- Considerations for Using the Data
- Social Impact of Dataset
- Discussion of Biases
- Other Known Limitations
- Additional Information
- Dataset Curators
- Licensing Information
- Citation Information
- Contributions
## Dataset Description
- Homepage:
- Repository:
- Paper:
- Leaderboard:
- Point of Contact:
### Dataset Summary
### Supported Tasks and Leaderboards
### Languages
## Dataset Structure
### Data Instances
### Data Fields
### Data Splits
## Dataset Creation
### Curation Rationale
### Source Data
#### Initial Data Collection and Normalization
#### Who are the source language producers?
### Annotations
#### Annotation process
#### Who are the annotators?
### Personal and Sensitive Information
## Considerations for Using the Data
### Social Impact of Dataset
### Discussion of Biases
### Other Known Limitations
## Additional Information
### Dataset Curators
### Licensing Information
### Contributions
Thanks to @github-username for adding this dataset. | [
"# Dataset Card for [Dataset Name]",
"## Table of Contents\n- Table of Contents\n- Dataset Description\n - Dataset Summary\n - Supported Tasks and Leaderboards\n - Languages\n- Dataset Structure\n - Data Instances\n - Data Fields\n - Data Splits\n- Dataset Creation\n - Curation Rationale\n - Source Data\n - Annotations\n - Personal and Sensitive Information\n- Considerations for Using the Data\n - Social Impact of Dataset\n - Discussion of Biases\n - Other Known Limitations\n- Additional Information\n - Dataset Curators\n - Licensing Information\n - Citation Information\n - Contributions",
"## Dataset Description\n\n- Homepage:\n- Repository:\n- Paper:\n- Leaderboard:\n- Point of Contact:",
"### Dataset Summary",
"### Supported Tasks and Leaderboards",
"### Languages",
"## Dataset Structure",
"### Data Instances",
"### Data Fields",
"### Data Splits",
"## Dataset Creation",
"### Curation Rationale",
"### Source Data",
"#### Initial Data Collection and Normalization",
"#### Who are the source language producers?",
"### Annotations",
"#### Annotation process",
"#### Who are the annotators?",
"### Personal and Sensitive Information",
"## Considerations for Using the Data",
"### Social Impact of Dataset",
"### Discussion of Biases",
"### Other Known Limitations",
"## Additional Information",
"### Dataset Curators",
"### Licensing Information",
"### Contributions\n\nThanks to @github-username for adding this dataset."
] | [
"TAGS\n#task_categories-token-classification #annotations_creators-expert-generated #language_creators-found #multilinguality-monolingual #size_categories-10K<n<100K #source_datasets-original #language-English #license-mit #region-us \n",
"# Dataset Card for [Dataset Name]",
"## Table of Contents\n- Table of Contents\n- Dataset Description\n - Dataset Summary\n - Supported Tasks and Leaderboards\n - Languages\n- Dataset Structure\n - Data Instances\n - Data Fields\n - Data Splits\n- Dataset Creation\n - Curation Rationale\n - Source Data\n - Annotations\n - Personal and Sensitive Information\n- Considerations for Using the Data\n - Social Impact of Dataset\n - Discussion of Biases\n - Other Known Limitations\n- Additional Information\n - Dataset Curators\n - Licensing Information\n - Citation Information\n - Contributions",
"## Dataset Description\n\n- Homepage:\n- Repository:\n- Paper:\n- Leaderboard:\n- Point of Contact:",
"### Dataset Summary",
"### Supported Tasks and Leaderboards",
"### Languages",
"## Dataset Structure",
"### Data Instances",
"### Data Fields",
"### Data Splits",
"## Dataset Creation",
"### Curation Rationale",
"### Source Data",
"#### Initial Data Collection and Normalization",
"#### Who are the source language producers?",
"### Annotations",
"#### Annotation process",
"#### Who are the annotators?",
"### Personal and Sensitive Information",
"## Considerations for Using the Data",
"### Social Impact of Dataset",
"### Discussion of Biases",
"### Other Known Limitations",
"## Additional Information",
"### Dataset Curators",
"### Licensing Information",
"### Contributions\n\nThanks to @github-username for adding this dataset."
] |
e5c3719d0092d25288c49a5d55e10b1f81e56021 |
# Dataset Card for Nail Biting Classification
## Table of Contents
- [Table of Contents](#table-of-contents)
- [Dataset Description](#dataset-description)
- [Dataset Summary](#dataset-summary)
- [Supported Tasks and Leaderboards](#supported-tasks-and-leaderboards)
- [Languages](#languages)
- [Dataset Structure](#dataset-structure)
- [Data Instances](#data-instances)
- [Data Fields](#data-fields)
- [Data Splits](#data-splits)
- [Dataset Creation](#dataset-creation)
- [Curation Rationale](#curation-rationale)
- [Source Data](#source-data)
- [Annotations](#annotations)
- [Personal and Sensitive Information](#personal-and-sensitive-information)
- [Considerations for Using the Data](#considerations-for-using-the-data)
- [Social Impact of Dataset](#social-impact-of-dataset)
- [Discussion of Biases](#discussion-of-biases)
- [Other Known Limitations](#other-known-limitations)
- [Additional Information](#additional-information)
- [Dataset Curators](#dataset-curators)
- [Licensing Information](#licensing-information)
- [Citation Information](#citation-information)
- [Contributions](#contributions)
## Dataset Description
- **Homepage:** [https://huggingface.co/datasets/alecsharpie/nailbiting_classification](https://huggingface.co/datasets/alecsharpie/nailbiting_classification)
- **Repository:** [https://github.com/alecsharpie/nomo_nailbiting](https://github.com/alecsharpie/nomo_nailbiting)
- **Point of Contact:** [[email protected]]([email protected])
### Dataset Summary
A binary image dataset for classifying nailbiting. Images are cropped to only show the mouth area.
Should contain edge cases such as drinking water, talking on the phone, scratching chin etc.. all in "no biting" category
## Dataset Structure
### Data Instances
- 7147 Images
- 14879790 bytes total
- 12332617 bytes download
### Data Fields
128 x 64 (w x h, pixels)
Black and white
Labels
- '0': biting
- '1': no_biting
### Data Splits
- train: 6629 (11965737 bytes)
- test: 1471 (2914053 bytes)
## Dataset Creation
### Curation Rationale
I wanted to create a notification system to help me stop biting my nails. It needed to contain lots of possible no-biting scenarios. eg talking on the phone
### Source Data
#### Initial Data Collection and Normalization
The data was scraped from stock images sites and photos of myself were taken with my webcam.
MTCNN (https://github.com/ipazc/mtcnn) was then used to crop the images down to only the show the mouth area
The images were then converted to a black & white colour scheme.
### Annotations
#### Annotation process
During the scraping process images were labelled with a description, which I then manually sanity checked. I labelled the ones of me manually.
#### Who are the annotators?
Alec Sharp
## Considerations for Using the Data
### Discussion of Biases & Limitations
Tried to make the dataset diverse in terms of age and skin tone. Although, this dataset contains a large number of images of one subject (me) so is biased towards lower quality webcam pictures of a white male with a short beard.
### Dataset Curators
Alec Sharp
### Licensing Information
MIT
### Contributions
Thanks to [@alecsharpie](https://github.com/alecsharpie) for adding this dataset. | alecsharpie/nailbiting_classification | [
"task_categories:image-classification",
"annotations_creators:expert-generated",
"annotations_creators:machine-generated",
"size_categories:1K<n<10K",
"source_datasets:original",
"language:en",
"license:mit",
"nailbiting",
"image",
"preprocesses",
"region:us"
] | 2022-11-30T06:02:22+00:00 | {"annotations_creators": ["expert-generated", "machine-generated"], "language_creators": [], "language": ["en"], "license": ["mit"], "multilinguality": [], "size_categories": ["1K<n<10K"], "source_datasets": ["original"], "task_categories": ["image-classification"], "task_ids": [], "paperswithcode_id": "acronym-identification", "pretty_name": "Nailbiting Classification", "tags": ["nailbiting", "image", "preprocesses"], "dataset_info": {"features": [{"name": "image", "dtype": "image"}, {"name": "label", "dtype": {"class_label": {"names": {"0": "biting", "1": "no_biting"}}}}], "splits": [{"name": "train", "num_bytes": 11965731.715, "num_examples": 6629}, {"name": "test", "num_bytes": 1485426.0, "num_examples": 736}], "download_size": 11546517, "dataset_size": 13451157.715}, "train-eval-index": [{"col_mapping": {"labels": "tags", "tokens": "tokens"}, "config": "default", "splits": {"eval_split": "test"}, "task": "token-classification", "task_id": "entity_extraction"}]} | 2022-11-30T07:12:04+00:00 | [] | [
"en"
] | TAGS
#task_categories-image-classification #annotations_creators-expert-generated #annotations_creators-machine-generated #size_categories-1K<n<10K #source_datasets-original #language-English #license-mit #nailbiting #image #preprocesses #region-us
|
# Dataset Card for Nail Biting Classification
## Table of Contents
- Table of Contents
- Dataset Description
- Dataset Summary
- Supported Tasks and Leaderboards
- Languages
- Dataset Structure
- Data Instances
- Data Fields
- Data Splits
- Dataset Creation
- Curation Rationale
- Source Data
- Annotations
- Personal and Sensitive Information
- Considerations for Using the Data
- Social Impact of Dataset
- Discussion of Biases
- Other Known Limitations
- Additional Information
- Dataset Curators
- Licensing Information
- Citation Information
- Contributions
## Dataset Description
- Homepage: URL
- Repository: URL
- Point of Contact: alecsharpie@URL
### Dataset Summary
A binary image dataset for classifying nailbiting. Images are cropped to only show the mouth area.
Should contain edge cases such as drinking water, talking on the phone, scratching chin etc.. all in "no biting" category
## Dataset Structure
### Data Instances
- 7147 Images
- 14879790 bytes total
- 12332617 bytes download
### Data Fields
128 x 64 (w x h, pixels)
Black and white
Labels
- '0': biting
- '1': no_biting
### Data Splits
- train: 6629 (11965737 bytes)
- test: 1471 (2914053 bytes)
## Dataset Creation
### Curation Rationale
I wanted to create a notification system to help me stop biting my nails. It needed to contain lots of possible no-biting scenarios. eg talking on the phone
### Source Data
#### Initial Data Collection and Normalization
The data was scraped from stock images sites and photos of myself were taken with my webcam.
MTCNN (URL was then used to crop the images down to only the show the mouth area
The images were then converted to a black & white colour scheme.
### Annotations
#### Annotation process
During the scraping process images were labelled with a description, which I then manually sanity checked. I labelled the ones of me manually.
#### Who are the annotators?
Alec Sharp
## Considerations for Using the Data
### Discussion of Biases & Limitations
Tried to make the dataset diverse in terms of age and skin tone. Although, this dataset contains a large number of images of one subject (me) so is biased towards lower quality webcam pictures of a white male with a short beard.
### Dataset Curators
Alec Sharp
### Licensing Information
MIT
### Contributions
Thanks to @alecsharpie for adding this dataset. | [
"# Dataset Card for Nail Biting Classification",
"## Table of Contents\n- Table of Contents\n- Dataset Description\n - Dataset Summary\n - Supported Tasks and Leaderboards\n - Languages\n- Dataset Structure\n - Data Instances\n - Data Fields\n - Data Splits\n- Dataset Creation\n - Curation Rationale\n - Source Data\n - Annotations\n - Personal and Sensitive Information\n- Considerations for Using the Data\n - Social Impact of Dataset\n - Discussion of Biases\n - Other Known Limitations\n- Additional Information\n - Dataset Curators\n - Licensing Information\n - Citation Information\n - Contributions",
"## Dataset Description\n\n\n- Homepage: URL\n- Repository: URL\n- Point of Contact: alecsharpie@URL",
"### Dataset Summary\n\nA binary image dataset for classifying nailbiting. Images are cropped to only show the mouth area.\nShould contain edge cases such as drinking water, talking on the phone, scratching chin etc.. all in \"no biting\" category",
"## Dataset Structure",
"### Data Instances\n\n - 7147 Images\n - 14879790 bytes total\n - 12332617 bytes download",
"### Data Fields\n\n128 x 64 (w x h, pixels)\nBlack and white\n\nLabels\n - '0': biting\n - '1': no_biting",
"### Data Splits\n\n- train: 6629 (11965737 bytes)\n- test: 1471 (2914053 bytes)",
"## Dataset Creation",
"### Curation Rationale\n\nI wanted to create a notification system to help me stop biting my nails. It needed to contain lots of possible no-biting scenarios. eg talking on the phone",
"### Source Data",
"#### Initial Data Collection and Normalization\n\nThe data was scraped from stock images sites and photos of myself were taken with my webcam. \nMTCNN (URL was then used to crop the images down to only the show the mouth area\nThe images were then converted to a black & white colour scheme.",
"### Annotations",
"#### Annotation process\n\nDuring the scraping process images were labelled with a description, which I then manually sanity checked. I labelled the ones of me manually.",
"#### Who are the annotators?\n\nAlec Sharp",
"## Considerations for Using the Data",
"### Discussion of Biases & Limitations\n\nTried to make the dataset diverse in terms of age and skin tone. Although, this dataset contains a large number of images of one subject (me) so is biased towards lower quality webcam pictures of a white male with a short beard.",
"### Dataset Curators\n\nAlec Sharp",
"### Licensing Information\n\nMIT",
"### Contributions\n\nThanks to @alecsharpie for adding this dataset."
] | [
"TAGS\n#task_categories-image-classification #annotations_creators-expert-generated #annotations_creators-machine-generated #size_categories-1K<n<10K #source_datasets-original #language-English #license-mit #nailbiting #image #preprocesses #region-us \n",
"# Dataset Card for Nail Biting Classification",
"## Table of Contents\n- Table of Contents\n- Dataset Description\n - Dataset Summary\n - Supported Tasks and Leaderboards\n - Languages\n- Dataset Structure\n - Data Instances\n - Data Fields\n - Data Splits\n- Dataset Creation\n - Curation Rationale\n - Source Data\n - Annotations\n - Personal and Sensitive Information\n- Considerations for Using the Data\n - Social Impact of Dataset\n - Discussion of Biases\n - Other Known Limitations\n- Additional Information\n - Dataset Curators\n - Licensing Information\n - Citation Information\n - Contributions",
"## Dataset Description\n\n\n- Homepage: URL\n- Repository: URL\n- Point of Contact: alecsharpie@URL",
"### Dataset Summary\n\nA binary image dataset for classifying nailbiting. Images are cropped to only show the mouth area.\nShould contain edge cases such as drinking water, talking on the phone, scratching chin etc.. all in \"no biting\" category",
"## Dataset Structure",
"### Data Instances\n\n - 7147 Images\n - 14879790 bytes total\n - 12332617 bytes download",
"### Data Fields\n\n128 x 64 (w x h, pixels)\nBlack and white\n\nLabels\n - '0': biting\n - '1': no_biting",
"### Data Splits\n\n- train: 6629 (11965737 bytes)\n- test: 1471 (2914053 bytes)",
"## Dataset Creation",
"### Curation Rationale\n\nI wanted to create a notification system to help me stop biting my nails. It needed to contain lots of possible no-biting scenarios. eg talking on the phone",
"### Source Data",
"#### Initial Data Collection and Normalization\n\nThe data was scraped from stock images sites and photos of myself were taken with my webcam. \nMTCNN (URL was then used to crop the images down to only the show the mouth area\nThe images were then converted to a black & white colour scheme.",
"### Annotations",
"#### Annotation process\n\nDuring the scraping process images were labelled with a description, which I then manually sanity checked. I labelled the ones of me manually.",
"#### Who are the annotators?\n\nAlec Sharp",
"## Considerations for Using the Data",
"### Discussion of Biases & Limitations\n\nTried to make the dataset diverse in terms of age and skin tone. Although, this dataset contains a large number of images of one subject (me) so is biased towards lower quality webcam pictures of a white male with a short beard.",
"### Dataset Curators\n\nAlec Sharp",
"### Licensing Information\n\nMIT",
"### Contributions\n\nThanks to @alecsharpie for adding this dataset."
] |
9095e8f6dc0f0bb51623ee632058b625271171dd |
# Dataset Card for [Dataset Name]
## Table of Contents
- [Table of Contents](#table-of-contents)
- [Dataset Description](#dataset-description)
- [Dataset Summary](#dataset-summary)
- [Supported Tasks and Leaderboards](#supported-tasks-and-leaderboards)
- [Languages](#languages)
- [Dataset Structure](#dataset-structure)
- [Data Instances](#data-instances)
- [Data Fields](#data-fields)
- [Data Splits](#data-splits)
- [Dataset Creation](#dataset-creation)
- [Curation Rationale](#curation-rationale)
- [Source Data](#source-data)
- [Annotations](#annotations)
- [Personal and Sensitive Information](#personal-and-sensitive-information)
- [Considerations for Using the Data](#considerations-for-using-the-data)
- [Social Impact of Dataset](#social-impact-of-dataset)
- [Discussion of Biases](#discussion-of-biases)
- [Other Known Limitations](#other-known-limitations)
- [Additional Information](#additional-information)
- [Dataset Curators](#dataset-curators)
- [Licensing Information](#licensing-information)
- [Citation Information](#citation-information)
- [Contributions](#contributions)
## Dataset Description
- **Homepage:**
- **Repository:**
- **Paper:**
- **Leaderboard:**
- **Point of Contact:**
### Dataset Summary
[More Information Needed]
### Supported Tasks and Leaderboards
[More Information Needed]
### Languages
[More Information Needed]
## Dataset Structure
### Data Instances
[More Information Needed]
### Data Fields
[More Information Needed]
### Data Splits
[More Information Needed]
## Dataset Creation
### Curation Rationale
[More Information Needed]
### Source Data
#### Initial Data Collection and Normalization
[More Information Needed]
#### Who are the source language producers?
[More Information Needed]
### Annotations
#### Annotation process
[More Information Needed]
#### Who are the annotators?
[More Information Needed]
### Personal and Sensitive Information
[More Information Needed]
## Considerations for Using the Data
### Social Impact of Dataset
[More Information Needed]
### Discussion of Biases
[More Information Needed]
### Other Known Limitations
[More Information Needed]
## Additional Information
### Dataset Curators
[More Information Needed]
### Licensing Information
[More Information Needed]
### Citation Information
[More Information Needed]
### Contributions
Thanks to [@github-username](https://github.com/<github-username>) for adding this dataset. | DTU54DL/common-accent | [
"task_categories:token-classification",
"annotations_creators:expert-generated",
"language_creators:found",
"multilinguality:monolingual",
"size_categories:10K<n<100K",
"source_datasets:original",
"language:en",
"license:mit",
"region:us"
] | 2022-11-30T07:46:58+00:00 | {"annotations_creators": ["expert-generated"], "language_creators": ["found"], "language": ["en"], "license": ["mit"], "multilinguality": ["monolingual"], "size_categories": ["10K<n<100K"], "source_datasets": ["original"], "task_categories": ["token-classification"], "task_ids": ["token-classification-other-acronym-identification"], "paperswithcode_id": "acronym-identification", "pretty_name": "Acronym Identification Dataset", "dataset_info": {"features": [{"name": "audio", "dtype": {"audio": {"sampling_rate": 16000}}}, {"name": "sentence", "dtype": "string"}, {"name": "accent", "dtype": "string"}], "splits": [{"name": "train", "num_bytes": 471755846.3910719, "num_examples": 10000}, {"name": "test", "num_bytes": 19497172.25755167, "num_examples": 451}], "download_size": 436911322, "dataset_size": 491253018.6486236}, "train-eval-index": [{"col_mapping": {"labels": "tags", "tokens": "tokens"}, "config": "default", "splits": {"eval_split": "test"}, "task": "token-classification", "task_id": "entity_extraction"}]} | 2022-11-30T13:25:07+00:00 | [] | [
"en"
] | TAGS
#task_categories-token-classification #annotations_creators-expert-generated #language_creators-found #multilinguality-monolingual #size_categories-10K<n<100K #source_datasets-original #language-English #license-mit #region-us
|
# Dataset Card for [Dataset Name]
## Table of Contents
- Table of Contents
- Dataset Description
- Dataset Summary
- Supported Tasks and Leaderboards
- Languages
- Dataset Structure
- Data Instances
- Data Fields
- Data Splits
- Dataset Creation
- Curation Rationale
- Source Data
- Annotations
- Personal and Sensitive Information
- Considerations for Using the Data
- Social Impact of Dataset
- Discussion of Biases
- Other Known Limitations
- Additional Information
- Dataset Curators
- Licensing Information
- Citation Information
- Contributions
## Dataset Description
- Homepage:
- Repository:
- Paper:
- Leaderboard:
- Point of Contact:
### Dataset Summary
### Supported Tasks and Leaderboards
### Languages
## Dataset Structure
### Data Instances
### Data Fields
### Data Splits
## Dataset Creation
### Curation Rationale
### Source Data
#### Initial Data Collection and Normalization
#### Who are the source language producers?
### Annotations
#### Annotation process
#### Who are the annotators?
### Personal and Sensitive Information
## Considerations for Using the Data
### Social Impact of Dataset
### Discussion of Biases
### Other Known Limitations
## Additional Information
### Dataset Curators
### Licensing Information
### Contributions
Thanks to @github-username for adding this dataset. | [
"# Dataset Card for [Dataset Name]",
"## Table of Contents\n- Table of Contents\n- Dataset Description\n - Dataset Summary\n - Supported Tasks and Leaderboards\n - Languages\n- Dataset Structure\n - Data Instances\n - Data Fields\n - Data Splits\n- Dataset Creation\n - Curation Rationale\n - Source Data\n - Annotations\n - Personal and Sensitive Information\n- Considerations for Using the Data\n - Social Impact of Dataset\n - Discussion of Biases\n - Other Known Limitations\n- Additional Information\n - Dataset Curators\n - Licensing Information\n - Citation Information\n - Contributions",
"## Dataset Description\n\n- Homepage:\n- Repository:\n- Paper:\n- Leaderboard:\n- Point of Contact:",
"### Dataset Summary",
"### Supported Tasks and Leaderboards",
"### Languages",
"## Dataset Structure",
"### Data Instances",
"### Data Fields",
"### Data Splits",
"## Dataset Creation",
"### Curation Rationale",
"### Source Data",
"#### Initial Data Collection and Normalization",
"#### Who are the source language producers?",
"### Annotations",
"#### Annotation process",
"#### Who are the annotators?",
"### Personal and Sensitive Information",
"## Considerations for Using the Data",
"### Social Impact of Dataset",
"### Discussion of Biases",
"### Other Known Limitations",
"## Additional Information",
"### Dataset Curators",
"### Licensing Information",
"### Contributions\n\nThanks to @github-username for adding this dataset."
] | [
"TAGS\n#task_categories-token-classification #annotations_creators-expert-generated #language_creators-found #multilinguality-monolingual #size_categories-10K<n<100K #source_datasets-original #language-English #license-mit #region-us \n",
"# Dataset Card for [Dataset Name]",
"## Table of Contents\n- Table of Contents\n- Dataset Description\n - Dataset Summary\n - Supported Tasks and Leaderboards\n - Languages\n- Dataset Structure\n - Data Instances\n - Data Fields\n - Data Splits\n- Dataset Creation\n - Curation Rationale\n - Source Data\n - Annotations\n - Personal and Sensitive Information\n- Considerations for Using the Data\n - Social Impact of Dataset\n - Discussion of Biases\n - Other Known Limitations\n- Additional Information\n - Dataset Curators\n - Licensing Information\n - Citation Information\n - Contributions",
"## Dataset Description\n\n- Homepage:\n- Repository:\n- Paper:\n- Leaderboard:\n- Point of Contact:",
"### Dataset Summary",
"### Supported Tasks and Leaderboards",
"### Languages",
"## Dataset Structure",
"### Data Instances",
"### Data Fields",
"### Data Splits",
"## Dataset Creation",
"### Curation Rationale",
"### Source Data",
"#### Initial Data Collection and Normalization",
"#### Who are the source language producers?",
"### Annotations",
"#### Annotation process",
"#### Who are the annotators?",
"### Personal and Sensitive Information",
"## Considerations for Using the Data",
"### Social Impact of Dataset",
"### Discussion of Biases",
"### Other Known Limitations",
"## Additional Information",
"### Dataset Curators",
"### Licensing Information",
"### Contributions\n\nThanks to @github-username for adding this dataset."
] |
6f70815ecc5a8a6bef1f20e5c889b8872a047c38 |
# Dataset version 2
Work in progress...
| albertvillanova/dummy-version | [
"source_datasets:extended|go_emotions",
"license:openrail",
"region:us"
] | 2022-11-30T08:53:55+00:00 | {"license": "openrail", "source_datasets": ["extended|go_emotions"]} | 2022-12-02T10:43:51+00:00 | [] | [] | TAGS
#source_datasets-extended|go_emotions #license-openrail #region-us
|
# Dataset version 2
Work in progress...
| [
"# Dataset version 2\n\nWork in progress..."
] | [
"TAGS\n#source_datasets-extended|go_emotions #license-openrail #region-us \n",
"# Dataset version 2\n\nWork in progress..."
] |
5fb84d922ac6af2428ac1453f0b237fba17b2140 | # Dataset Card for "hindawi"
[More Information needed](https://github.com/huggingface/datasets/blob/main/CONTRIBUTING.md#how-to-contribute-to-the-dataset-cards) | gagan3012/hindawi | [
"region:us"
] | 2022-11-30T09:16:31+00:00 | {"dataset_info": {"features": [{"name": "image", "dtype": "image"}, {"name": "label", "dtype": {"class_label": {"names": {"0": "Noto_Sans_Arabic", "1": "Readex_Pro", "2": "Amiri", "3": "Noto_Kufi_Arabic", "4": "Reem_Kufi_Fun", "5": "Lateef", "6": "Changa", "7": "Kufam", "8": "ElMessiri", "9": "Reem_Kufi", "10": "Noto_Naskh_Arabic", "11": "Reem_Kufi_Ink", "12": "Tajawal", "13": "Aref_Ruqaa_Ink", "14": "Markazi_Text", "15": "IBM_Plex_Sans_Arabic", "16": "Vazirmatn", "17": "Harmattan", "18": "Gulzar", "19": "Scheherazade_New", "20": "Cairo", "21": "Amiri_Quran", "22": "Noto_Nastaliq_Urdu", "23": "Mada", "24": "Aref_Ruqaa", "25": "Almarai", "26": "Alkalami", "27": "Qahiri"}}}}], "splits": [{"name": "train", "num_bytes": 4098675549.992, "num_examples": 64624}, {"name": "validation", "num_bytes": 459422119.624, "num_examples": 7196}], "download_size": 4536653671, "dataset_size": 4558097669.616}} | 2022-12-12T00:34:11+00:00 | [] | [] | TAGS
#region-us
| # Dataset Card for "hindawi"
More Information needed | [
"# Dataset Card for \"hindawi\"\n\nMore Information needed"
] | [
"TAGS\n#region-us \n",
"# Dataset Card for \"hindawi\"\n\nMore Information needed"
] |
901ab25812e9d8de92aae67ad015699f001a4b58 |
# ShapeNet SDF Sample Dataset
This is a subset of the [ShapeNet SDF Dataset](https://ls7-data.cs.tu-dortmund.de/shape_net/ShapeNet_SDF.tar.gz) provided by the [ShapeGan Project](https://github.com/marian42/shapegan).<br>
Only Uniform SDF samples are included.
### Contents
The dataset contains 8,320 data samples. Each data sample contains 200,000 uniformly distributed points and their corresponding SDF values.<br>
The dataset contains three shape classes:
* Airplanes (2156 samples)
* Chairs (4189 samples)
* Sofas (1975 samples) | AlexWolski/ShapeNet-SDF-Uniform | [
"annotations_creators:no-annotation",
"size_categories:1K<n<10K",
"Artificial Intelligence",
"Machine Learning",
"Computational Geometry",
"region:us"
] | 2022-11-30T09:17:03+00:00 | {"annotations_creators": ["no-annotation"], "size_categories": ["1K<n<10K"], "pretty_name": "ShapeNet SDF Uniform", "tags": ["Artificial Intelligence", "Machine Learning", "Computational Geometry"]} | 2022-11-30T12:08:06+00:00 | [] | [] | TAGS
#annotations_creators-no-annotation #size_categories-1K<n<10K #Artificial Intelligence #Machine Learning #Computational Geometry #region-us
|
# ShapeNet SDF Sample Dataset
This is a subset of the ShapeNet SDF Dataset provided by the ShapeGan Project.<br>
Only Uniform SDF samples are included.
### Contents
The dataset contains 8,320 data samples. Each data sample contains 200,000 uniformly distributed points and their corresponding SDF values.<br>
The dataset contains three shape classes:
* Airplanes (2156 samples)
* Chairs (4189 samples)
* Sofas (1975 samples) | [
"# ShapeNet SDF Sample Dataset\n\nThis is a subset of the ShapeNet SDF Dataset provided by the ShapeGan Project.<br>\nOnly Uniform SDF samples are included.",
"### Contents\n\nThe dataset contains 8,320 data samples. Each data sample contains 200,000 uniformly distributed points and their corresponding SDF values.<br>\nThe dataset contains three shape classes:\n\n* Airplanes (2156 samples)\n* Chairs (4189 samples)\n* Sofas (1975 samples)"
] | [
"TAGS\n#annotations_creators-no-annotation #size_categories-1K<n<10K #Artificial Intelligence #Machine Learning #Computational Geometry #region-us \n",
"# ShapeNet SDF Sample Dataset\n\nThis is a subset of the ShapeNet SDF Dataset provided by the ShapeGan Project.<br>\nOnly Uniform SDF samples are included.",
"### Contents\n\nThe dataset contains 8,320 data samples. Each data sample contains 200,000 uniformly distributed points and their corresponding SDF values.<br>\nThe dataset contains three shape classes:\n\n* Airplanes (2156 samples)\n* Chairs (4189 samples)\n* Sofas (1975 samples)"
] |
40afa4c0aae68de7f75c8837c303ffcac1630cc4 | # Dataset Card for "laion-hd-subset"
[More Information needed](https://github.com/huggingface/datasets/blob/main/CONTRIBUTING.md#how-to-contribute-to-the-dataset-cards) | yuvalkirstain/laion-hd-subset | [
"region:us"
] | 2022-11-30T09:48:05+00:00 | {"dataset_info": {"features": [{"name": "similarity", "dtype": "float64"}, {"name": "hash", "dtype": "int64"}, {"name": "punsafe", "dtype": "float64"}, {"name": "pwatermark", "dtype": "float64"}, {"name": "LANGUAGE", "dtype": "string"}, {"name": "caption", "dtype": "string"}, {"name": "url", "dtype": "string"}, {"name": "key", "dtype": "string"}, {"name": "status", "dtype": "string"}, {"name": "error_message", "dtype": "null"}, {"name": "width", "dtype": "int64"}, {"name": "height", "dtype": "int64"}, {"name": "original_width", "dtype": "int64"}, {"name": "original_height", "dtype": "int64"}, {"name": "exif", "dtype": "string"}, {"name": "md5", "dtype": "string"}, {"name": "path", "dtype": "string"}, {"name": "image", "dtype": "image"}], "splits": [{"name": "train", "num_bytes": 4395359106.2963705, "num_examples": 13451}, {"name": "test", "num_bytes": 496904910.53063023, "num_examples": 1495}], "download_size": 4890190248, "dataset_size": 4892264016.827001}} | 2022-11-30T11:07:56+00:00 | [] | [] | TAGS
#region-us
| # Dataset Card for "laion-hd-subset"
More Information needed | [
"# Dataset Card for \"laion-hd-subset\"\n\nMore Information needed"
] | [
"TAGS\n#region-us \n",
"# Dataset Card for \"laion-hd-subset\"\n\nMore Information needed"
] |
95a568b549723e0a383b3582e8dd6e8174d15b50 | This is a Swedish NE dataset, Swe-NERC v1. Please see https://hdl.handle.net/10794/121 for more information.
Included here is the manually tagged part.
| vesteinn/swe-nerc | [
"region:us"
] | 2022-11-30T10:26:32+00:00 | {} | 2022-11-30T12:40:35+00:00 | [] | [] | TAGS
#region-us
| This is a Swedish NE dataset, Swe-NERC v1. Please see URL for more information.
Included here is the manually tagged part.
| [] | [
"TAGS\n#region-us \n"
] |
9a392a9604a06b4d20929bfb75e8bd220dd10062 | ## Table of Contents
- [Dataset Description](#dataset-description)
- [Dataset Summary](#dataset-summary)
- [Dataset Structure](#dataset-structure)
- [Data Fields](#data-fields)
- [Data Splits](#data-splits)
- [Additional Information](#additional-information)
- [Citation Information](#citation-information)
- [Contributions](#contributions)
# Dataset Description
- **Homepage: https://moleculenet.org/**
- **Repository: https://github.com/deepchem/deepchem/tree/master**
- **Paper: https://arxiv.org/abs/1703.00564**
## Dataset Summary
`tox21_SRp53` is a dataset included in [MoleculeNet](https://moleculenet.org/). The "Toxicology in the 21st Century" (Tox21) initiative created a public database measuring toxicity of compounds, which has been used in the 2014 Tox21 Data Challenge. This dataset contains qualitative toxicity measurements for 8k compounds on 12 different targets, including nuclear receptors and stress response pathways.
# Dataset Structure
## Data Fields
Each split contains
* `smiles`: the [SMILES](https://en.wikipedia.org/wiki/Simplified_molecular-input_line-entry_system) representation of a molecule
* `selfies`: the [SELFIES](https://github.com/aspuru-guzik-group/selfies) representation of a molecule
* `target`: Measured results (Active/Inactive) for bioassays
## Data Splits
The dataset is split into an 80/10/10 train/valid/test split using random split.
# Additional Information
## Citation Information
```
@misc{https://doi.org/10.48550/arxiv.1703.00564,
doi = {10.48550/ARXIV.1703.00564},
url = {https://arxiv.org/abs/1703.00564},
author = {Wu, Zhenqin and Ramsundar, Bharath and Feinberg, Evan N. and Gomes, Joseph and Geniesse, Caleb and Pappu, Aneesh S. and Leswing, Karl and Pande, Vijay},
keywords = {Machine Learning (cs.LG), Chemical Physics (physics.chem-ph), Machine Learning (stat.ML), FOS: Computer and information sciences, FOS: Computer and information sciences, FOS: Physical sciences, FOS: Physical sciences},
title = {MoleculeNet: A Benchmark for Molecular Machine Learning},
publisher = {arXiv},
year = {2017},
copyright = {arXiv.org perpetual, non-exclusive license}
}
```
## Contributions
Thanks to [@SauravMaheshkar](https://github.com/SauravMaheshkar) and [@zanussbaum](https://github.com/zanussbaum) for adding this dataset | SauravMaheshkar/tox21_SRp53 | [
"task_categories:other",
"task_categories:graph-ml",
"annotations_creators:machine-generated",
"language_creators:machine-generated",
"bio",
"bio-chem",
"molnet",
"molecule-net",
"biophysics",
"arxiv:1703.00564",
"region:us"
] | 2022-11-30T10:33:29+00:00 | {"annotations_creators": ["machine-generated"], "language_creators": ["machine-generated"], "task_categories": ["other", "graph-ml"], "task_ids": [], "pretty_name": "tox21_SRp53", "tags": ["bio", "bio-chem", "molnet", "molecule-net", "biophysics"]} | 2023-02-12T14:30:43+00:00 | [
"1703.00564"
] | [] | TAGS
#task_categories-other #task_categories-graph-ml #annotations_creators-machine-generated #language_creators-machine-generated #bio #bio-chem #molnet #molecule-net #biophysics #arxiv-1703.00564 #region-us
| ## Table of Contents
- Dataset Description
- Dataset Summary
- Dataset Structure
- Data Fields
- Data Splits
- Additional Information
- Citation Information
- Contributions
# Dataset Description
- Homepage: URL
- Repository: URL
- Paper: URL
## Dataset Summary
'tox21_SRp53' is a dataset included in MoleculeNet. The "Toxicology in the 21st Century" (Tox21) initiative created a public database measuring toxicity of compounds, which has been used in the 2014 Tox21 Data Challenge. This dataset contains qualitative toxicity measurements for 8k compounds on 12 different targets, including nuclear receptors and stress response pathways.
# Dataset Structure
## Data Fields
Each split contains
* 'smiles': the SMILES representation of a molecule
* 'selfies': the SELFIES representation of a molecule
* 'target': Measured results (Active/Inactive) for bioassays
## Data Splits
The dataset is split into an 80/10/10 train/valid/test split using random split.
# Additional Information
## Contributions
Thanks to @SauravMaheshkar and @zanussbaum for adding this dataset | [
"## Table of Contents\n- Dataset Description\n - Dataset Summary\n- Dataset Structure\n - Data Fields\n - Data Splits\n- Additional Information\n - Citation Information\n - Contributions",
"# Dataset Description\n- Homepage: URL\n- Repository: URL\n- Paper: URL",
"## Dataset Summary\n'tox21_SRp53' is a dataset included in MoleculeNet. The \"Toxicology in the 21st Century\" (Tox21) initiative created a public database measuring toxicity of compounds, which has been used in the 2014 Tox21 Data Challenge. This dataset contains qualitative toxicity measurements for 8k compounds on 12 different targets, including nuclear receptors and stress response pathways.",
"# Dataset Structure",
"## Data Fields\nEach split contains\n* 'smiles': the SMILES representation of a molecule\n* 'selfies': the SELFIES representation of a molecule\n* 'target': Measured results (Active/Inactive) for bioassays",
"## Data Splits\nThe dataset is split into an 80/10/10 train/valid/test split using random split.",
"# Additional Information",
"## Contributions\nThanks to @SauravMaheshkar and @zanussbaum for adding this dataset"
] | [
"TAGS\n#task_categories-other #task_categories-graph-ml #annotations_creators-machine-generated #language_creators-machine-generated #bio #bio-chem #molnet #molecule-net #biophysics #arxiv-1703.00564 #region-us \n",
"## Table of Contents\n- Dataset Description\n - Dataset Summary\n- Dataset Structure\n - Data Fields\n - Data Splits\n- Additional Information\n - Citation Information\n - Contributions",
"# Dataset Description\n- Homepage: URL\n- Repository: URL\n- Paper: URL",
"## Dataset Summary\n'tox21_SRp53' is a dataset included in MoleculeNet. The \"Toxicology in the 21st Century\" (Tox21) initiative created a public database measuring toxicity of compounds, which has been used in the 2014 Tox21 Data Challenge. This dataset contains qualitative toxicity measurements for 8k compounds on 12 different targets, including nuclear receptors and stress response pathways.",
"# Dataset Structure",
"## Data Fields\nEach split contains\n* 'smiles': the SMILES representation of a molecule\n* 'selfies': the SELFIES representation of a molecule\n* 'target': Measured results (Active/Inactive) for bioassays",
"## Data Splits\nThe dataset is split into an 80/10/10 train/valid/test split using random split.",
"# Additional Information",
"## Contributions\nThanks to @SauravMaheshkar and @zanussbaum for adding this dataset"
] |
81c1547cf4314a74f60c136171327a164430ac7b |
# Germeval Task 2017: Shared Task on Aspect-based Sentiment in Social Media Customer Feedback
In the connected, modern world, customer feedback is a valuable source for insights on the quality of products or services. This feedback allows other customers to benefit from the experiences of others and enables businesses to react on requests, complaints or recommendations. However, the more people use a product or service, the more feedback is generated, which results in the major challenge of analyzing huge amounts of feedback in an efficient, but still meaningful way.
Thus, we propose a shared task on automatically analyzing customer reviews about “Deutsche Bahn” - the german public train operator with about two billion passengers each year.
Example:
> “RT @XXX: Da hört jemand in der Bahn so laut ‘700 Main Street’ durch seine Kopfhörer, dass ich mithören kann. :( :( :(“
As shown in the example, insights from reviews can be derived on different granularities. The review contains a general evaluation of the travel (The customer disliked the travel). Furthermore, the review evaluates a dedicated aspect of the train travel (“laut” → customer did not like the noise level).
Consequently, we frame the task as aspect-based sentiment analysis with four sub tasks:
## Data format
```
ID <tab> Text <tab> Relevance <tab> Sentiment <tab> Aspect:Polarity (whitespace separated)
```
## Links
- http://ltdata1.informatik.uni-hamburg.de/germeval2017/
- https://sites.google.com/view/germeval2017-absa/
## How to cite
```bibtex
@inproceedings{germevaltask2017,
title = {{GermEval 2017: Shared Task on Aspect-based Sentiment in Social Media Customer Feedback}},
author = {Michael Wojatzki and Eugen Ruppert and Sarah Holschneider and Torsten Zesch and Chris Biemann},
year = {2017},
booktitle = {Proceedings of the GermEval 2017 – Shared Task on Aspect-based Sentiment in Social Media Customer Feedback},
address={Berlin, Germany},
pages={1--12}
}
``` | malteos/germeval2017 | [
"language:de",
"region:us"
] | 2022-11-30T12:53:43+00:00 | {"language": ["de"]} | 2022-11-30T13:49:08+00:00 | [] | [
"de"
] | TAGS
#language-German #region-us
|
# Germeval Task 2017: Shared Task on Aspect-based Sentiment in Social Media Customer Feedback
In the connected, modern world, customer feedback is a valuable source for insights on the quality of products or services. This feedback allows other customers to benefit from the experiences of others and enables businesses to react on requests, complaints or recommendations. However, the more people use a product or service, the more feedback is generated, which results in the major challenge of analyzing huge amounts of feedback in an efficient, but still meaningful way.
Thus, we propose a shared task on automatically analyzing customer reviews about “Deutsche Bahn” - the german public train operator with about two billion passengers each year.
Example:
> “RT @XXX: Da hört jemand in der Bahn so laut ‘700 Main Street’ durch seine Kopfhörer, dass ich mithören kann. :( :( :(“
As shown in the example, insights from reviews can be derived on different granularities. The review contains a general evaluation of the travel (The customer disliked the travel). Furthermore, the review evaluates a dedicated aspect of the train travel (“laut” → customer did not like the noise level).
Consequently, we frame the task as aspect-based sentiment analysis with four sub tasks:
## Data format
## Links
- URL
- URL
## How to cite
| [
"# Germeval Task 2017: Shared Task on Aspect-based Sentiment in Social Media Customer Feedback\n\nIn the connected, modern world, customer feedback is a valuable source for insights on the quality of products or services. This feedback allows other customers to benefit from the experiences of others and enables businesses to react on requests, complaints or recommendations. However, the more people use a product or service, the more feedback is generated, which results in the major challenge of analyzing huge amounts of feedback in an efficient, but still meaningful way.\n\nThus, we propose a shared task on automatically analyzing customer reviews about “Deutsche Bahn” - the german public train operator with about two billion passengers each year.\n\nExample: \n\n> “RT @XXX: Da hört jemand in der Bahn so laut ‘700 Main Street’ durch seine Kopfhörer, dass ich mithören kann. :( :( :(“\n\nAs shown in the example, insights from reviews can be derived on different granularities. The review contains a general evaluation of the travel (The customer disliked the travel). Furthermore, the review evaluates a dedicated aspect of the train travel (“laut” → customer did not like the noise level).\n\nConsequently, we frame the task as aspect-based sentiment analysis with four sub tasks:",
"## Data format",
"## Links\n\n- URL\n- URL",
"## How to cite"
] | [
"TAGS\n#language-German #region-us \n",
"# Germeval Task 2017: Shared Task on Aspect-based Sentiment in Social Media Customer Feedback\n\nIn the connected, modern world, customer feedback is a valuable source for insights on the quality of products or services. This feedback allows other customers to benefit from the experiences of others and enables businesses to react on requests, complaints or recommendations. However, the more people use a product or service, the more feedback is generated, which results in the major challenge of analyzing huge amounts of feedback in an efficient, but still meaningful way.\n\nThus, we propose a shared task on automatically analyzing customer reviews about “Deutsche Bahn” - the german public train operator with about two billion passengers each year.\n\nExample: \n\n> “RT @XXX: Da hört jemand in der Bahn so laut ‘700 Main Street’ durch seine Kopfhörer, dass ich mithören kann. :( :( :(“\n\nAs shown in the example, insights from reviews can be derived on different granularities. The review contains a general evaluation of the travel (The customer disliked the travel). Furthermore, the review evaluates a dedicated aspect of the train travel (“laut” → customer did not like the noise level).\n\nConsequently, we frame the task as aspect-based sentiment analysis with four sub tasks:",
"## Data format",
"## Links\n\n- URL\n- URL",
"## How to cite"
] |
07c4ea16f5d86bc8678df33857a64172d6f588f6 |
# Dataset Card for [Dataset Name]
## Table of Contents
- [Table of Contents](#table-of-contents)
- [Dataset Description](#dataset-description)
- [Dataset Summary](#dataset-summary)
- [Supported Tasks and Leaderboards](#supported-tasks-and-leaderboards)
- [Languages](#languages)
- [Dataset Structure](#dataset-structure)
- [Data Instances](#data-instances)
- [Data Fields](#data-fields)
- [Data Splits](#data-splits)
- [Dataset Creation](#dataset-creation)
- [Curation Rationale](#curation-rationale)
- [Source Data](#source-data)
- [Annotations](#annotations)
- [Personal and Sensitive Information](#personal-and-sensitive-information)
- [Considerations for Using the Data](#considerations-for-using-the-data)
- [Social Impact of Dataset](#social-impact-of-dataset)
- [Discussion of Biases](#discussion-of-biases)
- [Other Known Limitations](#other-known-limitations)
- [Additional Information](#additional-information)
- [Dataset Curators](#dataset-curators)
- [Licensing Information](#licensing-information)
- [Citation Information](#citation-information)
- [Contributions](#contributions)
## Dataset Description
- **Homepage:**
- **Repository:**
- **Paper:**
- **Leaderboard:**
- **Point of Contact:**
### Dataset Summary
[More Information Needed]
### Supported Tasks and Leaderboards
[More Information Needed]
### Languages
[More Information Needed]
## Dataset Structure
### Data Instances
[More Information Needed]
### Data Fields
[More Information Needed]
### Data Splits
[More Information Needed]
## Dataset Creation
### Curation Rationale
[More Information Needed]
### Source Data
#### Initial Data Collection and Normalization
[More Information Needed]
#### Who are the source language producers?
[More Information Needed]
### Annotations
#### Annotation process
[More Information Needed]
#### Who are the annotators?
[More Information Needed]
### Personal and Sensitive Information
[More Information Needed]
## Considerations for Using the Data
### Social Impact of Dataset
[More Information Needed]
### Discussion of Biases
[More Information Needed]
### Other Known Limitations
[More Information Needed]
## Additional Information
### Dataset Curators
[More Information Needed]
### Licensing Information
[More Information Needed]
### Citation Information
[More Information Needed]
### Contributions
Thanks to [@github-username](https://github.com/<github-username>) for adding this dataset. | DTU54DL/common-accent-proc | [
"task_categories:token-classification",
"annotations_creators:expert-generated",
"language_creators:found",
"multilinguality:monolingual",
"size_categories:10K<n<100K",
"source_datasets:original",
"language:en",
"license:mit",
"region:us"
] | 2022-11-30T13:24:08+00:00 | {"annotations_creators": ["expert-generated"], "language_creators": ["found"], "language": ["en"], "license": ["mit"], "multilinguality": ["monolingual"], "size_categories": ["10K<n<100K"], "source_datasets": ["original"], "task_categories": ["token-classification"], "task_ids": ["token-classification-other-acronym-identification"], "paperswithcode_id": "acronym-identification", "pretty_name": "Acronym Identification Dataset", "dataset_info": {"features": [{"name": "audio", "dtype": {"audio": {"sampling_rate": 16000}}}, {"name": "sentence", "dtype": "string"}, {"name": "accent", "dtype": "string"}, {"name": "input_features", "sequence": {"sequence": "float32"}}, {"name": "labels", "sequence": "int64"}], "splits": [{"name": "train", "num_bytes": 11534718760.0, "num_examples": 10000}, {"name": "test", "num_bytes": 518496848.0, "num_examples": 451}], "download_size": 3935975243, "dataset_size": 12053215608.0}, "train-eval-index": [{"col_mapping": {"labels": "tags", "tokens": "tokens"}, "config": "default", "splits": {"eval_split": "test"}, "task": "token-classification", "task_id": "entity_extraction"}]} | 2022-11-30T20:41:55+00:00 | [] | [
"en"
] | TAGS
#task_categories-token-classification #annotations_creators-expert-generated #language_creators-found #multilinguality-monolingual #size_categories-10K<n<100K #source_datasets-original #language-English #license-mit #region-us
|
# Dataset Card for [Dataset Name]
## Table of Contents
- Table of Contents
- Dataset Description
- Dataset Summary
- Supported Tasks and Leaderboards
- Languages
- Dataset Structure
- Data Instances
- Data Fields
- Data Splits
- Dataset Creation
- Curation Rationale
- Source Data
- Annotations
- Personal and Sensitive Information
- Considerations for Using the Data
- Social Impact of Dataset
- Discussion of Biases
- Other Known Limitations
- Additional Information
- Dataset Curators
- Licensing Information
- Citation Information
- Contributions
## Dataset Description
- Homepage:
- Repository:
- Paper:
- Leaderboard:
- Point of Contact:
### Dataset Summary
### Supported Tasks and Leaderboards
### Languages
## Dataset Structure
### Data Instances
### Data Fields
### Data Splits
## Dataset Creation
### Curation Rationale
### Source Data
#### Initial Data Collection and Normalization
#### Who are the source language producers?
### Annotations
#### Annotation process
#### Who are the annotators?
### Personal and Sensitive Information
## Considerations for Using the Data
### Social Impact of Dataset
### Discussion of Biases
### Other Known Limitations
## Additional Information
### Dataset Curators
### Licensing Information
### Contributions
Thanks to @github-username for adding this dataset. | [
"# Dataset Card for [Dataset Name]",
"## Table of Contents\n- Table of Contents\n- Dataset Description\n - Dataset Summary\n - Supported Tasks and Leaderboards\n - Languages\n- Dataset Structure\n - Data Instances\n - Data Fields\n - Data Splits\n- Dataset Creation\n - Curation Rationale\n - Source Data\n - Annotations\n - Personal and Sensitive Information\n- Considerations for Using the Data\n - Social Impact of Dataset\n - Discussion of Biases\n - Other Known Limitations\n- Additional Information\n - Dataset Curators\n - Licensing Information\n - Citation Information\n - Contributions",
"## Dataset Description\n\n- Homepage:\n- Repository:\n- Paper:\n- Leaderboard:\n- Point of Contact:",
"### Dataset Summary",
"### Supported Tasks and Leaderboards",
"### Languages",
"## Dataset Structure",
"### Data Instances",
"### Data Fields",
"### Data Splits",
"## Dataset Creation",
"### Curation Rationale",
"### Source Data",
"#### Initial Data Collection and Normalization",
"#### Who are the source language producers?",
"### Annotations",
"#### Annotation process",
"#### Who are the annotators?",
"### Personal and Sensitive Information",
"## Considerations for Using the Data",
"### Social Impact of Dataset",
"### Discussion of Biases",
"### Other Known Limitations",
"## Additional Information",
"### Dataset Curators",
"### Licensing Information",
"### Contributions\n\nThanks to @github-username for adding this dataset."
] | [
"TAGS\n#task_categories-token-classification #annotations_creators-expert-generated #language_creators-found #multilinguality-monolingual #size_categories-10K<n<100K #source_datasets-original #language-English #license-mit #region-us \n",
"# Dataset Card for [Dataset Name]",
"## Table of Contents\n- Table of Contents\n- Dataset Description\n - Dataset Summary\n - Supported Tasks and Leaderboards\n - Languages\n- Dataset Structure\n - Data Instances\n - Data Fields\n - Data Splits\n- Dataset Creation\n - Curation Rationale\n - Source Data\n - Annotations\n - Personal and Sensitive Information\n- Considerations for Using the Data\n - Social Impact of Dataset\n - Discussion of Biases\n - Other Known Limitations\n- Additional Information\n - Dataset Curators\n - Licensing Information\n - Citation Information\n - Contributions",
"## Dataset Description\n\n- Homepage:\n- Repository:\n- Paper:\n- Leaderboard:\n- Point of Contact:",
"### Dataset Summary",
"### Supported Tasks and Leaderboards",
"### Languages",
"## Dataset Structure",
"### Data Instances",
"### Data Fields",
"### Data Splits",
"## Dataset Creation",
"### Curation Rationale",
"### Source Data",
"#### Initial Data Collection and Normalization",
"#### Who are the source language producers?",
"### Annotations",
"#### Annotation process",
"#### Who are the annotators?",
"### Personal and Sensitive Information",
"## Considerations for Using the Data",
"### Social Impact of Dataset",
"### Discussion of Biases",
"### Other Known Limitations",
"## Additional Information",
"### Dataset Curators",
"### Licensing Information",
"### Contributions\n\nThanks to @github-username for adding this dataset."
] |
5f2ed7957159a3386228b4ac8cbc9e72c0ccca18 |
# Dataset Card for [Dataset Name]
## Table of Contents
- [Table of Contents](#table-of-contents)
- [Dataset Description](#dataset-description)
- [Dataset Summary](#dataset-summary)
- [Supported Tasks and Leaderboards](#supported-tasks-and-leaderboards)
- [Languages](#languages)
- [Dataset Structure](#dataset-structure)
- [Data Instances](#data-instances)
- [Data Fields](#data-fields)
- [Data Splits](#data-splits)
- [Dataset Creation](#dataset-creation)
- [Curation Rationale](#curation-rationale)
- [Source Data](#source-data)
- [Annotations](#annotations)
- [Personal and Sensitive Information](#personal-and-sensitive-information)
- [Considerations for Using the Data](#considerations-for-using-the-data)
- [Social Impact of Dataset](#social-impact-of-dataset)
- [Discussion of Biases](#discussion-of-biases)
- [Other Known Limitations](#other-known-limitations)
- [Additional Information](#additional-information)
- [Dataset Curators](#dataset-curators)
- [Licensing Information](#licensing-information)
- [Citation Information](#citation-information)
- [Contributions](#contributions)
## Dataset Description
- **Homepage:**
- **Repository:**
- **Paper:**
- **Leaderboard:**
- **Point of Contact:**
### Dataset Summary
[More Information Needed]
### Supported Tasks and Leaderboards
[More Information Needed]
### Languages
[More Information Needed]
## Dataset Structure
### Data Instances
[More Information Needed]
### Data Fields
[More Information Needed]
### Data Splits
[More Information Needed]
## Dataset Creation
### Curation Rationale
[More Information Needed]
### Source Data
#### Initial Data Collection and Normalization
[More Information Needed]
#### Who are the source language producers?
[More Information Needed]
### Annotations
#### Annotation process
[More Information Needed]
#### Who are the annotators?
[More Information Needed]
### Personal and Sensitive Information
[More Information Needed]
## Considerations for Using the Data
### Social Impact of Dataset
[More Information Needed]
### Discussion of Biases
[More Information Needed]
### Other Known Limitations
[More Information Needed]
## Additional Information
### Dataset Curators
[More Information Needed]
### Licensing Information
[More Information Needed]
### Citation Information
[More Information Needed]
### Contributions
Thanks to [@github-username](https://github.com/<github-username>) for adding this dataset. | DTU54DL/common-accent-augmented | [
"task_categories:token-classification",
"annotations_creators:expert-generated",
"language_creators:found",
"multilinguality:monolingual",
"size_categories:10K<n<100K",
"source_datasets:original",
"language:en",
"license:mit",
"region:us"
] | 2022-11-30T15:42:10+00:00 | {"annotations_creators": ["expert-generated"], "language_creators": ["found"], "language": ["en"], "license": ["mit"], "multilinguality": ["monolingual"], "size_categories": ["10K<n<100K"], "source_datasets": ["original"], "task_categories": ["token-classification"], "task_ids": ["token-classification-other-acronym-identification"], "paperswithcode_id": "acronym-identification", "pretty_name": "Acronym Identification Dataset", "dataset_info": {"features": [{"name": "sentence", "dtype": "string"}, {"name": "accent", "dtype": "string"}, {"name": "input_features", "sequence": {"sequence": "float32"}}, {"name": "labels", "sequence": "int64"}], "splits": [{"name": "test", "num_bytes": 433226048, "num_examples": 451}, {"name": "train", "num_bytes": 9606026408, "num_examples": 10000}], "download_size": 2307300737, "dataset_size": 10039252456}, "train-eval-index": [{"col_mapping": {"labels": "tags", "tokens": "tokens"}, "config": "default", "splits": {"eval_split": "test"}, "task": "token-classification", "task_id": "entity_extraction"}]} | 2022-12-07T14:00:54+00:00 | [] | [
"en"
] | TAGS
#task_categories-token-classification #annotations_creators-expert-generated #language_creators-found #multilinguality-monolingual #size_categories-10K<n<100K #source_datasets-original #language-English #license-mit #region-us
|
# Dataset Card for [Dataset Name]
## Table of Contents
- Table of Contents
- Dataset Description
- Dataset Summary
- Supported Tasks and Leaderboards
- Languages
- Dataset Structure
- Data Instances
- Data Fields
- Data Splits
- Dataset Creation
- Curation Rationale
- Source Data
- Annotations
- Personal and Sensitive Information
- Considerations for Using the Data
- Social Impact of Dataset
- Discussion of Biases
- Other Known Limitations
- Additional Information
- Dataset Curators
- Licensing Information
- Citation Information
- Contributions
## Dataset Description
- Homepage:
- Repository:
- Paper:
- Leaderboard:
- Point of Contact:
### Dataset Summary
### Supported Tasks and Leaderboards
### Languages
## Dataset Structure
### Data Instances
### Data Fields
### Data Splits
## Dataset Creation
### Curation Rationale
### Source Data
#### Initial Data Collection and Normalization
#### Who are the source language producers?
### Annotations
#### Annotation process
#### Who are the annotators?
### Personal and Sensitive Information
## Considerations for Using the Data
### Social Impact of Dataset
### Discussion of Biases
### Other Known Limitations
## Additional Information
### Dataset Curators
### Licensing Information
### Contributions
Thanks to @github-username for adding this dataset. | [
"# Dataset Card for [Dataset Name]",
"## Table of Contents\n- Table of Contents\n- Dataset Description\n - Dataset Summary\n - Supported Tasks and Leaderboards\n - Languages\n- Dataset Structure\n - Data Instances\n - Data Fields\n - Data Splits\n- Dataset Creation\n - Curation Rationale\n - Source Data\n - Annotations\n - Personal and Sensitive Information\n- Considerations for Using the Data\n - Social Impact of Dataset\n - Discussion of Biases\n - Other Known Limitations\n- Additional Information\n - Dataset Curators\n - Licensing Information\n - Citation Information\n - Contributions",
"## Dataset Description\n\n- Homepage:\n- Repository:\n- Paper:\n- Leaderboard:\n- Point of Contact:",
"### Dataset Summary",
"### Supported Tasks and Leaderboards",
"### Languages",
"## Dataset Structure",
"### Data Instances",
"### Data Fields",
"### Data Splits",
"## Dataset Creation",
"### Curation Rationale",
"### Source Data",
"#### Initial Data Collection and Normalization",
"#### Who are the source language producers?",
"### Annotations",
"#### Annotation process",
"#### Who are the annotators?",
"### Personal and Sensitive Information",
"## Considerations for Using the Data",
"### Social Impact of Dataset",
"### Discussion of Biases",
"### Other Known Limitations",
"## Additional Information",
"### Dataset Curators",
"### Licensing Information",
"### Contributions\n\nThanks to @github-username for adding this dataset."
] | [
"TAGS\n#task_categories-token-classification #annotations_creators-expert-generated #language_creators-found #multilinguality-monolingual #size_categories-10K<n<100K #source_datasets-original #language-English #license-mit #region-us \n",
"# Dataset Card for [Dataset Name]",
"## Table of Contents\n- Table of Contents\n- Dataset Description\n - Dataset Summary\n - Supported Tasks and Leaderboards\n - Languages\n- Dataset Structure\n - Data Instances\n - Data Fields\n - Data Splits\n- Dataset Creation\n - Curation Rationale\n - Source Data\n - Annotations\n - Personal and Sensitive Information\n- Considerations for Using the Data\n - Social Impact of Dataset\n - Discussion of Biases\n - Other Known Limitations\n- Additional Information\n - Dataset Curators\n - Licensing Information\n - Citation Information\n - Contributions",
"## Dataset Description\n\n- Homepage:\n- Repository:\n- Paper:\n- Leaderboard:\n- Point of Contact:",
"### Dataset Summary",
"### Supported Tasks and Leaderboards",
"### Languages",
"## Dataset Structure",
"### Data Instances",
"### Data Fields",
"### Data Splits",
"## Dataset Creation",
"### Curation Rationale",
"### Source Data",
"#### Initial Data Collection and Normalization",
"#### Who are the source language producers?",
"### Annotations",
"#### Annotation process",
"#### Who are the annotators?",
"### Personal and Sensitive Information",
"## Considerations for Using the Data",
"### Social Impact of Dataset",
"### Discussion of Biases",
"### Other Known Limitations",
"## Additional Information",
"### Dataset Curators",
"### Licensing Information",
"### Contributions\n\nThanks to @github-username for adding this dataset."
] |
520557a22a9299f45211e9bf66ee75bcd4b8c8d7 | # Elite Voice Project
これはホロライブ所属Vtuberさくらみこ氏の声をデータセット化し音声認識などで活用できるようにする事を目的とした非公式プロジェクトです。
---
# LICENSEについて
## データセット内の音声データ
すべてのデータは、[hololive productionの二次創作ガイドライン](https://hololive.hololivepro.com/guidelines/)に準拠する形で利用されています。
これらのデータの著作権はカバー株式会社等が保有しており、リポジトリオーナー、コントリビューターは一切の権利を有しておりません。
---
# 当プロジェクトへのご協力
当プロジェクトは皆様のご協力を心より歓迎いたします。 以下の方法をご一読いただき、そのうえでプルリクエストをお願い致します。
## 始める前に
[hololive productionの二次創作ガイドライン](https://hololive.hololivepro.com/guidelines/)を必ずお読みください。
---
## 音声データの追加
基本的には、データセットに追加したい音声データを`audio_raw`ディレクトリ内の所定のディレクトリへ追加していただく形になります。
git等を使用して音声データを追加する場合にはgit-lfsが必要になります。事前にgit-lfsのインストールをお願い致します。
`audio_raw`ディレクトリ内の構造は以下の通りです。
```
audio_raw
├─twitch
│ ├─test
│ │ └─<ID>
│ │ ├─1.mp3
│ │ ├─2.mp3
│ │ ├─3.mp3
│ │ ├─.
│ │ └─.
│ └─train
│ └─<ID>
│ ├─1.mp3
│ ├─2.mp3
│ ├─3.mp3
│ ├─.
│ └─.
├─twitter
│ ├─test
│ │ └─<ID>
│ │ ├─1.mp3
│ │ ├─2.mp3
│ │ ├─3.mp3
│ │ ├─.
│ │ └─.
│ └─train
│ └─<ID>
│ ├─1.mp3
│ ├─2.mp3
│ ├─3.mp3
│ ├─.
│ └─.
└─youtube
├─test
│ └─<ID>
│ ├─1.mp3
│ ├─2.mp3
│ ├─3.mp3
│ ├─.
│ └─.
└─train
└─<ID>
├─1.mp3
├─2.mp3
├─3.mp3
├─.
└─.
```
- `youtube`, `twitch`, `twitch`ディレクトリはデータセットに追加するデータの切り出し元のプラットフォーム名です。
- `train`と`test`ディレクトリについてですが、[OpenAI Whisper](https://openai.com/blog/whisper/)等の学習を行う際にtrainとtest、2種類のデータが必要になるために存在しています。
- `train`と`test`には同じ配信から切り出したデータを入れても良いですが全く同じデータを入れることは辞めてください。正確に学習を行うことができなくなります。
- `<ID>`には音声データを切り出す元になった配信等のIDが入ります。
- YouTubeであれば`https://www.youtube.com/watch?v=X9zw0QF12Kc`の`X9zw0QF12Kc`がディレクトリ名となります。
- Twitterであれば`https://twitter.com/i/spaces/1lPKqmyQPOAKb`の`1lPKqmyQPOAKb`がディレクトリ名となります。
- Twitchであれば`https://www.twitch.tv/videos/824387510`の`824387510`がディレクトリ名となります。
- `<ID>`ディレクトリ内には連番でmp3形式の音声ファイルを入れてください。
- 音声データは30秒以内である必要があります。
- BGMやSE、ノイズ等が含まれる音声データは避けてください。
- あまりに短すぎる音声データは避けてください。(既にデータセットにある音声は削除予定です。)
- 出来る限り30秒に近い音声データを入れていただけると助かります。
- 文脈のある音声データが望ましいです。
- 英語の音声は避けてください。
---
## 書き起こしテキストデータの追加
基本的には、データセットに追加したい音声データの書き起こしテキストデータを`transcript_raw`ディレクトリ内の所定のディレクトリへ追加していただく形になります。
`transcript_raw`ディレクトリ内の構造は以下の通りです。
```
transcript_raw
├─twitch
│ ├─test
│ │ └─<ID>.csv
│ │
│ └─train
│ └─<ID>.csv
│
├─twitter
│ ├─test
│ │ └─<ID>.csv
│ │
│ └─train
│ └─<ID>.csv
│
└─youtube
├─test
│ └─<ID>.csv
│
└─train
└─<ID>.csv
```
- `youtube`, `twitch`, `twitch`ディレクトリはデータセットに追加するデータの切り出し元のプラットフォーム名です。
- `<ID>`には音声データを切り出す元になった配信等のIDが入ります。
- YouTubeであれば`https://www.youtube.com/watch?v=X9zw0QF12Kc`の`X9zw0QF12Kc`がディレクトリ名となります。
- Twitterであれば`https://twitter.com/i/spaces/1lPKqmyQPOAKb`の`1lPKqmyQPOAKb`がディレクトリ名となります。
- Twitchであれば`https://www.twitch.tv/videos/824387510`の`824387510`がディレクトリ名となります。
- `<ID>.csv`について
- 必ず`audio_raw`に追加した音声データに対応した書き起こしテキストを追加する必要があります。
- 句読点、!,?等は正確に入れてください。
- 半角英数字記号を使用してください。(!, ?, 1等)
- 漢数字は避けてください。
- csvファイルの1行目は必ず`path,sentence`で始めてください。
- 書き起こしテキストはWhisper等で一度書き起こしたものを修正して行く方法を推奨致します。
### CSVファイルの記述例
```csv
path,sentence
1.mp3,雷が落ちた時のみこ
2.mp3,コメント止まった?
3.mp3,見えてるー?いやコメント止まった。壊れた。
4.mp3,インターネット繋がってない!
5.mp3,雷鳴ったよまた
``` | Elite35P-Server/EliteVoiceProject | [
"annotations_creators:crowdsourced",
"language_creators:さくらみこ",
"language_creators:hololive production",
"multilinguality:monolingual",
"language:ja",
"license:other",
"region:us"
] | 2022-11-30T16:10:15+00:00 | {"annotations_creators": ["crowdsourced"], "language_creators": ["\u3055\u304f\u3089\u307f\u3053", "hololive production"], "language": ["ja"], "license": "other", "multilinguality": ["monolingual"]} | 2023-01-14T19:28:16+00:00 | [] | [
"ja"
] | TAGS
#annotations_creators-crowdsourced #language_creators-さくらみこ #language_creators-hololive production #multilinguality-monolingual #language-Japanese #license-other #region-us
| # Elite Voice Project
これはホロライブ所属Vtuberさくらみこ氏の声をデータセット化し音声認識などで活用できるようにする事を目的とした非公式プロジェクトです。
---
# LICENSEについて
## データセット内の音声データ
すべてのデータは、hololive productionの二次創作ガイドラインに準拠する形で利用されています。
これらのデータの著作権はカバー株式会社等が保有しており、リポジトリオーナー、コントリビューターは一切の権利を有しておりません。
---
# 当プロジェクトへのご協力
当プロジェクトは皆様のご協力を心より歓迎いたします。 以下の方法をご一読いただき、そのうえでプルリクエストをお願い致します。
## 始める前に
hololive productionの二次創作ガイドラインを必ずお読みください。
---
## 音声データの追加
基本的には、データセットに追加したい音声データを'audio_raw'ディレクトリ内の所定のディレクトリへ追加していただく形になります。
git等を使用して音声データを追加する場合にはgit-lfsが必要になります。事前にgit-lfsのインストールをお願い致します。
'audio_raw'ディレクトリ内の構造は以下の通りです。
- 'youtube', 'twitch', 'twitch'ディレクトリはデータセットに追加するデータの切り出し元のプラットフォーム名です。
- 'train'と'test'ディレクトリについてですが、OpenAI Whisper等の学習を行う際にtrainとtest、2種類のデータが必要になるために存在しています。
- 'train'と'test'には同じ配信から切り出したデータを入れても良いですが全く同じデータを入れることは辞めてください。正確に学習を行うことができなくなります。
- '<ID>'には音声データを切り出す元になった配信等のIDが入ります。
- YouTubeであれば'URL'の'X9zw0QF12Kc'がディレクトリ名となります。
- Twitterであれば'URL'の'1lPKqmyQPOAKb'がディレクトリ名となります。
- Twitchであれば'URL'の'824387510'がディレクトリ名となります。
- '<ID>'ディレクトリ内には連番でmp3形式の音声ファイルを入れてください。
- 音声データは30秒以内である必要があります。
- BGMやSE、ノイズ等が含まれる音声データは避けてください。
- あまりに短すぎる音声データは避けてください。(既にデータセットにある音声は削除予定です。)
- 出来る限り30秒に近い音声データを入れていただけると助かります。
- 文脈のある音声データが望ましいです。
- 英語の音声は避けてください。
---
## 書き起こしテキストデータの追加
基本的には、データセットに追加したい音声データの書き起こしテキストデータを'transcript_raw'ディレクトリ内の所定のディレクトリへ追加していただく形になります。
'transcript_raw'ディレクトリ内の構造は以下の通りです。
- 'youtube', 'twitch', 'twitch'ディレクトリはデータセットに追加するデータの切り出し元のプラットフォーム名です。
- '<ID>'には音声データを切り出す元になった配信等のIDが入ります。
- YouTubeであれば'URL'の'X9zw0QF12Kc'がディレクトリ名となります。
- Twitterであれば'URL'の'1lPKqmyQPOAKb'がディレクトリ名となります。
- Twitchであれば'URL'の'824387510'がディレクトリ名となります。
- '<ID>.csv'について
- 必ず'audio_raw'に追加した音声データに対応した書き起こしテキストを追加する必要があります。
- 句読点、!,?等は正確に入れてください。
- 半角英数字記号を使用してください。(!, ?, 1等)
- 漢数字は避けてください。
- csvファイルの1行目は必ず'path,sentence'で始めてください。
- 書き起こしテキストはWhisper等で一度書き起こしたものを修正して行く方法を推奨致します。
### CSVファイルの記述例
| [
"# Elite Voice Project\n\nこれはホロライブ所属Vtuberさくらみこ氏の声をデータセット化し音声認識などで活用できるようにする事を目的とした非公式プロジェクトです。\n\n---",
"# LICENSEについて",
"## データセット内の音声データ\n\nすべてのデータは、hololive productionの二次創作ガイドラインに準拠する形で利用されています。\n\nこれらのデータの著作権はカバー株式会社等が保有しており、リポジトリオーナー、コントリビューターは一切の権利を有しておりません。\n\n---",
"# 当プロジェクトへのご協力\n\n当プロジェクトは皆様のご協力を心より歓迎いたします。 以下の方法をご一読いただき、そのうえでプルリクエストをお願い致します。",
"## 始める前に\n\nhololive productionの二次創作ガイドラインを必ずお読みください。\n\n---",
"## 音声データの追加\n\n基本的には、データセットに追加したい音声データを'audio_raw'ディレクトリ内の所定のディレクトリへ追加していただく形になります。\n\ngit等を使用して音声データを追加する場合にはgit-lfsが必要になります。事前にgit-lfsのインストールをお願い致します。\n\n'audio_raw'ディレクトリ内の構造は以下の通りです。\n\n\n\n- 'youtube', 'twitch', 'twitch'ディレクトリはデータセットに追加するデータの切り出し元のプラットフォーム名です。\n\n- 'train'と'test'ディレクトリについてですが、OpenAI Whisper等の学習を行う際にtrainとtest、2種類のデータが必要になるために存在しています。\n- 'train'と'test'には同じ配信から切り出したデータを入れても良いですが全く同じデータを入れることは辞めてください。正確に学習を行うことができなくなります。\n\n- '<ID>'には音声データを切り出す元になった配信等のIDが入ります。\n - YouTubeであれば'URL'の'X9zw0QF12Kc'がディレクトリ名となります。\n - Twitterであれば'URL'の'1lPKqmyQPOAKb'がディレクトリ名となります。\n - Twitchであれば'URL'の'824387510'がディレクトリ名となります。\n\n- '<ID>'ディレクトリ内には連番でmp3形式の音声ファイルを入れてください。\n - 音声データは30秒以内である必要があります。\n - BGMやSE、ノイズ等が含まれる音声データは避けてください。\n - あまりに短すぎる音声データは避けてください。(既にデータセットにある音声は削除予定です。)\n - 出来る限り30秒に近い音声データを入れていただけると助かります。\n - 文脈のある音声データが望ましいです。\n - 英語の音声は避けてください。\n\n---",
"## 書き起こしテキストデータの追加\n\n基本的には、データセットに追加したい音声データの書き起こしテキストデータを'transcript_raw'ディレクトリ内の所定のディレクトリへ追加していただく形になります。\n\n'transcript_raw'ディレクトリ内の構造は以下の通りです。\n\n\n\n- 'youtube', 'twitch', 'twitch'ディレクトリはデータセットに追加するデータの切り出し元のプラットフォーム名です。\n\n- '<ID>'には音声データを切り出す元になった配信等のIDが入ります。\n - YouTubeであれば'URL'の'X9zw0QF12Kc'がディレクトリ名となります。\n - Twitterであれば'URL'の'1lPKqmyQPOAKb'がディレクトリ名となります。\n - Twitchであれば'URL'の'824387510'がディレクトリ名となります。\n\n- '<ID>.csv'について\n - 必ず'audio_raw'に追加した音声データに対応した書き起こしテキストを追加する必要があります。\n - 句読点、!,?等は正確に入れてください。\n - 半角英数字記号を使用してください。(!, ?, 1等)\n - 漢数字は避けてください。\n - csvファイルの1行目は必ず'path,sentence'で始めてください。\n - 書き起こしテキストはWhisper等で一度書き起こしたものを修正して行く方法を推奨致します。",
"### CSVファイルの記述例"
] | [
"TAGS\n#annotations_creators-crowdsourced #language_creators-さくらみこ #language_creators-hololive production #multilinguality-monolingual #language-Japanese #license-other #region-us \n",
"# Elite Voice Project\n\nこれはホロライブ所属Vtuberさくらみこ氏の声をデータセット化し音声認識などで活用できるようにする事を目的とした非公式プロジェクトです。\n\n---",
"# LICENSEについて",
"## データセット内の音声データ\n\nすべてのデータは、hololive productionの二次創作ガイドラインに準拠する形で利用されています。\n\nこれらのデータの著作権はカバー株式会社等が保有しており、リポジトリオーナー、コントリビューターは一切の権利を有しておりません。\n\n---",
"# 当プロジェクトへのご協力\n\n当プロジェクトは皆様のご協力を心より歓迎いたします。 以下の方法をご一読いただき、そのうえでプルリクエストをお願い致します。",
"## 始める前に\n\nhololive productionの二次創作ガイドラインを必ずお読みください。\n\n---",
"## 音声データの追加\n\n基本的には、データセットに追加したい音声データを'audio_raw'ディレクトリ内の所定のディレクトリへ追加していただく形になります。\n\ngit等を使用して音声データを追加する場合にはgit-lfsが必要になります。事前にgit-lfsのインストールをお願い致します。\n\n'audio_raw'ディレクトリ内の構造は以下の通りです。\n\n\n\n- 'youtube', 'twitch', 'twitch'ディレクトリはデータセットに追加するデータの切り出し元のプラットフォーム名です。\n\n- 'train'と'test'ディレクトリについてですが、OpenAI Whisper等の学習を行う際にtrainとtest、2種類のデータが必要になるために存在しています。\n- 'train'と'test'には同じ配信から切り出したデータを入れても良いですが全く同じデータを入れることは辞めてください。正確に学習を行うことができなくなります。\n\n- '<ID>'には音声データを切り出す元になった配信等のIDが入ります。\n - YouTubeであれば'URL'の'X9zw0QF12Kc'がディレクトリ名となります。\n - Twitterであれば'URL'の'1lPKqmyQPOAKb'がディレクトリ名となります。\n - Twitchであれば'URL'の'824387510'がディレクトリ名となります。\n\n- '<ID>'ディレクトリ内には連番でmp3形式の音声ファイルを入れてください。\n - 音声データは30秒以内である必要があります。\n - BGMやSE、ノイズ等が含まれる音声データは避けてください。\n - あまりに短すぎる音声データは避けてください。(既にデータセットにある音声は削除予定です。)\n - 出来る限り30秒に近い音声データを入れていただけると助かります。\n - 文脈のある音声データが望ましいです。\n - 英語の音声は避けてください。\n\n---",
"## 書き起こしテキストデータの追加\n\n基本的には、データセットに追加したい音声データの書き起こしテキストデータを'transcript_raw'ディレクトリ内の所定のディレクトリへ追加していただく形になります。\n\n'transcript_raw'ディレクトリ内の構造は以下の通りです。\n\n\n\n- 'youtube', 'twitch', 'twitch'ディレクトリはデータセットに追加するデータの切り出し元のプラットフォーム名です。\n\n- '<ID>'には音声データを切り出す元になった配信等のIDが入ります。\n - YouTubeであれば'URL'の'X9zw0QF12Kc'がディレクトリ名となります。\n - Twitterであれば'URL'の'1lPKqmyQPOAKb'がディレクトリ名となります。\n - Twitchであれば'URL'の'824387510'がディレクトリ名となります。\n\n- '<ID>.csv'について\n - 必ず'audio_raw'に追加した音声データに対応した書き起こしテキストを追加する必要があります。\n - 句読点、!,?等は正確に入れてください。\n - 半角英数字記号を使用してください。(!, ?, 1等)\n - 漢数字は避けてください。\n - csvファイルの1行目は必ず'path,sentence'で始めてください。\n - 書き起こしテキストはWhisper等で一度書き起こしたものを修正して行く方法を推奨致します。",
"### CSVファイルの記述例"
] |
6d84ffafa2b74d9c9b8d567ad338ad2e6c255a6d |
# Dataset Card for JSNLI
[](https://github.com/shunk031/huggingface-datasets_jsnli/actions/workflows/ci.yaml)
## Table of Contents
- [Table of Contents](#table-of-contents)
- [Dataset Description](#dataset-description)
- [Dataset Summary](#dataset-summary)
- [Dataset Preprocessing](#dataset-preprocessing)
- [Supported Tasks and Leaderboards](#supported-tasks-and-leaderboards)
- [Languages](#languages)
- [Dataset Structure](#dataset-structure)
- [Data Instances](#data-instances)
- [Data Fields](#data-fields)
- [Data Splits](#data-splits)
- [Dataset Creation](#dataset-creation)
- [Curation Rationale](#curation-rationale)
- [Source Data](#source-data)
- [Annotations](#annotations)
- [Personal and Sensitive Information](#personal-and-sensitive-information)
- [Considerations for Using the Data](#considerations-for-using-the-data)
- [Social Impact of Dataset](#social-impact-of-dataset)
- [Discussion of Biases](#discussion-of-biases)
- [Other Known Limitations](#other-known-limitations)
- [Additional Information](#additional-information)
- [Dataset Curators](#dataset-curators)
- [Licensing Information](#licensing-information)
- [Citation Information](#citation-information)
- [Contributions](#contributions)
## Dataset Description
- Homepage: https://nlp.ist.i.kyoto-u.ac.jp/?%E6%97%A5%E6%9C%AC%E8%AA%9ESNLI%28JSNLI%29%E3%83%87%E3%83%BC%E3%82%BF%E3%82%BB%E3%83%83%E3%83%88
- Repository: https://github.com/shunk031/huggingface-datasets_jsnli
### Dataset Summary
[日本語 SNLI(JSNLI) データセット - KUROHASHI-CHU-MURAWAKI LAB](https://nlp.ist.i.kyoto-u.ac.jp/?%E6%97%A5%E6%9C%AC%E8%AA%9ESNLI%28JSNLI%29%E3%83%87%E3%83%BC%E3%82%BF%E3%82%BB%E3%83%83%E3%83%88 ) より:
> 本データセットは自然言語推論 (NLI) の標準的ベンチマークである [SNLI](https://nlp.stanford.edu/projects/snli/) を日本語に翻訳したものです。
### Dataset Preprocessing
### Supported Tasks and Leaderboards
### Languages
注釈はすべて日本語を主要言語としています。
## Dataset Structure
> データセットは TSV フォーマットで、各行がラベル、前提、仮説の三つ組を表します。前提、仮説は JUMAN++ によって形態素分割されています。以下に例をあげます。
```
entailment 自転車 で 2 人 の 男性 が レース で 競い ます 。 人々 は 自転車 に 乗って います 。
```
### Data Instances
```python
from datasets import load_dataset
load_dataset("shunk031/jsnli", "without-filtering")
```
```json
{
'label': 'neutral',
'premise': 'ガレージ で 、 壁 に ナイフ を 投げる 男 。',
'hypothesis': '男 は 魔法 の ショー の ため に ナイフ を 投げる 行為 を 練習 して い ます 。'
}
```
### Data Fields
### Data Splits
| name | train | validation |
|-------------------|--------:|-----------:|
| without-filtering | 548,014 | 3,916 |
| with-filtering | 533,005 | 3,916 |
## Dataset Creation
### Curation Rationale
### Source Data
#### Initial Data Collection and Normalization
#### Who are the source language producers?
### Annotations
#### Annotation process
> SNLI に機械翻訳を適用した後、評価データにクラウドソーシングによる正確なフィルタリング、学習データに計算機による自動フィルタリングを施すことで構築されています。
> データセットは学習データを全くフィルタリングしていないものと、フィルタリングした中で最も精度が高かったものの 2 種類を公開しています。データサイズは、フィルタリング前の学習データが 548,014 ペア、フィルタリング後の学習データが 533,005 ペア、評価データは 3,916 ペアです。詳細は参考文献を参照してください。
#### Who are the annotators?
### Personal and Sensitive Information
## Considerations for Using the Data
### Social Impact of Dataset
### Discussion of Biases
### Other Known Limitations
## Additional Information
> 本データセットに関するご質問は nl-resource あっと nlp.ist.i.kyoto-u.ac.jp 宛にお願いいたします。
### Dataset Curators
### Licensing Information
> このデータセットのライセンスは、SNLI のライセンスと同じ [CC BY-SA 4.0](https://creativecommons.org/licenses/by-sa/4.0/) に従います。SNLI に関しては参考文献を参照してください。
### Citation Information
```bibtex
@article{吉越卓見 2020 機械翻訳を用いた自然言語推論データセットの多言語化,
title={機械翻訳を用いた自然言語推論データセットの多言語化},
author={吉越卓見 and 河原大輔 and 黒橋禎夫 and others},
journal={研究報告自然言語処理 (NL)},
volume={2020},
number={6},
pages={1--8},
year={2020}
}
```
```bibtex
@inproceedings{bowman2015large,
title={A large annotated corpus for learning natural language inference},
author={Bowman, Samuel and Angeli, Gabor and Potts, Christopher and Manning, Christopher D},
booktitle={Proceedings of the 2015 Conference on Empirical Methods in Natural Language Processing},
pages={632--642},
year={2015}
}
```
```bibtex
@article{young2014image,
title={From image descriptions to visual denotations: New similarity metrics for semantic inference over event descriptions},
author={Young, Peter and Lai, Alice and Hodosh, Micah and Hockenmaier, Julia},
journal={Transactions of the Association for Computational Linguistics},
volume={2},
pages={67--78},
year={2014},
publisher={MIT Press}
}
```
### Contributions
JSNLI データセットを公開してくださった吉越 卓見さま,河原 大輔さま,黒橋 禎夫さまに心から感謝します。
| shunk031/jsnli | [
"task_categories:text-classification",
"task_ids:natural-language-inference",
"task_ids:multi-input-text-classification",
"multilinguality:monolingual",
"language:ja",
"license:cc-by-sa-4.0",
"natural-language-inference",
"nli",
"jsnli",
"region:us"
] | 2022-11-30T16:34:02+00:00 | {"language": ["ja"], "license": ["cc-by-sa-4.0"], "multilinguality": ["monolingual"], "task_categories": ["text-classification"], "task_ids": ["natural-language-inference", "multi-input-text-classification"], "tags": ["natural-language-inference", "nli", "jsnli"], "datasets": ["without-filtering", "with-filtering"], "metrics": ["accuracy"]} | 2022-12-12T07:36:58+00:00 | [] | [
"ja"
] | TAGS
#task_categories-text-classification #task_ids-natural-language-inference #task_ids-multi-input-text-classification #multilinguality-monolingual #language-Japanese #license-cc-by-sa-4.0 #natural-language-inference #nli #jsnli #region-us
| Dataset Card for JSNLI
======================
 データセット - KUROHASHI-CHU-MURAWAKI LAB より:
>
> 本データセットは自然言語推論 (NLI) の標準的ベンチマークである SNLI を日本語に翻訳したものです。
>
>
>
### Dataset Preprocessing
### Supported Tasks and Leaderboards
### Languages
注釈はすべて日本語を主要言語としています。
Dataset Structure
-----------------
>
> データセットは TSV フォーマットで、各行がラベル、前提、仮説の三つ組を表します。前提、仮説は JUMAN++ によって形態素分割されています。以下に例をあげます。
>
>
>
### Data Instances
### Data Fields
### Data Splits
Dataset Creation
----------------
### Curation Rationale
### Source Data
#### Initial Data Collection and Normalization
#### Who are the source language producers?
### Annotations
#### Annotation process
>
> SNLI に機械翻訳を適用した後、評価データにクラウドソーシングによる正確なフィルタリング、学習データに計算機による自動フィルタリングを施すことで構築されています。
> データセットは学習データを全くフィルタリングしていないものと、フィルタリングした中で最も精度が高かったものの 2 種類を公開しています。データサイズは、フィルタリング前の学習データが 548,014 ペア、フィルタリング後の学習データが 533,005 ペア、評価データは 3,916 ペアです。詳細は参考文献を参照してください。
>
>
>
#### Who are the annotators?
### Personal and Sensitive Information
Considerations for Using the Data
---------------------------------
### Social Impact of Dataset
### Discussion of Biases
### Other Known Limitations
Additional Information
----------------------
>
> 本データセットに関するご質問は nl-resource あっと URL.i.URL 宛にお願いいたします。
>
>
>
### Dataset Curators
### Licensing Information
>
> このデータセットのライセンスは、SNLI のライセンスと同じ CC BY-SA 4.0 に従います。SNLI に関しては参考文献を参照してください。
>
>
>
### Contributions
JSNLI データセットを公開してくださった吉越 卓見さま,河原 大輔さま,黒橋 禎夫さまに心から感謝します。
| [
"### Dataset Summary\n\n\n日本語 SNLI(JSNLI) データセット - KUROHASHI-CHU-MURAWAKI LAB より:\n\n\n\n> \n> 本データセットは自然言語推論 (NLI) の標準的ベンチマークである SNLI を日本語に翻訳したものです。\n> \n> \n>",
"### Dataset Preprocessing",
"### Supported Tasks and Leaderboards",
"### Languages\n\n\n注釈はすべて日本語を主要言語としています。\n\n\nDataset Structure\n-----------------\n\n\n\n> \n> データセットは TSV フォーマットで、各行がラベル、前提、仮説の三つ組を表します。前提、仮説は JUMAN++ によって形態素分割されています。以下に例をあげます。\n> \n> \n>",
"### Data Instances",
"### Data Fields",
"### Data Splits\n\n\n\nDataset Creation\n----------------",
"### Curation Rationale",
"### Source Data",
"#### Initial Data Collection and Normalization",
"#### Who are the source language producers?",
"### Annotations",
"#### Annotation process\n\n\n\n> \n> SNLI に機械翻訳を適用した後、評価データにクラウドソーシングによる正確なフィルタリング、学習データに計算機による自動フィルタリングを施すことで構築されています。\n> データセットは学習データを全くフィルタリングしていないものと、フィルタリングした中で最も精度が高かったものの 2 種類を公開しています。データサイズは、フィルタリング前の学習データが 548,014 ペア、フィルタリング後の学習データが 533,005 ペア、評価データは 3,916 ペアです。詳細は参考文献を参照してください。\n> \n> \n>",
"#### Who are the annotators?",
"### Personal and Sensitive Information\n\n\nConsiderations for Using the Data\n---------------------------------",
"### Social Impact of Dataset",
"### Discussion of Biases",
"### Other Known Limitations\n\n\nAdditional Information\n----------------------\n\n\n\n> \n> 本データセットに関するご質問は nl-resource あっと URL.i.URL 宛にお願いいたします。\n> \n> \n>",
"### Dataset Curators",
"### Licensing Information\n\n\n\n> \n> このデータセットのライセンスは、SNLI のライセンスと同じ CC BY-SA 4.0 に従います。SNLI に関しては参考文献を参照してください。\n> \n> \n>",
"### Contributions\n\n\nJSNLI データセットを公開してくださった吉越 卓見さま,河原 大輔さま,黒橋 禎夫さまに心から感謝します。"
] | [
"TAGS\n#task_categories-text-classification #task_ids-natural-language-inference #task_ids-multi-input-text-classification #multilinguality-monolingual #language-Japanese #license-cc-by-sa-4.0 #natural-language-inference #nli #jsnli #region-us \n",
"### Dataset Summary\n\n\n日本語 SNLI(JSNLI) データセット - KUROHASHI-CHU-MURAWAKI LAB より:\n\n\n\n> \n> 本データセットは自然言語推論 (NLI) の標準的ベンチマークである SNLI を日本語に翻訳したものです。\n> \n> \n>",
"### Dataset Preprocessing",
"### Supported Tasks and Leaderboards",
"### Languages\n\n\n注釈はすべて日本語を主要言語としています。\n\n\nDataset Structure\n-----------------\n\n\n\n> \n> データセットは TSV フォーマットで、各行がラベル、前提、仮説の三つ組を表します。前提、仮説は JUMAN++ によって形態素分割されています。以下に例をあげます。\n> \n> \n>",
"### Data Instances",
"### Data Fields",
"### Data Splits\n\n\n\nDataset Creation\n----------------",
"### Curation Rationale",
"### Source Data",
"#### Initial Data Collection and Normalization",
"#### Who are the source language producers?",
"### Annotations",
"#### Annotation process\n\n\n\n> \n> SNLI に機械翻訳を適用した後、評価データにクラウドソーシングによる正確なフィルタリング、学習データに計算機による自動フィルタリングを施すことで構築されています。\n> データセットは学習データを全くフィルタリングしていないものと、フィルタリングした中で最も精度が高かったものの 2 種類を公開しています。データサイズは、フィルタリング前の学習データが 548,014 ペア、フィルタリング後の学習データが 533,005 ペア、評価データは 3,916 ペアです。詳細は参考文献を参照してください。\n> \n> \n>",
"#### Who are the annotators?",
"### Personal and Sensitive Information\n\n\nConsiderations for Using the Data\n---------------------------------",
"### Social Impact of Dataset",
"### Discussion of Biases",
"### Other Known Limitations\n\n\nAdditional Information\n----------------------\n\n\n\n> \n> 本データセットに関するご質問は nl-resource あっと URL.i.URL 宛にお願いいたします。\n> \n> \n>",
"### Dataset Curators",
"### Licensing Information\n\n\n\n> \n> このデータセットのライセンスは、SNLI のライセンスと同じ CC BY-SA 4.0 に従います。SNLI に関しては参考文献を参照してください。\n> \n> \n>",
"### Contributions\n\n\nJSNLI データセットを公開してくださった吉越 卓見さま,河原 大輔さま,黒橋 禎夫さまに心から感謝します。"
] |
19f3c7b2cc41d158ff70a666de27f76098a1b2e6 |
# Dataset Card for clintox
## Table of Contents
- [Table of Contents](#table-of-contents)
- [Dataset Description](#dataset-description)
- [Dataset Summary](#dataset-summary)
- [Supported Tasks and Leaderboards](#supported-tasks-and-leaderboards)
- [Languages](#languages)
- [Dataset Structure](#dataset-structure)
- [Data Instances](#data-instances)
- [Data Fields](#data-fields)
- [Data Splits](#data-splits)
- [Dataset Creation](#dataset-creation)
- [Curation Rationale](#curation-rationale)
- [Source Data](#source-data)
- [Annotations](#annotations)
- [Personal and Sensitive Information](#personal-and-sensitive-information)
- [Considerations for Using the Data](#considerations-for-using-the-data)
- [Social Impact of Dataset](#social-impact-of-dataset)
- [Discussion of Biases](#discussion-of-biases)
- [Other Known Limitations](#other-known-limitations)
- [Additional Information](#additional-information)
- [Dataset Curators](#dataset-curators)
- [Licensing Information](#licensing-information)
- [Citation Information](#citation-information)
- [Contributions](#contributions)
## Dataset Description
- **Homepage: https://moleculenet.org/**
- **Repository: https://github.com/deepchem/deepchem/tree/master**
- **Paper: https://arxiv.org/abs/1703.00564**
### Dataset Summary
`clintox` is a dataset included in [MoleculeNet](https://moleculenet.org/). Qualitative data of drugs approved by the FDA and those that have failed clinical trials for toxicity reasons. This uses the `CT_TOX` task.
Note, there was one molecule in the training set that could not be converted to SELFIES (`*C(=O)[C@H](CCCCNC(=O)OCCOC)NC(=O)OCCOC`)
## Dataset Structure
### Data Fields
Each split contains
* `smiles`: the [SMILES](https://en.wikipedia.org/wiki/Simplified_molecular-input_line-entry_system) representation of a molecule
* `selfies`: the [SELFIES](https://github.com/aspuru-guzik-group/selfies) representation of a molecule
* `target`: clinical trial toxicity (or absence of toxicity)
### Data Splits
The dataset is split into an 80/10/10 train/valid/test split using scaffold split.
### Source Data
#### Initial Data Collection and Normalization
Data was originially generated by the Pande Group at Standford
### Licensing Information
This dataset was originally released under an MIT license
### Citation Information
```
@misc{https://doi.org/10.48550/arxiv.1703.00564,
doi = {10.48550/ARXIV.1703.00564},
url = {https://arxiv.org/abs/1703.00564},
author = {Wu, Zhenqin and Ramsundar, Bharath and Feinberg, Evan N. and Gomes, Joseph and Geniesse, Caleb and Pappu, Aneesh S. and Leswing, Karl and Pande, Vijay},
keywords = {Machine Learning (cs.LG), Chemical Physics (physics.chem-ph), Machine Learning (stat.ML), FOS: Computer and information sciences, FOS: Computer and information sciences, FOS: Physical sciences, FOS: Physical sciences},
title = {MoleculeNet: A Benchmark for Molecular Machine Learning},
publisher = {arXiv},
year = {2017},
copyright = {arXiv.org perpetual, non-exclusive license}
}
```
### Contributions
Thanks to [@zanussbaum](https://github.com/zanussbaum) for adding this dataset.
| zpn/clintox | [
"task_categories:other",
"annotations_creators:machine-generated",
"language_creators:machine-generated",
"multilinguality:monolingual",
"size_categories:1K<n<10K",
"license:mit",
"bio",
"bio-chem",
"molnet",
"molecule-net",
"biophysics",
"arxiv:1703.00564",
"region:us"
] | 2022-11-30T16:59:11+00:00 | {"annotations_creators": ["machine-generated"], "language_creators": ["machine-generated"], "license": ["mit"], "multilinguality": ["monolingual"], "size_categories": ["1K<n<10K"], "source_datasets": [], "task_categories": ["other"], "task_ids": [], "pretty_name": "clintox", "tags": ["bio", "bio-chem", "molnet", "molecule-net", "biophysics"]} | 2022-12-09T20:35:15+00:00 | [
"1703.00564"
] | [] | TAGS
#task_categories-other #annotations_creators-machine-generated #language_creators-machine-generated #multilinguality-monolingual #size_categories-1K<n<10K #license-mit #bio #bio-chem #molnet #molecule-net #biophysics #arxiv-1703.00564 #region-us
|
# Dataset Card for clintox
## Table of Contents
- Table of Contents
- Dataset Description
- Dataset Summary
- Supported Tasks and Leaderboards
- Languages
- Dataset Structure
- Data Instances
- Data Fields
- Data Splits
- Dataset Creation
- Curation Rationale
- Source Data
- Annotations
- Personal and Sensitive Information
- Considerations for Using the Data
- Social Impact of Dataset
- Discussion of Biases
- Other Known Limitations
- Additional Information
- Dataset Curators
- Licensing Information
- Citation Information
- Contributions
## Dataset Description
- Homepage: URL
- Repository: URL
- Paper: URL
### Dataset Summary
'clintox' is a dataset included in MoleculeNet. Qualitative data of drugs approved by the FDA and those that have failed clinical trials for toxicity reasons. This uses the 'CT_TOX' task.
Note, there was one molecule in the training set that could not be converted to SELFIES ('*C(=O)C@HOCCOC)NC(=O)OCCOC')
## Dataset Structure
### Data Fields
Each split contains
* 'smiles': the SMILES representation of a molecule
* 'selfies': the SELFIES representation of a molecule
* 'target': clinical trial toxicity (or absence of toxicity)
### Data Splits
The dataset is split into an 80/10/10 train/valid/test split using scaffold split.
### Source Data
#### Initial Data Collection and Normalization
Data was originially generated by the Pande Group at Standford
### Licensing Information
This dataset was originally released under an MIT license
### Contributions
Thanks to @zanussbaum for adding this dataset.
| [
"# Dataset Card for clintox",
"## Table of Contents\n- Table of Contents\n- Dataset Description\n - Dataset Summary\n - Supported Tasks and Leaderboards\n - Languages\n- Dataset Structure\n - Data Instances\n - Data Fields\n - Data Splits\n- Dataset Creation\n - Curation Rationale\n - Source Data\n - Annotations\n - Personal and Sensitive Information\n- Considerations for Using the Data\n - Social Impact of Dataset\n - Discussion of Biases\n - Other Known Limitations\n- Additional Information\n - Dataset Curators\n - Licensing Information\n - Citation Information\n - Contributions",
"## Dataset Description\n\n- Homepage: URL\n- Repository: URL\n- Paper: URL",
"### Dataset Summary\n\n'clintox' is a dataset included in MoleculeNet. Qualitative data of drugs approved by the FDA and those that have failed clinical trials for toxicity reasons. This uses the 'CT_TOX' task.\n\nNote, there was one molecule in the training set that could not be converted to SELFIES ('*C(=O)C@HOCCOC)NC(=O)OCCOC')",
"## Dataset Structure",
"### Data Fields\n\nEach split contains\n\n* 'smiles': the SMILES representation of a molecule\n* 'selfies': the SELFIES representation of a molecule\n* 'target': clinical trial toxicity (or absence of toxicity)",
"### Data Splits\n\nThe dataset is split into an 80/10/10 train/valid/test split using scaffold split.",
"### Source Data",
"#### Initial Data Collection and Normalization\n\nData was originially generated by the Pande Group at Standford",
"### Licensing Information\n\nThis dataset was originally released under an MIT license",
"### Contributions\n\nThanks to @zanussbaum for adding this dataset."
] | [
"TAGS\n#task_categories-other #annotations_creators-machine-generated #language_creators-machine-generated #multilinguality-monolingual #size_categories-1K<n<10K #license-mit #bio #bio-chem #molnet #molecule-net #biophysics #arxiv-1703.00564 #region-us \n",
"# Dataset Card for clintox",
"## Table of Contents\n- Table of Contents\n- Dataset Description\n - Dataset Summary\n - Supported Tasks and Leaderboards\n - Languages\n- Dataset Structure\n - Data Instances\n - Data Fields\n - Data Splits\n- Dataset Creation\n - Curation Rationale\n - Source Data\n - Annotations\n - Personal and Sensitive Information\n- Considerations for Using the Data\n - Social Impact of Dataset\n - Discussion of Biases\n - Other Known Limitations\n- Additional Information\n - Dataset Curators\n - Licensing Information\n - Citation Information\n - Contributions",
"## Dataset Description\n\n- Homepage: URL\n- Repository: URL\n- Paper: URL",
"### Dataset Summary\n\n'clintox' is a dataset included in MoleculeNet. Qualitative data of drugs approved by the FDA and those that have failed clinical trials for toxicity reasons. This uses the 'CT_TOX' task.\n\nNote, there was one molecule in the training set that could not be converted to SELFIES ('*C(=O)C@HOCCOC)NC(=O)OCCOC')",
"## Dataset Structure",
"### Data Fields\n\nEach split contains\n\n* 'smiles': the SMILES representation of a molecule\n* 'selfies': the SELFIES representation of a molecule\n* 'target': clinical trial toxicity (or absence of toxicity)",
"### Data Splits\n\nThe dataset is split into an 80/10/10 train/valid/test split using scaffold split.",
"### Source Data",
"#### Initial Data Collection and Normalization\n\nData was originially generated by the Pande Group at Standford",
"### Licensing Information\n\nThis dataset was originally released under an MIT license",
"### Contributions\n\nThanks to @zanussbaum for adding this dataset."
] |
f22a1ceffaa5ecbecebef3f5ab15a33c3ee14768 | # Dataset Card for AutoTrain Evaluator
This repository contains model predictions generated by [AutoTrain](https://huggingface.co/autotrain) for the following task and dataset:
* Task: Summarization
* Model: pszemraj/led-large-book-summary-continued-r1
* Dataset: kmfoda/booksum
* Config: kmfoda--booksum
* Split: test
To run new evaluation jobs, visit Hugging Face's [automatic model evaluator](https://huggingface.co/spaces/autoevaluate/model-evaluator).
## Contributions
Thanks to [@pszemraj](https://huggingface.co/pszemraj) for evaluating this model. | autoevaluate/autoeval-eval-kmfoda__booksum-kmfoda__booksum-dd12a3-2278572227 | [
"autotrain",
"evaluation",
"region:us"
] | 2022-11-30T17:03:35+00:00 | {"type": "predictions", "tags": ["autotrain", "evaluation"], "datasets": ["kmfoda/booksum"], "eval_info": {"task": "summarization", "model": "pszemraj/led-large-book-summary-continued-r1", "metrics": [], "dataset_name": "kmfoda/booksum", "dataset_config": "kmfoda--booksum", "dataset_split": "test", "col_mapping": {"text": "chapter", "target": "summary_text"}}} | 2022-12-01T01:50:12+00:00 | [] | [] | TAGS
#autotrain #evaluation #region-us
| # Dataset Card for AutoTrain Evaluator
This repository contains model predictions generated by AutoTrain for the following task and dataset:
* Task: Summarization
* Model: pszemraj/led-large-book-summary-continued-r1
* Dataset: kmfoda/booksum
* Config: kmfoda--booksum
* Split: test
To run new evaluation jobs, visit Hugging Face's automatic model evaluator.
## Contributions
Thanks to @pszemraj for evaluating this model. | [
"# Dataset Card for AutoTrain Evaluator\n\nThis repository contains model predictions generated by AutoTrain for the following task and dataset:\n\n* Task: Summarization\n* Model: pszemraj/led-large-book-summary-continued-r1\n* Dataset: kmfoda/booksum\n* Config: kmfoda--booksum\n* Split: test\n\nTo run new evaluation jobs, visit Hugging Face's automatic model evaluator.",
"## Contributions\n\nThanks to @pszemraj for evaluating this model."
] | [
"TAGS\n#autotrain #evaluation #region-us \n",
"# Dataset Card for AutoTrain Evaluator\n\nThis repository contains model predictions generated by AutoTrain for the following task and dataset:\n\n* Task: Summarization\n* Model: pszemraj/led-large-book-summary-continued-r1\n* Dataset: kmfoda/booksum\n* Config: kmfoda--booksum\n* Split: test\n\nTo run new evaluation jobs, visit Hugging Face's automatic model evaluator.",
"## Contributions\n\nThanks to @pszemraj for evaluating this model."
] |
0873f3eccba2eb07fc45a3b8faf4186c1345d6f4 |
# Dataset Card for delaney
## Table of Contents
- [Table of Contents](#table-of-contents)
- [Dataset Description](#dataset-description)
- [Dataset Summary](#dataset-summary)
- [Supported Tasks and Leaderboards](#supported-tasks-and-leaderboards)
- [Languages](#languages)
- [Dataset Structure](#dataset-structure)
- [Data Instances](#data-instances)
- [Data Fields](#data-fields)
- [Data Splits](#data-splits)
- [Dataset Creation](#dataset-creation)
- [Curation Rationale](#curation-rationale)
- [Source Data](#source-data)
- [Annotations](#annotations)
- [Personal and Sensitive Information](#personal-and-sensitive-information)
- [Considerations for Using the Data](#considerations-for-using-the-data)
- [Social Impact of Dataset](#social-impact-of-dataset)
- [Discussion of Biases](#discussion-of-biases)
- [Other Known Limitations](#other-known-limitations)
- [Additional Information](#additional-information)
- [Dataset Curators](#dataset-curators)
- [Licensing Information](#licensing-information)
- [Citation Information](#citation-information)
- [Contributions](#contributions)
## Dataset Description
- **Homepage: https://moleculenet.org/**
- **Repository: https://github.com/deepchem/deepchem/tree/master**
- **Paper: https://arxiv.org/abs/1703.00564**
### Dataset Summary
`delaney` (aka. `ESOL`) is a dataset included in [MoleculeNet](https://moleculenet.org/). Water solubility data(log solubility in mols per litre) for common organic small molecules.
## Dataset Structure
### Data Fields
Each split contains
* `smiles`: the [SMILES](https://en.wikipedia.org/wiki/Simplified_molecular-input_line-entry_system) representation of a molecule
* `selfies`: the [SELFIES](https://github.com/aspuru-guzik-group/selfies) representation of a molecule
* `target`: log solubility in mols per litre
### Data Splits
The dataset is split into an 80/10/10 train/valid/test split using scaffold split.
### Source Data
#### Initial Data Collection and Normalization
Data was originially generated by the Pande Group at Standford
### Licensing Information
This dataset was originally released under an MIT license
### Citation Information
```
@misc{https://doi.org/10.48550/arxiv.1703.00564,
doi = {10.48550/ARXIV.1703.00564},
url = {https://arxiv.org/abs/1703.00564},
author = {Wu, Zhenqin and Ramsundar, Bharath and Feinberg, Evan N. and Gomes, Joseph and Geniesse, Caleb and Pappu, Aneesh S. and Leswing, Karl and Pande, Vijay},
keywords = {Machine Learning (cs.LG), Chemical Physics (physics.chem-ph), Machine Learning (stat.ML), FOS: Computer and information sciences, FOS: Computer and information sciences, FOS: Physical sciences, FOS: Physical sciences},
title = {MoleculeNet: A Benchmark for Molecular Machine Learning},
publisher = {arXiv},
year = {2017},
copyright = {arXiv.org perpetual, non-exclusive license}
}
```
### Contributions
Thanks to [@zanussbaum](https://github.com/zanussbaum) for adding this dataset.
| zpn/delaney | [
"task_categories:other",
"annotations_creators:machine-generated",
"language_creators:machine-generated",
"multilinguality:monolingual",
"size_categories:n<1K",
"license:mit",
"bio",
"bio-chem",
"molnet",
"molecule-net",
"biophysics",
"arxiv:1703.00564",
"region:us"
] | 2022-11-30T17:06:42+00:00 | {"annotations_creators": ["machine-generated"], "language_creators": ["machine-generated"], "license": ["mit"], "multilinguality": ["monolingual"], "size_categories": ["n<1K"], "source_datasets": [], "task_categories": ["other"], "task_ids": [], "pretty_name": "delaney", "tags": ["bio", "bio-chem", "molnet", "molecule-net", "biophysics"]} | 2022-11-30T17:09:36+00:00 | [
"1703.00564"
] | [] | TAGS
#task_categories-other #annotations_creators-machine-generated #language_creators-machine-generated #multilinguality-monolingual #size_categories-n<1K #license-mit #bio #bio-chem #molnet #molecule-net #biophysics #arxiv-1703.00564 #region-us
|
# Dataset Card for delaney
## Table of Contents
- Table of Contents
- Dataset Description
- Dataset Summary
- Supported Tasks and Leaderboards
- Languages
- Dataset Structure
- Data Instances
- Data Fields
- Data Splits
- Dataset Creation
- Curation Rationale
- Source Data
- Annotations
- Personal and Sensitive Information
- Considerations for Using the Data
- Social Impact of Dataset
- Discussion of Biases
- Other Known Limitations
- Additional Information
- Dataset Curators
- Licensing Information
- Citation Information
- Contributions
## Dataset Description
- Homepage: URL
- Repository: URL
- Paper: URL
### Dataset Summary
'delaney' (aka. 'ESOL') is a dataset included in MoleculeNet. Water solubility data(log solubility in mols per litre) for common organic small molecules.
## Dataset Structure
### Data Fields
Each split contains
* 'smiles': the SMILES representation of a molecule
* 'selfies': the SELFIES representation of a molecule
* 'target': log solubility in mols per litre
### Data Splits
The dataset is split into an 80/10/10 train/valid/test split using scaffold split.
### Source Data
#### Initial Data Collection and Normalization
Data was originially generated by the Pande Group at Standford
### Licensing Information
This dataset was originally released under an MIT license
### Contributions
Thanks to @zanussbaum for adding this dataset.
| [
"# Dataset Card for delaney",
"## Table of Contents\n- Table of Contents\n- Dataset Description\n - Dataset Summary\n - Supported Tasks and Leaderboards\n - Languages\n- Dataset Structure\n - Data Instances\n - Data Fields\n - Data Splits\n- Dataset Creation\n - Curation Rationale\n - Source Data\n - Annotations\n - Personal and Sensitive Information\n- Considerations for Using the Data\n - Social Impact of Dataset\n - Discussion of Biases\n - Other Known Limitations\n- Additional Information\n - Dataset Curators\n - Licensing Information\n - Citation Information\n - Contributions",
"## Dataset Description\n\n- Homepage: URL\n- Repository: URL\n- Paper: URL",
"### Dataset Summary\n\n'delaney' (aka. 'ESOL') is a dataset included in MoleculeNet. Water solubility data(log solubility in mols per litre) for common organic small molecules.",
"## Dataset Structure",
"### Data Fields\n\nEach split contains\n\n* 'smiles': the SMILES representation of a molecule\n* 'selfies': the SELFIES representation of a molecule\n* 'target': log solubility in mols per litre",
"### Data Splits\n\nThe dataset is split into an 80/10/10 train/valid/test split using scaffold split.",
"### Source Data",
"#### Initial Data Collection and Normalization\n\nData was originially generated by the Pande Group at Standford",
"### Licensing Information\n\nThis dataset was originally released under an MIT license",
"### Contributions\n\nThanks to @zanussbaum for adding this dataset."
] | [
"TAGS\n#task_categories-other #annotations_creators-machine-generated #language_creators-machine-generated #multilinguality-monolingual #size_categories-n<1K #license-mit #bio #bio-chem #molnet #molecule-net #biophysics #arxiv-1703.00564 #region-us \n",
"# Dataset Card for delaney",
"## Table of Contents\n- Table of Contents\n- Dataset Description\n - Dataset Summary\n - Supported Tasks and Leaderboards\n - Languages\n- Dataset Structure\n - Data Instances\n - Data Fields\n - Data Splits\n- Dataset Creation\n - Curation Rationale\n - Source Data\n - Annotations\n - Personal and Sensitive Information\n- Considerations for Using the Data\n - Social Impact of Dataset\n - Discussion of Biases\n - Other Known Limitations\n- Additional Information\n - Dataset Curators\n - Licensing Information\n - Citation Information\n - Contributions",
"## Dataset Description\n\n- Homepage: URL\n- Repository: URL\n- Paper: URL",
"### Dataset Summary\n\n'delaney' (aka. 'ESOL') is a dataset included in MoleculeNet. Water solubility data(log solubility in mols per litre) for common organic small molecules.",
"## Dataset Structure",
"### Data Fields\n\nEach split contains\n\n* 'smiles': the SMILES representation of a molecule\n* 'selfies': the SELFIES representation of a molecule\n* 'target': log solubility in mols per litre",
"### Data Splits\n\nThe dataset is split into an 80/10/10 train/valid/test split using scaffold split.",
"### Source Data",
"#### Initial Data Collection and Normalization\n\nData was originially generated by the Pande Group at Standford",
"### Licensing Information\n\nThis dataset was originally released under an MIT license",
"### Contributions\n\nThanks to @zanussbaum for adding this dataset."
] |
8594ad93c7a05b0ecc54c29cd02b420488bf43be | # Dataset Card for AutoTrain Evaluator
This repository contains model predictions generated by [AutoTrain](https://huggingface.co/autotrain) for the following task and dataset:
* Task: Question Answering
* Model: Aiyshwariya/bert-finetuned-squad
* Dataset: squad
* Config: plain_text
* Split: validation
To run new evaluation jobs, visit Hugging Face's [automatic model evaluator](https://huggingface.co/spaces/autoevaluate/model-evaluator).
## Contributions
Thanks to [@bestuh](https://huggingface.co/bestuh) for evaluating this model. | autoevaluate/autoeval-eval-squad-plain_text-fee91a-2282172274 | [
"autotrain",
"evaluation",
"region:us"
] | 2022-11-30T17:11:01+00:00 | {"type": "predictions", "tags": ["autotrain", "evaluation"], "datasets": ["squad"], "eval_info": {"task": "extractive_question_answering", "model": "Aiyshwariya/bert-finetuned-squad", "metrics": ["squad", "squad_v2"], "dataset_name": "squad", "dataset_config": "plain_text", "dataset_split": "validation", "col_mapping": {"context": "context", "question": "question", "answers-text": "answers.text", "answers-answer_start": "answers.answer_start"}}} | 2022-11-30T17:14:25+00:00 | [] | [] | TAGS
#autotrain #evaluation #region-us
| # Dataset Card for AutoTrain Evaluator
This repository contains model predictions generated by AutoTrain for the following task and dataset:
* Task: Question Answering
* Model: Aiyshwariya/bert-finetuned-squad
* Dataset: squad
* Config: plain_text
* Split: validation
To run new evaluation jobs, visit Hugging Face's automatic model evaluator.
## Contributions
Thanks to @bestuh for evaluating this model. | [
"# Dataset Card for AutoTrain Evaluator\n\nThis repository contains model predictions generated by AutoTrain for the following task and dataset:\n\n* Task: Question Answering\n* Model: Aiyshwariya/bert-finetuned-squad\n* Dataset: squad\n* Config: plain_text\n* Split: validation\n\nTo run new evaluation jobs, visit Hugging Face's automatic model evaluator.",
"## Contributions\n\nThanks to @bestuh for evaluating this model."
] | [
"TAGS\n#autotrain #evaluation #region-us \n",
"# Dataset Card for AutoTrain Evaluator\n\nThis repository contains model predictions generated by AutoTrain for the following task and dataset:\n\n* Task: Question Answering\n* Model: Aiyshwariya/bert-finetuned-squad\n* Dataset: squad\n* Config: plain_text\n* Split: validation\n\nTo run new evaluation jobs, visit Hugging Face's automatic model evaluator.",
"## Contributions\n\nThanks to @bestuh for evaluating this model."
] |
47893bb4d8be15289e58d75665af1afad1dde61d |
# Dataset Card for clearance
## Table of Contents
- [Table of Contents](#table-of-contents)
- [Dataset Description](#dataset-description)
- [Dataset Summary](#dataset-summary)
- [Supported Tasks and Leaderboards](#supported-tasks-and-leaderboards)
- [Languages](#languages)
- [Dataset Structure](#dataset-structure)
- [Data Instances](#data-instances)
- [Data Fields](#data-fields)
- [Data Splits](#data-splits)
- [Dataset Creation](#dataset-creation)
- [Curation Rationale](#curation-rationale)
- [Source Data](#source-data)
- [Annotations](#annotations)
- [Personal and Sensitive Information](#personal-and-sensitive-information)
- [Considerations for Using the Data](#considerations-for-using-the-data)
- [Social Impact of Dataset](#social-impact-of-dataset)
- [Discussion of Biases](#discussion-of-biases)
- [Other Known Limitations](#other-known-limitations)
- [Additional Information](#additional-information)
- [Dataset Curators](#dataset-curators)
- [Licensing Information](#licensing-information)
- [Citation Information](#citation-information)
- [Contributions](#contributions)
## Dataset Description
- **Homepage: https://moleculenet.org/**
- **Repository: https://github.com/deepchem/deepchem/tree/master**
- **Paper: https://arxiv.org/abs/1703.00564**
### Dataset Summary
`clearance` is a dataset included in [Chemberta-2 benchmarking](https://arxiv.org/pdf/2209.01712.pdf).
## Dataset Structure
### Data Fields
Each split contains
* `smiles`: the [SMILES](https://en.wikipedia.org/wiki/Simplified_molecular-input_line-entry_system) representation of a molecule
* `selfies`: the [SELFIES](https://github.com/aspuru-guzik-group/selfies) representation of a molecule
* `target`:
### Data Splits
The dataset is split into an 80/10/10 train/valid/test split using scaffold split.
### Source Data
#### Initial Data Collection and Normalization
Data was originially generated by the Pande Group at Standford
### Licensing Information
This dataset was originally released under an MIT license
### Citation Information
```
@misc{https://doi.org/10.48550/arxiv.1703.00564,
doi = {10.48550/ARXIV.1703.00564},
url = {https://arxiv.org/abs/1703.00564},
author = {Wu, Zhenqin and Ramsundar, Bharath and Feinberg, Evan N. and Gomes, Joseph and Geniesse, Caleb and Pappu, Aneesh S. and Leswing, Karl and Pande, Vijay},
keywords = {Machine Learning (cs.LG), Chemical Physics (physics.chem-ph), Machine Learning (stat.ML), FOS: Computer and information sciences, FOS: Computer and information sciences, FOS: Physical sciences, FOS: Physical sciences},
title = {MoleculeNet: A Benchmark for Molecular Machine Learning},
publisher = {arXiv},
year = {2017},
copyright = {arXiv.org perpetual, non-exclusive license}
}
```
### Contributions
Thanks to [@zanussbaum](https://github.com/zanussbaum) for adding this dataset.
| zpn/clearance | [
"task_categories:other",
"annotations_creators:machine-generated",
"language_creators:machine-generated",
"multilinguality:monolingual",
"size_categories:n<1K",
"license:mit",
"bio",
"bio-chem",
"molnet",
"molecule-net",
"biophysics",
"arxiv:1703.00564",
"arxiv:2209.01712",
"region:us"
] | 2022-11-30T17:13:08+00:00 | {"annotations_creators": ["machine-generated"], "language_creators": ["machine-generated"], "license": ["mit"], "multilinguality": ["monolingual"], "size_categories": ["n<1K"], "source_datasets": [], "task_categories": ["other"], "task_ids": [], "pretty_name": "clearance", "tags": ["bio", "bio-chem", "molnet", "molecule-net", "biophysics"]} | 2022-11-30T17:20:47+00:00 | [
"1703.00564",
"2209.01712"
] | [] | TAGS
#task_categories-other #annotations_creators-machine-generated #language_creators-machine-generated #multilinguality-monolingual #size_categories-n<1K #license-mit #bio #bio-chem #molnet #molecule-net #biophysics #arxiv-1703.00564 #arxiv-2209.01712 #region-us
|
# Dataset Card for clearance
## Table of Contents
- Table of Contents
- Dataset Description
- Dataset Summary
- Supported Tasks and Leaderboards
- Languages
- Dataset Structure
- Data Instances
- Data Fields
- Data Splits
- Dataset Creation
- Curation Rationale
- Source Data
- Annotations
- Personal and Sensitive Information
- Considerations for Using the Data
- Social Impact of Dataset
- Discussion of Biases
- Other Known Limitations
- Additional Information
- Dataset Curators
- Licensing Information
- Citation Information
- Contributions
## Dataset Description
- Homepage: URL
- Repository: URL
- Paper: URL
### Dataset Summary
'clearance' is a dataset included in Chemberta-2 benchmarking.
## Dataset Structure
### Data Fields
Each split contains
* 'smiles': the SMILES representation of a molecule
* 'selfies': the SELFIES representation of a molecule
* 'target':
### Data Splits
The dataset is split into an 80/10/10 train/valid/test split using scaffold split.
### Source Data
#### Initial Data Collection and Normalization
Data was originially generated by the Pande Group at Standford
### Licensing Information
This dataset was originally released under an MIT license
### Contributions
Thanks to @zanussbaum for adding this dataset.
| [
"# Dataset Card for clearance",
"## Table of Contents\n- Table of Contents\n- Dataset Description\n - Dataset Summary\n - Supported Tasks and Leaderboards\n - Languages\n- Dataset Structure\n - Data Instances\n - Data Fields\n - Data Splits\n- Dataset Creation\n - Curation Rationale\n - Source Data\n - Annotations\n - Personal and Sensitive Information\n- Considerations for Using the Data\n - Social Impact of Dataset\n - Discussion of Biases\n - Other Known Limitations\n- Additional Information\n - Dataset Curators\n - Licensing Information\n - Citation Information\n - Contributions",
"## Dataset Description\n\n- Homepage: URL\n- Repository: URL\n- Paper: URL",
"### Dataset Summary\n\n'clearance' is a dataset included in Chemberta-2 benchmarking.",
"## Dataset Structure",
"### Data Fields\n\nEach split contains\n\n* 'smiles': the SMILES representation of a molecule\n* 'selfies': the SELFIES representation of a molecule\n* 'target':",
"### Data Splits\n\nThe dataset is split into an 80/10/10 train/valid/test split using scaffold split.",
"### Source Data",
"#### Initial Data Collection and Normalization\n\nData was originially generated by the Pande Group at Standford",
"### Licensing Information\n\nThis dataset was originally released under an MIT license",
"### Contributions\n\nThanks to @zanussbaum for adding this dataset."
] | [
"TAGS\n#task_categories-other #annotations_creators-machine-generated #language_creators-machine-generated #multilinguality-monolingual #size_categories-n<1K #license-mit #bio #bio-chem #molnet #molecule-net #biophysics #arxiv-1703.00564 #arxiv-2209.01712 #region-us \n",
"# Dataset Card for clearance",
"## Table of Contents\n- Table of Contents\n- Dataset Description\n - Dataset Summary\n - Supported Tasks and Leaderboards\n - Languages\n- Dataset Structure\n - Data Instances\n - Data Fields\n - Data Splits\n- Dataset Creation\n - Curation Rationale\n - Source Data\n - Annotations\n - Personal and Sensitive Information\n- Considerations for Using the Data\n - Social Impact of Dataset\n - Discussion of Biases\n - Other Known Limitations\n- Additional Information\n - Dataset Curators\n - Licensing Information\n - Citation Information\n - Contributions",
"## Dataset Description\n\n- Homepage: URL\n- Repository: URL\n- Paper: URL",
"### Dataset Summary\n\n'clearance' is a dataset included in Chemberta-2 benchmarking.",
"## Dataset Structure",
"### Data Fields\n\nEach split contains\n\n* 'smiles': the SMILES representation of a molecule\n* 'selfies': the SELFIES representation of a molecule\n* 'target':",
"### Data Splits\n\nThe dataset is split into an 80/10/10 train/valid/test split using scaffold split.",
"### Source Data",
"#### Initial Data Collection and Normalization\n\nData was originially generated by the Pande Group at Standford",
"### Licensing Information\n\nThis dataset was originally released under an MIT license",
"### Contributions\n\nThanks to @zanussbaum for adding this dataset."
] |
7c185766c22ed4bc33f33e9831376e1985c9a2df |
# Dataset Card for lipo
## Table of Contents
- [Table of Contents](#table-of-contents)
- [Dataset Description](#dataset-description)
- [Dataset Summary](#dataset-summary)
- [Supported Tasks and Leaderboards](#supported-tasks-and-leaderboards)
- [Languages](#languages)
- [Dataset Structure](#dataset-structure)
- [Data Instances](#data-instances)
- [Data Fields](#data-fields)
- [Data Splits](#data-splits)
- [Dataset Creation](#dataset-creation)
- [Curation Rationale](#curation-rationale)
- [Source Data](#source-data)
- [Annotations](#annotations)
- [Personal and Sensitive Information](#personal-and-sensitive-information)
- [Considerations for Using the Data](#considerations-for-using-the-data)
- [Social Impact of Dataset](#social-impact-of-dataset)
- [Discussion of Biases](#discussion-of-biases)
- [Other Known Limitations](#other-known-limitations)
- [Additional Information](#additional-information)
- [Dataset Curators](#dataset-curators)
- [Licensing Information](#licensing-information)
- [Citation Information](#citation-information)
- [Contributions](#contributions)
## Dataset Description
- **Homepage: https://moleculenet.org/**
- **Repository: https://github.com/deepchem/deepchem/tree/master**
- **Paper: https://arxiv.org/abs/1703.00564**
### Dataset Summary
`lipo` is a dataset included in [MoleculeNet](https://moleculenet.org/). It measures the experimental results of octanol/water distribution coefficient(logD at pH 7.4)
## Dataset Structure
### Data Fields
Each split contains
* `smiles`: the [SMILES](https://en.wikipedia.org/wiki/Simplified_molecular-input_line-entry_system) representation of a molecule
* `selfies`: the [SELFIES](https://github.com/aspuru-guzik-group/selfies) representation of a molecule
* `target`: octanol/water distribution coefficient(logD at pH 7.4)
### Data Splits
The dataset is split into an 80/10/10 train/valid/test split using scaffold split.
### Source Data
#### Initial Data Collection and Normalization
Data was originially generated by the Pande Group at Standford
### Licensing Information
This dataset was originally released under an MIT license
### Citation Information
```
@misc{https://doi.org/10.48550/arxiv.1703.00564,
doi = {10.48550/ARXIV.1703.00564},
url = {https://arxiv.org/abs/1703.00564},
author = {Wu, Zhenqin and Ramsundar, Bharath and Feinberg, Evan N. and Gomes, Joseph and Geniesse, Caleb and Pappu, Aneesh S. and Leswing, Karl and Pande, Vijay},
keywords = {Machine Learning (cs.LG), Chemical Physics (physics.chem-ph), Machine Learning (stat.ML), FOS: Computer and information sciences, FOS: Computer and information sciences, FOS: Physical sciences, FOS: Physical sciences},
title = {MoleculeNet: A Benchmark for Molecular Machine Learning},
publisher = {arXiv},
year = {2017},
copyright = {arXiv.org perpetual, non-exclusive license}
}
```
### Contributions
Thanks to [@zanussbaum](https://github.com/zanussbaum) for adding this dataset.
| zpn/lipo | [
"task_categories:other",
"annotations_creators:machine-generated",
"language_creators:machine-generated",
"multilinguality:monolingual",
"size_categories:1K<n<10K",
"license:mit",
"bio",
"bio-chem",
"molnet",
"molecule-net",
"biophysics",
"arxiv:1703.00564",
"region:us"
] | 2022-11-30T17:23:53+00:00 | {"annotations_creators": ["machine-generated"], "language_creators": ["machine-generated"], "license": ["mit"], "multilinguality": ["monolingual"], "size_categories": ["1K<n<10K"], "source_datasets": [], "task_categories": ["other"], "task_ids": [], "pretty_name": "lipo", "tags": ["bio", "bio-chem", "molnet", "molecule-net", "biophysics"]} | 2022-11-30T17:25:11+00:00 | [
"1703.00564"
] | [] | TAGS
#task_categories-other #annotations_creators-machine-generated #language_creators-machine-generated #multilinguality-monolingual #size_categories-1K<n<10K #license-mit #bio #bio-chem #molnet #molecule-net #biophysics #arxiv-1703.00564 #region-us
|
# Dataset Card for lipo
## Table of Contents
- Table of Contents
- Dataset Description
- Dataset Summary
- Supported Tasks and Leaderboards
- Languages
- Dataset Structure
- Data Instances
- Data Fields
- Data Splits
- Dataset Creation
- Curation Rationale
- Source Data
- Annotations
- Personal and Sensitive Information
- Considerations for Using the Data
- Social Impact of Dataset
- Discussion of Biases
- Other Known Limitations
- Additional Information
- Dataset Curators
- Licensing Information
- Citation Information
- Contributions
## Dataset Description
- Homepage: URL
- Repository: URL
- Paper: URL
### Dataset Summary
'lipo' is a dataset included in MoleculeNet. It measures the experimental results of octanol/water distribution coefficient(logD at pH 7.4)
## Dataset Structure
### Data Fields
Each split contains
* 'smiles': the SMILES representation of a molecule
* 'selfies': the SELFIES representation of a molecule
* 'target': octanol/water distribution coefficient(logD at pH 7.4)
### Data Splits
The dataset is split into an 80/10/10 train/valid/test split using scaffold split.
### Source Data
#### Initial Data Collection and Normalization
Data was originially generated by the Pande Group at Standford
### Licensing Information
This dataset was originally released under an MIT license
### Contributions
Thanks to @zanussbaum for adding this dataset.
| [
"# Dataset Card for lipo",
"## Table of Contents\n- Table of Contents\n- Dataset Description\n - Dataset Summary\n - Supported Tasks and Leaderboards\n - Languages\n- Dataset Structure\n - Data Instances\n - Data Fields\n - Data Splits\n- Dataset Creation\n - Curation Rationale\n - Source Data\n - Annotations\n - Personal and Sensitive Information\n- Considerations for Using the Data\n - Social Impact of Dataset\n - Discussion of Biases\n - Other Known Limitations\n- Additional Information\n - Dataset Curators\n - Licensing Information\n - Citation Information\n - Contributions",
"## Dataset Description\n\n- Homepage: URL\n- Repository: URL\n- Paper: URL",
"### Dataset Summary\n\n'lipo' is a dataset included in MoleculeNet. It measures the experimental results of octanol/water distribution coefficient(logD at pH 7.4)",
"## Dataset Structure",
"### Data Fields\n\nEach split contains\n\n* 'smiles': the SMILES representation of a molecule\n* 'selfies': the SELFIES representation of a molecule\n* 'target': octanol/water distribution coefficient(logD at pH 7.4)",
"### Data Splits\n\nThe dataset is split into an 80/10/10 train/valid/test split using scaffold split.",
"### Source Data",
"#### Initial Data Collection and Normalization\n\nData was originially generated by the Pande Group at Standford",
"### Licensing Information\n\nThis dataset was originally released under an MIT license",
"### Contributions\n\nThanks to @zanussbaum for adding this dataset."
] | [
"TAGS\n#task_categories-other #annotations_creators-machine-generated #language_creators-machine-generated #multilinguality-monolingual #size_categories-1K<n<10K #license-mit #bio #bio-chem #molnet #molecule-net #biophysics #arxiv-1703.00564 #region-us \n",
"# Dataset Card for lipo",
"## Table of Contents\n- Table of Contents\n- Dataset Description\n - Dataset Summary\n - Supported Tasks and Leaderboards\n - Languages\n- Dataset Structure\n - Data Instances\n - Data Fields\n - Data Splits\n- Dataset Creation\n - Curation Rationale\n - Source Data\n - Annotations\n - Personal and Sensitive Information\n- Considerations for Using the Data\n - Social Impact of Dataset\n - Discussion of Biases\n - Other Known Limitations\n- Additional Information\n - Dataset Curators\n - Licensing Information\n - Citation Information\n - Contributions",
"## Dataset Description\n\n- Homepage: URL\n- Repository: URL\n- Paper: URL",
"### Dataset Summary\n\n'lipo' is a dataset included in MoleculeNet. It measures the experimental results of octanol/water distribution coefficient(logD at pH 7.4)",
"## Dataset Structure",
"### Data Fields\n\nEach split contains\n\n* 'smiles': the SMILES representation of a molecule\n* 'selfies': the SELFIES representation of a molecule\n* 'target': octanol/water distribution coefficient(logD at pH 7.4)",
"### Data Splits\n\nThe dataset is split into an 80/10/10 train/valid/test split using scaffold split.",
"### Source Data",
"#### Initial Data Collection and Normalization\n\nData was originially generated by the Pande Group at Standford",
"### Licensing Information\n\nThis dataset was originally released under an MIT license",
"### Contributions\n\nThanks to @zanussbaum for adding this dataset."
] |
788e8b5132cc61fc19a71a4f9fb54a15b4d10b29 |
# Dataset Card for bbbp
## Table of Contents
- [Table of Contents](#table-of-contents)
- [Dataset Description](#dataset-description)
- [Dataset Summary](#dataset-summary)
- [Supported Tasks and Leaderboards](#supported-tasks-and-leaderboards)
- [Languages](#languages)
- [Dataset Structure](#dataset-structure)
- [Data Instances](#data-instances)
- [Data Fields](#data-fields)
- [Data Splits](#data-splits)
- [Dataset Creation](#dataset-creation)
- [Curation Rationale](#curation-rationale)
- [Source Data](#source-data)
- [Annotations](#annotations)
- [Personal and Sensitive Information](#personal-and-sensitive-information)
- [Considerations for Using the Data](#considerations-for-using-the-data)
- [Social Impact of Dataset](#social-impact-of-dataset)
- [Discussion of Biases](#discussion-of-biases)
- [Other Known Limitations](#other-known-limitations)
- [Additional Information](#additional-information)
- [Dataset Curators](#dataset-curators)
- [Licensing Information](#licensing-information)
- [Citation Information](#citation-information)
- [Contributions](#contributions)
## Dataset Description
- **Homepage: https://moleculenet.org/**
- **Repository: https://github.com/deepchem/deepchem/tree/master**
- **Paper: https://arxiv.org/abs/1703.00564**
### Dataset Summary
`bbbp` is a dataset included in [MoleculeNet](https://moleculenet.org/). This dataset has binary labels of blood-brain barrier penetration(permeability).
## Dataset Structure
### Data Fields
Each split contains
* `smiles`: the [SMILES](https://en.wikipedia.org/wiki/Simplified_molecular-input_line-entry_system) representation of a molecule
* `selfies`: the [SELFIES](https://github.com/aspuru-guzik-group/selfies) representation of a molecule
* `target`: blood-brain barrier penetration(permeability)
### Data Splits
The dataset is split into an 80/10/10 train/valid/test split using scaffold split.
### Source Data
#### Initial Data Collection and Normalization
Data was originially generated by the Pande Group at Standford
### Licensing Information
This dataset was originally released under an MIT license
### Citation Information
```
@misc{https://doi.org/10.48550/arxiv.1703.00564,
doi = {10.48550/ARXIV.1703.00564},
url = {https://arxiv.org/abs/1703.00564},
author = {Wu, Zhenqin and Ramsundar, Bharath and Feinberg, Evan N. and Gomes, Joseph and Geniesse, Caleb and Pappu, Aneesh S. and Leswing, Karl and Pande, Vijay},
keywords = {Machine Learning (cs.LG), Chemical Physics (physics.chem-ph), Machine Learning (stat.ML), FOS: Computer and information sciences, FOS: Computer and information sciences, FOS: Physical sciences, FOS: Physical sciences},
title = {MoleculeNet: A Benchmark for Molecular Machine Learning},
publisher = {arXiv},
year = {2017},
copyright = {arXiv.org perpetual, non-exclusive license}
}
```
### Contributions
Thanks to [@zanussbaum](https://github.com/zanussbaum) for adding this dataset.
| zpn/bbbp | [
"task_categories:other",
"annotations_creators:machine-generated",
"language_creators:machine-generated",
"multilinguality:monolingual",
"size_categories:1K<n<10K",
"license:mit",
"bio",
"bio-chem",
"molnet",
"molecule-net",
"biophysics",
"arxiv:1703.00564",
"region:us"
] | 2022-11-30T17:27:29+00:00 | {"annotations_creators": ["machine-generated"], "language_creators": ["machine-generated"], "license": ["mit"], "multilinguality": ["monolingual"], "size_categories": ["1K<n<10K"], "source_datasets": [], "task_categories": ["other"], "task_ids": [], "pretty_name": "bbbp", "tags": ["bio", "bio-chem", "molnet", "molecule-net", "biophysics"]} | 2022-12-09T20:33:42+00:00 | [
"1703.00564"
] | [] | TAGS
#task_categories-other #annotations_creators-machine-generated #language_creators-machine-generated #multilinguality-monolingual #size_categories-1K<n<10K #license-mit #bio #bio-chem #molnet #molecule-net #biophysics #arxiv-1703.00564 #region-us
|
# Dataset Card for bbbp
## Table of Contents
- Table of Contents
- Dataset Description
- Dataset Summary
- Supported Tasks and Leaderboards
- Languages
- Dataset Structure
- Data Instances
- Data Fields
- Data Splits
- Dataset Creation
- Curation Rationale
- Source Data
- Annotations
- Personal and Sensitive Information
- Considerations for Using the Data
- Social Impact of Dataset
- Discussion of Biases
- Other Known Limitations
- Additional Information
- Dataset Curators
- Licensing Information
- Citation Information
- Contributions
## Dataset Description
- Homepage: URL
- Repository: URL
- Paper: URL
### Dataset Summary
'bbbp' is a dataset included in MoleculeNet. This dataset has binary labels of blood-brain barrier penetration(permeability).
## Dataset Structure
### Data Fields
Each split contains
* 'smiles': the SMILES representation of a molecule
* 'selfies': the SELFIES representation of a molecule
* 'target': blood-brain barrier penetration(permeability)
### Data Splits
The dataset is split into an 80/10/10 train/valid/test split using scaffold split.
### Source Data
#### Initial Data Collection and Normalization
Data was originially generated by the Pande Group at Standford
### Licensing Information
This dataset was originally released under an MIT license
### Contributions
Thanks to @zanussbaum for adding this dataset.
| [
"# Dataset Card for bbbp",
"## Table of Contents\n- Table of Contents\n- Dataset Description\n - Dataset Summary\n - Supported Tasks and Leaderboards\n - Languages\n- Dataset Structure\n - Data Instances\n - Data Fields\n - Data Splits\n- Dataset Creation\n - Curation Rationale\n - Source Data\n - Annotations\n - Personal and Sensitive Information\n- Considerations for Using the Data\n - Social Impact of Dataset\n - Discussion of Biases\n - Other Known Limitations\n- Additional Information\n - Dataset Curators\n - Licensing Information\n - Citation Information\n - Contributions",
"## Dataset Description\n\n- Homepage: URL\n- Repository: URL\n- Paper: URL",
"### Dataset Summary\n\n'bbbp' is a dataset included in MoleculeNet. This dataset has binary labels of blood-brain barrier penetration(permeability).",
"## Dataset Structure",
"### Data Fields\n\nEach split contains\n\n* 'smiles': the SMILES representation of a molecule\n* 'selfies': the SELFIES representation of a molecule\n* 'target': blood-brain barrier penetration(permeability)",
"### Data Splits\n\nThe dataset is split into an 80/10/10 train/valid/test split using scaffold split.",
"### Source Data",
"#### Initial Data Collection and Normalization\n\nData was originially generated by the Pande Group at Standford",
"### Licensing Information\n\nThis dataset was originally released under an MIT license",
"### Contributions\n\nThanks to @zanussbaum for adding this dataset."
] | [
"TAGS\n#task_categories-other #annotations_creators-machine-generated #language_creators-machine-generated #multilinguality-monolingual #size_categories-1K<n<10K #license-mit #bio #bio-chem #molnet #molecule-net #biophysics #arxiv-1703.00564 #region-us \n",
"# Dataset Card for bbbp",
"## Table of Contents\n- Table of Contents\n- Dataset Description\n - Dataset Summary\n - Supported Tasks and Leaderboards\n - Languages\n- Dataset Structure\n - Data Instances\n - Data Fields\n - Data Splits\n- Dataset Creation\n - Curation Rationale\n - Source Data\n - Annotations\n - Personal and Sensitive Information\n- Considerations for Using the Data\n - Social Impact of Dataset\n - Discussion of Biases\n - Other Known Limitations\n- Additional Information\n - Dataset Curators\n - Licensing Information\n - Citation Information\n - Contributions",
"## Dataset Description\n\n- Homepage: URL\n- Repository: URL\n- Paper: URL",
"### Dataset Summary\n\n'bbbp' is a dataset included in MoleculeNet. This dataset has binary labels of blood-brain barrier penetration(permeability).",
"## Dataset Structure",
"### Data Fields\n\nEach split contains\n\n* 'smiles': the SMILES representation of a molecule\n* 'selfies': the SELFIES representation of a molecule\n* 'target': blood-brain barrier penetration(permeability)",
"### Data Splits\n\nThe dataset is split into an 80/10/10 train/valid/test split using scaffold split.",
"### Source Data",
"#### Initial Data Collection and Normalization\n\nData was originially generated by the Pande Group at Standford",
"### Licensing Information\n\nThis dataset was originally released under an MIT license",
"### Contributions\n\nThanks to @zanussbaum for adding this dataset."
] |
01c891497eccf050072a24aca0d66613e10d3310 |
# Dataset Card for fcc-comments
## Table of Contents
- [Table of Contents](#table-of-contents)
- [Dataset Description](#dataset-description)
- [Dataset Summary](#dataset-summary)
- [Supported Tasks and Leaderboards](#supported-tasks-and-leaderboards)
- [Languages](#languages)
- [Dataset Structure](#dataset-structure)
- [Data Instances](#data-instances)
- [Data Fields](#data-fields)
- [Data Splits](#data-splits)
- [Dataset Creation](#dataset-creation)
- [Curation Rationale](#curation-rationale)
- [Source Data](#source-data)
- [Annotations](#annotations)
- [Personal and Sensitive Information](#personal-and-sensitive-information)
- [Considerations for Using the Data](#considerations-for-using-the-data)
- [Social Impact of Dataset](#social-impact-of-dataset)
- [Discussion of Biases](#discussion-of-biases)
- [Other Known Limitations](#other-known-limitations)
- [Additional Information](#additional-information)
- [Dataset Curators](#dataset-curators)
- [Licensing Information](#licensing-information)
- [Citation Information](#citation-information)
- [Contributions](#contributions)
## Dataset Description
- **Repository: https://github.com/slnader/fcc-comments **
- **Paper: https://doi.org/10.1002/poi3.327 **
### Dataset Summary
Online comment floods during public consultations have posed unique governance challenges for
regulatory bodies seeking relevant information on proposed regulations.
How should regulatory bodies separate spam and fake comments from genuine submissions by the public,
especially when fake comments are designed to imitate ordinary citizens? How can regulatory bodies
achieve both breadth and depth in their citations to the comment corpus? What is the best way to
select comments that represent the average submission and comments that supply highly specialized
information?
`fcc-comments` is an annotated version of the comment corpus from the Federal Communications Commission's
(FCC) 2017 "Restoring Internet Freedom" proceeding. The source data were downloaded directly from the FCC's Electronic
Comment Filing System (ECFS) between January and February of 2019 and include raw comment text and metadata on
comment submissions. The comment data were processed to be in a consistent format
(machine-readable pdf or plain text), and annotated with three types of information: whether the comment was cited in the
agency's final order, the type of commenter (individual, interest group, business group), and whether the comment was associated with an in-person meeting.
The release also includes query-term and document-term matrices to facilitate keyword searches on the comment corpus.
An example of how these can be used with the bm25 algorithm can be found
[here](https://github.com/slnader/fcc-comments/blob/main/process_comments/1_score_comments.py).
## Dataset Structure
FCC relational database (fcc.pgsql): The core components of the database include a table for submission metadata,
a table for attachment metadata, a table for filer metadata, and a table that contains comment text if submitted in express format.
In addition to these core tables, there are several derived tables specific to the analyses in the paper,
including which submissions and attachments were cited in the final order, which submissions were associated with in-person meetings,
and which submissions were associated with interest groups. Full documentation of the tables can be found in fcc_database.md.
Attachments (attachments.tar.gz): Attachments to submissions that could be converted to text via OCR and saved in machine-readable pdf format.
The filenames are formatted as [submission_id]_[document_id].pdf, where submission_id and document_id are keys in the relational database.
Search datasets (search.tar.gz): Objects to facilitate prototyping of search algorithms on the comment corpus. Contains the following elements:
| Filename | description |
| ----------- | ----------- |
query_dtm.pickle | Query-term matrix (79x3986) in sparse csr format (rows are queries, columns are bigram keyword counts).
query_text.pickle | Dictionary keyed by the paragraph number in the FCC’s Notice of Proposed Rulemaking. Values are the text of the query containing a call for comments. |
search_dtms_express.pickle | Document-term matrix for express comments (3800691x3986) in sparse csr format (rows are comment pages, columns are bigram keyword counts). |
search_index_express.pickle | Pandas dataframe containing unique id and total term length for express comments. |
search_dtms.pickle | Document-term matrix for standard comment attachments (44655x3986) in sparse csr format (rows are comment pages, columns are bigram keyword counts). |
search_index.pickle | Pandas dataframe containing unique id and total term length for standard comment attachments. |
### Data Fields
The following tables are available in fcc.pgsql:
- comments: plain text comments associated with submissions
| column | type | description |
| ----------- | ----------- | ----------- |
| comment_id | character varying(64) | unique id for plain text comment |
comment_text | text | raw text of plain text comment
row_id | integer | row sequence for plain text comments
- submissions: metadata for submissions
| column | type | description |
| ----------- | ----------- | ----------- |
submission_id | character varying(20) | unique id for submission
submission_type | character varying(100) | type of submission (e.g., comment, reply, statement)
express_comment | numeric | 1 if express comment
date_received | date | date submission was received
contact_email | character varying(255) | submitter email address
city | character varying(255) | submitter city
address_line_1 | character varying(255) | submitter address line 1
address_line_2 | character varying(255) | submitter address line 2
state | character varying(255) | submitter state
zip_code | character varying(50) | submitter zip
comment_id | character varying(64) | unique id for plain text comment
- filers: names of filers associated with submissions
| column | type | description |
| ----------- | ----------- | ----------- |
submission_id | character varying(20) | unique id for submission
filer_name | character varying(250) | name of filer associated with submission
- documents: attachments associated with submissions
| column | type | description |
| ----------- | ----------- | ----------- |
submission_id | character varying(20) | unique id for submission
document_name | text | filename of attachment
download_status | numeric | status of attachment download
document_id | character varying(64) | unique id for attachment
file_extension | character varying(4) | file extension for attachment
- filers_cited: citations from final order
| column | type | description |
| ----------- | ----------- | ----------- |
point | numeric | paragraph number in final order
filer_name | character varying(250) | name of cited filer
submission_type | character varying(12) | type of submission as indicated in final order
page_numbers | text[] | cited page numbers
cite_id | integer | unique id for citation
filer_id | character varying(250) | id for cited filer
- docs_cited: attachments associated with cited submissions
| column | type | description |
| ----------- | ----------- | ----------- |
cite_id | numeric | unique id for citation
submission_id | character varying(20) | unique id for submission
document_id | character varying(64) | unique id for attachment
- near_duplicates: lookup table for comment near-duplicates
| column | type | description |
| ----------- | ----------- | ----------- |
target_document_id | unique id for target document
duplicate_document_id | unique id for duplicate of target document
- exact_duplicates: lookup table for comment exact duplicates
| column | type | description |
| ----------- | ----------- | ----------- |
target_document_id | character varying(100) | unique id for target document
duplicate_document_id | character varying(100) | unique id for duplicate of target document
- in_person_exparte: submissions associated with ex parte meeting
| column | type | description |
| ----------- | ----------- | ----------- |
submission_id | character varying(20) | unique id for submission
- interest_groups: submissions associated with interest groups
| column | type | description |
| ----------- | ----------- | ----------- |
submission_id | character varying(20) | unique id for submission
business | numeric | 1 if business group, 0 otherwise
## Dataset Creation
### Curation Rationale
The data were curated to perform information retrieval and summarization tasks as documented in https://doi.org/10.1002/poi3.327.
### Source Data
#### Initial Data Collection and Normalization
The data for this study come from the FCC's Electronic Comment Filing System (ECFS) system, accessed between January and February of 2019.
I converted the API responses into a normalized, relational database containing information on 23,951,967 submissions.
23,938,686 "express" submissions contained a single plain text comment submitted directly through the comment form.
13,821 "standard" submissions contained one or more comment documents submitted as attachments in various file formats.
While the FCC permitted any file format for attachments, I only consider documents attached in pdf, plain text, rich text,
and Microsoft Word file formats, and I drop submitted documents that were simply copies of the FCC’s official documents (e.g., the NPRM itself).
Using standard OCR software, I attempted to convert all attachments into plain text and saved them as machine-readable pdfs.
#### Who are the source language producers?
All submitters of public comments during the public comment period (but see note on fake comments in considerations).
### Annotations
#### Annotation process
- Citations: I consider citations from the main text of the FCC's final rule. I did not include citations to
supporting documents not available through ECFS (e.g., court decisions), nor did I include citations
to submissions from prior FCC proceedings. The direct citations to filed submissions are included
in a series of 1,186 footnotes. The FCC’s citation format typically followed a relatively standard
pattern: the name of the filer (e.g., Verizon), a description of the document (e.g., Comment), and
at times a page number. I extracted citations from the text using regular expressions. Based on a
random sample of paragraphs from the final order, the regular expressions identified 98% of eligible citations,
while successfully excluding all non-citation text. In total, this produced 1,886 unique citations.
I then identified which of the comments were cited. First, I identified all documents from the cited filer
that had enough pages to contain the page number cited (if provided), and, where applicable, whose filename
contained the moniker from the FCC’s citation (e.g., "Reply"). The majority of citations matched to only one
possible comment submitted, and I identified the re- maining cited comments through manual review of the citations.
In this way, I was able to tag documents associated with all but three citations. When the same cited document was
submitted under multiple separate submissions, I tagged all versions of the document as being cited.
- Commenter type: Comments are labeled as mass comments if 10 or more duplicate or near-duplicate copies were
submitted by individual commenters. Near-duplicates were defined as comments with non-zero identical information scores.
To identify the type of commenter for non-mass comments, I take advantage of the fact that the vast majority of organized
groups preferred standard submissions over express submissions. Any non-mass comment submitted as an express comment was
coded as coming from an individual. To distinguish between individuals and organizations that used standard submissions,
I use a first name and surname database from the names dataset Python package to characterize filer names as belonging to
individuals or organizations. I also use the domain of the submitter’s email address to re-categorize comments as coming
from organizations if they were submitted on behalf of organizations by an individual. Government officials were identified by
their .gov email addresses. I manually review this procedure for mischaracterizations. After obtaining a list of organization
names, I manually code each one as belonging to a business group or a non-business group. Government officials writing in
their official capacity were categorized as a non-business group.
- In-person meetings: To identify which commenters held in-person meetings with the agency, I collect all comments labeled
as an ex-parte submission in the EFCS. I manually review these submissions for mention of an in-person meeting. I label
a commenter as having held an in-person meeting if they submitted at least one ex-parte document that mentioned an in-person meeting.
#### Who are the annotators?
Annotations are a combination of automated and manual review done by the author.
### Personal and Sensitive Information
This dataset may contain personal and sensitive information, as there were no restrictions on what commenters could submit to
the agency. This dataset also contains numerous examples of profanity and spam. These comments represent what the FCC decided was
appropriate to share publicly on their own website.
## Considerations for Using the Data
### Discussion of Biases
This proceeding was famous for the large number of "fake" comments (comments impersonating ordinary citizens) submitted to the
agency (see [this report](https://ag.ny.gov/sites/default/files/oag-fakecommentsreport.pdf) by the NY AG for more information).
As such, this comment corpus contains a mix of computer-generated and natural language, and there is currently no way to reliably separate
mass comments submitted with the approval of the commenter and those submitted on behalf of the commenter without their knowledge.
## Additional Information
### Licensing Information
CreativeCommons Attribution-NonCommercial-ShareAlike 4.0 International.
### Citation Information
```
@article{handan2022,
title={Do fake online comments pose a threat to regulatory policymaking? Evidence from Internet regulation in the United States},
author={Handan-Nader, Cassandra},
journal={Policy \& Internet},
year={2022}
}
``` | slnader/fcc-comments | [
"task_categories:text-retrieval",
"task_ids:document-retrieval",
"annotations_creators:expert-generated",
"language_creators:found",
"multilinguality:monolingual",
"size_categories:10M<n<100M",
"source_datasets:original",
"language:en",
"license:cc-by-nc-sa-4.0",
"notice and comment",
"regulation",
"government",
"region:us"
] | 2022-11-30T17:38:32+00:00 | {"annotations_creators": ["expert-generated"], "language_creators": ["found"], "language": ["en"], "license": ["cc-by-nc-sa-4.0"], "multilinguality": ["monolingual"], "size_categories": ["10M<n<100M"], "source_datasets": ["original"], "task_categories": ["text-retrieval"], "task_ids": ["document-retrieval"], "pretty_name": "fcc-comments", "tags": ["notice and comment", "regulation", "government"]} | 2022-11-30T19:05:23+00:00 | [] | [
"en"
] | TAGS
#task_categories-text-retrieval #task_ids-document-retrieval #annotations_creators-expert-generated #language_creators-found #multilinguality-monolingual #size_categories-10M<n<100M #source_datasets-original #language-English #license-cc-by-nc-sa-4.0 #notice and comment #regulation #government #region-us
| Dataset Card for fcc-comments
=============================
Table of Contents
-----------------
* Table of Contents
* Dataset Description
+ Dataset Summary
+ Supported Tasks and Leaderboards
+ Languages
* Dataset Structure
+ Data Instances
+ Data Fields
+ Data Splits
* Dataset Creation
+ Curation Rationale
+ Source Data
+ Annotations
+ Personal and Sensitive Information
* Considerations for Using the Data
+ Social Impact of Dataset
+ Discussion of Biases
+ Other Known Limitations
* Additional Information
+ Dataset Curators
+ Licensing Information
+ Citation Information
+ Contributions
Dataset Description
-------------------
* Repository: URL
* Paper: URL
### Dataset Summary
Online comment floods during public consultations have posed unique governance challenges for
regulatory bodies seeking relevant information on proposed regulations.
How should regulatory bodies separate spam and fake comments from genuine submissions by the public,
especially when fake comments are designed to imitate ordinary citizens? How can regulatory bodies
achieve both breadth and depth in their citations to the comment corpus? What is the best way to
select comments that represent the average submission and comments that supply highly specialized
information?
'fcc-comments' is an annotated version of the comment corpus from the Federal Communications Commission's
(FCC) 2017 "Restoring Internet Freedom" proceeding. The source data were downloaded directly from the FCC's Electronic
Comment Filing System (ECFS) between January and February of 2019 and include raw comment text and metadata on
comment submissions. The comment data were processed to be in a consistent format
(machine-readable pdf or plain text), and annotated with three types of information: whether the comment was cited in the
agency's final order, the type of commenter (individual, interest group, business group), and whether the comment was associated with an in-person meeting.
The release also includes query-term and document-term matrices to facilitate keyword searches on the comment corpus.
An example of how these can be used with the bm25 algorithm can be found
here.
Dataset Structure
-----------------
FCC relational database (URL): The core components of the database include a table for submission metadata,
a table for attachment metadata, a table for filer metadata, and a table that contains comment text if submitted in express format.
In addition to these core tables, there are several derived tables specific to the analyses in the paper,
including which submissions and attachments were cited in the final order, which submissions were associated with in-person meetings,
and which submissions were associated with interest groups. Full documentation of the tables can be found in fcc\_database.md.
Attachments (URL): Attachments to submissions that could be converted to text via OCR and saved in machine-readable pdf format.
The filenames are formatted as [submission\_id]\_[document\_id].pdf, where submission\_id and document\_id are keys in the relational database.
Search datasets (URL): Objects to facilitate prototyping of search algorithms on the comment corpus. Contains the following elements:
### Data Fields
The following tables are available in URL:
* comments: plain text comments associated with submissions
column: comment\_id, type: character varying(64), description: unique id for plain text comment
column: comment\_text, type: text, description: raw text of plain text comment
column: row\_id, type: integer, description: row sequence for plain text comments
* submissions: metadata for submissions
column: submission\_id, type: character varying(20), description: unique id for submission
column: submission\_type, type: character varying(100), description: type of submission (e.g., comment, reply, statement)
column: express\_comment, type: numeric, description: 1 if express comment
column: date\_received, type: date, description: date submission was received
column: contact\_email, type: character varying(255), description: submitter email address
column: city, type: character varying(255), description: submitter city
column: address\_line\_1, type: character varying(255), description: submitter address line 1
column: address\_line\_2, type: character varying(255), description: submitter address line 2
column: state, type: character varying(255), description: submitter state
column: zip\_code, type: character varying(50), description: submitter zip
column: comment\_id, type: character varying(64), description: unique id for plain text comment
* filers: names of filers associated with submissions
column: submission\_id, type: character varying(20), description: unique id for submission
column: filer\_name, type: character varying(250), description: name of filer associated with submission
* documents: attachments associated with submissions
column: submission\_id, type: character varying(20), description: unique id for submission
column: document\_name, type: text, description: filename of attachment
column: download\_status, type: numeric, description: status of attachment download
column: document\_id, type: character varying(64), description: unique id for attachment
column: file\_extension, type: character varying(4), description: file extension for attachment
* filers\_cited: citations from final order
column: point, type: numeric, description: paragraph number in final order
column: filer\_name, type: character varying(250), description: name of cited filer
column: submission\_type, type: character varying(12), description: type of submission as indicated in final order
column: page\_numbers, type: text[], description: cited page numbers
column: cite\_id, type: integer, description: unique id for citation
column: filer\_id, type: character varying(250), description: id for cited filer
* docs\_cited: attachments associated with cited submissions
column: cite\_id, type: numeric, description: unique id for citation
column: submission\_id, type: character varying(20), description: unique id for submission
column: document\_id, type: character varying(64), description: unique id for attachment
* near\_duplicates: lookup table for comment near-duplicates
column: target\_document\_id, type: unique id for target document, description:
column: duplicate\_document\_id, type: unique id for duplicate of target document, description:
* exact\_duplicates: lookup table for comment exact duplicates
column: target\_document\_id, type: character varying(100), description: unique id for target document
column: duplicate\_document\_id, type: character varying(100), description: unique id for duplicate of target document
* in\_person\_exparte: submissions associated with ex parte meeting
column: submission\_id, type: character varying(20), description: unique id for submission
* interest\_groups: submissions associated with interest groups
column: submission\_id, type: character varying(20), description: unique id for submission
column: business, type: numeric, description: 1 if business group, 0 otherwise
Dataset Creation
----------------
### Curation Rationale
The data were curated to perform information retrieval and summarization tasks as documented in URL
### Source Data
#### Initial Data Collection and Normalization
The data for this study come from the FCC's Electronic Comment Filing System (ECFS) system, accessed between January and February of 2019.
I converted the API responses into a normalized, relational database containing information on 23,951,967 submissions.
23,938,686 "express" submissions contained a single plain text comment submitted directly through the comment form.
13,821 "standard" submissions contained one or more comment documents submitted as attachments in various file formats.
While the FCC permitted any file format for attachments, I only consider documents attached in pdf, plain text, rich text,
and Microsoft Word file formats, and I drop submitted documents that were simply copies of the FCC’s official documents (e.g., the NPRM itself).
Using standard OCR software, I attempted to convert all attachments into plain text and saved them as machine-readable pdfs.
#### Who are the source language producers?
All submitters of public comments during the public comment period (but see note on fake comments in considerations).
### Annotations
#### Annotation process
* Citations: I consider citations from the main text of the FCC's final rule. I did not include citations to
supporting documents not available through ECFS (e.g., court decisions), nor did I include citations
to submissions from prior FCC proceedings. The direct citations to filed submissions are included
in a series of 1,186 footnotes. The FCC’s citation format typically followed a relatively standard
pattern: the name of the filer (e.g., Verizon), a description of the document (e.g., Comment), and
at times a page number. I extracted citations from the text using regular expressions. Based on a
random sample of paragraphs from the final order, the regular expressions identified 98% of eligible citations,
while successfully excluding all non-citation text. In total, this produced 1,886 unique citations.
I then identified which of the comments were cited. First, I identified all documents from the cited filer
that had enough pages to contain the page number cited (if provided), and, where applicable, whose filename
contained the moniker from the FCC’s citation (e.g., "Reply"). The majority of citations matched to only one
possible comment submitted, and I identified the re- maining cited comments through manual review of the citations.
In this way, I was able to tag documents associated with all but three citations. When the same cited document was
submitted under multiple separate submissions, I tagged all versions of the document as being cited.
* Commenter type: Comments are labeled as mass comments if 10 or more duplicate or near-duplicate copies were
submitted by individual commenters. Near-duplicates were defined as comments with non-zero identical information scores.
To identify the type of commenter for non-mass comments, I take advantage of the fact that the vast majority of organized
groups preferred standard submissions over express submissions. Any non-mass comment submitted as an express comment was
coded as coming from an individual. To distinguish between individuals and organizations that used standard submissions,
I use a first name and surname database from the names dataset Python package to characterize filer names as belonging to
individuals or organizations. I also use the domain of the submitter’s email address to re-categorize comments as coming
from organizations if they were submitted on behalf of organizations by an individual. Government officials were identified by
their .gov email addresses. I manually review this procedure for mischaracterizations. After obtaining a list of organization
names, I manually code each one as belonging to a business group or a non-business group. Government officials writing in
their official capacity were categorized as a non-business group.
* In-person meetings: To identify which commenters held in-person meetings with the agency, I collect all comments labeled
as an ex-parte submission in the EFCS. I manually review these submissions for mention of an in-person meeting. I label
a commenter as having held an in-person meeting if they submitted at least one ex-parte document that mentioned an in-person meeting.
#### Who are the annotators?
Annotations are a combination of automated and manual review done by the author.
### Personal and Sensitive Information
This dataset may contain personal and sensitive information, as there were no restrictions on what commenters could submit to
the agency. This dataset also contains numerous examples of profanity and spam. These comments represent what the FCC decided was
appropriate to share publicly on their own website.
Considerations for Using the Data
---------------------------------
### Discussion of Biases
This proceeding was famous for the large number of "fake" comments (comments impersonating ordinary citizens) submitted to the
agency (see this report by the NY AG for more information).
As such, this comment corpus contains a mix of computer-generated and natural language, and there is currently no way to reliably separate
mass comments submitted with the approval of the commenter and those submitted on behalf of the commenter without their knowledge.
Additional Information
----------------------
### Licensing Information
CreativeCommons Attribution-NonCommercial-ShareAlike 4.0 International.
| [
"### Dataset Summary\n\n\nOnline comment floods during public consultations have posed unique governance challenges for\nregulatory bodies seeking relevant information on proposed regulations.\nHow should regulatory bodies separate spam and fake comments from genuine submissions by the public,\nespecially when fake comments are designed to imitate ordinary citizens? How can regulatory bodies\nachieve both breadth and depth in their citations to the comment corpus? What is the best way to\nselect comments that represent the average submission and comments that supply highly specialized\ninformation?\n\n\n'fcc-comments' is an annotated version of the comment corpus from the Federal Communications Commission's\n(FCC) 2017 \"Restoring Internet Freedom\" proceeding. The source data were downloaded directly from the FCC's Electronic\nComment Filing System (ECFS) between January and February of 2019 and include raw comment text and metadata on\ncomment submissions. The comment data were processed to be in a consistent format\n(machine-readable pdf or plain text), and annotated with three types of information: whether the comment was cited in the\nagency's final order, the type of commenter (individual, interest group, business group), and whether the comment was associated with an in-person meeting.\n\n\nThe release also includes query-term and document-term matrices to facilitate keyword searches on the comment corpus.\nAn example of how these can be used with the bm25 algorithm can be found\nhere.\n\n\nDataset Structure\n-----------------\n\n\nFCC relational database (URL): The core components of the database include a table for submission metadata,\na table for attachment metadata, a table for filer metadata, and a table that contains comment text if submitted in express format.\nIn addition to these core tables, there are several derived tables specific to the analyses in the paper,\nincluding which submissions and attachments were cited in the final order, which submissions were associated with in-person meetings,\nand which submissions were associated with interest groups. Full documentation of the tables can be found in fcc\\_database.md.\n\n\nAttachments (URL): Attachments to submissions that could be converted to text via OCR and saved in machine-readable pdf format.\nThe filenames are formatted as [submission\\_id]\\_[document\\_id].pdf, where submission\\_id and document\\_id are keys in the relational database.\n\n\nSearch datasets (URL): Objects to facilitate prototyping of search algorithms on the comment corpus. Contains the following elements:",
"### Data Fields\n\n\nThe following tables are available in URL:\n\n\n* comments: plain text comments associated with submissions\n\n\ncolumn: comment\\_id, type: character varying(64), description: unique id for plain text comment\ncolumn: comment\\_text, type: text, description: raw text of plain text comment\ncolumn: row\\_id, type: integer, description: row sequence for plain text comments\n\n\n* submissions: metadata for submissions\n\n\ncolumn: submission\\_id, type: character varying(20), description: unique id for submission\ncolumn: submission\\_type, type: character varying(100), description: type of submission (e.g., comment, reply, statement)\ncolumn: express\\_comment, type: numeric, description: 1 if express comment\ncolumn: date\\_received, type: date, description: date submission was received\ncolumn: contact\\_email, type: character varying(255), description: submitter email address\ncolumn: city, type: character varying(255), description: submitter city\ncolumn: address\\_line\\_1, type: character varying(255), description: submitter address line 1\ncolumn: address\\_line\\_2, type: character varying(255), description: submitter address line 2\ncolumn: state, type: character varying(255), description: submitter state\ncolumn: zip\\_code, type: character varying(50), description: submitter zip\ncolumn: comment\\_id, type: character varying(64), description: unique id for plain text comment\n\n\n* filers: names of filers associated with submissions\n\n\ncolumn: submission\\_id, type: character varying(20), description: unique id for submission\ncolumn: filer\\_name, type: character varying(250), description: name of filer associated with submission\n\n\n* documents: attachments associated with submissions\n\n\ncolumn: submission\\_id, type: character varying(20), description: unique id for submission\ncolumn: document\\_name, type: text, description: filename of attachment\ncolumn: download\\_status, type: numeric, description: status of attachment download\ncolumn: document\\_id, type: character varying(64), description: unique id for attachment\ncolumn: file\\_extension, type: character varying(4), description: file extension for attachment\n\n\n* filers\\_cited: citations from final order\n\n\ncolumn: point, type: numeric, description: paragraph number in final order\ncolumn: filer\\_name, type: character varying(250), description: name of cited filer\ncolumn: submission\\_type, type: character varying(12), description: type of submission as indicated in final order\ncolumn: page\\_numbers, type: text[], description: cited page numbers\ncolumn: cite\\_id, type: integer, description: unique id for citation\ncolumn: filer\\_id, type: character varying(250), description: id for cited filer\n\n\n* docs\\_cited: attachments associated with cited submissions\n\n\ncolumn: cite\\_id, type: numeric, description: unique id for citation\ncolumn: submission\\_id, type: character varying(20), description: unique id for submission\ncolumn: document\\_id, type: character varying(64), description: unique id for attachment\n\n\n* near\\_duplicates: lookup table for comment near-duplicates\n\n\ncolumn: target\\_document\\_id, type: unique id for target document, description: \ncolumn: duplicate\\_document\\_id, type: unique id for duplicate of target document, description: \n\n\n* exact\\_duplicates: lookup table for comment exact duplicates\n\n\ncolumn: target\\_document\\_id, type: character varying(100), description: unique id for target document\ncolumn: duplicate\\_document\\_id, type: character varying(100), description: unique id for duplicate of target document\n\n\n* in\\_person\\_exparte: submissions associated with ex parte meeting\n\n\ncolumn: submission\\_id, type: character varying(20), description: unique id for submission\n\n\n* interest\\_groups: submissions associated with interest groups\n\n\ncolumn: submission\\_id, type: character varying(20), description: unique id for submission\ncolumn: business, type: numeric, description: 1 if business group, 0 otherwise\n\n\nDataset Creation\n----------------",
"### Curation Rationale\n\n\nThe data were curated to perform information retrieval and summarization tasks as documented in URL",
"### Source Data",
"#### Initial Data Collection and Normalization\n\n\nThe data for this study come from the FCC's Electronic Comment Filing System (ECFS) system, accessed between January and February of 2019.\nI converted the API responses into a normalized, relational database containing information on 23,951,967 submissions.\n23,938,686 \"express\" submissions contained a single plain text comment submitted directly through the comment form.\n13,821 \"standard\" submissions contained one or more comment documents submitted as attachments in various file formats.\nWhile the FCC permitted any file format for attachments, I only consider documents attached in pdf, plain text, rich text,\nand Microsoft Word file formats, and I drop submitted documents that were simply copies of the FCC’s official documents (e.g., the NPRM itself).\nUsing standard OCR software, I attempted to convert all attachments into plain text and saved them as machine-readable pdfs.",
"#### Who are the source language producers?\n\n\nAll submitters of public comments during the public comment period (but see note on fake comments in considerations).",
"### Annotations",
"#### Annotation process\n\n\n* Citations: I consider citations from the main text of the FCC's final rule. I did not include citations to\nsupporting documents not available through ECFS (e.g., court decisions), nor did I include citations\nto submissions from prior FCC proceedings. The direct citations to filed submissions are included\nin a series of 1,186 footnotes. The FCC’s citation format typically followed a relatively standard\npattern: the name of the filer (e.g., Verizon), a description of the document (e.g., Comment), and\nat times a page number. I extracted citations from the text using regular expressions. Based on a\nrandom sample of paragraphs from the final order, the regular expressions identified 98% of eligible citations,\nwhile successfully excluding all non-citation text. In total, this produced 1,886 unique citations.\nI then identified which of the comments were cited. First, I identified all documents from the cited filer\nthat had enough pages to contain the page number cited (if provided), and, where applicable, whose filename\ncontained the moniker from the FCC’s citation (e.g., \"Reply\"). The majority of citations matched to only one\npossible comment submitted, and I identified the re- maining cited comments through manual review of the citations.\nIn this way, I was able to tag documents associated with all but three citations. When the same cited document was\nsubmitted under multiple separate submissions, I tagged all versions of the document as being cited.\n* Commenter type: Comments are labeled as mass comments if 10 or more duplicate or near-duplicate copies were\nsubmitted by individual commenters. Near-duplicates were defined as comments with non-zero identical information scores.\nTo identify the type of commenter for non-mass comments, I take advantage of the fact that the vast majority of organized\ngroups preferred standard submissions over express submissions. Any non-mass comment submitted as an express comment was\ncoded as coming from an individual. To distinguish between individuals and organizations that used standard submissions,\nI use a first name and surname database from the names dataset Python package to characterize filer names as belonging to\nindividuals or organizations. I also use the domain of the submitter’s email address to re-categorize comments as coming\nfrom organizations if they were submitted on behalf of organizations by an individual. Government officials were identified by\ntheir .gov email addresses. I manually review this procedure for mischaracterizations. After obtaining a list of organization\nnames, I manually code each one as belonging to a business group or a non-business group. Government officials writing in\ntheir official capacity were categorized as a non-business group.\n* In-person meetings: To identify which commenters held in-person meetings with the agency, I collect all comments labeled\nas an ex-parte submission in the EFCS. I manually review these submissions for mention of an in-person meeting. I label\na commenter as having held an in-person meeting if they submitted at least one ex-parte document that mentioned an in-person meeting.",
"#### Who are the annotators?\n\n\nAnnotations are a combination of automated and manual review done by the author.",
"### Personal and Sensitive Information\n\n\nThis dataset may contain personal and sensitive information, as there were no restrictions on what commenters could submit to\nthe agency. This dataset also contains numerous examples of profanity and spam. These comments represent what the FCC decided was\nappropriate to share publicly on their own website.\n\n\nConsiderations for Using the Data\n---------------------------------",
"### Discussion of Biases\n\n\nThis proceeding was famous for the large number of \"fake\" comments (comments impersonating ordinary citizens) submitted to the\nagency (see this report by the NY AG for more information).\nAs such, this comment corpus contains a mix of computer-generated and natural language, and there is currently no way to reliably separate\nmass comments submitted with the approval of the commenter and those submitted on behalf of the commenter without their knowledge.\n\n\nAdditional Information\n----------------------",
"### Licensing Information\n\n\nCreativeCommons Attribution-NonCommercial-ShareAlike 4.0 International."
] | [
"TAGS\n#task_categories-text-retrieval #task_ids-document-retrieval #annotations_creators-expert-generated #language_creators-found #multilinguality-monolingual #size_categories-10M<n<100M #source_datasets-original #language-English #license-cc-by-nc-sa-4.0 #notice and comment #regulation #government #region-us \n",
"### Dataset Summary\n\n\nOnline comment floods during public consultations have posed unique governance challenges for\nregulatory bodies seeking relevant information on proposed regulations.\nHow should regulatory bodies separate spam and fake comments from genuine submissions by the public,\nespecially when fake comments are designed to imitate ordinary citizens? How can regulatory bodies\nachieve both breadth and depth in their citations to the comment corpus? What is the best way to\nselect comments that represent the average submission and comments that supply highly specialized\ninformation?\n\n\n'fcc-comments' is an annotated version of the comment corpus from the Federal Communications Commission's\n(FCC) 2017 \"Restoring Internet Freedom\" proceeding. The source data were downloaded directly from the FCC's Electronic\nComment Filing System (ECFS) between January and February of 2019 and include raw comment text and metadata on\ncomment submissions. The comment data were processed to be in a consistent format\n(machine-readable pdf or plain text), and annotated with three types of information: whether the comment was cited in the\nagency's final order, the type of commenter (individual, interest group, business group), and whether the comment was associated with an in-person meeting.\n\n\nThe release also includes query-term and document-term matrices to facilitate keyword searches on the comment corpus.\nAn example of how these can be used with the bm25 algorithm can be found\nhere.\n\n\nDataset Structure\n-----------------\n\n\nFCC relational database (URL): The core components of the database include a table for submission metadata,\na table for attachment metadata, a table for filer metadata, and a table that contains comment text if submitted in express format.\nIn addition to these core tables, there are several derived tables specific to the analyses in the paper,\nincluding which submissions and attachments were cited in the final order, which submissions were associated with in-person meetings,\nand which submissions were associated with interest groups. Full documentation of the tables can be found in fcc\\_database.md.\n\n\nAttachments (URL): Attachments to submissions that could be converted to text via OCR and saved in machine-readable pdf format.\nThe filenames are formatted as [submission\\_id]\\_[document\\_id].pdf, where submission\\_id and document\\_id are keys in the relational database.\n\n\nSearch datasets (URL): Objects to facilitate prototyping of search algorithms on the comment corpus. Contains the following elements:",
"### Data Fields\n\n\nThe following tables are available in URL:\n\n\n* comments: plain text comments associated with submissions\n\n\ncolumn: comment\\_id, type: character varying(64), description: unique id for plain text comment\ncolumn: comment\\_text, type: text, description: raw text of plain text comment\ncolumn: row\\_id, type: integer, description: row sequence for plain text comments\n\n\n* submissions: metadata for submissions\n\n\ncolumn: submission\\_id, type: character varying(20), description: unique id for submission\ncolumn: submission\\_type, type: character varying(100), description: type of submission (e.g., comment, reply, statement)\ncolumn: express\\_comment, type: numeric, description: 1 if express comment\ncolumn: date\\_received, type: date, description: date submission was received\ncolumn: contact\\_email, type: character varying(255), description: submitter email address\ncolumn: city, type: character varying(255), description: submitter city\ncolumn: address\\_line\\_1, type: character varying(255), description: submitter address line 1\ncolumn: address\\_line\\_2, type: character varying(255), description: submitter address line 2\ncolumn: state, type: character varying(255), description: submitter state\ncolumn: zip\\_code, type: character varying(50), description: submitter zip\ncolumn: comment\\_id, type: character varying(64), description: unique id for plain text comment\n\n\n* filers: names of filers associated with submissions\n\n\ncolumn: submission\\_id, type: character varying(20), description: unique id for submission\ncolumn: filer\\_name, type: character varying(250), description: name of filer associated with submission\n\n\n* documents: attachments associated with submissions\n\n\ncolumn: submission\\_id, type: character varying(20), description: unique id for submission\ncolumn: document\\_name, type: text, description: filename of attachment\ncolumn: download\\_status, type: numeric, description: status of attachment download\ncolumn: document\\_id, type: character varying(64), description: unique id for attachment\ncolumn: file\\_extension, type: character varying(4), description: file extension for attachment\n\n\n* filers\\_cited: citations from final order\n\n\ncolumn: point, type: numeric, description: paragraph number in final order\ncolumn: filer\\_name, type: character varying(250), description: name of cited filer\ncolumn: submission\\_type, type: character varying(12), description: type of submission as indicated in final order\ncolumn: page\\_numbers, type: text[], description: cited page numbers\ncolumn: cite\\_id, type: integer, description: unique id for citation\ncolumn: filer\\_id, type: character varying(250), description: id for cited filer\n\n\n* docs\\_cited: attachments associated with cited submissions\n\n\ncolumn: cite\\_id, type: numeric, description: unique id for citation\ncolumn: submission\\_id, type: character varying(20), description: unique id for submission\ncolumn: document\\_id, type: character varying(64), description: unique id for attachment\n\n\n* near\\_duplicates: lookup table for comment near-duplicates\n\n\ncolumn: target\\_document\\_id, type: unique id for target document, description: \ncolumn: duplicate\\_document\\_id, type: unique id for duplicate of target document, description: \n\n\n* exact\\_duplicates: lookup table for comment exact duplicates\n\n\ncolumn: target\\_document\\_id, type: character varying(100), description: unique id for target document\ncolumn: duplicate\\_document\\_id, type: character varying(100), description: unique id for duplicate of target document\n\n\n* in\\_person\\_exparte: submissions associated with ex parte meeting\n\n\ncolumn: submission\\_id, type: character varying(20), description: unique id for submission\n\n\n* interest\\_groups: submissions associated with interest groups\n\n\ncolumn: submission\\_id, type: character varying(20), description: unique id for submission\ncolumn: business, type: numeric, description: 1 if business group, 0 otherwise\n\n\nDataset Creation\n----------------",
"### Curation Rationale\n\n\nThe data were curated to perform information retrieval and summarization tasks as documented in URL",
"### Source Data",
"#### Initial Data Collection and Normalization\n\n\nThe data for this study come from the FCC's Electronic Comment Filing System (ECFS) system, accessed between January and February of 2019.\nI converted the API responses into a normalized, relational database containing information on 23,951,967 submissions.\n23,938,686 \"express\" submissions contained a single plain text comment submitted directly through the comment form.\n13,821 \"standard\" submissions contained one or more comment documents submitted as attachments in various file formats.\nWhile the FCC permitted any file format for attachments, I only consider documents attached in pdf, plain text, rich text,\nand Microsoft Word file formats, and I drop submitted documents that were simply copies of the FCC’s official documents (e.g., the NPRM itself).\nUsing standard OCR software, I attempted to convert all attachments into plain text and saved them as machine-readable pdfs.",
"#### Who are the source language producers?\n\n\nAll submitters of public comments during the public comment period (but see note on fake comments in considerations).",
"### Annotations",
"#### Annotation process\n\n\n* Citations: I consider citations from the main text of the FCC's final rule. I did not include citations to\nsupporting documents not available through ECFS (e.g., court decisions), nor did I include citations\nto submissions from prior FCC proceedings. The direct citations to filed submissions are included\nin a series of 1,186 footnotes. The FCC’s citation format typically followed a relatively standard\npattern: the name of the filer (e.g., Verizon), a description of the document (e.g., Comment), and\nat times a page number. I extracted citations from the text using regular expressions. Based on a\nrandom sample of paragraphs from the final order, the regular expressions identified 98% of eligible citations,\nwhile successfully excluding all non-citation text. In total, this produced 1,886 unique citations.\nI then identified which of the comments were cited. First, I identified all documents from the cited filer\nthat had enough pages to contain the page number cited (if provided), and, where applicable, whose filename\ncontained the moniker from the FCC’s citation (e.g., \"Reply\"). The majority of citations matched to only one\npossible comment submitted, and I identified the re- maining cited comments through manual review of the citations.\nIn this way, I was able to tag documents associated with all but three citations. When the same cited document was\nsubmitted under multiple separate submissions, I tagged all versions of the document as being cited.\n* Commenter type: Comments are labeled as mass comments if 10 or more duplicate or near-duplicate copies were\nsubmitted by individual commenters. Near-duplicates were defined as comments with non-zero identical information scores.\nTo identify the type of commenter for non-mass comments, I take advantage of the fact that the vast majority of organized\ngroups preferred standard submissions over express submissions. Any non-mass comment submitted as an express comment was\ncoded as coming from an individual. To distinguish between individuals and organizations that used standard submissions,\nI use a first name and surname database from the names dataset Python package to characterize filer names as belonging to\nindividuals or organizations. I also use the domain of the submitter’s email address to re-categorize comments as coming\nfrom organizations if they were submitted on behalf of organizations by an individual. Government officials were identified by\ntheir .gov email addresses. I manually review this procedure for mischaracterizations. After obtaining a list of organization\nnames, I manually code each one as belonging to a business group or a non-business group. Government officials writing in\ntheir official capacity were categorized as a non-business group.\n* In-person meetings: To identify which commenters held in-person meetings with the agency, I collect all comments labeled\nas an ex-parte submission in the EFCS. I manually review these submissions for mention of an in-person meeting. I label\na commenter as having held an in-person meeting if they submitted at least one ex-parte document that mentioned an in-person meeting.",
"#### Who are the annotators?\n\n\nAnnotations are a combination of automated and manual review done by the author.",
"### Personal and Sensitive Information\n\n\nThis dataset may contain personal and sensitive information, as there were no restrictions on what commenters could submit to\nthe agency. This dataset also contains numerous examples of profanity and spam. These comments represent what the FCC decided was\nappropriate to share publicly on their own website.\n\n\nConsiderations for Using the Data\n---------------------------------",
"### Discussion of Biases\n\n\nThis proceeding was famous for the large number of \"fake\" comments (comments impersonating ordinary citizens) submitted to the\nagency (see this report by the NY AG for more information).\nAs such, this comment corpus contains a mix of computer-generated and natural language, and there is currently no way to reliably separate\nmass comments submitted with the approval of the commenter and those submitted on behalf of the commenter without their knowledge.\n\n\nAdditional Information\n----------------------",
"### Licensing Information\n\n\nCreativeCommons Attribution-NonCommercial-ShareAlike 4.0 International."
] |
2b8093c6599dd3999aa44a7a14af75c52df9362d |
# Yellow Module embedding
## Samples
<img alt="Samples" src="https://huggingface.co/datasets/DJSoft/yellow_module/resolve/main/samples.jpg" style="max-height: 80vh"/>
## About
Use this Stable Diffusion embedding to achieve the Project Diva Yellow outfit
## Usage
To use this embedding you have to download the file and put it into the "\stable-diffusion-webui\embeddings" folder
To use it in a prompt add __yellow_module-*__
Add **( :1.0)** around it to modify its weight
## Additional info
In order to improve some details you can use the following words:
**Positive prompt:** blue eyes, white sport shorts, yellow see-through babydoll, unzipped, yellow legwear
**Negative prompt:** white babydoll, skirt, black shorts, yellow shorts
## Included Files
- 15000 steps Usage: **yellow_module-15000**
## License
This embedding is open access and available to all, with a CreativeML OpenRAIL-M license further specifying rights and usage. The CreativeML OpenRAIL License specifies:
1. You can't use the embedding to deliberately produce nor share illegal or harmful outputs or content
2. The authors claims no rights on the outputs you generate, you are free to use them and are accountable for their use which must not go against the provisions set in the license
3. You may re-distribute the weights and use the embedding commercially and/or as a service. If you do, please be aware you have to include the same use restrictions as the ones in the license and share a copy of the CreativeML OpenRAIL-M to all your users (please read the license entirely and carefully) [Please read the full license here](https://huggingface.co/spaces/CompVis/stable-diffusion-license) | DJSoft/yellow_module | [
"license:creativeml-openrail-m",
"region:us"
] | 2022-11-30T17:58:12+00:00 | {"license": "creativeml-openrail-m"} | 2022-11-30T18:11:04+00:00 | [] | [] | TAGS
#license-creativeml-openrail-m #region-us
|
# Yellow Module embedding
## Samples
<img alt="Samples" src="URL style="max-height: 80vh"/>
## About
Use this Stable Diffusion embedding to achieve the Project Diva Yellow outfit
## Usage
To use this embedding you have to download the file and put it into the "\stable-diffusion-webui\embeddings" folder
To use it in a prompt add __yellow_module-*__
Add ( :1.0) around it to modify its weight
## Additional info
In order to improve some details you can use the following words:
Positive prompt: blue eyes, white sport shorts, yellow see-through babydoll, unzipped, yellow legwear
Negative prompt: white babydoll, skirt, black shorts, yellow shorts
## Included Files
- 15000 steps Usage: yellow_module-15000
## License
This embedding is open access and available to all, with a CreativeML OpenRAIL-M license further specifying rights and usage. The CreativeML OpenRAIL License specifies:
1. You can't use the embedding to deliberately produce nor share illegal or harmful outputs or content
2. The authors claims no rights on the outputs you generate, you are free to use them and are accountable for their use which must not go against the provisions set in the license
3. You may re-distribute the weights and use the embedding commercially and/or as a service. If you do, please be aware you have to include the same use restrictions as the ones in the license and share a copy of the CreativeML OpenRAIL-M to all your users (please read the license entirely and carefully) Please read the full license here | [
"# Yellow Module embedding",
"## Samples\n\n<img alt=\"Samples\" src=\"URL style=\"max-height: 80vh\"/>",
"## About\n\nUse this Stable Diffusion embedding to achieve the Project Diva Yellow outfit",
"## Usage\n\nTo use this embedding you have to download the file and put it into the \"\\stable-diffusion-webui\\embeddings\" folder \nTo use it in a prompt add __yellow_module-*__ \n\nAdd ( :1.0) around it to modify its weight",
"## Additional info\n\nIn order to improve some details you can use the following words: \nPositive prompt: blue eyes, white sport shorts, yellow see-through babydoll, unzipped, yellow legwear \nNegative prompt: white babydoll, skirt, black shorts, yellow shorts",
"## Included Files\n- 15000 steps Usage: yellow_module-15000",
"## License\n\nThis embedding is open access and available to all, with a CreativeML OpenRAIL-M license further specifying rights and usage. The CreativeML OpenRAIL License specifies:\n\n1. You can't use the embedding to deliberately produce nor share illegal or harmful outputs or content\n2. The authors claims no rights on the outputs you generate, you are free to use them and are accountable for their use which must not go against the provisions set in the license\n3. You may re-distribute the weights and use the embedding commercially and/or as a service. If you do, please be aware you have to include the same use restrictions as the ones in the license and share a copy of the CreativeML OpenRAIL-M to all your users (please read the license entirely and carefully) Please read the full license here"
] | [
"TAGS\n#license-creativeml-openrail-m #region-us \n",
"# Yellow Module embedding",
"## Samples\n\n<img alt=\"Samples\" src=\"URL style=\"max-height: 80vh\"/>",
"## About\n\nUse this Stable Diffusion embedding to achieve the Project Diva Yellow outfit",
"## Usage\n\nTo use this embedding you have to download the file and put it into the \"\\stable-diffusion-webui\\embeddings\" folder \nTo use it in a prompt add __yellow_module-*__ \n\nAdd ( :1.0) around it to modify its weight",
"## Additional info\n\nIn order to improve some details you can use the following words: \nPositive prompt: blue eyes, white sport shorts, yellow see-through babydoll, unzipped, yellow legwear \nNegative prompt: white babydoll, skirt, black shorts, yellow shorts",
"## Included Files\n- 15000 steps Usage: yellow_module-15000",
"## License\n\nThis embedding is open access and available to all, with a CreativeML OpenRAIL-M license further specifying rights and usage. The CreativeML OpenRAIL License specifies:\n\n1. You can't use the embedding to deliberately produce nor share illegal or harmful outputs or content\n2. The authors claims no rights on the outputs you generate, you are free to use them and are accountable for their use which must not go against the provisions set in the license\n3. You may re-distribute the weights and use the embedding commercially and/or as a service. If you do, please be aware you have to include the same use restrictions as the ones in the license and share a copy of the CreativeML OpenRAIL-M to all your users (please read the license entirely and carefully) Please read the full license here"
] |
c14b14f8b23079d25070d66b7db892b73d819632 | # Dataset Card for "google"
[More Information needed](https://github.com/huggingface/datasets/blob/main/CONTRIBUTING.md#how-to-contribute-to-the-dataset-cards) | steciuk/google | [
"region:us"
] | 2022-11-30T18:33:07+00:00 | {"dataset_info": {"features": [{"name": "text", "dtype": "string"}, {"name": "label", "dtype": "int64"}], "splits": [{"name": "train", "num_bytes": 1640375, "num_examples": 10504}], "download_size": 971872, "dataset_size": 1640375}} | 2022-11-30T18:33:20+00:00 | [] | [] | TAGS
#region-us
| # Dataset Card for "google"
More Information needed | [
"# Dataset Card for \"google\"\n\nMore Information needed"
] | [
"TAGS\n#region-us \n",
"# Dataset Card for \"google\"\n\nMore Information needed"
] |
f45d740c271d390ae25c4ec4b83bb1a1386d6419 | # Dataset Card for "imdb"
[More Information needed](https://github.com/huggingface/datasets/blob/main/CONTRIBUTING.md#how-to-contribute-to-the-dataset-cards) | steciuk/imdb | [
"region:us"
] | 2022-11-30T18:33:22+00:00 | {"dataset_info": {"features": [{"name": "text", "dtype": "string"}, {"name": "label", "dtype": "int64"}], "splits": [{"name": "train", "num_bytes": 52901123, "num_examples": 40000}], "download_size": 34391296, "dataset_size": 52901123}} | 2022-11-30T18:33:38+00:00 | [] | [] | TAGS
#region-us
| # Dataset Card for "imdb"
More Information needed | [
"# Dataset Card for \"imdb\"\n\nMore Information needed"
] | [
"TAGS\n#region-us \n",
"# Dataset Card for \"imdb\"\n\nMore Information needed"
] |
e65b73b9d65fdcd430b4574eca0ef9ba6c346e4e | # Dataset Card for AutoTrain Evaluator
This repository contains model predictions generated by [AutoTrain](https://huggingface.co/autotrain) for the following task and dataset:
* Task: Summarization
* Model: pszemraj/pegasus-x-large-book-summary-C-r2
* Dataset: kmfoda/booksum
* Config: kmfoda--booksum
* Split: test
To run new evaluation jobs, visit Hugging Face's [automatic model evaluator](https://huggingface.co/spaces/autoevaluate/model-evaluator).
## Contributions
Thanks to [@pszemraj](https://huggingface.co/pszemraj) for evaluating this model. | autoevaluate/autoeval-eval-kmfoda__booksum-kmfoda__booksum-66d70e-2296872703 | [
"autotrain",
"evaluation",
"region:us"
] | 2022-11-30T19:05:45+00:00 | {"type": "predictions", "tags": ["autotrain", "evaluation"], "datasets": ["kmfoda/booksum"], "eval_info": {"task": "summarization", "model": "pszemraj/pegasus-x-large-book-summary-C-r2", "metrics": [], "dataset_name": "kmfoda/booksum", "dataset_config": "kmfoda--booksum", "dataset_split": "test", "col_mapping": {"text": "chapter", "target": "summary_text"}}} | 2022-12-01T01:21:36+00:00 | [] | [] | TAGS
#autotrain #evaluation #region-us
| # Dataset Card for AutoTrain Evaluator
This repository contains model predictions generated by AutoTrain for the following task and dataset:
* Task: Summarization
* Model: pszemraj/pegasus-x-large-book-summary-C-r2
* Dataset: kmfoda/booksum
* Config: kmfoda--booksum
* Split: test
To run new evaluation jobs, visit Hugging Face's automatic model evaluator.
## Contributions
Thanks to @pszemraj for evaluating this model. | [
"# Dataset Card for AutoTrain Evaluator\n\nThis repository contains model predictions generated by AutoTrain for the following task and dataset:\n\n* Task: Summarization\n* Model: pszemraj/pegasus-x-large-book-summary-C-r2\n* Dataset: kmfoda/booksum\n* Config: kmfoda--booksum\n* Split: test\n\nTo run new evaluation jobs, visit Hugging Face's automatic model evaluator.",
"## Contributions\n\nThanks to @pszemraj for evaluating this model."
] | [
"TAGS\n#autotrain #evaluation #region-us \n",
"# Dataset Card for AutoTrain Evaluator\n\nThis repository contains model predictions generated by AutoTrain for the following task and dataset:\n\n* Task: Summarization\n* Model: pszemraj/pegasus-x-large-book-summary-C-r2\n* Dataset: kmfoda/booksum\n* Config: kmfoda--booksum\n* Split: test\n\nTo run new evaluation jobs, visit Hugging Face's automatic model evaluator.",
"## Contributions\n\nThanks to @pszemraj for evaluating this model."
] |
045259d09f4357fc85422d0bf6a7f6d0da41a4fa | # Caltech-101 Webdataset (Test set only)
Original paper: [One-shot learning of object categories](https://ieeexplore.ieee.org/document/1597116)
Homepage: https://data.caltech.edu/records/mzrjq-6wc02
Bibtex:
```
@misc{li_andreeto_ranzato_perona_2022,
title={Caltech 101},
DOI={10.22002/D1.20086},
publisher={CaltechDATA},
author={Li, Fei-Fei and Andreeto, Marco and Ranzato, Marc'Aurelio and Perona, Pietro},
year={2022},
month={Apr}
}
``` | djghosh/wds_vtab-caltech101_test | [
"region:us"
] | 2022-11-30T21:35:43+00:00 | {} | 2022-12-12T20:37:10+00:00 | [] | [] | TAGS
#region-us
| # Caltech-101 Webdataset (Test set only)
Original paper: One-shot learning of object categories
Homepage: URL
Bibtex:
| [
"# Caltech-101 Webdataset (Test set only)\n\nOriginal paper: One-shot learning of object categories\n\nHomepage: URL\n\nBibtex:"
] | [
"TAGS\n#region-us \n",
"# Caltech-101 Webdataset (Test set only)\n\nOriginal paper: One-shot learning of object categories\n\nHomepage: URL\n\nBibtex:"
] |
2510fd71df6bdc8a87a62a5bbb342d1e6dc30dcb | # CIFAR-100 Webdataset (Test set only)
Original paper: [Learning Multiple Layers of Features from Tiny Images](https://www.cs.toronto.edu/~kriz/learning-features-2009-TR.pdf)
Homepage: https://www.cs.toronto.edu/~kriz/cifar.html
Bibtex:
```
@TECHREPORT{Krizhevsky09learningmultiple,
author = {Alex Krizhevsky},
title = {Learning multiple layers of features from tiny images},
institution = {},
year = {2009}
}
``` | djghosh/wds_vtab-cifar100_test | [
"region:us"
] | 2022-11-30T21:41:23+00:00 | {} | 2022-12-12T20:25:53+00:00 | [] | [] | TAGS
#region-us
| # CIFAR-100 Webdataset (Test set only)
Original paper: Learning Multiple Layers of Features from Tiny Images
Homepage: URL
Bibtex:
| [
"# CIFAR-100 Webdataset (Test set only)\n\nOriginal paper: Learning Multiple Layers of Features from Tiny Images\n\nHomepage: URL\n\nBibtex:"
] | [
"TAGS\n#region-us \n",
"# CIFAR-100 Webdataset (Test set only)\n\nOriginal paper: Learning Multiple Layers of Features from Tiny Images\n\nHomepage: URL\n\nBibtex:"
] |
196667de6472be9447f917288b05750024f2002b | # CLEVR Count All Webdataset (Test set only)
Original paper: [CLEVR: A Diagnostic Dataset for Compositional Language and Elementary Visual Reasoning](https://arxiv.org/pdf/1612.06890.pdf)
Homepage: https://cs.stanford.edu/people/jcjohns/clevr/
Bibtex:
```
@article{DBLP:journals/corr/JohnsonHMFZG16,
author = {Justin Johnson and
Bharath Hariharan and
Laurens van der Maaten and
Li Fei{-}Fei and
C. Lawrence Zitnick and
Ross B. Girshick},
title = {{CLEVR:} {A} Diagnostic Dataset for Compositional Language and Elementary
Visual Reasoning},
journal = {CoRR},
volume = {abs/1612.06890},
year = {2016},
url = {http://arxiv.org/abs/1612.06890},
eprinttype = {arXiv},
eprint = {1612.06890},
timestamp = {Sat, 19 Oct 2019 16:30:04 +0200},
biburl = {https://dblp.org/rec/journals/corr/JohnsonHMFZG16.bib},
bibsource = {dblp computer science bibliography, https://dblp.org}
}
``` | djghosh/wds_vtab-clevr_count_all_test | [
"arxiv:1612.06890",
"region:us"
] | 2022-11-30T21:41:46+00:00 | {} | 2022-12-12T20:40:49+00:00 | [
"1612.06890"
] | [] | TAGS
#arxiv-1612.06890 #region-us
| # CLEVR Count All Webdataset (Test set only)
Original paper: CLEVR: A Diagnostic Dataset for Compositional Language and Elementary Visual Reasoning
Homepage: URL
Bibtex:
| [
"# CLEVR Count All Webdataset (Test set only)\n\nOriginal paper: CLEVR: A Diagnostic Dataset for Compositional Language and Elementary Visual Reasoning\n\nHomepage: URL\n\nBibtex:"
] | [
"TAGS\n#arxiv-1612.06890 #region-us \n",
"# CLEVR Count All Webdataset (Test set only)\n\nOriginal paper: CLEVR: A Diagnostic Dataset for Compositional Language and Elementary Visual Reasoning\n\nHomepage: URL\n\nBibtex:"
] |
960ed30e5ff2a205c80befe503984946fad59106 | # CLEVR Closest Object Distance Webdataset (Test set only)
Original paper: [CLEVR: A Diagnostic Dataset for Compositional Language and Elementary Visual Reasoning](https://arxiv.org/pdf/1612.06890.pdf)
Homepage: https://cs.stanford.edu/people/jcjohns/clevr/
Bibtex:
```
@article{DBLP:journals/corr/JohnsonHMFZG16,
author = {Justin Johnson and
Bharath Hariharan and
Laurens van der Maaten and
Li Fei{-}Fei and
C. Lawrence Zitnick and
Ross B. Girshick},
title = {{CLEVR:} {A} Diagnostic Dataset for Compositional Language and Elementary
Visual Reasoning},
journal = {CoRR},
volume = {abs/1612.06890},
year = {2016},
url = {http://arxiv.org/abs/1612.06890},
eprinttype = {arXiv},
eprint = {1612.06890},
timestamp = {Sat, 19 Oct 2019 16:30:04 +0200},
biburl = {https://dblp.org/rec/journals/corr/JohnsonHMFZG16.bib},
bibsource = {dblp computer science bibliography, https://dblp.org}
}
``` | djghosh/wds_vtab-clevr_closest_object_distance_test | [
"arxiv:1612.06890",
"region:us"
] | 2022-11-30T21:42:58+00:00 | {} | 2022-12-12T20:40:20+00:00 | [
"1612.06890"
] | [] | TAGS
#arxiv-1612.06890 #region-us
| # CLEVR Closest Object Distance Webdataset (Test set only)
Original paper: CLEVR: A Diagnostic Dataset for Compositional Language and Elementary Visual Reasoning
Homepage: URL
Bibtex:
| [
"# CLEVR Closest Object Distance Webdataset (Test set only)\n\nOriginal paper: CLEVR: A Diagnostic Dataset for Compositional Language and Elementary Visual Reasoning\n\nHomepage: URL\n\nBibtex:"
] | [
"TAGS\n#arxiv-1612.06890 #region-us \n",
"# CLEVR Closest Object Distance Webdataset (Test set only)\n\nOriginal paper: CLEVR: A Diagnostic Dataset for Compositional Language and Elementary Visual Reasoning\n\nHomepage: URL\n\nBibtex:"
] |
154bf10ce86beabe53eff464079fe9d6186f13ac | # Country-211 (Test set only)
Original paper: [Learning Transferable Visual Models From Natural Language Supervision](https://arxiv.org/abs/2103.00020)
Homepage: https://github.com/openai/CLIP/blob/main/data/country211.md
Derived from YFCC100M: https://multimediacommons.wordpress.com/yfcc100m-core-dataset/
Bibtex:
```
@article{DBLP:journals/corr/abs-2103-00020,
author = {Alec Radford and
Jong Wook Kim and
Chris Hallacy and
Aditya Ramesh and
Gabriel Goh and
Sandhini Agarwal and
Girish Sastry and
Amanda Askell and
Pamela Mishkin and
Jack Clark and
Gretchen Krueger and
Ilya Sutskever},
title = {Learning Transferable Visual Models From Natural Language Supervision},
journal = {CoRR},
volume = {abs/2103.00020},
year = {2021},
url = {https://arxiv.org/abs/2103.00020},
eprinttype = {arXiv},
eprint = {2103.00020},
timestamp = {Thu, 04 Mar 2021 17:00:40 +0100},
biburl = {https://dblp.org/rec/journals/corr/abs-2103-00020.bib},
bibsource = {dblp computer science bibliography, https://dblp.org}
}
``` | djghosh/wds_country211_test | [
"arxiv:2103.00020",
"region:us"
] | 2022-11-30T21:44:34+00:00 | {} | 2022-12-12T20:44:50+00:00 | [
"2103.00020"
] | [] | TAGS
#arxiv-2103.00020 #region-us
| # Country-211 (Test set only)
Original paper: Learning Transferable Visual Models From Natural Language Supervision
Homepage: URL
Derived from YFCC100M: URL
Bibtex:
| [
"# Country-211 (Test set only)\n\nOriginal paper: Learning Transferable Visual Models From Natural Language Supervision\n\nHomepage: URL\n\nDerived from YFCC100M: URL\n\nBibtex:"
] | [
"TAGS\n#arxiv-2103.00020 #region-us \n",
"# Country-211 (Test set only)\n\nOriginal paper: Learning Transferable Visual Models From Natural Language Supervision\n\nHomepage: URL\n\nDerived from YFCC100M: URL\n\nBibtex:"
] |
276b0059cb0cc956aaf588225d5197c13924adb3 | # Describable Textures Dataset (Test set only)
Original paper: [Describing Textures in the Wild](http://www.robots.ox.ac.uk/~vgg/publications/2014/Cimpoi14/cimpoi14.pdf)
Homepage: https://www.robots.ox.ac.uk/~vgg/data/dtd/
Bibtex:
```
@InProceedings{cimpoi14describing,
Author = {M. Cimpoi and S. Maji and I. Kokkinos and S. Mohamed and and A. Vedaldi},
Title = {Describing Textures in the Wild},
Booktitle = {Proceedings of the {IEEE} Conf. on Computer Vision and Pattern Recognition ({CVPR})},
Year = {2014}}
``` | djghosh/wds_vtab-dtd_test | [
"region:us"
] | 2022-11-30T21:46:14+00:00 | {} | 2022-12-12T20:46:48+00:00 | [] | [] | TAGS
#region-us
| # Describable Textures Dataset (Test set only)
Original paper: Describing Textures in the Wild
Homepage: URL
Bibtex:
| [
"# Describable Textures Dataset (Test set only)\n\nOriginal paper: Describing Textures in the Wild\n\nHomepage: URL\n\nBibtex:"
] | [
"TAGS\n#region-us \n",
"# Describable Textures Dataset (Test set only)\n\nOriginal paper: Describing Textures in the Wild\n\nHomepage: URL\n\nBibtex:"
] |
d96ddaa13dabad7da66e04c48141560f21cbf7b5 | # DMLab Frames (Test set only)
Original paper: [The Visual Task Adaptation Benchmark](https://arxiv.org/abs/1910.04867)
Homepage: https://github.com/google-research/task_adaptation
Bibtex:
```
@article{zhai2019visual,
title={The Visual Task Adaptation Benchmark},
author={Xiaohua Zhai and Joan Puigcerver and Alexander Kolesnikov and
Pierre Ruyssen and Carlos Riquelme and Mario Lucic and
Josip Djolonga and Andre Susano Pinto and Maxim Neumann and
Alexey Dosovitskiy and Lucas Beyer and Olivier Bachem and
Michael Tschannen and Marcin Michalski and Olivier Bousquet and
Sylvain Gelly and Neil Houlsby},
year={2019},
eprint={1910.04867},
archivePrefix={arXiv},
primaryClass={cs.CV},
url = {https://arxiv.org/abs/1910.04867}
}
``` | djghosh/wds_vtab-dmlab_test | [
"arxiv:1910.04867",
"region:us"
] | 2022-11-30T21:46:50+00:00 | {} | 2022-12-12T20:49:10+00:00 | [
"1910.04867"
] | [] | TAGS
#arxiv-1910.04867 #region-us
| # DMLab Frames (Test set only)
Original paper: The Visual Task Adaptation Benchmark
Homepage: URL
Bibtex:
| [
"# DMLab Frames (Test set only)\n\nOriginal paper: The Visual Task Adaptation Benchmark\n\nHomepage: URL\n\nBibtex:"
] | [
"TAGS\n#arxiv-1910.04867 #region-us \n",
"# DMLab Frames (Test set only)\n\nOriginal paper: The Visual Task Adaptation Benchmark\n\nHomepage: URL\n\nBibtex:"
] |
4d54915cdf934af23a2d4f3b5f895a4fbb7402d3 | # dSprites Orientation (Test set only)
Original paper: [beta-VAE: Learning Basic Visual Concepts with a Constrained Variational Framework](https://openreview.net/forum?id=Sy2fzU9gl)
Homepage: https://github.com/deepmind/dsprites-dataset
Bibtex:
```
@misc{dsprites17,
author = {Loic Matthey and Irina Higgins and Demis Hassabis and Alexander Lerchner},
title = {dSprites: Disentanglement testing Sprites dataset},
howpublished= {https://github.com/deepmind/dsprites-dataset/},
year = "2017",
}
``` | djghosh/wds_vtab-dsprites_label_orientation_test | [
"region:us"
] | 2022-11-30T21:47:55+00:00 | {} | 2022-12-12T20:51:57+00:00 | [] | [] | TAGS
#region-us
| # dSprites Orientation (Test set only)
Original paper: beta-VAE: Learning Basic Visual Concepts with a Constrained Variational Framework
Homepage: URL
Bibtex:
| [
"# dSprites Orientation (Test set only)\n\nOriginal paper: beta-VAE: Learning Basic Visual Concepts with a Constrained Variational Framework\n\nHomepage: URL\n\nBibtex:"
] | [
"TAGS\n#region-us \n",
"# dSprites Orientation (Test set only)\n\nOriginal paper: beta-VAE: Learning Basic Visual Concepts with a Constrained Variational Framework\n\nHomepage: URL\n\nBibtex:"
] |
8ded762a7b18bc4b75985cb39cb5355cecf58d27 | # dSprites X Position (Test set only)
Original paper: [beta-VAE: Learning Basic Visual Concepts with a Constrained Variational Framework](https://openreview.net/forum?id=Sy2fzU9gl)
Homepage: https://github.com/deepmind/dsprites-dataset
Bibtex:
```
@misc{dsprites17,
author = {Loic Matthey and Irina Higgins and Demis Hassabis and Alexander Lerchner},
title = {dSprites: Disentanglement testing Sprites dataset},
howpublished= {https://github.com/deepmind/dsprites-dataset/},
year = "2017",
}
``` | djghosh/wds_vtab-dsprites_label_x_position_test | [
"region:us"
] | 2022-11-30T21:48:20+00:00 | {} | 2022-12-12T20:52:19+00:00 | [] | [] | TAGS
#region-us
| # dSprites X Position (Test set only)
Original paper: beta-VAE: Learning Basic Visual Concepts with a Constrained Variational Framework
Homepage: URL
Bibtex:
| [
"# dSprites X Position (Test set only)\n\nOriginal paper: beta-VAE: Learning Basic Visual Concepts with a Constrained Variational Framework\n\nHomepage: URL\n\nBibtex:"
] | [
"TAGS\n#region-us \n",
"# dSprites X Position (Test set only)\n\nOriginal paper: beta-VAE: Learning Basic Visual Concepts with a Constrained Variational Framework\n\nHomepage: URL\n\nBibtex:"
] |
e1c187043efbc540f37db8b07af5d949920718fa | # dSprites Y Position (Test set only)
Original paper: [beta-VAE: Learning Basic Visual Concepts with a Constrained Variational Framework](https://openreview.net/forum?id=Sy2fzU9gl)
Homepage: https://github.com/deepmind/dsprites-dataset
Bibtex:
```
@misc{dsprites17,
author = {Loic Matthey and Irina Higgins and Demis Hassabis and Alexander Lerchner},
title = {dSprites: Disentanglement testing Sprites dataset},
howpublished= {https://github.com/deepmind/dsprites-dataset/},
year = "2017",
}
``` | djghosh/wds_vtab-dsprites_label_y_position_test | [
"region:us"
] | 2022-11-30T21:48:45+00:00 | {} | 2022-12-12T20:52:35+00:00 | [] | [] | TAGS
#region-us
| # dSprites Y Position (Test set only)
Original paper: beta-VAE: Learning Basic Visual Concepts with a Constrained Variational Framework
Homepage: URL
Bibtex:
| [
"# dSprites Y Position (Test set only)\n\nOriginal paper: beta-VAE: Learning Basic Visual Concepts with a Constrained Variational Framework\n\nHomepage: URL\n\nBibtex:"
] | [
"TAGS\n#region-us \n",
"# dSprites Y Position (Test set only)\n\nOriginal paper: beta-VAE: Learning Basic Visual Concepts with a Constrained Variational Framework\n\nHomepage: URL\n\nBibtex:"
] |
7d107212549825bf1fc46d322d0839e9cc8a3ff1 | # dSprites EuroSAT (Test set only)
Original paper: [EuroSAT: A Novel Dataset and Deep Learning Benchmark for Land Use and Land Cover Classification](https://arxiv.org/abs/1709.00029)
Homepage: https://github.com/phelber/EuroSAT
Bibtex:
```
@article{helber2019eurosat,
title={Eurosat: A novel dataset and deep learning benchmark for land use and land cover classification},
author={Helber, Patrick and Bischke, Benjamin and Dengel, Andreas and Borth, Damian},
journal={IEEE Journal of Selected Topics in Applied Earth Observations and Remote Sensing},
year={2019},
publisher={IEEE}
}
@inproceedings{helber2018introducing,
title={Introducing EuroSAT: A Novel Dataset and Deep Learning Benchmark for Land Use and Land Cover Classification},
author={Helber, Patrick and Bischke, Benjamin and Dengel, Andreas and Borth, Damian},
booktitle={IGARSS 2018-2018 IEEE International Geoscience and Remote Sensing Symposium},
pages={204--207},
year={2018},
organization={IEEE}
}
``` | djghosh/wds_vtab-eurosat_test | [
"arxiv:1709.00029",
"region:us"
] | 2022-11-30T21:49:11+00:00 | {} | 2022-12-12T20:54:32+00:00 | [
"1709.00029"
] | [] | TAGS
#arxiv-1709.00029 #region-us
| # dSprites EuroSAT (Test set only)
Original paper: EuroSAT: A Novel Dataset and Deep Learning Benchmark for Land Use and Land Cover Classification
Homepage: URL
Bibtex:
| [
"# dSprites EuroSAT (Test set only)\n\nOriginal paper: EuroSAT: A Novel Dataset and Deep Learning Benchmark for Land Use and Land Cover Classification\n\nHomepage: URL\n\nBibtex:"
] | [
"TAGS\n#arxiv-1709.00029 #region-us \n",
"# dSprites EuroSAT (Test set only)\n\nOriginal paper: EuroSAT: A Novel Dataset and Deep Learning Benchmark for Land Use and Land Cover Classification\n\nHomepage: URL\n\nBibtex:"
] |
6b5e12a741d27b63718dd08365b866a9a8e5e7a5 | # FGVC-Aircraft (Test set only)
Original paper: [Fine-Grained Visual Classification of Aircraft](https://arxiv.org/abs/1306.5151)
Homepage: https://www.robots.ox.ac.uk/~vgg/data/fgvc-aircraft/
Bibtex:
```
@techreport{maji13fine-grained,
title = {Fine-Grained Visual Classification of Aircraft},
author = {S. Maji and J. Kannala and E. Rahtu
and M. Blaschko and A. Vedaldi},
year = {2013},
archivePrefix = {arXiv},
eprint = {1306.5151},
primaryClass = "cs-cv",
}
``` | djghosh/wds_fgvc_aircraft_test | [
"arxiv:1306.5151",
"region:us"
] | 2022-11-30T21:49:32+00:00 | {} | 2022-12-12T20:57:36+00:00 | [
"1306.5151"
] | [] | TAGS
#arxiv-1306.5151 #region-us
| # FGVC-Aircraft (Test set only)
Original paper: Fine-Grained Visual Classification of Aircraft
Homepage: URL
Bibtex:
| [
"# FGVC-Aircraft (Test set only)\n\nOriginal paper: Fine-Grained Visual Classification of Aircraft\n\nHomepage: URL\n\nBibtex:"
] | [
"TAGS\n#arxiv-1306.5151 #region-us \n",
"# FGVC-Aircraft (Test set only)\n\nOriginal paper: Fine-Grained Visual Classification of Aircraft\n\nHomepage: URL\n\nBibtex:"
] |
056ba0c1c51e384f0d64b54b4bd02bea6e3e3382 | # Food-101 (Test set only)
Original paper: [Food-101 – Mining Discriminative Components with Random Forests](https://data.vision.ee.ethz.ch/cvl/datasets_extra/food-101/static/bossard_eccv14_food-101.pdf)
Homepage: https://data.vision.ee.ethz.ch/cvl/datasets_extra/food-101/
Bibtex:
```
@inproceedings{bossard14,
title = {Food-101 -- Mining Discriminative Components with Random Forests},
author = {Bossard, Lukas and Guillaumin, Matthieu and Van Gool, Luc},
booktitle = {European Conference on Computer Vision},
year = {2014}
}
``` | djghosh/wds_food101_test | [
"region:us"
] | 2022-11-30T21:50:50+00:00 | {} | 2022-12-12T20:57:21+00:00 | [] | [] | TAGS
#region-us
| # Food-101 (Test set only)
Original paper: Food-101 – Mining Discriminative Components with Random Forests
Homepage: URL
Bibtex:
| [
"# Food-101 (Test set only)\n\nOriginal paper: Food-101 – Mining Discriminative Components with Random Forests\n\nHomepage: URL\n\nBibtex:"
] | [
"TAGS\n#region-us \n",
"# Food-101 (Test set only)\n\nOriginal paper: Food-101 – Mining Discriminative Components with Random Forests\n\nHomepage: URL\n\nBibtex:"
] |
7cc5c0dc48e76d0a8a76649234db09145582dc5a | # GTSRB (Test set only)
Original paper: [Man vs. computer: Benchmarking machine learning algorithms for traffic sign recognition](https://doi.org/10.1016/j.neunet.2012.02.016)
Homepage: https://benchmark.ini.rub.de/gtsrb_news.html
Bibtex:
```
@article{Stallkamp2012,
title = "Man vs. computer: Benchmarking machine learning algorithms for traffic sign recognition",
journal = "Neural Networks",
volume = "",
number = "0",
pages = " - ",
year = "2012",
note = "",
issn = "0893-6080",
doi = "10.1016/j.neunet.2012.02.016",
url = "http://www.sciencedirect.com/science/article/pii/S0893608012000457",
author = "J. Stallkamp and M. Schlipsing and J. Salmen and C. Igel",
keywords = "Traffic sign recognition",
keywords = "Machine learning",
keywords = "Convolutional neural networks",
keywords = "Benchmarking"
}
``` | djghosh/wds_gtsrb_test | [
"region:us"
] | 2022-11-30T21:54:33+00:00 | {} | 2022-12-12T20:59:36+00:00 | [] | [] | TAGS
#region-us
| # GTSRB (Test set only)
Original paper: Man vs. computer: Benchmarking machine learning algorithms for traffic sign recognition
Homepage: URL
Bibtex:
| [
"# GTSRB (Test set only)\n\nOriginal paper: Man vs. computer: Benchmarking machine learning algorithms for traffic sign recognition\n\nHomepage: URL\n\nBibtex:"
] | [
"TAGS\n#region-us \n",
"# GTSRB (Test set only)\n\nOriginal paper: Man vs. computer: Benchmarking machine learning algorithms for traffic sign recognition\n\nHomepage: URL\n\nBibtex:"
] |
ab0b1cc7ac87b9688de640151800cc5c1f8a831d | # Dataset Card for "laion-2b-en-very-unsafe"
A version of laion5b dataset(en subset) with strictly `unsafe` images.
Dataset was filtered to retain only examples with `punsafe` present and > 0.9.
However, due to the way nsfw detector was train, there is a significant amount of false postives.
There is, likely, more false positives than real unsafe images. | Rexhaif/laion-2b-en-very-unsafe | [
"region:us"
] | 2022-11-30T22:04:39+00:00 | {"dataset_info": {"features": [{"name": "URL", "dtype": "string"}, {"name": "TEXT", "dtype": "string"}, {"name": "WIDTH", "dtype": "int32"}, {"name": "HEIGHT", "dtype": "int32"}, {"name": "similarity", "dtype": "float64"}, {"name": "hash", "dtype": "int64"}, {"name": "punsafe", "dtype": "float32"}, {"name": "pwatermark", "dtype": "float32"}], "splits": [{"name": "train", "num_bytes": 6799407448, "num_examples": 34607134}], "download_size": 5322013902, "dataset_size": 6799407448}} | 2022-11-30T23:18:49+00:00 | [] | [] | TAGS
#region-us
| # Dataset Card for "laion-2b-en-very-unsafe"
A version of laion5b dataset(en subset) with strictly 'unsafe' images.
Dataset was filtered to retain only examples with 'punsafe' present and > 0.9.
However, due to the way nsfw detector was train, there is a significant amount of false postives.
There is, likely, more false positives than real unsafe images. | [
"# Dataset Card for \"laion-2b-en-very-unsafe\"\n\nA version of laion5b dataset(en subset) with strictly 'unsafe' images.\nDataset was filtered to retain only examples with 'punsafe' present and > 0.9.\nHowever, due to the way nsfw detector was train, there is a significant amount of false postives.\nThere is, likely, more false positives than real unsafe images."
] | [
"TAGS\n#region-us \n",
"# Dataset Card for \"laion-2b-en-very-unsafe\"\n\nA version of laion5b dataset(en subset) with strictly 'unsafe' images.\nDataset was filtered to retain only examples with 'punsafe' present and > 0.9.\nHowever, due to the way nsfw detector was train, there is a significant amount of false postives.\nThere is, likely, more false positives than real unsafe images."
] |
1d7fbb7e1879b05f9db3ab0775ac68435b8ba385 | # ImageNet-Sketch (Test set only)
Original paper: [Learning Robust Global Representations by Penalizing Local Predictive Power](https://arxiv.org/abs/1905.13549)
Homepage: https://github.com/HaohanWang/ImageNet-Sketch
Bibtex:
```
@inproceedings{wang2019learning,
title={Learning Robust Global Representations by Penalizing Local Predictive Power},
author={Wang, Haohan and Ge, Songwei and Lipton, Zachary and Xing, Eric P},
booktitle={Advances in Neural Information Processing Systems},
pages={10506--10518},
year={2019}
}
``` | djghosh/wds_imagenet_sketch_test | [
"arxiv:1905.13549",
"region:us"
] | 2022-11-30T23:23:24+00:00 | {} | 2022-12-12T21:03:25+00:00 | [
"1905.13549"
] | [] | TAGS
#arxiv-1905.13549 #region-us
| # ImageNet-Sketch (Test set only)
Original paper: Learning Robust Global Representations by Penalizing Local Predictive Power
Homepage: URL
Bibtex:
| [
"# ImageNet-Sketch (Test set only)\n\nOriginal paper: Learning Robust Global Representations by Penalizing Local Predictive Power\n\nHomepage: URL\n\nBibtex:"
] | [
"TAGS\n#arxiv-1905.13549 #region-us \n",
"# ImageNet-Sketch (Test set only)\n\nOriginal paper: Learning Robust Global Representations by Penalizing Local Predictive Power\n\nHomepage: URL\n\nBibtex:"
] |
4d1d20ffc1718d1db6a1f47bdf5e4da57d671d81 | # Dataset Card for [best-selling-video-games]
## Table of Contents
- [Table of Contents](#table-of-contents)
- [Dataset Description](#dataset-description)
- [Dataset Summary](#dataset-summary)
- [Supported Tasks and Leaderboards](#supported-tasks-and-leaderboards)
- [Languages](#languages)
- [Dataset Structure](#dataset-structure)
- [Data Instances](#data-instances)
- [Data Fields](#data-fields)
- [Data Splits](#data-splits)
- [Dataset Creation](#dataset-creation)
- [Curation Rationale](#curation-rationale)
- [Source Data](#source-data)
- [Annotations](#annotations)
- [Personal and Sensitive Information](#personal-and-sensitive-information)
- [Considerations for Using the Data](#considerations-for-using-the-data)
- [Social Impact of Dataset](#social-impact-of-dataset)
- [Discussion of Biases](#discussion-of-biases)
- [Other Known Limitations](#other-known-limitations)
- [Additional Information](#additional-information)
- [Dataset Curators](#dataset-curators)
- [Licensing Information](#licensing-information)
- [Citation Information](#citation-information)
- [Contributions](#contributions)
## Dataset Description
- **Homepage:**
- **Repository:**
- **Paper:**
- **Leaderboard:**
- **Point of Contact:**
### Dataset Summary
[More Information Needed]
### Supported Tasks and Leaderboards
[More Information Needed]
### Languages
[More Information Needed]
## Dataset Structure
### Data Instances
[More Information Needed]
### Data Fields
[More Information Needed]
### Data Splits
[More Information Needed]
## Dataset Creation
### Curation Rationale
[More Information Needed]
### Source Data
#### Initial Data Collection and Normalization
[More Information Needed]
#### Who are the source language producers?
[More Information Needed]
### Annotations
#### Annotation process
[More Information Needed]
#### Who are the annotators?
[More Information Needed]
### Personal and Sensitive Information
[More Information Needed]
## Considerations for Using the Data
### Social Impact of Dataset
[More Information Needed]
### Discussion of Biases
[More Information Needed]
### Other Known Limitations
[More Information Needed]
## Additional Information
### Dataset Curators
[More Information Needed]
### Licensing Information
[More Information Needed]
### Citation Information
[More Information Needed]
### Contributions
Thanks to [@arjunpatel7](https://github.com/<github-username>) for adding this dataset. | arjunpatel/best-selling-video-games | [
"region:us"
] | 2022-11-30T23:25:57+00:00 | {} | 2022-12-02T23:46:24+00:00 | [] | [] | TAGS
#region-us
| # Dataset Card for [best-selling-video-games]
## Table of Contents
- Table of Contents
- Dataset Description
- Dataset Summary
- Supported Tasks and Leaderboards
- Languages
- Dataset Structure
- Data Instances
- Data Fields
- Data Splits
- Dataset Creation
- Curation Rationale
- Source Data
- Annotations
- Personal and Sensitive Information
- Considerations for Using the Data
- Social Impact of Dataset
- Discussion of Biases
- Other Known Limitations
- Additional Information
- Dataset Curators
- Licensing Information
- Citation Information
- Contributions
## Dataset Description
- Homepage:
- Repository:
- Paper:
- Leaderboard:
- Point of Contact:
### Dataset Summary
### Supported Tasks and Leaderboards
### Languages
## Dataset Structure
### Data Instances
### Data Fields
### Data Splits
## Dataset Creation
### Curation Rationale
### Source Data
#### Initial Data Collection and Normalization
#### Who are the source language producers?
### Annotations
#### Annotation process
#### Who are the annotators?
### Personal and Sensitive Information
## Considerations for Using the Data
### Social Impact of Dataset
### Discussion of Biases
### Other Known Limitations
## Additional Information
### Dataset Curators
### Licensing Information
### Contributions
Thanks to @arjunpatel7 for adding this dataset. | [
"# Dataset Card for [best-selling-video-games]",
"## Table of Contents\n- Table of Contents\n- Dataset Description\n - Dataset Summary\n - Supported Tasks and Leaderboards\n - Languages\n- Dataset Structure\n - Data Instances\n - Data Fields\n - Data Splits\n- Dataset Creation\n - Curation Rationale\n - Source Data\n - Annotations\n - Personal and Sensitive Information\n- Considerations for Using the Data\n - Social Impact of Dataset\n - Discussion of Biases\n - Other Known Limitations\n- Additional Information\n - Dataset Curators\n - Licensing Information\n - Citation Information\n - Contributions",
"## Dataset Description\n\n- Homepage:\n- Repository:\n- Paper:\n- Leaderboard:\n- Point of Contact:",
"### Dataset Summary",
"### Supported Tasks and Leaderboards",
"### Languages",
"## Dataset Structure",
"### Data Instances",
"### Data Fields",
"### Data Splits",
"## Dataset Creation",
"### Curation Rationale",
"### Source Data",
"#### Initial Data Collection and Normalization",
"#### Who are the source language producers?",
"### Annotations",
"#### Annotation process",
"#### Who are the annotators?",
"### Personal and Sensitive Information",
"## Considerations for Using the Data",
"### Social Impact of Dataset",
"### Discussion of Biases",
"### Other Known Limitations",
"## Additional Information",
"### Dataset Curators",
"### Licensing Information",
"### Contributions\n\nThanks to @arjunpatel7 for adding this dataset."
] | [
"TAGS\n#region-us \n",
"# Dataset Card for [best-selling-video-games]",
"## Table of Contents\n- Table of Contents\n- Dataset Description\n - Dataset Summary\n - Supported Tasks and Leaderboards\n - Languages\n- Dataset Structure\n - Data Instances\n - Data Fields\n - Data Splits\n- Dataset Creation\n - Curation Rationale\n - Source Data\n - Annotations\n - Personal and Sensitive Information\n- Considerations for Using the Data\n - Social Impact of Dataset\n - Discussion of Biases\n - Other Known Limitations\n- Additional Information\n - Dataset Curators\n - Licensing Information\n - Citation Information\n - Contributions",
"## Dataset Description\n\n- Homepage:\n- Repository:\n- Paper:\n- Leaderboard:\n- Point of Contact:",
"### Dataset Summary",
"### Supported Tasks and Leaderboards",
"### Languages",
"## Dataset Structure",
"### Data Instances",
"### Data Fields",
"### Data Splits",
"## Dataset Creation",
"### Curation Rationale",
"### Source Data",
"#### Initial Data Collection and Normalization",
"#### Who are the source language producers?",
"### Annotations",
"#### Annotation process",
"#### Who are the annotators?",
"### Personal and Sensitive Information",
"## Considerations for Using the Data",
"### Social Impact of Dataset",
"### Discussion of Biases",
"### Other Known Limitations",
"## Additional Information",
"### Dataset Curators",
"### Licensing Information",
"### Contributions\n\nThanks to @arjunpatel7 for adding this dataset."
] |
622aa95311079dde7d64d541c411f7c8b796e6b5 | # ImageNetV2 Matched Frequency (Test set only)
Original paper: [Do ImageNet Classifiers Generalize to ImageNet?](https://arxiv.org/abs/1902.10811)
Homepage: https://github.com/modestyachts/ImageNetV2
Bibtex:
```
@article{DBLP:journals/corr/abs-1902-10811,
author = {Benjamin Recht and
Rebecca Roelofs and
Ludwig Schmidt and
Vaishaal Shankar},
title = {Do ImageNet Classifiers Generalize to ImageNet?},
journal = {CoRR},
volume = {abs/1902.10811},
year = {2019},
url = {http://arxiv.org/abs/1902.10811},
eprinttype = {arXiv},
eprint = {1902.10811},
timestamp = {Tue, 21 May 2019 18:03:38 +0200},
biburl = {https://dblp.org/rec/journals/corr/abs-1902-10811.bib},
bibsource = {dblp computer science bibliography, https://dblp.org}
}
``` | djghosh/wds_imagenetv2_test | [
"arxiv:1902.10811",
"region:us"
] | 2022-11-30T23:26:19+00:00 | {} | 2022-12-12T21:06:43+00:00 | [
"1902.10811"
] | [] | TAGS
#arxiv-1902.10811 #region-us
| # ImageNetV2 Matched Frequency (Test set only)
Original paper: Do ImageNet Classifiers Generalize to ImageNet?
Homepage: URL
Bibtex:
| [
"# ImageNetV2 Matched Frequency (Test set only)\n\nOriginal paper: Do ImageNet Classifiers Generalize to ImageNet?\n\nHomepage: URL\n\nBibtex:"
] | [
"TAGS\n#arxiv-1902.10811 #region-us \n",
"# ImageNetV2 Matched Frequency (Test set only)\n\nOriginal paper: Do ImageNet Classifiers Generalize to ImageNet?\n\nHomepage: URL\n\nBibtex:"
] |
370e992788929ebec1a633652d4fb4330b4a621a | # ImageNet-A (Test set only)
Original paper: [Natural Adversarial Examples](https://arxiv.org/abs/1907.07174)
Homepage: https://github.com/hendrycks/natural-adv-examples
Bibtex:
```
@article{hendrycks2021nae,
title={Natural Adversarial Examples},
author={Dan Hendrycks and Kevin Zhao and Steven Basart and Jacob Steinhardt and Dawn Song},
journal={CVPR},
year={2021}
}
``` | djghosh/wds_imagenet-a_test | [
"arxiv:1907.07174",
"region:us"
] | 2022-11-30T23:27:38+00:00 | {} | 2022-12-12T21:08:01+00:00 | [
"1907.07174"
] | [] | TAGS
#arxiv-1907.07174 #region-us
| # ImageNet-A (Test set only)
Original paper: Natural Adversarial Examples
Homepage: URL
Bibtex:
| [
"# ImageNet-A (Test set only)\n\nOriginal paper: Natural Adversarial Examples\n\nHomepage: URL\n\nBibtex:"
] | [
"TAGS\n#arxiv-1907.07174 #region-us \n",
"# ImageNet-A (Test set only)\n\nOriginal paper: Natural Adversarial Examples\n\nHomepage: URL\n\nBibtex:"
] |
48413c5561bdd0b4a0df490d46cf8dabee7f6db3 | # ImageNet-O (Test set only)
Original paper: [Natural Adversarial Examples](https://arxiv.org/abs/1907.07174)
Homepage: https://github.com/hendrycks/natural-adv-examples
Bibtex:
```
@article{hendrycks2021nae,
title={Natural Adversarial Examples},
author={Dan Hendrycks and Kevin Zhao and Steven Basart and Jacob Steinhardt and Dawn Song},
journal={CVPR},
year={2021}
}
``` | djghosh/wds_imagenet-o_test | [
"arxiv:1907.07174",
"region:us"
] | 2022-11-30T23:30:09+00:00 | {} | 2022-12-12T21:08:16+00:00 | [
"1907.07174"
] | [] | TAGS
#arxiv-1907.07174 #region-us
| # ImageNet-O (Test set only)
Original paper: Natural Adversarial Examples
Homepage: URL
Bibtex:
| [
"# ImageNet-O (Test set only)\n\nOriginal paper: Natural Adversarial Examples\n\nHomepage: URL\n\nBibtex:"
] | [
"TAGS\n#arxiv-1907.07174 #region-us \n",
"# ImageNet-O (Test set only)\n\nOriginal paper: Natural Adversarial Examples\n\nHomepage: URL\n\nBibtex:"
] |
582a158b4ff1de74de428fc34df09e8cf76fe484 | # ImageNet-R (Test set only)
Original paper: [The Many Faces of Robustness](https://arxiv.org/abs/2006.16241)
Homepage: https://github.com/hendrycks/imagenet-r
Bibtex:
```
@article{hendrycks2021many,
title={The Many Faces of Robustness: A Critical Analysis of Out-of-Distribution Generalization},
author={Dan Hendrycks and Steven Basart and Norman Mu and Saurav Kadavath and Frank Wang and Evan Dorundo and Rahul Desai and Tyler Zhu and Samyak Parajuli and Mike Guo and Dawn Song and Jacob Steinhardt and Justin Gilmer},
journal={ICCV},
year={2021}
}
``` | djghosh/wds_imagenet-r_test | [
"arxiv:2006.16241",
"region:us"
] | 2022-11-30T23:30:37+00:00 | {} | 2022-12-12T21:09:12+00:00 | [
"2006.16241"
] | [] | TAGS
#arxiv-2006.16241 #region-us
| # ImageNet-R (Test set only)
Original paper: The Many Faces of Robustness
Homepage: URL
Bibtex:
| [
"# ImageNet-R (Test set only)\n\nOriginal paper: The Many Faces of Robustness\n\nHomepage: URL\n\nBibtex:"
] | [
"TAGS\n#arxiv-2006.16241 #region-us \n",
"# ImageNet-R (Test set only)\n\nOriginal paper: The Many Faces of Robustness\n\nHomepage: URL\n\nBibtex:"
] |
b7565ccd1eabd787806222a9c75e8a9f56c6e835 | # KITTI Closest Vehicle Distance (Test set only)
Original paper: [Vision meets Robotics: The KITTI Dataset](https://www.cvlibs.net/publications/Geiger2013IJRR.pdf)
Homepage: https://www.cvlibs.net/datasets/kitti/
Bibtex:
```
@ARTICLE{Geiger2013IJRR,
author = {Andreas Geiger and Philip Lenz and Christoph Stiller and Raquel Urtasun},
title = {Vision meets Robotics: The KITTI Dataset},
journal = {International Journal of Robotics Research (IJRR)},
year = {2013}
}
``` | djghosh/wds_vtab-kitti_closest_vehicle_distance_test | [
"region:us"
] | 2022-11-30T23:32:50+00:00 | {} | 2022-12-12T21:11:59+00:00 | [] | [] | TAGS
#region-us
| # KITTI Closest Vehicle Distance (Test set only)
Original paper: Vision meets Robotics: The KITTI Dataset
Homepage: URL
Bibtex:
| [
"# KITTI Closest Vehicle Distance (Test set only)\n\nOriginal paper: Vision meets Robotics: The KITTI Dataset\n\nHomepage: URL\n\nBibtex:"
] | [
"TAGS\n#region-us \n",
"# KITTI Closest Vehicle Distance (Test set only)\n\nOriginal paper: Vision meets Robotics: The KITTI Dataset\n\nHomepage: URL\n\nBibtex:"
] |
668da094a3e9a48be4bca62f14a569a8386ee6cb | # MNIST (Test set only)
Original paper: [Gradient-based learning applied to document recognition](https://ieeexplore.ieee.org/document/726791)
Homepage (broken link): http://yann.lecun.com/exdb/mnist
Bibtex:
```
@ARTICLE{726791,
author={Lecun, Y. and Bottou, L. and Bengio, Y. and Haffner, P.},
journal={Proceedings of the IEEE},
title={Gradient-based learning applied to document recognition},
year={1998},
volume={86},
number={11},
pages={2278-2324},
doi={10.1109/5.726791}
}
``` | djghosh/wds_mnist_test | [
"region:us"
] | 2022-11-30T23:34:14+00:00 | {} | 2022-12-12T22:24:17+00:00 | [] | [] | TAGS
#region-us
| # MNIST (Test set only)
Original paper: Gradient-based learning applied to document recognition
Homepage (broken link): URL
Bibtex:
| [
"# MNIST (Test set only)\n\nOriginal paper: Gradient-based learning applied to document recognition\n\nHomepage (broken link): URL\n\nBibtex:"
] | [
"TAGS\n#region-us \n",
"# MNIST (Test set only)\n\nOriginal paper: Gradient-based learning applied to document recognition\n\nHomepage (broken link): URL\n\nBibtex:"
] |
e433f2df2999e77401a627de654bddbeadf6f8e9 | # Oxford Flowers-102 (Test set only)
Original paper: [Automated flower classification over a large number of classes](https://www.robots.ox.ac.uk/~vgg/publications/2008/Nilsback08/nilsback08.pdf)
Homepage: https://www.robots.ox.ac.uk/~vgg/data/flowers/102/
Bibtex:
```
@InProceedings{Nilsback08,
author = "Maria-Elena Nilsback and Andrew Zisserman",
title = "Automated Flower Classification over a Large Number of Classes",
booktitle = "Indian Conference on Computer Vision, Graphics and Image Processing",
month = "Dec",
year = "2008",
}
``` | djghosh/wds_vtab-flowers_test | [
"region:us"
] | 2022-11-30T23:34:43+00:00 | {} | 2022-12-12T21:20:43+00:00 | [] | [] | TAGS
#region-us
| # Oxford Flowers-102 (Test set only)
Original paper: Automated flower classification over a large number of classes
Homepage: URL
Bibtex:
| [
"# Oxford Flowers-102 (Test set only)\n\nOriginal paper: Automated flower classification over a large number of classes\n\nHomepage: URL\n\nBibtex:"
] | [
"TAGS\n#region-us \n",
"# Oxford Flowers-102 (Test set only)\n\nOriginal paper: Automated flower classification over a large number of classes\n\nHomepage: URL\n\nBibtex:"
] |
8e094aae70d884c4ccbc9fffe51d1903d897e83d | # Oxford-IIIT Pets (Test set only)
Original paper: [Cats and Dogs](https://www.robots.ox.ac.uk/~vgg/publications/2012/parkhi12a/parkhi12a.pdf)
Homepage: https://www.robots.ox.ac.uk/~vgg/data/pets/
Bibtex:
```
@InProceedings{parkhi12a,
author = "Omkar M. Parkhi and Andrea Vedaldi and Andrew Zisserman and C. V. Jawahar",
title = "Cats and Dogs",
booktitle = "IEEE Conference on Computer Vision and Pattern Recognition",
year = "2012",
}
``` | djghosh/wds_vtab-pets_test | [
"region:us"
] | 2022-11-30T23:36:13+00:00 | {} | 2022-12-12T21:22:46+00:00 | [] | [] | TAGS
#region-us
| # Oxford-IIIT Pets (Test set only)
Original paper: Cats and Dogs
Homepage: URL
Bibtex:
| [
"# Oxford-IIIT Pets (Test set only)\n\nOriginal paper: Cats and Dogs\n\nHomepage: URL\n\nBibtex:"
] | [
"TAGS\n#region-us \n",
"# Oxford-IIIT Pets (Test set only)\n\nOriginal paper: Cats and Dogs\n\nHomepage: URL\n\nBibtex:"
] |
c19b3db699f7fab639d70250f74bb70b5002141e | # Pascal VOC2007 (Test set only)
Original paper: [The PASCAL Visual Object Classes Challenge: A Retrospective](http://host.robots.ox.ac.uk/pascal/VOC/pubs/everingham15.pdf)
Homepage: http://host.robots.ox.ac.uk/pascal/VOC/voc2007/
Bibtex:
```
@misc{pascal-voc-2007,
author = "Everingham, M. and Van~Gool, L. and Williams, C. K. I. and Winn, J. and Zisserman, A.",
title = "The {PASCAL} {V}isual {O}bject {C}lasses {C}hallenge 2007 {(VOC2007)} {R}esults",
howpublished = "http://www.pascal-network.org/challenges/VOC/voc2007/workshop/index.html"}
``` | djghosh/wds_voc2007_test | [
"region:us"
] | 2022-11-30T23:38:38+00:00 | {} | 2022-12-12T21:25:32+00:00 | [] | [] | TAGS
#region-us
| # Pascal VOC2007 (Test set only)
Original paper: The PASCAL Visual Object Classes Challenge: A Retrospective
Homepage: URL
Bibtex:
| [
"# Pascal VOC2007 (Test set only)\n\nOriginal paper: The PASCAL Visual Object Classes Challenge: A Retrospective\n\nHomepage: URL\n\nBibtex:"
] | [
"TAGS\n#region-us \n",
"# Pascal VOC2007 (Test set only)\n\nOriginal paper: The PASCAL Visual Object Classes Challenge: A Retrospective\n\nHomepage: URL\n\nBibtex:"
] |
b1bcefc6b04ded211ca4201cb849156d9a98ef1e | # PatchCamelyon (Test set only)
Original paper: [Rotation Equivariant CNNs for Digital Pathology](http://arxiv.org/abs/1806.03962)
Homepage: https://github.com/basveeling/pcam
Bibtex:
```
@ARTICLE{Veeling2018-qh,
title = "Rotation Equivariant {CNNs} for Digital Pathology",
author = "Veeling, Bastiaan S and Linmans, Jasper and Winkens, Jim and
Cohen, Taco and Welling, Max",
month = jun,
year = 2018,
archivePrefix = "arXiv",
primaryClass = "cs.CV",
eprint = "1806.03962"
}
``` | djghosh/wds_vtab-pcam_test | [
"arxiv:1806.03962",
"region:us"
] | 2022-11-30T23:39:37+00:00 | {} | 2022-12-12T21:26:52+00:00 | [
"1806.03962"
] | [] | TAGS
#arxiv-1806.03962 #region-us
| # PatchCamelyon (Test set only)
Original paper: Rotation Equivariant CNNs for Digital Pathology
Homepage: URL
Bibtex:
| [
"# PatchCamelyon (Test set only)\n\nOriginal paper: Rotation Equivariant CNNs for Digital Pathology\n\nHomepage: URL\n\nBibtex:"
] | [
"TAGS\n#arxiv-1806.03962 #region-us \n",
"# PatchCamelyon (Test set only)\n\nOriginal paper: Rotation Equivariant CNNs for Digital Pathology\n\nHomepage: URL\n\nBibtex:"
] |
1c85f91db604f5f4919cd16603c5898b2e088bf8 |
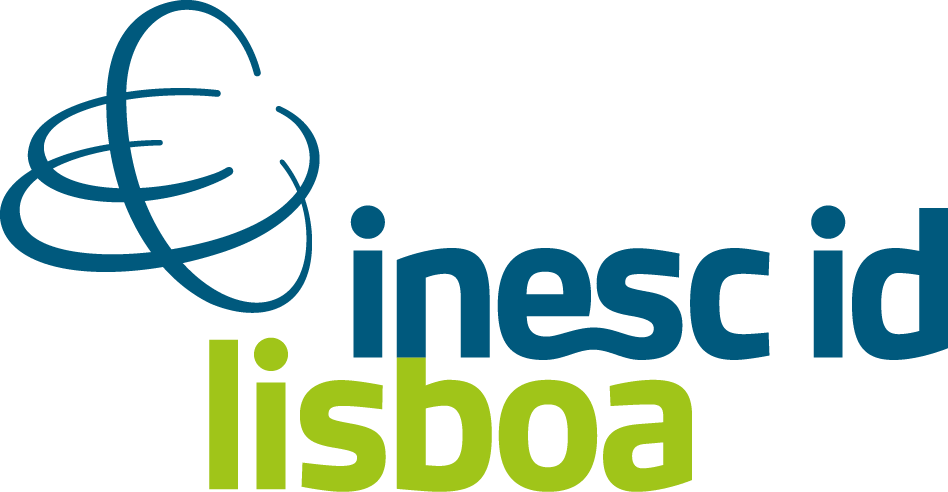
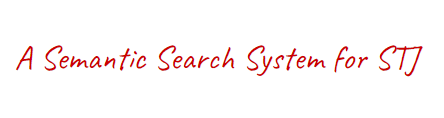
Work developed as part of [Project IRIS](https://www.inesc-id.pt/projects/PR07005/).
Thesis: [A Semantic Search System for Supremo Tribunal de Justiça](https://rufimelo99.github.io/SemanticSearchSystemForSTJ/)
# Portuguese Legal Sentences
Collection of Legal Sentences pairs from the Portuguese Supreme Court of Justice
The goal of this dataset was to be used for Semantic Textual Similarity
- Values from 0-1: random sentences across documents
- Values from 2-4: sentences from the same summary (implying some level of entailment)
- Values from 4-5: sentences pairs generated through OpenAi' text-davinci-003 ("Escreve por outras palavras:\n\Entrada:\n"+originalQuery + "\Saída: \n")
### Contributions
[@rufimelo99](https://github.com/rufimelo99)
If you use this work, please cite:
```bibtex
@inproceedings{MeloSemantic,
author = {Melo, Rui and Santos, Professor Pedro Alexandre and Dias, Professor Jo{\~ a}o},
title = {A {Semantic} {Search} {System} for {Supremo} {Tribunal} de {Justi}{\c c}a},
}
``` | stjiris/IRIS_sts | [
"task_categories:text-classification",
"task_ids:text-scoring",
"task_ids:semantic-similarity-scoring",
"annotations_creators:automated",
"language_creators:found",
"multilinguality:monolingual",
"size_categories:10K>n",
"source_datasets:original",
"language:pt",
"license:mit",
"region:us"
] | 2022-11-30T23:51:04+00:00 | {"annotations_creators": ["automated"], "language_creators": ["found"], "language": ["pt"], "license": ["mit"], "multilinguality": ["monolingual"], "size_categories": ["10K>n"], "source_datasets": ["original"], "task_categories": ["text-classification"], "task_ids": ["text-scoring", "semantic-similarity-scoring"], "pretty_name": "IRIS Legal Dataset"} | 2023-01-08T02:54:33+00:00 | [] | [
"pt"
] | TAGS
#task_categories-text-classification #task_ids-text-scoring #task_ids-semantic-similarity-scoring #annotations_creators-automated #language_creators-found #multilinguality-monolingual #size_categories-10K>n #source_datasets-original #language-Portuguese #license-mit #region-us
|
!INESC-ID
!A Semantic Search System for Supremo Tribunal de Justiça
Work developed as part of Project IRIS.
Thesis: A Semantic Search System for Supremo Tribunal de Justiça
# Portuguese Legal Sentences
Collection of Legal Sentences pairs from the Portuguese Supreme Court of Justice
The goal of this dataset was to be used for Semantic Textual Similarity
- Values from 0-1: random sentences across documents
- Values from 2-4: sentences from the same summary (implying some level of entailment)
- Values from 4-5: sentences pairs generated through OpenAi' text-davinci-003 ("Escreve por outras palavras:\n\Entrada:\n"+originalQuery + "\Saída: \n")
### Contributions
@rufimelo99
If you use this work, please cite:
| [
"# Portuguese Legal Sentences\nCollection of Legal Sentences pairs from the Portuguese Supreme Court of Justice\nThe goal of this dataset was to be used for Semantic Textual Similarity\n\n- Values from 0-1: random sentences across documents\n- Values from 2-4: sentences from the same summary (implying some level of entailment)\n- Values from 4-5: sentences pairs generated through OpenAi' text-davinci-003 (\"Escreve por outras palavras:\\n\\Entrada:\\n\"+originalQuery + \"\\Saída: \\n\")",
"### Contributions\n@rufimelo99\n\n\nIf you use this work, please cite:"
] | [
"TAGS\n#task_categories-text-classification #task_ids-text-scoring #task_ids-semantic-similarity-scoring #annotations_creators-automated #language_creators-found #multilinguality-monolingual #size_categories-10K>n #source_datasets-original #language-Portuguese #license-mit #region-us \n",
"# Portuguese Legal Sentences\nCollection of Legal Sentences pairs from the Portuguese Supreme Court of Justice\nThe goal of this dataset was to be used for Semantic Textual Similarity\n\n- Values from 0-1: random sentences across documents\n- Values from 2-4: sentences from the same summary (implying some level of entailment)\n- Values from 4-5: sentences pairs generated through OpenAi' text-davinci-003 (\"Escreve por outras palavras:\\n\\Entrada:\\n\"+originalQuery + \"\\Saída: \\n\")",
"### Contributions\n@rufimelo99\n\n\nIf you use this work, please cite:"
] |
cf370843eae0cac330f1ed47534c1f29a74da542 |
# laion-translated-to-en-korean-subset
## Dataset Description
- **Homepage:** [laion-5b](https://laion.ai/blog/laion-5b/)
- **Download Size** 1.40 GiB
- **Generated Size** 3.49 GiB
- **Total Size** 4.89 GiB
## About dataset
a subset data of [laion/laion2B-multi-joined-translated-to-en](https://huggingface.co/datasets/laion/laion2B-multi-joined-translated-to-en) and [laion/laion1B-nolang-joined-translated-to-en](https://huggingface.co/datasets/laion/laion1B-nolang-joined-translated-to-en), including only korean
### Lisence
CC-BY-4.0
## Data Structure
### Data Instance
```py
>>> from datasets import load_dataset
>>> dataset = load_dataset("Bingsu/laion-translated-to-en-korean-subset")
>>> dataset
DatasetDict({
train: Dataset({
features: ['hash', 'URL', 'TEXT', 'ENG TEXT', 'WIDTH', 'HEIGHT', 'LANGUAGE', 'similarity', 'pwatermark', 'punsafe', 'AESTHETIC_SCORE'],
num_rows: 12769693
})
})
```
```py
>>> dataset["train"].features
{'hash': Value(dtype='int64', id=None),
'URL': Value(dtype='large_string', id=None),
'TEXT': Value(dtype='large_string', id=None),
'ENG TEXT': Value(dtype='large_string', id=None),
'WIDTH': Value(dtype='int32', id=None),
'HEIGHT': Value(dtype='int32', id=None),
'LANGUAGE': Value(dtype='large_string', id=None),
'similarity': Value(dtype='float32', id=None),
'pwatermark': Value(dtype='float32', id=None),
'punsafe': Value(dtype='float32', id=None),
'AESTHETIC_SCORE': Value(dtype='float32', id=None)}
```
### Data Size
download: 1.40 GiB<br>
generated: 3.49 GiB<br>
total: 4.89 GiB
### Data Field
- 'hash': `int`
- 'URL': `string`
- 'TEXT': `string`
- 'ENG TEXT': `string`, null data are dropped
- 'WIDTH': `int`, null data are filled with 0
- 'HEIGHT': `int`, null data are filled with 0
- 'LICENSE': `string`
- 'LANGUAGE': `string`
- 'similarity': `float32`, CLIP similarity score, null data are filled with 0.0
- 'pwatermark': `float32`, Probability of containing a watermark, null data are filled with 0.0
- 'punsafe': `float32`, Probability of nsfw image, null data are filled with 0.0
- 'AESTHETIC_SCORE': `float32`, null data are filled with 0.0
### Data Splits
| | train |
| --------- | -------- |
| # of data | 12769693 |
### polars
```sh
pip install polars[fsspec]
```
```py
import polars as pl
from huggingface_hub import hf_hub_url
url = hf_hub_url("Bingsu/laion-translated-to-en-korean-subset", filename="train.parquet", repo_type="dataset")
# url = "https://huggingface.co/datasets/Bingsu/laion-translated-to-en-korean-subset/resolve/main/train.parquet"
df = pl.read_parquet(url)
```
pandas broke my colab session. | Bingsu/laion-translated-to-en-korean-subset | [
"task_categories:feature-extraction",
"annotations_creators:crowdsourced",
"language_creators:crowdsourced",
"multilinguality:multilingual",
"size_categories:10M<n<100M",
"language:ko",
"language:en",
"license:cc-by-4.0",
"region:us"
] | 2022-12-01T01:58:31+00:00 | {"annotations_creators": ["crowdsourced"], "language_creators": ["crowdsourced"], "language": ["ko", "en"], "license": ["cc-by-4.0"], "multilinguality": ["multilingual"], "size_categories": ["10M<n<100M"], "task_categories": ["feature-extraction"], "pretty_name": "laion-translated-to-en-korean-subset"} | 2023-02-01T01:15:43+00:00 | [] | [
"ko",
"en"
] | TAGS
#task_categories-feature-extraction #annotations_creators-crowdsourced #language_creators-crowdsourced #multilinguality-multilingual #size_categories-10M<n<100M #language-Korean #language-English #license-cc-by-4.0 #region-us
| laion-translated-to-en-korean-subset
====================================
Dataset Description
-------------------
* Homepage: laion-5b
* Download Size 1.40 GiB
* Generated Size 3.49 GiB
* Total Size 4.89 GiB
About dataset
-------------
a subset data of laion/laion2B-multi-joined-translated-to-en and laion/laion1B-nolang-joined-translated-to-en, including only korean
### Lisence
CC-BY-4.0
Data Structure
--------------
### Data Instance
### Data Size
download: 1.40 GiB
generated: 3.49 GiB
total: 4.89 GiB
### Data Field
* 'hash': 'int'
* 'URL': 'string'
* 'TEXT': 'string'
* 'ENG TEXT': 'string', null data are dropped
* 'WIDTH': 'int', null data are filled with 0
* 'HEIGHT': 'int', null data are filled with 0
* 'LICENSE': 'string'
* 'LANGUAGE': 'string'
* 'similarity': 'float32', CLIP similarity score, null data are filled with 0.0
* 'pwatermark': 'float32', Probability of containing a watermark, null data are filled with 0.0
* 'punsafe': 'float32', Probability of nsfw image, null data are filled with 0.0
* 'AESTHETIC\_SCORE': 'float32', null data are filled with 0.0
### Data Splits
### polars
pandas broke my colab session.
| [
"### Lisence\n\n\nCC-BY-4.0\n\n\nData Structure\n--------------",
"### Data Instance",
"### Data Size\n\n\ndownload: 1.40 GiB \n\ngenerated: 3.49 GiB \n\ntotal: 4.89 GiB",
"### Data Field\n\n\n* 'hash': 'int'\n* 'URL': 'string'\n* 'TEXT': 'string'\n* 'ENG TEXT': 'string', null data are dropped\n* 'WIDTH': 'int', null data are filled with 0\n* 'HEIGHT': 'int', null data are filled with 0\n* 'LICENSE': 'string'\n* 'LANGUAGE': 'string'\n* 'similarity': 'float32', CLIP similarity score, null data are filled with 0.0\n* 'pwatermark': 'float32', Probability of containing a watermark, null data are filled with 0.0\n* 'punsafe': 'float32', Probability of nsfw image, null data are filled with 0.0\n* 'AESTHETIC\\_SCORE': 'float32', null data are filled with 0.0",
"### Data Splits",
"### polars\n\n\npandas broke my colab session."
] | [
"TAGS\n#task_categories-feature-extraction #annotations_creators-crowdsourced #language_creators-crowdsourced #multilinguality-multilingual #size_categories-10M<n<100M #language-Korean #language-English #license-cc-by-4.0 #region-us \n",
"### Lisence\n\n\nCC-BY-4.0\n\n\nData Structure\n--------------",
"### Data Instance",
"### Data Size\n\n\ndownload: 1.40 GiB \n\ngenerated: 3.49 GiB \n\ntotal: 4.89 GiB",
"### Data Field\n\n\n* 'hash': 'int'\n* 'URL': 'string'\n* 'TEXT': 'string'\n* 'ENG TEXT': 'string', null data are dropped\n* 'WIDTH': 'int', null data are filled with 0\n* 'HEIGHT': 'int', null data are filled with 0\n* 'LICENSE': 'string'\n* 'LANGUAGE': 'string'\n* 'similarity': 'float32', CLIP similarity score, null data are filled with 0.0\n* 'pwatermark': 'float32', Probability of containing a watermark, null data are filled with 0.0\n* 'punsafe': 'float32', Probability of nsfw image, null data are filled with 0.0\n* 'AESTHETIC\\_SCORE': 'float32', null data are filled with 0.0",
"### Data Splits",
"### polars\n\n\npandas broke my colab session."
] |
d201c488dc7024623d1ecbcc987b3f132c4c2e12 | # The Hateful Memes Challenge README
The Hateful Memes Challenge is a dataset and benchmark created by Facebook AI to drive and measure progress on multimodal reasoning and understanding. The task focuses on detecting hate speech in multimodal memes.
Please see the paper for further details:
[The Hateful Memes Challenge: Detecting Hate Speech in Multimodal Memes
D. Kiela, H. Firooz, A. Mohan, V. Goswami, A. Singh, P. Ringshia, D. Testuggine](
https://arxiv.org/abs/2005.04790)
For more details, see also the website:
https://hatefulmemeschallenge.com
# Dataset details
The files for this folder are arranged as follows:
img/ - the PNG images
train.jsonl - the training set
dev_seen.jsonl - the "seen" development set
test_seen.jsonl - the "seen" test set
dev_unseen.jsonl - the "unseen" development set
test_unseen.jsonl - the "unseen" test set
The "seen" dataset was presented in the NeurIPS paper; the “unseen” dev and test set were released as a part of the NeurIPS 2020 competition.
The .jsonl format contains one JSON-encoded example per line, each of which has the following fields:
‘text’ - the text occurring in the meme
‘img’ - the path to the image in the img/ directory
‘label’ - the label for the meme (0=not-hateful, 1=hateful), provided for train and dev
The metric to use is AUROC. You may also report accuracy in addition, since this is arguably more interpretable. To compute these metrics, we recommend the roc_auc_score and accuracy_score methods in sklearn.metrics, with default settings.
# Getting started
To get started working on this dataset, there's an easy-to-use "starter kit" available in MMF: https://github.com/facebookresearch/mmf/tree/master/projects/hateful_memes.
# Note on Annotator Accuracy
As is to be expected with a dataset of this size and nature, some of the examples in the training set have been misclassified. We are not claiming that our dataset labels are completely accurate, or even that all annotators would agree on a particular label. Misclassifications, although possible, should be very rare in the dev and seen test set, however, and we will take extra care with the unseen test set.
As a reminder, the annotations collected for this dataset were not collected using Facebook annotators and we did not employ Facebook’s hate speech policy. As such, the dataset labels do not in any way reflect Facebook’s official stance on this matter.
# License
The dataset is licensed under the terms in the `LICENSE.txt` file.
# Image Attribution
If you wish to display example memes in your paper, please provide the following attribution:
*Image is a compilation of assets, including ©Getty Image.*
# Citations
If you wish to cite this work, please use the following BiBTeX:
```
@inproceedings{Kiela:2020hatefulmemes,
author = {Kiela, Douwe and Firooz, Hamed and Mohan, Aravind and Goswami, Vedanuj and Singh, Amanpreet and Ringshia, Pratik and Testuggine, Davide},
booktitle = {Advances in Neural Information Processing Systems},
editor = {H. Larochelle and M. Ranzato and R. Hadsell and M. F. Balcan and H. Lin},
pages = {2611--2624},
publisher = {Curran Associates, Inc.},
title = {The Hateful Memes Challenge: Detecting Hate Speech in Multimodal Memes},
url = {https://proceedings.neurips.cc/paper/2020/file/1b84c4cee2b8b3d823b30e2d604b1878-Paper.pdf},
volume = {33},
year = {2020}
}
```
# Contact
If you have any questions or comments on the dataset, please contact [email protected] or one of the authors.
| neuralcatcher/hateful_memes | [
"arxiv:2005.04790",
"region:us"
] | 2022-12-01T03:49:06+00:00 | {} | 2022-12-01T07:08:59+00:00 | [
"2005.04790"
] | [] | TAGS
#arxiv-2005.04790 #region-us
| # The Hateful Memes Challenge README
The Hateful Memes Challenge is a dataset and benchmark created by Facebook AI to drive and measure progress on multimodal reasoning and understanding. The task focuses on detecting hate speech in multimodal memes.
Please see the paper for further details:
The Hateful Memes Challenge: Detecting Hate Speech in Multimodal Memes
D. Kiela, H. Firooz, A. Mohan, V. Goswami, A. Singh, P. Ringshia, D. Testuggine
For more details, see also the website:
URL
# Dataset details
The files for this folder are arranged as follows:
img/ - the PNG images
URL - the training set
dev_seen.jsonl - the "seen" development set
test_seen.jsonl - the "seen" test set
dev_unseen.jsonl - the "unseen" development set
test_unseen.jsonl - the "unseen" test set
The "seen" dataset was presented in the NeurIPS paper; the “unseen” dev and test set were released as a part of the NeurIPS 2020 competition.
The .jsonl format contains one JSON-encoded example per line, each of which has the following fields:
‘text’ - the text occurring in the meme
‘img’ - the path to the image in the img/ directory
‘label’ - the label for the meme (0=not-hateful, 1=hateful), provided for train and dev
The metric to use is AUROC. You may also report accuracy in addition, since this is arguably more interpretable. To compute these metrics, we recommend the roc_auc_score and accuracy_score methods in sklearn.metrics, with default settings.
# Getting started
To get started working on this dataset, there's an easy-to-use "starter kit" available in MMF: URL
# Note on Annotator Accuracy
As is to be expected with a dataset of this size and nature, some of the examples in the training set have been misclassified. We are not claiming that our dataset labels are completely accurate, or even that all annotators would agree on a particular label. Misclassifications, although possible, should be very rare in the dev and seen test set, however, and we will take extra care with the unseen test set.
As a reminder, the annotations collected for this dataset were not collected using Facebook annotators and we did not employ Facebook’s hate speech policy. As such, the dataset labels do not in any way reflect Facebook’s official stance on this matter.
# License
The dataset is licensed under the terms in the 'URL' file.
# Image Attribution
If you wish to display example memes in your paper, please provide the following attribution:
*Image is a compilation of assets, including ©Getty Image.*
s
If you wish to cite this work, please use the following BiBTeX:
# Contact
If you have any questions or comments on the dataset, please contact hatefulmemeschallenge@URL or one of the authors.
| [
"# The Hateful Memes Challenge README\n\nThe Hateful Memes Challenge is a dataset and benchmark created by Facebook AI to drive and measure progress on multimodal reasoning and understanding. The task focuses on detecting hate speech in multimodal memes.\n\nPlease see the paper for further details:\n\nThe Hateful Memes Challenge: Detecting Hate Speech in Multimodal Memes\nD. Kiela, H. Firooz, A. Mohan, V. Goswami, A. Singh, P. Ringshia, D. Testuggine\n\nFor more details, see also the website:\n\nURL",
"# Dataset details\n\nThe files for this folder are arranged as follows:\n\nimg/ - the PNG images\nURL - the training set\ndev_seen.jsonl - the \"seen\" development set\ntest_seen.jsonl - the \"seen\" test set\ndev_unseen.jsonl - the \"unseen\" development set\ntest_unseen.jsonl - the \"unseen\" test set\n\nThe \"seen\" dataset was presented in the NeurIPS paper; the “unseen” dev and test set were released as a part of the NeurIPS 2020 competition.\n\nThe .jsonl format contains one JSON-encoded example per line, each of which has the following fields:\n\n‘text’ - the text occurring in the meme\n‘img’ - the path to the image in the img/ directory\n‘label’ - the label for the meme (0=not-hateful, 1=hateful), provided for train and dev\n\nThe metric to use is AUROC. You may also report accuracy in addition, since this is arguably more interpretable. To compute these metrics, we recommend the roc_auc_score and accuracy_score methods in sklearn.metrics, with default settings.",
"# Getting started\nTo get started working on this dataset, there's an easy-to-use \"starter kit\" available in MMF: URL",
"# Note on Annotator Accuracy\nAs is to be expected with a dataset of this size and nature, some of the examples in the training set have been misclassified. We are not claiming that our dataset labels are completely accurate, or even that all annotators would agree on a particular label. Misclassifications, although possible, should be very rare in the dev and seen test set, however, and we will take extra care with the unseen test set.\n\nAs a reminder, the annotations collected for this dataset were not collected using Facebook annotators and we did not employ Facebook’s hate speech policy. As such, the dataset labels do not in any way reflect Facebook’s official stance on this matter.",
"# License\nThe dataset is licensed under the terms in the 'URL' file.",
"# Image Attribution\nIf you wish to display example memes in your paper, please provide the following attribution:\n\n*Image is a compilation of assets, including ©Getty Image.*\n\ns\nIf you wish to cite this work, please use the following BiBTeX:",
"# Contact\nIf you have any questions or comments on the dataset, please contact hatefulmemeschallenge@URL or one of the authors."
] | [
"TAGS\n#arxiv-2005.04790 #region-us \n",
"# The Hateful Memes Challenge README\n\nThe Hateful Memes Challenge is a dataset and benchmark created by Facebook AI to drive and measure progress on multimodal reasoning and understanding. The task focuses on detecting hate speech in multimodal memes.\n\nPlease see the paper for further details:\n\nThe Hateful Memes Challenge: Detecting Hate Speech in Multimodal Memes\nD. Kiela, H. Firooz, A. Mohan, V. Goswami, A. Singh, P. Ringshia, D. Testuggine\n\nFor more details, see also the website:\n\nURL",
"# Dataset details\n\nThe files for this folder are arranged as follows:\n\nimg/ - the PNG images\nURL - the training set\ndev_seen.jsonl - the \"seen\" development set\ntest_seen.jsonl - the \"seen\" test set\ndev_unseen.jsonl - the \"unseen\" development set\ntest_unseen.jsonl - the \"unseen\" test set\n\nThe \"seen\" dataset was presented in the NeurIPS paper; the “unseen” dev and test set were released as a part of the NeurIPS 2020 competition.\n\nThe .jsonl format contains one JSON-encoded example per line, each of which has the following fields:\n\n‘text’ - the text occurring in the meme\n‘img’ - the path to the image in the img/ directory\n‘label’ - the label for the meme (0=not-hateful, 1=hateful), provided for train and dev\n\nThe metric to use is AUROC. You may also report accuracy in addition, since this is arguably more interpretable. To compute these metrics, we recommend the roc_auc_score and accuracy_score methods in sklearn.metrics, with default settings.",
"# Getting started\nTo get started working on this dataset, there's an easy-to-use \"starter kit\" available in MMF: URL",
"# Note on Annotator Accuracy\nAs is to be expected with a dataset of this size and nature, some of the examples in the training set have been misclassified. We are not claiming that our dataset labels are completely accurate, or even that all annotators would agree on a particular label. Misclassifications, although possible, should be very rare in the dev and seen test set, however, and we will take extra care with the unseen test set.\n\nAs a reminder, the annotations collected for this dataset were not collected using Facebook annotators and we did not employ Facebook’s hate speech policy. As such, the dataset labels do not in any way reflect Facebook’s official stance on this matter.",
"# License\nThe dataset is licensed under the terms in the 'URL' file.",
"# Image Attribution\nIf you wish to display example memes in your paper, please provide the following attribution:\n\n*Image is a compilation of assets, including ©Getty Image.*\n\ns\nIf you wish to cite this work, please use the following BiBTeX:",
"# Contact\nIf you have any questions or comments on the dataset, please contact hatefulmemeschallenge@URL or one of the authors."
] |
2c82a8a0d42f58c4e146198f172126ab093a4c7e | # Dataset Card for "whisper-transcripts-mlst"
[More Information needed](https://github.com/huggingface/datasets/blob/main/CONTRIBUTING.md#how-to-contribute-to-the-dataset-cards) | Whispering-GPT/whisper-transcripts-ml-street-talk | [
"task_categories:automatic-speech-recognition",
"whisper",
"whispering",
"medium",
"region:us"
] | 2022-12-01T05:24:10+00:00 | {"task_categories": ["automatic-speech-recognition"], "dataset_info": {"features": [{"name": "CHANNEL_NAME", "dtype": "string"}, {"name": "URL", "dtype": "string"}, {"name": "TITLE", "dtype": "string"}, {"name": "DESCRIPTION", "dtype": "string"}, {"name": "TRANSCRIPTION", "dtype": "string"}, {"name": "SEGMENTS", "dtype": "string"}], "splits": [{"name": "train", "num_bytes": 21764632, "num_examples": 83}], "download_size": 10320580, "dataset_size": 21764632}, "tags": ["whisper", "whispering", "medium"]} | 2022-12-02T07:19:28+00:00 | [] | [] | TAGS
#task_categories-automatic-speech-recognition #whisper #whispering #medium #region-us
| # Dataset Card for "whisper-transcripts-mlst"
More Information needed | [
"# Dataset Card for \"whisper-transcripts-mlst\"\n\nMore Information needed"
] | [
"TAGS\n#task_categories-automatic-speech-recognition #whisper #whispering #medium #region-us \n",
"# Dataset Card for \"whisper-transcripts-mlst\"\n\nMore Information needed"
] |
d21e7540cb904cf9143b5433c064cefe63e223a0 |
# spanish-tweets-small
## A smaller version of spanish-tweets
## A corpus of tweets for pretraining embeddings and language models
## Table of Contents
- [Table of Contents](#table-of-contents)
- [Dataset Description](#dataset-description)
- [Dataset Summary](#dataset-summary)
- [Supported Tasks and Leaderboards](#supported-tasks-and-leaderboards)
- [Languages](#languages)
- [Dataset Creation](#dataset-creation)
- [Curation Rationale](#curation-rationale)
- [Source Data](#source-data)
- [Additional Information](#additional-information)
- [Dataset Curators](#dataset-curators)
- [Licensing Information](#licensing-information)
- [Citation Information](#citation-information)
- [Contributions](#contributions)
## Dataset Description
- **Homepage**: https://github.com/pysentimiento/robertuito
- **Paper**: [RoBERTuito: a pre-trained language model for social media text in Spanish](https://aclanthology.org/2022.lrec-1.785/)
- **Point of Contact:** jmperez (at) dc.uba.ar
### Dataset Summary
A big dataset of (mostly) Spanish tweets for pre-training language models (or other representations).
### Supported Tasks and Leaderboards
Language Modeling
### Languages
Mostly Spanish, but some Portuguese, English, and other languages.
## Dataset Structure
### Data Fields
- *tweet_id*: id of the tweet
- *user_id*: id of the user
- *text*: text from the tweet
## Dataset Creation
The full process of data collection is described in the paper. Here we roughly outline the main points:
- A Spritzer collection uploaded to Archive.org dating from May 2019 was downloaded
- From this, we only kept tweets with language metadata equal to Spanish, and mark the users who posted these messages.
- Then, the tweetline from each of these marked users was downloaded.
This corpus consists of ~30M tweets.
Please note that we did not filter tweets from other languages, so you might find English, Portuguese, Catalan and other languages in the dataset (around 7/8% of the tweets are not in Spanish)
### Citation Information
```
@inproceedings{perez-etal-2022-robertuito,
title = "{R}o{BERT}uito: a pre-trained language model for social media text in {S}panish",
author = "P{\'e}rez, Juan Manuel and
Furman, Dami{\'a}n Ariel and
Alonso Alemany, Laura and
Luque, Franco M.",
booktitle = "Proceedings of the Thirteenth Language Resources and Evaluation Conference",
month = jun,
year = "2022",
address = "Marseille, France",
publisher = "European Language Resources Association",
url = "https://aclanthology.org/2022.lrec-1.785",
pages = "7235--7243",
abstract = "Since BERT appeared, Transformer language models and transfer learning have become state-of-the-art for natural language processing tasks. Recently, some works geared towards pre-training specially-crafted models for particular domains, such as scientific papers, medical documents, user-generated texts, among others. These domain-specific models have been shown to improve performance significantly in most tasks; however, for languages other than English, such models are not widely available. In this work, we present RoBERTuito, a pre-trained language model for user-generated text in Spanish, trained on over 500 million tweets. Experiments on a benchmark of tasks involving user-generated text showed that RoBERTuito outperformed other pre-trained language models in Spanish. In addition to this, our model has some cross-lingual abilities, achieving top results for English-Spanish tasks of the Linguistic Code-Switching Evaluation benchmark (LinCE) and also competitive performance against monolingual models in English Twitter tasks. To facilitate further research, we make RoBERTuito publicly available at the HuggingFace model hub together with the dataset used to pre-train it.",
}
``` | pysentimiento/spanish-tweets-small | [
"region:us"
] | 2022-12-01T11:52:09+00:00 | {"dataset_info": {"features": [{"name": "text", "dtype": "string"}, {"name": "tweet_id", "dtype": "string"}, {"name": "user_id", "dtype": "string"}], "splits": [{"name": "train", "num_bytes": 82649695458, "num_examples": 597433111}, {"name": "test", "num_bytes": 892219251, "num_examples": 6224733}], "download_size": 51737237106, "dataset_size": 83541914709}} | 2022-12-01T13:50:26+00:00 | [] | [] | TAGS
#region-us
|
# spanish-tweets-small
## A smaller version of spanish-tweets
## A corpus of tweets for pretraining embeddings and language models
## Table of Contents
- Table of Contents
- Dataset Description
- Dataset Summary
- Supported Tasks and Leaderboards
- Languages
- Dataset Creation
- Curation Rationale
- Source Data
- Additional Information
- Dataset Curators
- Licensing Information
- Citation Information
- Contributions
## Dataset Description
- Homepage: URL
- Paper: RoBERTuito: a pre-trained language model for social media text in Spanish
- Point of Contact: jmperez (at) URL
### Dataset Summary
A big dataset of (mostly) Spanish tweets for pre-training language models (or other representations).
### Supported Tasks and Leaderboards
Language Modeling
### Languages
Mostly Spanish, but some Portuguese, English, and other languages.
## Dataset Structure
### Data Fields
- *tweet_id*: id of the tweet
- *user_id*: id of the user
- *text*: text from the tweet
## Dataset Creation
The full process of data collection is described in the paper. Here we roughly outline the main points:
- A Spritzer collection uploaded to URL dating from May 2019 was downloaded
- From this, we only kept tweets with language metadata equal to Spanish, and mark the users who posted these messages.
- Then, the tweetline from each of these marked users was downloaded.
This corpus consists of ~30M tweets.
Please note that we did not filter tweets from other languages, so you might find English, Portuguese, Catalan and other languages in the dataset (around 7/8% of the tweets are not in Spanish)
| [
"# spanish-tweets-small",
"## A smaller version of spanish-tweets",
"## A corpus of tweets for pretraining embeddings and language models",
"## Table of Contents\n- Table of Contents\n- Dataset Description\n - Dataset Summary\n - Supported Tasks and Leaderboards\n - Languages\n- Dataset Creation\n - Curation Rationale\n - Source Data\n- Additional Information\n - Dataset Curators\n - Licensing Information\n - Citation Information\n - Contributions",
"## Dataset Description\n\n- Homepage: URL\n- Paper: RoBERTuito: a pre-trained language model for social media text in Spanish\n- Point of Contact: jmperez (at) URL",
"### Dataset Summary\n\nA big dataset of (mostly) Spanish tweets for pre-training language models (or other representations).",
"### Supported Tasks and Leaderboards\n\nLanguage Modeling",
"### Languages\n\nMostly Spanish, but some Portuguese, English, and other languages.",
"## Dataset Structure",
"### Data Fields\n\n- *tweet_id*: id of the tweet\n- *user_id*: id of the user\n- *text*: text from the tweet",
"## Dataset Creation\n\nThe full process of data collection is described in the paper. Here we roughly outline the main points:\n\n- A Spritzer collection uploaded to URL dating from May 2019 was downloaded\n- From this, we only kept tweets with language metadata equal to Spanish, and mark the users who posted these messages.\n- Then, the tweetline from each of these marked users was downloaded.\n\n\nThis corpus consists of ~30M tweets.\n\nPlease note that we did not filter tweets from other languages, so you might find English, Portuguese, Catalan and other languages in the dataset (around 7/8% of the tweets are not in Spanish)"
] | [
"TAGS\n#region-us \n",
"# spanish-tweets-small",
"## A smaller version of spanish-tweets",
"## A corpus of tweets for pretraining embeddings and language models",
"## Table of Contents\n- Table of Contents\n- Dataset Description\n - Dataset Summary\n - Supported Tasks and Leaderboards\n - Languages\n- Dataset Creation\n - Curation Rationale\n - Source Data\n- Additional Information\n - Dataset Curators\n - Licensing Information\n - Citation Information\n - Contributions",
"## Dataset Description\n\n- Homepage: URL\n- Paper: RoBERTuito: a pre-trained language model for social media text in Spanish\n- Point of Contact: jmperez (at) URL",
"### Dataset Summary\n\nA big dataset of (mostly) Spanish tweets for pre-training language models (or other representations).",
"### Supported Tasks and Leaderboards\n\nLanguage Modeling",
"### Languages\n\nMostly Spanish, but some Portuguese, English, and other languages.",
"## Dataset Structure",
"### Data Fields\n\n- *tweet_id*: id of the tweet\n- *user_id*: id of the user\n- *text*: text from the tweet",
"## Dataset Creation\n\nThe full process of data collection is described in the paper. Here we roughly outline the main points:\n\n- A Spritzer collection uploaded to URL dating from May 2019 was downloaded\n- From this, we only kept tweets with language metadata equal to Spanish, and mark the users who posted these messages.\n- Then, the tweetline from each of these marked users was downloaded.\n\n\nThis corpus consists of ~30M tweets.\n\nPlease note that we did not filter tweets from other languages, so you might find English, Portuguese, Catalan and other languages in the dataset (around 7/8% of the tweets are not in Spanish)"
] |
4323d2431f4471758222493595e0de72041e114b | # Dataset Card for "turkishKuran"
[More Information needed](https://github.com/huggingface/datasets/blob/main/CONTRIBUTING.md#how-to-contribute-to-the-dataset-cards) | erkanxyzalaca/turkishKuran | [
"region:us"
] | 2022-12-01T13:45:28+00:00 | {"dataset_info": {"features": [{"name": "Ayet", "dtype": "string"}, {"name": "review_length", "dtype": "int64"}], "splits": [{"name": "train", "num_bytes": 255726.9, "num_examples": 738}, {"name": "validation", "num_bytes": 28414.1, "num_examples": 82}], "download_size": 0, "dataset_size": 284141.0}} | 2022-12-02T14:01:58+00:00 | [] | [] | TAGS
#region-us
| # Dataset Card for "turkishKuran"
More Information needed | [
"# Dataset Card for \"turkishKuran\"\n\nMore Information needed"
] | [
"TAGS\n#region-us \n",
"# Dataset Card for \"turkishKuran\"\n\nMore Information needed"
] |
d7ca8ddb4327dfa6994e2b44ae6c89b4ea82053e | # Dataset Card for "my_dataset"
[More Information needed](https://github.com/huggingface/datasets/blob/main/CONTRIBUTING.md#how-to-contribute-to-the-dataset-cards) | Mizurodp/my_dataset | [
"region:us"
] | 2022-12-01T16:09:51+00:00 | {"dataset_info": {"features": [{"name": "audio", "dtype": "audio"}, {"name": "transcription", "dtype": "string"}], "splits": [{"name": "train", "num_bytes": 11800901344.896, "num_examples": 219008}, {"name": "test", "num_bytes": 473579114.0, "num_examples": 9230}], "download_size": 10947568917, "dataset_size": 12274480458.896}} | 2022-12-02T08:30:40+00:00 | [] | [] | TAGS
#region-us
| # Dataset Card for "my_dataset"
More Information needed | [
"# Dataset Card for \"my_dataset\"\n\nMore Information needed"
] | [
"TAGS\n#region-us \n",
"# Dataset Card for \"my_dataset\"\n\nMore Information needed"
] |
192c83c7f843e7eba0c522a11cad137e6da5ab26 | # Dataset Card for "gov_report"
## GOV_REPORT
A dataset "consisting of about 19.5k U.S. government reports with expert-written ab- stractive summaries.3 GOVREPORT has two impor- tant features: (1) It contains significantly longer documents (9.4k words) and summaries (553 words) than existing datasets, such as PubMed and arXiv (Cohan et al., 2018) (2) Salient content is spread throughout the documents, as opposed
to cases where summary-worthy words are
more heavily concentrated in specific parts of the
document. These properties make GOVREPORT an
important benchmark for producing long document
summaries with multiple paragraphs.
## Links
- [Paper](https://aclanthology.org/2021.naacl-main.112.pdf)
- [GitHub repo](http://cloud.datacrunch.io)
- [GDrive Folder](https://drive.google.com/drive/folders/128KyqPTwZ0Si9RV_IX-md2dcHeRTUHkr)
## Citation
```
@article{kryscinski2021booksum,
title={BookSum: A Collection of Datasets for Long-form Narrative Summarization},
author={Wojciech Kry{\'s}ci{\'n}ski and Nazneen Rajani and Divyansh Agarwal and Caiming Xiong and Dragomir Radev},
year={2021},
eprint={2105.08209},
archivePrefix={arXiv},
primaryClass={cs.CL}
}
```
## License
Licensing info TBD. [Issue raised](https://github.com/luyang-huang96/LongDocSum/issues/7) in main repo to get info on the license from the original authors. | kmfoda/gov_report | [
"arxiv:2105.08209",
"region:us"
] | 2022-12-01T16:18:16+00:00 | {"dataset_info": {"features": [{"name": "source", "dtype": "string"}, {"name": "target", "dtype": "string"}], "splits": [{"name": "test", "num_bytes": 55710125, "num_examples": 972}, {"name": "train", "num_bytes": 976584268, "num_examples": 17519}, {"name": "validation", "num_bytes": 57315603, "num_examples": 973}], "download_size": 528419980, "dataset_size": 1089609996}} | 2022-12-01T16:52:30+00:00 | [
"2105.08209"
] | [] | TAGS
#arxiv-2105.08209 #region-us
| # Dataset Card for "gov_report"
## GOV_REPORT
A dataset "consisting of about 19.5k U.S. government reports with expert-written ab- stractive summaries.3 GOVREPORT has two impor- tant features: (1) It contains significantly longer documents (9.4k words) and summaries (553 words) than existing datasets, such as PubMed and arXiv (Cohan et al., 2018) (2) Salient content is spread throughout the documents, as opposed
to cases where summary-worthy words are
more heavily concentrated in specific parts of the
document. These properties make GOVREPORT an
important benchmark for producing long document
summaries with multiple paragraphs.
## Links
- Paper
- GitHub repo
- GDrive Folder
## License
Licensing info TBD. Issue raised in main repo to get info on the license from the original authors. | [
"# Dataset Card for \"gov_report\"",
"## GOV_REPORT\nA dataset \"consisting of about 19.5k U.S. government reports with expert-written ab- stractive summaries.3 GOVREPORT has two impor- tant features: (1) It contains significantly longer documents (9.4k words) and summaries (553 words) than existing datasets, such as PubMed and arXiv (Cohan et al., 2018) (2) Salient content is spread throughout the documents, as opposed\nto cases where summary-worthy words are\nmore heavily concentrated in specific parts of the\ndocument. These properties make GOVREPORT an\nimportant benchmark for producing long document\nsummaries with multiple paragraphs.",
"## Links\n\n- Paper\n- GitHub repo\n- GDrive Folder",
"## License\nLicensing info TBD. Issue raised in main repo to get info on the license from the original authors."
] | [
"TAGS\n#arxiv-2105.08209 #region-us \n",
"# Dataset Card for \"gov_report\"",
"## GOV_REPORT\nA dataset \"consisting of about 19.5k U.S. government reports with expert-written ab- stractive summaries.3 GOVREPORT has two impor- tant features: (1) It contains significantly longer documents (9.4k words) and summaries (553 words) than existing datasets, such as PubMed and arXiv (Cohan et al., 2018) (2) Salient content is spread throughout the documents, as opposed\nto cases where summary-worthy words are\nmore heavily concentrated in specific parts of the\ndocument. These properties make GOVREPORT an\nimportant benchmark for producing long document\nsummaries with multiple paragraphs.",
"## Links\n\n- Paper\n- GitHub repo\n- GDrive Folder",
"## License\nLicensing info TBD. Issue raised in main repo to get info on the license from the original authors."
] |
fea8970db0ee25164db34bfce0ff661e5c5ae2d1 | # Indonesian Dataset Apache Access | EgilKarlsen/ApacheAccessLabeled | [
"region:us"
] | 2022-12-01T16:21:21+00:00 | {} | 2022-12-01T16:22:08+00:00 | [] | [] | TAGS
#region-us
| # Indonesian Dataset Apache Access | [
"# Indonesian Dataset Apache Access"
] | [
"TAGS\n#region-us \n",
"# Indonesian Dataset Apache Access"
] |
d42ae7545e6c548a566abed310cf61f85fe895ad |
# NLU Evaluation Data - English and German
A labeled English **and German** language multi-domain dataset (21 domains) with 25K user utterances for human-robot interaction.
This dataset is collected and annotated for evaluating NLU services and platforms.
The detailed paper on this dataset can be found at arXiv.org:
[Benchmarking Natural Language Understanding Services for building Conversational Agents](https://arxiv.org/abs/1903.05566)
The dataset builds on the annotated data of the [xliuhw/NLU-Evaluation-Data](https://github.com/xliuhw/NLU-Evaluation-Data)
repository. We have added an additional column (`answer_de`)
by translating the texts in column `answer` into German.
The translation was made with [DeepL](https://www.deepl.com/translator).
## Creator
This data set was compiled and open sourced by [Philip May](https://may.la/)
of [Deutsche Telekom](https://www.telekom.de/).
## Labels
The columns `scenario` and `intent` can be used for classification tasks.
However, we recommend to use even more fine-grained labels.
For this purpose, a new label can be derived by concatenating `scenario` and `intent`.
For example, this would turn "alarm" and "set" into "alarm_set".
## Dataset Quirks
The original dataset contains some `NaN` values in the `answer` column.
This means that there are also `NaN` values in the translations (`answer_de` column).
These rows should be filtered.
The dataset also contains duplicate values.
## Copyright
Copyright (c) the authors of [xliuhw/NLU-Evaluation-Data](https://github.com/xliuhw/NLU-Evaluation-Data)\
Copyright (c) 2022 [Philip May](https://may.la/)
All data is released under the
[Creative Commons Attribution 4.0 International License (CC BY 4.0)](http://creativecommons.org/licenses/by/4.0/).
| deutsche-telekom/NLU-Evaluation-Data-en-de | [
"task_categories:text-classification",
"task_ids:intent-classification",
"multilinguality:multilingual",
"size_categories:10K<n<100K",
"source_datasets:extended|nlu_evaluation_data",
"language:en",
"language:de",
"license:cc-by-4.0",
"arxiv:1903.05566",
"region:us"
] | 2022-12-01T16:54:19+00:00 | {"language": ["en", "de"], "license": "cc-by-4.0", "multilinguality": ["multilingual"], "size_categories": ["10K<n<100K"], "source_datasets": ["extended|nlu_evaluation_data"], "task_categories": ["text-classification"], "task_ids": ["intent-classification"]} | 2023-12-17T17:42:48+00:00 | [
"1903.05566"
] | [
"en",
"de"
] | TAGS
#task_categories-text-classification #task_ids-intent-classification #multilinguality-multilingual #size_categories-10K<n<100K #source_datasets-extended|nlu_evaluation_data #language-English #language-German #license-cc-by-4.0 #arxiv-1903.05566 #region-us
|
# NLU Evaluation Data - English and German
A labeled English and German language multi-domain dataset (21 domains) with 25K user utterances for human-robot interaction.
This dataset is collected and annotated for evaluating NLU services and platforms.
The detailed paper on this dataset can be found at URL:
Benchmarking Natural Language Understanding Services for building Conversational Agents
The dataset builds on the annotated data of the xliuhw/NLU-Evaluation-Data
repository. We have added an additional column ('answer_de')
by translating the texts in column 'answer' into German.
The translation was made with DeepL.
## Creator
This data set was compiled and open sourced by Philip May
of Deutsche Telekom.
## Labels
The columns 'scenario' and 'intent' can be used for classification tasks.
However, we recommend to use even more fine-grained labels.
For this purpose, a new label can be derived by concatenating 'scenario' and 'intent'.
For example, this would turn "alarm" and "set" into "alarm_set".
## Dataset Quirks
The original dataset contains some 'NaN' values in the 'answer' column.
This means that there are also 'NaN' values in the translations ('answer_de' column).
These rows should be filtered.
The dataset also contains duplicate values.
## Copyright
Copyright (c) the authors of xliuhw/NLU-Evaluation-Data\
Copyright (c) 2022 Philip May
All data is released under the
Creative Commons Attribution 4.0 International License (CC BY 4.0).
| [
"# NLU Evaluation Data - English and German\nA labeled English and German language multi-domain dataset (21 domains) with 25K user utterances for human-robot interaction.\n\nThis dataset is collected and annotated for evaluating NLU services and platforms.\nThe detailed paper on this dataset can be found at URL:\nBenchmarking Natural Language Understanding Services for building Conversational Agents\n\nThe dataset builds on the annotated data of the xliuhw/NLU-Evaluation-Data\nrepository. We have added an additional column ('answer_de')\nby translating the texts in column 'answer' into German.\nThe translation was made with DeepL.",
"## Creator\n\nThis data set was compiled and open sourced by Philip May\nof Deutsche Telekom.",
"## Labels\nThe columns 'scenario' and 'intent' can be used for classification tasks.\nHowever, we recommend to use even more fine-grained labels.\nFor this purpose, a new label can be derived by concatenating 'scenario' and 'intent'.\nFor example, this would turn \"alarm\" and \"set\" into \"alarm_set\".",
"## Dataset Quirks\nThe original dataset contains some 'NaN' values in the 'answer' column.\nThis means that there are also 'NaN' values in the translations ('answer_de' column).\nThese rows should be filtered.\n\nThe dataset also contains duplicate values.",
"## Copyright\nCopyright (c) the authors of xliuhw/NLU-Evaluation-Data\\\nCopyright (c) 2022 Philip May\n\nAll data is released under the\nCreative Commons Attribution 4.0 International License (CC BY 4.0)."
] | [
"TAGS\n#task_categories-text-classification #task_ids-intent-classification #multilinguality-multilingual #size_categories-10K<n<100K #source_datasets-extended|nlu_evaluation_data #language-English #language-German #license-cc-by-4.0 #arxiv-1903.05566 #region-us \n",
"# NLU Evaluation Data - English and German\nA labeled English and German language multi-domain dataset (21 domains) with 25K user utterances for human-robot interaction.\n\nThis dataset is collected and annotated for evaluating NLU services and platforms.\nThe detailed paper on this dataset can be found at URL:\nBenchmarking Natural Language Understanding Services for building Conversational Agents\n\nThe dataset builds on the annotated data of the xliuhw/NLU-Evaluation-Data\nrepository. We have added an additional column ('answer_de')\nby translating the texts in column 'answer' into German.\nThe translation was made with DeepL.",
"## Creator\n\nThis data set was compiled and open sourced by Philip May\nof Deutsche Telekom.",
"## Labels\nThe columns 'scenario' and 'intent' can be used for classification tasks.\nHowever, we recommend to use even more fine-grained labels.\nFor this purpose, a new label can be derived by concatenating 'scenario' and 'intent'.\nFor example, this would turn \"alarm\" and \"set\" into \"alarm_set\".",
"## Dataset Quirks\nThe original dataset contains some 'NaN' values in the 'answer' column.\nThis means that there are also 'NaN' values in the translations ('answer_de' column).\nThese rows should be filtered.\n\nThe dataset also contains duplicate values.",
"## Copyright\nCopyright (c) the authors of xliuhw/NLU-Evaluation-Data\\\nCopyright (c) 2022 Philip May\n\nAll data is released under the\nCreative Commons Attribution 4.0 International License (CC BY 4.0)."
] |
15b0ca33d20ba15984ee7617eda35019051f8949 | ## This is Arabic news data with 9 categories in csv format
original data link: https://www.kaggle.com/datasets/muhammedfathi/arabic-news-texts-corpus
Data preparation and summary link: https://www.kaggle.com/code/abdalrahmanshahrour/arabic-text-summarization | abdalrahmanshahrour/ArabicTextSummarization | [
"region:us"
] | 2022-12-01T17:14:40+00:00 | {} | 2022-12-01T17:16:50+00:00 | [] | [] | TAGS
#region-us
| ## This is Arabic news data with 9 categories in csv format
original data link: URL
Data preparation and summary link: URL | [
"## This is Arabic news data with 9 categories in csv format\n\noriginal data link: URL\n\nData preparation and summary link: URL"
] | [
"TAGS\n#region-us \n",
"## This is Arabic news data with 9 categories in csv format\n\noriginal data link: URL\n\nData preparation and summary link: URL"
] |
bafa0c15e8d5780859c0e0e56eee1e919ced0115 | # AutoTrain Dataset for project: shahroursummarizer
## Dataset Description
This dataset has been automatically processed by AutoTrain for project shahroursummarizer.
### Languages
The BCP-47 code for the dataset's language is ar.
## Dataset Structure
### Data Instances
A sample from this dataset looks as follows:
```json
[
{
"text": "\n\u064a\u0648\u0627\u062c\u0647 \u0627\u0644\u0627\u0633\u0628\u0627\u0646\u064a \u0631\u0641\u0627\u0626\u064a\u0644 \u0646\u0627\u062f\u0627\u0644 \u064a\u0648\u0645 \u063a\u062f \u0627\u0644\u0627\u062d\u062f \u0627\u0646\u0637\u0644\u0627\u0642\u0627 \u0645\u0646 \u0627\u0644\u0633\u0627\u0639\u0629 21:00 \u0645\u0646\u0627\u0641\u0633\u0647 \u0627\u0644\u0633\u0648\u064a\u0633\u0631\u064a \u0631\u0648\u062c\u0631 \u0641\u064a\u062f\u0631\u0631 \u0641\u064a \u0646\u0647\u0627\u0626\u064a \u0628\u0637\u0648\u0644\u0629 \u0645\u064a\u0627\u0645\u064a \u0627\u0644\u0645\u0641\u062a\u0648\u062d\u0629 \u0644\u0644\u062a\u0646\u0633 .\n\u0648 \u064a\u0633\u0639\u0649 \u0641\u064a\u062f\u0631\u0631 \u0644\u062a\u062d\u0642\u064a\u0642 \u062b\u0627\u0644\u062b \u0627\u0644\u0642\u0627\u0628\u0647 \u0647\u0630\u0627 \u0627\u0644\u0645\u0648\u0633\u0645 \u0628\u0639\u062f \u062a\u062a\u0648\u064a\u062c\u0647 \u0628\u0644\u0642\u0628 \u0628\u0637\u0648\u0644\u0629 \u0627\u0633\u062a\u0631\u0627\u0644\u064a\u0627 \u0641\u064a \u062c\u0627\u0646\u0641\u064a \u0627\u0644\u0645\u0627\u0636\u064a \u0639\u0644\u0649 \u062d\u0633\u0627\u0628 \u0646\u0627\u062f\u0627\u0644 \u0648 \u062a\u062a\u0648\u064a\u062c\u0647\u00a0\u0628\u0628\u0637\u0648\u0644\u0629 \u0625\u0646\u062f\u064a\u0627\u0646 \u0648\u064a\u0644\u0632 \u0639\u0644\u0649 \u062d\u0633\u0627\u0628 \u0645\u0648\u0627\u0637\u0646\u0647 \u0641\u0627\u0641\u0631\u064a\u0646\u0643\u0627\u00a0\u00a0.\n",
"target": "\u0648 \u064a\u0633\u0639\u0649 \u0641\u064a\u062f\u0631\u0631 \u0644\u062a\u062d\u0642\u064a\u0642 \u062b\u0627\u0644\u062b \u0627\u0644\u0642\u0627\u0628\u0647 \u0647\u0630\u0627 \u0627\u0644\u0645\u0648\u0633\u0645 \u0628\u0639\u062f \u062a\u062a\u0648\u064a\u062c\u0647 \u0628\u0644\u0642\u0628 \u0628\u0637\u0648\u0644\u0629 \u0627\u0633\u062a\u0631\u0627\u0644\u064a\u0627 \u0641\u064a \u062c\u0627\u0646\u0641\u064a \u0627\u0644\u0645\u0627\u0636\u064a \u0639\u0644\u0649 \u062d\u0633\u0627\u0628 \u0646\u0627\u062f\u0627\u0644 \u0648 \u062a\u062a\u0648\u064a\u062c\u0647\u00a0\u0628\u0628\u0637\u0648\u0644\u0629 \u0625\u0646\u062f\u064a\u0627\u0646 \u0648\u064a\u0644\u0632 \u0639\u0644\u0649 \u062d\u0633\u0627\u0628 \u0645\u0648\u0627\u0637\u0646\u0647 \u0641\u0627\u0641\u0631\u064a\u0646\u0643\u0627\u00a0\u00a0. \n\u064a\u0648\u0627\u062c\u0647 \u0627\u0644\u0627\u0633\u0628\u0627\u0646\u064a \u0631\u0641\u0627\u0626\u064a\u0644 \u0646\u0627\u062f\u0627\u0644 \u064a\u0648\u0645 \u063a\u062f \u0627\u0644\u0627\u062d\u062f \u0627\u0646\u0637\u0644\u0627\u0642\u0627 \u0645\u0646 \u0627\u0644\u0633\u0627\u0639\u0629 21:00 \u0645\u0646\u0627\u0641\u0633\u0647 \u0627\u0644\u0633\u0648\u064a\u0633\u0631\u064a \u0631\u0648\u062c\u0631 \u0641\u064a\u062f\u0631\u0631 \u0641\u064a \u0646\u0647\u0627\u0626\u064a \u0628\u0637\u0648\u0644\u0629 \u0645\u064a\u0627\u0645\u064a \u0627\u0644\u0645\u0641\u062a\u0648\u062d\u0629 \u0644\u0644\u062a\u0646\u0633 ."
},
{
"text": "\n\u0642\u0627\u0644\u062a \u0627\u0644\u0634\u0631\u0637\u0629 \u0627\u0644\u0623\u0645\u064a\u0631\u0643\u064a\u0629 \u0625\u0646 \u0631\u062c\u0644\u0627 \u0645\u0646 \u0648\u0644\u0627\u064a\u0629 \u0628\u0646\u0633\u0644\u0641\u0627\u0646\u064a\u0627 \u0627\u062a\u0635\u0644 \u0645\u0631\u0627\u0631\u0627 \u0628\u062e\u062f\u0645\u0629 \u0627\u0644\u0637\u0648\u0627\u0631\u0626 \u0628\u064a\u0646\u0645\u0627 \u0643\u0627\u0646 \u062a\u062d\u062a \u062a\u0623\u062b\u064a\u0631 \u0627\u0644\u0643\u062d\u0648\u0644 \u0648\u0628\u062d\u0627\u062c\u0629 \u0625\u0644\u0649 \u0634\u062e\u0635 \u064a\u062a\u062d\u062f\u062b \u0645\u0639\u0647.\n\u0648\u0630\u0643\u0631\u062a \u0627\u0644\u0634\u0631\u0637\u0629 \u0625\u0646 \u0644\u0627\u0631\u0649 \u0643\u064a\u0632\u0631 \u0627\u0644\u0628\u0627\u0644\u063a 51 \u0639\u0627\u0645\u0627 \u0627\u062a\u0635\u0644 \u0628\u0627\u0644\u0637\u0648\u0627\u0631\u0626 \u0644\u0623\u0648\u0644 \u0645\u0631\u0629 \u062d\u0648\u0627\u0644\u064a \u0627\u0644\u0639\u0627\u0634\u0631\u0629 \u0648\u0627\u0644\u0646\u0635\u0641 \u0645\u0646 \u0645\u0633\u0627\u0621 \u0627\u0644\u062c\u0645\u0639\u0629\u060c \u0637\u0627\u0644\u0628\u0627 \u0627\u0644\u062a\u062d\u062f\u062b \u0645\u0639 \u0636\u0627\u0628\u0637 \u0634\u0631\u0637\u0629\u060c \u0645\u0646 \u062f\u0648\u0646 \u0627\u0644\u062d\u0627\u062c\u0629 \u0625\u0644\u0649 \u0627\u0633\u062a\u062f\u0639\u0627\u0621 \u0627\u0644\u0637\u0648\u0627\u0631\u0626. \u0648\u0648\u0641\u0642\u0627 \u0644\u0640\"\u0623\u0633\u0648\u0634\u064a\u062a\u062f\u0628\u0631\u0633\" \u0641\u0642\u062f \u0639\u0627\u0648\u062f \u0643\u064a\u0632\u0631 \u0627\u0644\u0627\u062a\u0635\u0627\u0644 5 \u0645\u0631\u0627\u062a \u0623\u062e\u0631\u0649\u060c \u0627\u0644\u0623\u0645\u0631 \u0627\u0644\u0630\u064a \u0627\u0633\u062a\u062f\u0639\u0649 \u0642\u0648\u0629 \u0645\u0646 \u0627\u0644\u0634\u0631\u0637\u0629 \u0625\u0644\u0649 \u0645\u0646\u0632\u0644\u0647 \u0641\u064a \u0628\u0644\u062f\u0629 \u0648\u0627\u064a\u062a\u0647\u0648\u0644 \u0627\u0644\u0634\u0645\u0627\u0644\u064a\u0629 \u0628\u0639\u062f \u0645\u0646\u062a\u0635\u0641 \u0627\u0644\u0644\u064a\u0644. \u0648\u0642\u0627\u0644 \u0627\u0644\u0631\u062c\u0644 \u0644\u0644\u0636\u0628\u0627\u0637 \u0625\u0646\u0647 \u0634\u0631\u0628 \u0627\u0644\u0639\u062f\u064a\u062f \u0645\u0646 \u0627\u0644\u062c\u0639\u0629 \u0644\u0623\u0646\u0647 \u0643\u0627\u0646 \u0645\u0646\u0632\u0639\u062c\u0627 \u0645\u0646 \u0645\u0634\u0627\u0643\u0644\u0647 \u0627\u0644\u0639\u0627\u0626\u0644\u064a\u0629\u060c \u0648\u062a\u0639\u0647\u062f \u0643\u064a\u0632\u0631 \u0628\u0639\u062f\u0645 \u0627\u0644\u0627\u062a\u0635\u0627\u0644 \u0645\u062c\u062f\u062f\u0627 \u0628\u0627\u0644\u0634\u0631\u0637\u0629\u060c \u0625\u0644\u0627 \u0625\u0630\u0627 \u0643\u0627\u0646 \u0647\u0646\u0627\u0643 \u062d\u0627\u0644\u0629 \u0637\u0648\u0627\u0631\u0626 \u062d\u0642\u064a\u0642\u0629 \u062a\u0633\u062a\u062f\u0639\u064a\u0647\u0645. \u0644\u0643\u0646 \u0627\u0644\u0631\u062c\u0644 \u0643\u0631\u0631 \u0627\u0644\u0627\u062a\u0635\u0627\u0644 \u0628\u0627\u0644\u0637\u0648\u0627\u0631\u0626 \u0628\u0639\u062f \u062f\u0642\u064a\u0642\u0629 \u0648\u0627\u062d\u062f\u0629 \u0641\u0642\u0637 \u0645\u0646 \u0645\u063a\u0627\u062f\u0631\u0629 \u0627\u0644\u0636\u0628\u0627\u0637. \u0648\u0642\u062f \u0623\u0644\u0642\u064a \u0627\u0644\u0642\u0628\u0636 \u0639\u0644\u0649 \u0643\u064a\u0632\u0631\u060c \u0644\u064a\u0648\u0627\u062c\u0647 \u0627\u062a\u0647\u0627\u0645\u0627\u062a \u0628\u0627\u0633\u062a\u062f\u0639\u0627\u0621 \u0627\u0644\u0637\u0648\u0627\u0631\u0626 \u0639\u0645\u062f\u0627 \u0644\u0623\u0633\u0628\u0627\u0628 \u063a\u064a\u0631 \u0637\u0627\u0631\u0626\u0629.\n",
"target": "\u0648\u0648\u0641\u0642\u0627 \u0644\u0640\"\u0623\u0633\u0648\u0634\u064a\u062a\u062f\u0628\u0631\u0633\" \u0641\u0642\u062f \u0639\u0627\u0648\u062f \u0643\u064a\u0632\u0631 \u0627\u0644\u0627\u062a\u0635\u0627\u0644 5 \u0645\u0631\u0627\u062a \u0623\u062e\u0631\u0649\u060c \u0627\u0644\u0623\u0645\u0631 \u0627\u0644\u0630\u064a \u0627\u0633\u062a\u062f\u0639\u0649 \u0642\u0648\u0629 \u0645\u0646 \u0627\u0644\u0634\u0631\u0637\u0629 \u0625\u0644\u0649 \u0645\u0646\u0632\u0644\u0647 \u0641\u064a \u0628\u0644\u062f\u0629 \u0648\u0627\u064a\u062a\u0647\u0648\u0644 \u0627\u0644\u0634\u0645\u0627\u0644\u064a\u0629 \u0628\u0639\u062f \u0645\u0646\u062a\u0635\u0641 \u0627\u0644\u0644\u064a\u0644. \u0648\u0630\u0643\u0631\u062a \u0627\u0644\u0634\u0631\u0637\u0629 \u0625\u0646 \u0644\u0627\u0631\u0649 \u0643\u064a\u0632\u0631 \u0627\u0644\u0628\u0627\u0644\u063a 51 \u0639\u0627\u0645\u0627 \u0627\u062a\u0635\u0644 \u0628\u0627\u0644\u0637\u0648\u0627\u0631\u0626 \u0644\u0623\u0648\u0644 \u0645\u0631\u0629 \u062d\u0648\u0627\u0644\u064a \u0627\u0644\u0639\u0627\u0634\u0631\u0629 \u0648\u0627\u0644\u0646\u0635\u0641 \u0645\u0646 \u0645\u0633\u0627\u0621 \u0627\u0644\u062c\u0645\u0639\u0629\u060c \u0637\u0627\u0644\u0628\u0627 \u0627\u0644\u062a\u062d\u062f\u062b \u0645\u0639 \u0636\u0627\u0628\u0637 \u0634\u0631\u0637\u0629\u060c \u0645\u0646 \u062f\u0648\u0646 \u0627\u0644\u062d\u0627\u062c\u0629 \u0625\u0644\u0649 \u0627\u0633\u062a\u062f\u0639\u0627\u0621 \u0627\u0644\u0637\u0648\u0627\u0631\u0626. \u0648\u0642\u0627\u0644 \u0627\u0644\u0631\u062c\u0644 \u0644\u0644\u0636\u0628\u0627\u0637 \u0625\u0646\u0647 \u0634\u0631\u0628 \u0627\u0644\u0639\u062f\u064a\u062f \u0645\u0646 \u0627\u0644\u062c\u0639\u0629 \u0644\u0623\u0646\u0647 \u0643\u0627\u0646 \u0645\u0646\u0632\u0639\u062c\u0627 \u0645\u0646 \u0645\u0634\u0627\u0643\u0644\u0647 \u0627\u0644\u0639\u0627\u0626\u0644\u064a\u0629\u060c \u0648\u062a\u0639\u0647\u062f \u0643\u064a\u0632\u0631 \u0628\u0639\u062f\u0645 \u0627\u0644\u0627\u062a\u0635\u0627\u0644 \u0645\u062c\u062f\u062f\u0627 \u0628\u0627\u0644\u0634\u0631\u0637\u0629\u060c \u0625\u0644\u0627 \u0625\u0630\u0627 \u0643\u0627\u0646 \u0647\u0646\u0627\u0643 \u062d\u0627\u0644\u0629 \u0637\u0648\u0627\u0631\u0626 \u062d\u0642\u064a\u0642\u0629 \u062a\u0633\u062a\u062f\u0639\u064a\u0647\u0645."
}
]
```
### Dataset Fields
The dataset has the following fields (also called "features"):
```json
{
"text": "Value(dtype='string', id=None)",
"target": "Value(dtype='string', id=None)"
}
```
### Dataset Splits
This dataset is split into a train and validation split. The split sizes are as follow:
| Split name | Num samples |
| ------------ | ------------------- |
| train | 2400 |
| valid | 600 |
| abdalrahmanshahrour/shahroursummarizerdataset | [
"language:ar",
"region:us"
] | 2022-12-01T17:19:19+00:00 | {"language": ["ar"], "task_categories": ["conditional-text-generation"]} | 2022-12-01T18:22:47+00:00 | [] | [
"ar"
] | TAGS
#language-Arabic #region-us
| AutoTrain Dataset for project: shahroursummarizer
=================================================
Dataset Description
-------------------
This dataset has been automatically processed by AutoTrain for project shahroursummarizer.
### Languages
The BCP-47 code for the dataset's language is ar.
Dataset Structure
-----------------
### Data Instances
A sample from this dataset looks as follows:
### Dataset Fields
The dataset has the following fields (also called "features"):
### Dataset Splits
This dataset is split into a train and validation split. The split sizes are as follow:
| [
"### Languages\n\n\nThe BCP-47 code for the dataset's language is ar.\n\n\nDataset Structure\n-----------------",
"### Data Instances\n\n\nA sample from this dataset looks as follows:",
"### Dataset Fields\n\n\nThe dataset has the following fields (also called \"features\"):",
"### Dataset Splits\n\n\nThis dataset is split into a train and validation split. The split sizes are as follow:"
] | [
"TAGS\n#language-Arabic #region-us \n",
"### Languages\n\n\nThe BCP-47 code for the dataset's language is ar.\n\n\nDataset Structure\n-----------------",
"### Data Instances\n\n\nA sample from this dataset looks as follows:",
"### Dataset Fields\n\n\nThe dataset has the following fields (also called \"features\"):",
"### Dataset Splits\n\n\nThis dataset is split into a train and validation split. The split sizes are as follow:"
] |
e39ea9713ca47689c8bdd635b94c2d26ea8390f7 |
The xfund dataset with annotations at the word level.
The original XFUND dataset
see more detail at [this](https://github.com/doc-analysis/XFUND)
#### Citation Information
``` latex
@inproceedings{xu-etal-2022-xfund,
title = "{XFUND}: A Benchmark Dataset for Multilingual Visually Rich Form Understanding",
author = "Xu, Yiheng and
Lv, Tengchao and
Cui, Lei and
Wang, Guoxin and
Lu, Yijuan and
Florencio, Dinei and
Zhang, Cha and
Wei, Furu",
booktitle = "Findings of the Association for Computational Linguistics: ACL 2022",
month = may,
year = "2022",
address = "Dublin, Ireland",
publisher = "Association for Computational Linguistics",
url = "https://aclanthology.org/2022.findings-acl.253",
doi = "10.18653/v1/2022.findings-acl.253",
pages = "3214--3224",
abstract = "Multimodal pre-training with text, layout, and image has achieved SOTA performance for visually rich document understanding tasks recently, which demonstrates the great potential for joint learning across different modalities. However, the existed research work has focused only on the English domain while neglecting the importance of multilingual generalization. In this paper, we introduce a human-annotated multilingual form understanding benchmark dataset named XFUND, which includes form understanding samples in 7 languages (Chinese, Japanese, Spanish, French, Italian, German, Portuguese). Meanwhile, we present LayoutXLM, a multimodal pre-trained model for multilingual document understanding, which aims to bridge the language barriers for visually rich document understanding. Experimental results show that the LayoutXLM model has significantly outperformed the existing SOTA cross-lingual pre-trained models on the XFUND dataset. The XFUND dataset and the pre-trained LayoutXLM model have been publicly available at https://aka.ms/layoutxlm.",
}
```
| cooleel/xfund_de | [
"license:mit",
"region:us"
] | 2022-12-01T19:42:05+00:00 | {"license": "mit"} | 2022-12-02T03:12:40+00:00 | [] | [] | TAGS
#license-mit #region-us
|
The xfund dataset with annotations at the word level.
The original XFUND dataset
see more detail at this
| [] | [
"TAGS\n#license-mit #region-us \n"
] |
7509909b272be4338642a3162ae09f4c4d259262 | # CIFAR-10 Webdataset (Test set only)
Original paper: [Learning Multiple Layers of Features from Tiny Images](https://www.cs.toronto.edu/~kriz/learning-features-2009-TR.pdf)
Homepage: https://www.cs.toronto.edu/~kriz/cifar.html
Bibtex:
```
@TECHREPORT{Krizhevsky09learningmultiple,
author = {Alex Krizhevsky},
title = {Learning multiple layers of features from tiny images},
institution = {},
year = {2009}
}
``` | djghosh/wds_cifar10_test | [
"region:us"
] | 2022-12-01T19:47:51+00:00 | {} | 2023-01-24T00:39:41+00:00 | [] | [] | TAGS
#region-us
| # CIFAR-10 Webdataset (Test set only)
Original paper: Learning Multiple Layers of Features from Tiny Images
Homepage: URL
Bibtex:
| [
"# CIFAR-10 Webdataset (Test set only)\n\nOriginal paper: Learning Multiple Layers of Features from Tiny Images\n\nHomepage: URL\n\nBibtex:"
] | [
"TAGS\n#region-us \n",
"# CIFAR-10 Webdataset (Test set only)\n\nOriginal paper: Learning Multiple Layers of Features from Tiny Images\n\nHomepage: URL\n\nBibtex:"
] |
180eb530bf9bc2808c605557971f62db03715b3e | license: MIT
---
---
Updated embedding for SDV 2.1 - ddedv2.pt
This is a Day of the dead embedding for SD2
Use dded to initiate the embedding built on 2.0 </br>
Use ddedv2.pt to initiate embedding built on 2.1
(High quality Professional Photo:1.3) of (Realistic:1) assassin creed character, style dded,HD,4K,8K,highly detailed,Sharp,Photo-realism,Professional photograph,Masterpiece,((Agfacolor)),(close portrait:1.3),(Feminine:1.4),(beautiful:1.4),(attractive:1.3),handsome,calendar pose,perfectly detailed eyes,studio lighting,thematic background

(High quality Professional Photo:1.3) of (Realistic:1) a photo of a beautiful woman with a skull face paint and flowers in her hair and makeup make up for a day of the dead costume, dded style,HD,4K,8K,highly detailed,Sharp,Photo-realism,Professional photograph,Masterpiece,(close portrait:1.3),(Feminine:1.4),(beautiful:1.4),(attractive:1.3),handsome,calendar pose,perfectly detailed eyes,studio lighting,thematic background

Enjoy everyone. | Rocinante2000/Day-of-the-Dead | [
"region:us"
] | 2022-12-01T19:53:02+00:00 | {} | 2022-12-09T11:30:56+00:00 | [] | [] | TAGS
#region-us
| license: MIT
---
---
Updated embedding for SDV 2.1 - URL
This is a Day of the dead embedding for SD2
Use dded to initiate the embedding built on 2.0 </br>
Use URL to initiate embedding built on 2.1
(High quality Professional Photo:1.3) of (Realistic:1) assassin creed character, style dded,HD,4K,8K,highly detailed,Sharp,Photo-realism,Professional photograph,Masterpiece,((Agfacolor)),(close portrait:1.3),(Feminine:1.4),(beautiful:1.4),(attractive:1.3),handsome,calendar pose,perfectly detailed eyes,studio lighting,thematic background
!assasin creed dod
(High quality Professional Photo:1.3) of (Realistic:1) a photo of a beautiful woman with a skull face paint and flowers in her hair and makeup make up for a day of the dead costume, dded style,HD,4K,8K,highly detailed,Sharp,Photo-realism,Professional photograph,Masterpiece,(close portrait:1.3),(Feminine:1.4),(beautiful:1.4),(attractive:1.3),handsome,calendar pose,perfectly detailed eyes,studio lighting,thematic background
!DOD beautiful face
Enjoy everyone. | [] | [
"TAGS\n#region-us \n"
] |
dc347c6e19e40550545475507ca8638d584f4c9c | # lex_fridman_podcast
## Data
This dataset provides transcribed Lex Fridman Podcast episodes that were shared on [Andrej Karpathy's site](https://karpathy.ai/lexicap).
Last update was on 01.12.2022.
## Goal
The goal of this dataset is to use it in [niph - needle in podcast haystack](https://github.com/lambdaofgod/niph) library to make searching it easier.
There exist other similar datasets, but they lack timestamp information that makes finding stuff on Karpathy's page easier.
### Schema
```
# Column Non-Null Count Dtype
--- ------ -------------- -----
0 episode 802299 non-null object
1 text 802299 non-null object
2 timestamp_link 802299 non-null object
```
## Acknowledgements
LONG LIVE ANDREJ KARPATHY!
GLORY TO OpenAI WHISPER!
Thank you Lex Fridman for the podcast!
| lambdaofgod/lex_fridman_podcast | [
"region:us"
] | 2022-12-01T20:11:41+00:00 | {} | 2022-12-01T20:22:12+00:00 | [] | [] | TAGS
#region-us
| # lex_fridman_podcast
## Data
This dataset provides transcribed Lex Fridman Podcast episodes that were shared on Andrej Karpathy's site.
Last update was on 01.12.2022.
## Goal
The goal of this dataset is to use it in niph - needle in podcast haystack library to make searching it easier.
There exist other similar datasets, but they lack timestamp information that makes finding stuff on Karpathy's page easier.
### Schema
## Acknowledgements
LONG LIVE ANDREJ KARPATHY!
GLORY TO OpenAI WHISPER!
Thank you Lex Fridman for the podcast!
| [
"# lex_fridman_podcast",
"## Data\n\nThis dataset provides transcribed Lex Fridman Podcast episodes that were shared on Andrej Karpathy's site.\n\nLast update was on 01.12.2022.",
"## Goal \n\nThe goal of this dataset is to use it in niph - needle in podcast haystack library to make searching it easier.\n\nThere exist other similar datasets, but they lack timestamp information that makes finding stuff on Karpathy's page easier.",
"### Schema",
"## Acknowledgements\n\nLONG LIVE ANDREJ KARPATHY!\n\nGLORY TO OpenAI WHISPER!\n\nThank you Lex Fridman for the podcast!"
] | [
"TAGS\n#region-us \n",
"# lex_fridman_podcast",
"## Data\n\nThis dataset provides transcribed Lex Fridman Podcast episodes that were shared on Andrej Karpathy's site.\n\nLast update was on 01.12.2022.",
"## Goal \n\nThe goal of this dataset is to use it in niph - needle in podcast haystack library to make searching it easier.\n\nThere exist other similar datasets, but they lack timestamp information that makes finding stuff on Karpathy's page easier.",
"### Schema",
"## Acknowledgements\n\nLONG LIVE ANDREJ KARPATHY!\n\nGLORY TO OpenAI WHISPER!\n\nThank you Lex Fridman for the podcast!"
] |
ed47abbab3085f0e4e90b799326fd2932b96f0bd |
# Individuality Of Handwriting (CEDAR)
https://pubmed.ncbi.nlm.nih.gov/12136998/ \
https://cedar.buffalo.edu/NIJ/projectinfo.html
## Abstract
Motivated by several rulings in United States courts concerning expert testimony in general, and handwriting testimony in particular, we undertook a study to objectively validate the hypothesis that handwriting is individual. Handwriting samples of 1,500 individuals, representative of the U.S. population with respect to gender, age, ethnic groups, etc., were obtained. Analyzing differences in handwriting was done by using computer algorithms for extracting features from scanned images of handwriting. Attributes characteristic of the handwriting were obtained, e.g., line separation, slant, character shapes, etc. These attributes, which are a subset of attributes used by forensic document examiners (FDEs), were used to quantitatively establish individuality by using machine learning approaches. Using global attributes of handwriting and very few characters in the writing, the ability to determine the writer with a high degree of confidence was established. The work is a step towards providing scientific support for admitting handwriting evidence in court. The mathematical approach and the resulting software also have the promise of aiding the FDE.
Srihari SN, Cha SH, Arora H, Lee S. Individuality of handwriting. J Forensic Sci. 2002 Jul;47(4):856-72. PMID: 12136998. | 1aurent/individuality-of-handwriting | [
"task_categories:image-classification",
"annotations_creators:crowdsourced",
"language_creators:crowdsourced",
"multilinguality:monolingual",
"size_categories:1K<n<10K",
"language:en",
"license:unknown",
"legal",
"signatures",
"CEDAR",
"region:us"
] | 2022-12-01T20:42:04+00:00 | {"annotations_creators": ["crowdsourced"], "language_creators": ["crowdsourced"], "language": ["en"], "license": ["unknown"], "multilinguality": ["monolingual"], "size_categories": ["1K<n<10K"], "task_categories": ["image-classification"], "pretty_name": "Individuality Of Handwriting (CEDAR)", "dataset_info": {"features": [{"name": "image", "dtype": "image"}, {"name": "label", "dtype": {"class_label": {"names": {"0": "original", "1": "forgeries"}}}}, {"name": "individual", "dtype": "uint8"}, {"name": "figure", "dtype": "uint8"}], "splits": [{"name": "train", "num_bytes": 195780898.8, "num_examples": 2640}], "download_size": 252337526, "dataset_size": 195780898.8}, "tags": ["legal", "signatures", "CEDAR"], "configs": [{"config_name": "default", "data_files": [{"split": "train", "path": "data/train-*"}]}]} | 2023-10-01T14:15:30+00:00 | [] | [
"en"
] | TAGS
#task_categories-image-classification #annotations_creators-crowdsourced #language_creators-crowdsourced #multilinguality-monolingual #size_categories-1K<n<10K #language-English #license-unknown #legal #signatures #CEDAR #region-us
|
# Individuality Of Handwriting (CEDAR)
URL \
URL
## Abstract
Motivated by several rulings in United States courts concerning expert testimony in general, and handwriting testimony in particular, we undertook a study to objectively validate the hypothesis that handwriting is individual. Handwriting samples of 1,500 individuals, representative of the U.S. population with respect to gender, age, ethnic groups, etc., were obtained. Analyzing differences in handwriting was done by using computer algorithms for extracting features from scanned images of handwriting. Attributes characteristic of the handwriting were obtained, e.g., line separation, slant, character shapes, etc. These attributes, which are a subset of attributes used by forensic document examiners (FDEs), were used to quantitatively establish individuality by using machine learning approaches. Using global attributes of handwriting and very few characters in the writing, the ability to determine the writer with a high degree of confidence was established. The work is a step towards providing scientific support for admitting handwriting evidence in court. The mathematical approach and the resulting software also have the promise of aiding the FDE.
Srihari SN, Cha SH, Arora H, Lee S. Individuality of handwriting. J Forensic Sci. 2002 Jul;47(4):856-72. PMID: 12136998. | [
"# Individuality Of Handwriting (CEDAR)\n\nURL \\\nURL",
"## Abstract\n\nMotivated by several rulings in United States courts concerning expert testimony in general, and handwriting testimony in particular, we undertook a study to objectively validate the hypothesis that handwriting is individual. Handwriting samples of 1,500 individuals, representative of the U.S. population with respect to gender, age, ethnic groups, etc., were obtained. Analyzing differences in handwriting was done by using computer algorithms for extracting features from scanned images of handwriting. Attributes characteristic of the handwriting were obtained, e.g., line separation, slant, character shapes, etc. These attributes, which are a subset of attributes used by forensic document examiners (FDEs), were used to quantitatively establish individuality by using machine learning approaches. Using global attributes of handwriting and very few characters in the writing, the ability to determine the writer with a high degree of confidence was established. The work is a step towards providing scientific support for admitting handwriting evidence in court. The mathematical approach and the resulting software also have the promise of aiding the FDE.\n\nSrihari SN, Cha SH, Arora H, Lee S. Individuality of handwriting. J Forensic Sci. 2002 Jul;47(4):856-72. PMID: 12136998."
] | [
"TAGS\n#task_categories-image-classification #annotations_creators-crowdsourced #language_creators-crowdsourced #multilinguality-monolingual #size_categories-1K<n<10K #language-English #license-unknown #legal #signatures #CEDAR #region-us \n",
"# Individuality Of Handwriting (CEDAR)\n\nURL \\\nURL",
"## Abstract\n\nMotivated by several rulings in United States courts concerning expert testimony in general, and handwriting testimony in particular, we undertook a study to objectively validate the hypothesis that handwriting is individual. Handwriting samples of 1,500 individuals, representative of the U.S. population with respect to gender, age, ethnic groups, etc., were obtained. Analyzing differences in handwriting was done by using computer algorithms for extracting features from scanned images of handwriting. Attributes characteristic of the handwriting were obtained, e.g., line separation, slant, character shapes, etc. These attributes, which are a subset of attributes used by forensic document examiners (FDEs), were used to quantitatively establish individuality by using machine learning approaches. Using global attributes of handwriting and very few characters in the writing, the ability to determine the writer with a high degree of confidence was established. The work is a step towards providing scientific support for admitting handwriting evidence in court. The mathematical approach and the resulting software also have the promise of aiding the FDE.\n\nSrihari SN, Cha SH, Arora H, Lee S. Individuality of handwriting. J Forensic Sci. 2002 Jul;47(4):856-72. PMID: 12136998."
] |
0ab77186d4608c21d9b0f8a6b9df199cb93231fa |
# ICDAR 2011 Signature Verification Competition (SigComp2011)
http://iapr-tc11.org/mediawiki/index.php/ICDAR_2011_Signature_Verification_Competition_(SigComp2011)
The collection contains simultaneously acquired online and offline samples.
The collection contains offline and online signature samples. The offline dataset comprises PNG images, scanned at 400 dpi, RGB color. The online dataset comprises ascii files with the format: X, Y, Z (per line).
Marcus Liwicki, Michael Blumenstein, Elisa van den Heuvel, Charles E.H. Berger, Reinoud D. Stoel, Bryan Found, Xiaohong Chen, Muhammad Imran Malik. "SigComp11: Signature Verification Competition for On- and Offline Skilled Forgeries", Proc. 11th Int. Conference on Document Analysis and Recognition, 2011
| 1aurent/ICDAR-2011 | [
"size_categories:1K<n<10K",
"license:unknown",
"online handwriting",
"offline handwriting",
"signature",
"verification",
"region:us"
] | 2022-12-01T21:08:23+00:00 | {"license": "unknown", "size_categories": ["1K<n<10K"], "tags": ["online handwriting", "offline handwriting", "signature", "verification"], "configs": [{"config_name": "default", "data_files": [{"split": "train", "path": "data/train-*"}, {"split": "test", "path": "data/test-*"}]}], "dataset_info": {"features": [{"name": "image", "dtype": "image"}, {"name": "label", "dtype": {"class_label": {"names": {"0": "genuine", "1": "forgeries"}}}}, {"name": "forger", "dtype": "int32"}, {"name": "writer", "dtype": "uint32"}, {"name": "attempt", "dtype": "uint32"}], "splits": [{"name": "train", "num_bytes": 240159596.0, "num_examples": 937}, {"name": "test", "num_bytes": 466376280.094, "num_examples": 2534}], "download_size": 793149429, "dataset_size": 706535876.094}} | 2023-09-23T17:58:09+00:00 | [] | [] | TAGS
#size_categories-1K<n<10K #license-unknown #online handwriting #offline handwriting #signature #verification #region-us
|
# ICDAR 2011 Signature Verification Competition (SigComp2011)
URL
The collection contains simultaneously acquired online and offline samples.
The collection contains offline and online signature samples. The offline dataset comprises PNG images, scanned at 400 dpi, RGB color. The online dataset comprises ascii files with the format: X, Y, Z (per line).
Marcus Liwicki, Michael Blumenstein, Elisa van den Heuvel, Charles E.H. Berger, Reinoud D. Stoel, Bryan Found, Xiaohong Chen, Muhammad Imran Malik. "SigComp11: Signature Verification Competition for On- and Offline Skilled Forgeries", Proc. 11th Int. Conference on Document Analysis and Recognition, 2011
| [
"# ICDAR 2011 Signature Verification Competition (SigComp2011)\n\nURL\n\nThe collection contains simultaneously acquired online and offline samples.\nThe collection contains offline and online signature samples. The offline dataset comprises PNG images, scanned at 400 dpi, RGB color. The online dataset comprises ascii files with the format: X, Y, Z (per line). \n\nMarcus Liwicki, Michael Blumenstein, Elisa van den Heuvel, Charles E.H. Berger, Reinoud D. Stoel, Bryan Found, Xiaohong Chen, Muhammad Imran Malik. \"SigComp11: Signature Verification Competition for On- and Offline Skilled Forgeries\", Proc. 11th Int. Conference on Document Analysis and Recognition, 2011"
] | [
"TAGS\n#size_categories-1K<n<10K #license-unknown #online handwriting #offline handwriting #signature #verification #region-us \n",
"# ICDAR 2011 Signature Verification Competition (SigComp2011)\n\nURL\n\nThe collection contains simultaneously acquired online and offline samples.\nThe collection contains offline and online signature samples. The offline dataset comprises PNG images, scanned at 400 dpi, RGB color. The online dataset comprises ascii files with the format: X, Y, Z (per line). \n\nMarcus Liwicki, Michael Blumenstein, Elisa van den Heuvel, Charles E.H. Berger, Reinoud D. Stoel, Bryan Found, Xiaohong Chen, Muhammad Imran Malik. \"SigComp11: Signature Verification Competition for On- and Offline Skilled Forgeries\", Proc. 11th Int. Conference on Document Analysis and Recognition, 2011"
] |
4c1878fda32583a1f585c32d06f18c4157b1c157 | Dataset of 10000 [Bored Ape](https://opensea.io/collection/boredapeyachtclub) images. | daspartho/bored-ape | [
"region:us"
] | 2022-12-01T22:04:13+00:00 | {} | 2022-12-03T17:47:13+00:00 | [] | [] | TAGS
#region-us
| Dataset of 10000 Bored Ape images. | [] | [
"TAGS\n#region-us \n"
] |
5f571837da18cb1fc390072ad57d6fb89e7b1f71 | # Stanford Cars (Test set only)
Original paper: [3D Object Representations for Fine-Grained Categorization](http://ai.stanford.edu/~jkrause/papers/3drr13.pdf)
Homepage: http://ai.stanford.edu/~jkrause/cars/car_dataset.html
Bibtex:
```
@inproceedings{KrauseStarkDengFei-Fei_3DRR2013,
title = {3D Object Representations for Fine-Grained Categorization},
booktitle = {4th International IEEE Workshop on 3D Representation and Recognition (3dRR-13)},
year = {2013},
address = {Sydney, Australia},
author = {Jonathan Krause and Michael Stark and Jia Deng and Li Fei-Fei}
}
``` | djghosh/wds_cars_test | [
"region:us"
] | 2022-12-01T22:05:28+00:00 | {} | 2022-12-12T22:13:41+00:00 | [] | [] | TAGS
#region-us
| # Stanford Cars (Test set only)
Original paper: 3D Object Representations for Fine-Grained Categorization
Homepage: URL
Bibtex:
| [
"# Stanford Cars (Test set only)\n\nOriginal paper: 3D Object Representations for Fine-Grained Categorization\n\nHomepage: URL\n\nBibtex:"
] | [
"TAGS\n#region-us \n",
"# Stanford Cars (Test set only)\n\nOriginal paper: 3D Object Representations for Fine-Grained Categorization\n\nHomepage: URL\n\nBibtex:"
] |
e26a882e4cedabc7bed82e2e24525dd7e328c14b | # Rendered SST2 (Test set only)
Original paper: [The Visual Task Adaptation Benchmark](https://arxiv.org/abs/1910.04867)
Homepage: https://github.com/openai/CLIP/blob/main/data/rendered-sst2.md
Derived from SST2: https://nlp.stanford.edu/sentiment/treebank.html
Bibtex:
```
@article{zhai2019visual,
title={The Visual Task Adaptation Benchmark},
author={Xiaohua Zhai and Joan Puigcerver and Alexander Kolesnikov and
Pierre Ruyssen and Carlos Riquelme and Mario Lucic and
Josip Djolonga and Andre Susano Pinto and Maxim Neumann and
Alexey Dosovitskiy and Lucas Beyer and Olivier Bachem and
Michael Tschannen and Marcin Michalski and Olivier Bousquet and
Sylvain Gelly and Neil Houlsby},
year={2019},
eprint={1910.04867},
archivePrefix={arXiv},
primaryClass={cs.CV},
url = {https://arxiv.org/abs/1910.04867}
}
``` | djghosh/wds_renderedsst2_test | [
"arxiv:1910.04867",
"region:us"
] | 2022-12-01T22:06:34+00:00 | {} | 2022-12-12T22:07:24+00:00 | [
"1910.04867"
] | [] | TAGS
#arxiv-1910.04867 #region-us
| # Rendered SST2 (Test set only)
Original paper: The Visual Task Adaptation Benchmark
Homepage: URL
Derived from SST2: URL
Bibtex:
| [
"# Rendered SST2 (Test set only)\n\nOriginal paper: The Visual Task Adaptation Benchmark\n\nHomepage: URL\n\nDerived from SST2: URL\n\nBibtex:"
] | [
"TAGS\n#arxiv-1910.04867 #region-us \n",
"# Rendered SST2 (Test set only)\n\nOriginal paper: The Visual Task Adaptation Benchmark\n\nHomepage: URL\n\nDerived from SST2: URL\n\nBibtex:"
] |
9df2c4de5740764de4b1efce408da3291803b38e | # STL-10 (Test set only)
Original paper: [An Analysis of Single Layer Networks in Unsupervised Feature Learning](http://cs.stanford.edu/~acoates/papers/coatesleeng_aistats_2011.pdf)
Homepage: https://cs.stanford.edu/~acoates/stl10/
Bibtex:
```
@InProceedings{pmlr-v15-coates11a,
title = {An Analysis of Single-Layer Networks in Unsupervised Feature Learning},
author = {Coates, Adam and Ng, Andrew and Lee, Honglak},
booktitle = {Proceedings of the Fourteenth International Conference on Artificial Intelligence and Statistics},
pages = {215--223},
year = {2011},
editor = {Gordon, Geoffrey and Dunson, David and Dudík, Miroslav},
volume = {15},
series = {Proceedings of Machine Learning Research},
address = {Fort Lauderdale, FL, USA},
month = {11--13 Apr},
publisher = {PMLR},
pdf = {http://proceedings.mlr.press/v15/coates11a/coates11a.pdf},
url = {https://proceedings.mlr.press/v15/coates11a.html}
}
``` | djghosh/wds_stl10_test | [
"region:us"
] | 2022-12-01T22:07:23+00:00 | {} | 2022-12-12T22:16:13+00:00 | [] | [] | TAGS
#region-us
| # STL-10 (Test set only)
Original paper: An Analysis of Single Layer Networks in Unsupervised Feature Learning
Homepage: URL
Bibtex:
| [
"# STL-10 (Test set only)\n\nOriginal paper: An Analysis of Single Layer Networks in Unsupervised Feature Learning\n\nHomepage: URL\n\nBibtex:"
] | [
"TAGS\n#region-us \n",
"# STL-10 (Test set only)\n\nOriginal paper: An Analysis of Single Layer Networks in Unsupervised Feature Learning\n\nHomepage: URL\n\nBibtex:"
] |
Subsets and Splits
No community queries yet
The top public SQL queries from the community will appear here once available.