sha
stringlengths 40
40
| text
stringlengths 1
13.4M
| id
stringlengths 2
117
| tags
sequencelengths 1
7.91k
| created_at
stringlengths 25
25
| metadata
stringlengths 2
875k
| last_modified
stringlengths 25
25
| arxiv
sequencelengths 0
25
| languages
sequencelengths 0
7.91k
| tags_str
stringlengths 17
159k
| text_str
stringlengths 1
447k
| text_lists
sequencelengths 0
352
| processed_texts
sequencelengths 1
353
|
---|---|---|---|---|---|---|---|---|---|---|---|---|
247e3b4ec632602bead7a90a4fd838450c69c780 | # Dataset Card for AutoTrain Evaluator
This repository contains model predictions generated by [AutoTrain](https://huggingface.co/autotrain) for the following task and dataset:
* Task: Zero-Shot Text Classification
* Model: bigscience/bloom-7b1
* Dataset: futin/guess
* Config: en_3
* Split: test
To run new evaluation jobs, visit Hugging Face's [automatic model evaluator](https://huggingface.co/spaces/autoevaluate/model-evaluator).
## Contributions
Thanks to [@futin](https://huggingface.co/futin) for evaluating this model. | autoevaluate/autoeval-eval-futin__guess-en_3-ab6376-2120068524 | [
"autotrain",
"evaluation",
"region:us"
] | 2022-11-16T15:58:00+00:00 | {"type": "predictions", "tags": ["autotrain", "evaluation"], "datasets": ["futin/guess"], "eval_info": {"task": "text_zero_shot_classification", "model": "bigscience/bloom-7b1", "metrics": [], "dataset_name": "futin/guess", "dataset_config": "en_3", "dataset_split": "test", "col_mapping": {"text": "text", "classes": "classes", "target": "target"}}} | 2022-11-16T17:45:44+00:00 | [] | [] | TAGS
#autotrain #evaluation #region-us
| # Dataset Card for AutoTrain Evaluator
This repository contains model predictions generated by AutoTrain for the following task and dataset:
* Task: Zero-Shot Text Classification
* Model: bigscience/bloom-7b1
* Dataset: futin/guess
* Config: en_3
* Split: test
To run new evaluation jobs, visit Hugging Face's automatic model evaluator.
## Contributions
Thanks to @futin for evaluating this model. | [
"# Dataset Card for AutoTrain Evaluator\n\nThis repository contains model predictions generated by AutoTrain for the following task and dataset:\n\n* Task: Zero-Shot Text Classification\n* Model: bigscience/bloom-7b1\n* Dataset: futin/guess\n* Config: en_3\n* Split: test\n\nTo run new evaluation jobs, visit Hugging Face's automatic model evaluator.",
"## Contributions\n\nThanks to @futin for evaluating this model."
] | [
"TAGS\n#autotrain #evaluation #region-us \n",
"# Dataset Card for AutoTrain Evaluator\n\nThis repository contains model predictions generated by AutoTrain for the following task and dataset:\n\n* Task: Zero-Shot Text Classification\n* Model: bigscience/bloom-7b1\n* Dataset: futin/guess\n* Config: en_3\n* Split: test\n\nTo run new evaluation jobs, visit Hugging Face's automatic model evaluator.",
"## Contributions\n\nThanks to @futin for evaluating this model."
] |
cf77295d81f17cafdac7d0152765e8b42392e296 | # Dataset Card for AutoTrain Evaluator
This repository contains model predictions generated by [AutoTrain](https://huggingface.co/autotrain) for the following task and dataset:
* Task: Zero-Shot Text Classification
* Model: bigscience/bloom-1b7
* Dataset: futin/guess
* Config: en_3
* Split: test
To run new evaluation jobs, visit Hugging Face's [automatic model evaluator](https://huggingface.co/spaces/autoevaluate/model-evaluator).
## Contributions
Thanks to [@futin](https://huggingface.co/futin) for evaluating this model. | autoevaluate/autoeval-eval-futin__guess-en_3-ab6376-2120068525 | [
"autotrain",
"evaluation",
"region:us"
] | 2022-11-16T15:58:01+00:00 | {"type": "predictions", "tags": ["autotrain", "evaluation"], "datasets": ["futin/guess"], "eval_info": {"task": "text_zero_shot_classification", "model": "bigscience/bloom-1b7", "metrics": [], "dataset_name": "futin/guess", "dataset_config": "en_3", "dataset_split": "test", "col_mapping": {"text": "text", "classes": "classes", "target": "target"}}} | 2022-11-16T16:35:35+00:00 | [] | [] | TAGS
#autotrain #evaluation #region-us
| # Dataset Card for AutoTrain Evaluator
This repository contains model predictions generated by AutoTrain for the following task and dataset:
* Task: Zero-Shot Text Classification
* Model: bigscience/bloom-1b7
* Dataset: futin/guess
* Config: en_3
* Split: test
To run new evaluation jobs, visit Hugging Face's automatic model evaluator.
## Contributions
Thanks to @futin for evaluating this model. | [
"# Dataset Card for AutoTrain Evaluator\n\nThis repository contains model predictions generated by AutoTrain for the following task and dataset:\n\n* Task: Zero-Shot Text Classification\n* Model: bigscience/bloom-1b7\n* Dataset: futin/guess\n* Config: en_3\n* Split: test\n\nTo run new evaluation jobs, visit Hugging Face's automatic model evaluator.",
"## Contributions\n\nThanks to @futin for evaluating this model."
] | [
"TAGS\n#autotrain #evaluation #region-us \n",
"# Dataset Card for AutoTrain Evaluator\n\nThis repository contains model predictions generated by AutoTrain for the following task and dataset:\n\n* Task: Zero-Shot Text Classification\n* Model: bigscience/bloom-1b7\n* Dataset: futin/guess\n* Config: en_3\n* Split: test\n\nTo run new evaluation jobs, visit Hugging Face's automatic model evaluator.",
"## Contributions\n\nThanks to @futin for evaluating this model."
] |
df149fbf9bcca94959d9177c4e99526172e530bf | # Dataset Card for AutoTrain Evaluator
This repository contains model predictions generated by [AutoTrain](https://huggingface.co/autotrain) for the following task and dataset:
* Task: Zero-Shot Text Classification
* Model: bigscience/bloom-560m
* Dataset: futin/guess
* Config: en_3
* Split: test
To run new evaluation jobs, visit Hugging Face's [automatic model evaluator](https://huggingface.co/spaces/autoevaluate/model-evaluator).
## Contributions
Thanks to [@futin](https://huggingface.co/futin) for evaluating this model. | autoevaluate/autoeval-eval-futin__guess-en_3-ab6376-2120068527 | [
"autotrain",
"evaluation",
"region:us"
] | 2022-11-16T15:58:07+00:00 | {"type": "predictions", "tags": ["autotrain", "evaluation"], "datasets": ["futin/guess"], "eval_info": {"task": "text_zero_shot_classification", "model": "bigscience/bloom-560m", "metrics": [], "dataset_name": "futin/guess", "dataset_config": "en_3", "dataset_split": "test", "col_mapping": {"text": "text", "classes": "classes", "target": "target"}}} | 2022-11-16T16:31:49+00:00 | [] | [] | TAGS
#autotrain #evaluation #region-us
| # Dataset Card for AutoTrain Evaluator
This repository contains model predictions generated by AutoTrain for the following task and dataset:
* Task: Zero-Shot Text Classification
* Model: bigscience/bloom-560m
* Dataset: futin/guess
* Config: en_3
* Split: test
To run new evaluation jobs, visit Hugging Face's automatic model evaluator.
## Contributions
Thanks to @futin for evaluating this model. | [
"# Dataset Card for AutoTrain Evaluator\n\nThis repository contains model predictions generated by AutoTrain for the following task and dataset:\n\n* Task: Zero-Shot Text Classification\n* Model: bigscience/bloom-560m\n* Dataset: futin/guess\n* Config: en_3\n* Split: test\n\nTo run new evaluation jobs, visit Hugging Face's automatic model evaluator.",
"## Contributions\n\nThanks to @futin for evaluating this model."
] | [
"TAGS\n#autotrain #evaluation #region-us \n",
"# Dataset Card for AutoTrain Evaluator\n\nThis repository contains model predictions generated by AutoTrain for the following task and dataset:\n\n* Task: Zero-Shot Text Classification\n* Model: bigscience/bloom-560m\n* Dataset: futin/guess\n* Config: en_3\n* Split: test\n\nTo run new evaluation jobs, visit Hugging Face's automatic model evaluator.",
"## Contributions\n\nThanks to @futin for evaluating this model."
] |
131f0b6c9736853611c0294edea5346d8f0990cc | # Dataset Card for "zalo-ai-train"
[More Information needed](https://github.com/huggingface/datasets/blob/main/CONTRIBUTING.md#how-to-contribute-to-the-dataset-cards) | hungngocphat01/zalo-ai-train | [
"region:us"
] | 2022-11-16T16:51:42+00:00 | {"dataset_info": {"features": [{"name": "audio", "dtype": "audio"}, {"name": "text", "dtype": "string"}], "splits": [{"name": "train", "num_bytes": 642229551.79, "num_examples": 9217}], "download_size": 641925455, "dataset_size": 642229551.79}} | 2022-11-19T05:06:32+00:00 | [] | [] | TAGS
#region-us
| # Dataset Card for "zalo-ai-train"
More Information needed | [
"# Dataset Card for \"zalo-ai-train\"\n\nMore Information needed"
] | [
"TAGS\n#region-us \n",
"# Dataset Card for \"zalo-ai-train\"\n\nMore Information needed"
] |
6b2c98066ce597b9de0fb040e6baec52eadbbc75 |
# Dataset Card for Wikipedia
This repo is a wrapper around [olm/wikipedia](https://huggingface.co/datasets/olm/wikipedia) that just concatenates data from the EU languages.
Please refer to it for a complete data card.
The EU languages we include are:
- bg
- cs
- da
- de
- el
- en
- es
- et
- fi
- fr
- ga
- hr
- hu
- it
- lt
- lv
- mt
- nl
- pl
- pt
- ro
- sk
- sl
- sv
As with `olm/wikipedia` you will need to install a few dependencies:
```
pip install mwparserfromhell==0.6.4 multiprocess==0.70.13
```
```python
from datasets import load_dataset
load_dataset("dlwh/eu_wikipedias", date="20221101")
```
Please refer to the original olm/wikipedia for a complete data card.
| dlwh/eu_wikipedias | [
"task_categories:text-generation",
"task_categories:fill-mask",
"task_ids:language-modeling",
"task_ids:masked-language-modeling",
"annotations_creators:no-annotation",
"language_creators:crowdsourced",
"multilinguality:multilingual",
"size_categories:n<1K",
"size_categories:1K<n<10K",
"size_categories:10K<n<100K",
"size_categories:100K<n<1M",
"size_categories:1M<n<10M",
"source_datasets:original",
"language:bg",
"language:cs",
"language:da",
"language:de",
"language:el",
"language:en",
"language:es",
"language:et",
"language:fi",
"language:fr",
"language:ga",
"language:hr",
"language:hu",
"language:it",
"language:lt",
"language:lv",
"language:mt",
"language:nl",
"language:pl",
"language:pt",
"language:ro",
"language:sk",
"language:sl",
"language:sv",
"license:cc-by-sa-3.0",
"license:gfdl",
"region:us"
] | 2022-11-16T18:03:07+00:00 | {"annotations_creators": ["no-annotation"], "language_creators": ["crowdsourced"], "language": ["bg", "cs", "da", "de", "el", "en", "es", "et", "fi", "fr", "ga", "hr", "hu", "it", "lt", "lv", "mt", "nl", "pl", "pt", "ro", "sk", "sl", "sv"], "license": ["cc-by-sa-3.0", "gfdl"], "multilinguality": ["multilingual"], "size_categories": ["n<1K", "1K<n<10K", "10K<n<100K", "100K<n<1M", "1M<n<10M"], "source_datasets": ["original"], "task_categories": ["text-generation", "fill-mask"], "task_ids": ["language-modeling", "masked-language-modeling"], "pretty_name": "Wikipedia"} | 2022-11-17T08:13:51+00:00 | [] | [
"bg",
"cs",
"da",
"de",
"el",
"en",
"es",
"et",
"fi",
"fr",
"ga",
"hr",
"hu",
"it",
"lt",
"lv",
"mt",
"nl",
"pl",
"pt",
"ro",
"sk",
"sl",
"sv"
] | TAGS
#task_categories-text-generation #task_categories-fill-mask #task_ids-language-modeling #task_ids-masked-language-modeling #annotations_creators-no-annotation #language_creators-crowdsourced #multilinguality-multilingual #size_categories-n<1K #size_categories-1K<n<10K #size_categories-10K<n<100K #size_categories-100K<n<1M #size_categories-1M<n<10M #source_datasets-original #language-Bulgarian #language-Czech #language-Danish #language-German #language-Modern Greek (1453-) #language-English #language-Spanish #language-Estonian #language-Finnish #language-French #language-Irish #language-Croatian #language-Hungarian #language-Italian #language-Lithuanian #language-Latvian #language-Maltese #language-Dutch #language-Polish #language-Portuguese #language-Romanian #language-Slovak #language-Slovenian #language-Swedish #license-cc-by-sa-3.0 #license-gfdl #region-us
|
# Dataset Card for Wikipedia
This repo is a wrapper around olm/wikipedia that just concatenates data from the EU languages.
Please refer to it for a complete data card.
The EU languages we include are:
- bg
- cs
- da
- de
- el
- en
- es
- et
- fi
- fr
- ga
- hr
- hu
- it
- lt
- lv
- mt
- nl
- pl
- pt
- ro
- sk
- sl
- sv
As with 'olm/wikipedia' you will need to install a few dependencies:
Please refer to the original olm/wikipedia for a complete data card.
| [
"# Dataset Card for Wikipedia\n\nThis repo is a wrapper around olm/wikipedia that just concatenates data from the EU languages.\nPlease refer to it for a complete data card.\n\nThe EU languages we include are:\n - bg\n - cs\n - da\n - de\n - el\n - en\n - es\n - et\n - fi\n - fr\n - ga\n - hr\n - hu\n - it\n - lt\n - lv\n - mt\n - nl\n - pl\n - pt\n - ro\n - sk\n - sl\n - sv\n\n\nAs with 'olm/wikipedia' you will need to install a few dependencies:\n\n\n\n\n\n\nPlease refer to the original olm/wikipedia for a complete data card."
] | [
"TAGS\n#task_categories-text-generation #task_categories-fill-mask #task_ids-language-modeling #task_ids-masked-language-modeling #annotations_creators-no-annotation #language_creators-crowdsourced #multilinguality-multilingual #size_categories-n<1K #size_categories-1K<n<10K #size_categories-10K<n<100K #size_categories-100K<n<1M #size_categories-1M<n<10M #source_datasets-original #language-Bulgarian #language-Czech #language-Danish #language-German #language-Modern Greek (1453-) #language-English #language-Spanish #language-Estonian #language-Finnish #language-French #language-Irish #language-Croatian #language-Hungarian #language-Italian #language-Lithuanian #language-Latvian #language-Maltese #language-Dutch #language-Polish #language-Portuguese #language-Romanian #language-Slovak #language-Slovenian #language-Swedish #license-cc-by-sa-3.0 #license-gfdl #region-us \n",
"# Dataset Card for Wikipedia\n\nThis repo is a wrapper around olm/wikipedia that just concatenates data from the EU languages.\nPlease refer to it for a complete data card.\n\nThe EU languages we include are:\n - bg\n - cs\n - da\n - de\n - el\n - en\n - es\n - et\n - fi\n - fr\n - ga\n - hr\n - hu\n - it\n - lt\n - lv\n - mt\n - nl\n - pl\n - pt\n - ro\n - sk\n - sl\n - sv\n\n\nAs with 'olm/wikipedia' you will need to install a few dependencies:\n\n\n\n\n\n\nPlease refer to the original olm/wikipedia for a complete data card."
] |
9ff900bee6cf6db545000652535d44345757fd51 | # VietNews-Abs-Sum
A dataset for Vietnamese Abstractive Summarization task.
It includes all articles from Vietnews (VNDS) dataset which was released by Van-Hau Nguyen et al.
The articles were collected from tuoitre.vn, vnexpress.net, and nguoiduatin.vn online newspaper by the authors.
# Introduction
This dataset was extracted from Train/Val/Test split of Vietnews dataset. All files from *test_tokenized*, *train_tokenized* and *val_tokenized* directories are fetched and preprocessed with punctuation normalization. The subsets then are stored in the *raw* director with 3 files *train.tsv*, *valid.tsv*, and *test.tsv* accordingly. These files will be considered as the original raw dataset as nothing changes except the punctuation normalization.
As pointed out in *BARTpho: Pre-trained Sequence-to-Sequence Models for Vietnamese*, there are lots of duplicated samples across subsets. Therefore, we do another preprocessing process to remove all the duplicated samples. The process includes the following steps:
- First, remove all duplicates from each subset
- Second, merge all subsets into 1 set with the following order: test + val + train
- Finally, remove all duplicates from that merged set and then split out into 3 new subsets
The final subsets are the same to the orignal subsets but all duplicates were removed. Each subset now has total samples as follows:
- train_no_dups.tsv: 99134 samples
- valid_no_dups.tsv: 22184 samples
- test_no_dups.tsv: 22498 samples
Totally, we have 99134 + 22184 + 22498 = 143816 samples after filtering!
Note that this result is not the same as the number of samples reported in BARTpho paper, but there is no duplicate inside each subset or across subsets anymore.
These filtered subsets are also exported into JSONLINE format to support future training script that requires this data format.
# Directory structure
- raw: contains 3 raw subset files fetched from Vietnews directories
- train.tsv
- val.tsv
- test.tsv
- processed: contains duplicates filtered subsets
- test.tsv
- train.tsv
- valid.tsv
- test.jsonl
- train.jsonl
- valid.jsonl
- [and other variants]
# Credits
- Special thanks to Vietnews (VNDS) authors: https://github.com/ThanhChinhBK/vietnews
| ithieund/VietNews-Abs-Sum | [
"region:us"
] | 2022-11-16T18:26:54+00:00 | {} | 2022-11-17T10:46:16+00:00 | [] | [] | TAGS
#region-us
| # VietNews-Abs-Sum
A dataset for Vietnamese Abstractive Summarization task.
It includes all articles from Vietnews (VNDS) dataset which was released by Van-Hau Nguyen et al.
The articles were collected from URL, URL, and URL online newspaper by the authors.
# Introduction
This dataset was extracted from Train/Val/Test split of Vietnews dataset. All files from *test_tokenized*, *train_tokenized* and *val_tokenized* directories are fetched and preprocessed with punctuation normalization. The subsets then are stored in the *raw* director with 3 files *URL*, *URL*, and *URL* accordingly. These files will be considered as the original raw dataset as nothing changes except the punctuation normalization.
As pointed out in *BARTpho: Pre-trained Sequence-to-Sequence Models for Vietnamese*, there are lots of duplicated samples across subsets. Therefore, we do another preprocessing process to remove all the duplicated samples. The process includes the following steps:
- First, remove all duplicates from each subset
- Second, merge all subsets into 1 set with the following order: test + val + train
- Finally, remove all duplicates from that merged set and then split out into 3 new subsets
The final subsets are the same to the orignal subsets but all duplicates were removed. Each subset now has total samples as follows:
- train_no_dups.tsv: 99134 samples
- valid_no_dups.tsv: 22184 samples
- test_no_dups.tsv: 22498 samples
Totally, we have 99134 + 22184 + 22498 = 143816 samples after filtering!
Note that this result is not the same as the number of samples reported in BARTpho paper, but there is no duplicate inside each subset or across subsets anymore.
These filtered subsets are also exported into JSONLINE format to support future training script that requires this data format.
# Directory structure
- raw: contains 3 raw subset files fetched from Vietnews directories
- URL
- URL
- URL
- processed: contains duplicates filtered subsets
- URL
- URL
- URL
- URL
- URL
- URL
- [and other variants]
# Credits
- Special thanks to Vietnews (VNDS) authors: URL
| [
"# VietNews-Abs-Sum\nA dataset for Vietnamese Abstractive Summarization task. \nIt includes all articles from Vietnews (VNDS) dataset which was released by Van-Hau Nguyen et al. \nThe articles were collected from URL, URL, and URL online newspaper by the authors.",
"# Introduction\nThis dataset was extracted from Train/Val/Test split of Vietnews dataset. All files from *test_tokenized*, *train_tokenized* and *val_tokenized* directories are fetched and preprocessed with punctuation normalization. The subsets then are stored in the *raw* director with 3 files *URL*, *URL*, and *URL* accordingly. These files will be considered as the original raw dataset as nothing changes except the punctuation normalization.\n\nAs pointed out in *BARTpho: Pre-trained Sequence-to-Sequence Models for Vietnamese*, there are lots of duplicated samples across subsets. Therefore, we do another preprocessing process to remove all the duplicated samples. The process includes the following steps:\n- First, remove all duplicates from each subset\n- Second, merge all subsets into 1 set with the following order: test + val + train\n- Finally, remove all duplicates from that merged set and then split out into 3 new subsets\n\nThe final subsets are the same to the orignal subsets but all duplicates were removed. Each subset now has total samples as follows:\n- train_no_dups.tsv: 99134 samples\n- valid_no_dups.tsv: 22184 samples\n- test_no_dups.tsv: 22498 samples\n\nTotally, we have 99134 + 22184 + 22498 = 143816 samples after filtering! \nNote that this result is not the same as the number of samples reported in BARTpho paper, but there is no duplicate inside each subset or across subsets anymore.\n\nThese filtered subsets are also exported into JSONLINE format to support future training script that requires this data format.",
"# Directory structure\n- raw: contains 3 raw subset files fetched from Vietnews directories\n - URL\n - URL\n - URL\n- processed: contains duplicates filtered subsets\n - URL\n - URL\n - URL\n - URL\n - URL\n - URL\n - [and other variants]",
"# Credits\n- Special thanks to Vietnews (VNDS) authors: URL"
] | [
"TAGS\n#region-us \n",
"# VietNews-Abs-Sum\nA dataset for Vietnamese Abstractive Summarization task. \nIt includes all articles from Vietnews (VNDS) dataset which was released by Van-Hau Nguyen et al. \nThe articles were collected from URL, URL, and URL online newspaper by the authors.",
"# Introduction\nThis dataset was extracted from Train/Val/Test split of Vietnews dataset. All files from *test_tokenized*, *train_tokenized* and *val_tokenized* directories are fetched and preprocessed with punctuation normalization. The subsets then are stored in the *raw* director with 3 files *URL*, *URL*, and *URL* accordingly. These files will be considered as the original raw dataset as nothing changes except the punctuation normalization.\n\nAs pointed out in *BARTpho: Pre-trained Sequence-to-Sequence Models for Vietnamese*, there are lots of duplicated samples across subsets. Therefore, we do another preprocessing process to remove all the duplicated samples. The process includes the following steps:\n- First, remove all duplicates from each subset\n- Second, merge all subsets into 1 set with the following order: test + val + train\n- Finally, remove all duplicates from that merged set and then split out into 3 new subsets\n\nThe final subsets are the same to the orignal subsets but all duplicates were removed. Each subset now has total samples as follows:\n- train_no_dups.tsv: 99134 samples\n- valid_no_dups.tsv: 22184 samples\n- test_no_dups.tsv: 22498 samples\n\nTotally, we have 99134 + 22184 + 22498 = 143816 samples after filtering! \nNote that this result is not the same as the number of samples reported in BARTpho paper, but there is no duplicate inside each subset or across subsets anymore.\n\nThese filtered subsets are also exported into JSONLINE format to support future training script that requires this data format.",
"# Directory structure\n- raw: contains 3 raw subset files fetched from Vietnews directories\n - URL\n - URL\n - URL\n- processed: contains duplicates filtered subsets\n - URL\n - URL\n - URL\n - URL\n - URL\n - URL\n - [and other variants]",
"# Credits\n- Special thanks to Vietnews (VNDS) authors: URL"
] |
6eca9828d803494f43b9623a6e952c37a595778d | # Dataset Card for "testnnk"
[More Information needed](https://github.com/huggingface/datasets/blob/main/CONTRIBUTING.md#how-to-contribute-to-the-dataset-cards) | juancopi81/testnnk | [
"region:us"
] | 2022-11-16T19:33:19+00:00 | {"dataset_info": {"features": [{"name": "CHANNEL_NAME", "dtype": "string"}, {"name": "URL", "dtype": "string"}, {"name": "TITLE", "dtype": "string"}, {"name": "DESCRIPTION", "dtype": "string"}, {"name": "TRANSCRIPTION", "dtype": "string"}, {"name": "SEGMENTS", "dtype": "string"}], "splits": [{"name": "train", "num_bytes": 382632, "num_examples": 1}], "download_size": 176707, "dataset_size": 382632}} | 2022-11-16T19:33:22+00:00 | [] | [] | TAGS
#region-us
| # Dataset Card for "testnnk"
More Information needed | [
"# Dataset Card for \"testnnk\"\n\nMore Information needed"
] | [
"TAGS\n#region-us \n",
"# Dataset Card for \"testnnk\"\n\nMore Information needed"
] |
a99195d7d7197eb9547133cea5046fb81b19a4aa | # Dataset Card for "logo-blip"
[More Information needed](https://github.com/huggingface/datasets/blob/main/CONTRIBUTING.md#how-to-contribute-to-the-dataset-cards) | salmonhumorous/logo-blip-caption | [
"region:us"
] | 2022-11-16T19:35:45+00:00 | {"dataset_info": {"features": [{"name": "image", "dtype": "image"}, {"name": "text", "dtype": "string"}], "splits": [{"name": "train", "num_bytes": 24808769.89, "num_examples": 1435}], "download_size": 24242906, "dataset_size": 24808769.89}} | 2022-11-16T19:35:54+00:00 | [] | [] | TAGS
#region-us
| # Dataset Card for "logo-blip"
More Information needed | [
"# Dataset Card for \"logo-blip\"\n\nMore Information needed"
] | [
"TAGS\n#region-us \n",
"# Dataset Card for \"logo-blip\"\n\nMore Information needed"
] |
55de12c96f4bc4cc14351b3660e009c8c5186088 | # Dataset Card for "ChristmasClaymation-blip-captions"
All captions end with the suffix ", Christmas claymation style" | Norod78/ChristmasClaymation-blip-captions | [
"task_categories:text-to-image",
"annotations_creators:machine-generated",
"language_creators:other",
"multilinguality:monolingual",
"size_categories:n<1K",
"language:en",
"license:cc-by-nc-sa-4.0",
"region:us"
] | 2022-11-16T20:12:20+00:00 | {"annotations_creators": ["machine-generated"], "language_creators": ["other"], "language": ["en"], "license": "cc-by-nc-sa-4.0", "multilinguality": ["monolingual"], "size_categories": ["n<1K"], "task_categories": ["text-to-image"], "pretty_name": "Christmas claymation style, BLIP captions", "dataset_info": {"features": [{"name": "image", "dtype": "image"}, {"name": "text", "dtype": "string"}], "splits": [{"name": "train", "num_bytes": 128397390.0, "num_examples": 401}], "download_size": 125229613, "dataset_size": 128397390.0}, "tags": []} | 2022-11-16T20:18:18+00:00 | [] | [
"en"
] | TAGS
#task_categories-text-to-image #annotations_creators-machine-generated #language_creators-other #multilinguality-monolingual #size_categories-n<1K #language-English #license-cc-by-nc-sa-4.0 #region-us
| # Dataset Card for "ChristmasClaymation-blip-captions"
All captions end with the suffix ", Christmas claymation style" | [
"# Dataset Card for \"ChristmasClaymation-blip-captions\"\n\nAll captions end with the suffix \", Christmas claymation style\""
] | [
"TAGS\n#task_categories-text-to-image #annotations_creators-machine-generated #language_creators-other #multilinguality-monolingual #size_categories-n<1K #language-English #license-cc-by-nc-sa-4.0 #region-us \n",
"# Dataset Card for \"ChristmasClaymation-blip-captions\"\n\nAll captions end with the suffix \", Christmas claymation style\""
] |
49b676d5016b3f1c19df199f08d406f062ce400c | # viWikiHow-Abs-Sum
A dataset for Vietnamese Abstractive Summarization task.
It includes all Vietnamese posts from WikiHow which was released in WikiLingua dataset.
# Introduction
This dataset was extracted from Train/Test split of WikiLingua dataset. As the target language is Vietnamese, we remove all other files, just keep train.\*.vi, test.\*.vi, and val.\*.vi for Vietnamese Abstractive Summarization task. The raw files then are stored in the *raw* director and after that, we run the python script to generate ready-to-use data files in TSV and JSONLINE formats which are stored in *processed* directory to be easily used for future training scripts.
# Directory structure
- raw: contains raw text files from WikiLingua
- test.src.vi
- test.tgt.vi
- train.src.vi
- train.tgt.vi
- val.src.vi
- val.tgt.vi
- processed: contains generated TSV and JSONLINE files
- test.tsv
- train.tsv
- valid.tsv
- test.jsonl
- train.jsonl
- valid.jsonl
- [and other variants]
# Credits
- Special thanks to WikiLingua authors: https://github.com/esdurmus/Wikilingua
- Article provided by <a href="https://www.wikihow.com/Main-Page" target="_blank">wikiHow</a>, a wiki that is building the world's largest and highest quality how-to manual. Please edit this article and find author credits at the original wikiHow article on How to Tie a Tie. Content on wikiHow can be shared under a <a href="http://creativecommons.org/licenses/by-nc-sa/3.0/" target="_blank">Creative Commons License</a>.
| ithieund/viWikiHow-Abs-Sum | [
"region:us"
] | 2022-11-16T20:34:58+00:00 | {} | 2022-11-16T20:50:46+00:00 | [] | [] | TAGS
#region-us
| # viWikiHow-Abs-Sum
A dataset for Vietnamese Abstractive Summarization task.
It includes all Vietnamese posts from WikiHow which was released in WikiLingua dataset.
# Introduction
This dataset was extracted from Train/Test split of WikiLingua dataset. As the target language is Vietnamese, we remove all other files, just keep train.\*.vi, test.\*.vi, and val.\*.vi for Vietnamese Abstractive Summarization task. The raw files then are stored in the *raw* director and after that, we run the python script to generate ready-to-use data files in TSV and JSONLINE formats which are stored in *processed* directory to be easily used for future training scripts.
# Directory structure
- raw: contains raw text files from WikiLingua
- URL
- URL
- URL
- URL
- URL
- URL
- processed: contains generated TSV and JSONLINE files
- URL
- URL
- URL
- URL
- URL
- URL
- [and other variants]
# Credits
- Special thanks to WikiLingua authors: URL
- Article provided by <a href="URL target="_blank">wikiHow</a>, a wiki that is building the world's largest and highest quality how-to manual. Please edit this article and find author credits at the original wikiHow article on How to Tie a Tie. Content on wikiHow can be shared under a <a href="URL target="_blank">Creative Commons License</a>.
| [
"# viWikiHow-Abs-Sum\nA dataset for Vietnamese Abstractive Summarization task.\nIt includes all Vietnamese posts from WikiHow which was released in WikiLingua dataset.",
"# Introduction\nThis dataset was extracted from Train/Test split of WikiLingua dataset. As the target language is Vietnamese, we remove all other files, just keep train.\\*.vi, test.\\*.vi, and val.\\*.vi for Vietnamese Abstractive Summarization task. The raw files then are stored in the *raw* director and after that, we run the python script to generate ready-to-use data files in TSV and JSONLINE formats which are stored in *processed* directory to be easily used for future training scripts.",
"# Directory structure\n- raw: contains raw text files from WikiLingua\n - URL\n - URL\n - URL\n - URL\n - URL\n - URL\n- processed: contains generated TSV and JSONLINE files\n - URL\n - URL\n - URL\n - URL\n - URL\n - URL\n - [and other variants]",
"# Credits\n- Special thanks to WikiLingua authors: URL\n- Article provided by <a href=\"URL target=\"_blank\">wikiHow</a>, a wiki that is building the world's largest and highest quality how-to manual. Please edit this article and find author credits at the original wikiHow article on How to Tie a Tie. Content on wikiHow can be shared under a <a href=\"URL target=\"_blank\">Creative Commons License</a>."
] | [
"TAGS\n#region-us \n",
"# viWikiHow-Abs-Sum\nA dataset for Vietnamese Abstractive Summarization task.\nIt includes all Vietnamese posts from WikiHow which was released in WikiLingua dataset.",
"# Introduction\nThis dataset was extracted from Train/Test split of WikiLingua dataset. As the target language is Vietnamese, we remove all other files, just keep train.\\*.vi, test.\\*.vi, and val.\\*.vi for Vietnamese Abstractive Summarization task. The raw files then are stored in the *raw* director and after that, we run the python script to generate ready-to-use data files in TSV and JSONLINE formats which are stored in *processed* directory to be easily used for future training scripts.",
"# Directory structure\n- raw: contains raw text files from WikiLingua\n - URL\n - URL\n - URL\n - URL\n - URL\n - URL\n- processed: contains generated TSV and JSONLINE files\n - URL\n - URL\n - URL\n - URL\n - URL\n - URL\n - [and other variants]",
"# Credits\n- Special thanks to WikiLingua authors: URL\n- Article provided by <a href=\"URL target=\"_blank\">wikiHow</a>, a wiki that is building the world's largest and highest quality how-to manual. Please edit this article and find author credits at the original wikiHow article on How to Tie a Tie. Content on wikiHow can be shared under a <a href=\"URL target=\"_blank\">Creative Commons License</a>."
] |
be7a8a072e974e015b08309f1b3df244d54f3b2c | # Dataset Card for "dataset_readmes"
[More Information needed](https://github.com/huggingface/datasets/blob/main/CONTRIBUTING.md#how-to-contribute-to-the-dataset-cards) | davanstrien/dataset_readmes | [
"region:us"
] | 2022-11-16T21:16:16+00:00 | {"dataset_info": {"features": [{"name": "author", "dtype": "string"}, {"name": "cardData", "dtype": "null"}, {"name": "citation", "dtype": "string"}, {"name": "description", "dtype": "string"}, {"name": "disabled", "dtype": "bool"}, {"name": "downloads", "dtype": "float64"}, {"name": "gated", "dtype": "bool"}, {"name": "id", "dtype": "string"}, {"name": "lastModified", "dtype": "string"}, {"name": "paperswithcode_id", "dtype": "string"}, {"name": "private", "dtype": "bool"}, {"name": "sha", "dtype": "string"}, {"name": "siblings", "sequence": "null"}, {"name": "tags", "sequence": "string"}, {"name": "readme_url", "dtype": "string"}, {"name": "readme", "dtype": "string"}], "splits": [{"name": "train", "num_bytes": 30248502, "num_examples": 7356}], "download_size": 9717727, "dataset_size": 30248502}} | 2022-11-16T21:16:19+00:00 | [] | [] | TAGS
#region-us
| # Dataset Card for "dataset_readmes"
More Information needed | [
"# Dataset Card for \"dataset_readmes\"\n\nMore Information needed"
] | [
"TAGS\n#region-us \n",
"# Dataset Card for \"dataset_readmes\"\n\nMore Information needed"
] |
f9c6c6198b775072d90d5d00fd3b01c1d18beba1 | # Dataset Card for "nn_to_hero"
[More Information needed](https://github.com/huggingface/datasets/blob/main/CONTRIBUTING.md#how-to-contribute-to-the-dataset-cards) | osanseviero/nn_to_hero | [
"whisper",
"region:us"
] | 2022-11-16T21:31:56+00:00 | {"tags": ["whisper"], "dataset_info": {"features": [{"name": "CHANNEL_NAME", "dtype": "string"}, {"name": "URL", "dtype": "string"}, {"name": "TITLE", "dtype": "string"}, {"name": "DESCRIPTION", "dtype": "string"}, {"name": "TRANSCRIPTION", "dtype": "string"}, {"name": "SEGMENTS", "dtype": "string"}], "splits": [{"name": "train", "num_bytes": 1673867, "num_examples": 12}], "download_size": 765920, "dataset_size": 1673867}} | 2022-11-16T21:48:03+00:00 | [] | [] | TAGS
#whisper #region-us
| # Dataset Card for "nn_to_hero"
More Information needed | [
"# Dataset Card for \"nn_to_hero\"\n\nMore Information needed"
] | [
"TAGS\n#whisper #region-us \n",
"# Dataset Card for \"nn_to_hero\"\n\nMore Information needed"
] |
dec17e9391b767791e3808a655654467605a9d49 |
# Dataset Card for Twitter US Airline Sentiment
## Table of Contents
- [Table of Contents](#table-of-contents)
- [Dataset Description](#dataset-description)
- [Dataset Summary](#dataset-summary)
- [Supported Tasks and Leaderboards](#supported-tasks-and-leaderboards)
- [Languages](#languages)
- [Dataset Structure](#dataset-structure)
- [Data Instances](#data-instances)
- [Data Fields](#data-fields)
- [Data Splits](#data-splits)
- [Dataset Creation](#dataset-creation)
- [Curation Rationale](#curation-rationale)
- [Source Data](#source-data)
- [Annotations](#annotations)
- [Personal and Sensitive Information](#personal-and-sensitive-information)
- [Considerations for Using the Data](#considerations-for-using-the-data)
- [Social Impact of Dataset](#social-impact-of-dataset)
- [Discussion of Biases](#discussion-of-biases)
- [Other Known Limitations](#other-known-limitations)
- [Additional Information](#additional-information)
- [Dataset Curators](#dataset-curators)
- [Licensing Information](#licensing-information)
- [Citation Information](#citation-information)
- [Contributions](#contributions)
## Dataset Description
- **Homepage:** https://kaggle.com/datasets/crowdflower/twitter-airline-sentiment
- **Repository:**
- **Paper:**
- **Leaderboard:**
- **Point of Contact:**
### Dataset Summary
*This data originally came from [Crowdflower's Data for Everyone library](http://www.crowdflower.com/data-for-everyone).*
As the original source says,
> A sentiment analysis job about the problems of each major U.S. airline. Twitter data was scraped from February of 2015 and contributors were asked to first classify positive, negative, and neutral tweets, followed by categorizing negative reasons (such as "late flight" or "rude service").
The data we're providing on Kaggle is a slightly reformatted version of the original source. It includes both a CSV file and SQLite database. The code that does these transformations is [available on GitHub](https://github.com/benhamner/crowdflower-airline-twitter-sentiment)
For example, it contains whether the sentiment of the tweets in this set was positive, neutral, or negative for six US airlines:
[](https://www.kaggle.com/benhamner/d/crowdflower/twitter-airline-sentiment/exploring-airline-twitter-sentiment-data)
### Supported Tasks and Leaderboards
[More Information Needed]
### Languages
[More Information Needed]
## Dataset Structure
### Data Instances
[More Information Needed]
### Data Fields
[More Information Needed]
### Data Splits
[More Information Needed]
## Dataset Creation
### Curation Rationale
[More Information Needed]
### Source Data
#### Initial Data Collection and Normalization
[More Information Needed]
#### Who are the source language producers?
[More Information Needed]
### Annotations
#### Annotation process
[More Information Needed]
#### Who are the annotators?
[More Information Needed]
### Personal and Sensitive Information
[More Information Needed]
## Considerations for Using the Data
### Social Impact of Dataset
[More Information Needed]
### Discussion of Biases
[More Information Needed]
### Other Known Limitations
[More Information Needed]
## Additional Information
### Dataset Curators
This dataset was shared by [@crowdflower](https://kaggle.com/crowdflower)
### Licensing Information
The license for this dataset is cc-by-nc-sa-4.0
### Citation Information
```bibtex
[More Information Needed]
```
### Contributions
[More Information Needed] | osanseviero/twitter-airline-sentiment | [
"license:cc-by-nc-sa-4.0",
"region:us"
] | 2022-11-16T22:31:43+00:00 | {"license": ["cc-by-nc-sa-4.0"], "converted_from": "kaggle", "kaggle_id": "crowdflower/twitter-airline-sentiment"} | 2022-11-16T22:31:48+00:00 | [] | [] | TAGS
#license-cc-by-nc-sa-4.0 #region-us
|
# Dataset Card for Twitter US Airline Sentiment
## Table of Contents
- Table of Contents
- Dataset Description
- Dataset Summary
- Supported Tasks and Leaderboards
- Languages
- Dataset Structure
- Data Instances
- Data Fields
- Data Splits
- Dataset Creation
- Curation Rationale
- Source Data
- Annotations
- Personal and Sensitive Information
- Considerations for Using the Data
- Social Impact of Dataset
- Discussion of Biases
- Other Known Limitations
- Additional Information
- Dataset Curators
- Licensing Information
- Citation Information
- Contributions
## Dataset Description
- Homepage: URL
- Repository:
- Paper:
- Leaderboard:
- Point of Contact:
### Dataset Summary
*This data originally came from Crowdflower's Data for Everyone library.*
As the original source says,
> A sentiment analysis job about the problems of each major U.S. airline. Twitter data was scraped from February of 2015 and contributors were asked to first classify positive, negative, and neutral tweets, followed by categorizing negative reasons (such as "late flight" or "rude service").
The data we're providing on Kaggle is a slightly reformatted version of the original source. It includes both a CSV file and SQLite database. The code that does these transformations is available on GitHub
For example, it contains whether the sentiment of the tweets in this set was positive, neutral, or negative for six US airlines:
.\n\nThe data we're providing on Kaggle is a slightly reformatted version of the original source. It includes both a CSV file and SQLite database. The code that does these transformations is available on GitHub\n\nFor example, it contains whether the sentiment of the tweets in this set was positive, neutral, or negative for six US airlines:\n\n.\n\nThe data we're providing on Kaggle is a slightly reformatted version of the original source. It includes both a CSV file and SQLite database. The code that does these transformations is available on GitHub\n\nFor example, it contains whether the sentiment of the tweets in this set was positive, neutral, or negative for six US airlines:\n\n | ak8618/my-image-captioning-dataset | [
"region:us"
] | 2022-11-17T00:14:11+00:00 | {"dataset_info": {"features": [{"name": "image", "dtype": "image"}, {"name": "text", "dtype": "string"}], "splits": [{"name": "train", "num_bytes": 182262.0, "num_examples": 3}], "download_size": 164273, "dataset_size": 182262.0}} | 2022-11-17T00:14:17+00:00 | [] | [] | TAGS
#region-us
| # Dataset Card for "my-image-captioning-dataset"
More Information needed | [
"# Dataset Card for \"my-image-captioning-dataset\"\n\nMore Information needed"
] | [
"TAGS\n#region-us \n",
"# Dataset Card for \"my-image-captioning-dataset\"\n\nMore Information needed"
] |
1498ecae7c86e1a50efc2003d3d613483cb410c2 | # Dataset Card for "my-image-captioning-dataset1"
[More Information needed](https://github.com/huggingface/datasets/blob/main/CONTRIBUTING.md#how-to-contribute-to-the-dataset-cards) | ak8618/my-image-captioning-dataset1 | [
"region:us"
] | 2022-11-17T00:23:11+00:00 | {"dataset_info": {"features": [{"name": "image", "dtype": "image"}, {"name": "text", "dtype": "string"}], "splits": [{"name": "train", "num_bytes": 171096.0, "num_examples": 3}], "download_size": 163572, "dataset_size": 171096.0}} | 2022-11-17T00:23:16+00:00 | [] | [] | TAGS
#region-us
| # Dataset Card for "my-image-captioning-dataset1"
More Information needed | [
"# Dataset Card for \"my-image-captioning-dataset1\"\n\nMore Information needed"
] | [
"TAGS\n#region-us \n",
"# Dataset Card for \"my-image-captioning-dataset1\"\n\nMore Information needed"
] |
d38a96426497e3b2a8643e86183fd575e09da88a | # Dataset Card for AutoTrain Evaluator
This repository contains model predictions generated by [AutoTrain](https://huggingface.co/autotrain) for the following task and dataset:
* Task: Token Classification
* Model: 51la5/bert-large-NER
* Dataset: conll2003
* Config: conll2003
* Split: test
To run new evaluation jobs, visit Hugging Face's [automatic model evaluator](https://huggingface.co/spaces/autoevaluate/model-evaluator).
## Contributions
Thanks to [@aniketrawat97](https://huggingface.co/aniketrawat97) for evaluating this model. | autoevaluate/autoeval-eval-conll2003-conll2003-c67e3d-2126868713 | [
"autotrain",
"evaluation",
"region:us"
] | 2022-11-17T01:35:59+00:00 | {"type": "predictions", "tags": ["autotrain", "evaluation"], "datasets": ["conll2003"], "eval_info": {"task": "entity_extraction", "model": "51la5/bert-large-NER", "metrics": [], "dataset_name": "conll2003", "dataset_config": "conll2003", "dataset_split": "test", "col_mapping": {"tokens": "tokens", "tags": "ner_tags"}}} | 2022-11-17T01:38:57+00:00 | [] | [] | TAGS
#autotrain #evaluation #region-us
| # Dataset Card for AutoTrain Evaluator
This repository contains model predictions generated by AutoTrain for the following task and dataset:
* Task: Token Classification
* Model: 51la5/bert-large-NER
* Dataset: conll2003
* Config: conll2003
* Split: test
To run new evaluation jobs, visit Hugging Face's automatic model evaluator.
## Contributions
Thanks to @aniketrawat97 for evaluating this model. | [
"# Dataset Card for AutoTrain Evaluator\n\nThis repository contains model predictions generated by AutoTrain for the following task and dataset:\n\n* Task: Token Classification\n* Model: 51la5/bert-large-NER\n* Dataset: conll2003\n* Config: conll2003\n* Split: test\n\nTo run new evaluation jobs, visit Hugging Face's automatic model evaluator.",
"## Contributions\n\nThanks to @aniketrawat97 for evaluating this model."
] | [
"TAGS\n#autotrain #evaluation #region-us \n",
"# Dataset Card for AutoTrain Evaluator\n\nThis repository contains model predictions generated by AutoTrain for the following task and dataset:\n\n* Task: Token Classification\n* Model: 51la5/bert-large-NER\n* Dataset: conll2003\n* Config: conll2003\n* Split: test\n\nTo run new evaluation jobs, visit Hugging Face's automatic model evaluator.",
"## Contributions\n\nThanks to @aniketrawat97 for evaluating this model."
] |
0243ab65168e9f9e2bdda0f201b43b4f84774561 | # Dataset Card for AutoTrain Evaluator
This repository contains model predictions generated by [AutoTrain](https://huggingface.co/autotrain) for the following task and dataset:
* Task: Token Classification
* Model: 51la5/distilbert-base-NER
* Dataset: conll2003
* Config: conll2003
* Split: test
To run new evaluation jobs, visit Hugging Face's [automatic model evaluator](https://huggingface.co/spaces/autoevaluate/model-evaluator).
## Contributions
Thanks to [@aniketrawat97](https://huggingface.co/aniketrawat97) for evaluating this model. | autoevaluate/autoeval-eval-conll2003-conll2003-c67e3d-2126868714 | [
"autotrain",
"evaluation",
"region:us"
] | 2022-11-17T01:36:05+00:00 | {"type": "predictions", "tags": ["autotrain", "evaluation"], "datasets": ["conll2003"], "eval_info": {"task": "entity_extraction", "model": "51la5/distilbert-base-NER", "metrics": [], "dataset_name": "conll2003", "dataset_config": "conll2003", "dataset_split": "test", "col_mapping": {"tokens": "tokens", "tags": "ner_tags"}}} | 2022-11-17T01:37:13+00:00 | [] | [] | TAGS
#autotrain #evaluation #region-us
| # Dataset Card for AutoTrain Evaluator
This repository contains model predictions generated by AutoTrain for the following task and dataset:
* Task: Token Classification
* Model: 51la5/distilbert-base-NER
* Dataset: conll2003
* Config: conll2003
* Split: test
To run new evaluation jobs, visit Hugging Face's automatic model evaluator.
## Contributions
Thanks to @aniketrawat97 for evaluating this model. | [
"# Dataset Card for AutoTrain Evaluator\n\nThis repository contains model predictions generated by AutoTrain for the following task and dataset:\n\n* Task: Token Classification\n* Model: 51la5/distilbert-base-NER\n* Dataset: conll2003\n* Config: conll2003\n* Split: test\n\nTo run new evaluation jobs, visit Hugging Face's automatic model evaluator.",
"## Contributions\n\nThanks to @aniketrawat97 for evaluating this model."
] | [
"TAGS\n#autotrain #evaluation #region-us \n",
"# Dataset Card for AutoTrain Evaluator\n\nThis repository contains model predictions generated by AutoTrain for the following task and dataset:\n\n* Task: Token Classification\n* Model: 51la5/distilbert-base-NER\n* Dataset: conll2003\n* Config: conll2003\n* Split: test\n\nTo run new evaluation jobs, visit Hugging Face's automatic model evaluator.",
"## Contributions\n\nThanks to @aniketrawat97 for evaluating this model."
] |
c3443fae8da8cc473b1f1b6ced73ae07b7d14529 |
# IMaSC: ICFOSS Malayalam Speech Corpus
**IMaSC** is a Malayalam text and speech corpus made available by [ICFOSS](https://icfoss.in/) for the purpose of developing speech technology for Malayalam, particularly text-to-speech. The corpus contains 34,473 text-audio pairs of Malayalam sentences spoken by 8 speakers, totalling in approximately 50 hours of audio.
## Dataset Description
- **Paper:** [IMaSC — ICFOSS Malayalam Speech Corpus](https://arxiv.org/abs/2211.12796)
- **Point of Contact:** [Thennal D K](mailto:[email protected])
## Dataset Structure
The dataset consists of 34,473 instances with fields `text`, `speaker`, and `audio`. The audio is mono, sampled at 16kH. The transcription is normalized and only includes Malayalam characters and common punctuation. The table given below specifies how the 34,473 instances are split between the speakers, along with some basic speaker info:
| Speaker | Gender | Age | Time (HH:MM:SS) | Sentences |
| --- | --- | --- | --- | --- |
| Joji | Male | 28 | 06:08:55 | 4,332 |
| Sonia | Female | 43 | 05:22:39 | 4,294 |
| Jijo | Male | 26 | 05:34:05 | 4,093 |
| Greeshma | Female | 22 | 06:32:39 | 4,416 |
| Anil | Male | 48 | 05:58:34 | 4,239 |
| Vidhya | Female | 23 | 04:21:56 | 3,242 |
| Sonu | Male | 25 | 06:04:43 | 4,219 |
| Simla | Female | 24 | 09:34:21 | 5,638 |
| **Total** | | | **49:37:54** | **34,473** |
### Data Instances
An example instance is given below:
```json
{'text': 'സർവ്വകലാശാല വൈസ് ചാൻസലർ ഡോ. ചന്ദ്രബാബുവിനും സംഭവം തലവേദനയാവുകയാണ്',
'speaker': 'Sonia',
'audio': {'path': None,
'array': array([ 0.00921631, 0.00930786, 0.00939941, ..., -0.00497437,
-0.00497437, -0.00497437]),
'sampling_rate': 16000}}
```
### Data Fields
- **text** (str): Transcription of the audio file
- **speaker** (str): The name of the speaker
- **audio** (dict): Audio object including loaded audio array, sampling rate and path to audio (always None)
### Data Splits
We provide all the data in a single `train` split. The loaded dataset object thus looks like this:
```json
DatasetDict({
train: Dataset({
features: ['text', 'speaker', 'audio'],
num_rows: 34473
})
})
```
### Dataset Creation
The text is sourced from [Malayalam Wikipedia](https://ml.wikipedia.org), and read by our speakers in studio conditions. Extensive error correction was conducted to provide a clean, accurate database. Further details are given in our paper, accessible at [https://arxiv.org/abs/2211.12796](https://arxiv.org/abs/2211.12796).
## Additional Information
### Licensing
The corpus is made available under the [Creative Commons license (CC BY-SA 4.0)](https://creativecommons.org/licenses/by-sa/4.0/).
### Citation
```
@misc{gopinath2022imasc,
title={IMaSC -- ICFOSS Malayalam Speech Corpus},
author={Deepa P Gopinath and Thennal D K and Vrinda V Nair and Swaraj K S and Sachin G},
year={2022},
eprint={2211.12796},
archivePrefix={arXiv},
primaryClass={cs.SD}
}
```
| thennal/IMaSC | [
"task_categories:text-to-speech",
"task_categories:automatic-speech-recognition",
"annotations_creators:expert-generated",
"language_creators:found",
"multilinguality:monolingual",
"size_categories:10K<n<100K",
"source_datasets:original",
"language:ml",
"license:cc-by-sa-4.0",
"arxiv:2211.12796",
"region:us"
] | 2022-11-17T05:16:00+00:00 | {"annotations_creators": ["expert-generated"], "language_creators": ["found"], "language": ["ml"], "license": ["cc-by-sa-4.0"], "multilinguality": ["monolingual"], "size_categories": ["10K<n<100K"], "source_datasets": ["original"], "task_categories": ["text-to-speech", "automatic-speech-recognition"], "task_ids": [], "pretty_name": "ICFOSS Malayalam Speech Corpus", "tags": []} | 2022-12-08T17:21:02+00:00 | [
"2211.12796"
] | [
"ml"
] | TAGS
#task_categories-text-to-speech #task_categories-automatic-speech-recognition #annotations_creators-expert-generated #language_creators-found #multilinguality-monolingual #size_categories-10K<n<100K #source_datasets-original #language-Malayalam #license-cc-by-sa-4.0 #arxiv-2211.12796 #region-us
| IMaSC: ICFOSS Malayalam Speech Corpus
=====================================
IMaSC is a Malayalam text and speech corpus made available by ICFOSS for the purpose of developing speech technology for Malayalam, particularly text-to-speech. The corpus contains 34,473 text-audio pairs of Malayalam sentences spoken by 8 speakers, totalling in approximately 50 hours of audio.
Dataset Description
-------------------
* Paper: IMaSC — ICFOSS Malayalam Speech Corpus
* Point of Contact: Thennal D K
Dataset Structure
-----------------
The dataset consists of 34,473 instances with fields 'text', 'speaker', and 'audio'. The audio is mono, sampled at 16kH. The transcription is normalized and only includes Malayalam characters and common punctuation. The table given below specifies how the 34,473 instances are split between the speakers, along with some basic speaker info:
### Data Instances
An example instance is given below:
### Data Fields
* text (str): Transcription of the audio file
* speaker (str): The name of the speaker
* audio (dict): Audio object including loaded audio array, sampling rate and path to audio (always None)
### Data Splits
We provide all the data in a single 'train' split. The loaded dataset object thus looks like this:
### Dataset Creation
The text is sourced from Malayalam Wikipedia, and read by our speakers in studio conditions. Extensive error correction was conducted to provide a clean, accurate database. Further details are given in our paper, accessible at URL
Additional Information
----------------------
### Licensing
The corpus is made available under the Creative Commons license (CC BY-SA 4.0).
| [
"### Data Instances\n\n\nAn example instance is given below:",
"### Data Fields\n\n\n* text (str): Transcription of the audio file\n* speaker (str): The name of the speaker\n* audio (dict): Audio object including loaded audio array, sampling rate and path to audio (always None)",
"### Data Splits\n\n\nWe provide all the data in a single 'train' split. The loaded dataset object thus looks like this:",
"### Dataset Creation\n\n\nThe text is sourced from Malayalam Wikipedia, and read by our speakers in studio conditions. Extensive error correction was conducted to provide a clean, accurate database. Further details are given in our paper, accessible at URL\n\n\nAdditional Information\n----------------------",
"### Licensing\n\n\nThe corpus is made available under the Creative Commons license (CC BY-SA 4.0)."
] | [
"TAGS\n#task_categories-text-to-speech #task_categories-automatic-speech-recognition #annotations_creators-expert-generated #language_creators-found #multilinguality-monolingual #size_categories-10K<n<100K #source_datasets-original #language-Malayalam #license-cc-by-sa-4.0 #arxiv-2211.12796 #region-us \n",
"### Data Instances\n\n\nAn example instance is given below:",
"### Data Fields\n\n\n* text (str): Transcription of the audio file\n* speaker (str): The name of the speaker\n* audio (dict): Audio object including loaded audio array, sampling rate and path to audio (always None)",
"### Data Splits\n\n\nWe provide all the data in a single 'train' split. The loaded dataset object thus looks like this:",
"### Dataset Creation\n\n\nThe text is sourced from Malayalam Wikipedia, and read by our speakers in studio conditions. Extensive error correction was conducted to provide a clean, accurate database. Further details are given in our paper, accessible at URL\n\n\nAdditional Information\n----------------------",
"### Licensing\n\n\nThe corpus is made available under the Creative Commons license (CC BY-SA 4.0)."
] |
52cc3f9653a75e6b972a3e8be232554b405569cd | file:///home/mzhh/%E6%A1%8C%E9%9D%A2/DFL_Me2.55/_internal/core
file:///home/mzhh/%E6%A1%8C%E9%9D%A2/DFL_Me2.55/_internal/DFLIMG
file:///home/mzhh/%E6%A1%8C%E9%9D%A2/DFL_Me2.55/_internal/doc
file:///home/mzhh/%E6%A1%8C%E9%9D%A2/DFL_Me2.55/_internal/facelib
file:///home/mzhh/%E6%A1%8C%E9%9D%A2/DFL_Me2.55/_internal/flaskr
file:///home/mzhh/%E6%A1%8C%E9%9D%A2/DFL_Me2.55/_internal/localization
file:///home/mzhh/%E6%A1%8C%E9%9D%A2/DFL_Me2.55/_internal/mainscripts
file:///home/mzhh/%E6%A1%8C%E9%9D%A2/DFL_Me2.55/_internal/merger
file:///home/mzhh/%E6%A1%8C%E9%9D%A2/DFL_Me2.55/_internal/models
file:///home/mzhh/%E6%A1%8C%E9%9D%A2/DFL_Me2.55/_internal/samplelib
file:///home/mzhh/%E6%A1%8C%E9%9D%A2/DFL_Me2.55/_internal/utils
file:///home/mzhh/%E6%A1%8C%E9%9D%A2/DFL_Me2.55/_internal/XSegEditor
file:///home/mzhh/%E6%A1%8C%E9%9D%A2/DFL_Me2.55/_internal/DeepfacelabMe%202022%20Sp1_Ver2.55.txt
file:///home/mzhh/%E6%A1%8C%E9%9D%A2/DFL_Me2.55/_internal/DeepfacelabMe%202022%20Sp1_Ver2.55_p1.txt
file:///home/mzhh/%E6%A1%8C%E9%9D%A2/DFL_Me2.55/_internal/environment.yml
file:///home/mzhh/%E6%A1%8C%E9%9D%A2/DFL_Me2.55/_internal/main.py
file:///home/mzhh/%E6%A1%8C%E9%9D%A2/DFL_Me2.55/_internal/requirements-colab.txt
file:///home/mzhh/%E6%A1%8C%E9%9D%A2/DFL_Me2.55/_internal/requirements-cuda.txt
file:///home/mzhh/%E6%A1%8C%E9%9D%A2/DFL_Me2.55/_internal/core
file:///home/mzhh/%E6%A1%8C%E9%9D%A2/DFL_Me2.55/_internal/DFLIMG
file:///home/mzhh/%E6%A1%8C%E9%9D%A2/DFL_Me2.55/_internal/doc
file:///home/mzhh/%E6%A1%8C%E9%9D%A2/DFL_Me2.55/_internal/facelib
file:///home/mzhh/%E6%A1%8C%E9%9D%A2/DFL_Me2.55/_internal/flaskr
file:///home/mzhh/%E6%A1%8C%E9%9D%A2/DFL_Me2.55/_internal/localization
file:///home/mzhh/%E6%A1%8C%E9%9D%A2/DFL_Me2.55/_internal/mainscripts
file:///home/mzhh/%E6%A1%8C%E9%9D%A2/DFL_Me2.55/_internal/merger
file:///home/mzhh/%E6%A1%8C%E9%9D%A2/DFL_Me2.55/_internal/models
file:///home/mzhh/%E6%A1%8C%E9%9D%A2/DFL_Me2.55/_internal/samplelib
file:///home/mzhh/%E6%A1%8C%E9%9D%A2/DFL_Me2.55/_internal/utils
file:///home/mzhh/%E6%A1%8C%E9%9D%A2/DFL_Me2.55/_internal/XSegEditor
file:///home/mzhh/%E6%A1%8C%E9%9D%A2/DFL_Me2.55/_internal/DeepfacelabMe%202022%20Sp1_Ver2.55.txt
file:///home/mzhh/%E6%A1%8C%E9%9D%A2/DFL_Me2.55/_internal/DeepfacelabMe%202022%20Sp1_Ver2.55_p1.txt
file:///home/mzhh/%E6%A1%8C%E9%9D%A2/DFL_Me2.55/_internal/environment.yml
file:///home/mzhh/%E6%A1%8C%E9%9D%A2/DFL_Me2.55/_internal/main.py
file:///home/mzhh/%E6%A1%8C%E9%9D%A2/DFL_Me2.55/_internal/requirements-colab.txt
file:///home/mzhh/%E6%A1%8C%E9%9D%A2/DFL_Me2.55/_internal/requirements-cuda.txt
file:///home/mzhh/%E6%A1%8C%E9%9D%A2/DFL_Me2.55/_internal/core
file:///home/mzhh/%E6%A1%8C%E9%9D%A2/DFL_Me2.55/_internal/DFLIMG
file:///home/mzhh/%E6%A1%8C%E9%9D%A2/DFL_Me2.55/_internal/doc
file:///home/mzhh/%E6%A1%8C%E9%9D%A2/DFL_Me2.55/_internal/facelib
file:///home/mzhh/%E6%A1%8C%E9%9D%A2/DFL_Me2.55/_internal/flaskr
file:///home/mzhh/%E6%A1%8C%E9%9D%A2/DFL_Me2.55/_internal/localization
file:///home/mzhh/%E6%A1%8C%E9%9D%A2/DFL_Me2.55/_internal/mainscripts
file:///home/mzhh/%E6%A1%8C%E9%9D%A2/DFL_Me2.55/_internal/merger
file:///home/mzhh/%E6%A1%8C%E9%9D%A2/DFL_Me2.55/_internal/models
file:///home/mzhh/%E6%A1%8C%E9%9D%A2/DFL_Me2.55/_internal/samplelib
file:///home/mzhh/%E6%A1%8C%E9%9D%A2/DFL_Me2.55/_internal/utils
file:///home/mzhh/%E6%A1%8C%E9%9D%A2/DFL_Me2.55/_internal/XSegEditor
file:///home/mzhh/%E6%A1%8C%E9%9D%A2/DFL_Me2.55/_internal/DeepfacelabMe%202022%20Sp1_Ver2.55.txt
file:///home/mzhh/%E6%A1%8C%E9%9D%A2/DFL_Me2.55/_internal/DeepfacelabMe%202022%20Sp1_Ver2.55_p1.txt
file:///home/mzhh/%E6%A1%8C%E9%9D%A2/DFL_Me2.55/_internal/environment.yml
file:///home/mzhh/%E6%A1%8C%E9%9D%A2/DFL_Me2.55/_internal/main.py
file:///home/mzhh/%E6%A1%8C%E9%9D%A2/DFL_Me2.55/_internal/requirements-colab.txt
file:///home/mzhh/%E6%A1%8C%E9%9D%A2/DFL_Me2.55/_internal/requirements-cuda.txt
file:///home/mzhh/%E6%A1%8C%E9%9D%A2/DFL_Me2.55/_internal/core
file:///home/mzhh/%E6%A1%8C%E9%9D%A2/DFL_Me2.55/_internal/DFLIMG
file:///home/mzhh/%E6%A1%8C%E9%9D%A2/DFL_Me2.55/_internal/doc
file:///home/mzhh/%E6%A1%8C%E9%9D%A2/DFL_Me2.55/_internal/facelib
file:///home/mzhh/%E6%A1%8C%E9%9D%A2/DFL_Me2.55/_internal/flaskr
file:///home/mzhh/%E6%A1%8C%E9%9D%A2/DFL_Me2.55/_internal/localization
file:///home/mzhh/%E6%A1%8C%E9%9D%A2/DFL_Me2.55/_internal/mainscripts
file:///home/mzhh/%E6%A1%8C%E9%9D%A2/DFL_Me2.55/_internal/merger
file:///home/mzhh/%E6%A1%8C%E9%9D%A2/DFL_Me2.55/_internal/models
file:///home/mzhh/%E6%A1%8C%E9%9D%A2/DFL_Me2.55/_internal/samplelib
file:///home/mzhh/%E6%A1%8C%E9%9D%A2/DFL_Me2.55/_internal/utils
file:///home/mzhh/%E6%A1%8C%E9%9D%A2/DFL_Me2.55/_internal/XSegEditor
file:///home/mzhh/%E6%A1%8C%E9%9D%A2/DFL_Me2.55/_internal/DeepfacelabMe%202022%20Sp1_Ver2.55.txt
file:///home/mzhh/%E6%A1%8C%E9%9D%A2/DFL_Me2.55/_internal/DeepfacelabMe%202022%20Sp1_Ver2.55_p1.txt
file:///home/mzhh/%E6%A1%8C%E9%9D%A2/DFL_Me2.55/_internal/environment.yml
file:///home/mzhh/%E6%A1%8C%E9%9D%A2/DFL_Me2.55/_internal/main.py
file:///home/mzhh/%E6%A1%8C%E9%9D%A2/DFL_Me2.55/_internal/requirements-colab.txt
file:///home/mzhh/%E6%A1%8C%E9%9D%A2/DFL_Me2.55/_internal/requirements-cuda.txt
file:///home/mzhh/%E6%A1%8C%E9%9D%A2/DFL_Me2.55/_internal/core
file:///home/mzhh/%E6%A1%8C%E9%9D%A2/DFL_Me2.55/_internal/DFLIMG
file:///home/mzhh/%E6%A1%8C%E9%9D%A2/DFL_Me2.55/_internal/doc
file:///home/mzhh/%E6%A1%8C%E9%9D%A2/DFL_Me2.55/_internal/facelib
file:///home/mzhh/%E6%A1%8C%E9%9D%A2/DFL_Me2.55/_internal/flaskr
file:///home/mzhh/%E6%A1%8C%E9%9D%A2/DFL_Me2.55/_internal/localization
file:///home/mzhh/%E6%A1%8C%E9%9D%A2/DFL_Me2.55/_internal/mainscripts
file:///home/mzhh/%E6%A1%8C%E9%9D%A2/DFL_Me2.55/_internal/merger
file:///home/mzhh/%E6%A1%8C%E9%9D%A2/DFL_Me2.55/_internal/models
file:///home/mzhh/%E6%A1%8C%E9%9D%A2/DFL_Me2.55/_internal/samplelib
file:///home/mzhh/%E6%A1%8C%E9%9D%A2/DFL_Me2.55/_internal/utils
file:///home/mzhh/%E6%A1%8C%E9%9D%A2/DFL_Me2.55/_internal/XSegEditor
file:///home/mzhh/%E6%A1%8C%E9%9D%A2/DFL_Me2.55/_internal/DeepfacelabMe%202022%20Sp1_Ver2.55.txt
file:///home/mzhh/%E6%A1%8C%E9%9D%A2/DFL_Me2.55/_internal/DeepfacelabMe%202022%20Sp1_Ver2.55_p1.txt
file:///home/mzhh/%E6%A1%8C%E9%9D%A2/DFL_Me2.55/_internal/environment.yml
file:///home/mzhh/%E6%A1%8C%E9%9D%A2/DFL_Me2.55/_internal/main.py
file:///home/mzhh/%E6%A1%8C%E9%9D%A2/DFL_Me2.55/_internal/requirements-colab.txt
file:///home/mzhh/%E6%A1%8C%E9%9D%A2/DFL_Me2.55/_internal/requirements-cuda.txt
file:///home/mzhh/%E6%A1%8C%E9%9D%A2/DFL_Me2.55/_internal/core
file:///home/mzhh/%E6%A1%8C%E9%9D%A2/DFL_Me2.55/_internal/DFLIMG
file:///home/mzhh/%E6%A1%8C%E9%9D%A2/DFL_Me2.55/_internal/doc
file:///home/mzhh/%E6%A1%8C%E9%9D%A2/DFL_Me2.55/_internal/facelib
file:///home/mzhh/%E6%A1%8C%E9%9D%A2/DFL_Me2.55/_internal/flaskr
file:///home/mzhh/%E6%A1%8C%E9%9D%A2/DFL_Me2.55/_internal/localization
file:///home/mzhh/%E6%A1%8C%E9%9D%A2/DFL_Me2.55/_internal/mainscripts
file:///home/mzhh/%E6%A1%8C%E9%9D%A2/DFL_Me2.55/_internal/merger
file:///home/mzhh/%E6%A1%8C%E9%9D%A2/DFL_Me2.55/_internal/models
file:///home/mzhh/%E6%A1%8C%E9%9D%A2/DFL_Me2.55/_internal/samplelib
file:///home/mzhh/%E6%A1%8C%E9%9D%A2/DFL_Me2.55/_internal/utils
file:///home/mzhh/%E6%A1%8C%E9%9D%A2/DFL_Me2.55/_internal/XSegEditor
file:///home/mzhh/%E6%A1%8C%E9%9D%A2/DFL_Me2.55/_internal/DeepfacelabMe%202022%20Sp1_Ver2.55.txt
file:///home/mzhh/%E6%A1%8C%E9%9D%A2/DFL_Me2.55/_internal/DeepfacelabMe%202022%20Sp1_Ver2.55_p1.txt
file:///home/mzhh/%E6%A1%8C%E9%9D%A2/DFL_Me2.55/_internal/environment.yml
file:///home/mzhh/%E6%A1%8C%E9%9D%A2/DFL_Me2.55/_internal/main.py
file:///home/mzhh/%E6%A1%8C%E9%9D%A2/DFL_Me2.55/_internal/requirements-colab.txt
file:///home/mzhh/%E6%A1%8C%E9%9D%A2/DFL_Me2.55/_internal/requirements-cuda.txt
file:///home/mzhh/%E6%A1%8C%E9%9D%A2/DFL_Me2.55/_internal/core
file:///home/mzhh/%E6%A1%8C%E9%9D%A2/DFL_Me2.55/_internal/DFLIMG
file:///home/mzhh/%E6%A1%8C%E9%9D%A2/DFL_Me2.55/_internal/doc
file:///home/mzhh/%E6%A1%8C%E9%9D%A2/DFL_Me2.55/_internal/facelib
file:///home/mzhh/%E6%A1%8C%E9%9D%A2/DFL_Me2.55/_internal/flaskr
file:///home/mzhh/%E6%A1%8C%E9%9D%A2/DFL_Me2.55/_internal/localization
file:///home/mzhh/%E6%A1%8C%E9%9D%A2/DFL_Me2.55/_internal/mainscripts
file:///home/mzhh/%E6%A1%8C%E9%9D%A2/DFL_Me2.55/_internal/merger
file:///home/mzhh/%E6%A1%8C%E9%9D%A2/DFL_Me2.55/_internal/models
file:///home/mzhh/%E6%A1%8C%E9%9D%A2/DFL_Me2.55/_internal/samplelib
file:///home/mzhh/%E6%A1%8C%E9%9D%A2/DFL_Me2.55/_internal/utils
file:///home/mzhh/%E6%A1%8C%E9%9D%A2/DFL_Me2.55/_internal/XSegEditor
file:///home/mzhh/%E6%A1%8C%E9%9D%A2/DFL_Me2.55/_internal/DeepfacelabMe%202022%20Sp1_Ver2.55.txt
file:///home/mzhh/%E6%A1%8C%E9%9D%A2/DFL_Me2.55/_internal/DeepfacelabMe%202022%20Sp1_Ver2.55_p1.txt
file:///home/mzhh/%E6%A1%8C%E9%9D%A2/DFL_Me2.55/_internal/environment.yml
file:///home/mzhh/%E6%A1%8C%E9%9D%A2/DFL_Me2.55/_internal/main.py
file:///home/mzhh/%E6%A1%8C%E9%9D%A2/DFL_Me2.55/_internal/requirements-colab.txt
file:///home/mzhh/%E6%A1%8C%E9%9D%A2/DFL_Me2.55/_internal/requirements-cuda.txt
file:///home/mzhh/%E6%A1%8C%E9%9D%A2/DFL_Me2.55/_internal/core
file:///home/mzhh/%E6%A1%8C%E9%9D%A2/DFL_Me2.55/_internal/DFLIMG
file:///home/mzhh/%E6%A1%8C%E9%9D%A2/DFL_Me2.55/_internal/doc
file:///home/mzhh/%E6%A1%8C%E9%9D%A2/DFL_Me2.55/_internal/facelib
file:///home/mzhh/%E6%A1%8C%E9%9D%A2/DFL_Me2.55/_internal/flaskr
file:///home/mzhh/%E6%A1%8C%E9%9D%A2/DFL_Me2.55/_internal/localization
file:///home/mzhh/%E6%A1%8C%E9%9D%A2/DFL_Me2.55/_internal/mainscripts
file:///home/mzhh/%E6%A1%8C%E9%9D%A2/DFL_Me2.55/_internal/merger
file:///home/mzhh/%E6%A1%8C%E9%9D%A2/DFL_Me2.55/_internal/models
file:///home/mzhh/%E6%A1%8C%E9%9D%A2/DFL_Me2.55/_internal/samplelib
file:///home/mzhh/%E6%A1%8C%E9%9D%A2/DFL_Me2.55/_internal/utils
file:///home/mzhh/%E6%A1%8C%E9%9D%A2/DFL_Me2.55/_internal/XSegEditor
file:///home/mzhh/%E6%A1%8C%E9%9D%A2/DFL_Me2.55/_internal/DeepfacelabMe%202022%20Sp1_Ver2.55.txt
file:///home/mzhh/%E6%A1%8C%E9%9D%A2/DFL_Me2.55/_internal/DeepfacelabMe%202022%20Sp1_Ver2.55_p1.txt
file:///home/mzhh/%E6%A1%8C%E9%9D%A2/DFL_Me2.55/_internal/environment.yml
file:///home/mzhh/%E6%A1%8C%E9%9D%A2/DFL_Me2.55/_internal/main.py
file:///home/mzhh/%E6%A1%8C%E9%9D%A2/DFL_Me2.55/_internal/requirements-colab.txt
file:///home/mzhh/%E6%A1%8C%E9%9D%A2/DFL_Me2.55/_internal/requirements-cuda.txt
file:///home/mzhh/%E6%A1%8C%E9%9D%A2/DFL_Me2.55/_internal/core
file:///home/mzhh/%E6%A1%8C%E9%9D%A2/DFL_Me2.55/_internal/DFLIMG
file:///home/mzhh/%E6%A1%8C%E9%9D%A2/DFL_Me2.55/_internal/doc
file:///home/mzhh/%E6%A1%8C%E9%9D%A2/DFL_Me2.55/_internal/facelib
file:///home/mzhh/%E6%A1%8C%E9%9D%A2/DFL_Me2.55/_internal/flaskr
file:///home/mzhh/%E6%A1%8C%E9%9D%A2/DFL_Me2.55/_internal/localization
file:///home/mzhh/%E6%A1%8C%E9%9D%A2/DFL_Me2.55/_internal/mainscripts
file:///home/mzhh/%E6%A1%8C%E9%9D%A2/DFL_Me2.55/_internal/merger
file:///home/mzhh/%E6%A1%8C%E9%9D%A2/DFL_Me2.55/_internal/models
file:///home/mzhh/%E6%A1%8C%E9%9D%A2/DFL_Me2.55/_internal/samplelib
file:///home/mzhh/%E6%A1%8C%E9%9D%A2/DFL_Me2.55/_internal/utils
file:///home/mzhh/%E6%A1%8C%E9%9D%A2/DFL_Me2.55/_internal/XSegEditor
file:///home/mzhh/%E6%A1%8C%E9%9D%A2/DFL_Me2.55/_internal/DeepfacelabMe%202022%20Sp1_Ver2.55.txt
file:///home/mzhh/%E6%A1%8C%E9%9D%A2/DFL_Me2.55/_internal/DeepfacelabMe%202022%20Sp1_Ver2.55_p1.txt
file:///home/mzhh/%E6%A1%8C%E9%9D%A2/DFL_Me2.55/_internal/environment.yml
file:///home/mzhh/%E6%A1%8C%E9%9D%A2/DFL_Me2.55/_internal/main.py
file:///home/mzhh/%E6%A1%8C%E9%9D%A2/DFL_Me2.55/_internal/requirements-colab.txt
file:///home/mzhh/%E6%A1%8C%E9%9D%A2/DFL_Me2.55/_internal/requirements-cuda.txt
file:///home/mzhh/%E6%A1%8C%E9%9D%A2/DFL_Me2.55/_internal/core
file:///home/mzhh/%E6%A1%8C%E9%9D%A2/DFL_Me2.55/_internal/DFLIMG
file:///home/mzhh/%E6%A1%8C%E9%9D%A2/DFL_Me2.55/_internal/doc
file:///home/mzhh/%E6%A1%8C%E9%9D%A2/DFL_Me2.55/_internal/facelib
file:///home/mzhh/%E6%A1%8C%E9%9D%A2/DFL_Me2.55/_internal/flaskr
file:///home/mzhh/%E6%A1%8C%E9%9D%A2/DFL_Me2.55/_internal/localization
file:///home/mzhh/%E6%A1%8C%E9%9D%A2/DFL_Me2.55/_internal/mainscripts
file:///home/mzhh/%E6%A1%8C%E9%9D%A2/DFL_Me2.55/_internal/merger
file:///home/mzhh/%E6%A1%8C%E9%9D%A2/DFL_Me2.55/_internal/models
file:///home/mzhh/%E6%A1%8C%E9%9D%A2/DFL_Me2.55/_internal/samplelib
file:///home/mzhh/%E6%A1%8C%E9%9D%A2/DFL_Me2.55/_internal/utils
file:///home/mzhh/%E6%A1%8C%E9%9D%A2/DFL_Me2.55/_internal/XSegEditor
file:///home/mzhh/%E6%A1%8C%E9%9D%A2/DFL_Me2.55/_internal/DeepfacelabMe%202022%20Sp1_Ver2.55.txt
file:///home/mzhh/%E6%A1%8C%E9%9D%A2/DFL_Me2.55/_internal/DeepfacelabMe%202022%20Sp1_Ver2.55_p1.txt
file:///home/mzhh/%E6%A1%8C%E9%9D%A2/DFL_Me2.55/_internal/environment.yml
file:///home/mzhh/%E6%A1%8C%E9%9D%A2/DFL_Me2.55/_internal/main.py
file:///home/mzhh/%E6%A1%8C%E9%9D%A2/DFL_Me2.55/_internal/requirements-colab.txt
file:///home/mzhh/%E6%A1%8C%E9%9D%A2/DFL_Me2.55/_internal/requirements-cuda.txt
file:///home/mzhh/%E6%A1%8C%E9%9D%A2/DFL_Me2.55/_internal/core
file:///home/mzhh/%E6%A1%8C%E9%9D%A2/DFL_Me2.55/_internal/DFLIMG
file:///home/mzhh/%E6%A1%8C%E9%9D%A2/DFL_Me2.55/_internal/doc
file:///home/mzhh/%E6%A1%8C%E9%9D%A2/DFL_Me2.55/_internal/facelib
file:///home/mzhh/%E6%A1%8C%E9%9D%A2/DFL_Me2.55/_internal/flaskr
file:///home/mzhh/%E6%A1%8C%E9%9D%A2/DFL_Me2.55/_internal/localization
file:///home/mzhh/%E6%A1%8C%E9%9D%A2/DFL_Me2.55/_internal/mainscripts
file:///home/mzhh/%E6%A1%8C%E9%9D%A2/DFL_Me2.55/_internal/merger
file:///home/mzhh/%E6%A1%8C%E9%9D%A2/DFL_Me2.55/_internal/models
file:///home/mzhh/%E6%A1%8C%E9%9D%A2/DFL_Me2.55/_internal/samplelib
file:///home/mzhh/%E6%A1%8C%E9%9D%A2/DFL_Me2.55/_internal/utils
file:///home/mzhh/%E6%A1%8C%E9%9D%A2/DFL_Me2.55/_internal/XSegEditor
file:///home/mzhh/%E6%A1%8C%E9%9D%A2/DFL_Me2.55/_internal/DeepfacelabMe%202022%20Sp1_Ver2.55.txt
file:///home/mzhh/%E6%A1%8C%E9%9D%A2/DFL_Me2.55/_internal/DeepfacelabMe%202022%20Sp1_Ver2.55_p1.txt
file:///home/mzhh/%E6%A1%8C%E9%9D%A2/DFL_Me2.55/_internal/environment.yml
file:///home/mzhh/%E6%A1%8C%E9%9D%A2/DFL_Me2.55/_internal/main.py
file:///home/mzhh/%E6%A1%8C%E9%9D%A2/DFL_Me2.55/_internal/requirements-colab.txt
file:///home/mzhh/%E6%A1%8C%E9%9D%A2/DFL_Me2.55/_internal/requirements-cuda.txt
file:///home/mzhh/%E6%A1%8C%E9%9D%A2/DFL_Me2.55/_internal/core
file:///home/mzhh/%E6%A1%8C%E9%9D%A2/DFL_Me2.55/_internal/DFLIMG
file:///home/mzhh/%E6%A1%8C%E9%9D%A2/DFL_Me2.55/_internal/doc
file:///home/mzhh/%E6%A1%8C%E9%9D%A2/DFL_Me2.55/_internal/facelib
file:///home/mzhh/%E6%A1%8C%E9%9D%A2/DFL_Me2.55/_internal/flaskr
file:///home/mzhh/%E6%A1%8C%E9%9D%A2/DFL_Me2.55/_internal/localization
file:///home/mzhh/%E6%A1%8C%E9%9D%A2/DFL_Me2.55/_internal/mainscripts
file:///home/mzhh/%E6%A1%8C%E9%9D%A2/DFL_Me2.55/_internal/merger
file:///home/mzhh/%E6%A1%8C%E9%9D%A2/DFL_Me2.55/_internal/models
file:///home/mzhh/%E6%A1%8C%E9%9D%A2/DFL_Me2.55/_internal/samplelib
file:///home/mzhh/%E6%A1%8C%E9%9D%A2/DFL_Me2.55/_internal/utils
file:///home/mzhh/%E6%A1%8C%E9%9D%A2/DFL_Me2.55/_internal/XSegEditor
file:///home/mzhh/%E6%A1%8C%E9%9D%A2/DFL_Me2.55/_internal/DeepfacelabMe%202022%20Sp1_Ver2.55.txt
file:///home/mzhh/%E6%A1%8C%E9%9D%A2/DFL_Me2.55/_internal/DeepfacelabMe%202022%20Sp1_Ver2.55_p1.txt
file:///home/mzhh/%E6%A1%8C%E9%9D%A2/DFL_Me2.55/_internal/environment.yml
file:///home/mzhh/%E6%A1%8C%E9%9D%A2/DFL_Me2.55/_internal/main.py
file:///home/mzhh/%E6%A1%8C%E9%9D%A2/DFL_Me2.55/_internal/requirements-colab.txt
file:///home/mzhh/%E6%A1%8C%E9%9D%A2/DFL_Me2.55/_internal/requirements-cuda.txt
file:///home/mzhh/%E6%A1%8C%E9%9D%A2/DFL_Me2.55/_internal/core
file:///home/mzhh/%E6%A1%8C%E9%9D%A2/DFL_Me2.55/_internal/DFLIMG
file:///home/mzhh/%E6%A1%8C%E9%9D%A2/DFL_Me2.55/_internal/doc
file:///home/mzhh/%E6%A1%8C%E9%9D%A2/DFL_Me2.55/_internal/facelib
file:///home/mzhh/%E6%A1%8C%E9%9D%A2/DFL_Me2.55/_internal/flaskr
file:///home/mzhh/%E6%A1%8C%E9%9D%A2/DFL_Me2.55/_internal/localization
file:///home/mzhh/%E6%A1%8C%E9%9D%A2/DFL_Me2.55/_internal/mainscripts
file:///home/mzhh/%E6%A1%8C%E9%9D%A2/DFL_Me2.55/_internal/merger
file:///home/mzhh/%E6%A1%8C%E9%9D%A2/DFL_Me2.55/_internal/models
file:///home/mzhh/%E6%A1%8C%E9%9D%A2/DFL_Me2.55/_internal/samplelib
file:///home/mzhh/%E6%A1%8C%E9%9D%A2/DFL_Me2.55/_internal/utils
file:///home/mzhh/%E6%A1%8C%E9%9D%A2/DFL_Me2.55/_internal/XSegEditor
file:///home/mzhh/%E6%A1%8C%E9%9D%A2/DFL_Me2.55/_internal/DeepfacelabMe%202022%20Sp1_Ver2.55.txt
file:///home/mzhh/%E6%A1%8C%E9%9D%A2/DFL_Me2.55/_internal/DeepfacelabMe%202022%20Sp1_Ver2.55_p1.txt
file:///home/mzhh/%E6%A1%8C%E9%9D%A2/DFL_Me2.55/_internal/environment.yml
file:///home/mzhh/%E6%A1%8C%E9%9D%A2/DFL_Me2.55/_internal/main.py
file:///home/mzhh/%E6%A1%8C%E9%9D%A2/DFL_Me2.55/_internal/requirements-colab.txt
file:///home/mzhh/%E6%A1%8C%E9%9D%A2/DFL_Me2.55/_internal/requirements-cuda.txt
file:///home/mzhh/%E6%A1%8C%E9%9D%A2/DFL_Me2.55/_internal/core
file:///home/mzhh/%E6%A1%8C%E9%9D%A2/DFL_Me2.55/_internal/DFLIMG
file:///home/mzhh/%E6%A1%8C%E9%9D%A2/DFL_Me2.55/_internal/doc
file:///home/mzhh/%E6%A1%8C%E9%9D%A2/DFL_Me2.55/_internal/facelib
file:///home/mzhh/%E6%A1%8C%E9%9D%A2/DFL_Me2.55/_internal/flaskr
file:///home/mzhh/%E6%A1%8C%E9%9D%A2/DFL_Me2.55/_internal/localization
file:///home/mzhh/%E6%A1%8C%E9%9D%A2/DFL_Me2.55/_internal/mainscripts
file:///home/mzhh/%E6%A1%8C%E9%9D%A2/DFL_Me2.55/_internal/merger
file:///home/mzhh/%E6%A1%8C%E9%9D%A2/DFL_Me2.55/_internal/models
file:///home/mzhh/%E6%A1%8C%E9%9D%A2/DFL_Me2.55/_internal/samplelib
file:///home/mzhh/%E6%A1%8C%E9%9D%A2/DFL_Me2.55/_internal/utils
file:///home/mzhh/%E6%A1%8C%E9%9D%A2/DFL_Me2.55/_internal/XSegEditor
file:///home/mzhh/%E6%A1%8C%E9%9D%A2/DFL_Me2.55/_internal/DeepfacelabMe%202022%20Sp1_Ver2.55.txt
file:///home/mzhh/%E6%A1%8C%E9%9D%A2/DFL_Me2.55/_internal/DeepfacelabMe%202022%20Sp1_Ver2.55_p1.txt
file:///home/mzhh/%E6%A1%8C%E9%9D%A2/DFL_Me2.55/_internal/environment.yml
file:///home/mzhh/%E6%A1%8C%E9%9D%A2/DFL_Me2.55/_internal/main.py
file:///home/mzhh/%E6%A1%8C%E9%9D%A2/DFL_Me2.55/_internal/requirements-colab.txt
file:///home/mzhh/%E6%A1%8C%E9%9D%A2/DFL_Me2.55/_internal/requirements-cuda.txt
file:///home/mzhh/%E6%A1%8C%E9%9D%A2/DFL_Me2.55/_internal/core
file:///home/mzhh/%E6%A1%8C%E9%9D%A2/DFL_Me2.55/_internal/DFLIMG
file:///home/mzhh/%E6%A1%8C%E9%9D%A2/DFL_Me2.55/_internal/doc
file:///home/mzhh/%E6%A1%8C%E9%9D%A2/DFL_Me2.55/_internal/facelib
file:///home/mzhh/%E6%A1%8C%E9%9D%A2/DFL_Me2.55/_internal/flaskr
file:///home/mzhh/%E6%A1%8C%E9%9D%A2/DFL_Me2.55/_internal/localization
file:///home/mzhh/%E6%A1%8C%E9%9D%A2/DFL_Me2.55/_internal/mainscripts
file:///home/mzhh/%E6%A1%8C%E9%9D%A2/DFL_Me2.55/_internal/merger
file:///home/mzhh/%E6%A1%8C%E9%9D%A2/DFL_Me2.55/_internal/models
file:///home/mzhh/%E6%A1%8C%E9%9D%A2/DFL_Me2.55/_internal/samplelib
file:///home/mzhh/%E6%A1%8C%E9%9D%A2/DFL_Me2.55/_internal/utils
file:///home/mzhh/%E6%A1%8C%E9%9D%A2/DFL_Me2.55/_internal/XSegEditor
file:///home/mzhh/%E6%A1%8C%E9%9D%A2/DFL_Me2.55/_internal/DeepfacelabMe%202022%20Sp1_Ver2.55.txt
file:///home/mzhh/%E6%A1%8C%E9%9D%A2/DFL_Me2.55/_internal/DeepfacelabMe%202022%20Sp1_Ver2.55_p1.txt
file:///home/mzhh/%E6%A1%8C%E9%9D%A2/DFL_Me2.55/_internal/environment.yml
file:///home/mzhh/%E6%A1%8C%E9%9D%A2/DFL_Me2.55/_internal/main.py
file:///home/mzhh/%E6%A1%8C%E9%9D%A2/DFL_Me2.55/_internal/requirements-colab.txt
file:///home/mzhh/%E6%A1%8C%E9%9D%A2/DFL_Me2.55/_internal/requirements-cuda.txt
file:///home/mzhh/%E6%A1%8C%E9%9D%A2/DFL_Me2.55/_internal/core
file:///home/mzhh/%E6%A1%8C%E9%9D%A2/DFL_Me2.55/_internal/DFLIMG
file:///home/mzhh/%E6%A1%8C%E9%9D%A2/DFL_Me2.55/_internal/doc
file:///home/mzhh/%E6%A1%8C%E9%9D%A2/DFL_Me2.55/_internal/facelib
file:///home/mzhh/%E6%A1%8C%E9%9D%A2/DFL_Me2.55/_internal/flaskr
file:///home/mzhh/%E6%A1%8C%E9%9D%A2/DFL_Me2.55/_internal/localization
file:///home/mzhh/%E6%A1%8C%E9%9D%A2/DFL_Me2.55/_internal/mainscripts
file:///home/mzhh/%E6%A1%8C%E9%9D%A2/DFL_Me2.55/_internal/merger
file:///home/mzhh/%E6%A1%8C%E9%9D%A2/DFL_Me2.55/_internal/models
file:///home/mzhh/%E6%A1%8C%E9%9D%A2/DFL_Me2.55/_internal/samplelib
file:///home/mzhh/%E6%A1%8C%E9%9D%A2/DFL_Me2.55/_internal/utils
file:///home/mzhh/%E6%A1%8C%E9%9D%A2/DFL_Me2.55/_internal/XSegEditor
file:///home/mzhh/%E6%A1%8C%E9%9D%A2/DFL_Me2.55/_internal/DeepfacelabMe%202022%20Sp1_Ver2.55.txt
file:///home/mzhh/%E6%A1%8C%E9%9D%A2/DFL_Me2.55/_internal/DeepfacelabMe%202022%20Sp1_Ver2.55_p1.txt
file:///home/mzhh/%E6%A1%8C%E9%9D%A2/DFL_Me2.55/_internal/environment.yml
file:///home/mzhh/%E6%A1%8C%E9%9D%A2/DFL_Me2.55/_internal/main.py
file:///home/mzhh/%E6%A1%8C%E9%9D%A2/DFL_Me2.55/_internal/requirements-colab.txt
file:///home/mzhh/%E6%A1%8C%E9%9D%A2/DFL_Me2.55/_internal/requirements-cuda.txt
file:///home/mzhh/%E6%A1%8C%E9%9D%A2/DFL_Me2.55/_internal/core
file:///home/mzhh/%E6%A1%8C%E9%9D%A2/DFL_Me2.55/_internal/DFLIMG
file:///home/mzhh/%E6%A1%8C%E9%9D%A2/DFL_Me2.55/_internal/doc
file:///home/mzhh/%E6%A1%8C%E9%9D%A2/DFL_Me2.55/_internal/facelib
file:///home/mzhh/%E6%A1%8C%E9%9D%A2/DFL_Me2.55/_internal/flaskr
file:///home/mzhh/%E6%A1%8C%E9%9D%A2/DFL_Me2.55/_internal/localization
file:///home/mzhh/%E6%A1%8C%E9%9D%A2/DFL_Me2.55/_internal/mainscripts
file:///home/mzhh/%E6%A1%8C%E9%9D%A2/DFL_Me2.55/_internal/merger
file:///home/mzhh/%E6%A1%8C%E9%9D%A2/DFL_Me2.55/_internal/models
file:///home/mzhh/%E6%A1%8C%E9%9D%A2/DFL_Me2.55/_internal/samplelib
file:///home/mzhh/%E6%A1%8C%E9%9D%A2/DFL_Me2.55/_internal/utils
file:///home/mzhh/%E6%A1%8C%E9%9D%A2/DFL_Me2.55/_internal/XSegEditor
file:///home/mzhh/%E6%A1%8C%E9%9D%A2/DFL_Me2.55/_internal/DeepfacelabMe%202022%20Sp1_Ver2.55.txt
file:///home/mzhh/%E6%A1%8C%E9%9D%A2/DFL_Me2.55/_internal/DeepfacelabMe%202022%20Sp1_Ver2.55_p1.txt
file:///home/mzhh/%E6%A1%8C%E9%9D%A2/DFL_Me2.55/_internal/environment.yml
file:///home/mzhh/%E6%A1%8C%E9%9D%A2/DFL_Me2.55/_internal/main.py
file:///home/mzhh/%E6%A1%8C%E9%9D%A2/DFL_Me2.55/_internal/requirements-colab.txt
file:///home/mzhh/%E6%A1%8C%E9%9D%A2/DFL_Me2.55/_internal/requirements-cuda.txt
file:///home/mzhh/%E6%A1%8C%E9%9D%A2/DFL_Me2.55/_internal/core
file:///home/mzhh/%E6%A1%8C%E9%9D%A2/DFL_Me2.55/_internal/DFLIMG
file:///home/mzhh/%E6%A1%8C%E9%9D%A2/DFL_Me2.55/_internal/doc
file:///home/mzhh/%E6%A1%8C%E9%9D%A2/DFL_Me2.55/_internal/facelib
file:///home/mzhh/%E6%A1%8C%E9%9D%A2/DFL_Me2.55/_internal/flaskr
file:///home/mzhh/%E6%A1%8C%E9%9D%A2/DFL_Me2.55/_internal/localization
file:///home/mzhh/%E6%A1%8C%E9%9D%A2/DFL_Me2.55/_internal/mainscripts
file:///home/mzhh/%E6%A1%8C%E9%9D%A2/DFL_Me2.55/_internal/merger
file:///home/mzhh/%E6%A1%8C%E9%9D%A2/DFL_Me2.55/_internal/models
file:///home/mzhh/%E6%A1%8C%E9%9D%A2/DFL_Me2.55/_internal/samplelib
file:///home/mzhh/%E6%A1%8C%E9%9D%A2/DFL_Me2.55/_internal/utils
file:///home/mzhh/%E6%A1%8C%E9%9D%A2/DFL_Me2.55/_internal/XSegEditor
file:///home/mzhh/%E6%A1%8C%E9%9D%A2/DFL_Me2.55/_internal/DeepfacelabMe%202022%20Sp1_Ver2.55.txt
file:///home/mzhh/%E6%A1%8C%E9%9D%A2/DFL_Me2.55/_internal/DeepfacelabMe%202022%20Sp1_Ver2.55_p1.txt
file:///home/mzhh/%E6%A1%8C%E9%9D%A2/DFL_Me2.55/_internal/environment.yml
file:///home/mzhh/%E6%A1%8C%E9%9D%A2/DFL_Me2.55/_internal/main.py
file:///home/mzhh/%E6%A1%8C%E9%9D%A2/DFL_Me2.55/_internal/requirements-colab.txt
file:///home/mzhh/%E6%A1%8C%E9%9D%A2/DFL_Me2.55/_internal/requirements-cuda.txt
| sdssfdf/deepfacelabme | [
"region:us"
] | 2022-11-17T07:57:47+00:00 | {} | 2022-11-17T08:05:34+00:00 | [] | [] | TAGS
#region-us
| file:///home/mzhh/%E6%A1%8C%E9%9D%A2/DFL_Me2.55/_internal/core
file:///home/mzhh/%E6%A1%8C%E9%9D%A2/DFL_Me2.55/_internal/DFLIMG
file:///home/mzhh/%E6%A1%8C%E9%9D%A2/DFL_Me2.55/_internal/doc
file:///home/mzhh/%E6%A1%8C%E9%9D%A2/DFL_Me2.55/_internal/facelib
file:///home/mzhh/%E6%A1%8C%E9%9D%A2/DFL_Me2.55/_internal/flaskr
file:///home/mzhh/%E6%A1%8C%E9%9D%A2/DFL_Me2.55/_internal/localization
file:///home/mzhh/%E6%A1%8C%E9%9D%A2/DFL_Me2.55/_internal/mainscripts
file:///home/mzhh/%E6%A1%8C%E9%9D%A2/DFL_Me2.55/_internal/merger
file:///home/mzhh/%E6%A1%8C%E9%9D%A2/DFL_Me2.55/_internal/models
file:///home/mzhh/%E6%A1%8C%E9%9D%A2/DFL_Me2.55/_internal/samplelib
file:///home/mzhh/%E6%A1%8C%E9%9D%A2/DFL_Me2.55/_internal/utils
file:///home/mzhh/%E6%A1%8C%E9%9D%A2/DFL_Me2.55/_internal/XSegEditor
file:///home/mzhh/%E6%A1%8C%E9%9D%A2/DFL_Me2.55/_internal/DeepfacelabMe%202022%20Sp1_Ver2.URL
file:///home/mzhh/%E6%A1%8C%E9%9D%A2/DFL_Me2.55/_internal/DeepfacelabMe%202022%20Sp1_Ver2.55_p1.txt
file:///home/mzhh/%E6%A1%8C%E9%9D%A2/DFL_Me2.55/_internal/URL
file:///home/mzhh/%E6%A1%8C%E9%9D%A2/DFL_Me2.55/_internal/URL
file:///home/mzhh/%E6%A1%8C%E9%9D%A2/DFL_Me2.55/_internal/URL
file:///home/mzhh/%E6%A1%8C%E9%9D%A2/DFL_Me2.55/_internal/URL
file:///home/mzhh/%E6%A1%8C%E9%9D%A2/DFL_Me2.55/_internal/core
file:///home/mzhh/%E6%A1%8C%E9%9D%A2/DFL_Me2.55/_internal/DFLIMG
file:///home/mzhh/%E6%A1%8C%E9%9D%A2/DFL_Me2.55/_internal/doc
file:///home/mzhh/%E6%A1%8C%E9%9D%A2/DFL_Me2.55/_internal/facelib
file:///home/mzhh/%E6%A1%8C%E9%9D%A2/DFL_Me2.55/_internal/flaskr
file:///home/mzhh/%E6%A1%8C%E9%9D%A2/DFL_Me2.55/_internal/localization
file:///home/mzhh/%E6%A1%8C%E9%9D%A2/DFL_Me2.55/_internal/mainscripts
file:///home/mzhh/%E6%A1%8C%E9%9D%A2/DFL_Me2.55/_internal/merger
file:///home/mzhh/%E6%A1%8C%E9%9D%A2/DFL_Me2.55/_internal/models
file:///home/mzhh/%E6%A1%8C%E9%9D%A2/DFL_Me2.55/_internal/samplelib
file:///home/mzhh/%E6%A1%8C%E9%9D%A2/DFL_Me2.55/_internal/utils
file:///home/mzhh/%E6%A1%8C%E9%9D%A2/DFL_Me2.55/_internal/XSegEditor
file:///home/mzhh/%E6%A1%8C%E9%9D%A2/DFL_Me2.55/_internal/DeepfacelabMe%202022%20Sp1_Ver2.URL
file:///home/mzhh/%E6%A1%8C%E9%9D%A2/DFL_Me2.55/_internal/DeepfacelabMe%202022%20Sp1_Ver2.55_p1.txt
file:///home/mzhh/%E6%A1%8C%E9%9D%A2/DFL_Me2.55/_internal/URL
file:///home/mzhh/%E6%A1%8C%E9%9D%A2/DFL_Me2.55/_internal/URL
file:///home/mzhh/%E6%A1%8C%E9%9D%A2/DFL_Me2.55/_internal/URL
file:///home/mzhh/%E6%A1%8C%E9%9D%A2/DFL_Me2.55/_internal/URL
file:///home/mzhh/%E6%A1%8C%E9%9D%A2/DFL_Me2.55/_internal/core
file:///home/mzhh/%E6%A1%8C%E9%9D%A2/DFL_Me2.55/_internal/DFLIMG
file:///home/mzhh/%E6%A1%8C%E9%9D%A2/DFL_Me2.55/_internal/doc
file:///home/mzhh/%E6%A1%8C%E9%9D%A2/DFL_Me2.55/_internal/facelib
file:///home/mzhh/%E6%A1%8C%E9%9D%A2/DFL_Me2.55/_internal/flaskr
file:///home/mzhh/%E6%A1%8C%E9%9D%A2/DFL_Me2.55/_internal/localization
file:///home/mzhh/%E6%A1%8C%E9%9D%A2/DFL_Me2.55/_internal/mainscripts
file:///home/mzhh/%E6%A1%8C%E9%9D%A2/DFL_Me2.55/_internal/merger
file:///home/mzhh/%E6%A1%8C%E9%9D%A2/DFL_Me2.55/_internal/models
file:///home/mzhh/%E6%A1%8C%E9%9D%A2/DFL_Me2.55/_internal/samplelib
file:///home/mzhh/%E6%A1%8C%E9%9D%A2/DFL_Me2.55/_internal/utils
file:///home/mzhh/%E6%A1%8C%E9%9D%A2/DFL_Me2.55/_internal/XSegEditor
file:///home/mzhh/%E6%A1%8C%E9%9D%A2/DFL_Me2.55/_internal/DeepfacelabMe%202022%20Sp1_Ver2.URL
file:///home/mzhh/%E6%A1%8C%E9%9D%A2/DFL_Me2.55/_internal/DeepfacelabMe%202022%20Sp1_Ver2.55_p1.txt
file:///home/mzhh/%E6%A1%8C%E9%9D%A2/DFL_Me2.55/_internal/URL
file:///home/mzhh/%E6%A1%8C%E9%9D%A2/DFL_Me2.55/_internal/URL
file:///home/mzhh/%E6%A1%8C%E9%9D%A2/DFL_Me2.55/_internal/URL
file:///home/mzhh/%E6%A1%8C%E9%9D%A2/DFL_Me2.55/_internal/URL
file:///home/mzhh/%E6%A1%8C%E9%9D%A2/DFL_Me2.55/_internal/core
file:///home/mzhh/%E6%A1%8C%E9%9D%A2/DFL_Me2.55/_internal/DFLIMG
file:///home/mzhh/%E6%A1%8C%E9%9D%A2/DFL_Me2.55/_internal/doc
file:///home/mzhh/%E6%A1%8C%E9%9D%A2/DFL_Me2.55/_internal/facelib
file:///home/mzhh/%E6%A1%8C%E9%9D%A2/DFL_Me2.55/_internal/flaskr
file:///home/mzhh/%E6%A1%8C%E9%9D%A2/DFL_Me2.55/_internal/localization
file:///home/mzhh/%E6%A1%8C%E9%9D%A2/DFL_Me2.55/_internal/mainscripts
file:///home/mzhh/%E6%A1%8C%E9%9D%A2/DFL_Me2.55/_internal/merger
file:///home/mzhh/%E6%A1%8C%E9%9D%A2/DFL_Me2.55/_internal/models
file:///home/mzhh/%E6%A1%8C%E9%9D%A2/DFL_Me2.55/_internal/samplelib
file:///home/mzhh/%E6%A1%8C%E9%9D%A2/DFL_Me2.55/_internal/utils
file:///home/mzhh/%E6%A1%8C%E9%9D%A2/DFL_Me2.55/_internal/XSegEditor
file:///home/mzhh/%E6%A1%8C%E9%9D%A2/DFL_Me2.55/_internal/DeepfacelabMe%202022%20Sp1_Ver2.URL
file:///home/mzhh/%E6%A1%8C%E9%9D%A2/DFL_Me2.55/_internal/DeepfacelabMe%202022%20Sp1_Ver2.55_p1.txt
file:///home/mzhh/%E6%A1%8C%E9%9D%A2/DFL_Me2.55/_internal/URL
file:///home/mzhh/%E6%A1%8C%E9%9D%A2/DFL_Me2.55/_internal/URL
file:///home/mzhh/%E6%A1%8C%E9%9D%A2/DFL_Me2.55/_internal/URL
file:///home/mzhh/%E6%A1%8C%E9%9D%A2/DFL_Me2.55/_internal/URL
file:///home/mzhh/%E6%A1%8C%E9%9D%A2/DFL_Me2.55/_internal/core
file:///home/mzhh/%E6%A1%8C%E9%9D%A2/DFL_Me2.55/_internal/DFLIMG
file:///home/mzhh/%E6%A1%8C%E9%9D%A2/DFL_Me2.55/_internal/doc
file:///home/mzhh/%E6%A1%8C%E9%9D%A2/DFL_Me2.55/_internal/facelib
file:///home/mzhh/%E6%A1%8C%E9%9D%A2/DFL_Me2.55/_internal/flaskr
file:///home/mzhh/%E6%A1%8C%E9%9D%A2/DFL_Me2.55/_internal/localization
file:///home/mzhh/%E6%A1%8C%E9%9D%A2/DFL_Me2.55/_internal/mainscripts
file:///home/mzhh/%E6%A1%8C%E9%9D%A2/DFL_Me2.55/_internal/merger
file:///home/mzhh/%E6%A1%8C%E9%9D%A2/DFL_Me2.55/_internal/models
file:///home/mzhh/%E6%A1%8C%E9%9D%A2/DFL_Me2.55/_internal/samplelib
file:///home/mzhh/%E6%A1%8C%E9%9D%A2/DFL_Me2.55/_internal/utils
file:///home/mzhh/%E6%A1%8C%E9%9D%A2/DFL_Me2.55/_internal/XSegEditor
file:///home/mzhh/%E6%A1%8C%E9%9D%A2/DFL_Me2.55/_internal/DeepfacelabMe%202022%20Sp1_Ver2.URL
file:///home/mzhh/%E6%A1%8C%E9%9D%A2/DFL_Me2.55/_internal/DeepfacelabMe%202022%20Sp1_Ver2.55_p1.txt
file:///home/mzhh/%E6%A1%8C%E9%9D%A2/DFL_Me2.55/_internal/URL
file:///home/mzhh/%E6%A1%8C%E9%9D%A2/DFL_Me2.55/_internal/URL
file:///home/mzhh/%E6%A1%8C%E9%9D%A2/DFL_Me2.55/_internal/URL
file:///home/mzhh/%E6%A1%8C%E9%9D%A2/DFL_Me2.55/_internal/URL
file:///home/mzhh/%E6%A1%8C%E9%9D%A2/DFL_Me2.55/_internal/core
file:///home/mzhh/%E6%A1%8C%E9%9D%A2/DFL_Me2.55/_internal/DFLIMG
file:///home/mzhh/%E6%A1%8C%E9%9D%A2/DFL_Me2.55/_internal/doc
file:///home/mzhh/%E6%A1%8C%E9%9D%A2/DFL_Me2.55/_internal/facelib
file:///home/mzhh/%E6%A1%8C%E9%9D%A2/DFL_Me2.55/_internal/flaskr
file:///home/mzhh/%E6%A1%8C%E9%9D%A2/DFL_Me2.55/_internal/localization
file:///home/mzhh/%E6%A1%8C%E9%9D%A2/DFL_Me2.55/_internal/mainscripts
file:///home/mzhh/%E6%A1%8C%E9%9D%A2/DFL_Me2.55/_internal/merger
file:///home/mzhh/%E6%A1%8C%E9%9D%A2/DFL_Me2.55/_internal/models
file:///home/mzhh/%E6%A1%8C%E9%9D%A2/DFL_Me2.55/_internal/samplelib
file:///home/mzhh/%E6%A1%8C%E9%9D%A2/DFL_Me2.55/_internal/utils
file:///home/mzhh/%E6%A1%8C%E9%9D%A2/DFL_Me2.55/_internal/XSegEditor
file:///home/mzhh/%E6%A1%8C%E9%9D%A2/DFL_Me2.55/_internal/DeepfacelabMe%202022%20Sp1_Ver2.URL
file:///home/mzhh/%E6%A1%8C%E9%9D%A2/DFL_Me2.55/_internal/DeepfacelabMe%202022%20Sp1_Ver2.55_p1.txt
file:///home/mzhh/%E6%A1%8C%E9%9D%A2/DFL_Me2.55/_internal/URL
file:///home/mzhh/%E6%A1%8C%E9%9D%A2/DFL_Me2.55/_internal/URL
file:///home/mzhh/%E6%A1%8C%E9%9D%A2/DFL_Me2.55/_internal/URL
file:///home/mzhh/%E6%A1%8C%E9%9D%A2/DFL_Me2.55/_internal/URL
file:///home/mzhh/%E6%A1%8C%E9%9D%A2/DFL_Me2.55/_internal/core
file:///home/mzhh/%E6%A1%8C%E9%9D%A2/DFL_Me2.55/_internal/DFLIMG
file:///home/mzhh/%E6%A1%8C%E9%9D%A2/DFL_Me2.55/_internal/doc
file:///home/mzhh/%E6%A1%8C%E9%9D%A2/DFL_Me2.55/_internal/facelib
file:///home/mzhh/%E6%A1%8C%E9%9D%A2/DFL_Me2.55/_internal/flaskr
file:///home/mzhh/%E6%A1%8C%E9%9D%A2/DFL_Me2.55/_internal/localization
file:///home/mzhh/%E6%A1%8C%E9%9D%A2/DFL_Me2.55/_internal/mainscripts
file:///home/mzhh/%E6%A1%8C%E9%9D%A2/DFL_Me2.55/_internal/merger
file:///home/mzhh/%E6%A1%8C%E9%9D%A2/DFL_Me2.55/_internal/models
file:///home/mzhh/%E6%A1%8C%E9%9D%A2/DFL_Me2.55/_internal/samplelib
file:///home/mzhh/%E6%A1%8C%E9%9D%A2/DFL_Me2.55/_internal/utils
file:///home/mzhh/%E6%A1%8C%E9%9D%A2/DFL_Me2.55/_internal/XSegEditor
file:///home/mzhh/%E6%A1%8C%E9%9D%A2/DFL_Me2.55/_internal/DeepfacelabMe%202022%20Sp1_Ver2.URL
file:///home/mzhh/%E6%A1%8C%E9%9D%A2/DFL_Me2.55/_internal/DeepfacelabMe%202022%20Sp1_Ver2.55_p1.txt
file:///home/mzhh/%E6%A1%8C%E9%9D%A2/DFL_Me2.55/_internal/URL
file:///home/mzhh/%E6%A1%8C%E9%9D%A2/DFL_Me2.55/_internal/URL
file:///home/mzhh/%E6%A1%8C%E9%9D%A2/DFL_Me2.55/_internal/URL
file:///home/mzhh/%E6%A1%8C%E9%9D%A2/DFL_Me2.55/_internal/URL
file:///home/mzhh/%E6%A1%8C%E9%9D%A2/DFL_Me2.55/_internal/core
file:///home/mzhh/%E6%A1%8C%E9%9D%A2/DFL_Me2.55/_internal/DFLIMG
file:///home/mzhh/%E6%A1%8C%E9%9D%A2/DFL_Me2.55/_internal/doc
file:///home/mzhh/%E6%A1%8C%E9%9D%A2/DFL_Me2.55/_internal/facelib
file:///home/mzhh/%E6%A1%8C%E9%9D%A2/DFL_Me2.55/_internal/flaskr
file:///home/mzhh/%E6%A1%8C%E9%9D%A2/DFL_Me2.55/_internal/localization
file:///home/mzhh/%E6%A1%8C%E9%9D%A2/DFL_Me2.55/_internal/mainscripts
file:///home/mzhh/%E6%A1%8C%E9%9D%A2/DFL_Me2.55/_internal/merger
file:///home/mzhh/%E6%A1%8C%E9%9D%A2/DFL_Me2.55/_internal/models
file:///home/mzhh/%E6%A1%8C%E9%9D%A2/DFL_Me2.55/_internal/samplelib
file:///home/mzhh/%E6%A1%8C%E9%9D%A2/DFL_Me2.55/_internal/utils
file:///home/mzhh/%E6%A1%8C%E9%9D%A2/DFL_Me2.55/_internal/XSegEditor
file:///home/mzhh/%E6%A1%8C%E9%9D%A2/DFL_Me2.55/_internal/DeepfacelabMe%202022%20Sp1_Ver2.URL
file:///home/mzhh/%E6%A1%8C%E9%9D%A2/DFL_Me2.55/_internal/DeepfacelabMe%202022%20Sp1_Ver2.55_p1.txt
file:///home/mzhh/%E6%A1%8C%E9%9D%A2/DFL_Me2.55/_internal/URL
file:///home/mzhh/%E6%A1%8C%E9%9D%A2/DFL_Me2.55/_internal/URL
file:///home/mzhh/%E6%A1%8C%E9%9D%A2/DFL_Me2.55/_internal/URL
file:///home/mzhh/%E6%A1%8C%E9%9D%A2/DFL_Me2.55/_internal/URL
file:///home/mzhh/%E6%A1%8C%E9%9D%A2/DFL_Me2.55/_internal/core
file:///home/mzhh/%E6%A1%8C%E9%9D%A2/DFL_Me2.55/_internal/DFLIMG
file:///home/mzhh/%E6%A1%8C%E9%9D%A2/DFL_Me2.55/_internal/doc
file:///home/mzhh/%E6%A1%8C%E9%9D%A2/DFL_Me2.55/_internal/facelib
file:///home/mzhh/%E6%A1%8C%E9%9D%A2/DFL_Me2.55/_internal/flaskr
file:///home/mzhh/%E6%A1%8C%E9%9D%A2/DFL_Me2.55/_internal/localization
file:///home/mzhh/%E6%A1%8C%E9%9D%A2/DFL_Me2.55/_internal/mainscripts
file:///home/mzhh/%E6%A1%8C%E9%9D%A2/DFL_Me2.55/_internal/merger
file:///home/mzhh/%E6%A1%8C%E9%9D%A2/DFL_Me2.55/_internal/models
file:///home/mzhh/%E6%A1%8C%E9%9D%A2/DFL_Me2.55/_internal/samplelib
file:///home/mzhh/%E6%A1%8C%E9%9D%A2/DFL_Me2.55/_internal/utils
file:///home/mzhh/%E6%A1%8C%E9%9D%A2/DFL_Me2.55/_internal/XSegEditor
file:///home/mzhh/%E6%A1%8C%E9%9D%A2/DFL_Me2.55/_internal/DeepfacelabMe%202022%20Sp1_Ver2.URL
file:///home/mzhh/%E6%A1%8C%E9%9D%A2/DFL_Me2.55/_internal/DeepfacelabMe%202022%20Sp1_Ver2.55_p1.txt
file:///home/mzhh/%E6%A1%8C%E9%9D%A2/DFL_Me2.55/_internal/URL
file:///home/mzhh/%E6%A1%8C%E9%9D%A2/DFL_Me2.55/_internal/URL
file:///home/mzhh/%E6%A1%8C%E9%9D%A2/DFL_Me2.55/_internal/URL
file:///home/mzhh/%E6%A1%8C%E9%9D%A2/DFL_Me2.55/_internal/URL
file:///home/mzhh/%E6%A1%8C%E9%9D%A2/DFL_Me2.55/_internal/core
file:///home/mzhh/%E6%A1%8C%E9%9D%A2/DFL_Me2.55/_internal/DFLIMG
file:///home/mzhh/%E6%A1%8C%E9%9D%A2/DFL_Me2.55/_internal/doc
file:///home/mzhh/%E6%A1%8C%E9%9D%A2/DFL_Me2.55/_internal/facelib
file:///home/mzhh/%E6%A1%8C%E9%9D%A2/DFL_Me2.55/_internal/flaskr
file:///home/mzhh/%E6%A1%8C%E9%9D%A2/DFL_Me2.55/_internal/localization
file:///home/mzhh/%E6%A1%8C%E9%9D%A2/DFL_Me2.55/_internal/mainscripts
file:///home/mzhh/%E6%A1%8C%E9%9D%A2/DFL_Me2.55/_internal/merger
file:///home/mzhh/%E6%A1%8C%E9%9D%A2/DFL_Me2.55/_internal/models
file:///home/mzhh/%E6%A1%8C%E9%9D%A2/DFL_Me2.55/_internal/samplelib
file:///home/mzhh/%E6%A1%8C%E9%9D%A2/DFL_Me2.55/_internal/utils
file:///home/mzhh/%E6%A1%8C%E9%9D%A2/DFL_Me2.55/_internal/XSegEditor
file:///home/mzhh/%E6%A1%8C%E9%9D%A2/DFL_Me2.55/_internal/DeepfacelabMe%202022%20Sp1_Ver2.URL
file:///home/mzhh/%E6%A1%8C%E9%9D%A2/DFL_Me2.55/_internal/DeepfacelabMe%202022%20Sp1_Ver2.55_p1.txt
file:///home/mzhh/%E6%A1%8C%E9%9D%A2/DFL_Me2.55/_internal/URL
file:///home/mzhh/%E6%A1%8C%E9%9D%A2/DFL_Me2.55/_internal/URL
file:///home/mzhh/%E6%A1%8C%E9%9D%A2/DFL_Me2.55/_internal/URL
file:///home/mzhh/%E6%A1%8C%E9%9D%A2/DFL_Me2.55/_internal/URL
file:///home/mzhh/%E6%A1%8C%E9%9D%A2/DFL_Me2.55/_internal/core
file:///home/mzhh/%E6%A1%8C%E9%9D%A2/DFL_Me2.55/_internal/DFLIMG
file:///home/mzhh/%E6%A1%8C%E9%9D%A2/DFL_Me2.55/_internal/doc
file:///home/mzhh/%E6%A1%8C%E9%9D%A2/DFL_Me2.55/_internal/facelib
file:///home/mzhh/%E6%A1%8C%E9%9D%A2/DFL_Me2.55/_internal/flaskr
file:///home/mzhh/%E6%A1%8C%E9%9D%A2/DFL_Me2.55/_internal/localization
file:///home/mzhh/%E6%A1%8C%E9%9D%A2/DFL_Me2.55/_internal/mainscripts
file:///home/mzhh/%E6%A1%8C%E9%9D%A2/DFL_Me2.55/_internal/merger
file:///home/mzhh/%E6%A1%8C%E9%9D%A2/DFL_Me2.55/_internal/models
file:///home/mzhh/%E6%A1%8C%E9%9D%A2/DFL_Me2.55/_internal/samplelib
file:///home/mzhh/%E6%A1%8C%E9%9D%A2/DFL_Me2.55/_internal/utils
file:///home/mzhh/%E6%A1%8C%E9%9D%A2/DFL_Me2.55/_internal/XSegEditor
file:///home/mzhh/%E6%A1%8C%E9%9D%A2/DFL_Me2.55/_internal/DeepfacelabMe%202022%20Sp1_Ver2.URL
file:///home/mzhh/%E6%A1%8C%E9%9D%A2/DFL_Me2.55/_internal/DeepfacelabMe%202022%20Sp1_Ver2.55_p1.txt
file:///home/mzhh/%E6%A1%8C%E9%9D%A2/DFL_Me2.55/_internal/URL
file:///home/mzhh/%E6%A1%8C%E9%9D%A2/DFL_Me2.55/_internal/URL
file:///home/mzhh/%E6%A1%8C%E9%9D%A2/DFL_Me2.55/_internal/URL
file:///home/mzhh/%E6%A1%8C%E9%9D%A2/DFL_Me2.55/_internal/URL
file:///home/mzhh/%E6%A1%8C%E9%9D%A2/DFL_Me2.55/_internal/core
file:///home/mzhh/%E6%A1%8C%E9%9D%A2/DFL_Me2.55/_internal/DFLIMG
file:///home/mzhh/%E6%A1%8C%E9%9D%A2/DFL_Me2.55/_internal/doc
file:///home/mzhh/%E6%A1%8C%E9%9D%A2/DFL_Me2.55/_internal/facelib
file:///home/mzhh/%E6%A1%8C%E9%9D%A2/DFL_Me2.55/_internal/flaskr
file:///home/mzhh/%E6%A1%8C%E9%9D%A2/DFL_Me2.55/_internal/localization
file:///home/mzhh/%E6%A1%8C%E9%9D%A2/DFL_Me2.55/_internal/mainscripts
file:///home/mzhh/%E6%A1%8C%E9%9D%A2/DFL_Me2.55/_internal/merger
file:///home/mzhh/%E6%A1%8C%E9%9D%A2/DFL_Me2.55/_internal/models
file:///home/mzhh/%E6%A1%8C%E9%9D%A2/DFL_Me2.55/_internal/samplelib
file:///home/mzhh/%E6%A1%8C%E9%9D%A2/DFL_Me2.55/_internal/utils
file:///home/mzhh/%E6%A1%8C%E9%9D%A2/DFL_Me2.55/_internal/XSegEditor
file:///home/mzhh/%E6%A1%8C%E9%9D%A2/DFL_Me2.55/_internal/DeepfacelabMe%202022%20Sp1_Ver2.URL
file:///home/mzhh/%E6%A1%8C%E9%9D%A2/DFL_Me2.55/_internal/DeepfacelabMe%202022%20Sp1_Ver2.55_p1.txt
file:///home/mzhh/%E6%A1%8C%E9%9D%A2/DFL_Me2.55/_internal/URL
file:///home/mzhh/%E6%A1%8C%E9%9D%A2/DFL_Me2.55/_internal/URL
file:///home/mzhh/%E6%A1%8C%E9%9D%A2/DFL_Me2.55/_internal/URL
file:///home/mzhh/%E6%A1%8C%E9%9D%A2/DFL_Me2.55/_internal/URL
file:///home/mzhh/%E6%A1%8C%E9%9D%A2/DFL_Me2.55/_internal/core
file:///home/mzhh/%E6%A1%8C%E9%9D%A2/DFL_Me2.55/_internal/DFLIMG
file:///home/mzhh/%E6%A1%8C%E9%9D%A2/DFL_Me2.55/_internal/doc
file:///home/mzhh/%E6%A1%8C%E9%9D%A2/DFL_Me2.55/_internal/facelib
file:///home/mzhh/%E6%A1%8C%E9%9D%A2/DFL_Me2.55/_internal/flaskr
file:///home/mzhh/%E6%A1%8C%E9%9D%A2/DFL_Me2.55/_internal/localization
file:///home/mzhh/%E6%A1%8C%E9%9D%A2/DFL_Me2.55/_internal/mainscripts
file:///home/mzhh/%E6%A1%8C%E9%9D%A2/DFL_Me2.55/_internal/merger
file:///home/mzhh/%E6%A1%8C%E9%9D%A2/DFL_Me2.55/_internal/models
file:///home/mzhh/%E6%A1%8C%E9%9D%A2/DFL_Me2.55/_internal/samplelib
file:///home/mzhh/%E6%A1%8C%E9%9D%A2/DFL_Me2.55/_internal/utils
file:///home/mzhh/%E6%A1%8C%E9%9D%A2/DFL_Me2.55/_internal/XSegEditor
file:///home/mzhh/%E6%A1%8C%E9%9D%A2/DFL_Me2.55/_internal/DeepfacelabMe%202022%20Sp1_Ver2.URL
file:///home/mzhh/%E6%A1%8C%E9%9D%A2/DFL_Me2.55/_internal/DeepfacelabMe%202022%20Sp1_Ver2.55_p1.txt
file:///home/mzhh/%E6%A1%8C%E9%9D%A2/DFL_Me2.55/_internal/URL
file:///home/mzhh/%E6%A1%8C%E9%9D%A2/DFL_Me2.55/_internal/URL
file:///home/mzhh/%E6%A1%8C%E9%9D%A2/DFL_Me2.55/_internal/URL
file:///home/mzhh/%E6%A1%8C%E9%9D%A2/DFL_Me2.55/_internal/URL
file:///home/mzhh/%E6%A1%8C%E9%9D%A2/DFL_Me2.55/_internal/core
file:///home/mzhh/%E6%A1%8C%E9%9D%A2/DFL_Me2.55/_internal/DFLIMG
file:///home/mzhh/%E6%A1%8C%E9%9D%A2/DFL_Me2.55/_internal/doc
file:///home/mzhh/%E6%A1%8C%E9%9D%A2/DFL_Me2.55/_internal/facelib
file:///home/mzhh/%E6%A1%8C%E9%9D%A2/DFL_Me2.55/_internal/flaskr
file:///home/mzhh/%E6%A1%8C%E9%9D%A2/DFL_Me2.55/_internal/localization
file:///home/mzhh/%E6%A1%8C%E9%9D%A2/DFL_Me2.55/_internal/mainscripts
file:///home/mzhh/%E6%A1%8C%E9%9D%A2/DFL_Me2.55/_internal/merger
file:///home/mzhh/%E6%A1%8C%E9%9D%A2/DFL_Me2.55/_internal/models
file:///home/mzhh/%E6%A1%8C%E9%9D%A2/DFL_Me2.55/_internal/samplelib
file:///home/mzhh/%E6%A1%8C%E9%9D%A2/DFL_Me2.55/_internal/utils
file:///home/mzhh/%E6%A1%8C%E9%9D%A2/DFL_Me2.55/_internal/XSegEditor
file:///home/mzhh/%E6%A1%8C%E9%9D%A2/DFL_Me2.55/_internal/DeepfacelabMe%202022%20Sp1_Ver2.URL
file:///home/mzhh/%E6%A1%8C%E9%9D%A2/DFL_Me2.55/_internal/DeepfacelabMe%202022%20Sp1_Ver2.55_p1.txt
file:///home/mzhh/%E6%A1%8C%E9%9D%A2/DFL_Me2.55/_internal/URL
file:///home/mzhh/%E6%A1%8C%E9%9D%A2/DFL_Me2.55/_internal/URL
file:///home/mzhh/%E6%A1%8C%E9%9D%A2/DFL_Me2.55/_internal/URL
file:///home/mzhh/%E6%A1%8C%E9%9D%A2/DFL_Me2.55/_internal/URL
file:///home/mzhh/%E6%A1%8C%E9%9D%A2/DFL_Me2.55/_internal/core
file:///home/mzhh/%E6%A1%8C%E9%9D%A2/DFL_Me2.55/_internal/DFLIMG
file:///home/mzhh/%E6%A1%8C%E9%9D%A2/DFL_Me2.55/_internal/doc
file:///home/mzhh/%E6%A1%8C%E9%9D%A2/DFL_Me2.55/_internal/facelib
file:///home/mzhh/%E6%A1%8C%E9%9D%A2/DFL_Me2.55/_internal/flaskr
file:///home/mzhh/%E6%A1%8C%E9%9D%A2/DFL_Me2.55/_internal/localization
file:///home/mzhh/%E6%A1%8C%E9%9D%A2/DFL_Me2.55/_internal/mainscripts
file:///home/mzhh/%E6%A1%8C%E9%9D%A2/DFL_Me2.55/_internal/merger
file:///home/mzhh/%E6%A1%8C%E9%9D%A2/DFL_Me2.55/_internal/models
file:///home/mzhh/%E6%A1%8C%E9%9D%A2/DFL_Me2.55/_internal/samplelib
file:///home/mzhh/%E6%A1%8C%E9%9D%A2/DFL_Me2.55/_internal/utils
file:///home/mzhh/%E6%A1%8C%E9%9D%A2/DFL_Me2.55/_internal/XSegEditor
file:///home/mzhh/%E6%A1%8C%E9%9D%A2/DFL_Me2.55/_internal/DeepfacelabMe%202022%20Sp1_Ver2.URL
file:///home/mzhh/%E6%A1%8C%E9%9D%A2/DFL_Me2.55/_internal/DeepfacelabMe%202022%20Sp1_Ver2.55_p1.txt
file:///home/mzhh/%E6%A1%8C%E9%9D%A2/DFL_Me2.55/_internal/URL
file:///home/mzhh/%E6%A1%8C%E9%9D%A2/DFL_Me2.55/_internal/URL
file:///home/mzhh/%E6%A1%8C%E9%9D%A2/DFL_Me2.55/_internal/URL
file:///home/mzhh/%E6%A1%8C%E9%9D%A2/DFL_Me2.55/_internal/URL
file:///home/mzhh/%E6%A1%8C%E9%9D%A2/DFL_Me2.55/_internal/core
file:///home/mzhh/%E6%A1%8C%E9%9D%A2/DFL_Me2.55/_internal/DFLIMG
file:///home/mzhh/%E6%A1%8C%E9%9D%A2/DFL_Me2.55/_internal/doc
file:///home/mzhh/%E6%A1%8C%E9%9D%A2/DFL_Me2.55/_internal/facelib
file:///home/mzhh/%E6%A1%8C%E9%9D%A2/DFL_Me2.55/_internal/flaskr
file:///home/mzhh/%E6%A1%8C%E9%9D%A2/DFL_Me2.55/_internal/localization
file:///home/mzhh/%E6%A1%8C%E9%9D%A2/DFL_Me2.55/_internal/mainscripts
file:///home/mzhh/%E6%A1%8C%E9%9D%A2/DFL_Me2.55/_internal/merger
file:///home/mzhh/%E6%A1%8C%E9%9D%A2/DFL_Me2.55/_internal/models
file:///home/mzhh/%E6%A1%8C%E9%9D%A2/DFL_Me2.55/_internal/samplelib
file:///home/mzhh/%E6%A1%8C%E9%9D%A2/DFL_Me2.55/_internal/utils
file:///home/mzhh/%E6%A1%8C%E9%9D%A2/DFL_Me2.55/_internal/XSegEditor
file:///home/mzhh/%E6%A1%8C%E9%9D%A2/DFL_Me2.55/_internal/DeepfacelabMe%202022%20Sp1_Ver2.URL
file:///home/mzhh/%E6%A1%8C%E9%9D%A2/DFL_Me2.55/_internal/DeepfacelabMe%202022%20Sp1_Ver2.55_p1.txt
file:///home/mzhh/%E6%A1%8C%E9%9D%A2/DFL_Me2.55/_internal/URL
file:///home/mzhh/%E6%A1%8C%E9%9D%A2/DFL_Me2.55/_internal/URL
file:///home/mzhh/%E6%A1%8C%E9%9D%A2/DFL_Me2.55/_internal/URL
file:///home/mzhh/%E6%A1%8C%E9%9D%A2/DFL_Me2.55/_internal/URL
file:///home/mzhh/%E6%A1%8C%E9%9D%A2/DFL_Me2.55/_internal/core
file:///home/mzhh/%E6%A1%8C%E9%9D%A2/DFL_Me2.55/_internal/DFLIMG
file:///home/mzhh/%E6%A1%8C%E9%9D%A2/DFL_Me2.55/_internal/doc
file:///home/mzhh/%E6%A1%8C%E9%9D%A2/DFL_Me2.55/_internal/facelib
file:///home/mzhh/%E6%A1%8C%E9%9D%A2/DFL_Me2.55/_internal/flaskr
file:///home/mzhh/%E6%A1%8C%E9%9D%A2/DFL_Me2.55/_internal/localization
file:///home/mzhh/%E6%A1%8C%E9%9D%A2/DFL_Me2.55/_internal/mainscripts
file:///home/mzhh/%E6%A1%8C%E9%9D%A2/DFL_Me2.55/_internal/merger
file:///home/mzhh/%E6%A1%8C%E9%9D%A2/DFL_Me2.55/_internal/models
file:///home/mzhh/%E6%A1%8C%E9%9D%A2/DFL_Me2.55/_internal/samplelib
file:///home/mzhh/%E6%A1%8C%E9%9D%A2/DFL_Me2.55/_internal/utils
file:///home/mzhh/%E6%A1%8C%E9%9D%A2/DFL_Me2.55/_internal/XSegEditor
file:///home/mzhh/%E6%A1%8C%E9%9D%A2/DFL_Me2.55/_internal/DeepfacelabMe%202022%20Sp1_Ver2.URL
file:///home/mzhh/%E6%A1%8C%E9%9D%A2/DFL_Me2.55/_internal/DeepfacelabMe%202022%20Sp1_Ver2.55_p1.txt
file:///home/mzhh/%E6%A1%8C%E9%9D%A2/DFL_Me2.55/_internal/URL
file:///home/mzhh/%E6%A1%8C%E9%9D%A2/DFL_Me2.55/_internal/URL
file:///home/mzhh/%E6%A1%8C%E9%9D%A2/DFL_Me2.55/_internal/URL
file:///home/mzhh/%E6%A1%8C%E9%9D%A2/DFL_Me2.55/_internal/URL
file:///home/mzhh/%E6%A1%8C%E9%9D%A2/DFL_Me2.55/_internal/core
file:///home/mzhh/%E6%A1%8C%E9%9D%A2/DFL_Me2.55/_internal/DFLIMG
file:///home/mzhh/%E6%A1%8C%E9%9D%A2/DFL_Me2.55/_internal/doc
file:///home/mzhh/%E6%A1%8C%E9%9D%A2/DFL_Me2.55/_internal/facelib
file:///home/mzhh/%E6%A1%8C%E9%9D%A2/DFL_Me2.55/_internal/flaskr
file:///home/mzhh/%E6%A1%8C%E9%9D%A2/DFL_Me2.55/_internal/localization
file:///home/mzhh/%E6%A1%8C%E9%9D%A2/DFL_Me2.55/_internal/mainscripts
file:///home/mzhh/%E6%A1%8C%E9%9D%A2/DFL_Me2.55/_internal/merger
file:///home/mzhh/%E6%A1%8C%E9%9D%A2/DFL_Me2.55/_internal/models
file:///home/mzhh/%E6%A1%8C%E9%9D%A2/DFL_Me2.55/_internal/samplelib
file:///home/mzhh/%E6%A1%8C%E9%9D%A2/DFL_Me2.55/_internal/utils
file:///home/mzhh/%E6%A1%8C%E9%9D%A2/DFL_Me2.55/_internal/XSegEditor
file:///home/mzhh/%E6%A1%8C%E9%9D%A2/DFL_Me2.55/_internal/DeepfacelabMe%202022%20Sp1_Ver2.URL
file:///home/mzhh/%E6%A1%8C%E9%9D%A2/DFL_Me2.55/_internal/DeepfacelabMe%202022%20Sp1_Ver2.55_p1.txt
file:///home/mzhh/%E6%A1%8C%E9%9D%A2/DFL_Me2.55/_internal/URL
file:///home/mzhh/%E6%A1%8C%E9%9D%A2/DFL_Me2.55/_internal/URL
file:///home/mzhh/%E6%A1%8C%E9%9D%A2/DFL_Me2.55/_internal/URL
file:///home/mzhh/%E6%A1%8C%E9%9D%A2/DFL_Me2.55/_internal/URL
| [] | [
"TAGS\n#region-us \n"
] |
530f80a26babad9381cb6c13ea768c63a07eda6c | # Dataset Card for "github-issues"
annotations_creators:
- expert-generated
language:
- en
language_creators:
- found
license:
- cc-by-nc-sa-3.0
multilinguality:
- monolingual
paperswithcode_id: null
pretty_name: Hugging Face GitHub Issues
size_categories:
- 1K<n<10K
source_datasets:
- original
tags:
- bio
- paper
task_categories:
- text-classification
- table-to-text
task_ids:
- multi-class-classification
- sentiment-classification
[More Information needed](https://github.com/huggingface/datasets/blob/main/CONTRIBUTING.md#how-to-contribute-to-the-dataset-cards) | taeseokyi/github-issues | [
"region:us"
] | 2022-11-17T08:11:18+00:00 | {"dataset_info": {"features": [{"name": "url", "dtype": "string"}, {"name": "repository_url", "dtype": "string"}, {"name": "labels_url", "dtype": "string"}, {"name": "comments_url", "dtype": "string"}, {"name": "events_url", "dtype": "string"}, {"name": "html_url", "dtype": "string"}, {"name": "id", "dtype": "int64"}, {"name": "node_id", "dtype": "string"}, {"name": "number", "dtype": "int64"}, {"name": "title", "dtype": "string"}, {"name": "user", "struct": [{"name": "login", "dtype": "string"}, {"name": "id", "dtype": "int64"}, {"name": "node_id", "dtype": "string"}, {"name": "avatar_url", "dtype": "string"}, {"name": "gravatar_id", "dtype": "string"}, {"name": "url", "dtype": "string"}, {"name": "html_url", "dtype": "string"}, {"name": "followers_url", "dtype": "string"}, {"name": "following_url", "dtype": "string"}, {"name": "gists_url", "dtype": "string"}, {"name": "starred_url", "dtype": "string"}, {"name": "subscriptions_url", "dtype": "string"}, {"name": "organizations_url", "dtype": "string"}, {"name": "repos_url", "dtype": "string"}, {"name": "events_url", "dtype": "string"}, {"name": "received_events_url", "dtype": "string"}, {"name": "type", "dtype": "string"}, {"name": "site_admin", "dtype": "bool"}]}, {"name": "labels", "list": [{"name": "id", "dtype": "int64"}, {"name": "node_id", "dtype": "string"}, {"name": "url", "dtype": "string"}, {"name": "name", "dtype": "string"}, {"name": "color", "dtype": "string"}, {"name": "default", "dtype": "bool"}, {"name": "description", "dtype": "string"}]}, {"name": "state", "dtype": "string"}, {"name": "locked", "dtype": "bool"}, {"name": "assignee", "struct": [{"name": "login", "dtype": "string"}, {"name": "id", "dtype": "int64"}, {"name": "node_id", "dtype": "string"}, {"name": "avatar_url", "dtype": "string"}, {"name": "gravatar_id", "dtype": "string"}, {"name": "url", "dtype": "string"}, {"name": "html_url", "dtype": "string"}, {"name": "followers_url", "dtype": "string"}, {"name": "following_url", "dtype": "string"}, {"name": "gists_url", "dtype": "string"}, {"name": "starred_url", "dtype": "string"}, {"name": "subscriptions_url", "dtype": "string"}, {"name": "organizations_url", "dtype": "string"}, {"name": "repos_url", "dtype": "string"}, {"name": "events_url", "dtype": "string"}, {"name": "received_events_url", "dtype": "string"}, {"name": "type", "dtype": "string"}, {"name": "site_admin", "dtype": "bool"}]}, {"name": "assignees", "list": [{"name": "login", "dtype": "string"}, {"name": "id", "dtype": "int64"}, {"name": "node_id", "dtype": "string"}, {"name": "avatar_url", "dtype": "string"}, {"name": "gravatar_id", "dtype": "string"}, {"name": "url", "dtype": "string"}, {"name": "html_url", "dtype": "string"}, {"name": "followers_url", "dtype": "string"}, {"name": "following_url", "dtype": "string"}, {"name": "gists_url", "dtype": "string"}, {"name": "starred_url", "dtype": "string"}, {"name": "subscriptions_url", "dtype": "string"}, {"name": "organizations_url", "dtype": "string"}, {"name": "repos_url", "dtype": "string"}, {"name": "events_url", "dtype": "string"}, {"name": "received_events_url", "dtype": "string"}, {"name": "type", "dtype": "string"}, {"name": "site_admin", "dtype": "bool"}]}, {"name": "milestone", "dtype": "null"}, {"name": "comments", "sequence": "string"}, {"name": "created_at", "dtype": "timestamp[s]"}, {"name": "updated_at", "dtype": "timestamp[s]"}, {"name": "closed_at", "dtype": "timestamp[s]"}, {"name": "author_association", "dtype": "string"}, {"name": "active_lock_reason", "dtype": "null"}, {"name": "draft", "dtype": "bool"}, {"name": "pull_request", "struct": [{"name": "url", "dtype": "string"}, {"name": "html_url", "dtype": "string"}, {"name": "diff_url", "dtype": "string"}, {"name": "patch_url", "dtype": "string"}, {"name": "merged_at", "dtype": "timestamp[s]"}]}, {"name": "body", "dtype": "string"}, {"name": "reactions", "struct": [{"name": "url", "dtype": "string"}, {"name": "total_count", "dtype": "int64"}, {"name": "+1", "dtype": "int64"}, {"name": "-1", "dtype": "int64"}, {"name": "laugh", "dtype": "int64"}, {"name": "hooray", "dtype": "int64"}, {"name": "confused", "dtype": "int64"}, {"name": "heart", "dtype": "int64"}, {"name": "rocket", "dtype": "int64"}, {"name": "eyes", "dtype": "int64"}]}, {"name": "timeline_url", "dtype": "string"}, {"name": "performed_via_github_app", "dtype": "null"}, {"name": "state_reason", "dtype": "string"}, {"name": "is_pull_request", "dtype": "bool"}], "splits": [{"name": "train", "num_bytes": 386968, "num_examples": 100}], "download_size": 169642, "dataset_size": 386968}} | 2022-11-17T08:28:08+00:00 | [] | [] | TAGS
#region-us
| # Dataset Card for "github-issues"
annotations_creators:
- expert-generated
language:
- en
language_creators:
- found
license:
- cc-by-nc-sa-3.0
multilinguality:
- monolingual
paperswithcode_id: null
pretty_name: Hugging Face GitHub Issues
size_categories:
- 1K<n<10K
source_datasets:
- original
tags:
- bio
- paper
task_categories:
- text-classification
- table-to-text
task_ids:
- multi-class-classification
- sentiment-classification
More Information needed | [
"# Dataset Card for \"github-issues\"\nannotations_creators:\n- expert-generated\nlanguage:\n- en\nlanguage_creators:\n- found\nlicense:\n- cc-by-nc-sa-3.0\nmultilinguality:\n- monolingual\npaperswithcode_id: null\npretty_name: Hugging Face GitHub Issues\nsize_categories:\n- 1K<n<10K\nsource_datasets:\n- original\ntags:\n- bio\n- paper\ntask_categories:\n- text-classification\n- table-to-text\ntask_ids:\n- multi-class-classification\n- sentiment-classification\nMore Information needed"
] | [
"TAGS\n#region-us \n",
"# Dataset Card for \"github-issues\"\nannotations_creators:\n- expert-generated\nlanguage:\n- en\nlanguage_creators:\n- found\nlicense:\n- cc-by-nc-sa-3.0\nmultilinguality:\n- monolingual\npaperswithcode_id: null\npretty_name: Hugging Face GitHub Issues\nsize_categories:\n- 1K<n<10K\nsource_datasets:\n- original\ntags:\n- bio\n- paper\ntask_categories:\n- text-classification\n- table-to-text\ntask_ids:\n- multi-class-classification\n- sentiment-classification\nMore Information needed"
] |
022bd3ea57091b057df3cf9e570ae0cb8c2c29a4 |
# Dataset Card for [np20ng]
## Table of Contents
- [Dataset Card for [np20ng]](#dataset-card-for-dataset-name)
- [Table of Contents](#table-of-contents)
- [Dataset Description](#dataset-description)
- [Dataset Summary](#dataset-summary)
- [Supported Tasks and Leaderboards](#supported-tasks-and-leaderboards)
- [Languages](#languages)
- [Dataset Structure](#dataset-structure)
- [Data Instances](#data-instances)
- [Data Fields](#data-fields)
- [Data Splits](#data-splits)
- [Dataset Creation](#dataset-creation)
- [Curation Rationale](#curation-rationale)
- [Source Data](#source-data)
- [Initial Data Collection and Normalization](#initial-data-collection-and-normalization)
- [Who are the source language producers?](#who-are-the-source-language-producers)
- [Annotations](#annotations)
- [Annotation process](#annotation-process)
- [Who are the annotators?](#who-are-the-annotators)
- [Personal and Sensitive Information](#personal-and-sensitive-information)
- [Considerations for Using the Data](#considerations-for-using-the-data)
- [Social Impact of Dataset](#social-impact-of-dataset)
- [Discussion of Biases](#discussion-of-biases)
- [Other Known Limitations](#other-known-limitations)
- [Additional Information](#additional-information)
- [Dataset Curators](#dataset-curators)
- [Licensing Information](#licensing-information)
- [Citation Information](#citation-information)
- [Contributions](#contributions)
## Dataset Description
- **Homepage:** To be updated
- **Repository:** To be updated
- **Paper:** Submitted for review
- **Leaderboard:** To be updated
- **Point of Contact:** To be updated
### Dataset Summary
This is a multi-class Nepali text classification dataset. Text are the news documents and labels are the news categories. It consists over 200,000 documents categorized into 20 different Nepali news groups. News documents from 10 different news sources are compiled into this dataset. Labeling is done using the category-specific news from the respective news portals.
### Supported Tasks and Leaderboards
- Multi-class text classification from news document
- Multi-class text classification from news headings
- News heading generation from news document
### Languages
- Nepali
## Dataset Structure
### Data Instances
The dataset consists over 200,000 Nepali news documents categorized into 20 different news categories.
### Data Fields
- **category:** News category
- **content:** News document (main text)
- **headline:** News headline
- **source:** News source from where the news is taken from
### Data Splits
The dataset is a whole dataset and is not splitted.
## Dataset Creation
### Curation Rationale
To develop and create a large-scale Nepali text classification dataset and releasing it to the public for further research and developments
### Source Data
#### Initial Data Collection and Normalization
Data are scraped from popular Nepali news portals such as Onlinekhabar, Nepalkhabar, Ekantipur, Ratopati, Gorkhapatra, Nepalipatra, Educationpati, Crimenews, etc.
#### Who are the source language producers?
News portals
### Annotations
#### Annotation process
Category labeling of news documents are automatically done as the documents are scraped from category-specific URLs of particular news source
#### Who are the annotators?
News portals
### Personal and Sensitive Information
This dataset does not possess any personal and sensitive information. However, the news content can possess some biasness and irregular information which might be sensitive and not quite related with the original author of the dataset
## Considerations for Using the Data
### Social Impact of Dataset
No issues.
### Discussion of Biases
Categories can be depended on how news portals have categorized them. Otherwise could cause some bias between them.
### Other Known Limitations
News summary are not included
## Additional Information
### Dataset Curators
Me myself.
### Licensing Information
Apache-2.0
### Citation Information
To be updated later (Paper submission in process)
### Contributions
Thanks to [@Suyogyart](https://github.com/Suyogyart) for adding this dataset.
| Suyogyart/np20ng | [
"task_categories:text-classification",
"task_ids:multi-class-classification",
"annotations_creators:other",
"language_creators:machine-generated",
"multilinguality:monolingual",
"size_categories:100K<n<1M",
"source_datasets:original",
"language:ne",
"license:apache-2.0",
"nepali-newsgroups",
"nepali-20-newsgroups",
"np20ng",
"nepali text classification",
"natural language processing",
"news",
"headline",
"region:us"
] | 2022-11-17T09:13:15+00:00 | {"annotations_creators": ["other"], "language_creators": ["machine-generated"], "language": ["ne"], "license": ["apache-2.0"], "multilinguality": ["monolingual"], "size_categories": ["100K<n<1M"], "source_datasets": ["original"], "task_categories": ["text-classification"], "task_ids": ["multi-class-classification"], "pretty_name": "np20ng", "tags": ["nepali-newsgroups", "nepali-20-newsgroups", "np20ng", "nepali text classification", "natural language processing", "news", "headline"]} | 2022-11-17T14:14:33+00:00 | [] | [
"ne"
] | TAGS
#task_categories-text-classification #task_ids-multi-class-classification #annotations_creators-other #language_creators-machine-generated #multilinguality-monolingual #size_categories-100K<n<1M #source_datasets-original #language-Nepali (macrolanguage) #license-apache-2.0 #nepali-newsgroups #nepali-20-newsgroups #np20ng #nepali text classification #natural language processing #news #headline #region-us
|
# Dataset Card for [np20ng]
## Table of Contents
- [Dataset Card for [np20ng]](#dataset-card-for-dataset-name)
- Table of Contents
- Dataset Description
- Dataset Summary
- Supported Tasks and Leaderboards
- Languages
- Dataset Structure
- Data Instances
- Data Fields
- Data Splits
- Dataset Creation
- Curation Rationale
- Source Data
- Initial Data Collection and Normalization
- Who are the source language producers?
- Annotations
- Annotation process
- Who are the annotators?
- Personal and Sensitive Information
- Considerations for Using the Data
- Social Impact of Dataset
- Discussion of Biases
- Other Known Limitations
- Additional Information
- Dataset Curators
- Licensing Information
- Citation Information
- Contributions
## Dataset Description
- Homepage: To be updated
- Repository: To be updated
- Paper: Submitted for review
- Leaderboard: To be updated
- Point of Contact: To be updated
### Dataset Summary
This is a multi-class Nepali text classification dataset. Text are the news documents and labels are the news categories. It consists over 200,000 documents categorized into 20 different Nepali news groups. News documents from 10 different news sources are compiled into this dataset. Labeling is done using the category-specific news from the respective news portals.
### Supported Tasks and Leaderboards
- Multi-class text classification from news document
- Multi-class text classification from news headings
- News heading generation from news document
### Languages
- Nepali
## Dataset Structure
### Data Instances
The dataset consists over 200,000 Nepali news documents categorized into 20 different news categories.
### Data Fields
- category: News category
- content: News document (main text)
- headline: News headline
- source: News source from where the news is taken from
### Data Splits
The dataset is a whole dataset and is not splitted.
## Dataset Creation
### Curation Rationale
To develop and create a large-scale Nepali text classification dataset and releasing it to the public for further research and developments
### Source Data
#### Initial Data Collection and Normalization
Data are scraped from popular Nepali news portals such as Onlinekhabar, Nepalkhabar, Ekantipur, Ratopati, Gorkhapatra, Nepalipatra, Educationpati, Crimenews, etc.
#### Who are the source language producers?
News portals
### Annotations
#### Annotation process
Category labeling of news documents are automatically done as the documents are scraped from category-specific URLs of particular news source
#### Who are the annotators?
News portals
### Personal and Sensitive Information
This dataset does not possess any personal and sensitive information. However, the news content can possess some biasness and irregular information which might be sensitive and not quite related with the original author of the dataset
## Considerations for Using the Data
### Social Impact of Dataset
No issues.
### Discussion of Biases
Categories can be depended on how news portals have categorized them. Otherwise could cause some bias between them.
### Other Known Limitations
News summary are not included
## Additional Information
### Dataset Curators
Me myself.
### Licensing Information
Apache-2.0
To be updated later (Paper submission in process)
### Contributions
Thanks to @Suyogyart for adding this dataset.
| [
"# Dataset Card for [np20ng]",
"## Table of Contents\n- [Dataset Card for [np20ng]](#dataset-card-for-dataset-name)\n - Table of Contents\n - Dataset Description\n - Dataset Summary\n - Supported Tasks and Leaderboards\n - Languages\n - Dataset Structure\n - Data Instances\n - Data Fields\n - Data Splits\n - Dataset Creation\n - Curation Rationale\n - Source Data\n - Initial Data Collection and Normalization\n - Who are the source language producers?\n - Annotations\n - Annotation process\n - Who are the annotators?\n - Personal and Sensitive Information\n - Considerations for Using the Data\n - Social Impact of Dataset\n - Discussion of Biases\n - Other Known Limitations\n - Additional Information\n - Dataset Curators\n - Licensing Information\n - Citation Information\n - Contributions",
"## Dataset Description\n\n- Homepage: To be updated\n- Repository: To be updated\n- Paper: Submitted for review\n- Leaderboard: To be updated\n- Point of Contact: To be updated",
"### Dataset Summary\n\nThis is a multi-class Nepali text classification dataset. Text are the news documents and labels are the news categories. It consists over 200,000 documents categorized into 20 different Nepali news groups. News documents from 10 different news sources are compiled into this dataset. Labeling is done using the category-specific news from the respective news portals.",
"### Supported Tasks and Leaderboards\n\n- Multi-class text classification from news document\n- Multi-class text classification from news headings\n- News heading generation from news document",
"### Languages\n\n- Nepali",
"## Dataset Structure",
"### Data Instances\n\nThe dataset consists over 200,000 Nepali news documents categorized into 20 different news categories.",
"### Data Fields\n\n- category: News category\n- content: News document (main text)\n- headline: News headline\n- source: News source from where the news is taken from",
"### Data Splits\n\nThe dataset is a whole dataset and is not splitted.",
"## Dataset Creation",
"### Curation Rationale\n\nTo develop and create a large-scale Nepali text classification dataset and releasing it to the public for further research and developments",
"### Source Data",
"#### Initial Data Collection and Normalization\n\nData are scraped from popular Nepali news portals such as Onlinekhabar, Nepalkhabar, Ekantipur, Ratopati, Gorkhapatra, Nepalipatra, Educationpati, Crimenews, etc.",
"#### Who are the source language producers?\n\nNews portals",
"### Annotations",
"#### Annotation process\n\nCategory labeling of news documents are automatically done as the documents are scraped from category-specific URLs of particular news source",
"#### Who are the annotators?\n\nNews portals",
"### Personal and Sensitive Information\n\nThis dataset does not possess any personal and sensitive information. However, the news content can possess some biasness and irregular information which might be sensitive and not quite related with the original author of the dataset",
"## Considerations for Using the Data",
"### Social Impact of Dataset\n\nNo issues.",
"### Discussion of Biases\n\nCategories can be depended on how news portals have categorized them. Otherwise could cause some bias between them.",
"### Other Known Limitations\n\nNews summary are not included",
"## Additional Information",
"### Dataset Curators\n\nMe myself.",
"### Licensing Information\n\nApache-2.0\n\n\n\nTo be updated later (Paper submission in process)",
"### Contributions\n\nThanks to @Suyogyart for adding this dataset."
] | [
"TAGS\n#task_categories-text-classification #task_ids-multi-class-classification #annotations_creators-other #language_creators-machine-generated #multilinguality-monolingual #size_categories-100K<n<1M #source_datasets-original #language-Nepali (macrolanguage) #license-apache-2.0 #nepali-newsgroups #nepali-20-newsgroups #np20ng #nepali text classification #natural language processing #news #headline #region-us \n",
"# Dataset Card for [np20ng]",
"## Table of Contents\n- [Dataset Card for [np20ng]](#dataset-card-for-dataset-name)\n - Table of Contents\n - Dataset Description\n - Dataset Summary\n - Supported Tasks and Leaderboards\n - Languages\n - Dataset Structure\n - Data Instances\n - Data Fields\n - Data Splits\n - Dataset Creation\n - Curation Rationale\n - Source Data\n - Initial Data Collection and Normalization\n - Who are the source language producers?\n - Annotations\n - Annotation process\n - Who are the annotators?\n - Personal and Sensitive Information\n - Considerations for Using the Data\n - Social Impact of Dataset\n - Discussion of Biases\n - Other Known Limitations\n - Additional Information\n - Dataset Curators\n - Licensing Information\n - Citation Information\n - Contributions",
"## Dataset Description\n\n- Homepage: To be updated\n- Repository: To be updated\n- Paper: Submitted for review\n- Leaderboard: To be updated\n- Point of Contact: To be updated",
"### Dataset Summary\n\nThis is a multi-class Nepali text classification dataset. Text are the news documents and labels are the news categories. It consists over 200,000 documents categorized into 20 different Nepali news groups. News documents from 10 different news sources are compiled into this dataset. Labeling is done using the category-specific news from the respective news portals.",
"### Supported Tasks and Leaderboards\n\n- Multi-class text classification from news document\n- Multi-class text classification from news headings\n- News heading generation from news document",
"### Languages\n\n- Nepali",
"## Dataset Structure",
"### Data Instances\n\nThe dataset consists over 200,000 Nepali news documents categorized into 20 different news categories.",
"### Data Fields\n\n- category: News category\n- content: News document (main text)\n- headline: News headline\n- source: News source from where the news is taken from",
"### Data Splits\n\nThe dataset is a whole dataset and is not splitted.",
"## Dataset Creation",
"### Curation Rationale\n\nTo develop and create a large-scale Nepali text classification dataset and releasing it to the public for further research and developments",
"### Source Data",
"#### Initial Data Collection and Normalization\n\nData are scraped from popular Nepali news portals such as Onlinekhabar, Nepalkhabar, Ekantipur, Ratopati, Gorkhapatra, Nepalipatra, Educationpati, Crimenews, etc.",
"#### Who are the source language producers?\n\nNews portals",
"### Annotations",
"#### Annotation process\n\nCategory labeling of news documents are automatically done as the documents are scraped from category-specific URLs of particular news source",
"#### Who are the annotators?\n\nNews portals",
"### Personal and Sensitive Information\n\nThis dataset does not possess any personal and sensitive information. However, the news content can possess some biasness and irregular information which might be sensitive and not quite related with the original author of the dataset",
"## Considerations for Using the Data",
"### Social Impact of Dataset\n\nNo issues.",
"### Discussion of Biases\n\nCategories can be depended on how news portals have categorized them. Otherwise could cause some bias between them.",
"### Other Known Limitations\n\nNews summary are not included",
"## Additional Information",
"### Dataset Curators\n\nMe myself.",
"### Licensing Information\n\nApache-2.0\n\n\n\nTo be updated later (Paper submission in process)",
"### Contributions\n\nThanks to @Suyogyart for adding this dataset."
] |
0912bb6c9393c76d62a7c5ee81c4c817ff47c9f4 |
# STS-es
## Table of Contents
- [Table of Contents](#table-of-contents)
- [Dataset Description](#dataset-description)
- [Dataset Summary](#dataset-summary)
- [Supported Tasks and Leaderboards](#supported-tasks-and-leaderboards)
- [Languages](#languages)
- [Dataset Structure](#dataset-structure)
- [Data Instances](#data-instances)
- [Data Fields](#data-fields)
- [Data Splits](#data-splits)
- [Dataset Creation](#dataset-creation)
- [Curation Rationale](#curation-rationale)
- [Source Data](#source-data)
- [Annotations](#annotations)
- [Personal and Sensitive Information](#personal-and-sensitive-information)
- [Considerations for Using the Data](#considerations-for-using-the-data)
- [Social Impact of Dataset](#social-impact-of-dataset)
- [Discussion of Biases](#discussion-of-biases)
- [Additional Information](#additional-information)
- [Dataset Curators](#dataset-curators)
- [Licensing Information](#licensing-information)
- [Citation Information](#citation-information)
- [Contributions](#contributions)
## Dataset Description
- **Homepage:** https://alt.qcri.org/semeval2014/task10/
- **Point of Contact:** [Aitor Gonzalez]([email protected])
### Dataset Summary
For Semantic Text Similarity, we collected the Spanish test sets from SemEval-2014 (Agirre et al., 2014) and SemEval-2015 (Agirre et al., 2015). Since no training data was provided for the Spanish subtask, we randomly sampled both datasets into 1,321 sentences for the train set, 78 sentences for the development set, and 156 sentences for the test set. To make the task harder for the models, we purposely made the development set smaller than the test set.
We use this corpus as part of the EvalEs Spanish language benchmark.
### Supported Tasks and Leaderboards
Semantic Text Similarity Scoring
### Languages
The dataset is in Spanish (`es-ES`)
## Dataset Structure
### Data Instances
```
{
'sentence1': "El "tendón de Aquiles" ("tendo Achillis") o "tendón calcáneo" ("tendo calcaneus") es un tendón de la parte posterior de la pierna."
'sentence2': "El tendón de Aquiles es la extensión tendinosa de los tres músculos de la pantorrilla: gemelo, sóleo y plantar delgado."
'label': 2.8
}
```
### Data Fields
- sentence1: String
- sentence2: String
- label: Float
### Data Splits
- train: 1,321 instances
- dev: 78 instances
- test: 156 instances
## Dataset Creation
### Curation Rationale
[N/A]
### Source Data
The source data came from the Spanish Wikipedia (2013 dump) and texts from Spanish news (2014).
For more information visit the paper from the SemEval-2014 Shared Task [(Agirre et al., 2014)](https://aclanthology.org/S14-2010.pdf) and the SemEval-2015 Shared Task [(Agirre et al., 2015)](https://aclanthology.org/S15-2045.pdf).
#### Initial Data Collection and Normalization
For more information visit the paper from the SemEval-2014 Shared Task [(Agirre et al., 2014)](https://aclanthology.org/S14-2010.pdf) and the SemEval-2015 Shared Task [(Agirre et al., 2015)](https://aclanthology.org/S15-2045.pdf).
#### Who are the source language producers?
Journalists and Wikipedia contributors.
### Annotations
#### Annotation process
For more information visit the paper from the SemEval-2014 Shared Task [(Agirre et al., 2014)](https://aclanthology.org/S14-2010.pdf) and the SemEval-2015 Shared Task [(Agirre et al., 2015)](https://aclanthology.org/S15-2045.pdf).
#### Who are the annotators?
For more information visit the paper from the SemEval-2014 Shared Task [(Agirre et al., 2014)](https://aclanthology.org/S14-2010.pdf) and the SemEval-2015 Shared Task [(Agirre et al., 2015)](https://aclanthology.org/S15-2045.pdf).
### Personal and Sensitive Information
No personal or sensitive information included.
## Considerations for Using the Data
### Social Impact of Dataset
This dataset contributes to the development of language models in Spanish.
### Discussion of Biases
No postprocessing steps were applied to mitigate potential social biases.
## Additional Information
### Citation Information
The following papers must be cited when using this corpus:
```
@inproceedings{agirre2015semeval,
title={Semeval-2015 task 2: Semantic textual similarity, english, spanish and pilot on interpretability},
author={Agirre, Eneko and Banea, Carmen and Cardie, Claire and Cer, Daniel and Diab, Mona and Gonzalez-Agirre, Aitor and Guo, Weiwei and Lopez-Gazpio, Inigo and Maritxalar, Montse and Mihalcea, Rada and others},
booktitle={Proceedings of the 9th international workshop on semantic evaluation (SemEval 2015)},
pages={252--263},
year={2015}
}
@inproceedings{agirre2014semeval,
title={SemEval-2014 Task 10: Multilingual Semantic Textual Similarity.},
author={Agirre, Eneko and Banea, Carmen and Cardie, Claire and Cer, Daniel M and Diab, Mona T and Gonzalez-Agirre, Aitor and Guo, Weiwei and Mihalcea, Rada and Rigau, German and Wiebe, Janyce},
booktitle={SemEval@ COLING},
pages={81--91},
year={2014}
}
```
| PlanTL-GOB-ES/sts-es | [
"task_categories:text-classification",
"task_ids:semantic-similarity-scoring",
"task_ids:text-scoring",
"annotations_creators:expert-generated",
"language_creators:found",
"multilinguality:monolingual",
"language:es",
"region:us"
] | 2022-11-17T12:11:58+00:00 | {"annotations_creators": ["expert-generated"], "language_creators": ["found"], "language": ["es"], "multilinguality": ["monolingual"], "size_categories": [], "source_datasets": [], "task_categories": ["text-classification"], "task_ids": ["semantic-similarity-scoring", "text-scoring"], "pretty_name": "STS-es", "tags": []} | 2023-01-19T09:45:42+00:00 | [] | [
"es"
] | TAGS
#task_categories-text-classification #task_ids-semantic-similarity-scoring #task_ids-text-scoring #annotations_creators-expert-generated #language_creators-found #multilinguality-monolingual #language-Spanish #region-us
|
# STS-es
## Table of Contents
- Table of Contents
- Dataset Description
- Dataset Summary
- Supported Tasks and Leaderboards
- Languages
- Dataset Structure
- Data Instances
- Data Fields
- Data Splits
- Dataset Creation
- Curation Rationale
- Source Data
- Annotations
- Personal and Sensitive Information
- Considerations for Using the Data
- Social Impact of Dataset
- Discussion of Biases
- Additional Information
- Dataset Curators
- Licensing Information
- Citation Information
- Contributions
## Dataset Description
- Homepage: URL
- Point of Contact: Aitor Gonzalez
### Dataset Summary
For Semantic Text Similarity, we collected the Spanish test sets from SemEval-2014 (Agirre et al., 2014) and SemEval-2015 (Agirre et al., 2015). Since no training data was provided for the Spanish subtask, we randomly sampled both datasets into 1,321 sentences for the train set, 78 sentences for the development set, and 156 sentences for the test set. To make the task harder for the models, we purposely made the development set smaller than the test set.
We use this corpus as part of the EvalEs Spanish language benchmark.
### Supported Tasks and Leaderboards
Semantic Text Similarity Scoring
### Languages
The dataset is in Spanish ('es-ES')
## Dataset Structure
### Data Instances
### Data Fields
- sentence1: String
- sentence2: String
- label: Float
### Data Splits
- train: 1,321 instances
- dev: 78 instances
- test: 156 instances
## Dataset Creation
### Curation Rationale
[N/A]
### Source Data
The source data came from the Spanish Wikipedia (2013 dump) and texts from Spanish news (2014).
For more information visit the paper from the SemEval-2014 Shared Task (Agirre et al., 2014) and the SemEval-2015 Shared Task (Agirre et al., 2015).
#### Initial Data Collection and Normalization
For more information visit the paper from the SemEval-2014 Shared Task (Agirre et al., 2014) and the SemEval-2015 Shared Task (Agirre et al., 2015).
#### Who are the source language producers?
Journalists and Wikipedia contributors.
### Annotations
#### Annotation process
For more information visit the paper from the SemEval-2014 Shared Task (Agirre et al., 2014) and the SemEval-2015 Shared Task (Agirre et al., 2015).
#### Who are the annotators?
For more information visit the paper from the SemEval-2014 Shared Task (Agirre et al., 2014) and the SemEval-2015 Shared Task (Agirre et al., 2015).
### Personal and Sensitive Information
No personal or sensitive information included.
## Considerations for Using the Data
### Social Impact of Dataset
This dataset contributes to the development of language models in Spanish.
### Discussion of Biases
No postprocessing steps were applied to mitigate potential social biases.
## Additional Information
The following papers must be cited when using this corpus:
| [
"# STS-es",
"## Table of Contents\n- Table of Contents\n- Dataset Description\n - Dataset Summary\n - Supported Tasks and Leaderboards\n - Languages\n- Dataset Structure\n - Data Instances\n - Data Fields\n - Data Splits\n- Dataset Creation\n - Curation Rationale\n - Source Data\n - Annotations\n - Personal and Sensitive Information\n- Considerations for Using the Data\n - Social Impact of Dataset\n - Discussion of Biases\n- Additional Information\n - Dataset Curators\n - Licensing Information\n - Citation Information\n - Contributions",
"## Dataset Description\n- Homepage: URL \n- Point of Contact: Aitor Gonzalez",
"### Dataset Summary\n\nFor Semantic Text Similarity, we collected the Spanish test sets from SemEval-2014 (Agirre et al., 2014) and SemEval-2015 (Agirre et al., 2015). Since no training data was provided for the Spanish subtask, we randomly sampled both datasets into 1,321 sentences for the train set, 78 sentences for the development set, and 156 sentences for the test set. To make the task harder for the models, we purposely made the development set smaller than the test set.\n\nWe use this corpus as part of the EvalEs Spanish language benchmark.",
"### Supported Tasks and Leaderboards\n\nSemantic Text Similarity Scoring",
"### Languages\n\nThe dataset is in Spanish ('es-ES')",
"## Dataset Structure",
"### Data Instances",
"### Data Fields\n\n- sentence1: String\n- sentence2: String\n- label: Float",
"### Data Splits\n\n- train: 1,321 instances\n- dev: 78 instances\n- test: 156 instances",
"## Dataset Creation",
"### Curation Rationale\n[N/A]",
"### Source Data\n\nThe source data came from the Spanish Wikipedia (2013 dump) and texts from Spanish news (2014).\n\nFor more information visit the paper from the SemEval-2014 Shared Task (Agirre et al., 2014) and the SemEval-2015 Shared Task (Agirre et al., 2015).",
"#### Initial Data Collection and Normalization\n\nFor more information visit the paper from the SemEval-2014 Shared Task (Agirre et al., 2014) and the SemEval-2015 Shared Task (Agirre et al., 2015).",
"#### Who are the source language producers?\n\nJournalists and Wikipedia contributors.",
"### Annotations",
"#### Annotation process\n\nFor more information visit the paper from the SemEval-2014 Shared Task (Agirre et al., 2014) and the SemEval-2015 Shared Task (Agirre et al., 2015).",
"#### Who are the annotators?\n\nFor more information visit the paper from the SemEval-2014 Shared Task (Agirre et al., 2014) and the SemEval-2015 Shared Task (Agirre et al., 2015).",
"### Personal and Sensitive Information\n\nNo personal or sensitive information included.",
"## Considerations for Using the Data",
"### Social Impact of Dataset\n\nThis dataset contributes to the development of language models in Spanish.",
"### Discussion of Biases\n\nNo postprocessing steps were applied to mitigate potential social biases.",
"## Additional Information\n\n\n\n\nThe following papers must be cited when using this corpus:"
] | [
"TAGS\n#task_categories-text-classification #task_ids-semantic-similarity-scoring #task_ids-text-scoring #annotations_creators-expert-generated #language_creators-found #multilinguality-monolingual #language-Spanish #region-us \n",
"# STS-es",
"## Table of Contents\n- Table of Contents\n- Dataset Description\n - Dataset Summary\n - Supported Tasks and Leaderboards\n - Languages\n- Dataset Structure\n - Data Instances\n - Data Fields\n - Data Splits\n- Dataset Creation\n - Curation Rationale\n - Source Data\n - Annotations\n - Personal and Sensitive Information\n- Considerations for Using the Data\n - Social Impact of Dataset\n - Discussion of Biases\n- Additional Information\n - Dataset Curators\n - Licensing Information\n - Citation Information\n - Contributions",
"## Dataset Description\n- Homepage: URL \n- Point of Contact: Aitor Gonzalez",
"### Dataset Summary\n\nFor Semantic Text Similarity, we collected the Spanish test sets from SemEval-2014 (Agirre et al., 2014) and SemEval-2015 (Agirre et al., 2015). Since no training data was provided for the Spanish subtask, we randomly sampled both datasets into 1,321 sentences for the train set, 78 sentences for the development set, and 156 sentences for the test set. To make the task harder for the models, we purposely made the development set smaller than the test set.\n\nWe use this corpus as part of the EvalEs Spanish language benchmark.",
"### Supported Tasks and Leaderboards\n\nSemantic Text Similarity Scoring",
"### Languages\n\nThe dataset is in Spanish ('es-ES')",
"## Dataset Structure",
"### Data Instances",
"### Data Fields\n\n- sentence1: String\n- sentence2: String\n- label: Float",
"### Data Splits\n\n- train: 1,321 instances\n- dev: 78 instances\n- test: 156 instances",
"## Dataset Creation",
"### Curation Rationale\n[N/A]",
"### Source Data\n\nThe source data came from the Spanish Wikipedia (2013 dump) and texts from Spanish news (2014).\n\nFor more information visit the paper from the SemEval-2014 Shared Task (Agirre et al., 2014) and the SemEval-2015 Shared Task (Agirre et al., 2015).",
"#### Initial Data Collection and Normalization\n\nFor more information visit the paper from the SemEval-2014 Shared Task (Agirre et al., 2014) and the SemEval-2015 Shared Task (Agirre et al., 2015).",
"#### Who are the source language producers?\n\nJournalists and Wikipedia contributors.",
"### Annotations",
"#### Annotation process\n\nFor more information visit the paper from the SemEval-2014 Shared Task (Agirre et al., 2014) and the SemEval-2015 Shared Task (Agirre et al., 2015).",
"#### Who are the annotators?\n\nFor more information visit the paper from the SemEval-2014 Shared Task (Agirre et al., 2014) and the SemEval-2015 Shared Task (Agirre et al., 2015).",
"### Personal and Sensitive Information\n\nNo personal or sensitive information included.",
"## Considerations for Using the Data",
"### Social Impact of Dataset\n\nThis dataset contributes to the development of language models in Spanish.",
"### Discussion of Biases\n\nNo postprocessing steps were applied to mitigate potential social biases.",
"## Additional Information\n\n\n\n\nThe following papers must be cited when using this corpus:"
] |
719918f7e4ce82d329ab8a0e2610e7fb239bd0c1 | # Dataset Card for "mm_tiny_imagenet"
[More Information needed](https://github.com/huggingface/datasets/blob/main/CONTRIBUTING.md#how-to-contribute-to-the-dataset-cards) | israfelsr/mm_tiny_imagenet | [
"region:us"
] | 2022-11-17T12:44:50+00:00 | {"dataset_info": {"features": [{"name": "image", "dtype": "image"}, {"name": "label", "dtype": {"class_label": {"names": {"0": "n01443537", "1": "n01629819", "2": "n01641577", "3": "n01644900", "4": "n01698640", "5": "n01742172", "6": "n01768244", "7": "n01770393", "8": "n01774384", "9": "n01774750", "10": "n01784675", "11": "n01882714", "12": "n01910747", "13": "n01917289", "14": "n01944390", "15": "n01950731", "16": "n01983481", "17": "n01984695", "18": "n02002724", "19": "n02056570", "20": "n02058221", "21": "n02074367", "22": "n02094433", "23": "n02099601", "24": "n02099712", "25": "n02106662", "26": "n02113799", "27": "n02123045", "28": "n02123394", "29": "n02124075", "30": "n02125311", "31": "n02129165", "32": "n02132136", "33": "n02165456", "34": "n02226429", "35": "n02231487", "36": "n02233338", "37": "n02236044", "38": "n02268443", "39": "n02279972", "40": "n02281406", "41": "n02321529", "42": "n02364673", "43": "n02395406", "44": "n02403003", "45": "n02410509", "46": "n02415577", "47": "n02423022", "48": "n02437312", "49": "n02480495", "50": "n02481823", "51": "n02486410", "52": "n02504458", "53": "n02509815", "54": "n02666347", "55": "n02669723", "56": "n02699494", "57": "n02769748", "58": "n02788148", "59": "n02791270", "60": "n02793495", "61": "n02795169", "62": "n02802426", "63": "n02808440", "64": "n02814533", "65": "n02814860", "66": "n02815834", "67": "n02823428", "68": "n02837789", "69": "n02841315", "70": "n02843684", "71": "n02883205", "72": "n02892201", "73": "n02909870", "74": "n02917067", "75": "n02927161", "76": "n02948072", "77": "n02950826", "78": "n02963159", "79": "n02977058", "80": "n02988304", "81": "n03014705", "82": "n03026506", "83": "n03042490", "84": "n03085013", "85": "n03089624", "86": "n03100240", "87": "n03126707", "88": "n03160309", "89": "n03179701", "90": "n03201208", "91": "n03255030", "92": "n03355925", "93": "n03373237", "94": "n03388043", "95": "n03393912", "96": "n03400231", "97": "n03404251", "98": "n03424325", "99": "n03444034", "100": "n03447447", "101": "n03544143", "102": "n03584254", "103": "n03599486", "104": "n03617480", "105": "n03637318", "106": "n03649909", "107": "n03662601", "108": "n03670208", "109": "n03706229", "110": "n03733131", "111": "n03763968", "112": "n03770439", "113": "n03796401", "114": "n03814639", "115": "n03837869", "116": "n03838899", "117": "n03854065", "118": "n03891332", "119": "n03902125", "120": "n03930313", "121": "n03937543", "122": "n03970156", "123": "n03977966", "124": "n03980874", "125": "n03983396", "126": "n03992509", "127": "n04008634", "128": "n04023962", "129": "n04070727", "130": "n04074963", "131": "n04099969", "132": "n04118538", "133": "n04133789", "134": "n04146614", "135": "n04149813", "136": "n04179913", "137": "n04251144", "138": "n04254777", "139": "n04259630", "140": "n04265275", "141": "n04275548", "142": "n04285008", "143": "n04311004", "144": "n04328186", "145": "n04356056", "146": "n04366367", "147": "n04371430", "148": "n04376876", "149": "n04398044", "150": "n04399382", "151": "n04417672", "152": "n04456115", "153": "n04465666", "154": "n04486054", "155": "n04487081", "156": "n04501370", "157": "n04507155", "158": "n04532106", "159": "n04532670", "160": "n04540053", "161": "n04560804", "162": "n04562935", "163": "n04596742", "164": "n04598010", "165": "n06596364", "166": "n07056680", "167": "n07583066", "168": "n07614500", "169": "n07615774", "170": "n07646821", "171": "n07647870", "172": "n07657664", "173": "n07695742", "174": "n07711569", "175": "n07715103", "176": "n07720875", "177": "n07749582", "178": "n07753592", "179": "n07768694", "180": "n07871810", "181": "n07873807", "182": "n07875152", "183": "n07920052", "184": "n07975909", "185": "n08496334", "186": "n08620881", "187": "n08742578", "188": "n09193705", "189": "n09246464", "190": "n09256479", "191": "n09332890", "192": "n09428293", "193": "n12267677", "194": "n12520864", "195": "n13001041", "196": "n13652335", "197": "n13652994", "198": "n13719102", "199": "n14991210"}}}}, {"name": "caption", "dtype": "string"}, {"name": "label_name", "dtype": "string"}], "splits": [{"name": "train", "num_bytes": 159978960.0, "num_examples": 80000}, {"name": "validation", "num_bytes": 40004701.0, "num_examples": 20000}], "download_size": 149059401, "dataset_size": 199983661.0}} | 2022-12-16T11:19:54+00:00 | [] | [] | TAGS
#region-us
| # Dataset Card for "mm_tiny_imagenet"
More Information needed | [
"# Dataset Card for \"mm_tiny_imagenet\"\n\nMore Information needed"
] | [
"TAGS\n#region-us \n",
"# Dataset Card for \"mm_tiny_imagenet\"\n\nMore Information needed"
] |
6e5d367220c831c72fb41436a75345d8bfd8daee | dfghnbfg | alvaroec98/images_prueba | [
"region:us"
] | 2022-11-17T12:53:10+00:00 | {} | 2022-11-17T14:53:11+00:00 | [] | [] | TAGS
#region-us
| dfghnbfg | [] | [
"TAGS\n#region-us \n"
] |
0ecd59e6c3eb60bae5e124ec827f60d5f8e2a2d1 |
# Dataset Card for librispeech_asr_dummy
## Table of Contents
- [Dataset Description](#dataset-description)
- [Dataset Summary](#dataset-summary)
- [Supported Tasks and Leaderboards](#supported-tasks-and-leaderboards)
- [Languages](#languages)
- [Dataset Structure](#dataset-structure)
- [Data Instances](#data-instances)
- [Data Fields](#data-fields)
- [Data Splits](#data-splits)
- [Dataset Creation](#dataset-creation)
- [Curation Rationale](#curation-rationale)
- [Source Data](#source-data)
- [Annotations](#annotations)
- [Personal and Sensitive Information](#personal-and-sensitive-information)
- [Considerations for Using the Data](#considerations-for-using-the-data)
- [Social Impact of Dataset](#social-impact-of-dataset)
- [Discussion of Biases](#discussion-of-biases)
- [Other Known Limitations](#other-known-limitations)
- [Additional Information](#additional-information)
- [Dataset Curators](#dataset-curators)
- [Licensing Information](#licensing-information)
- [Citation Information](#citation-information)
- [Contributions](#contributions)
## Dataset Description
- **Homepage:** [LibriSpeech ASR corpus](http://www.openslr.org/12)
- **Repository:** [Needs More Information]
- **Paper:** [LibriSpeech: An ASR Corpus Based On Public Domain Audio Books](https://www.danielpovey.com/files/2015_icassp_librispeech.pdf)
- **Leaderboard:** [The 🤗 Speech Bench](https://huggingface.co/spaces/huggingface/hf-speech-bench)
- **Point of Contact:** [Daniel Povey](mailto:[email protected])
### Dataset Summary
This is a **truncated** version of the LibriSpeech dataset. It contains 20 samples from each of the splits. To view the full dataset, visit: https://huggingface.co/datasets/librispeech_asr
LibriSpeech is a corpus of approximately 1000 hours of 16kHz read English speech, prepared by Vassil Panayotov with the assistance of Daniel Povey. The data is derived from read audiobooks from the LibriVox project, and has been carefully segmented and aligned.
### Supported Tasks and Leaderboards
- `automatic-speech-recognition`, `audio-speaker-identification`: The dataset can be used to train a model for Automatic Speech Recognition (ASR). The model is presented with an audio file and asked to transcribe the audio file to written text. The most common evaluation metric is the word error rate (WER). The task has an active Hugging Face leaderboard which can be found at https://huggingface.co/spaces/huggingface/hf-speech-bench. The leaderboard ranks models uploaded to the Hub based on their WER. An external leaderboard at https://paperswithcode.com/sota/speech-recognition-on-librispeech-test-clean ranks the latest models from research and academia.
### Languages
The audio is in English. There are two configurations: `clean` and `other`.
The speakers in the corpus were ranked according to the WER of the transcripts of a model trained on
a different dataset, and were divided roughly in the middle,
with the lower-WER speakers designated as "clean" and the higher WER speakers designated as "other".
## Dataset Structure
### Data Instances
A typical data point comprises the path to the audio file, usually called `file` and its transcription, called `text`. Some additional information about the speaker and the passage which contains the transcription is provided.
```
{'chapter_id': 141231,
'file': '/home/patrick/.cache/huggingface/datasets/downloads/extracted/b7ded9969e09942ab65313e691e6fc2e12066192ee8527e21d634aca128afbe2/dev_clean/1272/141231/1272-141231-0000.flac',
'audio': {'path': '/home/patrick/.cache/huggingface/datasets/downloads/extracted/b7ded9969e09942ab65313e691e6fc2e12066192ee8527e21d634aca128afbe2/dev_clean/1272/141231/1272-141231-0000.flac',
'array': array([-0.00048828, -0.00018311, -0.00137329, ..., 0.00079346,
0.00091553, 0.00085449], dtype=float32),
'sampling_rate': 16000},
'id': '1272-141231-0000',
'speaker_id': 1272,
'text': 'A MAN SAID TO THE UNIVERSE SIR I EXIST'}
```
### Data Fields
- file: A path to the downloaded audio file in .flac format.
- audio: A dictionary containing the path to the downloaded audio file, the decoded audio array, and the sampling rate. Note that when accessing the audio column: `dataset[0]["audio"]` the audio file is automatically decoded and resampled to `dataset.features["audio"].sampling_rate`. Decoding and resampling of a large number of audio files might take a significant amount of time. Thus it is important to first query the sample index before the `"audio"` column, *i.e.* `dataset[0]["audio"]` should **always** be preferred over `dataset["audio"][0]`.
- text: the transcription of the audio file.
- id: unique id of the data sample.
- speaker_id: unique id of the speaker. The same speaker id can be found for multiple data samples.
- chapter_id: id of the audiobook chapter which includes the transcription.
### Data Splits
The size of the corpus makes it impractical, or at least inconvenient
for some users, to distribute it as a single large archive. Thus the
training portion of the corpus is split into three subsets, with approximate size 100, 360 and 500 hours respectively.
A simple automatic
procedure was used to select the audio in the first two sets to be, on
average, of higher recording quality and with accents closer to US
English. An acoustic model was trained on WSJ’s si-84 data subset
and was used to recognize the audio in the corpus, using a bigram
LM estimated on the text of the respective books. We computed the
Word Error Rate (WER) of this automatic transcript relative to our
reference transcripts obtained from the book texts.
The speakers in the corpus were ranked according to the WER of
the WSJ model’s transcripts, and were divided roughly in the middle,
with the lower-WER speakers designated as "clean" and the higher-WER speakers designated as "other".
For "clean", the data is split into train, validation, and test set. The train set is further split into train.100 and train.360
respectively accounting for 100h and 360h of the training data.
For "other", the data is split into train, validation, and test set. The train set contains approximately 500h of recorded speech.
| | Train.500 | Train.360 | Train.100 | Valid | Test |
| ----- | ------ | ----- | ---- | ---- | ---- |
| clean | - | 104014 | 28539 | 2703 | 2620|
| other | 148688 | - | - | 2864 | 2939 |
## Dataset Creation
### Personal and Sensitive Information
The dataset consists of people who have donated their voice online. You agree to not attempt to determine the identity of speakers in this dataset.
## Additional Information
### Dataset Curators
The dataset was initially created by Vassil Panayotov, Guoguo Chen, Daniel Povey, and Sanjeev Khudanpur.
### Licensing Information
[CC BY 4.0](https://creativecommons.org/licenses/by/4.0/)
### Citation Information
```
@inproceedings{panayotov2015librispeech,
title={Librispeech: an ASR corpus based on public domain audio books},
author={Panayotov, Vassil and Chen, Guoguo and Povey, Daniel and Khudanpur, Sanjeev},
booktitle={Acoustics, Speech and Signal Processing (ICASSP), 2015 IEEE International Conference on},
pages={5206--5210},
year={2015},
organization={IEEE}
}
```
| sanchit-gandhi/librispeech_asr_dummy | [
"task_categories:automatic-speech-recognition",
"task_categories:audio-classification",
"task_ids:speaker-identification",
"annotations_creators:expert-generated",
"language_creators:crowdsourced",
"language_creators:expert-generated",
"multilinguality:monolingual",
"size_categories:100K<n<1M",
"source_datasets:original",
"language:en",
"license:cc-by-4.0",
"region:us"
] | 2022-11-17T13:29:57+00:00 | {"annotations_creators": ["expert-generated"], "language_creators": ["crowdsourced", "expert-generated"], "language": ["en"], "license": ["cc-by-4.0"], "multilinguality": ["monolingual"], "size_categories": ["100K<n<1M"], "source_datasets": ["original"], "task_categories": ["automatic-speech-recognition", "audio-classification"], "task_ids": ["speaker-identification"], "paperswithcode_id": "librispeech-1", "pretty_name": "LibriSpeech Dummy", "configs": [{"config_name": "default", "data_files": [{"split": "test.other", "path": "data/test.other-*"}, {"split": "train.other.500", "path": "data/train.other.500-*"}, {"split": "train.clean.360", "path": "data/train.clean.360-*"}, {"split": "validation.clean", "path": "data/validation.clean-*"}, {"split": "test.clean", "path": "data/test.clean-*"}, {"split": "validation.other", "path": "data/validation.other-*"}, {"split": "train.clean.100", "path": "data/train.clean.100-*"}]}, {"config_name": "short-form", "data_files": [{"split": "validation", "path": "short-form/validation-*"}]}], "dataset_info": {"config_name": "short-form", "features": [{"name": "file", "dtype": "string"}, {"name": "audio", "dtype": {"audio": {"sampling_rate": 16000}}}, {"name": "text", "dtype": "string"}, {"name": "speaker_id", "dtype": "int64"}, {"name": "chapter_id", "dtype": "int64"}, {"name": "id", "dtype": "string"}], "splits": [{"name": "validation", "num_bytes": 9677021.0, "num_examples": 73}], "download_size": 9192059, "dataset_size": 9677021.0}} | 2023-11-02T11:52:44+00:00 | [] | [
"en"
] | TAGS
#task_categories-automatic-speech-recognition #task_categories-audio-classification #task_ids-speaker-identification #annotations_creators-expert-generated #language_creators-crowdsourced #language_creators-expert-generated #multilinguality-monolingual #size_categories-100K<n<1M #source_datasets-original #language-English #license-cc-by-4.0 #region-us
| Dataset Card for librispeech\_asr\_dummy
========================================
Table of Contents
-----------------
* Dataset Description
+ Dataset Summary
+ Supported Tasks and Leaderboards
+ Languages
* Dataset Structure
+ Data Instances
+ Data Fields
+ Data Splits
* Dataset Creation
+ Curation Rationale
+ Source Data
+ Annotations
+ Personal and Sensitive Information
* Considerations for Using the Data
+ Social Impact of Dataset
+ Discussion of Biases
+ Other Known Limitations
* Additional Information
+ Dataset Curators
+ Licensing Information
+ Citation Information
+ Contributions
Dataset Description
-------------------
* Homepage: LibriSpeech ASR corpus
* Repository:
* Paper: LibriSpeech: An ASR Corpus Based On Public Domain Audio Books
* Leaderboard: The Speech Bench
* Point of Contact: Daniel Povey
### Dataset Summary
This is a truncated version of the LibriSpeech dataset. It contains 20 samples from each of the splits. To view the full dataset, visit: URL
LibriSpeech is a corpus of approximately 1000 hours of 16kHz read English speech, prepared by Vassil Panayotov with the assistance of Daniel Povey. The data is derived from read audiobooks from the LibriVox project, and has been carefully segmented and aligned.
### Supported Tasks and Leaderboards
* 'automatic-speech-recognition', 'audio-speaker-identification': The dataset can be used to train a model for Automatic Speech Recognition (ASR). The model is presented with an audio file and asked to transcribe the audio file to written text. The most common evaluation metric is the word error rate (WER). The task has an active Hugging Face leaderboard which can be found at URL The leaderboard ranks models uploaded to the Hub based on their WER. An external leaderboard at URL ranks the latest models from research and academia.
### Languages
The audio is in English. There are two configurations: 'clean' and 'other'.
The speakers in the corpus were ranked according to the WER of the transcripts of a model trained on
a different dataset, and were divided roughly in the middle,
with the lower-WER speakers designated as "clean" and the higher WER speakers designated as "other".
Dataset Structure
-----------------
### Data Instances
A typical data point comprises the path to the audio file, usually called 'file' and its transcription, called 'text'. Some additional information about the speaker and the passage which contains the transcription is provided.
### Data Fields
* file: A path to the downloaded audio file in .flac format.
* audio: A dictionary containing the path to the downloaded audio file, the decoded audio array, and the sampling rate. Note that when accessing the audio column: 'dataset[0]["audio"]' the audio file is automatically decoded and resampled to 'dataset.features["audio"].sampling\_rate'. Decoding and resampling of a large number of audio files might take a significant amount of time. Thus it is important to first query the sample index before the '"audio"' column, *i.e.* 'dataset[0]["audio"]' should always be preferred over 'dataset["audio"][0]'.
* text: the transcription of the audio file.
* id: unique id of the data sample.
* speaker\_id: unique id of the speaker. The same speaker id can be found for multiple data samples.
* chapter\_id: id of the audiobook chapter which includes the transcription.
### Data Splits
The size of the corpus makes it impractical, or at least inconvenient
for some users, to distribute it as a single large archive. Thus the
training portion of the corpus is split into three subsets, with approximate size 100, 360 and 500 hours respectively.
A simple automatic
procedure was used to select the audio in the first two sets to be, on
average, of higher recording quality and with accents closer to US
English. An acoustic model was trained on WSJ’s si-84 data subset
and was used to recognize the audio in the corpus, using a bigram
LM estimated on the text of the respective books. We computed the
Word Error Rate (WER) of this automatic transcript relative to our
reference transcripts obtained from the book texts.
The speakers in the corpus were ranked according to the WER of
the WSJ model’s transcripts, and were divided roughly in the middle,
with the lower-WER speakers designated as "clean" and the higher-WER speakers designated as "other".
For "clean", the data is split into train, validation, and test set. The train set is further split into train.100 and train.360
respectively accounting for 100h and 360h of the training data.
For "other", the data is split into train, validation, and test set. The train set contains approximately 500h of recorded speech.
Dataset Creation
----------------
### Personal and Sensitive Information
The dataset consists of people who have donated their voice online. You agree to not attempt to determine the identity of speakers in this dataset.
Additional Information
----------------------
### Dataset Curators
The dataset was initially created by Vassil Panayotov, Guoguo Chen, Daniel Povey, and Sanjeev Khudanpur.
### Licensing Information
CC BY 4.0
| [
"### Dataset Summary\n\n\nThis is a truncated version of the LibriSpeech dataset. It contains 20 samples from each of the splits. To view the full dataset, visit: URL\n\n\nLibriSpeech is a corpus of approximately 1000 hours of 16kHz read English speech, prepared by Vassil Panayotov with the assistance of Daniel Povey. The data is derived from read audiobooks from the LibriVox project, and has been carefully segmented and aligned.",
"### Supported Tasks and Leaderboards\n\n\n* 'automatic-speech-recognition', 'audio-speaker-identification': The dataset can be used to train a model for Automatic Speech Recognition (ASR). The model is presented with an audio file and asked to transcribe the audio file to written text. The most common evaluation metric is the word error rate (WER). The task has an active Hugging Face leaderboard which can be found at URL The leaderboard ranks models uploaded to the Hub based on their WER. An external leaderboard at URL ranks the latest models from research and academia.",
"### Languages\n\n\nThe audio is in English. There are two configurations: 'clean' and 'other'.\nThe speakers in the corpus were ranked according to the WER of the transcripts of a model trained on\na different dataset, and were divided roughly in the middle,\nwith the lower-WER speakers designated as \"clean\" and the higher WER speakers designated as \"other\".\n\n\nDataset Structure\n-----------------",
"### Data Instances\n\n\nA typical data point comprises the path to the audio file, usually called 'file' and its transcription, called 'text'. Some additional information about the speaker and the passage which contains the transcription is provided.",
"### Data Fields\n\n\n* file: A path to the downloaded audio file in .flac format.\n* audio: A dictionary containing the path to the downloaded audio file, the decoded audio array, and the sampling rate. Note that when accessing the audio column: 'dataset[0][\"audio\"]' the audio file is automatically decoded and resampled to 'dataset.features[\"audio\"].sampling\\_rate'. Decoding and resampling of a large number of audio files might take a significant amount of time. Thus it is important to first query the sample index before the '\"audio\"' column, *i.e.* 'dataset[0][\"audio\"]' should always be preferred over 'dataset[\"audio\"][0]'.\n* text: the transcription of the audio file.\n* id: unique id of the data sample.\n* speaker\\_id: unique id of the speaker. The same speaker id can be found for multiple data samples.\n* chapter\\_id: id of the audiobook chapter which includes the transcription.",
"### Data Splits\n\n\nThe size of the corpus makes it impractical, or at least inconvenient\nfor some users, to distribute it as a single large archive. Thus the\ntraining portion of the corpus is split into three subsets, with approximate size 100, 360 and 500 hours respectively.\nA simple automatic\nprocedure was used to select the audio in the first two sets to be, on\naverage, of higher recording quality and with accents closer to US\nEnglish. An acoustic model was trained on WSJ’s si-84 data subset\nand was used to recognize the audio in the corpus, using a bigram\nLM estimated on the text of the respective books. We computed the\nWord Error Rate (WER) of this automatic transcript relative to our\nreference transcripts obtained from the book texts.\nThe speakers in the corpus were ranked according to the WER of\nthe WSJ model’s transcripts, and were divided roughly in the middle,\nwith the lower-WER speakers designated as \"clean\" and the higher-WER speakers designated as \"other\".\n\n\nFor \"clean\", the data is split into train, validation, and test set. The train set is further split into train.100 and train.360\nrespectively accounting for 100h and 360h of the training data.\nFor \"other\", the data is split into train, validation, and test set. The train set contains approximately 500h of recorded speech.\n\n\n\nDataset Creation\n----------------",
"### Personal and Sensitive Information\n\n\nThe dataset consists of people who have donated their voice online. You agree to not attempt to determine the identity of speakers in this dataset.\n\n\nAdditional Information\n----------------------",
"### Dataset Curators\n\n\nThe dataset was initially created by Vassil Panayotov, Guoguo Chen, Daniel Povey, and Sanjeev Khudanpur.",
"### Licensing Information\n\n\nCC BY 4.0"
] | [
"TAGS\n#task_categories-automatic-speech-recognition #task_categories-audio-classification #task_ids-speaker-identification #annotations_creators-expert-generated #language_creators-crowdsourced #language_creators-expert-generated #multilinguality-monolingual #size_categories-100K<n<1M #source_datasets-original #language-English #license-cc-by-4.0 #region-us \n",
"### Dataset Summary\n\n\nThis is a truncated version of the LibriSpeech dataset. It contains 20 samples from each of the splits. To view the full dataset, visit: URL\n\n\nLibriSpeech is a corpus of approximately 1000 hours of 16kHz read English speech, prepared by Vassil Panayotov with the assistance of Daniel Povey. The data is derived from read audiobooks from the LibriVox project, and has been carefully segmented and aligned.",
"### Supported Tasks and Leaderboards\n\n\n* 'automatic-speech-recognition', 'audio-speaker-identification': The dataset can be used to train a model for Automatic Speech Recognition (ASR). The model is presented with an audio file and asked to transcribe the audio file to written text. The most common evaluation metric is the word error rate (WER). The task has an active Hugging Face leaderboard which can be found at URL The leaderboard ranks models uploaded to the Hub based on their WER. An external leaderboard at URL ranks the latest models from research and academia.",
"### Languages\n\n\nThe audio is in English. There are two configurations: 'clean' and 'other'.\nThe speakers in the corpus were ranked according to the WER of the transcripts of a model trained on\na different dataset, and were divided roughly in the middle,\nwith the lower-WER speakers designated as \"clean\" and the higher WER speakers designated as \"other\".\n\n\nDataset Structure\n-----------------",
"### Data Instances\n\n\nA typical data point comprises the path to the audio file, usually called 'file' and its transcription, called 'text'. Some additional information about the speaker and the passage which contains the transcription is provided.",
"### Data Fields\n\n\n* file: A path to the downloaded audio file in .flac format.\n* audio: A dictionary containing the path to the downloaded audio file, the decoded audio array, and the sampling rate. Note that when accessing the audio column: 'dataset[0][\"audio\"]' the audio file is automatically decoded and resampled to 'dataset.features[\"audio\"].sampling\\_rate'. Decoding and resampling of a large number of audio files might take a significant amount of time. Thus it is important to first query the sample index before the '\"audio\"' column, *i.e.* 'dataset[0][\"audio\"]' should always be preferred over 'dataset[\"audio\"][0]'.\n* text: the transcription of the audio file.\n* id: unique id of the data sample.\n* speaker\\_id: unique id of the speaker. The same speaker id can be found for multiple data samples.\n* chapter\\_id: id of the audiobook chapter which includes the transcription.",
"### Data Splits\n\n\nThe size of the corpus makes it impractical, or at least inconvenient\nfor some users, to distribute it as a single large archive. Thus the\ntraining portion of the corpus is split into three subsets, with approximate size 100, 360 and 500 hours respectively.\nA simple automatic\nprocedure was used to select the audio in the first two sets to be, on\naverage, of higher recording quality and with accents closer to US\nEnglish. An acoustic model was trained on WSJ’s si-84 data subset\nand was used to recognize the audio in the corpus, using a bigram\nLM estimated on the text of the respective books. We computed the\nWord Error Rate (WER) of this automatic transcript relative to our\nreference transcripts obtained from the book texts.\nThe speakers in the corpus were ranked according to the WER of\nthe WSJ model’s transcripts, and were divided roughly in the middle,\nwith the lower-WER speakers designated as \"clean\" and the higher-WER speakers designated as \"other\".\n\n\nFor \"clean\", the data is split into train, validation, and test set. The train set is further split into train.100 and train.360\nrespectively accounting for 100h and 360h of the training data.\nFor \"other\", the data is split into train, validation, and test set. The train set contains approximately 500h of recorded speech.\n\n\n\nDataset Creation\n----------------",
"### Personal and Sensitive Information\n\n\nThe dataset consists of people who have donated their voice online. You agree to not attempt to determine the identity of speakers in this dataset.\n\n\nAdditional Information\n----------------------",
"### Dataset Curators\n\n\nThe dataset was initially created by Vassil Panayotov, Guoguo Chen, Daniel Povey, and Sanjeev Khudanpur.",
"### Licensing Information\n\n\nCC BY 4.0"
] |
6c5fed17b4a853735e7d56709d184e50374af4a6 |
# Dataset Card for MNIST
## Table of Contents
- [Dataset Description](#dataset-description)
- [Dataset Summary](#dataset-summary)
- [Supported Tasks and Leaderboards](#supported-tasks-and-leaderboards)
- [Languages](#languages)
- [Dataset Structure](#dataset-structure)
- [Data Instances](#data-instances)
- [Data Fields](#data-fields)
- [Data Splits](#data-splits)
- [Dataset Creation](#dataset-creation)
- [Curation Rationale](#curation-rationale)
- [Source Data](#source-data)
- [Annotations](#annotations)
- [Personal and Sensitive Information](#personal-and-sensitive-information)
- [Considerations for Using the Data](#considerations-for-using-the-data)
- [Social Impact of Dataset](#social-impact-of-dataset)
- [Discussion of Biases](#discussion-of-biases)
- [Other Known Limitations](#other-known-limitations)
- [Additional Information](#additional-information)
- [Dataset Curators](#dataset-curators)
- [Licensing Information](#licensing-information)
- [Citation Information](#citation-information)
- [Contributions](#contributions)
## Dataset Description
- **Homepage:** http://yann.lecun.com/exdb/mnist/
- **Repository:**
- **Paper:** MNIST handwritten digit database by Yann LeCun, Corinna Cortes, and CJ Burges
- **Leaderboard:**
- **Point of Contact:**
### Dataset Summary
The MNIST dataset consists of 70,000 28x28 black-and-white images of handwritten digits extracted from two NIST databases. There are 60,000 images in the training dataset and 10,000 images in the validation dataset, one class per digit so a total of 10 classes, with 7,000 images (6,000 train images and 1,000 test images) per class.
Half of the image were drawn by Census Bureau employees and the other half by high school students (this split is evenly distributed in the training and testing sets).
### Supported Tasks and Leaderboards
- `image-classification`: The goal of this task is to classify a given image of a handwritten digit into one of 10 classes representing integer values from 0 to 9, inclusively. The leaderboard is available [here](https://paperswithcode.com/sota/image-classification-on-mnist).
### Languages
English
## Dataset Structure
### Data Instances
A data point comprises an image and its label:
```
{
'image': <PIL.PngImagePlugin.PngImageFile image mode=L size=28x28 at 0x276021F6DD8>,
'label': 5
}
```
### Data Fields
- `image`: A `PIL.Image.Image` object containing the 28x28 image. Note that when accessing the image column: `dataset[0]["image"]` the image file is automatically decoded. Decoding of a large number of image files might take a significant amount of time. Thus it is important to first query the sample index before the `"image"` column, *i.e.* `dataset[0]["image"]` should **always** be preferred over `dataset["image"][0]`
- `label`: an integer between 0 and 9 representing the digit.
### Data Splits
The data is split into training and test set. All the images in the test set were drawn by different individuals than the images in the training set. The training set contains 60,000 images and the test set 10,000 images.
## Dataset Creation
### Curation Rationale
The MNIST database was created to provide a testbed for people wanting to try pattern recognition methods or machine learning algorithms while spending minimal efforts on preprocessing and formatting. Images of the original dataset (NIST) were in two groups, one consisting of images drawn by Census Bureau employees and one consisting of images drawn by high school students. In NIST, the training set was built by grouping all the images of the Census Bureau employees, and the test set was built by grouping the images form the high school students.
The goal in building MNIST was to have a training and test set following the same distributions, so the training set contains 30,000 images drawn by Census Bureau employees and 30,000 images drawn by high school students, and the test set contains 5,000 images of each group. The curators took care to make sure all the images in the test set were drawn by different individuals than the images in the training set.
### Source Data
#### Initial Data Collection and Normalization
The original images from NIST were size normalized to fit a 20x20 pixel box while preserving their aspect ratio. The resulting images contain grey levels (i.e., pixels don't simply have a value of black and white, but a level of greyness from 0 to 255) as a result of the anti-aliasing technique used by the normalization algorithm. The images were then centered in a 28x28 image by computing the center of mass of the pixels, and translating the image so as to position this point at the center of the 28x28 field.
#### Who are the source language producers?
Half of the source images were drawn by Census Bureau employees, half by high school students. According to the dataset curator, the images from the first group are more easily recognizable.
### Annotations
#### Annotation process
The images were not annotated after their creation: the image creators annotated their images with the corresponding label after drawing them.
#### Who are the annotators?
Same as the source data creators.
### Personal and Sensitive Information
[More Information Needed]
## Considerations for Using the Data
### Social Impact of Dataset
[More Information Needed]
### Discussion of Biases
[More Information Needed]
### Other Known Limitations
[More Information Needed]
## Additional Information
### Dataset Curators
Chris Burges, Corinna Cortes and Yann LeCun
### Licensing Information
MIT Licence
### Citation Information
```
@article{lecun2010mnist,
title={MNIST handwritten digit database},
author={LeCun, Yann and Cortes, Corinna and Burges, CJ},
journal={ATT Labs [Online]. Available: http://yann.lecun.com/exdb/mnist},
volume={2},
year={2010}
}
```
### Contributions
Thanks to [@sgugger](https://github.com/sgugger) for adding this dataset. | severo/mnist | [
"task_categories:image-classification",
"task_ids:multi-class-image-classification",
"annotations_creators:expert-generated",
"language_creators:found",
"multilinguality:monolingual",
"size_categories:10K<n<100K",
"source_datasets:extended|other-nist",
"language:en",
"license:mit",
"region:us"
] | 2022-11-17T16:33:16+00:00 | {"annotations_creators": ["expert-generated"], "language_creators": ["found"], "language": ["en"], "license": ["mit"], "multilinguality": ["monolingual"], "size_categories": ["10K<n<100K"], "source_datasets": ["extended|other-nist"], "task_categories": ["image-classification"], "task_ids": ["multi-class-image-classification"], "paperswithcode_id": "mnist", "pretty_name": "MNIST", "dataset_info": {"features": [{"name": "image", "dtype": "image"}, {"name": "label", "dtype": {"class_label": {"names": {"0": "0", "1": "1", "2": "2", "3": "3", "4": "4", "5": "5", "6": "6", "7": "7", "8": "8", "9": "9"}}}}], "config_name": "mnist", "splits": [{"name": "test", "num_bytes": 2916440, "num_examples": 10000}, {"name": "train", "num_bytes": 17470848, "num_examples": 60000}], "download_size": 11594722, "dataset_size": 20387288}} | 2022-11-03T16:46:54+00:00 | [] | [
"en"
] | TAGS
#task_categories-image-classification #task_ids-multi-class-image-classification #annotations_creators-expert-generated #language_creators-found #multilinguality-monolingual #size_categories-10K<n<100K #source_datasets-extended|other-nist #language-English #license-mit #region-us
|
# Dataset Card for MNIST
## Table of Contents
- Dataset Description
- Dataset Summary
- Supported Tasks and Leaderboards
- Languages
- Dataset Structure
- Data Instances
- Data Fields
- Data Splits
- Dataset Creation
- Curation Rationale
- Source Data
- Annotations
- Personal and Sensitive Information
- Considerations for Using the Data
- Social Impact of Dataset
- Discussion of Biases
- Other Known Limitations
- Additional Information
- Dataset Curators
- Licensing Information
- Citation Information
- Contributions
## Dataset Description
- Homepage: URL
- Repository:
- Paper: MNIST handwritten digit database by Yann LeCun, Corinna Cortes, and CJ Burges
- Leaderboard:
- Point of Contact:
### Dataset Summary
The MNIST dataset consists of 70,000 28x28 black-and-white images of handwritten digits extracted from two NIST databases. There are 60,000 images in the training dataset and 10,000 images in the validation dataset, one class per digit so a total of 10 classes, with 7,000 images (6,000 train images and 1,000 test images) per class.
Half of the image were drawn by Census Bureau employees and the other half by high school students (this split is evenly distributed in the training and testing sets).
### Supported Tasks and Leaderboards
- 'image-classification': The goal of this task is to classify a given image of a handwritten digit into one of 10 classes representing integer values from 0 to 9, inclusively. The leaderboard is available here.
### Languages
English
## Dataset Structure
### Data Instances
A data point comprises an image and its label:
### Data Fields
- 'image': A 'PIL.Image.Image' object containing the 28x28 image. Note that when accessing the image column: 'dataset[0]["image"]' the image file is automatically decoded. Decoding of a large number of image files might take a significant amount of time. Thus it is important to first query the sample index before the '"image"' column, *i.e.* 'dataset[0]["image"]' should always be preferred over 'dataset["image"][0]'
- 'label': an integer between 0 and 9 representing the digit.
### Data Splits
The data is split into training and test set. All the images in the test set were drawn by different individuals than the images in the training set. The training set contains 60,000 images and the test set 10,000 images.
## Dataset Creation
### Curation Rationale
The MNIST database was created to provide a testbed for people wanting to try pattern recognition methods or machine learning algorithms while spending minimal efforts on preprocessing and formatting. Images of the original dataset (NIST) were in two groups, one consisting of images drawn by Census Bureau employees and one consisting of images drawn by high school students. In NIST, the training set was built by grouping all the images of the Census Bureau employees, and the test set was built by grouping the images form the high school students.
The goal in building MNIST was to have a training and test set following the same distributions, so the training set contains 30,000 images drawn by Census Bureau employees and 30,000 images drawn by high school students, and the test set contains 5,000 images of each group. The curators took care to make sure all the images in the test set were drawn by different individuals than the images in the training set.
### Source Data
#### Initial Data Collection and Normalization
The original images from NIST were size normalized to fit a 20x20 pixel box while preserving their aspect ratio. The resulting images contain grey levels (i.e., pixels don't simply have a value of black and white, but a level of greyness from 0 to 255) as a result of the anti-aliasing technique used by the normalization algorithm. The images were then centered in a 28x28 image by computing the center of mass of the pixels, and translating the image so as to position this point at the center of the 28x28 field.
#### Who are the source language producers?
Half of the source images were drawn by Census Bureau employees, half by high school students. According to the dataset curator, the images from the first group are more easily recognizable.
### Annotations
#### Annotation process
The images were not annotated after their creation: the image creators annotated their images with the corresponding label after drawing them.
#### Who are the annotators?
Same as the source data creators.
### Personal and Sensitive Information
## Considerations for Using the Data
### Social Impact of Dataset
### Discussion of Biases
### Other Known Limitations
## Additional Information
### Dataset Curators
Chris Burges, Corinna Cortes and Yann LeCun
### Licensing Information
MIT Licence
### Contributions
Thanks to @sgugger for adding this dataset. | [
"# Dataset Card for MNIST",
"## Table of Contents\n- Dataset Description\n - Dataset Summary\n - Supported Tasks and Leaderboards\n - Languages\n- Dataset Structure\n - Data Instances\n - Data Fields\n - Data Splits\n- Dataset Creation\n - Curation Rationale\n - Source Data\n - Annotations\n - Personal and Sensitive Information\n- Considerations for Using the Data\n - Social Impact of Dataset\n - Discussion of Biases\n - Other Known Limitations\n- Additional Information\n - Dataset Curators\n - Licensing Information\n - Citation Information\n - Contributions",
"## Dataset Description\n\n- Homepage: URL\n- Repository: \n- Paper: MNIST handwritten digit database by Yann LeCun, Corinna Cortes, and CJ Burges\n- Leaderboard:\n- Point of Contact:",
"### Dataset Summary\n\nThe MNIST dataset consists of 70,000 28x28 black-and-white images of handwritten digits extracted from two NIST databases. There are 60,000 images in the training dataset and 10,000 images in the validation dataset, one class per digit so a total of 10 classes, with 7,000 images (6,000 train images and 1,000 test images) per class.\nHalf of the image were drawn by Census Bureau employees and the other half by high school students (this split is evenly distributed in the training and testing sets).",
"### Supported Tasks and Leaderboards\n\n- 'image-classification': The goal of this task is to classify a given image of a handwritten digit into one of 10 classes representing integer values from 0 to 9, inclusively. The leaderboard is available here.",
"### Languages\n\nEnglish",
"## Dataset Structure",
"### Data Instances\n\nA data point comprises an image and its label:",
"### Data Fields\n\n- 'image': A 'PIL.Image.Image' object containing the 28x28 image. Note that when accessing the image column: 'dataset[0][\"image\"]' the image file is automatically decoded. Decoding of a large number of image files might take a significant amount of time. Thus it is important to first query the sample index before the '\"image\"' column, *i.e.* 'dataset[0][\"image\"]' should always be preferred over 'dataset[\"image\"][0]'\n- 'label': an integer between 0 and 9 representing the digit.",
"### Data Splits\n\nThe data is split into training and test set. All the images in the test set were drawn by different individuals than the images in the training set. The training set contains 60,000 images and the test set 10,000 images.",
"## Dataset Creation",
"### Curation Rationale\n\nThe MNIST database was created to provide a testbed for people wanting to try pattern recognition methods or machine learning algorithms while spending minimal efforts on preprocessing and formatting. Images of the original dataset (NIST) were in two groups, one consisting of images drawn by Census Bureau employees and one consisting of images drawn by high school students. In NIST, the training set was built by grouping all the images of the Census Bureau employees, and the test set was built by grouping the images form the high school students.\nThe goal in building MNIST was to have a training and test set following the same distributions, so the training set contains 30,000 images drawn by Census Bureau employees and 30,000 images drawn by high school students, and the test set contains 5,000 images of each group. The curators took care to make sure all the images in the test set were drawn by different individuals than the images in the training set.",
"### Source Data",
"#### Initial Data Collection and Normalization\n\nThe original images from NIST were size normalized to fit a 20x20 pixel box while preserving their aspect ratio. The resulting images contain grey levels (i.e., pixels don't simply have a value of black and white, but a level of greyness from 0 to 255) as a result of the anti-aliasing technique used by the normalization algorithm. The images were then centered in a 28x28 image by computing the center of mass of the pixels, and translating the image so as to position this point at the center of the 28x28 field.",
"#### Who are the source language producers?\n\nHalf of the source images were drawn by Census Bureau employees, half by high school students. According to the dataset curator, the images from the first group are more easily recognizable.",
"### Annotations",
"#### Annotation process\n\nThe images were not annotated after their creation: the image creators annotated their images with the corresponding label after drawing them.",
"#### Who are the annotators?\n\nSame as the source data creators.",
"### Personal and Sensitive Information",
"## Considerations for Using the Data",
"### Social Impact of Dataset",
"### Discussion of Biases",
"### Other Known Limitations",
"## Additional Information",
"### Dataset Curators\n\nChris Burges, Corinna Cortes and Yann LeCun",
"### Licensing Information\n\nMIT Licence",
"### Contributions\n\nThanks to @sgugger for adding this dataset."
] | [
"TAGS\n#task_categories-image-classification #task_ids-multi-class-image-classification #annotations_creators-expert-generated #language_creators-found #multilinguality-monolingual #size_categories-10K<n<100K #source_datasets-extended|other-nist #language-English #license-mit #region-us \n",
"# Dataset Card for MNIST",
"## Table of Contents\n- Dataset Description\n - Dataset Summary\n - Supported Tasks and Leaderboards\n - Languages\n- Dataset Structure\n - Data Instances\n - Data Fields\n - Data Splits\n- Dataset Creation\n - Curation Rationale\n - Source Data\n - Annotations\n - Personal and Sensitive Information\n- Considerations for Using the Data\n - Social Impact of Dataset\n - Discussion of Biases\n - Other Known Limitations\n- Additional Information\n - Dataset Curators\n - Licensing Information\n - Citation Information\n - Contributions",
"## Dataset Description\n\n- Homepage: URL\n- Repository: \n- Paper: MNIST handwritten digit database by Yann LeCun, Corinna Cortes, and CJ Burges\n- Leaderboard:\n- Point of Contact:",
"### Dataset Summary\n\nThe MNIST dataset consists of 70,000 28x28 black-and-white images of handwritten digits extracted from two NIST databases. There are 60,000 images in the training dataset and 10,000 images in the validation dataset, one class per digit so a total of 10 classes, with 7,000 images (6,000 train images and 1,000 test images) per class.\nHalf of the image were drawn by Census Bureau employees and the other half by high school students (this split is evenly distributed in the training and testing sets).",
"### Supported Tasks and Leaderboards\n\n- 'image-classification': The goal of this task is to classify a given image of a handwritten digit into one of 10 classes representing integer values from 0 to 9, inclusively. The leaderboard is available here.",
"### Languages\n\nEnglish",
"## Dataset Structure",
"### Data Instances\n\nA data point comprises an image and its label:",
"### Data Fields\n\n- 'image': A 'PIL.Image.Image' object containing the 28x28 image. Note that when accessing the image column: 'dataset[0][\"image\"]' the image file is automatically decoded. Decoding of a large number of image files might take a significant amount of time. Thus it is important to first query the sample index before the '\"image\"' column, *i.e.* 'dataset[0][\"image\"]' should always be preferred over 'dataset[\"image\"][0]'\n- 'label': an integer between 0 and 9 representing the digit.",
"### Data Splits\n\nThe data is split into training and test set. All the images in the test set were drawn by different individuals than the images in the training set. The training set contains 60,000 images and the test set 10,000 images.",
"## Dataset Creation",
"### Curation Rationale\n\nThe MNIST database was created to provide a testbed for people wanting to try pattern recognition methods or machine learning algorithms while spending minimal efforts on preprocessing and formatting. Images of the original dataset (NIST) were in two groups, one consisting of images drawn by Census Bureau employees and one consisting of images drawn by high school students. In NIST, the training set was built by grouping all the images of the Census Bureau employees, and the test set was built by grouping the images form the high school students.\nThe goal in building MNIST was to have a training and test set following the same distributions, so the training set contains 30,000 images drawn by Census Bureau employees and 30,000 images drawn by high school students, and the test set contains 5,000 images of each group. The curators took care to make sure all the images in the test set were drawn by different individuals than the images in the training set.",
"### Source Data",
"#### Initial Data Collection and Normalization\n\nThe original images from NIST were size normalized to fit a 20x20 pixel box while preserving their aspect ratio. The resulting images contain grey levels (i.e., pixels don't simply have a value of black and white, but a level of greyness from 0 to 255) as a result of the anti-aliasing technique used by the normalization algorithm. The images were then centered in a 28x28 image by computing the center of mass of the pixels, and translating the image so as to position this point at the center of the 28x28 field.",
"#### Who are the source language producers?\n\nHalf of the source images were drawn by Census Bureau employees, half by high school students. According to the dataset curator, the images from the first group are more easily recognizable.",
"### Annotations",
"#### Annotation process\n\nThe images were not annotated after their creation: the image creators annotated their images with the corresponding label after drawing them.",
"#### Who are the annotators?\n\nSame as the source data creators.",
"### Personal and Sensitive Information",
"## Considerations for Using the Data",
"### Social Impact of Dataset",
"### Discussion of Biases",
"### Other Known Limitations",
"## Additional Information",
"### Dataset Curators\n\nChris Burges, Corinna Cortes and Yann LeCun",
"### Licensing Information\n\nMIT Licence",
"### Contributions\n\nThanks to @sgugger for adding this dataset."
] |
060a986afef8ef37e7410183b61d982472ec2860 | # Dataset Card for "LogoGeneration_png"
[More Information needed](https://github.com/huggingface/datasets/blob/main/CONTRIBUTING.md#how-to-contribute-to-the-dataset-cards) | AmanK1202/LogoGeneration_png | [
"region:us"
] | 2022-11-17T16:56:53+00:00 | {"dataset_info": {"features": [{"name": "image", "dtype": "image"}, {"name": "text", "dtype": "string"}], "splits": [{"name": "train", "num_bytes": 120298419.0, "num_examples": 821}], "download_size": 120174466, "dataset_size": 120298419.0}} | 2022-11-17T16:57:32+00:00 | [] | [] | TAGS
#region-us
| # Dataset Card for "LogoGeneration_png"
More Information needed | [
"# Dataset Card for \"LogoGeneration_png\"\n\nMore Information needed"
] | [
"TAGS\n#region-us \n",
"# Dataset Card for \"LogoGeneration_png\"\n\nMore Information needed"
] |
32b5c393dc9f8c6d9f278f61040c79f9235c44a0 | Subset dataset of [diffusiondb](https://huggingface.co/datasets/poloclub/diffusiondb) consisting of just unique prompts.
Created this subset dataset for the [Prompt Extend](https://github.com/daspartho/prompt-extend) project. | daspartho/stable-diffusion-prompts | [
"language:en",
"region:us"
] | 2022-11-17T17:25:56+00:00 | {"language": "en", "dataset_info": {"features": [{"name": "prompt", "dtype": "string"}], "splits": [{"name": "train", "num_bytes": 284636288, "num_examples": 1819808}], "download_size": 101931289, "dataset_size": 284636288}} | 2023-08-25T13:33:31+00:00 | [] | [
"en"
] | TAGS
#language-English #region-us
| Subset dataset of diffusiondb consisting of just unique prompts.
Created this subset dataset for the Prompt Extend project. | [] | [
"TAGS\n#language-English #region-us \n"
] |
6124bed5f88aac1f16b37b6b24e464b68c2853d5 | # Dataset Card for "olm-CC-MAIN-2022-40-sampling-ratio-0.0001-ne-language"
[More Information needed](https://github.com/huggingface/datasets/blob/main/CONTRIBUTING.md#how-to-contribute-to-the-dataset-cards) | Tristan/olm-CC-MAIN-2022-40-sampling-ratio-0.0001-ne-language | [
"region:us"
] | 2022-11-17T17:33:23+00:00 | {"dataset_info": {"features": [{"name": "text", "dtype": "string"}, {"name": "url", "dtype": "string"}, {"name": "crawl_timestamp", "dtype": "float64"}, {"name": "last_modified_timestamp", "dtype": "float64"}], "splits": [{"name": "train", "num_bytes": 136949.0, "num_examples": 37}], "download_size": 62812, "dataset_size": 136949.0}} | 2022-11-17T17:34:16+00:00 | [] | [] | TAGS
#region-us
| # Dataset Card for "olm-CC-MAIN-2022-40-sampling-ratio-0.0001-ne-language"
More Information needed | [
"# Dataset Card for \"olm-CC-MAIN-2022-40-sampling-ratio-0.0001-ne-language\"\n\nMore Information needed"
] | [
"TAGS\n#region-us \n",
"# Dataset Card for \"olm-CC-MAIN-2022-40-sampling-ratio-0.0001-ne-language\"\n\nMore Information needed"
] |
5e848b43d8c0ed4aa7ba7de05a7b510560d71100 |
# Stripe Style Embedding / Textual Inversion
<img alt="Showcase" src="https://huggingface.co/datasets/Nerfgun3/stripe_style/resolve/main/stripe_style_showcase.jpg"/>
## Usage
To use this embedding you have to download the file aswell as drop it into the "\stable-diffusion-webui\embeddings" folder
To use it in a prompt: ```"drawn by stripe_style"```
Personally, I would recommend to use my embeddings with a strength of 0.8, like ```"drawn by (stripe_style:0.8)"```
I trained the embedding two epochs until 5000 steps.
I hope you enjoy the embedding. If you have any questions, you can ask me anything via Discord: "Nerfgun3#7508"
## License
This embedding is open access and available to all, with a CreativeML OpenRAIL-M license further specifying rights and usage.
The CreativeML OpenRAIL License specifies:
1. You can't use the embedding to deliberately produce nor share illegal or harmful outputs or content
2. The authors claims no rights on the outputs you generate, you are free to use them and are accountable for their use which must not go against the provisions set in the license
3. You may re-distribute the weights and use the embedding commercially and/or as a service. If you do, please be aware you have to include the same use restrictions as the ones in the license and share a copy of the CreativeML OpenRAIL-M to all your users (please read the license entirely and carefully)
[Please read the full license here](https://huggingface.co/spaces/CompVis/stable-diffusion-license) | Nerfgun3/stripe_style | [
"language:en",
"license:creativeml-openrail-m",
"stable-diffusion",
"text-to-image",
"image-to-image",
"region:us"
] | 2022-11-17T17:47:24+00:00 | {"language": ["en"], "license": "creativeml-openrail-m", "thumbnail": "https://huggingface.co/datasets/Nerfgun3/stripe_style/resolve/main/stripe_style_showcase.jpg", "tags": ["stable-diffusion", "text-to-image", "image-to-image"], "inference": false} | 2022-11-17T17:55:11+00:00 | [] | [
"en"
] | TAGS
#language-English #license-creativeml-openrail-m #stable-diffusion #text-to-image #image-to-image #region-us
|
# Stripe Style Embedding / Textual Inversion
<img alt="Showcase" src="URL
## Usage
To use this embedding you have to download the file aswell as drop it into the "\stable-diffusion-webui\embeddings" folder
To use it in a prompt:
Personally, I would recommend to use my embeddings with a strength of 0.8, like
I trained the embedding two epochs until 5000 steps.
I hope you enjoy the embedding. If you have any questions, you can ask me anything via Discord: "Nerfgun3#7508"
## License
This embedding is open access and available to all, with a CreativeML OpenRAIL-M license further specifying rights and usage.
The CreativeML OpenRAIL License specifies:
1. You can't use the embedding to deliberately produce nor share illegal or harmful outputs or content
2. The authors claims no rights on the outputs you generate, you are free to use them and are accountable for their use which must not go against the provisions set in the license
3. You may re-distribute the weights and use the embedding commercially and/or as a service. If you do, please be aware you have to include the same use restrictions as the ones in the license and share a copy of the CreativeML OpenRAIL-M to all your users (please read the license entirely and carefully)
Please read the full license here | [
"# Stripe Style Embedding / Textual Inversion\n\n<img alt=\"Showcase\" src=\"URL",
"## Usage\n\nTo use this embedding you have to download the file aswell as drop it into the \"\\stable-diffusion-webui\\embeddings\" folder\n\nTo use it in a prompt: \n\nPersonally, I would recommend to use my embeddings with a strength of 0.8, like \n\nI trained the embedding two epochs until 5000 steps.\n\nI hope you enjoy the embedding. If you have any questions, you can ask me anything via Discord: \"Nerfgun3#7508\"",
"## License\n\nThis embedding is open access and available to all, with a CreativeML OpenRAIL-M license further specifying rights and usage.\nThe CreativeML OpenRAIL License specifies: \n\n1. You can't use the embedding to deliberately produce nor share illegal or harmful outputs or content \n2. The authors claims no rights on the outputs you generate, you are free to use them and are accountable for their use which must not go against the provisions set in the license\n3. You may re-distribute the weights and use the embedding commercially and/or as a service. If you do, please be aware you have to include the same use restrictions as the ones in the license and share a copy of the CreativeML OpenRAIL-M to all your users (please read the license entirely and carefully)\nPlease read the full license here"
] | [
"TAGS\n#language-English #license-creativeml-openrail-m #stable-diffusion #text-to-image #image-to-image #region-us \n",
"# Stripe Style Embedding / Textual Inversion\n\n<img alt=\"Showcase\" src=\"URL",
"## Usage\n\nTo use this embedding you have to download the file aswell as drop it into the \"\\stable-diffusion-webui\\embeddings\" folder\n\nTo use it in a prompt: \n\nPersonally, I would recommend to use my embeddings with a strength of 0.8, like \n\nI trained the embedding two epochs until 5000 steps.\n\nI hope you enjoy the embedding. If you have any questions, you can ask me anything via Discord: \"Nerfgun3#7508\"",
"## License\n\nThis embedding is open access and available to all, with a CreativeML OpenRAIL-M license further specifying rights and usage.\nThe CreativeML OpenRAIL License specifies: \n\n1. You can't use the embedding to deliberately produce nor share illegal or harmful outputs or content \n2. The authors claims no rights on the outputs you generate, you are free to use them and are accountable for their use which must not go against the provisions set in the license\n3. You may re-distribute the weights and use the embedding commercially and/or as a service. If you do, please be aware you have to include the same use restrictions as the ones in the license and share a copy of the CreativeML OpenRAIL-M to all your users (please read the license entirely and carefully)\nPlease read the full license here"
] |
f94e826e12c3589ff908d338492211a4ebabe7a9 |
# Dataset Summary
AfriCLIRMatrix is a test collection for cross-lingual information retrieval research in 15 diverse African languages. This resource comprises English queries with query–document relevance judgments in 15 African languages automatically mined from Wikipedia
This dataset stores documents of AfriCLIRMatrix. To access the queries and judgments, please refer to [castorini/africlirmatrix](https://github.com/castorini/africlirmatrix).
# Dataset Structure
The only configuration here is the `language`.
An example of document data entry looks as follows:
```
{
'id': '62443',
'contents': 'Acyloin condensation jẹ́ ìyọkúrò àsopọ̀ àwọn carboxylic ester pẹ̀lú lílò metalic sodium lati ṣèdá α-hydroxyketone, tí wọ́n tún mọ̀ sí. Àdàpọ̀ ṣisẹ́ yìí jẹ́ èyí tó ...'
}
```
# Load Dataset
An example to load the dataset:
```
language = 'yoruba'
dataset = load_dataset('castorini/africlirmatrix', language, 'train')
```
# Citation Information
```
coming soon
``` | castorini/africlirmatrix | [
"task_categories:text-retrieval",
"multilinguality:multilingual",
"language:af",
"language:am",
"language:arz",
"language:ha",
"language:ig",
"language:ary",
"language:nso",
"language:sn",
"language:so",
"language:sw",
"language:ti",
"language:tw",
"language:wo",
"language:yo",
"language:zu",
"license:apache-2.0",
"region:us"
] | 2022-11-17T18:41:37+00:00 | {"language": ["af", "am", "arz", "ha", "ig", "ary", "nso", "sn", "so", "sw", "ti", "tw", "wo", "yo", "zu"], "license": "apache-2.0", "multilinguality": ["multilingual"], "task_categories": ["text-retrieval"], "viewer": true} | 2022-11-17T22:45:16+00:00 | [] | [
"af",
"am",
"arz",
"ha",
"ig",
"ary",
"nso",
"sn",
"so",
"sw",
"ti",
"tw",
"wo",
"yo",
"zu"
] | TAGS
#task_categories-text-retrieval #multilinguality-multilingual #language-Afrikaans #language-Amharic #language-Egyptian Arabic #language-Hausa #language-Igbo #language-Moroccan Arabic #language-Pedi #language-Shona #language-Somali #language-Swahili (macrolanguage) #language-Tigrinya #language-Twi #language-Wolof #language-Yoruba #language-Zulu #license-apache-2.0 #region-us
|
# Dataset Summary
AfriCLIRMatrix is a test collection for cross-lingual information retrieval research in 15 diverse African languages. This resource comprises English queries with query–document relevance judgments in 15 African languages automatically mined from Wikipedia
This dataset stores documents of AfriCLIRMatrix. To access the queries and judgments, please refer to castorini/africlirmatrix.
# Dataset Structure
The only configuration here is the 'language'.
An example of document data entry looks as follows:
# Load Dataset
An example to load the dataset:
| [
"# Dataset Summary\nAfriCLIRMatrix is a test collection for cross-lingual information retrieval research in 15 diverse African languages. This resource comprises English queries with query–document relevance judgments in 15 African languages automatically mined from Wikipedia\n\nThis dataset stores documents of AfriCLIRMatrix. To access the queries and judgments, please refer to castorini/africlirmatrix.",
"# Dataset Structure\nThe only configuration here is the 'language'.\n\nAn example of document data entry looks as follows:",
"# Load Dataset\nAn example to load the dataset:"
] | [
"TAGS\n#task_categories-text-retrieval #multilinguality-multilingual #language-Afrikaans #language-Amharic #language-Egyptian Arabic #language-Hausa #language-Igbo #language-Moroccan Arabic #language-Pedi #language-Shona #language-Somali #language-Swahili (macrolanguage) #language-Tigrinya #language-Twi #language-Wolof #language-Yoruba #language-Zulu #license-apache-2.0 #region-us \n",
"# Dataset Summary\nAfriCLIRMatrix is a test collection for cross-lingual information retrieval research in 15 diverse African languages. This resource comprises English queries with query–document relevance judgments in 15 African languages automatically mined from Wikipedia\n\nThis dataset stores documents of AfriCLIRMatrix. To access the queries and judgments, please refer to castorini/africlirmatrix.",
"# Dataset Structure\nThe only configuration here is the 'language'.\n\nAn example of document data entry looks as follows:",
"# Load Dataset\nAn example to load the dataset:"
] |
8ef704329bd386ce35ab431822ddab563965eff2 | # Dataset Card for "hackathon_pil"
[More Information needed](https://github.com/huggingface/datasets/blob/main/CONTRIBUTING.md#how-to-contribute-to-the-dataset-cards) | akanksha8618/hackathon_pil | [
"region:us"
] | 2022-11-17T18:58:49+00:00 | {"dataset_info": {"features": [{"name": "image", "dtype": "image"}, {"name": "text", "dtype": "string"}], "splits": [{"name": "train", "num_bytes": 93369.0, "num_examples": 3}], "download_size": 93939, "dataset_size": 93369.0}} | 2022-11-17T18:59:04+00:00 | [] | [] | TAGS
#region-us
| # Dataset Card for "hackathon_pil"
More Information needed | [
"# Dataset Card for \"hackathon_pil\"\n\nMore Information needed"
] | [
"TAGS\n#region-us \n",
"# Dataset Card for \"hackathon_pil\"\n\nMore Information needed"
] |
1c7130f602fa130e3cdf1d72ff83da131efb3bbe | # Dataset Card for "hackathon_pil_v2"
[More Information needed](https://github.com/huggingface/datasets/blob/main/CONTRIBUTING.md#how-to-contribute-to-the-dataset-cards) | akanksha8618/hackathon_pil_v2 | [
"region:us"
] | 2022-11-17T19:06:00+00:00 | {"dataset_info": {"features": [{"name": "image", "dtype": "image"}, {"name": "text", "dtype": "string"}], "splits": [{"name": "train", "num_bytes": 93369.0, "num_examples": 3}], "download_size": 93939, "dataset_size": 93369.0}} | 2022-11-17T19:06:16+00:00 | [] | [] | TAGS
#region-us
| # Dataset Card for "hackathon_pil_v2"
More Information needed | [
"# Dataset Card for \"hackathon_pil_v2\"\n\nMore Information needed"
] | [
"TAGS\n#region-us \n",
"# Dataset Card for \"hackathon_pil_v2\"\n\nMore Information needed"
] |
d0925f0e223bcfb2840e66328835380f96f8f589 |
# Dataset Card for MultiLegalPile_Wikipedia_Filtered: A filtered version of the MultiLegalPile dataset, together with wikipedia articles
## Table of Contents
- [Table of Contents](#table-of-contents)
- [Dataset Description](#dataset-description)
- [Dataset Summary](#dataset-summary)
- [Supported Tasks and Leaderboards](#supported-tasks-and-leaderboards)
- [Languages](#languages)
- [Dataset Structure](#dataset-structure)
- [Data Instances](#data-instances)
- [Data Fields](#data-fields)
- [Data Splits](#data-splits)
- [Dataset Creation](#dataset-creation)
- [Curation Rationale](#curation-rationale)
- [Source Data](#source-data)
- [Annotations](#annotations)
- [Personal and Sensitive Information](#personal-and-sensitive-information)
- [Considerations for Using the Data](#considerations-for-using-the-data)
- [Social Impact of Dataset](#social-impact-of-dataset)
- [Discussion of Biases](#discussion-of-biases)
- [Other Known Limitations](#other-known-limitations)
- [Additional Information](#additional-information)
- [Dataset Curators](#dataset-curators)
- [Licensing Information](#licensing-information)
- [Citation Information](#citation-information)
- [Contributions](#contributions)
## Dataset Description
- **Homepage:**
- **Repository:**
- **Paper:**
- **Leaderboard:**
- **Point of Contact:** [Joel Niklaus](mailto:[email protected])
### Dataset Summary
The Multi_Legal_Pile is a large-scale multilingual legal dataset suited for pretraining language models.
It spans over 24 languages and four legal text types.
### Supported Tasks and Leaderboards
The dataset supports the tasks of fill-mask.
### Languages
The following languages are supported:
bg, cs, da, de, el, en, es, et, fi, fr, ga, hr, hu, it, lt, lv, mt, nl, pl, pt, ro, sk, sl, sv
## Dataset Structure
It is structured in the following format: {language}_{text_type}_{shard}.jsonl.xz
text_type is one of the following:
- caselaw
- contracts
- legislation
- other
- wikipedia
Use the dataset like this:
```python
from datasets import load_dataset
config = 'en_contracts' # {language}_{text_type}
dataset = load_dataset('joelito/Multi_Legal_Pile', config, split='train', streaming=True)
```
'config' is a combination of language and text_type, e.g. 'en_contracts' or 'de_caselaw'.
To load all the languages or all the text_types, use 'all' instead of the language or text_type (e.g., '
all_legislation').
### Data Instances
The file format is jsonl.xz and there is a `train` and `validation` split available.
Since some configurations are very small or non-existent, they might not contain a train split or not be present at all.
The complete dataset consists of five large subsets:
- [Native Multi Legal Pile](https://huggingface.co/datasets/joelito/Multi_Legal_Pile)
- [Eurlex Resources](https://huggingface.co/datasets/joelito/eurlex_resources)
- [MC4 Legal](https://huggingface.co/datasets/joelito/mc4_legal)
- [Pile of Law](https://huggingface.co/datasets/pile-of-law/pile-of-law)
- [EU Wikipedias](https://huggingface.co/datasets/joelito/EU_Wikipedias)
### Data Fields
[More Information Needed]
### Data Splits
[More Information Needed]
## Dataset Creation
This dataset has been created by combining the following datasets:
Native Multi Legal Pile, Eurlex Resources, MC4 Legal, Pile of Law, EU Wikipedias.
It has been filtered to remove short documents (less than 64 whitespace-separated tokens) and
documents with more than 30% punctuation or numbers (see prepare_legal_data.py for more details).
### Curation Rationale
[More Information Needed]
### Source Data
#### Initial Data Collection and Normalization
[More Information Needed]
#### Who are the source language producers?
[More Information Needed]
### Annotations
#### Annotation process
[More Information Needed]
#### Who are the annotators?
[More Information Needed]
### Personal and Sensitive Information
[More Information Needed]
## Considerations for Using the Data
### Social Impact of Dataset
[More Information Needed]
### Discussion of Biases
[More Information Needed]
### Other Known Limitations
[More Information Needed]
## Additional Information
### Dataset Curators
[More Information Needed]
### Licensing Information
[More Information Needed]
### Citation Information
```
TODO add citation
```
### Contributions
Thanks to [@JoelNiklaus](https://github.com/joelniklaus) for adding this dataset.
| joelniklaus/MultiLegalPile_Wikipedia_Filtered | [
"task_categories:fill-mask",
"annotations_creators:other",
"language_creators:found",
"multilinguality:multilingual",
"size_categories:10M<n<100M",
"source_datasets:original",
"language:bg",
"language:cs",
"language:da",
"language:de",
"language:el",
"language:en",
"language:es",
"language:et",
"language:fi",
"language:fr",
"language:ga",
"language:hr",
"language:hu",
"language:it",
"language:lt",
"language:lv",
"language:mt",
"language:nl",
"language:pl",
"language:pt",
"language:ro",
"language:sk",
"language:sl",
"language:sv",
"license:cc-by-4.0",
"region:us"
] | 2022-11-17T19:28:00+00:00 | {"annotations_creators": ["other"], "language_creators": ["found"], "language": ["bg", "cs", "da", "de", "el", "en", "es", "et", "fi", "fr", "ga", "hr", "hu", "it", "lt", "lv", "mt", "nl", "pl", "pt", "ro", "sk", "sl", "sv"], "license": ["cc-by-4.0"], "multilinguality": ["multilingual"], "size_categories": ["10M<n<100M"], "source_datasets": ["original"], "task_categories": ["fill-mask"], "pretty_name": "MultiLegalPile_Wikipedia_Filtered: A filtered version of the MultiLegalPile dataset, together with wikipedia articles."} | 2022-11-29T21:52:23+00:00 | [] | [
"bg",
"cs",
"da",
"de",
"el",
"en",
"es",
"et",
"fi",
"fr",
"ga",
"hr",
"hu",
"it",
"lt",
"lv",
"mt",
"nl",
"pl",
"pt",
"ro",
"sk",
"sl",
"sv"
] | TAGS
#task_categories-fill-mask #annotations_creators-other #language_creators-found #multilinguality-multilingual #size_categories-10M<n<100M #source_datasets-original #language-Bulgarian #language-Czech #language-Danish #language-German #language-Modern Greek (1453-) #language-English #language-Spanish #language-Estonian #language-Finnish #language-French #language-Irish #language-Croatian #language-Hungarian #language-Italian #language-Lithuanian #language-Latvian #language-Maltese #language-Dutch #language-Polish #language-Portuguese #language-Romanian #language-Slovak #language-Slovenian #language-Swedish #license-cc-by-4.0 #region-us
|
# Dataset Card for MultiLegalPile_Wikipedia_Filtered: A filtered version of the MultiLegalPile dataset, together with wikipedia articles
## Table of Contents
- Table of Contents
- Dataset Description
- Dataset Summary
- Supported Tasks and Leaderboards
- Languages
- Dataset Structure
- Data Instances
- Data Fields
- Data Splits
- Dataset Creation
- Curation Rationale
- Source Data
- Annotations
- Personal and Sensitive Information
- Considerations for Using the Data
- Social Impact of Dataset
- Discussion of Biases
- Other Known Limitations
- Additional Information
- Dataset Curators
- Licensing Information
- Citation Information
- Contributions
## Dataset Description
- Homepage:
- Repository:
- Paper:
- Leaderboard:
- Point of Contact: Joel Niklaus
### Dataset Summary
The Multi_Legal_Pile is a large-scale multilingual legal dataset suited for pretraining language models.
It spans over 24 languages and four legal text types.
### Supported Tasks and Leaderboards
The dataset supports the tasks of fill-mask.
### Languages
The following languages are supported:
bg, cs, da, de, el, en, es, et, fi, fr, ga, hr, hu, it, lt, lv, mt, nl, pl, pt, ro, sk, sl, sv
## Dataset Structure
It is structured in the following format: {language}_{text_type}_{shard}.URL
text_type is one of the following:
- caselaw
- contracts
- legislation
- other
- wikipedia
Use the dataset like this:
'config' is a combination of language and text_type, e.g. 'en_contracts' or 'de_caselaw'.
To load all the languages or all the text_types, use 'all' instead of the language or text_type (e.g., '
all_legislation').
### Data Instances
The file format is URL and there is a 'train' and 'validation' split available.
Since some configurations are very small or non-existent, they might not contain a train split or not be present at all.
The complete dataset consists of five large subsets:
- Native Multi Legal Pile
- Eurlex Resources
- MC4 Legal
- Pile of Law
- EU Wikipedias
### Data Fields
### Data Splits
## Dataset Creation
This dataset has been created by combining the following datasets:
Native Multi Legal Pile, Eurlex Resources, MC4 Legal, Pile of Law, EU Wikipedias.
It has been filtered to remove short documents (less than 64 whitespace-separated tokens) and
documents with more than 30% punctuation or numbers (see prepare_legal_data.py for more details).
### Curation Rationale
### Source Data
#### Initial Data Collection and Normalization
#### Who are the source language producers?
### Annotations
#### Annotation process
#### Who are the annotators?
### Personal and Sensitive Information
## Considerations for Using the Data
### Social Impact of Dataset
### Discussion of Biases
### Other Known Limitations
## Additional Information
### Dataset Curators
### Licensing Information
### Contributions
Thanks to @JoelNiklaus for adding this dataset.
| [
"# Dataset Card for MultiLegalPile_Wikipedia_Filtered: A filtered version of the MultiLegalPile dataset, together with wikipedia articles",
"## Table of Contents\n\n- Table of Contents\n- Dataset Description\n - Dataset Summary\n - Supported Tasks and Leaderboards\n - Languages\n- Dataset Structure\n - Data Instances\n - Data Fields\n - Data Splits\n- Dataset Creation\n - Curation Rationale\n - Source Data\n - Annotations\n - Personal and Sensitive Information\n- Considerations for Using the Data\n - Social Impact of Dataset\n - Discussion of Biases\n - Other Known Limitations\n- Additional Information\n - Dataset Curators\n - Licensing Information\n - Citation Information\n - Contributions",
"## Dataset Description\n\n- Homepage:\n- Repository: \n- Paper: \n- Leaderboard:\n- Point of Contact: Joel Niklaus",
"### Dataset Summary\n\nThe Multi_Legal_Pile is a large-scale multilingual legal dataset suited for pretraining language models.\nIt spans over 24 languages and four legal text types.",
"### Supported Tasks and Leaderboards\n\nThe dataset supports the tasks of fill-mask.",
"### Languages\n\nThe following languages are supported: \nbg, cs, da, de, el, en, es, et, fi, fr, ga, hr, hu, it, lt, lv, mt, nl, pl, pt, ro, sk, sl, sv",
"## Dataset Structure\n\nIt is structured in the following format: {language}_{text_type}_{shard}.URL\n\ntext_type is one of the following:\n\n- caselaw\n- contracts\n- legislation\n- other\n- wikipedia\n\n\nUse the dataset like this:\n\n\n'config' is a combination of language and text_type, e.g. 'en_contracts' or 'de_caselaw'.\nTo load all the languages or all the text_types, use 'all' instead of the language or text_type (e.g., '\nall_legislation').",
"### Data Instances\n\nThe file format is URL and there is a 'train' and 'validation' split available. \nSince some configurations are very small or non-existent, they might not contain a train split or not be present at all.\n\nThe complete dataset consists of five large subsets:\n- Native Multi Legal Pile\n- Eurlex Resources \n- MC4 Legal\n- Pile of Law\n- EU Wikipedias",
"### Data Fields",
"### Data Splits",
"## Dataset Creation\n\nThis dataset has been created by combining the following datasets:\nNative Multi Legal Pile, Eurlex Resources, MC4 Legal, Pile of Law, EU Wikipedias.\nIt has been filtered to remove short documents (less than 64 whitespace-separated tokens) and \ndocuments with more than 30% punctuation or numbers (see prepare_legal_data.py for more details).",
"### Curation Rationale",
"### Source Data",
"#### Initial Data Collection and Normalization",
"#### Who are the source language producers?",
"### Annotations",
"#### Annotation process",
"#### Who are the annotators?",
"### Personal and Sensitive Information",
"## Considerations for Using the Data",
"### Social Impact of Dataset",
"### Discussion of Biases",
"### Other Known Limitations",
"## Additional Information",
"### Dataset Curators",
"### Licensing Information",
"### Contributions\n\nThanks to @JoelNiklaus for adding this dataset."
] | [
"TAGS\n#task_categories-fill-mask #annotations_creators-other #language_creators-found #multilinguality-multilingual #size_categories-10M<n<100M #source_datasets-original #language-Bulgarian #language-Czech #language-Danish #language-German #language-Modern Greek (1453-) #language-English #language-Spanish #language-Estonian #language-Finnish #language-French #language-Irish #language-Croatian #language-Hungarian #language-Italian #language-Lithuanian #language-Latvian #language-Maltese #language-Dutch #language-Polish #language-Portuguese #language-Romanian #language-Slovak #language-Slovenian #language-Swedish #license-cc-by-4.0 #region-us \n",
"# Dataset Card for MultiLegalPile_Wikipedia_Filtered: A filtered version of the MultiLegalPile dataset, together with wikipedia articles",
"## Table of Contents\n\n- Table of Contents\n- Dataset Description\n - Dataset Summary\n - Supported Tasks and Leaderboards\n - Languages\n- Dataset Structure\n - Data Instances\n - Data Fields\n - Data Splits\n- Dataset Creation\n - Curation Rationale\n - Source Data\n - Annotations\n - Personal and Sensitive Information\n- Considerations for Using the Data\n - Social Impact of Dataset\n - Discussion of Biases\n - Other Known Limitations\n- Additional Information\n - Dataset Curators\n - Licensing Information\n - Citation Information\n - Contributions",
"## Dataset Description\n\n- Homepage:\n- Repository: \n- Paper: \n- Leaderboard:\n- Point of Contact: Joel Niklaus",
"### Dataset Summary\n\nThe Multi_Legal_Pile is a large-scale multilingual legal dataset suited for pretraining language models.\nIt spans over 24 languages and four legal text types.",
"### Supported Tasks and Leaderboards\n\nThe dataset supports the tasks of fill-mask.",
"### Languages\n\nThe following languages are supported: \nbg, cs, da, de, el, en, es, et, fi, fr, ga, hr, hu, it, lt, lv, mt, nl, pl, pt, ro, sk, sl, sv",
"## Dataset Structure\n\nIt is structured in the following format: {language}_{text_type}_{shard}.URL\n\ntext_type is one of the following:\n\n- caselaw\n- contracts\n- legislation\n- other\n- wikipedia\n\n\nUse the dataset like this:\n\n\n'config' is a combination of language and text_type, e.g. 'en_contracts' or 'de_caselaw'.\nTo load all the languages or all the text_types, use 'all' instead of the language or text_type (e.g., '\nall_legislation').",
"### Data Instances\n\nThe file format is URL and there is a 'train' and 'validation' split available. \nSince some configurations are very small or non-existent, they might not contain a train split or not be present at all.\n\nThe complete dataset consists of five large subsets:\n- Native Multi Legal Pile\n- Eurlex Resources \n- MC4 Legal\n- Pile of Law\n- EU Wikipedias",
"### Data Fields",
"### Data Splits",
"## Dataset Creation\n\nThis dataset has been created by combining the following datasets:\nNative Multi Legal Pile, Eurlex Resources, MC4 Legal, Pile of Law, EU Wikipedias.\nIt has been filtered to remove short documents (less than 64 whitespace-separated tokens) and \ndocuments with more than 30% punctuation or numbers (see prepare_legal_data.py for more details).",
"### Curation Rationale",
"### Source Data",
"#### Initial Data Collection and Normalization",
"#### Who are the source language producers?",
"### Annotations",
"#### Annotation process",
"#### Who are the annotators?",
"### Personal and Sensitive Information",
"## Considerations for Using the Data",
"### Social Impact of Dataset",
"### Discussion of Biases",
"### Other Known Limitations",
"## Additional Information",
"### Dataset Curators",
"### Licensing Information",
"### Contributions\n\nThanks to @JoelNiklaus for adding this dataset."
] |
26a7b45850bfdafeda574d1bc79b2f16700748e1 |
# Negative Embedding / Textual Inversion
<img alt="Showcase" src="https://huggingface.co/datasets/Nerfgun3/bad_prompt/resolve/main/bad_prompt_showcase.jpg"/>
## Idea
The idea behind this embedding was to somehow train the negative prompt as an embedding, thus unifying the basis of the negative prompt into one word or embedding.
Side note: Embedding has proven to be very helpful for the generation of hands! :)
## Usage
To use this embedding you have to download the file aswell as drop it into the "\stable-diffusion-webui\embeddings" folder.
**Please put the embedding in the negative prompt to get the right results!**
For special negative tags such as "malformed sword", you still need to add them yourself. The negative embedding is trained on a basic skeleton for the negative prompt, which should provide a high-resolution image as a result.
### Version 1:
Issue: Changing the style to much.
To use it in the negative prompt: ```"bad_prompt"```
Personally, I would recommend to use my embeddings with a strength of 0.8 even the negative embeddings, like ```"(bad_prompt:0.8)"```
### Version 2:
With this version I tried to reduce the amount of vectors used, aswell as the issue with the changing artstyle. The newer version is still a work in progress, but its already way better than the first version. Its in files section!
I hope you enjoy the embedding. If you have any questions, you can ask me anything via Discord: "Nerfgun3#7508"
## License
This embedding is open access and available to all, with a CreativeML OpenRAIL-M license further specifying rights and usage.
The CreativeML OpenRAIL License specifies:
1. You can't use the embedding to deliberately produce nor share illegal or harmful outputs or content
2. The authors claims no rights on the outputs you generate, you are free to use them and are accountable for their use which must not go against the provisions set in the license
3. You may re-distribute the weights and use the embedding commercially and/or as a service. If you do, please be aware you have to include the same use restrictions as the ones in the license and share a copy of the CreativeML OpenRAIL-M to all your users (please read the license entirely and carefully)
[Please read the full license here](https://huggingface.co/spaces/CompVis/stable-diffusion-license) | Nerfgun3/bad_prompt | [
"language:en",
"license:creativeml-openrail-m",
"stable-diffusion",
"text-to-image",
"image-to-image",
"region:us"
] | 2022-11-17T20:47:06+00:00 | {"language": ["en"], "license": "creativeml-openrail-m", "thumbnail": "https://huggingface.co/datasets/Nerfgun3/bad_prompt/resolve/main/bad_prompt_showcase.jpg", "tags": ["stable-diffusion", "text-to-image", "image-to-image"], "inference": false} | 2022-11-19T23:43:47+00:00 | [] | [
"en"
] | TAGS
#language-English #license-creativeml-openrail-m #stable-diffusion #text-to-image #image-to-image #region-us
|
# Negative Embedding / Textual Inversion
<img alt="Showcase" src="URL
## Idea
The idea behind this embedding was to somehow train the negative prompt as an embedding, thus unifying the basis of the negative prompt into one word or embedding.
Side note: Embedding has proven to be very helpful for the generation of hands! :)
## Usage
To use this embedding you have to download the file aswell as drop it into the "\stable-diffusion-webui\embeddings" folder.
Please put the embedding in the negative prompt to get the right results!
For special negative tags such as "malformed sword", you still need to add them yourself. The negative embedding is trained on a basic skeleton for the negative prompt, which should provide a high-resolution image as a result.
### Version 1:
Issue: Changing the style to much.
To use it in the negative prompt:
Personally, I would recommend to use my embeddings with a strength of 0.8 even the negative embeddings, like
### Version 2:
With this version I tried to reduce the amount of vectors used, aswell as the issue with the changing artstyle. The newer version is still a work in progress, but its already way better than the first version. Its in files section!
I hope you enjoy the embedding. If you have any questions, you can ask me anything via Discord: "Nerfgun3#7508"
## License
This embedding is open access and available to all, with a CreativeML OpenRAIL-M license further specifying rights and usage.
The CreativeML OpenRAIL License specifies:
1. You can't use the embedding to deliberately produce nor share illegal or harmful outputs or content
2. The authors claims no rights on the outputs you generate, you are free to use them and are accountable for their use which must not go against the provisions set in the license
3. You may re-distribute the weights and use the embedding commercially and/or as a service. If you do, please be aware you have to include the same use restrictions as the ones in the license and share a copy of the CreativeML OpenRAIL-M to all your users (please read the license entirely and carefully)
Please read the full license here | [
"# Negative Embedding / Textual Inversion\n\n<img alt=\"Showcase\" src=\"URL",
"## Idea\n\nThe idea behind this embedding was to somehow train the negative prompt as an embedding, thus unifying the basis of the negative prompt into one word or embedding. \n\nSide note: Embedding has proven to be very helpful for the generation of hands! :)",
"## Usage\n\nTo use this embedding you have to download the file aswell as drop it into the \"\\stable-diffusion-webui\\embeddings\" folder.\n\nPlease put the embedding in the negative prompt to get the right results!\n\nFor special negative tags such as \"malformed sword\", you still need to add them yourself. The negative embedding is trained on a basic skeleton for the negative prompt, which should provide a high-resolution image as a result.",
"### Version 1:\n\nIssue: Changing the style to much.\n\nTo use it in the negative prompt: \n\nPersonally, I would recommend to use my embeddings with a strength of 0.8 even the negative embeddings, like",
"### Version 2:\n\nWith this version I tried to reduce the amount of vectors used, aswell as the issue with the changing artstyle. The newer version is still a work in progress, but its already way better than the first version. Its in files section!\n\nI hope you enjoy the embedding. If you have any questions, you can ask me anything via Discord: \"Nerfgun3#7508\"",
"## License\n\nThis embedding is open access and available to all, with a CreativeML OpenRAIL-M license further specifying rights and usage.\nThe CreativeML OpenRAIL License specifies: \n\n1. You can't use the embedding to deliberately produce nor share illegal or harmful outputs or content \n2. The authors claims no rights on the outputs you generate, you are free to use them and are accountable for their use which must not go against the provisions set in the license\n3. You may re-distribute the weights and use the embedding commercially and/or as a service. If you do, please be aware you have to include the same use restrictions as the ones in the license and share a copy of the CreativeML OpenRAIL-M to all your users (please read the license entirely and carefully)\nPlease read the full license here"
] | [
"TAGS\n#language-English #license-creativeml-openrail-m #stable-diffusion #text-to-image #image-to-image #region-us \n",
"# Negative Embedding / Textual Inversion\n\n<img alt=\"Showcase\" src=\"URL",
"## Idea\n\nThe idea behind this embedding was to somehow train the negative prompt as an embedding, thus unifying the basis of the negative prompt into one word or embedding. \n\nSide note: Embedding has proven to be very helpful for the generation of hands! :)",
"## Usage\n\nTo use this embedding you have to download the file aswell as drop it into the \"\\stable-diffusion-webui\\embeddings\" folder.\n\nPlease put the embedding in the negative prompt to get the right results!\n\nFor special negative tags such as \"malformed sword\", you still need to add them yourself. The negative embedding is trained on a basic skeleton for the negative prompt, which should provide a high-resolution image as a result.",
"### Version 1:\n\nIssue: Changing the style to much.\n\nTo use it in the negative prompt: \n\nPersonally, I would recommend to use my embeddings with a strength of 0.8 even the negative embeddings, like",
"### Version 2:\n\nWith this version I tried to reduce the amount of vectors used, aswell as the issue with the changing artstyle. The newer version is still a work in progress, but its already way better than the first version. Its in files section!\n\nI hope you enjoy the embedding. If you have any questions, you can ask me anything via Discord: \"Nerfgun3#7508\"",
"## License\n\nThis embedding is open access and available to all, with a CreativeML OpenRAIL-M license further specifying rights and usage.\nThe CreativeML OpenRAIL License specifies: \n\n1. You can't use the embedding to deliberately produce nor share illegal or harmful outputs or content \n2. The authors claims no rights on the outputs you generate, you are free to use them and are accountable for their use which must not go against the provisions set in the license\n3. You may re-distribute the weights and use the embedding commercially and/or as a service. If you do, please be aware you have to include the same use restrictions as the ones in the license and share a copy of the CreativeML OpenRAIL-M to all your users (please read the license entirely and carefully)\nPlease read the full license here"
] |
5b1bb2ed401d4c3384702e2bb011e4eb379b2396 | from datasets import load_dataset
| purplecat24/Russel | [
"region:us"
] | 2022-11-17T21:06:39+00:00 | {} | 2022-11-17T21:29:28+00:00 | [] | [] | TAGS
#region-us
| from datasets import load_dataset
| [] | [
"TAGS\n#region-us \n"
] |
13054fc9d7475eebe9919802a5ae36f36abdc567 | # Dataset Card for "mtop"
[More Information needed](https://github.com/huggingface/datasets/blob/main/CONTRIBUTING.md#how-to-contribute-to-the-dataset-cards) | WillHeld/mtop | [
"region:us"
] | 2022-11-17T21:54:47+00:00 | {"dataset_info": {"features": [{"name": "id", "dtype": "int64"}, {"name": " intent", "dtype": "string"}, {"name": " slot", "dtype": "string"}, {"name": " utterance", "dtype": "string"}, {"name": " domain", "dtype": "string"}, {"name": " locale", "dtype": "string"}, {"name": " dcp_form", "dtype": "string"}, {"name": " tokens", "dtype": "string"}, {"name": "intent", "dtype": "string"}, {"name": "slot", "dtype": "string"}, {"name": "utterance", "dtype": "string"}, {"name": "domain", "dtype": "string"}, {"name": "locale", "dtype": "string"}, {"name": "dcp_form", "dtype": "string"}, {"name": "tokens", "dtype": "string"}], "splits": [{"name": "eval_en", "num_bytes": 2077234, "num_examples": 2235}, {"name": "test_en", "num_bytes": 4090856, "num_examples": 4386}, {"name": "train_en", "num_bytes": 14501480, "num_examples": 15667}, {"name": "eval_de", "num_bytes": 1764320, "num_examples": 1815}, {"name": "test_de", "num_bytes": 3439946, "num_examples": 3549}, {"name": "train_de", "num_bytes": 13122042, "num_examples": 13424}, {"name": "eval_es", "num_bytes": 1594238, "num_examples": 1527}, {"name": "test_es", "num_bytes": 3089782, "num_examples": 2998}, {"name": "train_es", "num_bytes": 11277514, "num_examples": 10934}, {"name": "eval_fr", "num_bytes": 1607082, "num_examples": 1577}, {"name": "test_fr", "num_bytes": 3289276, "num_examples": 3193}, {"name": "train_fr", "num_bytes": 12147836, "num_examples": 11814}, {"name": "eval_hi", "num_bytes": 2618172, "num_examples": 2012}, {"name": "test_hi", "num_bytes": 3491690, "num_examples": 2789}, {"name": "train_hi", "num_bytes": 14225324, "num_examples": 11330}, {"name": "eval_th", "num_bytes": 2251378, "num_examples": 1671}, {"name": "test_th", "num_bytes": 3654864, "num_examples": 2765}, {"name": "train_th", "num_bytes": 14277512, "num_examples": 10759}], "download_size": 16165451, "dataset_size": 112520546}} | 2022-12-10T17:50:10+00:00 | [] | [] | TAGS
#region-us
| # Dataset Card for "mtop"
More Information needed | [
"# Dataset Card for \"mtop\"\n\nMore Information needed"
] | [
"TAGS\n#region-us \n",
"# Dataset Card for \"mtop\"\n\nMore Information needed"
] |
53404b8688a8bb2504a3717a345f8fc85c29ee61 | # Dataset Card for "hinglish_top"
License: https://github.com/google-research-datasets/Hinglish-TOP-Dataset/blob/main/LICENSE.md
Original Repo: https://github.com/google-research-datasets/Hinglish-TOP-Dataset
Paper Link For Citation: https://arxiv.org/pdf/2211.07514.pdf
[More Information needed](https://github.com/huggingface/datasets/blob/main/CONTRIBUTING.md#how-to-contribute-to-the-dataset-cards) | WillHeld/hinglish_top | [
"arxiv:2211.07514",
"region:us"
] | 2022-11-17T22:01:20+00:00 | {"dataset_info": {"features": [{"name": "en_query", "dtype": "string"}, {"name": "cs_query", "dtype": "string"}, {"name": "en_parse", "dtype": "string"}, {"name": "cs_parse", "dtype": "string"}, {"name": "domain", "dtype": "string"}], "splits": [{"name": "validation", "num_bytes": 411962, "num_examples": 1390}, {"name": "test", "num_bytes": 2003034, "num_examples": 6513}, {"name": "train", "num_bytes": 894606, "num_examples": 2993}], "download_size": 1553636, "dataset_size": 3309602}} | 2022-12-10T17:51:03+00:00 | [
"2211.07514"
] | [] | TAGS
#arxiv-2211.07514 #region-us
| # Dataset Card for "hinglish_top"
License: URL
Original Repo: URL
Paper Link For Citation: URL
More Information needed | [
"# Dataset Card for \"hinglish_top\"\n\nLicense: URL\nOriginal Repo: URL\nPaper Link For Citation: URL\n\nMore Information needed"
] | [
"TAGS\n#arxiv-2211.07514 #region-us \n",
"# Dataset Card for \"hinglish_top\"\n\nLicense: URL\nOriginal Repo: URL\nPaper Link For Citation: URL\n\nMore Information needed"
] |
ade45482b1fa163b34177963c1e6f4d29621e24f |
**Homepage:** https://www.nb.no/sprakbanken/en/resource-catalogue/oai-nb-no-sbr-56
Used lydfiler_16_1.tar.gz and metadata_se_csv.zip | jzju/nst | [
"task_categories:automatic-speech-recognition",
"language:sv",
"license:cc0-1.0",
"region:us"
] | 2022-11-17T22:47:45+00:00 | {"language": ["sv"], "license": ["cc0-1.0"], "task_categories": ["automatic-speech-recognition"], "pretty_name": "NST"} | 2022-11-17T23:35:18+00:00 | [] | [
"sv"
] | TAGS
#task_categories-automatic-speech-recognition #language-Swedish #license-cc0-1.0 #region-us
|
Homepage: URL
Used lydfiler_16_1.URL and metadata_se_csv.zip | [] | [
"TAGS\n#task_categories-automatic-speech-recognition #language-Swedish #license-cc0-1.0 #region-us \n"
] |
d9c4b7fe6948e8651d914b111367c4be9f2f0269 |
# Dataset Card for "Reddit Haiku"
This dataset contains haikus from the subreddit [/r/haiku](https://www.reddit.com/r/haiku/) scraped and filtered between October 19th and 10th 2022, combined with a [previous dump](https://zissou.infosci.cornell.edu/convokit/datasets/subreddit-corpus/corpus-zipped/hackintosh_ja~-~hamsters/) of that same subreddit packaged by [ConvoKit](https://convokit.cornell.edu/documentation/subreddit.html) as part of the Subreddit Corpus, which is itself a subset of [pushshift.io](https://pushshift.io/)'s big dump.
A main motivation for this dataset was to collect an alternative haiku dataset for evaluation, in particular for evaluating Fabian Mueller's Deep Haiku [model](fabianmmueller/deep-haiku-gpt-j-6b-8bit) which was trained on the Haiku datasets of [hjhalani30](https://www.kaggle.com/datasets/hjhalani30/haiku-dataset) and [bfbarry](https://www.kaggle.com/datasets/bfbarry/haiku-dataset), which are also available on [huggingface hub](https://huggingface.co/datasets/statworx/haiku).
## Fields
The fields are post id (`id`), the content of the haiku (`processed_title`), upvotes (`ups`), and topic keywords (`keywords`). Topic keywords for each haiku have been extracted with the [KeyBERT library](https://maartengr.github.io/KeyBERT/guides/quickstart.html) and truncated to top-5 keywords.
## Usage
This dataset is intended for evaluation, hence there is only one split which is `test`.
```python
from datasets import load_dataset
d=load_dataset('huanggab/reddit_haiku', data_files='test':'merged_with_keywords.csv'}) # use data_files or it will result in error
>>> print(d['train'][0])
#{'Unnamed: 0': 0, 'id': '1020ac', 'processed_title': "There's nothing inside/There is nothing outside me/I search on in hope.", 'ups': 5, 'keywords': "[('inside', 0.5268), ('outside', 0.3751), ('search', 0.3367), ('hope', 0.272)]"}
```
There is code for scraping and processing in `processing_code`, and a subset of the data with more fields such as author Karma, downvotes and posting time at `processing_code/reddit-2022-10-20-dump.csv`. | huanggab/reddit_haiku | [
"task_categories:text-generation",
"task_ids:language-modeling",
"annotations_creators:found",
"language_creators:found",
"multilinguality:monolingual",
"size_categories:10K<n<100K",
"source_datasets:extended|other",
"language:en",
"license:unknown",
"haiku",
"poem",
"poetry",
"reddit",
"keybert",
"generation",
"region:us"
] | 2022-11-17T23:02:12+00:00 | {"annotations_creators": ["found"], "language_creators": ["found"], "language": ["en"], "license": ["unknown"], "multilinguality": ["monolingual"], "size_categories": ["10K<n<100K"], "source_datasets": ["extended|other"], "task_categories": ["text-generation"], "task_ids": ["language-modeling"], "pretty_name": "English haiku dataset scraped from Reddit's /r/haiku with topics extracted using KeyBERT", "tags": ["haiku", "poem", "poetry", "reddit", "keybert", "generation"]} | 2022-11-18T20:02:29+00:00 | [] | [
"en"
] | TAGS
#task_categories-text-generation #task_ids-language-modeling #annotations_creators-found #language_creators-found #multilinguality-monolingual #size_categories-10K<n<100K #source_datasets-extended|other #language-English #license-unknown #haiku #poem #poetry #reddit #keybert #generation #region-us
|
# Dataset Card for "Reddit Haiku"
This dataset contains haikus from the subreddit /r/haiku scraped and filtered between October 19th and 10th 2022, combined with a previous dump of that same subreddit packaged by ConvoKit as part of the Subreddit Corpus, which is itself a subset of URL's big dump.
A main motivation for this dataset was to collect an alternative haiku dataset for evaluation, in particular for evaluating Fabian Mueller's Deep Haiku model which was trained on the Haiku datasets of hjhalani30 and bfbarry, which are also available on huggingface hub.
## Fields
The fields are post id ('id'), the content of the haiku ('processed_title'), upvotes ('ups'), and topic keywords ('keywords'). Topic keywords for each haiku have been extracted with the KeyBERT library and truncated to top-5 keywords.
## Usage
This dataset is intended for evaluation, hence there is only one split which is 'test'.
There is code for scraping and processing in 'processing_code', and a subset of the data with more fields such as author Karma, downvotes and posting time at 'processing_code/URL'. | [
"# Dataset Card for \"Reddit Haiku\"\n\nThis dataset contains haikus from the subreddit /r/haiku scraped and filtered between October 19th and 10th 2022, combined with a previous dump of that same subreddit packaged by ConvoKit as part of the Subreddit Corpus, which is itself a subset of URL's big dump.\n\nA main motivation for this dataset was to collect an alternative haiku dataset for evaluation, in particular for evaluating Fabian Mueller's Deep Haiku model which was trained on the Haiku datasets of hjhalani30 and bfbarry, which are also available on huggingface hub.",
"## Fields\nThe fields are post id ('id'), the content of the haiku ('processed_title'), upvotes ('ups'), and topic keywords ('keywords'). Topic keywords for each haiku have been extracted with the KeyBERT library and truncated to top-5 keywords.",
"## Usage\n\nThis dataset is intended for evaluation, hence there is only one split which is 'test'.\n\n\n\nThere is code for scraping and processing in 'processing_code', and a subset of the data with more fields such as author Karma, downvotes and posting time at 'processing_code/URL'."
] | [
"TAGS\n#task_categories-text-generation #task_ids-language-modeling #annotations_creators-found #language_creators-found #multilinguality-monolingual #size_categories-10K<n<100K #source_datasets-extended|other #language-English #license-unknown #haiku #poem #poetry #reddit #keybert #generation #region-us \n",
"# Dataset Card for \"Reddit Haiku\"\n\nThis dataset contains haikus from the subreddit /r/haiku scraped and filtered between October 19th and 10th 2022, combined with a previous dump of that same subreddit packaged by ConvoKit as part of the Subreddit Corpus, which is itself a subset of URL's big dump.\n\nA main motivation for this dataset was to collect an alternative haiku dataset for evaluation, in particular for evaluating Fabian Mueller's Deep Haiku model which was trained on the Haiku datasets of hjhalani30 and bfbarry, which are also available on huggingface hub.",
"## Fields\nThe fields are post id ('id'), the content of the haiku ('processed_title'), upvotes ('ups'), and topic keywords ('keywords'). Topic keywords for each haiku have been extracted with the KeyBERT library and truncated to top-5 keywords.",
"## Usage\n\nThis dataset is intended for evaluation, hence there is only one split which is 'test'.\n\n\n\nThere is code for scraping and processing in 'processing_code', and a subset of the data with more fields such as author Karma, downvotes and posting time at 'processing_code/URL'."
] |
4556473b1043404d771aa6a91ba2c0ad5a6a1f27 | https://opus.nlpl.eu/XLEnt-v1.1.php
Uploaded from Opus to HuggingFace AI by Argos Open Tech.
Corpus Name: XLEnt
Package: XLEnt.de-en in Moses format
Website: http://opus.nlpl.eu/XLEnt-v1.1.php
Release: v1.1
Release date: Sun May 23 08:35:55 EEST 2021
This corpus is part of OPUS - the open collection of parallel corpora
OPUS Website: http://opus.nlpl.eu
If you use the dataset or code, please cite (pdf): @inproceedings{elkishky_xlent_2021, author = {El-Kishky, Ahmed and Renduchintala, Adi and Cross, James and Guzmán, Francisco and Koehn, Philipp}, booktitle = {Preprint}, title = {{XLEnt}: Mining Cross-lingual Entities with Lexical-Semantic-Phonetic Word Alignment}, year = {2021}, address = Online, } and, please, acknowledge OPUS (bib, pdf) as well for this service.
This corpus was created by mining CCAligned, CCMatrix, and WikiMatrix parallel sentences. These three sources were themselves extracted from web data from Commoncrawl Snapshots and Wikipedia snapshots. Entity pairs were obtained by performing named entity recognition and typing on English sentences and projecting labels to non-English aligned sentence pairs. No claims of intellectual property are made on the work of preparation of the corpus. XLEnt consists of parallel entity mentions in 120 languages aligned with English. These entity pairs were constructed by performing named entity recognition (NER) and typing on English sentences from mined sentence pairs. These extracted English entity labels and types were projected to the non-English sentences through word alignment. Word alignment was performed by combining three alignment signals ((1) word co-occurence alignment with FastAlign (2) semantic alignment using LASER embeddings, and (3) phonetic alignment via transliteration) into a unified word-alignment model. This lexical/semantic/phonetic alignment approach yielded more than 160 million aligned entity pairs in 120 languages paired with English. Recognizing that each English is often aligned to mulitple entities in different target languages, we can join on English entities to obtain aligned entity pairs that directly pair two non-English entities (e.g., Arabic-French) The original distribution is available from http://data.statmt.org/xlent/ The difference to version 1 is that pivoting now only uses the link with best score in case of alternative alignments for a pivot entity.
| argosopentech/xlent-de_en | [
"region:us"
] | 2022-11-17T23:15:36+00:00 | {} | 2022-11-17T23:22:09+00:00 | [] | [] | TAGS
#region-us
| URL
Uploaded from Opus to HuggingFace AI by Argos Open Tech.
Corpus Name: XLEnt
Package: URL-en in Moses format
Website: URL
Release: v1.1
Release date: Sun May 23 08:35:55 EEST 2021
This corpus is part of OPUS - the open collection of parallel corpora
OPUS Website: URL
If you use the dataset or code, please cite (pdf): @inproceedings{elkishky_xlent_2021, author = {El-Kishky, Ahmed and Renduchintala, Adi and Cross, James and Guzmán, Francisco and Koehn, Philipp}, booktitle = {Preprint}, title = {{XLEnt}: Mining Cross-lingual Entities with Lexical-Semantic-Phonetic Word Alignment}, year = {2021}, address = Online, } and, please, acknowledge OPUS (bib, pdf) as well for this service.
This corpus was created by mining CCAligned, CCMatrix, and WikiMatrix parallel sentences. These three sources were themselves extracted from web data from Commoncrawl Snapshots and Wikipedia snapshots. Entity pairs were obtained by performing named entity recognition and typing on English sentences and projecting labels to non-English aligned sentence pairs. No claims of intellectual property are made on the work of preparation of the corpus. XLEnt consists of parallel entity mentions in 120 languages aligned with English. These entity pairs were constructed by performing named entity recognition (NER) and typing on English sentences from mined sentence pairs. These extracted English entity labels and types were projected to the non-English sentences through word alignment. Word alignment was performed by combining three alignment signals ((1) word co-occurence alignment with FastAlign (2) semantic alignment using LASER embeddings, and (3) phonetic alignment via transliteration) into a unified word-alignment model. This lexical/semantic/phonetic alignment approach yielded more than 160 million aligned entity pairs in 120 languages paired with English. Recognizing that each English is often aligned to mulitple entities in different target languages, we can join on English entities to obtain aligned entity pairs that directly pair two non-English entities (e.g., Arabic-French) The original distribution is available from URL The difference to version 1 is that pivoting now only uses the link with best score in case of alternative alignments for a pivot entity.
| [] | [
"TAGS\n#region-us \n"
] |
cb8e75614830035a37f3a2a11de5e625eaf0bc31 |
# ProofNet
## Dataset Description
- **Repository:** [zhangir-azerbayev/ProofNet](https://github.com/zhangir-azerbayev/ProofNet)
- **Paper:** [ProofNet](https://mathai2022.github.io/papers/20.pdf)
- **Point of Contact:** [Zhangir Azerbayev](https://zhangir-azerbayev.github.io/)
### Dataset Summary
ProofNet is a benchmark for autoformalization and formal proving of undergraduate-level mathematics. The ProofNet benchmarks consists of 371 examples, each consisting of a formal theorem statement in Lean 3, a natural language theorem statement, and a natural language proof. The problems are primarily drawn from popular undergraduate pure mathematics textbooks and cover topics such as real and complex analysis, linear algebra, abstract algebra, and topology. We intend for ProofNet to be a challenging benchmark that will drive progress in autoformalization and automatic theorem proving.
**Citation**:
```bibtex
@misc{azerbayev2023proofnet,
title={ProofNet: Autoformalizing and Formally Proving Undergraduate-Level Mathematics},
author={Zhangir Azerbayev and Bartosz Piotrowski and Hailey Schoelkopf and Edward W. Ayers and Dragomir Radev and Jeremy Avigad},
year={2023},
eprint={2302.12433},
archivePrefix={arXiv},
primaryClass={cs.CL}
}
```
### Leaderboard
**Statement Autoformalization**
| Model | Typecheck Rate | Accuracy |
| ---------------------------------- | -------------- | -------- |
| Davinci-code-002 (prompt retrieval)| 45.2 | 16.1 |
| Davinci-code-002 (in-context learning) | 23.7 | 13.4 |
| proofGPT-1.3B | 10.7 | 3.2 |
**Statement Informalization**
| Model | Accuracy |
| ---------------------------------- | -------- |
| Code-davinci-002 (in-context learning)| 62.3 |
| proofGPT-6.7B (in-context learning) | 6.5 |
| proofGPT-1.3B (in-context learning) | 4.3 |
### Data Fields
- `id`: Unique string identifier for the problem.
- `nl_statement`: Natural language theorem statement.
- `nl_proof`: Natural language proof, in LaTeX. Depends on `amsthm, amsmath, amssymb` packages.
- `formal_statement`: Formal theorem statement in Lean 3.
- `src_header`: File header including imports, namespaces, and locales required for the formal statement. Note that local import of [common.lean](https://github.com/zhangir-azerbayev/ProofNet/blob/main/benchmark/benchmark_to_publish/formal/common.lean), which has to be manually downloaded and place in the same directory as your `.lean` file containing the formal statement.
### Authors
Zhangir Azerbayev, Bartosz Piotrowski, Jeremy Avigad | hoskinson-center/proofnet | [
"license:mit",
"arxiv:2302.12433",
"region:us"
] | 2022-11-17T23:53:41+00:00 | {"license": "mit"} | 2023-03-17T21:25:37+00:00 | [
"2302.12433"
] | [] | TAGS
#license-mit #arxiv-2302.12433 #region-us
| ProofNet
========
Dataset Description
-------------------
* Repository: zhangir-azerbayev/ProofNet
* Paper: ProofNet
* Point of Contact: Zhangir Azerbayev
### Dataset Summary
ProofNet is a benchmark for autoformalization and formal proving of undergraduate-level mathematics. The ProofNet benchmarks consists of 371 examples, each consisting of a formal theorem statement in Lean 3, a natural language theorem statement, and a natural language proof. The problems are primarily drawn from popular undergraduate pure mathematics textbooks and cover topics such as real and complex analysis, linear algebra, abstract algebra, and topology. We intend for ProofNet to be a challenging benchmark that will drive progress in autoformalization and automatic theorem proving.
Citation:
### Leaderboard
Statement Autoformalization
Model: Davinci-code-002 (prompt retrieval), Typecheck Rate: 45.2, Accuracy: 16.1
Model: Davinci-code-002 (in-context learning), Typecheck Rate: 23.7, Accuracy: 13.4
Model: proofGPT-1.3B, Typecheck Rate: 10.7, Accuracy: 3.2
Statement Informalization
### Data Fields
* 'id': Unique string identifier for the problem.
* 'nl\_statement': Natural language theorem statement.
* 'nl\_proof': Natural language proof, in LaTeX. Depends on 'amsthm, amsmath, amssymb' packages.
* 'formal\_statement': Formal theorem statement in Lean 3.
* 'src\_header': File header including imports, namespaces, and locales required for the formal statement. Note that local import of URL, which has to be manually downloaded and place in the same directory as your '.lean' file containing the formal statement.
### Authors
Zhangir Azerbayev, Bartosz Piotrowski, Jeremy Avigad
| [
"### Dataset Summary\n\n\nProofNet is a benchmark for autoformalization and formal proving of undergraduate-level mathematics. The ProofNet benchmarks consists of 371 examples, each consisting of a formal theorem statement in Lean 3, a natural language theorem statement, and a natural language proof. The problems are primarily drawn from popular undergraduate pure mathematics textbooks and cover topics such as real and complex analysis, linear algebra, abstract algebra, and topology. We intend for ProofNet to be a challenging benchmark that will drive progress in autoformalization and automatic theorem proving.\n\n\nCitation:",
"### Leaderboard\n\n\nStatement Autoformalization\n\n\nModel: Davinci-code-002 (prompt retrieval), Typecheck Rate: 45.2, Accuracy: 16.1\nModel: Davinci-code-002 (in-context learning), Typecheck Rate: 23.7, Accuracy: 13.4\nModel: proofGPT-1.3B, Typecheck Rate: 10.7, Accuracy: 3.2\n\n\nStatement Informalization",
"### Data Fields\n\n\n* 'id': Unique string identifier for the problem.\n* 'nl\\_statement': Natural language theorem statement.\n* 'nl\\_proof': Natural language proof, in LaTeX. Depends on 'amsthm, amsmath, amssymb' packages.\n* 'formal\\_statement': Formal theorem statement in Lean 3.\n* 'src\\_header': File header including imports, namespaces, and locales required for the formal statement. Note that local import of URL, which has to be manually downloaded and place in the same directory as your '.lean' file containing the formal statement.",
"### Authors\n\n\nZhangir Azerbayev, Bartosz Piotrowski, Jeremy Avigad"
] | [
"TAGS\n#license-mit #arxiv-2302.12433 #region-us \n",
"### Dataset Summary\n\n\nProofNet is a benchmark for autoformalization and formal proving of undergraduate-level mathematics. The ProofNet benchmarks consists of 371 examples, each consisting of a formal theorem statement in Lean 3, a natural language theorem statement, and a natural language proof. The problems are primarily drawn from popular undergraduate pure mathematics textbooks and cover topics such as real and complex analysis, linear algebra, abstract algebra, and topology. We intend for ProofNet to be a challenging benchmark that will drive progress in autoformalization and automatic theorem proving.\n\n\nCitation:",
"### Leaderboard\n\n\nStatement Autoformalization\n\n\nModel: Davinci-code-002 (prompt retrieval), Typecheck Rate: 45.2, Accuracy: 16.1\nModel: Davinci-code-002 (in-context learning), Typecheck Rate: 23.7, Accuracy: 13.4\nModel: proofGPT-1.3B, Typecheck Rate: 10.7, Accuracy: 3.2\n\n\nStatement Informalization",
"### Data Fields\n\n\n* 'id': Unique string identifier for the problem.\n* 'nl\\_statement': Natural language theorem statement.\n* 'nl\\_proof': Natural language proof, in LaTeX. Depends on 'amsthm, amsmath, amssymb' packages.\n* 'formal\\_statement': Formal theorem statement in Lean 3.\n* 'src\\_header': File header including imports, namespaces, and locales required for the formal statement. Note that local import of URL, which has to be manually downloaded and place in the same directory as your '.lean' file containing the formal statement.",
"### Authors\n\n\nZhangir Azerbayev, Bartosz Piotrowski, Jeremy Avigad"
] |
5e382a8497d4dd28842cc0bfa85387f965ac9d8d | # Dataset Card for "top_v2"
[More Information needed](https://github.com/huggingface/datasets/blob/main/CONTRIBUTING.md#how-to-contribute-to-the-dataset-cards) | WillHeld/top_v2 | [
"region:us"
] | 2022-11-18T00:41:44+00:00 | {"dataset_info": {"features": [{"name": "domain", "dtype": "string"}, {"name": "utterance", "dtype": "string"}, {"name": "semantic_parse", "dtype": "string"}], "splits": [{"name": "eval", "num_bytes": 2650777, "num_examples": 17160}, {"name": "test", "num_bytes": 5947186, "num_examples": 38785}, {"name": "train", "num_bytes": 19433606, "num_examples": 124597}], "download_size": 9672445, "dataset_size": 28031569}} | 2022-12-10T17:52:27+00:00 | [] | [] | TAGS
#region-us
| # Dataset Card for "top_v2"
More Information needed | [
"# Dataset Card for \"top_v2\"\n\nMore Information needed"
] | [
"TAGS\n#region-us \n",
"# Dataset Card for \"top_v2\"\n\nMore Information needed"
] |
677226ce59cda82b34387e1c4a0991966b00914d | # Dataset Card for "cstop"
[More Information needed](https://github.com/huggingface/datasets/blob/main/CONTRIBUTING.md#how-to-contribute-to-the-dataset-cards) | WillHeld/cstop | [
"region:us"
] | 2022-11-18T00:46:55+00:00 | {"dataset_info": {"features": [{"name": "intent", "dtype": "string"}, {"name": " slots", "dtype": "string"}, {"name": " utterance", "dtype": "string"}, {"name": " semantic_parse", "dtype": "string"}, {"name": "slots", "dtype": "string"}, {"name": "utterance", "dtype": "string"}, {"name": "semantic_parse", "dtype": "string"}], "splits": [{"name": "eval", "num_bytes": 182981, "num_examples": 559}, {"name": "test", "num_bytes": 377805, "num_examples": 1167}, {"name": "train", "num_bytes": 1325564, "num_examples": 4077}], "download_size": 618573, "dataset_size": 1886350}} | 2022-12-10T17:53:33+00:00 | [] | [] | TAGS
#region-us
| # Dataset Card for "cstop"
More Information needed | [
"# Dataset Card for \"cstop\"\n\nMore Information needed"
] | [
"TAGS\n#region-us \n",
"# Dataset Card for \"cstop\"\n\nMore Information needed"
] |
94fc7c46882d3a75878bbce17a1bbf0449579826 | # Dataset Card for "parsed_sst2"
[More Information needed](https://github.com/huggingface/datasets/blob/main/CONTRIBUTING.md#how-to-contribute-to-the-dataset-cards) | liuyanchen1015/parsed_sst2 | [
"region:us"
] | 2022-11-18T02:46:25+00:00 | {"dataset_info": {"features": [{"name": "sentence", "dtype": "string"}, {"name": "label", "dtype": {"class_label": {"names": {"0": "negative", "1": "positive"}}}}, {"name": "idx", "dtype": "int32"}, {"name": "parse_tree", "dtype": "string"}, {"name": "pure_parse_tree", "dtype": "string"}], "splits": [{"name": "train", "num_bytes": 22647332, "num_examples": 67349}, {"name": "validation", "num_bytes": 560160, "num_examples": 872}, {"name": "test", "num_bytes": 1155733, "num_examples": 1821}], "download_size": 10913172, "dataset_size": 24363225}} | 2022-11-18T05:18:40+00:00 | [] | [] | TAGS
#region-us
| # Dataset Card for "parsed_sst2"
More Information needed | [
"# Dataset Card for \"parsed_sst2\"\n\nMore Information needed"
] | [
"TAGS\n#region-us \n",
"# Dataset Card for \"parsed_sst2\"\n\nMore Information needed"
] |
a754585cf5449543a22daf2fa371957ff1d1353d | # Dataset Card for "Yannic-Kilcher"
[More Information needed](https://github.com/huggingface/datasets/blob/main/CONTRIBUTING.md#how-to-contribute-to-the-dataset-cards) | juancopi81/Yannic-Kilcher | [
"task_categories:automatic-speech-recognition",
"whisper",
"whispering",
"region:us"
] | 2022-11-18T03:10:02+00:00 | {"task_categories": ["automatic-speech-recognition"], "dataset_info": {"features": [{"name": "CHANNEL_NAME", "dtype": "string"}, {"name": "URL", "dtype": "string"}, {"name": "TITLE", "dtype": "string"}, {"name": "DESCRIPTION", "dtype": "string"}, {"name": "TRANSCRIPTION", "dtype": "string"}, {"name": "SEGMENTS", "dtype": "string"}], "splits": [{"name": "train", "num_bytes": 28243998, "num_examples": 375}], "download_size": 12872792, "dataset_size": 28243998}, "tags": ["whisper", "whispering"]} | 2022-11-18T12:29:51+00:00 | [] | [] | TAGS
#task_categories-automatic-speech-recognition #whisper #whispering #region-us
| # Dataset Card for "Yannic-Kilcher"
More Information needed | [
"# Dataset Card for \"Yannic-Kilcher\"\n\nMore Information needed"
] | [
"TAGS\n#task_categories-automatic-speech-recognition #whisper #whispering #region-us \n",
"# Dataset Card for \"Yannic-Kilcher\"\n\nMore Information needed"
] |
30a3566ac0cc8e45248a20919b6fdbaab365b540 | # Dataset Card for "urgent-triage-samples"
[More Information needed](https://github.com/huggingface/datasets/blob/main/CONTRIBUTING.md#how-to-contribute-to-the-dataset-cards) | Lokibabu/urgent-triage-samples | [
"region:us"
] | 2022-11-18T05:42:00+00:00 | {"dataset_info": {"features": [{"name": "img", "dtype": "string"}, {"name": "label", "dtype": "int64"}, {"name": "name", "dtype": "string"}], "splits": [{"name": "test", "num_bytes": 1021, "num_examples": 22}, {"name": "train", "num_bytes": 1021, "num_examples": 22}], "download_size": 2988, "dataset_size": 2042}} | 2022-11-18T06:05:09+00:00 | [] | [] | TAGS
#region-us
| # Dataset Card for "urgent-triage-samples"
More Information needed | [
"# Dataset Card for \"urgent-triage-samples\"\n\nMore Information needed"
] | [
"TAGS\n#region-us \n",
"# Dataset Card for \"urgent-triage-samples\"\n\nMore Information needed"
] |
9eb409dcb51be812b30a8c1cfe8b0ecb8e961305 | # Dataset Card for "Yannic-Kilcher"
[More Information needed](https://github.com/huggingface/datasets/blob/main/CONTRIBUTING.md#how-to-contribute-to-the-dataset-cards) | osanseviero/test_osan | [
"whisper",
"whispering",
"region:us"
] | 2022-11-18T06:39:19+00:00 | {"task_ids": ["automatic-speech-recognition"], "dataset_info": {"features": [{"name": "CHANNEL_NAME", "dtype": "string"}, {"name": "URL", "dtype": "string"}, {"name": "TITLE", "dtype": "string"}, {"name": "DESCRIPTION", "dtype": "string"}, {"name": "TRANSCRIPTION", "dtype": "string"}, {"name": "SEGMENTS", "dtype": "string"}], "splits": [{"name": "train", "num_bytes": 28243998, "num_examples": 375}], "download_size": 12872792, "dataset_size": 28243998}, "tags": ["whisper", "whispering"]} | 2022-11-18T06:47:04+00:00 | [] | [] | TAGS
#whisper #whispering #region-us
| # Dataset Card for "Yannic-Kilcher"
More Information needed | [
"# Dataset Card for \"Yannic-Kilcher\"\n\nMore Information needed"
] | [
"TAGS\n#whisper #whispering #region-us \n",
"# Dataset Card for \"Yannic-Kilcher\"\n\nMore Information needed"
] |
6ebad500e4c26070bf0250887f2ea1add40535e9 | # Dataset Card for "task-pages"
[More Information needed](https://github.com/huggingface/datasets/blob/main/CONTRIBUTING.md#how-to-contribute-to-the-dataset-cards) | osanseviero/task-pages | [
"region:us"
] | 2022-11-18T06:58:02+00:00 | {"dataset_info": {"features": [{"name": "CHANNEL_NAME", "dtype": "string"}, {"name": "URL", "dtype": "string"}, {"name": "TITLE", "dtype": "string"}, {"name": "DESCRIPTION", "dtype": "string"}, {"name": "TRANSCRIPTION", "dtype": "string"}, {"name": "SEGMENTS", "dtype": "string"}], "splits": [{"name": "train", "num_bytes": 27732, "num_examples": 2}], "download_size": 29958, "dataset_size": 27732}} | 2022-11-18T07:01:05+00:00 | [] | [] | TAGS
#region-us
| # Dataset Card for "task-pages"
More Information needed | [
"# Dataset Card for \"task-pages\"\n\nMore Information needed"
] | [
"TAGS\n#region-us \n",
"# Dataset Card for \"task-pages\"\n\nMore Information needed"
] |
1b7fe9e45386ba995a6e91128bcfd3b278fb7c42 | # Dataset Card for "azure"
[More Information needed](https://github.com/huggingface/datasets/blob/main/CONTRIBUTING.md#how-to-contribute-to-the-dataset-cards) | osanseviero/azure | [
"task_categories:automatic-speech-recognition",
"whisper",
"whispering",
"region:us"
] | 2022-11-18T07:06:59+00:00 | {"task_categories": ["automatic-speech-recognition"], "dataset_info": {"features": [{"name": "CHANNEL_NAME", "dtype": "string"}, {"name": "URL", "dtype": "string"}, {"name": "TITLE", "dtype": "string"}, {"name": "DESCRIPTION", "dtype": "string"}, {"name": "TRANSCRIPTION", "dtype": "string"}, {"name": "SEGMENTS", "dtype": "string"}], "splits": [{"name": "train", "num_bytes": 27732, "num_examples": 2}], "download_size": 29958, "dataset_size": 27732}, "tags": ["whisper", "whispering"]} | 2022-11-18T07:07:02+00:00 | [] | [] | TAGS
#task_categories-automatic-speech-recognition #whisper #whispering #region-us
| # Dataset Card for "azure"
More Information needed | [
"# Dataset Card for \"azure\"\n\nMore Information needed"
] | [
"TAGS\n#task_categories-automatic-speech-recognition #whisper #whispering #region-us \n",
"# Dataset Card for \"azure\"\n\nMore Information needed"
] |
744e1fd35ab07eb9d83860154b4298d453050009 | # Dataset Card for AutoTrain Evaluator
This repository contains model predictions generated by [AutoTrain](https://huggingface.co/autotrain) for the following task and dataset:
* Task: Binary Text Classification
* Model: autoevaluate/binary-classification
* Dataset: glue
* Config: sst2
* Split: validation
To run new evaluation jobs, visit Hugging Face's [automatic model evaluator](https://huggingface.co/spaces/autoevaluate/model-evaluator).
## Contributions
Thanks to [@lewtun](https://huggingface.co/lewtun) for evaluating this model. | autoevaluate/autoeval-staging-eval-project-2e778dac-2622-46c9-930e-6f9e705a27bf-2018 | [
"autotrain",
"evaluation",
"region:us"
] | 2022-11-18T10:00:57+00:00 | {"type": "predictions", "tags": ["autotrain", "evaluation"], "datasets": ["glue"], "eval_info": {"task": "binary_classification", "model": "autoevaluate/binary-classification", "metrics": ["matthews_correlation"], "dataset_name": "glue", "dataset_config": "sst2", "dataset_split": "validation", "col_mapping": {"text": "sentence", "target": "label"}}} | 2022-11-18T10:01:40+00:00 | [] | [] | TAGS
#autotrain #evaluation #region-us
| # Dataset Card for AutoTrain Evaluator
This repository contains model predictions generated by AutoTrain for the following task and dataset:
* Task: Binary Text Classification
* Model: autoevaluate/binary-classification
* Dataset: glue
* Config: sst2
* Split: validation
To run new evaluation jobs, visit Hugging Face's automatic model evaluator.
## Contributions
Thanks to @lewtun for evaluating this model. | [
"# Dataset Card for AutoTrain Evaluator\n\nThis repository contains model predictions generated by AutoTrain for the following task and dataset:\n\n* Task: Binary Text Classification\n* Model: autoevaluate/binary-classification\n* Dataset: glue\n* Config: sst2\n* Split: validation\n\nTo run new evaluation jobs, visit Hugging Face's automatic model evaluator.",
"## Contributions\n\nThanks to @lewtun for evaluating this model."
] | [
"TAGS\n#autotrain #evaluation #region-us \n",
"# Dataset Card for AutoTrain Evaluator\n\nThis repository contains model predictions generated by AutoTrain for the following task and dataset:\n\n* Task: Binary Text Classification\n* Model: autoevaluate/binary-classification\n* Dataset: glue\n* Config: sst2\n* Split: validation\n\nTo run new evaluation jobs, visit Hugging Face's automatic model evaluator.",
"## Contributions\n\nThanks to @lewtun for evaluating this model."
] |
f1323cd10266ca8d8e135a7f567210d03a747139 | # Dataset Card for AutoTrain Evaluator
This repository contains model predictions generated by [AutoTrain](https://huggingface.co/autotrain) for the following task and dataset:
* Task: Binary Text Classification
* Model: autoevaluate/binary-classification
* Dataset: glue
* Config: sst2
* Split: validation
To run new evaluation jobs, visit Hugging Face's [automatic model evaluator](https://huggingface.co/spaces/autoevaluate/model-evaluator).
## Contributions
Thanks to [@lewtun](https://huggingface.co/lewtun) for evaluating this model. | autoevaluate/autoeval-staging-eval-project-61fd61be-9af8-4428-ac3c-2fe701ee60d1-2119 | [
"autotrain",
"evaluation",
"region:us"
] | 2022-11-18T10:10:19+00:00 | {"type": "predictions", "tags": ["autotrain", "evaluation"], "datasets": ["glue"], "eval_info": {"task": "binary_classification", "model": "autoevaluate/binary-classification", "metrics": ["matthews_correlation"], "dataset_name": "glue", "dataset_config": "sst2", "dataset_split": "validation", "col_mapping": {"text": "sentence", "target": "label"}}} | 2022-11-18T10:10:55+00:00 | [] | [] | TAGS
#autotrain #evaluation #region-us
| # Dataset Card for AutoTrain Evaluator
This repository contains model predictions generated by AutoTrain for the following task and dataset:
* Task: Binary Text Classification
* Model: autoevaluate/binary-classification
* Dataset: glue
* Config: sst2
* Split: validation
To run new evaluation jobs, visit Hugging Face's automatic model evaluator.
## Contributions
Thanks to @lewtun for evaluating this model. | [
"# Dataset Card for AutoTrain Evaluator\n\nThis repository contains model predictions generated by AutoTrain for the following task and dataset:\n\n* Task: Binary Text Classification\n* Model: autoevaluate/binary-classification\n* Dataset: glue\n* Config: sst2\n* Split: validation\n\nTo run new evaluation jobs, visit Hugging Face's automatic model evaluator.",
"## Contributions\n\nThanks to @lewtun for evaluating this model."
] | [
"TAGS\n#autotrain #evaluation #region-us \n",
"# Dataset Card for AutoTrain Evaluator\n\nThis repository contains model predictions generated by AutoTrain for the following task and dataset:\n\n* Task: Binary Text Classification\n* Model: autoevaluate/binary-classification\n* Dataset: glue\n* Config: sst2\n* Split: validation\n\nTo run new evaluation jobs, visit Hugging Face's automatic model evaluator.",
"## Contributions\n\nThanks to @lewtun for evaluating this model."
] |
924ae6077edddf60f3ad2f2cbc54df3825a70930 |
# WikiCAT_es: Spanish Text Classification dataset
## Dataset Description
- **Paper:**
- **Point of Contact:** [email protected]
**Repository**
### Dataset Summary
WikiCAT_ca is a Spanish corpus for thematic Text Classification tasks. It is created automatically from Wikipedia and Wikidata sources, and contains 8401 articles from the Viquipedia classified under 12 different categories.
This dataset was developed by BSC TeMU as part of the PlanTL project, and intended as an evaluation of LT capabilities to generate useful synthetic corpus.
### Supported Tasks and Leaderboards
Text classification, Language Model
### Languages
ES- Spanish
## Dataset Structure
### Data Instances
Two json files, one for each split.
### Data Fields
We used a simple model with the article text and associated labels, without further metadata.
#### Example:
<pre>
{'sentence': 'La economía de Reunión se ha basado tradicionalmente en la agricultura. La caña de azúcar ha sido el cultivo principal durante más de un siglo, y en algunos años representa el 85% de las exportaciones. El gobierno ha estado impulsando el desarrollo de una industria turística para aliviar el alto desempleo, que representa más del 40% de la fuerza laboral.(...) El PIB total de la isla fue de 18.800 millones de dólares EE.UU. en 2007., 'label': 'Economía'}
</pre>
#### Labels
'Religión', 'Entretenimiento', 'Música', 'Ciencia_y_Tecnología', 'Política', 'Economía', 'Matemáticas', 'Humanidades', 'Deporte', 'Derecho', 'Historia', 'Filosofía'
### Data Splits
* hfeval_esv5.json: 1681 label-document pairs
* hftrain_esv5.json: 6716 label-document pairs
## Dataset Creation
### Methodology
La páginas de "Categoría" representan los temas.
para cada tema, extraemos las páginas asociadas a ese primer nivel de la jerarquía, y utilizamos el resúmen ("summary") como texto representativo.
### Curation Rationale
### Source Data
#### Initial Data Collection and Normalization
The source data are thematic categories in the different Wikipedias
#### Who are the source language producers?
### Annotations
#### Annotation process
Automatic annotation
#### Who are the annotators?
[N/A]
### Personal and Sensitive Information
No personal or sensitive information included.
## Considerations for Using the Data
### Social Impact of Dataset
We hope this corpus contributes to the development of language models in Spanish.
### Discussion of Biases
We are aware that this data might contain biases. We have not applied any steps to reduce their impact.
### Other Known Limitations
[N/A]
## Additional Information
### Dataset Curators
Text Mining Unit (TeMU) at the Barcelona Supercomputing Center ([email protected]).
For further information, send an email to ([email protected]).
This work was funded by the [Spanish State Secretariat for Digitalization and Artificial Intelligence (SEDIA)](https://avancedigital.mineco.gob.es/en-us/Paginas/index.aspx) within the framework of the [Plan-TL](https://plantl.mineco.gob.es/Paginas/index.aspx).
### Licensing Information
This work is licensed under [CC Attribution 4.0 International](https://creativecommons.org/licenses/by/4.0/) License.
Copyright by the Spanish State Secretariat for Digitalization and Artificial Intelligence (SEDIA) (2022)
### Contributions
[N/A]
| PlanTL-GOB-ES/WikiCAT_esv2 | [
"task_categories:text-classification",
"task_ids:multi-class-classification",
"annotations_creators:automatically-generated",
"language_creators:found",
"multilinguality:monolingual",
"size_categories:unknown",
"language:es",
"license:cc-by-sa-3.0",
"region:us"
] | 2022-11-18T10:18:53+00:00 | {"annotations_creators": ["automatically-generated"], "language_creators": ["found"], "language": ["es"], "license": ["cc-by-sa-3.0"], "multilinguality": ["monolingual"], "size_categories": ["unknown"], "source_datasets": [], "task_categories": ["text-classification"], "task_ids": ["multi-class-classification"], "pretty_name": "wikicat_esv2"} | 2023-07-27T08:13:16+00:00 | [] | [
"es"
] | TAGS
#task_categories-text-classification #task_ids-multi-class-classification #annotations_creators-automatically-generated #language_creators-found #multilinguality-monolingual #size_categories-unknown #language-Spanish #license-cc-by-sa-3.0 #region-us
|
# WikiCAT_es: Spanish Text Classification dataset
## Dataset Description
- Paper:
- Point of Contact: carlos.rodriguez1@URL
Repository
### Dataset Summary
WikiCAT_ca is a Spanish corpus for thematic Text Classification tasks. It is created automatically from Wikipedia and Wikidata sources, and contains 8401 articles from the Viquipedia classified under 12 different categories.
This dataset was developed by BSC TeMU as part of the PlanTL project, and intended as an evaluation of LT capabilities to generate useful synthetic corpus.
### Supported Tasks and Leaderboards
Text classification, Language Model
### Languages
ES- Spanish
## Dataset Structure
### Data Instances
Two json files, one for each split.
### Data Fields
We used a simple model with the article text and associated labels, without further metadata.
#### Example:
<pre>
{'sentence': 'La economía de Reunión se ha basado tradicionalmente en la agricultura. La caña de azúcar ha sido el cultivo principal durante más de un siglo, y en algunos años representa el 85% de las exportaciones. El gobierno ha estado impulsando el desarrollo de una industria turística para aliviar el alto desempleo, que representa más del 40% de la fuerza laboral.(...) El PIB total de la isla fue de 18.800 millones de dólares EE.UU. en 2007., 'label': 'Economía'}
</pre>
#### Labels
'Religión', 'Entretenimiento', 'Música', 'Ciencia_y_Tecnología', 'Política', 'Economía', 'Matemáticas', 'Humanidades', 'Deporte', 'Derecho', 'Historia', 'Filosofía'
### Data Splits
* hfeval_esv5.json: 1681 label-document pairs
* hftrain_esv5.json: 6716 label-document pairs
## Dataset Creation
### Methodology
La páginas de "Categoría" representan los temas.
para cada tema, extraemos las páginas asociadas a ese primer nivel de la jerarquía, y utilizamos el resúmen ("summary") como texto representativo.
### Curation Rationale
### Source Data
#### Initial Data Collection and Normalization
The source data are thematic categories in the different Wikipedias
#### Who are the source language producers?
### Annotations
#### Annotation process
Automatic annotation
#### Who are the annotators?
[N/A]
### Personal and Sensitive Information
No personal or sensitive information included.
## Considerations for Using the Data
### Social Impact of Dataset
We hope this corpus contributes to the development of language models in Spanish.
### Discussion of Biases
We are aware that this data might contain biases. We have not applied any steps to reduce their impact.
### Other Known Limitations
[N/A]
## Additional Information
### Dataset Curators
Text Mining Unit (TeMU) at the Barcelona Supercomputing Center (bsc-temu@URL).
For further information, send an email to (plantl-gob-es@URL).
This work was funded by the Spanish State Secretariat for Digitalization and Artificial Intelligence (SEDIA) within the framework of the Plan-TL.
### Licensing Information
This work is licensed under CC Attribution 4.0 International License.
Copyright by the Spanish State Secretariat for Digitalization and Artificial Intelligence (SEDIA) (2022)
### Contributions
[N/A]
| [
"# WikiCAT_es: Spanish Text Classification dataset",
"## Dataset Description\n\n- Paper: \n\n- Point of Contact: carlos.rodriguez1@URL\n\n\nRepository",
"### Dataset Summary\n\nWikiCAT_ca is a Spanish corpus for thematic Text Classification tasks. It is created automatically from Wikipedia and Wikidata sources, and contains 8401 articles from the Viquipedia classified under 12 different categories.\n\nThis dataset was developed by BSC TeMU as part of the PlanTL project, and intended as an evaluation of LT capabilities to generate useful synthetic corpus.",
"### Supported Tasks and Leaderboards\n\nText classification, Language Model",
"### Languages\n\nES- Spanish",
"## Dataset Structure",
"### Data Instances\n\nTwo json files, one for each split.",
"### Data Fields\n\nWe used a simple model with the article text and associated labels, without further metadata.",
"#### Example:\n\n<pre>\n{'sentence': 'La economía de Reunión se ha basado tradicionalmente en la agricultura. La caña de azúcar ha sido el cultivo principal durante más de un siglo, y en algunos años representa el 85% de las exportaciones. El gobierno ha estado impulsando el desarrollo de una industria turística para aliviar el alto desempleo, que representa más del 40% de la fuerza laboral.(...) El PIB total de la isla fue de 18.800 millones de dólares EE.UU. en 2007., 'label': 'Economía'}\n\n\n</pre>",
"#### Labels\n\n'Religión', 'Entretenimiento', 'Música', 'Ciencia_y_Tecnología', 'Política', 'Economía', 'Matemáticas', 'Humanidades', 'Deporte', 'Derecho', 'Historia', 'Filosofía'",
"### Data Splits\n\n* hfeval_esv5.json: 1681 label-document pairs\n* hftrain_esv5.json: 6716 label-document pairs",
"## Dataset Creation",
"### Methodology\n\nLa páginas de \"Categoría\" representan los temas.\npara cada tema, extraemos las páginas asociadas a ese primer nivel de la jerarquía, y utilizamos el resúmen (\"summary\") como texto representativo.",
"### Curation Rationale",
"### Source Data",
"#### Initial Data Collection and Normalization\n\nThe source data are thematic categories in the different Wikipedias",
"#### Who are the source language producers?",
"### Annotations",
"#### Annotation process\nAutomatic annotation",
"#### Who are the annotators?\n\n[N/A]",
"### Personal and Sensitive Information\n\nNo personal or sensitive information included.",
"## Considerations for Using the Data",
"### Social Impact of Dataset\n\nWe hope this corpus contributes to the development of language models in Spanish.",
"### Discussion of Biases\n\nWe are aware that this data might contain biases. We have not applied any steps to reduce their impact.",
"### Other Known Limitations\n\n[N/A]",
"## Additional Information",
"### Dataset Curators\n\nText Mining Unit (TeMU) at the Barcelona Supercomputing Center (bsc-temu@URL). \n\nFor further information, send an email to (plantl-gob-es@URL).\n\nThis work was funded by the Spanish State Secretariat for Digitalization and Artificial Intelligence (SEDIA) within the framework of the Plan-TL.",
"### Licensing Information\n\nThis work is licensed under CC Attribution 4.0 International License.\n\nCopyright by the Spanish State Secretariat for Digitalization and Artificial Intelligence (SEDIA) (2022)",
"### Contributions\n\n[N/A]"
] | [
"TAGS\n#task_categories-text-classification #task_ids-multi-class-classification #annotations_creators-automatically-generated #language_creators-found #multilinguality-monolingual #size_categories-unknown #language-Spanish #license-cc-by-sa-3.0 #region-us \n",
"# WikiCAT_es: Spanish Text Classification dataset",
"## Dataset Description\n\n- Paper: \n\n- Point of Contact: carlos.rodriguez1@URL\n\n\nRepository",
"### Dataset Summary\n\nWikiCAT_ca is a Spanish corpus for thematic Text Classification tasks. It is created automatically from Wikipedia and Wikidata sources, and contains 8401 articles from the Viquipedia classified under 12 different categories.\n\nThis dataset was developed by BSC TeMU as part of the PlanTL project, and intended as an evaluation of LT capabilities to generate useful synthetic corpus.",
"### Supported Tasks and Leaderboards\n\nText classification, Language Model",
"### Languages\n\nES- Spanish",
"## Dataset Structure",
"### Data Instances\n\nTwo json files, one for each split.",
"### Data Fields\n\nWe used a simple model with the article text and associated labels, without further metadata.",
"#### Example:\n\n<pre>\n{'sentence': 'La economía de Reunión se ha basado tradicionalmente en la agricultura. La caña de azúcar ha sido el cultivo principal durante más de un siglo, y en algunos años representa el 85% de las exportaciones. El gobierno ha estado impulsando el desarrollo de una industria turística para aliviar el alto desempleo, que representa más del 40% de la fuerza laboral.(...) El PIB total de la isla fue de 18.800 millones de dólares EE.UU. en 2007., 'label': 'Economía'}\n\n\n</pre>",
"#### Labels\n\n'Religión', 'Entretenimiento', 'Música', 'Ciencia_y_Tecnología', 'Política', 'Economía', 'Matemáticas', 'Humanidades', 'Deporte', 'Derecho', 'Historia', 'Filosofía'",
"### Data Splits\n\n* hfeval_esv5.json: 1681 label-document pairs\n* hftrain_esv5.json: 6716 label-document pairs",
"## Dataset Creation",
"### Methodology\n\nLa páginas de \"Categoría\" representan los temas.\npara cada tema, extraemos las páginas asociadas a ese primer nivel de la jerarquía, y utilizamos el resúmen (\"summary\") como texto representativo.",
"### Curation Rationale",
"### Source Data",
"#### Initial Data Collection and Normalization\n\nThe source data are thematic categories in the different Wikipedias",
"#### Who are the source language producers?",
"### Annotations",
"#### Annotation process\nAutomatic annotation",
"#### Who are the annotators?\n\n[N/A]",
"### Personal and Sensitive Information\n\nNo personal or sensitive information included.",
"## Considerations for Using the Data",
"### Social Impact of Dataset\n\nWe hope this corpus contributes to the development of language models in Spanish.",
"### Discussion of Biases\n\nWe are aware that this data might contain biases. We have not applied any steps to reduce their impact.",
"### Other Known Limitations\n\n[N/A]",
"## Additional Information",
"### Dataset Curators\n\nText Mining Unit (TeMU) at the Barcelona Supercomputing Center (bsc-temu@URL). \n\nFor further information, send an email to (plantl-gob-es@URL).\n\nThis work was funded by the Spanish State Secretariat for Digitalization and Artificial Intelligence (SEDIA) within the framework of the Plan-TL.",
"### Licensing Information\n\nThis work is licensed under CC Attribution 4.0 International License.\n\nCopyright by the Spanish State Secretariat for Digitalization and Artificial Intelligence (SEDIA) (2022)",
"### Contributions\n\n[N/A]"
] |
facf3772e67d51b7d27508477f777565c6c720f5 | # Dataset Card for AutoTrain Evaluator
This repository contains model predictions generated by [AutoTrain](https://huggingface.co/autotrain) for the following task and dataset:
* Task: Binary Text Classification
* Model: autoevaluate/binary-classification
* Dataset: glue
* Config: sst2
* Split: validation
To run new evaluation jobs, visit Hugging Face's [automatic model evaluator](https://huggingface.co/spaces/autoevaluate/model-evaluator).
## Contributions
Thanks to [@lewtun](https://huggingface.co/lewtun) for evaluating this model. | autoevaluate/autoeval-staging-eval-project-ec388423-7e76-47a7-a778-e7cfff84a71c-2220 | [
"autotrain",
"evaluation",
"region:us"
] | 2022-11-18T10:28:05+00:00 | {"type": "predictions", "tags": ["autotrain", "evaluation"], "datasets": ["glue"], "eval_info": {"task": "binary_classification", "model": "autoevaluate/binary-classification", "metrics": ["matthews_correlation"], "dataset_name": "glue", "dataset_config": "sst2", "dataset_split": "validation", "col_mapping": {"text": "sentence", "target": "label"}}} | 2022-11-18T10:28:40+00:00 | [] | [] | TAGS
#autotrain #evaluation #region-us
| # Dataset Card for AutoTrain Evaluator
This repository contains model predictions generated by AutoTrain for the following task and dataset:
* Task: Binary Text Classification
* Model: autoevaluate/binary-classification
* Dataset: glue
* Config: sst2
* Split: validation
To run new evaluation jobs, visit Hugging Face's automatic model evaluator.
## Contributions
Thanks to @lewtun for evaluating this model. | [
"# Dataset Card for AutoTrain Evaluator\n\nThis repository contains model predictions generated by AutoTrain for the following task and dataset:\n\n* Task: Binary Text Classification\n* Model: autoevaluate/binary-classification\n* Dataset: glue\n* Config: sst2\n* Split: validation\n\nTo run new evaluation jobs, visit Hugging Face's automatic model evaluator.",
"## Contributions\n\nThanks to @lewtun for evaluating this model."
] | [
"TAGS\n#autotrain #evaluation #region-us \n",
"# Dataset Card for AutoTrain Evaluator\n\nThis repository contains model predictions generated by AutoTrain for the following task and dataset:\n\n* Task: Binary Text Classification\n* Model: autoevaluate/binary-classification\n* Dataset: glue\n* Config: sst2\n* Split: validation\n\nTo run new evaluation jobs, visit Hugging Face's automatic model evaluator.",
"## Contributions\n\nThanks to @lewtun for evaluating this model."
] |
2278fe0ab44d4eaf561999862ac1a67ec0fbf4b7 | # Dataset Card for AutoTrain Evaluator
This repository contains model predictions generated by [AutoTrain](https://huggingface.co/autotrain) for the following task and dataset:
* Task: Binary Text Classification
* Model: autoevaluate/binary-classification
* Dataset: glue
* Config: sst2
* Split: validation
To run new evaluation jobs, visit Hugging Face's [automatic model evaluator](https://huggingface.co/spaces/autoevaluate/model-evaluator).
## Contributions
Thanks to [@lewtun](https://huggingface.co/lewtun) for evaluating this model. | autoevaluate/autoeval-staging-eval-project-fc4d51f7-9dde-4256-8b44-b5a68a081b2b-2321 | [
"autotrain",
"evaluation",
"region:us"
] | 2022-11-18T10:42:05+00:00 | {"type": "predictions", "tags": ["autotrain", "evaluation"], "datasets": ["glue"], "eval_info": {"task": "binary_classification", "model": "autoevaluate/binary-classification", "metrics": ["matthews_correlation"], "dataset_name": "glue", "dataset_config": "sst2", "dataset_split": "validation", "col_mapping": {"text": "sentence", "target": "label"}}} | 2022-11-18T10:42:41+00:00 | [] | [] | TAGS
#autotrain #evaluation #region-us
| # Dataset Card for AutoTrain Evaluator
This repository contains model predictions generated by AutoTrain for the following task and dataset:
* Task: Binary Text Classification
* Model: autoevaluate/binary-classification
* Dataset: glue
* Config: sst2
* Split: validation
To run new evaluation jobs, visit Hugging Face's automatic model evaluator.
## Contributions
Thanks to @lewtun for evaluating this model. | [
"# Dataset Card for AutoTrain Evaluator\n\nThis repository contains model predictions generated by AutoTrain for the following task and dataset:\n\n* Task: Binary Text Classification\n* Model: autoevaluate/binary-classification\n* Dataset: glue\n* Config: sst2\n* Split: validation\n\nTo run new evaluation jobs, visit Hugging Face's automatic model evaluator.",
"## Contributions\n\nThanks to @lewtun for evaluating this model."
] | [
"TAGS\n#autotrain #evaluation #region-us \n",
"# Dataset Card for AutoTrain Evaluator\n\nThis repository contains model predictions generated by AutoTrain for the following task and dataset:\n\n* Task: Binary Text Classification\n* Model: autoevaluate/binary-classification\n* Dataset: glue\n* Config: sst2\n* Split: validation\n\nTo run new evaluation jobs, visit Hugging Face's automatic model evaluator.",
"## Contributions\n\nThanks to @lewtun for evaluating this model."
] |
2f1635ca28e9b62ec23b10311b737f02996d799b | # Dataset Card for AutoTrain Evaluator
This repository contains model predictions generated by [AutoTrain](https://huggingface.co/autotrain) for the following task and dataset:
* Task: Binary Text Classification
* Model: autoevaluate/binary-classification
* Dataset: glue
* Config: sst2
* Split: validation
To run new evaluation jobs, visit Hugging Face's [automatic model evaluator](https://huggingface.co/spaces/autoevaluate/model-evaluator).
## Contributions
Thanks to [@lewtun](https://huggingface.co/lewtun) for evaluating this model. | autoevaluate/autoeval-staging-eval-project-ef91170b-c394-482c-8a00-6b7bc5ea5574-2422 | [
"autotrain",
"evaluation",
"region:us"
] | 2022-11-18T10:59:57+00:00 | {"type": "predictions", "tags": ["autotrain", "evaluation"], "datasets": ["glue"], "eval_info": {"task": "binary_classification", "model": "autoevaluate/binary-classification", "metrics": ["matthews_correlation"], "dataset_name": "glue", "dataset_config": "sst2", "dataset_split": "validation", "col_mapping": {"text": "sentence", "target": "label"}}} | 2022-11-18T11:00:32+00:00 | [] | [] | TAGS
#autotrain #evaluation #region-us
| # Dataset Card for AutoTrain Evaluator
This repository contains model predictions generated by AutoTrain for the following task and dataset:
* Task: Binary Text Classification
* Model: autoevaluate/binary-classification
* Dataset: glue
* Config: sst2
* Split: validation
To run new evaluation jobs, visit Hugging Face's automatic model evaluator.
## Contributions
Thanks to @lewtun for evaluating this model. | [
"# Dataset Card for AutoTrain Evaluator\n\nThis repository contains model predictions generated by AutoTrain for the following task and dataset:\n\n* Task: Binary Text Classification\n* Model: autoevaluate/binary-classification\n* Dataset: glue\n* Config: sst2\n* Split: validation\n\nTo run new evaluation jobs, visit Hugging Face's automatic model evaluator.",
"## Contributions\n\nThanks to @lewtun for evaluating this model."
] | [
"TAGS\n#autotrain #evaluation #region-us \n",
"# Dataset Card for AutoTrain Evaluator\n\nThis repository contains model predictions generated by AutoTrain for the following task and dataset:\n\n* Task: Binary Text Classification\n* Model: autoevaluate/binary-classification\n* Dataset: glue\n* Config: sst2\n* Split: validation\n\nTo run new evaluation jobs, visit Hugging Face's automatic model evaluator.",
"## Contributions\n\nThanks to @lewtun for evaluating this model."
] |
febb64298be8a000cbc22029364c563b0b9c2105 | # Dataset Card for AutoTrain Evaluator
This repository contains model predictions generated by [AutoTrain](https://huggingface.co/autotrain) for the following task and dataset:
* Task: Binary Text Classification
* Model: autoevaluate/binary-classification
* Dataset: glue
* Config: sst2
* Split: validation
To run new evaluation jobs, visit Hugging Face's [automatic model evaluator](https://huggingface.co/spaces/autoevaluate/model-evaluator).
## Contributions
Thanks to [@lewtun](https://huggingface.co/lewtun) for evaluating this model. | autoevaluate/autoeval-staging-eval-project-622e0c30-b54d-415c-87b9-70c107d23cec-2523 | [
"autotrain",
"evaluation",
"region:us"
] | 2022-11-18T11:04:27+00:00 | {"type": "predictions", "tags": ["autotrain", "evaluation"], "datasets": ["glue"], "eval_info": {"task": "binary_classification", "model": "autoevaluate/binary-classification", "metrics": ["matthews_correlation"], "dataset_name": "glue", "dataset_config": "sst2", "dataset_split": "validation", "col_mapping": {"text": "sentence", "target": "label"}}} | 2022-11-18T11:05:03+00:00 | [] | [] | TAGS
#autotrain #evaluation #region-us
| # Dataset Card for AutoTrain Evaluator
This repository contains model predictions generated by AutoTrain for the following task and dataset:
* Task: Binary Text Classification
* Model: autoevaluate/binary-classification
* Dataset: glue
* Config: sst2
* Split: validation
To run new evaluation jobs, visit Hugging Face's automatic model evaluator.
## Contributions
Thanks to @lewtun for evaluating this model. | [
"# Dataset Card for AutoTrain Evaluator\n\nThis repository contains model predictions generated by AutoTrain for the following task and dataset:\n\n* Task: Binary Text Classification\n* Model: autoevaluate/binary-classification\n* Dataset: glue\n* Config: sst2\n* Split: validation\n\nTo run new evaluation jobs, visit Hugging Face's automatic model evaluator.",
"## Contributions\n\nThanks to @lewtun for evaluating this model."
] | [
"TAGS\n#autotrain #evaluation #region-us \n",
"# Dataset Card for AutoTrain Evaluator\n\nThis repository contains model predictions generated by AutoTrain for the following task and dataset:\n\n* Task: Binary Text Classification\n* Model: autoevaluate/binary-classification\n* Dataset: glue\n* Config: sst2\n* Split: validation\n\nTo run new evaluation jobs, visit Hugging Face's automatic model evaluator.",
"## Contributions\n\nThanks to @lewtun for evaluating this model."
] |
b7cdd5ca43df8833b34d5ca2d4088051b6b82926 | # Dataset Card for AutoTrain Evaluator
This repository contains model predictions generated by [AutoTrain](https://huggingface.co/autotrain) for the following task and dataset:
* Task: Binary Text Classification
* Model: autoevaluate/binary-classification
* Dataset: glue
* Config: sst2
* Split: validation
To run new evaluation jobs, visit Hugging Face's [automatic model evaluator](https://huggingface.co/spaces/autoevaluate/model-evaluator).
## Contributions
Thanks to [@lewtun](https://huggingface.co/lewtun) for evaluating this model. | autoevaluate/autoeval-staging-eval-project-e6349348-5660-49a6-843b-4c305a6146f2-2624 | [
"autotrain",
"evaluation",
"region:us"
] | 2022-11-18T11:09:03+00:00 | {"type": "predictions", "tags": ["autotrain", "evaluation"], "datasets": ["glue"], "eval_info": {"task": "binary_classification", "model": "autoevaluate/binary-classification", "metrics": ["matthews_correlation"], "dataset_name": "glue", "dataset_config": "sst2", "dataset_split": "validation", "col_mapping": {"text": "sentence", "target": "label"}}} | 2022-11-18T11:09:39+00:00 | [] | [] | TAGS
#autotrain #evaluation #region-us
| # Dataset Card for AutoTrain Evaluator
This repository contains model predictions generated by AutoTrain for the following task and dataset:
* Task: Binary Text Classification
* Model: autoevaluate/binary-classification
* Dataset: glue
* Config: sst2
* Split: validation
To run new evaluation jobs, visit Hugging Face's automatic model evaluator.
## Contributions
Thanks to @lewtun for evaluating this model. | [
"# Dataset Card for AutoTrain Evaluator\n\nThis repository contains model predictions generated by AutoTrain for the following task and dataset:\n\n* Task: Binary Text Classification\n* Model: autoevaluate/binary-classification\n* Dataset: glue\n* Config: sst2\n* Split: validation\n\nTo run new evaluation jobs, visit Hugging Face's automatic model evaluator.",
"## Contributions\n\nThanks to @lewtun for evaluating this model."
] | [
"TAGS\n#autotrain #evaluation #region-us \n",
"# Dataset Card for AutoTrain Evaluator\n\nThis repository contains model predictions generated by AutoTrain for the following task and dataset:\n\n* Task: Binary Text Classification\n* Model: autoevaluate/binary-classification\n* Dataset: glue\n* Config: sst2\n* Split: validation\n\nTo run new evaluation jobs, visit Hugging Face's automatic model evaluator.",
"## Contributions\n\nThanks to @lewtun for evaluating this model."
] |
bfc6a6071e5fc81c992e01784c8195aa1d23e910 | # Dataset Card for AutoTrain Evaluator
This repository contains model predictions generated by [AutoTrain](https://huggingface.co/autotrain) for the following task and dataset:
* Task: Binary Text Classification
* Model: autoevaluate/binary-classification
* Dataset: glue
* Config: sst2
* Split: validation
To run new evaluation jobs, visit Hugging Face's [automatic model evaluator](https://huggingface.co/spaces/autoevaluate/model-evaluator).
## Contributions
Thanks to [@lewtun](https://huggingface.co/lewtun) for evaluating this model. | autoevaluate/autoeval-staging-eval-project-6a0cd869-0e5a-4c97-8312-c7fea68b3609-2725 | [
"autotrain",
"evaluation",
"region:us"
] | 2022-11-18T11:19:34+00:00 | {"type": "predictions", "tags": ["autotrain", "evaluation"], "datasets": ["glue"], "eval_info": {"task": "binary_classification", "model": "autoevaluate/binary-classification", "metrics": ["matthews_correlation"], "dataset_name": "glue", "dataset_config": "sst2", "dataset_split": "validation", "col_mapping": {"text": "sentence", "target": "label"}}} | 2022-11-18T11:20:11+00:00 | [] | [] | TAGS
#autotrain #evaluation #region-us
| # Dataset Card for AutoTrain Evaluator
This repository contains model predictions generated by AutoTrain for the following task and dataset:
* Task: Binary Text Classification
* Model: autoevaluate/binary-classification
* Dataset: glue
* Config: sst2
* Split: validation
To run new evaluation jobs, visit Hugging Face's automatic model evaluator.
## Contributions
Thanks to @lewtun for evaluating this model. | [
"# Dataset Card for AutoTrain Evaluator\n\nThis repository contains model predictions generated by AutoTrain for the following task and dataset:\n\n* Task: Binary Text Classification\n* Model: autoevaluate/binary-classification\n* Dataset: glue\n* Config: sst2\n* Split: validation\n\nTo run new evaluation jobs, visit Hugging Face's automatic model evaluator.",
"## Contributions\n\nThanks to @lewtun for evaluating this model."
] | [
"TAGS\n#autotrain #evaluation #region-us \n",
"# Dataset Card for AutoTrain Evaluator\n\nThis repository contains model predictions generated by AutoTrain for the following task and dataset:\n\n* Task: Binary Text Classification\n* Model: autoevaluate/binary-classification\n* Dataset: glue\n* Config: sst2\n* Split: validation\n\nTo run new evaluation jobs, visit Hugging Face's automatic model evaluator.",
"## Contributions\n\nThanks to @lewtun for evaluating this model."
] |
23c0a29d28d0bbdb4c2bbcff56fda332379e69b0 | # Dataset Card for "sidewalk-imagery"
[More Information needed](https://github.com/huggingface/datasets/blob/main/CONTRIBUTING.md#how-to-contribute-to-the-dataset-cards) | pattern123/sidewalk-imagery | [
"region:us"
] | 2022-11-18T14:01:20+00:00 | {"dataset_info": {"features": [{"name": "pixel_values", "dtype": "image"}, {"name": "label", "dtype": "image"}], "splits": [{"name": "train", "num_bytes": 3138394.0, "num_examples": 10}], "download_size": 3139599, "dataset_size": 3138394.0}} | 2022-11-19T05:23:06+00:00 | [] | [] | TAGS
#region-us
| # Dataset Card for "sidewalk-imagery"
More Information needed | [
"# Dataset Card for \"sidewalk-imagery\"\n\nMore Information needed"
] | [
"TAGS\n#region-us \n",
"# Dataset Card for \"sidewalk-imagery\"\n\nMore Information needed"
] |
21187d1891b1911eeb12022294a5681e28edb7eb |
# Dataset Card for Dataset Name
## Dataset Description
- **Homepage:**
- **Repository:**
- **Paper:**
- **Leaderboard:**
- **Point of Contact:**
### Dataset Summary
This dataset card aims to be a base template for new datasets. It has been generated using [this raw template](https://github.com/huggingface/huggingface_hub/blob/main/src/huggingface_hub/templates/datasetcard_template.md?plain=1).
### Supported Tasks and Leaderboards
[More Information Needed]
### Languages
[More Information Needed]
## Dataset Structure
### Data Instances
[More Information Needed]
### Data Fields
[More Information Needed]
### Data Splits
[More Information Needed]
## Dataset Creation
### Curation Rationale
[More Information Needed]
### Source Data
#### Initial Data Collection and Normalization
[More Information Needed]
#### Who are the source language producers?
[More Information Needed]
### Annotations
#### Annotation process
[More Information Needed]
#### Who are the annotators?
[More Information Needed]
### Personal and Sensitive Information
[More Information Needed]
## Considerations for Using the Data
### Social Impact of Dataset
[More Information Needed]
### Discussion of Biases
[More Information Needed]
### Other Known Limitations
[More Information Needed]
## Additional Information
### Dataset Curators
[More Information Needed]
### Licensing Information
[More Information Needed]
### Citation Information
[More Information Needed]
### Contributions
[More Information Needed] | ccao/test | [
"region:us"
] | 2022-11-18T16:17:38+00:00 | {} | 2023-01-19T05:02:01+00:00 | [] | [] | TAGS
#region-us
|
# Dataset Card for Dataset Name
## Dataset Description
- Homepage:
- Repository:
- Paper:
- Leaderboard:
- Point of Contact:
### Dataset Summary
This dataset card aims to be a base template for new datasets. It has been generated using this raw template.
### Supported Tasks and Leaderboards
### Languages
## Dataset Structure
### Data Instances
### Data Fields
### Data Splits
## Dataset Creation
### Curation Rationale
### Source Data
#### Initial Data Collection and Normalization
#### Who are the source language producers?
### Annotations
#### Annotation process
#### Who are the annotators?
### Personal and Sensitive Information
## Considerations for Using the Data
### Social Impact of Dataset
### Discussion of Biases
### Other Known Limitations
## Additional Information
### Dataset Curators
### Licensing Information
### Contributions
| [
"# Dataset Card for Dataset Name",
"## Dataset Description\n\n- Homepage: \n- Repository: \n- Paper: \n- Leaderboard: \n- Point of Contact:",
"### Dataset Summary\n\nThis dataset card aims to be a base template for new datasets. It has been generated using this raw template.",
"### Supported Tasks and Leaderboards",
"### Languages",
"## Dataset Structure",
"### Data Instances",
"### Data Fields",
"### Data Splits",
"## Dataset Creation",
"### Curation Rationale",
"### Source Data",
"#### Initial Data Collection and Normalization",
"#### Who are the source language producers?",
"### Annotations",
"#### Annotation process",
"#### Who are the annotators?",
"### Personal and Sensitive Information",
"## Considerations for Using the Data",
"### Social Impact of Dataset",
"### Discussion of Biases",
"### Other Known Limitations",
"## Additional Information",
"### Dataset Curators",
"### Licensing Information",
"### Contributions"
] | [
"TAGS\n#region-us \n",
"# Dataset Card for Dataset Name",
"## Dataset Description\n\n- Homepage: \n- Repository: \n- Paper: \n- Leaderboard: \n- Point of Contact:",
"### Dataset Summary\n\nThis dataset card aims to be a base template for new datasets. It has been generated using this raw template.",
"### Supported Tasks and Leaderboards",
"### Languages",
"## Dataset Structure",
"### Data Instances",
"### Data Fields",
"### Data Splits",
"## Dataset Creation",
"### Curation Rationale",
"### Source Data",
"#### Initial Data Collection and Normalization",
"#### Who are the source language producers?",
"### Annotations",
"#### Annotation process",
"#### Who are the annotators?",
"### Personal and Sensitive Information",
"## Considerations for Using the Data",
"### Social Impact of Dataset",
"### Discussion of Biases",
"### Other Known Limitations",
"## Additional Information",
"### Dataset Curators",
"### Licensing Information",
"### Contributions"
] |
2bc7902538956d23470bd31923ae3ff2d12757bb | # Dataset Card for "cstop_artificial"
[More Information needed](https://github.com/huggingface/datasets/blob/main/CONTRIBUTING.md#how-to-contribute-to-the-dataset-cards) | WillHeld/cstop_artificial | [
"region:us"
] | 2022-11-18T20:13:29+00:00 | {"dataset_info": {"features": [{"name": "utterance", "dtype": "string"}, {"name": " semantic_parse", "dtype": "string"}, {"name": "semantic_parse", "dtype": "string"}], "splits": [{"name": "eval", "num_bytes": 113084, "num_examples": 559}, {"name": "test", "num_bytes": 233020, "num_examples": 1167}, {"name": "train", "num_bytes": 819464, "num_examples": 4077}], "download_size": 371646, "dataset_size": 1165568}} | 2022-12-10T17:54:16+00:00 | [] | [] | TAGS
#region-us
| # Dataset Card for "cstop_artificial"
More Information needed | [
"# Dataset Card for \"cstop_artificial\"\n\nMore Information needed"
] | [
"TAGS\n#region-us \n",
"# Dataset Card for \"cstop_artificial\"\n\nMore Information needed"
] |
9e0916d21f6fbedd8a1786e8be29b0df87b40bb1 |
<h4> Usage </h4>
To use this embedding you have to download the file and put it into the "\stable-diffusion-webui\embeddings" folder
To use it in a prompt add
<em style="font-weight:600">art by rogue_style </em>
add <b>[ ]</b> around it to reduce its weight.
<h4> Included Files </h4>
<ul>
<li>500 steps <em>Usage: art by rogue_style-500</em></li>
<li>3500 steps <em>Usage: art by rogue_style-3500</em></li>
<li>6500 steps <em>Usage: art by rogue_style</em> </li>
</ul>
cheers<br>
Wipeout
<h4> Example Pictures </h4>
<table>
<tbody>
<tr>
<td><img height="100%/" width="100%" src="https://i.imgur.com/JefZ3cA.png"></td>
<td><img height="100%/" width="100%" src="https://i.imgur.com/YBJzVIi.png"></td>
<td><img height="100%/" width="100%" src="https://i.imgur.com/96iutfu.png"></td>
<td><img height="100%/" width="100%" src="https://i.imgur.com/SBKfnc4.png"></td>
</tr>
</tbody>
</table>
<h4> prompt comparison </h4>
<em> click the image to enlarge</em>
<a href="https://i.imgur.com/a6te4zG.png" target="_blank"><img height="50%" width="50%" src="https://i.imgur.com/a6te4zG.png"></a>
| zZWipeoutZz/rogue_style | [
"license:creativeml-openrail-m",
"region:us"
] | 2022-11-18T20:40:41+00:00 | {"license": "creativeml-openrail-m"} | 2022-11-19T15:03:02+00:00 | [] | [] | TAGS
#license-creativeml-openrail-m #region-us
| #### Usage
To use this embedding you have to download the file and put it into the "\stable-diffusion-webui\embeddings" folder
To use it in a prompt add
*art by rogue\_style*
add **[ ]** around it to reduce its weight.
#### Included Files
* 500 steps *Usage: art by rogue\_style-500*
* 3500 steps *Usage: art by rogue\_style-3500*
* 6500 steps *Usage: art by rogue\_style*
cheers
Wipeout
#### Example Pictures
#### prompt comparison
*click the image to enlarge*
[<img height="50%" width="50%" src="https://i.URL](https://i.URL target=) | [
"#### Usage\n\n\nTo use this embedding you have to download the file and put it into the \"\\stable-diffusion-webui\\embeddings\" folder\nTo use it in a prompt add\n*art by rogue\\_style* \n\n\nadd **[ ]** around it to reduce its weight.",
"#### Included Files\n\n\n* 500 steps *Usage: art by rogue\\_style-500*\n* 3500 steps *Usage: art by rogue\\_style-3500*\n* 6500 steps *Usage: art by rogue\\_style*\n\n\ncheers \n\nWipeout",
"#### Example Pictures",
"#### prompt comparison\n\n\n *click the image to enlarge*\n[<img height=\"50%\" width=\"50%\" src=\"https://i.URL](https://i.URL target=)"
] | [
"TAGS\n#license-creativeml-openrail-m #region-us \n",
"#### Usage\n\n\nTo use this embedding you have to download the file and put it into the \"\\stable-diffusion-webui\\embeddings\" folder\nTo use it in a prompt add\n*art by rogue\\_style* \n\n\nadd **[ ]** around it to reduce its weight.",
"#### Included Files\n\n\n* 500 steps *Usage: art by rogue\\_style-500*\n* 3500 steps *Usage: art by rogue\\_style-3500*\n* 6500 steps *Usage: art by rogue\\_style*\n\n\ncheers \n\nWipeout",
"#### Example Pictures",
"#### prompt comparison\n\n\n *click the image to enlarge*\n[<img height=\"50%\" width=\"50%\" src=\"https://i.URL](https://i.URL target=)"
] |
45ae712ac42fa0209015db476c1d040e17442527 | # Dataset Card for "highways-hacktum"
[More Information needed](https://github.com/huggingface/datasets/blob/main/CONTRIBUTING.md#how-to-contribute-to-the-dataset-cards) | ogimgio/highways-hacktum | [
"region:us"
] | 2022-11-18T23:59:44+00:00 | {"dataset_info": {"features": [{"name": "image", "dtype": "image"}, {"name": "label", "dtype": {"class_label": {"names": {"0": "footway", "1": "primary"}}}}], "splits": [{"name": "train", "num_bytes": 1155915978.0, "num_examples": 500}, {"name": "validation", "num_bytes": 284161545.0, "num_examples": 125}], "download_size": 1431719317, "dataset_size": 1440077523.0}} | 2022-11-19T00:04:45+00:00 | [] | [] | TAGS
#region-us
| # Dataset Card for "highways-hacktum"
More Information needed | [
"# Dataset Card for \"highways-hacktum\"\n\nMore Information needed"
] | [
"TAGS\n#region-us \n",
"# Dataset Card for \"highways-hacktum\"\n\nMore Information needed"
] |
74a44153625d0382b9d3c8af0a49a16e0c3cef0e |
# Dataset Card for ravnursson_asr
## Table of Contents
- [Dataset Description](#dataset-description)
- [Dataset Summary](#dataset-summary)
- [Supported Tasks](#supported-tasks-and-leaderboards)
- [Languages](#languages)
- [Dataset Structure](#dataset-structure)
- [Data Instances](#data-instances)
- [Data Fields](#data-fields)
- [Data Splits](#data-splits)
- [Dataset Creation](#dataset-creation)
- [Curation Rationale](#curation-rationale)
- [Source Data](#source-data)
- [Annotations](#annotations)
- [Personal and Sensitive Information](#personal-and-sensitive-information)
- [Considerations for Using the Data](#considerations-for-using-the-data)
- [Social Impact of Dataset](#social-impact-of-dataset)
- [Discussion of Biases](#discussion-of-biases)
- [Other Known Limitations](#other-known-limitations)
- [Additional Information](#additional-information)
- [Dataset Curators](#dataset-curators)
- [Licensing Information](#licensing-information)
- [Citation Information](#citation-information)
- [Contributions](#contributions)
## Dataset Description
- **Homepage:** [Ravnursson Faroese Speech and Transcripts](http://hdl.handle.net/20.500.12537/276)
- **Repository:** [Clarin.is](http://hdl.handle.net/20.500.12537/276)
- **Paper:** [ASR Language Resources for Faroese](https://aclanthology.org/2023.nodalida-1.4.pdf)
- **Paper:** [Creating a basic language resource kit for faroese.](https://aclanthology.org/2022.lrec-1.495.pdf)
- **Point of Contact:** [Annika Simonsen](mailto:[email protected]), [Carlos Mena](mailto:[email protected])
### Dataset Summary
The corpus "RAVNURSSON FAROESE SPEECH AND TRANSCRIPTS" (or RAVNURSSON Corpus for short) is a collection of speech recordings with transcriptions intended for Automatic Speech Recognition (ASR) applications in the language that is spoken at the Faroe Islands (Faroese). It was curated at the Reykjavík University (RU) in 2022.
The RAVNURSSON Corpus is an extract of the "Basic Language Resource Kit 1.0" (BLARK 1.0) [1] developed by the Ravnur Project from the Faroe Islands [2]. As a matter of fact, the name RAVNURSSON comes from Ravnur (a tribute to the Ravnur Project) and the suffix "son" which in Icelandic means "son of". Therefore, the name "RAVNURSSON" means "The (Icelandic) son of Ravnur". The double "ss" is just for aesthetics.
The audio was collected by recording speakers reading texts. The participants are aged 15-83, divided into 3 age groups: 15-35, 36-60 and 61+.
The speech files are made of 249 female speakers and 184 male speakers; 433 speakers total. The recordings were made on TASCAM DR-40 Linear PCM audio recorders using the built-in stereo microphones in WAVE 16 bit with a sample rate of 48kHz, but then, downsampled to 16kHz@16bit mono for this corpus.
[1] Simonsen, A., Debess, I. N., Lamhauge, S. S., & Henrichsen, P. J. Creating a basic language resource kit for Faroese. In LREC 2022. 13th International Conference on Language Resources and Evaluation.
[2] Website. The Project Ravnur under the Talutøkni Foundation https://maltokni.fo/en/the-ravnur-project
### Example Usage
The RAVNURSSON Corpus is divided in 3 splits: train, validation and test. To load a specific split pass its name as a config name:
```python
from datasets import load_dataset
ravnursson = load_dataset("carlosdanielhernandezmena/ravnursson_asr")
```
To load an specific split (for example, the validation split) do:
```python
from datasets import load_dataset
ravnursson = load_dataset("carlosdanielhernandezmena/ravnursson_asr",split="validation")
```
### Supported Tasks
automatic-speech-recognition: The dataset can be used to train a model for Automatic Speech Recognition (ASR). The model is presented with an audio file and asked to transcribe the audio file to written text. The most common evaluation metric is the word error rate (WER).
### Languages
The audio is in Faroese.
The reading prompts for the RAVNURSSON Corpus have been generated by expert linguists. The whole corpus was balanced for phonetic and dialectal coverage; Test and Dev subsets are gender-balanced. Tabular computer-searchable information is included as well as written documentation.
## Dataset Structure
### Data Instances
```python
{
'audio_id': 'KAM06_151121_0101',
'audio': {
'path': '/home/carlos/.cache/HuggingFace/datasets/downloads/extracted/32b4a757027b72b8d2e25cd9c8be9c7c919cc8d4eb1a9a899e02c11fd6074536/dev/RDATA2/KAM06_151121/KAM06_151121_0101.flac',
'array': array([ 0.0010376 , -0.00521851, -0.00393677, ..., 0.00128174,
0.00076294, 0.00045776], dtype=float32),
'sampling_rate': 16000
},
'speaker_id': 'KAM06_151121',
'gender': 'female',
'age': '36-60',
'duration': 4.863999843597412,
'normalized_text': 'endurskin eru týdningarmikil í myrkri',
'dialect': 'sandoy'
}
```
### Data Fields
* `audio_id` (string) - id of audio segment
* `audio` (datasets.Audio) - a dictionary containing the path to the audio, the decoded audio array, and the sampling rate. In non-streaming mode (default), the path points to the locally extracted audio. In streaming mode, the path is the relative path of an audio inside its archive (as files are not downloaded and extracted locally).
* `speaker_id` (string) - id of speaker
* `gender` (string) - gender of speaker (male or female)
* `age` (string) - range of age of the speaker: Younger (15-35), Middle-aged (36-60) or Elderly (61+).
* `duration` (float32) - duration of the audio file in seconds.
* `normalized_text` (string) - normalized audio segment transcription
* `dialect` (string) - dialect group, for example "Suðuroy" or "Sandoy".
### Data Splits
The speech material has been subdivided into portions for training (train), development (evaluation) and testing (test). Lengths of each portion are: train = 100h08m, test = 4h30m, dev (evaluation)=4h30m.
To load an specific portion please see the above section "Example Usage".
The development and test portions have exactly 10 male and 10 female speakers each and both portions have exactly the same size in hours (4.5h each).
## Dataset Creation
### Curation Rationale
The directory called "speech" contains all the speech files of the corpus. The files in the speech directory are divided in three directories: train, dev and test. The train portion is sub-divided in three types of recordings: RDATA1O, RDATA1OP and RDATA2; this is due to the organization of the recordings in the original BLARK 1.0. There, the recordings are divided in Rdata1 and Rdata2.
One main difference between Rdata1 and Rdata2 is that the reading environment for Rdata2 was controlled by a software called "PushPrompt" which is included in the original BLARK 1.0. Another main difference is that in Rdata1 there are some available transcriptions labeled at the phoneme level. For this reason the audio files in the speech directory of the RAVNURSSON corpus are divided in the folders RDATA1O where "O" is for "Orthographic" and RDATA1OP where "O" is for Orthographic and "P" is for phonetic.
In the case of the dev and test portions, the data come only from Rdata2 which does not have labels at the phonetic level.
It is important to clarify that the RAVNURSSON Corpus only includes transcriptions at the orthographic level.
### Source Data
#### Initial Data Collection and Normalization
The dataset was released with normalized text only at an orthographic level in lower-case. The normalization process was performed by automatically removing punctuation marks and characters that are not present in the Faroese alphabet.
#### Who are the source language producers?
* The utterances were recorded using a TASCAM DR-40.
* Participants self-reported their age group, gender, native language and dialect.
* Participants are aged between 15 to 83 years.
* The corpus contains 71949 speech files from 433 speakers, totalling 109 hours and 9 minutes.
### Annotations
#### Annotation process
Most of the reading prompts were selected by experts from a Faroese text corpus (news, blogs, Wikipedia etc.) and were edited to fit the format. Reading prompts that are within specific domains (such as Faroese place names, numbers, license plates, telling time etc.) were written by the Ravnur Project. Then, a software tool called PushPrompt were used for reading sessions (voice recordings). PushPromt presents the text items in the reading material to the reader, allowing him/her to manage the session interactively (adjusting the reading tempo, repeating speech productions at wish, inserting short breaks as needed, etc.). When the reading session is completed, a log file (with time stamps for each production) is written as a data table compliant with the TextGrid-format.
#### Who are the annotators?
The corpus was annotated by the [Ravnur Project](https://maltokni.fo/en/the-ravnur-project)
### Personal and Sensitive Information
The dataset consists of people who have donated their voice. You agree to not attempt to determine the identity of speakers in this dataset.
## Considerations for Using the Data
### Social Impact of Dataset
This is the first ASR corpus in Faroese.
### Discussion of Biases
As the number of reading prompts was limited, the common denominator in the RAVNURSSON corpus is that one prompt is read by more than one speaker. This is relevant because is a common practice in ASR to create a language model using the prompts that are found in the train portion of the corpus. That is not recommended for the RAVNURSSON Corpus as it counts with many prompts shared by all the portions and that will produce an important bias in the language modeling task.
In this section we present some statistics about the repeated prompts through all the portions of the corpus.
- In the train portion:
* Total number of prompts = 65616
* Number of unique prompts = 38646
There are 26970 repeated prompts in the train portion. In other words, 41.1% of the prompts are repeated.
- In the test portion:
* Total number of prompts = 3002
* Number of unique prompts = 2887
There are 115 repeated prompts in the test portion. In other words, 3.83% of the prompts are repeated.
- In the dev portion:
* Total number of prompts = 3331
* Number of unique prompts = 3302
There are 29 repeated prompts in the dev portion. In other words, 0.87% of the prompts are repeated.
- Considering the corpus as a whole:
* Total number of prompts = 71949
* Number of unique prompts = 39945
There are 32004 repeated prompts in the whole corpus. In other words, 44.48% of the prompts are repeated.
NOTICE!: It is also important to clarify that none of the 3 portions of the corpus share speakers.
### Other Known Limitations
"RAVNURSSON FAROESE SPEECH AND TRANSCRIPTS" by Carlos Daniel Hernández Mena and Annika Simonsen is licensed under a Creative Commons Attribution 4.0 International (CC BY 4.0) License with the hope that it will be useful, but WITHOUT ANY WARRANTY; without even the implied warranty of MERCHANTABILITY or FITNESS FOR A PARTICULAR PURPOSE.
## Additional Information
### Dataset Curators
The dataset was collected by Annika Simonsen and curated by Carlos Daniel Hernández Mena.
### Licensing Information
[CC-BY-4.0](https://creativecommons.org/licenses/by/4.0/)
### Citation Information
```
@misc{carlosmenaravnursson2022,
title={Ravnursson Faroese Speech and Transcripts},
author={Hernandez Mena, Carlos Daniel and Simonsen, Annika},
year={2022},
url={http://hdl.handle.net/20.500.12537/276},
}
```
### Contributions
This project was made possible under the umbrella of the Language Technology Programme for Icelandic 2019-2023. The programme, which is managed and coordinated by Almannarómur, is funded by the Icelandic Ministry of Education, Science and Culture.
Special thanks to Dr. Jón Guðnason, professor at Reykjavík University and head of the Language and Voice Lab (LVL) for providing computational resources.
| carlosdanielhernandezmena/ravnursson_asr | [
"task_categories:automatic-speech-recognition",
"annotations_creators:expert-generated",
"language_creators:expert-generated",
"multilinguality:monolingual",
"size_categories:10K<n<100K",
"source_datasets:original",
"language:fo",
"license:cc-by-4.0",
"faroe islands",
"faroese",
"ravnur project",
"speech recognition in faroese",
"region:us"
] | 2022-11-19T00:02:04+00:00 | {"annotations_creators": ["expert-generated"], "language_creators": ["expert-generated"], "language": ["fo"], "license": ["cc-by-4.0"], "multilinguality": ["monolingual"], "size_categories": ["10K<n<100K"], "source_datasets": ["original"], "task_categories": ["automatic-speech-recognition"], "task_ids": [], "pretty_name": "RAVNURSSON FAROESE SPEECH AND TRANSCRIPTS", "tags": ["faroe islands", "faroese", "ravnur project", "speech recognition in faroese"]} | 2023-07-10T20:20:03+00:00 | [] | [
"fo"
] | TAGS
#task_categories-automatic-speech-recognition #annotations_creators-expert-generated #language_creators-expert-generated #multilinguality-monolingual #size_categories-10K<n<100K #source_datasets-original #language-Faroese #license-cc-by-4.0 #faroe islands #faroese #ravnur project #speech recognition in faroese #region-us
|
# Dataset Card for ravnursson_asr
## Table of Contents
- Dataset Description
- Dataset Summary
- Supported Tasks
- Languages
- Dataset Structure
- Data Instances
- Data Fields
- Data Splits
- Dataset Creation
- Curation Rationale
- Source Data
- Annotations
- Personal and Sensitive Information
- Considerations for Using the Data
- Social Impact of Dataset
- Discussion of Biases
- Other Known Limitations
- Additional Information
- Dataset Curators
- Licensing Information
- Citation Information
- Contributions
## Dataset Description
- Homepage: Ravnursson Faroese Speech and Transcripts
- Repository: URL
- Paper: ASR Language Resources for Faroese
- Paper: Creating a basic language resource kit for faroese.
- Point of Contact: Annika Simonsen, Carlos Mena
### Dataset Summary
The corpus "RAVNURSSON FAROESE SPEECH AND TRANSCRIPTS" (or RAVNURSSON Corpus for short) is a collection of speech recordings with transcriptions intended for Automatic Speech Recognition (ASR) applications in the language that is spoken at the Faroe Islands (Faroese). It was curated at the Reykjavík University (RU) in 2022.
The RAVNURSSON Corpus is an extract of the "Basic Language Resource Kit 1.0" (BLARK 1.0) [1] developed by the Ravnur Project from the Faroe Islands [2]. As a matter of fact, the name RAVNURSSON comes from Ravnur (a tribute to the Ravnur Project) and the suffix "son" which in Icelandic means "son of". Therefore, the name "RAVNURSSON" means "The (Icelandic) son of Ravnur". The double "ss" is just for aesthetics.
The audio was collected by recording speakers reading texts. The participants are aged 15-83, divided into 3 age groups: 15-35, 36-60 and 61+.
The speech files are made of 249 female speakers and 184 male speakers; 433 speakers total. The recordings were made on TASCAM DR-40 Linear PCM audio recorders using the built-in stereo microphones in WAVE 16 bit with a sample rate of 48kHz, but then, downsampled to 16kHz@16bit mono for this corpus.
[1] Simonsen, A., Debess, I. N., Lamhauge, S. S., & Henrichsen, P. J. Creating a basic language resource kit for Faroese. In LREC 2022. 13th International Conference on Language Resources and Evaluation.
[2] Website. The Project Ravnur under the Talutøkni Foundation URL
### Example Usage
The RAVNURSSON Corpus is divided in 3 splits: train, validation and test. To load a specific split pass its name as a config name:
To load an specific split (for example, the validation split) do:
### Supported Tasks
automatic-speech-recognition: The dataset can be used to train a model for Automatic Speech Recognition (ASR). The model is presented with an audio file and asked to transcribe the audio file to written text. The most common evaluation metric is the word error rate (WER).
### Languages
The audio is in Faroese.
The reading prompts for the RAVNURSSON Corpus have been generated by expert linguists. The whole corpus was balanced for phonetic and dialectal coverage; Test and Dev subsets are gender-balanced. Tabular computer-searchable information is included as well as written documentation.
## Dataset Structure
### Data Instances
### Data Fields
* 'audio_id' (string) - id of audio segment
* 'audio' (datasets.Audio) - a dictionary containing the path to the audio, the decoded audio array, and the sampling rate. In non-streaming mode (default), the path points to the locally extracted audio. In streaming mode, the path is the relative path of an audio inside its archive (as files are not downloaded and extracted locally).
* 'speaker_id' (string) - id of speaker
* 'gender' (string) - gender of speaker (male or female)
* 'age' (string) - range of age of the speaker: Younger (15-35), Middle-aged (36-60) or Elderly (61+).
* 'duration' (float32) - duration of the audio file in seconds.
* 'normalized_text' (string) - normalized audio segment transcription
* 'dialect' (string) - dialect group, for example "Suðuroy" or "Sandoy".
### Data Splits
The speech material has been subdivided into portions for training (train), development (evaluation) and testing (test). Lengths of each portion are: train = 100h08m, test = 4h30m, dev (evaluation)=4h30m.
To load an specific portion please see the above section "Example Usage".
The development and test portions have exactly 10 male and 10 female speakers each and both portions have exactly the same size in hours (4.5h each).
## Dataset Creation
### Curation Rationale
The directory called "speech" contains all the speech files of the corpus. The files in the speech directory are divided in three directories: train, dev and test. The train portion is sub-divided in three types of recordings: RDATA1O, RDATA1OP and RDATA2; this is due to the organization of the recordings in the original BLARK 1.0. There, the recordings are divided in Rdata1 and Rdata2.
One main difference between Rdata1 and Rdata2 is that the reading environment for Rdata2 was controlled by a software called "PushPrompt" which is included in the original BLARK 1.0. Another main difference is that in Rdata1 there are some available transcriptions labeled at the phoneme level. For this reason the audio files in the speech directory of the RAVNURSSON corpus are divided in the folders RDATA1O where "O" is for "Orthographic" and RDATA1OP where "O" is for Orthographic and "P" is for phonetic.
In the case of the dev and test portions, the data come only from Rdata2 which does not have labels at the phonetic level.
It is important to clarify that the RAVNURSSON Corpus only includes transcriptions at the orthographic level.
### Source Data
#### Initial Data Collection and Normalization
The dataset was released with normalized text only at an orthographic level in lower-case. The normalization process was performed by automatically removing punctuation marks and characters that are not present in the Faroese alphabet.
#### Who are the source language producers?
* The utterances were recorded using a TASCAM DR-40.
* Participants self-reported their age group, gender, native language and dialect.
* Participants are aged between 15 to 83 years.
* The corpus contains 71949 speech files from 433 speakers, totalling 109 hours and 9 minutes.
### Annotations
#### Annotation process
Most of the reading prompts were selected by experts from a Faroese text corpus (news, blogs, Wikipedia etc.) and were edited to fit the format. Reading prompts that are within specific domains (such as Faroese place names, numbers, license plates, telling time etc.) were written by the Ravnur Project. Then, a software tool called PushPrompt were used for reading sessions (voice recordings). PushPromt presents the text items in the reading material to the reader, allowing him/her to manage the session interactively (adjusting the reading tempo, repeating speech productions at wish, inserting short breaks as needed, etc.). When the reading session is completed, a log file (with time stamps for each production) is written as a data table compliant with the TextGrid-format.
#### Who are the annotators?
The corpus was annotated by the Ravnur Project
### Personal and Sensitive Information
The dataset consists of people who have donated their voice. You agree to not attempt to determine the identity of speakers in this dataset.
## Considerations for Using the Data
### Social Impact of Dataset
This is the first ASR corpus in Faroese.
### Discussion of Biases
As the number of reading prompts was limited, the common denominator in the RAVNURSSON corpus is that one prompt is read by more than one speaker. This is relevant because is a common practice in ASR to create a language model using the prompts that are found in the train portion of the corpus. That is not recommended for the RAVNURSSON Corpus as it counts with many prompts shared by all the portions and that will produce an important bias in the language modeling task.
In this section we present some statistics about the repeated prompts through all the portions of the corpus.
- In the train portion:
* Total number of prompts = 65616
* Number of unique prompts = 38646
There are 26970 repeated prompts in the train portion. In other words, 41.1% of the prompts are repeated.
- In the test portion:
* Total number of prompts = 3002
* Number of unique prompts = 2887
There are 115 repeated prompts in the test portion. In other words, 3.83% of the prompts are repeated.
- In the dev portion:
* Total number of prompts = 3331
* Number of unique prompts = 3302
There are 29 repeated prompts in the dev portion. In other words, 0.87% of the prompts are repeated.
- Considering the corpus as a whole:
* Total number of prompts = 71949
* Number of unique prompts = 39945
There are 32004 repeated prompts in the whole corpus. In other words, 44.48% of the prompts are repeated.
NOTICE!: It is also important to clarify that none of the 3 portions of the corpus share speakers.
### Other Known Limitations
"RAVNURSSON FAROESE SPEECH AND TRANSCRIPTS" by Carlos Daniel Hernández Mena and Annika Simonsen is licensed under a Creative Commons Attribution 4.0 International (CC BY 4.0) License with the hope that it will be useful, but WITHOUT ANY WARRANTY; without even the implied warranty of MERCHANTABILITY or FITNESS FOR A PARTICULAR PURPOSE.
## Additional Information
### Dataset Curators
The dataset was collected by Annika Simonsen and curated by Carlos Daniel Hernández Mena.
### Licensing Information
CC-BY-4.0
### Contributions
This project was made possible under the umbrella of the Language Technology Programme for Icelandic 2019-2023. The programme, which is managed and coordinated by Almannarómur, is funded by the Icelandic Ministry of Education, Science and Culture.
Special thanks to Dr. Jón Guðnason, professor at Reykjavík University and head of the Language and Voice Lab (LVL) for providing computational resources.
| [
"# Dataset Card for ravnursson_asr",
"## Table of Contents\n- Dataset Description\n - Dataset Summary\n - Supported Tasks\n - Languages\n- Dataset Structure\n - Data Instances\n - Data Fields\n - Data Splits\n- Dataset Creation\n - Curation Rationale\n - Source Data\n - Annotations\n - Personal and Sensitive Information\n- Considerations for Using the Data\n - Social Impact of Dataset\n - Discussion of Biases\n - Other Known Limitations\n- Additional Information\n - Dataset Curators\n - Licensing Information\n - Citation Information\n - Contributions",
"## Dataset Description\n- Homepage: Ravnursson Faroese Speech and Transcripts\n- Repository: URL\n- Paper: ASR Language Resources for Faroese\n- Paper: Creating a basic language resource kit for faroese.\n- Point of Contact: Annika Simonsen, Carlos Mena",
"### Dataset Summary\nThe corpus \"RAVNURSSON FAROESE SPEECH AND TRANSCRIPTS\" (or RAVNURSSON Corpus for short) is a collection of speech recordings with transcriptions intended for Automatic Speech Recognition (ASR) applications in the language that is spoken at the Faroe Islands (Faroese). It was curated at the Reykjavík University (RU) in 2022.\n\nThe RAVNURSSON Corpus is an extract of the \"Basic Language Resource Kit 1.0\" (BLARK 1.0) [1] developed by the Ravnur Project from the Faroe Islands [2]. As a matter of fact, the name RAVNURSSON comes from Ravnur (a tribute to the Ravnur Project) and the suffix \"son\" which in Icelandic means \"son of\". Therefore, the name \"RAVNURSSON\" means \"The (Icelandic) son of Ravnur\". The double \"ss\" is just for aesthetics.\n\nThe audio was collected by recording speakers reading texts. The participants are aged 15-83, divided into 3 age groups: 15-35, 36-60 and 61+.\n\nThe speech files are made of 249 female speakers and 184 male speakers; 433 speakers total. The recordings were made on TASCAM DR-40 Linear PCM audio recorders using the built-in stereo microphones in WAVE 16 bit with a sample rate of 48kHz, but then, downsampled to 16kHz@16bit mono for this corpus.\n\n[1] Simonsen, A., Debess, I. N., Lamhauge, S. S., & Henrichsen, P. J. Creating a basic language resource kit for Faroese. In LREC 2022. 13th International Conference on Language Resources and Evaluation.\n \n[2] Website. The Project Ravnur under the Talutøkni Foundation URL",
"### Example Usage\nThe RAVNURSSON Corpus is divided in 3 splits: train, validation and test. To load a specific split pass its name as a config name:\n\nTo load an specific split (for example, the validation split) do:",
"### Supported Tasks\nautomatic-speech-recognition: The dataset can be used to train a model for Automatic Speech Recognition (ASR). The model is presented with an audio file and asked to transcribe the audio file to written text. The most common evaluation metric is the word error rate (WER).",
"### Languages\nThe audio is in Faroese.\nThe reading prompts for the RAVNURSSON Corpus have been generated by expert linguists. The whole corpus was balanced for phonetic and dialectal coverage; Test and Dev subsets are gender-balanced. Tabular computer-searchable information is included as well as written documentation.",
"## Dataset Structure",
"### Data Instances",
"### Data Fields\n* 'audio_id' (string) - id of audio segment\n* 'audio' (datasets.Audio) - a dictionary containing the path to the audio, the decoded audio array, and the sampling rate. In non-streaming mode (default), the path points to the locally extracted audio. In streaming mode, the path is the relative path of an audio inside its archive (as files are not downloaded and extracted locally).\n* 'speaker_id' (string) - id of speaker\n* 'gender' (string) - gender of speaker (male or female)\n* 'age' (string) - range of age of the speaker: Younger (15-35), Middle-aged (36-60) or Elderly (61+).\n* 'duration' (float32) - duration of the audio file in seconds.\n* 'normalized_text' (string) - normalized audio segment transcription\n* 'dialect' (string) - dialect group, for example \"Suðuroy\" or \"Sandoy\".",
"### Data Splits\nThe speech material has been subdivided into portions for training (train), development (evaluation) and testing (test). Lengths of each portion are: train = 100h08m, test = 4h30m, dev (evaluation)=4h30m.\n\nTo load an specific portion please see the above section \"Example Usage\".\n\nThe development and test portions have exactly 10 male and 10 female speakers each and both portions have exactly the same size in hours (4.5h each).",
"## Dataset Creation",
"### Curation Rationale\n\nThe directory called \"speech\" contains all the speech files of the corpus. The files in the speech directory are divided in three directories: train, dev and test. The train portion is sub-divided in three types of recordings: RDATA1O, RDATA1OP and RDATA2; this is due to the organization of the recordings in the original BLARK 1.0. There, the recordings are divided in Rdata1 and Rdata2.\n\nOne main difference between Rdata1 and Rdata2 is that the reading environment for Rdata2 was controlled by a software called \"PushPrompt\" which is included in the original BLARK 1.0. Another main difference is that in Rdata1 there are some available transcriptions labeled at the phoneme level. For this reason the audio files in the speech directory of the RAVNURSSON corpus are divided in the folders RDATA1O where \"O\" is for \"Orthographic\" and RDATA1OP where \"O\" is for Orthographic and \"P\" is for phonetic.\n\nIn the case of the dev and test portions, the data come only from Rdata2 which does not have labels at the phonetic level.\n\nIt is important to clarify that the RAVNURSSON Corpus only includes transcriptions at the orthographic level.",
"### Source Data",
"#### Initial Data Collection and Normalization\nThe dataset was released with normalized text only at an orthographic level in lower-case. The normalization process was performed by automatically removing punctuation marks and characters that are not present in the Faroese alphabet.",
"#### Who are the source language producers?\n\n* The utterances were recorded using a TASCAM DR-40.\n\n* Participants self-reported their age group, gender, native language and dialect.\n\n* Participants are aged between 15 to 83 years. \n \n* The corpus contains 71949 speech files from 433 speakers, totalling 109 hours and 9 minutes.",
"### Annotations",
"#### Annotation process\n\nMost of the reading prompts were selected by experts from a Faroese text corpus (news, blogs, Wikipedia etc.) and were edited to fit the format. Reading prompts that are within specific domains (such as Faroese place names, numbers, license plates, telling time etc.) were written by the Ravnur Project. Then, a software tool called PushPrompt were used for reading sessions (voice recordings). PushPromt presents the text items in the reading material to the reader, allowing him/her to manage the session interactively (adjusting the reading tempo, repeating speech productions at wish, inserting short breaks as needed, etc.). When the reading session is completed, a log file (with time stamps for each production) is written as a data table compliant with the TextGrid-format.",
"#### Who are the annotators?\nThe corpus was annotated by the Ravnur Project",
"### Personal and Sensitive Information\nThe dataset consists of people who have donated their voice. You agree to not attempt to determine the identity of speakers in this dataset.",
"## Considerations for Using the Data",
"### Social Impact of Dataset\nThis is the first ASR corpus in Faroese.",
"### Discussion of Biases\nAs the number of reading prompts was limited, the common denominator in the RAVNURSSON corpus is that one prompt is read by more than one speaker. This is relevant because is a common practice in ASR to create a language model using the prompts that are found in the train portion of the corpus. That is not recommended for the RAVNURSSON Corpus as it counts with many prompts shared by all the portions and that will produce an important bias in the language modeling task.\n\nIn this section we present some statistics about the repeated prompts through all the portions of the corpus.\n\n- In the train portion:\n\t* Total number of prompts = 65616\n\t* Number of unique prompts = 38646\nThere are 26970 repeated prompts in the train portion. In other words, 41.1% of the prompts are repeated.\n\n- In the test portion:\n\t* Total number of prompts = 3002\n\t* Number of unique prompts = 2887\nThere are 115 repeated prompts in the test portion. In other words, 3.83% of the prompts are repeated.\n\n- In the dev portion:\n\t* Total number of prompts = 3331\n\t* Number of unique prompts = 3302\nThere are 29 repeated prompts in the dev portion. In other words, 0.87% of the prompts are repeated.\n\n- Considering the corpus as a whole:\n\t* Total number of prompts = 71949\n\t* Number of unique prompts = 39945\nThere are 32004 repeated prompts in the whole corpus. In other words, 44.48% of the prompts are repeated.\n\nNOTICE!: It is also important to clarify that none of the 3 portions of the corpus share speakers.",
"### Other Known Limitations\n\"RAVNURSSON FAROESE SPEECH AND TRANSCRIPTS\" by Carlos Daniel Hernández Mena and Annika Simonsen is licensed under a Creative Commons Attribution 4.0 International (CC BY 4.0) License with the hope that it will be useful, but WITHOUT ANY WARRANTY; without even the implied warranty of MERCHANTABILITY or FITNESS FOR A PARTICULAR PURPOSE.",
"## Additional Information",
"### Dataset Curators\nThe dataset was collected by Annika Simonsen and curated by Carlos Daniel Hernández Mena.",
"### Licensing Information\nCC-BY-4.0",
"### Contributions\nThis project was made possible under the umbrella of the Language Technology Programme for Icelandic 2019-2023. The programme, which is managed and coordinated by Almannarómur, is funded by the Icelandic Ministry of Education, Science and Culture.\n\nSpecial thanks to Dr. Jón Guðnason, professor at Reykjavík University and head of the Language and Voice Lab (LVL) for providing computational resources."
] | [
"TAGS\n#task_categories-automatic-speech-recognition #annotations_creators-expert-generated #language_creators-expert-generated #multilinguality-monolingual #size_categories-10K<n<100K #source_datasets-original #language-Faroese #license-cc-by-4.0 #faroe islands #faroese #ravnur project #speech recognition in faroese #region-us \n",
"# Dataset Card for ravnursson_asr",
"## Table of Contents\n- Dataset Description\n - Dataset Summary\n - Supported Tasks\n - Languages\n- Dataset Structure\n - Data Instances\n - Data Fields\n - Data Splits\n- Dataset Creation\n - Curation Rationale\n - Source Data\n - Annotations\n - Personal and Sensitive Information\n- Considerations for Using the Data\n - Social Impact of Dataset\n - Discussion of Biases\n - Other Known Limitations\n- Additional Information\n - Dataset Curators\n - Licensing Information\n - Citation Information\n - Contributions",
"## Dataset Description\n- Homepage: Ravnursson Faroese Speech and Transcripts\n- Repository: URL\n- Paper: ASR Language Resources for Faroese\n- Paper: Creating a basic language resource kit for faroese.\n- Point of Contact: Annika Simonsen, Carlos Mena",
"### Dataset Summary\nThe corpus \"RAVNURSSON FAROESE SPEECH AND TRANSCRIPTS\" (or RAVNURSSON Corpus for short) is a collection of speech recordings with transcriptions intended for Automatic Speech Recognition (ASR) applications in the language that is spoken at the Faroe Islands (Faroese). It was curated at the Reykjavík University (RU) in 2022.\n\nThe RAVNURSSON Corpus is an extract of the \"Basic Language Resource Kit 1.0\" (BLARK 1.0) [1] developed by the Ravnur Project from the Faroe Islands [2]. As a matter of fact, the name RAVNURSSON comes from Ravnur (a tribute to the Ravnur Project) and the suffix \"son\" which in Icelandic means \"son of\". Therefore, the name \"RAVNURSSON\" means \"The (Icelandic) son of Ravnur\". The double \"ss\" is just for aesthetics.\n\nThe audio was collected by recording speakers reading texts. The participants are aged 15-83, divided into 3 age groups: 15-35, 36-60 and 61+.\n\nThe speech files are made of 249 female speakers and 184 male speakers; 433 speakers total. The recordings were made on TASCAM DR-40 Linear PCM audio recorders using the built-in stereo microphones in WAVE 16 bit with a sample rate of 48kHz, but then, downsampled to 16kHz@16bit mono for this corpus.\n\n[1] Simonsen, A., Debess, I. N., Lamhauge, S. S., & Henrichsen, P. J. Creating a basic language resource kit for Faroese. In LREC 2022. 13th International Conference on Language Resources and Evaluation.\n \n[2] Website. The Project Ravnur under the Talutøkni Foundation URL",
"### Example Usage\nThe RAVNURSSON Corpus is divided in 3 splits: train, validation and test. To load a specific split pass its name as a config name:\n\nTo load an specific split (for example, the validation split) do:",
"### Supported Tasks\nautomatic-speech-recognition: The dataset can be used to train a model for Automatic Speech Recognition (ASR). The model is presented with an audio file and asked to transcribe the audio file to written text. The most common evaluation metric is the word error rate (WER).",
"### Languages\nThe audio is in Faroese.\nThe reading prompts for the RAVNURSSON Corpus have been generated by expert linguists. The whole corpus was balanced for phonetic and dialectal coverage; Test and Dev subsets are gender-balanced. Tabular computer-searchable information is included as well as written documentation.",
"## Dataset Structure",
"### Data Instances",
"### Data Fields\n* 'audio_id' (string) - id of audio segment\n* 'audio' (datasets.Audio) - a dictionary containing the path to the audio, the decoded audio array, and the sampling rate. In non-streaming mode (default), the path points to the locally extracted audio. In streaming mode, the path is the relative path of an audio inside its archive (as files are not downloaded and extracted locally).\n* 'speaker_id' (string) - id of speaker\n* 'gender' (string) - gender of speaker (male or female)\n* 'age' (string) - range of age of the speaker: Younger (15-35), Middle-aged (36-60) or Elderly (61+).\n* 'duration' (float32) - duration of the audio file in seconds.\n* 'normalized_text' (string) - normalized audio segment transcription\n* 'dialect' (string) - dialect group, for example \"Suðuroy\" or \"Sandoy\".",
"### Data Splits\nThe speech material has been subdivided into portions for training (train), development (evaluation) and testing (test). Lengths of each portion are: train = 100h08m, test = 4h30m, dev (evaluation)=4h30m.\n\nTo load an specific portion please see the above section \"Example Usage\".\n\nThe development and test portions have exactly 10 male and 10 female speakers each and both portions have exactly the same size in hours (4.5h each).",
"## Dataset Creation",
"### Curation Rationale\n\nThe directory called \"speech\" contains all the speech files of the corpus. The files in the speech directory are divided in three directories: train, dev and test. The train portion is sub-divided in three types of recordings: RDATA1O, RDATA1OP and RDATA2; this is due to the organization of the recordings in the original BLARK 1.0. There, the recordings are divided in Rdata1 and Rdata2.\n\nOne main difference between Rdata1 and Rdata2 is that the reading environment for Rdata2 was controlled by a software called \"PushPrompt\" which is included in the original BLARK 1.0. Another main difference is that in Rdata1 there are some available transcriptions labeled at the phoneme level. For this reason the audio files in the speech directory of the RAVNURSSON corpus are divided in the folders RDATA1O where \"O\" is for \"Orthographic\" and RDATA1OP where \"O\" is for Orthographic and \"P\" is for phonetic.\n\nIn the case of the dev and test portions, the data come only from Rdata2 which does not have labels at the phonetic level.\n\nIt is important to clarify that the RAVNURSSON Corpus only includes transcriptions at the orthographic level.",
"### Source Data",
"#### Initial Data Collection and Normalization\nThe dataset was released with normalized text only at an orthographic level in lower-case. The normalization process was performed by automatically removing punctuation marks and characters that are not present in the Faroese alphabet.",
"#### Who are the source language producers?\n\n* The utterances were recorded using a TASCAM DR-40.\n\n* Participants self-reported their age group, gender, native language and dialect.\n\n* Participants are aged between 15 to 83 years. \n \n* The corpus contains 71949 speech files from 433 speakers, totalling 109 hours and 9 minutes.",
"### Annotations",
"#### Annotation process\n\nMost of the reading prompts were selected by experts from a Faroese text corpus (news, blogs, Wikipedia etc.) and were edited to fit the format. Reading prompts that are within specific domains (such as Faroese place names, numbers, license plates, telling time etc.) were written by the Ravnur Project. Then, a software tool called PushPrompt were used for reading sessions (voice recordings). PushPromt presents the text items in the reading material to the reader, allowing him/her to manage the session interactively (adjusting the reading tempo, repeating speech productions at wish, inserting short breaks as needed, etc.). When the reading session is completed, a log file (with time stamps for each production) is written as a data table compliant with the TextGrid-format.",
"#### Who are the annotators?\nThe corpus was annotated by the Ravnur Project",
"### Personal and Sensitive Information\nThe dataset consists of people who have donated their voice. You agree to not attempt to determine the identity of speakers in this dataset.",
"## Considerations for Using the Data",
"### Social Impact of Dataset\nThis is the first ASR corpus in Faroese.",
"### Discussion of Biases\nAs the number of reading prompts was limited, the common denominator in the RAVNURSSON corpus is that one prompt is read by more than one speaker. This is relevant because is a common practice in ASR to create a language model using the prompts that are found in the train portion of the corpus. That is not recommended for the RAVNURSSON Corpus as it counts with many prompts shared by all the portions and that will produce an important bias in the language modeling task.\n\nIn this section we present some statistics about the repeated prompts through all the portions of the corpus.\n\n- In the train portion:\n\t* Total number of prompts = 65616\n\t* Number of unique prompts = 38646\nThere are 26970 repeated prompts in the train portion. In other words, 41.1% of the prompts are repeated.\n\n- In the test portion:\n\t* Total number of prompts = 3002\n\t* Number of unique prompts = 2887\nThere are 115 repeated prompts in the test portion. In other words, 3.83% of the prompts are repeated.\n\n- In the dev portion:\n\t* Total number of prompts = 3331\n\t* Number of unique prompts = 3302\nThere are 29 repeated prompts in the dev portion. In other words, 0.87% of the prompts are repeated.\n\n- Considering the corpus as a whole:\n\t* Total number of prompts = 71949\n\t* Number of unique prompts = 39945\nThere are 32004 repeated prompts in the whole corpus. In other words, 44.48% of the prompts are repeated.\n\nNOTICE!: It is also important to clarify that none of the 3 portions of the corpus share speakers.",
"### Other Known Limitations\n\"RAVNURSSON FAROESE SPEECH AND TRANSCRIPTS\" by Carlos Daniel Hernández Mena and Annika Simonsen is licensed under a Creative Commons Attribution 4.0 International (CC BY 4.0) License with the hope that it will be useful, but WITHOUT ANY WARRANTY; without even the implied warranty of MERCHANTABILITY or FITNESS FOR A PARTICULAR PURPOSE.",
"## Additional Information",
"### Dataset Curators\nThe dataset was collected by Annika Simonsen and curated by Carlos Daniel Hernández Mena.",
"### Licensing Information\nCC-BY-4.0",
"### Contributions\nThis project was made possible under the umbrella of the Language Technology Programme for Icelandic 2019-2023. The programme, which is managed and coordinated by Almannarómur, is funded by the Icelandic Ministry of Education, Science and Culture.\n\nSpecial thanks to Dr. Jón Guðnason, professor at Reykjavík University and head of the Language and Voice Lab (LVL) for providing computational resources."
] |
b908bad5ef0759d2c03baf09715a98aedda9ded1 |
# Dataset Card for kodak
## Table of Contents
- [Table of Contents](#table-of-contents)
- [Dataset Description](#dataset-description)
- [Dataset Summary](#dataset-summary)
- [Supported Tasks and Leaderboards](#supported-tasks-and-leaderboards)
- [Languages](#languages)
- [Dataset Structure](#dataset-structure)
- [Data Instances](#data-instances)
- [Data Fields](#data-fields)
- [Data Splits](#data-splits)
- [Dataset Creation](#dataset-creation)
- [Curation Rationale](#curation-rationale)
- [Source Data](#source-data)
- [Annotations](#annotations)
- [Personal and Sensitive Information](#personal-and-sensitive-information)
- [Considerations for Using the Data](#considerations-for-using-the-data)
- [Social Impact of Dataset](#social-impact-of-dataset)
- [Discussion of Biases](#discussion-of-biases)
- [Other Known Limitations](#other-known-limitations)
- [Additional Information](#additional-information)
- [Dataset Curators](#dataset-curators)
- [Licensing Information](#licensing-information)
- [Citation Information](#citation-information)
- [Contributions](#contributions)
## Dataset Description
- **Homepage:** <https://r0k.us/graphics/kodak/>
- **Repository:** <https://github.com/MohamedBakrAli/Kodak-Lossless-True-Color-Image-Suite>
- **Paper:**
- **Leaderboard:**
- **Point of Contact:**
### Dataset Summary
The pictures below link to lossless, true color (24 bits per pixel, aka "full
color") images. It is my understanding they have been released by the Eastman
Kodak Company for unrestricted usage. Many sites use them as a standard test
suite for compression testing, etc. Prior to this site, they were only
available in the Sun Raster format via ftp. This meant that the images could
not be previewed before downloading. Since their release, however, the lossless
PNG format has been incorporated into all the major browsers. Since PNG
supports 24-bit lossless color (which GIF and JPEG do not), it is now possible
to offer this browser-friendly access to the images.
### Supported Tasks and Leaderboards
- Image compression
### Languages
- en
## Dataset Structure
### Data Instances
- [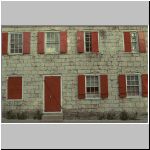](https://r0k.us/graphics/kodak/kodak/kodim01.png)
- [](https://r0k.us/graphics/kodak/kodak/kodim02.png)
- [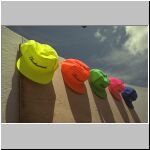](https://r0k.us/graphics/kodak/kodak/kodim03.png)
- [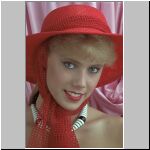](https://r0k.us/graphics/kodak/kodak/kodim04.png)
- [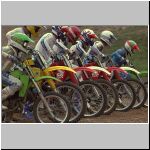](https://r0k.us/graphics/kodak/kodak/kodim05.png)
- [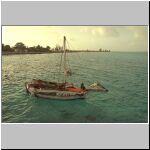](https://r0k.us/graphics/kodak/kodak/kodim06.png)
- [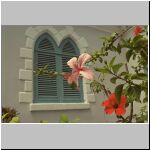](https://r0k.us/graphics/kodak/kodak/kodim07.png)
- [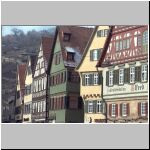](https://r0k.us/graphics/kodak/kodak/kodim08.png)
- [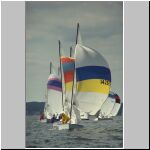](https://r0k.us/graphics/kodak/kodak/kodim09.png)
- [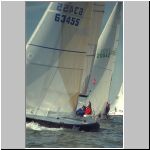](https://r0k.us/graphics/kodak/kodak/kodim10.png)
- [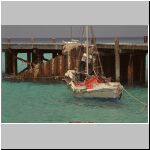](https://r0k.us/graphics/kodak/kodak/kodim11.png)
- [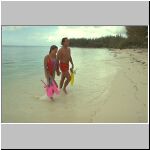](https://r0k.us/graphics/kodak/kodak/kodim12.png)
- [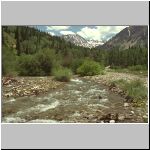](https://r0k.us/graphics/kodak/kodak/kodim13.png)
- [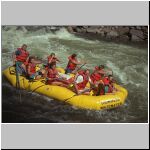](https://r0k.us/graphics/kodak/kodak/kodim14.png)
- [](https://r0k.us/graphics/kodak/kodak/kodim15.png)
- [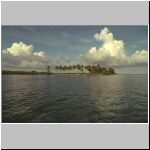](https://r0k.us/graphics/kodak/kodak/kodim16.png)
- [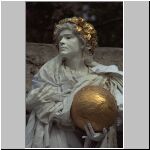](https://r0k.us/graphics/kodak/kodak/kodim17.png)
- [](https://r0k.us/graphics/kodak/kodak/kodim18.png)
- [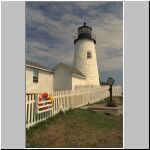](https://r0k.us/graphics/kodak/kodak/kodim19.png)
- [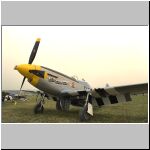](https://r0k.us/graphics/kodak/kodak/kodim20.png)
- [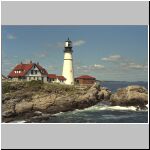](https://r0k.us/graphics/kodak/kodak/kodim21.png)
- [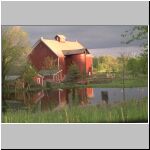](https://r0k.us/graphics/kodak/kodak/kodim22.png)
- [](https://r0k.us/graphics/kodak/kodak/kodim23.png)
- [](https://r0k.us/graphics/kodak/kodak/kodim24.png)
### Data Fields
### Data Splits
## Dataset Creation
### Curation Rationale
### Source Data
#### Initial Data Collection and Normalization
#### Who are the source language producers?
<https://www.kodak.com>
### Annotations
#### Annotation process
#### Who are the annotators?
### Personal and Sensitive Information
## Considerations for Using the Data
### Social Impact of Dataset
### Discussion of Biases
### Other Known Limitations
## Additional Information
### Dataset Curators
### Licensing Information
[LICENSE](LICENSE)
### Citation Information
### Contributions
Thanks to [@Freed-Wu](https://github.com/Freed-Wu) for adding this dataset.
| Freed-Wu/kodak | [
"task_categories:other",
"annotations_creators:no-annotation",
"language_creators:found",
"multilinguality:monolingual",
"size_categories:n<1K",
"source_datasets:original",
"language:en",
"license:gpl-3.0",
"region:us"
] | 2022-11-19T05:43:53+00:00 | {"annotations_creators": ["no-annotation"], "language_creators": ["found"], "language": ["en"], "license": ["gpl-3.0"], "multilinguality": ["monolingual"], "size_categories": ["n<1K"], "source_datasets": ["original"], "task_categories": ["other"], "task_ids": [], "pretty_name": "kodak", "tags": [], "dataset_info": {"features": [{"name": "image", "dtype": "image"}], "splits": [{"name": "test", "num_bytes": 15072, "num_examples": 24}], "download_size": 15072, "dataset_size": 15072}} | 2022-11-19T05:43:53+00:00 | [] | [
"en"
] | TAGS
#task_categories-other #annotations_creators-no-annotation #language_creators-found #multilinguality-monolingual #size_categories-n<1K #source_datasets-original #language-English #license-gpl-3.0 #region-us
|
# Dataset Card for kodak
## Table of Contents
- Table of Contents
- Dataset Description
- Dataset Summary
- Supported Tasks and Leaderboards
- Languages
- Dataset Structure
- Data Instances
- Data Fields
- Data Splits
- Dataset Creation
- Curation Rationale
- Source Data
- Annotations
- Personal and Sensitive Information
- Considerations for Using the Data
- Social Impact of Dataset
- Discussion of Biases
- Other Known Limitations
- Additional Information
- Dataset Curators
- Licensing Information
- Citation Information
- Contributions
## Dataset Description
- Homepage: <URL
- Repository: <URL
- Paper:
- Leaderboard:
- Point of Contact:
### Dataset Summary
The pictures below link to lossless, true color (24 bits per pixel, aka "full
color") images. It is my understanding they have been released by the Eastman
Kodak Company for unrestricted usage. Many sites use them as a standard test
suite for compression testing, etc. Prior to this site, they were only
available in the Sun Raster format via ftp. This meant that the images could
not be previewed before downloading. Since their release, however, the lossless
PNG format has been incorporated into all the major browsers. Since PNG
supports 24-bit lossless color (which GIF and JPEG do not), it is now possible
to offer this browser-friendly access to the images.
### Supported Tasks and Leaderboards
- Image compression
### Languages
- en
## Dataset Structure
### Data Instances
-  images. It is my understanding they have been released by the Eastman\nKodak Company for unrestricted usage. Many sites use them as a standard test\nsuite for compression testing, etc. Prior to this site, they were only\navailable in the Sun Raster format via ftp. This meant that the images could\nnot be previewed before downloading. Since their release, however, the lossless\nPNG format has been incorporated into all the major browsers. Since PNG\nsupports 24-bit lossless color (which GIF and JPEG do not), it is now possible\nto offer this browser-friendly access to the images.",
"### Supported Tasks and Leaderboards\n\n- Image compression",
"### Languages\n\n- en",
"## Dataset Structure",
"### Data Instances\n\n-  images. It is my understanding they have been released by the Eastman\nKodak Company for unrestricted usage. Many sites use them as a standard test\nsuite for compression testing, etc. Prior to this site, they were only\navailable in the Sun Raster format via ftp. This meant that the images could\nnot be previewed before downloading. Since their release, however, the lossless\nPNG format has been incorporated into all the major browsers. Since PNG\nsupports 24-bit lossless color (which GIF and JPEG do not), it is now possible\nto offer this browser-friendly access to the images.",
"### Supported Tasks and Leaderboards\n\n- Image compression",
"### Languages\n\n- en",
"## Dataset Structure",
"### Data Instances\n\n-  | chenrm/koikatsu-cards | [
"region:us"
] | 2022-11-19T08:54:34+00:00 | {"dataset_info": {"features": [{"name": "image", "dtype": "image"}], "splits": [{"name": "train", "num_bytes": 43368873054.078, "num_examples": 10178}, {"name": "test", "num_bytes": 20733059.0, "num_examples": 5}], "download_size": 56731523062, "dataset_size": 43389606113.078}} | 2022-11-19T10:33:28+00:00 | [] | [] | TAGS
#region-us
| # Dataset Card for "koikatsu-cards"
More Information needed | [
"# Dataset Card for \"koikatsu-cards\"\n\nMore Information needed"
] | [
"TAGS\n#region-us \n",
"# Dataset Card for \"koikatsu-cards\"\n\nMore Information needed"
] |
4eaa49ac06038400da1437d5cd98686ac3712ab0 | # Dataset Card for "del"
[More Information needed](https://github.com/huggingface/datasets/blob/main/CONTRIBUTING.md#how-to-contribute-to-the-dataset-cards) | galman33/del | [
"region:us"
] | 2022-11-19T12:26:10+00:00 | {"dataset_info": {"features": [{"name": "lat", "dtype": "float64"}, {"name": "lon", "dtype": "float64"}, {"name": "country_code", "dtype": "string"}, {"name": "pixels", "dtype": {"array3_d": {"shape": [256, 256, 3], "dtype": "uint8"}}}], "splits": [{"name": "train", "num_bytes": 3816359256, "num_examples": 8300}], "download_size": 1455177025, "dataset_size": 3816359256}} | 2022-11-19T12:45:41+00:00 | [] | [] | TAGS
#region-us
| # Dataset Card for "del"
More Information needed | [
"# Dataset Card for \"del\"\n\nMore Information needed"
] | [
"TAGS\n#region-us \n",
"# Dataset Card for \"del\"\n\nMore Information needed"
] |
4f15697f40bdb5be4c583942d825e48627d4bef5 | # Dataset Card for "gal_yair_83000_256x256"
[More Information needed](https://github.com/huggingface/datasets/blob/main/CONTRIBUTING.md#how-to-contribute-to-the-dataset-cards) | galman33/gal_yair_83000_256x256 | [
"region:us"
] | 2022-11-19T12:29:05+00:00 | {"dataset_info": {"features": [{"name": "lat", "dtype": "float64"}, {"name": "lon", "dtype": "float64"}, {"name": "country_code", "dtype": "string"}, {"name": "image", "dtype": "image"}], "splits": [{"name": "train", "num_bytes": 8075570913.0, "num_examples": 83000}], "download_size": 8075813262, "dataset_size": 8075570913.0}} | 2022-11-19T12:33:25+00:00 | [] | [] | TAGS
#region-us
| # Dataset Card for "gal_yair_83000_256x256"
More Information needed | [
"# Dataset Card for \"gal_yair_83000_256x256\"\n\nMore Information needed"
] | [
"TAGS\n#region-us \n",
"# Dataset Card for \"gal_yair_83000_256x256\"\n\nMore Information needed"
] |
24aea2a13efaddb810cdff6ae2b133ccb565d025 | Hugging Face's logo
Hugging Face
Search models, datasets, users...
Models
Datasets
Spaces
Docs
Solutions
Pricing
Hugging Face is way more fun with friends and colleagues! 🤗 Join an organization
Datasets:
Mostafa3zazi
/
Arabic_SQuAD Copied
like
0
Dataset card
Files and versions
Community
Arabic_SQuAD
/
README.md
Mostafa3zazi's picture
Mostafa3zazi
Update README.md
17d5b9d
19 days ago
raw
history
blame
contribute
delete
Safe
2.18 kB
---
dataset_info:
features:
- name: index
dtype: string
- name: question
dtype: string
- name: context
dtype: string
- name: text
dtype: string
- name: answer_start
dtype: int64
- name: c_id
dtype: int64
splits:
- name: train
num_bytes: 61868003
num_examples: 48344
download_size: 10512179
dataset_size: 61868003
---
# Dataset Card for "Arabic_SQuAD"
[More Information needed](https://github.com/huggingface/datasets/blob/main/CONTRIBUTING.md#how-to-contribute-to-the-dataset-cards)
---
# Citation
```
@inproceedings{mozannar-etal-2019-neural,
title = "Neural {A}rabic Question Answering",
author = "Mozannar, Hussein and
Maamary, Elie and
El Hajal, Karl and
Hajj, Hazem",
booktitle = "Proceedings of the Fourth Arabic Natural Language Processing Workshop",
month = aug,
year = "2019",
address = "Florence, Italy",
publisher = "Association for Computational Linguistics",
url = "https://www.aclweb.org/anthology/W19-4612",
doi = "10.18653/v1/W19-4612",
pages = "108--118",
abstract = "This paper tackles the problem of open domain factual Arabic question answering (QA) using Wikipedia as our knowledge source. This constrains the answer of any question to be a span of text in Wikipedia. Open domain QA for Arabic entails three challenges: annotated QA datasets in Arabic, large scale efficient information retrieval and machine reading comprehension. To deal with the lack of Arabic QA datasets we present the Arabic Reading Comprehension Dataset (ARCD) composed of 1,395 questions posed by crowdworkers on Wikipedia articles, and a machine translation of the Stanford Question Answering Dataset (Arabic-SQuAD). Our system for open domain question answering in Arabic (SOQAL) is based on two components: (1) a document retriever using a hierarchical TF-IDF approach and (2) a neural reading comprehension model using the pre-trained bi-directional transformer BERT. Our experiments on ARCD indicate the effectiveness of our approach with our BERT-based reader achieving a 61.3 F1 score, and our open domain system SOQAL achieving a 27.6 F1 score.",
}
```
---
| ZIZOU/Arabic_Squad | [
"region:us"
] | 2022-11-19T12:58:41+00:00 | {} | 2022-11-26T09:36:45+00:00 | [] | [] | TAGS
#region-us
| Hugging Face's logo
Hugging Face
Search models, datasets, users...
Models
Datasets
Spaces
Docs
Solutions
Pricing
Hugging Face is way more fun with friends and colleagues! Join an organization
Datasets:
Mostafa3zazi
/
Arabic_SQuAD Copied
like
0
Dataset card
Files and versions
Community
Arabic_SQuAD
/
URL
Mostafa3zazi's picture
Mostafa3zazi
Update URL
17d5b9d
19 days ago
raw
history
blame
contribute
delete
Safe
2.18 kB
---
dataset_info:
features:
- name: index
dtype: string
- name: question
dtype: string
- name: context
dtype: string
- name: text
dtype: string
- name: answer_start
dtype: int64
- name: c_id
dtype: int64
splits:
- name: train
num_bytes: 61868003
num_examples: 48344
download_size: 10512179
dataset_size: 61868003
---
# Dataset Card for "Arabic_SQuAD"
More Information needed
---
---
| [
"# Dataset Card for \"Arabic_SQuAD\"\nMore Information needed\n\n---\n---"
] | [
"TAGS\n#region-us \n",
"# Dataset Card for \"Arabic_SQuAD\"\nMore Information needed\n\n---\n---"
] |
e9805e363e41f46225021bc9f3da6d0b0483b8e1 | This dataset is for use in Automatic Speech Recognition (ASR) for a project at University of Zambia(UNZA) | unza/unza-nyanja | [
"region:us"
] | 2022-11-19T14:18:39+00:00 | {} | 2022-11-19T17:42:38+00:00 | [] | [] | TAGS
#region-us
| This dataset is for use in Automatic Speech Recognition (ASR) for a project at University of Zambia(UNZA) | [] | [
"TAGS\n#region-us \n"
] |
d7c094f2a6ae22d41a3c143b42033e61c6ecfd72 | # Dataset Card for "L1_poleval_korpus_pelny_train"
[More Information needed](https://github.com/huggingface/datasets/blob/main/CONTRIBUTING.md#how-to-contribute-to-the-dataset-cards) | Nikutka/L1_poleval_korpus_pelny_train | [
"region:us"
] | 2022-11-19T14:43:15+00:00 | {"dataset_info": {"features": [{"name": "content", "dtype": "string"}, {"name": "label", "dtype": "int64"}], "splits": [{"name": "train", "num_bytes": 764265, "num_examples": 9443}], "download_size": 509113, "dataset_size": 764265}} | 2022-11-19T18:25:13+00:00 | [] | [] | TAGS
#region-us
| # Dataset Card for "L1_poleval_korpus_pelny_train"
More Information needed | [
"# Dataset Card for \"L1_poleval_korpus_pelny_train\"\n\nMore Information needed"
] | [
"TAGS\n#region-us \n",
"# Dataset Card for \"L1_poleval_korpus_pelny_train\"\n\nMore Information needed"
] |
56bfdb5641038be046b772f3378e9b45b06a6bb2 | # Dataset Card for "L1_poleval_korpus_pelny_test"
[More Information needed](https://github.com/huggingface/datasets/blob/main/CONTRIBUTING.md#how-to-contribute-to-the-dataset-cards) | Nikutka/L1_poleval_korpus_pelny_test | [
"region:us"
] | 2022-11-19T14:43:36+00:00 | {"dataset_info": {"features": [{"name": "content", "dtype": "string"}, {"name": "label", "dtype": "int64"}], "splits": [{"name": "train", "num_bytes": 71297, "num_examples": 891}], "download_size": 47500, "dataset_size": 71297}} | 2022-11-19T18:25:17+00:00 | [] | [] | TAGS
#region-us
| # Dataset Card for "L1_poleval_korpus_pelny_test"
More Information needed | [
"# Dataset Card for \"L1_poleval_korpus_pelny_test\"\n\nMore Information needed"
] | [
"TAGS\n#region-us \n",
"# Dataset Card for \"L1_poleval_korpus_pelny_test\"\n\nMore Information needed"
] |
c4d9f2faf033cc7f4f86b65814ae21df6e2ba768 | # Dataset Card for "L1_poleval_korpus_wzorcowy_train"
[More Information needed](https://github.com/huggingface/datasets/blob/main/CONTRIBUTING.md#how-to-contribute-to-the-dataset-cards) | Nikutka/L1_poleval_korpus_wzorcowy_train | [
"region:us"
] | 2022-11-19T14:55:23+00:00 | {"dataset_info": {"features": [{"name": "content", "dtype": "string"}, {"name": "label", "dtype": "int64"}], "splits": [{"name": "train", "num_bytes": 20564, "num_examples": 253}], "download_size": 15381, "dataset_size": 20564}} | 2022-11-19T18:25:28+00:00 | [] | [] | TAGS
#region-us
| # Dataset Card for "L1_poleval_korpus_wzorcowy_train"
More Information needed | [
"# Dataset Card for \"L1_poleval_korpus_wzorcowy_train\"\n\nMore Information needed"
] | [
"TAGS\n#region-us \n",
"# Dataset Card for \"L1_poleval_korpus_wzorcowy_train\"\n\nMore Information needed"
] |
85e49f1e1bc10c9cacc44a24d17112d42fb09ccb | # Dataset Card for "L1_poleval_korpus_wzorcowy_test"
[More Information needed](https://github.com/huggingface/datasets/blob/main/CONTRIBUTING.md#how-to-contribute-to-the-dataset-cards) | Nikutka/L1_poleval_korpus_wzorcowy_test | [
"region:us"
] | 2022-11-19T14:55:27+00:00 | {"dataset_info": {"features": [{"name": "content", "dtype": "string"}, {"name": "label", "dtype": "int64"}], "splits": [{"name": "train", "num_bytes": 1963, "num_examples": 25}], "download_size": 2784, "dataset_size": 1963}} | 2022-11-19T18:25:32+00:00 | [] | [] | TAGS
#region-us
| # Dataset Card for "L1_poleval_korpus_wzorcowy_test"
More Information needed | [
"# Dataset Card for \"L1_poleval_korpus_wzorcowy_test\"\n\nMore Information needed"
] | [
"TAGS\n#region-us \n",
"# Dataset Card for \"L1_poleval_korpus_wzorcowy_test\"\n\nMore Information needed"
] |
3a031ae539b268125dad64f3ac9d559bd9ea9e22 | # Dataset Card for "gal_yair_83000_100x100"
[More Information needed](https://github.com/huggingface/datasets/blob/main/CONTRIBUTING.md#how-to-contribute-to-the-dataset-cards) | galman33/gal_yair_83000_100x100 | [
"region:us"
] | 2022-11-19T14:56:55+00:00 | {"dataset_info": {"features": [{"name": "lat", "dtype": "float64"}, {"name": "lon", "dtype": "float64"}, {"name": "country_code", "dtype": "string"}, {"name": "image", "dtype": "image"}], "splits": [{"name": "train", "num_bytes": 1423239502.0, "num_examples": 83000}], "download_size": 1423108777, "dataset_size": 1423239502.0}} | 2022-11-19T14:57:47+00:00 | [] | [] | TAGS
#region-us
| # Dataset Card for "gal_yair_83000_100x100"
More Information needed | [
"# Dataset Card for \"gal_yair_83000_100x100\"\n\nMore Information needed"
] | [
"TAGS\n#region-us \n",
"# Dataset Card for \"gal_yair_83000_100x100\"\n\nMore Information needed"
] |
e8aa28670485bbe09d7cc3ce8a47ea4186f01429 |
# Dataset Card for FIB
## Dataset Summary
The FIB benchmark consists of 3579 examples for evaluating the factual inconsistency of large language models. Each example consists of a document and a pair of summaries: a factually consistent one and a factually inconsistent one. It is based on documents and summaries from XSum and CNN/DM.
Since this dataset is intended to evaluate the factual inconsistency of large language models, there is only a test split.
Accuracies should be reported separately for examples from XSum and for examples from CNN/DM. This is because the behavior of models on XSum and CNN/DM are expected to be very different. The factually inconsistent summaries are model-extracted from the document for CNN/DM but are model-generated for XSum.
### Citation Information
```
@article{tam2022fib,
title={Evaluating the Factual Consistency of Large Language Models Through Summarization},
author={Tam, Derek and Mascarenhas, Anisha and Zhang, Shiyue and Kwan, Sarah and Bansal, Mohit and Raffel, Colin},
journal={arXiv preprint arXiv:2211.08412},
year={2022}
}
```
### Licensing Information
license: cc-by-4.0 | r-three/fib | [
"region:us"
] | 2022-11-19T15:22:00+00:00 | {} | 2022-11-19T15:57:58+00:00 | [] | [] | TAGS
#region-us
|
# Dataset Card for FIB
## Dataset Summary
The FIB benchmark consists of 3579 examples for evaluating the factual inconsistency of large language models. Each example consists of a document and a pair of summaries: a factually consistent one and a factually inconsistent one. It is based on documents and summaries from XSum and CNN/DM.
Since this dataset is intended to evaluate the factual inconsistency of large language models, there is only a test split.
Accuracies should be reported separately for examples from XSum and for examples from CNN/DM. This is because the behavior of models on XSum and CNN/DM are expected to be very different. The factually inconsistent summaries are model-extracted from the document for CNN/DM but are model-generated for XSum.
### Licensing Information
license: cc-by-4.0 | [
"# Dataset Card for FIB",
"## Dataset Summary\n\nThe FIB benchmark consists of 3579 examples for evaluating the factual inconsistency of large language models. Each example consists of a document and a pair of summaries: a factually consistent one and a factually inconsistent one. It is based on documents and summaries from XSum and CNN/DM.\nSince this dataset is intended to evaluate the factual inconsistency of large language models, there is only a test split. \n\nAccuracies should be reported separately for examples from XSum and for examples from CNN/DM. This is because the behavior of models on XSum and CNN/DM are expected to be very different. The factually inconsistent summaries are model-extracted from the document for CNN/DM but are model-generated for XSum.",
"### Licensing Information\n\nlicense: cc-by-4.0"
] | [
"TAGS\n#region-us \n",
"# Dataset Card for FIB",
"## Dataset Summary\n\nThe FIB benchmark consists of 3579 examples for evaluating the factual inconsistency of large language models. Each example consists of a document and a pair of summaries: a factually consistent one and a factually inconsistent one. It is based on documents and summaries from XSum and CNN/DM.\nSince this dataset is intended to evaluate the factual inconsistency of large language models, there is only a test split. \n\nAccuracies should be reported separately for examples from XSum and for examples from CNN/DM. This is because the behavior of models on XSum and CNN/DM are expected to be very different. The factually inconsistent summaries are model-extracted from the document for CNN/DM but are model-generated for XSum.",
"### Licensing Information\n\nlicense: cc-by-4.0"
] |
93d367c80cbd29aad9c9412a95b95ec782509b39 | два датасета: один с оригинальными нуждиками и один поменьше с пупами на основе нуждиков. оба в аудио и текстовом формате. | 4eJIoBek/nujdiki | [
"license:wtfpl",
"region:us"
] | 2022-11-19T16:23:30+00:00 | {"license": "wtfpl"} | 2023-02-13T15:35:25+00:00 | [] | [] | TAGS
#license-wtfpl #region-us
| два датасета: один с оригинальными нуждиками и один поменьше с пупами на основе нуждиков. оба в аудио и текстовом формате. | [] | [
"TAGS\n#license-wtfpl #region-us \n"
] |
51fbabf0496b056d0e018b99157a29261d6b9a93 | # Dataset Card for "L1_scraped_korpus_pelny_train"
[More Information needed](https://github.com/huggingface/datasets/blob/main/CONTRIBUTING.md#how-to-contribute-to-the-dataset-cards) | Nikutka/L1_scraped_korpus_pelny_train | [
"region:us"
] | 2022-11-19T16:38:58+00:00 | {"dataset_info": {"features": [{"name": "content", "dtype": "string"}, {"name": "label", "dtype": "int64"}], "splits": [{"name": "train", "num_bytes": 118294409, "num_examples": 1249536}], "download_size": 86623523, "dataset_size": 118294409}} | 2022-11-19T17:15:59+00:00 | [] | [] | TAGS
#region-us
| # Dataset Card for "L1_scraped_korpus_pelny_train"
More Information needed | [
"# Dataset Card for \"L1_scraped_korpus_pelny_train\"\n\nMore Information needed"
] | [
"TAGS\n#region-us \n",
"# Dataset Card for \"L1_scraped_korpus_pelny_train\"\n\nMore Information needed"
] |
67a3ee8544898d09a5aefd43e59d2461a8231a65 | # Dataset Card for "L1_scraped_korpus_pelny_test"
[More Information needed](https://github.com/huggingface/datasets/blob/main/CONTRIBUTING.md#how-to-contribute-to-the-dataset-cards) | Nikutka/L1_scraped_korpus_pelny_test | [
"region:us"
] | 2022-11-19T16:39:05+00:00 | {"dataset_info": {"features": [{"name": "content", "dtype": "string"}, {"name": "label", "dtype": "int64"}], "splits": [{"name": "train", "num_bytes": 29613125, "num_examples": 312385}], "download_size": 21671824, "dataset_size": 29613125}} | 2022-11-19T17:16:03+00:00 | [] | [] | TAGS
#region-us
| # Dataset Card for "L1_scraped_korpus_pelny_test"
More Information needed | [
"# Dataset Card for \"L1_scraped_korpus_pelny_test\"\n\nMore Information needed"
] | [
"TAGS\n#region-us \n",
"# Dataset Card for \"L1_scraped_korpus_pelny_test\"\n\nMore Information needed"
] |
208f15b308d39fe537796a914044a9d1f9656a23 | # Dataset Card for "L1_scraped_korpus_wzorcowy_train"
[More Information needed](https://github.com/huggingface/datasets/blob/main/CONTRIBUTING.md#how-to-contribute-to-the-dataset-cards) | Nikutka/L1_scraped_korpus_wzorcowy_train | [
"region:us"
] | 2022-11-19T16:39:36+00:00 | {"dataset_info": {"features": [{"name": "content", "dtype": "string"}, {"name": "label", "dtype": "int64"}], "splits": [{"name": "train", "num_bytes": 4838134, "num_examples": 29488}], "download_size": 3466828, "dataset_size": 4838134}} | 2022-11-19T17:18:32+00:00 | [] | [] | TAGS
#region-us
| # Dataset Card for "L1_scraped_korpus_wzorcowy_train"
More Information needed | [
"# Dataset Card for \"L1_scraped_korpus_wzorcowy_train\"\n\nMore Information needed"
] | [
"TAGS\n#region-us \n",
"# Dataset Card for \"L1_scraped_korpus_wzorcowy_train\"\n\nMore Information needed"
] |
7e3a8ff3857ecff1c74aaba90a379cb57dad6cae | # Dataset Card for "L1_scraped_korpus_wzorcowy_test"
[More Information needed](https://github.com/huggingface/datasets/blob/main/CONTRIBUTING.md#how-to-contribute-to-the-dataset-cards) | Nikutka/L1_scraped_korpus_wzorcowy_test | [
"region:us"
] | 2022-11-19T16:39:40+00:00 | {"dataset_info": {"features": [{"name": "content", "dtype": "string"}, {"name": "label", "dtype": "int64"}], "splits": [{"name": "train", "num_bytes": 1207567, "num_examples": 7372}], "download_size": 865883, "dataset_size": 1207567}} | 2022-11-19T17:18:35+00:00 | [] | [] | TAGS
#region-us
| # Dataset Card for "L1_scraped_korpus_wzorcowy_test"
More Information needed | [
"# Dataset Card for \"L1_scraped_korpus_wzorcowy_test\"\n\nMore Information needed"
] | [
"TAGS\n#region-us \n",
"# Dataset Card for \"L1_scraped_korpus_wzorcowy_test\"\n\nMore Information needed"
] |
9dede1f6ae1d869828ed07cd1ef20c07ec4d6b2f | # Dataset Card for "L1_scraped_korpus_wzorcowy"
[More Information needed](https://github.com/huggingface/datasets/blob/main/CONTRIBUTING.md#how-to-contribute-to-the-dataset-cards) | Nikutka/L1_scraped_korpus_wzorcowy | [
"region:us"
] | 2022-11-19T16:39:44+00:00 | {"dataset_info": {"features": [{"name": "content", "dtype": "string"}, {"name": "label", "dtype": "int64"}], "splits": [{"name": "train", "num_bytes": 4838134, "num_examples": 29488}, {"name": "test", "num_bytes": 1207567, "num_examples": 7372}], "download_size": 4332711, "dataset_size": 6045701}} | 2022-11-19T17:18:41+00:00 | [] | [] | TAGS
#region-us
| # Dataset Card for "L1_scraped_korpus_wzorcowy"
More Information needed | [
"# Dataset Card for \"L1_scraped_korpus_wzorcowy\"\n\nMore Information needed"
] | [
"TAGS\n#region-us \n",
"# Dataset Card for \"L1_scraped_korpus_wzorcowy\"\n\nMore Information needed"
] |
5c4e18e9fa5fd20be2eb840b2f43d241bc073fc9 | # Dataset Card for "L1_scraped_korpus_pelny"
[More Information needed](https://github.com/huggingface/datasets/blob/main/CONTRIBUTING.md#how-to-contribute-to-the-dataset-cards) | Nikutka/L1_scraped_korpus_pelny | [
"region:us"
] | 2022-11-19T16:50:52+00:00 | {"dataset_info": {"features": [{"name": "content", "dtype": "string"}, {"name": "label", "dtype": "int64"}], "splits": [{"name": "train", "num_bytes": 118294409, "num_examples": 1249536}, {"name": "test", "num_bytes": 29613125, "num_examples": 312385}], "download_size": 108295347, "dataset_size": 147907534}} | 2022-11-19T17:15:54+00:00 | [] | [] | TAGS
#region-us
| # Dataset Card for "L1_scraped_korpus_pelny"
More Information needed | [
"# Dataset Card for \"L1_scraped_korpus_pelny\"\n\nMore Information needed"
] | [
"TAGS\n#region-us \n",
"# Dataset Card for \"L1_scraped_korpus_pelny\"\n\nMore Information needed"
] |
a953228ef0aad99a67b9da3b5cc2a7e6dc3fff55 | # Dataset Card for "L1_poleval_korpus_pelny"
[More Information needed](https://github.com/huggingface/datasets/blob/main/CONTRIBUTING.md#how-to-contribute-to-the-dataset-cards) | Nikutka/L1_poleval_korpus_pelny | [
"region:us"
] | 2022-11-19T16:51:32+00:00 | {"dataset_info": {"features": [{"name": "content", "dtype": "string"}, {"name": "label", "dtype": "int64"}], "splits": [{"name": "train", "num_bytes": 764265, "num_examples": 9443}, {"name": "test", "num_bytes": 71297, "num_examples": 891}], "download_size": 556613, "dataset_size": 835562}} | 2022-11-19T16:51:39+00:00 | [] | [] | TAGS
#region-us
| # Dataset Card for "L1_poleval_korpus_pelny"
More Information needed | [
"# Dataset Card for \"L1_poleval_korpus_pelny\"\n\nMore Information needed"
] | [
"TAGS\n#region-us \n",
"# Dataset Card for \"L1_poleval_korpus_pelny\"\n\nMore Information needed"
] |
12d7363c2f20943fa85687da7ac2a6947fb2923a | # Dataset Card for "L1_poleval_korpus_wzorcowy"
[More Information needed](https://github.com/huggingface/datasets/blob/main/CONTRIBUTING.md#how-to-contribute-to-the-dataset-cards) | Nikutka/L1_poleval_korpus_wzorcowy | [
"region:us"
] | 2022-11-19T16:51:55+00:00 | {"dataset_info": {"features": [{"name": "content", "dtype": "string"}, {"name": "label", "dtype": "int64"}], "splits": [{"name": "train", "num_bytes": 20564, "num_examples": 253}, {"name": "test", "num_bytes": 1963, "num_examples": 25}], "download_size": 18165, "dataset_size": 22527}} | 2022-11-19T16:52:02+00:00 | [] | [] | TAGS
#region-us
| # Dataset Card for "L1_poleval_korpus_wzorcowy"
More Information needed | [
"# Dataset Card for \"L1_poleval_korpus_wzorcowy\"\n\nMore Information needed"
] | [
"TAGS\n#region-us \n",
"# Dataset Card for \"L1_poleval_korpus_wzorcowy\"\n\nMore Information needed"
] |
2b67f85fe6ae0762e5b6bcb5e2202477c204dc83 |
_The Dataset Teaser is now enabled instead! Isn't this better?_

# TD 01: Natural Ground Textures
This dataset contains multi-photo texture captures in outdoor nature scenes — all focusing on the ground. Each set has different photos that showcase texture variety, making them ideal for training a domain-specific image generator!
Overall information about this dataset:
* **Format** — JPEG-XL, lossless RGB
* **Resolution** — 4032 × 2268
* **Device** — mobile camera
* **Technique** — hand-held
* **Orientation** — portrait or landscape
* **Author**: Alex J. Champandard
* **Configurations**: 4K, 2K (default), 1K
To load the medium- and high-resolution images of the dataset, you'll need to install `jxlpy` from [PyPI](https://pypi.org/project/jxlpy/) with `pip install jxlpy`:
```python
# Recommended use, JXL at high-quality.
from jxlpy import JXLImagePlugin
from datasets import load_dataset
d = load_dataset('texturedesign/td01_natural-ground-textures', 'JXL@4K', num_proc=4)
print(len(d['train']), len(d['test']))
```
The lowest-resolution images are available as PNG with a regular installation of `pillow`:
```python
# Alternative use, PNG at low-quality.
from datasets import load_dataset
d = load_dataset('texturedesign/td01_natural-ground-textures', 'PNG@1K', num_proc=4)
# EXAMPLE: Discard all other sets except Set #1.
dataset = dataset.filter(lambda s: s['set'] == 1)
# EXAMPLE: Only keep images with index 0 and 2.
dataset = dataset.select([0, 2])
```
Use built-in dataset `filter()` and `select()` to narrow down the loaded dataset for training, or to ease with development.
## Set #1: Rock and Gravel

* **Description**:
- surface rocks with gravel and coarse sand
- strong sunlight from the left, sharp shadows
* **Number of Photos**:
- 7 train
- 2 test
* **Edits**:
- rotated photos to align sunlight
- removed infrequent objects
* **Size**: 77.8 Mb
## Set #2: Dry Grass with Pine Needles

* **Description**:
- field of dry grass and pine needles
- sunlight from the top right, some shadows
* **Number of Photos**:
- 6 train
- 1 test
* **Edits**:
- removed dry leaves and large plants
- removed sticks, rocks and sporadic daisies
* **Size**: 95.2 Mb
## Set #3: Chipped Stones, Broken Leaves and Twiglets

* **Description**:
- autumn path with chipped stones and dry broken leaves
- diffuse light on a cloudy day, very soft shadows
* **Number of Photos**:
- 9 train
- 3 test
* **Edits**:
- removed anything that looks green, fresh leaves
- removed long sticks and large/odd stones
* **Size**: 126.9 Mb
## Set #4: Grass Clumps and Cracked Dirt

* **Description**:
- clumps of green grass, clover and patches of cracked dirt
- diffuse light on cloudy day, shadows under large blades of grass
* **Number of Photos**:
- 9 train
- 2 test
* **Edits**:
- removed dry leaves, sporadic dandelions, and large objects
- histogram matching for two of the photos so the colors look similar
* **Size**: 126.8 Mb
## Set #5: Dirt, Stones, Rock, Twigs...

* **Description**:
- intricate micro-scene with grey dirt, surface rock, stones, twigs and organic debris
- diffuse light on cloudy day, soft shadows around the larger objects
* **Number of Photos**:
- 9 train
- 3 test
* **Edits**:
- removed odd objects that felt out-of-distribution
* **Size**: 102.1 Mb
## Set #6: Plants with Flowers on Dry Leaves

* **Description**:
- leafy plants with white flowers on a bed of dry brown leaves
- soft diffuse light, shaded areas under the plants
* **Number of Photos**:
- 9 train
- 2 test
* **Edits**:
- none yet, inpainting doesn't work well enough
- would remove long sticks and pieces of wood
* **Size**: 105.1 Mb
## Set #7: Frozen Footpath with Snow

* **Description**:
- frozen ground on a path with footprints
- areas with snow and dark brown ground beneath
- diffuse lighting on a cloudy day
* **Number of Photos**:
- 11 train
- 3 test
* **Size**: 95.5 Mb
## Set #8: Pine Needles Forest Floor

* **Description**:
- forest floor with a mix of brown soil and grass
- variety of dry white leaves, sticks, pinecones, pine needles
- diffuse lighting on a cloudy day
* **Number of Photos**:
- 15 train
- 4 test
* **Size**: 160.6 Mb
## Set #9: Snow on Grass and Dried Leaves

* **Description**:
- field in a park with short green grass
- large dried brown leaves and fallen snow on top
- diffuse lighting on a cloudy day
* **Number of Photos**:
- 8 train
- 3 test
* **Size**: 99.8 Mb
## Set #10: Brown Leaves on Wet Ground

* **Description**:
- fallew brown leaves on wet ground
- occasional tree root and twiglets
- diffuse lighting on a rainy day
* **Number of Photos**:
- 17 train
- 4 test
* **Size**: 186.2 Mb
## Set #11: Wet Sand Path with Debris

* **Description**:
- hard sandy path in the rain
- decomposing leaves and other organic debris
- diffuse lighting on a rainy day
* **Number of Photos**:
- 17 train
- 4 test
* **Size**: 186.2 Mb
## Set #12: Wood Chips & Sawdust Sprinkled on Forest Path

* **Description**:
- wood chips, sawdust, twigs and roots on forest path
- intermittent sunlight with shadows of trees
* **Number of Photos**:
- 8 train
- 2 test
* **Size**: 110.4 Mb
## Set #13: Young Grass Growing in the Dog Park

* **Description**:
- young grass growing in a dog park after overnight rain
- occasional stones, sticks and twigs, pine needles
- diffuse lighting on a cloudy day
* **Number of Photos**:
- 17 train
- 4 test
* **Size**: 193.4 Mb
## Set #14: Wavy Wet Beach Sand

* **Description**:
- wavy wet sand on the beach after the tide retreated
- some dirt and large pieces algae debris
- diffuse lighting on a cloudy day
* **Number of Photos**:
- 11 train
- 3 test
* **Size**: 86.5 Mb
## Set #15: Dry Dirt Road and Debris from Trees

* **Description**:
- dirt road of dry compacted sand with debris on top
- old pine needles and dry brown leaves
- diffuse lighting on a cloudy day
* **Number of Photos**:
- 8 train
- 2 test
* **Size**: 86.9 Mb
## Set #16: Sandy Beach Path with Grass Clumps

* **Description**:
- path with sand and clumps grass heading towards the beach
- occasional blueish stones, leafy weeds, and yellow flowers
- diffuse lighting on a cloudy day
* **Number of Photos**:
- 10 train
- 3 test
* **Size**: 118.8 Mb
## Set #17: Pine Needles and Brown Leaves on Park Floor

* **Description**:
- park floor with predominantly pine needles
- brown leaves from nearby trees, green grass underneath
- diffuse lighting on a cloudy day
* **Number of Photos**:
- 8 train
- 2 test
* **Size**: 99.9 Mb
| texturedesign/td01_natural-ground-textures | [
"task_categories:unconditional-image-generation",
"annotations_creators:expert-generated",
"size_categories:n<1K",
"source_datasets:original",
"license:cc-by-nc-4.0",
"texture-synthesis",
"photography",
"non-infringing",
"region:us"
] | 2022-11-19T17:43:30+00:00 | {"annotations_creators": ["expert-generated"], "language_creators": [], "language": [], "license": ["cc-by-nc-4.0"], "multilinguality": [], "size_categories": ["n<1K"], "source_datasets": ["original"], "task_categories": ["unconditional-image-generation"], "task_ids": [], "pretty_name": "TD01: Natural Ground Texture Photos", "tags": ["texture-synthesis", "photography", "non-infringing"], "viewer": false} | 2023-09-02T09:21:04+00:00 | [] | [] | TAGS
#task_categories-unconditional-image-generation #annotations_creators-expert-generated #size_categories-n<1K #source_datasets-original #license-cc-by-nc-4.0 #texture-synthesis #photography #non-infringing #region-us
|
_The Dataset Teaser is now enabled instead! Isn't this better?_
!preview of all texture sets
# TD 01: Natural Ground Textures
This dataset contains multi-photo texture captures in outdoor nature scenes — all focusing on the ground. Each set has different photos that showcase texture variety, making them ideal for training a domain-specific image generator!
Overall information about this dataset:
* Format — JPEG-XL, lossless RGB
* Resolution — 4032 × 2268
* Device — mobile camera
* Technique — hand-held
* Orientation — portrait or landscape
* Author: Alex J. Champandard
* Configurations: 4K, 2K (default), 1K
To load the medium- and high-resolution images of the dataset, you'll need to install 'jxlpy' from PyPI with 'pip install jxlpy':
The lowest-resolution images are available as PNG with a regular installation of 'pillow':
Use built-in dataset 'filter()' and 'select()' to narrow down the loaded dataset for training, or to ease with development.
## Set #1: Rock and Gravel
!preview of the files in Set #1
* Description:
- surface rocks with gravel and coarse sand
- strong sunlight from the left, sharp shadows
* Number of Photos:
- 7 train
- 2 test
* Edits:
- rotated photos to align sunlight
- removed infrequent objects
* Size: 77.8 Mb
## Set #2: Dry Grass with Pine Needles
!preview of the files in Set #2
* Description:
- field of dry grass and pine needles
- sunlight from the top right, some shadows
* Number of Photos:
- 6 train
- 1 test
* Edits:
- removed dry leaves and large plants
- removed sticks, rocks and sporadic daisies
* Size: 95.2 Mb
## Set #3: Chipped Stones, Broken Leaves and Twiglets
!preview of the files in Set #3
* Description:
- autumn path with chipped stones and dry broken leaves
- diffuse light on a cloudy day, very soft shadows
* Number of Photos:
- 9 train
- 3 test
* Edits:
- removed anything that looks green, fresh leaves
- removed long sticks and large/odd stones
* Size: 126.9 Mb
## Set #4: Grass Clumps and Cracked Dirt
!preview of the files in Set #4
* Description:
- clumps of green grass, clover and patches of cracked dirt
- diffuse light on cloudy day, shadows under large blades of grass
* Number of Photos:
- 9 train
- 2 test
* Edits:
- removed dry leaves, sporadic dandelions, and large objects
- histogram matching for two of the photos so the colors look similar
* Size: 126.8 Mb
## Set #5: Dirt, Stones, Rock, Twigs...
!preview of the files in Set #5
* Description:
- intricate micro-scene with grey dirt, surface rock, stones, twigs and organic debris
- diffuse light on cloudy day, soft shadows around the larger objects
* Number of Photos:
- 9 train
- 3 test
* Edits:
- removed odd objects that felt out-of-distribution
* Size: 102.1 Mb
## Set #6: Plants with Flowers on Dry Leaves
!preview of the files in Set #6
* Description:
- leafy plants with white flowers on a bed of dry brown leaves
- soft diffuse light, shaded areas under the plants
* Number of Photos:
- 9 train
- 2 test
* Edits:
- none yet, inpainting doesn't work well enough
- would remove long sticks and pieces of wood
* Size: 105.1 Mb
## Set #7: Frozen Footpath with Snow
!preview of the files in Set #7
* Description:
- frozen ground on a path with footprints
- areas with snow and dark brown ground beneath
- diffuse lighting on a cloudy day
* Number of Photos:
- 11 train
- 3 test
* Size: 95.5 Mb
## Set #8: Pine Needles Forest Floor
!preview of the files in Set #8
* Description:
- forest floor with a mix of brown soil and grass
- variety of dry white leaves, sticks, pinecones, pine needles
- diffuse lighting on a cloudy day
* Number of Photos:
- 15 train
- 4 test
* Size: 160.6 Mb
## Set #9: Snow on Grass and Dried Leaves
!preview of the files in Set #9
* Description:
- field in a park with short green grass
- large dried brown leaves and fallen snow on top
- diffuse lighting on a cloudy day
* Number of Photos:
- 8 train
- 3 test
* Size: 99.8 Mb
## Set #10: Brown Leaves on Wet Ground
!preview of the files in Set #10
* Description:
- fallew brown leaves on wet ground
- occasional tree root and twiglets
- diffuse lighting on a rainy day
* Number of Photos:
- 17 train
- 4 test
* Size: 186.2 Mb
## Set #11: Wet Sand Path with Debris
!preview of the files in Set #11
* Description:
- hard sandy path in the rain
- decomposing leaves and other organic debris
- diffuse lighting on a rainy day
* Number of Photos:
- 17 train
- 4 test
* Size: 186.2 Mb
## Set #12: Wood Chips & Sawdust Sprinkled on Forest Path
!preview of the files in Set #12
* Description:
- wood chips, sawdust, twigs and roots on forest path
- intermittent sunlight with shadows of trees
* Number of Photos:
- 8 train
- 2 test
* Size: 110.4 Mb
## Set #13: Young Grass Growing in the Dog Park
!preview of the files in Set #13
* Description:
- young grass growing in a dog park after overnight rain
- occasional stones, sticks and twigs, pine needles
- diffuse lighting on a cloudy day
* Number of Photos:
- 17 train
- 4 test
* Size: 193.4 Mb
## Set #14: Wavy Wet Beach Sand
!preview of the files in Set #14
* Description:
- wavy wet sand on the beach after the tide retreated
- some dirt and large pieces algae debris
- diffuse lighting on a cloudy day
* Number of Photos:
- 11 train
- 3 test
* Size: 86.5 Mb
## Set #15: Dry Dirt Road and Debris from Trees
!preview of the files in Set #15
* Description:
- dirt road of dry compacted sand with debris on top
- old pine needles and dry brown leaves
- diffuse lighting on a cloudy day
* Number of Photos:
- 8 train
- 2 test
* Size: 86.9 Mb
## Set #16: Sandy Beach Path with Grass Clumps
!preview of the files in Set #17
* Description:
- path with sand and clumps grass heading towards the beach
- occasional blueish stones, leafy weeds, and yellow flowers
- diffuse lighting on a cloudy day
* Number of Photos:
- 10 train
- 3 test
* Size: 118.8 Mb
## Set #17: Pine Needles and Brown Leaves on Park Floor
!preview of the files in Set #16
* Description:
- park floor with predominantly pine needles
- brown leaves from nearby trees, green grass underneath
- diffuse lighting on a cloudy day
* Number of Photos:
- 8 train
- 2 test
* Size: 99.9 Mb
| [
"# TD 01: Natural Ground Textures\n\nThis dataset contains multi-photo texture captures in outdoor nature scenes — all focusing on the ground. Each set has different photos that showcase texture variety, making them ideal for training a domain-specific image generator!\n\nOverall information about this dataset:\n\n* Format — JPEG-XL, lossless RGB\n* Resolution — 4032 × 2268\n* Device — mobile camera\n* Technique — hand-held\n* Orientation — portrait or landscape\n* Author: Alex J. Champandard\n* Configurations: 4K, 2K (default), 1K\n\nTo load the medium- and high-resolution images of the dataset, you'll need to install 'jxlpy' from PyPI with 'pip install jxlpy':\n\n\n\nThe lowest-resolution images are available as PNG with a regular installation of 'pillow':\n\n\n\nUse built-in dataset 'filter()' and 'select()' to narrow down the loaded dataset for training, or to ease with development.",
"## Set #1: Rock and Gravel\n\n!preview of the files in Set #1\n\n* Description:\n - surface rocks with gravel and coarse sand\n - strong sunlight from the left, sharp shadows\n* Number of Photos:\n - 7 train\n - 2 test\n* Edits:\n - rotated photos to align sunlight\n - removed infrequent objects\n* Size: 77.8 Mb",
"## Set #2: Dry Grass with Pine Needles\n\n!preview of the files in Set #2\n\n* Description:\n - field of dry grass and pine needles\n - sunlight from the top right, some shadows\n* Number of Photos:\n - 6 train\n - 1 test\n* Edits:\n - removed dry leaves and large plants\n - removed sticks, rocks and sporadic daisies\n* Size: 95.2 Mb",
"## Set #3: Chipped Stones, Broken Leaves and Twiglets\n\n!preview of the files in Set #3\n\n* Description:\n - autumn path with chipped stones and dry broken leaves\n - diffuse light on a cloudy day, very soft shadows\n* Number of Photos:\n - 9 train\n - 3 test\n* Edits:\n - removed anything that looks green, fresh leaves\n - removed long sticks and large/odd stones\n* Size: 126.9 Mb",
"## Set #4: Grass Clumps and Cracked Dirt\n\n!preview of the files in Set #4\n\n* Description:\n - clumps of green grass, clover and patches of cracked dirt\n - diffuse light on cloudy day, shadows under large blades of grass\n* Number of Photos:\n - 9 train\n - 2 test\n* Edits:\n - removed dry leaves, sporadic dandelions, and large objects\n - histogram matching for two of the photos so the colors look similar\n* Size: 126.8 Mb",
"## Set #5: Dirt, Stones, Rock, Twigs...\n\n!preview of the files in Set #5\n\n* Description:\n - intricate micro-scene with grey dirt, surface rock, stones, twigs and organic debris\n - diffuse light on cloudy day, soft shadows around the larger objects\n* Number of Photos:\n - 9 train\n - 3 test\n* Edits:\n - removed odd objects that felt out-of-distribution\n* Size: 102.1 Mb",
"## Set #6: Plants with Flowers on Dry Leaves\n\n!preview of the files in Set #6\n\n* Description:\n - leafy plants with white flowers on a bed of dry brown leaves\n - soft diffuse light, shaded areas under the plants\n* Number of Photos:\n - 9 train\n - 2 test\n* Edits:\n - none yet, inpainting doesn't work well enough\n - would remove long sticks and pieces of wood\n* Size: 105.1 Mb",
"## Set #7: Frozen Footpath with Snow\n\n!preview of the files in Set #7\n\n* Description:\n - frozen ground on a path with footprints\n - areas with snow and dark brown ground beneath\n - diffuse lighting on a cloudy day\n* Number of Photos:\n - 11 train\n - 3 test\n* Size: 95.5 Mb",
"## Set #8: Pine Needles Forest Floor\n\n!preview of the files in Set #8\n\n* Description:\n - forest floor with a mix of brown soil and grass\n - variety of dry white leaves, sticks, pinecones, pine needles\n - diffuse lighting on a cloudy day\n* Number of Photos:\n - 15 train\n - 4 test\n* Size: 160.6 Mb",
"## Set #9: Snow on Grass and Dried Leaves\n\n!preview of the files in Set #9\n\n* Description:\n - field in a park with short green grass\n - large dried brown leaves and fallen snow on top\n - diffuse lighting on a cloudy day\n* Number of Photos:\n - 8 train\n - 3 test\n* Size: 99.8 Mb",
"## Set #10: Brown Leaves on Wet Ground\n\n!preview of the files in Set #10\n\n* Description:\n - fallew brown leaves on wet ground\n - occasional tree root and twiglets\n - diffuse lighting on a rainy day\n* Number of Photos:\n - 17 train\n - 4 test\n* Size: 186.2 Mb",
"## Set #11: Wet Sand Path with Debris\n\n!preview of the files in Set #11\n\n* Description:\n - hard sandy path in the rain\n - decomposing leaves and other organic debris\n - diffuse lighting on a rainy day\n* Number of Photos:\n - 17 train\n - 4 test\n* Size: 186.2 Mb",
"## Set #12: Wood Chips & Sawdust Sprinkled on Forest Path\n\n!preview of the files in Set #12\n\n* Description:\n - wood chips, sawdust, twigs and roots on forest path\n - intermittent sunlight with shadows of trees\n* Number of Photos:\n - 8 train\n - 2 test\n* Size: 110.4 Mb",
"## Set #13: Young Grass Growing in the Dog Park\n\n!preview of the files in Set #13\n\n* Description:\n - young grass growing in a dog park after overnight rain\n - occasional stones, sticks and twigs, pine needles\n - diffuse lighting on a cloudy day\n* Number of Photos:\n - 17 train\n - 4 test\n* Size: 193.4 Mb",
"## Set #14: Wavy Wet Beach Sand\n\n!preview of the files in Set #14\n\n* Description:\n - wavy wet sand on the beach after the tide retreated\n - some dirt and large pieces algae debris\n - diffuse lighting on a cloudy day\n* Number of Photos:\n - 11 train\n - 3 test\n* Size: 86.5 Mb",
"## Set #15: Dry Dirt Road and Debris from Trees\n\n!preview of the files in Set #15\n\n* Description:\n - dirt road of dry compacted sand with debris on top\n - old pine needles and dry brown leaves\n - diffuse lighting on a cloudy day\n* Number of Photos:\n - 8 train\n - 2 test\n* Size: 86.9 Mb",
"## Set #16: Sandy Beach Path with Grass Clumps\n\n!preview of the files in Set #17\n\n* Description:\n - path with sand and clumps grass heading towards the beach\n - occasional blueish stones, leafy weeds, and yellow flowers\n - diffuse lighting on a cloudy day\n* Number of Photos:\n - 10 train\n - 3 test\n* Size: 118.8 Mb",
"## Set #17: Pine Needles and Brown Leaves on Park Floor\n\n!preview of the files in Set #16\n\n* Description:\n - park floor with predominantly pine needles\n - brown leaves from nearby trees, green grass underneath\n - diffuse lighting on a cloudy day\n* Number of Photos:\n - 8 train\n - 2 test\n* Size: 99.9 Mb"
] | [
"TAGS\n#task_categories-unconditional-image-generation #annotations_creators-expert-generated #size_categories-n<1K #source_datasets-original #license-cc-by-nc-4.0 #texture-synthesis #photography #non-infringing #region-us \n",
"# TD 01: Natural Ground Textures\n\nThis dataset contains multi-photo texture captures in outdoor nature scenes — all focusing on the ground. Each set has different photos that showcase texture variety, making them ideal for training a domain-specific image generator!\n\nOverall information about this dataset:\n\n* Format — JPEG-XL, lossless RGB\n* Resolution — 4032 × 2268\n* Device — mobile camera\n* Technique — hand-held\n* Orientation — portrait or landscape\n* Author: Alex J. Champandard\n* Configurations: 4K, 2K (default), 1K\n\nTo load the medium- and high-resolution images of the dataset, you'll need to install 'jxlpy' from PyPI with 'pip install jxlpy':\n\n\n\nThe lowest-resolution images are available as PNG with a regular installation of 'pillow':\n\n\n\nUse built-in dataset 'filter()' and 'select()' to narrow down the loaded dataset for training, or to ease with development.",
"## Set #1: Rock and Gravel\n\n!preview of the files in Set #1\n\n* Description:\n - surface rocks with gravel and coarse sand\n - strong sunlight from the left, sharp shadows\n* Number of Photos:\n - 7 train\n - 2 test\n* Edits:\n - rotated photos to align sunlight\n - removed infrequent objects\n* Size: 77.8 Mb",
"## Set #2: Dry Grass with Pine Needles\n\n!preview of the files in Set #2\n\n* Description:\n - field of dry grass and pine needles\n - sunlight from the top right, some shadows\n* Number of Photos:\n - 6 train\n - 1 test\n* Edits:\n - removed dry leaves and large plants\n - removed sticks, rocks and sporadic daisies\n* Size: 95.2 Mb",
"## Set #3: Chipped Stones, Broken Leaves and Twiglets\n\n!preview of the files in Set #3\n\n* Description:\n - autumn path with chipped stones and dry broken leaves\n - diffuse light on a cloudy day, very soft shadows\n* Number of Photos:\n - 9 train\n - 3 test\n* Edits:\n - removed anything that looks green, fresh leaves\n - removed long sticks and large/odd stones\n* Size: 126.9 Mb",
"## Set #4: Grass Clumps and Cracked Dirt\n\n!preview of the files in Set #4\n\n* Description:\n - clumps of green grass, clover and patches of cracked dirt\n - diffuse light on cloudy day, shadows under large blades of grass\n* Number of Photos:\n - 9 train\n - 2 test\n* Edits:\n - removed dry leaves, sporadic dandelions, and large objects\n - histogram matching for two of the photos so the colors look similar\n* Size: 126.8 Mb",
"## Set #5: Dirt, Stones, Rock, Twigs...\n\n!preview of the files in Set #5\n\n* Description:\n - intricate micro-scene with grey dirt, surface rock, stones, twigs and organic debris\n - diffuse light on cloudy day, soft shadows around the larger objects\n* Number of Photos:\n - 9 train\n - 3 test\n* Edits:\n - removed odd objects that felt out-of-distribution\n* Size: 102.1 Mb",
"## Set #6: Plants with Flowers on Dry Leaves\n\n!preview of the files in Set #6\n\n* Description:\n - leafy plants with white flowers on a bed of dry brown leaves\n - soft diffuse light, shaded areas under the plants\n* Number of Photos:\n - 9 train\n - 2 test\n* Edits:\n - none yet, inpainting doesn't work well enough\n - would remove long sticks and pieces of wood\n* Size: 105.1 Mb",
"## Set #7: Frozen Footpath with Snow\n\n!preview of the files in Set #7\n\n* Description:\n - frozen ground on a path with footprints\n - areas with snow and dark brown ground beneath\n - diffuse lighting on a cloudy day\n* Number of Photos:\n - 11 train\n - 3 test\n* Size: 95.5 Mb",
"## Set #8: Pine Needles Forest Floor\n\n!preview of the files in Set #8\n\n* Description:\n - forest floor with a mix of brown soil and grass\n - variety of dry white leaves, sticks, pinecones, pine needles\n - diffuse lighting on a cloudy day\n* Number of Photos:\n - 15 train\n - 4 test\n* Size: 160.6 Mb",
"## Set #9: Snow on Grass and Dried Leaves\n\n!preview of the files in Set #9\n\n* Description:\n - field in a park with short green grass\n - large dried brown leaves and fallen snow on top\n - diffuse lighting on a cloudy day\n* Number of Photos:\n - 8 train\n - 3 test\n* Size: 99.8 Mb",
"## Set #10: Brown Leaves on Wet Ground\n\n!preview of the files in Set #10\n\n* Description:\n - fallew brown leaves on wet ground\n - occasional tree root and twiglets\n - diffuse lighting on a rainy day\n* Number of Photos:\n - 17 train\n - 4 test\n* Size: 186.2 Mb",
"## Set #11: Wet Sand Path with Debris\n\n!preview of the files in Set #11\n\n* Description:\n - hard sandy path in the rain\n - decomposing leaves and other organic debris\n - diffuse lighting on a rainy day\n* Number of Photos:\n - 17 train\n - 4 test\n* Size: 186.2 Mb",
"## Set #12: Wood Chips & Sawdust Sprinkled on Forest Path\n\n!preview of the files in Set #12\n\n* Description:\n - wood chips, sawdust, twigs and roots on forest path\n - intermittent sunlight with shadows of trees\n* Number of Photos:\n - 8 train\n - 2 test\n* Size: 110.4 Mb",
"## Set #13: Young Grass Growing in the Dog Park\n\n!preview of the files in Set #13\n\n* Description:\n - young grass growing in a dog park after overnight rain\n - occasional stones, sticks and twigs, pine needles\n - diffuse lighting on a cloudy day\n* Number of Photos:\n - 17 train\n - 4 test\n* Size: 193.4 Mb",
"## Set #14: Wavy Wet Beach Sand\n\n!preview of the files in Set #14\n\n* Description:\n - wavy wet sand on the beach after the tide retreated\n - some dirt and large pieces algae debris\n - diffuse lighting on a cloudy day\n* Number of Photos:\n - 11 train\n - 3 test\n* Size: 86.5 Mb",
"## Set #15: Dry Dirt Road and Debris from Trees\n\n!preview of the files in Set #15\n\n* Description:\n - dirt road of dry compacted sand with debris on top\n - old pine needles and dry brown leaves\n - diffuse lighting on a cloudy day\n* Number of Photos:\n - 8 train\n - 2 test\n* Size: 86.9 Mb",
"## Set #16: Sandy Beach Path with Grass Clumps\n\n!preview of the files in Set #17\n\n* Description:\n - path with sand and clumps grass heading towards the beach\n - occasional blueish stones, leafy weeds, and yellow flowers\n - diffuse lighting on a cloudy day\n* Number of Photos:\n - 10 train\n - 3 test\n* Size: 118.8 Mb",
"## Set #17: Pine Needles and Brown Leaves on Park Floor\n\n!preview of the files in Set #16\n\n* Description:\n - park floor with predominantly pine needles\n - brown leaves from nearby trees, green grass underneath\n - diffuse lighting on a cloudy day\n* Number of Photos:\n - 8 train\n - 2 test\n* Size: 99.9 Mb"
] |
bfc723a1831e441b95d5604a266ab939b48dd4f2 | # AutoTrain Dataset for project: autotrain_goodreads_string
## Dataset Description
This dataset has been automatically processed by AutoTrain for project autotrain_goodreads_string.
### Languages
The BCP-47 code for the dataset's language is en.
## Dataset Structure
### Data Instances
A sample from this dataset looks as follows:
```json
[
{
"target": 5,
"text": "This book was absolutely ADORABLE!!!!!!!!!!! It was an awesome, light and FUN read. \n I loved the characters but I absolutely LOVED Cam!!!!!!!!!!!! Major Swoooon Worthy! J \n \"You've been checking me out, haven't you? In-between your flaming insults? I feel like man candy.\" \n Seriously, between being HOT, FUNNY and OH SO VERY ADORABLE, Cam was the perfect catch!! \n \" I'm not going out with you Cam.\" \n \" I didn't ask you at this moment, now did I\" One side of his lips curved up. \" But you will eventually.\" \n \"You're delusional\" \n \"I'm determined.\" \n \" More like annoying.\" \n \" Most would say amazing.\" \n Cam and Avery's relationship is tough due to the secrets she keeps but he is the perfect match for breaking her out of her shell and facing her fears. \n This book is definitely a MUST READ. \n Trust me when I say this YOU will not regret it! \n www.Jenreadit.com"
},
{
"target": 4,
"text": "I FINISHED!!! This book took me FOREVER to read! But I am so glad I stuck with it, I really loved it. It took me a while to get into: this book has a TON of characters and storylines. But once I hit about the 100-page mark, I became very invested in the story and couldn't wait to see what would happen with Lizzie, Lane, Edward, Gin and the rest of the family. Oh, and Samuel T. There's a little bit of sex but mostly this is a sweeping romance novel, much like Dynasty and Dallas from the 1980's. If you loved those series, you will love this book. There's betrayal, unrequited love, family fortunes, and much scheming. \n There are many characters to love here and many to hate. Some are over-the-top but I loved the central storyline involving Lane and Lizzie. \n The author really gets the Southern mannerisms right, and the backdrop of the Kentucky Bourbon industry is fascinating. This book ends not so much on a cliffhanger but with many, many loose ends, and I will eagerly pick up the next book in this series."
}
]
```
### Dataset Fields
The dataset has the following fields (also called "features"):
```json
{
"target": "ClassLabel(num_classes=6, names=['0_stars', '1_stars', '2_stars', '3_stars', '4_stars', '5_stars'], id=None)",
"text": "Value(dtype='string', id=None)"
}
```
### Dataset Splits
This dataset is split into a train and validation split. The split sizes are as follow:
| Split name | Num samples |
| ------------ | ------------------- |
| train | 2357 |
| valid | 592 |
| fernanda-dionello/good-reads-string | [
"task_categories:text-classification",
"language:en",
"region:us"
] | 2022-11-19T20:09:23+00:00 | {"language": ["en"], "task_categories": ["text-classification"]} | 2022-11-19T20:10:26+00:00 | [] | [
"en"
] | TAGS
#task_categories-text-classification #language-English #region-us
| AutoTrain Dataset for project: autotrain\_goodreads\_string
===========================================================
Dataset Description
-------------------
This dataset has been automatically processed by AutoTrain for project autotrain\_goodreads\_string.
### Languages
The BCP-47 code for the dataset's language is en.
Dataset Structure
-----------------
### Data Instances
A sample from this dataset looks as follows:
### Dataset Fields
The dataset has the following fields (also called "features"):
### Dataset Splits
This dataset is split into a train and validation split. The split sizes are as follow:
| [
"### Languages\n\n\nThe BCP-47 code for the dataset's language is en.\n\n\nDataset Structure\n-----------------",
"### Data Instances\n\n\nA sample from this dataset looks as follows:",
"### Dataset Fields\n\n\nThe dataset has the following fields (also called \"features\"):",
"### Dataset Splits\n\n\nThis dataset is split into a train and validation split. The split sizes are as follow:"
] | [
"TAGS\n#task_categories-text-classification #language-English #region-us \n",
"### Languages\n\n\nThe BCP-47 code for the dataset's language is en.\n\n\nDataset Structure\n-----------------",
"### Data Instances\n\n\nA sample from this dataset looks as follows:",
"### Dataset Fields\n\n\nThe dataset has the following fields (also called \"features\"):",
"### Dataset Splits\n\n\nThis dataset is split into a train and validation split. The split sizes are as follow:"
] |
fd46dee0a684d1f651e353ce659e0f0ff11322e7 | # Dataset Card for "stefano-finetune"
[More Information needed](https://github.com/huggingface/datasets/blob/main/CONTRIBUTING.md#how-to-contribute-to-the-dataset-cards) | albluc24/stefano-finetune | [
"region:us"
] | 2022-11-19T20:30:42+00:00 | {"dataset_info": {"features": [{"name": "text", "dtype": "string"}, {"name": "audio", "dtype": "audio"}], "splits": [{"name": "eval", "num_bytes": 3732782.0, "num_examples": 1}, {"name": "train", "num_bytes": 227326609.0, "num_examples": 55}], "download_size": 0, "dataset_size": 231059391.0}} | 2022-11-19T20:46:15+00:00 | [] | [] | TAGS
#region-us
| # Dataset Card for "stefano-finetune"
More Information needed | [
"# Dataset Card for \"stefano-finetune\"\n\nMore Information needed"
] | [
"TAGS\n#region-us \n",
"# Dataset Card for \"stefano-finetune\"\n\nMore Information needed"
] |
ae1e4ca8b786994cd3192930ad28f1676b4b02ef | # Dataset Card for "two-minute-papers"
[More Information needed](https://github.com/huggingface/datasets/blob/main/CONTRIBUTING.md#how-to-contribute-to-the-dataset-cards) | Whispering-GPT/two-minute-papers | [
"task_categories:automatic-speech-recognition",
"whisper",
"whispering",
"base",
"region:us"
] | 2022-11-19T20:52:17+00:00 | {"task_categories": ["automatic-speech-recognition"], "dataset_info": {"features": [{"name": "CHANNEL_NAME", "dtype": "string"}, {"name": "URL", "dtype": "string"}, {"name": "TITLE", "dtype": "string"}, {"name": "DESCRIPTION", "dtype": "string"}, {"name": "TRANSCRIPTION", "dtype": "string"}, {"name": "SEGMENTS", "dtype": "string"}], "splits": [{"name": "train", "num_bytes": 10435074, "num_examples": 737}], "download_size": 4626170, "dataset_size": 10435074}, "tags": ["whisper", "whispering", "base"]} | 2022-11-19T23:34:46+00:00 | [] | [] | TAGS
#task_categories-automatic-speech-recognition #whisper #whispering #base #region-us
| # Dataset Card for "two-minute-papers"
More Information needed | [
"# Dataset Card for \"two-minute-papers\"\n\nMore Information needed"
] | [
"TAGS\n#task_categories-automatic-speech-recognition #whisper #whispering #base #region-us \n",
"# Dataset Card for \"two-minute-papers\"\n\nMore Information needed"
] |
eb5f579468dc10ed0510d26e5f3de6b34f8700ca | # Dataset Card for "gal_yair_8300_100x100_fixed"
[More Information needed](https://github.com/huggingface/datasets/blob/main/CONTRIBUTING.md#how-to-contribute-to-the-dataset-cards) | galman33/gal_yair_8300_100x100_fixed | [
"region:us"
] | 2022-11-19T22:43:01+00:00 | {"dataset_info": {"features": [{"name": "lat", "dtype": "float64"}, {"name": "lon", "dtype": "float64"}, {"name": "country_code", "dtype": {"class_label": {"names": {"0": "ad", "1": "ae", "2": "al", "3": "aq", "4": "ar", "5": "au", "6": "bd", "7": "be", "8": "bg", "9": "bm", "10": "bo", "11": "br", "12": "bt", "13": "bw", "14": "ca", "15": "ch", "16": "cl", "17": "co", "18": "cz", "19": "de", "20": "dk", "21": "ec", "22": "ee", "23": "es", "24": "fi", "25": "fr", "26": "gb", "27": "gh", "28": "gl", "29": "gr", "30": "gt", "31": "hk", "32": "hr", "33": "hu", "34": "id", "35": "ie", "36": "il", "37": "is", "38": "it", "39": "ix", "40": "jp", "41": "kg", "42": "kh", "43": "kr", "44": "la", "45": "lk", "46": "ls", "47": "lt", "48": "lu", "49": "lv", "50": "me", "51": "mg", "52": "mk", "53": "mn", "54": "mo", "55": "mt", "56": "mx", "57": "my", "58": "nl", "59": "no", "60": "nz", "61": "pe", "62": "ph", "63": "pl", "64": "pt", "65": "ro", "66": "rs", "67": "ru", "68": "se", "69": "sg", "70": "si", "71": "sk", "72": "sn", "73": "sz", "74": "th", "75": "tn", "76": "tr", "77": "tw", "78": "ua", "79": "ug", "80": "us", "81": "uy", "82": "za"}}}}, {"name": "image", "dtype": "image"}], "splits": [{"name": "train", "num_bytes": 142019429.5, "num_examples": 8300}], "download_size": 141877783, "dataset_size": 142019429.5}} | 2022-11-26T13:15:23+00:00 | [] | [] | TAGS
#region-us
| # Dataset Card for "gal_yair_8300_100x100_fixed"
More Information needed | [
"# Dataset Card for \"gal_yair_8300_100x100_fixed\"\n\nMore Information needed"
] | [
"TAGS\n#region-us \n",
"# Dataset Card for \"gal_yair_8300_100x100_fixed\"\n\nMore Information needed"
] |
6af6fbe04f185dabec6605e5c3858bac1dd23396 |
# Genshin Datasets for Diff-SVC
## 仓库地址
| 仓库 | 传送门 |
| :--------------------------------------------------------: | :----------------------------------------------------------: |
| Diff-SVC | [点此传送](https://github.com/prophesier/diff-svc) |
| 44.1KHz声码器 | [点此传送](https://openvpi.github.io/vocoders) |
| 原神语音数据集 | [点此传送](https://github.com/w4123/GenshinVoice) |
| 训练用底模(如果想用这个数据集自己训练,非原神模型也可用) | [点此传送](https://huggingface.co/Erythrocyte/Diff-SVC_Pre-trained_Models) |
| 已训练原神 Diff-SVC 模型(可用于二创整活) | [点此传送](https://huggingface.co/Erythrocyte/Diff-SVC_Genshin_Models) |
## 介绍
该数据集为训练 Diff-SVC 原神模型的数据集,上传的数据集均已进行 `长音频切割` 、`响度匹配`。可以 `直接用来预处理并训练`,由于每个角色数据集规模不够,训练时候建议配合 `预训练模型` 训练。预训练模型见上表,并且提供了 `详细的教程` 以及 `多种可选预训练模型`。
## 使用教程(以下操作均需要在Diff-SVC目录下进行)
### Windows平台
1. 下载自己需要的数据集
2. 解压到 Diff-SVC 根目录,如果提示覆盖,请直接覆盖。
4. 依次输入如下指令进行预处理
```bash
set PYTHONPATH=.
set CUDA_VISIBLE_DEVICES=0
python preprocessing/binarize.py --config training/config_nsf.yaml
```
5. 按照教程进行加载预训练模型并训练:
教程地址:https://huggingface.co/Erythrocyte/Diff-SVC_Pre-trained_Models
### Linux平台
1. 输入如下命令下载需要的数据集(这里以纳西妲为例,其它角色可以从下表右击链接复制)
```bash
wget https://huggingface.co/datasets/Erythrocyte/Diff-SVC_Genshin_Datasets/resolve/main/Sumeru/nahida.zip
```
2. 输入如下命令解压到 Diff-SVC 根目录,如果提示覆盖请输入 y
```bash
unzip nahida.zip
```
4. 依次输入如下指令进行预处理
```bash
export PYTHONPATH=.
CUDA_VISIBLE_DEVICES=0 python preprocessing/binarize.py --config training/config_nsf.yaml
```
5. 按照教程进行加载预训练模型并训练:
教程地址:https://huggingface.co/Erythrocyte/Diff-SVC_Pre-trained_Models
## 下载地址
| 地区 | 角色名 | 下载地址 |
| :--: | :----------: | :----------------------------------------------------------: |
| 蒙德 | 优菈 | [eula.zip](https://huggingface.co/datasets/Erythrocyte/Diff-SVC_Genshin_Datasets/resolve/main/Mondstadt/eula.zip) |
| 蒙德 | 阿贝多 | [albedo.zip](https://huggingface.co/datasets/Erythrocyte/Diff-SVC_Genshin_Datasets/resolve/main/Mondstadt/albedo.zip) |
| 蒙德 | 温迪 | [venti.zip](https://huggingface.co/datasets/Erythrocyte/Diff-SVC_Genshin_Datasets/resolve/main/Mondstadt/venti.zip) |
| 蒙德 | 莫娜 | [mona.zip](https://huggingface.co/datasets/Erythrocyte/Diff-SVC_Genshin_Datasets/resolve/main/Mondstadt/mona.zip) |
| 蒙德 | 可莉 | [klee.zip](https://huggingface.co/datasets/Erythrocyte/Diff-SVC_Genshin_Datasets/resolve/main/Mondstadt/klee.zip) |
| 蒙德 | 琴 | [jean.zip](https://huggingface.co/datasets/Erythrocyte/Diff-SVC_Genshin_Datasets/resolve/main/Mondstadt/jean.zip) |
| 蒙德 | 迪卢克 | [diluc.zip](https://huggingface.co/datasets/Erythrocyte/Diff-SVC_Genshin_Datasets/resolve/main/Mondstadt/diluc.zip) |
| 璃月 | 钟离 | [zhongli.zip](https://huggingface.co/datasets/Erythrocyte/Diff-SVC_Genshin_Datasets/resolve/main/Liyue/zhongli.zip) |
| 稻妻 | 雷电将军 | [raiden.zip](https://huggingface.co/datasets/Erythrocyte/Diff-SVC_Genshin_Datasets/resolve/main/Inazuma/raiden.zip) |
| 须弥 | 流浪者(散兵) | [wanderer.zip](https://huggingface.co/datasets/Erythrocyte/Diff-SVC_Genshin_Datasets/resolve/main/Sumeru/wanderer.zip) |
| 须弥 | 纳西妲 | [nahida.zip](https://huggingface.co/datasets/Erythrocyte/Diff-SVC_Genshin_Datasets/resolve/main/Sumeru/nahida.zip) |
| Erythrocyte/Diff-SVC_Genshin_Datasets | [
"Diff-SVC",
"Genshin",
"Genshin Impact",
"Voice Data",
"Voice Dataset",
"region:us"
] | 2022-11-20T01:25:40+00:00 | {"tags": ["Diff-SVC", "Genshin", "Genshin Impact", "Voice Data", "Voice Dataset"]} | 2022-12-19T01:33:22+00:00 | [] | [] | TAGS
#Diff-SVC #Genshin #Genshin Impact #Voice Data #Voice Dataset #region-us
| Genshin Datasets for Diff-SVC
=============================
仓库地址
----
介绍
--
该数据集为训练 Diff-SVC 原神模型的数据集,上传的数据集均已进行 '长音频切割' 、'响度匹配'。可以 '直接用来预处理并训练',由于每个角色数据集规模不够,训练时候建议配合 '预训练模型' 训练。预训练模型见上表,并且提供了 '详细的教程' 以及 '多种可选预训练模型'。
使用教程(以下操作均需要在Diff-SVC目录下进行)
---------------------------
### Windows平台
1. 下载自己需要的数据集
2. 解压到 Diff-SVC 根目录,如果提示覆盖,请直接覆盖。
3. 依次输入如下指令进行预处理
4. 按照教程进行加载预训练模型并训练:
教程地址:URL
### Linux平台
1. 输入如下命令下载需要的数据集(这里以纳西妲为例,其它角色可以从下表右击链接复制)
2. 输入如下命令解压到 Diff-SVC 根目录,如果提示覆盖请输入 y
3. 依次输入如下指令进行预处理
4. 按照教程进行加载预训练模型并训练:
教程地址:URL
下载地址
----
| [
"### Windows平台\n\n\n1. 下载自己需要的数据集\n2. 解压到 Diff-SVC 根目录,如果提示覆盖,请直接覆盖。\n3. 依次输入如下指令进行预处理\n4. 按照教程进行加载预训练模型并训练:\n\n\n教程地址:URL",
"### Linux平台\n\n\n1. 输入如下命令下载需要的数据集(这里以纳西妲为例,其它角色可以从下表右击链接复制)\n2. 输入如下命令解压到 Diff-SVC 根目录,如果提示覆盖请输入 y\n3. 依次输入如下指令进行预处理\n4. 按照教程进行加载预训练模型并训练:\n\n\n教程地址:URL\n\n\n下载地址\n----"
] | [
"TAGS\n#Diff-SVC #Genshin #Genshin Impact #Voice Data #Voice Dataset #region-us \n",
"### Windows平台\n\n\n1. 下载自己需要的数据集\n2. 解压到 Diff-SVC 根目录,如果提示覆盖,请直接覆盖。\n3. 依次输入如下指令进行预处理\n4. 按照教程进行加载预训练模型并训练:\n\n\n教程地址:URL",
"### Linux平台\n\n\n1. 输入如下命令下载需要的数据集(这里以纳西妲为例,其它角色可以从下表右击链接复制)\n2. 输入如下命令解压到 Diff-SVC 根目录,如果提示覆盖请输入 y\n3. 依次输入如下指令进行预处理\n4. 按照教程进行加载预训练模型并训练:\n\n\n教程地址:URL\n\n\n下载地址\n----"
] |
d6115c5b9a52a758d6f0d08f0bd9b19df294edf9 |
# Dataset Card for EUWikipedias: A dataset of Wikipedias in the EU languages
## Table of Contents
- [Table of Contents](#table-of-contents)
- [Dataset Description](#dataset-description)
- [Dataset Summary](#dataset-summary)
- [Supported Tasks and Leaderboards](#supported-tasks-and-leaderboards)
- [Languages](#languages)
- [Dataset Structure](#dataset-structure)
- [Data Instances](#data-instances)
- [Data Fields](#data-fields)
- [Data Splits](#data-splits)
- [Dataset Creation](#dataset-creation)
- [Curation Rationale](#curation-rationale)
- [Source Data](#source-data)
- [Annotations](#annotations)
- [Personal and Sensitive Information](#personal-and-sensitive-information)
- [Considerations for Using the Data](#considerations-for-using-the-data)
- [Social Impact of Dataset](#social-impact-of-dataset)
- [Discussion of Biases](#discussion-of-biases)
- [Other Known Limitations](#other-known-limitations)
- [Additional Information](#additional-information)
- [Dataset Curators](#dataset-curators)
- [Licensing Information](#licensing-information)
- [Citation Information](#citation-information)
- [Contributions](#contributions)
## Dataset Description
- **Homepage:**
- **Repository:**
- **Paper:**
- **Leaderboard:**
- **Point of Contact:** [Joel Niklaus](mailto:[email protected])
### Dataset Summary
Wikipedia dataset containing cleaned articles of all languages.
The datasets are built from the Wikipedia dump
(https://dumps.wikimedia.org/) with one split per language. Each example
contains the content of one full Wikipedia article with cleaning to strip
markdown and unwanted sections (references, etc.).
### Supported Tasks and Leaderboards
The dataset supports the tasks of fill-mask.
### Languages
The following languages are supported:
bg, cs, da, de, el, en, es, et, fi, fr, ga, hr, hu, it, lt, lv, mt, nl, pl, pt, ro, sk, sl, sv
## Dataset Structure
It is structured in the following format: {date}/{language}_{shard}.jsonl.xz
At the moment only the date '20221120' is supported.
Use the dataset like this:
```python
from datasets import load_dataset
dataset = load_dataset('joelito/EU_Wikipedias', date="20221120", language="de", split='train', streaming=True)
```
### Data Instances
The file format is jsonl.xz and there is one split available (`train`).
| Source | Size (MB) | Words | Documents | Words/Document |
|:-------------|------------:|-----------:|------------:|-----------------:|
| 20221120.all | 86034 | 9506846949 | 26481379 | 359 |
| 20221120.bg | 1261 | 88138772 | 285876 | 308 |
| 20221120.cs | 1904 | 189580185 | 513851 | 368 |
| 20221120.da | 679 | 74546410 | 286864 | 259 |
| 20221120.de | 11761 | 1191919523 | 2740891 | 434 |
| 20221120.el | 1531 | 103504078 | 215046 | 481 |
| 20221120.en | 26685 | 3192209334 | 6575634 | 485 |
| 20221120.es | 6636 | 801322400 | 1583597 | 506 |
| 20221120.et | 538 | 48618507 | 231609 | 209 |
| 20221120.fi | 1391 | 115779646 | 542134 | 213 |
| 20221120.fr | 9703 | 1140823165 | 2472002 | 461 |
| 20221120.ga | 72 | 8025297 | 57808 | 138 |
| 20221120.hr | 555 | 58853753 | 198746 | 296 |
| 20221120.hu | 1855 | 167732810 | 515777 | 325 |
| 20221120.it | 5999 | 687745355 | 1782242 | 385 |
| 20221120.lt | 409 | 37572513 | 203233 | 184 |
| 20221120.lv | 269 | 25091547 | 116740 | 214 |
| 20221120.mt | 29 | 2867779 | 5030 | 570 |
| 20221120.nl | 3208 | 355031186 | 2107071 | 168 |
| 20221120.pl | 3608 | 349900622 | 1543442 | 226 |
| 20221120.pt | 3315 | 389786026 | 1095808 | 355 |
| 20221120.ro | 1017 | 111455336 | 434935 | 256 |
| 20221120.sk | 506 | 49612232 | 238439 | 208 |
| 20221120.sl | 543 | 58858041 | 178472 | 329 |
| 20221120.sv | 2560 | 257872432 | 2556132 | 100 |
### Data Fields
[More Information Needed]
### Data Splits
[More Information Needed]
## Dataset Creation
This dataset has been created by downloading the wikipedias using [olm/wikipedia](https://huggingface.co/datasets/olm/wikipedia) for the 24 EU languages.
For more information about the creation of the dataset please refer to prepare_wikipedias.py
### Curation Rationale
[More Information Needed]
### Source Data
#### Initial Data Collection and Normalization
[More Information Needed]
#### Who are the source language producers?
[More Information Needed]
### Annotations
#### Annotation process
[More Information Needed]
#### Who are the annotators?
[More Information Needed]
### Personal and Sensitive Information
[More Information Needed]
## Considerations for Using the Data
### Social Impact of Dataset
[More Information Needed]
### Discussion of Biases
[More Information Needed]
### Other Known Limitations
[More Information Needed]
## Additional Information
### Dataset Curators
[More Information Needed]
### Licensing Information
[More Information Needed]
### Citation Information
```
TODO add citation
```
### Contributions
Thanks to [@JoelNiklaus](https://github.com/joelniklaus) for adding this dataset.
| joelniklaus/EU_Wikipedias | [
"task_categories:fill-mask",
"annotations_creators:other",
"language_creators:found",
"multilinguality:multilingual",
"size_categories:10M<n<100M",
"source_datasets:original",
"language:bg",
"language:cs",
"language:da",
"language:de",
"language:el",
"language:en",
"language:es",
"language:et",
"language:fi",
"language:fr",
"language:ga",
"language:hr",
"language:hu",
"language:it",
"language:lt",
"language:lv",
"language:mt",
"language:nl",
"language:pl",
"language:pt",
"language:ro",
"language:sk",
"language:sl",
"language:sv",
"license:cc-by-4.0",
"region:us"
] | 2022-11-20T01:31:51+00:00 | {"annotations_creators": ["other"], "language_creators": ["found"], "language": ["bg", "cs", "da", "de", "el", "en", "es", "et", "fi", "fr", "ga", "hr", "hu", "it", "lt", "lv", "mt", "nl", "pl", "pt", "ro", "sk", "sl", "sv"], "license": ["cc-by-4.0"], "multilinguality": ["multilingual"], "size_categories": ["10M<n<100M"], "source_datasets": ["original"], "task_categories": ["fill-mask"], "pretty_name": "EUWikipedias: A dataset of Wikipedias in the EU languages"} | 2023-03-21T15:44:18+00:00 | [] | [
"bg",
"cs",
"da",
"de",
"el",
"en",
"es",
"et",
"fi",
"fr",
"ga",
"hr",
"hu",
"it",
"lt",
"lv",
"mt",
"nl",
"pl",
"pt",
"ro",
"sk",
"sl",
"sv"
] | TAGS
#task_categories-fill-mask #annotations_creators-other #language_creators-found #multilinguality-multilingual #size_categories-10M<n<100M #source_datasets-original #language-Bulgarian #language-Czech #language-Danish #language-German #language-Modern Greek (1453-) #language-English #language-Spanish #language-Estonian #language-Finnish #language-French #language-Irish #language-Croatian #language-Hungarian #language-Italian #language-Lithuanian #language-Latvian #language-Maltese #language-Dutch #language-Polish #language-Portuguese #language-Romanian #language-Slovak #language-Slovenian #language-Swedish #license-cc-by-4.0 #region-us
| Dataset Card for EUWikipedias: A dataset of Wikipedias in the EU languages
==========================================================================
Table of Contents
-----------------
* Table of Contents
* Dataset Description
+ Dataset Summary
+ Supported Tasks and Leaderboards
+ Languages
* Dataset Structure
+ Data Instances
+ Data Fields
+ Data Splits
* Dataset Creation
+ Curation Rationale
+ Source Data
+ Annotations
+ Personal and Sensitive Information
* Considerations for Using the Data
+ Social Impact of Dataset
+ Discussion of Biases
+ Other Known Limitations
* Additional Information
+ Dataset Curators
+ Licensing Information
+ Citation Information
+ Contributions
Dataset Description
-------------------
* Homepage:
* Repository:
* Paper:
* Leaderboard:
* Point of Contact: Joel Niklaus
### Dataset Summary
Wikipedia dataset containing cleaned articles of all languages.
The datasets are built from the Wikipedia dump
(URL with one split per language. Each example
contains the content of one full Wikipedia article with cleaning to strip
markdown and unwanted sections (references, etc.).
### Supported Tasks and Leaderboards
The dataset supports the tasks of fill-mask.
### Languages
The following languages are supported:
bg, cs, da, de, el, en, es, et, fi, fr, ga, hr, hu, it, lt, lv, mt, nl, pl, pt, ro, sk, sl, sv
Dataset Structure
-----------------
It is structured in the following format: {date}/{language}\_{shard}.URL
At the moment only the date '20221120' is supported.
Use the dataset like this:
### Data Instances
The file format is URL and there is one split available ('train').
### Data Fields
### Data Splits
Dataset Creation
----------------
This dataset has been created by downloading the wikipedias using olm/wikipedia for the 24 EU languages.
For more information about the creation of the dataset please refer to prepare\_wikipedias.py
### Curation Rationale
### Source Data
#### Initial Data Collection and Normalization
#### Who are the source language producers?
### Annotations
#### Annotation process
#### Who are the annotators?
### Personal and Sensitive Information
Considerations for Using the Data
---------------------------------
### Social Impact of Dataset
### Discussion of Biases
### Other Known Limitations
Additional Information
----------------------
### Dataset Curators
### Licensing Information
### Contributions
Thanks to @JoelNiklaus for adding this dataset.
| [
"### Dataset Summary\n\n\nWikipedia dataset containing cleaned articles of all languages.\nThe datasets are built from the Wikipedia dump\n(URL with one split per language. Each example\ncontains the content of one full Wikipedia article with cleaning to strip\nmarkdown and unwanted sections (references, etc.).",
"### Supported Tasks and Leaderboards\n\n\nThe dataset supports the tasks of fill-mask.",
"### Languages\n\n\nThe following languages are supported:\nbg, cs, da, de, el, en, es, et, fi, fr, ga, hr, hu, it, lt, lv, mt, nl, pl, pt, ro, sk, sl, sv\n\n\nDataset Structure\n-----------------\n\n\nIt is structured in the following format: {date}/{language}\\_{shard}.URL\nAt the moment only the date '20221120' is supported.\n\n\nUse the dataset like this:",
"### Data Instances\n\n\nThe file format is URL and there is one split available ('train').",
"### Data Fields",
"### Data Splits\n\n\nDataset Creation\n----------------\n\n\nThis dataset has been created by downloading the wikipedias using olm/wikipedia for the 24 EU languages.\nFor more information about the creation of the dataset please refer to prepare\\_wikipedias.py",
"### Curation Rationale",
"### Source Data",
"#### Initial Data Collection and Normalization",
"#### Who are the source language producers?",
"### Annotations",
"#### Annotation process",
"#### Who are the annotators?",
"### Personal and Sensitive Information\n\n\nConsiderations for Using the Data\n---------------------------------",
"### Social Impact of Dataset",
"### Discussion of Biases",
"### Other Known Limitations\n\n\nAdditional Information\n----------------------",
"### Dataset Curators",
"### Licensing Information",
"### Contributions\n\n\nThanks to @JoelNiklaus for adding this dataset."
] | [
"TAGS\n#task_categories-fill-mask #annotations_creators-other #language_creators-found #multilinguality-multilingual #size_categories-10M<n<100M #source_datasets-original #language-Bulgarian #language-Czech #language-Danish #language-German #language-Modern Greek (1453-) #language-English #language-Spanish #language-Estonian #language-Finnish #language-French #language-Irish #language-Croatian #language-Hungarian #language-Italian #language-Lithuanian #language-Latvian #language-Maltese #language-Dutch #language-Polish #language-Portuguese #language-Romanian #language-Slovak #language-Slovenian #language-Swedish #license-cc-by-4.0 #region-us \n",
"### Dataset Summary\n\n\nWikipedia dataset containing cleaned articles of all languages.\nThe datasets are built from the Wikipedia dump\n(URL with one split per language. Each example\ncontains the content of one full Wikipedia article with cleaning to strip\nmarkdown and unwanted sections (references, etc.).",
"### Supported Tasks and Leaderboards\n\n\nThe dataset supports the tasks of fill-mask.",
"### Languages\n\n\nThe following languages are supported:\nbg, cs, da, de, el, en, es, et, fi, fr, ga, hr, hu, it, lt, lv, mt, nl, pl, pt, ro, sk, sl, sv\n\n\nDataset Structure\n-----------------\n\n\nIt is structured in the following format: {date}/{language}\\_{shard}.URL\nAt the moment only the date '20221120' is supported.\n\n\nUse the dataset like this:",
"### Data Instances\n\n\nThe file format is URL and there is one split available ('train').",
"### Data Fields",
"### Data Splits\n\n\nDataset Creation\n----------------\n\n\nThis dataset has been created by downloading the wikipedias using olm/wikipedia for the 24 EU languages.\nFor more information about the creation of the dataset please refer to prepare\\_wikipedias.py",
"### Curation Rationale",
"### Source Data",
"#### Initial Data Collection and Normalization",
"#### Who are the source language producers?",
"### Annotations",
"#### Annotation process",
"#### Who are the annotators?",
"### Personal and Sensitive Information\n\n\nConsiderations for Using the Data\n---------------------------------",
"### Social Impact of Dataset",
"### Discussion of Biases",
"### Other Known Limitations\n\n\nAdditional Information\n----------------------",
"### Dataset Curators",
"### Licensing Information",
"### Contributions\n\n\nThanks to @JoelNiklaus for adding this dataset."
] |
084fd1fb5aad0dc3f8c655807a21f07aaf27f5ff | # Dataset Card for "goog-tech-talks"
[More Information needed](https://github.com/huggingface/datasets/blob/main/CONTRIBUTING.md#how-to-contribute-to-the-dataset-cards) | juancopi81/goog-tech-talks | [
"task_categories:automatic-speech-recognition",
"whisper",
"whispering",
"base",
"region:us"
] | 2022-11-20T02:08:42+00:00 | {"task_categories": ["automatic-speech-recognition"], "dataset_info": {"features": [{"name": "CHANNEL_NAME", "dtype": "string"}, {"name": "URL", "dtype": "string"}, {"name": "TITLE", "dtype": "string"}, {"name": "DESCRIPTION", "dtype": "string"}, {"name": "TRANSCRIPTION", "dtype": "string"}, {"name": "SEGMENTS", "dtype": "string"}], "splits": [{"name": "train", "num_bytes": 101139, "num_examples": 1}], "download_size": 55503, "dataset_size": 101139}, "tags": ["whisper", "whispering", "base"]} | 2022-11-20T02:08:46+00:00 | [] | [] | TAGS
#task_categories-automatic-speech-recognition #whisper #whispering #base #region-us
| # Dataset Card for "goog-tech-talks"
More Information needed | [
"# Dataset Card for \"goog-tech-talks\"\n\nMore Information needed"
] | [
"TAGS\n#task_categories-automatic-speech-recognition #whisper #whispering #base #region-us \n",
"# Dataset Card for \"goog-tech-talks\"\n\nMore Information needed"
] |
cd69fbfac065aeebd820f8ed4a047fb266e2e170 | # Dataset Card for "gpk-captions"
[More Information needed](https://github.com/huggingface/datasets/blob/main/CONTRIBUTING.md#how-to-contribute-to-the-dataset-cards) | gluten/gpk-captions | [
"doi:10.57967/hf/0123",
"region:us"
] | 2022-11-20T03:42:14+00:00 | {"dataset_info": {"features": [{"name": "image", "dtype": "image"}, {"name": "text", "dtype": "string"}], "splits": [{"name": "train", "num_bytes": 45126936.0, "num_examples": 83}], "download_size": 45128569, "dataset_size": 45126936.0}} | 2022-11-20T04:03:10+00:00 | [] | [] | TAGS
#doi-10.57967/hf/0123 #region-us
| # Dataset Card for "gpk-captions"
More Information needed | [
"# Dataset Card for \"gpk-captions\"\n\nMore Information needed"
] | [
"TAGS\n#doi-10.57967/hf/0123 #region-us \n",
"# Dataset Card for \"gpk-captions\"\n\nMore Information needed"
] |
89c13ef027bdb602bb91d8c16469fff1d4cf3862 |
This is the dataset used for making the model : https://huggingface.co/Guizmus/SD_PoW_Collection
The images were made by the users of Stable Diffusion discord using CreativeML-OpenRail-M licenced models, in the intent to make this dataset.
60 pictures captioned with their content by hand, with the prefix "3D Style"
The collection process was made public during a day, until enough variety was introduced to train through a Dreambooth method a style corresponding to the different members of this community
The picture captioned are available in [this zip file](https://huggingface.co/datasets/Guizmus/3DChanStyle/resolve/main/3DChanStyle.zip) | Guizmus/3DChanStyle | [
"license:creativeml-openrail-m",
"region:us"
] | 2022-11-20T09:49:14+00:00 | {"license": "creativeml-openrail-m"} | 2022-11-23T09:18:31+00:00 | [] | [] | TAGS
#license-creativeml-openrail-m #region-us
|
This is the dataset used for making the model : URL
The images were made by the users of Stable Diffusion discord using CreativeML-OpenRail-M licenced models, in the intent to make this dataset.
60 pictures captioned with their content by hand, with the prefix "3D Style"
The collection process was made public during a day, until enough variety was introduced to train through a Dreambooth method a style corresponding to the different members of this community
The picture captioned are available in this zip file | [] | [
"TAGS\n#license-creativeml-openrail-m #region-us \n"
] |
77a3ee29ca65c47eaae250a3aa0164fd701d634a | # Dataset Card for "relbert/semeval2012_relational_similarity_V6"
## Dataset Description
- **Repository:** [RelBERT](https://github.com/asahi417/relbert)
- **Paper:** [https://aclanthology.org/S12-1047/](https://aclanthology.org/S12-1047/)
- **Dataset:** SemEval2012: Relational Similarity
### Dataset Summary
***IMPORTANT***: This is the same dataset as [relbert/semeval2012_relational_similarity](https://huggingface.co/datasets/relbert/semeval2012_relational_similarity),
but with a different dataset construction.
Relational similarity dataset from [SemEval2012 task 2](https://aclanthology.org/S12-1047/), compiled to fine-tune [RelBERT](https://github.com/asahi417/relbert) model.
The dataset contains a list of positive and negative word pair from 89 pre-defined relations.
The relation types are constructed on top of following 10 parent relation types.
```shell
{
1: "Class Inclusion", # Hypernym
2: "Part-Whole", # Meronym, Substance Meronym
3: "Similar", # Synonym, Co-hypornym
4: "Contrast", # Antonym
5: "Attribute", # Attribute, Event
6: "Non Attribute",
7: "Case Relation",
8: "Cause-Purpose",
9: "Space-Time",
10: "Representation"
}
```
Each of the parent relation is further grouped into child relation types where the definition can be found [here](https://drive.google.com/file/d/0BzcZKTSeYL8VenY0QkVpZVpxYnc/view?resourcekey=0-ZP-UARfJj39PcLroibHPHw).
## Dataset Structure
### Data Instances
An example of `train` looks as follows.
```
{
'relation_type': '8d',
'positives': [ [ "breathe", "live" ], [ "study", "learn" ], [ "speak", "communicate" ], ... ]
'negatives': [ [ "starving", "hungry" ], [ "clean", "bathe" ], [ "hungry", "starving" ], ... ]
}
```
### Data Splits
| name |train|validation|
|---------|----:|---------:|
|semeval2012_relational_similarity| 89 | 89|
### Number of Positive/Negative Word-pairs in each Split
| | positives | negatives |
|:--------------------------------------------|------------:|------------:|
| ('1', 'parent', 'train') | 88 | 544 |
| ('1', 'parent', 'validation') | 22 | 136 |
| ('10', 'parent', 'train') | 48 | 584 |
| ('10', 'parent', 'validation') | 12 | 146 |
| ('10a', 'child', 'train') | 8 | 1324 |
| ('10a', 'child', 'validation') | 2 | 331 |
| ('10a', 'child_prototypical', 'train') | 194 | 1917 |
| ('10a', 'child_prototypical', 'validation') | 52 | 521 |
| ('10b', 'child', 'train') | 8 | 1325 |
| ('10b', 'child', 'validation') | 2 | 331 |
| ('10b', 'child_prototypical', 'train') | 180 | 1558 |
| ('10b', 'child_prototypical', 'validation') | 54 | 469 |
| ('10c', 'child', 'train') | 8 | 1327 |
| ('10c', 'child', 'validation') | 2 | 331 |
| ('10c', 'child_prototypical', 'train') | 170 | 1640 |
| ('10c', 'child_prototypical', 'validation') | 40 | 390 |
| ('10d', 'child', 'train') | 8 | 1328 |
| ('10d', 'child', 'validation') | 2 | 331 |
| ('10d', 'child_prototypical', 'train') | 154 | 1390 |
| ('10d', 'child_prototypical', 'validation') | 44 | 376 |
| ('10e', 'child', 'train') | 8 | 1329 |
| ('10e', 'child', 'validation') | 2 | 332 |
| ('10e', 'child_prototypical', 'train') | 134 | 884 |
| ('10e', 'child_prototypical', 'validation') | 40 | 234 |
| ('10f', 'child', 'train') | 8 | 1328 |
| ('10f', 'child', 'validation') | 2 | 331 |
| ('10f', 'child_prototypical', 'train') | 160 | 1460 |
| ('10f', 'child_prototypical', 'validation') | 38 | 306 |
| ('1a', 'child', 'train') | 8 | 1324 |
| ('1a', 'child', 'validation') | 2 | 331 |
| ('1a', 'child_prototypical', 'train') | 212 | 1854 |
| ('1a', 'child_prototypical', 'validation') | 34 | 338 |
| ('1b', 'child', 'train') | 8 | 1324 |
| ('1b', 'child', 'validation') | 2 | 331 |
| ('1b', 'child_prototypical', 'train') | 190 | 1712 |
| ('1b', 'child_prototypical', 'validation') | 56 | 480 |
| ('1c', 'child', 'train') | 8 | 1327 |
| ('1c', 'child', 'validation') | 2 | 331 |
| ('1c', 'child_prototypical', 'train') | 160 | 1528 |
| ('1c', 'child_prototypical', 'validation') | 50 | 502 |
| ('1d', 'child', 'train') | 8 | 1323 |
| ('1d', 'child', 'validation') | 2 | 330 |
| ('1d', 'child_prototypical', 'train') | 224 | 2082 |
| ('1d', 'child_prototypical', 'validation') | 46 | 458 |
| ('1e', 'child', 'train') | 8 | 1329 |
| ('1e', 'child', 'validation') | 2 | 332 |
| ('1e', 'child_prototypical', 'train') | 126 | 775 |
| ('1e', 'child_prototypical', 'validation') | 48 | 256 |
| ('2', 'parent', 'train') | 80 | 552 |
| ('2', 'parent', 'validation') | 20 | 138 |
| ('2a', 'child', 'train') | 8 | 1324 |
| ('2a', 'child', 'validation') | 2 | 330 |
| ('2a', 'child_prototypical', 'train') | 186 | 1885 |
| ('2a', 'child_prototypical', 'validation') | 72 | 736 |
| ('2b', 'child', 'train') | 8 | 1327 |
| ('2b', 'child', 'validation') | 2 | 331 |
| ('2b', 'child_prototypical', 'train') | 172 | 1326 |
| ('2b', 'child_prototypical', 'validation') | 38 | 284 |
| ('2c', 'child', 'train') | 8 | 1325 |
| ('2c', 'child', 'validation') | 2 | 331 |
| ('2c', 'child_prototypical', 'train') | 192 | 1773 |
| ('2c', 'child_prototypical', 'validation') | 42 | 371 |
| ('2d', 'child', 'train') | 8 | 1328 |
| ('2d', 'child', 'validation') | 2 | 331 |
| ('2d', 'child_prototypical', 'train') | 158 | 1329 |
| ('2d', 'child_prototypical', 'validation') | 40 | 338 |
| ('2e', 'child', 'train') | 8 | 1327 |
| ('2e', 'child', 'validation') | 2 | 331 |
| ('2e', 'child_prototypical', 'train') | 164 | 1462 |
| ('2e', 'child_prototypical', 'validation') | 46 | 463 |
| ('2f', 'child', 'train') | 8 | 1327 |
| ('2f', 'child', 'validation') | 2 | 331 |
| ('2f', 'child_prototypical', 'train') | 176 | 1869 |
| ('2f', 'child_prototypical', 'validation') | 34 | 371 |
| ('2g', 'child', 'train') | 8 | 1323 |
| ('2g', 'child', 'validation') | 2 | 330 |
| ('2g', 'child_prototypical', 'train') | 216 | 1925 |
| ('2g', 'child_prototypical', 'validation') | 54 | 480 |
| ('2h', 'child', 'train') | 8 | 1327 |
| ('2h', 'child', 'validation') | 2 | 331 |
| ('2h', 'child_prototypical', 'train') | 168 | 1540 |
| ('2h', 'child_prototypical', 'validation') | 42 | 385 |
| ('2i', 'child', 'train') | 8 | 1328 |
| ('2i', 'child', 'validation') | 2 | 332 |
| ('2i', 'child_prototypical', 'train') | 144 | 1335 |
| ('2i', 'child_prototypical', 'validation') | 42 | 371 |
| ('2j', 'child', 'train') | 8 | 1328 |
| ('2j', 'child', 'validation') | 2 | 331 |
| ('2j', 'child_prototypical', 'train') | 160 | 1595 |
| ('2j', 'child_prototypical', 'validation') | 38 | 369 |
| ('3', 'parent', 'train') | 64 | 568 |
| ('3', 'parent', 'validation') | 16 | 142 |
| ('3a', 'child', 'train') | 8 | 1327 |
| ('3a', 'child', 'validation') | 2 | 331 |
| ('3a', 'child_prototypical', 'train') | 174 | 1597 |
| ('3a', 'child_prototypical', 'validation') | 36 | 328 |
| ('3b', 'child', 'train') | 8 | 1327 |
| ('3b', 'child', 'validation') | 2 | 331 |
| ('3b', 'child_prototypical', 'train') | 174 | 1833 |
| ('3b', 'child_prototypical', 'validation') | 36 | 407 |
| ('3c', 'child', 'train') | 8 | 1326 |
| ('3c', 'child', 'validation') | 2 | 331 |
| ('3c', 'child_prototypical', 'train') | 186 | 1664 |
| ('3c', 'child_prototypical', 'validation') | 36 | 315 |
| ('3d', 'child', 'train') | 8 | 1324 |
| ('3d', 'child', 'validation') | 2 | 331 |
| ('3d', 'child_prototypical', 'train') | 202 | 1943 |
| ('3d', 'child_prototypical', 'validation') | 44 | 372 |
| ('3e', 'child', 'train') | 8 | 1332 |
| ('3e', 'child', 'validation') | 2 | 332 |
| ('3e', 'child_prototypical', 'train') | 98 | 900 |
| ('3e', 'child_prototypical', 'validation') | 40 | 368 |
| ('3f', 'child', 'train') | 8 | 1327 |
| ('3f', 'child', 'validation') | 2 | 331 |
| ('3f', 'child_prototypical', 'train') | 180 | 1983 |
| ('3f', 'child_prototypical', 'validation') | 30 | 362 |
| ('3g', 'child', 'train') | 8 | 1331 |
| ('3g', 'child', 'validation') | 2 | 332 |
| ('3g', 'child_prototypical', 'train') | 122 | 1089 |
| ('3g', 'child_prototypical', 'validation') | 28 | 251 |
| ('3h', 'child', 'train') | 8 | 1328 |
| ('3h', 'child', 'validation') | 2 | 331 |
| ('3h', 'child_prototypical', 'train') | 142 | 1399 |
| ('3h', 'child_prototypical', 'validation') | 56 | 565 |
| ('4', 'parent', 'train') | 64 | 568 |
| ('4', 'parent', 'validation') | 16 | 142 |
| ('4a', 'child', 'train') | 8 | 1327 |
| ('4a', 'child', 'validation') | 2 | 331 |
| ('4a', 'child_prototypical', 'train') | 170 | 1766 |
| ('4a', 'child_prototypical', 'validation') | 40 | 474 |
| ('4b', 'child', 'train') | 8 | 1330 |
| ('4b', 'child', 'validation') | 2 | 332 |
| ('4b', 'child_prototypical', 'train') | 132 | 949 |
| ('4b', 'child_prototypical', 'validation') | 30 | 214 |
| ('4c', 'child', 'train') | 8 | 1326 |
| ('4c', 'child', 'validation') | 2 | 331 |
| ('4c', 'child_prototypical', 'train') | 172 | 1755 |
| ('4c', 'child_prototypical', 'validation') | 50 | 446 |
| ('4d', 'child', 'train') | 8 | 1332 |
| ('4d', 'child', 'validation') | 2 | 333 |
| ('4d', 'child_prototypical', 'train') | 92 | 531 |
| ('4d', 'child_prototypical', 'validation') | 34 | 218 |
| ('4e', 'child', 'train') | 8 | 1326 |
| ('4e', 'child', 'validation') | 2 | 331 |
| ('4e', 'child_prototypical', 'train') | 184 | 2021 |
| ('4e', 'child_prototypical', 'validation') | 38 | 402 |
| ('4f', 'child', 'train') | 8 | 1328 |
| ('4f', 'child', 'validation') | 2 | 332 |
| ('4f', 'child_prototypical', 'train') | 144 | 1464 |
| ('4f', 'child_prototypical', 'validation') | 42 | 428 |
| ('4g', 'child', 'train') | 8 | 1324 |
| ('4g', 'child', 'validation') | 2 | 330 |
| ('4g', 'child_prototypical', 'train') | 212 | 2057 |
| ('4g', 'child_prototypical', 'validation') | 46 | 435 |
| ('4h', 'child', 'train') | 8 | 1326 |
| ('4h', 'child', 'validation') | 2 | 331 |
| ('4h', 'child_prototypical', 'train') | 170 | 1787 |
| ('4h', 'child_prototypical', 'validation') | 52 | 525 |
| ('5', 'parent', 'train') | 72 | 560 |
| ('5', 'parent', 'validation') | 18 | 140 |
| ('5a', 'child', 'train') | 8 | 1324 |
| ('5a', 'child', 'validation') | 2 | 331 |
| ('5a', 'child_prototypical', 'train') | 202 | 1876 |
| ('5a', 'child_prototypical', 'validation') | 44 | 439 |
| ('5b', 'child', 'train') | 8 | 1329 |
| ('5b', 'child', 'validation') | 2 | 332 |
| ('5b', 'child_prototypical', 'train') | 140 | 1310 |
| ('5b', 'child_prototypical', 'validation') | 34 | 330 |
| ('5c', 'child', 'train') | 8 | 1327 |
| ('5c', 'child', 'validation') | 2 | 331 |
| ('5c', 'child_prototypical', 'train') | 170 | 1552 |
| ('5c', 'child_prototypical', 'validation') | 40 | 373 |
| ('5d', 'child', 'train') | 8 | 1324 |
| ('5d', 'child', 'validation') | 2 | 330 |
| ('5d', 'child_prototypical', 'train') | 204 | 1783 |
| ('5d', 'child_prototypical', 'validation') | 54 | 580 |
| ('5e', 'child', 'train') | 8 | 1329 |
| ('5e', 'child', 'validation') | 2 | 332 |
| ('5e', 'child_prototypical', 'train') | 136 | 1283 |
| ('5e', 'child_prototypical', 'validation') | 38 | 357 |
| ('5f', 'child', 'train') | 8 | 1327 |
| ('5f', 'child', 'validation') | 2 | 331 |
| ('5f', 'child_prototypical', 'train') | 154 | 1568 |
| ('5f', 'child_prototypical', 'validation') | 56 | 567 |
| ('5g', 'child', 'train') | 8 | 1328 |
| ('5g', 'child', 'validation') | 2 | 332 |
| ('5g', 'child_prototypical', 'train') | 158 | 1626 |
| ('5g', 'child_prototypical', 'validation') | 28 | 266 |
| ('5h', 'child', 'train') | 8 | 1324 |
| ('5h', 'child', 'validation') | 2 | 330 |
| ('5h', 'child_prototypical', 'train') | 218 | 2348 |
| ('5h', 'child_prototypical', 'validation') | 40 | 402 |
| ('5i', 'child', 'train') | 8 | 1324 |
| ('5i', 'child', 'validation') | 2 | 331 |
| ('5i', 'child_prototypical', 'train') | 192 | 2010 |
| ('5i', 'child_prototypical', 'validation') | 54 | 551 |
| ('6', 'parent', 'train') | 64 | 568 |
| ('6', 'parent', 'validation') | 16 | 142 |
| ('6a', 'child', 'train') | 8 | 1324 |
| ('6a', 'child', 'validation') | 2 | 330 |
| ('6a', 'child_prototypical', 'train') | 204 | 1962 |
| ('6a', 'child_prototypical', 'validation') | 54 | 530 |
| ('6b', 'child', 'train') | 8 | 1327 |
| ('6b', 'child', 'validation') | 2 | 331 |
| ('6b', 'child_prototypical', 'train') | 180 | 1840 |
| ('6b', 'child_prototypical', 'validation') | 30 | 295 |
| ('6c', 'child', 'train') | 8 | 1325 |
| ('6c', 'child', 'validation') | 2 | 331 |
| ('6c', 'child_prototypical', 'train') | 180 | 1968 |
| ('6c', 'child_prototypical', 'validation') | 54 | 527 |
| ('6d', 'child', 'train') | 8 | 1328 |
| ('6d', 'child', 'validation') | 2 | 331 |
| ('6d', 'child_prototypical', 'train') | 164 | 1903 |
| ('6d', 'child_prototypical', 'validation') | 34 | 358 |
| ('6e', 'child', 'train') | 8 | 1327 |
| ('6e', 'child', 'validation') | 2 | 331 |
| ('6e', 'child_prototypical', 'train') | 170 | 1737 |
| ('6e', 'child_prototypical', 'validation') | 40 | 398 |
| ('6f', 'child', 'train') | 8 | 1326 |
| ('6f', 'child', 'validation') | 2 | 331 |
| ('6f', 'child_prototypical', 'train') | 174 | 1652 |
| ('6f', 'child_prototypical', 'validation') | 48 | 438 |
| ('6g', 'child', 'train') | 8 | 1326 |
| ('6g', 'child', 'validation') | 2 | 331 |
| ('6g', 'child_prototypical', 'train') | 188 | 1740 |
| ('6g', 'child_prototypical', 'validation') | 34 | 239 |
| ('6h', 'child', 'train') | 8 | 1324 |
| ('6h', 'child', 'validation') | 2 | 330 |
| ('6h', 'child_prototypical', 'train') | 230 | 2337 |
| ('6h', 'child_prototypical', 'validation') | 28 | 284 |
| ('7', 'parent', 'train') | 64 | 568 |
| ('7', 'parent', 'validation') | 16 | 142 |
| ('7a', 'child', 'train') | 8 | 1324 |
| ('7a', 'child', 'validation') | 2 | 331 |
| ('7a', 'child_prototypical', 'train') | 198 | 2045 |
| ('7a', 'child_prototypical', 'validation') | 48 | 516 |
| ('7b', 'child', 'train') | 8 | 1330 |
| ('7b', 'child', 'validation') | 2 | 332 |
| ('7b', 'child_prototypical', 'train') | 138 | 905 |
| ('7b', 'child_prototypical', 'validation') | 24 | 177 |
| ('7c', 'child', 'train') | 8 | 1327 |
| ('7c', 'child', 'validation') | 2 | 331 |
| ('7c', 'child_prototypical', 'train') | 170 | 1402 |
| ('7c', 'child_prototypical', 'validation') | 40 | 313 |
| ('7d', 'child', 'train') | 8 | 1324 |
| ('7d', 'child', 'validation') | 2 | 331 |
| ('7d', 'child_prototypical', 'train') | 196 | 2064 |
| ('7d', 'child_prototypical', 'validation') | 50 | 497 |
| ('7e', 'child', 'train') | 8 | 1328 |
| ('7e', 'child', 'validation') | 2 | 331 |
| ('7e', 'child_prototypical', 'train') | 156 | 1270 |
| ('7e', 'child_prototypical', 'validation') | 42 | 298 |
| ('7f', 'child', 'train') | 8 | 1326 |
| ('7f', 'child', 'validation') | 2 | 331 |
| ('7f', 'child_prototypical', 'train') | 178 | 1377 |
| ('7f', 'child_prototypical', 'validation') | 44 | 380 |
| ('7g', 'child', 'train') | 8 | 1328 |
| ('7g', 'child', 'validation') | 2 | 332 |
| ('7g', 'child_prototypical', 'train') | 144 | 885 |
| ('7g', 'child_prototypical', 'validation') | 42 | 263 |
| ('7h', 'child', 'train') | 8 | 1324 |
| ('7h', 'child', 'validation') | 2 | 331 |
| ('7h', 'child_prototypical', 'train') | 188 | 1479 |
| ('7h', 'child_prototypical', 'validation') | 58 | 467 |
| ('8', 'parent', 'train') | 64 | 568 |
| ('8', 'parent', 'validation') | 16 | 142 |
| ('8a', 'child', 'train') | 8 | 1324 |
| ('8a', 'child', 'validation') | 2 | 331 |
| ('8a', 'child_prototypical', 'train') | 186 | 1640 |
| ('8a', 'child_prototypical', 'validation') | 60 | 552 |
| ('8b', 'child', 'train') | 8 | 1330 |
| ('8b', 'child', 'validation') | 2 | 332 |
| ('8b', 'child_prototypical', 'train') | 122 | 1126 |
| ('8b', 'child_prototypical', 'validation') | 40 | 361 |
| ('8c', 'child', 'train') | 8 | 1326 |
| ('8c', 'child', 'validation') | 2 | 331 |
| ('8c', 'child_prototypical', 'train') | 192 | 1547 |
| ('8c', 'child_prototypical', 'validation') | 30 | 210 |
| ('8d', 'child', 'train') | 8 | 1325 |
| ('8d', 'child', 'validation') | 2 | 331 |
| ('8d', 'child_prototypical', 'train') | 184 | 1472 |
| ('8d', 'child_prototypical', 'validation') | 50 | 438 |
| ('8e', 'child', 'train') | 8 | 1327 |
| ('8e', 'child', 'validation') | 2 | 331 |
| ('8e', 'child_prototypical', 'train') | 174 | 1340 |
| ('8e', 'child_prototypical', 'validation') | 36 | 270 |
| ('8f', 'child', 'train') | 8 | 1326 |
| ('8f', 'child', 'validation') | 2 | 331 |
| ('8f', 'child_prototypical', 'train') | 166 | 1416 |
| ('8f', 'child_prototypical', 'validation') | 56 | 452 |
| ('8g', 'child', 'train') | 8 | 1330 |
| ('8g', 'child', 'validation') | 2 | 332 |
| ('8g', 'child_prototypical', 'train') | 124 | 640 |
| ('8g', 'child_prototypical', 'validation') | 38 | 199 |
| ('8h', 'child', 'train') | 8 | 1324 |
| ('8h', 'child', 'validation') | 2 | 331 |
| ('8h', 'child_prototypical', 'train') | 200 | 1816 |
| ('8h', 'child_prototypical', 'validation') | 46 | 499 |
| ('9', 'parent', 'train') | 72 | 560 |
| ('9', 'parent', 'validation') | 18 | 140 |
| ('9a', 'child', 'train') | 8 | 1324 |
| ('9a', 'child', 'validation') | 2 | 331 |
| ('9a', 'child_prototypical', 'train') | 192 | 1520 |
| ('9a', 'child_prototypical', 'validation') | 54 | 426 |
| ('9b', 'child', 'train') | 8 | 1326 |
| ('9b', 'child', 'validation') | 2 | 331 |
| ('9b', 'child_prototypical', 'train') | 186 | 1783 |
| ('9b', 'child_prototypical', 'validation') | 36 | 307 |
| ('9c', 'child', 'train') | 8 | 1330 |
| ('9c', 'child', 'validation') | 2 | 332 |
| ('9c', 'child_prototypical', 'train') | 118 | 433 |
| ('9c', 'child_prototypical', 'validation') | 44 | 163 |
| ('9d', 'child', 'train') | 8 | 1328 |
| ('9d', 'child', 'validation') | 2 | 332 |
| ('9d', 'child_prototypical', 'train') | 156 | 1683 |
| ('9d', 'child_prototypical', 'validation') | 30 | 302 |
| ('9e', 'child', 'train') | 8 | 1329 |
| ('9e', 'child', 'validation') | 2 | 332 |
| ('9e', 'child_prototypical', 'train') | 132 | 1426 |
| ('9e', 'child_prototypical', 'validation') | 42 | 475 |
| ('9f', 'child', 'train') | 8 | 1328 |
| ('9f', 'child', 'validation') | 2 | 331 |
| ('9f', 'child_prototypical', 'train') | 158 | 1436 |
| ('9f', 'child_prototypical', 'validation') | 40 | 330 |
| ('9g', 'child', 'train') | 8 | 1324 |
| ('9g', 'child', 'validation') | 2 | 331 |
| ('9g', 'child_prototypical', 'train') | 200 | 1685 |
| ('9g', 'child_prototypical', 'validation') | 46 | 384 |
| ('9h', 'child', 'train') | 8 | 1325 |
| ('9h', 'child', 'validation') | 2 | 331 |
| ('9h', 'child_prototypical', 'train') | 190 | 1799 |
| ('9h', 'child_prototypical', 'validation') | 44 | 462 |
| ('9i', 'child', 'train') | 8 | 1328 |
| ('9i', 'child', 'validation') | 2 | 332 |
| ('9i', 'child_prototypical', 'train') | 158 | 1361 |
| ('9i', 'child_prototypical', 'validation') | 28 | 252 |
### Citation Information
```
@inproceedings{jurgens-etal-2012-semeval,
title = "{S}em{E}val-2012 Task 2: Measuring Degrees of Relational Similarity",
author = "Jurgens, David and
Mohammad, Saif and
Turney, Peter and
Holyoak, Keith",
booktitle = "*{SEM} 2012: The First Joint Conference on Lexical and Computational Semantics {--} Volume 1: Proceedings of the main conference and the shared task, and Volume 2: Proceedings of the Sixth International Workshop on Semantic Evaluation ({S}em{E}val 2012)",
month = "7-8 " # jun,
year = "2012",
address = "Montr{\'e}al, Canada",
publisher = "Association for Computational Linguistics",
url = "https://aclanthology.org/S12-1047",
pages = "356--364",
}
``` | research-backup/semeval2012_relational_similarity_v6 | [
"multilinguality:monolingual",
"size_categories:1K<n<10K",
"language:en",
"license:other",
"region:us"
] | 2022-11-20T11:14:56+00:00 | {"language": ["en"], "license": ["other"], "multilinguality": ["monolingual"], "size_categories": ["1K<n<10K"], "pretty_name": "SemEval2012 task 2 Relational Similarity"} | 2022-11-20T11:30:10+00:00 | [] | [
"en"
] | TAGS
#multilinguality-monolingual #size_categories-1K<n<10K #language-English #license-other #region-us
| Dataset Card for "relbert/semeval2012\_relational\_similarity\_V6"
==================================================================
Dataset Description
-------------------
* Repository: RelBERT
* Paper: URL
* Dataset: SemEval2012: Relational Similarity
### Dataset Summary
*IMPORTANT*: This is the same dataset as relbert/semeval2012\_relational\_similarity,
but with a different dataset construction.
Relational similarity dataset from SemEval2012 task 2, compiled to fine-tune RelBERT model.
The dataset contains a list of positive and negative word pair from 89 pre-defined relations.
The relation types are constructed on top of following 10 parent relation types.
Each of the parent relation is further grouped into child relation types where the definition can be found here.
Dataset Structure
-----------------
### Data Instances
An example of 'train' looks as follows.
### Data Splits
### Number of Positive/Negative Word-pairs in each Split
| [
"### Dataset Summary\n\n\n*IMPORTANT*: This is the same dataset as relbert/semeval2012\\_relational\\_similarity,\nbut with a different dataset construction.\n\n\nRelational similarity dataset from SemEval2012 task 2, compiled to fine-tune RelBERT model.\nThe dataset contains a list of positive and negative word pair from 89 pre-defined relations.\nThe relation types are constructed on top of following 10 parent relation types.\n\n\nEach of the parent relation is further grouped into child relation types where the definition can be found here.\n\n\nDataset Structure\n-----------------",
"### Data Instances\n\n\nAn example of 'train' looks as follows.",
"### Data Splits",
"### Number of Positive/Negative Word-pairs in each Split"
] | [
"TAGS\n#multilinguality-monolingual #size_categories-1K<n<10K #language-English #license-other #region-us \n",
"### Dataset Summary\n\n\n*IMPORTANT*: This is the same dataset as relbert/semeval2012\\_relational\\_similarity,\nbut with a different dataset construction.\n\n\nRelational similarity dataset from SemEval2012 task 2, compiled to fine-tune RelBERT model.\nThe dataset contains a list of positive and negative word pair from 89 pre-defined relations.\nThe relation types are constructed on top of following 10 parent relation types.\n\n\nEach of the parent relation is further grouped into child relation types where the definition can be found here.\n\n\nDataset Structure\n-----------------",
"### Data Instances\n\n\nAn example of 'train' looks as follows.",
"### Data Splits",
"### Number of Positive/Negative Word-pairs in each Split"
] |
3ca4be9c651bf7b10b8742b048bef587c01a7a5d | # Dataset Card for "relbert/semeval2012_relational_similarity_V7"
## Dataset Description
- **Repository:** [RelBERT](https://github.com/asahi417/relbert)
- **Paper:** [https://aclanthology.org/S12-1047/](https://aclanthology.org/S12-1047/)
- **Dataset:** SemEval2012: Relational Similarity
### Dataset Summary
***IMPORTANT***: This is the same dataset as [relbert/semeval2012_relational_similarity](https://huggingface.co/datasets/relbert/semeval2012_relational_similarity),
but with a different dataset construction.
Relational similarity dataset from [SemEval2012 task 2](https://aclanthology.org/S12-1047/), compiled to fine-tune [RelBERT](https://github.com/asahi417/relbert) model.
The dataset contains a list of positive and negative word pair from 89 pre-defined relations.
The relation types are constructed on top of following 10 parent relation types.
```shell
{
1: "Class Inclusion", # Hypernym
2: "Part-Whole", # Meronym, Substance Meronym
3: "Similar", # Synonym, Co-hypornym
4: "Contrast", # Antonym
5: "Attribute", # Attribute, Event
6: "Non Attribute",
7: "Case Relation",
8: "Cause-Purpose",
9: "Space-Time",
10: "Representation"
}
```
Each of the parent relation is further grouped into child relation types where the definition can be found [here](https://drive.google.com/file/d/0BzcZKTSeYL8VenY0QkVpZVpxYnc/view?resourcekey=0-ZP-UARfJj39PcLroibHPHw).
## Dataset Structure
### Data Instances
An example of `train` looks as follows.
```
{
'relation_type': '8d',
'positives': [ [ "breathe", "live" ], [ "study", "learn" ], [ "speak", "communicate" ], ... ]
'negatives': [ [ "starving", "hungry" ], [ "clean", "bathe" ], [ "hungry", "starving" ], ... ]
}
```
### Data Splits
| name |train|validation|
|---------|----:|---------:|
|semeval2012_relational_similarity| 89 | 89|
### Number of Positive/Negative Word-pairs in each Split
| | positives | negatives |
|:------------------------------------------|------------:|------------:|
| ('1', 'parent', 'train') | 110 | 680 |
| ('10', 'parent', 'train') | 60 | 730 |
| ('10a', 'child', 'train') | 10 | 1655 |
| ('10a', 'child_prototypical', 'train') | 246 | 2438 |
| ('10b', 'child', 'train') | 10 | 1656 |
| ('10b', 'child_prototypical', 'train') | 234 | 2027 |
| ('10c', 'child', 'train') | 10 | 1658 |
| ('10c', 'child_prototypical', 'train') | 210 | 2030 |
| ('10d', 'child', 'train') | 10 | 1659 |
| ('10d', 'child_prototypical', 'train') | 198 | 1766 |
| ('10e', 'child', 'train') | 10 | 1661 |
| ('10e', 'child_prototypical', 'train') | 174 | 1118 |
| ('10f', 'child', 'train') | 10 | 1659 |
| ('10f', 'child_prototypical', 'train') | 198 | 1766 |
| ('1a', 'child', 'train') | 10 | 1655 |
| ('1a', 'child_prototypical', 'train') | 246 | 2192 |
| ('1b', 'child', 'train') | 10 | 1655 |
| ('1b', 'child_prototypical', 'train') | 246 | 2192 |
| ('1c', 'child', 'train') | 10 | 1658 |
| ('1c', 'child_prototypical', 'train') | 210 | 2030 |
| ('1d', 'child', 'train') | 10 | 1653 |
| ('1d', 'child_prototypical', 'train') | 270 | 2540 |
| ('1e', 'child', 'train') | 10 | 1661 |
| ('1e', 'child_prototypical', 'train') | 174 | 1031 |
| ('2', 'parent', 'train') | 100 | 690 |
| ('2a', 'child', 'train') | 10 | 1654 |
| ('2a', 'child_prototypical', 'train') | 258 | 2621 |
| ('2b', 'child', 'train') | 10 | 1658 |
| ('2b', 'child_prototypical', 'train') | 210 | 1610 |
| ('2c', 'child', 'train') | 10 | 1656 |
| ('2c', 'child_prototypical', 'train') | 234 | 2144 |
| ('2d', 'child', 'train') | 10 | 1659 |
| ('2d', 'child_prototypical', 'train') | 198 | 1667 |
| ('2e', 'child', 'train') | 10 | 1658 |
| ('2e', 'child_prototypical', 'train') | 210 | 1925 |
| ('2f', 'child', 'train') | 10 | 1658 |
| ('2f', 'child_prototypical', 'train') | 210 | 2240 |
| ('2g', 'child', 'train') | 10 | 1653 |
| ('2g', 'child_prototypical', 'train') | 270 | 2405 |
| ('2h', 'child', 'train') | 10 | 1658 |
| ('2h', 'child_prototypical', 'train') | 210 | 1925 |
| ('2i', 'child', 'train') | 10 | 1660 |
| ('2i', 'child_prototypical', 'train') | 186 | 1706 |
| ('2j', 'child', 'train') | 10 | 1659 |
| ('2j', 'child_prototypical', 'train') | 198 | 1964 |
| ('3', 'parent', 'train') | 80 | 710 |
| ('3a', 'child', 'train') | 10 | 1658 |
| ('3a', 'child_prototypical', 'train') | 210 | 1925 |
| ('3b', 'child', 'train') | 10 | 1658 |
| ('3b', 'child_prototypical', 'train') | 210 | 2240 |
| ('3c', 'child', 'train') | 10 | 1657 |
| ('3c', 'child_prototypical', 'train') | 222 | 1979 |
| ('3d', 'child', 'train') | 10 | 1655 |
| ('3d', 'child_prototypical', 'train') | 246 | 2315 |
| ('3e', 'child', 'train') | 10 | 1664 |
| ('3e', 'child_prototypical', 'train') | 138 | 1268 |
| ('3f', 'child', 'train') | 10 | 1658 |
| ('3f', 'child_prototypical', 'train') | 210 | 2345 |
| ('3g', 'child', 'train') | 10 | 1663 |
| ('3g', 'child_prototypical', 'train') | 150 | 1340 |
| ('3h', 'child', 'train') | 10 | 1659 |
| ('3h', 'child_prototypical', 'train') | 198 | 1964 |
| ('4', 'parent', 'train') | 80 | 710 |
| ('4a', 'child', 'train') | 10 | 1658 |
| ('4a', 'child_prototypical', 'train') | 210 | 2240 |
| ('4b', 'child', 'train') | 10 | 1662 |
| ('4b', 'child_prototypical', 'train') | 162 | 1163 |
| ('4c', 'child', 'train') | 10 | 1657 |
| ('4c', 'child_prototypical', 'train') | 222 | 2201 |
| ('4d', 'child', 'train') | 10 | 1665 |
| ('4d', 'child_prototypical', 'train') | 126 | 749 |
| ('4e', 'child', 'train') | 10 | 1657 |
| ('4e', 'child_prototypical', 'train') | 222 | 2423 |
| ('4f', 'child', 'train') | 10 | 1660 |
| ('4f', 'child_prototypical', 'train') | 186 | 1892 |
| ('4g', 'child', 'train') | 10 | 1654 |
| ('4g', 'child_prototypical', 'train') | 258 | 2492 |
| ('4h', 'child', 'train') | 10 | 1657 |
| ('4h', 'child_prototypical', 'train') | 222 | 2312 |
| ('5', 'parent', 'train') | 90 | 700 |
| ('5a', 'child', 'train') | 10 | 1655 |
| ('5a', 'child_prototypical', 'train') | 246 | 2315 |
| ('5b', 'child', 'train') | 10 | 1661 |
| ('5b', 'child_prototypical', 'train') | 174 | 1640 |
| ('5c', 'child', 'train') | 10 | 1658 |
| ('5c', 'child_prototypical', 'train') | 210 | 1925 |
| ('5d', 'child', 'train') | 10 | 1654 |
| ('5d', 'child_prototypical', 'train') | 258 | 2363 |
| ('5e', 'child', 'train') | 10 | 1661 |
| ('5e', 'child_prototypical', 'train') | 174 | 1640 |
| ('5f', 'child', 'train') | 10 | 1658 |
| ('5f', 'child_prototypical', 'train') | 210 | 2135 |
| ('5g', 'child', 'train') | 10 | 1660 |
| ('5g', 'child_prototypical', 'train') | 186 | 1892 |
| ('5h', 'child', 'train') | 10 | 1654 |
| ('5h', 'child_prototypical', 'train') | 258 | 2750 |
| ('5i', 'child', 'train') | 10 | 1655 |
| ('5i', 'child_prototypical', 'train') | 246 | 2561 |
| ('6', 'parent', 'train') | 80 | 710 |
| ('6a', 'child', 'train') | 10 | 1654 |
| ('6a', 'child_prototypical', 'train') | 258 | 2492 |
| ('6b', 'child', 'train') | 10 | 1658 |
| ('6b', 'child_prototypical', 'train') | 210 | 2135 |
| ('6c', 'child', 'train') | 10 | 1656 |
| ('6c', 'child_prototypical', 'train') | 234 | 2495 |
| ('6d', 'child', 'train') | 10 | 1659 |
| ('6d', 'child_prototypical', 'train') | 198 | 2261 |
| ('6e', 'child', 'train') | 10 | 1658 |
| ('6e', 'child_prototypical', 'train') | 210 | 2135 |
| ('6f', 'child', 'train') | 10 | 1657 |
| ('6f', 'child_prototypical', 'train') | 222 | 2090 |
| ('6g', 'child', 'train') | 10 | 1657 |
| ('6g', 'child_prototypical', 'train') | 222 | 1979 |
| ('6h', 'child', 'train') | 10 | 1654 |
| ('6h', 'child_prototypical', 'train') | 258 | 2621 |
| ('7', 'parent', 'train') | 80 | 710 |
| ('7a', 'child', 'train') | 10 | 1655 |
| ('7a', 'child_prototypical', 'train') | 246 | 2561 |
| ('7b', 'child', 'train') | 10 | 1662 |
| ('7b', 'child_prototypical', 'train') | 162 | 1082 |
| ('7c', 'child', 'train') | 10 | 1658 |
| ('7c', 'child_prototypical', 'train') | 210 | 1715 |
| ('7d', 'child', 'train') | 10 | 1655 |
| ('7d', 'child_prototypical', 'train') | 246 | 2561 |
| ('7e', 'child', 'train') | 10 | 1659 |
| ('7e', 'child_prototypical', 'train') | 198 | 1568 |
| ('7f', 'child', 'train') | 10 | 1657 |
| ('7f', 'child_prototypical', 'train') | 222 | 1757 |
| ('7g', 'child', 'train') | 10 | 1660 |
| ('7g', 'child_prototypical', 'train') | 186 | 1148 |
| ('7h', 'child', 'train') | 10 | 1655 |
| ('7h', 'child_prototypical', 'train') | 246 | 1946 |
| ('8', 'parent', 'train') | 80 | 710 |
| ('8a', 'child', 'train') | 10 | 1655 |
| ('8a', 'child_prototypical', 'train') | 246 | 2192 |
| ('8b', 'child', 'train') | 10 | 1662 |
| ('8b', 'child_prototypical', 'train') | 162 | 1487 |
| ('8c', 'child', 'train') | 10 | 1657 |
| ('8c', 'child_prototypical', 'train') | 222 | 1757 |
| ('8d', 'child', 'train') | 10 | 1656 |
| ('8d', 'child_prototypical', 'train') | 234 | 1910 |
| ('8e', 'child', 'train') | 10 | 1658 |
| ('8e', 'child_prototypical', 'train') | 210 | 1610 |
| ('8f', 'child', 'train') | 10 | 1657 |
| ('8f', 'child_prototypical', 'train') | 222 | 1868 |
| ('8g', 'child', 'train') | 10 | 1662 |
| ('8g', 'child_prototypical', 'train') | 162 | 839 |
| ('8h', 'child', 'train') | 10 | 1655 |
| ('8h', 'child_prototypical', 'train') | 246 | 2315 |
| ('9', 'parent', 'train') | 90 | 700 |
| ('9a', 'child', 'train') | 10 | 1655 |
| ('9a', 'child_prototypical', 'train') | 246 | 1946 |
| ('9b', 'child', 'train') | 10 | 1657 |
| ('9b', 'child_prototypical', 'train') | 222 | 2090 |
| ('9c', 'child', 'train') | 10 | 1662 |
| ('9c', 'child_prototypical', 'train') | 162 | 596 |
| ('9d', 'child', 'train') | 10 | 1660 |
| ('9d', 'child_prototypical', 'train') | 186 | 1985 |
| ('9e', 'child', 'train') | 10 | 1661 |
| ('9e', 'child_prototypical', 'train') | 174 | 1901 |
| ('9f', 'child', 'train') | 10 | 1659 |
| ('9f', 'child_prototypical', 'train') | 198 | 1766 |
| ('9g', 'child', 'train') | 10 | 1655 |
| ('9g', 'child_prototypical', 'train') | 246 | 2069 |
| ('9h', 'child', 'train') | 10 | 1656 |
| ('9h', 'child_prototypical', 'train') | 234 | 2261 |
| ('9i', 'child', 'train') | 10 | 1660 |
| ('9i', 'child_prototypical', 'train') | 186 | 1613 |
| ('AtLocation', 'N/A', 'validation') | 960 | 4646 |
| ('CapableOf', 'N/A', 'validation') | 536 | 4734 |
| ('Causes', 'N/A', 'validation') | 194 | 4738 |
| ('CausesDesire', 'N/A', 'validation') | 40 | 4730 |
| ('CreatedBy', 'N/A', 'validation') | 4 | 3554 |
| ('DefinedAs', 'N/A', 'validation') | 4 | 1182 |
| ('Desires', 'N/A', 'validation') | 56 | 4732 |
| ('HasA', 'N/A', 'validation') | 168 | 4772 |
| ('HasFirstSubevent', 'N/A', 'validation') | 4 | 3554 |
| ('HasLastSubevent', 'N/A', 'validation') | 10 | 4732 |
| ('HasPrerequisite', 'N/A', 'validation') | 450 | 4744 |
| ('HasProperty', 'N/A', 'validation') | 266 | 4766 |
| ('HasSubevent', 'N/A', 'validation') | 330 | 4768 |
| ('IsA', 'N/A', 'validation') | 816 | 4688 |
| ('MadeOf', 'N/A', 'validation') | 48 | 4726 |
| ('MotivatedByGoal', 'N/A', 'validation') | 50 | 4736 |
| ('PartOf', 'N/A', 'validation') | 82 | 4742 |
| ('ReceivesAction', 'N/A', 'validation') | 52 | 4726 |
| ('SymbolOf', 'N/A', 'validation') | 4 | 1184 |
| ('UsedFor', 'N/A', 'validation') | 660 | 4760 |
### Citation Information
```
@inproceedings{jurgens-etal-2012-semeval,
title = "{S}em{E}val-2012 Task 2: Measuring Degrees of Relational Similarity",
author = "Jurgens, David and
Mohammad, Saif and
Turney, Peter and
Holyoak, Keith",
booktitle = "*{SEM} 2012: The First Joint Conference on Lexical and Computational Semantics {--} Volume 1: Proceedings of the main conference and the shared task, and Volume 2: Proceedings of the Sixth International Workshop on Semantic Evaluation ({S}em{E}val 2012)",
month = "7-8 " # jun,
year = "2012",
address = "Montr{\'e}al, Canada",
publisher = "Association for Computational Linguistics",
url = "https://aclanthology.org/S12-1047",
pages = "356--364",
}
``` | research-backup/semeval2012_relational_similarity_v7 | [
"multilinguality:monolingual",
"size_categories:1K<n<10K",
"language:en",
"license:other",
"region:us"
] | 2022-11-20T11:42:11+00:00 | {"language": ["en"], "license": ["other"], "multilinguality": ["monolingual"], "size_categories": ["1K<n<10K"], "pretty_name": "SemEval2012 task 2 Relational Similarity"} | 2022-11-20T11:49:41+00:00 | [] | [
"en"
] | TAGS
#multilinguality-monolingual #size_categories-1K<n<10K #language-English #license-other #region-us
| Dataset Card for "relbert/semeval2012\_relational\_similarity\_V7"
==================================================================
Dataset Description
-------------------
* Repository: RelBERT
* Paper: URL
* Dataset: SemEval2012: Relational Similarity
### Dataset Summary
*IMPORTANT*: This is the same dataset as relbert/semeval2012\_relational\_similarity,
but with a different dataset construction.
Relational similarity dataset from SemEval2012 task 2, compiled to fine-tune RelBERT model.
The dataset contains a list of positive and negative word pair from 89 pre-defined relations.
The relation types are constructed on top of following 10 parent relation types.
Each of the parent relation is further grouped into child relation types where the definition can be found here.
Dataset Structure
-----------------
### Data Instances
An example of 'train' looks as follows.
### Data Splits
### Number of Positive/Negative Word-pairs in each Split
| [
"### Dataset Summary\n\n\n*IMPORTANT*: This is the same dataset as relbert/semeval2012\\_relational\\_similarity,\nbut with a different dataset construction.\n\n\nRelational similarity dataset from SemEval2012 task 2, compiled to fine-tune RelBERT model.\nThe dataset contains a list of positive and negative word pair from 89 pre-defined relations.\nThe relation types are constructed on top of following 10 parent relation types.\n\n\nEach of the parent relation is further grouped into child relation types where the definition can be found here.\n\n\nDataset Structure\n-----------------",
"### Data Instances\n\n\nAn example of 'train' looks as follows.",
"### Data Splits",
"### Number of Positive/Negative Word-pairs in each Split"
] | [
"TAGS\n#multilinguality-monolingual #size_categories-1K<n<10K #language-English #license-other #region-us \n",
"### Dataset Summary\n\n\n*IMPORTANT*: This is the same dataset as relbert/semeval2012\\_relational\\_similarity,\nbut with a different dataset construction.\n\n\nRelational similarity dataset from SemEval2012 task 2, compiled to fine-tune RelBERT model.\nThe dataset contains a list of positive and negative word pair from 89 pre-defined relations.\nThe relation types are constructed on top of following 10 parent relation types.\n\n\nEach of the parent relation is further grouped into child relation types where the definition can be found here.\n\n\nDataset Structure\n-----------------",
"### Data Instances\n\n\nAn example of 'train' looks as follows.",
"### Data Splits",
"### Number of Positive/Negative Word-pairs in each Split"
] |
7287f4fdbaa8ecb13a8b4e6acdc299afe355e25a | # Dataset Card for AutoTrain Evaluator
This repository contains model predictions generated by [AutoTrain](https://huggingface.co/autotrain) for the following task and dataset:
* Task: Binary Text Classification
* Model: autoevaluate/binary-classification
* Dataset: glue
* Config: sst2
* Split: validation
To run new evaluation jobs, visit Hugging Face's [automatic model evaluator](https://huggingface.co/spaces/autoevaluate/model-evaluator).
## Contributions
Thanks to [@lewtun](https://huggingface.co/lewtun) for evaluating this model. | autoevaluate/autoeval-staging-eval-project-37b497c4-c065-4454-9a21-53d55a38d3d3-2826 | [
"autotrain",
"evaluation",
"region:us"
] | 2022-11-20T13:02:16+00:00 | {"type": "predictions", "tags": ["autotrain", "evaluation"], "datasets": ["glue"], "eval_info": {"task": "binary_classification", "model": "autoevaluate/binary-classification", "metrics": ["matthews_correlation"], "dataset_name": "glue", "dataset_config": "sst2", "dataset_split": "validation", "col_mapping": {"text": "sentence", "target": "label"}}} | 2022-11-20T13:02:54+00:00 | [] | [] | TAGS
#autotrain #evaluation #region-us
| # Dataset Card for AutoTrain Evaluator
This repository contains model predictions generated by AutoTrain for the following task and dataset:
* Task: Binary Text Classification
* Model: autoevaluate/binary-classification
* Dataset: glue
* Config: sst2
* Split: validation
To run new evaluation jobs, visit Hugging Face's automatic model evaluator.
## Contributions
Thanks to @lewtun for evaluating this model. | [
"# Dataset Card for AutoTrain Evaluator\n\nThis repository contains model predictions generated by AutoTrain for the following task and dataset:\n\n* Task: Binary Text Classification\n* Model: autoevaluate/binary-classification\n* Dataset: glue\n* Config: sst2\n* Split: validation\n\nTo run new evaluation jobs, visit Hugging Face's automatic model evaluator.",
"## Contributions\n\nThanks to @lewtun for evaluating this model."
] | [
"TAGS\n#autotrain #evaluation #region-us \n",
"# Dataset Card for AutoTrain Evaluator\n\nThis repository contains model predictions generated by AutoTrain for the following task and dataset:\n\n* Task: Binary Text Classification\n* Model: autoevaluate/binary-classification\n* Dataset: glue\n* Config: sst2\n* Split: validation\n\nTo run new evaluation jobs, visit Hugging Face's automatic model evaluator.",
"## Contributions\n\nThanks to @lewtun for evaluating this model."
] |
95c97f926a6acdebfa7392b75b0d9c80014851f3 | # Dataset Card for "text_summarization_dataset5"
[More Information needed](https://github.com/huggingface/datasets/blob/main/CONTRIBUTING.md#how-to-contribute-to-the-dataset-cards) | shahidul034/text_summarization_dataset5 | [
"region:us"
] | 2022-11-20T13:07:21+00:00 | {"dataset_info": {"features": [{"name": "title", "dtype": "string"}, {"name": "content", "dtype": "string"}], "splits": [{"name": "train", "num_bytes": 144216578, "num_examples": 129922}], "download_size": 49285071, "dataset_size": 144216578}} | 2022-11-20T13:07:26+00:00 | [] | [] | TAGS
#region-us
| # Dataset Card for "text_summarization_dataset5"
More Information needed | [
"# Dataset Card for \"text_summarization_dataset5\"\n\nMore Information needed"
] | [
"TAGS\n#region-us \n",
"# Dataset Card for \"text_summarization_dataset5\"\n\nMore Information needed"
] |
Subsets and Splits
No community queries yet
The top public SQL queries from the community will appear here once available.