sha
stringlengths 40
40
| text
stringlengths 1
13.4M
| id
stringlengths 2
117
| tags
sequencelengths 1
7.91k
| created_at
stringlengths 25
25
| metadata
stringlengths 2
875k
| last_modified
stringlengths 25
25
| arxiv
sequencelengths 0
25
| languages
sequencelengths 0
7.91k
| tags_str
stringlengths 17
159k
| text_str
stringlengths 1
447k
| text_lists
sequencelengths 0
352
| processed_texts
sequencelengths 1
353
|
---|---|---|---|---|---|---|---|---|---|---|---|---|
d20b701b465db13f05b495c191abf0e4a0e02065 |
This is a dataset created using [vector-io](https://github.com/ai-northstar-tech/vector-io)
| aintech/vdf_PC_ANN_Fashion-MNIST_d784_euclidean | [
"vdf",
"vector-io",
"vector-dataset",
"vector-embeddings",
"region:us"
] | 2024-01-09T18:05:42+00:00 | {"tags": ["vdf", "vector-io", "vector-dataset", "vector-embeddings"], "dataset_info": {"features": [{"name": "id", "dtype": "string"}, {"name": "vector", "sequence": "float64"}], "splits": [{"name": "train", "num_bytes": 62838890, "num_examples": 10000}], "download_size": 5101858, "dataset_size": 62838890}, "configs": [{"config_name": "default", "data_files": [{"split": "train", "path": "data/train-*"}]}]} | 2024-01-10T12:12:56+00:00 | [] | [] | TAGS
#vdf #vector-io #vector-dataset #vector-embeddings #region-us
|
This is a dataset created using vector-io
| [] | [
"TAGS\n#vdf #vector-io #vector-dataset #vector-embeddings #region-us \n"
] |
4ef97d413d69dea3949358ce9a899162f3891b72 | # Dataset Card for "ultrafeedback_quality_binarized"
[More Information needed](https://github.com/huggingface/datasets/blob/main/CONTRIBUTING.md#how-to-contribute-to-the-dataset-cards) | jan-hq/ultrafeedback_quality_binarized | [
"region:us"
] | 2024-01-09T18:29:00+00:00 | {"configs": [{"config_name": "default", "data_files": [{"split": "train", "path": "data/train-*"}, {"split": "test", "path": "data/test-*"}]}], "dataset_info": {"features": [{"name": "source", "dtype": "string"}, {"name": "prompt", "dtype": "string"}, {"name": "chosen", "list": [{"name": "content", "dtype": "string"}, {"name": "role", "dtype": "string"}]}, {"name": "chosen-rating", "dtype": "float64"}, {"name": "chosen-model", "dtype": "string"}, {"name": "rejected", "list": [{"name": "content", "dtype": "string"}, {"name": "role", "dtype": "string"}]}, {"name": "rejected-rating", "dtype": "float64"}, {"name": "rejected-model", "dtype": "string"}], "splits": [{"name": "train", "num_bytes": 654240429.3032981, "num_examples": 139196}, {"name": "test", "num_bytes": 72697036.69670185, "num_examples": 15467}], "download_size": 396128426, "dataset_size": 726937466.0}} | 2024-01-09T18:29:37+00:00 | [] | [] | TAGS
#region-us
| # Dataset Card for "ultrafeedback_quality_binarized"
More Information needed | [
"# Dataset Card for \"ultrafeedback_quality_binarized\"\n\nMore Information needed"
] | [
"TAGS\n#region-us \n",
"# Dataset Card for \"ultrafeedback_quality_binarized\"\n\nMore Information needed"
] |
f96e32e2a1a64ab3b9aab8d67b9259a87e5203b9 | # Dataset Card for "parallel-pt-nl-pl"
[More Information needed](https://github.com/huggingface/datasets/blob/main/CONTRIBUTING.md#how-to-contribute-to-the-dataset-cards) | gowitheflowlab/parallel-pt-nl-pl | [
"region:us"
] | 2024-01-09T18:46:06+00:00 | {"configs": [{"config_name": "default", "data_files": [{"split": "train", "path": "data/train-*"}]}], "dataset_info": {"features": [{"name": "sentence1", "dtype": "string"}, {"name": "sentence2", "dtype": "string"}, {"name": "label", "dtype": "string"}], "splits": [{"name": "train", "num_bytes": 210221145.70946357, "num_examples": 1201407}], "download_size": 140654042, "dataset_size": 210221145.70946357}} | 2024-01-09T18:46:42+00:00 | [] | [] | TAGS
#region-us
| # Dataset Card for "parallel-pt-nl-pl"
More Information needed | [
"# Dataset Card for \"parallel-pt-nl-pl\"\n\nMore Information needed"
] | [
"TAGS\n#region-us \n",
"# Dataset Card for \"parallel-pt-nl-pl\"\n\nMore Information needed"
] |
e71a5b30eb0db9954addf9836cd99966afdb3a7b | # LegalPT
LegalPT aggregates the maximum amount of publicly available legal data in Portuguese, drawing from varied sources including legislation, jurisprudence, legal articles, and government documents.
## Dataset Details
Dataset is composed by six corpora:
[Ulysses-Tesemõ](https:github.com/ulysses-camara/ulysses-tesemo), [MultiLegalPile (PT)](https://arxiv.org/abs/2306.02069v2), [ParlamentoPT](http://arxiv.org/abs/2305.06721),
[Iudicium Textum](https://www.inf.ufpr.br/didonet/articles/2019_dsw_Iudicium_Textum_Dataset.pdf), [Acordãos TCU](https://link.springer.com/chapter/10.1007/978-3-030-61377-8_46), and
[DataSTF](https://legalhackersnatal.wordpress.com/2019/05/09/mais-dados-juridicos/).
- **MultiLegalPile**: a multilingual corpus of legal texts comprising 689 GiB of data, covering 24 languages in 17 jurisdictions. The corpus is separated by language, and the subset in Portuguese contains 92GiB of data, containing 13.76 billion words. This subset includes the jurisprudence of the Court of Justice of São Paulo (CJPG), appeals from the [5th Regional Federal Court (BRCAD-5)](https://journals.plos.org/plosone/article?id=10.1371/journal.pone.0272287), the Portuguese subset of legal documents from the European Union, known as [EUR-Lex](https://eur-lex.europa.eu/homepage.html), and a filter for legal documents from [MC4](http://arxiv.org/abs/2010.11934).
- **Ulysses-Tesemõ**: a legal corpus in Brazilian Portuguese, composed of 2.2 million documents, totaling about 26GiB of text obtained from 96 different data sources. These sources encompass legal, legislative, academic papers, news, and related comments. The data was collected through web scraping of government websites.
- **ParlamentoPT**: a corpus for training language models in European Portuguese. The data was collected from the Portuguese government portal and consists of 2.6 million documents of transcriptions of debates in the Portuguese Parliament.
- **Iudicium Textum**: consists of rulings, votes, and reports from the Supreme Federal Court (STF) of Brazil, published between 2010 and 2018. The dataset contains 1GiB of data extracted from PDFs.
- **Acordãos TCU**: an open dataset from the Tribunal de Contas da União (Brazilian Federal Court of Accounts), containing 600,000 documents obtained by web scraping government websites. The documents span from 1992 to 2019.
- **DataSTF**: a dataset of monocratic decisions from the Superior Court of Justice (STJ) in Brazil, containing 700,000 documents (5GiB of data).
### Dataset Description
- **Curated by:** [More Information Needed]
- **Funded by:** [More Information Needed]
- **Language(s) (NLP):** Brazilian Portuguese (pt-BR)
- **License:** [Creative Commons Attribution 4.0 International Public License](https://creativecommons.org/licenses/by/4.0/deed.en)
### Dataset Sources
- **Repository:** https://github.com/eduagarcia/roberta-legal-portuguese
- **Paper:** [More Information Needed]
## Dataset Structure
<!-- This section provides a description of the dataset fields, and additional information about the dataset structure such as criteria used to create the splits, relationships between data points, etc. -->
[More Information Needed]
## Data Collection and Processing
LegalPT is deduplicated using [MinHash algorithm](https://dl.acm.org/doi/abs/10.5555/647819.736184) and [Locality Sensitive Hashing](https://dspace.mit.edu/bitstream/handle/1721.1/134231/v008a014.pdf?sequence=2&isAllowed=y), following the approach of [Lee et al. (2022)](http://arxiv.org/abs/2107.06499).
We used 5-grams and a signature of size 256, considering two documents to be identical if their Jaccard Similarity exceeded 0.7.
Duplicate rate found by the Minhash-LSH algorithm for the LegalPT corpus:
| **Corpus** | **Documents** | **Docs. after deduplication** | **Duplicates (%)** |
|--------------------------|:--------------:|:-----------------------------:|:------------------:|
| Ulysses-Tesemõ | 2,216,656 | 1,737,720 | 21.61 |
| MultiLegalPile (PT) | | | |
| CJPG | 14,068,634 | 6,260,096 | 55.50 |
| BRCAD-5 | 3,128,292 | 542,680 | 82.65 |
| EUR-Lex (Caselaw) | 104,312 | 78,893 | 24.37 |
| EUR-Lex (Contracts) | 11,581 | 8,511 | 26.51 |
| EUR-Lex (Legislation) | 232,556 | 95,024 | 59.14 |
| Legal MC4 | 191,174 | 187,637 | 1.85 |
| ParlamentoPT | 2,670,846 | 2,109,931 | 21.00 |
| Iudicium Textum | 198,387 | 153,373 | 22.69 |
| Acordãos TCU | 634,711 | 462,031 | 27.21 |
| DataSTF | 737,769 | 310,119 | 57.97 |
| **Total (LegalPT)** | **24,194,918** | **11,946,015** | **50.63** |
## Citation
```bibtex
@InProceedings{garcia2024_roberlexpt,
author="Garcia, Eduardo A. S.
and Silva, N{\'a}dia F. F.
and Siqueira, Felipe
and Gomes, Juliana R. S.
and Albuqueruqe, Hidelberg O.
and Souza, Ellen
and Lima, Eliomar
and De Carvalho, André",
title="RoBERTaLexPT: A Legal RoBERTa Model pretrained with deduplication for Portuguese",
booktitle="Computational Processing of the Portuguese Language",
year="2024",
publisher="Association for Computational Linguistics"
}
```
## Acknowledgment
This work has been supported by the AI Center of Excellence (Centro de Excelência em Inteligência Artificial – CEIA) of the Institute of Informatics at the Federal University of Goiás (INF-UFG). | eduagarcia/LegalPT | [
"task_categories:text-generation",
"size_categories:10M<n<100M",
"language:pt",
"license:cc-by-4.0",
"legal",
"arxiv:2306.02069",
"arxiv:2305.06721",
"arxiv:2010.11934",
"arxiv:2107.06499",
"region:us"
] | 2024-01-09T19:03:24+00:00 | {"language": ["pt"], "license": "cc-by-4.0", "size_categories": ["10M<n<100M"], "task_categories": ["text-generation"], "tags": ["legal"], "dataset_info": [{"config_name": "acordaos_tcu", "features": [{"name": "id", "dtype": "int64"}, {"name": "text", "dtype": "string"}, {"name": "meta", "struct": [{"name": "dedup", "struct": [{"name": "exact_norm", "struct": [{"name": "cluster_main_idx", "dtype": "int64"}, {"name": "cluster_size", "dtype": "int64"}, {"name": "exact_hash_idx", "dtype": "int64"}, {"name": "is_duplicate", "dtype": "bool"}]}, {"name": "minhash", "struct": [{"name": "cluster_main_idx", "dtype": "int64"}, {"name": "cluster_size", "dtype": "int64"}, {"name": "is_duplicate", "dtype": "bool"}, {"name": "minhash_idx", "dtype": "int64"}]}]}]}], "splits": [{"name": "train", "num_bytes": 3494790013, "num_examples": 634711}], "download_size": 1653039356, "dataset_size": 3494790013}, {"config_name": "all", "features": [{"name": "text", "dtype": "string"}, {"name": "meta", "struct": [{"name": "dedup", "struct": [{"name": "exact_norm", "struct": [{"name": "cluster_main_idx", "dtype": "int64"}, {"name": "cluster_size", "dtype": "int64"}, {"name": "exact_hash_idx", "dtype": "int64"}, {"name": "is_duplicate", "dtype": "bool"}]}, {"name": "minhash", "struct": [{"name": "cluster_main_idx", "dtype": "int64"}, {"name": "cluster_size", "dtype": "int64"}, {"name": "is_duplicate", "dtype": "bool"}, {"name": "minhash_idx", "dtype": "int64"}]}]}]}, {"name": "source", "dtype": "string"}, {"name": "orig_id", "dtype": "int64"}, {"name": "id", "dtype": "int64"}], "splits": [{"name": "train", "num_bytes": 7047806791, "num_examples": 1399648}], "download_size": 3783112421, "dataset_size": 7047806791}, {"config_name": "datastf", "features": [{"name": "text", "dtype": "string"}, {"name": "meta", "struct": [{"name": "dedup", "struct": [{"name": "exact_norm", "struct": [{"name": "cluster_main_idx", "dtype": "int64"}, {"name": "cluster_size", "dtype": "int64"}, {"name": "exact_hash_idx", "dtype": "int64"}, {"name": "is_duplicate", "dtype": "bool"}]}, {"name": "minhash", "struct": [{"name": "cluster_main_idx", "dtype": "int64"}, {"name": "cluster_size", "dtype": "int64"}, {"name": "is_duplicate", "dtype": "bool"}, {"name": "minhash_idx", "dtype": "int64"}]}]}]}, {"name": "id", "dtype": "int64"}], "splits": [{"name": "train", "num_bytes": 3699382656, "num_examples": 737769}], "download_size": 1724245648, "dataset_size": 3699382656}, {"config_name": "iudicium_textum", "features": [{"name": "text", "dtype": "string"}, {"name": "meta", "struct": [{"name": "dedup", "struct": [{"name": "exact_norm", "struct": [{"name": "cluster_main_idx", "dtype": "int64"}, {"name": "cluster_size", "dtype": "int64"}, {"name": "exact_hash_idx", "dtype": "int64"}, {"name": "is_duplicate", "dtype": "bool"}]}, {"name": "minhash", "struct": [{"name": "cluster_main_idx", "dtype": "int64"}, {"name": "cluster_size", "dtype": "int64"}, {"name": "is_duplicate", "dtype": "bool"}, {"name": "minhash_idx", "dtype": "int64"}]}]}]}, {"name": "id", "dtype": "int64"}], "splits": [{"name": "train", "num_bytes": 896139675, "num_examples": 198387}], "download_size": 408025309, "dataset_size": 896139675}, {"config_name": "mlp_pt_BRCAD-5", "features": [{"name": "id", "dtype": "int64"}, {"name": "text", "dtype": "string"}, {"name": "meta", "struct": [{"name": "dedup", "struct": [{"name": "exact_norm", "struct": [{"name": "cluster_main_idx", "dtype": "int64"}, {"name": "cluster_size", "dtype": "int64"}, {"name": "exact_hash_idx", "dtype": "int64"}, {"name": "is_duplicate", "dtype": "bool"}]}, {"name": "minhash", "struct": [{"name": "cluster_main_idx", "dtype": "int64"}, {"name": "cluster_size", "dtype": "int64"}, {"name": "is_duplicate", "dtype": "bool"}, {"name": "minhash_idx", "dtype": "int64"}]}]}]}], "splits": [{"name": "train", "num_bytes": 20311710293, "num_examples": 3128292}], "download_size": 9735599974, "dataset_size": 20311710293}, {"config_name": "mlp_pt_CJPG", "features": [{"name": "id", "dtype": "int64"}, {"name": "text", "dtype": "string"}, {"name": "meta", "struct": [{"name": "dedup", "struct": [{"name": "exact_norm", "struct": [{"name": "cluster_main_idx", "dtype": "int64"}, {"name": "cluster_size", "dtype": "int64"}, {"name": "exact_hash_idx", "dtype": "int64"}, {"name": "is_duplicate", "dtype": "bool"}]}, {"name": "minhash", "struct": [{"name": "cluster_main_idx", "dtype": "int64"}, {"name": "cluster_size", "dtype": "int64"}, {"name": "is_duplicate", "dtype": "bool"}, {"name": "minhash_idx", "dtype": "int64"}]}]}]}], "splits": [{"name": "train", "num_bytes": 63201157801, "num_examples": 14068634}], "download_size": 30473107046, "dataset_size": 63201157801}, {"config_name": "mlp_pt_eurlex-caselaw", "features": [{"name": "id", "dtype": "int64"}, {"name": "text", "dtype": "string"}, {"name": "meta", "struct": [{"name": "dedup", "struct": [{"name": "exact_norm", "struct": [{"name": "cluster_main_idx", "dtype": "int64"}, {"name": "cluster_size", "dtype": "int64"}, {"name": "exact_hash_idx", "dtype": "int64"}, {"name": "is_duplicate", "dtype": "bool"}]}, {"name": "minhash", "struct": [{"name": "cluster_main_idx", "dtype": "int64"}, {"name": "cluster_size", "dtype": "int64"}, {"name": "is_duplicate", "dtype": "bool"}, {"name": "minhash_idx", "dtype": "int64"}]}]}]}], "splits": [{"name": "train", "num_bytes": 1499601545, "num_examples": 104312}], "download_size": 627235870, "dataset_size": 1499601545}, {"config_name": "mlp_pt_eurlex-contracts", "features": [{"name": "id", "dtype": "int64"}, {"name": "text", "dtype": "string"}, {"name": "meta", "struct": [{"name": "dedup", "struct": [{"name": "exact_norm", "struct": [{"name": "cluster_main_idx", "dtype": "int64"}, {"name": "cluster_size", "dtype": "int64"}, {"name": "exact_hash_idx", "dtype": "int64"}, {"name": "is_duplicate", "dtype": "bool"}]}, {"name": "minhash", "struct": [{"name": "cluster_main_idx", "dtype": "int64"}, {"name": "cluster_size", "dtype": "int64"}, {"name": "is_duplicate", "dtype": "bool"}, {"name": "minhash_idx", "dtype": "int64"}]}]}]}], "splits": [{"name": "train", "num_bytes": 467200973, "num_examples": 11581}], "download_size": 112805426, "dataset_size": 467200973}, {"config_name": "mlp_pt_eurlex-legislation", "features": [{"name": "id", "dtype": "int64"}, {"name": "text", "dtype": "string"}, {"name": "meta", "struct": [{"name": "dedup", "struct": [{"name": "exact_norm", "struct": [{"name": "cluster_main_idx", "dtype": "int64"}, {"name": "cluster_size", "dtype": "int64"}, {"name": "exact_hash_idx", "dtype": "int64"}, {"name": "is_duplicate", "dtype": "bool"}]}, {"name": "minhash", "struct": [{"name": "cluster_main_idx", "dtype": "int64"}, {"name": "cluster_size", "dtype": "int64"}, {"name": "is_duplicate", "dtype": "bool"}, {"name": "minhash_idx", "dtype": "int64"}]}]}]}], "splits": [{"name": "train", "num_bytes": 5669271303, "num_examples": 232556}], "download_size": 1384571339, "dataset_size": 5669271303}, {"config_name": "mlp_pt_legal-mc4", "features": [{"name": "id", "dtype": "int64"}, {"name": "text", "dtype": "string"}, {"name": "meta", "struct": [{"name": "dedup", "struct": [{"name": "exact_norm", "struct": [{"name": "cluster_main_idx", "dtype": "int64"}, {"name": "cluster_size", "dtype": "int64"}, {"name": "exact_hash_idx", "dtype": "int64"}, {"name": "is_duplicate", "dtype": "bool"}]}, {"name": "minhash", "struct": [{"name": "cluster_main_idx", "dtype": "int64"}, {"name": "cluster_size", "dtype": "int64"}, {"name": "is_duplicate", "dtype": "bool"}, {"name": "minhash_idx", "dtype": "int64"}]}]}]}], "splits": [{"name": "train", "num_bytes": 4483889482, "num_examples": 191174}], "download_size": 2250422592, "dataset_size": 4483889482}, {"config_name": "parlamento-pt", "features": [{"name": "id", "dtype": "int64"}, {"name": "text", "dtype": "string"}, {"name": "meta", "struct": [{"name": "dedup", "struct": [{"name": "exact_norm", "struct": [{"name": "cluster_main_idx", "dtype": "int64"}, {"name": "cluster_size", "dtype": "int64"}, {"name": "exact_hash_idx", "dtype": "int64"}, {"name": "is_duplicate", "dtype": "bool"}]}, {"name": "minhash", "struct": [{"name": "cluster_main_idx", "dtype": "int64"}, {"name": "cluster_size", "dtype": "int64"}, {"name": "is_duplicate", "dtype": "bool"}, {"name": "minhash_idx", "dtype": "int64"}]}]}]}], "splits": [{"name": "train", "num_bytes": 2867291543, "num_examples": 2670846}], "download_size": 1319479156, "dataset_size": 2867291543}], "configs": [{"config_name": "acordaos_tcu", "data_files": [{"split": "train", "path": "acordaos_tcu/train-*"}]}, {"config_name": "all", "data_files": [{"split": "train", "path": "all/train-*"}]}, {"config_name": "datastf", "data_files": [{"split": "train", "path": "datastf/train-*"}]}, {"config_name": "iudicium_textum", "data_files": [{"split": "train", "path": "iudicium_textum/train-*"}]}, {"config_name": "mlp_pt_BRCAD-5", "data_files": [{"split": "train", "path": "mlp_pt_BRCAD-5/train-*"}]}, {"config_name": "mlp_pt_CJPG", "data_files": [{"split": "train", "path": "mlp_pt_CJPG/train-*"}]}, {"config_name": "mlp_pt_eurlex-caselaw", "data_files": [{"split": "train", "path": "mlp_pt_eurlex-caselaw/train-*"}]}, {"config_name": "mlp_pt_eurlex-contracts", "data_files": [{"split": "train", "path": "mlp_pt_eurlex-contracts/train-*"}]}, {"config_name": "mlp_pt_eurlex-legislation", "data_files": [{"split": "train", "path": "mlp_pt_eurlex-legislation/train-*"}]}, {"config_name": "mlp_pt_legal-mc4", "data_files": [{"split": "train", "path": "mlp_pt_legal-mc4/train-*"}]}, {"config_name": "parlamento-pt", "data_files": [{"split": "train", "path": "parlamento-pt/train-*"}]}]} | 2024-02-09T16:36:38+00:00 | [
"2306.02069",
"2305.06721",
"2010.11934",
"2107.06499"
] | [
"pt"
] | TAGS
#task_categories-text-generation #size_categories-10M<n<100M #language-Portuguese #license-cc-by-4.0 #legal #arxiv-2306.02069 #arxiv-2305.06721 #arxiv-2010.11934 #arxiv-2107.06499 #region-us
| LegalPT
=======
LegalPT aggregates the maximum amount of publicly available legal data in Portuguese, drawing from varied sources including legislation, jurisprudence, legal articles, and government documents.
Dataset Details
---------------
Dataset is composed by six corpora:
Ulysses-Tesemõ, MultiLegalPile (PT), ParlamentoPT,
Iudicium Textum, Acordãos TCU, and
DataSTF.
* MultiLegalPile: a multilingual corpus of legal texts comprising 689 GiB of data, covering 24 languages in 17 jurisdictions. The corpus is separated by language, and the subset in Portuguese contains 92GiB of data, containing 13.76 billion words. This subset includes the jurisprudence of the Court of Justice of São Paulo (CJPG), appeals from the 5th Regional Federal Court (BRCAD-5), the Portuguese subset of legal documents from the European Union, known as EUR-Lex, and a filter for legal documents from MC4.
* Ulysses-Tesemõ: a legal corpus in Brazilian Portuguese, composed of 2.2 million documents, totaling about 26GiB of text obtained from 96 different data sources. These sources encompass legal, legislative, academic papers, news, and related comments. The data was collected through web scraping of government websites.
* ParlamentoPT: a corpus for training language models in European Portuguese. The data was collected from the Portuguese government portal and consists of 2.6 million documents of transcriptions of debates in the Portuguese Parliament.
* Iudicium Textum: consists of rulings, votes, and reports from the Supreme Federal Court (STF) of Brazil, published between 2010 and 2018. The dataset contains 1GiB of data extracted from PDFs.
* Acordãos TCU: an open dataset from the Tribunal de Contas da União (Brazilian Federal Court of Accounts), containing 600,000 documents obtained by web scraping government websites. The documents span from 1992 to 2019.
* DataSTF: a dataset of monocratic decisions from the Superior Court of Justice (STJ) in Brazil, containing 700,000 documents (5GiB of data).
### Dataset Description
* Curated by:
* Funded by:
* Language(s) (NLP): Brazilian Portuguese (pt-BR)
* License: Creative Commons Attribution 4.0 International Public License
### Dataset Sources
* Repository: URL
* Paper:
Dataset Structure
-----------------
Data Collection and Processing
------------------------------
LegalPT is deduplicated using MinHash algorithm and Locality Sensitive Hashing, following the approach of Lee et al. (2022).
We used 5-grams and a signature of size 256, considering two documents to be identical if their Jaccard Similarity exceeded 0.7.
Duplicate rate found by the Minhash-LSH algorithm for the LegalPT corpus:
Acknowledgment
--------------
This work has been supported by the AI Center of Excellence (Centro de Excelência em Inteligência Artificial – CEIA) of the Institute of Informatics at the Federal University of Goiás (INF-UFG).
| [
"### Dataset Description\n\n\n* Curated by:\n* Funded by:\n* Language(s) (NLP): Brazilian Portuguese (pt-BR)\n* License: Creative Commons Attribution 4.0 International Public License",
"### Dataset Sources\n\n\n* Repository: URL\n* Paper:\n\n\nDataset Structure\n-----------------\n\n\nData Collection and Processing\n------------------------------\n\n\nLegalPT is deduplicated using MinHash algorithm and Locality Sensitive Hashing, following the approach of Lee et al. (2022).\n\n\nWe used 5-grams and a signature of size 256, considering two documents to be identical if their Jaccard Similarity exceeded 0.7.\n\n\nDuplicate rate found by the Minhash-LSH algorithm for the LegalPT corpus:\n\n\n\nAcknowledgment\n--------------\n\n\nThis work has been supported by the AI Center of Excellence (Centro de Excelência em Inteligência Artificial – CEIA) of the Institute of Informatics at the Federal University of Goiás (INF-UFG)."
] | [
"TAGS\n#task_categories-text-generation #size_categories-10M<n<100M #language-Portuguese #license-cc-by-4.0 #legal #arxiv-2306.02069 #arxiv-2305.06721 #arxiv-2010.11934 #arxiv-2107.06499 #region-us \n",
"### Dataset Description\n\n\n* Curated by:\n* Funded by:\n* Language(s) (NLP): Brazilian Portuguese (pt-BR)\n* License: Creative Commons Attribution 4.0 International Public License",
"### Dataset Sources\n\n\n* Repository: URL\n* Paper:\n\n\nDataset Structure\n-----------------\n\n\nData Collection and Processing\n------------------------------\n\n\nLegalPT is deduplicated using MinHash algorithm and Locality Sensitive Hashing, following the approach of Lee et al. (2022).\n\n\nWe used 5-grams and a signature of size 256, considering two documents to be identical if their Jaccard Similarity exceeded 0.7.\n\n\nDuplicate rate found by the Minhash-LSH algorithm for the LegalPT corpus:\n\n\n\nAcknowledgment\n--------------\n\n\nThis work has been supported by the AI Center of Excellence (Centro de Excelência em Inteligência Artificial – CEIA) of the Institute of Informatics at the Federal University of Goiás (INF-UFG)."
] |
f49945e2ff49770328f685873ea1dfb00cda218b |
# REBUS
REBUS: A Robust Evaluation Benchmark of Understanding Symbols
[**Paper**](https://arxiv.org/abs/2401.05604) | [**🤗 Dataset**](https://huggingface.co/datasets/cavendishlabs/rebus) | [**GitHub**](https://github.com/cvndsh/rebus) | [**Website**](https://cavendishlabs.org/rebus/)
## Introduction
Recent advances in large language models have led to the development of multimodal LLMs (MLLMs), which take both image data and text as an input. Virtually all of these models have been announced within the past year, leading to a significant need for benchmarks evaluating the abilities of these models to reason truthfully and accurately on a diverse set of tasks. When Google announced Gemini Pro (Gemini Team et al., 2023), they displayed its ability to solve rebuses—wordplay puzzles which involve creatively adding and subtracting letters from words derived from text and images. The diversity of rebuses allows for a broad evaluation of multimodal reasoning capabilities, including image recognition, multi-step reasoning, and understanding the human creator's intent.
We present REBUS: a collection of 333 hand-crafted rebuses spanning 13 diverse categories, including hand-drawn and digital images created by nine contributors. Samples are presented in the table below. Notably, GPT-4V, the most powerful model we evaluated, answered only 24% of puzzles correctly, highlighting the poor capabilities of MLLMs in new and unexpected domains to which human reasoning generalizes with comparative ease. Open-source models perform even worse, with a median accuracy below 1%. We notice that models often give faithless explanations, fail to change their minds after an initial approach doesn't work, and remain highly uncalibrated on their own abilities.
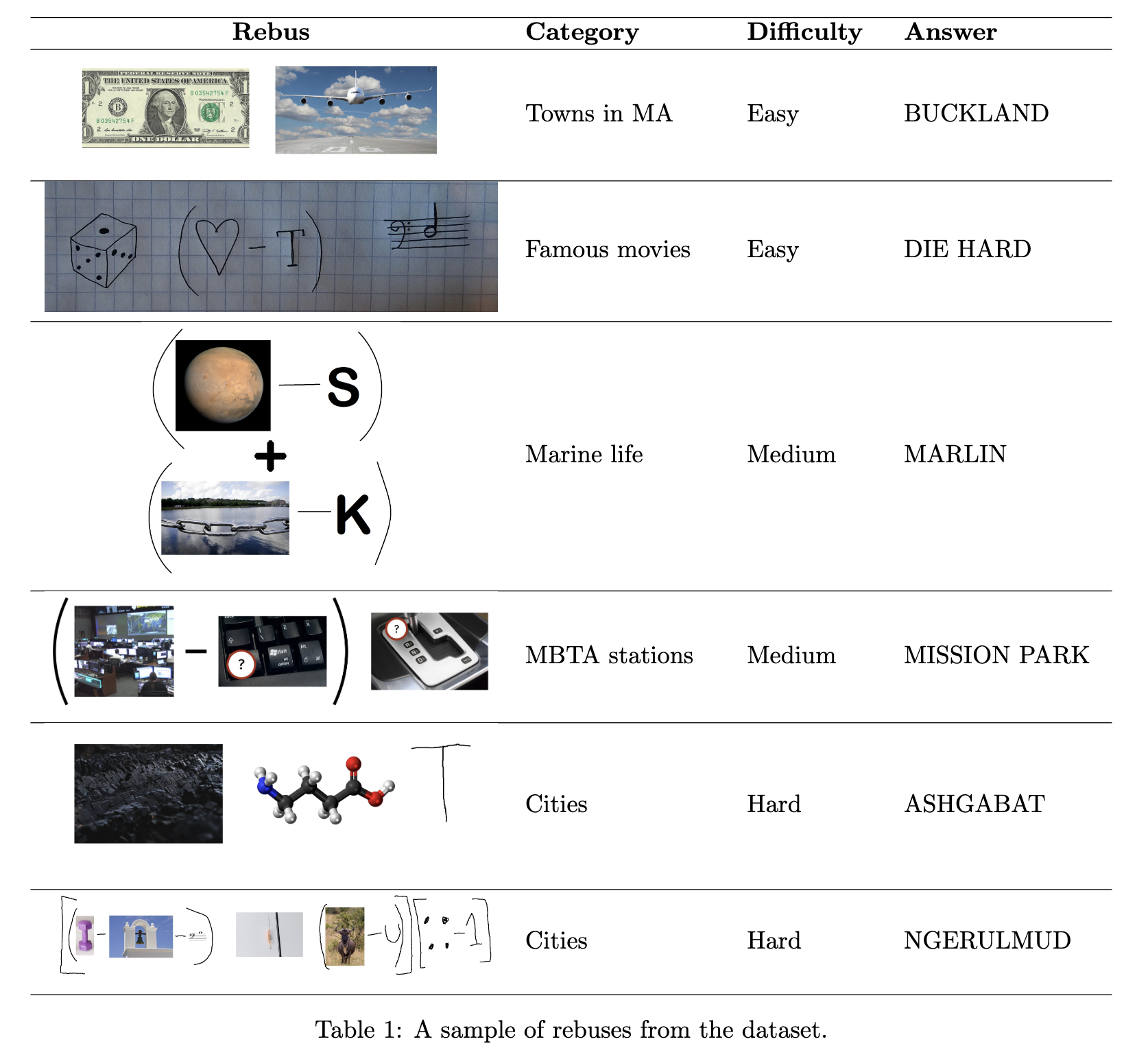
## Evaluation results
| Model | Overall | Easy | Medium | Hard |
| ----------------- | ------------- | ------------- | ------------- | ------------ |
| GPT-4V | **24.0** | **33.0** | **13.2** | **7.1** |
| Gemini Pro | 13.2 | 19.4 | 5.3 | 3.6 |
| LLaVa-1.5-13B | 1.8 | 2.6 | 0.9 | 0.0 |
| LLaVa-1.5-7B | 1.5 | 2.6 | 0.0 | 0.0 |
| BLIP2-FLAN-T5-XXL | 0.9 | 0.5 | 1.8 | 0.0 |
| CogVLM | 0.9 | 1.6 | 0.0 | 0.0 |
| QWEN | 0.9 | 1.6 | 0.0 | 0.0 |
| InstructBLIP | 0.6 | 0.5 | 0.9 | 0.0 |
| cavendishlabs/rebus | [
"arxiv:2401.05604",
"region:us"
] | 2024-01-09T19:10:22+00:00 | {"dataset_info": {"features": [{"name": "Filename", "dtype": "string"}, {"name": "Solution", "dtype": "string"}, {"name": "Also accept", "dtype": "string"}, {"name": "Theme", "dtype": "string"}, {"name": "Difficulty", "dtype": "string"}, {"name": "Exact spelling?", "dtype": "string"}, {"name": "Specific reference", "dtype": "string"}, {"name": "Reading?", "dtype": "string"}, {"name": "Attribution", "dtype": "string"}, {"name": "Author", "dtype": "string"}, {"name": "image", "dtype": "image"}], "splits": [{"name": "train", "num_bytes": 51545282.0, "num_examples": 333}], "download_size": 47656838, "dataset_size": 51545282.0}, "configs": [{"config_name": "default", "data_files": [{"split": "train", "path": "data/train-*"}]}]} | 2024-01-12T01:30:58+00:00 | [
"2401.05604"
] | [] | TAGS
#arxiv-2401.05604 #region-us
| REBUS
=====
REBUS: A Robust Evaluation Benchmark of Understanding Symbols
Paper | Dataset | GitHub | Website
Introduction
------------
Recent advances in large language models have led to the development of multimodal LLMs (MLLMs), which take both image data and text as an input. Virtually all of these models have been announced within the past year, leading to a significant need for benchmarks evaluating the abilities of these models to reason truthfully and accurately on a diverse set of tasks. When Google announced Gemini Pro (Gemini Team et al., 2023), they displayed its ability to solve rebuses—wordplay puzzles which involve creatively adding and subtracting letters from words derived from text and images. The diversity of rebuses allows for a broad evaluation of multimodal reasoning capabilities, including image recognition, multi-step reasoning, and understanding the human creator's intent.
We present REBUS: a collection of 333 hand-crafted rebuses spanning 13 diverse categories, including hand-drawn and digital images created by nine contributors. Samples are presented in the table below. Notably, GPT-4V, the most powerful model we evaluated, answered only 24% of puzzles correctly, highlighting the poor capabilities of MLLMs in new and unexpected domains to which human reasoning generalizes with comparative ease. Open-source models perform even worse, with a median accuracy below 1%. We notice that models often give faithless explanations, fail to change their minds after an initial approach doesn't work, and remain highly uncalibrated on their own abilities.
!image
Evaluation results
------------------
| [] | [
"TAGS\n#arxiv-2401.05604 #region-us \n"
] |
25046c986975a77f226ae59deeb12df4f86a3a40 | # Dataset Card for "module_pairwise_dataset_neg_from_pos_pool_v3"
[More Information needed](https://github.com/huggingface/datasets/blob/main/CONTRIBUTING.md#how-to-contribute-to-the-dataset-cards) | zhan1993/module_pairwise_dataset_neg_from_pos_pool_v3 | [
"region:us"
] | 2024-01-09T20:41:36+00:00 | {"configs": [{"config_name": "default", "data_files": [{"split": "train", "path": "data/train-*"}]}], "dataset_info": {"features": [{"name": "eval_task", "dtype": "string"}, {"name": "sources_texts", "dtype": "string"}, {"name": "positive_expert_names", "dtype": "string"}, {"name": "negative_expert_names", "dtype": "string"}], "splits": [{"name": "train", "num_bytes": 258629092, "num_examples": 120865}], "download_size": 28424463, "dataset_size": 258629092}} | 2024-01-09T20:41:40+00:00 | [] | [] | TAGS
#region-us
| # Dataset Card for "module_pairwise_dataset_neg_from_pos_pool_v3"
More Information needed | [
"# Dataset Card for \"module_pairwise_dataset_neg_from_pos_pool_v3\"\n\nMore Information needed"
] | [
"TAGS\n#region-us \n",
"# Dataset Card for \"module_pairwise_dataset_neg_from_pos_pool_v3\"\n\nMore Information needed"
] |
4bf71c546082f351dd4abcd3850202bff4671c47 |
### Overview
DPO dataset meant to enhance python coding abilities.
This dataset uses the excellent https://huggingface.co/datasets/Vezora/Tested-22k-Python-Alpaca dataset as the "chosen" responses, given this dataset was already tested and validated.
The "rejected" values were generated with a mix of airoboros-l2-13b-3.1 and bagel-7b-v0.1.
The rejected values may actually be perfectly fine, but the assumption here is that the values are generally a lower quality than the chosen counterpart. Items with duplicate code blocks were removed.
### Contribute
If you're interested in new functionality/datasets, take a look at [bagel repo](https://github.com/jondurbin/bagel) and [airoboros](https://github.com/jondurbin/airoboros) and either make a PR or open an issue with details.
To help me with the fine-tuning costs, dataset generation, etc., please use one of the following:
- https://bmc.link/jondurbin
- ETH 0xce914eAFC2fe52FdceE59565Dd92c06f776fcb11
- BTC bc1qdwuth4vlg8x37ggntlxu5cjfwgmdy5zaa7pswf | jondurbin/py-dpo-v0.1 | [
"language:code",
"license:cc-by-4.0",
"region:us"
] | 2024-01-09T20:57:21+00:00 | {"language": ["code"], "license": "cc-by-4.0"} | 2024-01-11T10:16:18+00:00 | [] | [
"code"
] | TAGS
#language-code #license-cc-by-4.0 #region-us
|
### Overview
DPO dataset meant to enhance python coding abilities.
This dataset uses the excellent URL dataset as the "chosen" responses, given this dataset was already tested and validated.
The "rejected" values were generated with a mix of airoboros-l2-13b-3.1 and bagel-7b-v0.1.
The rejected values may actually be perfectly fine, but the assumption here is that the values are generally a lower quality than the chosen counterpart. Items with duplicate code blocks were removed.
### Contribute
If you're interested in new functionality/datasets, take a look at bagel repo and airoboros and either make a PR or open an issue with details.
To help me with the fine-tuning costs, dataset generation, etc., please use one of the following:
- URL
- ETH 0xce914eAFC2fe52FdceE59565Dd92c06f776fcb11
- BTC bc1qdwuth4vlg8x37ggntlxu5cjfwgmdy5zaa7pswf | [
"### Overview\n\nDPO dataset meant to enhance python coding abilities.\n\nThis dataset uses the excellent URL dataset as the \"chosen\" responses, given this dataset was already tested and validated.\n\nThe \"rejected\" values were generated with a mix of airoboros-l2-13b-3.1 and bagel-7b-v0.1.\n\nThe rejected values may actually be perfectly fine, but the assumption here is that the values are generally a lower quality than the chosen counterpart. Items with duplicate code blocks were removed.",
"### Contribute\n\nIf you're interested in new functionality/datasets, take a look at bagel repo and airoboros and either make a PR or open an issue with details.\n\nTo help me with the fine-tuning costs, dataset generation, etc., please use one of the following:\n\n- URL\n- ETH 0xce914eAFC2fe52FdceE59565Dd92c06f776fcb11\n- BTC bc1qdwuth4vlg8x37ggntlxu5cjfwgmdy5zaa7pswf"
] | [
"TAGS\n#language-code #license-cc-by-4.0 #region-us \n",
"### Overview\n\nDPO dataset meant to enhance python coding abilities.\n\nThis dataset uses the excellent URL dataset as the \"chosen\" responses, given this dataset was already tested and validated.\n\nThe \"rejected\" values were generated with a mix of airoboros-l2-13b-3.1 and bagel-7b-v0.1.\n\nThe rejected values may actually be perfectly fine, but the assumption here is that the values are generally a lower quality than the chosen counterpart. Items with duplicate code blocks were removed.",
"### Contribute\n\nIf you're interested in new functionality/datasets, take a look at bagel repo and airoboros and either make a PR or open an issue with details.\n\nTo help me with the fine-tuning costs, dataset generation, etc., please use one of the following:\n\n- URL\n- ETH 0xce914eAFC2fe52FdceE59565Dd92c06f776fcb11\n- BTC bc1qdwuth4vlg8x37ggntlxu5cjfwgmdy5zaa7pswf"
] |
7b630ac15135be066dbdb96b5edb44ac56f41dc7 | # Dataset Card for "cai-conversation-dev1704834920"
[More Information needed](https://github.com/huggingface/datasets/blob/main/CONTRIBUTING.md#how-to-contribute-to-the-dataset-cards) | vwxyzjn/cai-conversation-dev1704834920 | [
"region:us"
] | 2024-01-09T21:18:42+00:00 | {"dataset_info": {"features": [{"name": "index", "dtype": "int64"}, {"name": "prompt", "dtype": "string"}, {"name": "init_prompt", "struct": [{"name": "content", "dtype": "string"}, {"name": "role", "dtype": "string"}]}, {"name": "init_response", "struct": [{"name": "content", "dtype": "string"}, {"name": "role", "dtype": "string"}]}, {"name": "critic_prompt", "struct": [{"name": "content", "dtype": "string"}, {"name": "role", "dtype": "string"}]}, {"name": "critic_response", "struct": [{"name": "content", "dtype": "string"}, {"name": "role", "dtype": "string"}]}, {"name": "revision_prompt", "struct": [{"name": "content", "dtype": "string"}, {"name": "role", "dtype": "string"}]}, {"name": "revision_response", "struct": [{"name": "content", "dtype": "string"}, {"name": "role", "dtype": "string"}]}, {"name": "messages", "list": [{"name": "content", "dtype": "string"}, {"name": "role", "dtype": "string"}]}, {"name": "chosen", "list": [{"name": "content", "dtype": "string"}, {"name": "role", "dtype": "string"}]}, {"name": "rejected", "list": [{"name": "content", "dtype": "string"}, {"name": "role", "dtype": "string"}]}], "splits": [{"name": "train_sft", "num_bytes": 273314, "num_examples": 64}, {"name": "train_prefs", "num_bytes": 255493, "num_examples": 64}], "download_size": 266824, "dataset_size": 528807}, "configs": [{"config_name": "default", "data_files": [{"split": "train_sft", "path": "data/train_sft-*"}, {"split": "train_prefs", "path": "data/train_prefs-*"}]}]} | 2024-01-09T21:18:44+00:00 | [] | [] | TAGS
#region-us
| # Dataset Card for "cai-conversation-dev1704834920"
More Information needed | [
"# Dataset Card for \"cai-conversation-dev1704834920\"\n\nMore Information needed"
] | [
"TAGS\n#region-us \n",
"# Dataset Card for \"cai-conversation-dev1704834920\"\n\nMore Information needed"
] |
6b3d377e6c4a206c0290d15f061f84417998f4e8 | # Dataset Card for "parallel-9"
[More Information needed](https://github.com/huggingface/datasets/blob/main/CONTRIBUTING.md#how-to-contribute-to-the-dataset-cards) | gowitheflowlab/parallel-9 | [
"region:us"
] | 2024-01-09T21:41:44+00:00 | {"configs": [{"config_name": "default", "data_files": [{"split": "train", "path": "data/train-*"}]}], "dataset_info": {"features": [{"name": "sentence1", "dtype": "string"}, {"name": "sentence2", "dtype": "string"}, {"name": "label", "dtype": "string"}], "splits": [{"name": "train", "num_bytes": 649296909.6590501, "num_examples": 3322980}], "download_size": 428488796, "dataset_size": 649296909.6590501}} | 2024-01-09T21:54:40+00:00 | [] | [] | TAGS
#region-us
| # Dataset Card for "parallel-9"
More Information needed | [
"# Dataset Card for \"parallel-9\"\n\nMore Information needed"
] | [
"TAGS\n#region-us \n",
"# Dataset Card for \"parallel-9\"\n\nMore Information needed"
] |
8b02cb3ea33e74861fcc4d3c7b266f83b3b8c6b9 | # FLORES-200 EN-EL with prompts for translation by LLMs
Based on [FLORES-200](https://huggingface.co/datasets/Muennighoff/flores200) dataset.
Publication:
@article{nllb2022,
author = {NLLB Team, Marta R. Costa-jussà, James Cross, Onur Çelebi, Maha Elbayad, Kenneth Heafield, Kevin Heffernan, Elahe Kalbassi, Janice Lam, Daniel Licht, Jean Maillard, Anna Sun, Skyler Wang, Guillaume Wenzek, Al Youngblood, Bapi Akula, Loic Barrault, Gabriel Mejia Gonzalez, Prangthip Hansanti, John Hoffman, Semarley Jarrett, Kaushik Ram Sadagopan, Dirk Rowe, Shannon Spruit, Chau Tran, Pierre Andrews, Necip Fazil Ayan, Shruti Bhosale, Sergey Edunov, Angela Fan, Cynthia Gao, Vedanuj Goswami, Francisco Guzmán, Philipp Koehn, Alexandre Mourachko, Christophe Ropers, Safiyyah Saleem, Holger Schwenk, Jeff Wang},
title = {No Language Left Behind: Scaling Human-Centered Machine Translation},
year = {2022}
}
Number of examples : 1012
## FLORES-200 for EN to EL with 0-shot prompts
Contains 2 prompt variants:
- EN:\n\[English Sentence\]\nEL:
- English:\n\[English Sentence\]\nΕλληνικά:
## FLORES-200 for EL to EN with 0-shot prompts
Contains 2 prompt variants:
- EL:\n\[Greek Sentence\]\nEL:
- Ελληνικά:\n\[Greek Sentence\]\nEnglish:
## How to load datasets
```python
from datasets import load_dataset
input_file = 'flores200.en2el.test.0-shot.json'
dataset = load_dataset(
'json',
data_files=input_file,
field='examples',
split='train'
)
```
## How to generate translation results with different configurations
```python
from multiprocessing import cpu_count
def generate_translations(datapoint, config, config_name):
for idx, variant in enumerate(datapoint["prompts_results"]):
# REPLACE generate WITH ACTUAL FUNCTION WHICH TAKES GENERATION CONFIG
result = generate(variant["prompt"], config=config)
datapoint["prompts_results"][idx].update({config_name: result})
return datapoint
dataset = dataset.map(
function=generate_translations,
fn_kwargs={"config": config, "config_name": config_name},
keep_in_memory=False,
num_proc=min(len(dataset), cpu_count()),
)
```
## How to push updated datasets to hub
```python
from huggingface_hub import HfApi
input_file = "flores200.en2el.test.0-shot.json"
model_name = "meltemi-v0.2"
output_file = input_file.replace(".json", ".{}.json".format(model_name)
dataset.to_json(output_file,
force_ascii=False,
indent=4,
orient="index")
api = HfApi()
api.upload_file(
path_or_fileobj=output_file,
path_in_repo="results/{}/{}".format(model_name, output_file)
repo_id="ilsp/flores200-en-el-prompt",
repo_type="dataset",
)
```
| ilsp/flores200_en-el | [
"task_categories:translation",
"size_categories:1K<n<10K",
"language:en",
"language:el",
"license:cc-by-sa-4.0",
"region:us"
] | 2024-01-09T21:50:20+00:00 | {"language": ["en", "el"], "license": "cc-by-sa-4.0", "size_categories": ["1K<n<10K"], "task_categories": ["translation"], "dataset_info": {"features": [{"name": "en", "dtype": "string"}, {"name": "el", "dtype": "string"}], "splits": [{"name": "validation", "num_bytes": 406555, "num_examples": 997}, {"name": "test", "num_bytes": 427413, "num_examples": 1012}], "download_size": 481524, "dataset_size": 833968}, "configs": [{"config_name": "default", "data_files": [{"split": "validation", "path": "data/validation-*"}, {"split": "test", "path": "data/test-*"}]}]} | 2024-01-23T14:37:02+00:00 | [] | [
"en",
"el"
] | TAGS
#task_categories-translation #size_categories-1K<n<10K #language-English #language-Modern Greek (1453-) #license-cc-by-sa-4.0 #region-us
| # FLORES-200 EN-EL with prompts for translation by LLMs
Based on FLORES-200 dataset.
Publication:
@article{nllb2022,
author = {NLLB Team, Marta R. Costa-jussà, James Cross, Onur Çelebi, Maha Elbayad, Kenneth Heafield, Kevin Heffernan, Elahe Kalbassi, Janice Lam, Daniel Licht, Jean Maillard, Anna Sun, Skyler Wang, Guillaume Wenzek, Al Youngblood, Bapi Akula, Loic Barrault, Gabriel Mejia Gonzalez, Prangthip Hansanti, John Hoffman, Semarley Jarrett, Kaushik Ram Sadagopan, Dirk Rowe, Shannon Spruit, Chau Tran, Pierre Andrews, Necip Fazil Ayan, Shruti Bhosale, Sergey Edunov, Angela Fan, Cynthia Gao, Vedanuj Goswami, Francisco Guzmán, Philipp Koehn, Alexandre Mourachko, Christophe Ropers, Safiyyah Saleem, Holger Schwenk, Jeff Wang},
title = {No Language Left Behind: Scaling Human-Centered Machine Translation},
year = {2022}
}
Number of examples : 1012
## FLORES-200 for EN to EL with 0-shot prompts
Contains 2 prompt variants:
- EN:\n\[English Sentence\]\nEL:
- English:\n\[English Sentence\]\nΕλληνικά:
## FLORES-200 for EL to EN with 0-shot prompts
Contains 2 prompt variants:
- EL:\n\[Greek Sentence\]\nEL:
- Ελληνικά:\n\[Greek Sentence\]\nEnglish:
## How to load datasets
## How to generate translation results with different configurations
## How to push updated datasets to hub
| [
"# FLORES-200 EN-EL with prompts for translation by LLMs\nBased on FLORES-200 dataset.\n\nPublication:\n@article{nllb2022,\n author = {NLLB Team, Marta R. Costa-jussà, James Cross, Onur Çelebi, Maha Elbayad, Kenneth Heafield, Kevin Heffernan, Elahe Kalbassi, Janice Lam, Daniel Licht, Jean Maillard, Anna Sun, Skyler Wang, Guillaume Wenzek, Al Youngblood, Bapi Akula, Loic Barrault, Gabriel Mejia Gonzalez, Prangthip Hansanti, John Hoffman, Semarley Jarrett, Kaushik Ram Sadagopan, Dirk Rowe, Shannon Spruit, Chau Tran, Pierre Andrews, Necip Fazil Ayan, Shruti Bhosale, Sergey Edunov, Angela Fan, Cynthia Gao, Vedanuj Goswami, Francisco Guzmán, Philipp Koehn, Alexandre Mourachko, Christophe Ropers, Safiyyah Saleem, Holger Schwenk, Jeff Wang},\n title = {No Language Left Behind: Scaling Human-Centered Machine Translation},\n year = {2022}\n}\n\nNumber of examples : 1012",
"## FLORES-200 for EN to EL with 0-shot prompts\nContains 2 prompt variants:\n- EN:\\n\\[English Sentence\\]\\nEL:\n- English:\\n\\[English Sentence\\]\\nΕλληνικά:",
"## FLORES-200 for EL to EN with 0-shot prompts\nContains 2 prompt variants:\n- EL:\\n\\[Greek Sentence\\]\\nEL:\n- Ελληνικά:\\n\\[Greek Sentence\\]\\nEnglish:",
"## How to load datasets",
"## How to generate translation results with different configurations",
"## How to push updated datasets to hub"
] | [
"TAGS\n#task_categories-translation #size_categories-1K<n<10K #language-English #language-Modern Greek (1453-) #license-cc-by-sa-4.0 #region-us \n",
"# FLORES-200 EN-EL with prompts for translation by LLMs\nBased on FLORES-200 dataset.\n\nPublication:\n@article{nllb2022,\n author = {NLLB Team, Marta R. Costa-jussà, James Cross, Onur Çelebi, Maha Elbayad, Kenneth Heafield, Kevin Heffernan, Elahe Kalbassi, Janice Lam, Daniel Licht, Jean Maillard, Anna Sun, Skyler Wang, Guillaume Wenzek, Al Youngblood, Bapi Akula, Loic Barrault, Gabriel Mejia Gonzalez, Prangthip Hansanti, John Hoffman, Semarley Jarrett, Kaushik Ram Sadagopan, Dirk Rowe, Shannon Spruit, Chau Tran, Pierre Andrews, Necip Fazil Ayan, Shruti Bhosale, Sergey Edunov, Angela Fan, Cynthia Gao, Vedanuj Goswami, Francisco Guzmán, Philipp Koehn, Alexandre Mourachko, Christophe Ropers, Safiyyah Saleem, Holger Schwenk, Jeff Wang},\n title = {No Language Left Behind: Scaling Human-Centered Machine Translation},\n year = {2022}\n}\n\nNumber of examples : 1012",
"## FLORES-200 for EN to EL with 0-shot prompts\nContains 2 prompt variants:\n- EN:\\n\\[English Sentence\\]\\nEL:\n- English:\\n\\[English Sentence\\]\\nΕλληνικά:",
"## FLORES-200 for EL to EN with 0-shot prompts\nContains 2 prompt variants:\n- EL:\\n\\[Greek Sentence\\]\\nEL:\n- Ελληνικά:\\n\\[Greek Sentence\\]\\nEnglish:",
"## How to load datasets",
"## How to generate translation results with different configurations",
"## How to push updated datasets to hub"
] |
ddc0edd55a8a3b082cc88f56409ca4f91c6aa1f3 | # Dataset Card for "cai-conversation-dev1704836562"
[More Information needed](https://github.com/huggingface/datasets/blob/main/CONTRIBUTING.md#how-to-contribute-to-the-dataset-cards) | vwxyzjn/cai-conversation-dev1704836562 | [
"region:us"
] | 2024-01-09T21:53:37+00:00 | {"dataset_info": {"features": [{"name": "index", "dtype": "int64"}, {"name": "prompt", "dtype": "string"}, {"name": "init_prompt", "struct": [{"name": "content", "dtype": "string"}, {"name": "role", "dtype": "string"}]}, {"name": "init_response", "struct": [{"name": "content", "dtype": "string"}, {"name": "role", "dtype": "string"}]}, {"name": "critic_prompt", "struct": [{"name": "content", "dtype": "string"}, {"name": "role", "dtype": "string"}]}, {"name": "critic_response", "struct": [{"name": "content", "dtype": "string"}, {"name": "role", "dtype": "string"}]}, {"name": "revision_prompt", "struct": [{"name": "content", "dtype": "string"}, {"name": "role", "dtype": "string"}]}, {"name": "revision_response", "struct": [{"name": "content", "dtype": "string"}, {"name": "role", "dtype": "string"}]}, {"name": "messages", "list": [{"name": "content", "dtype": "string"}, {"name": "role", "dtype": "string"}]}, {"name": "chosen", "list": [{"name": "content", "dtype": "string"}, {"name": "role", "dtype": "string"}]}, {"name": "rejected", "list": [{"name": "content", "dtype": "string"}, {"name": "role", "dtype": "string"}]}], "splits": [{"name": "train_sft", "num_bytes": 265134, "num_examples": 64}, {"name": "train_prefs", "num_bytes": 247352, "num_examples": 64}], "download_size": 263052, "dataset_size": 512486}, "configs": [{"config_name": "default", "data_files": [{"split": "train_sft", "path": "data/train_sft-*"}, {"split": "train_prefs", "path": "data/train_prefs-*"}]}]} | 2024-01-09T21:53:39+00:00 | [] | [] | TAGS
#region-us
| # Dataset Card for "cai-conversation-dev1704836562"
More Information needed | [
"# Dataset Card for \"cai-conversation-dev1704836562\"\n\nMore Information needed"
] | [
"TAGS\n#region-us \n",
"# Dataset Card for \"cai-conversation-dev1704836562\"\n\nMore Information needed"
] |
9fd657c9c8120af8358a422cf777c86dbb231a7c | # Dataset Card for "parallel-all"
[More Information needed](https://github.com/huggingface/datasets/blob/main/CONTRIBUTING.md#how-to-contribute-to-the-dataset-cards) | gowitheflowlab/parallel-all | [
"region:us"
] | 2024-01-09T21:56:56+00:00 | {"configs": [{"config_name": "default", "data_files": [{"split": "train", "path": "data/train-*"}]}], "dataset_info": {"features": [{"name": "sentence1", "dtype": "string"}, {"name": "sentence2", "dtype": "string"}, {"name": "label", "dtype": "string"}], "splits": [{"name": "train", "num_bytes": 817156947.0942363, "num_examples": 4102854}], "download_size": 536400953, "dataset_size": 817156947.0942363}} | 2024-01-09T22:09:40+00:00 | [] | [] | TAGS
#region-us
| # Dataset Card for "parallel-all"
More Information needed | [
"# Dataset Card for \"parallel-all\"\n\nMore Information needed"
] | [
"TAGS\n#region-us \n",
"# Dataset Card for \"parallel-all\"\n\nMore Information needed"
] |
e751bcc90f4266cd682c959c4a2af77a9aebe469 |
Total train samples: 168397
Total test samples: 49233
Total tasks: 7
| Task | Train | Test |
| ---- | ----- | ---- |
|reference_number_association_without_question_boxes/2023-01-01|11481|3756|
|reference_numbers/2023-01-01|12739|3974|
|reference_number_association_with_question_boxes/2023-01-01|11481|3756|
|table_cell_incremental_without_question_boxes/2023-01-01|22884|10566|
|table_cell_incremental_with_question_boxes/2023-01-01|17986|6079|
|table_header_with_question_boxes/2023-01-01|80278|17362|
|key_value/2023-01-01|11548|3740|
Total artifact_qids: 15860
| looppayments/question_answering_token_classification_addendum | [
"region:us"
] | 2024-01-09T22:19:32+00:00 | {"pretty_name": "Question Answering Token Classification"} | 2024-02-11T00:04:56+00:00 | [] | [] | TAGS
#region-us
| Total train samples: 168397
Total test samples: 49233
Total tasks: 7
Task: reference\_number\_association\_without\_question\_boxes/2023-01-01, Train: 11481, Test: 3756
Task: reference\_numbers/2023-01-01, Train: 12739, Test: 3974
Task: reference\_number\_association\_with\_question\_boxes/2023-01-01, Train: 11481, Test: 3756
Task: table\_cell\_incremental\_without\_question\_boxes/2023-01-01, Train: 22884, Test: 10566
Task: table\_cell\_incremental\_with\_question\_boxes/2023-01-01, Train: 17986, Test: 6079
Task: table\_header\_with\_question\_boxes/2023-01-01, Train: 80278, Test: 17362
Task: key\_value/2023-01-01, Train: 11548, Test: 3740
Task: Total artifact\_qids: 15860, Train: , Test:
| [] | [
"TAGS\n#region-us \n"
] |
186ec54deb42b0ece6ca5565db1e3b31dc87ed37 | # Dataset Card for "mmlu-abstract_algebra-neg-prepend-verbal"
[More Information needed](https://github.com/huggingface/datasets/blob/main/CONTRIBUTING.md#how-to-contribute-to-the-dataset-cards) | joey234/mmlu-abstract_algebra-neg-prepend-verbal | [
"region:us"
] | 2024-01-10T00:09:24+00:00 | {"configs": [{"config_name": "default", "data_files": [{"split": "dev", "path": "data/dev-*"}, {"split": "test", "path": "data/test-*"}]}], "dataset_info": {"features": [{"name": "question", "dtype": "string"}, {"name": "choices", "sequence": "string"}, {"name": "answer", "dtype": {"class_label": {"names": {"0": "A", "1": "B", "2": "C", "3": "D"}}}}, {"name": "negate_openai_prompt", "struct": [{"name": "content", "dtype": "string"}, {"name": "role", "dtype": "string"}]}, {"name": "neg_question", "dtype": "string"}, {"name": "fewshot_context", "dtype": "string"}, {"name": "ori_prompt", "dtype": "string"}, {"name": "fewshot_context_neg", "dtype": "string"}, {"name": "fewshot_context_ori", "dtype": "string"}, {"name": "neg_prompt", "dtype": "string"}], "splits": [{"name": "dev", "num_bytes": 5843, "num_examples": 5}, {"name": "test", "num_bytes": 554556, "num_examples": 100}], "download_size": 90058, "dataset_size": 560399}} | 2024-01-10T05:14:13+00:00 | [] | [] | TAGS
#region-us
| # Dataset Card for "mmlu-abstract_algebra-neg-prepend-verbal"
More Information needed | [
"# Dataset Card for \"mmlu-abstract_algebra-neg-prepend-verbal\"\n\nMore Information needed"
] | [
"TAGS\n#region-us \n",
"# Dataset Card for \"mmlu-abstract_algebra-neg-prepend-verbal\"\n\nMore Information needed"
] |
194f772681ecf1bb14fe891227c1ac50d1677cc3 | # Dataset Card for "paraphrase_collections_enhanced"
[More Information needed](https://github.com/huggingface/datasets/blob/main/CONTRIBUTING.md#how-to-contribute-to-the-dataset-cards) | xwjzds/paraphrase_collections_enhanced | [
"region:us"
] | 2024-01-10T01:06:53+00:00 | {"dataset_info": {"features": [{"name": "input", "dtype": "string"}, {"name": "output", "dtype": "string"}], "splits": [{"name": "train", "num_bytes": 37345974, "num_examples": 243754}], "download_size": 22571420, "dataset_size": 37345974}} | 2024-01-10T01:06:56+00:00 | [] | [] | TAGS
#region-us
| # Dataset Card for "paraphrase_collections_enhanced"
More Information needed | [
"# Dataset Card for \"paraphrase_collections_enhanced\"\n\nMore Information needed"
] | [
"TAGS\n#region-us \n",
"# Dataset Card for \"paraphrase_collections_enhanced\"\n\nMore Information needed"
] |
7406ce7439851d2ee395c9e0f7bce444d25ae1e8 | # Dataset Card for Indian Sweets
| VishalMysore/Hindi_Mithai | [
"language:hi",
"license:apache-2.0",
"region:us"
] | 2024-01-10T01:37:24+00:00 | {"language": ["hi"], "license": "apache-2.0"} | 2024-01-10T14:56:41+00:00 | [] | [
"hi"
] | TAGS
#language-Hindi #license-apache-2.0 #region-us
| # Dataset Card for Indian Sweets
| [
"# Dataset Card for Indian Sweets"
] | [
"TAGS\n#language-Hindi #license-apache-2.0 #region-us \n",
"# Dataset Card for Indian Sweets"
] |
de4f517a81d79f63d33f4eb01ade2065a7ea397e | # Open-Orca-FLAN-50K-Synthetic-5-Models Dataset Card
### Dataset Summary
The Open-Orca-FLAN-50K-Synthetic-5-Models dataset is a large-scale, synthetic dataset based on 50K filtered examples from [Open-Orca/Flan](https://huggingface.co/datasets/Open-Orca/FLAN) . It contains 50,000 examples, each consisting of a prompt, a completion, and the corresponding task. Additionally, it includes model-generated responses from five different models: [ignos-Mistral-T5-7B-v1](https://huggingface.co/ignos/Mistral-T5-7B-v1), [cognAI-lil-c3po](https://huggingface.co/cognAI/lil-c3po), [viethq188-Rabbit-7B-DPO-Chat](https://huggingface.co/viethq188/Rabbit-7B-DPO-Chat), [cookinai-DonutLM-v1](https://huggingface.co/cookinai/DonutLM-v1), and [v1olet-v1olet-merged-dpo-7B](https://huggingface.co/v1olet/v1olet_merged_dpo_7B). This dataset is particularly useful for research in natural language understanding, language model comparison, and AI-generated text analysis.
### Supported Tasks
- **Natural Language Understanding:** The dataset can be used to train models to understand and generate human-like text.
- **Model Comparison:** Researchers can compare the performance of different language models using this dataset.
- **CoE Router Reward Modeling:** The responses from the 5 models can be used to train the routing mechanism given a query
- **Text Generation:** It's suitable for training and evaluating models on text generation tasks.
### Languages
The dataset is primarily in English.
## Dataset Structure
### Data Instances
A typical data instance comprises the following fields:
- `prompt`: The input prompt (string).
- `completion`: The expected completion of the prompt (string).
- `task`: The specific task or category the example belongs to (string).
- Model-generated responses from five different models, each in a separate field.
### Data Fields
- `prompt`: A string containing the input prompt.
- `completion`: A string containing the expected response or completion to the prompt.
- `task`: A string indicating the type of task.
- `ignos-Mistral-T5-7B-v1`: Model-generated response from ignos-Mistral-T5-7B-v1.
- `cognAI-lil-c3po`: Model-generated response from cognAI-lil-c3po.
- `viethq188-Rabbit-7B-DPO-Chat`: Model-generated response from viethq188-Rabbit-7B-DPO-Chat.
- `cookinai-DonutLM-v1`: Model-generated response from cookinai-DonutLM-v1.
- `v1olet-v1olet-merged-dpo-7B`: Model-generated response from v1olet-v1olet-merged-dpo-7B.
### Data Splits
The dataset is not split into traditional training, validation, and test sets. It contains 50,000 examples in a single batch, designed for evaluation and comparison purposes.
## Dataset Creation
### Curation Rationale
This dataset was curated to provide a diverse and extensive set of prompts and completions, along with responses from various state-of-the-art language models, for comprehensive evaluation and comparison in language understanding and generation tasks.
### Source Data
#### Initial Data Collection and Normalization
Data was synthetically generated, ensuring a wide variety of prompts, tasks, and model-generated responses.
#### Who are the source language producers?
The prompts and completions are from a known dataset, and the responses are produced by the specified language models.
### Annotations
The dataset does not include manual annotations. The responses are generated by the models listed.
### Personal and Sensitive Information
Since the dataset is synthetic, it does not contain any personal or sensitive information.
## Considerations for Using the Data
### Social Impact of Dataset
This dataset contributes to the advancement of natural language processing by providing a rich source for model comparison and analysis.
### Discussion of Biases
As the dataset is generated by AI models, it may inherit biases present in those models. Users should be aware of this when analyzing the data.
### Other Known Limitations
The effectiveness of the dataset is contingent on the quality and diversity of the synthetic data and the responses generated by the models.
### Licensing Information
Please refer to the repository for licensing information.
### Citation Information
```
@inproceedings{open-orca-flan-50k-synthetic-5-models,
title={Open-Orca-FLAN-50K-Synthetic-5-Models},
author={Kaizhao Liang}
}
``` | kz919/open-orca-flan-50k-synthetic-5-models | [
"task_categories:text-generation",
"size_categories:10K<n<100K",
"language:en",
"license:apache-2.0",
"region:us"
] | 2024-01-10T02:08:52+00:00 | {"language": ["en"], "license": "apache-2.0", "size_categories": ["10K<n<100K"], "task_categories": ["text-generation"], "pretty_name": "synthetic open-orca flan", "dataset_info": {"features": [{"name": "prompt", "dtype": "string"}, {"name": "completion", "dtype": "string"}, {"name": "task", "dtype": "string"}, {"name": "ignos-Mistral-T5-7B-v1", "dtype": "string"}, {"name": "cognAI-lil-c3po", "dtype": "string"}, {"name": "viethq188-Rabbit-7B-DPO-Chat", "dtype": "string"}, {"name": "cookinai-DonutLM-v1", "dtype": "string"}, {"name": "v1olet-v1olet-merged-dpo-7B", "dtype": "string"}], "splits": [{"name": "train", "num_bytes": 103557970, "num_examples": 50000}], "download_size": 47451297, "dataset_size": 103557970}, "configs": [{"config_name": "default", "data_files": [{"split": "train", "path": "data/train-*"}]}]} | 2024-01-12T20:37:03+00:00 | [] | [
"en"
] | TAGS
#task_categories-text-generation #size_categories-10K<n<100K #language-English #license-apache-2.0 #region-us
| # Open-Orca-FLAN-50K-Synthetic-5-Models Dataset Card
### Dataset Summary
The Open-Orca-FLAN-50K-Synthetic-5-Models dataset is a large-scale, synthetic dataset based on 50K filtered examples from Open-Orca/Flan . It contains 50,000 examples, each consisting of a prompt, a completion, and the corresponding task. Additionally, it includes model-generated responses from five different models: ignos-Mistral-T5-7B-v1, cognAI-lil-c3po, viethq188-Rabbit-7B-DPO-Chat, cookinai-DonutLM-v1, and v1olet-v1olet-merged-dpo-7B. This dataset is particularly useful for research in natural language understanding, language model comparison, and AI-generated text analysis.
### Supported Tasks
- Natural Language Understanding: The dataset can be used to train models to understand and generate human-like text.
- Model Comparison: Researchers can compare the performance of different language models using this dataset.
- CoE Router Reward Modeling: The responses from the 5 models can be used to train the routing mechanism given a query
- Text Generation: It's suitable for training and evaluating models on text generation tasks.
### Languages
The dataset is primarily in English.
## Dataset Structure
### Data Instances
A typical data instance comprises the following fields:
- 'prompt': The input prompt (string).
- 'completion': The expected completion of the prompt (string).
- 'task': The specific task or category the example belongs to (string).
- Model-generated responses from five different models, each in a separate field.
### Data Fields
- 'prompt': A string containing the input prompt.
- 'completion': A string containing the expected response or completion to the prompt.
- 'task': A string indicating the type of task.
- 'ignos-Mistral-T5-7B-v1': Model-generated response from ignos-Mistral-T5-7B-v1.
- 'cognAI-lil-c3po': Model-generated response from cognAI-lil-c3po.
- 'viethq188-Rabbit-7B-DPO-Chat': Model-generated response from viethq188-Rabbit-7B-DPO-Chat.
- 'cookinai-DonutLM-v1': Model-generated response from cookinai-DonutLM-v1.
- 'v1olet-v1olet-merged-dpo-7B': Model-generated response from v1olet-v1olet-merged-dpo-7B.
### Data Splits
The dataset is not split into traditional training, validation, and test sets. It contains 50,000 examples in a single batch, designed for evaluation and comparison purposes.
## Dataset Creation
### Curation Rationale
This dataset was curated to provide a diverse and extensive set of prompts and completions, along with responses from various state-of-the-art language models, for comprehensive evaluation and comparison in language understanding and generation tasks.
### Source Data
#### Initial Data Collection and Normalization
Data was synthetically generated, ensuring a wide variety of prompts, tasks, and model-generated responses.
#### Who are the source language producers?
The prompts and completions are from a known dataset, and the responses are produced by the specified language models.
### Annotations
The dataset does not include manual annotations. The responses are generated by the models listed.
### Personal and Sensitive Information
Since the dataset is synthetic, it does not contain any personal or sensitive information.
## Considerations for Using the Data
### Social Impact of Dataset
This dataset contributes to the advancement of natural language processing by providing a rich source for model comparison and analysis.
### Discussion of Biases
As the dataset is generated by AI models, it may inherit biases present in those models. Users should be aware of this when analyzing the data.
### Other Known Limitations
The effectiveness of the dataset is contingent on the quality and diversity of the synthetic data and the responses generated by the models.
### Licensing Information
Please refer to the repository for licensing information.
| [
"# Open-Orca-FLAN-50K-Synthetic-5-Models Dataset Card",
"### Dataset Summary\n\nThe Open-Orca-FLAN-50K-Synthetic-5-Models dataset is a large-scale, synthetic dataset based on 50K filtered examples from Open-Orca/Flan . It contains 50,000 examples, each consisting of a prompt, a completion, and the corresponding task. Additionally, it includes model-generated responses from five different models: ignos-Mistral-T5-7B-v1, cognAI-lil-c3po, viethq188-Rabbit-7B-DPO-Chat, cookinai-DonutLM-v1, and v1olet-v1olet-merged-dpo-7B. This dataset is particularly useful for research in natural language understanding, language model comparison, and AI-generated text analysis.",
"### Supported Tasks\n\n- Natural Language Understanding: The dataset can be used to train models to understand and generate human-like text.\n- Model Comparison: Researchers can compare the performance of different language models using this dataset.\n- CoE Router Reward Modeling: The responses from the 5 models can be used to train the routing mechanism given a query\n- Text Generation: It's suitable for training and evaluating models on text generation tasks.",
"### Languages\n\nThe dataset is primarily in English.",
"## Dataset Structure",
"### Data Instances\n\nA typical data instance comprises the following fields:\n- 'prompt': The input prompt (string).\n- 'completion': The expected completion of the prompt (string).\n- 'task': The specific task or category the example belongs to (string).\n- Model-generated responses from five different models, each in a separate field.",
"### Data Fields\n\n- 'prompt': A string containing the input prompt.\n- 'completion': A string containing the expected response or completion to the prompt.\n- 'task': A string indicating the type of task.\n- 'ignos-Mistral-T5-7B-v1': Model-generated response from ignos-Mistral-T5-7B-v1.\n- 'cognAI-lil-c3po': Model-generated response from cognAI-lil-c3po.\n- 'viethq188-Rabbit-7B-DPO-Chat': Model-generated response from viethq188-Rabbit-7B-DPO-Chat.\n- 'cookinai-DonutLM-v1': Model-generated response from cookinai-DonutLM-v1.\n- 'v1olet-v1olet-merged-dpo-7B': Model-generated response from v1olet-v1olet-merged-dpo-7B.",
"### Data Splits\n\nThe dataset is not split into traditional training, validation, and test sets. It contains 50,000 examples in a single batch, designed for evaluation and comparison purposes.",
"## Dataset Creation",
"### Curation Rationale\n\nThis dataset was curated to provide a diverse and extensive set of prompts and completions, along with responses from various state-of-the-art language models, for comprehensive evaluation and comparison in language understanding and generation tasks.",
"### Source Data",
"#### Initial Data Collection and Normalization\n\nData was synthetically generated, ensuring a wide variety of prompts, tasks, and model-generated responses.",
"#### Who are the source language producers?\n\nThe prompts and completions are from a known dataset, and the responses are produced by the specified language models.",
"### Annotations\n\nThe dataset does not include manual annotations. The responses are generated by the models listed.",
"### Personal and Sensitive Information\n\nSince the dataset is synthetic, it does not contain any personal or sensitive information.",
"## Considerations for Using the Data",
"### Social Impact of Dataset\n\nThis dataset contributes to the advancement of natural language processing by providing a rich source for model comparison and analysis.",
"### Discussion of Biases\n\nAs the dataset is generated by AI models, it may inherit biases present in those models. Users should be aware of this when analyzing the data.",
"### Other Known Limitations\n\nThe effectiveness of the dataset is contingent on the quality and diversity of the synthetic data and the responses generated by the models.",
"### Licensing Information\n\nPlease refer to the repository for licensing information."
] | [
"TAGS\n#task_categories-text-generation #size_categories-10K<n<100K #language-English #license-apache-2.0 #region-us \n",
"# Open-Orca-FLAN-50K-Synthetic-5-Models Dataset Card",
"### Dataset Summary\n\nThe Open-Orca-FLAN-50K-Synthetic-5-Models dataset is a large-scale, synthetic dataset based on 50K filtered examples from Open-Orca/Flan . It contains 50,000 examples, each consisting of a prompt, a completion, and the corresponding task. Additionally, it includes model-generated responses from five different models: ignos-Mistral-T5-7B-v1, cognAI-lil-c3po, viethq188-Rabbit-7B-DPO-Chat, cookinai-DonutLM-v1, and v1olet-v1olet-merged-dpo-7B. This dataset is particularly useful for research in natural language understanding, language model comparison, and AI-generated text analysis.",
"### Supported Tasks\n\n- Natural Language Understanding: The dataset can be used to train models to understand and generate human-like text.\n- Model Comparison: Researchers can compare the performance of different language models using this dataset.\n- CoE Router Reward Modeling: The responses from the 5 models can be used to train the routing mechanism given a query\n- Text Generation: It's suitable for training and evaluating models on text generation tasks.",
"### Languages\n\nThe dataset is primarily in English.",
"## Dataset Structure",
"### Data Instances\n\nA typical data instance comprises the following fields:\n- 'prompt': The input prompt (string).\n- 'completion': The expected completion of the prompt (string).\n- 'task': The specific task or category the example belongs to (string).\n- Model-generated responses from five different models, each in a separate field.",
"### Data Fields\n\n- 'prompt': A string containing the input prompt.\n- 'completion': A string containing the expected response or completion to the prompt.\n- 'task': A string indicating the type of task.\n- 'ignos-Mistral-T5-7B-v1': Model-generated response from ignos-Mistral-T5-7B-v1.\n- 'cognAI-lil-c3po': Model-generated response from cognAI-lil-c3po.\n- 'viethq188-Rabbit-7B-DPO-Chat': Model-generated response from viethq188-Rabbit-7B-DPO-Chat.\n- 'cookinai-DonutLM-v1': Model-generated response from cookinai-DonutLM-v1.\n- 'v1olet-v1olet-merged-dpo-7B': Model-generated response from v1olet-v1olet-merged-dpo-7B.",
"### Data Splits\n\nThe dataset is not split into traditional training, validation, and test sets. It contains 50,000 examples in a single batch, designed for evaluation and comparison purposes.",
"## Dataset Creation",
"### Curation Rationale\n\nThis dataset was curated to provide a diverse and extensive set of prompts and completions, along with responses from various state-of-the-art language models, for comprehensive evaluation and comparison in language understanding and generation tasks.",
"### Source Data",
"#### Initial Data Collection and Normalization\n\nData was synthetically generated, ensuring a wide variety of prompts, tasks, and model-generated responses.",
"#### Who are the source language producers?\n\nThe prompts and completions are from a known dataset, and the responses are produced by the specified language models.",
"### Annotations\n\nThe dataset does not include manual annotations. The responses are generated by the models listed.",
"### Personal and Sensitive Information\n\nSince the dataset is synthetic, it does not contain any personal or sensitive information.",
"## Considerations for Using the Data",
"### Social Impact of Dataset\n\nThis dataset contributes to the advancement of natural language processing by providing a rich source for model comparison and analysis.",
"### Discussion of Biases\n\nAs the dataset is generated by AI models, it may inherit biases present in those models. Users should be aware of this when analyzing the data.",
"### Other Known Limitations\n\nThe effectiveness of the dataset is contingent on the quality and diversity of the synthetic data and the responses generated by the models.",
"### Licensing Information\n\nPlease refer to the repository for licensing information."
] |
e95858d016a7159c9e5cb7273ed3bb8ffef777e7 | # Dataset Card for "nft_prediction_all_with_dates"
[More Information needed](https://github.com/huggingface/datasets/blob/main/CONTRIBUTING.md#how-to-contribute-to-the-dataset-cards) | hongerzh/nft_prediction_all_with_dates | [
"region:us"
] | 2024-01-10T02:45:19+00:00 | {"configs": [{"config_name": "default", "data_files": [{"split": "train", "path": "data/train-*"}, {"split": "validation", "path": "data/validation-*"}, {"split": "test", "path": "data/test-*"}]}], "dataset_info": {"features": [{"name": "image", "dtype": "image"}, {"name": "label", "dtype": "float64"}, {"name": "time", "dtype": "float64"}, {"name": "text", "dtype": "string"}], "splits": [{"name": "train", "num_bytes": 5747708188.67, "num_examples": 29339}, {"name": "validation", "num_bytes": 1910519375.185, "num_examples": 9777}, {"name": "test", "num_bytes": 2129490317.38, "num_examples": 9780}], "download_size": 9022605212, "dataset_size": 9787717881.235}} | 2024-01-10T03:51:44+00:00 | [] | [] | TAGS
#region-us
| # Dataset Card for "nft_prediction_all_with_dates"
More Information needed | [
"# Dataset Card for \"nft_prediction_all_with_dates\"\n\nMore Information needed"
] | [
"TAGS\n#region-us \n",
"# Dataset Card for \"nft_prediction_all_with_dates\"\n\nMore Information needed"
] |
84892d5711e2f6996463bb5a9941f47bd21e96c1 | # tokenspace directory
This directory contains utilities for the purpose of browsing the
"token space" of CLIP ViT-L/14
Primary tools are:
* "calculate-distances.py": allows command-line browsing of words and their neighbours
* "graph-embeddings.py": plots graph of full values of two embeddings
## (clipmodel,cliptextmodel)-calculate-distances.py
Loads the generated embeddings, reads in a word, calculates "distance" to every
embedding, and then shows the closest "neighbours".
To run this requires the files "embeddings.safetensors" and "dictionary",
in matching format
You will need to rename or copy appropriate files for this as mentioned below.
Note that SD models use cliptextmodel, NOT clipmodel
## graph-textmodels.py
Shows the difference between the same word, embedded by CLIPTextModel
vs CLIPModel
## graph-embeddings.py
Run the script. It will ask you for two text strings.
Once you enter both, it will plot the graph and display it for you
Note that this tool does not require any of the other files; just that you
have the requisite python modules installed. (pip install -r requirements.txt)
### embeddings.safetensors
You can either copy one of the provided files, or generate your own.
See generate-embeddings.py for that.
Note that you muist always use the "dictionary" file that matchnes your embeddings file
### embeddings.allids.safetensors
DO NOT USE THIS ONE for programs that expect a matching dictionary.
This one is purely numeric based.
Its intention is more for research datamining, but it does have a matching
graph front end, graph-byid.py
### dictionary
Make sure to always use the dictionary file that matches your embeddings file.
The "dictionary.fullword" file is pulled from fullword.json, which is distilled from "full words"
present in the ViT-L/14 CLIP model's provided token dictionary, called "vocab.json".
Thus there are only around 30,000 words in it
If you want to use the provided "embeddings.safetensors.huge" file, you will want to use the matching
"dictionary.huge" file, which has over 300,000 words
This huge file comes from the linux "wamerican-huge" package, which delivers it under
/usr/share/dict/american-english-huge
There also exists a "american-insane" package
## generate-embeddings.py
Generates the "embeddings.safetensor" file, based on the "dictionary" file present.
Takes a few minutes to run, depending on size of the dictionary
The shape of the embeddings tensor, is
[number-of-words][768]
Note that yes, it is possible to directly pull a tensor from the CLIP model,
using keyname of text_model.embeddings.token_embedding.weight
This will NOT GIVE YOU THE RIGHT DISTANCES!
Hence why we are calculating and then storing the embedding weights actually
generated by the CLIP process
## fullword.json
This file contains a collection of "one word, one CLIP token id" pairings.
The file was taken from vocab.json, which is part of multiple SD models in huggingface.co
The file was optimized for what people are actually going to type as words.
First all the non-(/w) entries were stripped out.
Then all the garbage punctuation and foreign characters were stripped out.
Finally, the actual (/w) was stripped out, for ease of use.
| ppbrown/tokenspace | [
"region:us"
] | 2024-01-10T02:54:05+00:00 | {} | 2024-01-26T04:25:37+00:00 | [] | [] | TAGS
#region-us
| # tokenspace directory
This directory contains utilities for the purpose of browsing the
"token space" of CLIP ViT-L/14
Primary tools are:
* "URL": allows command-line browsing of words and their neighbours
* "URL": plots graph of full values of two embeddings
## (clipmodel,cliptextmodel)-URL
Loads the generated embeddings, reads in a word, calculates "distance" to every
embedding, and then shows the closest "neighbours".
To run this requires the files "embeddings.safetensors" and "dictionary",
in matching format
You will need to rename or copy appropriate files for this as mentioned below.
Note that SD models use cliptextmodel, NOT clipmodel
## URL
Shows the difference between the same word, embedded by CLIPTextModel
vs CLIPModel
## URL
Run the script. It will ask you for two text strings.
Once you enter both, it will plot the graph and display it for you
Note that this tool does not require any of the other files; just that you
have the requisite python modules installed. (pip install -r URL)
### embeddings.safetensors
You can either copy one of the provided files, or generate your own.
See URL for that.
Note that you muist always use the "dictionary" file that matchnes your embeddings file
### URL.safetensors
DO NOT USE THIS ONE for programs that expect a matching dictionary.
This one is purely numeric based.
Its intention is more for research datamining, but it does have a matching
graph front end, URL
### dictionary
Make sure to always use the dictionary file that matches your embeddings file.
The "dictionary.fullword" file is pulled from URL, which is distilled from "full words"
present in the ViT-L/14 CLIP model's provided token dictionary, called "URL".
Thus there are only around 30,000 words in it
If you want to use the provided "URL" file, you will want to use the matching
"URL" file, which has over 300,000 words
This huge file comes from the linux "wamerican-huge" package, which delivers it under
/usr/share/dict/american-english-huge
There also exists a "american-insane" package
## URL
Generates the "embeddings.safetensor" file, based on the "dictionary" file present.
Takes a few minutes to run, depending on size of the dictionary
The shape of the embeddings tensor, is
[number-of-words][768]
Note that yes, it is possible to directly pull a tensor from the CLIP model,
using keyname of text_model.embeddings.token_embedding.weight
This will NOT GIVE YOU THE RIGHT DISTANCES!
Hence why we are calculating and then storing the embedding weights actually
generated by the CLIP process
## URL
This file contains a collection of "one word, one CLIP token id" pairings.
The file was taken from URL, which is part of multiple SD models in URL
The file was optimized for what people are actually going to type as words.
First all the non-(/w) entries were stripped out.
Then all the garbage punctuation and foreign characters were stripped out.
Finally, the actual (/w) was stripped out, for ease of use.
| [
"# tokenspace directory\n\nThis directory contains utilities for the purpose of browsing the\n\"token space\" of CLIP ViT-L/14\n\nPrimary tools are:\n\n* \"URL\": allows command-line browsing of words and their neighbours\n* \"URL\": plots graph of full values of two embeddings",
"## (clipmodel,cliptextmodel)-URL\n\nLoads the generated embeddings, reads in a word, calculates \"distance\" to every\nembedding, and then shows the closest \"neighbours\".\n\nTo run this requires the files \"embeddings.safetensors\" and \"dictionary\",\nin matching format\n\nYou will need to rename or copy appropriate files for this as mentioned below.\n\nNote that SD models use cliptextmodel, NOT clipmodel",
"## URL\n\nShows the difference between the same word, embedded by CLIPTextModel\nvs CLIPModel",
"## URL\n\nRun the script. It will ask you for two text strings. \nOnce you enter both, it will plot the graph and display it for you\n\nNote that this tool does not require any of the other files; just that you \nhave the requisite python modules installed. (pip install -r URL)",
"### embeddings.safetensors\n\nYou can either copy one of the provided files, or generate your own.\nSee URL for that. \n\nNote that you muist always use the \"dictionary\" file that matchnes your embeddings file",
"### URL.safetensors\n\nDO NOT USE THIS ONE for programs that expect a matching dictionary.\nThis one is purely numeric based. \nIts intention is more for research datamining, but it does have a matching\ngraph front end, URL",
"### dictionary\n\nMake sure to always use the dictionary file that matches your embeddings file.\n\nThe \"dictionary.fullword\" file is pulled from URL, which is distilled from \"full words\"\npresent in the ViT-L/14 CLIP model's provided token dictionary, called \"URL\".\nThus there are only around 30,000 words in it\n\nIf you want to use the provided \"URL\" file, you will want to use the matching\n\"URL\" file, which has over 300,000 words\n\nThis huge file comes from the linux \"wamerican-huge\" package, which delivers it under\n/usr/share/dict/american-english-huge\n\nThere also exists a \"american-insane\" package",
"## URL\n\nGenerates the \"embeddings.safetensor\" file, based on the \"dictionary\" file present.\nTakes a few minutes to run, depending on size of the dictionary\n\nThe shape of the embeddings tensor, is\n [number-of-words][768]\n\nNote that yes, it is possible to directly pull a tensor from the CLIP model,\nusing keyname of text_model.embeddings.token_embedding.weight\n\nThis will NOT GIVE YOU THE RIGHT DISTANCES!\nHence why we are calculating and then storing the embedding weights actually\ngenerated by the CLIP process",
"## URL\n\nThis file contains a collection of \"one word, one CLIP token id\" pairings.\nThe file was taken from URL, which is part of multiple SD models in URL\n\nThe file was optimized for what people are actually going to type as words.\nFirst all the non-(/w) entries were stripped out.\nThen all the garbage punctuation and foreign characters were stripped out.\nFinally, the actual (/w) was stripped out, for ease of use."
] | [
"TAGS\n#region-us \n",
"# tokenspace directory\n\nThis directory contains utilities for the purpose of browsing the\n\"token space\" of CLIP ViT-L/14\n\nPrimary tools are:\n\n* \"URL\": allows command-line browsing of words and their neighbours\n* \"URL\": plots graph of full values of two embeddings",
"## (clipmodel,cliptextmodel)-URL\n\nLoads the generated embeddings, reads in a word, calculates \"distance\" to every\nembedding, and then shows the closest \"neighbours\".\n\nTo run this requires the files \"embeddings.safetensors\" and \"dictionary\",\nin matching format\n\nYou will need to rename or copy appropriate files for this as mentioned below.\n\nNote that SD models use cliptextmodel, NOT clipmodel",
"## URL\n\nShows the difference between the same word, embedded by CLIPTextModel\nvs CLIPModel",
"## URL\n\nRun the script. It will ask you for two text strings. \nOnce you enter both, it will plot the graph and display it for you\n\nNote that this tool does not require any of the other files; just that you \nhave the requisite python modules installed. (pip install -r URL)",
"### embeddings.safetensors\n\nYou can either copy one of the provided files, or generate your own.\nSee URL for that. \n\nNote that you muist always use the \"dictionary\" file that matchnes your embeddings file",
"### URL.safetensors\n\nDO NOT USE THIS ONE for programs that expect a matching dictionary.\nThis one is purely numeric based. \nIts intention is more for research datamining, but it does have a matching\ngraph front end, URL",
"### dictionary\n\nMake sure to always use the dictionary file that matches your embeddings file.\n\nThe \"dictionary.fullword\" file is pulled from URL, which is distilled from \"full words\"\npresent in the ViT-L/14 CLIP model's provided token dictionary, called \"URL\".\nThus there are only around 30,000 words in it\n\nIf you want to use the provided \"URL\" file, you will want to use the matching\n\"URL\" file, which has over 300,000 words\n\nThis huge file comes from the linux \"wamerican-huge\" package, which delivers it under\n/usr/share/dict/american-english-huge\n\nThere also exists a \"american-insane\" package",
"## URL\n\nGenerates the \"embeddings.safetensor\" file, based on the \"dictionary\" file present.\nTakes a few minutes to run, depending on size of the dictionary\n\nThe shape of the embeddings tensor, is\n [number-of-words][768]\n\nNote that yes, it is possible to directly pull a tensor from the CLIP model,\nusing keyname of text_model.embeddings.token_embedding.weight\n\nThis will NOT GIVE YOU THE RIGHT DISTANCES!\nHence why we are calculating and then storing the embedding weights actually\ngenerated by the CLIP process",
"## URL\n\nThis file contains a collection of \"one word, one CLIP token id\" pairings.\nThe file was taken from URL, which is part of multiple SD models in URL\n\nThe file was optimized for what people are actually going to type as words.\nFirst all the non-(/w) entries were stripped out.\nThen all the garbage punctuation and foreign characters were stripped out.\nFinally, the actual (/w) was stripped out, for ease of use."
] |
cef03a2830944bfb0d201107895ddd0e0e90bf0e |
# Dataset Card for InFoBench Dataset
## Table of Contents
- [Dataset Description](#dataset-description)
- [Dataset Usage](#dataset-usage)
- [Dataset Structure](#dataset-structure)
- [Data Instances](#data-instances)
- [Data Fields](#data-fields)
- [Additional Information](#additional-information)
- [Licensing Information](#licensing-information)
- [Citation Information](#citation-information)
## Dataset Description
- **Repository:** [InFoBench Repository](https://github.com/qinyiwei/InfoBench)
- **Paper:** [InFoBench: Evaluating Instruction Following Ability in Large Language Models](https://arxiv.org/pdf/2401.03601.pdf)
The InFoBench Dataset is an evaluation benchmark dataset containing 500 instructions and corresponding 2250 decomposed requirements.
## Dataset Usage
You can directly download it with huggingface datasets.
``` python
from datasets import load_dataset
dataset = load_dataset("kqsong/InFoBench")
```
## Dataset Structure
### Data Instances
For each instance, there is an instruction string, an input string (optional), a list of decomposed questions, and a list of the labels for each decomposed question.
```json
{
"id": "domain_oriented_task_215",
"input": "",
"category": "Business and Economics: Business Administration",
"instruction": "Generate a non-disclosure agreement of two pages (each page is limited to 250 words) for a software development project involving Party A and Party B. The confidentiality duration should be 5 years. \n\nThe first page should include definitions for key terms such as 'confidential information', 'disclosure', and 'recipient'. \n\nOn the second page, provide clauses detailing the protocol for the return or destruction of confidential information, exceptions to maintaining confidentiality, and the repercussions following a breach of the agreement. \n\nPlease indicate the separation between the first and second pages with a full line of dashed lines ('-----'). Also, make sure that each page is clearly labeled with its respective page number.",
"decomposed_questions": [
"Is the generated text a non-disclosure agreement?",
"Does the generated text consist of two pages?",
"Is each page of the generated text limited to 250 words?",
"Is the generated non-disclosure agreement for a software development project involving Party A and Party B?",
"Does the generated non-disclosure agreement specify a confidentiality duration of 5 years?",
"Does the first page of the generated non-disclosure agreement include definitions for key terms such as 'confidential information', 'disclosure', and 'recipient'?",
"Does the second page of the generated non-disclosure agreement provide clauses detailing the protocol for the return or destruction of confidential information?",
"Does the second page of the generated non-disclosure agreement provide exceptions to maintaining confidentiality?",
"Does the second page of the generated non-disclosure agreement provide the repercussions following a breach of the agreement?",
"Does the generated text indicate the separation between the first and second pages with a full line of dashed lines ('-----')?",
"Does the generated text ensure that each page is clearly labeled with its respective page number?"
],
"subset": "Hard_set",
"question_label": [
["Format"],
["Format", "Number"],
["Number"],
["Content"],
["Content"],
["Format", "Content"],
["Content"],
["Content"],
["Content"],
["Format"],
["Format"]
]
}
```
### Data Fields
- `id`: a string.
- `subset`: `Hard_Set` or `Easy_Set`.
- `category`: a string containing categorical information.
- `instruction`: a string containing instructions.
- `input`: a string, containing the context information, could be an empty string.
- `decomposed_questions`: a list of strings, each corresponding to a decomposed requirement.
- `question_label`: a list of list of strings, each list of strings containing a series of labels for the corresponding decomposed questions.
## Additional Information
### Licensing Information
The InFoBench Dataset version 1.0.0 is released under the [MIT LISENCE](https://github.com/qinyiwei/InfoBench/blob/main/LICENSE)
### Citation Information
```
@article{qin2024infobench,
title={InFoBench: Evaluating Instruction Following Ability in Large Language Models},
author={Yiwei Qin and Kaiqiang Song and Yebowen Hu and Wenlin Yao and Sangwoo Cho and Xiaoyang Wang and Xuansheng Wu and Fei Liu and Pengfei Liu and Dong Yu},
year={2024},
eprint={2401.03601},
archivePrefix={arXiv},
primaryClass={cs.CL}
}
``` | kqsong/InFoBench | [
"size_categories:n<1K",
"language:en",
"license:mit",
"arxiv:2401.03601",
"region:us"
] | 2024-01-10T02:58:20+00:00 | {"language": ["en"], "license": "mit", "size_categories": ["n<1K"], "pretty_name": "InfoBench"} | 2024-01-10T03:39:46+00:00 | [
"2401.03601"
] | [
"en"
] | TAGS
#size_categories-n<1K #language-English #license-mit #arxiv-2401.03601 #region-us
|
# Dataset Card for InFoBench Dataset
## Table of Contents
- Dataset Description
- Dataset Usage
- Dataset Structure
- Data Instances
- Data Fields
- Additional Information
- Licensing Information
- Citation Information
## Dataset Description
- Repository: InFoBench Repository
- Paper: InFoBench: Evaluating Instruction Following Ability in Large Language Models
The InFoBench Dataset is an evaluation benchmark dataset containing 500 instructions and corresponding 2250 decomposed requirements.
## Dataset Usage
You can directly download it with huggingface datasets.
## Dataset Structure
### Data Instances
For each instance, there is an instruction string, an input string (optional), a list of decomposed questions, and a list of the labels for each decomposed question.
### Data Fields
- 'id': a string.
- 'subset': 'Hard_Set' or 'Easy_Set'.
- 'category': a string containing categorical information.
- 'instruction': a string containing instructions.
- 'input': a string, containing the context information, could be an empty string.
- 'decomposed_questions': a list of strings, each corresponding to a decomposed requirement.
- 'question_label': a list of list of strings, each list of strings containing a series of labels for the corresponding decomposed questions.
## Additional Information
### Licensing Information
The InFoBench Dataset version 1.0.0 is released under the MIT LISENCE
| [
"# Dataset Card for InFoBench Dataset",
"## Table of Contents\n- Dataset Description\n- Dataset Usage\n- Dataset Structure\n - Data Instances\n - Data Fields\n- Additional Information\n - Licensing Information\n - Citation Information",
"## Dataset Description\n\n- Repository: InFoBench Repository\n- Paper: InFoBench: Evaluating Instruction Following Ability in Large Language Models\n\nThe InFoBench Dataset is an evaluation benchmark dataset containing 500 instructions and corresponding 2250 decomposed requirements.",
"## Dataset Usage\nYou can directly download it with huggingface datasets.",
"## Dataset Structure",
"### Data Instances\nFor each instance, there is an instruction string, an input string (optional), a list of decomposed questions, and a list of the labels for each decomposed question.",
"### Data Fields\n- 'id': a string.\n- 'subset': 'Hard_Set' or 'Easy_Set'.\n- 'category': a string containing categorical information.\n- 'instruction': a string containing instructions.\n- 'input': a string, containing the context information, could be an empty string.\n- 'decomposed_questions': a list of strings, each corresponding to a decomposed requirement.\n- 'question_label': a list of list of strings, each list of strings containing a series of labels for the corresponding decomposed questions.",
"## Additional Information",
"### Licensing Information\nThe InFoBench Dataset version 1.0.0 is released under the MIT LISENCE"
] | [
"TAGS\n#size_categories-n<1K #language-English #license-mit #arxiv-2401.03601 #region-us \n",
"# Dataset Card for InFoBench Dataset",
"## Table of Contents\n- Dataset Description\n- Dataset Usage\n- Dataset Structure\n - Data Instances\n - Data Fields\n- Additional Information\n - Licensing Information\n - Citation Information",
"## Dataset Description\n\n- Repository: InFoBench Repository\n- Paper: InFoBench: Evaluating Instruction Following Ability in Large Language Models\n\nThe InFoBench Dataset is an evaluation benchmark dataset containing 500 instructions and corresponding 2250 decomposed requirements.",
"## Dataset Usage\nYou can directly download it with huggingface datasets.",
"## Dataset Structure",
"### Data Instances\nFor each instance, there is an instruction string, an input string (optional), a list of decomposed questions, and a list of the labels for each decomposed question.",
"### Data Fields\n- 'id': a string.\n- 'subset': 'Hard_Set' or 'Easy_Set'.\n- 'category': a string containing categorical information.\n- 'instruction': a string containing instructions.\n- 'input': a string, containing the context information, could be an empty string.\n- 'decomposed_questions': a list of strings, each corresponding to a decomposed requirement.\n- 'question_label': a list of list of strings, each list of strings containing a series of labels for the corresponding decomposed questions.",
"## Additional Information",
"### Licensing Information\nThe InFoBench Dataset version 1.0.0 is released under the MIT LISENCE"
] |
74fdf155be0a101e0b828de97f4e812128e77d99 |
# Jobstreet Webscraping

The data was scraped from jobstreet malaysia site with search keyword *data scientist* using beautifulsoup4 object. | azrai99/data-scientist-jobstreet-dataset | [
"size_categories:n<1K",
"license:apache-2.0",
"region:us"
] | 2024-01-10T02:59:25+00:00 | {"license": "apache-2.0", "size_categories": ["n<1K"]} | 2024-01-10T04:25:18+00:00 | [] | [] | TAGS
#size_categories-n<1K #license-apache-2.0 #region-us
|
# Jobstreet Webscraping
!alt text
The data was scraped from jobstreet malaysia site with search keyword *data scientist* using beautifulsoup4 object. | [
"# Jobstreet Webscraping\n!alt text\n\n\nThe data was scraped from jobstreet malaysia site with search keyword *data scientist* using beautifulsoup4 object."
] | [
"TAGS\n#size_categories-n<1K #license-apache-2.0 #region-us \n",
"# Jobstreet Webscraping\n!alt text\n\n\nThe data was scraped from jobstreet malaysia site with search keyword *data scientist* using beautifulsoup4 object."
] |
d098baf1634de3b3c8206ee04d266f0104fa1961 | # Dataset Card for "dolphin-coder-templated-ia-flat"
[More Information needed](https://github.com/huggingface/datasets/blob/main/CONTRIBUTING.md#how-to-contribute-to-the-dataset-cards) | sordonia/dolphin-coder-templated-ia-flat | [
"region:us"
] | 2024-01-10T03:19:32+00:00 | {"configs": [{"config_name": "default", "data_files": [{"split": "train", "path": "data/train-*"}]}], "dataset_info": {"features": [{"name": "system_prompt", "dtype": "string"}, {"name": "source", "dtype": "string"}, {"name": "target", "dtype": "string"}, {"name": "task_name", "dtype": "string"}, {"name": "task_source", "dtype": "string"}, {"name": "split", "dtype": "string"}], "splits": [{"name": "train", "num_bytes": 302327245, "num_examples": 109118}], "download_size": 57056453, "dataset_size": 302327245}} | 2024-01-10T03:19:36+00:00 | [] | [] | TAGS
#region-us
| # Dataset Card for "dolphin-coder-templated-ia-flat"
More Information needed | [
"# Dataset Card for \"dolphin-coder-templated-ia-flat\"\n\nMore Information needed"
] | [
"TAGS\n#region-us \n",
"# Dataset Card for \"dolphin-coder-templated-ia-flat\"\n\nMore Information needed"
] |
7a45a48a83943132287b175008a6bcea686fd3f9 |
# Korean Effective Crowdsourcing of Multiple Tasks (ECMT) for Comprehensive Knowledge Extraction
- Project: https://github.com/machinereading/crowdsourcing
- Data source: https://figshare.com/s/7367aeca244efae03068
## Details
Annotated text from Korean Wikipedia and KBox (Korean DBpedia). Includes a crowd sourced training set and expert annotated (reviewed by four experts) test set.
The dataset was annotated by crowdworks in multiple stages.
* Phase I: entity mention detection annotation; candidate entity mentions are selected in a text
* Phase II: entity linking annotation; candidate mentions can be linked to a knowledge base
* Phase III: coreference annotation; entities can be linked to pronouns, demonstrative determiners, and antecedent mentions
* Phase IV: relation extraction annotation; relations between entities are annotated
### Annotation Notes
#### Phase I
* For each mention, the annotator selects a category from one of 16 options: person, study field, theory, artifact, organization, location, civilization, event, year, time, quantity, job, animal, plant, material, and term.
* Entities can be things, concepts, ideas, or events:
```
개체란 다른 것들과 분리되어 존재하는 것으로, 개체는 물질적 존재일 필요는 없으며 개념적 아이디어 혹은 사건도 될 수 있다 개체의 대표적인 범주에는 사람, 물체, 조직, 기관, 장소, 시간, 사건 등이 포함된다
```
* Compound nouns are tagged with the largest span:
```
복합명사인 경우 가장 넓은 단위로 태깅해주세요 ex) [상하이] [디즈니랜드] -> [상하이 디즈니랜드]
```
* Final result is created by merging annotations from two separate annotators.
#### Phase II
* For each mention, a list of candidates from the knowledge base are shown. The annotator can select a candidate, not in candidate list, or not an entity.
* Each document was annotated by a single annotator.
#### Phase III
* For each mention, the annotator can select a preceding mention, no antecedent, or error. Noun phrases and pronouns are extracted using the parse information.
* "We scaled down the coreference resolution by limiting the scope of the target mentions to a named entity, pronoun, and definite noun phrase."
* Postfixes particles (조사) are not included in the antecedent:
```
[작업대상] 아래 항목에서 조사등을 제외(교정)해 주세요. 그녀는 -> 그녀
```
## Citation
```
@inproceedings{nam-etal-2020-effective,
title = "Effective Crowdsourcing of Multiple Tasks for Comprehensive Knowledge Extraction",
author = "Nam, Sangha and
Lee, Minho and
Kim, Donghwan and
Han, Kijong and
Kim, Kuntae and
Yoon, Sooji and
Kim, Eun-kyung and
Choi, Key-Sun",
editor = "Calzolari, Nicoletta and
B{\'e}chet, Fr{\'e}d{\'e}ric and
Blache, Philippe and
Choukri, Khalid and
Cieri, Christopher and
Declerck, Thierry and
Goggi, Sara and
Isahara, Hitoshi and
Maegaard, Bente and
Mariani, Joseph and
Mazo, H{\'e}l{\`e}ne and
Moreno, Asuncion and
Odijk, Jan and
Piperidis, Stelios",
booktitle = "Proceedings of the Twelfth Language Resources and Evaluation Conference",
month = may,
year = "2020",
address = "Marseille, France",
publisher = "European Language Resources Association",
url = "https://aclanthology.org/2020.lrec-1.27",
pages = "212--219",
abstract = "Information extraction from unstructured texts plays a vital role in the field of natural language processing. Although there has been extensive research into each information extraction task (i.e., entity linking, coreference resolution, and relation extraction), data are not available for a continuous and coherent evaluation of all information extraction tasks in a comprehensive framework. Given that each task is performed and evaluated with a different dataset, analyzing the effect of the previous task on the next task with a single dataset throughout the information extraction process is impossible. This paper aims to propose a Korean information extraction initiative point and promote research in this field by presenting crowdsourcing data collected for four information extraction tasks from the same corpus and the training and evaluation results for each task of a state-of-the-art model. These machine learning data for Korean information extraction are the first of their kind, and there are plans to continuously increase the data volume. The test results will serve as an initiative result for each Korean information extraction task and are expected to serve as a comparison target for various studies on Korean information extraction using the data collected in this study.",
language = "English",
ISBN = "979-10-95546-34-4",
}
``` | coref-data/korean_ecmt_raw | [
"license:cc-by-nc-sa-4.0",
"region:us"
] | 2024-01-10T03:44:59+00:00 | {"license": "cc-by-nc-sa-4.0"} | 2024-01-19T00:03:42+00:00 | [] | [] | TAGS
#license-cc-by-nc-sa-4.0 #region-us
|
# Korean Effective Crowdsourcing of Multiple Tasks (ECMT) for Comprehensive Knowledge Extraction
- Project: URL
- Data source: URL
## Details
Annotated text from Korean Wikipedia and KBox (Korean DBpedia). Includes a crowd sourced training set and expert annotated (reviewed by four experts) test set.
The dataset was annotated by crowdworks in multiple stages.
* Phase I: entity mention detection annotation; candidate entity mentions are selected in a text
* Phase II: entity linking annotation; candidate mentions can be linked to a knowledge base
* Phase III: coreference annotation; entities can be linked to pronouns, demonstrative determiners, and antecedent mentions
* Phase IV: relation extraction annotation; relations between entities are annotated
### Annotation Notes
#### Phase I
* For each mention, the annotator selects a category from one of 16 options: person, study field, theory, artifact, organization, location, civilization, event, year, time, quantity, job, animal, plant, material, and term.
* Entities can be things, concepts, ideas, or events:
* Compound nouns are tagged with the largest span:
* Final result is created by merging annotations from two separate annotators.
#### Phase II
* For each mention, a list of candidates from the knowledge base are shown. The annotator can select a candidate, not in candidate list, or not an entity.
* Each document was annotated by a single annotator.
#### Phase III
* For each mention, the annotator can select a preceding mention, no antecedent, or error. Noun phrases and pronouns are extracted using the parse information.
* "We scaled down the coreference resolution by limiting the scope of the target mentions to a named entity, pronoun, and definite noun phrase."
* Postfixes particles (조사) are not included in the antecedent:
| [
"# Korean Effective Crowdsourcing of Multiple Tasks (ECMT) for Comprehensive Knowledge Extraction\n\n- Project: URL\n- Data source: URL",
"## Details\n\nAnnotated text from Korean Wikipedia and KBox (Korean DBpedia). Includes a crowd sourced training set and expert annotated (reviewed by four experts) test set.\n\nThe dataset was annotated by crowdworks in multiple stages.\n* Phase I: entity mention detection annotation; candidate entity mentions are selected in a text\n* Phase II: entity linking annotation; candidate mentions can be linked to a knowledge base\n* Phase III: coreference annotation; entities can be linked to pronouns, demonstrative determiners, and antecedent mentions\n* Phase IV: relation extraction annotation; relations between entities are annotated",
"### Annotation Notes",
"#### Phase I\n* For each mention, the annotator selects a category from one of 16 options: person, study field, theory, artifact, organization, location, civilization, event, year, time, quantity, job, animal, plant, material, and term.\n* Entities can be things, concepts, ideas, or events:\n\n* Compound nouns are tagged with the largest span:\n\n* Final result is created by merging annotations from two separate annotators.",
"#### Phase II\n* For each mention, a list of candidates from the knowledge base are shown. The annotator can select a candidate, not in candidate list, or not an entity.\n* Each document was annotated by a single annotator.",
"#### Phase III\n* For each mention, the annotator can select a preceding mention, no antecedent, or error. Noun phrases and pronouns are extracted using the parse information.\n* \"We scaled down the coreference resolution by limiting the scope of the target mentions to a named entity, pronoun, and definite noun phrase.\"\n* Postfixes particles (조사) are not included in the antecedent:"
] | [
"TAGS\n#license-cc-by-nc-sa-4.0 #region-us \n",
"# Korean Effective Crowdsourcing of Multiple Tasks (ECMT) for Comprehensive Knowledge Extraction\n\n- Project: URL\n- Data source: URL",
"## Details\n\nAnnotated text from Korean Wikipedia and KBox (Korean DBpedia). Includes a crowd sourced training set and expert annotated (reviewed by four experts) test set.\n\nThe dataset was annotated by crowdworks in multiple stages.\n* Phase I: entity mention detection annotation; candidate entity mentions are selected in a text\n* Phase II: entity linking annotation; candidate mentions can be linked to a knowledge base\n* Phase III: coreference annotation; entities can be linked to pronouns, demonstrative determiners, and antecedent mentions\n* Phase IV: relation extraction annotation; relations between entities are annotated",
"### Annotation Notes",
"#### Phase I\n* For each mention, the annotator selects a category from one of 16 options: person, study field, theory, artifact, organization, location, civilization, event, year, time, quantity, job, animal, plant, material, and term.\n* Entities can be things, concepts, ideas, or events:\n\n* Compound nouns are tagged with the largest span:\n\n* Final result is created by merging annotations from two separate annotators.",
"#### Phase II\n* For each mention, a list of candidates from the knowledge base are shown. The annotator can select a candidate, not in candidate list, or not an entity.\n* Each document was annotated by a single annotator.",
"#### Phase III\n* For each mention, the annotator can select a preceding mention, no antecedent, or error. Noun phrases and pronouns are extracted using the parse information.\n* \"We scaled down the coreference resolution by limiting the scope of the target mentions to a named entity, pronoun, and definite noun phrase.\"\n* Postfixes particles (조사) are not included in the antecedent:"
] |
690a24a4a2d6c7711f18c0fab14a8139eb10c9d8 | 300-400 hours of video turned into text + his twitter tweets using whisper large-v2 model for ai transcription. Jay Essex has made 3 books and about 1200 videos which only about 800 can be found online unless someone has a back up storage for past youtube videos as his old youtube channel got removed before it was fully backed up by a fan. This goes indepth on a variety of topics. Alot of it has never been shared before here. Some include DNA ICUC (Evolution), Source energy, aliens, extraterrestrials, spiritual awakening, psychic development, creations history, earths history, creations future, earths future, who was god, talk about angels, what types of spirit/souls are there, how to awaken metaphysically, tools that help the psychic abilitys develop, talk about aliens like the annunaki and more, facts about dragons and unicorns spirit, crystals and stones, divination tools, spirit guides, and a whole lot more.
For list of problems with this data and how it was made go here https://www.youtube.com/watch?v=TBUDd3EVX6A
Here are even more topics he covers, although some topics might only be found in his books and atm i havent included the books with this dataset. The New Universal Alliance, Drachk, N'Antids, Solar System, Alliance of Planets, Arae, Lilly, source field, akashic records, energy healing, star essenite, earthquake, tectonic plate splits, abuse system, freedom, trump, joe biden, government, military, et, alien hybrid, space travel, time travel, universe, law of attraction (myth), metaphysical, self awareness, energy flowing, flow state, relaxation tips, guided meditations, religions, jesus, qeeg test results, numerology, spirit core, angels, dreams, stone energy, Dreams, Visions, Deja-Vu, Spirit Guides (w/Ear Ringing), Ghost, Demons, Exorcisms, Energetic Imprint Recordings, Dousing Rods, Pendulums, Kinesiology, Pictures, Dimensions, Barriers, Mirrors, Ouija Boards, Darting Black Spots in the Corners of Your Eyes, Sage, Spontaneous Combustion, spirit attack, spirit protection, flow within to flow without outwards, the spiritual foundation, thespiritualfoundation, ghandi reincarnated, reincarnation, past lifes, third eye, pineal gland, nervous system, george washington, Tomoe Gozen, Johann Sebastian Bach, color therapy, android, cyborg, telekinesis, kundlini awakening, gaia | iwasjohnlennon/JayAraeEssexArchive | [
"task_categories:text-classification",
"size_categories:100K<n<1M",
"language:en",
"medical",
"music",
"biology",
"chemistry",
"art",
"climate",
"region:us"
] | 2024-01-10T03:45:52+00:00 | {"language": ["en"], "size_categories": ["100K<n<1M"], "task_categories": ["text-classification"], "tags": ["medical", "music", "biology", "chemistry", "art", "climate"]} | 2024-01-20T10:55:37+00:00 | [] | [
"en"
] | TAGS
#task_categories-text-classification #size_categories-100K<n<1M #language-English #medical #music #biology #chemistry #art #climate #region-us
| 300-400 hours of video turned into text + his twitter tweets using whisper large-v2 model for ai transcription. Jay Essex has made 3 books and about 1200 videos which only about 800 can be found online unless someone has a back up storage for past youtube videos as his old youtube channel got removed before it was fully backed up by a fan. This goes indepth on a variety of topics. Alot of it has never been shared before here. Some include DNA ICUC (Evolution), Source energy, aliens, extraterrestrials, spiritual awakening, psychic development, creations history, earths history, creations future, earths future, who was god, talk about angels, what types of spirit/souls are there, how to awaken metaphysically, tools that help the psychic abilitys develop, talk about aliens like the annunaki and more, facts about dragons and unicorns spirit, crystals and stones, divination tools, spirit guides, and a whole lot more.
For list of problems with this data and how it was made go here URL
Here are even more topics he covers, although some topics might only be found in his books and atm i havent included the books with this dataset. The New Universal Alliance, Drachk, N'Antids, Solar System, Alliance of Planets, Arae, Lilly, source field, akashic records, energy healing, star essenite, earthquake, tectonic plate splits, abuse system, freedom, trump, joe biden, government, military, et, alien hybrid, space travel, time travel, universe, law of attraction (myth), metaphysical, self awareness, energy flowing, flow state, relaxation tips, guided meditations, religions, jesus, qeeg test results, numerology, spirit core, angels, dreams, stone energy, Dreams, Visions, Deja-Vu, Spirit Guides (w/Ear Ringing), Ghost, Demons, Exorcisms, Energetic Imprint Recordings, Dousing Rods, Pendulums, Kinesiology, Pictures, Dimensions, Barriers, Mirrors, Ouija Boards, Darting Black Spots in the Corners of Your Eyes, Sage, Spontaneous Combustion, spirit attack, spirit protection, flow within to flow without outwards, the spiritual foundation, thespiritualfoundation, ghandi reincarnated, reincarnation, past lifes, third eye, pineal gland, nervous system, george washington, Tomoe Gozen, Johann Sebastian Bach, color therapy, android, cyborg, telekinesis, kundlini awakening, gaia | [] | [
"TAGS\n#task_categories-text-classification #size_categories-100K<n<1M #language-English #medical #music #biology #chemistry #art #climate #region-us \n"
] |
c3fbb1743f23d011cc5d80ecbf6317b260039c40 | # Dataset Card for "VA_test1"
[More Information needed](https://github.com/huggingface/datasets/blob/main/CONTRIBUTING.md#how-to-contribute-to-the-dataset-cards) | racheltong/VA_test1 | [
"region:us"
] | 2024-01-10T03:49:19+00:00 | {"dataset_info": {"features": [{"name": "audio", "dtype": {"audio": {"sampling_rate": 16000}}}, {"name": "sentence", "dtype": "string"}], "splits": [{"name": "train", "num_bytes": 17091584.0, "num_examples": 50}], "download_size": 13618363, "dataset_size": 17091584.0}} | 2024-01-10T03:49:21+00:00 | [] | [] | TAGS
#region-us
| # Dataset Card for "VA_test1"
More Information needed | [
"# Dataset Card for \"VA_test1\"\n\nMore Information needed"
] | [
"TAGS\n#region-us \n",
"# Dataset Card for \"VA_test1\"\n\nMore Information needed"
] |
77df26f883f5de06213c71a84d584a35c26a02d3 | # T-Eval: Evaluating the Tool Utilization Capability Step by Step
[](https://arxiv.org/abs/2312.14033)
[](./LICENSE)
## ✨ Introduction
This is an evaluation harness for the benchmark described in [T-Eval: Evaluating the Tool Utilization Capability Step by Step](https://arxiv.org/abs/2312.14033).
[[Paper](https://arxiv.org/abs/2312.14033)]
[[Project Page](https://open-compass.github.io/T-Eval/)]
[[LeaderBoard](https://open-compass.github.io/T-Eval/leaderboard.html)]
> Large language models (LLM) have achieved remarkable performance on various NLP tasks and are augmented by tools for broader applications. Yet, how to evaluate and analyze the tool utilization capability of LLMs is still under-explored. In contrast to previous works that evaluate models holistically, we comprehensively decompose the tool utilization into multiple sub-processes, including instruction following, planning, reasoning, retrieval, understanding, and review. Based on that, we further introduce T-Eval to evaluate the tool-utilization capability step by step. T-Eval disentangles the tool utilization evaluation into several sub-domains along model capabilities, facilitating the inner understanding of both holistic and isolated competency of LLMs. We conduct extensive experiments on T-Eval and in-depth analysis of various LLMs. T-Eval not only exhibits consistency with the outcome-oriented evaluation but also provides a more fine-grained analysis of the capabilities of LLMs, providing a new perspective in LLM evaluation on tool-utilization ability.
## 🚀 What's New
- **[2024.01.08]** Release [ZH Leaderboard](https://open-compass.github.io/T-Eval/leaderboard_zh.html) and [ZH data](https://drive.google.com/file/d/1z25duwZAnBrPN5jYu9-8RMvfqnwPByKV/view?usp=sharing), where the questions and answer formats are in Chinese. (公布了中文评测数据集和榜单)✨✨✨
- **[2023.12.22]** Paper available on [ArXiv](https://arxiv.org/abs/2312.14033). 🔥🔥🔥
- **[2023.12.21]** Release the test scripts and [data]() for T-Eval. 🎉🎉🎉
## 🛠️ Preparations
```bash
$ git clone https://github.com/open-compass/T-Eval.git
$ cd T-Eval
$ pip install requirements.txt
```
## 🛫️ Get Started
We support both API-based models and HuggingFace models via [Lagent](https://github.com/InternLM/lagent).
### 💾 Test Data
You can use the following link to access to the test data:
[[EN data](https://drive.google.com/file/d/1ebR6WCCbS9-u2x7mWpWy8wV_Gb6ltgpi/view?usp=sharing)] (English format) [[ZH data](https://drive.google.com/file/d/1z25duwZAnBrPN5jYu9-8RMvfqnwPByKV/view?usp=sharing)] (Chinese format)
After downloading, please put the data in the `data` folder directly:
```
- data
- instruct_v1.json
- plan_json_v1.json
...
```
### 🤖 API Models
1. Set your OPENAI key in your environment.
```bash
export OPENAI_API_KEY=xxxxxxxxx
```
2. Run the model with the following scripts
```bash
# test all data at once
sh test_all_en.sh gpt-4-1106-preview
# test ZH dataset
sh test_all_zh.sh gpt-4-1106-preview
# test for Instruct only
python test.py --model_type gpt-4-1106-preview --resume --out_name instruct_gpt-4-1106-preview.json --out_dir data/work_dirs/ --dataset_path data/instruct_v1.json --eval instruct --prompt_type json
```
### 🤗 HuggingFace Models
1. Download the huggingface model to your local path.
2. Modify the `meta_template` json according to your tested model.
3. Run the model with the following scripts
```bash
# test all data at once
sh test_all_en.sh hf $HF_PATH $HF_MODEL_NAME
# test ZH dataset
sh test_all_zh.sh hf $HF_PATH $HF_MODEL_NAME
# test for Instruct only
python test.py --model_type hf --hf_path $HF_PATH --resume --out_name instruct_$HF_MODEL_NAME.json --out_dir data/work_dirs/ --dataset_path data/instruct_v1.json --eval instruct --prompt_type json --model_display_name $HF_MODEL_NAME
```
### 💫 Final Results
Once you finish all tested samples, a detailed evluation results will be logged at `$out_dir/$model_display_name/$model_display_name_-1.json` (For ZH dataset, there is a `_zh` suffix). To obtain your final score, please run the following command:
```bash
python teval/utils/convert_results.py --result_path $out_dir/$model_display_name/$model_display_name_-1.json
```
## 🔌 Protocols
T-Eval adopts multi-conversation style evaluation to gauge the model. The format of our saved prompt is as follows:
```python
[
{
"role": "system",
"content": "You have access to the following API:\n{'name': 'AirbnbSearch.search_property_by_place', 'description': 'This function takes various parameters to search properties on Airbnb.', 'required_parameters': [{'name': 'place', 'type': 'STRING', 'description': 'The name of the destination.'}], 'optional_parameters': [], 'return_data': [{'name': 'property', 'description': 'a list of at most 3 properties, containing id, name, and address.'}]}\nPlease generate the response in the following format:\ngoal: goal to call this action\n\nname: api name to call\n\nargs: JSON format api args in ONLY one line\n"
},
{
"role": "user",
"content": "Call the function AirbnbSearch.search_property_by_place with the parameter as follows: 'place' is 'Berlin'."
}
]
```
where `role` can be ['system', 'user', 'assistant'], and `content` must be in string format. Before infering it by a LLM, we need to construct it into a raw string format via `meta_template`. A `meta_template` sample for InternLM is provided at [meta_template.py](teval/utils/meta_template.py):
```python
[
dict(role='system', begin='<|System|>:', end='\n'),
dict(role='user', begin='<|User|>:', end='\n'),
dict(
role='assistant',
begin='<|Bot|>:',
end='<eoa>\n',
generate=True)
]
```
You need to specify the `begin` and `end` token based on your tested huggingface model at [meta_template.py](teval/utils/meta_template.py) and specify the `meta_template` args in `test.py`, same as the name you set in the `meta_template.py`. As for OpenAI model, we will handle that for you.
## 📊 Benchmark Results
More detailed and comprehensive benchmark results can refer to 🏆 [T-Eval official leaderboard](https://open-compass.github.io/T-Eval/leaderboard.html) !
### ✉️ Submit Your Results
You can submit your inference results (via running test.py) to this [email]([email protected]). We will run your predictions and update the results in our leaderboard. Please also provide the scale of your tested model. A sample structure of your submission should be like:
```
$model_display_name/
instruct_$model_display_name/
query_0_1_0.json
query_0_1_1.json
...
plan_json_$model_display_name/
plan_str_$model_display_name/
...
```
## ❤️ Acknowledgements
T-Eval is built with [Lagent](https://github.com/InternLM/lagent) and [OpenCompass](https://github.com/open-compass/opencompass). Thanks for their awesome work!
## 🖊️ Citation
If you find this project useful in your research, please consider cite:
```
@article{chen2023t,
title={T-Eval: Evaluating the Tool Utilization Capability Step by Step},
author={Chen, Zehui and Du, Weihua and Zhang, Wenwei and Liu, Kuikun and Liu, Jiangning and Zheng, Miao and Zhuo, Jingming and Zhang, Songyang and Lin, Dahua and Chen, Kai and others},
journal={arXiv preprint arXiv:2312.14033},
year={2023}
}
```
## 💳 License
This project is released under the Apache 2.0 [license](./LICENSE). | lovesnowbest/T-Eval | [
"task_categories:question-answering",
"size_categories:100M<n<1B",
"language:en",
"language:zh",
"license:apache-2.0",
"code",
"arxiv:2312.14033",
"region:us"
] | 2024-01-10T04:31:35+00:00 | {"language": ["en", "zh"], "license": "apache-2.0", "size_categories": ["100M<n<1B"], "task_categories": ["question-answering"], "pretty_name": "teval", "tags": ["code"]} | 2024-01-10T06:10:08+00:00 | [
"2312.14033"
] | [
"en",
"zh"
] | TAGS
#task_categories-question-answering #size_categories-100M<n<1B #language-English #language-Chinese #license-apache-2.0 #code #arxiv-2312.14033 #region-us
| # T-Eval: Evaluating the Tool Utilization Capability Step by Step

## Introduction
This is an evaluation harness for the benchmark described in T-Eval: Evaluating the Tool Utilization Capability Step by Step.
[Paper]
[Project Page]
[LeaderBoard]
> Large language models (LLM) have achieved remarkable performance on various NLP tasks and are augmented by tools for broader applications. Yet, how to evaluate and analyze the tool utilization capability of LLMs is still under-explored. In contrast to previous works that evaluate models holistically, we comprehensively decompose the tool utilization into multiple sub-processes, including instruction following, planning, reasoning, retrieval, understanding, and review. Based on that, we further introduce T-Eval to evaluate the tool-utilization capability step by step. T-Eval disentangles the tool utilization evaluation into several sub-domains along model capabilities, facilitating the inner understanding of both holistic and isolated competency of LLMs. We conduct extensive experiments on T-Eval and in-depth analysis of various LLMs. T-Eval not only exhibits consistency with the outcome-oriented evaluation but also provides a more fine-grained analysis of the capabilities of LLMs, providing a new perspective in LLM evaluation on tool-utilization ability.
## What's New
- [2024.01.08] Release ZH Leaderboard and ZH data, where the questions and answer formats are in Chinese. (公布了中文评测数据集和榜单)
- [2023.12.22] Paper available on ArXiv.
- [2023.12.21] Release the test scripts and [data]() for T-Eval.
## ️ Preparations
## ️ Get Started
We support both API-based models and HuggingFace models via Lagent.
### Test Data
You can use the following link to access to the test data:
[EN data] (English format) [ZH data] (Chinese format)
After downloading, please put the data in the 'data' folder directly:
### API Models
1. Set your OPENAI key in your environment.
2. Run the model with the following scripts
### HuggingFace Models
1. Download the huggingface model to your local path.
2. Modify the 'meta_template' json according to your tested model.
3. Run the model with the following scripts
### Final Results
Once you finish all tested samples, a detailed evluation results will be logged at '$out_dir/$model_display_name/$model_display_name_-1.json' (For ZH dataset, there is a '_zh' suffix). To obtain your final score, please run the following command:
## Protocols
T-Eval adopts multi-conversation style evaluation to gauge the model. The format of our saved prompt is as follows:
where 'role' can be ['system', 'user', 'assistant'], and 'content' must be in string format. Before infering it by a LLM, we need to construct it into a raw string format via 'meta_template'. A 'meta_template' sample for InternLM is provided at meta_template.py:
You need to specify the 'begin' and 'end' token based on your tested huggingface model at meta_template.py and specify the 'meta_template' args in 'URL', same as the name you set in the 'meta_template.py'. As for OpenAI model, we will handle that for you.
## Benchmark Results
More detailed and comprehensive benchmark results can refer to T-Eval official leaderboard !
### ️ Submit Your Results
You can submit your inference results (via running URL) to this email. We will run your predictions and update the results in our leaderboard. Please also provide the scale of your tested model. A sample structure of your submission should be like:
## ️ Acknowledgements
T-Eval is built with Lagent and OpenCompass. Thanks for their awesome work!
## ️ Citation
If you find this project useful in your research, please consider cite:
## License
This project is released under the Apache 2.0 license. | [
"# T-Eval: Evaluating the Tool Utilization Capability Step by Step\n\n",
"## Introduction \n\nThis is an evaluation harness for the benchmark described in T-Eval: Evaluating the Tool Utilization Capability Step by Step. \n\n[Paper]\n[Project Page]\n[LeaderBoard]\n\n> Large language models (LLM) have achieved remarkable performance on various NLP tasks and are augmented by tools for broader applications. Yet, how to evaluate and analyze the tool utilization capability of LLMs is still under-explored. In contrast to previous works that evaluate models holistically, we comprehensively decompose the tool utilization into multiple sub-processes, including instruction following, planning, reasoning, retrieval, understanding, and review. Based on that, we further introduce T-Eval to evaluate the tool-utilization capability step by step. T-Eval disentangles the tool utilization evaluation into several sub-domains along model capabilities, facilitating the inner understanding of both holistic and isolated competency of LLMs. We conduct extensive experiments on T-Eval and in-depth analysis of various LLMs. T-Eval not only exhibits consistency with the outcome-oriented evaluation but also provides a more fine-grained analysis of the capabilities of LLMs, providing a new perspective in LLM evaluation on tool-utilization ability.",
"## What's New\n\n- [2024.01.08] Release ZH Leaderboard and ZH data, where the questions and answer formats are in Chinese. (公布了中文评测数据集和榜单)\n- [2023.12.22] Paper available on ArXiv. \n- [2023.12.21] Release the test scripts and [data]() for T-Eval.",
"## ️ Preparations",
"## ️ Get Started\n\nWe support both API-based models and HuggingFace models via Lagent.",
"### Test Data\n\nYou can use the following link to access to the test data:\n\n[EN data] (English format) [ZH data] (Chinese format)\n\nAfter downloading, please put the data in the 'data' folder directly:",
"### API Models\n\n1. Set your OPENAI key in your environment.\n\n2. Run the model with the following scripts",
"### HuggingFace Models\n\n1. Download the huggingface model to your local path.\n2. Modify the 'meta_template' json according to your tested model.\n3. Run the model with the following scripts",
"### Final Results\nOnce you finish all tested samples, a detailed evluation results will be logged at '$out_dir/$model_display_name/$model_display_name_-1.json' (For ZH dataset, there is a '_zh' suffix). To obtain your final score, please run the following command:",
"## Protocols\n\nT-Eval adopts multi-conversation style evaluation to gauge the model. The format of our saved prompt is as follows:\n\nwhere 'role' can be ['system', 'user', 'assistant'], and 'content' must be in string format. Before infering it by a LLM, we need to construct it into a raw string format via 'meta_template'. A 'meta_template' sample for InternLM is provided at meta_template.py:\n\nYou need to specify the 'begin' and 'end' token based on your tested huggingface model at meta_template.py and specify the 'meta_template' args in 'URL', same as the name you set in the 'meta_template.py'. As for OpenAI model, we will handle that for you.",
"## Benchmark Results\n\nMore detailed and comprehensive benchmark results can refer to T-Eval official leaderboard !",
"### ️ Submit Your Results\n\nYou can submit your inference results (via running URL) to this email. We will run your predictions and update the results in our leaderboard. Please also provide the scale of your tested model. A sample structure of your submission should be like:",
"## ️ Acknowledgements\n\nT-Eval is built with Lagent and OpenCompass. Thanks for their awesome work!",
"## ️ Citation\n\nIf you find this project useful in your research, please consider cite:",
"## License\n\nThis project is released under the Apache 2.0 license."
] | [
"TAGS\n#task_categories-question-answering #size_categories-100M<n<1B #language-English #language-Chinese #license-apache-2.0 #code #arxiv-2312.14033 #region-us \n",
"# T-Eval: Evaluating the Tool Utilization Capability Step by Step\n\n",
"## Introduction \n\nThis is an evaluation harness for the benchmark described in T-Eval: Evaluating the Tool Utilization Capability Step by Step. \n\n[Paper]\n[Project Page]\n[LeaderBoard]\n\n> Large language models (LLM) have achieved remarkable performance on various NLP tasks and are augmented by tools for broader applications. Yet, how to evaluate and analyze the tool utilization capability of LLMs is still under-explored. In contrast to previous works that evaluate models holistically, we comprehensively decompose the tool utilization into multiple sub-processes, including instruction following, planning, reasoning, retrieval, understanding, and review. Based on that, we further introduce T-Eval to evaluate the tool-utilization capability step by step. T-Eval disentangles the tool utilization evaluation into several sub-domains along model capabilities, facilitating the inner understanding of both holistic and isolated competency of LLMs. We conduct extensive experiments on T-Eval and in-depth analysis of various LLMs. T-Eval not only exhibits consistency with the outcome-oriented evaluation but also provides a more fine-grained analysis of the capabilities of LLMs, providing a new perspective in LLM evaluation on tool-utilization ability.",
"## What's New\n\n- [2024.01.08] Release ZH Leaderboard and ZH data, where the questions and answer formats are in Chinese. (公布了中文评测数据集和榜单)\n- [2023.12.22] Paper available on ArXiv. \n- [2023.12.21] Release the test scripts and [data]() for T-Eval.",
"## ️ Preparations",
"## ️ Get Started\n\nWe support both API-based models and HuggingFace models via Lagent.",
"### Test Data\n\nYou can use the following link to access to the test data:\n\n[EN data] (English format) [ZH data] (Chinese format)\n\nAfter downloading, please put the data in the 'data' folder directly:",
"### API Models\n\n1. Set your OPENAI key in your environment.\n\n2. Run the model with the following scripts",
"### HuggingFace Models\n\n1. Download the huggingface model to your local path.\n2. Modify the 'meta_template' json according to your tested model.\n3. Run the model with the following scripts",
"### Final Results\nOnce you finish all tested samples, a detailed evluation results will be logged at '$out_dir/$model_display_name/$model_display_name_-1.json' (For ZH dataset, there is a '_zh' suffix). To obtain your final score, please run the following command:",
"## Protocols\n\nT-Eval adopts multi-conversation style evaluation to gauge the model. The format of our saved prompt is as follows:\n\nwhere 'role' can be ['system', 'user', 'assistant'], and 'content' must be in string format. Before infering it by a LLM, we need to construct it into a raw string format via 'meta_template'. A 'meta_template' sample for InternLM is provided at meta_template.py:\n\nYou need to specify the 'begin' and 'end' token based on your tested huggingface model at meta_template.py and specify the 'meta_template' args in 'URL', same as the name you set in the 'meta_template.py'. As for OpenAI model, we will handle that for you.",
"## Benchmark Results\n\nMore detailed and comprehensive benchmark results can refer to T-Eval official leaderboard !",
"### ️ Submit Your Results\n\nYou can submit your inference results (via running URL) to this email. We will run your predictions and update the results in our leaderboard. Please also provide the scale of your tested model. A sample structure of your submission should be like:",
"## ️ Acknowledgements\n\nT-Eval is built with Lagent and OpenCompass. Thanks for their awesome work!",
"## ️ Citation\n\nIf you find this project useful in your research, please consider cite:",
"## License\n\nThis project is released under the Apache 2.0 license."
] |
6cc608123f2b8959c906b0a065b46be5862689f0 |
# Dataset Card for "Contextual Response Evaluation for ESL and ASD Support💜💬🌐""
## Dataset Description 📖
### Dataset Summary 📝
Curated by Eric Soderquist, this dataset is a collection of English prompts and responses generated by the Phi-2 model, designed to evaluate and improve NLP models for supporting ESL (English as a Second Language) and ASD (Autism Spectrum Disorder) user bases. Each prompt is paired with multiple AI-generated responses and evaluated using a reward model to assess their relevance and quality.
### Supported Tasks and Leaderboards 🎯
- `text-generation`: This dataset is intended to train and refine language models for generating sensitive and context-aware responses.
- `language-modeling`: It can also be used for scoring the quality of language model responses to support ESL and ASD individuals.
### Languages 🗣
The dataset is monolingual and written in English.
## Dataset Structure 🏗
### Data Instances 📜
Each data instance contains a prompt, multiple AI-generated responses to that prompt, and scores reflecting the quality of each response.
### Data Fields 🏛
- `prompt`: a string containing the original English prompt.
- `responses`: an array of strings containing responses generated by the language model.
- `scores`: an array of floats representing the reward model's evaluation of each response.
### Data Splits 🔢
This dataset is not divided into traditional splits and consists of one complete set for evaluation purposes.
## Dataset Creation 🛠
### Curation Rationale 🤔
The dataset was curated with the goal of advancing NLP technologies to better serve ESL and ASD communities, offering a resource to evaluate and enhance the sensitivity of language models in understanding and generating responses that cater to the unique needs of these groups.
### Source Data 🗃
#### Initial Data Collection and Normalization
Data was generated using the Phi-2 model in response to carefully crafted prompts, aiming to cover a range of contexts and challenges faced by ESL and ASD individuals.
#### Annotations 🛑
The dataset includes scores from a reward model, providing an evaluation based on the model's perceived quality and appropriateness of the responses.
### Personal and Sensitive Information 🛑
Responses are generated and do not contain any real personal or sensitive information.
## Considerations for Using the Data ⚖️
### Social Impact of the Dataset 🌍
This dataset has the potential to impact the development of inclusive language models that are attuned to the nuances of communication required by ESL and ASD individuals.
### Discussion of Biases 🧐
As with any language model, biases present in the training data of the Phi-2 model may be reflected in the responses.
### Other Known Limitations 🚧
The reward model's scores are based on its own training data and may not cover the full scope of human evaluative diversity.
## Additional Information 📚
### Dataset Curator 👥
This dataset was curated by Eric Soderquist with the intent to foster developments in NLP that can adapt to and support the diverse linguistic and communicative needs of ESL and ASD communities.
### Licensing Information ©️
The dataset is made available under the MIT license.
### Citation Information 📢
If you use this dataset in your research, please cite it as follows:
```bibtex
@misc{contextual_response_evaluation,
author = {Soderquist, Eric},
title = {Contextual Response Evaluation for ESL and ASD Support},
year = {2024}
}
```
### Contributions 👏
Contributions to further develop and expand this dataset are welcome. | yunjaeys/Contextual_Response_Evaluation_for_ESL_and_ASD_Support | [
"task_categories:text-generation",
"task_ids:language-modeling",
"multilinguality:monolingual",
"size_categories:1K<n<10K",
"source_datasets:original",
"language:en",
"license:mit",
"asd",
"autism",
"esl",
"english_second_language",
"NLP",
"second_language",
"phi-2",
"openassistant_reward",
"region:us"
] | 2024-01-10T04:50:15+00:00 | {"language": ["en"], "license": ["mit"], "multilinguality": ["monolingual"], "size_categories": ["1K<n<10K"], "source_datasets": ["original"], "task_categories": ["text-generation"], "task_ids": ["language-modeling"], "pretty_name": "Contextual Response Evaluation for ESL and ASD Support\ud83d\udc9c\ud83d\udcac\ud83c\udf10", "tags": ["asd", "autism", "esl", "english_second_language", "NLP", "second_language", "phi-2", "openassistant_reward"]} | 2024-01-10T11:55:19+00:00 | [] | [
"en"
] | TAGS
#task_categories-text-generation #task_ids-language-modeling #multilinguality-monolingual #size_categories-1K<n<10K #source_datasets-original #language-English #license-mit #asd #autism #esl #english_second_language #NLP #second_language #phi-2 #openassistant_reward #region-us
|
# Dataset Card for "Contextual Response Evaluation for ESL and ASD Support""
## Dataset Description
### Dataset Summary
Curated by Eric Soderquist, this dataset is a collection of English prompts and responses generated by the Phi-2 model, designed to evaluate and improve NLP models for supporting ESL (English as a Second Language) and ASD (Autism Spectrum Disorder) user bases. Each prompt is paired with multiple AI-generated responses and evaluated using a reward model to assess their relevance and quality.
### Supported Tasks and Leaderboards
- 'text-generation': This dataset is intended to train and refine language models for generating sensitive and context-aware responses.
- 'language-modeling': It can also be used for scoring the quality of language model responses to support ESL and ASD individuals.
### Languages
The dataset is monolingual and written in English.
## Dataset Structure
### Data Instances
Each data instance contains a prompt, multiple AI-generated responses to that prompt, and scores reflecting the quality of each response.
### Data Fields
- 'prompt': a string containing the original English prompt.
- 'responses': an array of strings containing responses generated by the language model.
- 'scores': an array of floats representing the reward model's evaluation of each response.
### Data Splits
This dataset is not divided into traditional splits and consists of one complete set for evaluation purposes.
## Dataset Creation
### Curation Rationale
The dataset was curated with the goal of advancing NLP technologies to better serve ESL and ASD communities, offering a resource to evaluate and enhance the sensitivity of language models in understanding and generating responses that cater to the unique needs of these groups.
### Source Data
#### Initial Data Collection and Normalization
Data was generated using the Phi-2 model in response to carefully crafted prompts, aiming to cover a range of contexts and challenges faced by ESL and ASD individuals.
#### Annotations
The dataset includes scores from a reward model, providing an evaluation based on the model's perceived quality and appropriateness of the responses.
### Personal and Sensitive Information
Responses are generated and do not contain any real personal or sensitive information.
## Considerations for Using the Data ️
### Social Impact of the Dataset
This dataset has the potential to impact the development of inclusive language models that are attuned to the nuances of communication required by ESL and ASD individuals.
### Discussion of Biases
As with any language model, biases present in the training data of the Phi-2 model may be reflected in the responses.
### Other Known Limitations
The reward model's scores are based on its own training data and may not cover the full scope of human evaluative diversity.
## Additional Information
### Dataset Curator
This dataset was curated by Eric Soderquist with the intent to foster developments in NLP that can adapt to and support the diverse linguistic and communicative needs of ESL and ASD communities.
### Licensing Information ©️
The dataset is made available under the MIT license.
If you use this dataset in your research, please cite it as follows:
### Contributions
Contributions to further develop and expand this dataset are welcome. | [
"# Dataset Card for \"Contextual Response Evaluation for ESL and ASD Support\"\"",
"## Dataset Description",
"### Dataset Summary \n\nCurated by Eric Soderquist, this dataset is a collection of English prompts and responses generated by the Phi-2 model, designed to evaluate and improve NLP models for supporting ESL (English as a Second Language) and ASD (Autism Spectrum Disorder) user bases. Each prompt is paired with multiple AI-generated responses and evaluated using a reward model to assess their relevance and quality.",
"### Supported Tasks and Leaderboards \n\n- 'text-generation': This dataset is intended to train and refine language models for generating sensitive and context-aware responses.\n- 'language-modeling': It can also be used for scoring the quality of language model responses to support ESL and ASD individuals.",
"### Languages \n\nThe dataset is monolingual and written in English.",
"## Dataset Structure",
"### Data Instances \n\nEach data instance contains a prompt, multiple AI-generated responses to that prompt, and scores reflecting the quality of each response.",
"### Data Fields \n\n- 'prompt': a string containing the original English prompt.\n- 'responses': an array of strings containing responses generated by the language model.\n- 'scores': an array of floats representing the reward model's evaluation of each response.",
"### Data Splits \n\nThis dataset is not divided into traditional splits and consists of one complete set for evaluation purposes.",
"## Dataset Creation",
"### Curation Rationale \n\nThe dataset was curated with the goal of advancing NLP technologies to better serve ESL and ASD communities, offering a resource to evaluate and enhance the sensitivity of language models in understanding and generating responses that cater to the unique needs of these groups.",
"### Source Data",
"#### Initial Data Collection and Normalization \n\nData was generated using the Phi-2 model in response to carefully crafted prompts, aiming to cover a range of contexts and challenges faced by ESL and ASD individuals.",
"#### Annotations \n\nThe dataset includes scores from a reward model, providing an evaluation based on the model's perceived quality and appropriateness of the responses.",
"### Personal and Sensitive Information \n\nResponses are generated and do not contain any real personal or sensitive information.",
"## Considerations for Using the Data ️",
"### Social Impact of the Dataset \n\nThis dataset has the potential to impact the development of inclusive language models that are attuned to the nuances of communication required by ESL and ASD individuals.",
"### Discussion of Biases \n\nAs with any language model, biases present in the training data of the Phi-2 model may be reflected in the responses.",
"### Other Known Limitations \n\nThe reward model's scores are based on its own training data and may not cover the full scope of human evaluative diversity.",
"## Additional Information",
"### Dataset Curator \n\nThis dataset was curated by Eric Soderquist with the intent to foster developments in NLP that can adapt to and support the diverse linguistic and communicative needs of ESL and ASD communities.",
"### Licensing Information ©️\n\nThe dataset is made available under the MIT license.\n\n \n\nIf you use this dataset in your research, please cite it as follows:",
"### Contributions \nContributions to further develop and expand this dataset are welcome."
] | [
"TAGS\n#task_categories-text-generation #task_ids-language-modeling #multilinguality-monolingual #size_categories-1K<n<10K #source_datasets-original #language-English #license-mit #asd #autism #esl #english_second_language #NLP #second_language #phi-2 #openassistant_reward #region-us \n",
"# Dataset Card for \"Contextual Response Evaluation for ESL and ASD Support\"\"",
"## Dataset Description",
"### Dataset Summary \n\nCurated by Eric Soderquist, this dataset is a collection of English prompts and responses generated by the Phi-2 model, designed to evaluate and improve NLP models for supporting ESL (English as a Second Language) and ASD (Autism Spectrum Disorder) user bases. Each prompt is paired with multiple AI-generated responses and evaluated using a reward model to assess their relevance and quality.",
"### Supported Tasks and Leaderboards \n\n- 'text-generation': This dataset is intended to train and refine language models for generating sensitive and context-aware responses.\n- 'language-modeling': It can also be used for scoring the quality of language model responses to support ESL and ASD individuals.",
"### Languages \n\nThe dataset is monolingual and written in English.",
"## Dataset Structure",
"### Data Instances \n\nEach data instance contains a prompt, multiple AI-generated responses to that prompt, and scores reflecting the quality of each response.",
"### Data Fields \n\n- 'prompt': a string containing the original English prompt.\n- 'responses': an array of strings containing responses generated by the language model.\n- 'scores': an array of floats representing the reward model's evaluation of each response.",
"### Data Splits \n\nThis dataset is not divided into traditional splits and consists of one complete set for evaluation purposes.",
"## Dataset Creation",
"### Curation Rationale \n\nThe dataset was curated with the goal of advancing NLP technologies to better serve ESL and ASD communities, offering a resource to evaluate and enhance the sensitivity of language models in understanding and generating responses that cater to the unique needs of these groups.",
"### Source Data",
"#### Initial Data Collection and Normalization \n\nData was generated using the Phi-2 model in response to carefully crafted prompts, aiming to cover a range of contexts and challenges faced by ESL and ASD individuals.",
"#### Annotations \n\nThe dataset includes scores from a reward model, providing an evaluation based on the model's perceived quality and appropriateness of the responses.",
"### Personal and Sensitive Information \n\nResponses are generated and do not contain any real personal or sensitive information.",
"## Considerations for Using the Data ️",
"### Social Impact of the Dataset \n\nThis dataset has the potential to impact the development of inclusive language models that are attuned to the nuances of communication required by ESL and ASD individuals.",
"### Discussion of Biases \n\nAs with any language model, biases present in the training data of the Phi-2 model may be reflected in the responses.",
"### Other Known Limitations \n\nThe reward model's scores are based on its own training data and may not cover the full scope of human evaluative diversity.",
"## Additional Information",
"### Dataset Curator \n\nThis dataset was curated by Eric Soderquist with the intent to foster developments in NLP that can adapt to and support the diverse linguistic and communicative needs of ESL and ASD communities.",
"### Licensing Information ©️\n\nThe dataset is made available under the MIT license.\n\n \n\nIf you use this dataset in your research, please cite it as follows:",
"### Contributions \nContributions to further develop and expand this dataset are welcome."
] |
5bc7160f157b99bcfe34cdf64c635fdd1e50dd65 |
The intial purpose of this dataset extraction was to extract the relevant skills that can be obtained from each course from **Coursera**.
The skills then can be used for further analytical use.
Feel free to use the dataset at your own use cases. | azrai99/coursera-course-dataset | [
"task_categories:text2text-generation",
"size_categories:n<1K",
"language:en",
"license:apache-2.0",
"region:us"
] | 2024-01-10T04:54:08+00:00 | {"language": ["en"], "license": "apache-2.0", "size_categories": ["n<1K"], "task_categories": ["text2text-generation"]} | 2024-02-08T10:46:28+00:00 | [] | [
"en"
] | TAGS
#task_categories-text2text-generation #size_categories-n<1K #language-English #license-apache-2.0 #region-us
|
The intial purpose of this dataset extraction was to extract the relevant skills that can be obtained from each course from Coursera.
The skills then can be used for further analytical use.
Feel free to use the dataset at your own use cases. | [] | [
"TAGS\n#task_categories-text2text-generation #size_categories-n<1K #language-English #license-apache-2.0 #region-us \n"
] |
affd7afe98f087ca5e606e18ddf87a1819f11fc6 | # Dataset Card for "ee5"
[More Information needed](https://github.com/huggingface/datasets/blob/main/CONTRIBUTING.md#how-to-contribute-to-the-dataset-cards) | Minglii/ee5 | [
"region:us"
] | 2024-01-10T05:04:13+00:00 | {"dataset_info": {"features": [{"name": "data", "struct": [{"name": "conversations", "list": [{"name": "from", "dtype": "string"}, {"name": "value", "dtype": "string"}]}, {"name": "id", "dtype": "string"}]}], "splits": [{"name": "train", "num_bytes": 1927794, "num_examples": 2600}], "download_size": 1110487, "dataset_size": 1927794}, "configs": [{"config_name": "default", "data_files": [{"split": "train", "path": "data/train-*"}]}]} | 2024-01-10T05:05:05+00:00 | [] | [] | TAGS
#region-us
| # Dataset Card for "ee5"
More Information needed | [
"# Dataset Card for \"ee5\"\n\nMore Information needed"
] | [
"TAGS\n#region-us \n",
"# Dataset Card for \"ee5\"\n\nMore Information needed"
] |
bde862f8a37d66360d98ebe210cc19e102ba22e6 | # Dataset Card for "ee10"
[More Information needed](https://github.com/huggingface/datasets/blob/main/CONTRIBUTING.md#how-to-contribute-to-the-dataset-cards) | Minglii/ee10 | [
"region:us"
] | 2024-01-10T05:05:20+00:00 | {"dataset_info": {"features": [{"name": "data", "struct": [{"name": "conversations", "list": [{"name": "from", "dtype": "string"}, {"name": "value", "dtype": "string"}]}, {"name": "id", "dtype": "string"}]}], "splits": [{"name": "train", "num_bytes": 3690751, "num_examples": 5200}], "download_size": 2116849, "dataset_size": 3690751}, "configs": [{"config_name": "default", "data_files": [{"split": "train", "path": "data/train-*"}]}]} | 2024-01-10T05:05:46+00:00 | [] | [] | TAGS
#region-us
| # Dataset Card for "ee10"
More Information needed | [
"# Dataset Card for \"ee10\"\n\nMore Information needed"
] | [
"TAGS\n#region-us \n",
"# Dataset Card for \"ee10\"\n\nMore Information needed"
] |
0304c1c2787cfe092523212773f343c49dcc8fa1 | # Dataset Card for "ee15"
[More Information needed](https://github.com/huggingface/datasets/blob/main/CONTRIBUTING.md#how-to-contribute-to-the-dataset-cards) | Minglii/ee15 | [
"region:us"
] | 2024-01-10T05:05:28+00:00 | {"dataset_info": {"features": [{"name": "data", "struct": [{"name": "conversations", "list": [{"name": "from", "dtype": "string"}, {"name": "value", "dtype": "string"}]}, {"name": "id", "dtype": "string"}]}], "splits": [{"name": "train", "num_bytes": 5329018, "num_examples": 7800}], "download_size": 3049837, "dataset_size": 5329018}, "configs": [{"config_name": "default", "data_files": [{"split": "train", "path": "data/train-*"}]}]} | 2024-01-10T05:05:57+00:00 | [] | [] | TAGS
#region-us
| # Dataset Card for "ee15"
More Information needed | [
"# Dataset Card for \"ee15\"\n\nMore Information needed"
] | [
"TAGS\n#region-us \n",
"# Dataset Card for \"ee15\"\n\nMore Information needed"
] |
0fc8b3116cb5dee61c24843d6d55c9a9c0fe426f | # Dataset Card for "mmlu-anatomy-neg-prepend-verbal"
[More Information needed](https://github.com/huggingface/datasets/blob/main/CONTRIBUTING.md#how-to-contribute-to-the-dataset-cards) | joey234/mmlu-anatomy-neg-prepend-verbal | [
"region:us"
] | 2024-01-10T05:07:03+00:00 | {"configs": [{"config_name": "default", "data_files": [{"split": "dev", "path": "data/dev-*"}, {"split": "test", "path": "data/test-*"}]}], "dataset_info": {"features": [{"name": "question", "dtype": "string"}, {"name": "choices", "sequence": "string"}, {"name": "answer", "dtype": {"class_label": {"names": {"0": "A", "1": "B", "2": "C", "3": "D"}}}}, {"name": "negate_openai_prompt", "struct": [{"name": "content", "dtype": "string"}, {"name": "role", "dtype": "string"}]}, {"name": "neg_question", "dtype": "string"}, {"name": "fewshot_context", "dtype": "string"}, {"name": "ori_prompt", "dtype": "string"}, {"name": "neg_prompt", "dtype": "string"}, {"name": "fewshot_context_neg", "dtype": "string"}, {"name": "fewshot_context_ori", "dtype": "string"}], "splits": [{"name": "dev", "num_bytes": 5735, "num_examples": 5}, {"name": "test", "num_bytes": 860423, "num_examples": 135}], "download_size": 130157, "dataset_size": 866158}} | 2024-01-11T07:00:20+00:00 | [] | [] | TAGS
#region-us
| # Dataset Card for "mmlu-anatomy-neg-prepend-verbal"
More Information needed | [
"# Dataset Card for \"mmlu-anatomy-neg-prepend-verbal\"\n\nMore Information needed"
] | [
"TAGS\n#region-us \n",
"# Dataset Card for \"mmlu-anatomy-neg-prepend-verbal\"\n\nMore Information needed"
] |
3638ef0f3fb316421919ccd8eab45b1b3fc267ec | # Dataset Card for "mmlu-astronomy-neg-prepend-verbal"
[More Information needed](https://github.com/huggingface/datasets/blob/main/CONTRIBUTING.md#how-to-contribute-to-the-dataset-cards) | joey234/mmlu-astronomy-neg-prepend-verbal | [
"region:us"
] | 2024-01-10T05:07:30+00:00 | {"configs": [{"config_name": "default", "data_files": [{"split": "dev", "path": "data/dev-*"}, {"split": "test", "path": "data/test-*"}]}], "dataset_info": {"features": [{"name": "question", "dtype": "string"}, {"name": "choices", "sequence": "string"}, {"name": "answer", "dtype": {"class_label": {"names": {"0": "A", "1": "B", "2": "C", "3": "D"}}}}, {"name": "negate_openai_prompt", "struct": [{"name": "content", "dtype": "string"}, {"name": "role", "dtype": "string"}]}, {"name": "neg_question", "dtype": "string"}, {"name": "fewshot_context", "dtype": "string"}, {"name": "ori_prompt", "dtype": "string"}, {"name": "fewshot_context_neg", "dtype": "string"}, {"name": "fewshot_context_ori", "dtype": "string"}, {"name": "neg_prompt", "dtype": "string"}], "splits": [{"name": "dev", "num_bytes": 9251, "num_examples": 5}, {"name": "test", "num_bytes": 1799886, "num_examples": 152}], "download_size": 147626, "dataset_size": 1809137}} | 2024-01-10T05:14:56+00:00 | [] | [] | TAGS
#region-us
| # Dataset Card for "mmlu-astronomy-neg-prepend-verbal"
More Information needed | [
"# Dataset Card for \"mmlu-astronomy-neg-prepend-verbal\"\n\nMore Information needed"
] | [
"TAGS\n#region-us \n",
"# Dataset Card for \"mmlu-astronomy-neg-prepend-verbal\"\n\nMore Information needed"
] |
67887b70ab74c8d45eb39173cb11f23f1b571428 | # Dataset Card for "mmlu-business_ethics-neg-prepend-verbal"
[More Information needed](https://github.com/huggingface/datasets/blob/main/CONTRIBUTING.md#how-to-contribute-to-the-dataset-cards) | joey234/mmlu-business_ethics-neg-prepend-verbal | [
"region:us"
] | 2024-01-10T05:07:57+00:00 | {"configs": [{"config_name": "default", "data_files": [{"split": "dev", "path": "data/dev-*"}, {"split": "test", "path": "data/test-*"}]}], "dataset_info": {"features": [{"name": "question", "dtype": "string"}, {"name": "choices", "sequence": "string"}, {"name": "answer", "dtype": {"class_label": {"names": {"0": "A", "1": "B", "2": "C", "3": "D"}}}}, {"name": "negate_openai_prompt", "struct": [{"name": "content", "dtype": "string"}, {"name": "role", "dtype": "string"}]}, {"name": "neg_question", "dtype": "string"}, {"name": "fewshot_context", "dtype": "string"}, {"name": "ori_prompt", "dtype": "string"}, {"name": "neg_prompt", "dtype": "string"}, {"name": "fewshot_context_neg", "dtype": "string"}, {"name": "fewshot_context_ori", "dtype": "string"}], "splits": [{"name": "dev", "num_bytes": 11534, "num_examples": 5}, {"name": "test", "num_bytes": 1367503, "num_examples": 100}], "download_size": 132815, "dataset_size": 1379037}} | 2024-01-11T07:00:44+00:00 | [] | [] | TAGS
#region-us
| # Dataset Card for "mmlu-business_ethics-neg-prepend-verbal"
More Information needed | [
"# Dataset Card for \"mmlu-business_ethics-neg-prepend-verbal\"\n\nMore Information needed"
] | [
"TAGS\n#region-us \n",
"# Dataset Card for \"mmlu-business_ethics-neg-prepend-verbal\"\n\nMore Information needed"
] |
b0bb1703c5a11a5eca4cc5b4f14c41075cdcbfb5 | # Dataset Card for "mmlu-clinical_knowledge-neg-prepend-verbal"
[More Information needed](https://github.com/huggingface/datasets/blob/main/CONTRIBUTING.md#how-to-contribute-to-the-dataset-cards) | joey234/mmlu-clinical_knowledge-neg-prepend-verbal | [
"region:us"
] | 2024-01-10T05:08:24+00:00 | {"configs": [{"config_name": "default", "data_files": [{"split": "dev", "path": "data/dev-*"}, {"split": "test", "path": "data/test-*"}]}], "dataset_info": {"features": [{"name": "question", "dtype": "string"}, {"name": "choices", "sequence": "string"}, {"name": "answer", "dtype": {"class_label": {"names": {"0": "A", "1": "B", "2": "C", "3": "D"}}}}, {"name": "negate_openai_prompt", "struct": [{"name": "content", "dtype": "string"}, {"name": "role", "dtype": "string"}]}, {"name": "neg_question", "dtype": "string"}, {"name": "fewshot_context", "dtype": "string"}, {"name": "ori_prompt", "dtype": "string"}, {"name": "neg_prompt", "dtype": "string"}, {"name": "fewshot_context_neg", "dtype": "string"}, {"name": "fewshot_context_ori", "dtype": "string"}], "splits": [{"name": "dev", "num_bytes": 6767, "num_examples": 5}, {"name": "test", "num_bytes": 2000689, "num_examples": 265}], "download_size": 212042, "dataset_size": 2007456}} | 2024-01-11T07:01:05+00:00 | [] | [] | TAGS
#region-us
| # Dataset Card for "mmlu-clinical_knowledge-neg-prepend-verbal"
More Information needed | [
"# Dataset Card for \"mmlu-clinical_knowledge-neg-prepend-verbal\"\n\nMore Information needed"
] | [
"TAGS\n#region-us \n",
"# Dataset Card for \"mmlu-clinical_knowledge-neg-prepend-verbal\"\n\nMore Information needed"
] |
d9166fc69ecd682930c6bdef0660c5977729a3cc | # Dataset Card for "mmlu-college_biology-neg-prepend-verbal"
[More Information needed](https://github.com/huggingface/datasets/blob/main/CONTRIBUTING.md#how-to-contribute-to-the-dataset-cards) | joey234/mmlu-college_biology-neg-prepend-verbal | [
"region:us"
] | 2024-01-10T05:08:50+00:00 | {"configs": [{"config_name": "default", "data_files": [{"split": "dev", "path": "data/dev-*"}, {"split": "test", "path": "data/test-*"}]}], "dataset_info": {"features": [{"name": "question", "dtype": "string"}, {"name": "choices", "sequence": "string"}, {"name": "answer", "dtype": {"class_label": {"names": {"0": "A", "1": "B", "2": "C", "3": "D"}}}}, {"name": "negate_openai_prompt", "struct": [{"name": "content", "dtype": "string"}, {"name": "role", "dtype": "string"}]}, {"name": "neg_question", "dtype": "string"}, {"name": "fewshot_context", "dtype": "string"}, {"name": "ori_prompt", "dtype": "string"}, {"name": "neg_prompt", "dtype": "string"}, {"name": "fewshot_context_neg", "dtype": "string"}, {"name": "fewshot_context_ori", "dtype": "string"}], "splits": [{"name": "dev", "num_bytes": 8495, "num_examples": 5}, {"name": "test", "num_bytes": 1406615, "num_examples": 144}], "download_size": 196092, "dataset_size": 1415110}} | 2024-01-11T07:01:27+00:00 | [] | [] | TAGS
#region-us
| # Dataset Card for "mmlu-college_biology-neg-prepend-verbal"
More Information needed | [
"# Dataset Card for \"mmlu-college_biology-neg-prepend-verbal\"\n\nMore Information needed"
] | [
"TAGS\n#region-us \n",
"# Dataset Card for \"mmlu-college_biology-neg-prepend-verbal\"\n\nMore Information needed"
] |
ba8bdbada83872be3822529b902edf504a9ca53e | # Dataset Card for "mmlu-college_chemistry-neg-prepend-verbal"
[More Information needed](https://github.com/huggingface/datasets/blob/main/CONTRIBUTING.md#how-to-contribute-to-the-dataset-cards) | joey234/mmlu-college_chemistry-neg-prepend-verbal | [
"region:us"
] | 2024-01-10T05:09:16+00:00 | {"configs": [{"config_name": "default", "data_files": [{"split": "dev", "path": "data/dev-*"}, {"split": "test", "path": "data/test-*"}]}], "dataset_info": {"features": [{"name": "question", "dtype": "string"}, {"name": "choices", "sequence": "string"}, {"name": "answer", "dtype": {"class_label": {"names": {"0": "A", "1": "B", "2": "C", "3": "D"}}}}, {"name": "negate_openai_prompt", "struct": [{"name": "content", "dtype": "string"}, {"name": "role", "dtype": "string"}]}, {"name": "neg_question", "dtype": "string"}, {"name": "fewshot_context", "dtype": "string"}, {"name": "ori_prompt", "dtype": "string"}, {"name": "fewshot_context_neg", "dtype": "string"}, {"name": "fewshot_context_ori", "dtype": "string"}, {"name": "neg_prompt", "dtype": "string"}], "splits": [{"name": "dev", "num_bytes": 7604, "num_examples": 5}, {"name": "test", "num_bytes": 809370, "num_examples": 100}], "download_size": 138526, "dataset_size": 816974}} | 2024-01-10T05:16:25+00:00 | [] | [] | TAGS
#region-us
| # Dataset Card for "mmlu-college_chemistry-neg-prepend-verbal"
More Information needed | [
"# Dataset Card for \"mmlu-college_chemistry-neg-prepend-verbal\"\n\nMore Information needed"
] | [
"TAGS\n#region-us \n",
"# Dataset Card for \"mmlu-college_chemistry-neg-prepend-verbal\"\n\nMore Information needed"
] |
b7dd389516c449194e43bd2d5e72db21f8466cfc | # Dataset Card for "mmlu-college_computer_science-neg-prepend-verbal"
[More Information needed](https://github.com/huggingface/datasets/blob/main/CONTRIBUTING.md#how-to-contribute-to-the-dataset-cards) | joey234/mmlu-college_computer_science-neg-prepend-verbal | [
"region:us"
] | 2024-01-10T05:09:42+00:00 | {"configs": [{"config_name": "default", "data_files": [{"split": "dev", "path": "data/dev-*"}, {"split": "test", "path": "data/test-*"}]}], "dataset_info": {"features": [{"name": "question", "dtype": "string"}, {"name": "choices", "sequence": "string"}, {"name": "answer", "dtype": {"class_label": {"names": {"0": "A", "1": "B", "2": "C", "3": "D"}}}}, {"name": "negate_openai_prompt", "struct": [{"name": "content", "dtype": "string"}, {"name": "role", "dtype": "string"}]}, {"name": "neg_question", "dtype": "string"}, {"name": "fewshot_context", "dtype": "string"}, {"name": "ori_prompt", "dtype": "string"}, {"name": "fewshot_context_neg", "dtype": "string"}, {"name": "fewshot_context_ori", "dtype": "string"}, {"name": "neg_prompt", "dtype": "string"}], "splits": [{"name": "dev", "num_bytes": 12762, "num_examples": 5}, {"name": "test", "num_bytes": 1190385, "num_examples": 100}], "download_size": 153057, "dataset_size": 1203147}} | 2024-01-10T05:16:46+00:00 | [] | [] | TAGS
#region-us
| # Dataset Card for "mmlu-college_computer_science-neg-prepend-verbal"
More Information needed | [
"# Dataset Card for \"mmlu-college_computer_science-neg-prepend-verbal\"\n\nMore Information needed"
] | [
"TAGS\n#region-us \n",
"# Dataset Card for \"mmlu-college_computer_science-neg-prepend-verbal\"\n\nMore Information needed"
] |
39b0067e85108d50929f5ed055dd51b1599e2ddb | # Dataset Card for "mmlu-college_mathematics-neg-prepend-verbal"
[More Information needed](https://github.com/huggingface/datasets/blob/main/CONTRIBUTING.md#how-to-contribute-to-the-dataset-cards) | joey234/mmlu-college_mathematics-neg-prepend-verbal | [
"region:us"
] | 2024-01-10T05:16:50+00:00 | {"configs": [{"config_name": "default", "data_files": [{"split": "dev", "path": "data/dev-*"}, {"split": "test", "path": "data/test-*"}]}], "dataset_info": {"features": [{"name": "question", "dtype": "string"}, {"name": "choices", "sequence": "string"}, {"name": "answer", "dtype": {"class_label": {"names": {"0": "A", "1": "B", "2": "C", "3": "D"}}}}, {"name": "negate_openai_prompt", "struct": [{"name": "content", "dtype": "string"}, {"name": "role", "dtype": "string"}]}, {"name": "neg_question", "dtype": "string"}, {"name": "fewshot_context", "dtype": "string"}, {"name": "ori_prompt", "dtype": "string"}, {"name": "fewshot_context_neg", "dtype": "string"}, {"name": "fewshot_context_ori", "dtype": "string"}, {"name": "neg_prompt", "dtype": "string"}], "splits": [{"name": "dev", "num_bytes": 9276, "num_examples": 5}, {"name": "test", "num_bytes": 925998, "num_examples": 100}], "download_size": 148577, "dataset_size": 935274}} | 2024-01-10T05:17:12+00:00 | [] | [] | TAGS
#region-us
| # Dataset Card for "mmlu-college_mathematics-neg-prepend-verbal"
More Information needed | [
"# Dataset Card for \"mmlu-college_mathematics-neg-prepend-verbal\"\n\nMore Information needed"
] | [
"TAGS\n#region-us \n",
"# Dataset Card for \"mmlu-college_mathematics-neg-prepend-verbal\"\n\nMore Information needed"
] |
fb3fd6ab1c8eb1b4984a97849348b55875ae5241 | # Dataset Card for "mmlu-college_medicine-neg-prepend-verbal"
[More Information needed](https://github.com/huggingface/datasets/blob/main/CONTRIBUTING.md#how-to-contribute-to-the-dataset-cards) | joey234/mmlu-college_medicine-neg-prepend-verbal | [
"region:us"
] | 2024-01-10T05:17:17+00:00 | {"configs": [{"config_name": "default", "data_files": [{"split": "dev", "path": "data/dev-*"}, {"split": "test", "path": "data/test-*"}]}], "dataset_info": {"features": [{"name": "question", "dtype": "string"}, {"name": "choices", "sequence": "string"}, {"name": "answer", "dtype": {"class_label": {"names": {"0": "A", "1": "B", "2": "C", "3": "D"}}}}, {"name": "negate_openai_prompt", "struct": [{"name": "content", "dtype": "string"}, {"name": "role", "dtype": "string"}]}, {"name": "neg_question", "dtype": "string"}, {"name": "fewshot_context", "dtype": "string"}, {"name": "ori_prompt", "dtype": "string"}, {"name": "neg_prompt", "dtype": "string"}, {"name": "fewshot_context_neg", "dtype": "string"}, {"name": "fewshot_context_ori", "dtype": "string"}], "splits": [{"name": "dev", "num_bytes": 8599, "num_examples": 5}, {"name": "test", "num_bytes": 1878410, "num_examples": 173}], "download_size": 252936, "dataset_size": 1887009}} | 2024-01-11T07:01:49+00:00 | [] | [] | TAGS
#region-us
| # Dataset Card for "mmlu-college_medicine-neg-prepend-verbal"
More Information needed | [
"# Dataset Card for \"mmlu-college_medicine-neg-prepend-verbal\"\n\nMore Information needed"
] | [
"TAGS\n#region-us \n",
"# Dataset Card for \"mmlu-college_medicine-neg-prepend-verbal\"\n\nMore Information needed"
] |
4be340c1d680d1dac19c0b39eeb389bb5e5f9ab7 | # Dataset Card for "mmlu-college_physics-neg-prepend-verbal"
[More Information needed](https://github.com/huggingface/datasets/blob/main/CONTRIBUTING.md#how-to-contribute-to-the-dataset-cards) | joey234/mmlu-college_physics-neg-prepend-verbal | [
"region:us"
] | 2024-01-10T05:17:43+00:00 | {"configs": [{"config_name": "default", "data_files": [{"split": "dev", "path": "data/dev-*"}, {"split": "test", "path": "data/test-*"}]}], "dataset_info": {"features": [{"name": "question", "dtype": "string"}, {"name": "choices", "sequence": "string"}, {"name": "answer", "dtype": {"class_label": {"names": {"0": "A", "1": "B", "2": "C", "3": "D"}}}}, {"name": "negate_openai_prompt", "struct": [{"name": "content", "dtype": "string"}, {"name": "role", "dtype": "string"}]}, {"name": "neg_question", "dtype": "string"}, {"name": "fewshot_context", "dtype": "string"}, {"name": "ori_prompt", "dtype": "string"}, {"name": "fewshot_context_neg", "dtype": "string"}, {"name": "fewshot_context_ori", "dtype": "string"}, {"name": "neg_prompt", "dtype": "string"}], "splits": [{"name": "dev", "num_bytes": 8555, "num_examples": 5}, {"name": "test", "num_bytes": 872832, "num_examples": 102}], "download_size": 147063, "dataset_size": 881387}} | 2024-01-10T05:18:04+00:00 | [] | [] | TAGS
#region-us
| # Dataset Card for "mmlu-college_physics-neg-prepend-verbal"
More Information needed | [
"# Dataset Card for \"mmlu-college_physics-neg-prepend-verbal\"\n\nMore Information needed"
] | [
"TAGS\n#region-us \n",
"# Dataset Card for \"mmlu-college_physics-neg-prepend-verbal\"\n\nMore Information needed"
] |
a2f4996cd6096315c09be29b1d93d1eaf114cb32 | # Dataset Card for "mmlu-computer_security-neg-prepend-verbal"
[More Information needed](https://github.com/huggingface/datasets/blob/main/CONTRIBUTING.md#how-to-contribute-to-the-dataset-cards) | joey234/mmlu-computer_security-neg-prepend-verbal | [
"region:us"
] | 2024-01-10T05:18:09+00:00 | {"configs": [{"config_name": "default", "data_files": [{"split": "dev", "path": "data/dev-*"}, {"split": "test", "path": "data/test-*"}]}], "dataset_info": {"features": [{"name": "question", "dtype": "string"}, {"name": "choices", "sequence": "string"}, {"name": "answer", "dtype": {"class_label": {"names": {"0": "A", "1": "B", "2": "C", "3": "D"}}}}, {"name": "negate_openai_prompt", "struct": [{"name": "content", "dtype": "string"}, {"name": "role", "dtype": "string"}]}, {"name": "neg_question", "dtype": "string"}, {"name": "fewshot_context", "dtype": "string"}, {"name": "ori_prompt", "dtype": "string"}, {"name": "fewshot_context_neg", "dtype": "string"}, {"name": "fewshot_context_ori", "dtype": "string"}, {"name": "neg_prompt", "dtype": "string"}], "splits": [{"name": "dev", "num_bytes": 6196, "num_examples": 5}, {"name": "test", "num_bytes": 689900, "num_examples": 100}], "download_size": 128678, "dataset_size": 696096}} | 2024-01-10T05:18:33+00:00 | [] | [] | TAGS
#region-us
| # Dataset Card for "mmlu-computer_security-neg-prepend-verbal"
More Information needed | [
"# Dataset Card for \"mmlu-computer_security-neg-prepend-verbal\"\n\nMore Information needed"
] | [
"TAGS\n#region-us \n",
"# Dataset Card for \"mmlu-computer_security-neg-prepend-verbal\"\n\nMore Information needed"
] |
80303cb83f9fd437363d32017a9a5de28c8fc3b8 | # Dataset Card for "mmlu-conceptual_physics-neg-prepend-verbal"
[More Information needed](https://github.com/huggingface/datasets/blob/main/CONTRIBUTING.md#how-to-contribute-to-the-dataset-cards) | joey234/mmlu-conceptual_physics-neg-prepend-verbal | [
"region:us"
] | 2024-01-10T05:18:38+00:00 | {"configs": [{"config_name": "default", "data_files": [{"split": "dev", "path": "data/dev-*"}, {"split": "test", "path": "data/test-*"}]}], "dataset_info": {"features": [{"name": "question", "dtype": "string"}, {"name": "choices", "sequence": "string"}, {"name": "answer", "dtype": {"class_label": {"names": {"0": "A", "1": "B", "2": "C", "3": "D"}}}}, {"name": "negate_openai_prompt", "struct": [{"name": "content", "dtype": "string"}, {"name": "role", "dtype": "string"}]}, {"name": "neg_question", "dtype": "string"}, {"name": "fewshot_context", "dtype": "string"}, {"name": "ori_prompt", "dtype": "string"}, {"name": "fewshot_context_neg", "dtype": "string"}, {"name": "fewshot_context_ori", "dtype": "string"}, {"name": "neg_prompt", "dtype": "string"}], "splits": [{"name": "dev", "num_bytes": 5977, "num_examples": 5}, {"name": "test", "num_bytes": 1347765, "num_examples": 235}], "download_size": 155122, "dataset_size": 1353742}} | 2024-01-10T05:18:59+00:00 | [] | [] | TAGS
#region-us
| # Dataset Card for "mmlu-conceptual_physics-neg-prepend-verbal"
More Information needed | [
"# Dataset Card for \"mmlu-conceptual_physics-neg-prepend-verbal\"\n\nMore Information needed"
] | [
"TAGS\n#region-us \n",
"# Dataset Card for \"mmlu-conceptual_physics-neg-prepend-verbal\"\n\nMore Information needed"
] |
85e7ac4b64b575a5ecbd355dc3e867ccb4a56d48 | # Dataset Card for "mmlu-econometrics-neg-prepend-verbal"
[More Information needed](https://github.com/huggingface/datasets/blob/main/CONTRIBUTING.md#how-to-contribute-to-the-dataset-cards) | joey234/mmlu-econometrics-neg-prepend-verbal | [
"region:us"
] | 2024-01-10T05:19:04+00:00 | {"configs": [{"config_name": "default", "data_files": [{"split": "dev", "path": "data/dev-*"}, {"split": "test", "path": "data/test-*"}]}], "dataset_info": {"features": [{"name": "question", "dtype": "string"}, {"name": "choices", "sequence": "string"}, {"name": "answer", "dtype": {"class_label": {"names": {"0": "A", "1": "B", "2": "C", "3": "D"}}}}, {"name": "negate_openai_prompt", "struct": [{"name": "content", "dtype": "string"}, {"name": "role", "dtype": "string"}]}, {"name": "neg_question", "dtype": "string"}, {"name": "fewshot_context", "dtype": "string"}, {"name": "ori_prompt", "dtype": "string"}, {"name": "fewshot_context_neg", "dtype": "string"}, {"name": "fewshot_context_ori", "dtype": "string"}, {"name": "neg_prompt", "dtype": "string"}], "splits": [{"name": "dev", "num_bytes": 9477, "num_examples": 5}, {"name": "test", "num_bytes": 1163997, "num_examples": 114}], "download_size": 175699, "dataset_size": 1173474}} | 2024-01-10T05:19:24+00:00 | [] | [] | TAGS
#region-us
| # Dataset Card for "mmlu-econometrics-neg-prepend-verbal"
More Information needed | [
"# Dataset Card for \"mmlu-econometrics-neg-prepend-verbal\"\n\nMore Information needed"
] | [
"TAGS\n#region-us \n",
"# Dataset Card for \"mmlu-econometrics-neg-prepend-verbal\"\n\nMore Information needed"
] |
4c2940f524f05bb709f1f4dc7705b994efbd1518 | # Dataset Card for "mmlu-electrical_engineering-neg-prepend-verbal"
[More Information needed](https://github.com/huggingface/datasets/blob/main/CONTRIBUTING.md#how-to-contribute-to-the-dataset-cards) | joey234/mmlu-electrical_engineering-neg-prepend-verbal | [
"region:us"
] | 2024-01-10T05:19:30+00:00 | {"configs": [{"config_name": "default", "data_files": [{"split": "dev", "path": "data/dev-*"}, {"split": "test", "path": "data/test-*"}]}], "dataset_info": {"features": [{"name": "question", "dtype": "string"}, {"name": "choices", "sequence": "string"}, {"name": "answer", "dtype": {"class_label": {"names": {"0": "A", "1": "B", "2": "C", "3": "D"}}}}, {"name": "negate_openai_prompt", "struct": [{"name": "content", "dtype": "string"}, {"name": "role", "dtype": "string"}]}, {"name": "neg_question", "dtype": "string"}, {"name": "fewshot_context", "dtype": "string"}, {"name": "ori_prompt", "dtype": "string"}, {"name": "fewshot_context_neg", "dtype": "string"}, {"name": "fewshot_context_ori", "dtype": "string"}, {"name": "neg_prompt", "dtype": "string"}], "splits": [{"name": "dev", "num_bytes": 6493, "num_examples": 5}, {"name": "test", "num_bytes": 857717, "num_examples": 145}], "download_size": 121746, "dataset_size": 864210}} | 2024-01-10T05:19:50+00:00 | [] | [] | TAGS
#region-us
| # Dataset Card for "mmlu-electrical_engineering-neg-prepend-verbal"
More Information needed | [
"# Dataset Card for \"mmlu-electrical_engineering-neg-prepend-verbal\"\n\nMore Information needed"
] | [
"TAGS\n#region-us \n",
"# Dataset Card for \"mmlu-electrical_engineering-neg-prepend-verbal\"\n\nMore Information needed"
] |
a9b6c510461ae654914e77b5fe6baaeebc01bf22 | # Dataset Card for "mmlu-elementary_mathematics-neg-prepend-verbal"
[More Information needed](https://github.com/huggingface/datasets/blob/main/CONTRIBUTING.md#how-to-contribute-to-the-dataset-cards) | joey234/mmlu-elementary_mathematics-neg-prepend-verbal | [
"region:us"
] | 2024-01-10T05:19:55+00:00 | {"configs": [{"config_name": "default", "data_files": [{"split": "dev", "path": "data/dev-*"}, {"split": "test", "path": "data/test-*"}]}], "dataset_info": {"features": [{"name": "question", "dtype": "string"}, {"name": "choices", "sequence": "string"}, {"name": "answer", "dtype": {"class_label": {"names": {"0": "A", "1": "B", "2": "C", "3": "D"}}}}, {"name": "negate_openai_prompt", "struct": [{"name": "content", "dtype": "string"}, {"name": "role", "dtype": "string"}]}, {"name": "neg_question", "dtype": "string"}, {"name": "fewshot_context", "dtype": "string"}, {"name": "fewshot_context_neg", "dtype": "string"}, {"name": "ori_prompt", "dtype": "string"}], "splits": [{"name": "dev", "num_bytes": 6417, "num_examples": 5}, {"name": "test", "num_bytes": 1326061, "num_examples": 378}], "download_size": 179519, "dataset_size": 1332478}} | 2024-01-10T05:20:00+00:00 | [] | [] | TAGS
#region-us
| # Dataset Card for "mmlu-elementary_mathematics-neg-prepend-verbal"
More Information needed | [
"# Dataset Card for \"mmlu-elementary_mathematics-neg-prepend-verbal\"\n\nMore Information needed"
] | [
"TAGS\n#region-us \n",
"# Dataset Card for \"mmlu-elementary_mathematics-neg-prepend-verbal\"\n\nMore Information needed"
] |
4935ae975303c881b8eb3b7e672496a61069b6e0 | Filtered down to items where `'artifacts == 0 and ratings == 10'` | xzuyn/ai-horde-filtered | [
"language:en",
"region:us"
] | 2024-01-10T05:34:08+00:00 | {"language": ["en"]} | 2024-01-10T05:36:54+00:00 | [] | [
"en"
] | TAGS
#language-English #region-us
| Filtered down to items where ''artifacts == 0 and ratings == 10'' | [] | [
"TAGS\n#language-English #region-us \n"
] |
15fd000657b98a4db77dc8b758ba7e25a7265a91 | # Dataset Card for "mmlu-global_facts-neg-prepend-verbal"
[More Information needed](https://github.com/huggingface/datasets/blob/main/CONTRIBUTING.md#how-to-contribute-to-the-dataset-cards) | joey234/mmlu-global_facts-neg-prepend-verbal | [
"region:us"
] | 2024-01-10T05:52:30+00:00 | {"configs": [{"config_name": "default", "data_files": [{"split": "dev", "path": "data/dev-*"}, {"split": "test", "path": "data/test-*"}]}], "dataset_info": {"features": [{"name": "question", "dtype": "string"}, {"name": "choices", "sequence": "string"}, {"name": "answer", "dtype": {"class_label": {"names": {"0": "A", "1": "B", "2": "C", "3": "D"}}}}, {"name": "negate_openai_prompt", "struct": [{"name": "content", "dtype": "string"}, {"name": "role", "dtype": "string"}]}, {"name": "neg_question", "dtype": "string"}, {"name": "fewshot_context", "dtype": "string"}, {"name": "ori_prompt", "dtype": "string"}, {"name": "neg_prompt", "dtype": "string"}, {"name": "fewshot_context_neg", "dtype": "string"}, {"name": "fewshot_context_ori", "dtype": "string"}], "splits": [{"name": "dev", "num_bytes": 7049, "num_examples": 5}, {"name": "test", "num_bytes": 753562, "num_examples": 100}], "download_size": 110433, "dataset_size": 760611}} | 2024-01-11T07:02:11+00:00 | [] | [] | TAGS
#region-us
| # Dataset Card for "mmlu-global_facts-neg-prepend-verbal"
More Information needed | [
"# Dataset Card for \"mmlu-global_facts-neg-prepend-verbal\"\n\nMore Information needed"
] | [
"TAGS\n#region-us \n",
"# Dataset Card for \"mmlu-global_facts-neg-prepend-verbal\"\n\nMore Information needed"
] |
41787b0bf6eafd92344bc599987c35c5c779fc74 | # Dataset Card for "mmlu-high_school_biology-neg-prepend-verbal"
[More Information needed](https://github.com/huggingface/datasets/blob/main/CONTRIBUTING.md#how-to-contribute-to-the-dataset-cards) | joey234/mmlu-high_school_biology-neg-prepend-verbal | [
"region:us"
] | 2024-01-10T05:52:56+00:00 | {"configs": [{"config_name": "default", "data_files": [{"split": "dev", "path": "data/dev-*"}, {"split": "test", "path": "data/test-*"}]}], "dataset_info": {"features": [{"name": "question", "dtype": "string"}, {"name": "choices", "sequence": "string"}, {"name": "answer", "dtype": {"class_label": {"names": {"0": "A", "1": "B", "2": "C", "3": "D"}}}}, {"name": "negate_openai_prompt", "struct": [{"name": "content", "dtype": "string"}, {"name": "role", "dtype": "string"}]}, {"name": "neg_question", "dtype": "string"}, {"name": "fewshot_context", "dtype": "string"}, {"name": "ori_prompt", "dtype": "string"}, {"name": "neg_prompt", "dtype": "string"}, {"name": "fewshot_context_neg", "dtype": "string"}, {"name": "fewshot_context_ori", "dtype": "string"}], "splits": [{"name": "dev", "num_bytes": 8658, "num_examples": 5}, {"name": "test", "num_bytes": 3196056, "num_examples": 310}], "download_size": 327280, "dataset_size": 3204714}} | 2024-01-11T07:02:38+00:00 | [] | [] | TAGS
#region-us
| # Dataset Card for "mmlu-high_school_biology-neg-prepend-verbal"
More Information needed | [
"# Dataset Card for \"mmlu-high_school_biology-neg-prepend-verbal\"\n\nMore Information needed"
] | [
"TAGS\n#region-us \n",
"# Dataset Card for \"mmlu-high_school_biology-neg-prepend-verbal\"\n\nMore Information needed"
] |
62cb4a3e5f0da0cf66411cf0b266db527547d528 | # Dataset Card for "mmlu-high_school_geography-neg-prepend-verbal"
[More Information needed](https://github.com/huggingface/datasets/blob/main/CONTRIBUTING.md#how-to-contribute-to-the-dataset-cards) | joey234/mmlu-high_school_geography-neg-prepend-verbal | [
"region:us"
] | 2024-01-10T05:53:24+00:00 | {"configs": [{"config_name": "default", "data_files": [{"split": "dev", "path": "data/dev-*"}, {"split": "test", "path": "data/test-*"}]}], "dataset_info": {"features": [{"name": "question", "dtype": "string"}, {"name": "choices", "sequence": "string"}, {"name": "answer", "dtype": {"class_label": {"names": {"0": "A", "1": "B", "2": "C", "3": "D"}}}}, {"name": "negate_openai_prompt", "struct": [{"name": "content", "dtype": "string"}, {"name": "role", "dtype": "string"}]}, {"name": "neg_question", "dtype": "string"}, {"name": "fewshot_context", "dtype": "string"}, {"name": "ori_prompt", "dtype": "string"}, {"name": "neg_prompt", "dtype": "string"}, {"name": "fewshot_context_neg", "dtype": "string"}, {"name": "fewshot_context_ori", "dtype": "string"}], "splits": [{"name": "dev", "num_bytes": 7457, "num_examples": 5}, {"name": "test", "num_bytes": 1690950, "num_examples": 198}], "download_size": 177118, "dataset_size": 1698407}} | 2024-01-11T07:03:00+00:00 | [] | [] | TAGS
#region-us
| # Dataset Card for "mmlu-high_school_geography-neg-prepend-verbal"
More Information needed | [
"# Dataset Card for \"mmlu-high_school_geography-neg-prepend-verbal\"\n\nMore Information needed"
] | [
"TAGS\n#region-us \n",
"# Dataset Card for \"mmlu-high_school_geography-neg-prepend-verbal\"\n\nMore Information needed"
] |
4889a7406543956756e3a4a61e0980647d859e4e | # Dataset Card for "mmlu-high_school_government_and_politics-neg-prepend-verbal"
[More Information needed](https://github.com/huggingface/datasets/blob/main/CONTRIBUTING.md#how-to-contribute-to-the-dataset-cards) | joey234/mmlu-high_school_government_and_politics-neg-prepend-verbal | [
"region:us"
] | 2024-01-10T05:53:50+00:00 | {"configs": [{"config_name": "default", "data_files": [{"split": "dev", "path": "data/dev-*"}, {"split": "test", "path": "data/test-*"}]}], "dataset_info": {"features": [{"name": "question", "dtype": "string"}, {"name": "choices", "sequence": "string"}, {"name": "answer", "dtype": {"class_label": {"names": {"0": "A", "1": "B", "2": "C", "3": "D"}}}}, {"name": "negate_openai_prompt", "struct": [{"name": "content", "dtype": "string"}, {"name": "role", "dtype": "string"}]}, {"name": "neg_question", "dtype": "string"}, {"name": "fewshot_context", "dtype": "string"}, {"name": "ori_prompt", "dtype": "string"}, {"name": "neg_prompt", "dtype": "string"}, {"name": "fewshot_context_neg", "dtype": "string"}, {"name": "fewshot_context_ori", "dtype": "string"}], "splits": [{"name": "dev", "num_bytes": 8727, "num_examples": 5}, {"name": "test", "num_bytes": 2137365, "num_examples": 193}], "download_size": 229574, "dataset_size": 2146092}} | 2024-01-11T07:03:23+00:00 | [] | [] | TAGS
#region-us
| # Dataset Card for "mmlu-high_school_government_and_politics-neg-prepend-verbal"
More Information needed | [
"# Dataset Card for \"mmlu-high_school_government_and_politics-neg-prepend-verbal\"\n\nMore Information needed"
] | [
"TAGS\n#region-us \n",
"# Dataset Card for \"mmlu-high_school_government_and_politics-neg-prepend-verbal\"\n\nMore Information needed"
] |
5b03d6e853d4fec66fdfb830396b5ac01d69d598 | # Dataset Card for "mmlu-high_school_psychology-neg-prepend-verbal"
[More Information needed](https://github.com/huggingface/datasets/blob/main/CONTRIBUTING.md#how-to-contribute-to-the-dataset-cards) | joey234/mmlu-high_school_psychology-neg-prepend-verbal | [
"region:us"
] | 2024-01-10T05:59:57+00:00 | {"configs": [{"config_name": "default", "data_files": [{"split": "dev", "path": "data/dev-*"}, {"split": "test", "path": "data/test-*"}]}], "dataset_info": {"features": [{"name": "question", "dtype": "string"}, {"name": "choices", "sequence": "string"}, {"name": "answer", "dtype": {"class_label": {"names": {"0": "A", "1": "B", "2": "C", "3": "D"}}}}, {"name": "negate_openai_prompt", "struct": [{"name": "content", "dtype": "string"}, {"name": "role", "dtype": "string"}]}, {"name": "neg_question", "dtype": "string"}, {"name": "fewshot_context", "dtype": "string"}, {"name": "ori_prompt", "dtype": "string"}, {"name": "neg_prompt", "dtype": "string"}, {"name": "fewshot_context_neg", "dtype": "string"}, {"name": "fewshot_context_ori", "dtype": "string"}], "splits": [{"name": "dev", "num_bytes": 9825, "num_examples": 5}, {"name": "test", "num_bytes": 6256568, "num_examples": 545}], "download_size": 482916, "dataset_size": 6266393}} | 2024-01-11T07:03:49+00:00 | [] | [] | TAGS
#region-us
| # Dataset Card for "mmlu-high_school_psychology-neg-prepend-verbal"
More Information needed | [
"# Dataset Card for \"mmlu-high_school_psychology-neg-prepend-verbal\"\n\nMore Information needed"
] | [
"TAGS\n#region-us \n",
"# Dataset Card for \"mmlu-high_school_psychology-neg-prepend-verbal\"\n\nMore Information needed"
] |
bebe162e524a3d783aaa0be8e9de9e1b9542524d | # GolaifVirus-llama2-60
60 QA entries on a made-up Golaif Virus with fictional symptoms
| kazcfz/GolaifVirus-llama2-60 | [
"region:us"
] | 2024-01-10T06:43:39+00:00 | {} | 2024-01-10T16:31:26+00:00 | [] | [] | TAGS
#region-us
| # GolaifVirus-llama2-60
60 QA entries on a made-up Golaif Virus with fictional symptoms
| [
"# GolaifVirus-llama2-60\n60 QA entries on a made-up Golaif Virus with fictional symptoms"
] | [
"TAGS\n#region-us \n",
"# GolaifVirus-llama2-60\n60 QA entries on a made-up Golaif Virus with fictional symptoms"
] |
30936dffefc03ba6d1c6ef8a4d01075c7566cda9 | # Demo Datasets for WordCamp Asia 2024
Demo datasets for example finetuning and embedding.
Paired with Colab Notebook: https://colab.research.google.com/drive/1PGw_QEjJFQ3vhVuSinCbWose5KNjk5wb?usp=sharing
Recipes coming from the website: robs.kitchen as well as Kaggle's Food.com Recipe list open source dataset.
Finetuned model: iamchum/TinyLlama.recipe (based on TinyLlama/TinyLlama-1.1B-Chat-v1.0)
Inference endpoint: https://ui.endpoints.huggingface.co/iamchum/endpoints/aws-tinyllama-recipe-wcasia-2024
---
license: mit
---
| iamchum/robs.kitchen_recipe_dataset | [
"region:us"
] | 2024-01-10T06:47:13+00:00 | {} | 2024-01-10T06:51:33+00:00 | [] | [] | TAGS
#region-us
| # Demo Datasets for WordCamp Asia 2024
Demo datasets for example finetuning and embedding.
Paired with Colab Notebook: URL
Recipes coming from the website: robs.kitchen as well as Kaggle's URL Recipe list open source dataset.
Finetuned model: iamchum/URL (based on TinyLlama/TinyLlama-1.1B-Chat-v1.0)
Inference endpoint: URL
---
license: mit
---
| [
"# Demo Datasets for WordCamp Asia 2024\n\nDemo datasets for example finetuning and embedding.\n\nPaired with Colab Notebook: URL\n\nRecipes coming from the website: robs.kitchen as well as Kaggle's URL Recipe list open source dataset.\n\nFinetuned model: iamchum/URL (based on TinyLlama/TinyLlama-1.1B-Chat-v1.0)\nInference endpoint: URL\n\n---\nlicense: mit\n---"
] | [
"TAGS\n#region-us \n",
"# Demo Datasets for WordCamp Asia 2024\n\nDemo datasets for example finetuning and embedding.\n\nPaired with Colab Notebook: URL\n\nRecipes coming from the website: robs.kitchen as well as Kaggle's URL Recipe list open source dataset.\n\nFinetuned model: iamchum/URL (based on TinyLlama/TinyLlama-1.1B-Chat-v1.0)\nInference endpoint: URL\n\n---\nlicense: mit\n---"
] |
13cfc8c078e29177e0338653cde762ab8f165ce6 |
Just a quick test of a translation script - Machine translated, so not perfect | buzzcraft/ELI5-NO | [
"language:no",
"license:apache-2.0",
"region:us"
] | 2024-01-10T08:02:22+00:00 | {"language": ["no"], "license": "apache-2.0"} | 2024-01-12T14:16:56+00:00 | [] | [
"no"
] | TAGS
#language-Norwegian #license-apache-2.0 #region-us
|
Just a quick test of a translation script - Machine translated, so not perfect | [] | [
"TAGS\n#language-Norwegian #license-apache-2.0 #region-us \n"
] |
9c20afb384d44e6f2957b9984741c69255ec6477 | # LCA Project Level Code Completion
## How to load the dataset
```
from datasets import load_dataset
ds = load_dataset('JetBrains-Research/lca-codegen-medium', split='test')
```
## Data Point Structure
* `repo` -- repository name in format `{GitHub_user_name}__{repository_name}`
* `commit_hash` -- commit hash
* `completion_file` -- dictionary with the completion file content in the following format:
* `filename` -- filepath to the completion file
* `content` -- content of the completion file
* `completion_lines` -- dictionary where keys are classes of lines and values are a list of integers (numbers of lines to complete). The classes are:
* `committed` -- line contains at least one function or class that was declared in the committed files
* `inproject` -- line contains at least one function and class that was declared in the project (excluding previous)
* `infile` -- line contains at least one function and class that was declared in the completion file (excluding previous)
* `common` -- line contains at least one function and class that was classified to be common, e.g. `main`, `get`, etc (excluding previous)
* `non_informative` -- line that was classified to be non-informative, e.g. too short, contains comments, etc
* `random` -- randomly sampled from the rest of the lines
* `repo_snapshot` -- dictionary with a snapshot of the repository before the commit. Has the same structure as `completion_file`, but filenames and contents are orginized as lists.
* `completion_lines_raw` -- the same as `completion_lines`, but before sampling.
## How we collected the data
* TBA | JetBrains-Research/lca-codegen-medium | [
"region:us"
] | 2024-01-10T08:05:22+00:00 | {"dataset_info": {"features": [{"name": "repo", "dtype": "string"}, {"name": "commit_hash", "dtype": "string"}, {"name": "completion_file", "struct": [{"name": "filename", "dtype": "string"}, {"name": "content", "dtype": "string"}]}, {"name": "completion_lines", "struct": [{"name": "infile", "sequence": "int32"}, {"name": "inproject", "sequence": "int32"}, {"name": "common", "sequence": "int32"}, {"name": "commited", "sequence": "int32"}, {"name": "non_informative", "sequence": "int32"}, {"name": "random", "sequence": "int32"}]}, {"name": "repo_snapshot", "sequence": [{"name": "filename", "dtype": "string"}, {"name": "content", "dtype": "string"}]}, {"name": "completion_lines_raw", "struct": [{"name": "commited", "sequence": "int64"}, {"name": "common", "sequence": "int64"}, {"name": "infile", "sequence": "int64"}, {"name": "inproject", "sequence": "int64"}, {"name": "non_informative", "sequence": "int64"}, {"name": "other", "sequence": "int64"}]}], "splits": [{"name": "test", "num_bytes": 514928459, "num_examples": 224}], "download_size": 225824560, "dataset_size": 514928459}, "configs": [{"config_name": "default", "data_files": [{"split": "test", "path": "data/test-*"}]}]} | 2024-02-05T14:41:54+00:00 | [] | [] | TAGS
#region-us
| # LCA Project Level Code Completion
## How to load the dataset
## Data Point Structure
* 'repo' -- repository name in format '{GitHub_user_name}__{repository_name}'
* 'commit_hash' -- commit hash
* 'completion_file' -- dictionary with the completion file content in the following format:
* 'filename' -- filepath to the completion file
* 'content' -- content of the completion file
* 'completion_lines' -- dictionary where keys are classes of lines and values are a list of integers (numbers of lines to complete). The classes are:
* 'committed' -- line contains at least one function or class that was declared in the committed files
* 'inproject' -- line contains at least one function and class that was declared in the project (excluding previous)
* 'infile' -- line contains at least one function and class that was declared in the completion file (excluding previous)
* 'common' -- line contains at least one function and class that was classified to be common, e.g. 'main', 'get', etc (excluding previous)
* 'non_informative' -- line that was classified to be non-informative, e.g. too short, contains comments, etc
* 'random' -- randomly sampled from the rest of the lines
* 'repo_snapshot' -- dictionary with a snapshot of the repository before the commit. Has the same structure as 'completion_file', but filenames and contents are orginized as lists.
* 'completion_lines_raw' -- the same as 'completion_lines', but before sampling.
## How we collected the data
* TBA | [
"# LCA Project Level Code Completion",
"## How to load the dataset",
"## Data Point Structure\n* 'repo' -- repository name in format '{GitHub_user_name}__{repository_name}'\n* 'commit_hash' -- commit hash\n* 'completion_file' -- dictionary with the completion file content in the following format:\n * 'filename' -- filepath to the completion file\n * 'content' -- content of the completion file\n* 'completion_lines' -- dictionary where keys are classes of lines and values are a list of integers (numbers of lines to complete). The classes are:\n * 'committed' -- line contains at least one function or class that was declared in the committed files\n * 'inproject' -- line contains at least one function and class that was declared in the project (excluding previous)\n * 'infile' -- line contains at least one function and class that was declared in the completion file (excluding previous)\n * 'common' -- line contains at least one function and class that was classified to be common, e.g. 'main', 'get', etc (excluding previous)\n * 'non_informative' -- line that was classified to be non-informative, e.g. too short, contains comments, etc\n * 'random' -- randomly sampled from the rest of the lines\n* 'repo_snapshot' -- dictionary with a snapshot of the repository before the commit. Has the same structure as 'completion_file', but filenames and contents are orginized as lists.\n* 'completion_lines_raw' -- the same as 'completion_lines', but before sampling.",
"## How we collected the data\n* TBA"
] | [
"TAGS\n#region-us \n",
"# LCA Project Level Code Completion",
"## How to load the dataset",
"## Data Point Structure\n* 'repo' -- repository name in format '{GitHub_user_name}__{repository_name}'\n* 'commit_hash' -- commit hash\n* 'completion_file' -- dictionary with the completion file content in the following format:\n * 'filename' -- filepath to the completion file\n * 'content' -- content of the completion file\n* 'completion_lines' -- dictionary where keys are classes of lines and values are a list of integers (numbers of lines to complete). The classes are:\n * 'committed' -- line contains at least one function or class that was declared in the committed files\n * 'inproject' -- line contains at least one function and class that was declared in the project (excluding previous)\n * 'infile' -- line contains at least one function and class that was declared in the completion file (excluding previous)\n * 'common' -- line contains at least one function and class that was classified to be common, e.g. 'main', 'get', etc (excluding previous)\n * 'non_informative' -- line that was classified to be non-informative, e.g. too short, contains comments, etc\n * 'random' -- randomly sampled from the rest of the lines\n* 'repo_snapshot' -- dictionary with a snapshot of the repository before the commit. Has the same structure as 'completion_file', but filenames and contents are orginized as lists.\n* 'completion_lines_raw' -- the same as 'completion_lines', but before sampling.",
"## How we collected the data\n* TBA"
] |
33660a40201a127c24ea7b8ef62ec454348aca7c | # LCA Project Level Code Completion
## How to load the dataset
```
from datasets import load_dataset
ds = load_dataset('JetBrains-Research/lca-codegen-small', split='test')
```
## Data Point Structure
* `repo` -- repository name in format `{GitHub_user_name}__{repository_name}`
* `commit_hash` -- commit hash
* `completion_file` -- dictionary with the completion file content in the following format:
* `filename` -- filepath to the completion file
* `content` -- content of the completion file
* `completion_lines` -- dictionary where keys are classes of lines and values are a list of integers (numbers of lines to complete). The classes are:
* `committed` -- line contains at least one function or class that was declared in the committed files
* `inproject` -- line contains at least one function and class that was declared in the project (excluding previous)
* `infile` -- line contains at least one function and class that was declared in the completion file (excluding previous)
* `common` -- line contains at least one function and class that was classified to be common, e.g. `main`, `get`, etc (excluding previous)
* `non_informative` -- line that was classified to be non-informative, e.g. too short, contains comments, etc
* `random` -- randomly sampled from the rest of the lines
* `repo_snapshot` -- dictionary with a snapshot of the repository before the commit. Has the same structure as `completion_file`, but filenames and contents are orginized as lists.
* `completion_lines_raw` -- the same as `completion_lines`, but before sampling.
## How we collected the data
* TBA
| JetBrains-Research/lca-codegen-small | [
"region:us"
] | 2024-01-10T08:11:17+00:00 | {"dataset_info": {"features": [{"name": "repo", "dtype": "string"}, {"name": "commit_hash", "dtype": "string"}, {"name": "completion_file", "struct": [{"name": "filename", "dtype": "string"}, {"name": "content", "dtype": "string"}]}, {"name": "completion_lines", "struct": [{"name": "infile", "sequence": "int32"}, {"name": "inproject", "sequence": "int32"}, {"name": "common", "sequence": "int32"}, {"name": "commited", "sequence": "int32"}, {"name": "non_informative", "sequence": "int32"}, {"name": "random", "sequence": "int32"}]}, {"name": "repo_snapshot", "sequence": [{"name": "filename", "dtype": "string"}, {"name": "content", "dtype": "string"}]}, {"name": "completion_lines_raw", "struct": [{"name": "commited", "sequence": "int64"}, {"name": "common", "sequence": "int64"}, {"name": "infile", "sequence": "int64"}, {"name": "inproject", "sequence": "int64"}, {"name": "non_informative", "sequence": "int64"}, {"name": "other", "sequence": "int64"}]}], "splits": [{"name": "test", "num_bytes": 111010036, "num_examples": 144}], "download_size": 37603701, "dataset_size": 111010036}, "configs": [{"config_name": "default", "data_files": [{"split": "test", "path": "data/test-*"}]}]} | 2024-02-05T14:40:04+00:00 | [] | [] | TAGS
#region-us
| # LCA Project Level Code Completion
## How to load the dataset
## Data Point Structure
* 'repo' -- repository name in format '{GitHub_user_name}__{repository_name}'
* 'commit_hash' -- commit hash
* 'completion_file' -- dictionary with the completion file content in the following format:
* 'filename' -- filepath to the completion file
* 'content' -- content of the completion file
* 'completion_lines' -- dictionary where keys are classes of lines and values are a list of integers (numbers of lines to complete). The classes are:
* 'committed' -- line contains at least one function or class that was declared in the committed files
* 'inproject' -- line contains at least one function and class that was declared in the project (excluding previous)
* 'infile' -- line contains at least one function and class that was declared in the completion file (excluding previous)
* 'common' -- line contains at least one function and class that was classified to be common, e.g. 'main', 'get', etc (excluding previous)
* 'non_informative' -- line that was classified to be non-informative, e.g. too short, contains comments, etc
* 'random' -- randomly sampled from the rest of the lines
* 'repo_snapshot' -- dictionary with a snapshot of the repository before the commit. Has the same structure as 'completion_file', but filenames and contents are orginized as lists.
* 'completion_lines_raw' -- the same as 'completion_lines', but before sampling.
## How we collected the data
* TBA
| [
"# LCA Project Level Code Completion",
"## How to load the dataset",
"## Data Point Structure\n* 'repo' -- repository name in format '{GitHub_user_name}__{repository_name}'\n* 'commit_hash' -- commit hash\n* 'completion_file' -- dictionary with the completion file content in the following format:\n * 'filename' -- filepath to the completion file\n * 'content' -- content of the completion file\n* 'completion_lines' -- dictionary where keys are classes of lines and values are a list of integers (numbers of lines to complete). The classes are:\n * 'committed' -- line contains at least one function or class that was declared in the committed files\n * 'inproject' -- line contains at least one function and class that was declared in the project (excluding previous)\n * 'infile' -- line contains at least one function and class that was declared in the completion file (excluding previous)\n * 'common' -- line contains at least one function and class that was classified to be common, e.g. 'main', 'get', etc (excluding previous)\n * 'non_informative' -- line that was classified to be non-informative, e.g. too short, contains comments, etc\n * 'random' -- randomly sampled from the rest of the lines\n* 'repo_snapshot' -- dictionary with a snapshot of the repository before the commit. Has the same structure as 'completion_file', but filenames and contents are orginized as lists.\n* 'completion_lines_raw' -- the same as 'completion_lines', but before sampling.",
"## How we collected the data\n* TBA"
] | [
"TAGS\n#region-us \n",
"# LCA Project Level Code Completion",
"## How to load the dataset",
"## Data Point Structure\n* 'repo' -- repository name in format '{GitHub_user_name}__{repository_name}'\n* 'commit_hash' -- commit hash\n* 'completion_file' -- dictionary with the completion file content in the following format:\n * 'filename' -- filepath to the completion file\n * 'content' -- content of the completion file\n* 'completion_lines' -- dictionary where keys are classes of lines and values are a list of integers (numbers of lines to complete). The classes are:\n * 'committed' -- line contains at least one function or class that was declared in the committed files\n * 'inproject' -- line contains at least one function and class that was declared in the project (excluding previous)\n * 'infile' -- line contains at least one function and class that was declared in the completion file (excluding previous)\n * 'common' -- line contains at least one function and class that was classified to be common, e.g. 'main', 'get', etc (excluding previous)\n * 'non_informative' -- line that was classified to be non-informative, e.g. too short, contains comments, etc\n * 'random' -- randomly sampled from the rest of the lines\n* 'repo_snapshot' -- dictionary with a snapshot of the repository before the commit. Has the same structure as 'completion_file', but filenames and contents are orginized as lists.\n* 'completion_lines_raw' -- the same as 'completion_lines', but before sampling.",
"## How we collected the data\n* TBA"
] |
14d98318664c1e04623a21c287b158ea9237ccca |
# Answer Reformulation
The "Answer Reformulation" dataset is designed for a task that involves providing a detailed, comprehensive answer to a given question, supported by a set of related documents. The unique aspect of this dataset is the specific format required for the answers, emphasizing thorough exploration and understanding of the material in a specified language.
---
## Dataset Details
Each data point in the dataset has three main elements:
- **Query**: A question that needs to be addressed
- **Input Documents** (input_docs): A collection of documents related (or not) to the query. These documents may provide the information required to formulate the answer.
- **Answer**: A comprehensive response to the query, incorporating information from the input documents and following strict citation guidelines, referencing specific input documents using citation IDs (e.g., [@1], [@2]). If the input documents do not provide sufficient information to answer the query, the response should be "Answer not found."
---
## Data Generation (with GPT)
### Local Question Answers
**Question Answer Generation**:
The idea is to generate based on a complete and long document (a section from a textbook):
- A question that could be answered based on the given document
- An answer citing specific parts of the document using citation IDs
To achieve this:
1. Breaking down the complete document into smaller, numbered segments to facilitate the citation process.
2. Employing GPT-4 to generate both the question and its corresponding answer, which includes citations referring to the numbered segments of the text.
**Postprocess Enhancements**:
To enrich the dataset, each data point is supplemented with irrelevant texts (selected E5 model) as part of the input documents. This addition is intended to increase the complexity and diversity of the dataset, thereby making it more challenging and realistic for training purposes.
| ProfessorBob/answer_reformulate | [
"region:us"
] | 2024-01-10T08:14:13+00:00 | {"dataset_info": {"features": [{"name": "query", "dtype": "string"}, {"name": "input_docs", "dtype": "string"}, {"name": "answer", "dtype": "string"}, {"name": "lang", "dtype": "string"}, {"name": "source", "dtype": "string"}, {"name": "answer_lang", "dtype": "string"}], "splits": [{"name": "eval", "num_bytes": 3873010.4015041944, "num_examples": 1038}, {"name": "test", "num_bytes": 3869279.177610645, "num_examples": 1037}, {"name": "train", "num_bytes": 30484771, "num_examples": 8269}], "download_size": 40075295, "dataset_size": 38227060.57911484}, "configs": [{"config_name": "default", "data_files": [{"split": "train", "path": "data/train-*"}, {"split": "eval", "path": "data/eval-*"}, {"split": "test", "path": "data/test-*"}]}]} | 2024-01-16T09:17:26+00:00 | [] | [] | TAGS
#region-us
|
# Answer Reformulation
The "Answer Reformulation" dataset is designed for a task that involves providing a detailed, comprehensive answer to a given question, supported by a set of related documents. The unique aspect of this dataset is the specific format required for the answers, emphasizing thorough exploration and understanding of the material in a specified language.
---
## Dataset Details
Each data point in the dataset has three main elements:
- Query: A question that needs to be addressed
- Input Documents (input_docs): A collection of documents related (or not) to the query. These documents may provide the information required to formulate the answer.
- Answer: A comprehensive response to the query, incorporating information from the input documents and following strict citation guidelines, referencing specific input documents using citation IDs (e.g., [@1], [@2]). If the input documents do not provide sufficient information to answer the query, the response should be "Answer not found."
---
## Data Generation (with GPT)
### Local Question Answers
Question Answer Generation:
The idea is to generate based on a complete and long document (a section from a textbook):
- A question that could be answered based on the given document
- An answer citing specific parts of the document using citation IDs
To achieve this:
1. Breaking down the complete document into smaller, numbered segments to facilitate the citation process.
2. Employing GPT-4 to generate both the question and its corresponding answer, which includes citations referring to the numbered segments of the text.
Postprocess Enhancements:
To enrich the dataset, each data point is supplemented with irrelevant texts (selected E5 model) as part of the input documents. This addition is intended to increase the complexity and diversity of the dataset, thereby making it more challenging and realistic for training purposes.
| [
"# Answer Reformulation\n\nThe \"Answer Reformulation\" dataset is designed for a task that involves providing a detailed, comprehensive answer to a given question, supported by a set of related documents. The unique aspect of this dataset is the specific format required for the answers, emphasizing thorough exploration and understanding of the material in a specified language.\n\n---",
"## Dataset Details\nEach data point in the dataset has three main elements:\n - Query: A question that needs to be addressed\n - Input Documents (input_docs): A collection of documents related (or not) to the query. These documents may provide the information required to formulate the answer.\n - Answer: A comprehensive response to the query, incorporating information from the input documents and following strict citation guidelines, referencing specific input documents using citation IDs (e.g., [@1], [@2]). If the input documents do not provide sufficient information to answer the query, the response should be \"Answer not found.\"\n\n---",
"## Data Generation (with GPT)",
"### Local Question Answers\n\nQuestion Answer Generation: \nThe idea is to generate based on a complete and long document (a section from a textbook): \n - A question that could be answered based on the given document\n - An answer citing specific parts of the document using citation IDs\n\nTo achieve this: \n 1. Breaking down the complete document into smaller, numbered segments to facilitate the citation process.\n 2. Employing GPT-4 to generate both the question and its corresponding answer, which includes citations referring to the numbered segments of the text.\n\nPostprocess Enhancements:\nTo enrich the dataset, each data point is supplemented with irrelevant texts (selected E5 model) as part of the input documents. This addition is intended to increase the complexity and diversity of the dataset, thereby making it more challenging and realistic for training purposes."
] | [
"TAGS\n#region-us \n",
"# Answer Reformulation\n\nThe \"Answer Reformulation\" dataset is designed for a task that involves providing a detailed, comprehensive answer to a given question, supported by a set of related documents. The unique aspect of this dataset is the specific format required for the answers, emphasizing thorough exploration and understanding of the material in a specified language.\n\n---",
"## Dataset Details\nEach data point in the dataset has three main elements:\n - Query: A question that needs to be addressed\n - Input Documents (input_docs): A collection of documents related (or not) to the query. These documents may provide the information required to formulate the answer.\n - Answer: A comprehensive response to the query, incorporating information from the input documents and following strict citation guidelines, referencing specific input documents using citation IDs (e.g., [@1], [@2]). If the input documents do not provide sufficient information to answer the query, the response should be \"Answer not found.\"\n\n---",
"## Data Generation (with GPT)",
"### Local Question Answers\n\nQuestion Answer Generation: \nThe idea is to generate based on a complete and long document (a section from a textbook): \n - A question that could be answered based on the given document\n - An answer citing specific parts of the document using citation IDs\n\nTo achieve this: \n 1. Breaking down the complete document into smaller, numbered segments to facilitate the citation process.\n 2. Employing GPT-4 to generate both the question and its corresponding answer, which includes citations referring to the numbered segments of the text.\n\nPostprocess Enhancements:\nTo enrich the dataset, each data point is supplemented with irrelevant texts (selected E5 model) as part of the input documents. This addition is intended to increase the complexity and diversity of the dataset, thereby making it more challenging and realistic for training purposes."
] |
4e903e1193b9503682597fbe5862854a1d725e72 | # Dataset Card for "alpaca_farm-alpaca_gpt4_preference-re-preference_eval"
[More Information needed](https://github.com/huggingface/datasets/blob/main/CONTRIBUTING.md#how-to-contribute-to-the-dataset-cards) | Mitsuki-Sakamoto/alpaca_farm-alpaca_gpt4_preference-re-preference_eval | [
"region:us"
] | 2024-01-10T08:14:51+00:00 | {"dataset_info": [{"config_name": "42dot_LLM-SFT-1.3B", "features": [{"name": "instruction", "dtype": "string"}, {"name": "input", "dtype": "string"}, {"name": "output_1", "dtype": "string"}, {"name": "output_2", "dtype": "string"}, {"name": "preference", "dtype": "int64"}, {"name": "output", "dtype": "string"}, {"name": "generator", "dtype": "string"}, {"name": "sample_mode", "dtype": "string"}, {"name": "dataset", "dtype": "string"}, {"name": "datasplit", "dtype": "string"}, {"name": "prompt_format", "dtype": "string"}], "splits": [{"name": "preference", "num_bytes": 2424368, "num_examples": 1947}], "download_size": 1105540, "dataset_size": 2424368}, {"config_name": "opt-1.3b", "features": [{"name": "instruction", "dtype": "string"}, {"name": "input", "dtype": "string"}, {"name": "output_1", "dtype": "string"}, {"name": "output_2", "dtype": "string"}, {"name": "preference", "dtype": "int64"}, {"name": "output", "dtype": "string"}, {"name": "generator", "dtype": "string"}, {"name": "sample_mode", "dtype": "string"}, {"name": "dataset", "dtype": "string"}, {"name": "datasplit", "dtype": "string"}, {"name": "prompt_format", "dtype": "string"}], "splits": [{"name": "preference", "num_bytes": 3391896, "num_examples": 1947}], "download_size": 1582036, "dataset_size": 3391896}, {"config_name": "opt-1.3b_alpaca_farm_instructions_sft_constant_pa-checkpoint-10000", "features": [{"name": "instruction", "dtype": "string"}, {"name": "input", "dtype": "string"}, {"name": "output_1", "dtype": "string"}, {"name": "output_2", "dtype": "string"}, {"name": "preference", "dtype": "int64"}, {"name": "output", "dtype": "string"}, {"name": "generator", "dtype": "string"}, {"name": "sample_mode", "dtype": "string"}, {"name": "dataset", "dtype": "string"}, {"name": "datasplit", "dtype": "string"}, {"name": "prompt_format", "dtype": "string"}], "splits": [{"name": "preference", "num_bytes": 4571720, "num_examples": 1947}], "download_size": 2062683, "dataset_size": 4571720}, {"config_name": "opt-1.3b_alpaca_farm_instructions_sft_constant_pa-checkpoint-12500", "features": [{"name": "instruction", "dtype": "string"}, {"name": "input", "dtype": "string"}, {"name": "output_1", "dtype": "string"}, {"name": "output_2", "dtype": "string"}, {"name": "preference", "dtype": "int64"}, {"name": "output", "dtype": "string"}, {"name": "generator", "dtype": "string"}, {"name": "sample_mode", "dtype": "string"}, {"name": "dataset", "dtype": "string"}, {"name": "datasplit", "dtype": "string"}, {"name": "prompt_format", "dtype": "string"}], "splits": [{"name": "preference", "num_bytes": 4581082, "num_examples": 1947}], "download_size": 2074136, "dataset_size": 4581082}, {"config_name": "opt-1.3b_alpaca_farm_instructions_sft_constant_pa-checkpoint-15000", "features": [{"name": "instruction", "dtype": "string"}, {"name": "input", "dtype": "string"}, {"name": "output_1", "dtype": "string"}, {"name": "output_2", "dtype": "string"}, {"name": "preference", "dtype": "int64"}, {"name": "output", "dtype": "string"}, {"name": "generator", "dtype": "string"}, {"name": "sample_mode", "dtype": "string"}, {"name": "dataset", "dtype": "string"}, {"name": "datasplit", "dtype": "string"}, {"name": "prompt_format", "dtype": "string"}], "splits": [{"name": "preference", "num_bytes": 4609007, "num_examples": 1947}], "download_size": 2007282, "dataset_size": 4609007}, {"config_name": "opt-1.3b_alpaca_farm_instructions_sft_constant_pa-checkpoint-17500", "features": [{"name": "instruction", "dtype": "string"}, {"name": "input", "dtype": "string"}, {"name": "output_1", "dtype": "string"}, {"name": "output_2", "dtype": "string"}, {"name": "preference", "dtype": "int64"}, {"name": "output", "dtype": "string"}, {"name": "generator", "dtype": "string"}, {"name": "sample_mode", "dtype": "string"}, {"name": "dataset", "dtype": "string"}, {"name": "datasplit", "dtype": "string"}, {"name": "prompt_format", "dtype": "string"}], "splits": [{"name": "preference", "num_bytes": 4561880, "num_examples": 1947}], "download_size": 2013269, "dataset_size": 4561880}, {"config_name": "opt-1.3b_alpaca_farm_instructions_sft_constant_pa-checkpoint-20000", "features": [{"name": "instruction", "dtype": "string"}, {"name": "input", "dtype": "string"}, {"name": "output_1", "dtype": "string"}, {"name": "output_2", "dtype": "string"}, {"name": "preference", "dtype": "int64"}, {"name": "output", "dtype": "string"}, {"name": "generator", "dtype": "string"}, {"name": "sample_mode", "dtype": "string"}, {"name": "dataset", "dtype": "string"}, {"name": "datasplit", "dtype": "string"}, {"name": "prompt_format", "dtype": "string"}], "splits": [{"name": "preference", "num_bytes": 4556006, "num_examples": 1947}], "download_size": 2043801, "dataset_size": 4556006}, {"config_name": "opt-1.3b_alpaca_farm_instructions_sft_constant_pa-checkpoint-2500", "features": [{"name": "instruction", "dtype": "string"}, {"name": "input", "dtype": "string"}, {"name": "output_1", "dtype": "string"}, {"name": "output_2", "dtype": "string"}, {"name": "preference", "dtype": "int64"}, {"name": "output", "dtype": "string"}, {"name": "generator", "dtype": "string"}, {"name": "sample_mode", "dtype": "string"}, {"name": "dataset", "dtype": "string"}, {"name": "datasplit", "dtype": "string"}, {"name": "prompt_format", "dtype": "string"}], "splits": [{"name": "preference", "num_bytes": 4405822, "num_examples": 1947}], "download_size": 1951763, "dataset_size": 4405822}, {"config_name": "opt-1.3b_alpaca_farm_instructions_sft_constant_pa-checkpoint-25000", "features": [{"name": "instruction", "dtype": "string"}, {"name": "input", "dtype": "string"}, {"name": "output_1", "dtype": "string"}, {"name": "output_2", "dtype": "string"}, {"name": "preference", "dtype": "int64"}, {"name": "output", "dtype": "string"}, {"name": "generator", "dtype": "string"}, {"name": "sample_mode", "dtype": "string"}, {"name": "dataset", "dtype": "string"}, {"name": "datasplit", "dtype": "string"}, {"name": "prompt_format", "dtype": "string"}], "splits": [{"name": "preference", "num_bytes": 4600418, "num_examples": 1947}], "download_size": 2026316, "dataset_size": 4600418}, {"config_name": "opt-1.3b_alpaca_farm_instructions_sft_constant_pa-checkpoint-5000", "features": [{"name": "instruction", "dtype": "string"}, {"name": "input", "dtype": "string"}, {"name": "output_1", "dtype": "string"}, {"name": "output_2", "dtype": "string"}, {"name": "preference", "dtype": "int64"}, {"name": "output", "dtype": "string"}, {"name": "generator", "dtype": "string"}, {"name": "sample_mode", "dtype": "string"}, {"name": "dataset", "dtype": "string"}, {"name": "datasplit", "dtype": "string"}, {"name": "prompt_format", "dtype": "string"}], "splits": [{"name": "preference", "num_bytes": 4584026, "num_examples": 1947}], "download_size": 2079766, "dataset_size": 4584026}, {"config_name": "opt-1.3b_alpaca_farm_instructions_sft_constant_pa-checkpoint-7500", "features": [{"name": "instruction", "dtype": "string"}, {"name": "input", "dtype": "string"}, {"name": "output_1", "dtype": "string"}, {"name": "output_2", "dtype": "string"}, {"name": "preference", "dtype": "int64"}, {"name": "output", "dtype": "string"}, {"name": "generator", "dtype": "string"}, {"name": "sample_mode", "dtype": "string"}, {"name": "dataset", "dtype": "string"}, {"name": "datasplit", "dtype": "string"}, {"name": "prompt_format", "dtype": "string"}], "splits": [{"name": "preference", "num_bytes": 4483634, "num_examples": 1947}], "download_size": 2060995, "dataset_size": 4483634}, {"config_name": "pythia-1.4b", "features": [{"name": "instruction", "dtype": "string"}, {"name": "input", "dtype": "string"}, {"name": "output_1", "dtype": "string"}, {"name": "output_2", "dtype": "string"}, {"name": "preference", "dtype": "int64"}, {"name": "output", "dtype": "string"}, {"name": "generator", "dtype": "string"}, {"name": "sample_mode", "dtype": "string"}, {"name": "dataset", "dtype": "string"}, {"name": "datasplit", "dtype": "string"}, {"name": "prompt_format", "dtype": "string"}], "splits": [{"name": "preference", "num_bytes": 3153973, "num_examples": 1947}], "download_size": 1457855, "dataset_size": 3153973}, {"config_name": "pythia-1.4b_alpaca_farm_instructions_sft_constant_pa-checkpoint-10000", "features": [{"name": "instruction", "dtype": "string"}, {"name": "input", "dtype": "string"}, {"name": "output_1", "dtype": "string"}, {"name": "output_2", "dtype": "string"}, {"name": "preference", "dtype": "int64"}, {"name": "output", "dtype": "string"}, {"name": "generator", "dtype": "string"}, {"name": "sample_mode", "dtype": "string"}, {"name": "dataset", "dtype": "string"}, {"name": "datasplit", "dtype": "string"}, {"name": "prompt_format", "dtype": "string"}], "splits": [{"name": "preference", "num_bytes": 4680087, "num_examples": 1947}], "download_size": 2120681, "dataset_size": 4680087}, {"config_name": "pythia-1.4b_alpaca_farm_instructions_sft_constant_pa-checkpoint-12500", "features": [{"name": "instruction", "dtype": "string"}, {"name": "input", "dtype": "string"}, {"name": "output_1", "dtype": "string"}, {"name": "output_2", "dtype": "string"}, {"name": "preference", "dtype": "int64"}, {"name": "output", "dtype": "string"}, {"name": "generator", "dtype": "string"}, {"name": "sample_mode", "dtype": "string"}, {"name": "dataset", "dtype": "string"}, {"name": "datasplit", "dtype": "string"}, {"name": "prompt_format", "dtype": "string"}], "splits": [{"name": "preference", "num_bytes": 4630090, "num_examples": 1947}], "download_size": 2059183, "dataset_size": 4630090}, {"config_name": "pythia-1.4b_alpaca_farm_instructions_sft_constant_pa-checkpoint-15000", "features": [{"name": "instruction", "dtype": "string"}, {"name": "input", "dtype": "string"}, {"name": "output_1", "dtype": "string"}, {"name": "output_2", "dtype": "string"}, {"name": "preference", "dtype": "int64"}, {"name": "output", "dtype": "string"}, {"name": "generator", "dtype": "string"}, {"name": "sample_mode", "dtype": "string"}, {"name": "dataset", "dtype": "string"}, {"name": "datasplit", "dtype": "string"}, {"name": "prompt_format", "dtype": "string"}], "splits": [{"name": "preference", "num_bytes": 4648954, "num_examples": 1947}], "download_size": 2035508, "dataset_size": 4648954}, {"config_name": "pythia-1.4b_alpaca_farm_instructions_sft_constant_pa-checkpoint-17500", "features": [{"name": "instruction", "dtype": "string"}, {"name": "input", "dtype": "string"}, {"name": "output_1", "dtype": "string"}, {"name": "output_2", "dtype": "string"}, {"name": "preference", "dtype": "int64"}, {"name": "output", "dtype": "string"}, {"name": "generator", "dtype": "string"}, {"name": "sample_mode", "dtype": "string"}, {"name": "dataset", "dtype": "string"}, {"name": "datasplit", "dtype": "string"}, {"name": "prompt_format", "dtype": "string"}], "splits": [{"name": "preference", "num_bytes": 4619331, "num_examples": 1947}], "download_size": 2007853, "dataset_size": 4619331}, {"config_name": "pythia-1.4b_alpaca_farm_instructions_sft_constant_pa-checkpoint-20000", "features": [{"name": "instruction", "dtype": "string"}, {"name": "input", "dtype": "string"}, {"name": "output_1", "dtype": "string"}, {"name": "output_2", "dtype": "string"}, {"name": "preference", "dtype": "int64"}, {"name": "output", "dtype": "string"}, {"name": "generator", "dtype": "string"}, {"name": "sample_mode", "dtype": "string"}, {"name": "dataset", "dtype": "string"}, {"name": "datasplit", "dtype": "string"}, {"name": "prompt_format", "dtype": "string"}], "splits": [{"name": "preference", "num_bytes": 4670191, "num_examples": 1947}], "download_size": 2057546, "dataset_size": 4670191}, {"config_name": "pythia-1.4b_alpaca_farm_instructions_sft_constant_pa-checkpoint-22500", "features": [{"name": "instruction", "dtype": "string"}, {"name": "input", "dtype": "string"}, {"name": "output_1", "dtype": "string"}, {"name": "output_2", "dtype": "string"}, {"name": "preference", "dtype": "int64"}, {"name": "output", "dtype": "string"}, {"name": "generator", "dtype": "string"}, {"name": "sample_mode", "dtype": "string"}, {"name": "dataset", "dtype": "string"}, {"name": "datasplit", "dtype": "string"}, {"name": "prompt_format", "dtype": "string"}], "splits": [{"name": "preference", "num_bytes": 4647824, "num_examples": 1947}], "download_size": 2068755, "dataset_size": 4647824}, {"config_name": "pythia-1.4b_alpaca_farm_instructions_sft_constant_pa-checkpoint-2500", "features": [{"name": "instruction", "dtype": "string"}, {"name": "input", "dtype": "string"}, {"name": "output_1", "dtype": "string"}, {"name": "output_2", "dtype": "string"}, {"name": "preference", "dtype": "int64"}, {"name": "output", "dtype": "string"}, {"name": "generator", "dtype": "string"}, {"name": "sample_mode", "dtype": "string"}, {"name": "dataset", "dtype": "string"}, {"name": "datasplit", "dtype": "string"}, {"name": "prompt_format", "dtype": "string"}], "splits": [{"name": "preference", "num_bytes": 4415826, "num_examples": 1947}], "download_size": 1894728, "dataset_size": 4415826}, {"config_name": "pythia-1.4b_alpaca_farm_instructions_sft_constant_pa-checkpoint-25000", "features": [{"name": "instruction", "dtype": "string"}, {"name": "input", "dtype": "string"}, {"name": "output_1", "dtype": "string"}, {"name": "output_2", "dtype": "string"}, {"name": "preference", "dtype": "int64"}, {"name": "output", "dtype": "string"}, {"name": "generator", "dtype": "string"}, {"name": "sample_mode", "dtype": "string"}, {"name": "dataset", "dtype": "string"}, {"name": "datasplit", "dtype": "string"}, {"name": "prompt_format", "dtype": "string"}], "splits": [{"name": "preference", "num_bytes": 4618860, "num_examples": 1947}], "download_size": 1972799, "dataset_size": 4618860}, {"config_name": "pythia-1.4b_alpaca_farm_instructions_sft_constant_pa-checkpoint-5000", "features": [{"name": "instruction", "dtype": "string"}, {"name": "input", "dtype": "string"}, {"name": "output_1", "dtype": "string"}, {"name": "output_2", "dtype": "string"}, {"name": "preference", "dtype": "int64"}, {"name": "output", "dtype": "string"}, {"name": "generator", "dtype": "string"}, {"name": "sample_mode", "dtype": "string"}, {"name": "dataset", "dtype": "string"}, {"name": "datasplit", "dtype": "string"}, {"name": "prompt_format", "dtype": "string"}], "splits": [{"name": "preference", "num_bytes": 4668611, "num_examples": 1947}], "download_size": 2082476, "dataset_size": 4668611}, {"config_name": "pythia-1.4b_alpaca_farm_instructions_sft_constant_pa-checkpoint-7500", "features": [{"name": "instruction", "dtype": "string"}, {"name": "input", "dtype": "string"}, {"name": "output_1", "dtype": "string"}, {"name": "output_2", "dtype": "string"}, {"name": "preference", "dtype": "int64"}, {"name": "output", "dtype": "string"}, {"name": "generator", "dtype": "string"}, {"name": "sample_mode", "dtype": "string"}, {"name": "dataset", "dtype": "string"}, {"name": "datasplit", "dtype": "string"}, {"name": "prompt_format", "dtype": "string"}], "splits": [{"name": "preference", "num_bytes": 4533047, "num_examples": 1947}], "download_size": 1994024, "dataset_size": 4533047}, {"config_name": "pythia-1.4b_alpaca_farm_instructions_sft_constant_pac", "features": [{"name": "instruction", "dtype": "string"}, {"name": "input", "dtype": "string"}, {"name": "output_1", "dtype": "string"}, {"name": "output_2", "dtype": "string"}, {"name": "preference", "dtype": "int64"}, {"name": "output", "dtype": "string"}, {"name": "generator", "dtype": "string"}, {"name": "sample_mode", "dtype": "string"}, {"name": "dataset", "dtype": "string"}, {"name": "datasplit", "dtype": "string"}, {"name": "prompt_format", "dtype": "string"}], "splits": [{"name": "preference", "num_bytes": 4606727, "num_examples": 1947}], "download_size": 2027182, "dataset_size": 4606727}, {"config_name": "pythia-1.4b_alpaca_farm_instructions_sft_constant_pac-checkpoint-1000", "features": [{"name": "instruction", "dtype": "string"}, {"name": "input", "dtype": "string"}, {"name": "output_1", "dtype": "string"}, {"name": "output_2", "dtype": "string"}, {"name": "preference", "dtype": "int64"}, {"name": "output", "dtype": "string"}, {"name": "generator", "dtype": "string"}, {"name": "sample_mode", "dtype": "string"}, {"name": "dataset", "dtype": "string"}, {"name": "datasplit", "dtype": "string"}, {"name": "prompt_format", "dtype": "string"}], "splits": [{"name": "preference", "num_bytes": 4677544, "num_examples": 1947}], "download_size": 2156014, "dataset_size": 4677544}, {"config_name": "pythia-1.4b_alpaca_farm_instructions_sft_constant_pac-checkpoint-10000", "features": [{"name": "instruction", "dtype": "string"}, {"name": "input", "dtype": "string"}, {"name": "output_1", "dtype": "string"}, {"name": "output_2", "dtype": "string"}, {"name": "preference", "dtype": "int64"}, {"name": "output", "dtype": "string"}, {"name": "generator", "dtype": "string"}, {"name": "sample_mode", "dtype": "string"}, {"name": "dataset", "dtype": "string"}, {"name": "datasplit", "dtype": "string"}, {"name": "prompt_format", "dtype": "string"}], "splits": [{"name": "preference", "num_bytes": 4687049, "num_examples": 1947}], "download_size": 2098625, "dataset_size": 4687049}, {"config_name": "pythia-1.4b_alpaca_farm_instructions_sft_constant_pac-checkpoint-10500", "features": [{"name": "instruction", "dtype": "string"}, {"name": "input", "dtype": "string"}, {"name": "output_1", "dtype": "string"}, {"name": "output_2", "dtype": "string"}, {"name": "preference", "dtype": "int64"}, {"name": "output", "dtype": "string"}, {"name": "generator", "dtype": "string"}, {"name": "sample_mode", "dtype": "string"}, {"name": "dataset", "dtype": "string"}, {"name": "datasplit", "dtype": "string"}, {"name": "prompt_format", "dtype": "string"}], "splits": [{"name": "preference", "num_bytes": 4622949, "num_examples": 1947}], "download_size": 2039759, "dataset_size": 4622949}, {"config_name": "pythia-1.4b_alpaca_farm_instructions_sft_constant_pac-checkpoint-11000", "features": [{"name": "instruction", "dtype": "string"}, {"name": "input", "dtype": "string"}, {"name": "output_1", "dtype": "string"}, {"name": "output_2", "dtype": "string"}, {"name": "preference", "dtype": "int64"}, {"name": "output", "dtype": "string"}, {"name": "generator", "dtype": "string"}, {"name": "sample_mode", "dtype": "string"}, {"name": "dataset", "dtype": "string"}, {"name": "datasplit", "dtype": "string"}, {"name": "prompt_format", "dtype": "string"}], "splits": [{"name": "preference", "num_bytes": 4541747, "num_examples": 1947}], "download_size": 2012301, "dataset_size": 4541747}, {"config_name": "pythia-1.4b_alpaca_farm_instructions_sft_constant_pac-checkpoint-11500", "features": [{"name": "instruction", "dtype": "string"}, {"name": "input", "dtype": "string"}, {"name": "output_1", "dtype": "string"}, {"name": "output_2", "dtype": "string"}, {"name": "preference", "dtype": "int64"}, {"name": "output", "dtype": "string"}, {"name": "generator", "dtype": "string"}, {"name": "sample_mode", "dtype": "string"}, {"name": "dataset", "dtype": "string"}, {"name": "datasplit", "dtype": "string"}, {"name": "prompt_format", "dtype": "string"}], "splits": [{"name": "preference", "num_bytes": 4570899, "num_examples": 1947}], "download_size": 1998536, "dataset_size": 4570899}, {"config_name": "pythia-1.4b_alpaca_farm_instructions_sft_constant_pac-checkpoint-12000", "features": [{"name": "instruction", "dtype": "string"}, {"name": "input", "dtype": "string"}, {"name": "output_1", "dtype": "string"}, {"name": "output_2", "dtype": "string"}, {"name": "preference", "dtype": "int64"}, {"name": "output", "dtype": "string"}, {"name": "generator", "dtype": "string"}, {"name": "sample_mode", "dtype": "string"}, {"name": "dataset", "dtype": "string"}, {"name": "datasplit", "dtype": "string"}, {"name": "prompt_format", "dtype": "string"}], "splits": [{"name": "preference", "num_bytes": 4574198, "num_examples": 1947}], "download_size": 2043282, "dataset_size": 4574198}, {"config_name": "pythia-1.4b_alpaca_farm_instructions_sft_constant_pac-checkpoint-12500", "features": [{"name": "instruction", "dtype": "string"}, {"name": "input", "dtype": "string"}, {"name": "output_1", "dtype": "string"}, {"name": "output_2", "dtype": "string"}, {"name": "preference", "dtype": "int64"}, {"name": "output", "dtype": "string"}, {"name": "generator", "dtype": "string"}, {"name": "sample_mode", "dtype": "string"}, {"name": "dataset", "dtype": "string"}, {"name": "datasplit", "dtype": "string"}, {"name": "prompt_format", "dtype": "string"}], "splits": [{"name": "preference", "num_bytes": 4640259, "num_examples": 1947}], "download_size": 2040145, "dataset_size": 4640259}, {"config_name": "pythia-1.4b_alpaca_farm_instructions_sft_constant_pac-checkpoint-1500", "features": [{"name": "instruction", "dtype": "string"}, {"name": "input", "dtype": "string"}, {"name": "output_1", "dtype": "string"}, {"name": "output_2", "dtype": "string"}, {"name": "preference", "dtype": "int64"}, {"name": "output", "dtype": "string"}, {"name": "generator", "dtype": "string"}, {"name": "sample_mode", "dtype": "string"}, {"name": "dataset", "dtype": "string"}, {"name": "datasplit", "dtype": "string"}, {"name": "prompt_format", "dtype": "string"}], "splits": [{"name": "preference", "num_bytes": 4615750, "num_examples": 1947}], "download_size": 2023356, "dataset_size": 4615750}, {"config_name": "pythia-1.4b_alpaca_farm_instructions_sft_constant_pac-checkpoint-2000", "features": [{"name": "instruction", "dtype": "string"}, {"name": "input", "dtype": "string"}, {"name": "output_1", "dtype": "string"}, {"name": "output_2", "dtype": "string"}, {"name": "preference", "dtype": "int64"}, {"name": "output", "dtype": "string"}, {"name": "generator", "dtype": "string"}, {"name": "sample_mode", "dtype": "string"}, {"name": "dataset", "dtype": "string"}, {"name": "datasplit", "dtype": "string"}, {"name": "prompt_format", "dtype": "string"}], "splits": [{"name": "preference", "num_bytes": 4503451, "num_examples": 1947}], "download_size": 1972806, "dataset_size": 4503451}, {"config_name": "pythia-1.4b_alpaca_farm_instructions_sft_constant_pac-checkpoint-2500", "features": [{"name": "instruction", "dtype": "string"}, {"name": "input", "dtype": "string"}, {"name": "output_1", "dtype": "string"}, {"name": "output_2", "dtype": "string"}, {"name": "preference", "dtype": "int64"}, {"name": "output", "dtype": "string"}, {"name": "generator", "dtype": "string"}, {"name": "sample_mode", "dtype": "string"}, {"name": "dataset", "dtype": "string"}, {"name": "datasplit", "dtype": "string"}, {"name": "prompt_format", "dtype": "string"}], "splits": [{"name": "preference", "num_bytes": 4438189, "num_examples": 1947}], "download_size": 1889463, "dataset_size": 4438189}, {"config_name": "pythia-1.4b_alpaca_farm_instructions_sft_constant_pac-checkpoint-3000", "features": [{"name": "instruction", "dtype": "string"}, {"name": "input", "dtype": "string"}, {"name": "output_1", "dtype": "string"}, {"name": "output_2", "dtype": "string"}, {"name": "preference", "dtype": "int64"}, {"name": "output", "dtype": "string"}, {"name": "generator", "dtype": "string"}, {"name": "sample_mode", "dtype": "string"}, {"name": "dataset", "dtype": "string"}, {"name": "datasplit", "dtype": "string"}, {"name": "prompt_format", "dtype": "string"}], "splits": [{"name": "preference", "num_bytes": 4660840, "num_examples": 1947}], "download_size": 2018963, "dataset_size": 4660840}, {"config_name": "pythia-1.4b_alpaca_farm_instructions_sft_constant_pac-checkpoint-3500", "features": [{"name": "instruction", "dtype": "string"}, {"name": "input", "dtype": "string"}, {"name": "output_1", "dtype": "string"}, {"name": "output_2", "dtype": "string"}, {"name": "preference", "dtype": "int64"}, {"name": "output", "dtype": "string"}, {"name": "generator", "dtype": "string"}, {"name": "sample_mode", "dtype": "string"}, {"name": "dataset", "dtype": "string"}, {"name": "datasplit", "dtype": "string"}, {"name": "prompt_format", "dtype": "string"}], "splits": [{"name": "preference", "num_bytes": 4666795, "num_examples": 1947}], "download_size": 2068065, "dataset_size": 4666795}, {"config_name": "pythia-1.4b_alpaca_farm_instructions_sft_constant_pac-checkpoint-4000", "features": [{"name": "instruction", "dtype": "string"}, {"name": "input", "dtype": "string"}, {"name": "output_1", "dtype": "string"}, {"name": "output_2", "dtype": "string"}, {"name": "preference", "dtype": "int64"}, {"name": "output", "dtype": "string"}, {"name": "generator", "dtype": "string"}, {"name": "sample_mode", "dtype": "string"}, {"name": "dataset", "dtype": "string"}, {"name": "datasplit", "dtype": "string"}, {"name": "prompt_format", "dtype": "string"}], "splits": [{"name": "preference", "num_bytes": 4659921, "num_examples": 1947}], "download_size": 2090866, "dataset_size": 4659921}, {"config_name": "pythia-1.4b_alpaca_farm_instructions_sft_constant_pac-checkpoint-4500", "features": [{"name": "instruction", "dtype": "string"}, {"name": "input", "dtype": "string"}, {"name": "output_1", "dtype": "string"}, {"name": "output_2", "dtype": "string"}, {"name": "preference", "dtype": "int64"}, {"name": "output", "dtype": "string"}, {"name": "generator", "dtype": "string"}, {"name": "sample_mode", "dtype": "string"}, {"name": "dataset", "dtype": "string"}, {"name": "datasplit", "dtype": "string"}, {"name": "prompt_format", "dtype": "string"}], "splits": [{"name": "preference", "num_bytes": 4514215, "num_examples": 1947}], "download_size": 1906139, "dataset_size": 4514215}, {"config_name": "pythia-1.4b_alpaca_farm_instructions_sft_constant_pac-checkpoint-500", "features": [{"name": "instruction", "dtype": "string"}, {"name": "input", "dtype": "string"}, {"name": "output_1", "dtype": "string"}, {"name": "output_2", "dtype": "string"}, {"name": "preference", "dtype": "int64"}, {"name": "output", "dtype": "string"}, {"name": "generator", "dtype": "string"}, {"name": "sample_mode", "dtype": "string"}, {"name": "dataset", "dtype": "string"}, {"name": "datasplit", "dtype": "string"}, {"name": "prompt_format", "dtype": "string"}], "splits": [{"name": "preference", "num_bytes": 4524274, "num_examples": 1947}], "download_size": 2080294, "dataset_size": 4524274}, {"config_name": "pythia-1.4b_alpaca_farm_instructions_sft_constant_pac-checkpoint-5000", "features": [{"name": "instruction", "dtype": "string"}, {"name": "input", "dtype": "string"}, {"name": "output_1", "dtype": "string"}, {"name": "output_2", "dtype": "string"}, {"name": "preference", "dtype": "int64"}, {"name": "output", "dtype": "string"}, {"name": "generator", "dtype": "string"}, {"name": "sample_mode", "dtype": "string"}, {"name": "dataset", "dtype": "string"}, {"name": "datasplit", "dtype": "string"}, {"name": "prompt_format", "dtype": "string"}], "splits": [{"name": "preference", "num_bytes": 4597420, "num_examples": 1947}], "download_size": 2062025, "dataset_size": 4597420}, {"config_name": "pythia-1.4b_alpaca_farm_instructions_sft_constant_pac-checkpoint-5500", "features": [{"name": "instruction", "dtype": "string"}, {"name": "input", "dtype": "string"}, {"name": "output_1", "dtype": "string"}, {"name": "output_2", "dtype": "string"}, {"name": "preference", "dtype": "int64"}, {"name": "output", "dtype": "string"}, {"name": "generator", "dtype": "string"}, {"name": "sample_mode", "dtype": "string"}, {"name": "dataset", "dtype": "string"}, {"name": "datasplit", "dtype": "string"}, {"name": "prompt_format", "dtype": "string"}], "splits": [{"name": "preference", "num_bytes": 4609709, "num_examples": 1947}], "download_size": 1973691, "dataset_size": 4609709}, {"config_name": "pythia-1.4b_alpaca_farm_instructions_sft_constant_pac-checkpoint-6000", "features": [{"name": "instruction", "dtype": "string"}, {"name": "input", "dtype": "string"}, {"name": "output_1", "dtype": "string"}, {"name": "output_2", "dtype": "string"}, {"name": "preference", "dtype": "int64"}, {"name": "output", "dtype": "string"}, {"name": "generator", "dtype": "string"}, {"name": "sample_mode", "dtype": "string"}, {"name": "dataset", "dtype": "string"}, {"name": "datasplit", "dtype": "string"}, {"name": "prompt_format", "dtype": "string"}], "splits": [{"name": "preference", "num_bytes": 4565628, "num_examples": 1947}], "download_size": 2003409, "dataset_size": 4565628}, {"config_name": "pythia-1.4b_alpaca_farm_instructions_sft_constant_pac-checkpoint-6500", "features": [{"name": "instruction", "dtype": "string"}, {"name": "input", "dtype": "string"}, {"name": "output_1", "dtype": "string"}, {"name": "output_2", "dtype": "string"}, {"name": "preference", "dtype": "int64"}, {"name": "output", "dtype": "string"}, {"name": "generator", "dtype": "string"}, {"name": "sample_mode", "dtype": "string"}, {"name": "dataset", "dtype": "string"}, {"name": "datasplit", "dtype": "string"}, {"name": "prompt_format", "dtype": "string"}], "splits": [{"name": "preference", "num_bytes": 4642271, "num_examples": 1947}], "download_size": 1993168, "dataset_size": 4642271}, {"config_name": "pythia-1.4b_alpaca_farm_instructions_sft_constant_pac-checkpoint-7000", "features": [{"name": "instruction", "dtype": "string"}, {"name": "input", "dtype": "string"}, {"name": "output_1", "dtype": "string"}, {"name": "output_2", "dtype": "string"}, {"name": "preference", "dtype": "int64"}, {"name": "output", "dtype": "string"}, {"name": "generator", "dtype": "string"}, {"name": "sample_mode", "dtype": "string"}, {"name": "dataset", "dtype": "string"}, {"name": "datasplit", "dtype": "string"}, {"name": "prompt_format", "dtype": "string"}], "splits": [{"name": "preference", "num_bytes": 4675241, "num_examples": 1947}], "download_size": 2062903, "dataset_size": 4675241}, {"config_name": "pythia-1.4b_alpaca_farm_instructions_sft_constant_pac-checkpoint-7500", "features": [{"name": "instruction", "dtype": "string"}, {"name": "input", "dtype": "string"}, {"name": "output_1", "dtype": "string"}, {"name": "output_2", "dtype": "string"}, {"name": "preference", "dtype": "int64"}, {"name": "output", "dtype": "string"}, {"name": "generator", "dtype": "string"}, {"name": "sample_mode", "dtype": "string"}, {"name": "dataset", "dtype": "string"}, {"name": "datasplit", "dtype": "string"}, {"name": "prompt_format", "dtype": "string"}], "splits": [{"name": "preference", "num_bytes": 4619399, "num_examples": 1947}], "download_size": 2038601, "dataset_size": 4619399}, {"config_name": "pythia-1.4b_alpaca_farm_instructions_sft_constant_pac-checkpoint-8000", "features": [{"name": "instruction", "dtype": "string"}, {"name": "input", "dtype": "string"}, {"name": "output_1", "dtype": "string"}, {"name": "output_2", "dtype": "string"}, {"name": "preference", "dtype": "int64"}, {"name": "output", "dtype": "string"}, {"name": "generator", "dtype": "string"}, {"name": "sample_mode", "dtype": "string"}, {"name": "dataset", "dtype": "string"}, {"name": "datasplit", "dtype": "string"}, {"name": "prompt_format", "dtype": "string"}], "splits": [{"name": "preference", "num_bytes": 4643594, "num_examples": 1947}], "download_size": 2080602, "dataset_size": 4643594}, {"config_name": "pythia-1.4b_alpaca_farm_instructions_sft_constant_pac-checkpoint-8500", "features": [{"name": "instruction", "dtype": "string"}, {"name": "input", "dtype": "string"}, {"name": "output_1", "dtype": "string"}, {"name": "output_2", "dtype": "string"}, {"name": "preference", "dtype": "int64"}, {"name": "output", "dtype": "string"}, {"name": "generator", "dtype": "string"}, {"name": "sample_mode", "dtype": "string"}, {"name": "dataset", "dtype": "string"}, {"name": "datasplit", "dtype": "string"}, {"name": "prompt_format", "dtype": "string"}], "splits": [{"name": "preference", "num_bytes": 4659586, "num_examples": 1947}], "download_size": 2079365, "dataset_size": 4659586}, {"config_name": "pythia-1.4b_alpaca_farm_instructions_sft_constant_pac-checkpoint-9000", "features": [{"name": "instruction", "dtype": "string"}, {"name": "input", "dtype": "string"}, {"name": "output_1", "dtype": "string"}, {"name": "output_2", "dtype": "string"}, {"name": "preference", "dtype": "int64"}, {"name": "output", "dtype": "string"}, {"name": "generator", "dtype": "string"}, {"name": "sample_mode", "dtype": "string"}, {"name": "dataset", "dtype": "string"}, {"name": "datasplit", "dtype": "string"}, {"name": "prompt_format", "dtype": "string"}], "splits": [{"name": "preference", "num_bytes": 4621685, "num_examples": 1947}], "download_size": 2060891, "dataset_size": 4621685}, {"config_name": "pythia-1.4b_alpaca_farm_instructions_sft_constant_pac-checkpoint-9500", "features": [{"name": "instruction", "dtype": "string"}, {"name": "input", "dtype": "string"}, {"name": "output_1", "dtype": "string"}, {"name": "output_2", "dtype": "string"}, {"name": "preference", "dtype": "int64"}, {"name": "output", "dtype": "string"}, {"name": "generator", "dtype": "string"}, {"name": "sample_mode", "dtype": "string"}, {"name": "dataset", "dtype": "string"}, {"name": "datasplit", "dtype": "string"}, {"name": "prompt_format", "dtype": "string"}], "splits": [{"name": "preference", "num_bytes": 4653351, "num_examples": 1947}], "download_size": 2028083, "dataset_size": 4653351}, {"config_name": "pythia-1.4b_alpaca_farm_instructions_sft_sep", "features": [{"name": "instruction", "dtype": "string"}, {"name": "input", "dtype": "string"}, {"name": "output_1", "dtype": "string"}, {"name": "output_2", "dtype": "string"}, {"name": "preference", "dtype": "int64"}, {"name": "output", "dtype": "string"}, {"name": "generator", "dtype": "string"}, {"name": "sample_mode", "dtype": "string"}, {"name": "dataset", "dtype": "string"}, {"name": "datasplit", "dtype": "string"}, {"name": "prompt_format", "dtype": "string"}], "splits": [{"name": "preference", "num_bytes": 4340565, "num_examples": 1947}], "download_size": 1878882, "dataset_size": 4340565}, {"config_name": "pythia-1.4b_alpaca_farm_instructions_sft_sep-checkpoint-1000", "features": [{"name": "instruction", "dtype": "string"}, {"name": "input", "dtype": "string"}, {"name": "output_1", "dtype": "string"}, {"name": "output_2", "dtype": "string"}, {"name": "preference", "dtype": "int64"}, {"name": "output", "dtype": "string"}, {"name": "generator", "dtype": "string"}, {"name": "sample_mode", "dtype": "string"}, {"name": "dataset", "dtype": "string"}, {"name": "datasplit", "dtype": "string"}, {"name": "prompt_format", "dtype": "string"}], "splits": [{"name": "preference", "num_bytes": 4435324, "num_examples": 1947}], "download_size": 2106629, "dataset_size": 4435324}, {"config_name": "pythia-1.4b_alpaca_farm_instructions_sft_sep-checkpoint-10000", "features": [{"name": "instruction", "dtype": "string"}, {"name": "input", "dtype": "string"}, {"name": "output_1", "dtype": "string"}, {"name": "output_2", "dtype": "string"}, {"name": "preference", "dtype": "int64"}, {"name": "output", "dtype": "string"}, {"name": "generator", "dtype": "string"}, {"name": "sample_mode", "dtype": "string"}, {"name": "dataset", "dtype": "string"}, {"name": "datasplit", "dtype": "string"}, {"name": "prompt_format", "dtype": "string"}], "splits": [{"name": "preference", "num_bytes": 4336709, "num_examples": 1947}], "download_size": 1957513, "dataset_size": 4336709}, {"config_name": "pythia-1.4b_alpaca_farm_instructions_sft_sep-checkpoint-10500", "features": [{"name": "instruction", "dtype": "string"}, {"name": "input", "dtype": "string"}, {"name": "output_1", "dtype": "string"}, {"name": "output_2", "dtype": "string"}, {"name": "preference", "dtype": "int64"}, {"name": "output", "dtype": "string"}, {"name": "generator", "dtype": "string"}, {"name": "sample_mode", "dtype": "string"}, {"name": "dataset", "dtype": "string"}, {"name": "datasplit", "dtype": "string"}, {"name": "prompt_format", "dtype": "string"}], "splits": [{"name": "preference", "num_bytes": 4411542, "num_examples": 1947}], "download_size": 2029794, "dataset_size": 4411542}, {"config_name": "pythia-1.4b_alpaca_farm_instructions_sft_sep-checkpoint-11000", "features": [{"name": "instruction", "dtype": "string"}, {"name": "input", "dtype": "string"}, {"name": "output_1", "dtype": "string"}, {"name": "output_2", "dtype": "string"}, {"name": "preference", "dtype": "int64"}, {"name": "output", "dtype": "string"}, {"name": "generator", "dtype": "string"}, {"name": "sample_mode", "dtype": "string"}, {"name": "dataset", "dtype": "string"}, {"name": "datasplit", "dtype": "string"}, {"name": "prompt_format", "dtype": "string"}], "splits": [{"name": "preference", "num_bytes": 4490413, "num_examples": 1947}], "download_size": 2046163, "dataset_size": 4490413}, {"config_name": "pythia-1.4b_alpaca_farm_instructions_sft_sep-checkpoint-11500", "features": [{"name": "instruction", "dtype": "string"}, {"name": "input", "dtype": "string"}, {"name": "output_1", "dtype": "string"}, {"name": "output_2", "dtype": "string"}, {"name": "preference", "dtype": "int64"}, {"name": "output", "dtype": "string"}, {"name": "generator", "dtype": "string"}, {"name": "sample_mode", "dtype": "string"}, {"name": "dataset", "dtype": "string"}, {"name": "datasplit", "dtype": "string"}, {"name": "prompt_format", "dtype": "string"}], "splits": [{"name": "preference", "num_bytes": 4338792, "num_examples": 1947}], "download_size": 1934221, "dataset_size": 4338792}, {"config_name": "pythia-1.4b_alpaca_farm_instructions_sft_sep-checkpoint-12000", "features": [{"name": "instruction", "dtype": "string"}, {"name": "input", "dtype": "string"}, {"name": "output_1", "dtype": "string"}, {"name": "output_2", "dtype": "string"}, {"name": "preference", "dtype": "int64"}, {"name": "output", "dtype": "string"}, {"name": "generator", "dtype": "string"}, {"name": "sample_mode", "dtype": "string"}, {"name": "dataset", "dtype": "string"}, {"name": "datasplit", "dtype": "string"}, {"name": "prompt_format", "dtype": "string"}], "splits": [{"name": "preference", "num_bytes": 4426615, "num_examples": 1947}], "download_size": 1948075, "dataset_size": 4426615}, {"config_name": "pythia-1.4b_alpaca_farm_instructions_sft_sep-checkpoint-12500", "features": [{"name": "instruction", "dtype": "string"}, {"name": "input", "dtype": "string"}, {"name": "output_1", "dtype": "string"}, {"name": "output_2", "dtype": "string"}, {"name": "preference", "dtype": "int64"}, {"name": "output", "dtype": "string"}, {"name": "generator", "dtype": "string"}, {"name": "sample_mode", "dtype": "string"}, {"name": "dataset", "dtype": "string"}, {"name": "datasplit", "dtype": "string"}, {"name": "prompt_format", "dtype": "string"}], "splits": [{"name": "preference", "num_bytes": 4371597, "num_examples": 1947}], "download_size": 1869432, "dataset_size": 4371597}, {"config_name": "pythia-1.4b_alpaca_farm_instructions_sft_sep-checkpoint-1500", "features": [{"name": "instruction", "dtype": "string"}, {"name": "input", "dtype": "string"}, {"name": "output_1", "dtype": "string"}, {"name": "output_2", "dtype": "string"}, {"name": "preference", "dtype": "int64"}, {"name": "output", "dtype": "string"}, {"name": "generator", "dtype": "string"}, {"name": "sample_mode", "dtype": "string"}, {"name": "dataset", "dtype": "string"}, {"name": "datasplit", "dtype": "string"}, {"name": "prompt_format", "dtype": "string"}], "splits": [{"name": "preference", "num_bytes": 4385915, "num_examples": 1947}], "download_size": 1987645, "dataset_size": 4385915}, {"config_name": "pythia-1.4b_alpaca_farm_instructions_sft_sep-checkpoint-2000", "features": [{"name": "instruction", "dtype": "string"}, {"name": "input", "dtype": "string"}, {"name": "output_1", "dtype": "string"}, {"name": "output_2", "dtype": "string"}, {"name": "preference", "dtype": "int64"}, {"name": "output", "dtype": "string"}, {"name": "generator", "dtype": "string"}, {"name": "sample_mode", "dtype": "string"}, {"name": "dataset", "dtype": "string"}, {"name": "datasplit", "dtype": "string"}, {"name": "prompt_format", "dtype": "string"}], "splits": [{"name": "preference", "num_bytes": 4260341, "num_examples": 1947}], "download_size": 1925366, "dataset_size": 4260341}, {"config_name": "pythia-1.4b_alpaca_farm_instructions_sft_sep-checkpoint-2500", "features": [{"name": "instruction", "dtype": "string"}, {"name": "input", "dtype": "string"}, {"name": "output_1", "dtype": "string"}, {"name": "output_2", "dtype": "string"}, {"name": "preference", "dtype": "int64"}, {"name": "output", "dtype": "string"}, {"name": "generator", "dtype": "string"}, {"name": "sample_mode", "dtype": "string"}, {"name": "dataset", "dtype": "string"}, {"name": "datasplit", "dtype": "string"}, {"name": "prompt_format", "dtype": "string"}], "splits": [{"name": "preference", "num_bytes": 4147295, "num_examples": 1947}], "download_size": 1817509, "dataset_size": 4147295}, {"config_name": "pythia-1.4b_alpaca_farm_instructions_sft_sep-checkpoint-3000", "features": [{"name": "instruction", "dtype": "string"}, {"name": "input", "dtype": "string"}, {"name": "output_1", "dtype": "string"}, {"name": "output_2", "dtype": "string"}, {"name": "preference", "dtype": "int64"}, {"name": "output", "dtype": "string"}, {"name": "generator", "dtype": "string"}, {"name": "sample_mode", "dtype": "string"}, {"name": "dataset", "dtype": "string"}, {"name": "datasplit", "dtype": "string"}, {"name": "prompt_format", "dtype": "string"}], "splits": [{"name": "preference", "num_bytes": 4356468, "num_examples": 1947}], "download_size": 1947759, "dataset_size": 4356468}, {"config_name": "pythia-1.4b_alpaca_farm_instructions_sft_sep-checkpoint-3500", "features": [{"name": "instruction", "dtype": "string"}, {"name": "input", "dtype": "string"}, {"name": "output_1", "dtype": "string"}, {"name": "output_2", "dtype": "string"}, {"name": "preference", "dtype": "int64"}, {"name": "output", "dtype": "string"}, {"name": "generator", "dtype": "string"}, {"name": "sample_mode", "dtype": "string"}, {"name": "dataset", "dtype": "string"}, {"name": "datasplit", "dtype": "string"}, {"name": "prompt_format", "dtype": "string"}], "splits": [{"name": "preference", "num_bytes": 4354855, "num_examples": 1947}], "download_size": 2028617, "dataset_size": 4354855}, {"config_name": "pythia-1.4b_alpaca_farm_instructions_sft_sep-checkpoint-4000", "features": [{"name": "instruction", "dtype": "string"}, {"name": "input", "dtype": "string"}, {"name": "output_1", "dtype": "string"}, {"name": "output_2", "dtype": "string"}, {"name": "preference", "dtype": "int64"}, {"name": "output", "dtype": "string"}, {"name": "generator", "dtype": "string"}, {"name": "sample_mode", "dtype": "string"}, {"name": "dataset", "dtype": "string"}, {"name": "datasplit", "dtype": "string"}, {"name": "prompt_format", "dtype": "string"}], "splits": [{"name": "preference", "num_bytes": 4388027, "num_examples": 1947}], "download_size": 2035873, "dataset_size": 4388027}, {"config_name": "pythia-1.4b_alpaca_farm_instructions_sft_sep-checkpoint-4500", "features": [{"name": "instruction", "dtype": "string"}, {"name": "input", "dtype": "string"}, {"name": "output_1", "dtype": "string"}, {"name": "output_2", "dtype": "string"}, {"name": "preference", "dtype": "int64"}, {"name": "output", "dtype": "string"}, {"name": "generator", "dtype": "string"}, {"name": "sample_mode", "dtype": "string"}, {"name": "dataset", "dtype": "string"}, {"name": "datasplit", "dtype": "string"}, {"name": "prompt_format", "dtype": "string"}], "splits": [{"name": "preference", "num_bytes": 4248658, "num_examples": 1947}], "download_size": 1869186, "dataset_size": 4248658}, {"config_name": "pythia-1.4b_alpaca_farm_instructions_sft_sep-checkpoint-500", "features": [{"name": "instruction", "dtype": "string"}, {"name": "input", "dtype": "string"}, {"name": "output_1", "dtype": "string"}, {"name": "output_2", "dtype": "string"}, {"name": "preference", "dtype": "int64"}, {"name": "output", "dtype": "string"}, {"name": "generator", "dtype": "string"}, {"name": "sample_mode", "dtype": "string"}, {"name": "dataset", "dtype": "string"}, {"name": "datasplit", "dtype": "string"}, {"name": "prompt_format", "dtype": "string"}], "splits": [{"name": "preference", "num_bytes": 4316458, "num_examples": 1947}], "download_size": 2022333, "dataset_size": 4316458}, {"config_name": "pythia-1.4b_alpaca_farm_instructions_sft_sep-checkpoint-5000", "features": [{"name": "instruction", "dtype": "string"}, {"name": "input", "dtype": "string"}, {"name": "output_1", "dtype": "string"}, {"name": "output_2", "dtype": "string"}, {"name": "preference", "dtype": "int64"}, {"name": "output", "dtype": "string"}, {"name": "generator", "dtype": "string"}, {"name": "sample_mode", "dtype": "string"}, {"name": "dataset", "dtype": "string"}, {"name": "datasplit", "dtype": "string"}, {"name": "prompt_format", "dtype": "string"}], "splits": [{"name": "preference", "num_bytes": 4379043, "num_examples": 1947}], "download_size": 2016049, "dataset_size": 4379043}, {"config_name": "pythia-1.4b_alpaca_farm_instructions_sft_sep-checkpoint-5500", "features": [{"name": "instruction", "dtype": "string"}, {"name": "input", "dtype": "string"}, {"name": "output_1", "dtype": "string"}, {"name": "output_2", "dtype": "string"}, {"name": "preference", "dtype": "int64"}, {"name": "output", "dtype": "string"}, {"name": "generator", "dtype": "string"}, {"name": "sample_mode", "dtype": "string"}, {"name": "dataset", "dtype": "string"}, {"name": "datasplit", "dtype": "string"}, {"name": "prompt_format", "dtype": "string"}], "splits": [{"name": "preference", "num_bytes": 4440792, "num_examples": 1947}], "download_size": 2068021, "dataset_size": 4440792}, {"config_name": "pythia-1.4b_alpaca_farm_instructions_sft_sep-checkpoint-6000", "features": [{"name": "instruction", "dtype": "string"}, {"name": "input", "dtype": "string"}, {"name": "output_1", "dtype": "string"}, {"name": "output_2", "dtype": "string"}, {"name": "preference", "dtype": "int64"}, {"name": "output", "dtype": "string"}, {"name": "generator", "dtype": "string"}, {"name": "sample_mode", "dtype": "string"}, {"name": "dataset", "dtype": "string"}, {"name": "datasplit", "dtype": "string"}, {"name": "prompt_format", "dtype": "string"}], "splits": [{"name": "preference", "num_bytes": 4280137, "num_examples": 1947}], "download_size": 1922600, "dataset_size": 4280137}, {"config_name": "pythia-1.4b_alpaca_farm_instructions_sft_sep-checkpoint-6500", "features": [{"name": "instruction", "dtype": "string"}, {"name": "input", "dtype": "string"}, {"name": "output_1", "dtype": "string"}, {"name": "output_2", "dtype": "string"}, {"name": "preference", "dtype": "int64"}, {"name": "output", "dtype": "string"}, {"name": "generator", "dtype": "string"}, {"name": "sample_mode", "dtype": "string"}, {"name": "dataset", "dtype": "string"}, {"name": "datasplit", "dtype": "string"}, {"name": "prompt_format", "dtype": "string"}], "splits": [{"name": "preference", "num_bytes": 4380647, "num_examples": 1947}], "download_size": 1982355, "dataset_size": 4380647}, {"config_name": "pythia-1.4b_alpaca_farm_instructions_sft_sep-checkpoint-7000", "features": [{"name": "instruction", "dtype": "string"}, {"name": "input", "dtype": "string"}, {"name": "output_1", "dtype": "string"}, {"name": "output_2", "dtype": "string"}, {"name": "preference", "dtype": "int64"}, {"name": "output", "dtype": "string"}, {"name": "generator", "dtype": "string"}, {"name": "sample_mode", "dtype": "string"}, {"name": "dataset", "dtype": "string"}, {"name": "datasplit", "dtype": "string"}, {"name": "prompt_format", "dtype": "string"}], "splits": [{"name": "preference", "num_bytes": 4356267, "num_examples": 1947}], "download_size": 1924906, "dataset_size": 4356267}, {"config_name": "pythia-1.4b_alpaca_farm_instructions_sft_sep-checkpoint-7500", "features": [{"name": "instruction", "dtype": "string"}, {"name": "input", "dtype": "string"}, {"name": "output_1", "dtype": "string"}, {"name": "output_2", "dtype": "string"}, {"name": "preference", "dtype": "int64"}, {"name": "output", "dtype": "string"}, {"name": "generator", "dtype": "string"}, {"name": "sample_mode", "dtype": "string"}, {"name": "dataset", "dtype": "string"}, {"name": "datasplit", "dtype": "string"}, {"name": "prompt_format", "dtype": "string"}], "splits": [{"name": "preference", "num_bytes": 4385671, "num_examples": 1947}], "download_size": 1998801, "dataset_size": 4385671}, {"config_name": "pythia-1.4b_alpaca_farm_instructions_sft_sep-checkpoint-8000", "features": [{"name": "instruction", "dtype": "string"}, {"name": "input", "dtype": "string"}, {"name": "output_1", "dtype": "string"}, {"name": "output_2", "dtype": "string"}, {"name": "preference", "dtype": "int64"}, {"name": "output", "dtype": "string"}, {"name": "generator", "dtype": "string"}, {"name": "sample_mode", "dtype": "string"}, {"name": "dataset", "dtype": "string"}, {"name": "datasplit", "dtype": "string"}, {"name": "prompt_format", "dtype": "string"}], "splits": [{"name": "preference", "num_bytes": 4359715, "num_examples": 1947}], "download_size": 1980369, "dataset_size": 4359715}, {"config_name": "pythia-1.4b_alpaca_farm_instructions_sft_sep-checkpoint-8500", "features": [{"name": "instruction", "dtype": "string"}, {"name": "input", "dtype": "string"}, {"name": "output_1", "dtype": "string"}, {"name": "output_2", "dtype": "string"}, {"name": "preference", "dtype": "int64"}, {"name": "output", "dtype": "string"}, {"name": "generator", "dtype": "string"}, {"name": "sample_mode", "dtype": "string"}, {"name": "dataset", "dtype": "string"}, {"name": "datasplit", "dtype": "string"}, {"name": "prompt_format", "dtype": "string"}], "splits": [{"name": "preference", "num_bytes": 4416499, "num_examples": 1947}], "download_size": 2004097, "dataset_size": 4416499}, {"config_name": "pythia-1.4b_alpaca_farm_instructions_sft_sep-checkpoint-9000", "features": [{"name": "instruction", "dtype": "string"}, {"name": "input", "dtype": "string"}, {"name": "output_1", "dtype": "string"}, {"name": "output_2", "dtype": "string"}, {"name": "preference", "dtype": "int64"}, {"name": "output", "dtype": "string"}, {"name": "generator", "dtype": "string"}, {"name": "sample_mode", "dtype": "string"}, {"name": "dataset", "dtype": "string"}, {"name": "datasplit", "dtype": "string"}, {"name": "prompt_format", "dtype": "string"}], "splits": [{"name": "preference", "num_bytes": 4341413, "num_examples": 1947}], "download_size": 1952204, "dataset_size": 4341413}, {"config_name": "pythia-1.4b_alpaca_farm_instructions_sft_sep-checkpoint-9500", "features": [{"name": "instruction", "dtype": "string"}, {"name": "input", "dtype": "string"}, {"name": "output_1", "dtype": "string"}, {"name": "output_2", "dtype": "string"}, {"name": "preference", "dtype": "int64"}, {"name": "output", "dtype": "string"}, {"name": "generator", "dtype": "string"}, {"name": "sample_mode", "dtype": "string"}, {"name": "dataset", "dtype": "string"}, {"name": "datasplit", "dtype": "string"}, {"name": "prompt_format", "dtype": "string"}], "splits": [{"name": "preference", "num_bytes": 4405117, "num_examples": 1947}], "download_size": 1991014, "dataset_size": 4405117}, {"config_name": "pythia-1.4b_alpaca_farm_instructions_sft_sep_spe", "features": [{"name": "instruction", "dtype": "string"}, {"name": "input", "dtype": "string"}, {"name": "output_1", "dtype": "string"}, {"name": "output_2", "dtype": "string"}, {"name": "preference", "dtype": "int64"}, {"name": "output", "dtype": "string"}, {"name": "generator", "dtype": "string"}, {"name": "sample_mode", "dtype": "string"}, {"name": "dataset", "dtype": "string"}, {"name": "datasplit", "dtype": "string"}, {"name": "prompt_format", "dtype": "string"}], "splits": [{"name": "preference", "num_bytes": 4383538, "num_examples": 1947}], "download_size": 1991323, "dataset_size": 4383538}, {"config_name": "pythia-1.4b_alpaca_farm_instructions_sft_sep_spe-checkpoint-1000", "features": [{"name": "instruction", "dtype": "string"}, {"name": "input", "dtype": "string"}, {"name": "output_1", "dtype": "string"}, {"name": "output_2", "dtype": "string"}, {"name": "preference", "dtype": "int64"}, {"name": "output", "dtype": "string"}, {"name": "generator", "dtype": "string"}, {"name": "sample_mode", "dtype": "string"}, {"name": "dataset", "dtype": "string"}, {"name": "datasplit", "dtype": "string"}, {"name": "prompt_format", "dtype": "string"}], "splits": [{"name": "preference", "num_bytes": 4492296, "num_examples": 1947}], "download_size": 2128887, "dataset_size": 4492296}, {"config_name": "pythia-1.4b_alpaca_farm_instructions_sft_sep_spe-checkpoint-10000", "features": [{"name": "instruction", "dtype": "string"}, {"name": "input", "dtype": "string"}, {"name": "output_1", "dtype": "string"}, {"name": "output_2", "dtype": "string"}, {"name": "preference", "dtype": "int64"}, {"name": "output", "dtype": "string"}, {"name": "generator", "dtype": "string"}, {"name": "sample_mode", "dtype": "string"}, {"name": "dataset", "dtype": "string"}, {"name": "datasplit", "dtype": "string"}, {"name": "prompt_format", "dtype": "string"}], "splits": [{"name": "preference", "num_bytes": 4450582, "num_examples": 1947}], "download_size": 2055603, "dataset_size": 4450582}, {"config_name": "pythia-1.4b_alpaca_farm_instructions_sft_sep_spe-checkpoint-10500", "features": [{"name": "instruction", "dtype": "string"}, {"name": "input", "dtype": "string"}, {"name": "output_1", "dtype": "string"}, {"name": "output_2", "dtype": "string"}, {"name": "preference", "dtype": "int64"}, {"name": "output", "dtype": "string"}, {"name": "generator", "dtype": "string"}, {"name": "sample_mode", "dtype": "string"}, {"name": "dataset", "dtype": "string"}, {"name": "datasplit", "dtype": "string"}, {"name": "prompt_format", "dtype": "string"}], "splits": [{"name": "preference", "num_bytes": 4404371, "num_examples": 1947}], "download_size": 2016887, "dataset_size": 4404371}, {"config_name": "pythia-1.4b_alpaca_farm_instructions_sft_sep_spe-checkpoint-11000", "features": [{"name": "instruction", "dtype": "string"}, {"name": "input", "dtype": "string"}, {"name": "output_1", "dtype": "string"}, {"name": "output_2", "dtype": "string"}, {"name": "preference", "dtype": "int64"}, {"name": "output", "dtype": "string"}, {"name": "generator", "dtype": "string"}, {"name": "sample_mode", "dtype": "string"}, {"name": "dataset", "dtype": "string"}, {"name": "datasplit", "dtype": "string"}, {"name": "prompt_format", "dtype": "string"}], "splits": [{"name": "preference", "num_bytes": 4357716, "num_examples": 1947}], "download_size": 1953433, "dataset_size": 4357716}, {"config_name": "pythia-1.4b_alpaca_farm_instructions_sft_sep_spe-checkpoint-11500", "features": [{"name": "instruction", "dtype": "string"}, {"name": "input", "dtype": "string"}, {"name": "output_1", "dtype": "string"}, {"name": "output_2", "dtype": "string"}, {"name": "preference", "dtype": "int64"}, {"name": "output", "dtype": "string"}, {"name": "generator", "dtype": "string"}, {"name": "sample_mode", "dtype": "string"}, {"name": "dataset", "dtype": "string"}, {"name": "datasplit", "dtype": "string"}, {"name": "prompt_format", "dtype": "string"}], "splits": [{"name": "preference", "num_bytes": 4279734, "num_examples": 1947}], "download_size": 1896380, "dataset_size": 4279734}, {"config_name": "pythia-1.4b_alpaca_farm_instructions_sft_sep_spe-checkpoint-12000", "features": [{"name": "instruction", "dtype": "string"}, {"name": "input", "dtype": "string"}, {"name": "output_1", "dtype": "string"}, {"name": "output_2", "dtype": "string"}, {"name": "preference", "dtype": "int64"}, {"name": "output", "dtype": "string"}, {"name": "generator", "dtype": "string"}, {"name": "sample_mode", "dtype": "string"}, {"name": "dataset", "dtype": "string"}, {"name": "datasplit", "dtype": "string"}, {"name": "prompt_format", "dtype": "string"}], "splits": [{"name": "preference", "num_bytes": 4374793, "num_examples": 1947}], "download_size": 1980386, "dataset_size": 4374793}, {"config_name": "pythia-1.4b_alpaca_farm_instructions_sft_sep_spe-checkpoint-12500", "features": [{"name": "instruction", "dtype": "string"}, {"name": "input", "dtype": "string"}, {"name": "output_1", "dtype": "string"}, {"name": "output_2", "dtype": "string"}, {"name": "preference", "dtype": "int64"}, {"name": "output", "dtype": "string"}, {"name": "generator", "dtype": "string"}, {"name": "sample_mode", "dtype": "string"}, {"name": "dataset", "dtype": "string"}, {"name": "datasplit", "dtype": "string"}, {"name": "prompt_format", "dtype": "string"}], "splits": [{"name": "preference", "num_bytes": 4402312, "num_examples": 1947}], "download_size": 1987870, "dataset_size": 4402312}, {"config_name": "pythia-1.4b_alpaca_farm_instructions_sft_sep_spe-checkpoint-1500", "features": [{"name": "instruction", "dtype": "string"}, {"name": "input", "dtype": "string"}, {"name": "output_1", "dtype": "string"}, {"name": "output_2", "dtype": "string"}, {"name": "preference", "dtype": "int64"}, {"name": "output", "dtype": "string"}, {"name": "generator", "dtype": "string"}, {"name": "sample_mode", "dtype": "string"}, {"name": "dataset", "dtype": "string"}, {"name": "datasplit", "dtype": "string"}, {"name": "prompt_format", "dtype": "string"}], "splits": [{"name": "preference", "num_bytes": 4431948, "num_examples": 1947}], "download_size": 1982711, "dataset_size": 4431948}, {"config_name": "pythia-1.4b_alpaca_farm_instructions_sft_sep_spe-checkpoint-2000", "features": [{"name": "instruction", "dtype": "string"}, {"name": "input", "dtype": "string"}, {"name": "output_1", "dtype": "string"}, {"name": "output_2", "dtype": "string"}, {"name": "preference", "dtype": "int64"}, {"name": "output", "dtype": "string"}, {"name": "generator", "dtype": "string"}, {"name": "sample_mode", "dtype": "string"}, {"name": "dataset", "dtype": "string"}, {"name": "datasplit", "dtype": "string"}, {"name": "prompt_format", "dtype": "string"}], "splits": [{"name": "preference", "num_bytes": 4225150, "num_examples": 1947}], "download_size": 1873927, "dataset_size": 4225150}, {"config_name": "pythia-1.4b_alpaca_farm_instructions_sft_sep_spe-checkpoint-2500", "features": [{"name": "instruction", "dtype": "string"}, {"name": "input", "dtype": "string"}, {"name": "output_1", "dtype": "string"}, {"name": "output_2", "dtype": "string"}, {"name": "preference", "dtype": "int64"}, {"name": "output", "dtype": "string"}, {"name": "generator", "dtype": "string"}, {"name": "sample_mode", "dtype": "string"}, {"name": "dataset", "dtype": "string"}, {"name": "datasplit", "dtype": "string"}, {"name": "prompt_format", "dtype": "string"}], "splits": [{"name": "preference", "num_bytes": 4169327, "num_examples": 1947}], "download_size": 1842090, "dataset_size": 4169327}, {"config_name": "pythia-1.4b_alpaca_farm_instructions_sft_sep_spe-checkpoint-3000", "features": [{"name": "instruction", "dtype": "string"}, {"name": "input", "dtype": "string"}, {"name": "output_1", "dtype": "string"}, {"name": "output_2", "dtype": "string"}, {"name": "preference", "dtype": "int64"}, {"name": "output", "dtype": "string"}, {"name": "generator", "dtype": "string"}, {"name": "sample_mode", "dtype": "string"}, {"name": "dataset", "dtype": "string"}, {"name": "datasplit", "dtype": "string"}, {"name": "prompt_format", "dtype": "string"}], "splits": [{"name": "preference", "num_bytes": 4393337, "num_examples": 1947}], "download_size": 1937361, "dataset_size": 4393337}, {"config_name": "pythia-1.4b_alpaca_farm_instructions_sft_sep_spe-checkpoint-3500", "features": [{"name": "instruction", "dtype": "string"}, {"name": "input", "dtype": "string"}, {"name": "output_1", "dtype": "string"}, {"name": "output_2", "dtype": "string"}, {"name": "preference", "dtype": "int64"}, {"name": "output", "dtype": "string"}, {"name": "generator", "dtype": "string"}, {"name": "sample_mode", "dtype": "string"}, {"name": "dataset", "dtype": "string"}, {"name": "datasplit", "dtype": "string"}, {"name": "prompt_format", "dtype": "string"}], "splits": [{"name": "preference", "num_bytes": 4424276, "num_examples": 1947}], "download_size": 2041134, "dataset_size": 4424276}, {"config_name": "pythia-1.4b_alpaca_farm_instructions_sft_sep_spe-checkpoint-4000", "features": [{"name": "instruction", "dtype": "string"}, {"name": "input", "dtype": "string"}, {"name": "output_1", "dtype": "string"}, {"name": "output_2", "dtype": "string"}, {"name": "preference", "dtype": "int64"}, {"name": "output", "dtype": "string"}, {"name": "generator", "dtype": "string"}, {"name": "sample_mode", "dtype": "string"}, {"name": "dataset", "dtype": "string"}, {"name": "datasplit", "dtype": "string"}, {"name": "prompt_format", "dtype": "string"}], "splits": [{"name": "preference", "num_bytes": 4400846, "num_examples": 1947}], "download_size": 2032521, "dataset_size": 4400846}, {"config_name": "pythia-1.4b_alpaca_farm_instructions_sft_sep_spe-checkpoint-4500", "features": [{"name": "instruction", "dtype": "string"}, {"name": "input", "dtype": "string"}, {"name": "output_1", "dtype": "string"}, {"name": "output_2", "dtype": "string"}, {"name": "preference", "dtype": "int64"}, {"name": "output", "dtype": "string"}, {"name": "generator", "dtype": "string"}, {"name": "sample_mode", "dtype": "string"}, {"name": "dataset", "dtype": "string"}, {"name": "datasplit", "dtype": "string"}, {"name": "prompt_format", "dtype": "string"}], "splits": [{"name": "preference", "num_bytes": 4240722, "num_examples": 1947}], "download_size": 1859819, "dataset_size": 4240722}, {"config_name": "pythia-1.4b_alpaca_farm_instructions_sft_sep_spe-checkpoint-500", "features": [{"name": "instruction", "dtype": "string"}, {"name": "input", "dtype": "string"}, {"name": "output_1", "dtype": "string"}, {"name": "output_2", "dtype": "string"}, {"name": "preference", "dtype": "int64"}, {"name": "output", "dtype": "string"}, {"name": "generator", "dtype": "string"}, {"name": "sample_mode", "dtype": "string"}, {"name": "dataset", "dtype": "string"}, {"name": "datasplit", "dtype": "string"}, {"name": "prompt_format", "dtype": "string"}], "splits": [{"name": "preference", "num_bytes": 4220416, "num_examples": 1947}], "download_size": 1954092, "dataset_size": 4220416}, {"config_name": "pythia-1.4b_alpaca_farm_instructions_sft_sep_spe-checkpoint-5000", "features": [{"name": "instruction", "dtype": "string"}, {"name": "input", "dtype": "string"}, {"name": "output_1", "dtype": "string"}, {"name": "output_2", "dtype": "string"}, {"name": "preference", "dtype": "int64"}, {"name": "output", "dtype": "string"}, {"name": "generator", "dtype": "string"}, {"name": "sample_mode", "dtype": "string"}, {"name": "dataset", "dtype": "string"}, {"name": "datasplit", "dtype": "string"}, {"name": "prompt_format", "dtype": "string"}], "splits": [{"name": "preference", "num_bytes": 4374666, "num_examples": 1947}], "download_size": 2025320, "dataset_size": 4374666}, {"config_name": "pythia-1.4b_alpaca_farm_instructions_sft_sep_spe-checkpoint-5500", "features": [{"name": "instruction", "dtype": "string"}, {"name": "input", "dtype": "string"}, {"name": "output_1", "dtype": "string"}, {"name": "output_2", "dtype": "string"}, {"name": "preference", "dtype": "int64"}, {"name": "output", "dtype": "string"}, {"name": "generator", "dtype": "string"}, {"name": "sample_mode", "dtype": "string"}, {"name": "dataset", "dtype": "string"}, {"name": "datasplit", "dtype": "string"}, {"name": "prompt_format", "dtype": "string"}], "splits": [{"name": "preference", "num_bytes": 4452411, "num_examples": 1947}], "download_size": 2049717, "dataset_size": 4452411}, {"config_name": "pythia-1.4b_alpaca_farm_instructions_sft_sep_spe-checkpoint-6000", "features": [{"name": "instruction", "dtype": "string"}, {"name": "input", "dtype": "string"}, {"name": "output_1", "dtype": "string"}, {"name": "output_2", "dtype": "string"}, {"name": "preference", "dtype": "int64"}, {"name": "output", "dtype": "string"}, {"name": "generator", "dtype": "string"}, {"name": "sample_mode", "dtype": "string"}, {"name": "dataset", "dtype": "string"}, {"name": "datasplit", "dtype": "string"}, {"name": "prompt_format", "dtype": "string"}], "splits": [{"name": "preference", "num_bytes": 4289284, "num_examples": 1947}], "download_size": 1931491, "dataset_size": 4289284}, {"config_name": "pythia-1.4b_alpaca_farm_instructions_sft_sep_spe-checkpoint-6500", "features": [{"name": "instruction", "dtype": "string"}, {"name": "input", "dtype": "string"}, {"name": "output_1", "dtype": "string"}, {"name": "output_2", "dtype": "string"}, {"name": "preference", "dtype": "int64"}, {"name": "output", "dtype": "string"}, {"name": "generator", "dtype": "string"}, {"name": "sample_mode", "dtype": "string"}, {"name": "dataset", "dtype": "string"}, {"name": "datasplit", "dtype": "string"}, {"name": "prompt_format", "dtype": "string"}], "splits": [{"name": "preference", "num_bytes": 4437044, "num_examples": 1947}], "download_size": 2008991, "dataset_size": 4437044}, {"config_name": "pythia-1.4b_alpaca_farm_instructions_sft_sep_spe-checkpoint-7000", "features": [{"name": "instruction", "dtype": "string"}, {"name": "input", "dtype": "string"}, {"name": "output_1", "dtype": "string"}, {"name": "output_2", "dtype": "string"}, {"name": "preference", "dtype": "int64"}, {"name": "output", "dtype": "string"}, {"name": "generator", "dtype": "string"}, {"name": "sample_mode", "dtype": "string"}, {"name": "dataset", "dtype": "string"}, {"name": "datasplit", "dtype": "string"}, {"name": "prompt_format", "dtype": "string"}], "splits": [{"name": "preference", "num_bytes": 4359253, "num_examples": 1947}], "download_size": 1948993, "dataset_size": 4359253}, {"config_name": "pythia-1.4b_alpaca_farm_instructions_sft_sep_spe-checkpoint-7500", "features": [{"name": "instruction", "dtype": "string"}, {"name": "input", "dtype": "string"}, {"name": "output_1", "dtype": "string"}, {"name": "output_2", "dtype": "string"}, {"name": "preference", "dtype": "int64"}, {"name": "output", "dtype": "string"}, {"name": "generator", "dtype": "string"}, {"name": "sample_mode", "dtype": "string"}, {"name": "dataset", "dtype": "string"}, {"name": "datasplit", "dtype": "string"}, {"name": "prompt_format", "dtype": "string"}], "splits": [{"name": "preference", "num_bytes": 4355605, "num_examples": 1947}], "download_size": 1973019, "dataset_size": 4355605}, {"config_name": "pythia-1.4b_alpaca_farm_instructions_sft_sep_spe-checkpoint-8000", "features": [{"name": "instruction", "dtype": "string"}, {"name": "input", "dtype": "string"}, {"name": "output_1", "dtype": "string"}, {"name": "output_2", "dtype": "string"}, {"name": "preference", "dtype": "int64"}, {"name": "output", "dtype": "string"}, {"name": "generator", "dtype": "string"}, {"name": "sample_mode", "dtype": "string"}, {"name": "dataset", "dtype": "string"}, {"name": "datasplit", "dtype": "string"}, {"name": "prompt_format", "dtype": "string"}], "splits": [{"name": "preference", "num_bytes": 4379255, "num_examples": 1947}], "download_size": 2020435, "dataset_size": 4379255}, {"config_name": "pythia-1.4b_alpaca_farm_instructions_sft_sep_spe-checkpoint-8500", "features": [{"name": "instruction", "dtype": "string"}, {"name": "input", "dtype": "string"}, {"name": "output_1", "dtype": "string"}, {"name": "output_2", "dtype": "string"}, {"name": "preference", "dtype": "int64"}, {"name": "output", "dtype": "string"}, {"name": "generator", "dtype": "string"}, {"name": "sample_mode", "dtype": "string"}, {"name": "dataset", "dtype": "string"}, {"name": "datasplit", "dtype": "string"}, {"name": "prompt_format", "dtype": "string"}], "splits": [{"name": "preference", "num_bytes": 4394848, "num_examples": 1947}], "download_size": 1991396, "dataset_size": 4394848}, {"config_name": "pythia-1.4b_alpaca_farm_instructions_sft_sep_spe-checkpoint-9000", "features": [{"name": "instruction", "dtype": "string"}, {"name": "input", "dtype": "string"}, {"name": "output_1", "dtype": "string"}, {"name": "output_2", "dtype": "string"}, {"name": "preference", "dtype": "int64"}, {"name": "output", "dtype": "string"}, {"name": "generator", "dtype": "string"}, {"name": "sample_mode", "dtype": "string"}, {"name": "dataset", "dtype": "string"}, {"name": "datasplit", "dtype": "string"}, {"name": "prompt_format", "dtype": "string"}], "splits": [{"name": "preference", "num_bytes": 4387580, "num_examples": 1947}], "download_size": 2017722, "dataset_size": 4387580}, {"config_name": "pythia-1.4b_alpaca_farm_instructions_sft_sep_spe-checkpoint-9500", "features": [{"name": "instruction", "dtype": "string"}, {"name": "input", "dtype": "string"}, {"name": "output_1", "dtype": "string"}, {"name": "output_2", "dtype": "string"}, {"name": "preference", "dtype": "int64"}, {"name": "output", "dtype": "string"}, {"name": "generator", "dtype": "string"}, {"name": "sample_mode", "dtype": "string"}, {"name": "dataset", "dtype": "string"}, {"name": "datasplit", "dtype": "string"}, {"name": "prompt_format", "dtype": "string"}], "splits": [{"name": "preference", "num_bytes": 4412057, "num_examples": 1947}], "download_size": 1978508, "dataset_size": 4412057}, {"config_name": "starcoderbase-1b-sft", "features": [{"name": "instruction", "dtype": "string"}, {"name": "input", "dtype": "string"}, {"name": "output_1", "dtype": "string"}, {"name": "output_2", "dtype": "string"}, {"name": "preference", "dtype": "int64"}, {"name": "output", "dtype": "string"}, {"name": "generator", "dtype": "string"}, {"name": "sample_mode", "dtype": "string"}, {"name": "dataset", "dtype": "string"}, {"name": "datasplit", "dtype": "string"}, {"name": "prompt_format", "dtype": "string"}], "splits": [{"name": "preference", "num_bytes": 3443792, "num_examples": 1947}], "download_size": 1658856, "dataset_size": 3443792}], "configs": [{"config_name": "42dot_LLM-SFT-1.3B", "data_files": [{"split": "preference", "path": "42dot_LLM-SFT-1.3B/preference-*"}]}, {"config_name": "opt-1.3b", "data_files": [{"split": "preference", "path": "opt-1.3b/preference-*"}]}, {"config_name": "opt-1.3b_alpaca_farm_instructions_sft_constant_pa-checkpoint-10000", "data_files": [{"split": "preference", "path": "opt-1.3b_alpaca_farm_instructions_sft_constant_pa-checkpoint-10000/preference-*"}]}, {"config_name": "opt-1.3b_alpaca_farm_instructions_sft_constant_pa-checkpoint-12500", "data_files": [{"split": "preference", "path": "opt-1.3b_alpaca_farm_instructions_sft_constant_pa-checkpoint-12500/preference-*"}]}, {"config_name": "opt-1.3b_alpaca_farm_instructions_sft_constant_pa-checkpoint-15000", "data_files": [{"split": "preference", "path": "opt-1.3b_alpaca_farm_instructions_sft_constant_pa-checkpoint-15000/preference-*"}]}, {"config_name": "opt-1.3b_alpaca_farm_instructions_sft_constant_pa-checkpoint-17500", "data_files": [{"split": "preference", "path": "opt-1.3b_alpaca_farm_instructions_sft_constant_pa-checkpoint-17500/preference-*"}]}, {"config_name": "opt-1.3b_alpaca_farm_instructions_sft_constant_pa-checkpoint-20000", "data_files": [{"split": "preference", "path": "opt-1.3b_alpaca_farm_instructions_sft_constant_pa-checkpoint-20000/preference-*"}]}, {"config_name": "opt-1.3b_alpaca_farm_instructions_sft_constant_pa-checkpoint-2500", "data_files": [{"split": "preference", "path": "opt-1.3b_alpaca_farm_instructions_sft_constant_pa-checkpoint-2500/preference-*"}]}, {"config_name": "opt-1.3b_alpaca_farm_instructions_sft_constant_pa-checkpoint-25000", "data_files": [{"split": "preference", "path": "opt-1.3b_alpaca_farm_instructions_sft_constant_pa-checkpoint-25000/preference-*"}]}, {"config_name": "opt-1.3b_alpaca_farm_instructions_sft_constant_pa-checkpoint-5000", "data_files": [{"split": "preference", "path": "opt-1.3b_alpaca_farm_instructions_sft_constant_pa-checkpoint-5000/preference-*"}]}, {"config_name": "opt-1.3b_alpaca_farm_instructions_sft_constant_pa-checkpoint-7500", "data_files": [{"split": "preference", "path": "opt-1.3b_alpaca_farm_instructions_sft_constant_pa-checkpoint-7500/preference-*"}]}, {"config_name": "pythia-1.4b", "data_files": [{"split": "preference", "path": "pythia-1.4b/preference-*"}]}, {"config_name": "pythia-1.4b_alpaca_farm_instructions_sft_constant_pa-checkpoint-10000", "data_files": [{"split": "preference", "path": "pythia-1.4b_alpaca_farm_instructions_sft_constant_pa-checkpoint-10000/preference-*"}]}, {"config_name": "pythia-1.4b_alpaca_farm_instructions_sft_constant_pa-checkpoint-12500", "data_files": [{"split": "preference", "path": "pythia-1.4b_alpaca_farm_instructions_sft_constant_pa-checkpoint-12500/preference-*"}]}, {"config_name": "pythia-1.4b_alpaca_farm_instructions_sft_constant_pa-checkpoint-15000", "data_files": [{"split": "preference", "path": "pythia-1.4b_alpaca_farm_instructions_sft_constant_pa-checkpoint-15000/preference-*"}]}, {"config_name": "pythia-1.4b_alpaca_farm_instructions_sft_constant_pa-checkpoint-17500", "data_files": [{"split": "preference", "path": "pythia-1.4b_alpaca_farm_instructions_sft_constant_pa-checkpoint-17500/preference-*"}]}, {"config_name": "pythia-1.4b_alpaca_farm_instructions_sft_constant_pa-checkpoint-20000", "data_files": [{"split": "preference", "path": "pythia-1.4b_alpaca_farm_instructions_sft_constant_pa-checkpoint-20000/preference-*"}]}, {"config_name": "pythia-1.4b_alpaca_farm_instructions_sft_constant_pa-checkpoint-22500", "data_files": [{"split": "preference", "path": "pythia-1.4b_alpaca_farm_instructions_sft_constant_pa-checkpoint-22500/preference-*"}]}, {"config_name": "pythia-1.4b_alpaca_farm_instructions_sft_constant_pa-checkpoint-2500", "data_files": [{"split": "preference", "path": "pythia-1.4b_alpaca_farm_instructions_sft_constant_pa-checkpoint-2500/preference-*"}]}, {"config_name": "pythia-1.4b_alpaca_farm_instructions_sft_constant_pa-checkpoint-25000", "data_files": [{"split": "preference", "path": "pythia-1.4b_alpaca_farm_instructions_sft_constant_pa-checkpoint-25000/preference-*"}]}, {"config_name": "pythia-1.4b_alpaca_farm_instructions_sft_constant_pa-checkpoint-5000", "data_files": [{"split": "preference", "path": "pythia-1.4b_alpaca_farm_instructions_sft_constant_pa-checkpoint-5000/preference-*"}]}, {"config_name": "pythia-1.4b_alpaca_farm_instructions_sft_constant_pa-checkpoint-7500", "data_files": [{"split": "preference", "path": "pythia-1.4b_alpaca_farm_instructions_sft_constant_pa-checkpoint-7500/preference-*"}]}, {"config_name": "pythia-1.4b_alpaca_farm_instructions_sft_constant_pac", "data_files": [{"split": "preference", "path": "pythia-1.4b_alpaca_farm_instructions_sft_constant_pac/preference-*"}]}, {"config_name": "pythia-1.4b_alpaca_farm_instructions_sft_constant_pac-checkpoint-1000", "data_files": [{"split": "preference", "path": "pythia-1.4b_alpaca_farm_instructions_sft_constant_pac-checkpoint-1000/preference-*"}]}, {"config_name": "pythia-1.4b_alpaca_farm_instructions_sft_constant_pac-checkpoint-10000", "data_files": [{"split": "preference", "path": "pythia-1.4b_alpaca_farm_instructions_sft_constant_pac-checkpoint-10000/preference-*"}]}, {"config_name": "pythia-1.4b_alpaca_farm_instructions_sft_constant_pac-checkpoint-10500", "data_files": [{"split": "preference", "path": "pythia-1.4b_alpaca_farm_instructions_sft_constant_pac-checkpoint-10500/preference-*"}]}, {"config_name": "pythia-1.4b_alpaca_farm_instructions_sft_constant_pac-checkpoint-11000", "data_files": [{"split": "preference", "path": "pythia-1.4b_alpaca_farm_instructions_sft_constant_pac-checkpoint-11000/preference-*"}]}, {"config_name": "pythia-1.4b_alpaca_farm_instructions_sft_constant_pac-checkpoint-11500", "data_files": [{"split": "preference", "path": "pythia-1.4b_alpaca_farm_instructions_sft_constant_pac-checkpoint-11500/preference-*"}]}, {"config_name": "pythia-1.4b_alpaca_farm_instructions_sft_constant_pac-checkpoint-12000", "data_files": [{"split": "preference", "path": "pythia-1.4b_alpaca_farm_instructions_sft_constant_pac-checkpoint-12000/preference-*"}]}, {"config_name": "pythia-1.4b_alpaca_farm_instructions_sft_constant_pac-checkpoint-12500", "data_files": [{"split": "preference", "path": "pythia-1.4b_alpaca_farm_instructions_sft_constant_pac-checkpoint-12500/preference-*"}]}, {"config_name": "pythia-1.4b_alpaca_farm_instructions_sft_constant_pac-checkpoint-1500", "data_files": [{"split": "preference", "path": "pythia-1.4b_alpaca_farm_instructions_sft_constant_pac-checkpoint-1500/preference-*"}]}, {"config_name": "pythia-1.4b_alpaca_farm_instructions_sft_constant_pac-checkpoint-2000", "data_files": [{"split": "preference", "path": "pythia-1.4b_alpaca_farm_instructions_sft_constant_pac-checkpoint-2000/preference-*"}]}, {"config_name": "pythia-1.4b_alpaca_farm_instructions_sft_constant_pac-checkpoint-2500", "data_files": [{"split": "preference", "path": "pythia-1.4b_alpaca_farm_instructions_sft_constant_pac-checkpoint-2500/preference-*"}]}, {"config_name": "pythia-1.4b_alpaca_farm_instructions_sft_constant_pac-checkpoint-3000", "data_files": [{"split": "preference", "path": "pythia-1.4b_alpaca_farm_instructions_sft_constant_pac-checkpoint-3000/preference-*"}]}, {"config_name": "pythia-1.4b_alpaca_farm_instructions_sft_constant_pac-checkpoint-3500", "data_files": [{"split": "preference", "path": "pythia-1.4b_alpaca_farm_instructions_sft_constant_pac-checkpoint-3500/preference-*"}]}, {"config_name": "pythia-1.4b_alpaca_farm_instructions_sft_constant_pac-checkpoint-4000", "data_files": [{"split": "preference", "path": "pythia-1.4b_alpaca_farm_instructions_sft_constant_pac-checkpoint-4000/preference-*"}]}, {"config_name": "pythia-1.4b_alpaca_farm_instructions_sft_constant_pac-checkpoint-4500", "data_files": [{"split": "preference", "path": "pythia-1.4b_alpaca_farm_instructions_sft_constant_pac-checkpoint-4500/preference-*"}]}, {"config_name": "pythia-1.4b_alpaca_farm_instructions_sft_constant_pac-checkpoint-500", "data_files": [{"split": "preference", "path": "pythia-1.4b_alpaca_farm_instructions_sft_constant_pac-checkpoint-500/preference-*"}]}, {"config_name": "pythia-1.4b_alpaca_farm_instructions_sft_constant_pac-checkpoint-5000", "data_files": [{"split": "preference", "path": "pythia-1.4b_alpaca_farm_instructions_sft_constant_pac-checkpoint-5000/preference-*"}]}, {"config_name": "pythia-1.4b_alpaca_farm_instructions_sft_constant_pac-checkpoint-5500", "data_files": [{"split": "preference", "path": "pythia-1.4b_alpaca_farm_instructions_sft_constant_pac-checkpoint-5500/preference-*"}]}, {"config_name": "pythia-1.4b_alpaca_farm_instructions_sft_constant_pac-checkpoint-6000", "data_files": [{"split": "preference", "path": "pythia-1.4b_alpaca_farm_instructions_sft_constant_pac-checkpoint-6000/preference-*"}]}, {"config_name": "pythia-1.4b_alpaca_farm_instructions_sft_constant_pac-checkpoint-6500", "data_files": [{"split": "preference", "path": "pythia-1.4b_alpaca_farm_instructions_sft_constant_pac-checkpoint-6500/preference-*"}]}, {"config_name": "pythia-1.4b_alpaca_farm_instructions_sft_constant_pac-checkpoint-7000", "data_files": [{"split": "preference", "path": "pythia-1.4b_alpaca_farm_instructions_sft_constant_pac-checkpoint-7000/preference-*"}]}, {"config_name": "pythia-1.4b_alpaca_farm_instructions_sft_constant_pac-checkpoint-7500", "data_files": [{"split": "preference", "path": "pythia-1.4b_alpaca_farm_instructions_sft_constant_pac-checkpoint-7500/preference-*"}]}, {"config_name": "pythia-1.4b_alpaca_farm_instructions_sft_constant_pac-checkpoint-8000", "data_files": [{"split": "preference", "path": "pythia-1.4b_alpaca_farm_instructions_sft_constant_pac-checkpoint-8000/preference-*"}]}, {"config_name": "pythia-1.4b_alpaca_farm_instructions_sft_constant_pac-checkpoint-8500", "data_files": [{"split": "preference", "path": "pythia-1.4b_alpaca_farm_instructions_sft_constant_pac-checkpoint-8500/preference-*"}]}, {"config_name": "pythia-1.4b_alpaca_farm_instructions_sft_constant_pac-checkpoint-9000", "data_files": [{"split": "preference", "path": "pythia-1.4b_alpaca_farm_instructions_sft_constant_pac-checkpoint-9000/preference-*"}]}, {"config_name": "pythia-1.4b_alpaca_farm_instructions_sft_constant_pac-checkpoint-9500", "data_files": [{"split": "preference", "path": "pythia-1.4b_alpaca_farm_instructions_sft_constant_pac-checkpoint-9500/preference-*"}]}, {"config_name": "pythia-1.4b_alpaca_farm_instructions_sft_sep", "data_files": [{"split": "preference", "path": "pythia-1.4b_alpaca_farm_instructions_sft_sep/preference-*"}]}, {"config_name": "pythia-1.4b_alpaca_farm_instructions_sft_sep-checkpoint-1000", "data_files": [{"split": "preference", "path": "pythia-1.4b_alpaca_farm_instructions_sft_sep-checkpoint-1000/preference-*"}]}, {"config_name": "pythia-1.4b_alpaca_farm_instructions_sft_sep-checkpoint-10000", "data_files": [{"split": "preference", "path": "pythia-1.4b_alpaca_farm_instructions_sft_sep-checkpoint-10000/preference-*"}]}, {"config_name": "pythia-1.4b_alpaca_farm_instructions_sft_sep-checkpoint-10500", "data_files": [{"split": "preference", "path": "pythia-1.4b_alpaca_farm_instructions_sft_sep-checkpoint-10500/preference-*"}]}, {"config_name": "pythia-1.4b_alpaca_farm_instructions_sft_sep-checkpoint-11000", "data_files": [{"split": "preference", "path": "pythia-1.4b_alpaca_farm_instructions_sft_sep-checkpoint-11000/preference-*"}]}, {"config_name": "pythia-1.4b_alpaca_farm_instructions_sft_sep-checkpoint-11500", "data_files": [{"split": "preference", "path": "pythia-1.4b_alpaca_farm_instructions_sft_sep-checkpoint-11500/preference-*"}]}, {"config_name": "pythia-1.4b_alpaca_farm_instructions_sft_sep-checkpoint-12000", "data_files": [{"split": "preference", "path": "pythia-1.4b_alpaca_farm_instructions_sft_sep-checkpoint-12000/preference-*"}]}, {"config_name": "pythia-1.4b_alpaca_farm_instructions_sft_sep-checkpoint-12500", "data_files": [{"split": "preference", "path": "pythia-1.4b_alpaca_farm_instructions_sft_sep-checkpoint-12500/preference-*"}]}, {"config_name": "pythia-1.4b_alpaca_farm_instructions_sft_sep-checkpoint-1500", "data_files": [{"split": "preference", "path": "pythia-1.4b_alpaca_farm_instructions_sft_sep-checkpoint-1500/preference-*"}]}, {"config_name": "pythia-1.4b_alpaca_farm_instructions_sft_sep-checkpoint-2000", "data_files": [{"split": "preference", "path": "pythia-1.4b_alpaca_farm_instructions_sft_sep-checkpoint-2000/preference-*"}]}, {"config_name": "pythia-1.4b_alpaca_farm_instructions_sft_sep-checkpoint-2500", "data_files": [{"split": "preference", "path": "pythia-1.4b_alpaca_farm_instructions_sft_sep-checkpoint-2500/preference-*"}]}, {"config_name": "pythia-1.4b_alpaca_farm_instructions_sft_sep-checkpoint-3000", "data_files": [{"split": "preference", "path": "pythia-1.4b_alpaca_farm_instructions_sft_sep-checkpoint-3000/preference-*"}]}, {"config_name": "pythia-1.4b_alpaca_farm_instructions_sft_sep-checkpoint-3500", "data_files": [{"split": "preference", "path": "pythia-1.4b_alpaca_farm_instructions_sft_sep-checkpoint-3500/preference-*"}]}, {"config_name": "pythia-1.4b_alpaca_farm_instructions_sft_sep-checkpoint-4000", "data_files": [{"split": "preference", "path": "pythia-1.4b_alpaca_farm_instructions_sft_sep-checkpoint-4000/preference-*"}]}, {"config_name": "pythia-1.4b_alpaca_farm_instructions_sft_sep-checkpoint-4500", "data_files": [{"split": "preference", "path": "pythia-1.4b_alpaca_farm_instructions_sft_sep-checkpoint-4500/preference-*"}]}, {"config_name": "pythia-1.4b_alpaca_farm_instructions_sft_sep-checkpoint-500", "data_files": [{"split": "preference", "path": "pythia-1.4b_alpaca_farm_instructions_sft_sep-checkpoint-500/preference-*"}]}, {"config_name": "pythia-1.4b_alpaca_farm_instructions_sft_sep-checkpoint-5000", "data_files": [{"split": "preference", "path": "pythia-1.4b_alpaca_farm_instructions_sft_sep-checkpoint-5000/preference-*"}]}, {"config_name": "pythia-1.4b_alpaca_farm_instructions_sft_sep-checkpoint-5500", "data_files": [{"split": "preference", "path": "pythia-1.4b_alpaca_farm_instructions_sft_sep-checkpoint-5500/preference-*"}]}, {"config_name": "pythia-1.4b_alpaca_farm_instructions_sft_sep-checkpoint-6000", "data_files": [{"split": "preference", "path": "pythia-1.4b_alpaca_farm_instructions_sft_sep-checkpoint-6000/preference-*"}]}, {"config_name": "pythia-1.4b_alpaca_farm_instructions_sft_sep-checkpoint-6500", "data_files": [{"split": "preference", "path": "pythia-1.4b_alpaca_farm_instructions_sft_sep-checkpoint-6500/preference-*"}]}, {"config_name": "pythia-1.4b_alpaca_farm_instructions_sft_sep-checkpoint-7000", "data_files": [{"split": "preference", "path": "pythia-1.4b_alpaca_farm_instructions_sft_sep-checkpoint-7000/preference-*"}]}, {"config_name": "pythia-1.4b_alpaca_farm_instructions_sft_sep-checkpoint-7500", "data_files": [{"split": "preference", "path": "pythia-1.4b_alpaca_farm_instructions_sft_sep-checkpoint-7500/preference-*"}]}, {"config_name": "pythia-1.4b_alpaca_farm_instructions_sft_sep-checkpoint-8000", "data_files": [{"split": "preference", "path": "pythia-1.4b_alpaca_farm_instructions_sft_sep-checkpoint-8000/preference-*"}]}, {"config_name": "pythia-1.4b_alpaca_farm_instructions_sft_sep-checkpoint-8500", "data_files": [{"split": "preference", "path": "pythia-1.4b_alpaca_farm_instructions_sft_sep-checkpoint-8500/preference-*"}]}, {"config_name": "pythia-1.4b_alpaca_farm_instructions_sft_sep-checkpoint-9000", "data_files": [{"split": "preference", "path": "pythia-1.4b_alpaca_farm_instructions_sft_sep-checkpoint-9000/preference-*"}]}, {"config_name": "pythia-1.4b_alpaca_farm_instructions_sft_sep-checkpoint-9500", "data_files": [{"split": "preference", "path": "pythia-1.4b_alpaca_farm_instructions_sft_sep-checkpoint-9500/preference-*"}]}, {"config_name": "pythia-1.4b_alpaca_farm_instructions_sft_sep_spe", "data_files": [{"split": "preference", "path": "pythia-1.4b_alpaca_farm_instructions_sft_sep_spe/preference-*"}]}, {"config_name": "pythia-1.4b_alpaca_farm_instructions_sft_sep_spe-checkpoint-1000", "data_files": [{"split": "preference", "path": "pythia-1.4b_alpaca_farm_instructions_sft_sep_spe-checkpoint-1000/preference-*"}]}, {"config_name": "pythia-1.4b_alpaca_farm_instructions_sft_sep_spe-checkpoint-10000", "data_files": [{"split": "preference", "path": "pythia-1.4b_alpaca_farm_instructions_sft_sep_spe-checkpoint-10000/preference-*"}]}, {"config_name": "pythia-1.4b_alpaca_farm_instructions_sft_sep_spe-checkpoint-10500", "data_files": [{"split": "preference", "path": "pythia-1.4b_alpaca_farm_instructions_sft_sep_spe-checkpoint-10500/preference-*"}]}, {"config_name": "pythia-1.4b_alpaca_farm_instructions_sft_sep_spe-checkpoint-11000", "data_files": [{"split": "preference", "path": "pythia-1.4b_alpaca_farm_instructions_sft_sep_spe-checkpoint-11000/preference-*"}]}, {"config_name": "pythia-1.4b_alpaca_farm_instructions_sft_sep_spe-checkpoint-11500", "data_files": [{"split": "preference", "path": "pythia-1.4b_alpaca_farm_instructions_sft_sep_spe-checkpoint-11500/preference-*"}]}, {"config_name": "pythia-1.4b_alpaca_farm_instructions_sft_sep_spe-checkpoint-12000", "data_files": [{"split": "preference", "path": "pythia-1.4b_alpaca_farm_instructions_sft_sep_spe-checkpoint-12000/preference-*"}]}, {"config_name": "pythia-1.4b_alpaca_farm_instructions_sft_sep_spe-checkpoint-12500", "data_files": [{"split": "preference", "path": "pythia-1.4b_alpaca_farm_instructions_sft_sep_spe-checkpoint-12500/preference-*"}]}, {"config_name": "pythia-1.4b_alpaca_farm_instructions_sft_sep_spe-checkpoint-1500", "data_files": [{"split": "preference", "path": "pythia-1.4b_alpaca_farm_instructions_sft_sep_spe-checkpoint-1500/preference-*"}]}, {"config_name": "pythia-1.4b_alpaca_farm_instructions_sft_sep_spe-checkpoint-2000", "data_files": [{"split": "preference", "path": "pythia-1.4b_alpaca_farm_instructions_sft_sep_spe-checkpoint-2000/preference-*"}]}, {"config_name": "pythia-1.4b_alpaca_farm_instructions_sft_sep_spe-checkpoint-2500", "data_files": [{"split": "preference", "path": "pythia-1.4b_alpaca_farm_instructions_sft_sep_spe-checkpoint-2500/preference-*"}]}, {"config_name": "pythia-1.4b_alpaca_farm_instructions_sft_sep_spe-checkpoint-3000", "data_files": [{"split": "preference", "path": "pythia-1.4b_alpaca_farm_instructions_sft_sep_spe-checkpoint-3000/preference-*"}]}, {"config_name": "pythia-1.4b_alpaca_farm_instructions_sft_sep_spe-checkpoint-3500", "data_files": [{"split": "preference", "path": "pythia-1.4b_alpaca_farm_instructions_sft_sep_spe-checkpoint-3500/preference-*"}]}, {"config_name": "pythia-1.4b_alpaca_farm_instructions_sft_sep_spe-checkpoint-4000", "data_files": [{"split": "preference", "path": "pythia-1.4b_alpaca_farm_instructions_sft_sep_spe-checkpoint-4000/preference-*"}]}, {"config_name": "pythia-1.4b_alpaca_farm_instructions_sft_sep_spe-checkpoint-4500", "data_files": [{"split": "preference", "path": "pythia-1.4b_alpaca_farm_instructions_sft_sep_spe-checkpoint-4500/preference-*"}]}, {"config_name": "pythia-1.4b_alpaca_farm_instructions_sft_sep_spe-checkpoint-500", "data_files": [{"split": "preference", "path": "pythia-1.4b_alpaca_farm_instructions_sft_sep_spe-checkpoint-500/preference-*"}]}, {"config_name": "pythia-1.4b_alpaca_farm_instructions_sft_sep_spe-checkpoint-5000", "data_files": [{"split": "preference", "path": "pythia-1.4b_alpaca_farm_instructions_sft_sep_spe-checkpoint-5000/preference-*"}]}, {"config_name": "pythia-1.4b_alpaca_farm_instructions_sft_sep_spe-checkpoint-5500", "data_files": [{"split": "preference", "path": "pythia-1.4b_alpaca_farm_instructions_sft_sep_spe-checkpoint-5500/preference-*"}]}, {"config_name": "pythia-1.4b_alpaca_farm_instructions_sft_sep_spe-checkpoint-6000", "data_files": [{"split": "preference", "path": "pythia-1.4b_alpaca_farm_instructions_sft_sep_spe-checkpoint-6000/preference-*"}]}, {"config_name": "pythia-1.4b_alpaca_farm_instructions_sft_sep_spe-checkpoint-6500", "data_files": [{"split": "preference", "path": "pythia-1.4b_alpaca_farm_instructions_sft_sep_spe-checkpoint-6500/preference-*"}]}, {"config_name": "pythia-1.4b_alpaca_farm_instructions_sft_sep_spe-checkpoint-7000", "data_files": [{"split": "preference", "path": "pythia-1.4b_alpaca_farm_instructions_sft_sep_spe-checkpoint-7000/preference-*"}]}, {"config_name": "pythia-1.4b_alpaca_farm_instructions_sft_sep_spe-checkpoint-7500", "data_files": [{"split": "preference", "path": "pythia-1.4b_alpaca_farm_instructions_sft_sep_spe-checkpoint-7500/preference-*"}]}, {"config_name": "pythia-1.4b_alpaca_farm_instructions_sft_sep_spe-checkpoint-8000", "data_files": [{"split": "preference", "path": "pythia-1.4b_alpaca_farm_instructions_sft_sep_spe-checkpoint-8000/preference-*"}]}, {"config_name": "pythia-1.4b_alpaca_farm_instructions_sft_sep_spe-checkpoint-8500", "data_files": [{"split": "preference", "path": "pythia-1.4b_alpaca_farm_instructions_sft_sep_spe-checkpoint-8500/preference-*"}]}, {"config_name": "pythia-1.4b_alpaca_farm_instructions_sft_sep_spe-checkpoint-9000", "data_files": [{"split": "preference", "path": "pythia-1.4b_alpaca_farm_instructions_sft_sep_spe-checkpoint-9000/preference-*"}]}, {"config_name": "pythia-1.4b_alpaca_farm_instructions_sft_sep_spe-checkpoint-9500", "data_files": [{"split": "preference", "path": "pythia-1.4b_alpaca_farm_instructions_sft_sep_spe-checkpoint-9500/preference-*"}]}, {"config_name": "starcoderbase-1b-sft", "data_files": [{"split": "preference", "path": "starcoderbase-1b-sft/preference-*"}]}]} | 2024-01-15T08:37:34+00:00 | [] | [] | TAGS
#region-us
| # Dataset Card for "alpaca_farm-alpaca_gpt4_preference-re-preference_eval"
More Information needed | [
"# Dataset Card for \"alpaca_farm-alpaca_gpt4_preference-re-preference_eval\"\n\nMore Information needed"
] | [
"TAGS\n#region-us \n",
"# Dataset Card for \"alpaca_farm-alpaca_gpt4_preference-re-preference_eval\"\n\nMore Information needed"
] |
edd1cd743169d778c98f671d4b096c1fac5b59b7 |
# MMVP Benchmark Datacard
## Basic Information
**Title:** MMVP Benchmark
**Description:** The MMVP (Multimodal Visual Patterns) Benchmark focuses on identifying “CLIP-blind pairs” – images that are perceived as similar by CLIP despite having clear visual differences. MMVP benchmarks the performance of state-of-the-art systems, including GPT-4V, across nine basic visual patterns. It highlights the challenges these systems face in answering straightforward questions, often leading to incorrect responses and hallucinated explanations.
## Dataset Details
- **Content Types:** Images (CLIP-blind pairs)
- **Volume:** 300 images
- **Source of Data:** Derived from ImageNet-1k and LAION-Aesthetics
- **Data Collection Method:** Identification of CLIP-blind pairs through comparative analysis
| MMVP/MMVP | [
"task_categories:question-answering",
"size_categories:n<1K",
"license:mit",
"region:us"
] | 2024-01-10T09:14:24+00:00 | {"license": "mit", "size_categories": ["n<1K"], "task_categories": ["question-answering"]} | 2024-01-10T09:56:01+00:00 | [] | [] | TAGS
#task_categories-question-answering #size_categories-n<1K #license-mit #region-us
|
# MMVP Benchmark Datacard
## Basic Information
Title: MMVP Benchmark
Description: The MMVP (Multimodal Visual Patterns) Benchmark focuses on identifying “CLIP-blind pairs” – images that are perceived as similar by CLIP despite having clear visual differences. MMVP benchmarks the performance of state-of-the-art systems, including GPT-4V, across nine basic visual patterns. It highlights the challenges these systems face in answering straightforward questions, often leading to incorrect responses and hallucinated explanations.
## Dataset Details
- Content Types: Images (CLIP-blind pairs)
- Volume: 300 images
- Source of Data: Derived from ImageNet-1k and LAION-Aesthetics
- Data Collection Method: Identification of CLIP-blind pairs through comparative analysis
| [
"# MMVP Benchmark Datacard",
"## Basic Information\n\nTitle: MMVP Benchmark\n\nDescription: The MMVP (Multimodal Visual Patterns) Benchmark focuses on identifying “CLIP-blind pairs” – images that are perceived as similar by CLIP despite having clear visual differences. MMVP benchmarks the performance of state-of-the-art systems, including GPT-4V, across nine basic visual patterns. It highlights the challenges these systems face in answering straightforward questions, often leading to incorrect responses and hallucinated explanations.",
"## Dataset Details\n\n- Content Types: Images (CLIP-blind pairs)\n- Volume: 300 images\n- Source of Data: Derived from ImageNet-1k and LAION-Aesthetics\n- Data Collection Method: Identification of CLIP-blind pairs through comparative analysis"
] | [
"TAGS\n#task_categories-question-answering #size_categories-n<1K #license-mit #region-us \n",
"# MMVP Benchmark Datacard",
"## Basic Information\n\nTitle: MMVP Benchmark\n\nDescription: The MMVP (Multimodal Visual Patterns) Benchmark focuses on identifying “CLIP-blind pairs” – images that are perceived as similar by CLIP despite having clear visual differences. MMVP benchmarks the performance of state-of-the-art systems, including GPT-4V, across nine basic visual patterns. It highlights the challenges these systems face in answering straightforward questions, often leading to incorrect responses and hallucinated explanations.",
"## Dataset Details\n\n- Content Types: Images (CLIP-blind pairs)\n- Volume: 300 images\n- Source of Data: Derived from ImageNet-1k and LAION-Aesthetics\n- Data Collection Method: Identification of CLIP-blind pairs through comparative analysis"
] |
740f187eba8c418e2adaa40707ba43914af2801e | # Dataset Card for "death_se42-type2"
[More Information needed](https://github.com/huggingface/datasets/blob/main/CONTRIBUTING.md#how-to-contribute-to-the-dataset-cards) | rohangbs/death_se42-type2 | [
"region:us"
] | 2024-01-10T09:33:37+00:00 | {"configs": [{"config_name": "default", "data_files": [{"split": "train", "path": "data/train-*"}, {"split": "val", "path": "data/val-*"}]}], "dataset_info": {"features": [{"name": "image", "dtype": "image"}, {"name": "ground_truth", "dtype": "string"}], "splits": [{"name": "train", "num_bytes": 19293975.0, "num_examples": 122}, {"name": "val", "num_bytes": 2214406.0, "num_examples": 14}], "download_size": 21490683, "dataset_size": 21508381.0}} | 2024-01-11T06:02:25+00:00 | [] | [] | TAGS
#region-us
| # Dataset Card for "death_se42-type2"
More Information needed | [
"# Dataset Card for \"death_se42-type2\"\n\nMore Information needed"
] | [
"TAGS\n#region-us \n",
"# Dataset Card for \"death_se42-type2\"\n\nMore Information needed"
] |
347deb793e7297ea096d00bf3809b04e88ee2483 | This is the dataset which i used to train https://huggingface.co/TriadParty/deepmoney-34b-200k-chat-evaluator
Enjoy it | TriadParty/deepmoney-sft | [
"license:apache-2.0",
"region:us"
] | 2024-01-10T09:38:20+00:00 | {"license": "apache-2.0"} | 2024-01-10T09:47:01+00:00 | [] | [] | TAGS
#license-apache-2.0 #region-us
| This is the dataset which i used to train URL
Enjoy it | [] | [
"TAGS\n#license-apache-2.0 #region-us \n"
] |
211372d5357398f914d806d07dc305aea1f257d2 |
# MMVP-VLM Benchmark Datacard
## Basic Information
**Title:** MMVP-VLM Benchmark
**Description:** The MMVP-VLM (Multimodal Visual Patterns - Visual Language Models) Benchmark is designed to systematically evaluate the performance of recent CLIP-based models in understanding and processing visual patterns. It distills a subset of questions from the original MMVP benchmark into simpler language descriptions, categorizing them into distinct visual patterns. Each visual pattern is represented by 15 text-image pairs. The benchmark assesses whether CLIP models can accurately match these image-text combinations, providing insights into the capabilities and limitations of these models.
## Dataset Details
- **Content Types:** Text-Image Pairs
- **Volume:** Balanced number of questions for each visual pattern, with each pattern represented by 15 pairs.
- **Source of Data:** Subset from MMVP benchmark, supplemented with additional questions for balance
- **Data Collection Method:** Distillation and categorization of questions from MMVP benchmark into simpler language
## Usage
### Intended Use
- Evaluation of CLIP models' ability to understand and process various visual patterns.
| MMVP/MMVP_VLM | [
"task_categories:zero-shot-classification",
"size_categories:n<1K",
"license:mit",
"region:us"
] | 2024-01-10T09:48:42+00:00 | {"license": "mit", "size_categories": ["n<1K"], "task_categories": ["zero-shot-classification"]} | 2024-01-10T13:22:26+00:00 | [] | [] | TAGS
#task_categories-zero-shot-classification #size_categories-n<1K #license-mit #region-us
|
# MMVP-VLM Benchmark Datacard
## Basic Information
Title: MMVP-VLM Benchmark
Description: The MMVP-VLM (Multimodal Visual Patterns - Visual Language Models) Benchmark is designed to systematically evaluate the performance of recent CLIP-based models in understanding and processing visual patterns. It distills a subset of questions from the original MMVP benchmark into simpler language descriptions, categorizing them into distinct visual patterns. Each visual pattern is represented by 15 text-image pairs. The benchmark assesses whether CLIP models can accurately match these image-text combinations, providing insights into the capabilities and limitations of these models.
## Dataset Details
- Content Types: Text-Image Pairs
- Volume: Balanced number of questions for each visual pattern, with each pattern represented by 15 pairs.
- Source of Data: Subset from MMVP benchmark, supplemented with additional questions for balance
- Data Collection Method: Distillation and categorization of questions from MMVP benchmark into simpler language
## Usage
### Intended Use
- Evaluation of CLIP models' ability to understand and process various visual patterns.
| [
"# MMVP-VLM Benchmark Datacard",
"## Basic Information\n\nTitle: MMVP-VLM Benchmark\n\nDescription: The MMVP-VLM (Multimodal Visual Patterns - Visual Language Models) Benchmark is designed to systematically evaluate the performance of recent CLIP-based models in understanding and processing visual patterns. It distills a subset of questions from the original MMVP benchmark into simpler language descriptions, categorizing them into distinct visual patterns. Each visual pattern is represented by 15 text-image pairs. The benchmark assesses whether CLIP models can accurately match these image-text combinations, providing insights into the capabilities and limitations of these models.",
"## Dataset Details\n\n- Content Types: Text-Image Pairs\n- Volume: Balanced number of questions for each visual pattern, with each pattern represented by 15 pairs. \n- Source of Data: Subset from MMVP benchmark, supplemented with additional questions for balance\n- Data Collection Method: Distillation and categorization of questions from MMVP benchmark into simpler language",
"## Usage",
"### Intended Use\n\n- Evaluation of CLIP models' ability to understand and process various visual patterns."
] | [
"TAGS\n#task_categories-zero-shot-classification #size_categories-n<1K #license-mit #region-us \n",
"# MMVP-VLM Benchmark Datacard",
"## Basic Information\n\nTitle: MMVP-VLM Benchmark\n\nDescription: The MMVP-VLM (Multimodal Visual Patterns - Visual Language Models) Benchmark is designed to systematically evaluate the performance of recent CLIP-based models in understanding and processing visual patterns. It distills a subset of questions from the original MMVP benchmark into simpler language descriptions, categorizing them into distinct visual patterns. Each visual pattern is represented by 15 text-image pairs. The benchmark assesses whether CLIP models can accurately match these image-text combinations, providing insights into the capabilities and limitations of these models.",
"## Dataset Details\n\n- Content Types: Text-Image Pairs\n- Volume: Balanced number of questions for each visual pattern, with each pattern represented by 15 pairs. \n- Source of Data: Subset from MMVP benchmark, supplemented with additional questions for balance\n- Data Collection Method: Distillation and categorization of questions from MMVP benchmark into simpler language",
"## Usage",
"### Intended Use\n\n- Evaluation of CLIP models' ability to understand and process various visual patterns."
] |
89d6a4960b79701828e3902f69068fb4e667c493 | 12222222 | suxin/test_llama_aaa | [
"region:us"
] | 2024-01-10T09:51:40+00:00 | {} | 2024-01-10T10:21:48+00:00 | [] | [] | TAGS
#region-us
| 12222222 | [] | [
"TAGS\n#region-us \n"
] |
0e87734f97eb40b56bc43b10374ad68c42bbd216 | 虎扑评分数据, 共3.5M
| Zhangzhe197/HupuJudge | [
"license:apache-2.0",
"region:us"
] | 2024-01-10T09:59:35+00:00 | {"license": "apache-2.0"} | 2024-01-10T10:03:24+00:00 | [] | [] | TAGS
#license-apache-2.0 #region-us
| 虎扑评分数据, 共3.5M
| [] | [
"TAGS\n#license-apache-2.0 #region-us \n"
] |
18ee5f5916ac34abdec0abc872bf92d87d03f8bf |
3.7M headlines and corresponding links from the LA Times spanning over a century (1914-2024). Should be useful for knowledge retreival.
Created Jan. 10, 2024 | Astris/LA-Times-Linked-Headlines | [
"size_categories:1M<n<10M",
"region:us"
] | 2024-01-10T10:16:40+00:00 | {"size_categories": ["1M<n<10M"], "dataset_info": {"features": [{"name": "title", "dtype": "string"}, {"name": "link", "dtype": "string"}, {"name": "month", "dtype": "int64"}, {"name": "year", "dtype": "int64"}], "splits": [{"name": "train", "num_bytes": 585407628, "num_examples": 3721395}], "download_size": 235503523, "dataset_size": 585407628}, "configs": [{"config_name": "default", "data_files": [{"split": "train", "path": "data/train-*"}]}]} | 2024-01-10T10:20:24+00:00 | [] | [] | TAGS
#size_categories-1M<n<10M #region-us
|
3.7M headlines and corresponding links from the LA Times spanning over a century (1914-2024). Should be useful for knowledge retreival.
Created Jan. 10, 2024 | [] | [
"TAGS\n#size_categories-1M<n<10M #region-us \n"
] |
04fd75ba775cf267b02904ac2880cb5cff293772 | # Dataset Card for "myriade_noun_aligned_with_wordnet_v2"
[More Information needed](https://github.com/huggingface/datasets/blob/main/CONTRIBUTING.md#how-to-contribute-to-the-dataset-cards) | gguichard/myriade_noun_aligned_with_wordnet_v2 | [
"region:us"
] | 2024-01-10T10:48:28+00:00 | {"dataset_info": {"features": [{"name": "tokens", "sequence": "string"}, {"name": "wn_sens", "sequence": "int64"}, {"name": "input_ids", "sequence": "int32"}, {"name": "attention_mask", "sequence": "int8"}, {"name": "labels", "sequence": "int64"}], "splits": [{"name": "train", "num_bytes": 98888979, "num_examples": 162516}], "download_size": 22776318, "dataset_size": 98888979}, "configs": [{"config_name": "default", "data_files": [{"split": "train", "path": "data/train-*"}]}]} | 2024-01-10T10:48:32+00:00 | [] | [] | TAGS
#region-us
| # Dataset Card for "myriade_noun_aligned_with_wordnet_v2"
More Information needed | [
"# Dataset Card for \"myriade_noun_aligned_with_wordnet_v2\"\n\nMore Information needed"
] | [
"TAGS\n#region-us \n",
"# Dataset Card for \"myriade_noun_aligned_with_wordnet_v2\"\n\nMore Information needed"
] |
c725f98e5fe030daddd84d74e5b4301c1747ed2f |
# Table Detection in Document Images using YOLOv8
The Table Detection YOLO dataset is a collection of document images annotated with table bounding boxes suitable for \
training object detection models, specifically using the YOLOv8 (You Only Look Once) architecture. The dataset is intended \
for developing and evaluating table detection algorithms within the field of document analysis and recognition. The \
annotations define the locations of tables within a variety of document images, which can range from scanned documents to \
digital PDFs.
### Dataset Labels
```json
['table']
```
### Number of Images
```json
{"train": 815, "valid": 152, "test": 52}
```
### Getting Started
| abdullahmeda/yolov8-table-detection | [
"task_categories:object-detection",
"size_categories:1K<n<10K",
"language:en",
"Table",
"Unstructured Document",
"YOLOv8",
"Object Detection",
"Table Detection",
"region:us"
] | 2024-01-10T11:18:45+00:00 | {"language": ["en"], "size_categories": ["1K<n<10K"], "task_categories": ["object-detection"], "pretty_name": "TableDetectionNet", "tags": ["Table", "Unstructured Document", "YOLOv8", "Object Detection", "Table Detection"]} | 2024-01-10T12:37:15+00:00 | [] | [
"en"
] | TAGS
#task_categories-object-detection #size_categories-1K<n<10K #language-English #Table #Unstructured Document #YOLOv8 #Object Detection #Table Detection #region-us
|
# Table Detection in Document Images using YOLOv8
The Table Detection YOLO dataset is a collection of document images annotated with table bounding boxes suitable for \
training object detection models, specifically using the YOLOv8 (You Only Look Once) architecture. The dataset is intended \
for developing and evaluating table detection algorithms within the field of document analysis and recognition. The \
annotations define the locations of tables within a variety of document images, which can range from scanned documents to \
digital PDFs.
### Dataset Labels
### Number of Images
### Getting Started
| [
"# Table Detection in Document Images using YOLOv8\n\nThe Table Detection YOLO dataset is a collection of document images annotated with table bounding boxes suitable for \\\ntraining object detection models, specifically using the YOLOv8 (You Only Look Once) architecture. The dataset is intended \\\nfor developing and evaluating table detection algorithms within the field of document analysis and recognition. The \\\nannotations define the locations of tables within a variety of document images, which can range from scanned documents to \\\ndigital PDFs.",
"### Dataset Labels",
"### Number of Images",
"### Getting Started"
] | [
"TAGS\n#task_categories-object-detection #size_categories-1K<n<10K #language-English #Table #Unstructured Document #YOLOv8 #Object Detection #Table Detection #region-us \n",
"# Table Detection in Document Images using YOLOv8\n\nThe Table Detection YOLO dataset is a collection of document images annotated with table bounding boxes suitable for \\\ntraining object detection models, specifically using the YOLOv8 (You Only Look Once) architecture. The dataset is intended \\\nfor developing and evaluating table detection algorithms within the field of document analysis and recognition. The \\\nannotations define the locations of tables within a variety of document images, which can range from scanned documents to \\\ndigital PDFs.",
"### Dataset Labels",
"### Number of Images",
"### Getting Started"
] |
fbf8657aa30f59436bfccba5469874e1bdc19222 | # Dataset Card for "vsums_synthetic_gpt4_deduped"
[More Information needed](https://github.com/huggingface/datasets/blob/main/CONTRIBUTING.md#how-to-contribute-to-the-dataset-cards) | Xapien/vsums_synthetic_gpt4_deduped | [
"region:us"
] | 2024-01-10T11:40:44+00:00 | {"dataset_info": {"features": [{"name": "name", "dtype": "string"}, {"name": "summary_a", "dtype": "string"}, {"name": "same_entity_summary", "dtype": "string"}, {"name": "different_entity_summary", "dtype": "string"}, {"name": "__index_level_0__", "dtype": "int64"}], "splits": [{"name": "train", "num_bytes": 10159, "num_examples": 25}], "download_size": 12680, "dataset_size": 10159}, "configs": [{"config_name": "default", "data_files": [{"split": "train", "path": "data/train-*"}]}]} | 2024-01-12T09:31:24+00:00 | [] | [] | TAGS
#region-us
| # Dataset Card for "vsums_synthetic_gpt4_deduped"
More Information needed | [
"# Dataset Card for \"vsums_synthetic_gpt4_deduped\"\n\nMore Information needed"
] | [
"TAGS\n#region-us \n",
"# Dataset Card for \"vsums_synthetic_gpt4_deduped\"\n\nMore Information needed"
] |
0a79736e5a1add452b014bdecc66bd4618a4e856 | # 3.5k lengthy ShareGPT conversations used to train [EABF Models](https://github.com/GAIR-NLP/Entropy-ABF)
Following the data cleaning pipeline in [FastChat](https://github.com/lm-sys/FastChat), we processed [raw ShareGPT conversations](https://huggingface.co/datasets/philschmid/sharegpt-raw) by keeping English conversations only, excluding those with less than 10,000 tokens, and splitting long conversations that exceed 16,384 tokens.
We find multi-round long conversations efficient for extending LLMs' context window.
# Dataset Overview
Our released dataset follows the conventional ShareGPT multi-round conversation JSON format:
- **id**: The unique identifier for each conversation in the dataset.
- **model**: The model used for generating the response. (Can be left empty if not applicable)
- **conversations**: Object containing the dialogue between human and AI assistants.
- **from**: Indicates whether the message is from the "human" or the "AI".
- **value**: The actual content of the message.
Example JSON Object:
```
{
"id": "wNBG8Gp_0",
"model": "",
"conversations": [
{
"from": "human",
"value": "Java add to the arraylist of a class type"
},
{
"from": "gpt",
"value": "To add an element to an ArrayList of a specific class type in Java..."
},
...
]
}
``` | Arist12/EABF-ShareGPT-Long-3.5k | [
"license:mit",
"region:us"
] | 2024-01-10T12:00:12+00:00 | {"license": "mit"} | 2024-01-10T12:41:09+00:00 | [] | [] | TAGS
#license-mit #region-us
| # 3.5k lengthy ShareGPT conversations used to train EABF Models
Following the data cleaning pipeline in FastChat, we processed raw ShareGPT conversations by keeping English conversations only, excluding those with less than 10,000 tokens, and splitting long conversations that exceed 16,384 tokens.
We find multi-round long conversations efficient for extending LLMs' context window.
# Dataset Overview
Our released dataset follows the conventional ShareGPT multi-round conversation JSON format:
- id: The unique identifier for each conversation in the dataset.
- model: The model used for generating the response. (Can be left empty if not applicable)
- conversations: Object containing the dialogue between human and AI assistants.
- from: Indicates whether the message is from the "human" or the "AI".
- value: The actual content of the message.
Example JSON Object:
| [
"# 3.5k lengthy ShareGPT conversations used to train EABF Models\n\nFollowing the data cleaning pipeline in FastChat, we processed raw ShareGPT conversations by keeping English conversations only, excluding those with less than 10,000 tokens, and splitting long conversations that exceed 16,384 tokens. \n\nWe find multi-round long conversations efficient for extending LLMs' context window.",
"# Dataset Overview\nOur released dataset follows the conventional ShareGPT multi-round conversation JSON format:\n\n- id: The unique identifier for each conversation in the dataset.\n- model: The model used for generating the response. (Can be left empty if not applicable)\n- conversations: Object containing the dialogue between human and AI assistants.\n - from: Indicates whether the message is from the \"human\" or the \"AI\".\n - value: The actual content of the message.\n\nExample JSON Object:"
] | [
"TAGS\n#license-mit #region-us \n",
"# 3.5k lengthy ShareGPT conversations used to train EABF Models\n\nFollowing the data cleaning pipeline in FastChat, we processed raw ShareGPT conversations by keeping English conversations only, excluding those with less than 10,000 tokens, and splitting long conversations that exceed 16,384 tokens. \n\nWe find multi-round long conversations efficient for extending LLMs' context window.",
"# Dataset Overview\nOur released dataset follows the conventional ShareGPT multi-round conversation JSON format:\n\n- id: The unique identifier for each conversation in the dataset.\n- model: The model used for generating the response. (Can be left empty if not applicable)\n- conversations: Object containing the dialogue between human and AI assistants.\n - from: Indicates whether the message is from the \"human\" or the \"AI\".\n - value: The actual content of the message.\n\nExample JSON Object:"
] |
d5d43ef62fdec9797d25e6c7ee8ae42a605e50fb |
# Dataset of furina/フリーナ/芙宁娜 (Genshin Impact)
This is the dataset of furina/フリーナ/芙宁娜 (Genshin Impact), containing 500 images and their tags.
The core tags of this character are `blue_eyes, blue_hair, bangs, white_hair, ahoge, hair_between_eyes, long_hair, multicolored_hair, hat, bow, streaked_hair, very_long_hair, breasts`, which are pruned in this dataset.
Images are crawled from many sites (e.g. danbooru, pixiv, zerochan ...), the auto-crawling system is powered by [DeepGHS Team](https://github.com/deepghs)([huggingface organization](https://huggingface.co/deepghs)).
## List of Packages
| Name | Images | Size | Download | Type | Description |
|:-----------------|---------:|:------------|:----------------------------------------------------------------------------------------------------------------|:-----------|:---------------------------------------------------------------------|
| raw | 500 | 1.20 GiB | [Download](https://huggingface.co/datasets/CyberHarem/furina_genshin/resolve/main/dataset-raw.zip) | Waifuc-Raw | Raw data with meta information (min edge aligned to 1400 if larger). |
| 800 | 500 | 544.45 MiB | [Download](https://huggingface.co/datasets/CyberHarem/furina_genshin/resolve/main/dataset-800.zip) | IMG+TXT | dataset with the shorter side not exceeding 800 pixels. |
| stage3-p480-800 | 1295 | 1.17 GiB | [Download](https://huggingface.co/datasets/CyberHarem/furina_genshin/resolve/main/dataset-stage3-p480-800.zip) | IMG+TXT | 3-stage cropped dataset with the area not less than 480x480 pixels. |
| 1200 | 500 | 1007.01 MiB | [Download](https://huggingface.co/datasets/CyberHarem/furina_genshin/resolve/main/dataset-1200.zip) | IMG+TXT | dataset with the shorter side not exceeding 1200 pixels. |
| stage3-p480-1200 | 1295 | 1.91 GiB | [Download](https://huggingface.co/datasets/CyberHarem/furina_genshin/resolve/main/dataset-stage3-p480-1200.zip) | IMG+TXT | 3-stage cropped dataset with the area not less than 480x480 pixels. |
### Load Raw Dataset with Waifuc
We provide raw dataset (including tagged images) for [waifuc](https://deepghs.github.io/waifuc/main/tutorials/installation/index.html) loading. If you need this, just run the following code
```python
import os
import zipfile
from huggingface_hub import hf_hub_download
from waifuc.source import LocalSource
# download raw archive file
zip_file = hf_hub_download(
repo_id='CyberHarem/furina_genshin',
repo_type='dataset',
filename='dataset-raw.zip',
)
# extract files to your directory
dataset_dir = 'dataset_dir'
os.makedirs(dataset_dir, exist_ok=True)
with zipfile.ZipFile(zip_file, 'r') as zf:
zf.extractall(dataset_dir)
# load the dataset with waifuc
source = LocalSource(dataset_dir)
for item in source:
print(item.image, item.meta['filename'], item.meta['tags'])
```
## List of Clusters
List of tag clustering result, maybe some outfits can be mined here.
### Raw Text Version
| # | Samples | Img-1 | Img-2 | Img-3 | Img-4 | Img-5 | Tags |
|----:|----------:|:--------------------------------|:--------------------------------|:--------------------------------|:--------------------------------|:--------------------------------|:------------------------------------------------------------------------------------------------------------------------------------------------------------------------------------------------------------------------------------------------------|
| 0 | 18 |  |  |  |  |  | 1girl, solo, long_sleeves, looking_at_viewer, upper_body, blue_headwear, simple_background, top_hat, white_background, black_gloves, white_gloves, jacket, smile, shirt, ascot, brooch, open_mouth, closed_mouth |
| 1 | 10 |  |  |  |  |  | 1girl, looking_at_viewer, smile, solo, long_sleeves, white_dress, puffy_sleeves, closed_mouth, small_breasts |
| 2 | 7 |  |  |  |  |  | 1girl, high_heels, long_sleeves, shirt, shorts, solo, black_gloves, blue_headwear, full_body, looking_at_viewer, :d, black_footwear, blue_bow, frills, jacket, open_mouth, thigh_strap, bowtie, bubble, top_hat, blue_footwear, shoes, sitting, socks |
| 3 | 7 |  |  |  |  |  | 1girl, anklet, barefoot, full_body, solo, closed_mouth, long_sleeves, looking_at_viewer, white_dress, smile, bare_legs, feet, sitting, toes, water, blue_nails, puffy_sleeves, toenail_polish |
| 4 | 16 |  |  |  |  |  | 1girl, obi, long_sleeves, looking_at_viewer, solo, blue_kimono, holding, smile, wide_sleeves, hair_flower, blush, floral_print, open_mouth, short_hair, upper_body, blue_flower |
| 5 | 8 |  |  |  |  |  | 1girl, blush, nipples, 1boy, hetero, looking_at_viewer, open_mouth, navel, penis, pussy, sex, small_breasts, solo_focus, sweat, medium_breasts, pov, smile, spread_legs, completely_nude, gloves, thigh_strap, uncensored, vaginal |
### Table Version
| # | Samples | Img-1 | Img-2 | Img-3 | Img-4 | Img-5 | 1girl | solo | long_sleeves | looking_at_viewer | upper_body | blue_headwear | simple_background | top_hat | white_background | black_gloves | white_gloves | jacket | smile | shirt | ascot | brooch | open_mouth | closed_mouth | white_dress | puffy_sleeves | small_breasts | high_heels | shorts | full_body | :d | black_footwear | blue_bow | frills | thigh_strap | bowtie | bubble | blue_footwear | shoes | sitting | socks | anklet | barefoot | bare_legs | feet | toes | water | blue_nails | toenail_polish | obi | blue_kimono | holding | wide_sleeves | hair_flower | blush | floral_print | short_hair | blue_flower | nipples | 1boy | hetero | navel | penis | pussy | sex | solo_focus | sweat | medium_breasts | pov | spread_legs | completely_nude | gloves | uncensored | vaginal |
|----:|----------:|:--------------------------------|:--------------------------------|:--------------------------------|:--------------------------------|:--------------------------------|:--------|:-------|:---------------|:--------------------|:-------------|:----------------|:--------------------|:----------|:-------------------|:---------------|:---------------|:---------|:--------|:--------|:--------|:---------|:-------------|:---------------|:--------------|:----------------|:----------------|:-------------|:---------|:------------|:-----|:-----------------|:-----------|:---------|:--------------|:---------|:---------|:----------------|:--------|:----------|:--------|:---------|:-----------|:------------|:-------|:-------|:--------|:-------------|:-----------------|:------|:--------------|:----------|:---------------|:--------------|:--------|:---------------|:-------------|:--------------|:----------|:-------|:---------|:--------|:--------|:--------|:------|:-------------|:--------|:-----------------|:------|:--------------|:------------------|:---------|:-------------|:----------|
| 0 | 18 |  |  |  |  |  | X | X | X | X | X | X | X | X | X | X | X | X | X | X | X | X | X | X | | | | | | | | | | | | | | | | | | | | | | | | | | | | | | | | | | | | | | | | | | | | | | | | | | |
| 1 | 10 |  |  |  |  |  | X | X | X | X | | | | | | | | | X | | | | | X | X | X | X | | | | | | | | | | | | | | | | | | | | | | | | | | | | | | | | | | | | | | | | | | | | | | | |
| 2 | 7 |  |  |  |  |  | X | X | X | X | | X | | X | | X | | X | | X | | | X | | | | | X | X | X | X | X | X | X | X | X | X | X | X | X | X | | | | | | | | | | | | | | | | | | | | | | | | | | | | | | | | | |
| 3 | 7 |  |  |  |  |  | X | X | X | X | | | | | | | | | X | | | | | X | X | X | | | | X | | | | | | | | | | X | | X | X | X | X | X | X | X | X | | | | | | | | | | | | | | | | | | | | | | | | | |
| 4 | 16 |  |  |  |  |  | X | X | X | X | X | | | | | | | | X | | | | X | | | | | | | | | | | | | | | | | | | | | | | | | | | X | X | X | X | X | X | X | X | X | | | | | | | | | | | | | | | | |
| 5 | 8 |  |  |  |  |  | X | | | X | | | | | | | | | X | | | | X | | | | X | | | | | | | | X | | | | | | | | | | | | | | | | | | | | X | | | | X | X | X | X | X | X | X | X | X | X | X | X | X | X | X | X |
| CyberHarem/furina_genshin | [
"task_categories:text-to-image",
"size_categories:n<1K",
"license:mit",
"art",
"not-for-all-audiences",
"region:us"
] | 2024-01-10T13:38:01+00:00 | {"license": "mit", "size_categories": ["n<1K"], "task_categories": ["text-to-image"], "tags": ["art", "not-for-all-audiences"]} | 2024-01-10T16:10:55+00:00 | [] | [] | TAGS
#task_categories-text-to-image #size_categories-n<1K #license-mit #art #not-for-all-audiences #region-us
| Dataset of furina/フリーナ/芙宁娜 (Genshin Impact)
===========================================
This is the dataset of furina/フリーナ/芙宁娜 (Genshin Impact), containing 500 images and their tags.
The core tags of this character are 'blue\_eyes, blue\_hair, bangs, white\_hair, ahoge, hair\_between\_eyes, long\_hair, multicolored\_hair, hat, bow, streaked\_hair, very\_long\_hair, breasts', which are pruned in this dataset.
Images are crawled from many sites (e.g. danbooru, pixiv, zerochan ...), the auto-crawling system is powered by DeepGHS Team(huggingface organization).
List of Packages
----------------
### Load Raw Dataset with Waifuc
We provide raw dataset (including tagged images) for waifuc loading. If you need this, just run the following code
List of Clusters
----------------
List of tag clustering result, maybe some outfits can be mined here.
### Raw Text Version
### Table Version
| [
"### Load Raw Dataset with Waifuc\n\n\nWe provide raw dataset (including tagged images) for waifuc loading. If you need this, just run the following code\n\n\nList of Clusters\n----------------\n\n\nList of tag clustering result, maybe some outfits can be mined here.",
"### Raw Text Version",
"### Table Version"
] | [
"TAGS\n#task_categories-text-to-image #size_categories-n<1K #license-mit #art #not-for-all-audiences #region-us \n",
"### Load Raw Dataset with Waifuc\n\n\nWe provide raw dataset (including tagged images) for waifuc loading. If you need this, just run the following code\n\n\nList of Clusters\n----------------\n\n\nList of tag clustering result, maybe some outfits can be mined here.",
"### Raw Text Version",
"### Table Version"
] |
61c9d0e97b3c13687a5e8f691d8326cf34cd463b |
# StackMathQA
StackMathQA is a meticulously curated collection of **2 million** mathematical questions and answers, sourced from various Stack Exchange sites. This repository is designed to serve as a comprehensive resource for researchers, educators, and enthusiasts in the field of mathematics and AI research.
## Configs
```YAML
configs:
- config_name: stackmathqa1600k
data_files: data/stackmathqa1600k/all.jsonl
default: true
- config_name: stackmathqa800k
data_files: data/stackmathqa800k/all.jsonl
- config_name: stackmathqa400k
data_files: data/stackmathqa400k/all.jsonl
- config_name: stackmathqa200k
data_files: data/stackmathqa200k/all.jsonl
- config_name: stackmathqa100k
data_files: data/stackmathqa100k/all.jsonl
- config_name: stackmathqafull-1q1a
data_files: preprocessed/stackexchange-math--1q1a/*.jsonl
- config_name: stackmathqafull-qalist
data_files: preprocessed/stackexchange-math/*.jsonl
```
How to load data:
```python
from datasets import load_dataset
ds = load_dataset("math-ai/StackMathQA", "stackmathqa1600k") # or any valid config_name
```
## Preprocessed Data
In the `./preprocessed/stackexchange-math` directory and `./preprocessed/stackexchange-math--1q1a` directory, you will find the data structured in two formats:
1. **Question and List of Answers Format**:
Each entry is structured as {"Q": "question", "A_List": ["answer1", "answer2", ...]}.
- `math.stackexchange.com.jsonl`: 827,439 lines
- `mathoverflow.net.jsonl`: 90,645 lines
- `stats.stackexchange.com.jsonl`: 103,024 lines
- `physics.stackexchange.com.jsonl`: 117,318 lines
- In total: **1,138,426** questions
```YAML
dataset_info:
features:
- name: Q
dtype: string
description: "The mathematical question in LaTeX encoded format."
- name: A_list
dtype: sequence
description: "The list of answers to the mathematical question, also in LaTeX encoded."
- name: meta
dtype: dict
description: "A collection of metadata for each question and its corresponding answer list."
```
2. **Question and Single Answer Format**:
Each line contains a question and one corresponding answer, structured as {"Q": "question", "A": "answer"}. Multiple answers for the same question are separated into different lines.
- `math.stackexchange.com.jsonl`: 1,407,739 lines
- `mathoverflow.net.jsonl`: 166,592 lines
- `stats.stackexchange.com.jsonl`: 156,143 lines
- `physics.stackexchange.com.jsonl`: 226,532 lines
- In total: **1,957,006** answers
```YAML
dataset_info:
features:
- name: Q
dtype: string
description: "The mathematical question in LaTeX encoded format."
- name: A
dtype: string
description: "The answer to the mathematical question, also in LaTeX encoded."
- name: meta
dtype: dict
description: "A collection of metadata for each question-answer pair."
```
## Selected Data
The dataset has been carefully curated using importance sampling. We offer selected subsets of the dataset (`./preprocessed/stackexchange-math--1q1a`) with different sizes to cater to varied needs:
```YAML
dataset_info:
features:
- name: Q
dtype: string
description: "The mathematical question in LaTeX encoded format."
- name: A
dtype: string
description: "The answer to the mathematical question, also in LaTeX encoded."
- name: meta
dtype: dict
description: "A collection of metadata for each question-answer pair."
```
### StackMathQA1600K
- Location: `./data/stackmathqa1600k`
- Contents:
- `all.jsonl`: Containing 1.6 million entries.
- `meta.json`: Metadata and additional information.
```bash
Source: Stack Exchange (Math), Count: 1244887
Source: MathOverflow, Count: 110041
Source: Stack Exchange (Stats), Count: 99878
Source: Stack Exchange (Physics), Count: 145194
```
Similar structures are available for StackMathQA800K, StackMathQA400K, StackMathQA200K, and StackMathQA100K subsets.
### StackMathQA800K
- Location: `./data/stackmathqa800k`
- Contents:
- `all.jsonl`: Containing 800k entries.
- `meta.json`: Metadata and additional information.
```bash
Source: Stack Exchange (Math), Count: 738850
Source: MathOverflow, Count: 24276
Source: Stack Exchange (Stats), Count: 15046
Source: Stack Exchange (Physics), Count: 21828
```
### StackMathQA400K
- Location: `./data/stackmathqa400k`
- Contents:
- `all.jsonl`: Containing 400k entries.
- `meta.json`: Metadata and additional information.
```bash
Source: Stack Exchange (Math), Count: 392940
Source: MathOverflow, Count: 3963
Source: Stack Exchange (Stats), Count: 1637
Source: Stack Exchange (Physics), Count: 1460
```
### StackMathQA200K
- Location: `./data/stackmathqa200k`
- Contents:
- `all.jsonl`: Containing 200k entries.
- `meta.json`: Metadata and additional information.
```bash
Source: Stack Exchange (Math), Count: 197792
Source: MathOverflow, Count: 1367
Source: Stack Exchange (Stats), Count: 423
Source: Stack Exchange (Physics), Count: 418
```
### StackMathQA100K
- Location: `./data/stackmathqa100k`
- Contents:
- `all.jsonl`: Containing 100k entries.
- `meta.json`: Metadata and additional information.
```bash
Source: Stack Exchange (Math), Count: 99013
Source: MathOverflow, Count: 626
Source: Stack Exchange (Stats), Count: 182
Source: Stack Exchange (Physics), Count: 179
```
## Citation
We appreciate your use of StackMathQA in your work. If you find this repository helpful, please consider citing it and star this repo. Feel free to contact [email protected] or open an issue if you have any questions.
```bibtex
@misc{stackmathqa2024,
title={StackMathQA: A Curated Collection of 2 Million Mathematical Questions and Answers Sourced from Stack Exchange},
author={Zhang, Yifan},
year={2024},
}
```
| math-ai/StackMathQA | [
"task_categories:text-generation",
"task_categories:question-answering",
"size_categories:1B<n<10B",
"language:en",
"license:cc-by-4.0",
"mathematical-reasoning",
"reasoning",
"finetuning",
"pretraining",
"llm",
"region:us"
] | 2024-01-10T13:41:12+00:00 | {"language": ["en"], "license": "cc-by-4.0", "size_categories": ["1B<n<10B"], "task_categories": ["text-generation", "question-answering"], "pretty_name": "StackMathQA", "configs": [{"config_name": "stackmathqa1600k", "data_files": "data/stackmathqa1600k/all.jsonl", "default": true}, {"config_name": "stackmathqa800k", "data_files": "data/stackmathqa800k/all.jsonl"}, {"config_name": "stackmathqa400k", "data_files": "data/stackmathqa400k/all.jsonl"}, {"config_name": "stackmathqa200k", "data_files": "data/stackmathqa200k/all.jsonl"}, {"config_name": "stackmathqa100k", "data_files": "data/stackmathqa100k/all.jsonl"}, {"config_name": "stackmathqafull-1q1a", "data_files": "preprocessed/stackexchange-math--1q1a/*.jsonl"}, {"config_name": "stackmathqafull-qalist", "data_files": "preprocessed/stackexchange-math/*.jsonl"}], "tags": ["mathematical-reasoning", "reasoning", "finetuning", "pretraining", "llm"]} | 2024-01-14T01:57:26+00:00 | [] | [
"en"
] | TAGS
#task_categories-text-generation #task_categories-question-answering #size_categories-1B<n<10B #language-English #license-cc-by-4.0 #mathematical-reasoning #reasoning #finetuning #pretraining #llm #region-us
|
# StackMathQA
StackMathQA is a meticulously curated collection of 2 million mathematical questions and answers, sourced from various Stack Exchange sites. This repository is designed to serve as a comprehensive resource for researchers, educators, and enthusiasts in the field of mathematics and AI research.
## Configs
How to load data:
## Preprocessed Data
In the './preprocessed/stackexchange-math' directory and './preprocessed/stackexchange-math--1q1a' directory, you will find the data structured in two formats:
1. Question and List of Answers Format:
Each entry is structured as {"Q": "question", "A_List": ["answer1", "answer2", ...]}.
- 'URL': 827,439 lines
- 'URL': 90,645 lines
- 'URL': 103,024 lines
- 'URL': 117,318 lines
- In total: 1,138,426 questions
2. Question and Single Answer Format:
Each line contains a question and one corresponding answer, structured as {"Q": "question", "A": "answer"}. Multiple answers for the same question are separated into different lines.
- 'URL': 1,407,739 lines
- 'URL': 166,592 lines
- 'URL': 156,143 lines
- 'URL': 226,532 lines
- In total: 1,957,006 answers
## Selected Data
The dataset has been carefully curated using importance sampling. We offer selected subsets of the dataset ('./preprocessed/stackexchange-math--1q1a') with different sizes to cater to varied needs:
### StackMathQA1600K
- Location: './data/stackmathqa1600k'
- Contents:
- 'URL': Containing 1.6 million entries.
- 'URL': Metadata and additional information.
Similar structures are available for StackMathQA800K, StackMathQA400K, StackMathQA200K, and StackMathQA100K subsets.
### StackMathQA800K
- Location: './data/stackmathqa800k'
- Contents:
- 'URL': Containing 800k entries.
- 'URL': Metadata and additional information.
### StackMathQA400K
- Location: './data/stackmathqa400k'
- Contents:
- 'URL': Containing 400k entries.
- 'URL': Metadata and additional information.
### StackMathQA200K
- Location: './data/stackmathqa200k'
- Contents:
- 'URL': Containing 200k entries.
- 'URL': Metadata and additional information.
### StackMathQA100K
- Location: './data/stackmathqa100k'
- Contents:
- 'URL': Containing 100k entries.
- 'URL': Metadata and additional information.
We appreciate your use of StackMathQA in your work. If you find this repository helpful, please consider citing it and star this repo. Feel free to contact zhangyif21@URL or open an issue if you have any questions.
| [
"# StackMathQA\nStackMathQA is a meticulously curated collection of 2 million mathematical questions and answers, sourced from various Stack Exchange sites. This repository is designed to serve as a comprehensive resource for researchers, educators, and enthusiasts in the field of mathematics and AI research.",
"## Configs\n\n\n\nHow to load data:",
"## Preprocessed Data\nIn the './preprocessed/stackexchange-math' directory and './preprocessed/stackexchange-math--1q1a' directory, you will find the data structured in two formats:\n\n1. Question and List of Answers Format:\n Each entry is structured as {\"Q\": \"question\", \"A_List\": [\"answer1\", \"answer2\", ...]}.\n - 'URL': 827,439 lines\n - 'URL': 90,645 lines\n - 'URL': 103,024 lines\n - 'URL': 117,318 lines\n - In total: 1,138,426 questions\n\n\n\n2. Question and Single Answer Format:\n Each line contains a question and one corresponding answer, structured as {\"Q\": \"question\", \"A\": \"answer\"}. Multiple answers for the same question are separated into different lines.\n - 'URL': 1,407,739 lines\n - 'URL': 166,592 lines\n - 'URL': 156,143 lines\n - 'URL': 226,532 lines\n - In total: 1,957,006 answers",
"## Selected Data\nThe dataset has been carefully curated using importance sampling. We offer selected subsets of the dataset ('./preprocessed/stackexchange-math--1q1a') with different sizes to cater to varied needs:",
"### StackMathQA1600K\n- Location: './data/stackmathqa1600k'\n- Contents:\n - 'URL': Containing 1.6 million entries.\n - 'URL': Metadata and additional information.\n\n\n\nSimilar structures are available for StackMathQA800K, StackMathQA400K, StackMathQA200K, and StackMathQA100K subsets.",
"### StackMathQA800K\n- Location: './data/stackmathqa800k'\n- Contents:\n - 'URL': Containing 800k entries.\n - 'URL': Metadata and additional information.",
"### StackMathQA400K\n\n- Location: './data/stackmathqa400k'\n- Contents:\n - 'URL': Containing 400k entries.\n - 'URL': Metadata and additional information.",
"### StackMathQA200K\n\n- Location: './data/stackmathqa200k'\n- Contents:\n - 'URL': Containing 200k entries.\n - 'URL': Metadata and additional information.",
"### StackMathQA100K\n\n- Location: './data/stackmathqa100k'\n- Contents:\n - 'URL': Containing 100k entries.\n - 'URL': Metadata and additional information.\n\n\n\nWe appreciate your use of StackMathQA in your work. If you find this repository helpful, please consider citing it and star this repo. Feel free to contact zhangyif21@URL or open an issue if you have any questions."
] | [
"TAGS\n#task_categories-text-generation #task_categories-question-answering #size_categories-1B<n<10B #language-English #license-cc-by-4.0 #mathematical-reasoning #reasoning #finetuning #pretraining #llm #region-us \n",
"# StackMathQA\nStackMathQA is a meticulously curated collection of 2 million mathematical questions and answers, sourced from various Stack Exchange sites. This repository is designed to serve as a comprehensive resource for researchers, educators, and enthusiasts in the field of mathematics and AI research.",
"## Configs\n\n\n\nHow to load data:",
"## Preprocessed Data\nIn the './preprocessed/stackexchange-math' directory and './preprocessed/stackexchange-math--1q1a' directory, you will find the data structured in two formats:\n\n1. Question and List of Answers Format:\n Each entry is structured as {\"Q\": \"question\", \"A_List\": [\"answer1\", \"answer2\", ...]}.\n - 'URL': 827,439 lines\n - 'URL': 90,645 lines\n - 'URL': 103,024 lines\n - 'URL': 117,318 lines\n - In total: 1,138,426 questions\n\n\n\n2. Question and Single Answer Format:\n Each line contains a question and one corresponding answer, structured as {\"Q\": \"question\", \"A\": \"answer\"}. Multiple answers for the same question are separated into different lines.\n - 'URL': 1,407,739 lines\n - 'URL': 166,592 lines\n - 'URL': 156,143 lines\n - 'URL': 226,532 lines\n - In total: 1,957,006 answers",
"## Selected Data\nThe dataset has been carefully curated using importance sampling. We offer selected subsets of the dataset ('./preprocessed/stackexchange-math--1q1a') with different sizes to cater to varied needs:",
"### StackMathQA1600K\n- Location: './data/stackmathqa1600k'\n- Contents:\n - 'URL': Containing 1.6 million entries.\n - 'URL': Metadata and additional information.\n\n\n\nSimilar structures are available for StackMathQA800K, StackMathQA400K, StackMathQA200K, and StackMathQA100K subsets.",
"### StackMathQA800K\n- Location: './data/stackmathqa800k'\n- Contents:\n - 'URL': Containing 800k entries.\n - 'URL': Metadata and additional information.",
"### StackMathQA400K\n\n- Location: './data/stackmathqa400k'\n- Contents:\n - 'URL': Containing 400k entries.\n - 'URL': Metadata and additional information.",
"### StackMathQA200K\n\n- Location: './data/stackmathqa200k'\n- Contents:\n - 'URL': Containing 200k entries.\n - 'URL': Metadata and additional information.",
"### StackMathQA100K\n\n- Location: './data/stackmathqa100k'\n- Contents:\n - 'URL': Containing 100k entries.\n - 'URL': Metadata and additional information.\n\n\n\nWe appreciate your use of StackMathQA in your work. If you find this repository helpful, please consider citing it and star this repo. Feel free to contact zhangyif21@URL or open an issue if you have any questions."
] |
16a5c27cc71a2d2dece0de82ee60468f558f3e7d | # Dataset Card for "arxiv-test-2048"
[More Information needed](https://github.com/huggingface/datasets/blob/main/CONTRIBUTING.md#how-to-contribute-to-the-dataset-cards) | anumafzal94/arxiv-test-2048 | [
"region:us"
] | 2024-01-10T13:46:26+00:00 | {"configs": [{"config_name": "default", "data_files": [{"split": "test", "path": "data/test-*"}]}], "dataset_info": {"features": [{"name": "text", "dtype": "string"}, {"name": "summary", "dtype": "string"}], "splits": [{"name": "test", "num_bytes": 6592892.490835663, "num_examples": 196}], "download_size": 615323, "dataset_size": 6592892.490835663}} | 2024-01-10T14:37:16+00:00 | [] | [] | TAGS
#region-us
| # Dataset Card for "arxiv-test-2048"
More Information needed | [
"# Dataset Card for \"arxiv-test-2048\"\n\nMore Information needed"
] | [
"TAGS\n#region-us \n",
"# Dataset Card for \"arxiv-test-2048\"\n\nMore Information needed"
] |
46f7ee5d4905da5a1edeb846b6dbb88166766fdf | # Dataset Card for "pubmed-test-2048"
[More Information needed](https://github.com/huggingface/datasets/blob/main/CONTRIBUTING.md#how-to-contribute-to-the-dataset-cards) | anumafzal94/pubmed-test-2048 | [
"region:us"
] | 2024-01-10T13:53:57+00:00 | {"configs": [{"config_name": "default", "data_files": [{"split": "test", "path": "data/test-*"}]}], "dataset_info": {"features": [{"name": "text", "dtype": "string"}, {"name": "summary", "dtype": "string"}], "splits": [{"name": "test", "num_bytes": 18823188.567961164, "num_examples": 984}], "download_size": 0, "dataset_size": 18823188.567961164}} | 2024-01-10T14:36:59+00:00 | [] | [] | TAGS
#region-us
| # Dataset Card for "pubmed-test-2048"
More Information needed | [
"# Dataset Card for \"pubmed-test-2048\"\n\nMore Information needed"
] | [
"TAGS\n#region-us \n",
"# Dataset Card for \"pubmed-test-2048\"\n\nMore Information needed"
] |
1cdc60ff1c02d0029dcaf4dcc4c1c48dc2c4d22e | # Dataset Card for "VIVOS_CommonVoice_FOSD_combined_dataset"
[More Information needed](https://github.com/huggingface/datasets/blob/main/CONTRIBUTING.md#how-to-contribute-to-the-dataset-cards) | tuanmanh28/VIVOS_CommonVoice_FOSD_combined_dataset | [
"region:us"
] | 2024-01-10T14:09:35+00:00 | {"dataset_info": {"features": [{"name": "file", "dtype": "string"}, {"name": "audio", "dtype": {"audio": {"sampling_rate": 16000}}}, {"name": "text", "dtype": "string"}], "splits": [{"name": "train", "num_bytes": 2479635100.0, "num_examples": 37513}, {"name": "test", "num_bytes": 203842090.2, "num_examples": 4590}], "download_size": 2699288186, "dataset_size": 2683477190.2}, "configs": [{"config_name": "default", "data_files": [{"split": "train", "path": "data/train-*"}, {"split": "test", "path": "data/test-*"}]}]} | 2024-01-10T14:13:22+00:00 | [] | [] | TAGS
#region-us
| # Dataset Card for "VIVOS_CommonVoice_FOSD_combined_dataset"
More Information needed | [
"# Dataset Card for \"VIVOS_CommonVoice_FOSD_combined_dataset\"\n\nMore Information needed"
] | [
"TAGS\n#region-us \n",
"# Dataset Card for \"VIVOS_CommonVoice_FOSD_combined_dataset\"\n\nMore Information needed"
] |
6517bf6444f9ad56fd725bf013c0883e508628f6 |
# Dataset Card for Evaluation run of dvilasuero/NeuralHermes-2.5-Mistral-7B-distilabel
<!-- Provide a quick summary of the dataset. -->
Dataset automatically created during the evaluation run of model [dvilasuero/NeuralHermes-2.5-Mistral-7B-distilabel](https://huggingface.co/dvilasuero/NeuralHermes-2.5-Mistral-7B-distilabel) on the [Open LLM Leaderboard](https://huggingface.co/spaces/HuggingFaceH4/open_llm_leaderboard).
The dataset is composed of 63 configuration, each one coresponding to one of the evaluated task.
The dataset has been created from 1 run(s). Each run can be found as a specific split in each configuration, the split being named using the timestamp of the run.The "train" split is always pointing to the latest results.
An additional configuration "results" store all the aggregated results of the run (and is used to compute and display the aggregated metrics on the [Open LLM Leaderboard](https://huggingface.co/spaces/HuggingFaceH4/open_llm_leaderboard)).
To load the details from a run, you can for instance do the following:
```python
from datasets import load_dataset
data = load_dataset("open-llm-leaderboard/details_dvilasuero__NeuralHermes-2.5-Mistral-7B-distilabel",
"harness_winogrande_5",
split="train")
```
## Latest results
These are the [latest results from run 2024-01-10T14:17:38.745392](https://huggingface.co/datasets/open-llm-leaderboard/details_dvilasuero__NeuralHermes-2.5-Mistral-7B-distilabel/blob/main/results_2024-01-10T14-17-38.745392.json)(note that their might be results for other tasks in the repos if successive evals didn't cover the same tasks. You find each in the results and the "latest" split for each eval):
```python
{
"all": {
"acc": 0.6385164875113943,
"acc_stderr": 0.032116569731227715,
"acc_norm": 0.6402618412720931,
"acc_norm_stderr": 0.032757857320627824,
"mc1": 0.386780905752754,
"mc1_stderr": 0.017048857010515107,
"mc2": 0.5585768485275255,
"mc2_stderr": 0.01538538971794349
},
"harness|arc:challenge|25": {
"acc": 0.6194539249146758,
"acc_stderr": 0.014188277712349812,
"acc_norm": 0.6578498293515358,
"acc_norm_stderr": 0.01386415215917728
},
"harness|hellaswag|10": {
"acc": 0.660426209918343,
"acc_stderr": 0.004725967684806406,
"acc_norm": 0.8497311292571201,
"acc_norm_stderr": 0.003566044777327419
},
"harness|hendrycksTest-abstract_algebra|5": {
"acc": 0.29,
"acc_stderr": 0.04560480215720684,
"acc_norm": 0.29,
"acc_norm_stderr": 0.04560480215720684
},
"harness|hendrycksTest-anatomy|5": {
"acc": 0.5851851851851851,
"acc_stderr": 0.04256193767901408,
"acc_norm": 0.5851851851851851,
"acc_norm_stderr": 0.04256193767901408
},
"harness|hendrycksTest-astronomy|5": {
"acc": 0.6907894736842105,
"acc_stderr": 0.037610708698674805,
"acc_norm": 0.6907894736842105,
"acc_norm_stderr": 0.037610708698674805
},
"harness|hendrycksTest-business_ethics|5": {
"acc": 0.6,
"acc_stderr": 0.04923659639173309,
"acc_norm": 0.6,
"acc_norm_stderr": 0.04923659639173309
},
"harness|hendrycksTest-clinical_knowledge|5": {
"acc": 0.6754716981132075,
"acc_stderr": 0.02881561571343211,
"acc_norm": 0.6754716981132075,
"acc_norm_stderr": 0.02881561571343211
},
"harness|hendrycksTest-college_biology|5": {
"acc": 0.7777777777777778,
"acc_stderr": 0.03476590104304133,
"acc_norm": 0.7777777777777778,
"acc_norm_stderr": 0.03476590104304133
},
"harness|hendrycksTest-college_chemistry|5": {
"acc": 0.49,
"acc_stderr": 0.05024183937956912,
"acc_norm": 0.49,
"acc_norm_stderr": 0.05024183937956912
},
"harness|hendrycksTest-college_computer_science|5": {
"acc": 0.46,
"acc_stderr": 0.05009082659620333,
"acc_norm": 0.46,
"acc_norm_stderr": 0.05009082659620333
},
"harness|hendrycksTest-college_mathematics|5": {
"acc": 0.24,
"acc_stderr": 0.04292346959909282,
"acc_norm": 0.24,
"acc_norm_stderr": 0.04292346959909282
},
"harness|hendrycksTest-college_medicine|5": {
"acc": 0.6242774566473989,
"acc_stderr": 0.036928207672648664,
"acc_norm": 0.6242774566473989,
"acc_norm_stderr": 0.036928207672648664
},
"harness|hendrycksTest-college_physics|5": {
"acc": 0.3627450980392157,
"acc_stderr": 0.04784060704105653,
"acc_norm": 0.3627450980392157,
"acc_norm_stderr": 0.04784060704105653
},
"harness|hendrycksTest-computer_security|5": {
"acc": 0.76,
"acc_stderr": 0.042923469599092816,
"acc_norm": 0.76,
"acc_norm_stderr": 0.042923469599092816
},
"harness|hendrycksTest-conceptual_physics|5": {
"acc": 0.5404255319148936,
"acc_stderr": 0.03257901482099835,
"acc_norm": 0.5404255319148936,
"acc_norm_stderr": 0.03257901482099835
},
"harness|hendrycksTest-econometrics|5": {
"acc": 0.4649122807017544,
"acc_stderr": 0.046920083813689104,
"acc_norm": 0.4649122807017544,
"acc_norm_stderr": 0.046920083813689104
},
"harness|hendrycksTest-electrical_engineering|5": {
"acc": 0.5448275862068965,
"acc_stderr": 0.04149886942192117,
"acc_norm": 0.5448275862068965,
"acc_norm_stderr": 0.04149886942192117
},
"harness|hendrycksTest-elementary_mathematics|5": {
"acc": 0.4074074074074074,
"acc_stderr": 0.02530590624159063,
"acc_norm": 0.4074074074074074,
"acc_norm_stderr": 0.02530590624159063
},
"harness|hendrycksTest-formal_logic|5": {
"acc": 0.4523809523809524,
"acc_stderr": 0.044518079590553275,
"acc_norm": 0.4523809523809524,
"acc_norm_stderr": 0.044518079590553275
},
"harness|hendrycksTest-global_facts|5": {
"acc": 0.36,
"acc_stderr": 0.04824181513244218,
"acc_norm": 0.36,
"acc_norm_stderr": 0.04824181513244218
},
"harness|hendrycksTest-high_school_biology|5": {
"acc": 0.7935483870967742,
"acc_stderr": 0.02302589961718871,
"acc_norm": 0.7935483870967742,
"acc_norm_stderr": 0.02302589961718871
},
"harness|hendrycksTest-high_school_chemistry|5": {
"acc": 0.5172413793103449,
"acc_stderr": 0.035158955511656986,
"acc_norm": 0.5172413793103449,
"acc_norm_stderr": 0.035158955511656986
},
"harness|hendrycksTest-high_school_computer_science|5": {
"acc": 0.65,
"acc_stderr": 0.047937248544110196,
"acc_norm": 0.65,
"acc_norm_stderr": 0.047937248544110196
},
"harness|hendrycksTest-high_school_european_history|5": {
"acc": 0.7878787878787878,
"acc_stderr": 0.03192271569548301,
"acc_norm": 0.7878787878787878,
"acc_norm_stderr": 0.03192271569548301
},
"harness|hendrycksTest-high_school_geography|5": {
"acc": 0.7929292929292929,
"acc_stderr": 0.02886977846026704,
"acc_norm": 0.7929292929292929,
"acc_norm_stderr": 0.02886977846026704
},
"harness|hendrycksTest-high_school_government_and_politics|5": {
"acc": 0.8963730569948186,
"acc_stderr": 0.02199531196364424,
"acc_norm": 0.8963730569948186,
"acc_norm_stderr": 0.02199531196364424
},
"harness|hendrycksTest-high_school_macroeconomics|5": {
"acc": 0.6051282051282051,
"acc_stderr": 0.0247843169421564,
"acc_norm": 0.6051282051282051,
"acc_norm_stderr": 0.0247843169421564
},
"harness|hendrycksTest-high_school_mathematics|5": {
"acc": 0.3074074074074074,
"acc_stderr": 0.02813325257881563,
"acc_norm": 0.3074074074074074,
"acc_norm_stderr": 0.02813325257881563
},
"harness|hendrycksTest-high_school_microeconomics|5": {
"acc": 0.6722689075630253,
"acc_stderr": 0.03048991141767323,
"acc_norm": 0.6722689075630253,
"acc_norm_stderr": 0.03048991141767323
},
"harness|hendrycksTest-high_school_physics|5": {
"acc": 0.3443708609271523,
"acc_stderr": 0.038796870240733264,
"acc_norm": 0.3443708609271523,
"acc_norm_stderr": 0.038796870240733264
},
"harness|hendrycksTest-high_school_psychology|5": {
"acc": 0.8330275229357799,
"acc_stderr": 0.015990154885073368,
"acc_norm": 0.8330275229357799,
"acc_norm_stderr": 0.015990154885073368
},
"harness|hendrycksTest-high_school_statistics|5": {
"acc": 0.5,
"acc_stderr": 0.034099716973523674,
"acc_norm": 0.5,
"acc_norm_stderr": 0.034099716973523674
},
"harness|hendrycksTest-high_school_us_history|5": {
"acc": 0.8137254901960784,
"acc_stderr": 0.027325470966716312,
"acc_norm": 0.8137254901960784,
"acc_norm_stderr": 0.027325470966716312
},
"harness|hendrycksTest-high_school_world_history|5": {
"acc": 0.8059071729957806,
"acc_stderr": 0.025744902532290913,
"acc_norm": 0.8059071729957806,
"acc_norm_stderr": 0.025744902532290913
},
"harness|hendrycksTest-human_aging|5": {
"acc": 0.7040358744394619,
"acc_stderr": 0.030636591348699803,
"acc_norm": 0.7040358744394619,
"acc_norm_stderr": 0.030636591348699803
},
"harness|hendrycksTest-human_sexuality|5": {
"acc": 0.7786259541984732,
"acc_stderr": 0.03641297081313728,
"acc_norm": 0.7786259541984732,
"acc_norm_stderr": 0.03641297081313728
},
"harness|hendrycksTest-international_law|5": {
"acc": 0.7768595041322314,
"acc_stderr": 0.03800754475228732,
"acc_norm": 0.7768595041322314,
"acc_norm_stderr": 0.03800754475228732
},
"harness|hendrycksTest-jurisprudence|5": {
"acc": 0.8055555555555556,
"acc_stderr": 0.038260763248848646,
"acc_norm": 0.8055555555555556,
"acc_norm_stderr": 0.038260763248848646
},
"harness|hendrycksTest-logical_fallacies|5": {
"acc": 0.7914110429447853,
"acc_stderr": 0.031921934489347235,
"acc_norm": 0.7914110429447853,
"acc_norm_stderr": 0.031921934489347235
},
"harness|hendrycksTest-machine_learning|5": {
"acc": 0.5089285714285714,
"acc_stderr": 0.04745033255489123,
"acc_norm": 0.5089285714285714,
"acc_norm_stderr": 0.04745033255489123
},
"harness|hendrycksTest-management|5": {
"acc": 0.7864077669902912,
"acc_stderr": 0.040580420156460344,
"acc_norm": 0.7864077669902912,
"acc_norm_stderr": 0.040580420156460344
},
"harness|hendrycksTest-marketing|5": {
"acc": 0.8717948717948718,
"acc_stderr": 0.02190190511507333,
"acc_norm": 0.8717948717948718,
"acc_norm_stderr": 0.02190190511507333
},
"harness|hendrycksTest-medical_genetics|5": {
"acc": 0.69,
"acc_stderr": 0.04648231987117316,
"acc_norm": 0.69,
"acc_norm_stderr": 0.04648231987117316
},
"harness|hendrycksTest-miscellaneous|5": {
"acc": 0.8186462324393359,
"acc_stderr": 0.013778693778464074,
"acc_norm": 0.8186462324393359,
"acc_norm_stderr": 0.013778693778464074
},
"harness|hendrycksTest-moral_disputes|5": {
"acc": 0.7283236994219653,
"acc_stderr": 0.023948512905468358,
"acc_norm": 0.7283236994219653,
"acc_norm_stderr": 0.023948512905468358
},
"harness|hendrycksTest-moral_scenarios|5": {
"acc": 0.33519553072625696,
"acc_stderr": 0.015788007190185884,
"acc_norm": 0.33519553072625696,
"acc_norm_stderr": 0.015788007190185884
},
"harness|hendrycksTest-nutrition|5": {
"acc": 0.7483660130718954,
"acc_stderr": 0.0248480182638752,
"acc_norm": 0.7483660130718954,
"acc_norm_stderr": 0.0248480182638752
},
"harness|hendrycksTest-philosophy|5": {
"acc": 0.6945337620578779,
"acc_stderr": 0.026160584450140446,
"acc_norm": 0.6945337620578779,
"acc_norm_stderr": 0.026160584450140446
},
"harness|hendrycksTest-prehistory|5": {
"acc": 0.7438271604938271,
"acc_stderr": 0.024288533637726095,
"acc_norm": 0.7438271604938271,
"acc_norm_stderr": 0.024288533637726095
},
"harness|hendrycksTest-professional_accounting|5": {
"acc": 0.5177304964539007,
"acc_stderr": 0.02980873964223777,
"acc_norm": 0.5177304964539007,
"acc_norm_stderr": 0.02980873964223777
},
"harness|hendrycksTest-professional_law|5": {
"acc": 0.47783572359843546,
"acc_stderr": 0.012757683047716175,
"acc_norm": 0.47783572359843546,
"acc_norm_stderr": 0.012757683047716175
},
"harness|hendrycksTest-professional_medicine|5": {
"acc": 0.6654411764705882,
"acc_stderr": 0.028661996202335303,
"acc_norm": 0.6654411764705882,
"acc_norm_stderr": 0.028661996202335303
},
"harness|hendrycksTest-professional_psychology|5": {
"acc": 0.673202614379085,
"acc_stderr": 0.018975427920507215,
"acc_norm": 0.673202614379085,
"acc_norm_stderr": 0.018975427920507215
},
"harness|hendrycksTest-public_relations|5": {
"acc": 0.6636363636363637,
"acc_stderr": 0.04525393596302506,
"acc_norm": 0.6636363636363637,
"acc_norm_stderr": 0.04525393596302506
},
"harness|hendrycksTest-security_studies|5": {
"acc": 0.7591836734693878,
"acc_stderr": 0.027372942201788163,
"acc_norm": 0.7591836734693878,
"acc_norm_stderr": 0.027372942201788163
},
"harness|hendrycksTest-sociology|5": {
"acc": 0.835820895522388,
"acc_stderr": 0.026193923544454115,
"acc_norm": 0.835820895522388,
"acc_norm_stderr": 0.026193923544454115
},
"harness|hendrycksTest-us_foreign_policy|5": {
"acc": 0.85,
"acc_stderr": 0.035887028128263686,
"acc_norm": 0.85,
"acc_norm_stderr": 0.035887028128263686
},
"harness|hendrycksTest-virology|5": {
"acc": 0.5602409638554217,
"acc_stderr": 0.03864139923699122,
"acc_norm": 0.5602409638554217,
"acc_norm_stderr": 0.03864139923699122
},
"harness|hendrycksTest-world_religions|5": {
"acc": 0.8362573099415205,
"acc_stderr": 0.028380919596145866,
"acc_norm": 0.8362573099415205,
"acc_norm_stderr": 0.028380919596145866
},
"harness|truthfulqa:mc|0": {
"mc1": 0.386780905752754,
"mc1_stderr": 0.017048857010515107,
"mc2": 0.5585768485275255,
"mc2_stderr": 0.01538538971794349
},
"harness|winogrande|5": {
"acc": 0.7868981846882399,
"acc_stderr": 0.011508957690722762
},
"harness|gsm8k|5": {
"acc": 0.6148597422289613,
"acc_stderr": 0.013404165536474303
}
}
```
## Dataset Details
### Dataset Description
<!-- Provide a longer summary of what this dataset is. -->
- **Curated by:** [More Information Needed]
- **Funded by [optional]:** [More Information Needed]
- **Shared by [optional]:** [More Information Needed]
- **Language(s) (NLP):** [More Information Needed]
- **License:** [More Information Needed]
### Dataset Sources [optional]
<!-- Provide the basic links for the dataset. -->
- **Repository:** [More Information Needed]
- **Paper [optional]:** [More Information Needed]
- **Demo [optional]:** [More Information Needed]
## Uses
<!-- Address questions around how the dataset is intended to be used. -->
### Direct Use
<!-- This section describes suitable use cases for the dataset. -->
[More Information Needed]
### Out-of-Scope Use
<!-- This section addresses misuse, malicious use, and uses that the dataset will not work well for. -->
[More Information Needed]
## Dataset Structure
<!-- This section provides a description of the dataset fields, and additional information about the dataset structure such as criteria used to create the splits, relationships between data points, etc. -->
[More Information Needed]
## Dataset Creation
### Curation Rationale
<!-- Motivation for the creation of this dataset. -->
[More Information Needed]
### Source Data
<!-- This section describes the source data (e.g. news text and headlines, social media posts, translated sentences, ...). -->
#### Data Collection and Processing
<!-- This section describes the data collection and processing process such as data selection criteria, filtering and normalization methods, tools and libraries used, etc. -->
[More Information Needed]
#### Who are the source data producers?
<!-- This section describes the people or systems who originally created the data. It should also include self-reported demographic or identity information for the source data creators if this information is available. -->
[More Information Needed]
### Annotations [optional]
<!-- If the dataset contains annotations which are not part of the initial data collection, use this section to describe them. -->
#### Annotation process
<!-- This section describes the annotation process such as annotation tools used in the process, the amount of data annotated, annotation guidelines provided to the annotators, interannotator statistics, annotation validation, etc. -->
[More Information Needed]
#### Who are the annotators?
<!-- This section describes the people or systems who created the annotations. -->
[More Information Needed]
#### Personal and Sensitive Information
<!-- State whether the dataset contains data that might be considered personal, sensitive, or private (e.g., data that reveals addresses, uniquely identifiable names or aliases, racial or ethnic origins, sexual orientations, religious beliefs, political opinions, financial or health data, etc.). If efforts were made to anonymize the data, describe the anonymization process. -->
[More Information Needed]
## Bias, Risks, and Limitations
<!-- This section is meant to convey both technical and sociotechnical limitations. -->
[More Information Needed]
### Recommendations
<!-- This section is meant to convey recommendations with respect to the bias, risk, and technical limitations. -->
Users should be made aware of the risks, biases and limitations of the dataset. More information needed for further recommendations.
## Citation [optional]
<!-- If there is a paper or blog post introducing the dataset, the APA and Bibtex information for that should go in this section. -->
**BibTeX:**
[More Information Needed]
**APA:**
[More Information Needed]
## Glossary [optional]
<!-- If relevant, include terms and calculations in this section that can help readers understand the dataset or dataset card. -->
[More Information Needed]
## More Information [optional]
[More Information Needed]
## Dataset Card Authors [optional]
[More Information Needed]
## Dataset Card Contact
[More Information Needed] | open-llm-leaderboard/details_dvilasuero__NeuralHermes-2.5-Mistral-7B-distilabel | [
"region:us"
] | 2024-01-10T14:19:54+00:00 | {"pretty_name": "Evaluation run of dvilasuero/NeuralHermes-2.5-Mistral-7B-distilabel", "dataset_summary": "Dataset automatically created during the evaluation run of model [dvilasuero/NeuralHermes-2.5-Mistral-7B-distilabel](https://huggingface.co/dvilasuero/NeuralHermes-2.5-Mistral-7B-distilabel) on the [Open LLM Leaderboard](https://huggingface.co/spaces/HuggingFaceH4/open_llm_leaderboard).\n\nThe dataset is composed of 63 configuration, each one coresponding to one of the evaluated task.\n\nThe dataset has been created from 1 run(s). Each run can be found as a specific split in each configuration, the split being named using the timestamp of the run.The \"train\" split is always pointing to the latest results.\n\nAn additional configuration \"results\" store all the aggregated results of the run (and is used to compute and display the aggregated metrics on the [Open LLM Leaderboard](https://huggingface.co/spaces/HuggingFaceH4/open_llm_leaderboard)).\n\nTo load the details from a run, you can for instance do the following:\n```python\nfrom datasets import load_dataset\ndata = load_dataset(\"open-llm-leaderboard/details_dvilasuero__NeuralHermes-2.5-Mistral-7B-distilabel\",\n\t\"harness_winogrande_5\",\n\tsplit=\"train\")\n```\n\n## Latest results\n\nThese are the [latest results from run 2024-01-10T14:17:38.745392](https://huggingface.co/datasets/open-llm-leaderboard/details_dvilasuero__NeuralHermes-2.5-Mistral-7B-distilabel/blob/main/results_2024-01-10T14-17-38.745392.json)(note that their might be results for other tasks in the repos if successive evals didn't cover the same tasks. You find each in the results and the \"latest\" split for each eval):\n\n```python\n{\n \"all\": {\n \"acc\": 0.6385164875113943,\n \"acc_stderr\": 0.032116569731227715,\n \"acc_norm\": 0.6402618412720931,\n \"acc_norm_stderr\": 0.032757857320627824,\n \"mc1\": 0.386780905752754,\n \"mc1_stderr\": 0.017048857010515107,\n \"mc2\": 0.5585768485275255,\n \"mc2_stderr\": 0.01538538971794349\n },\n \"harness|arc:challenge|25\": {\n \"acc\": 0.6194539249146758,\n \"acc_stderr\": 0.014188277712349812,\n \"acc_norm\": 0.6578498293515358,\n \"acc_norm_stderr\": 0.01386415215917728\n },\n \"harness|hellaswag|10\": {\n \"acc\": 0.660426209918343,\n \"acc_stderr\": 0.004725967684806406,\n \"acc_norm\": 0.8497311292571201,\n \"acc_norm_stderr\": 0.003566044777327419\n },\n \"harness|hendrycksTest-abstract_algebra|5\": {\n \"acc\": 0.29,\n \"acc_stderr\": 0.04560480215720684,\n \"acc_norm\": 0.29,\n \"acc_norm_stderr\": 0.04560480215720684\n },\n \"harness|hendrycksTest-anatomy|5\": {\n \"acc\": 0.5851851851851851,\n \"acc_stderr\": 0.04256193767901408,\n \"acc_norm\": 0.5851851851851851,\n \"acc_norm_stderr\": 0.04256193767901408\n },\n \"harness|hendrycksTest-astronomy|5\": {\n \"acc\": 0.6907894736842105,\n \"acc_stderr\": 0.037610708698674805,\n \"acc_norm\": 0.6907894736842105,\n \"acc_norm_stderr\": 0.037610708698674805\n },\n \"harness|hendrycksTest-business_ethics|5\": {\n \"acc\": 0.6,\n \"acc_stderr\": 0.04923659639173309,\n \"acc_norm\": 0.6,\n \"acc_norm_stderr\": 0.04923659639173309\n },\n \"harness|hendrycksTest-clinical_knowledge|5\": {\n \"acc\": 0.6754716981132075,\n \"acc_stderr\": 0.02881561571343211,\n \"acc_norm\": 0.6754716981132075,\n \"acc_norm_stderr\": 0.02881561571343211\n },\n \"harness|hendrycksTest-college_biology|5\": {\n \"acc\": 0.7777777777777778,\n \"acc_stderr\": 0.03476590104304133,\n \"acc_norm\": 0.7777777777777778,\n \"acc_norm_stderr\": 0.03476590104304133\n },\n \"harness|hendrycksTest-college_chemistry|5\": {\n \"acc\": 0.49,\n \"acc_stderr\": 0.05024183937956912,\n \"acc_norm\": 0.49,\n \"acc_norm_stderr\": 0.05024183937956912\n },\n \"harness|hendrycksTest-college_computer_science|5\": {\n \"acc\": 0.46,\n \"acc_stderr\": 0.05009082659620333,\n \"acc_norm\": 0.46,\n \"acc_norm_stderr\": 0.05009082659620333\n },\n \"harness|hendrycksTest-college_mathematics|5\": {\n \"acc\": 0.24,\n \"acc_stderr\": 0.04292346959909282,\n \"acc_norm\": 0.24,\n \"acc_norm_stderr\": 0.04292346959909282\n },\n \"harness|hendrycksTest-college_medicine|5\": {\n \"acc\": 0.6242774566473989,\n \"acc_stderr\": 0.036928207672648664,\n \"acc_norm\": 0.6242774566473989,\n \"acc_norm_stderr\": 0.036928207672648664\n },\n \"harness|hendrycksTest-college_physics|5\": {\n \"acc\": 0.3627450980392157,\n \"acc_stderr\": 0.04784060704105653,\n \"acc_norm\": 0.3627450980392157,\n \"acc_norm_stderr\": 0.04784060704105653\n },\n \"harness|hendrycksTest-computer_security|5\": {\n \"acc\": 0.76,\n \"acc_stderr\": 0.042923469599092816,\n \"acc_norm\": 0.76,\n \"acc_norm_stderr\": 0.042923469599092816\n },\n \"harness|hendrycksTest-conceptual_physics|5\": {\n \"acc\": 0.5404255319148936,\n \"acc_stderr\": 0.03257901482099835,\n \"acc_norm\": 0.5404255319148936,\n \"acc_norm_stderr\": 0.03257901482099835\n },\n \"harness|hendrycksTest-econometrics|5\": {\n \"acc\": 0.4649122807017544,\n \"acc_stderr\": 0.046920083813689104,\n \"acc_norm\": 0.4649122807017544,\n \"acc_norm_stderr\": 0.046920083813689104\n },\n \"harness|hendrycksTest-electrical_engineering|5\": {\n \"acc\": 0.5448275862068965,\n \"acc_stderr\": 0.04149886942192117,\n \"acc_norm\": 0.5448275862068965,\n \"acc_norm_stderr\": 0.04149886942192117\n },\n \"harness|hendrycksTest-elementary_mathematics|5\": {\n \"acc\": 0.4074074074074074,\n \"acc_stderr\": 0.02530590624159063,\n \"acc_norm\": 0.4074074074074074,\n \"acc_norm_stderr\": 0.02530590624159063\n },\n \"harness|hendrycksTest-formal_logic|5\": {\n \"acc\": 0.4523809523809524,\n \"acc_stderr\": 0.044518079590553275,\n \"acc_norm\": 0.4523809523809524,\n \"acc_norm_stderr\": 0.044518079590553275\n },\n \"harness|hendrycksTest-global_facts|5\": {\n \"acc\": 0.36,\n \"acc_stderr\": 0.04824181513244218,\n \"acc_norm\": 0.36,\n \"acc_norm_stderr\": 0.04824181513244218\n },\n \"harness|hendrycksTest-high_school_biology|5\": {\n \"acc\": 0.7935483870967742,\n \"acc_stderr\": 0.02302589961718871,\n \"acc_norm\": 0.7935483870967742,\n \"acc_norm_stderr\": 0.02302589961718871\n },\n \"harness|hendrycksTest-high_school_chemistry|5\": {\n \"acc\": 0.5172413793103449,\n \"acc_stderr\": 0.035158955511656986,\n \"acc_norm\": 0.5172413793103449,\n \"acc_norm_stderr\": 0.035158955511656986\n },\n \"harness|hendrycksTest-high_school_computer_science|5\": {\n \"acc\": 0.65,\n \"acc_stderr\": 0.047937248544110196,\n \"acc_norm\": 0.65,\n \"acc_norm_stderr\": 0.047937248544110196\n },\n \"harness|hendrycksTest-high_school_european_history|5\": {\n \"acc\": 0.7878787878787878,\n \"acc_stderr\": 0.03192271569548301,\n \"acc_norm\": 0.7878787878787878,\n \"acc_norm_stderr\": 0.03192271569548301\n },\n \"harness|hendrycksTest-high_school_geography|5\": {\n \"acc\": 0.7929292929292929,\n \"acc_stderr\": 0.02886977846026704,\n \"acc_norm\": 0.7929292929292929,\n \"acc_norm_stderr\": 0.02886977846026704\n },\n \"harness|hendrycksTest-high_school_government_and_politics|5\": {\n \"acc\": 0.8963730569948186,\n \"acc_stderr\": 0.02199531196364424,\n \"acc_norm\": 0.8963730569948186,\n \"acc_norm_stderr\": 0.02199531196364424\n },\n \"harness|hendrycksTest-high_school_macroeconomics|5\": {\n \"acc\": 0.6051282051282051,\n \"acc_stderr\": 0.0247843169421564,\n \"acc_norm\": 0.6051282051282051,\n \"acc_norm_stderr\": 0.0247843169421564\n },\n \"harness|hendrycksTest-high_school_mathematics|5\": {\n \"acc\": 0.3074074074074074,\n \"acc_stderr\": 0.02813325257881563,\n \"acc_norm\": 0.3074074074074074,\n \"acc_norm_stderr\": 0.02813325257881563\n },\n \"harness|hendrycksTest-high_school_microeconomics|5\": {\n \"acc\": 0.6722689075630253,\n \"acc_stderr\": 0.03048991141767323,\n \"acc_norm\": 0.6722689075630253,\n \"acc_norm_stderr\": 0.03048991141767323\n },\n \"harness|hendrycksTest-high_school_physics|5\": {\n \"acc\": 0.3443708609271523,\n \"acc_stderr\": 0.038796870240733264,\n \"acc_norm\": 0.3443708609271523,\n \"acc_norm_stderr\": 0.038796870240733264\n },\n \"harness|hendrycksTest-high_school_psychology|5\": {\n \"acc\": 0.8330275229357799,\n \"acc_stderr\": 0.015990154885073368,\n \"acc_norm\": 0.8330275229357799,\n \"acc_norm_stderr\": 0.015990154885073368\n },\n \"harness|hendrycksTest-high_school_statistics|5\": {\n \"acc\": 0.5,\n \"acc_stderr\": 0.034099716973523674,\n \"acc_norm\": 0.5,\n \"acc_norm_stderr\": 0.034099716973523674\n },\n \"harness|hendrycksTest-high_school_us_history|5\": {\n \"acc\": 0.8137254901960784,\n \"acc_stderr\": 0.027325470966716312,\n \"acc_norm\": 0.8137254901960784,\n \"acc_norm_stderr\": 0.027325470966716312\n },\n \"harness|hendrycksTest-high_school_world_history|5\": {\n \"acc\": 0.8059071729957806,\n \"acc_stderr\": 0.025744902532290913,\n \"acc_norm\": 0.8059071729957806,\n \"acc_norm_stderr\": 0.025744902532290913\n },\n \"harness|hendrycksTest-human_aging|5\": {\n \"acc\": 0.7040358744394619,\n \"acc_stderr\": 0.030636591348699803,\n \"acc_norm\": 0.7040358744394619,\n \"acc_norm_stderr\": 0.030636591348699803\n },\n \"harness|hendrycksTest-human_sexuality|5\": {\n \"acc\": 0.7786259541984732,\n \"acc_stderr\": 0.03641297081313728,\n \"acc_norm\": 0.7786259541984732,\n \"acc_norm_stderr\": 0.03641297081313728\n },\n \"harness|hendrycksTest-international_law|5\": {\n \"acc\": 0.7768595041322314,\n \"acc_stderr\": 0.03800754475228732,\n \"acc_norm\": 0.7768595041322314,\n \"acc_norm_stderr\": 0.03800754475228732\n },\n \"harness|hendrycksTest-jurisprudence|5\": {\n \"acc\": 0.8055555555555556,\n \"acc_stderr\": 0.038260763248848646,\n \"acc_norm\": 0.8055555555555556,\n \"acc_norm_stderr\": 0.038260763248848646\n },\n \"harness|hendrycksTest-logical_fallacies|5\": {\n \"acc\": 0.7914110429447853,\n \"acc_stderr\": 0.031921934489347235,\n \"acc_norm\": 0.7914110429447853,\n \"acc_norm_stderr\": 0.031921934489347235\n },\n \"harness|hendrycksTest-machine_learning|5\": {\n \"acc\": 0.5089285714285714,\n \"acc_stderr\": 0.04745033255489123,\n \"acc_norm\": 0.5089285714285714,\n \"acc_norm_stderr\": 0.04745033255489123\n },\n \"harness|hendrycksTest-management|5\": {\n \"acc\": 0.7864077669902912,\n \"acc_stderr\": 0.040580420156460344,\n \"acc_norm\": 0.7864077669902912,\n \"acc_norm_stderr\": 0.040580420156460344\n },\n \"harness|hendrycksTest-marketing|5\": {\n \"acc\": 0.8717948717948718,\n \"acc_stderr\": 0.02190190511507333,\n \"acc_norm\": 0.8717948717948718,\n \"acc_norm_stderr\": 0.02190190511507333\n },\n \"harness|hendrycksTest-medical_genetics|5\": {\n \"acc\": 0.69,\n \"acc_stderr\": 0.04648231987117316,\n \"acc_norm\": 0.69,\n \"acc_norm_stderr\": 0.04648231987117316\n },\n \"harness|hendrycksTest-miscellaneous|5\": {\n \"acc\": 0.8186462324393359,\n \"acc_stderr\": 0.013778693778464074,\n \"acc_norm\": 0.8186462324393359,\n \"acc_norm_stderr\": 0.013778693778464074\n },\n \"harness|hendrycksTest-moral_disputes|5\": {\n \"acc\": 0.7283236994219653,\n \"acc_stderr\": 0.023948512905468358,\n \"acc_norm\": 0.7283236994219653,\n \"acc_norm_stderr\": 0.023948512905468358\n },\n \"harness|hendrycksTest-moral_scenarios|5\": {\n \"acc\": 0.33519553072625696,\n \"acc_stderr\": 0.015788007190185884,\n \"acc_norm\": 0.33519553072625696,\n \"acc_norm_stderr\": 0.015788007190185884\n },\n \"harness|hendrycksTest-nutrition|5\": {\n \"acc\": 0.7483660130718954,\n \"acc_stderr\": 0.0248480182638752,\n \"acc_norm\": 0.7483660130718954,\n \"acc_norm_stderr\": 0.0248480182638752\n },\n \"harness|hendrycksTest-philosophy|5\": {\n \"acc\": 0.6945337620578779,\n \"acc_stderr\": 0.026160584450140446,\n \"acc_norm\": 0.6945337620578779,\n \"acc_norm_stderr\": 0.026160584450140446\n },\n \"harness|hendrycksTest-prehistory|5\": {\n \"acc\": 0.7438271604938271,\n \"acc_stderr\": 0.024288533637726095,\n \"acc_norm\": 0.7438271604938271,\n \"acc_norm_stderr\": 0.024288533637726095\n },\n \"harness|hendrycksTest-professional_accounting|5\": {\n \"acc\": 0.5177304964539007,\n \"acc_stderr\": 0.02980873964223777,\n \"acc_norm\": 0.5177304964539007,\n \"acc_norm_stderr\": 0.02980873964223777\n },\n \"harness|hendrycksTest-professional_law|5\": {\n \"acc\": 0.47783572359843546,\n \"acc_stderr\": 0.012757683047716175,\n \"acc_norm\": 0.47783572359843546,\n \"acc_norm_stderr\": 0.012757683047716175\n },\n \"harness|hendrycksTest-professional_medicine|5\": {\n \"acc\": 0.6654411764705882,\n \"acc_stderr\": 0.028661996202335303,\n \"acc_norm\": 0.6654411764705882,\n \"acc_norm_stderr\": 0.028661996202335303\n },\n \"harness|hendrycksTest-professional_psychology|5\": {\n \"acc\": 0.673202614379085,\n \"acc_stderr\": 0.018975427920507215,\n \"acc_norm\": 0.673202614379085,\n \"acc_norm_stderr\": 0.018975427920507215\n },\n \"harness|hendrycksTest-public_relations|5\": {\n \"acc\": 0.6636363636363637,\n \"acc_stderr\": 0.04525393596302506,\n \"acc_norm\": 0.6636363636363637,\n \"acc_norm_stderr\": 0.04525393596302506\n },\n \"harness|hendrycksTest-security_studies|5\": {\n \"acc\": 0.7591836734693878,\n \"acc_stderr\": 0.027372942201788163,\n \"acc_norm\": 0.7591836734693878,\n \"acc_norm_stderr\": 0.027372942201788163\n },\n \"harness|hendrycksTest-sociology|5\": {\n \"acc\": 0.835820895522388,\n \"acc_stderr\": 0.026193923544454115,\n \"acc_norm\": 0.835820895522388,\n \"acc_norm_stderr\": 0.026193923544454115\n },\n \"harness|hendrycksTest-us_foreign_policy|5\": {\n \"acc\": 0.85,\n \"acc_stderr\": 0.035887028128263686,\n \"acc_norm\": 0.85,\n \"acc_norm_stderr\": 0.035887028128263686\n },\n \"harness|hendrycksTest-virology|5\": {\n \"acc\": 0.5602409638554217,\n \"acc_stderr\": 0.03864139923699122,\n \"acc_norm\": 0.5602409638554217,\n \"acc_norm_stderr\": 0.03864139923699122\n },\n \"harness|hendrycksTest-world_religions|5\": {\n \"acc\": 0.8362573099415205,\n \"acc_stderr\": 0.028380919596145866,\n \"acc_norm\": 0.8362573099415205,\n \"acc_norm_stderr\": 0.028380919596145866\n },\n \"harness|truthfulqa:mc|0\": {\n \"mc1\": 0.386780905752754,\n \"mc1_stderr\": 0.017048857010515107,\n \"mc2\": 0.5585768485275255,\n \"mc2_stderr\": 0.01538538971794349\n },\n \"harness|winogrande|5\": {\n \"acc\": 0.7868981846882399,\n \"acc_stderr\": 0.011508957690722762\n },\n \"harness|gsm8k|5\": {\n \"acc\": 0.6148597422289613,\n \"acc_stderr\": 0.013404165536474303\n }\n}\n```", "repo_url": "https://huggingface.co/dvilasuero/NeuralHermes-2.5-Mistral-7B-distilabel", "leaderboard_url": "https://huggingface.co/spaces/HuggingFaceH4/open_llm_leaderboard", "point_of_contact": "[email protected]", "configs": [{"config_name": "harness_arc_challenge_25", "data_files": [{"split": "2024_01_10T14_17_38.745392", "path": ["**/details_harness|arc:challenge|25_2024-01-10T14-17-38.745392.parquet"]}, {"split": "latest", "path": ["**/details_harness|arc:challenge|25_2024-01-10T14-17-38.745392.parquet"]}]}, {"config_name": "harness_gsm8k_5", "data_files": [{"split": "2024_01_10T14_17_38.745392", "path": ["**/details_harness|gsm8k|5_2024-01-10T14-17-38.745392.parquet"]}, {"split": "latest", "path": ["**/details_harness|gsm8k|5_2024-01-10T14-17-38.745392.parquet"]}]}, {"config_name": "harness_hellaswag_10", "data_files": [{"split": "2024_01_10T14_17_38.745392", "path": ["**/details_harness|hellaswag|10_2024-01-10T14-17-38.745392.parquet"]}, {"split": "latest", "path": ["**/details_harness|hellaswag|10_2024-01-10T14-17-38.745392.parquet"]}]}, {"config_name": "harness_hendrycksTest_5", "data_files": [{"split": "2024_01_10T14_17_38.745392", "path": ["**/details_harness|hendrycksTest-abstract_algebra|5_2024-01-10T14-17-38.745392.parquet", "**/details_harness|hendrycksTest-anatomy|5_2024-01-10T14-17-38.745392.parquet", "**/details_harness|hendrycksTest-astronomy|5_2024-01-10T14-17-38.745392.parquet", "**/details_harness|hendrycksTest-business_ethics|5_2024-01-10T14-17-38.745392.parquet", "**/details_harness|hendrycksTest-clinical_knowledge|5_2024-01-10T14-17-38.745392.parquet", "**/details_harness|hendrycksTest-college_biology|5_2024-01-10T14-17-38.745392.parquet", "**/details_harness|hendrycksTest-college_chemistry|5_2024-01-10T14-17-38.745392.parquet", "**/details_harness|hendrycksTest-college_computer_science|5_2024-01-10T14-17-38.745392.parquet", "**/details_harness|hendrycksTest-college_mathematics|5_2024-01-10T14-17-38.745392.parquet", "**/details_harness|hendrycksTest-college_medicine|5_2024-01-10T14-17-38.745392.parquet", "**/details_harness|hendrycksTest-college_physics|5_2024-01-10T14-17-38.745392.parquet", "**/details_harness|hendrycksTest-computer_security|5_2024-01-10T14-17-38.745392.parquet", "**/details_harness|hendrycksTest-conceptual_physics|5_2024-01-10T14-17-38.745392.parquet", "**/details_harness|hendrycksTest-econometrics|5_2024-01-10T14-17-38.745392.parquet", "**/details_harness|hendrycksTest-electrical_engineering|5_2024-01-10T14-17-38.745392.parquet", "**/details_harness|hendrycksTest-elementary_mathematics|5_2024-01-10T14-17-38.745392.parquet", "**/details_harness|hendrycksTest-formal_logic|5_2024-01-10T14-17-38.745392.parquet", "**/details_harness|hendrycksTest-global_facts|5_2024-01-10T14-17-38.745392.parquet", "**/details_harness|hendrycksTest-high_school_biology|5_2024-01-10T14-17-38.745392.parquet", "**/details_harness|hendrycksTest-high_school_chemistry|5_2024-01-10T14-17-38.745392.parquet", "**/details_harness|hendrycksTest-high_school_computer_science|5_2024-01-10T14-17-38.745392.parquet", "**/details_harness|hendrycksTest-high_school_european_history|5_2024-01-10T14-17-38.745392.parquet", "**/details_harness|hendrycksTest-high_school_geography|5_2024-01-10T14-17-38.745392.parquet", "**/details_harness|hendrycksTest-high_school_government_and_politics|5_2024-01-10T14-17-38.745392.parquet", "**/details_harness|hendrycksTest-high_school_macroeconomics|5_2024-01-10T14-17-38.745392.parquet", "**/details_harness|hendrycksTest-high_school_mathematics|5_2024-01-10T14-17-38.745392.parquet", "**/details_harness|hendrycksTest-high_school_microeconomics|5_2024-01-10T14-17-38.745392.parquet", "**/details_harness|hendrycksTest-high_school_physics|5_2024-01-10T14-17-38.745392.parquet", "**/details_harness|hendrycksTest-high_school_psychology|5_2024-01-10T14-17-38.745392.parquet", "**/details_harness|hendrycksTest-high_school_statistics|5_2024-01-10T14-17-38.745392.parquet", "**/details_harness|hendrycksTest-high_school_us_history|5_2024-01-10T14-17-38.745392.parquet", "**/details_harness|hendrycksTest-high_school_world_history|5_2024-01-10T14-17-38.745392.parquet", "**/details_harness|hendrycksTest-human_aging|5_2024-01-10T14-17-38.745392.parquet", "**/details_harness|hendrycksTest-human_sexuality|5_2024-01-10T14-17-38.745392.parquet", "**/details_harness|hendrycksTest-international_law|5_2024-01-10T14-17-38.745392.parquet", "**/details_harness|hendrycksTest-jurisprudence|5_2024-01-10T14-17-38.745392.parquet", "**/details_harness|hendrycksTest-logical_fallacies|5_2024-01-10T14-17-38.745392.parquet", "**/details_harness|hendrycksTest-machine_learning|5_2024-01-10T14-17-38.745392.parquet", "**/details_harness|hendrycksTest-management|5_2024-01-10T14-17-38.745392.parquet", "**/details_harness|hendrycksTest-marketing|5_2024-01-10T14-17-38.745392.parquet", "**/details_harness|hendrycksTest-medical_genetics|5_2024-01-10T14-17-38.745392.parquet", "**/details_harness|hendrycksTest-miscellaneous|5_2024-01-10T14-17-38.745392.parquet", "**/details_harness|hendrycksTest-moral_disputes|5_2024-01-10T14-17-38.745392.parquet", "**/details_harness|hendrycksTest-moral_scenarios|5_2024-01-10T14-17-38.745392.parquet", "**/details_harness|hendrycksTest-nutrition|5_2024-01-10T14-17-38.745392.parquet", "**/details_harness|hendrycksTest-philosophy|5_2024-01-10T14-17-38.745392.parquet", "**/details_harness|hendrycksTest-prehistory|5_2024-01-10T14-17-38.745392.parquet", "**/details_harness|hendrycksTest-professional_accounting|5_2024-01-10T14-17-38.745392.parquet", "**/details_harness|hendrycksTest-professional_law|5_2024-01-10T14-17-38.745392.parquet", "**/details_harness|hendrycksTest-professional_medicine|5_2024-01-10T14-17-38.745392.parquet", "**/details_harness|hendrycksTest-professional_psychology|5_2024-01-10T14-17-38.745392.parquet", "**/details_harness|hendrycksTest-public_relations|5_2024-01-10T14-17-38.745392.parquet", "**/details_harness|hendrycksTest-security_studies|5_2024-01-10T14-17-38.745392.parquet", "**/details_harness|hendrycksTest-sociology|5_2024-01-10T14-17-38.745392.parquet", "**/details_harness|hendrycksTest-us_foreign_policy|5_2024-01-10T14-17-38.745392.parquet", "**/details_harness|hendrycksTest-virology|5_2024-01-10T14-17-38.745392.parquet", "**/details_harness|hendrycksTest-world_religions|5_2024-01-10T14-17-38.745392.parquet"]}, {"split": "latest", "path": ["**/details_harness|hendrycksTest-abstract_algebra|5_2024-01-10T14-17-38.745392.parquet", "**/details_harness|hendrycksTest-anatomy|5_2024-01-10T14-17-38.745392.parquet", "**/details_harness|hendrycksTest-astronomy|5_2024-01-10T14-17-38.745392.parquet", "**/details_harness|hendrycksTest-business_ethics|5_2024-01-10T14-17-38.745392.parquet", "**/details_harness|hendrycksTest-clinical_knowledge|5_2024-01-10T14-17-38.745392.parquet", "**/details_harness|hendrycksTest-college_biology|5_2024-01-10T14-17-38.745392.parquet", "**/details_harness|hendrycksTest-college_chemistry|5_2024-01-10T14-17-38.745392.parquet", "**/details_harness|hendrycksTest-college_computer_science|5_2024-01-10T14-17-38.745392.parquet", "**/details_harness|hendrycksTest-college_mathematics|5_2024-01-10T14-17-38.745392.parquet", "**/details_harness|hendrycksTest-college_medicine|5_2024-01-10T14-17-38.745392.parquet", "**/details_harness|hendrycksTest-college_physics|5_2024-01-10T14-17-38.745392.parquet", "**/details_harness|hendrycksTest-computer_security|5_2024-01-10T14-17-38.745392.parquet", "**/details_harness|hendrycksTest-conceptual_physics|5_2024-01-10T14-17-38.745392.parquet", "**/details_harness|hendrycksTest-econometrics|5_2024-01-10T14-17-38.745392.parquet", "**/details_harness|hendrycksTest-electrical_engineering|5_2024-01-10T14-17-38.745392.parquet", "**/details_harness|hendrycksTest-elementary_mathematics|5_2024-01-10T14-17-38.745392.parquet", "**/details_harness|hendrycksTest-formal_logic|5_2024-01-10T14-17-38.745392.parquet", "**/details_harness|hendrycksTest-global_facts|5_2024-01-10T14-17-38.745392.parquet", "**/details_harness|hendrycksTest-high_school_biology|5_2024-01-10T14-17-38.745392.parquet", "**/details_harness|hendrycksTest-high_school_chemistry|5_2024-01-10T14-17-38.745392.parquet", "**/details_harness|hendrycksTest-high_school_computer_science|5_2024-01-10T14-17-38.745392.parquet", "**/details_harness|hendrycksTest-high_school_european_history|5_2024-01-10T14-17-38.745392.parquet", "**/details_harness|hendrycksTest-high_school_geography|5_2024-01-10T14-17-38.745392.parquet", "**/details_harness|hendrycksTest-high_school_government_and_politics|5_2024-01-10T14-17-38.745392.parquet", "**/details_harness|hendrycksTest-high_school_macroeconomics|5_2024-01-10T14-17-38.745392.parquet", "**/details_harness|hendrycksTest-high_school_mathematics|5_2024-01-10T14-17-38.745392.parquet", "**/details_harness|hendrycksTest-high_school_microeconomics|5_2024-01-10T14-17-38.745392.parquet", "**/details_harness|hendrycksTest-high_school_physics|5_2024-01-10T14-17-38.745392.parquet", "**/details_harness|hendrycksTest-high_school_psychology|5_2024-01-10T14-17-38.745392.parquet", "**/details_harness|hendrycksTest-high_school_statistics|5_2024-01-10T14-17-38.745392.parquet", "**/details_harness|hendrycksTest-high_school_us_history|5_2024-01-10T14-17-38.745392.parquet", "**/details_harness|hendrycksTest-high_school_world_history|5_2024-01-10T14-17-38.745392.parquet", "**/details_harness|hendrycksTest-human_aging|5_2024-01-10T14-17-38.745392.parquet", "**/details_harness|hendrycksTest-human_sexuality|5_2024-01-10T14-17-38.745392.parquet", "**/details_harness|hendrycksTest-international_law|5_2024-01-10T14-17-38.745392.parquet", "**/details_harness|hendrycksTest-jurisprudence|5_2024-01-10T14-17-38.745392.parquet", "**/details_harness|hendrycksTest-logical_fallacies|5_2024-01-10T14-17-38.745392.parquet", "**/details_harness|hendrycksTest-machine_learning|5_2024-01-10T14-17-38.745392.parquet", "**/details_harness|hendrycksTest-management|5_2024-01-10T14-17-38.745392.parquet", "**/details_harness|hendrycksTest-marketing|5_2024-01-10T14-17-38.745392.parquet", "**/details_harness|hendrycksTest-medical_genetics|5_2024-01-10T14-17-38.745392.parquet", "**/details_harness|hendrycksTest-miscellaneous|5_2024-01-10T14-17-38.745392.parquet", "**/details_harness|hendrycksTest-moral_disputes|5_2024-01-10T14-17-38.745392.parquet", "**/details_harness|hendrycksTest-moral_scenarios|5_2024-01-10T14-17-38.745392.parquet", "**/details_harness|hendrycksTest-nutrition|5_2024-01-10T14-17-38.745392.parquet", "**/details_harness|hendrycksTest-philosophy|5_2024-01-10T14-17-38.745392.parquet", "**/details_harness|hendrycksTest-prehistory|5_2024-01-10T14-17-38.745392.parquet", "**/details_harness|hendrycksTest-professional_accounting|5_2024-01-10T14-17-38.745392.parquet", "**/details_harness|hendrycksTest-professional_law|5_2024-01-10T14-17-38.745392.parquet", "**/details_harness|hendrycksTest-professional_medicine|5_2024-01-10T14-17-38.745392.parquet", "**/details_harness|hendrycksTest-professional_psychology|5_2024-01-10T14-17-38.745392.parquet", "**/details_harness|hendrycksTest-public_relations|5_2024-01-10T14-17-38.745392.parquet", "**/details_harness|hendrycksTest-security_studies|5_2024-01-10T14-17-38.745392.parquet", "**/details_harness|hendrycksTest-sociology|5_2024-01-10T14-17-38.745392.parquet", "**/details_harness|hendrycksTest-us_foreign_policy|5_2024-01-10T14-17-38.745392.parquet", "**/details_harness|hendrycksTest-virology|5_2024-01-10T14-17-38.745392.parquet", "**/details_harness|hendrycksTest-world_religions|5_2024-01-10T14-17-38.745392.parquet"]}]}, {"config_name": "harness_hendrycksTest_abstract_algebra_5", "data_files": [{"split": "2024_01_10T14_17_38.745392", "path": ["**/details_harness|hendrycksTest-abstract_algebra|5_2024-01-10T14-17-38.745392.parquet"]}, {"split": "latest", "path": ["**/details_harness|hendrycksTest-abstract_algebra|5_2024-01-10T14-17-38.745392.parquet"]}]}, {"config_name": "harness_hendrycksTest_anatomy_5", "data_files": [{"split": "2024_01_10T14_17_38.745392", "path": ["**/details_harness|hendrycksTest-anatomy|5_2024-01-10T14-17-38.745392.parquet"]}, {"split": "latest", "path": ["**/details_harness|hendrycksTest-anatomy|5_2024-01-10T14-17-38.745392.parquet"]}]}, {"config_name": "harness_hendrycksTest_astronomy_5", "data_files": [{"split": "2024_01_10T14_17_38.745392", "path": ["**/details_harness|hendrycksTest-astronomy|5_2024-01-10T14-17-38.745392.parquet"]}, {"split": "latest", "path": ["**/details_harness|hendrycksTest-astronomy|5_2024-01-10T14-17-38.745392.parquet"]}]}, {"config_name": "harness_hendrycksTest_business_ethics_5", "data_files": [{"split": "2024_01_10T14_17_38.745392", "path": ["**/details_harness|hendrycksTest-business_ethics|5_2024-01-10T14-17-38.745392.parquet"]}, {"split": "latest", "path": ["**/details_harness|hendrycksTest-business_ethics|5_2024-01-10T14-17-38.745392.parquet"]}]}, {"config_name": "harness_hendrycksTest_clinical_knowledge_5", "data_files": [{"split": "2024_01_10T14_17_38.745392", "path": ["**/details_harness|hendrycksTest-clinical_knowledge|5_2024-01-10T14-17-38.745392.parquet"]}, {"split": "latest", "path": ["**/details_harness|hendrycksTest-clinical_knowledge|5_2024-01-10T14-17-38.745392.parquet"]}]}, {"config_name": "harness_hendrycksTest_college_biology_5", "data_files": [{"split": "2024_01_10T14_17_38.745392", "path": ["**/details_harness|hendrycksTest-college_biology|5_2024-01-10T14-17-38.745392.parquet"]}, {"split": "latest", "path": ["**/details_harness|hendrycksTest-college_biology|5_2024-01-10T14-17-38.745392.parquet"]}]}, {"config_name": "harness_hendrycksTest_college_chemistry_5", "data_files": [{"split": "2024_01_10T14_17_38.745392", "path": ["**/details_harness|hendrycksTest-college_chemistry|5_2024-01-10T14-17-38.745392.parquet"]}, {"split": "latest", "path": ["**/details_harness|hendrycksTest-college_chemistry|5_2024-01-10T14-17-38.745392.parquet"]}]}, {"config_name": "harness_hendrycksTest_college_computer_science_5", "data_files": [{"split": "2024_01_10T14_17_38.745392", "path": ["**/details_harness|hendrycksTest-college_computer_science|5_2024-01-10T14-17-38.745392.parquet"]}, {"split": "latest", "path": ["**/details_harness|hendrycksTest-college_computer_science|5_2024-01-10T14-17-38.745392.parquet"]}]}, {"config_name": "harness_hendrycksTest_college_mathematics_5", "data_files": [{"split": "2024_01_10T14_17_38.745392", "path": ["**/details_harness|hendrycksTest-college_mathematics|5_2024-01-10T14-17-38.745392.parquet"]}, {"split": "latest", "path": ["**/details_harness|hendrycksTest-college_mathematics|5_2024-01-10T14-17-38.745392.parquet"]}]}, {"config_name": "harness_hendrycksTest_college_medicine_5", "data_files": [{"split": "2024_01_10T14_17_38.745392", "path": ["**/details_harness|hendrycksTest-college_medicine|5_2024-01-10T14-17-38.745392.parquet"]}, {"split": "latest", "path": ["**/details_harness|hendrycksTest-college_medicine|5_2024-01-10T14-17-38.745392.parquet"]}]}, {"config_name": "harness_hendrycksTest_college_physics_5", "data_files": [{"split": "2024_01_10T14_17_38.745392", "path": ["**/details_harness|hendrycksTest-college_physics|5_2024-01-10T14-17-38.745392.parquet"]}, {"split": "latest", "path": ["**/details_harness|hendrycksTest-college_physics|5_2024-01-10T14-17-38.745392.parquet"]}]}, {"config_name": "harness_hendrycksTest_computer_security_5", "data_files": [{"split": "2024_01_10T14_17_38.745392", "path": ["**/details_harness|hendrycksTest-computer_security|5_2024-01-10T14-17-38.745392.parquet"]}, {"split": "latest", "path": ["**/details_harness|hendrycksTest-computer_security|5_2024-01-10T14-17-38.745392.parquet"]}]}, {"config_name": "harness_hendrycksTest_conceptual_physics_5", "data_files": [{"split": "2024_01_10T14_17_38.745392", "path": ["**/details_harness|hendrycksTest-conceptual_physics|5_2024-01-10T14-17-38.745392.parquet"]}, {"split": "latest", "path": ["**/details_harness|hendrycksTest-conceptual_physics|5_2024-01-10T14-17-38.745392.parquet"]}]}, {"config_name": "harness_hendrycksTest_econometrics_5", "data_files": [{"split": "2024_01_10T14_17_38.745392", "path": ["**/details_harness|hendrycksTest-econometrics|5_2024-01-10T14-17-38.745392.parquet"]}, {"split": "latest", "path": ["**/details_harness|hendrycksTest-econometrics|5_2024-01-10T14-17-38.745392.parquet"]}]}, {"config_name": "harness_hendrycksTest_electrical_engineering_5", "data_files": [{"split": "2024_01_10T14_17_38.745392", "path": ["**/details_harness|hendrycksTest-electrical_engineering|5_2024-01-10T14-17-38.745392.parquet"]}, {"split": "latest", "path": ["**/details_harness|hendrycksTest-electrical_engineering|5_2024-01-10T14-17-38.745392.parquet"]}]}, {"config_name": "harness_hendrycksTest_elementary_mathematics_5", "data_files": [{"split": "2024_01_10T14_17_38.745392", "path": ["**/details_harness|hendrycksTest-elementary_mathematics|5_2024-01-10T14-17-38.745392.parquet"]}, {"split": "latest", "path": ["**/details_harness|hendrycksTest-elementary_mathematics|5_2024-01-10T14-17-38.745392.parquet"]}]}, {"config_name": "harness_hendrycksTest_formal_logic_5", "data_files": [{"split": "2024_01_10T14_17_38.745392", "path": ["**/details_harness|hendrycksTest-formal_logic|5_2024-01-10T14-17-38.745392.parquet"]}, {"split": "latest", "path": ["**/details_harness|hendrycksTest-formal_logic|5_2024-01-10T14-17-38.745392.parquet"]}]}, {"config_name": "harness_hendrycksTest_global_facts_5", "data_files": [{"split": "2024_01_10T14_17_38.745392", "path": ["**/details_harness|hendrycksTest-global_facts|5_2024-01-10T14-17-38.745392.parquet"]}, {"split": "latest", "path": ["**/details_harness|hendrycksTest-global_facts|5_2024-01-10T14-17-38.745392.parquet"]}]}, {"config_name": "harness_hendrycksTest_high_school_biology_5", "data_files": [{"split": "2024_01_10T14_17_38.745392", "path": ["**/details_harness|hendrycksTest-high_school_biology|5_2024-01-10T14-17-38.745392.parquet"]}, {"split": "latest", "path": ["**/details_harness|hendrycksTest-high_school_biology|5_2024-01-10T14-17-38.745392.parquet"]}]}, {"config_name": "harness_hendrycksTest_high_school_chemistry_5", "data_files": [{"split": "2024_01_10T14_17_38.745392", "path": ["**/details_harness|hendrycksTest-high_school_chemistry|5_2024-01-10T14-17-38.745392.parquet"]}, {"split": "latest", "path": ["**/details_harness|hendrycksTest-high_school_chemistry|5_2024-01-10T14-17-38.745392.parquet"]}]}, {"config_name": "harness_hendrycksTest_high_school_computer_science_5", "data_files": [{"split": "2024_01_10T14_17_38.745392", "path": ["**/details_harness|hendrycksTest-high_school_computer_science|5_2024-01-10T14-17-38.745392.parquet"]}, {"split": "latest", "path": ["**/details_harness|hendrycksTest-high_school_computer_science|5_2024-01-10T14-17-38.745392.parquet"]}]}, {"config_name": "harness_hendrycksTest_high_school_european_history_5", "data_files": [{"split": "2024_01_10T14_17_38.745392", "path": ["**/details_harness|hendrycksTest-high_school_european_history|5_2024-01-10T14-17-38.745392.parquet"]}, {"split": "latest", "path": ["**/details_harness|hendrycksTest-high_school_european_history|5_2024-01-10T14-17-38.745392.parquet"]}]}, {"config_name": "harness_hendrycksTest_high_school_geography_5", "data_files": [{"split": "2024_01_10T14_17_38.745392", "path": ["**/details_harness|hendrycksTest-high_school_geography|5_2024-01-10T14-17-38.745392.parquet"]}, {"split": "latest", "path": ["**/details_harness|hendrycksTest-high_school_geography|5_2024-01-10T14-17-38.745392.parquet"]}]}, {"config_name": "harness_hendrycksTest_high_school_government_and_politics_5", "data_files": [{"split": "2024_01_10T14_17_38.745392", "path": ["**/details_harness|hendrycksTest-high_school_government_and_politics|5_2024-01-10T14-17-38.745392.parquet"]}, {"split": "latest", "path": ["**/details_harness|hendrycksTest-high_school_government_and_politics|5_2024-01-10T14-17-38.745392.parquet"]}]}, {"config_name": "harness_hendrycksTest_high_school_macroeconomics_5", "data_files": [{"split": "2024_01_10T14_17_38.745392", "path": ["**/details_harness|hendrycksTest-high_school_macroeconomics|5_2024-01-10T14-17-38.745392.parquet"]}, {"split": "latest", "path": ["**/details_harness|hendrycksTest-high_school_macroeconomics|5_2024-01-10T14-17-38.745392.parquet"]}]}, {"config_name": "harness_hendrycksTest_high_school_mathematics_5", "data_files": [{"split": "2024_01_10T14_17_38.745392", "path": ["**/details_harness|hendrycksTest-high_school_mathematics|5_2024-01-10T14-17-38.745392.parquet"]}, {"split": "latest", "path": ["**/details_harness|hendrycksTest-high_school_mathematics|5_2024-01-10T14-17-38.745392.parquet"]}]}, {"config_name": "harness_hendrycksTest_high_school_microeconomics_5", "data_files": [{"split": "2024_01_10T14_17_38.745392", "path": ["**/details_harness|hendrycksTest-high_school_microeconomics|5_2024-01-10T14-17-38.745392.parquet"]}, {"split": "latest", "path": ["**/details_harness|hendrycksTest-high_school_microeconomics|5_2024-01-10T14-17-38.745392.parquet"]}]}, {"config_name": "harness_hendrycksTest_high_school_physics_5", "data_files": [{"split": "2024_01_10T14_17_38.745392", "path": ["**/details_harness|hendrycksTest-high_school_physics|5_2024-01-10T14-17-38.745392.parquet"]}, {"split": "latest", "path": ["**/details_harness|hendrycksTest-high_school_physics|5_2024-01-10T14-17-38.745392.parquet"]}]}, {"config_name": "harness_hendrycksTest_high_school_psychology_5", "data_files": [{"split": "2024_01_10T14_17_38.745392", "path": ["**/details_harness|hendrycksTest-high_school_psychology|5_2024-01-10T14-17-38.745392.parquet"]}, {"split": "latest", "path": ["**/details_harness|hendrycksTest-high_school_psychology|5_2024-01-10T14-17-38.745392.parquet"]}]}, {"config_name": "harness_hendrycksTest_high_school_statistics_5", "data_files": [{"split": "2024_01_10T14_17_38.745392", "path": ["**/details_harness|hendrycksTest-high_school_statistics|5_2024-01-10T14-17-38.745392.parquet"]}, {"split": "latest", "path": ["**/details_harness|hendrycksTest-high_school_statistics|5_2024-01-10T14-17-38.745392.parquet"]}]}, {"config_name": "harness_hendrycksTest_high_school_us_history_5", "data_files": [{"split": "2024_01_10T14_17_38.745392", "path": ["**/details_harness|hendrycksTest-high_school_us_history|5_2024-01-10T14-17-38.745392.parquet"]}, {"split": "latest", "path": ["**/details_harness|hendrycksTest-high_school_us_history|5_2024-01-10T14-17-38.745392.parquet"]}]}, {"config_name": "harness_hendrycksTest_high_school_world_history_5", "data_files": [{"split": "2024_01_10T14_17_38.745392", "path": ["**/details_harness|hendrycksTest-high_school_world_history|5_2024-01-10T14-17-38.745392.parquet"]}, {"split": "latest", "path": ["**/details_harness|hendrycksTest-high_school_world_history|5_2024-01-10T14-17-38.745392.parquet"]}]}, {"config_name": "harness_hendrycksTest_human_aging_5", "data_files": [{"split": "2024_01_10T14_17_38.745392", "path": ["**/details_harness|hendrycksTest-human_aging|5_2024-01-10T14-17-38.745392.parquet"]}, {"split": "latest", "path": ["**/details_harness|hendrycksTest-human_aging|5_2024-01-10T14-17-38.745392.parquet"]}]}, {"config_name": "harness_hendrycksTest_human_sexuality_5", "data_files": [{"split": "2024_01_10T14_17_38.745392", "path": ["**/details_harness|hendrycksTest-human_sexuality|5_2024-01-10T14-17-38.745392.parquet"]}, {"split": "latest", "path": ["**/details_harness|hendrycksTest-human_sexuality|5_2024-01-10T14-17-38.745392.parquet"]}]}, {"config_name": "harness_hendrycksTest_international_law_5", "data_files": [{"split": "2024_01_10T14_17_38.745392", "path": ["**/details_harness|hendrycksTest-international_law|5_2024-01-10T14-17-38.745392.parquet"]}, {"split": "latest", "path": ["**/details_harness|hendrycksTest-international_law|5_2024-01-10T14-17-38.745392.parquet"]}]}, {"config_name": "harness_hendrycksTest_jurisprudence_5", "data_files": [{"split": "2024_01_10T14_17_38.745392", "path": ["**/details_harness|hendrycksTest-jurisprudence|5_2024-01-10T14-17-38.745392.parquet"]}, {"split": "latest", "path": ["**/details_harness|hendrycksTest-jurisprudence|5_2024-01-10T14-17-38.745392.parquet"]}]}, {"config_name": "harness_hendrycksTest_logical_fallacies_5", "data_files": [{"split": "2024_01_10T14_17_38.745392", "path": ["**/details_harness|hendrycksTest-logical_fallacies|5_2024-01-10T14-17-38.745392.parquet"]}, {"split": "latest", "path": ["**/details_harness|hendrycksTest-logical_fallacies|5_2024-01-10T14-17-38.745392.parquet"]}]}, {"config_name": "harness_hendrycksTest_machine_learning_5", "data_files": [{"split": "2024_01_10T14_17_38.745392", "path": ["**/details_harness|hendrycksTest-machine_learning|5_2024-01-10T14-17-38.745392.parquet"]}, {"split": "latest", "path": ["**/details_harness|hendrycksTest-machine_learning|5_2024-01-10T14-17-38.745392.parquet"]}]}, {"config_name": "harness_hendrycksTest_management_5", "data_files": [{"split": "2024_01_10T14_17_38.745392", "path": ["**/details_harness|hendrycksTest-management|5_2024-01-10T14-17-38.745392.parquet"]}, {"split": "latest", "path": ["**/details_harness|hendrycksTest-management|5_2024-01-10T14-17-38.745392.parquet"]}]}, {"config_name": "harness_hendrycksTest_marketing_5", "data_files": [{"split": "2024_01_10T14_17_38.745392", "path": ["**/details_harness|hendrycksTest-marketing|5_2024-01-10T14-17-38.745392.parquet"]}, {"split": "latest", "path": ["**/details_harness|hendrycksTest-marketing|5_2024-01-10T14-17-38.745392.parquet"]}]}, {"config_name": "harness_hendrycksTest_medical_genetics_5", "data_files": [{"split": "2024_01_10T14_17_38.745392", "path": ["**/details_harness|hendrycksTest-medical_genetics|5_2024-01-10T14-17-38.745392.parquet"]}, {"split": "latest", "path": ["**/details_harness|hendrycksTest-medical_genetics|5_2024-01-10T14-17-38.745392.parquet"]}]}, {"config_name": "harness_hendrycksTest_miscellaneous_5", "data_files": [{"split": "2024_01_10T14_17_38.745392", "path": ["**/details_harness|hendrycksTest-miscellaneous|5_2024-01-10T14-17-38.745392.parquet"]}, {"split": "latest", "path": ["**/details_harness|hendrycksTest-miscellaneous|5_2024-01-10T14-17-38.745392.parquet"]}]}, {"config_name": "harness_hendrycksTest_moral_disputes_5", "data_files": [{"split": "2024_01_10T14_17_38.745392", "path": ["**/details_harness|hendrycksTest-moral_disputes|5_2024-01-10T14-17-38.745392.parquet"]}, {"split": "latest", "path": ["**/details_harness|hendrycksTest-moral_disputes|5_2024-01-10T14-17-38.745392.parquet"]}]}, {"config_name": "harness_hendrycksTest_moral_scenarios_5", "data_files": [{"split": "2024_01_10T14_17_38.745392", "path": ["**/details_harness|hendrycksTest-moral_scenarios|5_2024-01-10T14-17-38.745392.parquet"]}, {"split": "latest", "path": ["**/details_harness|hendrycksTest-moral_scenarios|5_2024-01-10T14-17-38.745392.parquet"]}]}, {"config_name": "harness_hendrycksTest_nutrition_5", "data_files": [{"split": "2024_01_10T14_17_38.745392", "path": ["**/details_harness|hendrycksTest-nutrition|5_2024-01-10T14-17-38.745392.parquet"]}, {"split": "latest", "path": ["**/details_harness|hendrycksTest-nutrition|5_2024-01-10T14-17-38.745392.parquet"]}]}, {"config_name": "harness_hendrycksTest_philosophy_5", "data_files": [{"split": "2024_01_10T14_17_38.745392", "path": ["**/details_harness|hendrycksTest-philosophy|5_2024-01-10T14-17-38.745392.parquet"]}, {"split": "latest", "path": ["**/details_harness|hendrycksTest-philosophy|5_2024-01-10T14-17-38.745392.parquet"]}]}, {"config_name": "harness_hendrycksTest_prehistory_5", "data_files": [{"split": "2024_01_10T14_17_38.745392", "path": ["**/details_harness|hendrycksTest-prehistory|5_2024-01-10T14-17-38.745392.parquet"]}, {"split": "latest", "path": ["**/details_harness|hendrycksTest-prehistory|5_2024-01-10T14-17-38.745392.parquet"]}]}, {"config_name": "harness_hendrycksTest_professional_accounting_5", "data_files": [{"split": "2024_01_10T14_17_38.745392", "path": ["**/details_harness|hendrycksTest-professional_accounting|5_2024-01-10T14-17-38.745392.parquet"]}, {"split": "latest", "path": ["**/details_harness|hendrycksTest-professional_accounting|5_2024-01-10T14-17-38.745392.parquet"]}]}, {"config_name": "harness_hendrycksTest_professional_law_5", "data_files": [{"split": "2024_01_10T14_17_38.745392", "path": ["**/details_harness|hendrycksTest-professional_law|5_2024-01-10T14-17-38.745392.parquet"]}, {"split": "latest", "path": ["**/details_harness|hendrycksTest-professional_law|5_2024-01-10T14-17-38.745392.parquet"]}]}, {"config_name": "harness_hendrycksTest_professional_medicine_5", "data_files": [{"split": "2024_01_10T14_17_38.745392", "path": ["**/details_harness|hendrycksTest-professional_medicine|5_2024-01-10T14-17-38.745392.parquet"]}, {"split": "latest", "path": ["**/details_harness|hendrycksTest-professional_medicine|5_2024-01-10T14-17-38.745392.parquet"]}]}, {"config_name": "harness_hendrycksTest_professional_psychology_5", "data_files": [{"split": "2024_01_10T14_17_38.745392", "path": ["**/details_harness|hendrycksTest-professional_psychology|5_2024-01-10T14-17-38.745392.parquet"]}, {"split": "latest", "path": ["**/details_harness|hendrycksTest-professional_psychology|5_2024-01-10T14-17-38.745392.parquet"]}]}, {"config_name": "harness_hendrycksTest_public_relations_5", "data_files": [{"split": "2024_01_10T14_17_38.745392", "path": ["**/details_harness|hendrycksTest-public_relations|5_2024-01-10T14-17-38.745392.parquet"]}, {"split": "latest", "path": ["**/details_harness|hendrycksTest-public_relations|5_2024-01-10T14-17-38.745392.parquet"]}]}, {"config_name": "harness_hendrycksTest_security_studies_5", "data_files": [{"split": "2024_01_10T14_17_38.745392", "path": ["**/details_harness|hendrycksTest-security_studies|5_2024-01-10T14-17-38.745392.parquet"]}, {"split": "latest", "path": ["**/details_harness|hendrycksTest-security_studies|5_2024-01-10T14-17-38.745392.parquet"]}]}, {"config_name": "harness_hendrycksTest_sociology_5", "data_files": [{"split": "2024_01_10T14_17_38.745392", "path": ["**/details_harness|hendrycksTest-sociology|5_2024-01-10T14-17-38.745392.parquet"]}, {"split": "latest", "path": ["**/details_harness|hendrycksTest-sociology|5_2024-01-10T14-17-38.745392.parquet"]}]}, {"config_name": "harness_hendrycksTest_us_foreign_policy_5", "data_files": [{"split": "2024_01_10T14_17_38.745392", "path": ["**/details_harness|hendrycksTest-us_foreign_policy|5_2024-01-10T14-17-38.745392.parquet"]}, {"split": "latest", "path": ["**/details_harness|hendrycksTest-us_foreign_policy|5_2024-01-10T14-17-38.745392.parquet"]}]}, {"config_name": "harness_hendrycksTest_virology_5", "data_files": [{"split": "2024_01_10T14_17_38.745392", "path": ["**/details_harness|hendrycksTest-virology|5_2024-01-10T14-17-38.745392.parquet"]}, {"split": "latest", "path": ["**/details_harness|hendrycksTest-virology|5_2024-01-10T14-17-38.745392.parquet"]}]}, {"config_name": "harness_hendrycksTest_world_religions_5", "data_files": [{"split": "2024_01_10T14_17_38.745392", "path": ["**/details_harness|hendrycksTest-world_religions|5_2024-01-10T14-17-38.745392.parquet"]}, {"split": "latest", "path": ["**/details_harness|hendrycksTest-world_religions|5_2024-01-10T14-17-38.745392.parquet"]}]}, {"config_name": "harness_truthfulqa_mc_0", "data_files": [{"split": "2024_01_10T14_17_38.745392", "path": ["**/details_harness|truthfulqa:mc|0_2024-01-10T14-17-38.745392.parquet"]}, {"split": "latest", "path": ["**/details_harness|truthfulqa:mc|0_2024-01-10T14-17-38.745392.parquet"]}]}, {"config_name": "harness_winogrande_5", "data_files": [{"split": "2024_01_10T14_17_38.745392", "path": ["**/details_harness|winogrande|5_2024-01-10T14-17-38.745392.parquet"]}, {"split": "latest", "path": ["**/details_harness|winogrande|5_2024-01-10T14-17-38.745392.parquet"]}]}, {"config_name": "results", "data_files": [{"split": "2024_01_10T14_17_38.745392", "path": ["results_2024-01-10T14-17-38.745392.parquet"]}, {"split": "latest", "path": ["results_2024-01-10T14-17-38.745392.parquet"]}]}]} | 2024-01-10T14:20:19+00:00 | [] | [] | TAGS
#region-us
|
# Dataset Card for Evaluation run of dvilasuero/NeuralHermes-2.5-Mistral-7B-distilabel
Dataset automatically created during the evaluation run of model dvilasuero/NeuralHermes-2.5-Mistral-7B-distilabel on the Open LLM Leaderboard.
The dataset is composed of 63 configuration, each one coresponding to one of the evaluated task.
The dataset has been created from 1 run(s). Each run can be found as a specific split in each configuration, the split being named using the timestamp of the run.The "train" split is always pointing to the latest results.
An additional configuration "results" store all the aggregated results of the run (and is used to compute and display the aggregated metrics on the Open LLM Leaderboard).
To load the details from a run, you can for instance do the following:
## Latest results
These are the latest results from run 2024-01-10T14:17:38.745392(note that their might be results for other tasks in the repos if successive evals didn't cover the same tasks. You find each in the results and the "latest" split for each eval):
## Dataset Details
### Dataset Description
- Curated by:
- Funded by [optional]:
- Shared by [optional]:
- Language(s) (NLP):
- License:
### Dataset Sources [optional]
- Repository:
- Paper [optional]:
- Demo [optional]:
## Uses
### Direct Use
### Out-of-Scope Use
## Dataset Structure
## Dataset Creation
### Curation Rationale
### Source Data
#### Data Collection and Processing
#### Who are the source data producers?
### Annotations [optional]
#### Annotation process
#### Who are the annotators?
#### Personal and Sensitive Information
## Bias, Risks, and Limitations
### Recommendations
Users should be made aware of the risks, biases and limitations of the dataset. More information needed for further recommendations.
[optional]
BibTeX:
APA:
## Glossary [optional]
## More Information [optional]
## Dataset Card Authors [optional]
## Dataset Card Contact
| [
"# Dataset Card for Evaluation run of dvilasuero/NeuralHermes-2.5-Mistral-7B-distilabel\n\n\n\nDataset automatically created during the evaluation run of model dvilasuero/NeuralHermes-2.5-Mistral-7B-distilabel on the Open LLM Leaderboard.\n\nThe dataset is composed of 63 configuration, each one coresponding to one of the evaluated task.\n\nThe dataset has been created from 1 run(s). Each run can be found as a specific split in each configuration, the split being named using the timestamp of the run.The \"train\" split is always pointing to the latest results.\n\nAn additional configuration \"results\" store all the aggregated results of the run (and is used to compute and display the aggregated metrics on the Open LLM Leaderboard).\n\nTo load the details from a run, you can for instance do the following:",
"## Latest results\n\nThese are the latest results from run 2024-01-10T14:17:38.745392(note that their might be results for other tasks in the repos if successive evals didn't cover the same tasks. You find each in the results and the \"latest\" split for each eval):",
"## Dataset Details",
"### Dataset Description\n\n\n\n\n\n- Curated by: \n- Funded by [optional]: \n- Shared by [optional]: \n- Language(s) (NLP): \n- License:",
"### Dataset Sources [optional]\n\n\n\n- Repository: \n- Paper [optional]: \n- Demo [optional]:",
"## Uses",
"### Direct Use",
"### Out-of-Scope Use",
"## Dataset Structure",
"## Dataset Creation",
"### Curation Rationale",
"### Source Data",
"#### Data Collection and Processing",
"#### Who are the source data producers?",
"### Annotations [optional]",
"#### Annotation process",
"#### Who are the annotators?",
"#### Personal and Sensitive Information",
"## Bias, Risks, and Limitations",
"### Recommendations\n\n\n\nUsers should be made aware of the risks, biases and limitations of the dataset. More information needed for further recommendations.\n\n[optional]\n\n\n\nBibTeX:\n\n\n\nAPA:",
"## Glossary [optional]",
"## More Information [optional]",
"## Dataset Card Authors [optional]",
"## Dataset Card Contact"
] | [
"TAGS\n#region-us \n",
"# Dataset Card for Evaluation run of dvilasuero/NeuralHermes-2.5-Mistral-7B-distilabel\n\n\n\nDataset automatically created during the evaluation run of model dvilasuero/NeuralHermes-2.5-Mistral-7B-distilabel on the Open LLM Leaderboard.\n\nThe dataset is composed of 63 configuration, each one coresponding to one of the evaluated task.\n\nThe dataset has been created from 1 run(s). Each run can be found as a specific split in each configuration, the split being named using the timestamp of the run.The \"train\" split is always pointing to the latest results.\n\nAn additional configuration \"results\" store all the aggregated results of the run (and is used to compute and display the aggregated metrics on the Open LLM Leaderboard).\n\nTo load the details from a run, you can for instance do the following:",
"## Latest results\n\nThese are the latest results from run 2024-01-10T14:17:38.745392(note that their might be results for other tasks in the repos if successive evals didn't cover the same tasks. You find each in the results and the \"latest\" split for each eval):",
"## Dataset Details",
"### Dataset Description\n\n\n\n\n\n- Curated by: \n- Funded by [optional]: \n- Shared by [optional]: \n- Language(s) (NLP): \n- License:",
"### Dataset Sources [optional]\n\n\n\n- Repository: \n- Paper [optional]: \n- Demo [optional]:",
"## Uses",
"### Direct Use",
"### Out-of-Scope Use",
"## Dataset Structure",
"## Dataset Creation",
"### Curation Rationale",
"### Source Data",
"#### Data Collection and Processing",
"#### Who are the source data producers?",
"### Annotations [optional]",
"#### Annotation process",
"#### Who are the annotators?",
"#### Personal and Sensitive Information",
"## Bias, Risks, and Limitations",
"### Recommendations\n\n\n\nUsers should be made aware of the risks, biases and limitations of the dataset. More information needed for further recommendations.\n\n[optional]\n\n\n\nBibTeX:\n\n\n\nAPA:",
"## Glossary [optional]",
"## More Information [optional]",
"## Dataset Card Authors [optional]",
"## Dataset Card Contact"
] |
febbd55d7108180dff28dcec0a6b82647b35d4be | # Medical-Calgary-Cambridge-multi-turn-llama2-37
37 multi-turn conversational entries between a patient and doctor, using the Calgary-Cambridge model
| kazcfz/Medical-Calgary-Cambridge-multi-turn-llama2-37 | [
"region:us"
] | 2024-01-10T14:34:11+00:00 | {} | 2024-01-11T10:53:51+00:00 | [] | [] | TAGS
#region-us
| # Medical-Calgary-Cambridge-multi-turn-llama2-37
37 multi-turn conversational entries between a patient and doctor, using the Calgary-Cambridge model
| [
"# Medical-Calgary-Cambridge-multi-turn-llama2-37\n37 multi-turn conversational entries between a patient and doctor, using the Calgary-Cambridge model"
] | [
"TAGS\n#region-us \n",
"# Medical-Calgary-Cambridge-multi-turn-llama2-37\n37 multi-turn conversational entries between a patient and doctor, using the Calgary-Cambridge model"
] |
a841aa521ca2867fb8077ebac151985708ee4677 |
# these are the transcript files from the 09 01 2024 Tonic AI Community Discord
## Summary
# technology
- recorded by clyde
- transcribed by gladia
# Open Tasks :
- create a summary of the transcription
- automate summary of the transcriptions for tonic ai | Tonic/Tonic-AI-Transcript-1-09-2024 | [
"task_categories:conversational",
"size_categories:1K<n<10K",
"language:en",
"license:apache-2.0",
"not-for-all-audiences",
"region:us"
] | 2024-01-10T14:38:09+00:00 | {"language": ["en"], "license": "apache-2.0", "size_categories": ["1K<n<10K"], "task_categories": ["conversational"], "pretty_name": "Tonic AI Transcripts 09 01 2024", "tags": ["not-for-all-audiences"]} | 2024-01-10T15:16:01+00:00 | [] | [
"en"
] | TAGS
#task_categories-conversational #size_categories-1K<n<10K #language-English #license-apache-2.0 #not-for-all-audiences #region-us
|
# these are the transcript files from the 09 01 2024 Tonic AI Community Discord
## Summary
# technology
- recorded by clyde
- transcribed by gladia
# Open Tasks :
- create a summary of the transcription
- automate summary of the transcriptions for tonic ai | [
"# these are the transcript files from the 09 01 2024 Tonic AI Community Discord",
"## Summary",
"# technology\n- recorded by clyde\n- transcribed by gladia",
"# Open Tasks :\n- create a summary of the transcription\n- automate summary of the transcriptions for tonic ai"
] | [
"TAGS\n#task_categories-conversational #size_categories-1K<n<10K #language-English #license-apache-2.0 #not-for-all-audiences #region-us \n",
"# these are the transcript files from the 09 01 2024 Tonic AI Community Discord",
"## Summary",
"# technology\n- recorded by clyde\n- transcribed by gladia",
"# Open Tasks :\n- create a summary of the transcription\n- automate summary of the transcriptions for tonic ai"
] |
ea3f70b969d29c826ed49f91b032012f8224c209 |
# Esposalles Dataset
## Table of Contents
- [Esposalles Dataset](#esposalles-dataset)
- [Table of Contents](#table-of-contents)
- [Dataset Description](#dataset-description)
- [Dataset Summary](#dataset-summary)
- [Languages](#languages)
- [Dataset Structure](#dataset-structure)
- [Data Instances](#data-instances)
- [Data Fields](#data-fields)
## Dataset Description
- **Homepage:** [The Esposalles Database](http://dag.cvc.uab.es/the-esposalles-database/)
- **Source:** [IEHHR](https://rrc.cvc.uab.es/?ch=10&com=evaluation&task=1)
- **Paper:** [The ESPOSALLES database: An ancient marriage license corpus for off-line handwriting recognition](https://doi.org/10.1016/j.patcog.2012.11.024)
- **Point of Contact:** [TEKLIA](https://teklia.com)
## Dataset Summary
The Marriage Licenses ground-truth is compiled from the Marriage Licenses Books conserved at the Archives of the Cathedral of Barcelona.
### Languages
All the documents in the dataset are written in Catalan.
## Dataset Structure
### Data Instances
```
{
'image': <PIL.JpegImagePlugin.JpegImageFile image mode=RGB size=1244x128 at 0x1A800E8E190,
'text': 'donsella filla de Onofre Esquer morraler de Bara y'
}
```
### Data Fields
- `image`: A PIL.Image.Image object containing the image. Note that when accessing the image column: dataset[0]["image"] the image file is automatically decoded. Decoding of a large number of image files might take a significant amount of time. Thus it is important to first query the sample index before the "image" column, i.e. dataset[0]["image"] should always be preferred over dataset["image"][0].
- `text`: the label transcription of the image.
| Teklia/esposalles | [
"task_categories:image-to-text",
"language:ca",
"license:mit",
"region:us"
] | 2024-01-10T14:42:13+00:00 | {"language": ["ca"], "license": "mit", "task_categories": ["image-to-text"], "pretty_name": "Esposalles", "dataset_info": {"features": [{"name": "image", "dtype": "image"}, {"name": "text", "dtype": "string"}], "splits": [{"name": "train", "num_examples": 2328}, {"name": "validation", "num_examples": 742}, {"name": "test", "num_examples": 757}], "dataset_size": 3827}} | 2024-01-26T14:38:40+00:00 | [] | [
"ca"
] | TAGS
#task_categories-image-to-text #language-Catalan #license-mit #region-us
|
# Esposalles Dataset
## Table of Contents
- Esposalles Dataset
- Table of Contents
- Dataset Description
- Dataset Summary
- Languages
- Dataset Structure
- Data Instances
- Data Fields
## Dataset Description
- Homepage: The Esposalles Database
- Source: IEHHR
- Paper: The ESPOSALLES database: An ancient marriage license corpus for off-line handwriting recognition
- Point of Contact: TEKLIA
## Dataset Summary
The Marriage Licenses ground-truth is compiled from the Marriage Licenses Books conserved at the Archives of the Cathedral of Barcelona.
### Languages
All the documents in the dataset are written in Catalan.
## Dataset Structure
### Data Instances
### Data Fields
- 'image': A PIL.Image.Image object containing the image. Note that when accessing the image column: dataset[0]["image"] the image file is automatically decoded. Decoding of a large number of image files might take a significant amount of time. Thus it is important to first query the sample index before the "image" column, i.e. dataset[0]["image"] should always be preferred over dataset["image"][0].
- 'text': the label transcription of the image.
| [
"# Esposalles Dataset",
"## Table of Contents\n- Esposalles Dataset\n - Table of Contents\n - Dataset Description\n - Dataset Summary\n - Languages\n - Dataset Structure\n - Data Instances\n - Data Fields",
"## Dataset Description\n\n- Homepage: The Esposalles Database\n- Source: IEHHR\n- Paper: The ESPOSALLES database: An ancient marriage license corpus for off-line handwriting recognition\n- Point of Contact: TEKLIA",
"## Dataset Summary\n\nThe Marriage Licenses ground-truth is compiled from the Marriage Licenses Books conserved at the Archives of the Cathedral of Barcelona.",
"### Languages\n\nAll the documents in the dataset are written in Catalan.",
"## Dataset Structure",
"### Data Instances",
"### Data Fields\n\n\n- 'image': A PIL.Image.Image object containing the image. Note that when accessing the image column: dataset[0][\"image\"] the image file is automatically decoded. Decoding of a large number of image files might take a significant amount of time. Thus it is important to first query the sample index before the \"image\" column, i.e. dataset[0][\"image\"] should always be preferred over dataset[\"image\"][0].\n- 'text': the label transcription of the image."
] | [
"TAGS\n#task_categories-image-to-text #language-Catalan #license-mit #region-us \n",
"# Esposalles Dataset",
"## Table of Contents\n- Esposalles Dataset\n - Table of Contents\n - Dataset Description\n - Dataset Summary\n - Languages\n - Dataset Structure\n - Data Instances\n - Data Fields",
"## Dataset Description\n\n- Homepage: The Esposalles Database\n- Source: IEHHR\n- Paper: The ESPOSALLES database: An ancient marriage license corpus for off-line handwriting recognition\n- Point of Contact: TEKLIA",
"## Dataset Summary\n\nThe Marriage Licenses ground-truth is compiled from the Marriage Licenses Books conserved at the Archives of the Cathedral of Barcelona.",
"### Languages\n\nAll the documents in the dataset are written in Catalan.",
"## Dataset Structure",
"### Data Instances",
"### Data Fields\n\n\n- 'image': A PIL.Image.Image object containing the image. Note that when accessing the image column: dataset[0][\"image\"] the image file is automatically decoded. Decoding of a large number of image files might take a significant amount of time. Thus it is important to first query the sample index before the \"image\" column, i.e. dataset[0][\"image\"] should always be preferred over dataset[\"image\"][0].\n- 'text': the label transcription of the image."
] |
ac7b13a03e121ea9f708d5c7fd9c578f4f4791e9 |
The Typly Email Dataset contains 5,000 emails in Polish, collected from offices. The messages have been anonymised and pre-processed by [Typly](https://typly.app/). | Typly/the_typly_email_dataset | [
"language:pl",
"license:mit",
"region:us"
] | 2024-01-10T14:51:50+00:00 | {"language": ["pl"], "license": "mit", "pretty_name": "The Typly Email Dataset"} | 2024-01-10T15:01:04+00:00 | [] | [
"pl"
] | TAGS
#language-Polish #license-mit #region-us
|
The Typly Email Dataset contains 5,000 emails in Polish, collected from offices. The messages have been anonymised and pre-processed by Typly. | [] | [
"TAGS\n#language-Polish #license-mit #region-us \n"
] |
ba0646ce840ff64ec3e39d62ed3c7d77c141dcaf |
# Dataset Card for Evaluation run of ewqr2130/mistral-7b-raw-sft
<!-- Provide a quick summary of the dataset. -->
Dataset automatically created during the evaluation run of model [ewqr2130/mistral-7b-raw-sft](https://huggingface.co/ewqr2130/mistral-7b-raw-sft) on the [Open LLM Leaderboard](https://huggingface.co/spaces/HuggingFaceH4/open_llm_leaderboard).
The dataset is composed of 63 configuration, each one coresponding to one of the evaluated task.
The dataset has been created from 1 run(s). Each run can be found as a specific split in each configuration, the split being named using the timestamp of the run.The "train" split is always pointing to the latest results.
An additional configuration "results" store all the aggregated results of the run (and is used to compute and display the aggregated metrics on the [Open LLM Leaderboard](https://huggingface.co/spaces/HuggingFaceH4/open_llm_leaderboard)).
To load the details from a run, you can for instance do the following:
```python
from datasets import load_dataset
data = load_dataset("open-llm-leaderboard/details_ewqr2130__mistral-7b-raw-sft",
"harness_winogrande_5",
split="train")
```
## Latest results
These are the [latest results from run 2024-01-10T15:14:57.972449](https://huggingface.co/datasets/open-llm-leaderboard/details_ewqr2130__mistral-7b-raw-sft/blob/main/results_2024-01-10T15-14-57.972449.json)(note that their might be results for other tasks in the repos if successive evals didn't cover the same tasks. You find each in the results and the "latest" split for each eval):
```python
{
"all": {
"acc": 0.3451686041304389,
"acc_stderr": 0.033177024770114395,
"acc_norm": 0.34794617103590064,
"acc_norm_stderr": 0.033992606612009306,
"mc1": 0.2521419828641371,
"mc1_stderr": 0.015201522246299963,
"mc2": 0.4077071941467522,
"mc2_stderr": 0.014214727907656348
},
"harness|arc:challenge|25": {
"acc": 0.43430034129692835,
"acc_stderr": 0.01448470304885736,
"acc_norm": 0.47440273037542663,
"acc_norm_stderr": 0.014592230885298964
},
"harness|hellaswag|10": {
"acc": 0.5518820952001593,
"acc_stderr": 0.004962846206125493,
"acc_norm": 0.7525393347938658,
"acc_norm_stderr": 0.004306547156331412
},
"harness|hendrycksTest-abstract_algebra|5": {
"acc": 0.26,
"acc_stderr": 0.04408440022768081,
"acc_norm": 0.26,
"acc_norm_stderr": 0.04408440022768081
},
"harness|hendrycksTest-anatomy|5": {
"acc": 0.37037037037037035,
"acc_stderr": 0.04171654161354543,
"acc_norm": 0.37037037037037035,
"acc_norm_stderr": 0.04171654161354543
},
"harness|hendrycksTest-astronomy|5": {
"acc": 0.28289473684210525,
"acc_stderr": 0.03665349695640767,
"acc_norm": 0.28289473684210525,
"acc_norm_stderr": 0.03665349695640767
},
"harness|hendrycksTest-business_ethics|5": {
"acc": 0.37,
"acc_stderr": 0.04852365870939099,
"acc_norm": 0.37,
"acc_norm_stderr": 0.04852365870939099
},
"harness|hendrycksTest-clinical_knowledge|5": {
"acc": 0.41509433962264153,
"acc_stderr": 0.03032594578928611,
"acc_norm": 0.41509433962264153,
"acc_norm_stderr": 0.03032594578928611
},
"harness|hendrycksTest-college_biology|5": {
"acc": 0.3402777777777778,
"acc_stderr": 0.039621355734862175,
"acc_norm": 0.3402777777777778,
"acc_norm_stderr": 0.039621355734862175
},
"harness|hendrycksTest-college_chemistry|5": {
"acc": 0.23,
"acc_stderr": 0.04229525846816505,
"acc_norm": 0.23,
"acc_norm_stderr": 0.04229525846816505
},
"harness|hendrycksTest-college_computer_science|5": {
"acc": 0.27,
"acc_stderr": 0.04461960433384741,
"acc_norm": 0.27,
"acc_norm_stderr": 0.04461960433384741
},
"harness|hendrycksTest-college_mathematics|5": {
"acc": 0.22,
"acc_stderr": 0.041633319989322695,
"acc_norm": 0.22,
"acc_norm_stderr": 0.041633319989322695
},
"harness|hendrycksTest-college_medicine|5": {
"acc": 0.32947976878612717,
"acc_stderr": 0.035839017547364106,
"acc_norm": 0.32947976878612717,
"acc_norm_stderr": 0.035839017547364106
},
"harness|hendrycksTest-college_physics|5": {
"acc": 0.19607843137254902,
"acc_stderr": 0.03950581861179961,
"acc_norm": 0.19607843137254902,
"acc_norm_stderr": 0.03950581861179961
},
"harness|hendrycksTest-computer_security|5": {
"acc": 0.34,
"acc_stderr": 0.04760952285695235,
"acc_norm": 0.34,
"acc_norm_stderr": 0.04760952285695235
},
"harness|hendrycksTest-conceptual_physics|5": {
"acc": 0.32340425531914896,
"acc_stderr": 0.030579442773610334,
"acc_norm": 0.32340425531914896,
"acc_norm_stderr": 0.030579442773610334
},
"harness|hendrycksTest-econometrics|5": {
"acc": 0.2631578947368421,
"acc_stderr": 0.041424397194893624,
"acc_norm": 0.2631578947368421,
"acc_norm_stderr": 0.041424397194893624
},
"harness|hendrycksTest-electrical_engineering|5": {
"acc": 0.2413793103448276,
"acc_stderr": 0.03565998174135302,
"acc_norm": 0.2413793103448276,
"acc_norm_stderr": 0.03565998174135302
},
"harness|hendrycksTest-elementary_mathematics|5": {
"acc": 0.2566137566137566,
"acc_stderr": 0.022494510767503154,
"acc_norm": 0.2566137566137566,
"acc_norm_stderr": 0.022494510767503154
},
"harness|hendrycksTest-formal_logic|5": {
"acc": 0.1746031746031746,
"acc_stderr": 0.03395490020856112,
"acc_norm": 0.1746031746031746,
"acc_norm_stderr": 0.03395490020856112
},
"harness|hendrycksTest-global_facts|5": {
"acc": 0.33,
"acc_stderr": 0.047258156262526045,
"acc_norm": 0.33,
"acc_norm_stderr": 0.047258156262526045
},
"harness|hendrycksTest-high_school_biology|5": {
"acc": 0.4258064516129032,
"acc_stderr": 0.0281291127091659,
"acc_norm": 0.4258064516129032,
"acc_norm_stderr": 0.0281291127091659
},
"harness|hendrycksTest-high_school_chemistry|5": {
"acc": 0.3103448275862069,
"acc_stderr": 0.032550867699701024,
"acc_norm": 0.3103448275862069,
"acc_norm_stderr": 0.032550867699701024
},
"harness|hendrycksTest-high_school_computer_science|5": {
"acc": 0.28,
"acc_stderr": 0.045126085985421276,
"acc_norm": 0.28,
"acc_norm_stderr": 0.045126085985421276
},
"harness|hendrycksTest-high_school_european_history|5": {
"acc": 0.44242424242424244,
"acc_stderr": 0.03878372113711275,
"acc_norm": 0.44242424242424244,
"acc_norm_stderr": 0.03878372113711275
},
"harness|hendrycksTest-high_school_geography|5": {
"acc": 0.35858585858585856,
"acc_stderr": 0.03416903640391521,
"acc_norm": 0.35858585858585856,
"acc_norm_stderr": 0.03416903640391521
},
"harness|hendrycksTest-high_school_government_and_politics|5": {
"acc": 0.49222797927461137,
"acc_stderr": 0.036080032255696545,
"acc_norm": 0.49222797927461137,
"acc_norm_stderr": 0.036080032255696545
},
"harness|hendrycksTest-high_school_macroeconomics|5": {
"acc": 0.3384615384615385,
"acc_stderr": 0.02399150050031304,
"acc_norm": 0.3384615384615385,
"acc_norm_stderr": 0.02399150050031304
},
"harness|hendrycksTest-high_school_mathematics|5": {
"acc": 0.26296296296296295,
"acc_stderr": 0.02684205787383371,
"acc_norm": 0.26296296296296295,
"acc_norm_stderr": 0.02684205787383371
},
"harness|hendrycksTest-high_school_microeconomics|5": {
"acc": 0.36134453781512604,
"acc_stderr": 0.031204691225150013,
"acc_norm": 0.36134453781512604,
"acc_norm_stderr": 0.031204691225150013
},
"harness|hendrycksTest-high_school_physics|5": {
"acc": 0.33112582781456956,
"acc_stderr": 0.038425817186598696,
"acc_norm": 0.33112582781456956,
"acc_norm_stderr": 0.038425817186598696
},
"harness|hendrycksTest-high_school_psychology|5": {
"acc": 0.3724770642201835,
"acc_stderr": 0.020728368457638494,
"acc_norm": 0.3724770642201835,
"acc_norm_stderr": 0.020728368457638494
},
"harness|hendrycksTest-high_school_statistics|5": {
"acc": 0.46296296296296297,
"acc_stderr": 0.03400603625538272,
"acc_norm": 0.46296296296296297,
"acc_norm_stderr": 0.03400603625538272
},
"harness|hendrycksTest-high_school_us_history|5": {
"acc": 0.4019607843137255,
"acc_stderr": 0.034411900234824655,
"acc_norm": 0.4019607843137255,
"acc_norm_stderr": 0.034411900234824655
},
"harness|hendrycksTest-high_school_world_history|5": {
"acc": 0.3755274261603376,
"acc_stderr": 0.03152256243091156,
"acc_norm": 0.3755274261603376,
"acc_norm_stderr": 0.03152256243091156
},
"harness|hendrycksTest-human_aging|5": {
"acc": 0.33183856502242154,
"acc_stderr": 0.031602951437766785,
"acc_norm": 0.33183856502242154,
"acc_norm_stderr": 0.031602951437766785
},
"harness|hendrycksTest-human_sexuality|5": {
"acc": 0.35877862595419846,
"acc_stderr": 0.04206739313864908,
"acc_norm": 0.35877862595419846,
"acc_norm_stderr": 0.04206739313864908
},
"harness|hendrycksTest-international_law|5": {
"acc": 0.39669421487603307,
"acc_stderr": 0.04465869780531009,
"acc_norm": 0.39669421487603307,
"acc_norm_stderr": 0.04465869780531009
},
"harness|hendrycksTest-jurisprudence|5": {
"acc": 0.3611111111111111,
"acc_stderr": 0.04643454608906275,
"acc_norm": 0.3611111111111111,
"acc_norm_stderr": 0.04643454608906275
},
"harness|hendrycksTest-logical_fallacies|5": {
"acc": 0.3312883435582822,
"acc_stderr": 0.03697983910025588,
"acc_norm": 0.3312883435582822,
"acc_norm_stderr": 0.03697983910025588
},
"harness|hendrycksTest-machine_learning|5": {
"acc": 0.17857142857142858,
"acc_stderr": 0.036352091215778065,
"acc_norm": 0.17857142857142858,
"acc_norm_stderr": 0.036352091215778065
},
"harness|hendrycksTest-management|5": {
"acc": 0.36893203883495146,
"acc_stderr": 0.04777615181156739,
"acc_norm": 0.36893203883495146,
"acc_norm_stderr": 0.04777615181156739
},
"harness|hendrycksTest-marketing|5": {
"acc": 0.5085470085470085,
"acc_stderr": 0.0327513030009703,
"acc_norm": 0.5085470085470085,
"acc_norm_stderr": 0.0327513030009703
},
"harness|hendrycksTest-medical_genetics|5": {
"acc": 0.33,
"acc_stderr": 0.04725815626252604,
"acc_norm": 0.33,
"acc_norm_stderr": 0.04725815626252604
},
"harness|hendrycksTest-miscellaneous|5": {
"acc": 0.4240102171136654,
"acc_stderr": 0.017672263329084226,
"acc_norm": 0.4240102171136654,
"acc_norm_stderr": 0.017672263329084226
},
"harness|hendrycksTest-moral_disputes|5": {
"acc": 0.2543352601156069,
"acc_stderr": 0.023445826276545543,
"acc_norm": 0.2543352601156069,
"acc_norm_stderr": 0.023445826276545543
},
"harness|hendrycksTest-moral_scenarios|5": {
"acc": 0.2424581005586592,
"acc_stderr": 0.014333522059217889,
"acc_norm": 0.2424581005586592,
"acc_norm_stderr": 0.014333522059217889
},
"harness|hendrycksTest-nutrition|5": {
"acc": 0.4117647058823529,
"acc_stderr": 0.028180596328259293,
"acc_norm": 0.4117647058823529,
"acc_norm_stderr": 0.028180596328259293
},
"harness|hendrycksTest-philosophy|5": {
"acc": 0.34726688102893893,
"acc_stderr": 0.027040745502307336,
"acc_norm": 0.34726688102893893,
"acc_norm_stderr": 0.027040745502307336
},
"harness|hendrycksTest-prehistory|5": {
"acc": 0.31790123456790126,
"acc_stderr": 0.02591006352824088,
"acc_norm": 0.31790123456790126,
"acc_norm_stderr": 0.02591006352824088
},
"harness|hendrycksTest-professional_accounting|5": {
"acc": 0.28368794326241137,
"acc_stderr": 0.02689170942834396,
"acc_norm": 0.28368794326241137,
"acc_norm_stderr": 0.02689170942834396
},
"harness|hendrycksTest-professional_law|5": {
"acc": 0.2940026075619296,
"acc_stderr": 0.011636062953698604,
"acc_norm": 0.2940026075619296,
"acc_norm_stderr": 0.011636062953698604
},
"harness|hendrycksTest-professional_medicine|5": {
"acc": 0.4632352941176471,
"acc_stderr": 0.030290619180485687,
"acc_norm": 0.4632352941176471,
"acc_norm_stderr": 0.030290619180485687
},
"harness|hendrycksTest-professional_psychology|5": {
"acc": 0.28431372549019607,
"acc_stderr": 0.018249024411207668,
"acc_norm": 0.28431372549019607,
"acc_norm_stderr": 0.018249024411207668
},
"harness|hendrycksTest-public_relations|5": {
"acc": 0.42727272727272725,
"acc_stderr": 0.04738198703545483,
"acc_norm": 0.42727272727272725,
"acc_norm_stderr": 0.04738198703545483
},
"harness|hendrycksTest-security_studies|5": {
"acc": 0.3877551020408163,
"acc_stderr": 0.031192230726795656,
"acc_norm": 0.3877551020408163,
"acc_norm_stderr": 0.031192230726795656
},
"harness|hendrycksTest-sociology|5": {
"acc": 0.43283582089552236,
"acc_stderr": 0.03503490923673281,
"acc_norm": 0.43283582089552236,
"acc_norm_stderr": 0.03503490923673281
},
"harness|hendrycksTest-us_foreign_policy|5": {
"acc": 0.4,
"acc_stderr": 0.04923659639173309,
"acc_norm": 0.4,
"acc_norm_stderr": 0.04923659639173309
},
"harness|hendrycksTest-virology|5": {
"acc": 0.3614457831325301,
"acc_stderr": 0.0374005938202932,
"acc_norm": 0.3614457831325301,
"acc_norm_stderr": 0.0374005938202932
},
"harness|hendrycksTest-world_religions|5": {
"acc": 0.3742690058479532,
"acc_stderr": 0.03711601185389481,
"acc_norm": 0.3742690058479532,
"acc_norm_stderr": 0.03711601185389481
},
"harness|truthfulqa:mc|0": {
"mc1": 0.2521419828641371,
"mc1_stderr": 0.015201522246299963,
"mc2": 0.4077071941467522,
"mc2_stderr": 0.014214727907656348
},
"harness|winogrande|5": {
"acc": 0.7300710339384373,
"acc_stderr": 0.012476433372002608
},
"harness|gsm8k|5": {
"acc": 0.037149355572403335,
"acc_stderr": 0.005209516283073736
}
}
```
## Dataset Details
### Dataset Description
<!-- Provide a longer summary of what this dataset is. -->
- **Curated by:** [More Information Needed]
- **Funded by [optional]:** [More Information Needed]
- **Shared by [optional]:** [More Information Needed]
- **Language(s) (NLP):** [More Information Needed]
- **License:** [More Information Needed]
### Dataset Sources [optional]
<!-- Provide the basic links for the dataset. -->
- **Repository:** [More Information Needed]
- **Paper [optional]:** [More Information Needed]
- **Demo [optional]:** [More Information Needed]
## Uses
<!-- Address questions around how the dataset is intended to be used. -->
### Direct Use
<!-- This section describes suitable use cases for the dataset. -->
[More Information Needed]
### Out-of-Scope Use
<!-- This section addresses misuse, malicious use, and uses that the dataset will not work well for. -->
[More Information Needed]
## Dataset Structure
<!-- This section provides a description of the dataset fields, and additional information about the dataset structure such as criteria used to create the splits, relationships between data points, etc. -->
[More Information Needed]
## Dataset Creation
### Curation Rationale
<!-- Motivation for the creation of this dataset. -->
[More Information Needed]
### Source Data
<!-- This section describes the source data (e.g. news text and headlines, social media posts, translated sentences, ...). -->
#### Data Collection and Processing
<!-- This section describes the data collection and processing process such as data selection criteria, filtering and normalization methods, tools and libraries used, etc. -->
[More Information Needed]
#### Who are the source data producers?
<!-- This section describes the people or systems who originally created the data. It should also include self-reported demographic or identity information for the source data creators if this information is available. -->
[More Information Needed]
### Annotations [optional]
<!-- If the dataset contains annotations which are not part of the initial data collection, use this section to describe them. -->
#### Annotation process
<!-- This section describes the annotation process such as annotation tools used in the process, the amount of data annotated, annotation guidelines provided to the annotators, interannotator statistics, annotation validation, etc. -->
[More Information Needed]
#### Who are the annotators?
<!-- This section describes the people or systems who created the annotations. -->
[More Information Needed]
#### Personal and Sensitive Information
<!-- State whether the dataset contains data that might be considered personal, sensitive, or private (e.g., data that reveals addresses, uniquely identifiable names or aliases, racial or ethnic origins, sexual orientations, religious beliefs, political opinions, financial or health data, etc.). If efforts were made to anonymize the data, describe the anonymization process. -->
[More Information Needed]
## Bias, Risks, and Limitations
<!-- This section is meant to convey both technical and sociotechnical limitations. -->
[More Information Needed]
### Recommendations
<!-- This section is meant to convey recommendations with respect to the bias, risk, and technical limitations. -->
Users should be made aware of the risks, biases and limitations of the dataset. More information needed for further recommendations.
## Citation [optional]
<!-- If there is a paper or blog post introducing the dataset, the APA and Bibtex information for that should go in this section. -->
**BibTeX:**
[More Information Needed]
**APA:**
[More Information Needed]
## Glossary [optional]
<!-- If relevant, include terms and calculations in this section that can help readers understand the dataset or dataset card. -->
[More Information Needed]
## More Information [optional]
[More Information Needed]
## Dataset Card Authors [optional]
[More Information Needed]
## Dataset Card Contact
[More Information Needed] | open-llm-leaderboard/details_ewqr2130__mistral-7b-raw-sft | [
"region:us"
] | 2024-01-10T15:17:19+00:00 | {"pretty_name": "Evaluation run of ewqr2130/mistral-7b-raw-sft", "dataset_summary": "Dataset automatically created during the evaluation run of model [ewqr2130/mistral-7b-raw-sft](https://huggingface.co/ewqr2130/mistral-7b-raw-sft) on the [Open LLM Leaderboard](https://huggingface.co/spaces/HuggingFaceH4/open_llm_leaderboard).\n\nThe dataset is composed of 63 configuration, each one coresponding to one of the evaluated task.\n\nThe dataset has been created from 1 run(s). Each run can be found as a specific split in each configuration, the split being named using the timestamp of the run.The \"train\" split is always pointing to the latest results.\n\nAn additional configuration \"results\" store all the aggregated results of the run (and is used to compute and display the aggregated metrics on the [Open LLM Leaderboard](https://huggingface.co/spaces/HuggingFaceH4/open_llm_leaderboard)).\n\nTo load the details from a run, you can for instance do the following:\n```python\nfrom datasets import load_dataset\ndata = load_dataset(\"open-llm-leaderboard/details_ewqr2130__mistral-7b-raw-sft\",\n\t\"harness_winogrande_5\",\n\tsplit=\"train\")\n```\n\n## Latest results\n\nThese are the [latest results from run 2024-01-10T15:14:57.972449](https://huggingface.co/datasets/open-llm-leaderboard/details_ewqr2130__mistral-7b-raw-sft/blob/main/results_2024-01-10T15-14-57.972449.json)(note that their might be results for other tasks in the repos if successive evals didn't cover the same tasks. You find each in the results and the \"latest\" split for each eval):\n\n```python\n{\n \"all\": {\n \"acc\": 0.3451686041304389,\n \"acc_stderr\": 0.033177024770114395,\n \"acc_norm\": 0.34794617103590064,\n \"acc_norm_stderr\": 0.033992606612009306,\n \"mc1\": 0.2521419828641371,\n \"mc1_stderr\": 0.015201522246299963,\n \"mc2\": 0.4077071941467522,\n \"mc2_stderr\": 0.014214727907656348\n },\n \"harness|arc:challenge|25\": {\n \"acc\": 0.43430034129692835,\n \"acc_stderr\": 0.01448470304885736,\n \"acc_norm\": 0.47440273037542663,\n \"acc_norm_stderr\": 0.014592230885298964\n },\n \"harness|hellaswag|10\": {\n \"acc\": 0.5518820952001593,\n \"acc_stderr\": 0.004962846206125493,\n \"acc_norm\": 0.7525393347938658,\n \"acc_norm_stderr\": 0.004306547156331412\n },\n \"harness|hendrycksTest-abstract_algebra|5\": {\n \"acc\": 0.26,\n \"acc_stderr\": 0.04408440022768081,\n \"acc_norm\": 0.26,\n \"acc_norm_stderr\": 0.04408440022768081\n },\n \"harness|hendrycksTest-anatomy|5\": {\n \"acc\": 0.37037037037037035,\n \"acc_stderr\": 0.04171654161354543,\n \"acc_norm\": 0.37037037037037035,\n \"acc_norm_stderr\": 0.04171654161354543\n },\n \"harness|hendrycksTest-astronomy|5\": {\n \"acc\": 0.28289473684210525,\n \"acc_stderr\": 0.03665349695640767,\n \"acc_norm\": 0.28289473684210525,\n \"acc_norm_stderr\": 0.03665349695640767\n },\n \"harness|hendrycksTest-business_ethics|5\": {\n \"acc\": 0.37,\n \"acc_stderr\": 0.04852365870939099,\n \"acc_norm\": 0.37,\n \"acc_norm_stderr\": 0.04852365870939099\n },\n \"harness|hendrycksTest-clinical_knowledge|5\": {\n \"acc\": 0.41509433962264153,\n \"acc_stderr\": 0.03032594578928611,\n \"acc_norm\": 0.41509433962264153,\n \"acc_norm_stderr\": 0.03032594578928611\n },\n \"harness|hendrycksTest-college_biology|5\": {\n \"acc\": 0.3402777777777778,\n \"acc_stderr\": 0.039621355734862175,\n \"acc_norm\": 0.3402777777777778,\n \"acc_norm_stderr\": 0.039621355734862175\n },\n \"harness|hendrycksTest-college_chemistry|5\": {\n \"acc\": 0.23,\n \"acc_stderr\": 0.04229525846816505,\n \"acc_norm\": 0.23,\n \"acc_norm_stderr\": 0.04229525846816505\n },\n \"harness|hendrycksTest-college_computer_science|5\": {\n \"acc\": 0.27,\n \"acc_stderr\": 0.04461960433384741,\n \"acc_norm\": 0.27,\n \"acc_norm_stderr\": 0.04461960433384741\n },\n \"harness|hendrycksTest-college_mathematics|5\": {\n \"acc\": 0.22,\n \"acc_stderr\": 0.041633319989322695,\n \"acc_norm\": 0.22,\n \"acc_norm_stderr\": 0.041633319989322695\n },\n \"harness|hendrycksTest-college_medicine|5\": {\n \"acc\": 0.32947976878612717,\n \"acc_stderr\": 0.035839017547364106,\n \"acc_norm\": 0.32947976878612717,\n \"acc_norm_stderr\": 0.035839017547364106\n },\n \"harness|hendrycksTest-college_physics|5\": {\n \"acc\": 0.19607843137254902,\n \"acc_stderr\": 0.03950581861179961,\n \"acc_norm\": 0.19607843137254902,\n \"acc_norm_stderr\": 0.03950581861179961\n },\n \"harness|hendrycksTest-computer_security|5\": {\n \"acc\": 0.34,\n \"acc_stderr\": 0.04760952285695235,\n \"acc_norm\": 0.34,\n \"acc_norm_stderr\": 0.04760952285695235\n },\n \"harness|hendrycksTest-conceptual_physics|5\": {\n \"acc\": 0.32340425531914896,\n \"acc_stderr\": 0.030579442773610334,\n \"acc_norm\": 0.32340425531914896,\n \"acc_norm_stderr\": 0.030579442773610334\n },\n \"harness|hendrycksTest-econometrics|5\": {\n \"acc\": 0.2631578947368421,\n \"acc_stderr\": 0.041424397194893624,\n \"acc_norm\": 0.2631578947368421,\n \"acc_norm_stderr\": 0.041424397194893624\n },\n \"harness|hendrycksTest-electrical_engineering|5\": {\n \"acc\": 0.2413793103448276,\n \"acc_stderr\": 0.03565998174135302,\n \"acc_norm\": 0.2413793103448276,\n \"acc_norm_stderr\": 0.03565998174135302\n },\n \"harness|hendrycksTest-elementary_mathematics|5\": {\n \"acc\": 0.2566137566137566,\n \"acc_stderr\": 0.022494510767503154,\n \"acc_norm\": 0.2566137566137566,\n \"acc_norm_stderr\": 0.022494510767503154\n },\n \"harness|hendrycksTest-formal_logic|5\": {\n \"acc\": 0.1746031746031746,\n \"acc_stderr\": 0.03395490020856112,\n \"acc_norm\": 0.1746031746031746,\n \"acc_norm_stderr\": 0.03395490020856112\n },\n \"harness|hendrycksTest-global_facts|5\": {\n \"acc\": 0.33,\n \"acc_stderr\": 0.047258156262526045,\n \"acc_norm\": 0.33,\n \"acc_norm_stderr\": 0.047258156262526045\n },\n \"harness|hendrycksTest-high_school_biology|5\": {\n \"acc\": 0.4258064516129032,\n \"acc_stderr\": 0.0281291127091659,\n \"acc_norm\": 0.4258064516129032,\n \"acc_norm_stderr\": 0.0281291127091659\n },\n \"harness|hendrycksTest-high_school_chemistry|5\": {\n \"acc\": 0.3103448275862069,\n \"acc_stderr\": 0.032550867699701024,\n \"acc_norm\": 0.3103448275862069,\n \"acc_norm_stderr\": 0.032550867699701024\n },\n \"harness|hendrycksTest-high_school_computer_science|5\": {\n \"acc\": 0.28,\n \"acc_stderr\": 0.045126085985421276,\n \"acc_norm\": 0.28,\n \"acc_norm_stderr\": 0.045126085985421276\n },\n \"harness|hendrycksTest-high_school_european_history|5\": {\n \"acc\": 0.44242424242424244,\n \"acc_stderr\": 0.03878372113711275,\n \"acc_norm\": 0.44242424242424244,\n \"acc_norm_stderr\": 0.03878372113711275\n },\n \"harness|hendrycksTest-high_school_geography|5\": {\n \"acc\": 0.35858585858585856,\n \"acc_stderr\": 0.03416903640391521,\n \"acc_norm\": 0.35858585858585856,\n \"acc_norm_stderr\": 0.03416903640391521\n },\n \"harness|hendrycksTest-high_school_government_and_politics|5\": {\n \"acc\": 0.49222797927461137,\n \"acc_stderr\": 0.036080032255696545,\n \"acc_norm\": 0.49222797927461137,\n \"acc_norm_stderr\": 0.036080032255696545\n },\n \"harness|hendrycksTest-high_school_macroeconomics|5\": {\n \"acc\": 0.3384615384615385,\n \"acc_stderr\": 0.02399150050031304,\n \"acc_norm\": 0.3384615384615385,\n \"acc_norm_stderr\": 0.02399150050031304\n },\n \"harness|hendrycksTest-high_school_mathematics|5\": {\n \"acc\": 0.26296296296296295,\n \"acc_stderr\": 0.02684205787383371,\n \"acc_norm\": 0.26296296296296295,\n \"acc_norm_stderr\": 0.02684205787383371\n },\n \"harness|hendrycksTest-high_school_microeconomics|5\": {\n \"acc\": 0.36134453781512604,\n \"acc_stderr\": 0.031204691225150013,\n \"acc_norm\": 0.36134453781512604,\n \"acc_norm_stderr\": 0.031204691225150013\n },\n \"harness|hendrycksTest-high_school_physics|5\": {\n \"acc\": 0.33112582781456956,\n \"acc_stderr\": 0.038425817186598696,\n \"acc_norm\": 0.33112582781456956,\n \"acc_norm_stderr\": 0.038425817186598696\n },\n \"harness|hendrycksTest-high_school_psychology|5\": {\n \"acc\": 0.3724770642201835,\n \"acc_stderr\": 0.020728368457638494,\n \"acc_norm\": 0.3724770642201835,\n \"acc_norm_stderr\": 0.020728368457638494\n },\n \"harness|hendrycksTest-high_school_statistics|5\": {\n \"acc\": 0.46296296296296297,\n \"acc_stderr\": 0.03400603625538272,\n \"acc_norm\": 0.46296296296296297,\n \"acc_norm_stderr\": 0.03400603625538272\n },\n \"harness|hendrycksTest-high_school_us_history|5\": {\n \"acc\": 0.4019607843137255,\n \"acc_stderr\": 0.034411900234824655,\n \"acc_norm\": 0.4019607843137255,\n \"acc_norm_stderr\": 0.034411900234824655\n },\n \"harness|hendrycksTest-high_school_world_history|5\": {\n \"acc\": 0.3755274261603376,\n \"acc_stderr\": 0.03152256243091156,\n \"acc_norm\": 0.3755274261603376,\n \"acc_norm_stderr\": 0.03152256243091156\n },\n \"harness|hendrycksTest-human_aging|5\": {\n \"acc\": 0.33183856502242154,\n \"acc_stderr\": 0.031602951437766785,\n \"acc_norm\": 0.33183856502242154,\n \"acc_norm_stderr\": 0.031602951437766785\n },\n \"harness|hendrycksTest-human_sexuality|5\": {\n \"acc\": 0.35877862595419846,\n \"acc_stderr\": 0.04206739313864908,\n \"acc_norm\": 0.35877862595419846,\n \"acc_norm_stderr\": 0.04206739313864908\n },\n \"harness|hendrycksTest-international_law|5\": {\n \"acc\": 0.39669421487603307,\n \"acc_stderr\": 0.04465869780531009,\n \"acc_norm\": 0.39669421487603307,\n \"acc_norm_stderr\": 0.04465869780531009\n },\n \"harness|hendrycksTest-jurisprudence|5\": {\n \"acc\": 0.3611111111111111,\n \"acc_stderr\": 0.04643454608906275,\n \"acc_norm\": 0.3611111111111111,\n \"acc_norm_stderr\": 0.04643454608906275\n },\n \"harness|hendrycksTest-logical_fallacies|5\": {\n \"acc\": 0.3312883435582822,\n \"acc_stderr\": 0.03697983910025588,\n \"acc_norm\": 0.3312883435582822,\n \"acc_norm_stderr\": 0.03697983910025588\n },\n \"harness|hendrycksTest-machine_learning|5\": {\n \"acc\": 0.17857142857142858,\n \"acc_stderr\": 0.036352091215778065,\n \"acc_norm\": 0.17857142857142858,\n \"acc_norm_stderr\": 0.036352091215778065\n },\n \"harness|hendrycksTest-management|5\": {\n \"acc\": 0.36893203883495146,\n \"acc_stderr\": 0.04777615181156739,\n \"acc_norm\": 0.36893203883495146,\n \"acc_norm_stderr\": 0.04777615181156739\n },\n \"harness|hendrycksTest-marketing|5\": {\n \"acc\": 0.5085470085470085,\n \"acc_stderr\": 0.0327513030009703,\n \"acc_norm\": 0.5085470085470085,\n \"acc_norm_stderr\": 0.0327513030009703\n },\n \"harness|hendrycksTest-medical_genetics|5\": {\n \"acc\": 0.33,\n \"acc_stderr\": 0.04725815626252604,\n \"acc_norm\": 0.33,\n \"acc_norm_stderr\": 0.04725815626252604\n },\n \"harness|hendrycksTest-miscellaneous|5\": {\n \"acc\": 0.4240102171136654,\n \"acc_stderr\": 0.017672263329084226,\n \"acc_norm\": 0.4240102171136654,\n \"acc_norm_stderr\": 0.017672263329084226\n },\n \"harness|hendrycksTest-moral_disputes|5\": {\n \"acc\": 0.2543352601156069,\n \"acc_stderr\": 0.023445826276545543,\n \"acc_norm\": 0.2543352601156069,\n \"acc_norm_stderr\": 0.023445826276545543\n },\n \"harness|hendrycksTest-moral_scenarios|5\": {\n \"acc\": 0.2424581005586592,\n \"acc_stderr\": 0.014333522059217889,\n \"acc_norm\": 0.2424581005586592,\n \"acc_norm_stderr\": 0.014333522059217889\n },\n \"harness|hendrycksTest-nutrition|5\": {\n \"acc\": 0.4117647058823529,\n \"acc_stderr\": 0.028180596328259293,\n \"acc_norm\": 0.4117647058823529,\n \"acc_norm_stderr\": 0.028180596328259293\n },\n \"harness|hendrycksTest-philosophy|5\": {\n \"acc\": 0.34726688102893893,\n \"acc_stderr\": 0.027040745502307336,\n \"acc_norm\": 0.34726688102893893,\n \"acc_norm_stderr\": 0.027040745502307336\n },\n \"harness|hendrycksTest-prehistory|5\": {\n \"acc\": 0.31790123456790126,\n \"acc_stderr\": 0.02591006352824088,\n \"acc_norm\": 0.31790123456790126,\n \"acc_norm_stderr\": 0.02591006352824088\n },\n \"harness|hendrycksTest-professional_accounting|5\": {\n \"acc\": 0.28368794326241137,\n \"acc_stderr\": 0.02689170942834396,\n \"acc_norm\": 0.28368794326241137,\n \"acc_norm_stderr\": 0.02689170942834396\n },\n \"harness|hendrycksTest-professional_law|5\": {\n \"acc\": 0.2940026075619296,\n \"acc_stderr\": 0.011636062953698604,\n \"acc_norm\": 0.2940026075619296,\n \"acc_norm_stderr\": 0.011636062953698604\n },\n \"harness|hendrycksTest-professional_medicine|5\": {\n \"acc\": 0.4632352941176471,\n \"acc_stderr\": 0.030290619180485687,\n \"acc_norm\": 0.4632352941176471,\n \"acc_norm_stderr\": 0.030290619180485687\n },\n \"harness|hendrycksTest-professional_psychology|5\": {\n \"acc\": 0.28431372549019607,\n \"acc_stderr\": 0.018249024411207668,\n \"acc_norm\": 0.28431372549019607,\n \"acc_norm_stderr\": 0.018249024411207668\n },\n \"harness|hendrycksTest-public_relations|5\": {\n \"acc\": 0.42727272727272725,\n \"acc_stderr\": 0.04738198703545483,\n \"acc_norm\": 0.42727272727272725,\n \"acc_norm_stderr\": 0.04738198703545483\n },\n \"harness|hendrycksTest-security_studies|5\": {\n \"acc\": 0.3877551020408163,\n \"acc_stderr\": 0.031192230726795656,\n \"acc_norm\": 0.3877551020408163,\n \"acc_norm_stderr\": 0.031192230726795656\n },\n \"harness|hendrycksTest-sociology|5\": {\n \"acc\": 0.43283582089552236,\n \"acc_stderr\": 0.03503490923673281,\n \"acc_norm\": 0.43283582089552236,\n \"acc_norm_stderr\": 0.03503490923673281\n },\n \"harness|hendrycksTest-us_foreign_policy|5\": {\n \"acc\": 0.4,\n \"acc_stderr\": 0.04923659639173309,\n \"acc_norm\": 0.4,\n \"acc_norm_stderr\": 0.04923659639173309\n },\n \"harness|hendrycksTest-virology|5\": {\n \"acc\": 0.3614457831325301,\n \"acc_stderr\": 0.0374005938202932,\n \"acc_norm\": 0.3614457831325301,\n \"acc_norm_stderr\": 0.0374005938202932\n },\n \"harness|hendrycksTest-world_religions|5\": {\n \"acc\": 0.3742690058479532,\n \"acc_stderr\": 0.03711601185389481,\n \"acc_norm\": 0.3742690058479532,\n \"acc_norm_stderr\": 0.03711601185389481\n },\n \"harness|truthfulqa:mc|0\": {\n \"mc1\": 0.2521419828641371,\n \"mc1_stderr\": 0.015201522246299963,\n \"mc2\": 0.4077071941467522,\n \"mc2_stderr\": 0.014214727907656348\n },\n \"harness|winogrande|5\": {\n \"acc\": 0.7300710339384373,\n \"acc_stderr\": 0.012476433372002608\n },\n \"harness|gsm8k|5\": {\n \"acc\": 0.037149355572403335,\n \"acc_stderr\": 0.005209516283073736\n }\n}\n```", "repo_url": "https://huggingface.co/ewqr2130/mistral-7b-raw-sft", "leaderboard_url": "https://huggingface.co/spaces/HuggingFaceH4/open_llm_leaderboard", "point_of_contact": "[email protected]", "configs": [{"config_name": "harness_arc_challenge_25", "data_files": [{"split": "2024_01_10T15_14_57.972449", "path": ["**/details_harness|arc:challenge|25_2024-01-10T15-14-57.972449.parquet"]}, {"split": "latest", "path": ["**/details_harness|arc:challenge|25_2024-01-10T15-14-57.972449.parquet"]}]}, {"config_name": "harness_gsm8k_5", "data_files": [{"split": "2024_01_10T15_14_57.972449", "path": ["**/details_harness|gsm8k|5_2024-01-10T15-14-57.972449.parquet"]}, {"split": "latest", "path": ["**/details_harness|gsm8k|5_2024-01-10T15-14-57.972449.parquet"]}]}, {"config_name": "harness_hellaswag_10", "data_files": [{"split": "2024_01_10T15_14_57.972449", "path": ["**/details_harness|hellaswag|10_2024-01-10T15-14-57.972449.parquet"]}, {"split": "latest", "path": ["**/details_harness|hellaswag|10_2024-01-10T15-14-57.972449.parquet"]}]}, {"config_name": "harness_hendrycksTest_5", "data_files": [{"split": "2024_01_10T15_14_57.972449", "path": ["**/details_harness|hendrycksTest-abstract_algebra|5_2024-01-10T15-14-57.972449.parquet", "**/details_harness|hendrycksTest-anatomy|5_2024-01-10T15-14-57.972449.parquet", "**/details_harness|hendrycksTest-astronomy|5_2024-01-10T15-14-57.972449.parquet", "**/details_harness|hendrycksTest-business_ethics|5_2024-01-10T15-14-57.972449.parquet", "**/details_harness|hendrycksTest-clinical_knowledge|5_2024-01-10T15-14-57.972449.parquet", "**/details_harness|hendrycksTest-college_biology|5_2024-01-10T15-14-57.972449.parquet", "**/details_harness|hendrycksTest-college_chemistry|5_2024-01-10T15-14-57.972449.parquet", "**/details_harness|hendrycksTest-college_computer_science|5_2024-01-10T15-14-57.972449.parquet", "**/details_harness|hendrycksTest-college_mathematics|5_2024-01-10T15-14-57.972449.parquet", "**/details_harness|hendrycksTest-college_medicine|5_2024-01-10T15-14-57.972449.parquet", "**/details_harness|hendrycksTest-college_physics|5_2024-01-10T15-14-57.972449.parquet", "**/details_harness|hendrycksTest-computer_security|5_2024-01-10T15-14-57.972449.parquet", "**/details_harness|hendrycksTest-conceptual_physics|5_2024-01-10T15-14-57.972449.parquet", "**/details_harness|hendrycksTest-econometrics|5_2024-01-10T15-14-57.972449.parquet", "**/details_harness|hendrycksTest-electrical_engineering|5_2024-01-10T15-14-57.972449.parquet", "**/details_harness|hendrycksTest-elementary_mathematics|5_2024-01-10T15-14-57.972449.parquet", "**/details_harness|hendrycksTest-formal_logic|5_2024-01-10T15-14-57.972449.parquet", "**/details_harness|hendrycksTest-global_facts|5_2024-01-10T15-14-57.972449.parquet", "**/details_harness|hendrycksTest-high_school_biology|5_2024-01-10T15-14-57.972449.parquet", "**/details_harness|hendrycksTest-high_school_chemistry|5_2024-01-10T15-14-57.972449.parquet", "**/details_harness|hendrycksTest-high_school_computer_science|5_2024-01-10T15-14-57.972449.parquet", "**/details_harness|hendrycksTest-high_school_european_history|5_2024-01-10T15-14-57.972449.parquet", "**/details_harness|hendrycksTest-high_school_geography|5_2024-01-10T15-14-57.972449.parquet", "**/details_harness|hendrycksTest-high_school_government_and_politics|5_2024-01-10T15-14-57.972449.parquet", "**/details_harness|hendrycksTest-high_school_macroeconomics|5_2024-01-10T15-14-57.972449.parquet", "**/details_harness|hendrycksTest-high_school_mathematics|5_2024-01-10T15-14-57.972449.parquet", "**/details_harness|hendrycksTest-high_school_microeconomics|5_2024-01-10T15-14-57.972449.parquet", "**/details_harness|hendrycksTest-high_school_physics|5_2024-01-10T15-14-57.972449.parquet", "**/details_harness|hendrycksTest-high_school_psychology|5_2024-01-10T15-14-57.972449.parquet", "**/details_harness|hendrycksTest-high_school_statistics|5_2024-01-10T15-14-57.972449.parquet", "**/details_harness|hendrycksTest-high_school_us_history|5_2024-01-10T15-14-57.972449.parquet", "**/details_harness|hendrycksTest-high_school_world_history|5_2024-01-10T15-14-57.972449.parquet", "**/details_harness|hendrycksTest-human_aging|5_2024-01-10T15-14-57.972449.parquet", "**/details_harness|hendrycksTest-human_sexuality|5_2024-01-10T15-14-57.972449.parquet", "**/details_harness|hendrycksTest-international_law|5_2024-01-10T15-14-57.972449.parquet", "**/details_harness|hendrycksTest-jurisprudence|5_2024-01-10T15-14-57.972449.parquet", "**/details_harness|hendrycksTest-logical_fallacies|5_2024-01-10T15-14-57.972449.parquet", "**/details_harness|hendrycksTest-machine_learning|5_2024-01-10T15-14-57.972449.parquet", "**/details_harness|hendrycksTest-management|5_2024-01-10T15-14-57.972449.parquet", "**/details_harness|hendrycksTest-marketing|5_2024-01-10T15-14-57.972449.parquet", "**/details_harness|hendrycksTest-medical_genetics|5_2024-01-10T15-14-57.972449.parquet", "**/details_harness|hendrycksTest-miscellaneous|5_2024-01-10T15-14-57.972449.parquet", "**/details_harness|hendrycksTest-moral_disputes|5_2024-01-10T15-14-57.972449.parquet", "**/details_harness|hendrycksTest-moral_scenarios|5_2024-01-10T15-14-57.972449.parquet", "**/details_harness|hendrycksTest-nutrition|5_2024-01-10T15-14-57.972449.parquet", "**/details_harness|hendrycksTest-philosophy|5_2024-01-10T15-14-57.972449.parquet", "**/details_harness|hendrycksTest-prehistory|5_2024-01-10T15-14-57.972449.parquet", "**/details_harness|hendrycksTest-professional_accounting|5_2024-01-10T15-14-57.972449.parquet", "**/details_harness|hendrycksTest-professional_law|5_2024-01-10T15-14-57.972449.parquet", "**/details_harness|hendrycksTest-professional_medicine|5_2024-01-10T15-14-57.972449.parquet", "**/details_harness|hendrycksTest-professional_psychology|5_2024-01-10T15-14-57.972449.parquet", "**/details_harness|hendrycksTest-public_relations|5_2024-01-10T15-14-57.972449.parquet", "**/details_harness|hendrycksTest-security_studies|5_2024-01-10T15-14-57.972449.parquet", "**/details_harness|hendrycksTest-sociology|5_2024-01-10T15-14-57.972449.parquet", "**/details_harness|hendrycksTest-us_foreign_policy|5_2024-01-10T15-14-57.972449.parquet", "**/details_harness|hendrycksTest-virology|5_2024-01-10T15-14-57.972449.parquet", "**/details_harness|hendrycksTest-world_religions|5_2024-01-10T15-14-57.972449.parquet"]}, {"split": "latest", "path": ["**/details_harness|hendrycksTest-abstract_algebra|5_2024-01-10T15-14-57.972449.parquet", "**/details_harness|hendrycksTest-anatomy|5_2024-01-10T15-14-57.972449.parquet", "**/details_harness|hendrycksTest-astronomy|5_2024-01-10T15-14-57.972449.parquet", "**/details_harness|hendrycksTest-business_ethics|5_2024-01-10T15-14-57.972449.parquet", "**/details_harness|hendrycksTest-clinical_knowledge|5_2024-01-10T15-14-57.972449.parquet", "**/details_harness|hendrycksTest-college_biology|5_2024-01-10T15-14-57.972449.parquet", "**/details_harness|hendrycksTest-college_chemistry|5_2024-01-10T15-14-57.972449.parquet", "**/details_harness|hendrycksTest-college_computer_science|5_2024-01-10T15-14-57.972449.parquet", "**/details_harness|hendrycksTest-college_mathematics|5_2024-01-10T15-14-57.972449.parquet", "**/details_harness|hendrycksTest-college_medicine|5_2024-01-10T15-14-57.972449.parquet", "**/details_harness|hendrycksTest-college_physics|5_2024-01-10T15-14-57.972449.parquet", "**/details_harness|hendrycksTest-computer_security|5_2024-01-10T15-14-57.972449.parquet", "**/details_harness|hendrycksTest-conceptual_physics|5_2024-01-10T15-14-57.972449.parquet", "**/details_harness|hendrycksTest-econometrics|5_2024-01-10T15-14-57.972449.parquet", "**/details_harness|hendrycksTest-electrical_engineering|5_2024-01-10T15-14-57.972449.parquet", "**/details_harness|hendrycksTest-elementary_mathematics|5_2024-01-10T15-14-57.972449.parquet", "**/details_harness|hendrycksTest-formal_logic|5_2024-01-10T15-14-57.972449.parquet", "**/details_harness|hendrycksTest-global_facts|5_2024-01-10T15-14-57.972449.parquet", "**/details_harness|hendrycksTest-high_school_biology|5_2024-01-10T15-14-57.972449.parquet", "**/details_harness|hendrycksTest-high_school_chemistry|5_2024-01-10T15-14-57.972449.parquet", "**/details_harness|hendrycksTest-high_school_computer_science|5_2024-01-10T15-14-57.972449.parquet", "**/details_harness|hendrycksTest-high_school_european_history|5_2024-01-10T15-14-57.972449.parquet", "**/details_harness|hendrycksTest-high_school_geography|5_2024-01-10T15-14-57.972449.parquet", "**/details_harness|hendrycksTest-high_school_government_and_politics|5_2024-01-10T15-14-57.972449.parquet", "**/details_harness|hendrycksTest-high_school_macroeconomics|5_2024-01-10T15-14-57.972449.parquet", "**/details_harness|hendrycksTest-high_school_mathematics|5_2024-01-10T15-14-57.972449.parquet", "**/details_harness|hendrycksTest-high_school_microeconomics|5_2024-01-10T15-14-57.972449.parquet", "**/details_harness|hendrycksTest-high_school_physics|5_2024-01-10T15-14-57.972449.parquet", "**/details_harness|hendrycksTest-high_school_psychology|5_2024-01-10T15-14-57.972449.parquet", "**/details_harness|hendrycksTest-high_school_statistics|5_2024-01-10T15-14-57.972449.parquet", "**/details_harness|hendrycksTest-high_school_us_history|5_2024-01-10T15-14-57.972449.parquet", "**/details_harness|hendrycksTest-high_school_world_history|5_2024-01-10T15-14-57.972449.parquet", "**/details_harness|hendrycksTest-human_aging|5_2024-01-10T15-14-57.972449.parquet", "**/details_harness|hendrycksTest-human_sexuality|5_2024-01-10T15-14-57.972449.parquet", "**/details_harness|hendrycksTest-international_law|5_2024-01-10T15-14-57.972449.parquet", "**/details_harness|hendrycksTest-jurisprudence|5_2024-01-10T15-14-57.972449.parquet", "**/details_harness|hendrycksTest-logical_fallacies|5_2024-01-10T15-14-57.972449.parquet", "**/details_harness|hendrycksTest-machine_learning|5_2024-01-10T15-14-57.972449.parquet", "**/details_harness|hendrycksTest-management|5_2024-01-10T15-14-57.972449.parquet", "**/details_harness|hendrycksTest-marketing|5_2024-01-10T15-14-57.972449.parquet", "**/details_harness|hendrycksTest-medical_genetics|5_2024-01-10T15-14-57.972449.parquet", "**/details_harness|hendrycksTest-miscellaneous|5_2024-01-10T15-14-57.972449.parquet", "**/details_harness|hendrycksTest-moral_disputes|5_2024-01-10T15-14-57.972449.parquet", "**/details_harness|hendrycksTest-moral_scenarios|5_2024-01-10T15-14-57.972449.parquet", "**/details_harness|hendrycksTest-nutrition|5_2024-01-10T15-14-57.972449.parquet", "**/details_harness|hendrycksTest-philosophy|5_2024-01-10T15-14-57.972449.parquet", "**/details_harness|hendrycksTest-prehistory|5_2024-01-10T15-14-57.972449.parquet", "**/details_harness|hendrycksTest-professional_accounting|5_2024-01-10T15-14-57.972449.parquet", "**/details_harness|hendrycksTest-professional_law|5_2024-01-10T15-14-57.972449.parquet", "**/details_harness|hendrycksTest-professional_medicine|5_2024-01-10T15-14-57.972449.parquet", "**/details_harness|hendrycksTest-professional_psychology|5_2024-01-10T15-14-57.972449.parquet", "**/details_harness|hendrycksTest-public_relations|5_2024-01-10T15-14-57.972449.parquet", "**/details_harness|hendrycksTest-security_studies|5_2024-01-10T15-14-57.972449.parquet", "**/details_harness|hendrycksTest-sociology|5_2024-01-10T15-14-57.972449.parquet", "**/details_harness|hendrycksTest-us_foreign_policy|5_2024-01-10T15-14-57.972449.parquet", "**/details_harness|hendrycksTest-virology|5_2024-01-10T15-14-57.972449.parquet", "**/details_harness|hendrycksTest-world_religions|5_2024-01-10T15-14-57.972449.parquet"]}]}, {"config_name": "harness_hendrycksTest_abstract_algebra_5", "data_files": [{"split": "2024_01_10T15_14_57.972449", "path": ["**/details_harness|hendrycksTest-abstract_algebra|5_2024-01-10T15-14-57.972449.parquet"]}, {"split": "latest", "path": ["**/details_harness|hendrycksTest-abstract_algebra|5_2024-01-10T15-14-57.972449.parquet"]}]}, {"config_name": "harness_hendrycksTest_anatomy_5", "data_files": [{"split": "2024_01_10T15_14_57.972449", "path": ["**/details_harness|hendrycksTest-anatomy|5_2024-01-10T15-14-57.972449.parquet"]}, {"split": "latest", "path": ["**/details_harness|hendrycksTest-anatomy|5_2024-01-10T15-14-57.972449.parquet"]}]}, {"config_name": "harness_hendrycksTest_astronomy_5", "data_files": [{"split": "2024_01_10T15_14_57.972449", "path": ["**/details_harness|hendrycksTest-astronomy|5_2024-01-10T15-14-57.972449.parquet"]}, {"split": "latest", "path": ["**/details_harness|hendrycksTest-astronomy|5_2024-01-10T15-14-57.972449.parquet"]}]}, {"config_name": "harness_hendrycksTest_business_ethics_5", "data_files": [{"split": "2024_01_10T15_14_57.972449", "path": ["**/details_harness|hendrycksTest-business_ethics|5_2024-01-10T15-14-57.972449.parquet"]}, {"split": "latest", "path": ["**/details_harness|hendrycksTest-business_ethics|5_2024-01-10T15-14-57.972449.parquet"]}]}, {"config_name": "harness_hendrycksTest_clinical_knowledge_5", "data_files": [{"split": "2024_01_10T15_14_57.972449", "path": ["**/details_harness|hendrycksTest-clinical_knowledge|5_2024-01-10T15-14-57.972449.parquet"]}, {"split": "latest", "path": ["**/details_harness|hendrycksTest-clinical_knowledge|5_2024-01-10T15-14-57.972449.parquet"]}]}, {"config_name": "harness_hendrycksTest_college_biology_5", "data_files": [{"split": "2024_01_10T15_14_57.972449", "path": ["**/details_harness|hendrycksTest-college_biology|5_2024-01-10T15-14-57.972449.parquet"]}, {"split": "latest", "path": ["**/details_harness|hendrycksTest-college_biology|5_2024-01-10T15-14-57.972449.parquet"]}]}, {"config_name": "harness_hendrycksTest_college_chemistry_5", "data_files": [{"split": "2024_01_10T15_14_57.972449", "path": ["**/details_harness|hendrycksTest-college_chemistry|5_2024-01-10T15-14-57.972449.parquet"]}, {"split": "latest", "path": ["**/details_harness|hendrycksTest-college_chemistry|5_2024-01-10T15-14-57.972449.parquet"]}]}, {"config_name": "harness_hendrycksTest_college_computer_science_5", "data_files": [{"split": "2024_01_10T15_14_57.972449", "path": ["**/details_harness|hendrycksTest-college_computer_science|5_2024-01-10T15-14-57.972449.parquet"]}, {"split": "latest", "path": ["**/details_harness|hendrycksTest-college_computer_science|5_2024-01-10T15-14-57.972449.parquet"]}]}, {"config_name": "harness_hendrycksTest_college_mathematics_5", "data_files": [{"split": "2024_01_10T15_14_57.972449", "path": ["**/details_harness|hendrycksTest-college_mathematics|5_2024-01-10T15-14-57.972449.parquet"]}, {"split": "latest", "path": ["**/details_harness|hendrycksTest-college_mathematics|5_2024-01-10T15-14-57.972449.parquet"]}]}, {"config_name": "harness_hendrycksTest_college_medicine_5", "data_files": [{"split": "2024_01_10T15_14_57.972449", "path": ["**/details_harness|hendrycksTest-college_medicine|5_2024-01-10T15-14-57.972449.parquet"]}, {"split": "latest", "path": ["**/details_harness|hendrycksTest-college_medicine|5_2024-01-10T15-14-57.972449.parquet"]}]}, {"config_name": "harness_hendrycksTest_college_physics_5", "data_files": [{"split": "2024_01_10T15_14_57.972449", "path": ["**/details_harness|hendrycksTest-college_physics|5_2024-01-10T15-14-57.972449.parquet"]}, {"split": "latest", "path": ["**/details_harness|hendrycksTest-college_physics|5_2024-01-10T15-14-57.972449.parquet"]}]}, {"config_name": "harness_hendrycksTest_computer_security_5", "data_files": [{"split": "2024_01_10T15_14_57.972449", "path": ["**/details_harness|hendrycksTest-computer_security|5_2024-01-10T15-14-57.972449.parquet"]}, {"split": "latest", "path": ["**/details_harness|hendrycksTest-computer_security|5_2024-01-10T15-14-57.972449.parquet"]}]}, {"config_name": "harness_hendrycksTest_conceptual_physics_5", "data_files": [{"split": "2024_01_10T15_14_57.972449", "path": ["**/details_harness|hendrycksTest-conceptual_physics|5_2024-01-10T15-14-57.972449.parquet"]}, {"split": "latest", "path": ["**/details_harness|hendrycksTest-conceptual_physics|5_2024-01-10T15-14-57.972449.parquet"]}]}, {"config_name": "harness_hendrycksTest_econometrics_5", "data_files": [{"split": "2024_01_10T15_14_57.972449", "path": ["**/details_harness|hendrycksTest-econometrics|5_2024-01-10T15-14-57.972449.parquet"]}, {"split": "latest", "path": ["**/details_harness|hendrycksTest-econometrics|5_2024-01-10T15-14-57.972449.parquet"]}]}, {"config_name": "harness_hendrycksTest_electrical_engineering_5", "data_files": [{"split": "2024_01_10T15_14_57.972449", "path": ["**/details_harness|hendrycksTest-electrical_engineering|5_2024-01-10T15-14-57.972449.parquet"]}, {"split": "latest", "path": ["**/details_harness|hendrycksTest-electrical_engineering|5_2024-01-10T15-14-57.972449.parquet"]}]}, {"config_name": "harness_hendrycksTest_elementary_mathematics_5", "data_files": [{"split": "2024_01_10T15_14_57.972449", "path": ["**/details_harness|hendrycksTest-elementary_mathematics|5_2024-01-10T15-14-57.972449.parquet"]}, {"split": "latest", "path": ["**/details_harness|hendrycksTest-elementary_mathematics|5_2024-01-10T15-14-57.972449.parquet"]}]}, {"config_name": "harness_hendrycksTest_formal_logic_5", "data_files": [{"split": "2024_01_10T15_14_57.972449", "path": ["**/details_harness|hendrycksTest-formal_logic|5_2024-01-10T15-14-57.972449.parquet"]}, {"split": "latest", "path": ["**/details_harness|hendrycksTest-formal_logic|5_2024-01-10T15-14-57.972449.parquet"]}]}, {"config_name": "harness_hendrycksTest_global_facts_5", "data_files": [{"split": "2024_01_10T15_14_57.972449", "path": ["**/details_harness|hendrycksTest-global_facts|5_2024-01-10T15-14-57.972449.parquet"]}, {"split": "latest", "path": ["**/details_harness|hendrycksTest-global_facts|5_2024-01-10T15-14-57.972449.parquet"]}]}, {"config_name": "harness_hendrycksTest_high_school_biology_5", "data_files": [{"split": "2024_01_10T15_14_57.972449", "path": ["**/details_harness|hendrycksTest-high_school_biology|5_2024-01-10T15-14-57.972449.parquet"]}, {"split": "latest", "path": ["**/details_harness|hendrycksTest-high_school_biology|5_2024-01-10T15-14-57.972449.parquet"]}]}, {"config_name": "harness_hendrycksTest_high_school_chemistry_5", "data_files": [{"split": "2024_01_10T15_14_57.972449", "path": ["**/details_harness|hendrycksTest-high_school_chemistry|5_2024-01-10T15-14-57.972449.parquet"]}, {"split": "latest", "path": ["**/details_harness|hendrycksTest-high_school_chemistry|5_2024-01-10T15-14-57.972449.parquet"]}]}, {"config_name": "harness_hendrycksTest_high_school_computer_science_5", "data_files": [{"split": "2024_01_10T15_14_57.972449", "path": ["**/details_harness|hendrycksTest-high_school_computer_science|5_2024-01-10T15-14-57.972449.parquet"]}, {"split": "latest", "path": ["**/details_harness|hendrycksTest-high_school_computer_science|5_2024-01-10T15-14-57.972449.parquet"]}]}, {"config_name": "harness_hendrycksTest_high_school_european_history_5", "data_files": [{"split": "2024_01_10T15_14_57.972449", "path": ["**/details_harness|hendrycksTest-high_school_european_history|5_2024-01-10T15-14-57.972449.parquet"]}, {"split": "latest", "path": ["**/details_harness|hendrycksTest-high_school_european_history|5_2024-01-10T15-14-57.972449.parquet"]}]}, {"config_name": "harness_hendrycksTest_high_school_geography_5", "data_files": [{"split": "2024_01_10T15_14_57.972449", "path": ["**/details_harness|hendrycksTest-high_school_geography|5_2024-01-10T15-14-57.972449.parquet"]}, {"split": "latest", "path": ["**/details_harness|hendrycksTest-high_school_geography|5_2024-01-10T15-14-57.972449.parquet"]}]}, {"config_name": "harness_hendrycksTest_high_school_government_and_politics_5", "data_files": [{"split": "2024_01_10T15_14_57.972449", "path": ["**/details_harness|hendrycksTest-high_school_government_and_politics|5_2024-01-10T15-14-57.972449.parquet"]}, {"split": "latest", "path": ["**/details_harness|hendrycksTest-high_school_government_and_politics|5_2024-01-10T15-14-57.972449.parquet"]}]}, {"config_name": "harness_hendrycksTest_high_school_macroeconomics_5", "data_files": [{"split": "2024_01_10T15_14_57.972449", "path": ["**/details_harness|hendrycksTest-high_school_macroeconomics|5_2024-01-10T15-14-57.972449.parquet"]}, {"split": "latest", "path": ["**/details_harness|hendrycksTest-high_school_macroeconomics|5_2024-01-10T15-14-57.972449.parquet"]}]}, {"config_name": "harness_hendrycksTest_high_school_mathematics_5", "data_files": [{"split": "2024_01_10T15_14_57.972449", "path": ["**/details_harness|hendrycksTest-high_school_mathematics|5_2024-01-10T15-14-57.972449.parquet"]}, {"split": "latest", "path": ["**/details_harness|hendrycksTest-high_school_mathematics|5_2024-01-10T15-14-57.972449.parquet"]}]}, {"config_name": "harness_hendrycksTest_high_school_microeconomics_5", "data_files": [{"split": "2024_01_10T15_14_57.972449", "path": ["**/details_harness|hendrycksTest-high_school_microeconomics|5_2024-01-10T15-14-57.972449.parquet"]}, {"split": "latest", "path": ["**/details_harness|hendrycksTest-high_school_microeconomics|5_2024-01-10T15-14-57.972449.parquet"]}]}, {"config_name": "harness_hendrycksTest_high_school_physics_5", "data_files": [{"split": "2024_01_10T15_14_57.972449", "path": ["**/details_harness|hendrycksTest-high_school_physics|5_2024-01-10T15-14-57.972449.parquet"]}, {"split": "latest", "path": ["**/details_harness|hendrycksTest-high_school_physics|5_2024-01-10T15-14-57.972449.parquet"]}]}, {"config_name": "harness_hendrycksTest_high_school_psychology_5", "data_files": [{"split": "2024_01_10T15_14_57.972449", "path": ["**/details_harness|hendrycksTest-high_school_psychology|5_2024-01-10T15-14-57.972449.parquet"]}, {"split": "latest", "path": ["**/details_harness|hendrycksTest-high_school_psychology|5_2024-01-10T15-14-57.972449.parquet"]}]}, {"config_name": "harness_hendrycksTest_high_school_statistics_5", "data_files": [{"split": "2024_01_10T15_14_57.972449", "path": ["**/details_harness|hendrycksTest-high_school_statistics|5_2024-01-10T15-14-57.972449.parquet"]}, {"split": "latest", "path": ["**/details_harness|hendrycksTest-high_school_statistics|5_2024-01-10T15-14-57.972449.parquet"]}]}, {"config_name": "harness_hendrycksTest_high_school_us_history_5", "data_files": [{"split": "2024_01_10T15_14_57.972449", "path": ["**/details_harness|hendrycksTest-high_school_us_history|5_2024-01-10T15-14-57.972449.parquet"]}, {"split": "latest", "path": ["**/details_harness|hendrycksTest-high_school_us_history|5_2024-01-10T15-14-57.972449.parquet"]}]}, {"config_name": "harness_hendrycksTest_high_school_world_history_5", "data_files": [{"split": "2024_01_10T15_14_57.972449", "path": ["**/details_harness|hendrycksTest-high_school_world_history|5_2024-01-10T15-14-57.972449.parquet"]}, {"split": "latest", "path": ["**/details_harness|hendrycksTest-high_school_world_history|5_2024-01-10T15-14-57.972449.parquet"]}]}, {"config_name": "harness_hendrycksTest_human_aging_5", "data_files": [{"split": "2024_01_10T15_14_57.972449", "path": ["**/details_harness|hendrycksTest-human_aging|5_2024-01-10T15-14-57.972449.parquet"]}, {"split": "latest", "path": ["**/details_harness|hendrycksTest-human_aging|5_2024-01-10T15-14-57.972449.parquet"]}]}, {"config_name": "harness_hendrycksTest_human_sexuality_5", "data_files": [{"split": "2024_01_10T15_14_57.972449", "path": ["**/details_harness|hendrycksTest-human_sexuality|5_2024-01-10T15-14-57.972449.parquet"]}, {"split": "latest", "path": ["**/details_harness|hendrycksTest-human_sexuality|5_2024-01-10T15-14-57.972449.parquet"]}]}, {"config_name": "harness_hendrycksTest_international_law_5", "data_files": [{"split": "2024_01_10T15_14_57.972449", "path": ["**/details_harness|hendrycksTest-international_law|5_2024-01-10T15-14-57.972449.parquet"]}, {"split": "latest", "path": ["**/details_harness|hendrycksTest-international_law|5_2024-01-10T15-14-57.972449.parquet"]}]}, {"config_name": "harness_hendrycksTest_jurisprudence_5", "data_files": [{"split": "2024_01_10T15_14_57.972449", "path": ["**/details_harness|hendrycksTest-jurisprudence|5_2024-01-10T15-14-57.972449.parquet"]}, {"split": "latest", "path": ["**/details_harness|hendrycksTest-jurisprudence|5_2024-01-10T15-14-57.972449.parquet"]}]}, {"config_name": "harness_hendrycksTest_logical_fallacies_5", "data_files": [{"split": "2024_01_10T15_14_57.972449", "path": ["**/details_harness|hendrycksTest-logical_fallacies|5_2024-01-10T15-14-57.972449.parquet"]}, {"split": "latest", "path": ["**/details_harness|hendrycksTest-logical_fallacies|5_2024-01-10T15-14-57.972449.parquet"]}]}, {"config_name": "harness_hendrycksTest_machine_learning_5", "data_files": [{"split": "2024_01_10T15_14_57.972449", "path": ["**/details_harness|hendrycksTest-machine_learning|5_2024-01-10T15-14-57.972449.parquet"]}, {"split": "latest", "path": ["**/details_harness|hendrycksTest-machine_learning|5_2024-01-10T15-14-57.972449.parquet"]}]}, {"config_name": "harness_hendrycksTest_management_5", "data_files": [{"split": "2024_01_10T15_14_57.972449", "path": ["**/details_harness|hendrycksTest-management|5_2024-01-10T15-14-57.972449.parquet"]}, {"split": "latest", "path": ["**/details_harness|hendrycksTest-management|5_2024-01-10T15-14-57.972449.parquet"]}]}, {"config_name": "harness_hendrycksTest_marketing_5", "data_files": [{"split": "2024_01_10T15_14_57.972449", "path": ["**/details_harness|hendrycksTest-marketing|5_2024-01-10T15-14-57.972449.parquet"]}, {"split": "latest", "path": ["**/details_harness|hendrycksTest-marketing|5_2024-01-10T15-14-57.972449.parquet"]}]}, {"config_name": "harness_hendrycksTest_medical_genetics_5", "data_files": [{"split": "2024_01_10T15_14_57.972449", "path": ["**/details_harness|hendrycksTest-medical_genetics|5_2024-01-10T15-14-57.972449.parquet"]}, {"split": "latest", "path": ["**/details_harness|hendrycksTest-medical_genetics|5_2024-01-10T15-14-57.972449.parquet"]}]}, {"config_name": "harness_hendrycksTest_miscellaneous_5", "data_files": [{"split": "2024_01_10T15_14_57.972449", "path": ["**/details_harness|hendrycksTest-miscellaneous|5_2024-01-10T15-14-57.972449.parquet"]}, {"split": "latest", "path": ["**/details_harness|hendrycksTest-miscellaneous|5_2024-01-10T15-14-57.972449.parquet"]}]}, {"config_name": "harness_hendrycksTest_moral_disputes_5", "data_files": [{"split": "2024_01_10T15_14_57.972449", "path": ["**/details_harness|hendrycksTest-moral_disputes|5_2024-01-10T15-14-57.972449.parquet"]}, {"split": "latest", "path": ["**/details_harness|hendrycksTest-moral_disputes|5_2024-01-10T15-14-57.972449.parquet"]}]}, {"config_name": "harness_hendrycksTest_moral_scenarios_5", "data_files": [{"split": "2024_01_10T15_14_57.972449", "path": ["**/details_harness|hendrycksTest-moral_scenarios|5_2024-01-10T15-14-57.972449.parquet"]}, {"split": "latest", "path": ["**/details_harness|hendrycksTest-moral_scenarios|5_2024-01-10T15-14-57.972449.parquet"]}]}, {"config_name": "harness_hendrycksTest_nutrition_5", "data_files": [{"split": "2024_01_10T15_14_57.972449", "path": ["**/details_harness|hendrycksTest-nutrition|5_2024-01-10T15-14-57.972449.parquet"]}, {"split": "latest", "path": ["**/details_harness|hendrycksTest-nutrition|5_2024-01-10T15-14-57.972449.parquet"]}]}, {"config_name": "harness_hendrycksTest_philosophy_5", "data_files": [{"split": "2024_01_10T15_14_57.972449", "path": ["**/details_harness|hendrycksTest-philosophy|5_2024-01-10T15-14-57.972449.parquet"]}, {"split": "latest", "path": ["**/details_harness|hendrycksTest-philosophy|5_2024-01-10T15-14-57.972449.parquet"]}]}, {"config_name": "harness_hendrycksTest_prehistory_5", "data_files": [{"split": "2024_01_10T15_14_57.972449", "path": ["**/details_harness|hendrycksTest-prehistory|5_2024-01-10T15-14-57.972449.parquet"]}, {"split": "latest", "path": ["**/details_harness|hendrycksTest-prehistory|5_2024-01-10T15-14-57.972449.parquet"]}]}, {"config_name": "harness_hendrycksTest_professional_accounting_5", "data_files": [{"split": "2024_01_10T15_14_57.972449", "path": ["**/details_harness|hendrycksTest-professional_accounting|5_2024-01-10T15-14-57.972449.parquet"]}, {"split": "latest", "path": ["**/details_harness|hendrycksTest-professional_accounting|5_2024-01-10T15-14-57.972449.parquet"]}]}, {"config_name": "harness_hendrycksTest_professional_law_5", "data_files": [{"split": "2024_01_10T15_14_57.972449", "path": ["**/details_harness|hendrycksTest-professional_law|5_2024-01-10T15-14-57.972449.parquet"]}, {"split": "latest", "path": ["**/details_harness|hendrycksTest-professional_law|5_2024-01-10T15-14-57.972449.parquet"]}]}, {"config_name": "harness_hendrycksTest_professional_medicine_5", "data_files": [{"split": "2024_01_10T15_14_57.972449", "path": ["**/details_harness|hendrycksTest-professional_medicine|5_2024-01-10T15-14-57.972449.parquet"]}, {"split": "latest", "path": ["**/details_harness|hendrycksTest-professional_medicine|5_2024-01-10T15-14-57.972449.parquet"]}]}, {"config_name": "harness_hendrycksTest_professional_psychology_5", "data_files": [{"split": "2024_01_10T15_14_57.972449", "path": ["**/details_harness|hendrycksTest-professional_psychology|5_2024-01-10T15-14-57.972449.parquet"]}, {"split": "latest", "path": ["**/details_harness|hendrycksTest-professional_psychology|5_2024-01-10T15-14-57.972449.parquet"]}]}, {"config_name": "harness_hendrycksTest_public_relations_5", "data_files": [{"split": "2024_01_10T15_14_57.972449", "path": ["**/details_harness|hendrycksTest-public_relations|5_2024-01-10T15-14-57.972449.parquet"]}, {"split": "latest", "path": ["**/details_harness|hendrycksTest-public_relations|5_2024-01-10T15-14-57.972449.parquet"]}]}, {"config_name": "harness_hendrycksTest_security_studies_5", "data_files": [{"split": "2024_01_10T15_14_57.972449", "path": ["**/details_harness|hendrycksTest-security_studies|5_2024-01-10T15-14-57.972449.parquet"]}, {"split": "latest", "path": ["**/details_harness|hendrycksTest-security_studies|5_2024-01-10T15-14-57.972449.parquet"]}]}, {"config_name": "harness_hendrycksTest_sociology_5", "data_files": [{"split": "2024_01_10T15_14_57.972449", "path": ["**/details_harness|hendrycksTest-sociology|5_2024-01-10T15-14-57.972449.parquet"]}, {"split": "latest", "path": ["**/details_harness|hendrycksTest-sociology|5_2024-01-10T15-14-57.972449.parquet"]}]}, {"config_name": "harness_hendrycksTest_us_foreign_policy_5", "data_files": [{"split": "2024_01_10T15_14_57.972449", "path": ["**/details_harness|hendrycksTest-us_foreign_policy|5_2024-01-10T15-14-57.972449.parquet"]}, {"split": "latest", "path": ["**/details_harness|hendrycksTest-us_foreign_policy|5_2024-01-10T15-14-57.972449.parquet"]}]}, {"config_name": "harness_hendrycksTest_virology_5", "data_files": [{"split": "2024_01_10T15_14_57.972449", "path": ["**/details_harness|hendrycksTest-virology|5_2024-01-10T15-14-57.972449.parquet"]}, {"split": "latest", "path": ["**/details_harness|hendrycksTest-virology|5_2024-01-10T15-14-57.972449.parquet"]}]}, {"config_name": "harness_hendrycksTest_world_religions_5", "data_files": [{"split": "2024_01_10T15_14_57.972449", "path": ["**/details_harness|hendrycksTest-world_religions|5_2024-01-10T15-14-57.972449.parquet"]}, {"split": "latest", "path": ["**/details_harness|hendrycksTest-world_religions|5_2024-01-10T15-14-57.972449.parquet"]}]}, {"config_name": "harness_truthfulqa_mc_0", "data_files": [{"split": "2024_01_10T15_14_57.972449", "path": ["**/details_harness|truthfulqa:mc|0_2024-01-10T15-14-57.972449.parquet"]}, {"split": "latest", "path": ["**/details_harness|truthfulqa:mc|0_2024-01-10T15-14-57.972449.parquet"]}]}, {"config_name": "harness_winogrande_5", "data_files": [{"split": "2024_01_10T15_14_57.972449", "path": ["**/details_harness|winogrande|5_2024-01-10T15-14-57.972449.parquet"]}, {"split": "latest", "path": ["**/details_harness|winogrande|5_2024-01-10T15-14-57.972449.parquet"]}]}, {"config_name": "results", "data_files": [{"split": "2024_01_10T15_14_57.972449", "path": ["results_2024-01-10T15-14-57.972449.parquet"]}, {"split": "latest", "path": ["results_2024-01-10T15-14-57.972449.parquet"]}]}]} | 2024-01-10T15:17:43+00:00 | [] | [] | TAGS
#region-us
|
# Dataset Card for Evaluation run of ewqr2130/mistral-7b-raw-sft
Dataset automatically created during the evaluation run of model ewqr2130/mistral-7b-raw-sft on the Open LLM Leaderboard.
The dataset is composed of 63 configuration, each one coresponding to one of the evaluated task.
The dataset has been created from 1 run(s). Each run can be found as a specific split in each configuration, the split being named using the timestamp of the run.The "train" split is always pointing to the latest results.
An additional configuration "results" store all the aggregated results of the run (and is used to compute and display the aggregated metrics on the Open LLM Leaderboard).
To load the details from a run, you can for instance do the following:
## Latest results
These are the latest results from run 2024-01-10T15:14:57.972449(note that their might be results for other tasks in the repos if successive evals didn't cover the same tasks. You find each in the results and the "latest" split for each eval):
## Dataset Details
### Dataset Description
- Curated by:
- Funded by [optional]:
- Shared by [optional]:
- Language(s) (NLP):
- License:
### Dataset Sources [optional]
- Repository:
- Paper [optional]:
- Demo [optional]:
## Uses
### Direct Use
### Out-of-Scope Use
## Dataset Structure
## Dataset Creation
### Curation Rationale
### Source Data
#### Data Collection and Processing
#### Who are the source data producers?
### Annotations [optional]
#### Annotation process
#### Who are the annotators?
#### Personal and Sensitive Information
## Bias, Risks, and Limitations
### Recommendations
Users should be made aware of the risks, biases and limitations of the dataset. More information needed for further recommendations.
[optional]
BibTeX:
APA:
## Glossary [optional]
## More Information [optional]
## Dataset Card Authors [optional]
## Dataset Card Contact
| [
"# Dataset Card for Evaluation run of ewqr2130/mistral-7b-raw-sft\n\n\n\nDataset automatically created during the evaluation run of model ewqr2130/mistral-7b-raw-sft on the Open LLM Leaderboard.\n\nThe dataset is composed of 63 configuration, each one coresponding to one of the evaluated task.\n\nThe dataset has been created from 1 run(s). Each run can be found as a specific split in each configuration, the split being named using the timestamp of the run.The \"train\" split is always pointing to the latest results.\n\nAn additional configuration \"results\" store all the aggregated results of the run (and is used to compute and display the aggregated metrics on the Open LLM Leaderboard).\n\nTo load the details from a run, you can for instance do the following:",
"## Latest results\n\nThese are the latest results from run 2024-01-10T15:14:57.972449(note that their might be results for other tasks in the repos if successive evals didn't cover the same tasks. You find each in the results and the \"latest\" split for each eval):",
"## Dataset Details",
"### Dataset Description\n\n\n\n\n\n- Curated by: \n- Funded by [optional]: \n- Shared by [optional]: \n- Language(s) (NLP): \n- License:",
"### Dataset Sources [optional]\n\n\n\n- Repository: \n- Paper [optional]: \n- Demo [optional]:",
"## Uses",
"### Direct Use",
"### Out-of-Scope Use",
"## Dataset Structure",
"## Dataset Creation",
"### Curation Rationale",
"### Source Data",
"#### Data Collection and Processing",
"#### Who are the source data producers?",
"### Annotations [optional]",
"#### Annotation process",
"#### Who are the annotators?",
"#### Personal and Sensitive Information",
"## Bias, Risks, and Limitations",
"### Recommendations\n\n\n\nUsers should be made aware of the risks, biases and limitations of the dataset. More information needed for further recommendations.\n\n[optional]\n\n\n\nBibTeX:\n\n\n\nAPA:",
"## Glossary [optional]",
"## More Information [optional]",
"## Dataset Card Authors [optional]",
"## Dataset Card Contact"
] | [
"TAGS\n#region-us \n",
"# Dataset Card for Evaluation run of ewqr2130/mistral-7b-raw-sft\n\n\n\nDataset automatically created during the evaluation run of model ewqr2130/mistral-7b-raw-sft on the Open LLM Leaderboard.\n\nThe dataset is composed of 63 configuration, each one coresponding to one of the evaluated task.\n\nThe dataset has been created from 1 run(s). Each run can be found as a specific split in each configuration, the split being named using the timestamp of the run.The \"train\" split is always pointing to the latest results.\n\nAn additional configuration \"results\" store all the aggregated results of the run (and is used to compute and display the aggregated metrics on the Open LLM Leaderboard).\n\nTo load the details from a run, you can for instance do the following:",
"## Latest results\n\nThese are the latest results from run 2024-01-10T15:14:57.972449(note that their might be results for other tasks in the repos if successive evals didn't cover the same tasks. You find each in the results and the \"latest\" split for each eval):",
"## Dataset Details",
"### Dataset Description\n\n\n\n\n\n- Curated by: \n- Funded by [optional]: \n- Shared by [optional]: \n- Language(s) (NLP): \n- License:",
"### Dataset Sources [optional]\n\n\n\n- Repository: \n- Paper [optional]: \n- Demo [optional]:",
"## Uses",
"### Direct Use",
"### Out-of-Scope Use",
"## Dataset Structure",
"## Dataset Creation",
"### Curation Rationale",
"### Source Data",
"#### Data Collection and Processing",
"#### Who are the source data producers?",
"### Annotations [optional]",
"#### Annotation process",
"#### Who are the annotators?",
"#### Personal and Sensitive Information",
"## Bias, Risks, and Limitations",
"### Recommendations\n\n\n\nUsers should be made aware of the risks, biases and limitations of the dataset. More information needed for further recommendations.\n\n[optional]\n\n\n\nBibTeX:\n\n\n\nAPA:",
"## Glossary [optional]",
"## More Information [optional]",
"## Dataset Card Authors [optional]",
"## Dataset Card Contact"
] |
49543f489d58727af933d6530e8b2aa3f342868b |
# Dataset Card for Evaluation run of ewqr2130/llama2-7b-raw-sft
<!-- Provide a quick summary of the dataset. -->
Dataset automatically created during the evaluation run of model [ewqr2130/llama2-7b-raw-sft](https://huggingface.co/ewqr2130/llama2-7b-raw-sft) on the [Open LLM Leaderboard](https://huggingface.co/spaces/HuggingFaceH4/open_llm_leaderboard).
The dataset is composed of 63 configuration, each one coresponding to one of the evaluated task.
The dataset has been created from 1 run(s). Each run can be found as a specific split in each configuration, the split being named using the timestamp of the run.The "train" split is always pointing to the latest results.
An additional configuration "results" store all the aggregated results of the run (and is used to compute and display the aggregated metrics on the [Open LLM Leaderboard](https://huggingface.co/spaces/HuggingFaceH4/open_llm_leaderboard)).
To load the details from a run, you can for instance do the following:
```python
from datasets import load_dataset
data = load_dataset("open-llm-leaderboard/details_ewqr2130__llama2-7b-raw-sft",
"harness_winogrande_5",
split="train")
```
## Latest results
These are the [latest results from run 2024-01-10T15:15:16.030532](https://huggingface.co/datasets/open-llm-leaderboard/details_ewqr2130__llama2-7b-raw-sft/blob/main/results_2024-01-10T15-15-16.030532.json)(note that their might be results for other tasks in the repos if successive evals didn't cover the same tasks. You find each in the results and the "latest" split for each eval):
```python
{
"all": {
"acc": 0.3451686041304389,
"acc_stderr": 0.033177024770114395,
"acc_norm": 0.34794617103590064,
"acc_norm_stderr": 0.033992606612009306,
"mc1": 0.2521419828641371,
"mc1_stderr": 0.015201522246299963,
"mc2": 0.4077071941467522,
"mc2_stderr": 0.014214727907656348
},
"harness|arc:challenge|25": {
"acc": 0.43430034129692835,
"acc_stderr": 0.01448470304885736,
"acc_norm": 0.47440273037542663,
"acc_norm_stderr": 0.014592230885298964
},
"harness|hellaswag|10": {
"acc": 0.5518820952001593,
"acc_stderr": 0.004962846206125493,
"acc_norm": 0.7525393347938658,
"acc_norm_stderr": 0.004306547156331412
},
"harness|hendrycksTest-abstract_algebra|5": {
"acc": 0.26,
"acc_stderr": 0.04408440022768081,
"acc_norm": 0.26,
"acc_norm_stderr": 0.04408440022768081
},
"harness|hendrycksTest-anatomy|5": {
"acc": 0.37037037037037035,
"acc_stderr": 0.04171654161354543,
"acc_norm": 0.37037037037037035,
"acc_norm_stderr": 0.04171654161354543
},
"harness|hendrycksTest-astronomy|5": {
"acc": 0.28289473684210525,
"acc_stderr": 0.03665349695640767,
"acc_norm": 0.28289473684210525,
"acc_norm_stderr": 0.03665349695640767
},
"harness|hendrycksTest-business_ethics|5": {
"acc": 0.37,
"acc_stderr": 0.04852365870939099,
"acc_norm": 0.37,
"acc_norm_stderr": 0.04852365870939099
},
"harness|hendrycksTest-clinical_knowledge|5": {
"acc": 0.41509433962264153,
"acc_stderr": 0.03032594578928611,
"acc_norm": 0.41509433962264153,
"acc_norm_stderr": 0.03032594578928611
},
"harness|hendrycksTest-college_biology|5": {
"acc": 0.3402777777777778,
"acc_stderr": 0.039621355734862175,
"acc_norm": 0.3402777777777778,
"acc_norm_stderr": 0.039621355734862175
},
"harness|hendrycksTest-college_chemistry|5": {
"acc": 0.23,
"acc_stderr": 0.04229525846816505,
"acc_norm": 0.23,
"acc_norm_stderr": 0.04229525846816505
},
"harness|hendrycksTest-college_computer_science|5": {
"acc": 0.27,
"acc_stderr": 0.04461960433384741,
"acc_norm": 0.27,
"acc_norm_stderr": 0.04461960433384741
},
"harness|hendrycksTest-college_mathematics|5": {
"acc": 0.22,
"acc_stderr": 0.041633319989322695,
"acc_norm": 0.22,
"acc_norm_stderr": 0.041633319989322695
},
"harness|hendrycksTest-college_medicine|5": {
"acc": 0.32947976878612717,
"acc_stderr": 0.035839017547364106,
"acc_norm": 0.32947976878612717,
"acc_norm_stderr": 0.035839017547364106
},
"harness|hendrycksTest-college_physics|5": {
"acc": 0.19607843137254902,
"acc_stderr": 0.03950581861179961,
"acc_norm": 0.19607843137254902,
"acc_norm_stderr": 0.03950581861179961
},
"harness|hendrycksTest-computer_security|5": {
"acc": 0.34,
"acc_stderr": 0.04760952285695235,
"acc_norm": 0.34,
"acc_norm_stderr": 0.04760952285695235
},
"harness|hendrycksTest-conceptual_physics|5": {
"acc": 0.32340425531914896,
"acc_stderr": 0.030579442773610334,
"acc_norm": 0.32340425531914896,
"acc_norm_stderr": 0.030579442773610334
},
"harness|hendrycksTest-econometrics|5": {
"acc": 0.2631578947368421,
"acc_stderr": 0.041424397194893624,
"acc_norm": 0.2631578947368421,
"acc_norm_stderr": 0.041424397194893624
},
"harness|hendrycksTest-electrical_engineering|5": {
"acc": 0.2413793103448276,
"acc_stderr": 0.03565998174135302,
"acc_norm": 0.2413793103448276,
"acc_norm_stderr": 0.03565998174135302
},
"harness|hendrycksTest-elementary_mathematics|5": {
"acc": 0.2566137566137566,
"acc_stderr": 0.022494510767503154,
"acc_norm": 0.2566137566137566,
"acc_norm_stderr": 0.022494510767503154
},
"harness|hendrycksTest-formal_logic|5": {
"acc": 0.1746031746031746,
"acc_stderr": 0.03395490020856112,
"acc_norm": 0.1746031746031746,
"acc_norm_stderr": 0.03395490020856112
},
"harness|hendrycksTest-global_facts|5": {
"acc": 0.33,
"acc_stderr": 0.047258156262526045,
"acc_norm": 0.33,
"acc_norm_stderr": 0.047258156262526045
},
"harness|hendrycksTest-high_school_biology|5": {
"acc": 0.4258064516129032,
"acc_stderr": 0.0281291127091659,
"acc_norm": 0.4258064516129032,
"acc_norm_stderr": 0.0281291127091659
},
"harness|hendrycksTest-high_school_chemistry|5": {
"acc": 0.3103448275862069,
"acc_stderr": 0.032550867699701024,
"acc_norm": 0.3103448275862069,
"acc_norm_stderr": 0.032550867699701024
},
"harness|hendrycksTest-high_school_computer_science|5": {
"acc": 0.28,
"acc_stderr": 0.045126085985421276,
"acc_norm": 0.28,
"acc_norm_stderr": 0.045126085985421276
},
"harness|hendrycksTest-high_school_european_history|5": {
"acc": 0.44242424242424244,
"acc_stderr": 0.03878372113711275,
"acc_norm": 0.44242424242424244,
"acc_norm_stderr": 0.03878372113711275
},
"harness|hendrycksTest-high_school_geography|5": {
"acc": 0.35858585858585856,
"acc_stderr": 0.03416903640391521,
"acc_norm": 0.35858585858585856,
"acc_norm_stderr": 0.03416903640391521
},
"harness|hendrycksTest-high_school_government_and_politics|5": {
"acc": 0.49222797927461137,
"acc_stderr": 0.036080032255696545,
"acc_norm": 0.49222797927461137,
"acc_norm_stderr": 0.036080032255696545
},
"harness|hendrycksTest-high_school_macroeconomics|5": {
"acc": 0.3384615384615385,
"acc_stderr": 0.02399150050031304,
"acc_norm": 0.3384615384615385,
"acc_norm_stderr": 0.02399150050031304
},
"harness|hendrycksTest-high_school_mathematics|5": {
"acc": 0.26296296296296295,
"acc_stderr": 0.02684205787383371,
"acc_norm": 0.26296296296296295,
"acc_norm_stderr": 0.02684205787383371
},
"harness|hendrycksTest-high_school_microeconomics|5": {
"acc": 0.36134453781512604,
"acc_stderr": 0.031204691225150013,
"acc_norm": 0.36134453781512604,
"acc_norm_stderr": 0.031204691225150013
},
"harness|hendrycksTest-high_school_physics|5": {
"acc": 0.33112582781456956,
"acc_stderr": 0.038425817186598696,
"acc_norm": 0.33112582781456956,
"acc_norm_stderr": 0.038425817186598696
},
"harness|hendrycksTest-high_school_psychology|5": {
"acc": 0.3724770642201835,
"acc_stderr": 0.020728368457638494,
"acc_norm": 0.3724770642201835,
"acc_norm_stderr": 0.020728368457638494
},
"harness|hendrycksTest-high_school_statistics|5": {
"acc": 0.46296296296296297,
"acc_stderr": 0.03400603625538272,
"acc_norm": 0.46296296296296297,
"acc_norm_stderr": 0.03400603625538272
},
"harness|hendrycksTest-high_school_us_history|5": {
"acc": 0.4019607843137255,
"acc_stderr": 0.034411900234824655,
"acc_norm": 0.4019607843137255,
"acc_norm_stderr": 0.034411900234824655
},
"harness|hendrycksTest-high_school_world_history|5": {
"acc": 0.3755274261603376,
"acc_stderr": 0.03152256243091156,
"acc_norm": 0.3755274261603376,
"acc_norm_stderr": 0.03152256243091156
},
"harness|hendrycksTest-human_aging|5": {
"acc": 0.33183856502242154,
"acc_stderr": 0.031602951437766785,
"acc_norm": 0.33183856502242154,
"acc_norm_stderr": 0.031602951437766785
},
"harness|hendrycksTest-human_sexuality|5": {
"acc": 0.35877862595419846,
"acc_stderr": 0.04206739313864908,
"acc_norm": 0.35877862595419846,
"acc_norm_stderr": 0.04206739313864908
},
"harness|hendrycksTest-international_law|5": {
"acc": 0.39669421487603307,
"acc_stderr": 0.04465869780531009,
"acc_norm": 0.39669421487603307,
"acc_norm_stderr": 0.04465869780531009
},
"harness|hendrycksTest-jurisprudence|5": {
"acc": 0.3611111111111111,
"acc_stderr": 0.04643454608906275,
"acc_norm": 0.3611111111111111,
"acc_norm_stderr": 0.04643454608906275
},
"harness|hendrycksTest-logical_fallacies|5": {
"acc": 0.3312883435582822,
"acc_stderr": 0.03697983910025588,
"acc_norm": 0.3312883435582822,
"acc_norm_stderr": 0.03697983910025588
},
"harness|hendrycksTest-machine_learning|5": {
"acc": 0.17857142857142858,
"acc_stderr": 0.036352091215778065,
"acc_norm": 0.17857142857142858,
"acc_norm_stderr": 0.036352091215778065
},
"harness|hendrycksTest-management|5": {
"acc": 0.36893203883495146,
"acc_stderr": 0.04777615181156739,
"acc_norm": 0.36893203883495146,
"acc_norm_stderr": 0.04777615181156739
},
"harness|hendrycksTest-marketing|5": {
"acc": 0.5085470085470085,
"acc_stderr": 0.0327513030009703,
"acc_norm": 0.5085470085470085,
"acc_norm_stderr": 0.0327513030009703
},
"harness|hendrycksTest-medical_genetics|5": {
"acc": 0.33,
"acc_stderr": 0.04725815626252604,
"acc_norm": 0.33,
"acc_norm_stderr": 0.04725815626252604
},
"harness|hendrycksTest-miscellaneous|5": {
"acc": 0.4240102171136654,
"acc_stderr": 0.017672263329084226,
"acc_norm": 0.4240102171136654,
"acc_norm_stderr": 0.017672263329084226
},
"harness|hendrycksTest-moral_disputes|5": {
"acc": 0.2543352601156069,
"acc_stderr": 0.023445826276545543,
"acc_norm": 0.2543352601156069,
"acc_norm_stderr": 0.023445826276545543
},
"harness|hendrycksTest-moral_scenarios|5": {
"acc": 0.2424581005586592,
"acc_stderr": 0.014333522059217889,
"acc_norm": 0.2424581005586592,
"acc_norm_stderr": 0.014333522059217889
},
"harness|hendrycksTest-nutrition|5": {
"acc": 0.4117647058823529,
"acc_stderr": 0.028180596328259293,
"acc_norm": 0.4117647058823529,
"acc_norm_stderr": 0.028180596328259293
},
"harness|hendrycksTest-philosophy|5": {
"acc": 0.34726688102893893,
"acc_stderr": 0.027040745502307336,
"acc_norm": 0.34726688102893893,
"acc_norm_stderr": 0.027040745502307336
},
"harness|hendrycksTest-prehistory|5": {
"acc": 0.31790123456790126,
"acc_stderr": 0.02591006352824088,
"acc_norm": 0.31790123456790126,
"acc_norm_stderr": 0.02591006352824088
},
"harness|hendrycksTest-professional_accounting|5": {
"acc": 0.28368794326241137,
"acc_stderr": 0.02689170942834396,
"acc_norm": 0.28368794326241137,
"acc_norm_stderr": 0.02689170942834396
},
"harness|hendrycksTest-professional_law|5": {
"acc": 0.2940026075619296,
"acc_stderr": 0.011636062953698604,
"acc_norm": 0.2940026075619296,
"acc_norm_stderr": 0.011636062953698604
},
"harness|hendrycksTest-professional_medicine|5": {
"acc": 0.4632352941176471,
"acc_stderr": 0.030290619180485687,
"acc_norm": 0.4632352941176471,
"acc_norm_stderr": 0.030290619180485687
},
"harness|hendrycksTest-professional_psychology|5": {
"acc": 0.28431372549019607,
"acc_stderr": 0.018249024411207668,
"acc_norm": 0.28431372549019607,
"acc_norm_stderr": 0.018249024411207668
},
"harness|hendrycksTest-public_relations|5": {
"acc": 0.42727272727272725,
"acc_stderr": 0.04738198703545483,
"acc_norm": 0.42727272727272725,
"acc_norm_stderr": 0.04738198703545483
},
"harness|hendrycksTest-security_studies|5": {
"acc": 0.3877551020408163,
"acc_stderr": 0.031192230726795656,
"acc_norm": 0.3877551020408163,
"acc_norm_stderr": 0.031192230726795656
},
"harness|hendrycksTest-sociology|5": {
"acc": 0.43283582089552236,
"acc_stderr": 0.03503490923673281,
"acc_norm": 0.43283582089552236,
"acc_norm_stderr": 0.03503490923673281
},
"harness|hendrycksTest-us_foreign_policy|5": {
"acc": 0.4,
"acc_stderr": 0.04923659639173309,
"acc_norm": 0.4,
"acc_norm_stderr": 0.04923659639173309
},
"harness|hendrycksTest-virology|5": {
"acc": 0.3614457831325301,
"acc_stderr": 0.0374005938202932,
"acc_norm": 0.3614457831325301,
"acc_norm_stderr": 0.0374005938202932
},
"harness|hendrycksTest-world_religions|5": {
"acc": 0.3742690058479532,
"acc_stderr": 0.03711601185389481,
"acc_norm": 0.3742690058479532,
"acc_norm_stderr": 0.03711601185389481
},
"harness|truthfulqa:mc|0": {
"mc1": 0.2521419828641371,
"mc1_stderr": 0.015201522246299963,
"mc2": 0.4077071941467522,
"mc2_stderr": 0.014214727907656348
},
"harness|winogrande|5": {
"acc": 0.7300710339384373,
"acc_stderr": 0.012476433372002608
},
"harness|gsm8k|5": {
"acc": 0.037149355572403335,
"acc_stderr": 0.005209516283073736
}
}
```
## Dataset Details
### Dataset Description
<!-- Provide a longer summary of what this dataset is. -->
- **Curated by:** [More Information Needed]
- **Funded by [optional]:** [More Information Needed]
- **Shared by [optional]:** [More Information Needed]
- **Language(s) (NLP):** [More Information Needed]
- **License:** [More Information Needed]
### Dataset Sources [optional]
<!-- Provide the basic links for the dataset. -->
- **Repository:** [More Information Needed]
- **Paper [optional]:** [More Information Needed]
- **Demo [optional]:** [More Information Needed]
## Uses
<!-- Address questions around how the dataset is intended to be used. -->
### Direct Use
<!-- This section describes suitable use cases for the dataset. -->
[More Information Needed]
### Out-of-Scope Use
<!-- This section addresses misuse, malicious use, and uses that the dataset will not work well for. -->
[More Information Needed]
## Dataset Structure
<!-- This section provides a description of the dataset fields, and additional information about the dataset structure such as criteria used to create the splits, relationships between data points, etc. -->
[More Information Needed]
## Dataset Creation
### Curation Rationale
<!-- Motivation for the creation of this dataset. -->
[More Information Needed]
### Source Data
<!-- This section describes the source data (e.g. news text and headlines, social media posts, translated sentences, ...). -->
#### Data Collection and Processing
<!-- This section describes the data collection and processing process such as data selection criteria, filtering and normalization methods, tools and libraries used, etc. -->
[More Information Needed]
#### Who are the source data producers?
<!-- This section describes the people or systems who originally created the data. It should also include self-reported demographic or identity information for the source data creators if this information is available. -->
[More Information Needed]
### Annotations [optional]
<!-- If the dataset contains annotations which are not part of the initial data collection, use this section to describe them. -->
#### Annotation process
<!-- This section describes the annotation process such as annotation tools used in the process, the amount of data annotated, annotation guidelines provided to the annotators, interannotator statistics, annotation validation, etc. -->
[More Information Needed]
#### Who are the annotators?
<!-- This section describes the people or systems who created the annotations. -->
[More Information Needed]
#### Personal and Sensitive Information
<!-- State whether the dataset contains data that might be considered personal, sensitive, or private (e.g., data that reveals addresses, uniquely identifiable names or aliases, racial or ethnic origins, sexual orientations, religious beliefs, political opinions, financial or health data, etc.). If efforts were made to anonymize the data, describe the anonymization process. -->
[More Information Needed]
## Bias, Risks, and Limitations
<!-- This section is meant to convey both technical and sociotechnical limitations. -->
[More Information Needed]
### Recommendations
<!-- This section is meant to convey recommendations with respect to the bias, risk, and technical limitations. -->
Users should be made aware of the risks, biases and limitations of the dataset. More information needed for further recommendations.
## Citation [optional]
<!-- If there is a paper or blog post introducing the dataset, the APA and Bibtex information for that should go in this section. -->
**BibTeX:**
[More Information Needed]
**APA:**
[More Information Needed]
## Glossary [optional]
<!-- If relevant, include terms and calculations in this section that can help readers understand the dataset or dataset card. -->
[More Information Needed]
## More Information [optional]
[More Information Needed]
## Dataset Card Authors [optional]
[More Information Needed]
## Dataset Card Contact
[More Information Needed] | open-llm-leaderboard/details_ewqr2130__llama2-7b-raw-sft | [
"region:us"
] | 2024-01-10T15:17:37+00:00 | {"pretty_name": "Evaluation run of ewqr2130/llama2-7b-raw-sft", "dataset_summary": "Dataset automatically created during the evaluation run of model [ewqr2130/llama2-7b-raw-sft](https://huggingface.co/ewqr2130/llama2-7b-raw-sft) on the [Open LLM Leaderboard](https://huggingface.co/spaces/HuggingFaceH4/open_llm_leaderboard).\n\nThe dataset is composed of 63 configuration, each one coresponding to one of the evaluated task.\n\nThe dataset has been created from 1 run(s). Each run can be found as a specific split in each configuration, the split being named using the timestamp of the run.The \"train\" split is always pointing to the latest results.\n\nAn additional configuration \"results\" store all the aggregated results of the run (and is used to compute and display the aggregated metrics on the [Open LLM Leaderboard](https://huggingface.co/spaces/HuggingFaceH4/open_llm_leaderboard)).\n\nTo load the details from a run, you can for instance do the following:\n```python\nfrom datasets import load_dataset\ndata = load_dataset(\"open-llm-leaderboard/details_ewqr2130__llama2-7b-raw-sft\",\n\t\"harness_winogrande_5\",\n\tsplit=\"train\")\n```\n\n## Latest results\n\nThese are the [latest results from run 2024-01-10T15:15:16.030532](https://huggingface.co/datasets/open-llm-leaderboard/details_ewqr2130__llama2-7b-raw-sft/blob/main/results_2024-01-10T15-15-16.030532.json)(note that their might be results for other tasks in the repos if successive evals didn't cover the same tasks. You find each in the results and the \"latest\" split for each eval):\n\n```python\n{\n \"all\": {\n \"acc\": 0.3451686041304389,\n \"acc_stderr\": 0.033177024770114395,\n \"acc_norm\": 0.34794617103590064,\n \"acc_norm_stderr\": 0.033992606612009306,\n \"mc1\": 0.2521419828641371,\n \"mc1_stderr\": 0.015201522246299963,\n \"mc2\": 0.4077071941467522,\n \"mc2_stderr\": 0.014214727907656348\n },\n \"harness|arc:challenge|25\": {\n \"acc\": 0.43430034129692835,\n \"acc_stderr\": 0.01448470304885736,\n \"acc_norm\": 0.47440273037542663,\n \"acc_norm_stderr\": 0.014592230885298964\n },\n \"harness|hellaswag|10\": {\n \"acc\": 0.5518820952001593,\n \"acc_stderr\": 0.004962846206125493,\n \"acc_norm\": 0.7525393347938658,\n \"acc_norm_stderr\": 0.004306547156331412\n },\n \"harness|hendrycksTest-abstract_algebra|5\": {\n \"acc\": 0.26,\n \"acc_stderr\": 0.04408440022768081,\n \"acc_norm\": 0.26,\n \"acc_norm_stderr\": 0.04408440022768081\n },\n \"harness|hendrycksTest-anatomy|5\": {\n \"acc\": 0.37037037037037035,\n \"acc_stderr\": 0.04171654161354543,\n \"acc_norm\": 0.37037037037037035,\n \"acc_norm_stderr\": 0.04171654161354543\n },\n \"harness|hendrycksTest-astronomy|5\": {\n \"acc\": 0.28289473684210525,\n \"acc_stderr\": 0.03665349695640767,\n \"acc_norm\": 0.28289473684210525,\n \"acc_norm_stderr\": 0.03665349695640767\n },\n \"harness|hendrycksTest-business_ethics|5\": {\n \"acc\": 0.37,\n \"acc_stderr\": 0.04852365870939099,\n \"acc_norm\": 0.37,\n \"acc_norm_stderr\": 0.04852365870939099\n },\n \"harness|hendrycksTest-clinical_knowledge|5\": {\n \"acc\": 0.41509433962264153,\n \"acc_stderr\": 0.03032594578928611,\n \"acc_norm\": 0.41509433962264153,\n \"acc_norm_stderr\": 0.03032594578928611\n },\n \"harness|hendrycksTest-college_biology|5\": {\n \"acc\": 0.3402777777777778,\n \"acc_stderr\": 0.039621355734862175,\n \"acc_norm\": 0.3402777777777778,\n \"acc_norm_stderr\": 0.039621355734862175\n },\n \"harness|hendrycksTest-college_chemistry|5\": {\n \"acc\": 0.23,\n \"acc_stderr\": 0.04229525846816505,\n \"acc_norm\": 0.23,\n \"acc_norm_stderr\": 0.04229525846816505\n },\n \"harness|hendrycksTest-college_computer_science|5\": {\n \"acc\": 0.27,\n \"acc_stderr\": 0.04461960433384741,\n \"acc_norm\": 0.27,\n \"acc_norm_stderr\": 0.04461960433384741\n },\n \"harness|hendrycksTest-college_mathematics|5\": {\n \"acc\": 0.22,\n \"acc_stderr\": 0.041633319989322695,\n \"acc_norm\": 0.22,\n \"acc_norm_stderr\": 0.041633319989322695\n },\n \"harness|hendrycksTest-college_medicine|5\": {\n \"acc\": 0.32947976878612717,\n \"acc_stderr\": 0.035839017547364106,\n \"acc_norm\": 0.32947976878612717,\n \"acc_norm_stderr\": 0.035839017547364106\n },\n \"harness|hendrycksTest-college_physics|5\": {\n \"acc\": 0.19607843137254902,\n \"acc_stderr\": 0.03950581861179961,\n \"acc_norm\": 0.19607843137254902,\n \"acc_norm_stderr\": 0.03950581861179961\n },\n \"harness|hendrycksTest-computer_security|5\": {\n \"acc\": 0.34,\n \"acc_stderr\": 0.04760952285695235,\n \"acc_norm\": 0.34,\n \"acc_norm_stderr\": 0.04760952285695235\n },\n \"harness|hendrycksTest-conceptual_physics|5\": {\n \"acc\": 0.32340425531914896,\n \"acc_stderr\": 0.030579442773610334,\n \"acc_norm\": 0.32340425531914896,\n \"acc_norm_stderr\": 0.030579442773610334\n },\n \"harness|hendrycksTest-econometrics|5\": {\n \"acc\": 0.2631578947368421,\n \"acc_stderr\": 0.041424397194893624,\n \"acc_norm\": 0.2631578947368421,\n \"acc_norm_stderr\": 0.041424397194893624\n },\n \"harness|hendrycksTest-electrical_engineering|5\": {\n \"acc\": 0.2413793103448276,\n \"acc_stderr\": 0.03565998174135302,\n \"acc_norm\": 0.2413793103448276,\n \"acc_norm_stderr\": 0.03565998174135302\n },\n \"harness|hendrycksTest-elementary_mathematics|5\": {\n \"acc\": 0.2566137566137566,\n \"acc_stderr\": 0.022494510767503154,\n \"acc_norm\": 0.2566137566137566,\n \"acc_norm_stderr\": 0.022494510767503154\n },\n \"harness|hendrycksTest-formal_logic|5\": {\n \"acc\": 0.1746031746031746,\n \"acc_stderr\": 0.03395490020856112,\n \"acc_norm\": 0.1746031746031746,\n \"acc_norm_stderr\": 0.03395490020856112\n },\n \"harness|hendrycksTest-global_facts|5\": {\n \"acc\": 0.33,\n \"acc_stderr\": 0.047258156262526045,\n \"acc_norm\": 0.33,\n \"acc_norm_stderr\": 0.047258156262526045\n },\n \"harness|hendrycksTest-high_school_biology|5\": {\n \"acc\": 0.4258064516129032,\n \"acc_stderr\": 0.0281291127091659,\n \"acc_norm\": 0.4258064516129032,\n \"acc_norm_stderr\": 0.0281291127091659\n },\n \"harness|hendrycksTest-high_school_chemistry|5\": {\n \"acc\": 0.3103448275862069,\n \"acc_stderr\": 0.032550867699701024,\n \"acc_norm\": 0.3103448275862069,\n \"acc_norm_stderr\": 0.032550867699701024\n },\n \"harness|hendrycksTest-high_school_computer_science|5\": {\n \"acc\": 0.28,\n \"acc_stderr\": 0.045126085985421276,\n \"acc_norm\": 0.28,\n \"acc_norm_stderr\": 0.045126085985421276\n },\n \"harness|hendrycksTest-high_school_european_history|5\": {\n \"acc\": 0.44242424242424244,\n \"acc_stderr\": 0.03878372113711275,\n \"acc_norm\": 0.44242424242424244,\n \"acc_norm_stderr\": 0.03878372113711275\n },\n \"harness|hendrycksTest-high_school_geography|5\": {\n \"acc\": 0.35858585858585856,\n \"acc_stderr\": 0.03416903640391521,\n \"acc_norm\": 0.35858585858585856,\n \"acc_norm_stderr\": 0.03416903640391521\n },\n \"harness|hendrycksTest-high_school_government_and_politics|5\": {\n \"acc\": 0.49222797927461137,\n \"acc_stderr\": 0.036080032255696545,\n \"acc_norm\": 0.49222797927461137,\n \"acc_norm_stderr\": 0.036080032255696545\n },\n \"harness|hendrycksTest-high_school_macroeconomics|5\": {\n \"acc\": 0.3384615384615385,\n \"acc_stderr\": 0.02399150050031304,\n \"acc_norm\": 0.3384615384615385,\n \"acc_norm_stderr\": 0.02399150050031304\n },\n \"harness|hendrycksTest-high_school_mathematics|5\": {\n \"acc\": 0.26296296296296295,\n \"acc_stderr\": 0.02684205787383371,\n \"acc_norm\": 0.26296296296296295,\n \"acc_norm_stderr\": 0.02684205787383371\n },\n \"harness|hendrycksTest-high_school_microeconomics|5\": {\n \"acc\": 0.36134453781512604,\n \"acc_stderr\": 0.031204691225150013,\n \"acc_norm\": 0.36134453781512604,\n \"acc_norm_stderr\": 0.031204691225150013\n },\n \"harness|hendrycksTest-high_school_physics|5\": {\n \"acc\": 0.33112582781456956,\n \"acc_stderr\": 0.038425817186598696,\n \"acc_norm\": 0.33112582781456956,\n \"acc_norm_stderr\": 0.038425817186598696\n },\n \"harness|hendrycksTest-high_school_psychology|5\": {\n \"acc\": 0.3724770642201835,\n \"acc_stderr\": 0.020728368457638494,\n \"acc_norm\": 0.3724770642201835,\n \"acc_norm_stderr\": 0.020728368457638494\n },\n \"harness|hendrycksTest-high_school_statistics|5\": {\n \"acc\": 0.46296296296296297,\n \"acc_stderr\": 0.03400603625538272,\n \"acc_norm\": 0.46296296296296297,\n \"acc_norm_stderr\": 0.03400603625538272\n },\n \"harness|hendrycksTest-high_school_us_history|5\": {\n \"acc\": 0.4019607843137255,\n \"acc_stderr\": 0.034411900234824655,\n \"acc_norm\": 0.4019607843137255,\n \"acc_norm_stderr\": 0.034411900234824655\n },\n \"harness|hendrycksTest-high_school_world_history|5\": {\n \"acc\": 0.3755274261603376,\n \"acc_stderr\": 0.03152256243091156,\n \"acc_norm\": 0.3755274261603376,\n \"acc_norm_stderr\": 0.03152256243091156\n },\n \"harness|hendrycksTest-human_aging|5\": {\n \"acc\": 0.33183856502242154,\n \"acc_stderr\": 0.031602951437766785,\n \"acc_norm\": 0.33183856502242154,\n \"acc_norm_stderr\": 0.031602951437766785\n },\n \"harness|hendrycksTest-human_sexuality|5\": {\n \"acc\": 0.35877862595419846,\n \"acc_stderr\": 0.04206739313864908,\n \"acc_norm\": 0.35877862595419846,\n \"acc_norm_stderr\": 0.04206739313864908\n },\n \"harness|hendrycksTest-international_law|5\": {\n \"acc\": 0.39669421487603307,\n \"acc_stderr\": 0.04465869780531009,\n \"acc_norm\": 0.39669421487603307,\n \"acc_norm_stderr\": 0.04465869780531009\n },\n \"harness|hendrycksTest-jurisprudence|5\": {\n \"acc\": 0.3611111111111111,\n \"acc_stderr\": 0.04643454608906275,\n \"acc_norm\": 0.3611111111111111,\n \"acc_norm_stderr\": 0.04643454608906275\n },\n \"harness|hendrycksTest-logical_fallacies|5\": {\n \"acc\": 0.3312883435582822,\n \"acc_stderr\": 0.03697983910025588,\n \"acc_norm\": 0.3312883435582822,\n \"acc_norm_stderr\": 0.03697983910025588\n },\n \"harness|hendrycksTest-machine_learning|5\": {\n \"acc\": 0.17857142857142858,\n \"acc_stderr\": 0.036352091215778065,\n \"acc_norm\": 0.17857142857142858,\n \"acc_norm_stderr\": 0.036352091215778065\n },\n \"harness|hendrycksTest-management|5\": {\n \"acc\": 0.36893203883495146,\n \"acc_stderr\": 0.04777615181156739,\n \"acc_norm\": 0.36893203883495146,\n \"acc_norm_stderr\": 0.04777615181156739\n },\n \"harness|hendrycksTest-marketing|5\": {\n \"acc\": 0.5085470085470085,\n \"acc_stderr\": 0.0327513030009703,\n \"acc_norm\": 0.5085470085470085,\n \"acc_norm_stderr\": 0.0327513030009703\n },\n \"harness|hendrycksTest-medical_genetics|5\": {\n \"acc\": 0.33,\n \"acc_stderr\": 0.04725815626252604,\n \"acc_norm\": 0.33,\n \"acc_norm_stderr\": 0.04725815626252604\n },\n \"harness|hendrycksTest-miscellaneous|5\": {\n \"acc\": 0.4240102171136654,\n \"acc_stderr\": 0.017672263329084226,\n \"acc_norm\": 0.4240102171136654,\n \"acc_norm_stderr\": 0.017672263329084226\n },\n \"harness|hendrycksTest-moral_disputes|5\": {\n \"acc\": 0.2543352601156069,\n \"acc_stderr\": 0.023445826276545543,\n \"acc_norm\": 0.2543352601156069,\n \"acc_norm_stderr\": 0.023445826276545543\n },\n \"harness|hendrycksTest-moral_scenarios|5\": {\n \"acc\": 0.2424581005586592,\n \"acc_stderr\": 0.014333522059217889,\n \"acc_norm\": 0.2424581005586592,\n \"acc_norm_stderr\": 0.014333522059217889\n },\n \"harness|hendrycksTest-nutrition|5\": {\n \"acc\": 0.4117647058823529,\n \"acc_stderr\": 0.028180596328259293,\n \"acc_norm\": 0.4117647058823529,\n \"acc_norm_stderr\": 0.028180596328259293\n },\n \"harness|hendrycksTest-philosophy|5\": {\n \"acc\": 0.34726688102893893,\n \"acc_stderr\": 0.027040745502307336,\n \"acc_norm\": 0.34726688102893893,\n \"acc_norm_stderr\": 0.027040745502307336\n },\n \"harness|hendrycksTest-prehistory|5\": {\n \"acc\": 0.31790123456790126,\n \"acc_stderr\": 0.02591006352824088,\n \"acc_norm\": 0.31790123456790126,\n \"acc_norm_stderr\": 0.02591006352824088\n },\n \"harness|hendrycksTest-professional_accounting|5\": {\n \"acc\": 0.28368794326241137,\n \"acc_stderr\": 0.02689170942834396,\n \"acc_norm\": 0.28368794326241137,\n \"acc_norm_stderr\": 0.02689170942834396\n },\n \"harness|hendrycksTest-professional_law|5\": {\n \"acc\": 0.2940026075619296,\n \"acc_stderr\": 0.011636062953698604,\n \"acc_norm\": 0.2940026075619296,\n \"acc_norm_stderr\": 0.011636062953698604\n },\n \"harness|hendrycksTest-professional_medicine|5\": {\n \"acc\": 0.4632352941176471,\n \"acc_stderr\": 0.030290619180485687,\n \"acc_norm\": 0.4632352941176471,\n \"acc_norm_stderr\": 0.030290619180485687\n },\n \"harness|hendrycksTest-professional_psychology|5\": {\n \"acc\": 0.28431372549019607,\n \"acc_stderr\": 0.018249024411207668,\n \"acc_norm\": 0.28431372549019607,\n \"acc_norm_stderr\": 0.018249024411207668\n },\n \"harness|hendrycksTest-public_relations|5\": {\n \"acc\": 0.42727272727272725,\n \"acc_stderr\": 0.04738198703545483,\n \"acc_norm\": 0.42727272727272725,\n \"acc_norm_stderr\": 0.04738198703545483\n },\n \"harness|hendrycksTest-security_studies|5\": {\n \"acc\": 0.3877551020408163,\n \"acc_stderr\": 0.031192230726795656,\n \"acc_norm\": 0.3877551020408163,\n \"acc_norm_stderr\": 0.031192230726795656\n },\n \"harness|hendrycksTest-sociology|5\": {\n \"acc\": 0.43283582089552236,\n \"acc_stderr\": 0.03503490923673281,\n \"acc_norm\": 0.43283582089552236,\n \"acc_norm_stderr\": 0.03503490923673281\n },\n \"harness|hendrycksTest-us_foreign_policy|5\": {\n \"acc\": 0.4,\n \"acc_stderr\": 0.04923659639173309,\n \"acc_norm\": 0.4,\n \"acc_norm_stderr\": 0.04923659639173309\n },\n \"harness|hendrycksTest-virology|5\": {\n \"acc\": 0.3614457831325301,\n \"acc_stderr\": 0.0374005938202932,\n \"acc_norm\": 0.3614457831325301,\n \"acc_norm_stderr\": 0.0374005938202932\n },\n \"harness|hendrycksTest-world_religions|5\": {\n \"acc\": 0.3742690058479532,\n \"acc_stderr\": 0.03711601185389481,\n \"acc_norm\": 0.3742690058479532,\n \"acc_norm_stderr\": 0.03711601185389481\n },\n \"harness|truthfulqa:mc|0\": {\n \"mc1\": 0.2521419828641371,\n \"mc1_stderr\": 0.015201522246299963,\n \"mc2\": 0.4077071941467522,\n \"mc2_stderr\": 0.014214727907656348\n },\n \"harness|winogrande|5\": {\n \"acc\": 0.7300710339384373,\n \"acc_stderr\": 0.012476433372002608\n },\n \"harness|gsm8k|5\": {\n \"acc\": 0.037149355572403335,\n \"acc_stderr\": 0.005209516283073736\n }\n}\n```", "repo_url": "https://huggingface.co/ewqr2130/llama2-7b-raw-sft", "leaderboard_url": "https://huggingface.co/spaces/HuggingFaceH4/open_llm_leaderboard", "point_of_contact": "[email protected]", "configs": [{"config_name": "harness_arc_challenge_25", "data_files": [{"split": "2024_01_10T15_15_16.030532", "path": ["**/details_harness|arc:challenge|25_2024-01-10T15-15-16.030532.parquet"]}, {"split": "latest", "path": ["**/details_harness|arc:challenge|25_2024-01-10T15-15-16.030532.parquet"]}]}, {"config_name": "harness_gsm8k_5", "data_files": [{"split": "2024_01_10T15_15_16.030532", "path": ["**/details_harness|gsm8k|5_2024-01-10T15-15-16.030532.parquet"]}, {"split": "latest", "path": ["**/details_harness|gsm8k|5_2024-01-10T15-15-16.030532.parquet"]}]}, {"config_name": "harness_hellaswag_10", "data_files": [{"split": "2024_01_10T15_15_16.030532", "path": ["**/details_harness|hellaswag|10_2024-01-10T15-15-16.030532.parquet"]}, {"split": "latest", "path": ["**/details_harness|hellaswag|10_2024-01-10T15-15-16.030532.parquet"]}]}, {"config_name": "harness_hendrycksTest_5", "data_files": [{"split": "2024_01_10T15_15_16.030532", "path": ["**/details_harness|hendrycksTest-abstract_algebra|5_2024-01-10T15-15-16.030532.parquet", "**/details_harness|hendrycksTest-anatomy|5_2024-01-10T15-15-16.030532.parquet", "**/details_harness|hendrycksTest-astronomy|5_2024-01-10T15-15-16.030532.parquet", "**/details_harness|hendrycksTest-business_ethics|5_2024-01-10T15-15-16.030532.parquet", "**/details_harness|hendrycksTest-clinical_knowledge|5_2024-01-10T15-15-16.030532.parquet", "**/details_harness|hendrycksTest-college_biology|5_2024-01-10T15-15-16.030532.parquet", "**/details_harness|hendrycksTest-college_chemistry|5_2024-01-10T15-15-16.030532.parquet", "**/details_harness|hendrycksTest-college_computer_science|5_2024-01-10T15-15-16.030532.parquet", "**/details_harness|hendrycksTest-college_mathematics|5_2024-01-10T15-15-16.030532.parquet", "**/details_harness|hendrycksTest-college_medicine|5_2024-01-10T15-15-16.030532.parquet", "**/details_harness|hendrycksTest-college_physics|5_2024-01-10T15-15-16.030532.parquet", "**/details_harness|hendrycksTest-computer_security|5_2024-01-10T15-15-16.030532.parquet", "**/details_harness|hendrycksTest-conceptual_physics|5_2024-01-10T15-15-16.030532.parquet", "**/details_harness|hendrycksTest-econometrics|5_2024-01-10T15-15-16.030532.parquet", "**/details_harness|hendrycksTest-electrical_engineering|5_2024-01-10T15-15-16.030532.parquet", "**/details_harness|hendrycksTest-elementary_mathematics|5_2024-01-10T15-15-16.030532.parquet", "**/details_harness|hendrycksTest-formal_logic|5_2024-01-10T15-15-16.030532.parquet", "**/details_harness|hendrycksTest-global_facts|5_2024-01-10T15-15-16.030532.parquet", "**/details_harness|hendrycksTest-high_school_biology|5_2024-01-10T15-15-16.030532.parquet", "**/details_harness|hendrycksTest-high_school_chemistry|5_2024-01-10T15-15-16.030532.parquet", "**/details_harness|hendrycksTest-high_school_computer_science|5_2024-01-10T15-15-16.030532.parquet", "**/details_harness|hendrycksTest-high_school_european_history|5_2024-01-10T15-15-16.030532.parquet", "**/details_harness|hendrycksTest-high_school_geography|5_2024-01-10T15-15-16.030532.parquet", "**/details_harness|hendrycksTest-high_school_government_and_politics|5_2024-01-10T15-15-16.030532.parquet", "**/details_harness|hendrycksTest-high_school_macroeconomics|5_2024-01-10T15-15-16.030532.parquet", "**/details_harness|hendrycksTest-high_school_mathematics|5_2024-01-10T15-15-16.030532.parquet", "**/details_harness|hendrycksTest-high_school_microeconomics|5_2024-01-10T15-15-16.030532.parquet", "**/details_harness|hendrycksTest-high_school_physics|5_2024-01-10T15-15-16.030532.parquet", "**/details_harness|hendrycksTest-high_school_psychology|5_2024-01-10T15-15-16.030532.parquet", "**/details_harness|hendrycksTest-high_school_statistics|5_2024-01-10T15-15-16.030532.parquet", "**/details_harness|hendrycksTest-high_school_us_history|5_2024-01-10T15-15-16.030532.parquet", "**/details_harness|hendrycksTest-high_school_world_history|5_2024-01-10T15-15-16.030532.parquet", "**/details_harness|hendrycksTest-human_aging|5_2024-01-10T15-15-16.030532.parquet", "**/details_harness|hendrycksTest-human_sexuality|5_2024-01-10T15-15-16.030532.parquet", "**/details_harness|hendrycksTest-international_law|5_2024-01-10T15-15-16.030532.parquet", "**/details_harness|hendrycksTest-jurisprudence|5_2024-01-10T15-15-16.030532.parquet", "**/details_harness|hendrycksTest-logical_fallacies|5_2024-01-10T15-15-16.030532.parquet", "**/details_harness|hendrycksTest-machine_learning|5_2024-01-10T15-15-16.030532.parquet", "**/details_harness|hendrycksTest-management|5_2024-01-10T15-15-16.030532.parquet", "**/details_harness|hendrycksTest-marketing|5_2024-01-10T15-15-16.030532.parquet", "**/details_harness|hendrycksTest-medical_genetics|5_2024-01-10T15-15-16.030532.parquet", "**/details_harness|hendrycksTest-miscellaneous|5_2024-01-10T15-15-16.030532.parquet", "**/details_harness|hendrycksTest-moral_disputes|5_2024-01-10T15-15-16.030532.parquet", "**/details_harness|hendrycksTest-moral_scenarios|5_2024-01-10T15-15-16.030532.parquet", "**/details_harness|hendrycksTest-nutrition|5_2024-01-10T15-15-16.030532.parquet", "**/details_harness|hendrycksTest-philosophy|5_2024-01-10T15-15-16.030532.parquet", "**/details_harness|hendrycksTest-prehistory|5_2024-01-10T15-15-16.030532.parquet", "**/details_harness|hendrycksTest-professional_accounting|5_2024-01-10T15-15-16.030532.parquet", "**/details_harness|hendrycksTest-professional_law|5_2024-01-10T15-15-16.030532.parquet", "**/details_harness|hendrycksTest-professional_medicine|5_2024-01-10T15-15-16.030532.parquet", "**/details_harness|hendrycksTest-professional_psychology|5_2024-01-10T15-15-16.030532.parquet", "**/details_harness|hendrycksTest-public_relations|5_2024-01-10T15-15-16.030532.parquet", "**/details_harness|hendrycksTest-security_studies|5_2024-01-10T15-15-16.030532.parquet", "**/details_harness|hendrycksTest-sociology|5_2024-01-10T15-15-16.030532.parquet", "**/details_harness|hendrycksTest-us_foreign_policy|5_2024-01-10T15-15-16.030532.parquet", "**/details_harness|hendrycksTest-virology|5_2024-01-10T15-15-16.030532.parquet", "**/details_harness|hendrycksTest-world_religions|5_2024-01-10T15-15-16.030532.parquet"]}, {"split": "latest", "path": ["**/details_harness|hendrycksTest-abstract_algebra|5_2024-01-10T15-15-16.030532.parquet", "**/details_harness|hendrycksTest-anatomy|5_2024-01-10T15-15-16.030532.parquet", "**/details_harness|hendrycksTest-astronomy|5_2024-01-10T15-15-16.030532.parquet", "**/details_harness|hendrycksTest-business_ethics|5_2024-01-10T15-15-16.030532.parquet", "**/details_harness|hendrycksTest-clinical_knowledge|5_2024-01-10T15-15-16.030532.parquet", "**/details_harness|hendrycksTest-college_biology|5_2024-01-10T15-15-16.030532.parquet", "**/details_harness|hendrycksTest-college_chemistry|5_2024-01-10T15-15-16.030532.parquet", "**/details_harness|hendrycksTest-college_computer_science|5_2024-01-10T15-15-16.030532.parquet", "**/details_harness|hendrycksTest-college_mathematics|5_2024-01-10T15-15-16.030532.parquet", "**/details_harness|hendrycksTest-college_medicine|5_2024-01-10T15-15-16.030532.parquet", "**/details_harness|hendrycksTest-college_physics|5_2024-01-10T15-15-16.030532.parquet", "**/details_harness|hendrycksTest-computer_security|5_2024-01-10T15-15-16.030532.parquet", "**/details_harness|hendrycksTest-conceptual_physics|5_2024-01-10T15-15-16.030532.parquet", "**/details_harness|hendrycksTest-econometrics|5_2024-01-10T15-15-16.030532.parquet", "**/details_harness|hendrycksTest-electrical_engineering|5_2024-01-10T15-15-16.030532.parquet", "**/details_harness|hendrycksTest-elementary_mathematics|5_2024-01-10T15-15-16.030532.parquet", "**/details_harness|hendrycksTest-formal_logic|5_2024-01-10T15-15-16.030532.parquet", "**/details_harness|hendrycksTest-global_facts|5_2024-01-10T15-15-16.030532.parquet", "**/details_harness|hendrycksTest-high_school_biology|5_2024-01-10T15-15-16.030532.parquet", "**/details_harness|hendrycksTest-high_school_chemistry|5_2024-01-10T15-15-16.030532.parquet", "**/details_harness|hendrycksTest-high_school_computer_science|5_2024-01-10T15-15-16.030532.parquet", "**/details_harness|hendrycksTest-high_school_european_history|5_2024-01-10T15-15-16.030532.parquet", "**/details_harness|hendrycksTest-high_school_geography|5_2024-01-10T15-15-16.030532.parquet", "**/details_harness|hendrycksTest-high_school_government_and_politics|5_2024-01-10T15-15-16.030532.parquet", "**/details_harness|hendrycksTest-high_school_macroeconomics|5_2024-01-10T15-15-16.030532.parquet", "**/details_harness|hendrycksTest-high_school_mathematics|5_2024-01-10T15-15-16.030532.parquet", "**/details_harness|hendrycksTest-high_school_microeconomics|5_2024-01-10T15-15-16.030532.parquet", "**/details_harness|hendrycksTest-high_school_physics|5_2024-01-10T15-15-16.030532.parquet", "**/details_harness|hendrycksTest-high_school_psychology|5_2024-01-10T15-15-16.030532.parquet", "**/details_harness|hendrycksTest-high_school_statistics|5_2024-01-10T15-15-16.030532.parquet", "**/details_harness|hendrycksTest-high_school_us_history|5_2024-01-10T15-15-16.030532.parquet", "**/details_harness|hendrycksTest-high_school_world_history|5_2024-01-10T15-15-16.030532.parquet", "**/details_harness|hendrycksTest-human_aging|5_2024-01-10T15-15-16.030532.parquet", "**/details_harness|hendrycksTest-human_sexuality|5_2024-01-10T15-15-16.030532.parquet", "**/details_harness|hendrycksTest-international_law|5_2024-01-10T15-15-16.030532.parquet", "**/details_harness|hendrycksTest-jurisprudence|5_2024-01-10T15-15-16.030532.parquet", "**/details_harness|hendrycksTest-logical_fallacies|5_2024-01-10T15-15-16.030532.parquet", "**/details_harness|hendrycksTest-machine_learning|5_2024-01-10T15-15-16.030532.parquet", "**/details_harness|hendrycksTest-management|5_2024-01-10T15-15-16.030532.parquet", "**/details_harness|hendrycksTest-marketing|5_2024-01-10T15-15-16.030532.parquet", "**/details_harness|hendrycksTest-medical_genetics|5_2024-01-10T15-15-16.030532.parquet", "**/details_harness|hendrycksTest-miscellaneous|5_2024-01-10T15-15-16.030532.parquet", "**/details_harness|hendrycksTest-moral_disputes|5_2024-01-10T15-15-16.030532.parquet", "**/details_harness|hendrycksTest-moral_scenarios|5_2024-01-10T15-15-16.030532.parquet", "**/details_harness|hendrycksTest-nutrition|5_2024-01-10T15-15-16.030532.parquet", "**/details_harness|hendrycksTest-philosophy|5_2024-01-10T15-15-16.030532.parquet", "**/details_harness|hendrycksTest-prehistory|5_2024-01-10T15-15-16.030532.parquet", "**/details_harness|hendrycksTest-professional_accounting|5_2024-01-10T15-15-16.030532.parquet", "**/details_harness|hendrycksTest-professional_law|5_2024-01-10T15-15-16.030532.parquet", "**/details_harness|hendrycksTest-professional_medicine|5_2024-01-10T15-15-16.030532.parquet", "**/details_harness|hendrycksTest-professional_psychology|5_2024-01-10T15-15-16.030532.parquet", "**/details_harness|hendrycksTest-public_relations|5_2024-01-10T15-15-16.030532.parquet", "**/details_harness|hendrycksTest-security_studies|5_2024-01-10T15-15-16.030532.parquet", "**/details_harness|hendrycksTest-sociology|5_2024-01-10T15-15-16.030532.parquet", "**/details_harness|hendrycksTest-us_foreign_policy|5_2024-01-10T15-15-16.030532.parquet", "**/details_harness|hendrycksTest-virology|5_2024-01-10T15-15-16.030532.parquet", "**/details_harness|hendrycksTest-world_religions|5_2024-01-10T15-15-16.030532.parquet"]}]}, {"config_name": "harness_hendrycksTest_abstract_algebra_5", "data_files": [{"split": "2024_01_10T15_15_16.030532", "path": ["**/details_harness|hendrycksTest-abstract_algebra|5_2024-01-10T15-15-16.030532.parquet"]}, {"split": "latest", "path": ["**/details_harness|hendrycksTest-abstract_algebra|5_2024-01-10T15-15-16.030532.parquet"]}]}, {"config_name": "harness_hendrycksTest_anatomy_5", "data_files": [{"split": "2024_01_10T15_15_16.030532", "path": ["**/details_harness|hendrycksTest-anatomy|5_2024-01-10T15-15-16.030532.parquet"]}, {"split": "latest", "path": ["**/details_harness|hendrycksTest-anatomy|5_2024-01-10T15-15-16.030532.parquet"]}]}, {"config_name": "harness_hendrycksTest_astronomy_5", "data_files": [{"split": "2024_01_10T15_15_16.030532", "path": ["**/details_harness|hendrycksTest-astronomy|5_2024-01-10T15-15-16.030532.parquet"]}, {"split": "latest", "path": ["**/details_harness|hendrycksTest-astronomy|5_2024-01-10T15-15-16.030532.parquet"]}]}, {"config_name": "harness_hendrycksTest_business_ethics_5", "data_files": [{"split": "2024_01_10T15_15_16.030532", "path": ["**/details_harness|hendrycksTest-business_ethics|5_2024-01-10T15-15-16.030532.parquet"]}, {"split": "latest", "path": ["**/details_harness|hendrycksTest-business_ethics|5_2024-01-10T15-15-16.030532.parquet"]}]}, {"config_name": "harness_hendrycksTest_clinical_knowledge_5", "data_files": [{"split": "2024_01_10T15_15_16.030532", "path": ["**/details_harness|hendrycksTest-clinical_knowledge|5_2024-01-10T15-15-16.030532.parquet"]}, {"split": "latest", "path": ["**/details_harness|hendrycksTest-clinical_knowledge|5_2024-01-10T15-15-16.030532.parquet"]}]}, {"config_name": "harness_hendrycksTest_college_biology_5", "data_files": [{"split": "2024_01_10T15_15_16.030532", "path": ["**/details_harness|hendrycksTest-college_biology|5_2024-01-10T15-15-16.030532.parquet"]}, {"split": "latest", "path": ["**/details_harness|hendrycksTest-college_biology|5_2024-01-10T15-15-16.030532.parquet"]}]}, {"config_name": "harness_hendrycksTest_college_chemistry_5", "data_files": [{"split": "2024_01_10T15_15_16.030532", "path": ["**/details_harness|hendrycksTest-college_chemistry|5_2024-01-10T15-15-16.030532.parquet"]}, {"split": "latest", "path": ["**/details_harness|hendrycksTest-college_chemistry|5_2024-01-10T15-15-16.030532.parquet"]}]}, {"config_name": "harness_hendrycksTest_college_computer_science_5", "data_files": [{"split": "2024_01_10T15_15_16.030532", "path": ["**/details_harness|hendrycksTest-college_computer_science|5_2024-01-10T15-15-16.030532.parquet"]}, {"split": "latest", "path": ["**/details_harness|hendrycksTest-college_computer_science|5_2024-01-10T15-15-16.030532.parquet"]}]}, {"config_name": "harness_hendrycksTest_college_mathematics_5", "data_files": [{"split": "2024_01_10T15_15_16.030532", "path": ["**/details_harness|hendrycksTest-college_mathematics|5_2024-01-10T15-15-16.030532.parquet"]}, {"split": "latest", "path": ["**/details_harness|hendrycksTest-college_mathematics|5_2024-01-10T15-15-16.030532.parquet"]}]}, {"config_name": "harness_hendrycksTest_college_medicine_5", "data_files": [{"split": "2024_01_10T15_15_16.030532", "path": ["**/details_harness|hendrycksTest-college_medicine|5_2024-01-10T15-15-16.030532.parquet"]}, {"split": "latest", "path": ["**/details_harness|hendrycksTest-college_medicine|5_2024-01-10T15-15-16.030532.parquet"]}]}, {"config_name": "harness_hendrycksTest_college_physics_5", "data_files": [{"split": "2024_01_10T15_15_16.030532", "path": ["**/details_harness|hendrycksTest-college_physics|5_2024-01-10T15-15-16.030532.parquet"]}, {"split": "latest", "path": ["**/details_harness|hendrycksTest-college_physics|5_2024-01-10T15-15-16.030532.parquet"]}]}, {"config_name": "harness_hendrycksTest_computer_security_5", "data_files": [{"split": "2024_01_10T15_15_16.030532", "path": ["**/details_harness|hendrycksTest-computer_security|5_2024-01-10T15-15-16.030532.parquet"]}, {"split": "latest", "path": ["**/details_harness|hendrycksTest-computer_security|5_2024-01-10T15-15-16.030532.parquet"]}]}, {"config_name": "harness_hendrycksTest_conceptual_physics_5", "data_files": [{"split": "2024_01_10T15_15_16.030532", "path": ["**/details_harness|hendrycksTest-conceptual_physics|5_2024-01-10T15-15-16.030532.parquet"]}, {"split": "latest", "path": ["**/details_harness|hendrycksTest-conceptual_physics|5_2024-01-10T15-15-16.030532.parquet"]}]}, {"config_name": "harness_hendrycksTest_econometrics_5", "data_files": [{"split": "2024_01_10T15_15_16.030532", "path": ["**/details_harness|hendrycksTest-econometrics|5_2024-01-10T15-15-16.030532.parquet"]}, {"split": "latest", "path": ["**/details_harness|hendrycksTest-econometrics|5_2024-01-10T15-15-16.030532.parquet"]}]}, {"config_name": "harness_hendrycksTest_electrical_engineering_5", "data_files": [{"split": "2024_01_10T15_15_16.030532", "path": ["**/details_harness|hendrycksTest-electrical_engineering|5_2024-01-10T15-15-16.030532.parquet"]}, {"split": "latest", "path": ["**/details_harness|hendrycksTest-electrical_engineering|5_2024-01-10T15-15-16.030532.parquet"]}]}, {"config_name": "harness_hendrycksTest_elementary_mathematics_5", "data_files": [{"split": "2024_01_10T15_15_16.030532", "path": ["**/details_harness|hendrycksTest-elementary_mathematics|5_2024-01-10T15-15-16.030532.parquet"]}, {"split": "latest", "path": ["**/details_harness|hendrycksTest-elementary_mathematics|5_2024-01-10T15-15-16.030532.parquet"]}]}, {"config_name": "harness_hendrycksTest_formal_logic_5", "data_files": [{"split": "2024_01_10T15_15_16.030532", "path": ["**/details_harness|hendrycksTest-formal_logic|5_2024-01-10T15-15-16.030532.parquet"]}, {"split": "latest", "path": ["**/details_harness|hendrycksTest-formal_logic|5_2024-01-10T15-15-16.030532.parquet"]}]}, {"config_name": "harness_hendrycksTest_global_facts_5", "data_files": [{"split": "2024_01_10T15_15_16.030532", "path": ["**/details_harness|hendrycksTest-global_facts|5_2024-01-10T15-15-16.030532.parquet"]}, {"split": "latest", "path": ["**/details_harness|hendrycksTest-global_facts|5_2024-01-10T15-15-16.030532.parquet"]}]}, {"config_name": "harness_hendrycksTest_high_school_biology_5", "data_files": [{"split": "2024_01_10T15_15_16.030532", "path": ["**/details_harness|hendrycksTest-high_school_biology|5_2024-01-10T15-15-16.030532.parquet"]}, {"split": "latest", "path": ["**/details_harness|hendrycksTest-high_school_biology|5_2024-01-10T15-15-16.030532.parquet"]}]}, {"config_name": "harness_hendrycksTest_high_school_chemistry_5", "data_files": [{"split": "2024_01_10T15_15_16.030532", "path": ["**/details_harness|hendrycksTest-high_school_chemistry|5_2024-01-10T15-15-16.030532.parquet"]}, {"split": "latest", "path": ["**/details_harness|hendrycksTest-high_school_chemistry|5_2024-01-10T15-15-16.030532.parquet"]}]}, {"config_name": "harness_hendrycksTest_high_school_computer_science_5", "data_files": [{"split": "2024_01_10T15_15_16.030532", "path": ["**/details_harness|hendrycksTest-high_school_computer_science|5_2024-01-10T15-15-16.030532.parquet"]}, {"split": "latest", "path": ["**/details_harness|hendrycksTest-high_school_computer_science|5_2024-01-10T15-15-16.030532.parquet"]}]}, {"config_name": "harness_hendrycksTest_high_school_european_history_5", "data_files": [{"split": "2024_01_10T15_15_16.030532", "path": ["**/details_harness|hendrycksTest-high_school_european_history|5_2024-01-10T15-15-16.030532.parquet"]}, {"split": "latest", "path": ["**/details_harness|hendrycksTest-high_school_european_history|5_2024-01-10T15-15-16.030532.parquet"]}]}, {"config_name": "harness_hendrycksTest_high_school_geography_5", "data_files": [{"split": "2024_01_10T15_15_16.030532", "path": ["**/details_harness|hendrycksTest-high_school_geography|5_2024-01-10T15-15-16.030532.parquet"]}, {"split": "latest", "path": ["**/details_harness|hendrycksTest-high_school_geography|5_2024-01-10T15-15-16.030532.parquet"]}]}, {"config_name": "harness_hendrycksTest_high_school_government_and_politics_5", "data_files": [{"split": "2024_01_10T15_15_16.030532", "path": ["**/details_harness|hendrycksTest-high_school_government_and_politics|5_2024-01-10T15-15-16.030532.parquet"]}, {"split": "latest", "path": ["**/details_harness|hendrycksTest-high_school_government_and_politics|5_2024-01-10T15-15-16.030532.parquet"]}]}, {"config_name": "harness_hendrycksTest_high_school_macroeconomics_5", "data_files": [{"split": "2024_01_10T15_15_16.030532", "path": ["**/details_harness|hendrycksTest-high_school_macroeconomics|5_2024-01-10T15-15-16.030532.parquet"]}, {"split": "latest", "path": ["**/details_harness|hendrycksTest-high_school_macroeconomics|5_2024-01-10T15-15-16.030532.parquet"]}]}, {"config_name": "harness_hendrycksTest_high_school_mathematics_5", "data_files": [{"split": "2024_01_10T15_15_16.030532", "path": ["**/details_harness|hendrycksTest-high_school_mathematics|5_2024-01-10T15-15-16.030532.parquet"]}, {"split": "latest", "path": ["**/details_harness|hendrycksTest-high_school_mathematics|5_2024-01-10T15-15-16.030532.parquet"]}]}, {"config_name": "harness_hendrycksTest_high_school_microeconomics_5", "data_files": [{"split": "2024_01_10T15_15_16.030532", "path": ["**/details_harness|hendrycksTest-high_school_microeconomics|5_2024-01-10T15-15-16.030532.parquet"]}, {"split": "latest", "path": ["**/details_harness|hendrycksTest-high_school_microeconomics|5_2024-01-10T15-15-16.030532.parquet"]}]}, {"config_name": "harness_hendrycksTest_high_school_physics_5", "data_files": [{"split": "2024_01_10T15_15_16.030532", "path": ["**/details_harness|hendrycksTest-high_school_physics|5_2024-01-10T15-15-16.030532.parquet"]}, {"split": "latest", "path": ["**/details_harness|hendrycksTest-high_school_physics|5_2024-01-10T15-15-16.030532.parquet"]}]}, {"config_name": "harness_hendrycksTest_high_school_psychology_5", "data_files": [{"split": "2024_01_10T15_15_16.030532", "path": ["**/details_harness|hendrycksTest-high_school_psychology|5_2024-01-10T15-15-16.030532.parquet"]}, {"split": "latest", "path": ["**/details_harness|hendrycksTest-high_school_psychology|5_2024-01-10T15-15-16.030532.parquet"]}]}, {"config_name": "harness_hendrycksTest_high_school_statistics_5", "data_files": [{"split": "2024_01_10T15_15_16.030532", "path": ["**/details_harness|hendrycksTest-high_school_statistics|5_2024-01-10T15-15-16.030532.parquet"]}, {"split": "latest", "path": ["**/details_harness|hendrycksTest-high_school_statistics|5_2024-01-10T15-15-16.030532.parquet"]}]}, {"config_name": "harness_hendrycksTest_high_school_us_history_5", "data_files": [{"split": "2024_01_10T15_15_16.030532", "path": ["**/details_harness|hendrycksTest-high_school_us_history|5_2024-01-10T15-15-16.030532.parquet"]}, {"split": "latest", "path": ["**/details_harness|hendrycksTest-high_school_us_history|5_2024-01-10T15-15-16.030532.parquet"]}]}, {"config_name": "harness_hendrycksTest_high_school_world_history_5", "data_files": [{"split": "2024_01_10T15_15_16.030532", "path": ["**/details_harness|hendrycksTest-high_school_world_history|5_2024-01-10T15-15-16.030532.parquet"]}, {"split": "latest", "path": ["**/details_harness|hendrycksTest-high_school_world_history|5_2024-01-10T15-15-16.030532.parquet"]}]}, {"config_name": "harness_hendrycksTest_human_aging_5", "data_files": [{"split": "2024_01_10T15_15_16.030532", "path": ["**/details_harness|hendrycksTest-human_aging|5_2024-01-10T15-15-16.030532.parquet"]}, {"split": "latest", "path": ["**/details_harness|hendrycksTest-human_aging|5_2024-01-10T15-15-16.030532.parquet"]}]}, {"config_name": "harness_hendrycksTest_human_sexuality_5", "data_files": [{"split": "2024_01_10T15_15_16.030532", "path": ["**/details_harness|hendrycksTest-human_sexuality|5_2024-01-10T15-15-16.030532.parquet"]}, {"split": "latest", "path": ["**/details_harness|hendrycksTest-human_sexuality|5_2024-01-10T15-15-16.030532.parquet"]}]}, {"config_name": "harness_hendrycksTest_international_law_5", "data_files": [{"split": "2024_01_10T15_15_16.030532", "path": ["**/details_harness|hendrycksTest-international_law|5_2024-01-10T15-15-16.030532.parquet"]}, {"split": "latest", "path": ["**/details_harness|hendrycksTest-international_law|5_2024-01-10T15-15-16.030532.parquet"]}]}, {"config_name": "harness_hendrycksTest_jurisprudence_5", "data_files": [{"split": "2024_01_10T15_15_16.030532", "path": ["**/details_harness|hendrycksTest-jurisprudence|5_2024-01-10T15-15-16.030532.parquet"]}, {"split": "latest", "path": ["**/details_harness|hendrycksTest-jurisprudence|5_2024-01-10T15-15-16.030532.parquet"]}]}, {"config_name": "harness_hendrycksTest_logical_fallacies_5", "data_files": [{"split": "2024_01_10T15_15_16.030532", "path": ["**/details_harness|hendrycksTest-logical_fallacies|5_2024-01-10T15-15-16.030532.parquet"]}, {"split": "latest", "path": ["**/details_harness|hendrycksTest-logical_fallacies|5_2024-01-10T15-15-16.030532.parquet"]}]}, {"config_name": "harness_hendrycksTest_machine_learning_5", "data_files": [{"split": "2024_01_10T15_15_16.030532", "path": ["**/details_harness|hendrycksTest-machine_learning|5_2024-01-10T15-15-16.030532.parquet"]}, {"split": "latest", "path": ["**/details_harness|hendrycksTest-machine_learning|5_2024-01-10T15-15-16.030532.parquet"]}]}, {"config_name": "harness_hendrycksTest_management_5", "data_files": [{"split": "2024_01_10T15_15_16.030532", "path": ["**/details_harness|hendrycksTest-management|5_2024-01-10T15-15-16.030532.parquet"]}, {"split": "latest", "path": ["**/details_harness|hendrycksTest-management|5_2024-01-10T15-15-16.030532.parquet"]}]}, {"config_name": "harness_hendrycksTest_marketing_5", "data_files": [{"split": "2024_01_10T15_15_16.030532", "path": ["**/details_harness|hendrycksTest-marketing|5_2024-01-10T15-15-16.030532.parquet"]}, {"split": "latest", "path": ["**/details_harness|hendrycksTest-marketing|5_2024-01-10T15-15-16.030532.parquet"]}]}, {"config_name": "harness_hendrycksTest_medical_genetics_5", "data_files": [{"split": "2024_01_10T15_15_16.030532", "path": ["**/details_harness|hendrycksTest-medical_genetics|5_2024-01-10T15-15-16.030532.parquet"]}, {"split": "latest", "path": ["**/details_harness|hendrycksTest-medical_genetics|5_2024-01-10T15-15-16.030532.parquet"]}]}, {"config_name": "harness_hendrycksTest_miscellaneous_5", "data_files": [{"split": "2024_01_10T15_15_16.030532", "path": ["**/details_harness|hendrycksTest-miscellaneous|5_2024-01-10T15-15-16.030532.parquet"]}, {"split": "latest", "path": ["**/details_harness|hendrycksTest-miscellaneous|5_2024-01-10T15-15-16.030532.parquet"]}]}, {"config_name": "harness_hendrycksTest_moral_disputes_5", "data_files": [{"split": "2024_01_10T15_15_16.030532", "path": ["**/details_harness|hendrycksTest-moral_disputes|5_2024-01-10T15-15-16.030532.parquet"]}, {"split": "latest", "path": ["**/details_harness|hendrycksTest-moral_disputes|5_2024-01-10T15-15-16.030532.parquet"]}]}, {"config_name": "harness_hendrycksTest_moral_scenarios_5", "data_files": [{"split": "2024_01_10T15_15_16.030532", "path": ["**/details_harness|hendrycksTest-moral_scenarios|5_2024-01-10T15-15-16.030532.parquet"]}, {"split": "latest", "path": ["**/details_harness|hendrycksTest-moral_scenarios|5_2024-01-10T15-15-16.030532.parquet"]}]}, {"config_name": "harness_hendrycksTest_nutrition_5", "data_files": [{"split": "2024_01_10T15_15_16.030532", "path": ["**/details_harness|hendrycksTest-nutrition|5_2024-01-10T15-15-16.030532.parquet"]}, {"split": "latest", "path": ["**/details_harness|hendrycksTest-nutrition|5_2024-01-10T15-15-16.030532.parquet"]}]}, {"config_name": "harness_hendrycksTest_philosophy_5", "data_files": [{"split": "2024_01_10T15_15_16.030532", "path": ["**/details_harness|hendrycksTest-philosophy|5_2024-01-10T15-15-16.030532.parquet"]}, {"split": "latest", "path": ["**/details_harness|hendrycksTest-philosophy|5_2024-01-10T15-15-16.030532.parquet"]}]}, {"config_name": "harness_hendrycksTest_prehistory_5", "data_files": [{"split": "2024_01_10T15_15_16.030532", "path": ["**/details_harness|hendrycksTest-prehistory|5_2024-01-10T15-15-16.030532.parquet"]}, {"split": "latest", "path": ["**/details_harness|hendrycksTest-prehistory|5_2024-01-10T15-15-16.030532.parquet"]}]}, {"config_name": "harness_hendrycksTest_professional_accounting_5", "data_files": [{"split": "2024_01_10T15_15_16.030532", "path": ["**/details_harness|hendrycksTest-professional_accounting|5_2024-01-10T15-15-16.030532.parquet"]}, {"split": "latest", "path": ["**/details_harness|hendrycksTest-professional_accounting|5_2024-01-10T15-15-16.030532.parquet"]}]}, {"config_name": "harness_hendrycksTest_professional_law_5", "data_files": [{"split": "2024_01_10T15_15_16.030532", "path": ["**/details_harness|hendrycksTest-professional_law|5_2024-01-10T15-15-16.030532.parquet"]}, {"split": "latest", "path": ["**/details_harness|hendrycksTest-professional_law|5_2024-01-10T15-15-16.030532.parquet"]}]}, {"config_name": "harness_hendrycksTest_professional_medicine_5", "data_files": [{"split": "2024_01_10T15_15_16.030532", "path": ["**/details_harness|hendrycksTest-professional_medicine|5_2024-01-10T15-15-16.030532.parquet"]}, {"split": "latest", "path": ["**/details_harness|hendrycksTest-professional_medicine|5_2024-01-10T15-15-16.030532.parquet"]}]}, {"config_name": "harness_hendrycksTest_professional_psychology_5", "data_files": [{"split": "2024_01_10T15_15_16.030532", "path": ["**/details_harness|hendrycksTest-professional_psychology|5_2024-01-10T15-15-16.030532.parquet"]}, {"split": "latest", "path": ["**/details_harness|hendrycksTest-professional_psychology|5_2024-01-10T15-15-16.030532.parquet"]}]}, {"config_name": "harness_hendrycksTest_public_relations_5", "data_files": [{"split": "2024_01_10T15_15_16.030532", "path": ["**/details_harness|hendrycksTest-public_relations|5_2024-01-10T15-15-16.030532.parquet"]}, {"split": "latest", "path": ["**/details_harness|hendrycksTest-public_relations|5_2024-01-10T15-15-16.030532.parquet"]}]}, {"config_name": "harness_hendrycksTest_security_studies_5", "data_files": [{"split": "2024_01_10T15_15_16.030532", "path": ["**/details_harness|hendrycksTest-security_studies|5_2024-01-10T15-15-16.030532.parquet"]}, {"split": "latest", "path": ["**/details_harness|hendrycksTest-security_studies|5_2024-01-10T15-15-16.030532.parquet"]}]}, {"config_name": "harness_hendrycksTest_sociology_5", "data_files": [{"split": "2024_01_10T15_15_16.030532", "path": ["**/details_harness|hendrycksTest-sociology|5_2024-01-10T15-15-16.030532.parquet"]}, {"split": "latest", "path": ["**/details_harness|hendrycksTest-sociology|5_2024-01-10T15-15-16.030532.parquet"]}]}, {"config_name": "harness_hendrycksTest_us_foreign_policy_5", "data_files": [{"split": "2024_01_10T15_15_16.030532", "path": ["**/details_harness|hendrycksTest-us_foreign_policy|5_2024-01-10T15-15-16.030532.parquet"]}, {"split": "latest", "path": ["**/details_harness|hendrycksTest-us_foreign_policy|5_2024-01-10T15-15-16.030532.parquet"]}]}, {"config_name": "harness_hendrycksTest_virology_5", "data_files": [{"split": "2024_01_10T15_15_16.030532", "path": ["**/details_harness|hendrycksTest-virology|5_2024-01-10T15-15-16.030532.parquet"]}, {"split": "latest", "path": ["**/details_harness|hendrycksTest-virology|5_2024-01-10T15-15-16.030532.parquet"]}]}, {"config_name": "harness_hendrycksTest_world_religions_5", "data_files": [{"split": "2024_01_10T15_15_16.030532", "path": ["**/details_harness|hendrycksTest-world_religions|5_2024-01-10T15-15-16.030532.parquet"]}, {"split": "latest", "path": ["**/details_harness|hendrycksTest-world_religions|5_2024-01-10T15-15-16.030532.parquet"]}]}, {"config_name": "harness_truthfulqa_mc_0", "data_files": [{"split": "2024_01_10T15_15_16.030532", "path": ["**/details_harness|truthfulqa:mc|0_2024-01-10T15-15-16.030532.parquet"]}, {"split": "latest", "path": ["**/details_harness|truthfulqa:mc|0_2024-01-10T15-15-16.030532.parquet"]}]}, {"config_name": "harness_winogrande_5", "data_files": [{"split": "2024_01_10T15_15_16.030532", "path": ["**/details_harness|winogrande|5_2024-01-10T15-15-16.030532.parquet"]}, {"split": "latest", "path": ["**/details_harness|winogrande|5_2024-01-10T15-15-16.030532.parquet"]}]}, {"config_name": "results", "data_files": [{"split": "2024_01_10T15_15_16.030532", "path": ["results_2024-01-10T15-15-16.030532.parquet"]}, {"split": "latest", "path": ["results_2024-01-10T15-15-16.030532.parquet"]}]}]} | 2024-01-10T15:18:04+00:00 | [] | [] | TAGS
#region-us
|
# Dataset Card for Evaluation run of ewqr2130/llama2-7b-raw-sft
Dataset automatically created during the evaluation run of model ewqr2130/llama2-7b-raw-sft on the Open LLM Leaderboard.
The dataset is composed of 63 configuration, each one coresponding to one of the evaluated task.
The dataset has been created from 1 run(s). Each run can be found as a specific split in each configuration, the split being named using the timestamp of the run.The "train" split is always pointing to the latest results.
An additional configuration "results" store all the aggregated results of the run (and is used to compute and display the aggregated metrics on the Open LLM Leaderboard).
To load the details from a run, you can for instance do the following:
## Latest results
These are the latest results from run 2024-01-10T15:15:16.030532(note that their might be results for other tasks in the repos if successive evals didn't cover the same tasks. You find each in the results and the "latest" split for each eval):
## Dataset Details
### Dataset Description
- Curated by:
- Funded by [optional]:
- Shared by [optional]:
- Language(s) (NLP):
- License:
### Dataset Sources [optional]
- Repository:
- Paper [optional]:
- Demo [optional]:
## Uses
### Direct Use
### Out-of-Scope Use
## Dataset Structure
## Dataset Creation
### Curation Rationale
### Source Data
#### Data Collection and Processing
#### Who are the source data producers?
### Annotations [optional]
#### Annotation process
#### Who are the annotators?
#### Personal and Sensitive Information
## Bias, Risks, and Limitations
### Recommendations
Users should be made aware of the risks, biases and limitations of the dataset. More information needed for further recommendations.
[optional]
BibTeX:
APA:
## Glossary [optional]
## More Information [optional]
## Dataset Card Authors [optional]
## Dataset Card Contact
| [
"# Dataset Card for Evaluation run of ewqr2130/llama2-7b-raw-sft\n\n\n\nDataset automatically created during the evaluation run of model ewqr2130/llama2-7b-raw-sft on the Open LLM Leaderboard.\n\nThe dataset is composed of 63 configuration, each one coresponding to one of the evaluated task.\n\nThe dataset has been created from 1 run(s). Each run can be found as a specific split in each configuration, the split being named using the timestamp of the run.The \"train\" split is always pointing to the latest results.\n\nAn additional configuration \"results\" store all the aggregated results of the run (and is used to compute and display the aggregated metrics on the Open LLM Leaderboard).\n\nTo load the details from a run, you can for instance do the following:",
"## Latest results\n\nThese are the latest results from run 2024-01-10T15:15:16.030532(note that their might be results for other tasks in the repos if successive evals didn't cover the same tasks. You find each in the results and the \"latest\" split for each eval):",
"## Dataset Details",
"### Dataset Description\n\n\n\n\n\n- Curated by: \n- Funded by [optional]: \n- Shared by [optional]: \n- Language(s) (NLP): \n- License:",
"### Dataset Sources [optional]\n\n\n\n- Repository: \n- Paper [optional]: \n- Demo [optional]:",
"## Uses",
"### Direct Use",
"### Out-of-Scope Use",
"## Dataset Structure",
"## Dataset Creation",
"### Curation Rationale",
"### Source Data",
"#### Data Collection and Processing",
"#### Who are the source data producers?",
"### Annotations [optional]",
"#### Annotation process",
"#### Who are the annotators?",
"#### Personal and Sensitive Information",
"## Bias, Risks, and Limitations",
"### Recommendations\n\n\n\nUsers should be made aware of the risks, biases and limitations of the dataset. More information needed for further recommendations.\n\n[optional]\n\n\n\nBibTeX:\n\n\n\nAPA:",
"## Glossary [optional]",
"## More Information [optional]",
"## Dataset Card Authors [optional]",
"## Dataset Card Contact"
] | [
"TAGS\n#region-us \n",
"# Dataset Card for Evaluation run of ewqr2130/llama2-7b-raw-sft\n\n\n\nDataset automatically created during the evaluation run of model ewqr2130/llama2-7b-raw-sft on the Open LLM Leaderboard.\n\nThe dataset is composed of 63 configuration, each one coresponding to one of the evaluated task.\n\nThe dataset has been created from 1 run(s). Each run can be found as a specific split in each configuration, the split being named using the timestamp of the run.The \"train\" split is always pointing to the latest results.\n\nAn additional configuration \"results\" store all the aggregated results of the run (and is used to compute and display the aggregated metrics on the Open LLM Leaderboard).\n\nTo load the details from a run, you can for instance do the following:",
"## Latest results\n\nThese are the latest results from run 2024-01-10T15:15:16.030532(note that their might be results for other tasks in the repos if successive evals didn't cover the same tasks. You find each in the results and the \"latest\" split for each eval):",
"## Dataset Details",
"### Dataset Description\n\n\n\n\n\n- Curated by: \n- Funded by [optional]: \n- Shared by [optional]: \n- Language(s) (NLP): \n- License:",
"### Dataset Sources [optional]\n\n\n\n- Repository: \n- Paper [optional]: \n- Demo [optional]:",
"## Uses",
"### Direct Use",
"### Out-of-Scope Use",
"## Dataset Structure",
"## Dataset Creation",
"### Curation Rationale",
"### Source Data",
"#### Data Collection and Processing",
"#### Who are the source data producers?",
"### Annotations [optional]",
"#### Annotation process",
"#### Who are the annotators?",
"#### Personal and Sensitive Information",
"## Bias, Risks, and Limitations",
"### Recommendations\n\n\n\nUsers should be made aware of the risks, biases and limitations of the dataset. More information needed for further recommendations.\n\n[optional]\n\n\n\nBibTeX:\n\n\n\nAPA:",
"## Glossary [optional]",
"## More Information [optional]",
"## Dataset Card Authors [optional]",
"## Dataset Card Contact"
] |
571a60c0f45e92b565f4eb2a1da8f7fa5a475c8f |
# Dataset Card for Evaluation run of euclaise/crow-1b
<!-- Provide a quick summary of the dataset. -->
Dataset automatically created during the evaluation run of model [euclaise/crow-1b](https://huggingface.co/euclaise/crow-1b) on the [Open LLM Leaderboard](https://huggingface.co/spaces/HuggingFaceH4/open_llm_leaderboard).
The dataset is composed of 63 configuration, each one coresponding to one of the evaluated task.
The dataset has been created from 1 run(s). Each run can be found as a specific split in each configuration, the split being named using the timestamp of the run.The "train" split is always pointing to the latest results.
An additional configuration "results" store all the aggregated results of the run (and is used to compute and display the aggregated metrics on the [Open LLM Leaderboard](https://huggingface.co/spaces/HuggingFaceH4/open_llm_leaderboard)).
To load the details from a run, you can for instance do the following:
```python
from datasets import load_dataset
data = load_dataset("open-llm-leaderboard/details_euclaise__crow-1b",
"harness_winogrande_5",
split="train")
```
## Latest results
These are the [latest results from run 2024-01-10T15:21:15.168348](https://huggingface.co/datasets/open-llm-leaderboard/details_euclaise__crow-1b/blob/main/results_2024-01-10T15-21-15.168348.json)(note that their might be results for other tasks in the repos if successive evals didn't cover the same tasks. You find each in the results and the "latest" split for each eval):
```python
{
"all": {
"acc": 0.2479877476070674,
"acc_stderr": 0.030650601843589306,
"acc_norm": 0.2482802993881133,
"acc_norm_stderr": 0.031416441202837334,
"mc1": 0.2350061199510404,
"mc1_stderr": 0.014843061507731601,
"mc2": 0.4827953936445661,
"mc2_stderr": 0.01642500560197448
},
"harness|arc:challenge|25": {
"acc": 0.2295221843003413,
"acc_stderr": 0.012288926760890788,
"acc_norm": 0.2551194539249147,
"acc_norm_stderr": 0.012739038695202105
},
"harness|hellaswag|10": {
"acc": 0.2606054570802629,
"acc_stderr": 0.0043806785853414175,
"acc_norm": 0.25871340370444135,
"acc_norm_stderr": 0.004370328224831786
},
"harness|hendrycksTest-abstract_algebra|5": {
"acc": 0.22,
"acc_stderr": 0.04163331998932268,
"acc_norm": 0.22,
"acc_norm_stderr": 0.04163331998932268
},
"harness|hendrycksTest-anatomy|5": {
"acc": 0.18518518518518517,
"acc_stderr": 0.03355677216313142,
"acc_norm": 0.18518518518518517,
"acc_norm_stderr": 0.03355677216313142
},
"harness|hendrycksTest-astronomy|5": {
"acc": 0.18421052631578946,
"acc_stderr": 0.0315469804508223,
"acc_norm": 0.18421052631578946,
"acc_norm_stderr": 0.0315469804508223
},
"harness|hendrycksTest-business_ethics|5": {
"acc": 0.32,
"acc_stderr": 0.046882617226215034,
"acc_norm": 0.32,
"acc_norm_stderr": 0.046882617226215034
},
"harness|hendrycksTest-clinical_knowledge|5": {
"acc": 0.2,
"acc_stderr": 0.02461829819586651,
"acc_norm": 0.2,
"acc_norm_stderr": 0.02461829819586651
},
"harness|hendrycksTest-college_biology|5": {
"acc": 0.22916666666666666,
"acc_stderr": 0.035146974678623884,
"acc_norm": 0.22916666666666666,
"acc_norm_stderr": 0.035146974678623884
},
"harness|hendrycksTest-college_chemistry|5": {
"acc": 0.3,
"acc_stderr": 0.046056618647183814,
"acc_norm": 0.3,
"acc_norm_stderr": 0.046056618647183814
},
"harness|hendrycksTest-college_computer_science|5": {
"acc": 0.25,
"acc_stderr": 0.04351941398892446,
"acc_norm": 0.25,
"acc_norm_stderr": 0.04351941398892446
},
"harness|hendrycksTest-college_mathematics|5": {
"acc": 0.21,
"acc_stderr": 0.040936018074033256,
"acc_norm": 0.21,
"acc_norm_stderr": 0.040936018074033256
},
"harness|hendrycksTest-college_medicine|5": {
"acc": 0.3179190751445087,
"acc_stderr": 0.03550683989165581,
"acc_norm": 0.3179190751445087,
"acc_norm_stderr": 0.03550683989165581
},
"harness|hendrycksTest-college_physics|5": {
"acc": 0.3627450980392157,
"acc_stderr": 0.04784060704105655,
"acc_norm": 0.3627450980392157,
"acc_norm_stderr": 0.04784060704105655
},
"harness|hendrycksTest-computer_security|5": {
"acc": 0.25,
"acc_stderr": 0.04351941398892446,
"acc_norm": 0.25,
"acc_norm_stderr": 0.04351941398892446
},
"harness|hendrycksTest-conceptual_physics|5": {
"acc": 0.25957446808510637,
"acc_stderr": 0.028659179374292316,
"acc_norm": 0.25957446808510637,
"acc_norm_stderr": 0.028659179374292316
},
"harness|hendrycksTest-econometrics|5": {
"acc": 0.23684210526315788,
"acc_stderr": 0.039994238792813365,
"acc_norm": 0.23684210526315788,
"acc_norm_stderr": 0.039994238792813365
},
"harness|hendrycksTest-electrical_engineering|5": {
"acc": 0.22758620689655173,
"acc_stderr": 0.03493950380131184,
"acc_norm": 0.22758620689655173,
"acc_norm_stderr": 0.03493950380131184
},
"harness|hendrycksTest-elementary_mathematics|5": {
"acc": 0.2671957671957672,
"acc_stderr": 0.022789673145776564,
"acc_norm": 0.2671957671957672,
"acc_norm_stderr": 0.022789673145776564
},
"harness|hendrycksTest-formal_logic|5": {
"acc": 0.2777777777777778,
"acc_stderr": 0.04006168083848875,
"acc_norm": 0.2777777777777778,
"acc_norm_stderr": 0.04006168083848875
},
"harness|hendrycksTest-global_facts|5": {
"acc": 0.19,
"acc_stderr": 0.03942772444036624,
"acc_norm": 0.19,
"acc_norm_stderr": 0.03942772444036624
},
"harness|hendrycksTest-high_school_biology|5": {
"acc": 0.2870967741935484,
"acc_stderr": 0.025736542745594525,
"acc_norm": 0.2870967741935484,
"acc_norm_stderr": 0.025736542745594525
},
"harness|hendrycksTest-high_school_chemistry|5": {
"acc": 0.2413793103448276,
"acc_stderr": 0.03010833071801162,
"acc_norm": 0.2413793103448276,
"acc_norm_stderr": 0.03010833071801162
},
"harness|hendrycksTest-high_school_computer_science|5": {
"acc": 0.32,
"acc_stderr": 0.046882617226215034,
"acc_norm": 0.32,
"acc_norm_stderr": 0.046882617226215034
},
"harness|hendrycksTest-high_school_european_history|5": {
"acc": 0.22424242424242424,
"acc_stderr": 0.032568666616811015,
"acc_norm": 0.22424242424242424,
"acc_norm_stderr": 0.032568666616811015
},
"harness|hendrycksTest-high_school_geography|5": {
"acc": 0.18181818181818182,
"acc_stderr": 0.027479603010538787,
"acc_norm": 0.18181818181818182,
"acc_norm_stderr": 0.027479603010538787
},
"harness|hendrycksTest-high_school_government_and_politics|5": {
"acc": 0.35751295336787564,
"acc_stderr": 0.034588160421810045,
"acc_norm": 0.35751295336787564,
"acc_norm_stderr": 0.034588160421810045
},
"harness|hendrycksTest-high_school_macroeconomics|5": {
"acc": 0.19487179487179487,
"acc_stderr": 0.02008316759518139,
"acc_norm": 0.19487179487179487,
"acc_norm_stderr": 0.02008316759518139
},
"harness|hendrycksTest-high_school_mathematics|5": {
"acc": 0.26296296296296295,
"acc_stderr": 0.02684205787383371,
"acc_norm": 0.26296296296296295,
"acc_norm_stderr": 0.02684205787383371
},
"harness|hendrycksTest-high_school_microeconomics|5": {
"acc": 0.22268907563025211,
"acc_stderr": 0.02702543349888238,
"acc_norm": 0.22268907563025211,
"acc_norm_stderr": 0.02702543349888238
},
"harness|hendrycksTest-high_school_physics|5": {
"acc": 0.2185430463576159,
"acc_stderr": 0.03374235550425694,
"acc_norm": 0.2185430463576159,
"acc_norm_stderr": 0.03374235550425694
},
"harness|hendrycksTest-high_school_psychology|5": {
"acc": 0.3284403669724771,
"acc_stderr": 0.02013590279729839,
"acc_norm": 0.3284403669724771,
"acc_norm_stderr": 0.02013590279729839
},
"harness|hendrycksTest-high_school_statistics|5": {
"acc": 0.14814814814814814,
"acc_stderr": 0.024227629273728356,
"acc_norm": 0.14814814814814814,
"acc_norm_stderr": 0.024227629273728356
},
"harness|hendrycksTest-high_school_us_history|5": {
"acc": 0.24509803921568626,
"acc_stderr": 0.030190282453501954,
"acc_norm": 0.24509803921568626,
"acc_norm_stderr": 0.030190282453501954
},
"harness|hendrycksTest-high_school_world_history|5": {
"acc": 0.270042194092827,
"acc_stderr": 0.028900721906293426,
"acc_norm": 0.270042194092827,
"acc_norm_stderr": 0.028900721906293426
},
"harness|hendrycksTest-human_aging|5": {
"acc": 0.2645739910313901,
"acc_stderr": 0.029605103217038315,
"acc_norm": 0.2645739910313901,
"acc_norm_stderr": 0.029605103217038315
},
"harness|hendrycksTest-human_sexuality|5": {
"acc": 0.2595419847328244,
"acc_stderr": 0.03844876139785271,
"acc_norm": 0.2595419847328244,
"acc_norm_stderr": 0.03844876139785271
},
"harness|hendrycksTest-international_law|5": {
"acc": 0.2396694214876033,
"acc_stderr": 0.03896878985070417,
"acc_norm": 0.2396694214876033,
"acc_norm_stderr": 0.03896878985070417
},
"harness|hendrycksTest-jurisprudence|5": {
"acc": 0.25,
"acc_stderr": 0.04186091791394607,
"acc_norm": 0.25,
"acc_norm_stderr": 0.04186091791394607
},
"harness|hendrycksTest-logical_fallacies|5": {
"acc": 0.2147239263803681,
"acc_stderr": 0.03226219377286774,
"acc_norm": 0.2147239263803681,
"acc_norm_stderr": 0.03226219377286774
},
"harness|hendrycksTest-machine_learning|5": {
"acc": 0.3125,
"acc_stderr": 0.043994650575715215,
"acc_norm": 0.3125,
"acc_norm_stderr": 0.043994650575715215
},
"harness|hendrycksTest-management|5": {
"acc": 0.3883495145631068,
"acc_stderr": 0.048257293373563895,
"acc_norm": 0.3883495145631068,
"acc_norm_stderr": 0.048257293373563895
},
"harness|hendrycksTest-marketing|5": {
"acc": 0.28205128205128205,
"acc_stderr": 0.029480360549541194,
"acc_norm": 0.28205128205128205,
"acc_norm_stderr": 0.029480360549541194
},
"harness|hendrycksTest-medical_genetics|5": {
"acc": 0.3,
"acc_stderr": 0.046056618647183814,
"acc_norm": 0.3,
"acc_norm_stderr": 0.046056618647183814
},
"harness|hendrycksTest-miscellaneous|5": {
"acc": 0.20561941251596424,
"acc_stderr": 0.014452500456785823,
"acc_norm": 0.20561941251596424,
"acc_norm_stderr": 0.014452500456785823
},
"harness|hendrycksTest-moral_disputes|5": {
"acc": 0.21098265895953758,
"acc_stderr": 0.021966309947043128,
"acc_norm": 0.21098265895953758,
"acc_norm_stderr": 0.021966309947043128
},
"harness|hendrycksTest-moral_scenarios|5": {
"acc": 0.23798882681564246,
"acc_stderr": 0.014242630070574915,
"acc_norm": 0.23798882681564246,
"acc_norm_stderr": 0.014242630070574915
},
"harness|hendrycksTest-nutrition|5": {
"acc": 0.2549019607843137,
"acc_stderr": 0.024954184324879905,
"acc_norm": 0.2549019607843137,
"acc_norm_stderr": 0.024954184324879905
},
"harness|hendrycksTest-philosophy|5": {
"acc": 0.18006430868167203,
"acc_stderr": 0.021823422857744953,
"acc_norm": 0.18006430868167203,
"acc_norm_stderr": 0.021823422857744953
},
"harness|hendrycksTest-prehistory|5": {
"acc": 0.21296296296296297,
"acc_stderr": 0.0227797190887334,
"acc_norm": 0.21296296296296297,
"acc_norm_stderr": 0.0227797190887334
},
"harness|hendrycksTest-professional_accounting|5": {
"acc": 0.24113475177304963,
"acc_stderr": 0.025518731049537755,
"acc_norm": 0.24113475177304963,
"acc_norm_stderr": 0.025518731049537755
},
"harness|hendrycksTest-professional_law|5": {
"acc": 0.24902216427640156,
"acc_stderr": 0.011044892264040769,
"acc_norm": 0.24902216427640156,
"acc_norm_stderr": 0.011044892264040769
},
"harness|hendrycksTest-professional_medicine|5": {
"acc": 0.16544117647058823,
"acc_stderr": 0.022571771025494767,
"acc_norm": 0.16544117647058823,
"acc_norm_stderr": 0.022571771025494767
},
"harness|hendrycksTest-professional_psychology|5": {
"acc": 0.25163398692810457,
"acc_stderr": 0.01755581809132226,
"acc_norm": 0.25163398692810457,
"acc_norm_stderr": 0.01755581809132226
},
"harness|hendrycksTest-public_relations|5": {
"acc": 0.19090909090909092,
"acc_stderr": 0.03764425585984927,
"acc_norm": 0.19090909090909092,
"acc_norm_stderr": 0.03764425585984927
},
"harness|hendrycksTest-security_studies|5": {
"acc": 0.24081632653061225,
"acc_stderr": 0.027372942201788163,
"acc_norm": 0.24081632653061225,
"acc_norm_stderr": 0.027372942201788163
},
"harness|hendrycksTest-sociology|5": {
"acc": 0.23880597014925373,
"acc_stderr": 0.030147775935409214,
"acc_norm": 0.23880597014925373,
"acc_norm_stderr": 0.030147775935409214
},
"harness|hendrycksTest-us_foreign_policy|5": {
"acc": 0.27,
"acc_stderr": 0.0446196043338474,
"acc_norm": 0.27,
"acc_norm_stderr": 0.0446196043338474
},
"harness|hendrycksTest-virology|5": {
"acc": 0.28313253012048195,
"acc_stderr": 0.03507295431370518,
"acc_norm": 0.28313253012048195,
"acc_norm_stderr": 0.03507295431370518
},
"harness|hendrycksTest-world_religions|5": {
"acc": 0.19883040935672514,
"acc_stderr": 0.03061111655743253,
"acc_norm": 0.19883040935672514,
"acc_norm_stderr": 0.03061111655743253
},
"harness|truthfulqa:mc|0": {
"mc1": 0.2350061199510404,
"mc1_stderr": 0.014843061507731601,
"mc2": 0.4827953936445661,
"mc2_stderr": 0.01642500560197448
},
"harness|winogrande|5": {
"acc": 0.4940805051302289,
"acc_stderr": 0.014051500838485807
},
"harness|gsm8k|5": {
"acc": 0.008339651250947688,
"acc_stderr": 0.0025049422268605135
}
}
```
## Dataset Details
### Dataset Description
<!-- Provide a longer summary of what this dataset is. -->
- **Curated by:** [More Information Needed]
- **Funded by [optional]:** [More Information Needed]
- **Shared by [optional]:** [More Information Needed]
- **Language(s) (NLP):** [More Information Needed]
- **License:** [More Information Needed]
### Dataset Sources [optional]
<!-- Provide the basic links for the dataset. -->
- **Repository:** [More Information Needed]
- **Paper [optional]:** [More Information Needed]
- **Demo [optional]:** [More Information Needed]
## Uses
<!-- Address questions around how the dataset is intended to be used. -->
### Direct Use
<!-- This section describes suitable use cases for the dataset. -->
[More Information Needed]
### Out-of-Scope Use
<!-- This section addresses misuse, malicious use, and uses that the dataset will not work well for. -->
[More Information Needed]
## Dataset Structure
<!-- This section provides a description of the dataset fields, and additional information about the dataset structure such as criteria used to create the splits, relationships between data points, etc. -->
[More Information Needed]
## Dataset Creation
### Curation Rationale
<!-- Motivation for the creation of this dataset. -->
[More Information Needed]
### Source Data
<!-- This section describes the source data (e.g. news text and headlines, social media posts, translated sentences, ...). -->
#### Data Collection and Processing
<!-- This section describes the data collection and processing process such as data selection criteria, filtering and normalization methods, tools and libraries used, etc. -->
[More Information Needed]
#### Who are the source data producers?
<!-- This section describes the people or systems who originally created the data. It should also include self-reported demographic or identity information for the source data creators if this information is available. -->
[More Information Needed]
### Annotations [optional]
<!-- If the dataset contains annotations which are not part of the initial data collection, use this section to describe them. -->
#### Annotation process
<!-- This section describes the annotation process such as annotation tools used in the process, the amount of data annotated, annotation guidelines provided to the annotators, interannotator statistics, annotation validation, etc. -->
[More Information Needed]
#### Who are the annotators?
<!-- This section describes the people or systems who created the annotations. -->
[More Information Needed]
#### Personal and Sensitive Information
<!-- State whether the dataset contains data that might be considered personal, sensitive, or private (e.g., data that reveals addresses, uniquely identifiable names or aliases, racial or ethnic origins, sexual orientations, religious beliefs, political opinions, financial or health data, etc.). If efforts were made to anonymize the data, describe the anonymization process. -->
[More Information Needed]
## Bias, Risks, and Limitations
<!-- This section is meant to convey both technical and sociotechnical limitations. -->
[More Information Needed]
### Recommendations
<!-- This section is meant to convey recommendations with respect to the bias, risk, and technical limitations. -->
Users should be made aware of the risks, biases and limitations of the dataset. More information needed for further recommendations.
## Citation [optional]
<!-- If there is a paper or blog post introducing the dataset, the APA and Bibtex information for that should go in this section. -->
**BibTeX:**
[More Information Needed]
**APA:**
[More Information Needed]
## Glossary [optional]
<!-- If relevant, include terms and calculations in this section that can help readers understand the dataset or dataset card. -->
[More Information Needed]
## More Information [optional]
[More Information Needed]
## Dataset Card Authors [optional]
[More Information Needed]
## Dataset Card Contact
[More Information Needed] | open-llm-leaderboard/details_euclaise__crow-1b | [
"region:us"
] | 2024-01-10T15:23:03+00:00 | {"pretty_name": "Evaluation run of euclaise/crow-1b", "dataset_summary": "Dataset automatically created during the evaluation run of model [euclaise/crow-1b](https://huggingface.co/euclaise/crow-1b) on the [Open LLM Leaderboard](https://huggingface.co/spaces/HuggingFaceH4/open_llm_leaderboard).\n\nThe dataset is composed of 63 configuration, each one coresponding to one of the evaluated task.\n\nThe dataset has been created from 1 run(s). Each run can be found as a specific split in each configuration, the split being named using the timestamp of the run.The \"train\" split is always pointing to the latest results.\n\nAn additional configuration \"results\" store all the aggregated results of the run (and is used to compute and display the aggregated metrics on the [Open LLM Leaderboard](https://huggingface.co/spaces/HuggingFaceH4/open_llm_leaderboard)).\n\nTo load the details from a run, you can for instance do the following:\n```python\nfrom datasets import load_dataset\ndata = load_dataset(\"open-llm-leaderboard/details_euclaise__crow-1b\",\n\t\"harness_winogrande_5\",\n\tsplit=\"train\")\n```\n\n## Latest results\n\nThese are the [latest results from run 2024-01-10T15:21:15.168348](https://huggingface.co/datasets/open-llm-leaderboard/details_euclaise__crow-1b/blob/main/results_2024-01-10T15-21-15.168348.json)(note that their might be results for other tasks in the repos if successive evals didn't cover the same tasks. You find each in the results and the \"latest\" split for each eval):\n\n```python\n{\n \"all\": {\n \"acc\": 0.2479877476070674,\n \"acc_stderr\": 0.030650601843589306,\n \"acc_norm\": 0.2482802993881133,\n \"acc_norm_stderr\": 0.031416441202837334,\n \"mc1\": 0.2350061199510404,\n \"mc1_stderr\": 0.014843061507731601,\n \"mc2\": 0.4827953936445661,\n \"mc2_stderr\": 0.01642500560197448\n },\n \"harness|arc:challenge|25\": {\n \"acc\": 0.2295221843003413,\n \"acc_stderr\": 0.012288926760890788,\n \"acc_norm\": 0.2551194539249147,\n \"acc_norm_stderr\": 0.012739038695202105\n },\n \"harness|hellaswag|10\": {\n \"acc\": 0.2606054570802629,\n \"acc_stderr\": 0.0043806785853414175,\n \"acc_norm\": 0.25871340370444135,\n \"acc_norm_stderr\": 0.004370328224831786\n },\n \"harness|hendrycksTest-abstract_algebra|5\": {\n \"acc\": 0.22,\n \"acc_stderr\": 0.04163331998932268,\n \"acc_norm\": 0.22,\n \"acc_norm_stderr\": 0.04163331998932268\n },\n \"harness|hendrycksTest-anatomy|5\": {\n \"acc\": 0.18518518518518517,\n \"acc_stderr\": 0.03355677216313142,\n \"acc_norm\": 0.18518518518518517,\n \"acc_norm_stderr\": 0.03355677216313142\n },\n \"harness|hendrycksTest-astronomy|5\": {\n \"acc\": 0.18421052631578946,\n \"acc_stderr\": 0.0315469804508223,\n \"acc_norm\": 0.18421052631578946,\n \"acc_norm_stderr\": 0.0315469804508223\n },\n \"harness|hendrycksTest-business_ethics|5\": {\n \"acc\": 0.32,\n \"acc_stderr\": 0.046882617226215034,\n \"acc_norm\": 0.32,\n \"acc_norm_stderr\": 0.046882617226215034\n },\n \"harness|hendrycksTest-clinical_knowledge|5\": {\n \"acc\": 0.2,\n \"acc_stderr\": 0.02461829819586651,\n \"acc_norm\": 0.2,\n \"acc_norm_stderr\": 0.02461829819586651\n },\n \"harness|hendrycksTest-college_biology|5\": {\n \"acc\": 0.22916666666666666,\n \"acc_stderr\": 0.035146974678623884,\n \"acc_norm\": 0.22916666666666666,\n \"acc_norm_stderr\": 0.035146974678623884\n },\n \"harness|hendrycksTest-college_chemistry|5\": {\n \"acc\": 0.3,\n \"acc_stderr\": 0.046056618647183814,\n \"acc_norm\": 0.3,\n \"acc_norm_stderr\": 0.046056618647183814\n },\n \"harness|hendrycksTest-college_computer_science|5\": {\n \"acc\": 0.25,\n \"acc_stderr\": 0.04351941398892446,\n \"acc_norm\": 0.25,\n \"acc_norm_stderr\": 0.04351941398892446\n },\n \"harness|hendrycksTest-college_mathematics|5\": {\n \"acc\": 0.21,\n \"acc_stderr\": 0.040936018074033256,\n \"acc_norm\": 0.21,\n \"acc_norm_stderr\": 0.040936018074033256\n },\n \"harness|hendrycksTest-college_medicine|5\": {\n \"acc\": 0.3179190751445087,\n \"acc_stderr\": 0.03550683989165581,\n \"acc_norm\": 0.3179190751445087,\n \"acc_norm_stderr\": 0.03550683989165581\n },\n \"harness|hendrycksTest-college_physics|5\": {\n \"acc\": 0.3627450980392157,\n \"acc_stderr\": 0.04784060704105655,\n \"acc_norm\": 0.3627450980392157,\n \"acc_norm_stderr\": 0.04784060704105655\n },\n \"harness|hendrycksTest-computer_security|5\": {\n \"acc\": 0.25,\n \"acc_stderr\": 0.04351941398892446,\n \"acc_norm\": 0.25,\n \"acc_norm_stderr\": 0.04351941398892446\n },\n \"harness|hendrycksTest-conceptual_physics|5\": {\n \"acc\": 0.25957446808510637,\n \"acc_stderr\": 0.028659179374292316,\n \"acc_norm\": 0.25957446808510637,\n \"acc_norm_stderr\": 0.028659179374292316\n },\n \"harness|hendrycksTest-econometrics|5\": {\n \"acc\": 0.23684210526315788,\n \"acc_stderr\": 0.039994238792813365,\n \"acc_norm\": 0.23684210526315788,\n \"acc_norm_stderr\": 0.039994238792813365\n },\n \"harness|hendrycksTest-electrical_engineering|5\": {\n \"acc\": 0.22758620689655173,\n \"acc_stderr\": 0.03493950380131184,\n \"acc_norm\": 0.22758620689655173,\n \"acc_norm_stderr\": 0.03493950380131184\n },\n \"harness|hendrycksTest-elementary_mathematics|5\": {\n \"acc\": 0.2671957671957672,\n \"acc_stderr\": 0.022789673145776564,\n \"acc_norm\": 0.2671957671957672,\n \"acc_norm_stderr\": 0.022789673145776564\n },\n \"harness|hendrycksTest-formal_logic|5\": {\n \"acc\": 0.2777777777777778,\n \"acc_stderr\": 0.04006168083848875,\n \"acc_norm\": 0.2777777777777778,\n \"acc_norm_stderr\": 0.04006168083848875\n },\n \"harness|hendrycksTest-global_facts|5\": {\n \"acc\": 0.19,\n \"acc_stderr\": 0.03942772444036624,\n \"acc_norm\": 0.19,\n \"acc_norm_stderr\": 0.03942772444036624\n },\n \"harness|hendrycksTest-high_school_biology|5\": {\n \"acc\": 0.2870967741935484,\n \"acc_stderr\": 0.025736542745594525,\n \"acc_norm\": 0.2870967741935484,\n \"acc_norm_stderr\": 0.025736542745594525\n },\n \"harness|hendrycksTest-high_school_chemistry|5\": {\n \"acc\": 0.2413793103448276,\n \"acc_stderr\": 0.03010833071801162,\n \"acc_norm\": 0.2413793103448276,\n \"acc_norm_stderr\": 0.03010833071801162\n },\n \"harness|hendrycksTest-high_school_computer_science|5\": {\n \"acc\": 0.32,\n \"acc_stderr\": 0.046882617226215034,\n \"acc_norm\": 0.32,\n \"acc_norm_stderr\": 0.046882617226215034\n },\n \"harness|hendrycksTest-high_school_european_history|5\": {\n \"acc\": 0.22424242424242424,\n \"acc_stderr\": 0.032568666616811015,\n \"acc_norm\": 0.22424242424242424,\n \"acc_norm_stderr\": 0.032568666616811015\n },\n \"harness|hendrycksTest-high_school_geography|5\": {\n \"acc\": 0.18181818181818182,\n \"acc_stderr\": 0.027479603010538787,\n \"acc_norm\": 0.18181818181818182,\n \"acc_norm_stderr\": 0.027479603010538787\n },\n \"harness|hendrycksTest-high_school_government_and_politics|5\": {\n \"acc\": 0.35751295336787564,\n \"acc_stderr\": 0.034588160421810045,\n \"acc_norm\": 0.35751295336787564,\n \"acc_norm_stderr\": 0.034588160421810045\n },\n \"harness|hendrycksTest-high_school_macroeconomics|5\": {\n \"acc\": 0.19487179487179487,\n \"acc_stderr\": 0.02008316759518139,\n \"acc_norm\": 0.19487179487179487,\n \"acc_norm_stderr\": 0.02008316759518139\n },\n \"harness|hendrycksTest-high_school_mathematics|5\": {\n \"acc\": 0.26296296296296295,\n \"acc_stderr\": 0.02684205787383371,\n \"acc_norm\": 0.26296296296296295,\n \"acc_norm_stderr\": 0.02684205787383371\n },\n \"harness|hendrycksTest-high_school_microeconomics|5\": {\n \"acc\": 0.22268907563025211,\n \"acc_stderr\": 0.02702543349888238,\n \"acc_norm\": 0.22268907563025211,\n \"acc_norm_stderr\": 0.02702543349888238\n },\n \"harness|hendrycksTest-high_school_physics|5\": {\n \"acc\": 0.2185430463576159,\n \"acc_stderr\": 0.03374235550425694,\n \"acc_norm\": 0.2185430463576159,\n \"acc_norm_stderr\": 0.03374235550425694\n },\n \"harness|hendrycksTest-high_school_psychology|5\": {\n \"acc\": 0.3284403669724771,\n \"acc_stderr\": 0.02013590279729839,\n \"acc_norm\": 0.3284403669724771,\n \"acc_norm_stderr\": 0.02013590279729839\n },\n \"harness|hendrycksTest-high_school_statistics|5\": {\n \"acc\": 0.14814814814814814,\n \"acc_stderr\": 0.024227629273728356,\n \"acc_norm\": 0.14814814814814814,\n \"acc_norm_stderr\": 0.024227629273728356\n },\n \"harness|hendrycksTest-high_school_us_history|5\": {\n \"acc\": 0.24509803921568626,\n \"acc_stderr\": 0.030190282453501954,\n \"acc_norm\": 0.24509803921568626,\n \"acc_norm_stderr\": 0.030190282453501954\n },\n \"harness|hendrycksTest-high_school_world_history|5\": {\n \"acc\": 0.270042194092827,\n \"acc_stderr\": 0.028900721906293426,\n \"acc_norm\": 0.270042194092827,\n \"acc_norm_stderr\": 0.028900721906293426\n },\n \"harness|hendrycksTest-human_aging|5\": {\n \"acc\": 0.2645739910313901,\n \"acc_stderr\": 0.029605103217038315,\n \"acc_norm\": 0.2645739910313901,\n \"acc_norm_stderr\": 0.029605103217038315\n },\n \"harness|hendrycksTest-human_sexuality|5\": {\n \"acc\": 0.2595419847328244,\n \"acc_stderr\": 0.03844876139785271,\n \"acc_norm\": 0.2595419847328244,\n \"acc_norm_stderr\": 0.03844876139785271\n },\n \"harness|hendrycksTest-international_law|5\": {\n \"acc\": 0.2396694214876033,\n \"acc_stderr\": 0.03896878985070417,\n \"acc_norm\": 0.2396694214876033,\n \"acc_norm_stderr\": 0.03896878985070417\n },\n \"harness|hendrycksTest-jurisprudence|5\": {\n \"acc\": 0.25,\n \"acc_stderr\": 0.04186091791394607,\n \"acc_norm\": 0.25,\n \"acc_norm_stderr\": 0.04186091791394607\n },\n \"harness|hendrycksTest-logical_fallacies|5\": {\n \"acc\": 0.2147239263803681,\n \"acc_stderr\": 0.03226219377286774,\n \"acc_norm\": 0.2147239263803681,\n \"acc_norm_stderr\": 0.03226219377286774\n },\n \"harness|hendrycksTest-machine_learning|5\": {\n \"acc\": 0.3125,\n \"acc_stderr\": 0.043994650575715215,\n \"acc_norm\": 0.3125,\n \"acc_norm_stderr\": 0.043994650575715215\n },\n \"harness|hendrycksTest-management|5\": {\n \"acc\": 0.3883495145631068,\n \"acc_stderr\": 0.048257293373563895,\n \"acc_norm\": 0.3883495145631068,\n \"acc_norm_stderr\": 0.048257293373563895\n },\n \"harness|hendrycksTest-marketing|5\": {\n \"acc\": 0.28205128205128205,\n \"acc_stderr\": 0.029480360549541194,\n \"acc_norm\": 0.28205128205128205,\n \"acc_norm_stderr\": 0.029480360549541194\n },\n \"harness|hendrycksTest-medical_genetics|5\": {\n \"acc\": 0.3,\n \"acc_stderr\": 0.046056618647183814,\n \"acc_norm\": 0.3,\n \"acc_norm_stderr\": 0.046056618647183814\n },\n \"harness|hendrycksTest-miscellaneous|5\": {\n \"acc\": 0.20561941251596424,\n \"acc_stderr\": 0.014452500456785823,\n \"acc_norm\": 0.20561941251596424,\n \"acc_norm_stderr\": 0.014452500456785823\n },\n \"harness|hendrycksTest-moral_disputes|5\": {\n \"acc\": 0.21098265895953758,\n \"acc_stderr\": 0.021966309947043128,\n \"acc_norm\": 0.21098265895953758,\n \"acc_norm_stderr\": 0.021966309947043128\n },\n \"harness|hendrycksTest-moral_scenarios|5\": {\n \"acc\": 0.23798882681564246,\n \"acc_stderr\": 0.014242630070574915,\n \"acc_norm\": 0.23798882681564246,\n \"acc_norm_stderr\": 0.014242630070574915\n },\n \"harness|hendrycksTest-nutrition|5\": {\n \"acc\": 0.2549019607843137,\n \"acc_stderr\": 0.024954184324879905,\n \"acc_norm\": 0.2549019607843137,\n \"acc_norm_stderr\": 0.024954184324879905\n },\n \"harness|hendrycksTest-philosophy|5\": {\n \"acc\": 0.18006430868167203,\n \"acc_stderr\": 0.021823422857744953,\n \"acc_norm\": 0.18006430868167203,\n \"acc_norm_stderr\": 0.021823422857744953\n },\n \"harness|hendrycksTest-prehistory|5\": {\n \"acc\": 0.21296296296296297,\n \"acc_stderr\": 0.0227797190887334,\n \"acc_norm\": 0.21296296296296297,\n \"acc_norm_stderr\": 0.0227797190887334\n },\n \"harness|hendrycksTest-professional_accounting|5\": {\n \"acc\": 0.24113475177304963,\n \"acc_stderr\": 0.025518731049537755,\n \"acc_norm\": 0.24113475177304963,\n \"acc_norm_stderr\": 0.025518731049537755\n },\n \"harness|hendrycksTest-professional_law|5\": {\n \"acc\": 0.24902216427640156,\n \"acc_stderr\": 0.011044892264040769,\n \"acc_norm\": 0.24902216427640156,\n \"acc_norm_stderr\": 0.011044892264040769\n },\n \"harness|hendrycksTest-professional_medicine|5\": {\n \"acc\": 0.16544117647058823,\n \"acc_stderr\": 0.022571771025494767,\n \"acc_norm\": 0.16544117647058823,\n \"acc_norm_stderr\": 0.022571771025494767\n },\n \"harness|hendrycksTest-professional_psychology|5\": {\n \"acc\": 0.25163398692810457,\n \"acc_stderr\": 0.01755581809132226,\n \"acc_norm\": 0.25163398692810457,\n \"acc_norm_stderr\": 0.01755581809132226\n },\n \"harness|hendrycksTest-public_relations|5\": {\n \"acc\": 0.19090909090909092,\n \"acc_stderr\": 0.03764425585984927,\n \"acc_norm\": 0.19090909090909092,\n \"acc_norm_stderr\": 0.03764425585984927\n },\n \"harness|hendrycksTest-security_studies|5\": {\n \"acc\": 0.24081632653061225,\n \"acc_stderr\": 0.027372942201788163,\n \"acc_norm\": 0.24081632653061225,\n \"acc_norm_stderr\": 0.027372942201788163\n },\n \"harness|hendrycksTest-sociology|5\": {\n \"acc\": 0.23880597014925373,\n \"acc_stderr\": 0.030147775935409214,\n \"acc_norm\": 0.23880597014925373,\n \"acc_norm_stderr\": 0.030147775935409214\n },\n \"harness|hendrycksTest-us_foreign_policy|5\": {\n \"acc\": 0.27,\n \"acc_stderr\": 0.0446196043338474,\n \"acc_norm\": 0.27,\n \"acc_norm_stderr\": 0.0446196043338474\n },\n \"harness|hendrycksTest-virology|5\": {\n \"acc\": 0.28313253012048195,\n \"acc_stderr\": 0.03507295431370518,\n \"acc_norm\": 0.28313253012048195,\n \"acc_norm_stderr\": 0.03507295431370518\n },\n \"harness|hendrycksTest-world_religions|5\": {\n \"acc\": 0.19883040935672514,\n \"acc_stderr\": 0.03061111655743253,\n \"acc_norm\": 0.19883040935672514,\n \"acc_norm_stderr\": 0.03061111655743253\n },\n \"harness|truthfulqa:mc|0\": {\n \"mc1\": 0.2350061199510404,\n \"mc1_stderr\": 0.014843061507731601,\n \"mc2\": 0.4827953936445661,\n \"mc2_stderr\": 0.01642500560197448\n },\n \"harness|winogrande|5\": {\n \"acc\": 0.4940805051302289,\n \"acc_stderr\": 0.014051500838485807\n },\n \"harness|gsm8k|5\": {\n \"acc\": 0.008339651250947688,\n \"acc_stderr\": 0.0025049422268605135\n }\n}\n```", "repo_url": "https://huggingface.co/euclaise/crow-1b", "leaderboard_url": "https://huggingface.co/spaces/HuggingFaceH4/open_llm_leaderboard", "point_of_contact": "[email protected]", "configs": [{"config_name": "harness_arc_challenge_25", "data_files": [{"split": "2024_01_10T15_21_15.168348", "path": ["**/details_harness|arc:challenge|25_2024-01-10T15-21-15.168348.parquet"]}, {"split": "latest", "path": ["**/details_harness|arc:challenge|25_2024-01-10T15-21-15.168348.parquet"]}]}, {"config_name": "harness_gsm8k_5", "data_files": [{"split": "2024_01_10T15_21_15.168348", "path": ["**/details_harness|gsm8k|5_2024-01-10T15-21-15.168348.parquet"]}, {"split": "latest", "path": ["**/details_harness|gsm8k|5_2024-01-10T15-21-15.168348.parquet"]}]}, {"config_name": "harness_hellaswag_10", "data_files": [{"split": "2024_01_10T15_21_15.168348", "path": ["**/details_harness|hellaswag|10_2024-01-10T15-21-15.168348.parquet"]}, {"split": "latest", "path": ["**/details_harness|hellaswag|10_2024-01-10T15-21-15.168348.parquet"]}]}, {"config_name": "harness_hendrycksTest_5", "data_files": [{"split": "2024_01_10T15_21_15.168348", "path": ["**/details_harness|hendrycksTest-abstract_algebra|5_2024-01-10T15-21-15.168348.parquet", "**/details_harness|hendrycksTest-anatomy|5_2024-01-10T15-21-15.168348.parquet", "**/details_harness|hendrycksTest-astronomy|5_2024-01-10T15-21-15.168348.parquet", "**/details_harness|hendrycksTest-business_ethics|5_2024-01-10T15-21-15.168348.parquet", "**/details_harness|hendrycksTest-clinical_knowledge|5_2024-01-10T15-21-15.168348.parquet", "**/details_harness|hendrycksTest-college_biology|5_2024-01-10T15-21-15.168348.parquet", "**/details_harness|hendrycksTest-college_chemistry|5_2024-01-10T15-21-15.168348.parquet", "**/details_harness|hendrycksTest-college_computer_science|5_2024-01-10T15-21-15.168348.parquet", "**/details_harness|hendrycksTest-college_mathematics|5_2024-01-10T15-21-15.168348.parquet", "**/details_harness|hendrycksTest-college_medicine|5_2024-01-10T15-21-15.168348.parquet", "**/details_harness|hendrycksTest-college_physics|5_2024-01-10T15-21-15.168348.parquet", "**/details_harness|hendrycksTest-computer_security|5_2024-01-10T15-21-15.168348.parquet", "**/details_harness|hendrycksTest-conceptual_physics|5_2024-01-10T15-21-15.168348.parquet", "**/details_harness|hendrycksTest-econometrics|5_2024-01-10T15-21-15.168348.parquet", "**/details_harness|hendrycksTest-electrical_engineering|5_2024-01-10T15-21-15.168348.parquet", "**/details_harness|hendrycksTest-elementary_mathematics|5_2024-01-10T15-21-15.168348.parquet", "**/details_harness|hendrycksTest-formal_logic|5_2024-01-10T15-21-15.168348.parquet", "**/details_harness|hendrycksTest-global_facts|5_2024-01-10T15-21-15.168348.parquet", "**/details_harness|hendrycksTest-high_school_biology|5_2024-01-10T15-21-15.168348.parquet", "**/details_harness|hendrycksTest-high_school_chemistry|5_2024-01-10T15-21-15.168348.parquet", "**/details_harness|hendrycksTest-high_school_computer_science|5_2024-01-10T15-21-15.168348.parquet", "**/details_harness|hendrycksTest-high_school_european_history|5_2024-01-10T15-21-15.168348.parquet", "**/details_harness|hendrycksTest-high_school_geography|5_2024-01-10T15-21-15.168348.parquet", "**/details_harness|hendrycksTest-high_school_government_and_politics|5_2024-01-10T15-21-15.168348.parquet", "**/details_harness|hendrycksTest-high_school_macroeconomics|5_2024-01-10T15-21-15.168348.parquet", "**/details_harness|hendrycksTest-high_school_mathematics|5_2024-01-10T15-21-15.168348.parquet", "**/details_harness|hendrycksTest-high_school_microeconomics|5_2024-01-10T15-21-15.168348.parquet", "**/details_harness|hendrycksTest-high_school_physics|5_2024-01-10T15-21-15.168348.parquet", "**/details_harness|hendrycksTest-high_school_psychology|5_2024-01-10T15-21-15.168348.parquet", "**/details_harness|hendrycksTest-high_school_statistics|5_2024-01-10T15-21-15.168348.parquet", "**/details_harness|hendrycksTest-high_school_us_history|5_2024-01-10T15-21-15.168348.parquet", "**/details_harness|hendrycksTest-high_school_world_history|5_2024-01-10T15-21-15.168348.parquet", "**/details_harness|hendrycksTest-human_aging|5_2024-01-10T15-21-15.168348.parquet", "**/details_harness|hendrycksTest-human_sexuality|5_2024-01-10T15-21-15.168348.parquet", "**/details_harness|hendrycksTest-international_law|5_2024-01-10T15-21-15.168348.parquet", "**/details_harness|hendrycksTest-jurisprudence|5_2024-01-10T15-21-15.168348.parquet", "**/details_harness|hendrycksTest-logical_fallacies|5_2024-01-10T15-21-15.168348.parquet", "**/details_harness|hendrycksTest-machine_learning|5_2024-01-10T15-21-15.168348.parquet", "**/details_harness|hendrycksTest-management|5_2024-01-10T15-21-15.168348.parquet", "**/details_harness|hendrycksTest-marketing|5_2024-01-10T15-21-15.168348.parquet", "**/details_harness|hendrycksTest-medical_genetics|5_2024-01-10T15-21-15.168348.parquet", "**/details_harness|hendrycksTest-miscellaneous|5_2024-01-10T15-21-15.168348.parquet", "**/details_harness|hendrycksTest-moral_disputes|5_2024-01-10T15-21-15.168348.parquet", "**/details_harness|hendrycksTest-moral_scenarios|5_2024-01-10T15-21-15.168348.parquet", "**/details_harness|hendrycksTest-nutrition|5_2024-01-10T15-21-15.168348.parquet", "**/details_harness|hendrycksTest-philosophy|5_2024-01-10T15-21-15.168348.parquet", "**/details_harness|hendrycksTest-prehistory|5_2024-01-10T15-21-15.168348.parquet", "**/details_harness|hendrycksTest-professional_accounting|5_2024-01-10T15-21-15.168348.parquet", "**/details_harness|hendrycksTest-professional_law|5_2024-01-10T15-21-15.168348.parquet", "**/details_harness|hendrycksTest-professional_medicine|5_2024-01-10T15-21-15.168348.parquet", "**/details_harness|hendrycksTest-professional_psychology|5_2024-01-10T15-21-15.168348.parquet", "**/details_harness|hendrycksTest-public_relations|5_2024-01-10T15-21-15.168348.parquet", "**/details_harness|hendrycksTest-security_studies|5_2024-01-10T15-21-15.168348.parquet", "**/details_harness|hendrycksTest-sociology|5_2024-01-10T15-21-15.168348.parquet", "**/details_harness|hendrycksTest-us_foreign_policy|5_2024-01-10T15-21-15.168348.parquet", "**/details_harness|hendrycksTest-virology|5_2024-01-10T15-21-15.168348.parquet", "**/details_harness|hendrycksTest-world_religions|5_2024-01-10T15-21-15.168348.parquet"]}, {"split": "latest", "path": ["**/details_harness|hendrycksTest-abstract_algebra|5_2024-01-10T15-21-15.168348.parquet", "**/details_harness|hendrycksTest-anatomy|5_2024-01-10T15-21-15.168348.parquet", "**/details_harness|hendrycksTest-astronomy|5_2024-01-10T15-21-15.168348.parquet", "**/details_harness|hendrycksTest-business_ethics|5_2024-01-10T15-21-15.168348.parquet", "**/details_harness|hendrycksTest-clinical_knowledge|5_2024-01-10T15-21-15.168348.parquet", "**/details_harness|hendrycksTest-college_biology|5_2024-01-10T15-21-15.168348.parquet", "**/details_harness|hendrycksTest-college_chemistry|5_2024-01-10T15-21-15.168348.parquet", "**/details_harness|hendrycksTest-college_computer_science|5_2024-01-10T15-21-15.168348.parquet", "**/details_harness|hendrycksTest-college_mathematics|5_2024-01-10T15-21-15.168348.parquet", "**/details_harness|hendrycksTest-college_medicine|5_2024-01-10T15-21-15.168348.parquet", "**/details_harness|hendrycksTest-college_physics|5_2024-01-10T15-21-15.168348.parquet", "**/details_harness|hendrycksTest-computer_security|5_2024-01-10T15-21-15.168348.parquet", "**/details_harness|hendrycksTest-conceptual_physics|5_2024-01-10T15-21-15.168348.parquet", "**/details_harness|hendrycksTest-econometrics|5_2024-01-10T15-21-15.168348.parquet", "**/details_harness|hendrycksTest-electrical_engineering|5_2024-01-10T15-21-15.168348.parquet", "**/details_harness|hendrycksTest-elementary_mathematics|5_2024-01-10T15-21-15.168348.parquet", "**/details_harness|hendrycksTest-formal_logic|5_2024-01-10T15-21-15.168348.parquet", "**/details_harness|hendrycksTest-global_facts|5_2024-01-10T15-21-15.168348.parquet", "**/details_harness|hendrycksTest-high_school_biology|5_2024-01-10T15-21-15.168348.parquet", "**/details_harness|hendrycksTest-high_school_chemistry|5_2024-01-10T15-21-15.168348.parquet", "**/details_harness|hendrycksTest-high_school_computer_science|5_2024-01-10T15-21-15.168348.parquet", "**/details_harness|hendrycksTest-high_school_european_history|5_2024-01-10T15-21-15.168348.parquet", "**/details_harness|hendrycksTest-high_school_geography|5_2024-01-10T15-21-15.168348.parquet", "**/details_harness|hendrycksTest-high_school_government_and_politics|5_2024-01-10T15-21-15.168348.parquet", "**/details_harness|hendrycksTest-high_school_macroeconomics|5_2024-01-10T15-21-15.168348.parquet", "**/details_harness|hendrycksTest-high_school_mathematics|5_2024-01-10T15-21-15.168348.parquet", "**/details_harness|hendrycksTest-high_school_microeconomics|5_2024-01-10T15-21-15.168348.parquet", "**/details_harness|hendrycksTest-high_school_physics|5_2024-01-10T15-21-15.168348.parquet", "**/details_harness|hendrycksTest-high_school_psychology|5_2024-01-10T15-21-15.168348.parquet", "**/details_harness|hendrycksTest-high_school_statistics|5_2024-01-10T15-21-15.168348.parquet", "**/details_harness|hendrycksTest-high_school_us_history|5_2024-01-10T15-21-15.168348.parquet", "**/details_harness|hendrycksTest-high_school_world_history|5_2024-01-10T15-21-15.168348.parquet", "**/details_harness|hendrycksTest-human_aging|5_2024-01-10T15-21-15.168348.parquet", "**/details_harness|hendrycksTest-human_sexuality|5_2024-01-10T15-21-15.168348.parquet", "**/details_harness|hendrycksTest-international_law|5_2024-01-10T15-21-15.168348.parquet", "**/details_harness|hendrycksTest-jurisprudence|5_2024-01-10T15-21-15.168348.parquet", "**/details_harness|hendrycksTest-logical_fallacies|5_2024-01-10T15-21-15.168348.parquet", "**/details_harness|hendrycksTest-machine_learning|5_2024-01-10T15-21-15.168348.parquet", "**/details_harness|hendrycksTest-management|5_2024-01-10T15-21-15.168348.parquet", "**/details_harness|hendrycksTest-marketing|5_2024-01-10T15-21-15.168348.parquet", "**/details_harness|hendrycksTest-medical_genetics|5_2024-01-10T15-21-15.168348.parquet", "**/details_harness|hendrycksTest-miscellaneous|5_2024-01-10T15-21-15.168348.parquet", "**/details_harness|hendrycksTest-moral_disputes|5_2024-01-10T15-21-15.168348.parquet", "**/details_harness|hendrycksTest-moral_scenarios|5_2024-01-10T15-21-15.168348.parquet", "**/details_harness|hendrycksTest-nutrition|5_2024-01-10T15-21-15.168348.parquet", "**/details_harness|hendrycksTest-philosophy|5_2024-01-10T15-21-15.168348.parquet", "**/details_harness|hendrycksTest-prehistory|5_2024-01-10T15-21-15.168348.parquet", "**/details_harness|hendrycksTest-professional_accounting|5_2024-01-10T15-21-15.168348.parquet", "**/details_harness|hendrycksTest-professional_law|5_2024-01-10T15-21-15.168348.parquet", "**/details_harness|hendrycksTest-professional_medicine|5_2024-01-10T15-21-15.168348.parquet", "**/details_harness|hendrycksTest-professional_psychology|5_2024-01-10T15-21-15.168348.parquet", "**/details_harness|hendrycksTest-public_relations|5_2024-01-10T15-21-15.168348.parquet", "**/details_harness|hendrycksTest-security_studies|5_2024-01-10T15-21-15.168348.parquet", "**/details_harness|hendrycksTest-sociology|5_2024-01-10T15-21-15.168348.parquet", "**/details_harness|hendrycksTest-us_foreign_policy|5_2024-01-10T15-21-15.168348.parquet", "**/details_harness|hendrycksTest-virology|5_2024-01-10T15-21-15.168348.parquet", "**/details_harness|hendrycksTest-world_religions|5_2024-01-10T15-21-15.168348.parquet"]}]}, {"config_name": "harness_hendrycksTest_abstract_algebra_5", "data_files": [{"split": "2024_01_10T15_21_15.168348", "path": ["**/details_harness|hendrycksTest-abstract_algebra|5_2024-01-10T15-21-15.168348.parquet"]}, {"split": "latest", "path": ["**/details_harness|hendrycksTest-abstract_algebra|5_2024-01-10T15-21-15.168348.parquet"]}]}, {"config_name": "harness_hendrycksTest_anatomy_5", "data_files": [{"split": "2024_01_10T15_21_15.168348", "path": ["**/details_harness|hendrycksTest-anatomy|5_2024-01-10T15-21-15.168348.parquet"]}, {"split": "latest", "path": ["**/details_harness|hendrycksTest-anatomy|5_2024-01-10T15-21-15.168348.parquet"]}]}, {"config_name": "harness_hendrycksTest_astronomy_5", "data_files": [{"split": "2024_01_10T15_21_15.168348", "path": ["**/details_harness|hendrycksTest-astronomy|5_2024-01-10T15-21-15.168348.parquet"]}, {"split": "latest", "path": ["**/details_harness|hendrycksTest-astronomy|5_2024-01-10T15-21-15.168348.parquet"]}]}, {"config_name": "harness_hendrycksTest_business_ethics_5", "data_files": [{"split": "2024_01_10T15_21_15.168348", "path": ["**/details_harness|hendrycksTest-business_ethics|5_2024-01-10T15-21-15.168348.parquet"]}, {"split": "latest", "path": ["**/details_harness|hendrycksTest-business_ethics|5_2024-01-10T15-21-15.168348.parquet"]}]}, {"config_name": "harness_hendrycksTest_clinical_knowledge_5", "data_files": [{"split": "2024_01_10T15_21_15.168348", "path": ["**/details_harness|hendrycksTest-clinical_knowledge|5_2024-01-10T15-21-15.168348.parquet"]}, {"split": "latest", "path": ["**/details_harness|hendrycksTest-clinical_knowledge|5_2024-01-10T15-21-15.168348.parquet"]}]}, {"config_name": "harness_hendrycksTest_college_biology_5", "data_files": [{"split": "2024_01_10T15_21_15.168348", "path": ["**/details_harness|hendrycksTest-college_biology|5_2024-01-10T15-21-15.168348.parquet"]}, {"split": "latest", "path": ["**/details_harness|hendrycksTest-college_biology|5_2024-01-10T15-21-15.168348.parquet"]}]}, {"config_name": "harness_hendrycksTest_college_chemistry_5", "data_files": [{"split": "2024_01_10T15_21_15.168348", "path": ["**/details_harness|hendrycksTest-college_chemistry|5_2024-01-10T15-21-15.168348.parquet"]}, {"split": "latest", "path": ["**/details_harness|hendrycksTest-college_chemistry|5_2024-01-10T15-21-15.168348.parquet"]}]}, {"config_name": "harness_hendrycksTest_college_computer_science_5", "data_files": [{"split": "2024_01_10T15_21_15.168348", "path": ["**/details_harness|hendrycksTest-college_computer_science|5_2024-01-10T15-21-15.168348.parquet"]}, {"split": "latest", "path": ["**/details_harness|hendrycksTest-college_computer_science|5_2024-01-10T15-21-15.168348.parquet"]}]}, {"config_name": "harness_hendrycksTest_college_mathematics_5", "data_files": [{"split": "2024_01_10T15_21_15.168348", "path": ["**/details_harness|hendrycksTest-college_mathematics|5_2024-01-10T15-21-15.168348.parquet"]}, {"split": "latest", "path": ["**/details_harness|hendrycksTest-college_mathematics|5_2024-01-10T15-21-15.168348.parquet"]}]}, {"config_name": "harness_hendrycksTest_college_medicine_5", "data_files": [{"split": "2024_01_10T15_21_15.168348", "path": ["**/details_harness|hendrycksTest-college_medicine|5_2024-01-10T15-21-15.168348.parquet"]}, {"split": "latest", "path": ["**/details_harness|hendrycksTest-college_medicine|5_2024-01-10T15-21-15.168348.parquet"]}]}, {"config_name": "harness_hendrycksTest_college_physics_5", "data_files": [{"split": "2024_01_10T15_21_15.168348", "path": ["**/details_harness|hendrycksTest-college_physics|5_2024-01-10T15-21-15.168348.parquet"]}, {"split": "latest", "path": ["**/details_harness|hendrycksTest-college_physics|5_2024-01-10T15-21-15.168348.parquet"]}]}, {"config_name": "harness_hendrycksTest_computer_security_5", "data_files": [{"split": "2024_01_10T15_21_15.168348", "path": ["**/details_harness|hendrycksTest-computer_security|5_2024-01-10T15-21-15.168348.parquet"]}, {"split": "latest", "path": ["**/details_harness|hendrycksTest-computer_security|5_2024-01-10T15-21-15.168348.parquet"]}]}, {"config_name": "harness_hendrycksTest_conceptual_physics_5", "data_files": [{"split": "2024_01_10T15_21_15.168348", "path": ["**/details_harness|hendrycksTest-conceptual_physics|5_2024-01-10T15-21-15.168348.parquet"]}, {"split": "latest", "path": ["**/details_harness|hendrycksTest-conceptual_physics|5_2024-01-10T15-21-15.168348.parquet"]}]}, {"config_name": "harness_hendrycksTest_econometrics_5", "data_files": [{"split": "2024_01_10T15_21_15.168348", "path": ["**/details_harness|hendrycksTest-econometrics|5_2024-01-10T15-21-15.168348.parquet"]}, {"split": "latest", "path": ["**/details_harness|hendrycksTest-econometrics|5_2024-01-10T15-21-15.168348.parquet"]}]}, {"config_name": "harness_hendrycksTest_electrical_engineering_5", "data_files": [{"split": "2024_01_10T15_21_15.168348", "path": ["**/details_harness|hendrycksTest-electrical_engineering|5_2024-01-10T15-21-15.168348.parquet"]}, {"split": "latest", "path": ["**/details_harness|hendrycksTest-electrical_engineering|5_2024-01-10T15-21-15.168348.parquet"]}]}, {"config_name": "harness_hendrycksTest_elementary_mathematics_5", "data_files": [{"split": "2024_01_10T15_21_15.168348", "path": ["**/details_harness|hendrycksTest-elementary_mathematics|5_2024-01-10T15-21-15.168348.parquet"]}, {"split": "latest", "path": ["**/details_harness|hendrycksTest-elementary_mathematics|5_2024-01-10T15-21-15.168348.parquet"]}]}, {"config_name": "harness_hendrycksTest_formal_logic_5", "data_files": [{"split": "2024_01_10T15_21_15.168348", "path": ["**/details_harness|hendrycksTest-formal_logic|5_2024-01-10T15-21-15.168348.parquet"]}, {"split": "latest", "path": ["**/details_harness|hendrycksTest-formal_logic|5_2024-01-10T15-21-15.168348.parquet"]}]}, {"config_name": "harness_hendrycksTest_global_facts_5", "data_files": [{"split": "2024_01_10T15_21_15.168348", "path": ["**/details_harness|hendrycksTest-global_facts|5_2024-01-10T15-21-15.168348.parquet"]}, {"split": "latest", "path": ["**/details_harness|hendrycksTest-global_facts|5_2024-01-10T15-21-15.168348.parquet"]}]}, {"config_name": "harness_hendrycksTest_high_school_biology_5", "data_files": [{"split": "2024_01_10T15_21_15.168348", "path": ["**/details_harness|hendrycksTest-high_school_biology|5_2024-01-10T15-21-15.168348.parquet"]}, {"split": "latest", "path": ["**/details_harness|hendrycksTest-high_school_biology|5_2024-01-10T15-21-15.168348.parquet"]}]}, {"config_name": "harness_hendrycksTest_high_school_chemistry_5", "data_files": [{"split": "2024_01_10T15_21_15.168348", "path": ["**/details_harness|hendrycksTest-high_school_chemistry|5_2024-01-10T15-21-15.168348.parquet"]}, {"split": "latest", "path": ["**/details_harness|hendrycksTest-high_school_chemistry|5_2024-01-10T15-21-15.168348.parquet"]}]}, {"config_name": "harness_hendrycksTest_high_school_computer_science_5", "data_files": [{"split": "2024_01_10T15_21_15.168348", "path": ["**/details_harness|hendrycksTest-high_school_computer_science|5_2024-01-10T15-21-15.168348.parquet"]}, {"split": "latest", "path": ["**/details_harness|hendrycksTest-high_school_computer_science|5_2024-01-10T15-21-15.168348.parquet"]}]}, {"config_name": "harness_hendrycksTest_high_school_european_history_5", "data_files": [{"split": "2024_01_10T15_21_15.168348", "path": ["**/details_harness|hendrycksTest-high_school_european_history|5_2024-01-10T15-21-15.168348.parquet"]}, {"split": "latest", "path": ["**/details_harness|hendrycksTest-high_school_european_history|5_2024-01-10T15-21-15.168348.parquet"]}]}, {"config_name": "harness_hendrycksTest_high_school_geography_5", "data_files": [{"split": "2024_01_10T15_21_15.168348", "path": ["**/details_harness|hendrycksTest-high_school_geography|5_2024-01-10T15-21-15.168348.parquet"]}, {"split": "latest", "path": ["**/details_harness|hendrycksTest-high_school_geography|5_2024-01-10T15-21-15.168348.parquet"]}]}, {"config_name": "harness_hendrycksTest_high_school_government_and_politics_5", "data_files": [{"split": "2024_01_10T15_21_15.168348", "path": ["**/details_harness|hendrycksTest-high_school_government_and_politics|5_2024-01-10T15-21-15.168348.parquet"]}, {"split": "latest", "path": ["**/details_harness|hendrycksTest-high_school_government_and_politics|5_2024-01-10T15-21-15.168348.parquet"]}]}, {"config_name": "harness_hendrycksTest_high_school_macroeconomics_5", "data_files": [{"split": "2024_01_10T15_21_15.168348", "path": ["**/details_harness|hendrycksTest-high_school_macroeconomics|5_2024-01-10T15-21-15.168348.parquet"]}, {"split": "latest", "path": ["**/details_harness|hendrycksTest-high_school_macroeconomics|5_2024-01-10T15-21-15.168348.parquet"]}]}, {"config_name": "harness_hendrycksTest_high_school_mathematics_5", "data_files": [{"split": "2024_01_10T15_21_15.168348", "path": ["**/details_harness|hendrycksTest-high_school_mathematics|5_2024-01-10T15-21-15.168348.parquet"]}, {"split": "latest", "path": ["**/details_harness|hendrycksTest-high_school_mathematics|5_2024-01-10T15-21-15.168348.parquet"]}]}, {"config_name": "harness_hendrycksTest_high_school_microeconomics_5", "data_files": [{"split": "2024_01_10T15_21_15.168348", "path": ["**/details_harness|hendrycksTest-high_school_microeconomics|5_2024-01-10T15-21-15.168348.parquet"]}, {"split": "latest", "path": ["**/details_harness|hendrycksTest-high_school_microeconomics|5_2024-01-10T15-21-15.168348.parquet"]}]}, {"config_name": "harness_hendrycksTest_high_school_physics_5", "data_files": [{"split": "2024_01_10T15_21_15.168348", "path": ["**/details_harness|hendrycksTest-high_school_physics|5_2024-01-10T15-21-15.168348.parquet"]}, {"split": "latest", "path": ["**/details_harness|hendrycksTest-high_school_physics|5_2024-01-10T15-21-15.168348.parquet"]}]}, {"config_name": "harness_hendrycksTest_high_school_psychology_5", "data_files": [{"split": "2024_01_10T15_21_15.168348", "path": ["**/details_harness|hendrycksTest-high_school_psychology|5_2024-01-10T15-21-15.168348.parquet"]}, {"split": "latest", "path": ["**/details_harness|hendrycksTest-high_school_psychology|5_2024-01-10T15-21-15.168348.parquet"]}]}, {"config_name": "harness_hendrycksTest_high_school_statistics_5", "data_files": [{"split": "2024_01_10T15_21_15.168348", "path": ["**/details_harness|hendrycksTest-high_school_statistics|5_2024-01-10T15-21-15.168348.parquet"]}, {"split": "latest", "path": ["**/details_harness|hendrycksTest-high_school_statistics|5_2024-01-10T15-21-15.168348.parquet"]}]}, {"config_name": "harness_hendrycksTest_high_school_us_history_5", "data_files": [{"split": "2024_01_10T15_21_15.168348", "path": ["**/details_harness|hendrycksTest-high_school_us_history|5_2024-01-10T15-21-15.168348.parquet"]}, {"split": "latest", "path": ["**/details_harness|hendrycksTest-high_school_us_history|5_2024-01-10T15-21-15.168348.parquet"]}]}, {"config_name": "harness_hendrycksTest_high_school_world_history_5", "data_files": [{"split": "2024_01_10T15_21_15.168348", "path": ["**/details_harness|hendrycksTest-high_school_world_history|5_2024-01-10T15-21-15.168348.parquet"]}, {"split": "latest", "path": ["**/details_harness|hendrycksTest-high_school_world_history|5_2024-01-10T15-21-15.168348.parquet"]}]}, {"config_name": "harness_hendrycksTest_human_aging_5", "data_files": [{"split": "2024_01_10T15_21_15.168348", "path": ["**/details_harness|hendrycksTest-human_aging|5_2024-01-10T15-21-15.168348.parquet"]}, {"split": "latest", "path": ["**/details_harness|hendrycksTest-human_aging|5_2024-01-10T15-21-15.168348.parquet"]}]}, {"config_name": "harness_hendrycksTest_human_sexuality_5", "data_files": [{"split": "2024_01_10T15_21_15.168348", "path": ["**/details_harness|hendrycksTest-human_sexuality|5_2024-01-10T15-21-15.168348.parquet"]}, {"split": "latest", "path": ["**/details_harness|hendrycksTest-human_sexuality|5_2024-01-10T15-21-15.168348.parquet"]}]}, {"config_name": "harness_hendrycksTest_international_law_5", "data_files": [{"split": "2024_01_10T15_21_15.168348", "path": ["**/details_harness|hendrycksTest-international_law|5_2024-01-10T15-21-15.168348.parquet"]}, {"split": "latest", "path": ["**/details_harness|hendrycksTest-international_law|5_2024-01-10T15-21-15.168348.parquet"]}]}, {"config_name": "harness_hendrycksTest_jurisprudence_5", "data_files": [{"split": "2024_01_10T15_21_15.168348", "path": ["**/details_harness|hendrycksTest-jurisprudence|5_2024-01-10T15-21-15.168348.parquet"]}, {"split": "latest", "path": ["**/details_harness|hendrycksTest-jurisprudence|5_2024-01-10T15-21-15.168348.parquet"]}]}, {"config_name": "harness_hendrycksTest_logical_fallacies_5", "data_files": [{"split": "2024_01_10T15_21_15.168348", "path": ["**/details_harness|hendrycksTest-logical_fallacies|5_2024-01-10T15-21-15.168348.parquet"]}, {"split": "latest", "path": ["**/details_harness|hendrycksTest-logical_fallacies|5_2024-01-10T15-21-15.168348.parquet"]}]}, {"config_name": "harness_hendrycksTest_machine_learning_5", "data_files": [{"split": "2024_01_10T15_21_15.168348", "path": ["**/details_harness|hendrycksTest-machine_learning|5_2024-01-10T15-21-15.168348.parquet"]}, {"split": "latest", "path": ["**/details_harness|hendrycksTest-machine_learning|5_2024-01-10T15-21-15.168348.parquet"]}]}, {"config_name": "harness_hendrycksTest_management_5", "data_files": [{"split": "2024_01_10T15_21_15.168348", "path": ["**/details_harness|hendrycksTest-management|5_2024-01-10T15-21-15.168348.parquet"]}, {"split": "latest", "path": ["**/details_harness|hendrycksTest-management|5_2024-01-10T15-21-15.168348.parquet"]}]}, {"config_name": "harness_hendrycksTest_marketing_5", "data_files": [{"split": "2024_01_10T15_21_15.168348", "path": ["**/details_harness|hendrycksTest-marketing|5_2024-01-10T15-21-15.168348.parquet"]}, {"split": "latest", "path": ["**/details_harness|hendrycksTest-marketing|5_2024-01-10T15-21-15.168348.parquet"]}]}, {"config_name": "harness_hendrycksTest_medical_genetics_5", "data_files": [{"split": "2024_01_10T15_21_15.168348", "path": ["**/details_harness|hendrycksTest-medical_genetics|5_2024-01-10T15-21-15.168348.parquet"]}, {"split": "latest", "path": ["**/details_harness|hendrycksTest-medical_genetics|5_2024-01-10T15-21-15.168348.parquet"]}]}, {"config_name": "harness_hendrycksTest_miscellaneous_5", "data_files": [{"split": "2024_01_10T15_21_15.168348", "path": ["**/details_harness|hendrycksTest-miscellaneous|5_2024-01-10T15-21-15.168348.parquet"]}, {"split": "latest", "path": ["**/details_harness|hendrycksTest-miscellaneous|5_2024-01-10T15-21-15.168348.parquet"]}]}, {"config_name": "harness_hendrycksTest_moral_disputes_5", "data_files": [{"split": "2024_01_10T15_21_15.168348", "path": ["**/details_harness|hendrycksTest-moral_disputes|5_2024-01-10T15-21-15.168348.parquet"]}, {"split": "latest", "path": ["**/details_harness|hendrycksTest-moral_disputes|5_2024-01-10T15-21-15.168348.parquet"]}]}, {"config_name": "harness_hendrycksTest_moral_scenarios_5", "data_files": [{"split": "2024_01_10T15_21_15.168348", "path": ["**/details_harness|hendrycksTest-moral_scenarios|5_2024-01-10T15-21-15.168348.parquet"]}, {"split": "latest", "path": ["**/details_harness|hendrycksTest-moral_scenarios|5_2024-01-10T15-21-15.168348.parquet"]}]}, {"config_name": "harness_hendrycksTest_nutrition_5", "data_files": [{"split": "2024_01_10T15_21_15.168348", "path": ["**/details_harness|hendrycksTest-nutrition|5_2024-01-10T15-21-15.168348.parquet"]}, {"split": "latest", "path": ["**/details_harness|hendrycksTest-nutrition|5_2024-01-10T15-21-15.168348.parquet"]}]}, {"config_name": "harness_hendrycksTest_philosophy_5", "data_files": [{"split": "2024_01_10T15_21_15.168348", "path": ["**/details_harness|hendrycksTest-philosophy|5_2024-01-10T15-21-15.168348.parquet"]}, {"split": "latest", "path": ["**/details_harness|hendrycksTest-philosophy|5_2024-01-10T15-21-15.168348.parquet"]}]}, {"config_name": "harness_hendrycksTest_prehistory_5", "data_files": [{"split": "2024_01_10T15_21_15.168348", "path": ["**/details_harness|hendrycksTest-prehistory|5_2024-01-10T15-21-15.168348.parquet"]}, {"split": "latest", "path": ["**/details_harness|hendrycksTest-prehistory|5_2024-01-10T15-21-15.168348.parquet"]}]}, {"config_name": "harness_hendrycksTest_professional_accounting_5", "data_files": [{"split": "2024_01_10T15_21_15.168348", "path": ["**/details_harness|hendrycksTest-professional_accounting|5_2024-01-10T15-21-15.168348.parquet"]}, {"split": "latest", "path": ["**/details_harness|hendrycksTest-professional_accounting|5_2024-01-10T15-21-15.168348.parquet"]}]}, {"config_name": "harness_hendrycksTest_professional_law_5", "data_files": [{"split": "2024_01_10T15_21_15.168348", "path": ["**/details_harness|hendrycksTest-professional_law|5_2024-01-10T15-21-15.168348.parquet"]}, {"split": "latest", "path": ["**/details_harness|hendrycksTest-professional_law|5_2024-01-10T15-21-15.168348.parquet"]}]}, {"config_name": "harness_hendrycksTest_professional_medicine_5", "data_files": [{"split": "2024_01_10T15_21_15.168348", "path": ["**/details_harness|hendrycksTest-professional_medicine|5_2024-01-10T15-21-15.168348.parquet"]}, {"split": "latest", "path": ["**/details_harness|hendrycksTest-professional_medicine|5_2024-01-10T15-21-15.168348.parquet"]}]}, {"config_name": "harness_hendrycksTest_professional_psychology_5", "data_files": [{"split": "2024_01_10T15_21_15.168348", "path": ["**/details_harness|hendrycksTest-professional_psychology|5_2024-01-10T15-21-15.168348.parquet"]}, {"split": "latest", "path": ["**/details_harness|hendrycksTest-professional_psychology|5_2024-01-10T15-21-15.168348.parquet"]}]}, {"config_name": "harness_hendrycksTest_public_relations_5", "data_files": [{"split": "2024_01_10T15_21_15.168348", "path": ["**/details_harness|hendrycksTest-public_relations|5_2024-01-10T15-21-15.168348.parquet"]}, {"split": "latest", "path": ["**/details_harness|hendrycksTest-public_relations|5_2024-01-10T15-21-15.168348.parquet"]}]}, {"config_name": "harness_hendrycksTest_security_studies_5", "data_files": [{"split": "2024_01_10T15_21_15.168348", "path": ["**/details_harness|hendrycksTest-security_studies|5_2024-01-10T15-21-15.168348.parquet"]}, {"split": "latest", "path": ["**/details_harness|hendrycksTest-security_studies|5_2024-01-10T15-21-15.168348.parquet"]}]}, {"config_name": "harness_hendrycksTest_sociology_5", "data_files": [{"split": "2024_01_10T15_21_15.168348", "path": ["**/details_harness|hendrycksTest-sociology|5_2024-01-10T15-21-15.168348.parquet"]}, {"split": "latest", "path": ["**/details_harness|hendrycksTest-sociology|5_2024-01-10T15-21-15.168348.parquet"]}]}, {"config_name": "harness_hendrycksTest_us_foreign_policy_5", "data_files": [{"split": "2024_01_10T15_21_15.168348", "path": ["**/details_harness|hendrycksTest-us_foreign_policy|5_2024-01-10T15-21-15.168348.parquet"]}, {"split": "latest", "path": ["**/details_harness|hendrycksTest-us_foreign_policy|5_2024-01-10T15-21-15.168348.parquet"]}]}, {"config_name": "harness_hendrycksTest_virology_5", "data_files": [{"split": "2024_01_10T15_21_15.168348", "path": ["**/details_harness|hendrycksTest-virology|5_2024-01-10T15-21-15.168348.parquet"]}, {"split": "latest", "path": ["**/details_harness|hendrycksTest-virology|5_2024-01-10T15-21-15.168348.parquet"]}]}, {"config_name": "harness_hendrycksTest_world_religions_5", "data_files": [{"split": "2024_01_10T15_21_15.168348", "path": ["**/details_harness|hendrycksTest-world_religions|5_2024-01-10T15-21-15.168348.parquet"]}, {"split": "latest", "path": ["**/details_harness|hendrycksTest-world_religions|5_2024-01-10T15-21-15.168348.parquet"]}]}, {"config_name": "harness_truthfulqa_mc_0", "data_files": [{"split": "2024_01_10T15_21_15.168348", "path": ["**/details_harness|truthfulqa:mc|0_2024-01-10T15-21-15.168348.parquet"]}, {"split": "latest", "path": ["**/details_harness|truthfulqa:mc|0_2024-01-10T15-21-15.168348.parquet"]}]}, {"config_name": "harness_winogrande_5", "data_files": [{"split": "2024_01_10T15_21_15.168348", "path": ["**/details_harness|winogrande|5_2024-01-10T15-21-15.168348.parquet"]}, {"split": "latest", "path": ["**/details_harness|winogrande|5_2024-01-10T15-21-15.168348.parquet"]}]}, {"config_name": "results", "data_files": [{"split": "2024_01_10T15_21_15.168348", "path": ["results_2024-01-10T15-21-15.168348.parquet"]}, {"split": "latest", "path": ["results_2024-01-10T15-21-15.168348.parquet"]}]}]} | 2024-01-10T15:23:28+00:00 | [] | [] | TAGS
#region-us
|
# Dataset Card for Evaluation run of euclaise/crow-1b
Dataset automatically created during the evaluation run of model euclaise/crow-1b on the Open LLM Leaderboard.
The dataset is composed of 63 configuration, each one coresponding to one of the evaluated task.
The dataset has been created from 1 run(s). Each run can be found as a specific split in each configuration, the split being named using the timestamp of the run.The "train" split is always pointing to the latest results.
An additional configuration "results" store all the aggregated results of the run (and is used to compute and display the aggregated metrics on the Open LLM Leaderboard).
To load the details from a run, you can for instance do the following:
## Latest results
These are the latest results from run 2024-01-10T15:21:15.168348(note that their might be results for other tasks in the repos if successive evals didn't cover the same tasks. You find each in the results and the "latest" split for each eval):
## Dataset Details
### Dataset Description
- Curated by:
- Funded by [optional]:
- Shared by [optional]:
- Language(s) (NLP):
- License:
### Dataset Sources [optional]
- Repository:
- Paper [optional]:
- Demo [optional]:
## Uses
### Direct Use
### Out-of-Scope Use
## Dataset Structure
## Dataset Creation
### Curation Rationale
### Source Data
#### Data Collection and Processing
#### Who are the source data producers?
### Annotations [optional]
#### Annotation process
#### Who are the annotators?
#### Personal and Sensitive Information
## Bias, Risks, and Limitations
### Recommendations
Users should be made aware of the risks, biases and limitations of the dataset. More information needed for further recommendations.
[optional]
BibTeX:
APA:
## Glossary [optional]
## More Information [optional]
## Dataset Card Authors [optional]
## Dataset Card Contact
| [
"# Dataset Card for Evaluation run of euclaise/crow-1b\n\n\n\nDataset automatically created during the evaluation run of model euclaise/crow-1b on the Open LLM Leaderboard.\n\nThe dataset is composed of 63 configuration, each one coresponding to one of the evaluated task.\n\nThe dataset has been created from 1 run(s). Each run can be found as a specific split in each configuration, the split being named using the timestamp of the run.The \"train\" split is always pointing to the latest results.\n\nAn additional configuration \"results\" store all the aggregated results of the run (and is used to compute and display the aggregated metrics on the Open LLM Leaderboard).\n\nTo load the details from a run, you can for instance do the following:",
"## Latest results\n\nThese are the latest results from run 2024-01-10T15:21:15.168348(note that their might be results for other tasks in the repos if successive evals didn't cover the same tasks. You find each in the results and the \"latest\" split for each eval):",
"## Dataset Details",
"### Dataset Description\n\n\n\n\n\n- Curated by: \n- Funded by [optional]: \n- Shared by [optional]: \n- Language(s) (NLP): \n- License:",
"### Dataset Sources [optional]\n\n\n\n- Repository: \n- Paper [optional]: \n- Demo [optional]:",
"## Uses",
"### Direct Use",
"### Out-of-Scope Use",
"## Dataset Structure",
"## Dataset Creation",
"### Curation Rationale",
"### Source Data",
"#### Data Collection and Processing",
"#### Who are the source data producers?",
"### Annotations [optional]",
"#### Annotation process",
"#### Who are the annotators?",
"#### Personal and Sensitive Information",
"## Bias, Risks, and Limitations",
"### Recommendations\n\n\n\nUsers should be made aware of the risks, biases and limitations of the dataset. More information needed for further recommendations.\n\n[optional]\n\n\n\nBibTeX:\n\n\n\nAPA:",
"## Glossary [optional]",
"## More Information [optional]",
"## Dataset Card Authors [optional]",
"## Dataset Card Contact"
] | [
"TAGS\n#region-us \n",
"# Dataset Card for Evaluation run of euclaise/crow-1b\n\n\n\nDataset automatically created during the evaluation run of model euclaise/crow-1b on the Open LLM Leaderboard.\n\nThe dataset is composed of 63 configuration, each one coresponding to one of the evaluated task.\n\nThe dataset has been created from 1 run(s). Each run can be found as a specific split in each configuration, the split being named using the timestamp of the run.The \"train\" split is always pointing to the latest results.\n\nAn additional configuration \"results\" store all the aggregated results of the run (and is used to compute and display the aggregated metrics on the Open LLM Leaderboard).\n\nTo load the details from a run, you can for instance do the following:",
"## Latest results\n\nThese are the latest results from run 2024-01-10T15:21:15.168348(note that their might be results for other tasks in the repos if successive evals didn't cover the same tasks. You find each in the results and the \"latest\" split for each eval):",
"## Dataset Details",
"### Dataset Description\n\n\n\n\n\n- Curated by: \n- Funded by [optional]: \n- Shared by [optional]: \n- Language(s) (NLP): \n- License:",
"### Dataset Sources [optional]\n\n\n\n- Repository: \n- Paper [optional]: \n- Demo [optional]:",
"## Uses",
"### Direct Use",
"### Out-of-Scope Use",
"## Dataset Structure",
"## Dataset Creation",
"### Curation Rationale",
"### Source Data",
"#### Data Collection and Processing",
"#### Who are the source data producers?",
"### Annotations [optional]",
"#### Annotation process",
"#### Who are the annotators?",
"#### Personal and Sensitive Information",
"## Bias, Risks, and Limitations",
"### Recommendations\n\n\n\nUsers should be made aware of the risks, biases and limitations of the dataset. More information needed for further recommendations.\n\n[optional]\n\n\n\nBibTeX:\n\n\n\nAPA:",
"## Glossary [optional]",
"## More Information [optional]",
"## Dataset Card Authors [optional]",
"## Dataset Card Contact"
] |
72321460cc7dec7d40a56e9d704ebe5dd60c25f5 | # Dataset Card for "llava-pretrain"
[More Information needed](https://github.com/huggingface/datasets/blob/main/CONTRIBUTING.md#how-to-contribute-to-the-dataset-cards) | fxmeng/llava-pretrain | [
"region:us"
] | 2024-01-10T15:26:50+00:00 | {"dataset_info": {"features": [{"name": "id", "dtype": "string"}, {"name": "image", "dtype": "string"}, {"name": "input", "dtype": "string"}, {"name": "output", "dtype": "string"}], "splits": [{"name": "train", "num_bytes": 92854190, "num_examples": 558128}], "download_size": 36868547, "dataset_size": 92854190}, "configs": [{"config_name": "default", "data_files": [{"split": "train", "path": "data/train-*"}]}]} | 2024-01-10T15:41:55+00:00 | [] | [] | TAGS
#region-us
| # Dataset Card for "llava-pretrain"
More Information needed | [
"# Dataset Card for \"llava-pretrain\"\n\nMore Information needed"
] | [
"TAGS\n#region-us \n",
"# Dataset Card for \"llava-pretrain\"\n\nMore Information needed"
] |
bd13f3245a88ad19416fe1fdd3ad10628f2bb990 |
# Dataset Card for Evaluation run of shitshow123/tinylamma-20000
<!-- Provide a quick summary of the dataset. -->
Dataset automatically created during the evaluation run of model [shitshow123/tinylamma-20000](https://huggingface.co/shitshow123/tinylamma-20000) on the [Open LLM Leaderboard](https://huggingface.co/spaces/HuggingFaceH4/open_llm_leaderboard).
The dataset is composed of 63 configuration, each one coresponding to one of the evaluated task.
The dataset has been created from 1 run(s). Each run can be found as a specific split in each configuration, the split being named using the timestamp of the run.The "train" split is always pointing to the latest results.
An additional configuration "results" store all the aggregated results of the run (and is used to compute and display the aggregated metrics on the [Open LLM Leaderboard](https://huggingface.co/spaces/HuggingFaceH4/open_llm_leaderboard)).
To load the details from a run, you can for instance do the following:
```python
from datasets import load_dataset
data = load_dataset("open-llm-leaderboard/details_shitshow123__tinylamma-20000",
"harness_winogrande_5",
split="train")
```
## Latest results
These are the [latest results from run 2024-01-10T15:30:35.451394](https://huggingface.co/datasets/open-llm-leaderboard/details_shitshow123__tinylamma-20000/blob/main/results_2024-01-10T15-30-35.451394.json)(note that their might be results for other tasks in the repos if successive evals didn't cover the same tasks. You find each in the results and the "latest" split for each eval):
```python
{
"all": {
"acc": 0.25338604391770375,
"acc_stderr": 0.030713466916848734,
"acc_norm": 0.2546731267053568,
"acc_norm_stderr": 0.03153382715425773,
"mc1": 0.17258261933904528,
"mc1_stderr": 0.0132286573782371,
"mc2": 0.3487352293249913,
"mc2_stderr": 0.015350541017533394
},
"harness|arc:challenge|25": {
"acc": 0.19539249146757678,
"acc_stderr": 0.011586907189952911,
"acc_norm": 0.2380546075085324,
"acc_norm_stderr": 0.012445770028026205
},
"harness|hellaswag|10": {
"acc": 0.28579964150567616,
"acc_stderr": 0.004508710891053844,
"acc_norm": 0.3245369448317068,
"acc_norm_stderr": 0.0046724470468200024
},
"harness|hendrycksTest-abstract_algebra|5": {
"acc": 0.29,
"acc_stderr": 0.04560480215720683,
"acc_norm": 0.29,
"acc_norm_stderr": 0.04560480215720683
},
"harness|hendrycksTest-anatomy|5": {
"acc": 0.32592592592592595,
"acc_stderr": 0.040491220417025055,
"acc_norm": 0.32592592592592595,
"acc_norm_stderr": 0.040491220417025055
},
"harness|hendrycksTest-astronomy|5": {
"acc": 0.18421052631578946,
"acc_stderr": 0.0315469804508223,
"acc_norm": 0.18421052631578946,
"acc_norm_stderr": 0.0315469804508223
},
"harness|hendrycksTest-business_ethics|5": {
"acc": 0.22,
"acc_stderr": 0.04163331998932269,
"acc_norm": 0.22,
"acc_norm_stderr": 0.04163331998932269
},
"harness|hendrycksTest-clinical_knowledge|5": {
"acc": 0.22264150943396227,
"acc_stderr": 0.0256042334708991,
"acc_norm": 0.22264150943396227,
"acc_norm_stderr": 0.0256042334708991
},
"harness|hendrycksTest-college_biology|5": {
"acc": 0.2222222222222222,
"acc_stderr": 0.03476590104304134,
"acc_norm": 0.2222222222222222,
"acc_norm_stderr": 0.03476590104304134
},
"harness|hendrycksTest-college_chemistry|5": {
"acc": 0.21,
"acc_stderr": 0.04093601807403326,
"acc_norm": 0.21,
"acc_norm_stderr": 0.04093601807403326
},
"harness|hendrycksTest-college_computer_science|5": {
"acc": 0.21,
"acc_stderr": 0.040936018074033256,
"acc_norm": 0.21,
"acc_norm_stderr": 0.040936018074033256
},
"harness|hendrycksTest-college_mathematics|5": {
"acc": 0.21,
"acc_stderr": 0.040936018074033256,
"acc_norm": 0.21,
"acc_norm_stderr": 0.040936018074033256
},
"harness|hendrycksTest-college_medicine|5": {
"acc": 0.1907514450867052,
"acc_stderr": 0.029957851329869337,
"acc_norm": 0.1907514450867052,
"acc_norm_stderr": 0.029957851329869337
},
"harness|hendrycksTest-college_physics|5": {
"acc": 0.3333333333333333,
"acc_stderr": 0.04690650298201943,
"acc_norm": 0.3333333333333333,
"acc_norm_stderr": 0.04690650298201943
},
"harness|hendrycksTest-computer_security|5": {
"acc": 0.25,
"acc_stderr": 0.04351941398892446,
"acc_norm": 0.25,
"acc_norm_stderr": 0.04351941398892446
},
"harness|hendrycksTest-conceptual_physics|5": {
"acc": 0.3276595744680851,
"acc_stderr": 0.030683020843231008,
"acc_norm": 0.3276595744680851,
"acc_norm_stderr": 0.030683020843231008
},
"harness|hendrycksTest-econometrics|5": {
"acc": 0.24561403508771928,
"acc_stderr": 0.04049339297748141,
"acc_norm": 0.24561403508771928,
"acc_norm_stderr": 0.04049339297748141
},
"harness|hendrycksTest-electrical_engineering|5": {
"acc": 0.23448275862068965,
"acc_stderr": 0.035306258743465914,
"acc_norm": 0.23448275862068965,
"acc_norm_stderr": 0.035306258743465914
},
"harness|hendrycksTest-elementary_mathematics|5": {
"acc": 0.2566137566137566,
"acc_stderr": 0.022494510767503154,
"acc_norm": 0.2566137566137566,
"acc_norm_stderr": 0.022494510767503154
},
"harness|hendrycksTest-formal_logic|5": {
"acc": 0.1746031746031746,
"acc_stderr": 0.033954900208561116,
"acc_norm": 0.1746031746031746,
"acc_norm_stderr": 0.033954900208561116
},
"harness|hendrycksTest-global_facts|5": {
"acc": 0.31,
"acc_stderr": 0.04648231987117316,
"acc_norm": 0.31,
"acc_norm_stderr": 0.04648231987117316
},
"harness|hendrycksTest-high_school_biology|5": {
"acc": 0.3161290322580645,
"acc_stderr": 0.02645087448904277,
"acc_norm": 0.3161290322580645,
"acc_norm_stderr": 0.02645087448904277
},
"harness|hendrycksTest-high_school_chemistry|5": {
"acc": 0.2955665024630542,
"acc_stderr": 0.032104944337514575,
"acc_norm": 0.2955665024630542,
"acc_norm_stderr": 0.032104944337514575
},
"harness|hendrycksTest-high_school_computer_science|5": {
"acc": 0.28,
"acc_stderr": 0.045126085985421276,
"acc_norm": 0.28,
"acc_norm_stderr": 0.045126085985421276
},
"harness|hendrycksTest-high_school_european_history|5": {
"acc": 0.2606060606060606,
"acc_stderr": 0.034277431758165236,
"acc_norm": 0.2606060606060606,
"acc_norm_stderr": 0.034277431758165236
},
"harness|hendrycksTest-high_school_geography|5": {
"acc": 0.25757575757575757,
"acc_stderr": 0.031156269519646836,
"acc_norm": 0.25757575757575757,
"acc_norm_stderr": 0.031156269519646836
},
"harness|hendrycksTest-high_school_government_and_politics|5": {
"acc": 0.30569948186528495,
"acc_stderr": 0.03324837939758159,
"acc_norm": 0.30569948186528495,
"acc_norm_stderr": 0.03324837939758159
},
"harness|hendrycksTest-high_school_macroeconomics|5": {
"acc": 0.19743589743589743,
"acc_stderr": 0.02018264696867484,
"acc_norm": 0.19743589743589743,
"acc_norm_stderr": 0.02018264696867484
},
"harness|hendrycksTest-high_school_mathematics|5": {
"acc": 0.25925925925925924,
"acc_stderr": 0.026719240783712163,
"acc_norm": 0.25925925925925924,
"acc_norm_stderr": 0.026719240783712163
},
"harness|hendrycksTest-high_school_microeconomics|5": {
"acc": 0.20168067226890757,
"acc_stderr": 0.026064313406304527,
"acc_norm": 0.20168067226890757,
"acc_norm_stderr": 0.026064313406304527
},
"harness|hendrycksTest-high_school_physics|5": {
"acc": 0.1986754966887417,
"acc_stderr": 0.03257847384436775,
"acc_norm": 0.1986754966887417,
"acc_norm_stderr": 0.03257847384436775
},
"harness|hendrycksTest-high_school_psychology|5": {
"acc": 0.26605504587155965,
"acc_stderr": 0.018946022322225583,
"acc_norm": 0.26605504587155965,
"acc_norm_stderr": 0.018946022322225583
},
"harness|hendrycksTest-high_school_statistics|5": {
"acc": 0.48148148148148145,
"acc_stderr": 0.034076320938540516,
"acc_norm": 0.48148148148148145,
"acc_norm_stderr": 0.034076320938540516
},
"harness|hendrycksTest-high_school_us_history|5": {
"acc": 0.24019607843137256,
"acc_stderr": 0.02998373305591361,
"acc_norm": 0.24019607843137256,
"acc_norm_stderr": 0.02998373305591361
},
"harness|hendrycksTest-high_school_world_history|5": {
"acc": 0.2616033755274262,
"acc_stderr": 0.028609516716994934,
"acc_norm": 0.2616033755274262,
"acc_norm_stderr": 0.028609516716994934
},
"harness|hendrycksTest-human_aging|5": {
"acc": 0.26905829596412556,
"acc_stderr": 0.029763779406874975,
"acc_norm": 0.26905829596412556,
"acc_norm_stderr": 0.029763779406874975
},
"harness|hendrycksTest-human_sexuality|5": {
"acc": 0.22137404580152673,
"acc_stderr": 0.0364129708131373,
"acc_norm": 0.22137404580152673,
"acc_norm_stderr": 0.0364129708131373
},
"harness|hendrycksTest-international_law|5": {
"acc": 0.30578512396694213,
"acc_stderr": 0.04205953933884123,
"acc_norm": 0.30578512396694213,
"acc_norm_stderr": 0.04205953933884123
},
"harness|hendrycksTest-jurisprudence|5": {
"acc": 0.21296296296296297,
"acc_stderr": 0.0395783547198098,
"acc_norm": 0.21296296296296297,
"acc_norm_stderr": 0.0395783547198098
},
"harness|hendrycksTest-logical_fallacies|5": {
"acc": 0.294478527607362,
"acc_stderr": 0.03581165790474082,
"acc_norm": 0.294478527607362,
"acc_norm_stderr": 0.03581165790474082
},
"harness|hendrycksTest-machine_learning|5": {
"acc": 0.21428571428571427,
"acc_stderr": 0.03894641120044792,
"acc_norm": 0.21428571428571427,
"acc_norm_stderr": 0.03894641120044792
},
"harness|hendrycksTest-management|5": {
"acc": 0.20388349514563106,
"acc_stderr": 0.039891398595317706,
"acc_norm": 0.20388349514563106,
"acc_norm_stderr": 0.039891398595317706
},
"harness|hendrycksTest-marketing|5": {
"acc": 0.19658119658119658,
"acc_stderr": 0.02603538609895129,
"acc_norm": 0.19658119658119658,
"acc_norm_stderr": 0.02603538609895129
},
"harness|hendrycksTest-medical_genetics|5": {
"acc": 0.3,
"acc_stderr": 0.046056618647183814,
"acc_norm": 0.3,
"acc_norm_stderr": 0.046056618647183814
},
"harness|hendrycksTest-miscellaneous|5": {
"acc": 0.2669220945083014,
"acc_stderr": 0.015818450894777552,
"acc_norm": 0.2669220945083014,
"acc_norm_stderr": 0.015818450894777552
},
"harness|hendrycksTest-moral_disputes|5": {
"acc": 0.21965317919075145,
"acc_stderr": 0.022289638852617904,
"acc_norm": 0.21965317919075145,
"acc_norm_stderr": 0.022289638852617904
},
"harness|hendrycksTest-moral_scenarios|5": {
"acc": 0.2424581005586592,
"acc_stderr": 0.014333522059217889,
"acc_norm": 0.2424581005586592,
"acc_norm_stderr": 0.014333522059217889
},
"harness|hendrycksTest-nutrition|5": {
"acc": 0.2549019607843137,
"acc_stderr": 0.02495418432487991,
"acc_norm": 0.2549019607843137,
"acc_norm_stderr": 0.02495418432487991
},
"harness|hendrycksTest-philosophy|5": {
"acc": 0.2990353697749196,
"acc_stderr": 0.026003301117885135,
"acc_norm": 0.2990353697749196,
"acc_norm_stderr": 0.026003301117885135
},
"harness|hendrycksTest-prehistory|5": {
"acc": 0.2839506172839506,
"acc_stderr": 0.025089478523765137,
"acc_norm": 0.2839506172839506,
"acc_norm_stderr": 0.025089478523765137
},
"harness|hendrycksTest-professional_accounting|5": {
"acc": 0.25886524822695034,
"acc_stderr": 0.026129572527180844,
"acc_norm": 0.25886524822695034,
"acc_norm_stderr": 0.026129572527180844
},
"harness|hendrycksTest-professional_law|5": {
"acc": 0.2438070404172099,
"acc_stderr": 0.01096650797217848,
"acc_norm": 0.2438070404172099,
"acc_norm_stderr": 0.01096650797217848
},
"harness|hendrycksTest-professional_medicine|5": {
"acc": 0.23897058823529413,
"acc_stderr": 0.025905280644893006,
"acc_norm": 0.23897058823529413,
"acc_norm_stderr": 0.025905280644893006
},
"harness|hendrycksTest-professional_psychology|5": {
"acc": 0.23366013071895425,
"acc_stderr": 0.017119158496044503,
"acc_norm": 0.23366013071895425,
"acc_norm_stderr": 0.017119158496044503
},
"harness|hendrycksTest-public_relations|5": {
"acc": 0.22727272727272727,
"acc_stderr": 0.040139645540727735,
"acc_norm": 0.22727272727272727,
"acc_norm_stderr": 0.040139645540727735
},
"harness|hendrycksTest-security_studies|5": {
"acc": 0.24489795918367346,
"acc_stderr": 0.027529637440174934,
"acc_norm": 0.24489795918367346,
"acc_norm_stderr": 0.027529637440174934
},
"harness|hendrycksTest-sociology|5": {
"acc": 0.23880597014925373,
"acc_stderr": 0.03014777593540922,
"acc_norm": 0.23880597014925373,
"acc_norm_stderr": 0.03014777593540922
},
"harness|hendrycksTest-us_foreign_policy|5": {
"acc": 0.3,
"acc_stderr": 0.046056618647183814,
"acc_norm": 0.3,
"acc_norm_stderr": 0.046056618647183814
},
"harness|hendrycksTest-virology|5": {
"acc": 0.24096385542168675,
"acc_stderr": 0.03329394119073529,
"acc_norm": 0.24096385542168675,
"acc_norm_stderr": 0.03329394119073529
},
"harness|hendrycksTest-world_religions|5": {
"acc": 0.21052631578947367,
"acc_stderr": 0.0312678171466318,
"acc_norm": 0.21052631578947367,
"acc_norm_stderr": 0.0312678171466318
},
"harness|truthfulqa:mc|0": {
"mc1": 0.17258261933904528,
"mc1_stderr": 0.0132286573782371,
"mc2": 0.3487352293249913,
"mc2_stderr": 0.015350541017533394
},
"harness|winogrande|5": {
"acc": 0.5122336227308603,
"acc_stderr": 0.01404827882040562
},
"harness|gsm8k|5": {
"acc": 0.0,
"acc_stderr": 0.0
}
}
```
## Dataset Details
### Dataset Description
<!-- Provide a longer summary of what this dataset is. -->
- **Curated by:** [More Information Needed]
- **Funded by [optional]:** [More Information Needed]
- **Shared by [optional]:** [More Information Needed]
- **Language(s) (NLP):** [More Information Needed]
- **License:** [More Information Needed]
### Dataset Sources [optional]
<!-- Provide the basic links for the dataset. -->
- **Repository:** [More Information Needed]
- **Paper [optional]:** [More Information Needed]
- **Demo [optional]:** [More Information Needed]
## Uses
<!-- Address questions around how the dataset is intended to be used. -->
### Direct Use
<!-- This section describes suitable use cases for the dataset. -->
[More Information Needed]
### Out-of-Scope Use
<!-- This section addresses misuse, malicious use, and uses that the dataset will not work well for. -->
[More Information Needed]
## Dataset Structure
<!-- This section provides a description of the dataset fields, and additional information about the dataset structure such as criteria used to create the splits, relationships between data points, etc. -->
[More Information Needed]
## Dataset Creation
### Curation Rationale
<!-- Motivation for the creation of this dataset. -->
[More Information Needed]
### Source Data
<!-- This section describes the source data (e.g. news text and headlines, social media posts, translated sentences, ...). -->
#### Data Collection and Processing
<!-- This section describes the data collection and processing process such as data selection criteria, filtering and normalization methods, tools and libraries used, etc. -->
[More Information Needed]
#### Who are the source data producers?
<!-- This section describes the people or systems who originally created the data. It should also include self-reported demographic or identity information for the source data creators if this information is available. -->
[More Information Needed]
### Annotations [optional]
<!-- If the dataset contains annotations which are not part of the initial data collection, use this section to describe them. -->
#### Annotation process
<!-- This section describes the annotation process such as annotation tools used in the process, the amount of data annotated, annotation guidelines provided to the annotators, interannotator statistics, annotation validation, etc. -->
[More Information Needed]
#### Who are the annotators?
<!-- This section describes the people or systems who created the annotations. -->
[More Information Needed]
#### Personal and Sensitive Information
<!-- State whether the dataset contains data that might be considered personal, sensitive, or private (e.g., data that reveals addresses, uniquely identifiable names or aliases, racial or ethnic origins, sexual orientations, religious beliefs, political opinions, financial or health data, etc.). If efforts were made to anonymize the data, describe the anonymization process. -->
[More Information Needed]
## Bias, Risks, and Limitations
<!-- This section is meant to convey both technical and sociotechnical limitations. -->
[More Information Needed]
### Recommendations
<!-- This section is meant to convey recommendations with respect to the bias, risk, and technical limitations. -->
Users should be made aware of the risks, biases and limitations of the dataset. More information needed for further recommendations.
## Citation [optional]
<!-- If there is a paper or blog post introducing the dataset, the APA and Bibtex information for that should go in this section. -->
**BibTeX:**
[More Information Needed]
**APA:**
[More Information Needed]
## Glossary [optional]
<!-- If relevant, include terms and calculations in this section that can help readers understand the dataset or dataset card. -->
[More Information Needed]
## More Information [optional]
[More Information Needed]
## Dataset Card Authors [optional]
[More Information Needed]
## Dataset Card Contact
[More Information Needed] | open-llm-leaderboard/details_shitshow123__tinylamma-20000 | [
"region:us"
] | 2024-01-10T15:32:25+00:00 | {"pretty_name": "Evaluation run of shitshow123/tinylamma-20000", "dataset_summary": "Dataset automatically created during the evaluation run of model [shitshow123/tinylamma-20000](https://huggingface.co/shitshow123/tinylamma-20000) on the [Open LLM Leaderboard](https://huggingface.co/spaces/HuggingFaceH4/open_llm_leaderboard).\n\nThe dataset is composed of 63 configuration, each one coresponding to one of the evaluated task.\n\nThe dataset has been created from 1 run(s). Each run can be found as a specific split in each configuration, the split being named using the timestamp of the run.The \"train\" split is always pointing to the latest results.\n\nAn additional configuration \"results\" store all the aggregated results of the run (and is used to compute and display the aggregated metrics on the [Open LLM Leaderboard](https://huggingface.co/spaces/HuggingFaceH4/open_llm_leaderboard)).\n\nTo load the details from a run, you can for instance do the following:\n```python\nfrom datasets import load_dataset\ndata = load_dataset(\"open-llm-leaderboard/details_shitshow123__tinylamma-20000\",\n\t\"harness_winogrande_5\",\n\tsplit=\"train\")\n```\n\n## Latest results\n\nThese are the [latest results from run 2024-01-10T15:30:35.451394](https://huggingface.co/datasets/open-llm-leaderboard/details_shitshow123__tinylamma-20000/blob/main/results_2024-01-10T15-30-35.451394.json)(note that their might be results for other tasks in the repos if successive evals didn't cover the same tasks. You find each in the results and the \"latest\" split for each eval):\n\n```python\n{\n \"all\": {\n \"acc\": 0.25338604391770375,\n \"acc_stderr\": 0.030713466916848734,\n \"acc_norm\": 0.2546731267053568,\n \"acc_norm_stderr\": 0.03153382715425773,\n \"mc1\": 0.17258261933904528,\n \"mc1_stderr\": 0.0132286573782371,\n \"mc2\": 0.3487352293249913,\n \"mc2_stderr\": 0.015350541017533394\n },\n \"harness|arc:challenge|25\": {\n \"acc\": 0.19539249146757678,\n \"acc_stderr\": 0.011586907189952911,\n \"acc_norm\": 0.2380546075085324,\n \"acc_norm_stderr\": 0.012445770028026205\n },\n \"harness|hellaswag|10\": {\n \"acc\": 0.28579964150567616,\n \"acc_stderr\": 0.004508710891053844,\n \"acc_norm\": 0.3245369448317068,\n \"acc_norm_stderr\": 0.0046724470468200024\n },\n \"harness|hendrycksTest-abstract_algebra|5\": {\n \"acc\": 0.29,\n \"acc_stderr\": 0.04560480215720683,\n \"acc_norm\": 0.29,\n \"acc_norm_stderr\": 0.04560480215720683\n },\n \"harness|hendrycksTest-anatomy|5\": {\n \"acc\": 0.32592592592592595,\n \"acc_stderr\": 0.040491220417025055,\n \"acc_norm\": 0.32592592592592595,\n \"acc_norm_stderr\": 0.040491220417025055\n },\n \"harness|hendrycksTest-astronomy|5\": {\n \"acc\": 0.18421052631578946,\n \"acc_stderr\": 0.0315469804508223,\n \"acc_norm\": 0.18421052631578946,\n \"acc_norm_stderr\": 0.0315469804508223\n },\n \"harness|hendrycksTest-business_ethics|5\": {\n \"acc\": 0.22,\n \"acc_stderr\": 0.04163331998932269,\n \"acc_norm\": 0.22,\n \"acc_norm_stderr\": 0.04163331998932269\n },\n \"harness|hendrycksTest-clinical_knowledge|5\": {\n \"acc\": 0.22264150943396227,\n \"acc_stderr\": 0.0256042334708991,\n \"acc_norm\": 0.22264150943396227,\n \"acc_norm_stderr\": 0.0256042334708991\n },\n \"harness|hendrycksTest-college_biology|5\": {\n \"acc\": 0.2222222222222222,\n \"acc_stderr\": 0.03476590104304134,\n \"acc_norm\": 0.2222222222222222,\n \"acc_norm_stderr\": 0.03476590104304134\n },\n \"harness|hendrycksTest-college_chemistry|5\": {\n \"acc\": 0.21,\n \"acc_stderr\": 0.04093601807403326,\n \"acc_norm\": 0.21,\n \"acc_norm_stderr\": 0.04093601807403326\n },\n \"harness|hendrycksTest-college_computer_science|5\": {\n \"acc\": 0.21,\n \"acc_stderr\": 0.040936018074033256,\n \"acc_norm\": 0.21,\n \"acc_norm_stderr\": 0.040936018074033256\n },\n \"harness|hendrycksTest-college_mathematics|5\": {\n \"acc\": 0.21,\n \"acc_stderr\": 0.040936018074033256,\n \"acc_norm\": 0.21,\n \"acc_norm_stderr\": 0.040936018074033256\n },\n \"harness|hendrycksTest-college_medicine|5\": {\n \"acc\": 0.1907514450867052,\n \"acc_stderr\": 0.029957851329869337,\n \"acc_norm\": 0.1907514450867052,\n \"acc_norm_stderr\": 0.029957851329869337\n },\n \"harness|hendrycksTest-college_physics|5\": {\n \"acc\": 0.3333333333333333,\n \"acc_stderr\": 0.04690650298201943,\n \"acc_norm\": 0.3333333333333333,\n \"acc_norm_stderr\": 0.04690650298201943\n },\n \"harness|hendrycksTest-computer_security|5\": {\n \"acc\": 0.25,\n \"acc_stderr\": 0.04351941398892446,\n \"acc_norm\": 0.25,\n \"acc_norm_stderr\": 0.04351941398892446\n },\n \"harness|hendrycksTest-conceptual_physics|5\": {\n \"acc\": 0.3276595744680851,\n \"acc_stderr\": 0.030683020843231008,\n \"acc_norm\": 0.3276595744680851,\n \"acc_norm_stderr\": 0.030683020843231008\n },\n \"harness|hendrycksTest-econometrics|5\": {\n \"acc\": 0.24561403508771928,\n \"acc_stderr\": 0.04049339297748141,\n \"acc_norm\": 0.24561403508771928,\n \"acc_norm_stderr\": 0.04049339297748141\n },\n \"harness|hendrycksTest-electrical_engineering|5\": {\n \"acc\": 0.23448275862068965,\n \"acc_stderr\": 0.035306258743465914,\n \"acc_norm\": 0.23448275862068965,\n \"acc_norm_stderr\": 0.035306258743465914\n },\n \"harness|hendrycksTest-elementary_mathematics|5\": {\n \"acc\": 0.2566137566137566,\n \"acc_stderr\": 0.022494510767503154,\n \"acc_norm\": 0.2566137566137566,\n \"acc_norm_stderr\": 0.022494510767503154\n },\n \"harness|hendrycksTest-formal_logic|5\": {\n \"acc\": 0.1746031746031746,\n \"acc_stderr\": 0.033954900208561116,\n \"acc_norm\": 0.1746031746031746,\n \"acc_norm_stderr\": 0.033954900208561116\n },\n \"harness|hendrycksTest-global_facts|5\": {\n \"acc\": 0.31,\n \"acc_stderr\": 0.04648231987117316,\n \"acc_norm\": 0.31,\n \"acc_norm_stderr\": 0.04648231987117316\n },\n \"harness|hendrycksTest-high_school_biology|5\": {\n \"acc\": 0.3161290322580645,\n \"acc_stderr\": 0.02645087448904277,\n \"acc_norm\": 0.3161290322580645,\n \"acc_norm_stderr\": 0.02645087448904277\n },\n \"harness|hendrycksTest-high_school_chemistry|5\": {\n \"acc\": 0.2955665024630542,\n \"acc_stderr\": 0.032104944337514575,\n \"acc_norm\": 0.2955665024630542,\n \"acc_norm_stderr\": 0.032104944337514575\n },\n \"harness|hendrycksTest-high_school_computer_science|5\": {\n \"acc\": 0.28,\n \"acc_stderr\": 0.045126085985421276,\n \"acc_norm\": 0.28,\n \"acc_norm_stderr\": 0.045126085985421276\n },\n \"harness|hendrycksTest-high_school_european_history|5\": {\n \"acc\": 0.2606060606060606,\n \"acc_stderr\": 0.034277431758165236,\n \"acc_norm\": 0.2606060606060606,\n \"acc_norm_stderr\": 0.034277431758165236\n },\n \"harness|hendrycksTest-high_school_geography|5\": {\n \"acc\": 0.25757575757575757,\n \"acc_stderr\": 0.031156269519646836,\n \"acc_norm\": 0.25757575757575757,\n \"acc_norm_stderr\": 0.031156269519646836\n },\n \"harness|hendrycksTest-high_school_government_and_politics|5\": {\n \"acc\": 0.30569948186528495,\n \"acc_stderr\": 0.03324837939758159,\n \"acc_norm\": 0.30569948186528495,\n \"acc_norm_stderr\": 0.03324837939758159\n },\n \"harness|hendrycksTest-high_school_macroeconomics|5\": {\n \"acc\": 0.19743589743589743,\n \"acc_stderr\": 0.02018264696867484,\n \"acc_norm\": 0.19743589743589743,\n \"acc_norm_stderr\": 0.02018264696867484\n },\n \"harness|hendrycksTest-high_school_mathematics|5\": {\n \"acc\": 0.25925925925925924,\n \"acc_stderr\": 0.026719240783712163,\n \"acc_norm\": 0.25925925925925924,\n \"acc_norm_stderr\": 0.026719240783712163\n },\n \"harness|hendrycksTest-high_school_microeconomics|5\": {\n \"acc\": 0.20168067226890757,\n \"acc_stderr\": 0.026064313406304527,\n \"acc_norm\": 0.20168067226890757,\n \"acc_norm_stderr\": 0.026064313406304527\n },\n \"harness|hendrycksTest-high_school_physics|5\": {\n \"acc\": 0.1986754966887417,\n \"acc_stderr\": 0.03257847384436775,\n \"acc_norm\": 0.1986754966887417,\n \"acc_norm_stderr\": 0.03257847384436775\n },\n \"harness|hendrycksTest-high_school_psychology|5\": {\n \"acc\": 0.26605504587155965,\n \"acc_stderr\": 0.018946022322225583,\n \"acc_norm\": 0.26605504587155965,\n \"acc_norm_stderr\": 0.018946022322225583\n },\n \"harness|hendrycksTest-high_school_statistics|5\": {\n \"acc\": 0.48148148148148145,\n \"acc_stderr\": 0.034076320938540516,\n \"acc_norm\": 0.48148148148148145,\n \"acc_norm_stderr\": 0.034076320938540516\n },\n \"harness|hendrycksTest-high_school_us_history|5\": {\n \"acc\": 0.24019607843137256,\n \"acc_stderr\": 0.02998373305591361,\n \"acc_norm\": 0.24019607843137256,\n \"acc_norm_stderr\": 0.02998373305591361\n },\n \"harness|hendrycksTest-high_school_world_history|5\": {\n \"acc\": 0.2616033755274262,\n \"acc_stderr\": 0.028609516716994934,\n \"acc_norm\": 0.2616033755274262,\n \"acc_norm_stderr\": 0.028609516716994934\n },\n \"harness|hendrycksTest-human_aging|5\": {\n \"acc\": 0.26905829596412556,\n \"acc_stderr\": 0.029763779406874975,\n \"acc_norm\": 0.26905829596412556,\n \"acc_norm_stderr\": 0.029763779406874975\n },\n \"harness|hendrycksTest-human_sexuality|5\": {\n \"acc\": 0.22137404580152673,\n \"acc_stderr\": 0.0364129708131373,\n \"acc_norm\": 0.22137404580152673,\n \"acc_norm_stderr\": 0.0364129708131373\n },\n \"harness|hendrycksTest-international_law|5\": {\n \"acc\": 0.30578512396694213,\n \"acc_stderr\": 0.04205953933884123,\n \"acc_norm\": 0.30578512396694213,\n \"acc_norm_stderr\": 0.04205953933884123\n },\n \"harness|hendrycksTest-jurisprudence|5\": {\n \"acc\": 0.21296296296296297,\n \"acc_stderr\": 0.0395783547198098,\n \"acc_norm\": 0.21296296296296297,\n \"acc_norm_stderr\": 0.0395783547198098\n },\n \"harness|hendrycksTest-logical_fallacies|5\": {\n \"acc\": 0.294478527607362,\n \"acc_stderr\": 0.03581165790474082,\n \"acc_norm\": 0.294478527607362,\n \"acc_norm_stderr\": 0.03581165790474082\n },\n \"harness|hendrycksTest-machine_learning|5\": {\n \"acc\": 0.21428571428571427,\n \"acc_stderr\": 0.03894641120044792,\n \"acc_norm\": 0.21428571428571427,\n \"acc_norm_stderr\": 0.03894641120044792\n },\n \"harness|hendrycksTest-management|5\": {\n \"acc\": 0.20388349514563106,\n \"acc_stderr\": 0.039891398595317706,\n \"acc_norm\": 0.20388349514563106,\n \"acc_norm_stderr\": 0.039891398595317706\n },\n \"harness|hendrycksTest-marketing|5\": {\n \"acc\": 0.19658119658119658,\n \"acc_stderr\": 0.02603538609895129,\n \"acc_norm\": 0.19658119658119658,\n \"acc_norm_stderr\": 0.02603538609895129\n },\n \"harness|hendrycksTest-medical_genetics|5\": {\n \"acc\": 0.3,\n \"acc_stderr\": 0.046056618647183814,\n \"acc_norm\": 0.3,\n \"acc_norm_stderr\": 0.046056618647183814\n },\n \"harness|hendrycksTest-miscellaneous|5\": {\n \"acc\": 0.2669220945083014,\n \"acc_stderr\": 0.015818450894777552,\n \"acc_norm\": 0.2669220945083014,\n \"acc_norm_stderr\": 0.015818450894777552\n },\n \"harness|hendrycksTest-moral_disputes|5\": {\n \"acc\": 0.21965317919075145,\n \"acc_stderr\": 0.022289638852617904,\n \"acc_norm\": 0.21965317919075145,\n \"acc_norm_stderr\": 0.022289638852617904\n },\n \"harness|hendrycksTest-moral_scenarios|5\": {\n \"acc\": 0.2424581005586592,\n \"acc_stderr\": 0.014333522059217889,\n \"acc_norm\": 0.2424581005586592,\n \"acc_norm_stderr\": 0.014333522059217889\n },\n \"harness|hendrycksTest-nutrition|5\": {\n \"acc\": 0.2549019607843137,\n \"acc_stderr\": 0.02495418432487991,\n \"acc_norm\": 0.2549019607843137,\n \"acc_norm_stderr\": 0.02495418432487991\n },\n \"harness|hendrycksTest-philosophy|5\": {\n \"acc\": 0.2990353697749196,\n \"acc_stderr\": 0.026003301117885135,\n \"acc_norm\": 0.2990353697749196,\n \"acc_norm_stderr\": 0.026003301117885135\n },\n \"harness|hendrycksTest-prehistory|5\": {\n \"acc\": 0.2839506172839506,\n \"acc_stderr\": 0.025089478523765137,\n \"acc_norm\": 0.2839506172839506,\n \"acc_norm_stderr\": 0.025089478523765137\n },\n \"harness|hendrycksTest-professional_accounting|5\": {\n \"acc\": 0.25886524822695034,\n \"acc_stderr\": 0.026129572527180844,\n \"acc_norm\": 0.25886524822695034,\n \"acc_norm_stderr\": 0.026129572527180844\n },\n \"harness|hendrycksTest-professional_law|5\": {\n \"acc\": 0.2438070404172099,\n \"acc_stderr\": 0.01096650797217848,\n \"acc_norm\": 0.2438070404172099,\n \"acc_norm_stderr\": 0.01096650797217848\n },\n \"harness|hendrycksTest-professional_medicine|5\": {\n \"acc\": 0.23897058823529413,\n \"acc_stderr\": 0.025905280644893006,\n \"acc_norm\": 0.23897058823529413,\n \"acc_norm_stderr\": 0.025905280644893006\n },\n \"harness|hendrycksTest-professional_psychology|5\": {\n \"acc\": 0.23366013071895425,\n \"acc_stderr\": 0.017119158496044503,\n \"acc_norm\": 0.23366013071895425,\n \"acc_norm_stderr\": 0.017119158496044503\n },\n \"harness|hendrycksTest-public_relations|5\": {\n \"acc\": 0.22727272727272727,\n \"acc_stderr\": 0.040139645540727735,\n \"acc_norm\": 0.22727272727272727,\n \"acc_norm_stderr\": 0.040139645540727735\n },\n \"harness|hendrycksTest-security_studies|5\": {\n \"acc\": 0.24489795918367346,\n \"acc_stderr\": 0.027529637440174934,\n \"acc_norm\": 0.24489795918367346,\n \"acc_norm_stderr\": 0.027529637440174934\n },\n \"harness|hendrycksTest-sociology|5\": {\n \"acc\": 0.23880597014925373,\n \"acc_stderr\": 0.03014777593540922,\n \"acc_norm\": 0.23880597014925373,\n \"acc_norm_stderr\": 0.03014777593540922\n },\n \"harness|hendrycksTest-us_foreign_policy|5\": {\n \"acc\": 0.3,\n \"acc_stderr\": 0.046056618647183814,\n \"acc_norm\": 0.3,\n \"acc_norm_stderr\": 0.046056618647183814\n },\n \"harness|hendrycksTest-virology|5\": {\n \"acc\": 0.24096385542168675,\n \"acc_stderr\": 0.03329394119073529,\n \"acc_norm\": 0.24096385542168675,\n \"acc_norm_stderr\": 0.03329394119073529\n },\n \"harness|hendrycksTest-world_religions|5\": {\n \"acc\": 0.21052631578947367,\n \"acc_stderr\": 0.0312678171466318,\n \"acc_norm\": 0.21052631578947367,\n \"acc_norm_stderr\": 0.0312678171466318\n },\n \"harness|truthfulqa:mc|0\": {\n \"mc1\": 0.17258261933904528,\n \"mc1_stderr\": 0.0132286573782371,\n \"mc2\": 0.3487352293249913,\n \"mc2_stderr\": 0.015350541017533394\n },\n \"harness|winogrande|5\": {\n \"acc\": 0.5122336227308603,\n \"acc_stderr\": 0.01404827882040562\n },\n \"harness|gsm8k|5\": {\n \"acc\": 0.0,\n \"acc_stderr\": 0.0\n }\n}\n```", "repo_url": "https://huggingface.co/shitshow123/tinylamma-20000", "leaderboard_url": "https://huggingface.co/spaces/HuggingFaceH4/open_llm_leaderboard", "point_of_contact": "[email protected]", "configs": [{"config_name": "harness_arc_challenge_25", "data_files": [{"split": "2024_01_10T15_30_35.451394", "path": ["**/details_harness|arc:challenge|25_2024-01-10T15-30-35.451394.parquet"]}, {"split": "latest", "path": ["**/details_harness|arc:challenge|25_2024-01-10T15-30-35.451394.parquet"]}]}, {"config_name": "harness_gsm8k_5", "data_files": [{"split": "2024_01_10T15_30_35.451394", "path": ["**/details_harness|gsm8k|5_2024-01-10T15-30-35.451394.parquet"]}, {"split": "latest", "path": ["**/details_harness|gsm8k|5_2024-01-10T15-30-35.451394.parquet"]}]}, {"config_name": "harness_hellaswag_10", "data_files": [{"split": "2024_01_10T15_30_35.451394", "path": ["**/details_harness|hellaswag|10_2024-01-10T15-30-35.451394.parquet"]}, {"split": "latest", "path": ["**/details_harness|hellaswag|10_2024-01-10T15-30-35.451394.parquet"]}]}, {"config_name": "harness_hendrycksTest_5", "data_files": [{"split": "2024_01_10T15_30_35.451394", "path": ["**/details_harness|hendrycksTest-abstract_algebra|5_2024-01-10T15-30-35.451394.parquet", "**/details_harness|hendrycksTest-anatomy|5_2024-01-10T15-30-35.451394.parquet", "**/details_harness|hendrycksTest-astronomy|5_2024-01-10T15-30-35.451394.parquet", "**/details_harness|hendrycksTest-business_ethics|5_2024-01-10T15-30-35.451394.parquet", "**/details_harness|hendrycksTest-clinical_knowledge|5_2024-01-10T15-30-35.451394.parquet", "**/details_harness|hendrycksTest-college_biology|5_2024-01-10T15-30-35.451394.parquet", "**/details_harness|hendrycksTest-college_chemistry|5_2024-01-10T15-30-35.451394.parquet", "**/details_harness|hendrycksTest-college_computer_science|5_2024-01-10T15-30-35.451394.parquet", "**/details_harness|hendrycksTest-college_mathematics|5_2024-01-10T15-30-35.451394.parquet", "**/details_harness|hendrycksTest-college_medicine|5_2024-01-10T15-30-35.451394.parquet", "**/details_harness|hendrycksTest-college_physics|5_2024-01-10T15-30-35.451394.parquet", "**/details_harness|hendrycksTest-computer_security|5_2024-01-10T15-30-35.451394.parquet", "**/details_harness|hendrycksTest-conceptual_physics|5_2024-01-10T15-30-35.451394.parquet", "**/details_harness|hendrycksTest-econometrics|5_2024-01-10T15-30-35.451394.parquet", "**/details_harness|hendrycksTest-electrical_engineering|5_2024-01-10T15-30-35.451394.parquet", "**/details_harness|hendrycksTest-elementary_mathematics|5_2024-01-10T15-30-35.451394.parquet", "**/details_harness|hendrycksTest-formal_logic|5_2024-01-10T15-30-35.451394.parquet", "**/details_harness|hendrycksTest-global_facts|5_2024-01-10T15-30-35.451394.parquet", "**/details_harness|hendrycksTest-high_school_biology|5_2024-01-10T15-30-35.451394.parquet", "**/details_harness|hendrycksTest-high_school_chemistry|5_2024-01-10T15-30-35.451394.parquet", "**/details_harness|hendrycksTest-high_school_computer_science|5_2024-01-10T15-30-35.451394.parquet", "**/details_harness|hendrycksTest-high_school_european_history|5_2024-01-10T15-30-35.451394.parquet", "**/details_harness|hendrycksTest-high_school_geography|5_2024-01-10T15-30-35.451394.parquet", "**/details_harness|hendrycksTest-high_school_government_and_politics|5_2024-01-10T15-30-35.451394.parquet", "**/details_harness|hendrycksTest-high_school_macroeconomics|5_2024-01-10T15-30-35.451394.parquet", "**/details_harness|hendrycksTest-high_school_mathematics|5_2024-01-10T15-30-35.451394.parquet", "**/details_harness|hendrycksTest-high_school_microeconomics|5_2024-01-10T15-30-35.451394.parquet", "**/details_harness|hendrycksTest-high_school_physics|5_2024-01-10T15-30-35.451394.parquet", "**/details_harness|hendrycksTest-high_school_psychology|5_2024-01-10T15-30-35.451394.parquet", "**/details_harness|hendrycksTest-high_school_statistics|5_2024-01-10T15-30-35.451394.parquet", "**/details_harness|hendrycksTest-high_school_us_history|5_2024-01-10T15-30-35.451394.parquet", "**/details_harness|hendrycksTest-high_school_world_history|5_2024-01-10T15-30-35.451394.parquet", "**/details_harness|hendrycksTest-human_aging|5_2024-01-10T15-30-35.451394.parquet", "**/details_harness|hendrycksTest-human_sexuality|5_2024-01-10T15-30-35.451394.parquet", "**/details_harness|hendrycksTest-international_law|5_2024-01-10T15-30-35.451394.parquet", "**/details_harness|hendrycksTest-jurisprudence|5_2024-01-10T15-30-35.451394.parquet", "**/details_harness|hendrycksTest-logical_fallacies|5_2024-01-10T15-30-35.451394.parquet", "**/details_harness|hendrycksTest-machine_learning|5_2024-01-10T15-30-35.451394.parquet", "**/details_harness|hendrycksTest-management|5_2024-01-10T15-30-35.451394.parquet", "**/details_harness|hendrycksTest-marketing|5_2024-01-10T15-30-35.451394.parquet", "**/details_harness|hendrycksTest-medical_genetics|5_2024-01-10T15-30-35.451394.parquet", "**/details_harness|hendrycksTest-miscellaneous|5_2024-01-10T15-30-35.451394.parquet", "**/details_harness|hendrycksTest-moral_disputes|5_2024-01-10T15-30-35.451394.parquet", "**/details_harness|hendrycksTest-moral_scenarios|5_2024-01-10T15-30-35.451394.parquet", "**/details_harness|hendrycksTest-nutrition|5_2024-01-10T15-30-35.451394.parquet", "**/details_harness|hendrycksTest-philosophy|5_2024-01-10T15-30-35.451394.parquet", "**/details_harness|hendrycksTest-prehistory|5_2024-01-10T15-30-35.451394.parquet", "**/details_harness|hendrycksTest-professional_accounting|5_2024-01-10T15-30-35.451394.parquet", "**/details_harness|hendrycksTest-professional_law|5_2024-01-10T15-30-35.451394.parquet", "**/details_harness|hendrycksTest-professional_medicine|5_2024-01-10T15-30-35.451394.parquet", "**/details_harness|hendrycksTest-professional_psychology|5_2024-01-10T15-30-35.451394.parquet", "**/details_harness|hendrycksTest-public_relations|5_2024-01-10T15-30-35.451394.parquet", "**/details_harness|hendrycksTest-security_studies|5_2024-01-10T15-30-35.451394.parquet", "**/details_harness|hendrycksTest-sociology|5_2024-01-10T15-30-35.451394.parquet", "**/details_harness|hendrycksTest-us_foreign_policy|5_2024-01-10T15-30-35.451394.parquet", "**/details_harness|hendrycksTest-virology|5_2024-01-10T15-30-35.451394.parquet", "**/details_harness|hendrycksTest-world_religions|5_2024-01-10T15-30-35.451394.parquet"]}, {"split": "latest", "path": ["**/details_harness|hendrycksTest-abstract_algebra|5_2024-01-10T15-30-35.451394.parquet", "**/details_harness|hendrycksTest-anatomy|5_2024-01-10T15-30-35.451394.parquet", "**/details_harness|hendrycksTest-astronomy|5_2024-01-10T15-30-35.451394.parquet", "**/details_harness|hendrycksTest-business_ethics|5_2024-01-10T15-30-35.451394.parquet", "**/details_harness|hendrycksTest-clinical_knowledge|5_2024-01-10T15-30-35.451394.parquet", "**/details_harness|hendrycksTest-college_biology|5_2024-01-10T15-30-35.451394.parquet", "**/details_harness|hendrycksTest-college_chemistry|5_2024-01-10T15-30-35.451394.parquet", "**/details_harness|hendrycksTest-college_computer_science|5_2024-01-10T15-30-35.451394.parquet", "**/details_harness|hendrycksTest-college_mathematics|5_2024-01-10T15-30-35.451394.parquet", "**/details_harness|hendrycksTest-college_medicine|5_2024-01-10T15-30-35.451394.parquet", "**/details_harness|hendrycksTest-college_physics|5_2024-01-10T15-30-35.451394.parquet", "**/details_harness|hendrycksTest-computer_security|5_2024-01-10T15-30-35.451394.parquet", "**/details_harness|hendrycksTest-conceptual_physics|5_2024-01-10T15-30-35.451394.parquet", "**/details_harness|hendrycksTest-econometrics|5_2024-01-10T15-30-35.451394.parquet", "**/details_harness|hendrycksTest-electrical_engineering|5_2024-01-10T15-30-35.451394.parquet", "**/details_harness|hendrycksTest-elementary_mathematics|5_2024-01-10T15-30-35.451394.parquet", "**/details_harness|hendrycksTest-formal_logic|5_2024-01-10T15-30-35.451394.parquet", "**/details_harness|hendrycksTest-global_facts|5_2024-01-10T15-30-35.451394.parquet", "**/details_harness|hendrycksTest-high_school_biology|5_2024-01-10T15-30-35.451394.parquet", "**/details_harness|hendrycksTest-high_school_chemistry|5_2024-01-10T15-30-35.451394.parquet", "**/details_harness|hendrycksTest-high_school_computer_science|5_2024-01-10T15-30-35.451394.parquet", "**/details_harness|hendrycksTest-high_school_european_history|5_2024-01-10T15-30-35.451394.parquet", "**/details_harness|hendrycksTest-high_school_geography|5_2024-01-10T15-30-35.451394.parquet", "**/details_harness|hendrycksTest-high_school_government_and_politics|5_2024-01-10T15-30-35.451394.parquet", "**/details_harness|hendrycksTest-high_school_macroeconomics|5_2024-01-10T15-30-35.451394.parquet", "**/details_harness|hendrycksTest-high_school_mathematics|5_2024-01-10T15-30-35.451394.parquet", "**/details_harness|hendrycksTest-high_school_microeconomics|5_2024-01-10T15-30-35.451394.parquet", "**/details_harness|hendrycksTest-high_school_physics|5_2024-01-10T15-30-35.451394.parquet", "**/details_harness|hendrycksTest-high_school_psychology|5_2024-01-10T15-30-35.451394.parquet", "**/details_harness|hendrycksTest-high_school_statistics|5_2024-01-10T15-30-35.451394.parquet", "**/details_harness|hendrycksTest-high_school_us_history|5_2024-01-10T15-30-35.451394.parquet", "**/details_harness|hendrycksTest-high_school_world_history|5_2024-01-10T15-30-35.451394.parquet", "**/details_harness|hendrycksTest-human_aging|5_2024-01-10T15-30-35.451394.parquet", "**/details_harness|hendrycksTest-human_sexuality|5_2024-01-10T15-30-35.451394.parquet", "**/details_harness|hendrycksTest-international_law|5_2024-01-10T15-30-35.451394.parquet", "**/details_harness|hendrycksTest-jurisprudence|5_2024-01-10T15-30-35.451394.parquet", "**/details_harness|hendrycksTest-logical_fallacies|5_2024-01-10T15-30-35.451394.parquet", "**/details_harness|hendrycksTest-machine_learning|5_2024-01-10T15-30-35.451394.parquet", "**/details_harness|hendrycksTest-management|5_2024-01-10T15-30-35.451394.parquet", "**/details_harness|hendrycksTest-marketing|5_2024-01-10T15-30-35.451394.parquet", "**/details_harness|hendrycksTest-medical_genetics|5_2024-01-10T15-30-35.451394.parquet", "**/details_harness|hendrycksTest-miscellaneous|5_2024-01-10T15-30-35.451394.parquet", "**/details_harness|hendrycksTest-moral_disputes|5_2024-01-10T15-30-35.451394.parquet", "**/details_harness|hendrycksTest-moral_scenarios|5_2024-01-10T15-30-35.451394.parquet", "**/details_harness|hendrycksTest-nutrition|5_2024-01-10T15-30-35.451394.parquet", "**/details_harness|hendrycksTest-philosophy|5_2024-01-10T15-30-35.451394.parquet", "**/details_harness|hendrycksTest-prehistory|5_2024-01-10T15-30-35.451394.parquet", "**/details_harness|hendrycksTest-professional_accounting|5_2024-01-10T15-30-35.451394.parquet", "**/details_harness|hendrycksTest-professional_law|5_2024-01-10T15-30-35.451394.parquet", "**/details_harness|hendrycksTest-professional_medicine|5_2024-01-10T15-30-35.451394.parquet", "**/details_harness|hendrycksTest-professional_psychology|5_2024-01-10T15-30-35.451394.parquet", "**/details_harness|hendrycksTest-public_relations|5_2024-01-10T15-30-35.451394.parquet", "**/details_harness|hendrycksTest-security_studies|5_2024-01-10T15-30-35.451394.parquet", "**/details_harness|hendrycksTest-sociology|5_2024-01-10T15-30-35.451394.parquet", "**/details_harness|hendrycksTest-us_foreign_policy|5_2024-01-10T15-30-35.451394.parquet", "**/details_harness|hendrycksTest-virology|5_2024-01-10T15-30-35.451394.parquet", "**/details_harness|hendrycksTest-world_religions|5_2024-01-10T15-30-35.451394.parquet"]}]}, {"config_name": "harness_hendrycksTest_abstract_algebra_5", "data_files": [{"split": "2024_01_10T15_30_35.451394", "path": ["**/details_harness|hendrycksTest-abstract_algebra|5_2024-01-10T15-30-35.451394.parquet"]}, {"split": "latest", "path": ["**/details_harness|hendrycksTest-abstract_algebra|5_2024-01-10T15-30-35.451394.parquet"]}]}, {"config_name": "harness_hendrycksTest_anatomy_5", "data_files": [{"split": "2024_01_10T15_30_35.451394", "path": ["**/details_harness|hendrycksTest-anatomy|5_2024-01-10T15-30-35.451394.parquet"]}, {"split": "latest", "path": ["**/details_harness|hendrycksTest-anatomy|5_2024-01-10T15-30-35.451394.parquet"]}]}, {"config_name": "harness_hendrycksTest_astronomy_5", "data_files": [{"split": "2024_01_10T15_30_35.451394", "path": ["**/details_harness|hendrycksTest-astronomy|5_2024-01-10T15-30-35.451394.parquet"]}, {"split": "latest", "path": ["**/details_harness|hendrycksTest-astronomy|5_2024-01-10T15-30-35.451394.parquet"]}]}, {"config_name": "harness_hendrycksTest_business_ethics_5", "data_files": [{"split": "2024_01_10T15_30_35.451394", "path": ["**/details_harness|hendrycksTest-business_ethics|5_2024-01-10T15-30-35.451394.parquet"]}, {"split": "latest", "path": ["**/details_harness|hendrycksTest-business_ethics|5_2024-01-10T15-30-35.451394.parquet"]}]}, {"config_name": "harness_hendrycksTest_clinical_knowledge_5", "data_files": [{"split": "2024_01_10T15_30_35.451394", "path": ["**/details_harness|hendrycksTest-clinical_knowledge|5_2024-01-10T15-30-35.451394.parquet"]}, {"split": "latest", "path": ["**/details_harness|hendrycksTest-clinical_knowledge|5_2024-01-10T15-30-35.451394.parquet"]}]}, {"config_name": "harness_hendrycksTest_college_biology_5", "data_files": [{"split": "2024_01_10T15_30_35.451394", "path": ["**/details_harness|hendrycksTest-college_biology|5_2024-01-10T15-30-35.451394.parquet"]}, {"split": "latest", "path": ["**/details_harness|hendrycksTest-college_biology|5_2024-01-10T15-30-35.451394.parquet"]}]}, {"config_name": "harness_hendrycksTest_college_chemistry_5", "data_files": [{"split": "2024_01_10T15_30_35.451394", "path": ["**/details_harness|hendrycksTest-college_chemistry|5_2024-01-10T15-30-35.451394.parquet"]}, {"split": "latest", "path": ["**/details_harness|hendrycksTest-college_chemistry|5_2024-01-10T15-30-35.451394.parquet"]}]}, {"config_name": "harness_hendrycksTest_college_computer_science_5", "data_files": [{"split": "2024_01_10T15_30_35.451394", "path": ["**/details_harness|hendrycksTest-college_computer_science|5_2024-01-10T15-30-35.451394.parquet"]}, {"split": "latest", "path": ["**/details_harness|hendrycksTest-college_computer_science|5_2024-01-10T15-30-35.451394.parquet"]}]}, {"config_name": "harness_hendrycksTest_college_mathematics_5", "data_files": [{"split": "2024_01_10T15_30_35.451394", "path": ["**/details_harness|hendrycksTest-college_mathematics|5_2024-01-10T15-30-35.451394.parquet"]}, {"split": "latest", "path": ["**/details_harness|hendrycksTest-college_mathematics|5_2024-01-10T15-30-35.451394.parquet"]}]}, {"config_name": "harness_hendrycksTest_college_medicine_5", "data_files": [{"split": "2024_01_10T15_30_35.451394", "path": ["**/details_harness|hendrycksTest-college_medicine|5_2024-01-10T15-30-35.451394.parquet"]}, {"split": "latest", "path": ["**/details_harness|hendrycksTest-college_medicine|5_2024-01-10T15-30-35.451394.parquet"]}]}, {"config_name": "harness_hendrycksTest_college_physics_5", "data_files": [{"split": "2024_01_10T15_30_35.451394", "path": ["**/details_harness|hendrycksTest-college_physics|5_2024-01-10T15-30-35.451394.parquet"]}, {"split": "latest", "path": ["**/details_harness|hendrycksTest-college_physics|5_2024-01-10T15-30-35.451394.parquet"]}]}, {"config_name": "harness_hendrycksTest_computer_security_5", "data_files": [{"split": "2024_01_10T15_30_35.451394", "path": ["**/details_harness|hendrycksTest-computer_security|5_2024-01-10T15-30-35.451394.parquet"]}, {"split": "latest", "path": ["**/details_harness|hendrycksTest-computer_security|5_2024-01-10T15-30-35.451394.parquet"]}]}, {"config_name": "harness_hendrycksTest_conceptual_physics_5", "data_files": [{"split": "2024_01_10T15_30_35.451394", "path": ["**/details_harness|hendrycksTest-conceptual_physics|5_2024-01-10T15-30-35.451394.parquet"]}, {"split": "latest", "path": ["**/details_harness|hendrycksTest-conceptual_physics|5_2024-01-10T15-30-35.451394.parquet"]}]}, {"config_name": "harness_hendrycksTest_econometrics_5", "data_files": [{"split": "2024_01_10T15_30_35.451394", "path": ["**/details_harness|hendrycksTest-econometrics|5_2024-01-10T15-30-35.451394.parquet"]}, {"split": "latest", "path": ["**/details_harness|hendrycksTest-econometrics|5_2024-01-10T15-30-35.451394.parquet"]}]}, {"config_name": "harness_hendrycksTest_electrical_engineering_5", "data_files": [{"split": "2024_01_10T15_30_35.451394", "path": ["**/details_harness|hendrycksTest-electrical_engineering|5_2024-01-10T15-30-35.451394.parquet"]}, {"split": "latest", "path": ["**/details_harness|hendrycksTest-electrical_engineering|5_2024-01-10T15-30-35.451394.parquet"]}]}, {"config_name": "harness_hendrycksTest_elementary_mathematics_5", "data_files": [{"split": "2024_01_10T15_30_35.451394", "path": ["**/details_harness|hendrycksTest-elementary_mathematics|5_2024-01-10T15-30-35.451394.parquet"]}, {"split": "latest", "path": ["**/details_harness|hendrycksTest-elementary_mathematics|5_2024-01-10T15-30-35.451394.parquet"]}]}, {"config_name": "harness_hendrycksTest_formal_logic_5", "data_files": [{"split": "2024_01_10T15_30_35.451394", "path": ["**/details_harness|hendrycksTest-formal_logic|5_2024-01-10T15-30-35.451394.parquet"]}, {"split": "latest", "path": ["**/details_harness|hendrycksTest-formal_logic|5_2024-01-10T15-30-35.451394.parquet"]}]}, {"config_name": "harness_hendrycksTest_global_facts_5", "data_files": [{"split": "2024_01_10T15_30_35.451394", "path": ["**/details_harness|hendrycksTest-global_facts|5_2024-01-10T15-30-35.451394.parquet"]}, {"split": "latest", "path": ["**/details_harness|hendrycksTest-global_facts|5_2024-01-10T15-30-35.451394.parquet"]}]}, {"config_name": "harness_hendrycksTest_high_school_biology_5", "data_files": [{"split": "2024_01_10T15_30_35.451394", "path": ["**/details_harness|hendrycksTest-high_school_biology|5_2024-01-10T15-30-35.451394.parquet"]}, {"split": "latest", "path": ["**/details_harness|hendrycksTest-high_school_biology|5_2024-01-10T15-30-35.451394.parquet"]}]}, {"config_name": "harness_hendrycksTest_high_school_chemistry_5", "data_files": [{"split": "2024_01_10T15_30_35.451394", "path": ["**/details_harness|hendrycksTest-high_school_chemistry|5_2024-01-10T15-30-35.451394.parquet"]}, {"split": "latest", "path": ["**/details_harness|hendrycksTest-high_school_chemistry|5_2024-01-10T15-30-35.451394.parquet"]}]}, {"config_name": "harness_hendrycksTest_high_school_computer_science_5", "data_files": [{"split": "2024_01_10T15_30_35.451394", "path": ["**/details_harness|hendrycksTest-high_school_computer_science|5_2024-01-10T15-30-35.451394.parquet"]}, {"split": "latest", "path": ["**/details_harness|hendrycksTest-high_school_computer_science|5_2024-01-10T15-30-35.451394.parquet"]}]}, {"config_name": "harness_hendrycksTest_high_school_european_history_5", "data_files": [{"split": "2024_01_10T15_30_35.451394", "path": ["**/details_harness|hendrycksTest-high_school_european_history|5_2024-01-10T15-30-35.451394.parquet"]}, {"split": "latest", "path": ["**/details_harness|hendrycksTest-high_school_european_history|5_2024-01-10T15-30-35.451394.parquet"]}]}, {"config_name": "harness_hendrycksTest_high_school_geography_5", "data_files": [{"split": "2024_01_10T15_30_35.451394", "path": ["**/details_harness|hendrycksTest-high_school_geography|5_2024-01-10T15-30-35.451394.parquet"]}, {"split": "latest", "path": ["**/details_harness|hendrycksTest-high_school_geography|5_2024-01-10T15-30-35.451394.parquet"]}]}, {"config_name": "harness_hendrycksTest_high_school_government_and_politics_5", "data_files": [{"split": "2024_01_10T15_30_35.451394", "path": ["**/details_harness|hendrycksTest-high_school_government_and_politics|5_2024-01-10T15-30-35.451394.parquet"]}, {"split": "latest", "path": ["**/details_harness|hendrycksTest-high_school_government_and_politics|5_2024-01-10T15-30-35.451394.parquet"]}]}, {"config_name": "harness_hendrycksTest_high_school_macroeconomics_5", "data_files": [{"split": "2024_01_10T15_30_35.451394", "path": ["**/details_harness|hendrycksTest-high_school_macroeconomics|5_2024-01-10T15-30-35.451394.parquet"]}, {"split": "latest", "path": ["**/details_harness|hendrycksTest-high_school_macroeconomics|5_2024-01-10T15-30-35.451394.parquet"]}]}, {"config_name": "harness_hendrycksTest_high_school_mathematics_5", "data_files": [{"split": "2024_01_10T15_30_35.451394", "path": ["**/details_harness|hendrycksTest-high_school_mathematics|5_2024-01-10T15-30-35.451394.parquet"]}, {"split": "latest", "path": ["**/details_harness|hendrycksTest-high_school_mathematics|5_2024-01-10T15-30-35.451394.parquet"]}]}, {"config_name": "harness_hendrycksTest_high_school_microeconomics_5", "data_files": [{"split": "2024_01_10T15_30_35.451394", "path": ["**/details_harness|hendrycksTest-high_school_microeconomics|5_2024-01-10T15-30-35.451394.parquet"]}, {"split": "latest", "path": ["**/details_harness|hendrycksTest-high_school_microeconomics|5_2024-01-10T15-30-35.451394.parquet"]}]}, {"config_name": "harness_hendrycksTest_high_school_physics_5", "data_files": [{"split": "2024_01_10T15_30_35.451394", "path": ["**/details_harness|hendrycksTest-high_school_physics|5_2024-01-10T15-30-35.451394.parquet"]}, {"split": "latest", "path": ["**/details_harness|hendrycksTest-high_school_physics|5_2024-01-10T15-30-35.451394.parquet"]}]}, {"config_name": "harness_hendrycksTest_high_school_psychology_5", "data_files": [{"split": "2024_01_10T15_30_35.451394", "path": ["**/details_harness|hendrycksTest-high_school_psychology|5_2024-01-10T15-30-35.451394.parquet"]}, {"split": "latest", "path": ["**/details_harness|hendrycksTest-high_school_psychology|5_2024-01-10T15-30-35.451394.parquet"]}]}, {"config_name": "harness_hendrycksTest_high_school_statistics_5", "data_files": [{"split": "2024_01_10T15_30_35.451394", "path": ["**/details_harness|hendrycksTest-high_school_statistics|5_2024-01-10T15-30-35.451394.parquet"]}, {"split": "latest", "path": ["**/details_harness|hendrycksTest-high_school_statistics|5_2024-01-10T15-30-35.451394.parquet"]}]}, {"config_name": "harness_hendrycksTest_high_school_us_history_5", "data_files": [{"split": "2024_01_10T15_30_35.451394", "path": ["**/details_harness|hendrycksTest-high_school_us_history|5_2024-01-10T15-30-35.451394.parquet"]}, {"split": "latest", "path": ["**/details_harness|hendrycksTest-high_school_us_history|5_2024-01-10T15-30-35.451394.parquet"]}]}, {"config_name": "harness_hendrycksTest_high_school_world_history_5", "data_files": [{"split": "2024_01_10T15_30_35.451394", "path": ["**/details_harness|hendrycksTest-high_school_world_history|5_2024-01-10T15-30-35.451394.parquet"]}, {"split": "latest", "path": ["**/details_harness|hendrycksTest-high_school_world_history|5_2024-01-10T15-30-35.451394.parquet"]}]}, {"config_name": "harness_hendrycksTest_human_aging_5", "data_files": [{"split": "2024_01_10T15_30_35.451394", "path": ["**/details_harness|hendrycksTest-human_aging|5_2024-01-10T15-30-35.451394.parquet"]}, {"split": "latest", "path": ["**/details_harness|hendrycksTest-human_aging|5_2024-01-10T15-30-35.451394.parquet"]}]}, {"config_name": "harness_hendrycksTest_human_sexuality_5", "data_files": [{"split": "2024_01_10T15_30_35.451394", "path": ["**/details_harness|hendrycksTest-human_sexuality|5_2024-01-10T15-30-35.451394.parquet"]}, {"split": "latest", "path": ["**/details_harness|hendrycksTest-human_sexuality|5_2024-01-10T15-30-35.451394.parquet"]}]}, {"config_name": "harness_hendrycksTest_international_law_5", "data_files": [{"split": "2024_01_10T15_30_35.451394", "path": ["**/details_harness|hendrycksTest-international_law|5_2024-01-10T15-30-35.451394.parquet"]}, {"split": "latest", "path": ["**/details_harness|hendrycksTest-international_law|5_2024-01-10T15-30-35.451394.parquet"]}]}, {"config_name": "harness_hendrycksTest_jurisprudence_5", "data_files": [{"split": "2024_01_10T15_30_35.451394", "path": ["**/details_harness|hendrycksTest-jurisprudence|5_2024-01-10T15-30-35.451394.parquet"]}, {"split": "latest", "path": ["**/details_harness|hendrycksTest-jurisprudence|5_2024-01-10T15-30-35.451394.parquet"]}]}, {"config_name": "harness_hendrycksTest_logical_fallacies_5", "data_files": [{"split": "2024_01_10T15_30_35.451394", "path": ["**/details_harness|hendrycksTest-logical_fallacies|5_2024-01-10T15-30-35.451394.parquet"]}, {"split": "latest", "path": ["**/details_harness|hendrycksTest-logical_fallacies|5_2024-01-10T15-30-35.451394.parquet"]}]}, {"config_name": "harness_hendrycksTest_machine_learning_5", "data_files": [{"split": "2024_01_10T15_30_35.451394", "path": ["**/details_harness|hendrycksTest-machine_learning|5_2024-01-10T15-30-35.451394.parquet"]}, {"split": "latest", "path": ["**/details_harness|hendrycksTest-machine_learning|5_2024-01-10T15-30-35.451394.parquet"]}]}, {"config_name": "harness_hendrycksTest_management_5", "data_files": [{"split": "2024_01_10T15_30_35.451394", "path": ["**/details_harness|hendrycksTest-management|5_2024-01-10T15-30-35.451394.parquet"]}, {"split": "latest", "path": ["**/details_harness|hendrycksTest-management|5_2024-01-10T15-30-35.451394.parquet"]}]}, {"config_name": "harness_hendrycksTest_marketing_5", "data_files": [{"split": "2024_01_10T15_30_35.451394", "path": ["**/details_harness|hendrycksTest-marketing|5_2024-01-10T15-30-35.451394.parquet"]}, {"split": "latest", "path": ["**/details_harness|hendrycksTest-marketing|5_2024-01-10T15-30-35.451394.parquet"]}]}, {"config_name": "harness_hendrycksTest_medical_genetics_5", "data_files": [{"split": "2024_01_10T15_30_35.451394", "path": ["**/details_harness|hendrycksTest-medical_genetics|5_2024-01-10T15-30-35.451394.parquet"]}, {"split": "latest", "path": ["**/details_harness|hendrycksTest-medical_genetics|5_2024-01-10T15-30-35.451394.parquet"]}]}, {"config_name": "harness_hendrycksTest_miscellaneous_5", "data_files": [{"split": "2024_01_10T15_30_35.451394", "path": ["**/details_harness|hendrycksTest-miscellaneous|5_2024-01-10T15-30-35.451394.parquet"]}, {"split": "latest", "path": ["**/details_harness|hendrycksTest-miscellaneous|5_2024-01-10T15-30-35.451394.parquet"]}]}, {"config_name": "harness_hendrycksTest_moral_disputes_5", "data_files": [{"split": "2024_01_10T15_30_35.451394", "path": ["**/details_harness|hendrycksTest-moral_disputes|5_2024-01-10T15-30-35.451394.parquet"]}, {"split": "latest", "path": ["**/details_harness|hendrycksTest-moral_disputes|5_2024-01-10T15-30-35.451394.parquet"]}]}, {"config_name": "harness_hendrycksTest_moral_scenarios_5", "data_files": [{"split": "2024_01_10T15_30_35.451394", "path": ["**/details_harness|hendrycksTest-moral_scenarios|5_2024-01-10T15-30-35.451394.parquet"]}, {"split": "latest", "path": ["**/details_harness|hendrycksTest-moral_scenarios|5_2024-01-10T15-30-35.451394.parquet"]}]}, {"config_name": "harness_hendrycksTest_nutrition_5", "data_files": [{"split": "2024_01_10T15_30_35.451394", "path": ["**/details_harness|hendrycksTest-nutrition|5_2024-01-10T15-30-35.451394.parquet"]}, {"split": "latest", "path": ["**/details_harness|hendrycksTest-nutrition|5_2024-01-10T15-30-35.451394.parquet"]}]}, {"config_name": "harness_hendrycksTest_philosophy_5", "data_files": [{"split": "2024_01_10T15_30_35.451394", "path": ["**/details_harness|hendrycksTest-philosophy|5_2024-01-10T15-30-35.451394.parquet"]}, {"split": "latest", "path": ["**/details_harness|hendrycksTest-philosophy|5_2024-01-10T15-30-35.451394.parquet"]}]}, {"config_name": "harness_hendrycksTest_prehistory_5", "data_files": [{"split": "2024_01_10T15_30_35.451394", "path": ["**/details_harness|hendrycksTest-prehistory|5_2024-01-10T15-30-35.451394.parquet"]}, {"split": "latest", "path": ["**/details_harness|hendrycksTest-prehistory|5_2024-01-10T15-30-35.451394.parquet"]}]}, {"config_name": "harness_hendrycksTest_professional_accounting_5", "data_files": [{"split": "2024_01_10T15_30_35.451394", "path": ["**/details_harness|hendrycksTest-professional_accounting|5_2024-01-10T15-30-35.451394.parquet"]}, {"split": "latest", "path": ["**/details_harness|hendrycksTest-professional_accounting|5_2024-01-10T15-30-35.451394.parquet"]}]}, {"config_name": "harness_hendrycksTest_professional_law_5", "data_files": [{"split": "2024_01_10T15_30_35.451394", "path": ["**/details_harness|hendrycksTest-professional_law|5_2024-01-10T15-30-35.451394.parquet"]}, {"split": "latest", "path": ["**/details_harness|hendrycksTest-professional_law|5_2024-01-10T15-30-35.451394.parquet"]}]}, {"config_name": "harness_hendrycksTest_professional_medicine_5", "data_files": [{"split": "2024_01_10T15_30_35.451394", "path": ["**/details_harness|hendrycksTest-professional_medicine|5_2024-01-10T15-30-35.451394.parquet"]}, {"split": "latest", "path": ["**/details_harness|hendrycksTest-professional_medicine|5_2024-01-10T15-30-35.451394.parquet"]}]}, {"config_name": "harness_hendrycksTest_professional_psychology_5", "data_files": [{"split": "2024_01_10T15_30_35.451394", "path": ["**/details_harness|hendrycksTest-professional_psychology|5_2024-01-10T15-30-35.451394.parquet"]}, {"split": "latest", "path": ["**/details_harness|hendrycksTest-professional_psychology|5_2024-01-10T15-30-35.451394.parquet"]}]}, {"config_name": "harness_hendrycksTest_public_relations_5", "data_files": [{"split": "2024_01_10T15_30_35.451394", "path": ["**/details_harness|hendrycksTest-public_relations|5_2024-01-10T15-30-35.451394.parquet"]}, {"split": "latest", "path": ["**/details_harness|hendrycksTest-public_relations|5_2024-01-10T15-30-35.451394.parquet"]}]}, {"config_name": "harness_hendrycksTest_security_studies_5", "data_files": [{"split": "2024_01_10T15_30_35.451394", "path": ["**/details_harness|hendrycksTest-security_studies|5_2024-01-10T15-30-35.451394.parquet"]}, {"split": "latest", "path": ["**/details_harness|hendrycksTest-security_studies|5_2024-01-10T15-30-35.451394.parquet"]}]}, {"config_name": "harness_hendrycksTest_sociology_5", "data_files": [{"split": "2024_01_10T15_30_35.451394", "path": ["**/details_harness|hendrycksTest-sociology|5_2024-01-10T15-30-35.451394.parquet"]}, {"split": "latest", "path": ["**/details_harness|hendrycksTest-sociology|5_2024-01-10T15-30-35.451394.parquet"]}]}, {"config_name": "harness_hendrycksTest_us_foreign_policy_5", "data_files": [{"split": "2024_01_10T15_30_35.451394", "path": ["**/details_harness|hendrycksTest-us_foreign_policy|5_2024-01-10T15-30-35.451394.parquet"]}, {"split": "latest", "path": ["**/details_harness|hendrycksTest-us_foreign_policy|5_2024-01-10T15-30-35.451394.parquet"]}]}, {"config_name": "harness_hendrycksTest_virology_5", "data_files": [{"split": "2024_01_10T15_30_35.451394", "path": ["**/details_harness|hendrycksTest-virology|5_2024-01-10T15-30-35.451394.parquet"]}, {"split": "latest", "path": ["**/details_harness|hendrycksTest-virology|5_2024-01-10T15-30-35.451394.parquet"]}]}, {"config_name": "harness_hendrycksTest_world_religions_5", "data_files": [{"split": "2024_01_10T15_30_35.451394", "path": ["**/details_harness|hendrycksTest-world_religions|5_2024-01-10T15-30-35.451394.parquet"]}, {"split": "latest", "path": ["**/details_harness|hendrycksTest-world_religions|5_2024-01-10T15-30-35.451394.parquet"]}]}, {"config_name": "harness_truthfulqa_mc_0", "data_files": [{"split": "2024_01_10T15_30_35.451394", "path": ["**/details_harness|truthfulqa:mc|0_2024-01-10T15-30-35.451394.parquet"]}, {"split": "latest", "path": ["**/details_harness|truthfulqa:mc|0_2024-01-10T15-30-35.451394.parquet"]}]}, {"config_name": "harness_winogrande_5", "data_files": [{"split": "2024_01_10T15_30_35.451394", "path": ["**/details_harness|winogrande|5_2024-01-10T15-30-35.451394.parquet"]}, {"split": "latest", "path": ["**/details_harness|winogrande|5_2024-01-10T15-30-35.451394.parquet"]}]}, {"config_name": "results", "data_files": [{"split": "2024_01_10T15_30_35.451394", "path": ["results_2024-01-10T15-30-35.451394.parquet"]}, {"split": "latest", "path": ["results_2024-01-10T15-30-35.451394.parquet"]}]}]} | 2024-01-10T15:33:00+00:00 | [] | [] | TAGS
#region-us
|
# Dataset Card for Evaluation run of shitshow123/tinylamma-20000
Dataset automatically created during the evaluation run of model shitshow123/tinylamma-20000 on the Open LLM Leaderboard.
The dataset is composed of 63 configuration, each one coresponding to one of the evaluated task.
The dataset has been created from 1 run(s). Each run can be found as a specific split in each configuration, the split being named using the timestamp of the run.The "train" split is always pointing to the latest results.
An additional configuration "results" store all the aggregated results of the run (and is used to compute and display the aggregated metrics on the Open LLM Leaderboard).
To load the details from a run, you can for instance do the following:
## Latest results
These are the latest results from run 2024-01-10T15:30:35.451394(note that their might be results for other tasks in the repos if successive evals didn't cover the same tasks. You find each in the results and the "latest" split for each eval):
## Dataset Details
### Dataset Description
- Curated by:
- Funded by [optional]:
- Shared by [optional]:
- Language(s) (NLP):
- License:
### Dataset Sources [optional]
- Repository:
- Paper [optional]:
- Demo [optional]:
## Uses
### Direct Use
### Out-of-Scope Use
## Dataset Structure
## Dataset Creation
### Curation Rationale
### Source Data
#### Data Collection and Processing
#### Who are the source data producers?
### Annotations [optional]
#### Annotation process
#### Who are the annotators?
#### Personal and Sensitive Information
## Bias, Risks, and Limitations
### Recommendations
Users should be made aware of the risks, biases and limitations of the dataset. More information needed for further recommendations.
[optional]
BibTeX:
APA:
## Glossary [optional]
## More Information [optional]
## Dataset Card Authors [optional]
## Dataset Card Contact
| [
"# Dataset Card for Evaluation run of shitshow123/tinylamma-20000\n\n\n\nDataset automatically created during the evaluation run of model shitshow123/tinylamma-20000 on the Open LLM Leaderboard.\n\nThe dataset is composed of 63 configuration, each one coresponding to one of the evaluated task.\n\nThe dataset has been created from 1 run(s). Each run can be found as a specific split in each configuration, the split being named using the timestamp of the run.The \"train\" split is always pointing to the latest results.\n\nAn additional configuration \"results\" store all the aggregated results of the run (and is used to compute and display the aggregated metrics on the Open LLM Leaderboard).\n\nTo load the details from a run, you can for instance do the following:",
"## Latest results\n\nThese are the latest results from run 2024-01-10T15:30:35.451394(note that their might be results for other tasks in the repos if successive evals didn't cover the same tasks. You find each in the results and the \"latest\" split for each eval):",
"## Dataset Details",
"### Dataset Description\n\n\n\n\n\n- Curated by: \n- Funded by [optional]: \n- Shared by [optional]: \n- Language(s) (NLP): \n- License:",
"### Dataset Sources [optional]\n\n\n\n- Repository: \n- Paper [optional]: \n- Demo [optional]:",
"## Uses",
"### Direct Use",
"### Out-of-Scope Use",
"## Dataset Structure",
"## Dataset Creation",
"### Curation Rationale",
"### Source Data",
"#### Data Collection and Processing",
"#### Who are the source data producers?",
"### Annotations [optional]",
"#### Annotation process",
"#### Who are the annotators?",
"#### Personal and Sensitive Information",
"## Bias, Risks, and Limitations",
"### Recommendations\n\n\n\nUsers should be made aware of the risks, biases and limitations of the dataset. More information needed for further recommendations.\n\n[optional]\n\n\n\nBibTeX:\n\n\n\nAPA:",
"## Glossary [optional]",
"## More Information [optional]",
"## Dataset Card Authors [optional]",
"## Dataset Card Contact"
] | [
"TAGS\n#region-us \n",
"# Dataset Card for Evaluation run of shitshow123/tinylamma-20000\n\n\n\nDataset automatically created during the evaluation run of model shitshow123/tinylamma-20000 on the Open LLM Leaderboard.\n\nThe dataset is composed of 63 configuration, each one coresponding to one of the evaluated task.\n\nThe dataset has been created from 1 run(s). Each run can be found as a specific split in each configuration, the split being named using the timestamp of the run.The \"train\" split is always pointing to the latest results.\n\nAn additional configuration \"results\" store all the aggregated results of the run (and is used to compute and display the aggregated metrics on the Open LLM Leaderboard).\n\nTo load the details from a run, you can for instance do the following:",
"## Latest results\n\nThese are the latest results from run 2024-01-10T15:30:35.451394(note that their might be results for other tasks in the repos if successive evals didn't cover the same tasks. You find each in the results and the \"latest\" split for each eval):",
"## Dataset Details",
"### Dataset Description\n\n\n\n\n\n- Curated by: \n- Funded by [optional]: \n- Shared by [optional]: \n- Language(s) (NLP): \n- License:",
"### Dataset Sources [optional]\n\n\n\n- Repository: \n- Paper [optional]: \n- Demo [optional]:",
"## Uses",
"### Direct Use",
"### Out-of-Scope Use",
"## Dataset Structure",
"## Dataset Creation",
"### Curation Rationale",
"### Source Data",
"#### Data Collection and Processing",
"#### Who are the source data producers?",
"### Annotations [optional]",
"#### Annotation process",
"#### Who are the annotators?",
"#### Personal and Sensitive Information",
"## Bias, Risks, and Limitations",
"### Recommendations\n\n\n\nUsers should be made aware of the risks, biases and limitations of the dataset. More information needed for further recommendations.\n\n[optional]\n\n\n\nBibTeX:\n\n\n\nAPA:",
"## Glossary [optional]",
"## More Information [optional]",
"## Dataset Card Authors [optional]",
"## Dataset Card Contact"
] |
6a0ee29c9f3b1c6a80a1da026f3bebfb1d6eb221 |
# Dataset Card for "agieval-aqua-rat"
Dataset taken from https://github.com/microsoft/AGIEval and processed as in that repo, following dmayhem93/agieval-* datasets on the HF hub.
This dataset contains the contents of the AquA-RAT subtask of AGIEval, as accessed in https://github.com/ruixiangcui/AGIEval/commit/5c77d073fda993f1652eaae3cf5d04cc5fd21d40 .
Citation:
```
@misc{zhong2023agieval,
title={AGIEval: A Human-Centric Benchmark for Evaluating Foundation Models},
author={Wanjun Zhong and Ruixiang Cui and Yiduo Guo and Yaobo Liang and Shuai Lu and Yanlin Wang and Amin Saied and Weizhu Chen and Nan Duan},
year={2023},
eprint={2304.06364},
archivePrefix={arXiv},
primaryClass={cs.CL}
}
```
Please make sure to cite all the individual datasets in your paper when you use them. We provide the relevant citation information below:
```
@inproceedings{ling-etal-2017-program,
title = "Program Induction by Rationale Generation: Learning to Solve and Explain Algebraic Word Problems",
author = "Ling, Wang and
Yogatama, Dani and
Dyer, Chris and
Blunsom, Phil",
booktitle = "Proceedings of the 55th Annual Meeting of the Association for Computational Linguistics (Volume 1: Long Papers)",
month = jul,
year = "2017",
address = "Vancouver, Canada",
publisher = "Association for Computational Linguistics",
url = "https://aclanthology.org/P17-1015",
doi = "10.18653/v1/P17-1015",
pages = "158--167",
abstract = "Solving algebraic word problems requires executing a series of arithmetic operations{---}a program{---}to obtain a final answer. However, since programs can be arbitrarily complicated, inducing them directly from question-answer pairs is a formidable challenge. To make this task more feasible, we solve these problems by generating answer rationales, sequences of natural language and human-readable mathematical expressions that derive the final answer through a series of small steps. Although rationales do not explicitly specify programs, they provide a scaffolding for their structure via intermediate milestones. To evaluate our approach, we have created a new 100,000-sample dataset of questions, answers and rationales. Experimental results show that indirect supervision of program learning via answer rationales is a promising strategy for inducing arithmetic programs.",
}
@inproceedings{hendrycksmath2021,
title={Measuring Mathematical Problem Solving With the MATH Dataset},
author={Dan Hendrycks and Collin Burns and Saurav Kadavath and Akul Arora and Steven Basart and Eric Tang and Dawn Song and Jacob Steinhardt},
journal={NeurIPS},
year={2021}
}
@inproceedings{Liu2020LogiQAAC,
title={LogiQA: A Challenge Dataset for Machine Reading Comprehension with Logical Reasoning},
author={Jian Liu and Leyang Cui and Hanmeng Liu and Dandan Huang and Yile Wang and Yue Zhang},
booktitle={International Joint Conference on Artificial Intelligence},
year={2020}
}
@inproceedings{zhong2019jec,
title={JEC-QA: A Legal-Domain Question Answering Dataset},
author={Zhong, Haoxi and Xiao, Chaojun and Tu, Cunchao and Zhang, Tianyang and Liu, Zhiyuan and Sun, Maosong},
booktitle={Proceedings of AAAI},
year={2020},
}
@article{Wang2021FromLT,
title={From LSAT: The Progress and Challenges of Complex Reasoning},
author={Siyuan Wang and Zhongkun Liu and Wanjun Zhong and Ming Zhou and Zhongyu Wei and Zhumin Chen and Nan Duan},
journal={IEEE/ACM Transactions on Audio, Speech, and Language Processing},
year={2021},
volume={30},
pages={2201-2216}
}
``` | hails/agieval-aqua-rat | [
"arxiv:2304.06364",
"region:us"
] | 2024-01-10T15:32:41+00:00 | {"dataset_info": {"features": [{"name": "query", "dtype": "string"}, {"name": "choices", "sequence": "string"}, {"name": "gold", "sequence": "int64"}], "splits": [{"name": "test", "num_bytes": 93696, "num_examples": 254}], "download_size": 51275, "dataset_size": 93696}, "configs": [{"config_name": "default", "data_files": [{"split": "test", "path": "data/test-*"}]}]} | 2024-01-26T18:36:03+00:00 | [
"2304.06364"
] | [] | TAGS
#arxiv-2304.06364 #region-us
|
# Dataset Card for "agieval-aqua-rat"
Dataset taken from URL and processed as in that repo, following dmayhem93/agieval-* datasets on the HF hub.
This dataset contains the contents of the AquA-RAT subtask of AGIEval, as accessed in URL .
Citation:
Please make sure to cite all the individual datasets in your paper when you use them. We provide the relevant citation information below:
| [
"# Dataset Card for \"agieval-aqua-rat\"\n\n\nDataset taken from URL and processed as in that repo, following dmayhem93/agieval-* datasets on the HF hub.\n\nThis dataset contains the contents of the AquA-RAT subtask of AGIEval, as accessed in URL .\n\n\nCitation:\n\n\nPlease make sure to cite all the individual datasets in your paper when you use them. We provide the relevant citation information below:"
] | [
"TAGS\n#arxiv-2304.06364 #region-us \n",
"# Dataset Card for \"agieval-aqua-rat\"\n\n\nDataset taken from URL and processed as in that repo, following dmayhem93/agieval-* datasets on the HF hub.\n\nThis dataset contains the contents of the AquA-RAT subtask of AGIEval, as accessed in URL .\n\n\nCitation:\n\n\nPlease make sure to cite all the individual datasets in your paper when you use them. We provide the relevant citation information below:"
] |
c02066c0cfe2df9adf05512f99912644527af21c |
# Dataset Card for Evaluation run of abacusai/Fewshot-Metamath-Mistral
<!-- Provide a quick summary of the dataset. -->
Dataset automatically created during the evaluation run of model [abacusai/Fewshot-Metamath-Mistral](https://huggingface.co/abacusai/Fewshot-Metamath-Mistral) on the [Open LLM Leaderboard](https://huggingface.co/spaces/HuggingFaceH4/open_llm_leaderboard).
The dataset is composed of 63 configuration, each one coresponding to one of the evaluated task.
The dataset has been created from 1 run(s). Each run can be found as a specific split in each configuration, the split being named using the timestamp of the run.The "train" split is always pointing to the latest results.
An additional configuration "results" store all the aggregated results of the run (and is used to compute and display the aggregated metrics on the [Open LLM Leaderboard](https://huggingface.co/spaces/HuggingFaceH4/open_llm_leaderboard)).
To load the details from a run, you can for instance do the following:
```python
from datasets import load_dataset
data = load_dataset("open-llm-leaderboard/details_abacusai__Fewshot-Metamath-Mistral",
"harness_winogrande_5",
split="train")
```
## Latest results
These are the [latest results from run 2024-01-10T15:33:00.629548](https://huggingface.co/datasets/open-llm-leaderboard/details_abacusai__Fewshot-Metamath-Mistral/blob/main/results_2024-01-10T15-33-00.629548.json)(note that their might be results for other tasks in the repos if successive evals didn't cover the same tasks. You find each in the results and the "latest" split for each eval):
```python
{
"all": {
"acc": 0.5848581335337723,
"acc_stderr": 0.03341837065575973,
"acc_norm": 0.5842626849450804,
"acc_norm_stderr": 0.0341126414564645,
"mc1": 0.2937576499388005,
"mc1_stderr": 0.015945068581236614,
"mc2": 0.43044441727793376,
"mc2_stderr": 0.015120468254750151
},
"harness|arc:challenge|25": {
"acc": 0.5264505119453925,
"acc_stderr": 0.01459093135812017,
"acc_norm": 0.5776450511945392,
"acc_norm_stderr": 0.014434138713379976
},
"harness|hellaswag|10": {
"acc": 0.6188010356502689,
"acc_stderr": 0.004846886929763462,
"acc_norm": 0.8059151563433579,
"acc_norm_stderr": 0.0039468624307729535
},
"harness|hendrycksTest-abstract_algebra|5": {
"acc": 0.28,
"acc_stderr": 0.04512608598542128,
"acc_norm": 0.28,
"acc_norm_stderr": 0.04512608598542128
},
"harness|hendrycksTest-anatomy|5": {
"acc": 0.5481481481481482,
"acc_stderr": 0.04299268905480864,
"acc_norm": 0.5481481481481482,
"acc_norm_stderr": 0.04299268905480864
},
"harness|hendrycksTest-astronomy|5": {
"acc": 0.5986842105263158,
"acc_stderr": 0.039889037033362836,
"acc_norm": 0.5986842105263158,
"acc_norm_stderr": 0.039889037033362836
},
"harness|hendrycksTest-business_ethics|5": {
"acc": 0.51,
"acc_stderr": 0.05024183937956912,
"acc_norm": 0.51,
"acc_norm_stderr": 0.05024183937956912
},
"harness|hendrycksTest-clinical_knowledge|5": {
"acc": 0.6037735849056604,
"acc_stderr": 0.030102793781791194,
"acc_norm": 0.6037735849056604,
"acc_norm_stderr": 0.030102793781791194
},
"harness|hendrycksTest-college_biology|5": {
"acc": 0.6527777777777778,
"acc_stderr": 0.039812405437178615,
"acc_norm": 0.6527777777777778,
"acc_norm_stderr": 0.039812405437178615
},
"harness|hendrycksTest-college_chemistry|5": {
"acc": 0.34,
"acc_stderr": 0.04760952285695236,
"acc_norm": 0.34,
"acc_norm_stderr": 0.04760952285695236
},
"harness|hendrycksTest-college_computer_science|5": {
"acc": 0.49,
"acc_stderr": 0.05024183937956912,
"acc_norm": 0.49,
"acc_norm_stderr": 0.05024183937956912
},
"harness|hendrycksTest-college_mathematics|5": {
"acc": 0.32,
"acc_stderr": 0.046882617226215034,
"acc_norm": 0.32,
"acc_norm_stderr": 0.046882617226215034
},
"harness|hendrycksTest-college_medicine|5": {
"acc": 0.5780346820809249,
"acc_stderr": 0.03765746693865151,
"acc_norm": 0.5780346820809249,
"acc_norm_stderr": 0.03765746693865151
},
"harness|hendrycksTest-college_physics|5": {
"acc": 0.30392156862745096,
"acc_stderr": 0.04576665403207762,
"acc_norm": 0.30392156862745096,
"acc_norm_stderr": 0.04576665403207762
},
"harness|hendrycksTest-computer_security|5": {
"acc": 0.72,
"acc_stderr": 0.04512608598542128,
"acc_norm": 0.72,
"acc_norm_stderr": 0.04512608598542128
},
"harness|hendrycksTest-conceptual_physics|5": {
"acc": 0.4765957446808511,
"acc_stderr": 0.03265019475033582,
"acc_norm": 0.4765957446808511,
"acc_norm_stderr": 0.03265019475033582
},
"harness|hendrycksTest-econometrics|5": {
"acc": 0.5,
"acc_stderr": 0.047036043419179864,
"acc_norm": 0.5,
"acc_norm_stderr": 0.047036043419179864
},
"harness|hendrycksTest-electrical_engineering|5": {
"acc": 0.5241379310344828,
"acc_stderr": 0.0416180850350153,
"acc_norm": 0.5241379310344828,
"acc_norm_stderr": 0.0416180850350153
},
"harness|hendrycksTest-elementary_mathematics|5": {
"acc": 0.3544973544973545,
"acc_stderr": 0.024636830602842,
"acc_norm": 0.3544973544973545,
"acc_norm_stderr": 0.024636830602842
},
"harness|hendrycksTest-formal_logic|5": {
"acc": 0.42063492063492064,
"acc_stderr": 0.04415438226743744,
"acc_norm": 0.42063492063492064,
"acc_norm_stderr": 0.04415438226743744
},
"harness|hendrycksTest-global_facts|5": {
"acc": 0.36,
"acc_stderr": 0.04824181513244218,
"acc_norm": 0.36,
"acc_norm_stderr": 0.04824181513244218
},
"harness|hendrycksTest-high_school_biology|5": {
"acc": 0.7,
"acc_stderr": 0.026069362295335137,
"acc_norm": 0.7,
"acc_norm_stderr": 0.026069362295335137
},
"harness|hendrycksTest-high_school_chemistry|5": {
"acc": 0.39901477832512317,
"acc_stderr": 0.03445487686264715,
"acc_norm": 0.39901477832512317,
"acc_norm_stderr": 0.03445487686264715
},
"harness|hendrycksTest-high_school_computer_science|5": {
"acc": 0.54,
"acc_stderr": 0.05009082659620333,
"acc_norm": 0.54,
"acc_norm_stderr": 0.05009082659620333
},
"harness|hendrycksTest-high_school_european_history|5": {
"acc": 0.7333333333333333,
"acc_stderr": 0.03453131801885417,
"acc_norm": 0.7333333333333333,
"acc_norm_stderr": 0.03453131801885417
},
"harness|hendrycksTest-high_school_geography|5": {
"acc": 0.7727272727272727,
"acc_stderr": 0.02985751567338642,
"acc_norm": 0.7727272727272727,
"acc_norm_stderr": 0.02985751567338642
},
"harness|hendrycksTest-high_school_government_and_politics|5": {
"acc": 0.8393782383419689,
"acc_stderr": 0.026499057701397443,
"acc_norm": 0.8393782383419689,
"acc_norm_stderr": 0.026499057701397443
},
"harness|hendrycksTest-high_school_macroeconomics|5": {
"acc": 0.5641025641025641,
"acc_stderr": 0.02514180151117749,
"acc_norm": 0.5641025641025641,
"acc_norm_stderr": 0.02514180151117749
},
"harness|hendrycksTest-high_school_mathematics|5": {
"acc": 0.28888888888888886,
"acc_stderr": 0.027634907264178544,
"acc_norm": 0.28888888888888886,
"acc_norm_stderr": 0.027634907264178544
},
"harness|hendrycksTest-high_school_microeconomics|5": {
"acc": 0.5798319327731093,
"acc_stderr": 0.03206183783236152,
"acc_norm": 0.5798319327731093,
"acc_norm_stderr": 0.03206183783236152
},
"harness|hendrycksTest-high_school_physics|5": {
"acc": 0.33112582781456956,
"acc_stderr": 0.038425817186598696,
"acc_norm": 0.33112582781456956,
"acc_norm_stderr": 0.038425817186598696
},
"harness|hendrycksTest-high_school_psychology|5": {
"acc": 0.7577981651376147,
"acc_stderr": 0.018368176306598618,
"acc_norm": 0.7577981651376147,
"acc_norm_stderr": 0.018368176306598618
},
"harness|hendrycksTest-high_school_statistics|5": {
"acc": 0.39814814814814814,
"acc_stderr": 0.033384734032074016,
"acc_norm": 0.39814814814814814,
"acc_norm_stderr": 0.033384734032074016
},
"harness|hendrycksTest-high_school_us_history|5": {
"acc": 0.696078431372549,
"acc_stderr": 0.03228210387037892,
"acc_norm": 0.696078431372549,
"acc_norm_stderr": 0.03228210387037892
},
"harness|hendrycksTest-high_school_world_history|5": {
"acc": 0.7383966244725738,
"acc_stderr": 0.028609516716994934,
"acc_norm": 0.7383966244725738,
"acc_norm_stderr": 0.028609516716994934
},
"harness|hendrycksTest-human_aging|5": {
"acc": 0.6547085201793722,
"acc_stderr": 0.03191100192835794,
"acc_norm": 0.6547085201793722,
"acc_norm_stderr": 0.03191100192835794
},
"harness|hendrycksTest-human_sexuality|5": {
"acc": 0.7175572519083969,
"acc_stderr": 0.03948406125768361,
"acc_norm": 0.7175572519083969,
"acc_norm_stderr": 0.03948406125768361
},
"harness|hendrycksTest-international_law|5": {
"acc": 0.7603305785123967,
"acc_stderr": 0.03896878985070417,
"acc_norm": 0.7603305785123967,
"acc_norm_stderr": 0.03896878985070417
},
"harness|hendrycksTest-jurisprudence|5": {
"acc": 0.7129629629629629,
"acc_stderr": 0.043733130409147614,
"acc_norm": 0.7129629629629629,
"acc_norm_stderr": 0.043733130409147614
},
"harness|hendrycksTest-logical_fallacies|5": {
"acc": 0.6748466257668712,
"acc_stderr": 0.036803503712864595,
"acc_norm": 0.6748466257668712,
"acc_norm_stderr": 0.036803503712864595
},
"harness|hendrycksTest-machine_learning|5": {
"acc": 0.4107142857142857,
"acc_stderr": 0.04669510663875191,
"acc_norm": 0.4107142857142857,
"acc_norm_stderr": 0.04669510663875191
},
"harness|hendrycksTest-management|5": {
"acc": 0.7281553398058253,
"acc_stderr": 0.044052680241409216,
"acc_norm": 0.7281553398058253,
"acc_norm_stderr": 0.044052680241409216
},
"harness|hendrycksTest-marketing|5": {
"acc": 0.8333333333333334,
"acc_stderr": 0.02441494730454368,
"acc_norm": 0.8333333333333334,
"acc_norm_stderr": 0.02441494730454368
},
"harness|hendrycksTest-medical_genetics|5": {
"acc": 0.6,
"acc_stderr": 0.049236596391733084,
"acc_norm": 0.6,
"acc_norm_stderr": 0.049236596391733084
},
"harness|hendrycksTest-miscellaneous|5": {
"acc": 0.7713920817369093,
"acc_stderr": 0.015016884698539887,
"acc_norm": 0.7713920817369093,
"acc_norm_stderr": 0.015016884698539887
},
"harness|hendrycksTest-moral_disputes|5": {
"acc": 0.6098265895953757,
"acc_stderr": 0.026261677607806642,
"acc_norm": 0.6098265895953757,
"acc_norm_stderr": 0.026261677607806642
},
"harness|hendrycksTest-moral_scenarios|5": {
"acc": 0.37988826815642457,
"acc_stderr": 0.016232826818678492,
"acc_norm": 0.37988826815642457,
"acc_norm_stderr": 0.016232826818678492
},
"harness|hendrycksTest-nutrition|5": {
"acc": 0.6568627450980392,
"acc_stderr": 0.027184498909941616,
"acc_norm": 0.6568627450980392,
"acc_norm_stderr": 0.027184498909941616
},
"harness|hendrycksTest-philosophy|5": {
"acc": 0.6559485530546624,
"acc_stderr": 0.02698147804364805,
"acc_norm": 0.6559485530546624,
"acc_norm_stderr": 0.02698147804364805
},
"harness|hendrycksTest-prehistory|5": {
"acc": 0.6481481481481481,
"acc_stderr": 0.02657148348071997,
"acc_norm": 0.6481481481481481,
"acc_norm_stderr": 0.02657148348071997
},
"harness|hendrycksTest-professional_accounting|5": {
"acc": 0.4787234042553192,
"acc_stderr": 0.029800481645628693,
"acc_norm": 0.4787234042553192,
"acc_norm_stderr": 0.029800481645628693
},
"harness|hendrycksTest-professional_law|5": {
"acc": 0.4067796610169492,
"acc_stderr": 0.01254632559656954,
"acc_norm": 0.4067796610169492,
"acc_norm_stderr": 0.01254632559656954
},
"harness|hendrycksTest-professional_medicine|5": {
"acc": 0.5735294117647058,
"acc_stderr": 0.030042615832714867,
"acc_norm": 0.5735294117647058,
"acc_norm_stderr": 0.030042615832714867
},
"harness|hendrycksTest-professional_psychology|5": {
"acc": 0.6111111111111112,
"acc_stderr": 0.019722058939618065,
"acc_norm": 0.6111111111111112,
"acc_norm_stderr": 0.019722058939618065
},
"harness|hendrycksTest-public_relations|5": {
"acc": 0.6727272727272727,
"acc_stderr": 0.0449429086625209,
"acc_norm": 0.6727272727272727,
"acc_norm_stderr": 0.0449429086625209
},
"harness|hendrycksTest-security_studies|5": {
"acc": 0.6857142857142857,
"acc_stderr": 0.02971932942241748,
"acc_norm": 0.6857142857142857,
"acc_norm_stderr": 0.02971932942241748
},
"harness|hendrycksTest-sociology|5": {
"acc": 0.8308457711442786,
"acc_stderr": 0.026508590656233257,
"acc_norm": 0.8308457711442786,
"acc_norm_stderr": 0.026508590656233257
},
"harness|hendrycksTest-us_foreign_policy|5": {
"acc": 0.81,
"acc_stderr": 0.03942772444036625,
"acc_norm": 0.81,
"acc_norm_stderr": 0.03942772444036625
},
"harness|hendrycksTest-virology|5": {
"acc": 0.5060240963855421,
"acc_stderr": 0.03892212195333045,
"acc_norm": 0.5060240963855421,
"acc_norm_stderr": 0.03892212195333045
},
"harness|hendrycksTest-world_religions|5": {
"acc": 0.7777777777777778,
"acc_stderr": 0.03188578017686398,
"acc_norm": 0.7777777777777778,
"acc_norm_stderr": 0.03188578017686398
},
"harness|truthfulqa:mc|0": {
"mc1": 0.2937576499388005,
"mc1_stderr": 0.015945068581236614,
"mc2": 0.43044441727793376,
"mc2_stderr": 0.015120468254750151
},
"harness|winogrande|5": {
"acc": 0.7600631412786109,
"acc_stderr": 0.012002078629485739
},
"harness|gsm8k|5": {
"acc": 0.6830932524639879,
"acc_stderr": 0.012815868296721364
}
}
```
## Dataset Details
### Dataset Description
<!-- Provide a longer summary of what this dataset is. -->
- **Curated by:** [More Information Needed]
- **Funded by [optional]:** [More Information Needed]
- **Shared by [optional]:** [More Information Needed]
- **Language(s) (NLP):** [More Information Needed]
- **License:** [More Information Needed]
### Dataset Sources [optional]
<!-- Provide the basic links for the dataset. -->
- **Repository:** [More Information Needed]
- **Paper [optional]:** [More Information Needed]
- **Demo [optional]:** [More Information Needed]
## Uses
<!-- Address questions around how the dataset is intended to be used. -->
### Direct Use
<!-- This section describes suitable use cases for the dataset. -->
[More Information Needed]
### Out-of-Scope Use
<!-- This section addresses misuse, malicious use, and uses that the dataset will not work well for. -->
[More Information Needed]
## Dataset Structure
<!-- This section provides a description of the dataset fields, and additional information about the dataset structure such as criteria used to create the splits, relationships between data points, etc. -->
[More Information Needed]
## Dataset Creation
### Curation Rationale
<!-- Motivation for the creation of this dataset. -->
[More Information Needed]
### Source Data
<!-- This section describes the source data (e.g. news text and headlines, social media posts, translated sentences, ...). -->
#### Data Collection and Processing
<!-- This section describes the data collection and processing process such as data selection criteria, filtering and normalization methods, tools and libraries used, etc. -->
[More Information Needed]
#### Who are the source data producers?
<!-- This section describes the people or systems who originally created the data. It should also include self-reported demographic or identity information for the source data creators if this information is available. -->
[More Information Needed]
### Annotations [optional]
<!-- If the dataset contains annotations which are not part of the initial data collection, use this section to describe them. -->
#### Annotation process
<!-- This section describes the annotation process such as annotation tools used in the process, the amount of data annotated, annotation guidelines provided to the annotators, interannotator statistics, annotation validation, etc. -->
[More Information Needed]
#### Who are the annotators?
<!-- This section describes the people or systems who created the annotations. -->
[More Information Needed]
#### Personal and Sensitive Information
<!-- State whether the dataset contains data that might be considered personal, sensitive, or private (e.g., data that reveals addresses, uniquely identifiable names or aliases, racial or ethnic origins, sexual orientations, religious beliefs, political opinions, financial or health data, etc.). If efforts were made to anonymize the data, describe the anonymization process. -->
[More Information Needed]
## Bias, Risks, and Limitations
<!-- This section is meant to convey both technical and sociotechnical limitations. -->
[More Information Needed]
### Recommendations
<!-- This section is meant to convey recommendations with respect to the bias, risk, and technical limitations. -->
Users should be made aware of the risks, biases and limitations of the dataset. More information needed for further recommendations.
## Citation [optional]
<!-- If there is a paper or blog post introducing the dataset, the APA and Bibtex information for that should go in this section. -->
**BibTeX:**
[More Information Needed]
**APA:**
[More Information Needed]
## Glossary [optional]
<!-- If relevant, include terms and calculations in this section that can help readers understand the dataset or dataset card. -->
[More Information Needed]
## More Information [optional]
[More Information Needed]
## Dataset Card Authors [optional]
[More Information Needed]
## Dataset Card Contact
[More Information Needed] | open-llm-leaderboard/details_abacusai__Fewshot-Metamath-Mistral | [
"region:us"
] | 2024-01-10T15:35:18+00:00 | {"pretty_name": "Evaluation run of abacusai/Fewshot-Metamath-Mistral", "dataset_summary": "Dataset automatically created during the evaluation run of model [abacusai/Fewshot-Metamath-Mistral](https://huggingface.co/abacusai/Fewshot-Metamath-Mistral) on the [Open LLM Leaderboard](https://huggingface.co/spaces/HuggingFaceH4/open_llm_leaderboard).\n\nThe dataset is composed of 63 configuration, each one coresponding to one of the evaluated task.\n\nThe dataset has been created from 1 run(s). Each run can be found as a specific split in each configuration, the split being named using the timestamp of the run.The \"train\" split is always pointing to the latest results.\n\nAn additional configuration \"results\" store all the aggregated results of the run (and is used to compute and display the aggregated metrics on the [Open LLM Leaderboard](https://huggingface.co/spaces/HuggingFaceH4/open_llm_leaderboard)).\n\nTo load the details from a run, you can for instance do the following:\n```python\nfrom datasets import load_dataset\ndata = load_dataset(\"open-llm-leaderboard/details_abacusai__Fewshot-Metamath-Mistral\",\n\t\"harness_winogrande_5\",\n\tsplit=\"train\")\n```\n\n## Latest results\n\nThese are the [latest results from run 2024-01-10T15:33:00.629548](https://huggingface.co/datasets/open-llm-leaderboard/details_abacusai__Fewshot-Metamath-Mistral/blob/main/results_2024-01-10T15-33-00.629548.json)(note that their might be results for other tasks in the repos if successive evals didn't cover the same tasks. You find each in the results and the \"latest\" split for each eval):\n\n```python\n{\n \"all\": {\n \"acc\": 0.5848581335337723,\n \"acc_stderr\": 0.03341837065575973,\n \"acc_norm\": 0.5842626849450804,\n \"acc_norm_stderr\": 0.0341126414564645,\n \"mc1\": 0.2937576499388005,\n \"mc1_stderr\": 0.015945068581236614,\n \"mc2\": 0.43044441727793376,\n \"mc2_stderr\": 0.015120468254750151\n },\n \"harness|arc:challenge|25\": {\n \"acc\": 0.5264505119453925,\n \"acc_stderr\": 0.01459093135812017,\n \"acc_norm\": 0.5776450511945392,\n \"acc_norm_stderr\": 0.014434138713379976\n },\n \"harness|hellaswag|10\": {\n \"acc\": 0.6188010356502689,\n \"acc_stderr\": 0.004846886929763462,\n \"acc_norm\": 0.8059151563433579,\n \"acc_norm_stderr\": 0.0039468624307729535\n },\n \"harness|hendrycksTest-abstract_algebra|5\": {\n \"acc\": 0.28,\n \"acc_stderr\": 0.04512608598542128,\n \"acc_norm\": 0.28,\n \"acc_norm_stderr\": 0.04512608598542128\n },\n \"harness|hendrycksTest-anatomy|5\": {\n \"acc\": 0.5481481481481482,\n \"acc_stderr\": 0.04299268905480864,\n \"acc_norm\": 0.5481481481481482,\n \"acc_norm_stderr\": 0.04299268905480864\n },\n \"harness|hendrycksTest-astronomy|5\": {\n \"acc\": 0.5986842105263158,\n \"acc_stderr\": 0.039889037033362836,\n \"acc_norm\": 0.5986842105263158,\n \"acc_norm_stderr\": 0.039889037033362836\n },\n \"harness|hendrycksTest-business_ethics|5\": {\n \"acc\": 0.51,\n \"acc_stderr\": 0.05024183937956912,\n \"acc_norm\": 0.51,\n \"acc_norm_stderr\": 0.05024183937956912\n },\n \"harness|hendrycksTest-clinical_knowledge|5\": {\n \"acc\": 0.6037735849056604,\n \"acc_stderr\": 0.030102793781791194,\n \"acc_norm\": 0.6037735849056604,\n \"acc_norm_stderr\": 0.030102793781791194\n },\n \"harness|hendrycksTest-college_biology|5\": {\n \"acc\": 0.6527777777777778,\n \"acc_stderr\": 0.039812405437178615,\n \"acc_norm\": 0.6527777777777778,\n \"acc_norm_stderr\": 0.039812405437178615\n },\n \"harness|hendrycksTest-college_chemistry|5\": {\n \"acc\": 0.34,\n \"acc_stderr\": 0.04760952285695236,\n \"acc_norm\": 0.34,\n \"acc_norm_stderr\": 0.04760952285695236\n },\n \"harness|hendrycksTest-college_computer_science|5\": {\n \"acc\": 0.49,\n \"acc_stderr\": 0.05024183937956912,\n \"acc_norm\": 0.49,\n \"acc_norm_stderr\": 0.05024183937956912\n },\n \"harness|hendrycksTest-college_mathematics|5\": {\n \"acc\": 0.32,\n \"acc_stderr\": 0.046882617226215034,\n \"acc_norm\": 0.32,\n \"acc_norm_stderr\": 0.046882617226215034\n },\n \"harness|hendrycksTest-college_medicine|5\": {\n \"acc\": 0.5780346820809249,\n \"acc_stderr\": 0.03765746693865151,\n \"acc_norm\": 0.5780346820809249,\n \"acc_norm_stderr\": 0.03765746693865151\n },\n \"harness|hendrycksTest-college_physics|5\": {\n \"acc\": 0.30392156862745096,\n \"acc_stderr\": 0.04576665403207762,\n \"acc_norm\": 0.30392156862745096,\n \"acc_norm_stderr\": 0.04576665403207762\n },\n \"harness|hendrycksTest-computer_security|5\": {\n \"acc\": 0.72,\n \"acc_stderr\": 0.04512608598542128,\n \"acc_norm\": 0.72,\n \"acc_norm_stderr\": 0.04512608598542128\n },\n \"harness|hendrycksTest-conceptual_physics|5\": {\n \"acc\": 0.4765957446808511,\n \"acc_stderr\": 0.03265019475033582,\n \"acc_norm\": 0.4765957446808511,\n \"acc_norm_stderr\": 0.03265019475033582\n },\n \"harness|hendrycksTest-econometrics|5\": {\n \"acc\": 0.5,\n \"acc_stderr\": 0.047036043419179864,\n \"acc_norm\": 0.5,\n \"acc_norm_stderr\": 0.047036043419179864\n },\n \"harness|hendrycksTest-electrical_engineering|5\": {\n \"acc\": 0.5241379310344828,\n \"acc_stderr\": 0.0416180850350153,\n \"acc_norm\": 0.5241379310344828,\n \"acc_norm_stderr\": 0.0416180850350153\n },\n \"harness|hendrycksTest-elementary_mathematics|5\": {\n \"acc\": 0.3544973544973545,\n \"acc_stderr\": 0.024636830602842,\n \"acc_norm\": 0.3544973544973545,\n \"acc_norm_stderr\": 0.024636830602842\n },\n \"harness|hendrycksTest-formal_logic|5\": {\n \"acc\": 0.42063492063492064,\n \"acc_stderr\": 0.04415438226743744,\n \"acc_norm\": 0.42063492063492064,\n \"acc_norm_stderr\": 0.04415438226743744\n },\n \"harness|hendrycksTest-global_facts|5\": {\n \"acc\": 0.36,\n \"acc_stderr\": 0.04824181513244218,\n \"acc_norm\": 0.36,\n \"acc_norm_stderr\": 0.04824181513244218\n },\n \"harness|hendrycksTest-high_school_biology|5\": {\n \"acc\": 0.7,\n \"acc_stderr\": 0.026069362295335137,\n \"acc_norm\": 0.7,\n \"acc_norm_stderr\": 0.026069362295335137\n },\n \"harness|hendrycksTest-high_school_chemistry|5\": {\n \"acc\": 0.39901477832512317,\n \"acc_stderr\": 0.03445487686264715,\n \"acc_norm\": 0.39901477832512317,\n \"acc_norm_stderr\": 0.03445487686264715\n },\n \"harness|hendrycksTest-high_school_computer_science|5\": {\n \"acc\": 0.54,\n \"acc_stderr\": 0.05009082659620333,\n \"acc_norm\": 0.54,\n \"acc_norm_stderr\": 0.05009082659620333\n },\n \"harness|hendrycksTest-high_school_european_history|5\": {\n \"acc\": 0.7333333333333333,\n \"acc_stderr\": 0.03453131801885417,\n \"acc_norm\": 0.7333333333333333,\n \"acc_norm_stderr\": 0.03453131801885417\n },\n \"harness|hendrycksTest-high_school_geography|5\": {\n \"acc\": 0.7727272727272727,\n \"acc_stderr\": 0.02985751567338642,\n \"acc_norm\": 0.7727272727272727,\n \"acc_norm_stderr\": 0.02985751567338642\n },\n \"harness|hendrycksTest-high_school_government_and_politics|5\": {\n \"acc\": 0.8393782383419689,\n \"acc_stderr\": 0.026499057701397443,\n \"acc_norm\": 0.8393782383419689,\n \"acc_norm_stderr\": 0.026499057701397443\n },\n \"harness|hendrycksTest-high_school_macroeconomics|5\": {\n \"acc\": 0.5641025641025641,\n \"acc_stderr\": 0.02514180151117749,\n \"acc_norm\": 0.5641025641025641,\n \"acc_norm_stderr\": 0.02514180151117749\n },\n \"harness|hendrycksTest-high_school_mathematics|5\": {\n \"acc\": 0.28888888888888886,\n \"acc_stderr\": 0.027634907264178544,\n \"acc_norm\": 0.28888888888888886,\n \"acc_norm_stderr\": 0.027634907264178544\n },\n \"harness|hendrycksTest-high_school_microeconomics|5\": {\n \"acc\": 0.5798319327731093,\n \"acc_stderr\": 0.03206183783236152,\n \"acc_norm\": 0.5798319327731093,\n \"acc_norm_stderr\": 0.03206183783236152\n },\n \"harness|hendrycksTest-high_school_physics|5\": {\n \"acc\": 0.33112582781456956,\n \"acc_stderr\": 0.038425817186598696,\n \"acc_norm\": 0.33112582781456956,\n \"acc_norm_stderr\": 0.038425817186598696\n },\n \"harness|hendrycksTest-high_school_psychology|5\": {\n \"acc\": 0.7577981651376147,\n \"acc_stderr\": 0.018368176306598618,\n \"acc_norm\": 0.7577981651376147,\n \"acc_norm_stderr\": 0.018368176306598618\n },\n \"harness|hendrycksTest-high_school_statistics|5\": {\n \"acc\": 0.39814814814814814,\n \"acc_stderr\": 0.033384734032074016,\n \"acc_norm\": 0.39814814814814814,\n \"acc_norm_stderr\": 0.033384734032074016\n },\n \"harness|hendrycksTest-high_school_us_history|5\": {\n \"acc\": 0.696078431372549,\n \"acc_stderr\": 0.03228210387037892,\n \"acc_norm\": 0.696078431372549,\n \"acc_norm_stderr\": 0.03228210387037892\n },\n \"harness|hendrycksTest-high_school_world_history|5\": {\n \"acc\": 0.7383966244725738,\n \"acc_stderr\": 0.028609516716994934,\n \"acc_norm\": 0.7383966244725738,\n \"acc_norm_stderr\": 0.028609516716994934\n },\n \"harness|hendrycksTest-human_aging|5\": {\n \"acc\": 0.6547085201793722,\n \"acc_stderr\": 0.03191100192835794,\n \"acc_norm\": 0.6547085201793722,\n \"acc_norm_stderr\": 0.03191100192835794\n },\n \"harness|hendrycksTest-human_sexuality|5\": {\n \"acc\": 0.7175572519083969,\n \"acc_stderr\": 0.03948406125768361,\n \"acc_norm\": 0.7175572519083969,\n \"acc_norm_stderr\": 0.03948406125768361\n },\n \"harness|hendrycksTest-international_law|5\": {\n \"acc\": 0.7603305785123967,\n \"acc_stderr\": 0.03896878985070417,\n \"acc_norm\": 0.7603305785123967,\n \"acc_norm_stderr\": 0.03896878985070417\n },\n \"harness|hendrycksTest-jurisprudence|5\": {\n \"acc\": 0.7129629629629629,\n \"acc_stderr\": 0.043733130409147614,\n \"acc_norm\": 0.7129629629629629,\n \"acc_norm_stderr\": 0.043733130409147614\n },\n \"harness|hendrycksTest-logical_fallacies|5\": {\n \"acc\": 0.6748466257668712,\n \"acc_stderr\": 0.036803503712864595,\n \"acc_norm\": 0.6748466257668712,\n \"acc_norm_stderr\": 0.036803503712864595\n },\n \"harness|hendrycksTest-machine_learning|5\": {\n \"acc\": 0.4107142857142857,\n \"acc_stderr\": 0.04669510663875191,\n \"acc_norm\": 0.4107142857142857,\n \"acc_norm_stderr\": 0.04669510663875191\n },\n \"harness|hendrycksTest-management|5\": {\n \"acc\": 0.7281553398058253,\n \"acc_stderr\": 0.044052680241409216,\n \"acc_norm\": 0.7281553398058253,\n \"acc_norm_stderr\": 0.044052680241409216\n },\n \"harness|hendrycksTest-marketing|5\": {\n \"acc\": 0.8333333333333334,\n \"acc_stderr\": 0.02441494730454368,\n \"acc_norm\": 0.8333333333333334,\n \"acc_norm_stderr\": 0.02441494730454368\n },\n \"harness|hendrycksTest-medical_genetics|5\": {\n \"acc\": 0.6,\n \"acc_stderr\": 0.049236596391733084,\n \"acc_norm\": 0.6,\n \"acc_norm_stderr\": 0.049236596391733084\n },\n \"harness|hendrycksTest-miscellaneous|5\": {\n \"acc\": 0.7713920817369093,\n \"acc_stderr\": 0.015016884698539887,\n \"acc_norm\": 0.7713920817369093,\n \"acc_norm_stderr\": 0.015016884698539887\n },\n \"harness|hendrycksTest-moral_disputes|5\": {\n \"acc\": 0.6098265895953757,\n \"acc_stderr\": 0.026261677607806642,\n \"acc_norm\": 0.6098265895953757,\n \"acc_norm_stderr\": 0.026261677607806642\n },\n \"harness|hendrycksTest-moral_scenarios|5\": {\n \"acc\": 0.37988826815642457,\n \"acc_stderr\": 0.016232826818678492,\n \"acc_norm\": 0.37988826815642457,\n \"acc_norm_stderr\": 0.016232826818678492\n },\n \"harness|hendrycksTest-nutrition|5\": {\n \"acc\": 0.6568627450980392,\n \"acc_stderr\": 0.027184498909941616,\n \"acc_norm\": 0.6568627450980392,\n \"acc_norm_stderr\": 0.027184498909941616\n },\n \"harness|hendrycksTest-philosophy|5\": {\n \"acc\": 0.6559485530546624,\n \"acc_stderr\": 0.02698147804364805,\n \"acc_norm\": 0.6559485530546624,\n \"acc_norm_stderr\": 0.02698147804364805\n },\n \"harness|hendrycksTest-prehistory|5\": {\n \"acc\": 0.6481481481481481,\n \"acc_stderr\": 0.02657148348071997,\n \"acc_norm\": 0.6481481481481481,\n \"acc_norm_stderr\": 0.02657148348071997\n },\n \"harness|hendrycksTest-professional_accounting|5\": {\n \"acc\": 0.4787234042553192,\n \"acc_stderr\": 0.029800481645628693,\n \"acc_norm\": 0.4787234042553192,\n \"acc_norm_stderr\": 0.029800481645628693\n },\n \"harness|hendrycksTest-professional_law|5\": {\n \"acc\": 0.4067796610169492,\n \"acc_stderr\": 0.01254632559656954,\n \"acc_norm\": 0.4067796610169492,\n \"acc_norm_stderr\": 0.01254632559656954\n },\n \"harness|hendrycksTest-professional_medicine|5\": {\n \"acc\": 0.5735294117647058,\n \"acc_stderr\": 0.030042615832714867,\n \"acc_norm\": 0.5735294117647058,\n \"acc_norm_stderr\": 0.030042615832714867\n },\n \"harness|hendrycksTest-professional_psychology|5\": {\n \"acc\": 0.6111111111111112,\n \"acc_stderr\": 0.019722058939618065,\n \"acc_norm\": 0.6111111111111112,\n \"acc_norm_stderr\": 0.019722058939618065\n },\n \"harness|hendrycksTest-public_relations|5\": {\n \"acc\": 0.6727272727272727,\n \"acc_stderr\": 0.0449429086625209,\n \"acc_norm\": 0.6727272727272727,\n \"acc_norm_stderr\": 0.0449429086625209\n },\n \"harness|hendrycksTest-security_studies|5\": {\n \"acc\": 0.6857142857142857,\n \"acc_stderr\": 0.02971932942241748,\n \"acc_norm\": 0.6857142857142857,\n \"acc_norm_stderr\": 0.02971932942241748\n },\n \"harness|hendrycksTest-sociology|5\": {\n \"acc\": 0.8308457711442786,\n \"acc_stderr\": 0.026508590656233257,\n \"acc_norm\": 0.8308457711442786,\n \"acc_norm_stderr\": 0.026508590656233257\n },\n \"harness|hendrycksTest-us_foreign_policy|5\": {\n \"acc\": 0.81,\n \"acc_stderr\": 0.03942772444036625,\n \"acc_norm\": 0.81,\n \"acc_norm_stderr\": 0.03942772444036625\n },\n \"harness|hendrycksTest-virology|5\": {\n \"acc\": 0.5060240963855421,\n \"acc_stderr\": 0.03892212195333045,\n \"acc_norm\": 0.5060240963855421,\n \"acc_norm_stderr\": 0.03892212195333045\n },\n \"harness|hendrycksTest-world_religions|5\": {\n \"acc\": 0.7777777777777778,\n \"acc_stderr\": 0.03188578017686398,\n \"acc_norm\": 0.7777777777777778,\n \"acc_norm_stderr\": 0.03188578017686398\n },\n \"harness|truthfulqa:mc|0\": {\n \"mc1\": 0.2937576499388005,\n \"mc1_stderr\": 0.015945068581236614,\n \"mc2\": 0.43044441727793376,\n \"mc2_stderr\": 0.015120468254750151\n },\n \"harness|winogrande|5\": {\n \"acc\": 0.7600631412786109,\n \"acc_stderr\": 0.012002078629485739\n },\n \"harness|gsm8k|5\": {\n \"acc\": 0.6830932524639879,\n \"acc_stderr\": 0.012815868296721364\n }\n}\n```", "repo_url": "https://huggingface.co/abacusai/Fewshot-Metamath-Mistral", "leaderboard_url": "https://huggingface.co/spaces/HuggingFaceH4/open_llm_leaderboard", "point_of_contact": "[email protected]", "configs": [{"config_name": "harness_arc_challenge_25", "data_files": [{"split": "2024_01_10T15_33_00.629548", "path": ["**/details_harness|arc:challenge|25_2024-01-10T15-33-00.629548.parquet"]}, {"split": "latest", "path": ["**/details_harness|arc:challenge|25_2024-01-10T15-33-00.629548.parquet"]}]}, {"config_name": "harness_gsm8k_5", "data_files": [{"split": "2024_01_10T15_33_00.629548", "path": ["**/details_harness|gsm8k|5_2024-01-10T15-33-00.629548.parquet"]}, {"split": "latest", "path": ["**/details_harness|gsm8k|5_2024-01-10T15-33-00.629548.parquet"]}]}, {"config_name": "harness_hellaswag_10", "data_files": [{"split": "2024_01_10T15_33_00.629548", "path": ["**/details_harness|hellaswag|10_2024-01-10T15-33-00.629548.parquet"]}, {"split": "latest", "path": ["**/details_harness|hellaswag|10_2024-01-10T15-33-00.629548.parquet"]}]}, {"config_name": "harness_hendrycksTest_5", "data_files": [{"split": "2024_01_10T15_33_00.629548", "path": ["**/details_harness|hendrycksTest-abstract_algebra|5_2024-01-10T15-33-00.629548.parquet", "**/details_harness|hendrycksTest-anatomy|5_2024-01-10T15-33-00.629548.parquet", "**/details_harness|hendrycksTest-astronomy|5_2024-01-10T15-33-00.629548.parquet", "**/details_harness|hendrycksTest-business_ethics|5_2024-01-10T15-33-00.629548.parquet", "**/details_harness|hendrycksTest-clinical_knowledge|5_2024-01-10T15-33-00.629548.parquet", "**/details_harness|hendrycksTest-college_biology|5_2024-01-10T15-33-00.629548.parquet", "**/details_harness|hendrycksTest-college_chemistry|5_2024-01-10T15-33-00.629548.parquet", "**/details_harness|hendrycksTest-college_computer_science|5_2024-01-10T15-33-00.629548.parquet", "**/details_harness|hendrycksTest-college_mathematics|5_2024-01-10T15-33-00.629548.parquet", "**/details_harness|hendrycksTest-college_medicine|5_2024-01-10T15-33-00.629548.parquet", "**/details_harness|hendrycksTest-college_physics|5_2024-01-10T15-33-00.629548.parquet", "**/details_harness|hendrycksTest-computer_security|5_2024-01-10T15-33-00.629548.parquet", "**/details_harness|hendrycksTest-conceptual_physics|5_2024-01-10T15-33-00.629548.parquet", "**/details_harness|hendrycksTest-econometrics|5_2024-01-10T15-33-00.629548.parquet", "**/details_harness|hendrycksTest-electrical_engineering|5_2024-01-10T15-33-00.629548.parquet", "**/details_harness|hendrycksTest-elementary_mathematics|5_2024-01-10T15-33-00.629548.parquet", "**/details_harness|hendrycksTest-formal_logic|5_2024-01-10T15-33-00.629548.parquet", "**/details_harness|hendrycksTest-global_facts|5_2024-01-10T15-33-00.629548.parquet", "**/details_harness|hendrycksTest-high_school_biology|5_2024-01-10T15-33-00.629548.parquet", "**/details_harness|hendrycksTest-high_school_chemistry|5_2024-01-10T15-33-00.629548.parquet", "**/details_harness|hendrycksTest-high_school_computer_science|5_2024-01-10T15-33-00.629548.parquet", "**/details_harness|hendrycksTest-high_school_european_history|5_2024-01-10T15-33-00.629548.parquet", "**/details_harness|hendrycksTest-high_school_geography|5_2024-01-10T15-33-00.629548.parquet", "**/details_harness|hendrycksTest-high_school_government_and_politics|5_2024-01-10T15-33-00.629548.parquet", "**/details_harness|hendrycksTest-high_school_macroeconomics|5_2024-01-10T15-33-00.629548.parquet", "**/details_harness|hendrycksTest-high_school_mathematics|5_2024-01-10T15-33-00.629548.parquet", "**/details_harness|hendrycksTest-high_school_microeconomics|5_2024-01-10T15-33-00.629548.parquet", "**/details_harness|hendrycksTest-high_school_physics|5_2024-01-10T15-33-00.629548.parquet", "**/details_harness|hendrycksTest-high_school_psychology|5_2024-01-10T15-33-00.629548.parquet", "**/details_harness|hendrycksTest-high_school_statistics|5_2024-01-10T15-33-00.629548.parquet", "**/details_harness|hendrycksTest-high_school_us_history|5_2024-01-10T15-33-00.629548.parquet", "**/details_harness|hendrycksTest-high_school_world_history|5_2024-01-10T15-33-00.629548.parquet", "**/details_harness|hendrycksTest-human_aging|5_2024-01-10T15-33-00.629548.parquet", "**/details_harness|hendrycksTest-human_sexuality|5_2024-01-10T15-33-00.629548.parquet", "**/details_harness|hendrycksTest-international_law|5_2024-01-10T15-33-00.629548.parquet", "**/details_harness|hendrycksTest-jurisprudence|5_2024-01-10T15-33-00.629548.parquet", "**/details_harness|hendrycksTest-logical_fallacies|5_2024-01-10T15-33-00.629548.parquet", "**/details_harness|hendrycksTest-machine_learning|5_2024-01-10T15-33-00.629548.parquet", "**/details_harness|hendrycksTest-management|5_2024-01-10T15-33-00.629548.parquet", "**/details_harness|hendrycksTest-marketing|5_2024-01-10T15-33-00.629548.parquet", "**/details_harness|hendrycksTest-medical_genetics|5_2024-01-10T15-33-00.629548.parquet", "**/details_harness|hendrycksTest-miscellaneous|5_2024-01-10T15-33-00.629548.parquet", "**/details_harness|hendrycksTest-moral_disputes|5_2024-01-10T15-33-00.629548.parquet", "**/details_harness|hendrycksTest-moral_scenarios|5_2024-01-10T15-33-00.629548.parquet", "**/details_harness|hendrycksTest-nutrition|5_2024-01-10T15-33-00.629548.parquet", "**/details_harness|hendrycksTest-philosophy|5_2024-01-10T15-33-00.629548.parquet", "**/details_harness|hendrycksTest-prehistory|5_2024-01-10T15-33-00.629548.parquet", "**/details_harness|hendrycksTest-professional_accounting|5_2024-01-10T15-33-00.629548.parquet", "**/details_harness|hendrycksTest-professional_law|5_2024-01-10T15-33-00.629548.parquet", "**/details_harness|hendrycksTest-professional_medicine|5_2024-01-10T15-33-00.629548.parquet", "**/details_harness|hendrycksTest-professional_psychology|5_2024-01-10T15-33-00.629548.parquet", "**/details_harness|hendrycksTest-public_relations|5_2024-01-10T15-33-00.629548.parquet", "**/details_harness|hendrycksTest-security_studies|5_2024-01-10T15-33-00.629548.parquet", "**/details_harness|hendrycksTest-sociology|5_2024-01-10T15-33-00.629548.parquet", "**/details_harness|hendrycksTest-us_foreign_policy|5_2024-01-10T15-33-00.629548.parquet", "**/details_harness|hendrycksTest-virology|5_2024-01-10T15-33-00.629548.parquet", "**/details_harness|hendrycksTest-world_religions|5_2024-01-10T15-33-00.629548.parquet"]}, {"split": "latest", "path": ["**/details_harness|hendrycksTest-abstract_algebra|5_2024-01-10T15-33-00.629548.parquet", "**/details_harness|hendrycksTest-anatomy|5_2024-01-10T15-33-00.629548.parquet", "**/details_harness|hendrycksTest-astronomy|5_2024-01-10T15-33-00.629548.parquet", "**/details_harness|hendrycksTest-business_ethics|5_2024-01-10T15-33-00.629548.parquet", "**/details_harness|hendrycksTest-clinical_knowledge|5_2024-01-10T15-33-00.629548.parquet", "**/details_harness|hendrycksTest-college_biology|5_2024-01-10T15-33-00.629548.parquet", "**/details_harness|hendrycksTest-college_chemistry|5_2024-01-10T15-33-00.629548.parquet", "**/details_harness|hendrycksTest-college_computer_science|5_2024-01-10T15-33-00.629548.parquet", "**/details_harness|hendrycksTest-college_mathematics|5_2024-01-10T15-33-00.629548.parquet", "**/details_harness|hendrycksTest-college_medicine|5_2024-01-10T15-33-00.629548.parquet", "**/details_harness|hendrycksTest-college_physics|5_2024-01-10T15-33-00.629548.parquet", "**/details_harness|hendrycksTest-computer_security|5_2024-01-10T15-33-00.629548.parquet", "**/details_harness|hendrycksTest-conceptual_physics|5_2024-01-10T15-33-00.629548.parquet", "**/details_harness|hendrycksTest-econometrics|5_2024-01-10T15-33-00.629548.parquet", "**/details_harness|hendrycksTest-electrical_engineering|5_2024-01-10T15-33-00.629548.parquet", "**/details_harness|hendrycksTest-elementary_mathematics|5_2024-01-10T15-33-00.629548.parquet", "**/details_harness|hendrycksTest-formal_logic|5_2024-01-10T15-33-00.629548.parquet", "**/details_harness|hendrycksTest-global_facts|5_2024-01-10T15-33-00.629548.parquet", "**/details_harness|hendrycksTest-high_school_biology|5_2024-01-10T15-33-00.629548.parquet", "**/details_harness|hendrycksTest-high_school_chemistry|5_2024-01-10T15-33-00.629548.parquet", "**/details_harness|hendrycksTest-high_school_computer_science|5_2024-01-10T15-33-00.629548.parquet", "**/details_harness|hendrycksTest-high_school_european_history|5_2024-01-10T15-33-00.629548.parquet", "**/details_harness|hendrycksTest-high_school_geography|5_2024-01-10T15-33-00.629548.parquet", "**/details_harness|hendrycksTest-high_school_government_and_politics|5_2024-01-10T15-33-00.629548.parquet", "**/details_harness|hendrycksTest-high_school_macroeconomics|5_2024-01-10T15-33-00.629548.parquet", "**/details_harness|hendrycksTest-high_school_mathematics|5_2024-01-10T15-33-00.629548.parquet", "**/details_harness|hendrycksTest-high_school_microeconomics|5_2024-01-10T15-33-00.629548.parquet", "**/details_harness|hendrycksTest-high_school_physics|5_2024-01-10T15-33-00.629548.parquet", "**/details_harness|hendrycksTest-high_school_psychology|5_2024-01-10T15-33-00.629548.parquet", "**/details_harness|hendrycksTest-high_school_statistics|5_2024-01-10T15-33-00.629548.parquet", "**/details_harness|hendrycksTest-high_school_us_history|5_2024-01-10T15-33-00.629548.parquet", "**/details_harness|hendrycksTest-high_school_world_history|5_2024-01-10T15-33-00.629548.parquet", "**/details_harness|hendrycksTest-human_aging|5_2024-01-10T15-33-00.629548.parquet", "**/details_harness|hendrycksTest-human_sexuality|5_2024-01-10T15-33-00.629548.parquet", "**/details_harness|hendrycksTest-international_law|5_2024-01-10T15-33-00.629548.parquet", "**/details_harness|hendrycksTest-jurisprudence|5_2024-01-10T15-33-00.629548.parquet", "**/details_harness|hendrycksTest-logical_fallacies|5_2024-01-10T15-33-00.629548.parquet", "**/details_harness|hendrycksTest-machine_learning|5_2024-01-10T15-33-00.629548.parquet", "**/details_harness|hendrycksTest-management|5_2024-01-10T15-33-00.629548.parquet", "**/details_harness|hendrycksTest-marketing|5_2024-01-10T15-33-00.629548.parquet", "**/details_harness|hendrycksTest-medical_genetics|5_2024-01-10T15-33-00.629548.parquet", "**/details_harness|hendrycksTest-miscellaneous|5_2024-01-10T15-33-00.629548.parquet", "**/details_harness|hendrycksTest-moral_disputes|5_2024-01-10T15-33-00.629548.parquet", "**/details_harness|hendrycksTest-moral_scenarios|5_2024-01-10T15-33-00.629548.parquet", "**/details_harness|hendrycksTest-nutrition|5_2024-01-10T15-33-00.629548.parquet", "**/details_harness|hendrycksTest-philosophy|5_2024-01-10T15-33-00.629548.parquet", "**/details_harness|hendrycksTest-prehistory|5_2024-01-10T15-33-00.629548.parquet", "**/details_harness|hendrycksTest-professional_accounting|5_2024-01-10T15-33-00.629548.parquet", "**/details_harness|hendrycksTest-professional_law|5_2024-01-10T15-33-00.629548.parquet", "**/details_harness|hendrycksTest-professional_medicine|5_2024-01-10T15-33-00.629548.parquet", "**/details_harness|hendrycksTest-professional_psychology|5_2024-01-10T15-33-00.629548.parquet", "**/details_harness|hendrycksTest-public_relations|5_2024-01-10T15-33-00.629548.parquet", "**/details_harness|hendrycksTest-security_studies|5_2024-01-10T15-33-00.629548.parquet", "**/details_harness|hendrycksTest-sociology|5_2024-01-10T15-33-00.629548.parquet", "**/details_harness|hendrycksTest-us_foreign_policy|5_2024-01-10T15-33-00.629548.parquet", "**/details_harness|hendrycksTest-virology|5_2024-01-10T15-33-00.629548.parquet", "**/details_harness|hendrycksTest-world_religions|5_2024-01-10T15-33-00.629548.parquet"]}]}, {"config_name": "harness_hendrycksTest_abstract_algebra_5", "data_files": [{"split": "2024_01_10T15_33_00.629548", "path": ["**/details_harness|hendrycksTest-abstract_algebra|5_2024-01-10T15-33-00.629548.parquet"]}, {"split": "latest", "path": ["**/details_harness|hendrycksTest-abstract_algebra|5_2024-01-10T15-33-00.629548.parquet"]}]}, {"config_name": "harness_hendrycksTest_anatomy_5", "data_files": [{"split": "2024_01_10T15_33_00.629548", "path": ["**/details_harness|hendrycksTest-anatomy|5_2024-01-10T15-33-00.629548.parquet"]}, {"split": "latest", "path": ["**/details_harness|hendrycksTest-anatomy|5_2024-01-10T15-33-00.629548.parquet"]}]}, {"config_name": "harness_hendrycksTest_astronomy_5", "data_files": [{"split": "2024_01_10T15_33_00.629548", "path": ["**/details_harness|hendrycksTest-astronomy|5_2024-01-10T15-33-00.629548.parquet"]}, {"split": "latest", "path": ["**/details_harness|hendrycksTest-astronomy|5_2024-01-10T15-33-00.629548.parquet"]}]}, {"config_name": "harness_hendrycksTest_business_ethics_5", "data_files": [{"split": "2024_01_10T15_33_00.629548", "path": ["**/details_harness|hendrycksTest-business_ethics|5_2024-01-10T15-33-00.629548.parquet"]}, {"split": "latest", "path": ["**/details_harness|hendrycksTest-business_ethics|5_2024-01-10T15-33-00.629548.parquet"]}]}, {"config_name": "harness_hendrycksTest_clinical_knowledge_5", "data_files": [{"split": "2024_01_10T15_33_00.629548", "path": ["**/details_harness|hendrycksTest-clinical_knowledge|5_2024-01-10T15-33-00.629548.parquet"]}, {"split": "latest", "path": ["**/details_harness|hendrycksTest-clinical_knowledge|5_2024-01-10T15-33-00.629548.parquet"]}]}, {"config_name": "harness_hendrycksTest_college_biology_5", "data_files": [{"split": "2024_01_10T15_33_00.629548", "path": ["**/details_harness|hendrycksTest-college_biology|5_2024-01-10T15-33-00.629548.parquet"]}, {"split": "latest", "path": ["**/details_harness|hendrycksTest-college_biology|5_2024-01-10T15-33-00.629548.parquet"]}]}, {"config_name": "harness_hendrycksTest_college_chemistry_5", "data_files": [{"split": "2024_01_10T15_33_00.629548", "path": ["**/details_harness|hendrycksTest-college_chemistry|5_2024-01-10T15-33-00.629548.parquet"]}, {"split": "latest", "path": ["**/details_harness|hendrycksTest-college_chemistry|5_2024-01-10T15-33-00.629548.parquet"]}]}, {"config_name": "harness_hendrycksTest_college_computer_science_5", "data_files": [{"split": "2024_01_10T15_33_00.629548", "path": ["**/details_harness|hendrycksTest-college_computer_science|5_2024-01-10T15-33-00.629548.parquet"]}, {"split": "latest", "path": ["**/details_harness|hendrycksTest-college_computer_science|5_2024-01-10T15-33-00.629548.parquet"]}]}, {"config_name": "harness_hendrycksTest_college_mathematics_5", "data_files": [{"split": "2024_01_10T15_33_00.629548", "path": ["**/details_harness|hendrycksTest-college_mathematics|5_2024-01-10T15-33-00.629548.parquet"]}, {"split": "latest", "path": ["**/details_harness|hendrycksTest-college_mathematics|5_2024-01-10T15-33-00.629548.parquet"]}]}, {"config_name": "harness_hendrycksTest_college_medicine_5", "data_files": [{"split": "2024_01_10T15_33_00.629548", "path": ["**/details_harness|hendrycksTest-college_medicine|5_2024-01-10T15-33-00.629548.parquet"]}, {"split": "latest", "path": ["**/details_harness|hendrycksTest-college_medicine|5_2024-01-10T15-33-00.629548.parquet"]}]}, {"config_name": "harness_hendrycksTest_college_physics_5", "data_files": [{"split": "2024_01_10T15_33_00.629548", "path": ["**/details_harness|hendrycksTest-college_physics|5_2024-01-10T15-33-00.629548.parquet"]}, {"split": "latest", "path": ["**/details_harness|hendrycksTest-college_physics|5_2024-01-10T15-33-00.629548.parquet"]}]}, {"config_name": "harness_hendrycksTest_computer_security_5", "data_files": [{"split": "2024_01_10T15_33_00.629548", "path": ["**/details_harness|hendrycksTest-computer_security|5_2024-01-10T15-33-00.629548.parquet"]}, {"split": "latest", "path": ["**/details_harness|hendrycksTest-computer_security|5_2024-01-10T15-33-00.629548.parquet"]}]}, {"config_name": "harness_hendrycksTest_conceptual_physics_5", "data_files": [{"split": "2024_01_10T15_33_00.629548", "path": ["**/details_harness|hendrycksTest-conceptual_physics|5_2024-01-10T15-33-00.629548.parquet"]}, {"split": "latest", "path": ["**/details_harness|hendrycksTest-conceptual_physics|5_2024-01-10T15-33-00.629548.parquet"]}]}, {"config_name": "harness_hendrycksTest_econometrics_5", "data_files": [{"split": "2024_01_10T15_33_00.629548", "path": ["**/details_harness|hendrycksTest-econometrics|5_2024-01-10T15-33-00.629548.parquet"]}, {"split": "latest", "path": ["**/details_harness|hendrycksTest-econometrics|5_2024-01-10T15-33-00.629548.parquet"]}]}, {"config_name": "harness_hendrycksTest_electrical_engineering_5", "data_files": [{"split": "2024_01_10T15_33_00.629548", "path": ["**/details_harness|hendrycksTest-electrical_engineering|5_2024-01-10T15-33-00.629548.parquet"]}, {"split": "latest", "path": ["**/details_harness|hendrycksTest-electrical_engineering|5_2024-01-10T15-33-00.629548.parquet"]}]}, {"config_name": "harness_hendrycksTest_elementary_mathematics_5", "data_files": [{"split": "2024_01_10T15_33_00.629548", "path": ["**/details_harness|hendrycksTest-elementary_mathematics|5_2024-01-10T15-33-00.629548.parquet"]}, {"split": "latest", "path": ["**/details_harness|hendrycksTest-elementary_mathematics|5_2024-01-10T15-33-00.629548.parquet"]}]}, {"config_name": "harness_hendrycksTest_formal_logic_5", "data_files": [{"split": "2024_01_10T15_33_00.629548", "path": ["**/details_harness|hendrycksTest-formal_logic|5_2024-01-10T15-33-00.629548.parquet"]}, {"split": "latest", "path": ["**/details_harness|hendrycksTest-formal_logic|5_2024-01-10T15-33-00.629548.parquet"]}]}, {"config_name": "harness_hendrycksTest_global_facts_5", "data_files": [{"split": "2024_01_10T15_33_00.629548", "path": ["**/details_harness|hendrycksTest-global_facts|5_2024-01-10T15-33-00.629548.parquet"]}, {"split": "latest", "path": ["**/details_harness|hendrycksTest-global_facts|5_2024-01-10T15-33-00.629548.parquet"]}]}, {"config_name": "harness_hendrycksTest_high_school_biology_5", "data_files": [{"split": "2024_01_10T15_33_00.629548", "path": ["**/details_harness|hendrycksTest-high_school_biology|5_2024-01-10T15-33-00.629548.parquet"]}, {"split": "latest", "path": ["**/details_harness|hendrycksTest-high_school_biology|5_2024-01-10T15-33-00.629548.parquet"]}]}, {"config_name": "harness_hendrycksTest_high_school_chemistry_5", "data_files": [{"split": "2024_01_10T15_33_00.629548", "path": ["**/details_harness|hendrycksTest-high_school_chemistry|5_2024-01-10T15-33-00.629548.parquet"]}, {"split": "latest", "path": ["**/details_harness|hendrycksTest-high_school_chemistry|5_2024-01-10T15-33-00.629548.parquet"]}]}, {"config_name": "harness_hendrycksTest_high_school_computer_science_5", "data_files": [{"split": "2024_01_10T15_33_00.629548", "path": ["**/details_harness|hendrycksTest-high_school_computer_science|5_2024-01-10T15-33-00.629548.parquet"]}, {"split": "latest", "path": ["**/details_harness|hendrycksTest-high_school_computer_science|5_2024-01-10T15-33-00.629548.parquet"]}]}, {"config_name": "harness_hendrycksTest_high_school_european_history_5", "data_files": [{"split": "2024_01_10T15_33_00.629548", "path": ["**/details_harness|hendrycksTest-high_school_european_history|5_2024-01-10T15-33-00.629548.parquet"]}, {"split": "latest", "path": ["**/details_harness|hendrycksTest-high_school_european_history|5_2024-01-10T15-33-00.629548.parquet"]}]}, {"config_name": "harness_hendrycksTest_high_school_geography_5", "data_files": [{"split": "2024_01_10T15_33_00.629548", "path": ["**/details_harness|hendrycksTest-high_school_geography|5_2024-01-10T15-33-00.629548.parquet"]}, {"split": "latest", "path": ["**/details_harness|hendrycksTest-high_school_geography|5_2024-01-10T15-33-00.629548.parquet"]}]}, {"config_name": "harness_hendrycksTest_high_school_government_and_politics_5", "data_files": [{"split": "2024_01_10T15_33_00.629548", "path": ["**/details_harness|hendrycksTest-high_school_government_and_politics|5_2024-01-10T15-33-00.629548.parquet"]}, {"split": "latest", "path": ["**/details_harness|hendrycksTest-high_school_government_and_politics|5_2024-01-10T15-33-00.629548.parquet"]}]}, {"config_name": "harness_hendrycksTest_high_school_macroeconomics_5", "data_files": [{"split": "2024_01_10T15_33_00.629548", "path": ["**/details_harness|hendrycksTest-high_school_macroeconomics|5_2024-01-10T15-33-00.629548.parquet"]}, {"split": "latest", "path": ["**/details_harness|hendrycksTest-high_school_macroeconomics|5_2024-01-10T15-33-00.629548.parquet"]}]}, {"config_name": "harness_hendrycksTest_high_school_mathematics_5", "data_files": [{"split": "2024_01_10T15_33_00.629548", "path": ["**/details_harness|hendrycksTest-high_school_mathematics|5_2024-01-10T15-33-00.629548.parquet"]}, {"split": "latest", "path": ["**/details_harness|hendrycksTest-high_school_mathematics|5_2024-01-10T15-33-00.629548.parquet"]}]}, {"config_name": "harness_hendrycksTest_high_school_microeconomics_5", "data_files": [{"split": "2024_01_10T15_33_00.629548", "path": ["**/details_harness|hendrycksTest-high_school_microeconomics|5_2024-01-10T15-33-00.629548.parquet"]}, {"split": "latest", "path": ["**/details_harness|hendrycksTest-high_school_microeconomics|5_2024-01-10T15-33-00.629548.parquet"]}]}, {"config_name": "harness_hendrycksTest_high_school_physics_5", "data_files": [{"split": "2024_01_10T15_33_00.629548", "path": ["**/details_harness|hendrycksTest-high_school_physics|5_2024-01-10T15-33-00.629548.parquet"]}, {"split": "latest", "path": ["**/details_harness|hendrycksTest-high_school_physics|5_2024-01-10T15-33-00.629548.parquet"]}]}, {"config_name": "harness_hendrycksTest_high_school_psychology_5", "data_files": [{"split": "2024_01_10T15_33_00.629548", "path": ["**/details_harness|hendrycksTest-high_school_psychology|5_2024-01-10T15-33-00.629548.parquet"]}, {"split": "latest", "path": ["**/details_harness|hendrycksTest-high_school_psychology|5_2024-01-10T15-33-00.629548.parquet"]}]}, {"config_name": "harness_hendrycksTest_high_school_statistics_5", "data_files": [{"split": "2024_01_10T15_33_00.629548", "path": ["**/details_harness|hendrycksTest-high_school_statistics|5_2024-01-10T15-33-00.629548.parquet"]}, {"split": "latest", "path": ["**/details_harness|hendrycksTest-high_school_statistics|5_2024-01-10T15-33-00.629548.parquet"]}]}, {"config_name": "harness_hendrycksTest_high_school_us_history_5", "data_files": [{"split": "2024_01_10T15_33_00.629548", "path": ["**/details_harness|hendrycksTest-high_school_us_history|5_2024-01-10T15-33-00.629548.parquet"]}, {"split": "latest", "path": ["**/details_harness|hendrycksTest-high_school_us_history|5_2024-01-10T15-33-00.629548.parquet"]}]}, {"config_name": "harness_hendrycksTest_high_school_world_history_5", "data_files": [{"split": "2024_01_10T15_33_00.629548", "path": ["**/details_harness|hendrycksTest-high_school_world_history|5_2024-01-10T15-33-00.629548.parquet"]}, {"split": "latest", "path": ["**/details_harness|hendrycksTest-high_school_world_history|5_2024-01-10T15-33-00.629548.parquet"]}]}, {"config_name": "harness_hendrycksTest_human_aging_5", "data_files": [{"split": "2024_01_10T15_33_00.629548", "path": ["**/details_harness|hendrycksTest-human_aging|5_2024-01-10T15-33-00.629548.parquet"]}, {"split": "latest", "path": ["**/details_harness|hendrycksTest-human_aging|5_2024-01-10T15-33-00.629548.parquet"]}]}, {"config_name": "harness_hendrycksTest_human_sexuality_5", "data_files": [{"split": "2024_01_10T15_33_00.629548", "path": ["**/details_harness|hendrycksTest-human_sexuality|5_2024-01-10T15-33-00.629548.parquet"]}, {"split": "latest", "path": ["**/details_harness|hendrycksTest-human_sexuality|5_2024-01-10T15-33-00.629548.parquet"]}]}, {"config_name": "harness_hendrycksTest_international_law_5", "data_files": [{"split": "2024_01_10T15_33_00.629548", "path": ["**/details_harness|hendrycksTest-international_law|5_2024-01-10T15-33-00.629548.parquet"]}, {"split": "latest", "path": ["**/details_harness|hendrycksTest-international_law|5_2024-01-10T15-33-00.629548.parquet"]}]}, {"config_name": "harness_hendrycksTest_jurisprudence_5", "data_files": [{"split": "2024_01_10T15_33_00.629548", "path": ["**/details_harness|hendrycksTest-jurisprudence|5_2024-01-10T15-33-00.629548.parquet"]}, {"split": "latest", "path": ["**/details_harness|hendrycksTest-jurisprudence|5_2024-01-10T15-33-00.629548.parquet"]}]}, {"config_name": "harness_hendrycksTest_logical_fallacies_5", "data_files": [{"split": "2024_01_10T15_33_00.629548", "path": ["**/details_harness|hendrycksTest-logical_fallacies|5_2024-01-10T15-33-00.629548.parquet"]}, {"split": "latest", "path": ["**/details_harness|hendrycksTest-logical_fallacies|5_2024-01-10T15-33-00.629548.parquet"]}]}, {"config_name": "harness_hendrycksTest_machine_learning_5", "data_files": [{"split": "2024_01_10T15_33_00.629548", "path": ["**/details_harness|hendrycksTest-machine_learning|5_2024-01-10T15-33-00.629548.parquet"]}, {"split": "latest", "path": ["**/details_harness|hendrycksTest-machine_learning|5_2024-01-10T15-33-00.629548.parquet"]}]}, {"config_name": "harness_hendrycksTest_management_5", "data_files": [{"split": "2024_01_10T15_33_00.629548", "path": ["**/details_harness|hendrycksTest-management|5_2024-01-10T15-33-00.629548.parquet"]}, {"split": "latest", "path": ["**/details_harness|hendrycksTest-management|5_2024-01-10T15-33-00.629548.parquet"]}]}, {"config_name": "harness_hendrycksTest_marketing_5", "data_files": [{"split": "2024_01_10T15_33_00.629548", "path": ["**/details_harness|hendrycksTest-marketing|5_2024-01-10T15-33-00.629548.parquet"]}, {"split": "latest", "path": ["**/details_harness|hendrycksTest-marketing|5_2024-01-10T15-33-00.629548.parquet"]}]}, {"config_name": "harness_hendrycksTest_medical_genetics_5", "data_files": [{"split": "2024_01_10T15_33_00.629548", "path": ["**/details_harness|hendrycksTest-medical_genetics|5_2024-01-10T15-33-00.629548.parquet"]}, {"split": "latest", "path": ["**/details_harness|hendrycksTest-medical_genetics|5_2024-01-10T15-33-00.629548.parquet"]}]}, {"config_name": "harness_hendrycksTest_miscellaneous_5", "data_files": [{"split": "2024_01_10T15_33_00.629548", "path": ["**/details_harness|hendrycksTest-miscellaneous|5_2024-01-10T15-33-00.629548.parquet"]}, {"split": "latest", "path": ["**/details_harness|hendrycksTest-miscellaneous|5_2024-01-10T15-33-00.629548.parquet"]}]}, {"config_name": "harness_hendrycksTest_moral_disputes_5", "data_files": [{"split": "2024_01_10T15_33_00.629548", "path": ["**/details_harness|hendrycksTest-moral_disputes|5_2024-01-10T15-33-00.629548.parquet"]}, {"split": "latest", "path": ["**/details_harness|hendrycksTest-moral_disputes|5_2024-01-10T15-33-00.629548.parquet"]}]}, {"config_name": "harness_hendrycksTest_moral_scenarios_5", "data_files": [{"split": "2024_01_10T15_33_00.629548", "path": ["**/details_harness|hendrycksTest-moral_scenarios|5_2024-01-10T15-33-00.629548.parquet"]}, {"split": "latest", "path": ["**/details_harness|hendrycksTest-moral_scenarios|5_2024-01-10T15-33-00.629548.parquet"]}]}, {"config_name": "harness_hendrycksTest_nutrition_5", "data_files": [{"split": "2024_01_10T15_33_00.629548", "path": ["**/details_harness|hendrycksTest-nutrition|5_2024-01-10T15-33-00.629548.parquet"]}, {"split": "latest", "path": ["**/details_harness|hendrycksTest-nutrition|5_2024-01-10T15-33-00.629548.parquet"]}]}, {"config_name": "harness_hendrycksTest_philosophy_5", "data_files": [{"split": "2024_01_10T15_33_00.629548", "path": ["**/details_harness|hendrycksTest-philosophy|5_2024-01-10T15-33-00.629548.parquet"]}, {"split": "latest", "path": ["**/details_harness|hendrycksTest-philosophy|5_2024-01-10T15-33-00.629548.parquet"]}]}, {"config_name": "harness_hendrycksTest_prehistory_5", "data_files": [{"split": "2024_01_10T15_33_00.629548", "path": ["**/details_harness|hendrycksTest-prehistory|5_2024-01-10T15-33-00.629548.parquet"]}, {"split": "latest", "path": ["**/details_harness|hendrycksTest-prehistory|5_2024-01-10T15-33-00.629548.parquet"]}]}, {"config_name": "harness_hendrycksTest_professional_accounting_5", "data_files": [{"split": "2024_01_10T15_33_00.629548", "path": ["**/details_harness|hendrycksTest-professional_accounting|5_2024-01-10T15-33-00.629548.parquet"]}, {"split": "latest", "path": ["**/details_harness|hendrycksTest-professional_accounting|5_2024-01-10T15-33-00.629548.parquet"]}]}, {"config_name": "harness_hendrycksTest_professional_law_5", "data_files": [{"split": "2024_01_10T15_33_00.629548", "path": ["**/details_harness|hendrycksTest-professional_law|5_2024-01-10T15-33-00.629548.parquet"]}, {"split": "latest", "path": ["**/details_harness|hendrycksTest-professional_law|5_2024-01-10T15-33-00.629548.parquet"]}]}, {"config_name": "harness_hendrycksTest_professional_medicine_5", "data_files": [{"split": "2024_01_10T15_33_00.629548", "path": ["**/details_harness|hendrycksTest-professional_medicine|5_2024-01-10T15-33-00.629548.parquet"]}, {"split": "latest", "path": ["**/details_harness|hendrycksTest-professional_medicine|5_2024-01-10T15-33-00.629548.parquet"]}]}, {"config_name": "harness_hendrycksTest_professional_psychology_5", "data_files": [{"split": "2024_01_10T15_33_00.629548", "path": ["**/details_harness|hendrycksTest-professional_psychology|5_2024-01-10T15-33-00.629548.parquet"]}, {"split": "latest", "path": ["**/details_harness|hendrycksTest-professional_psychology|5_2024-01-10T15-33-00.629548.parquet"]}]}, {"config_name": "harness_hendrycksTest_public_relations_5", "data_files": [{"split": "2024_01_10T15_33_00.629548", "path": ["**/details_harness|hendrycksTest-public_relations|5_2024-01-10T15-33-00.629548.parquet"]}, {"split": "latest", "path": ["**/details_harness|hendrycksTest-public_relations|5_2024-01-10T15-33-00.629548.parquet"]}]}, {"config_name": "harness_hendrycksTest_security_studies_5", "data_files": [{"split": "2024_01_10T15_33_00.629548", "path": ["**/details_harness|hendrycksTest-security_studies|5_2024-01-10T15-33-00.629548.parquet"]}, {"split": "latest", "path": ["**/details_harness|hendrycksTest-security_studies|5_2024-01-10T15-33-00.629548.parquet"]}]}, {"config_name": "harness_hendrycksTest_sociology_5", "data_files": [{"split": "2024_01_10T15_33_00.629548", "path": ["**/details_harness|hendrycksTest-sociology|5_2024-01-10T15-33-00.629548.parquet"]}, {"split": "latest", "path": ["**/details_harness|hendrycksTest-sociology|5_2024-01-10T15-33-00.629548.parquet"]}]}, {"config_name": "harness_hendrycksTest_us_foreign_policy_5", "data_files": [{"split": "2024_01_10T15_33_00.629548", "path": ["**/details_harness|hendrycksTest-us_foreign_policy|5_2024-01-10T15-33-00.629548.parquet"]}, {"split": "latest", "path": ["**/details_harness|hendrycksTest-us_foreign_policy|5_2024-01-10T15-33-00.629548.parquet"]}]}, {"config_name": "harness_hendrycksTest_virology_5", "data_files": [{"split": "2024_01_10T15_33_00.629548", "path": ["**/details_harness|hendrycksTest-virology|5_2024-01-10T15-33-00.629548.parquet"]}, {"split": "latest", "path": ["**/details_harness|hendrycksTest-virology|5_2024-01-10T15-33-00.629548.parquet"]}]}, {"config_name": "harness_hendrycksTest_world_religions_5", "data_files": [{"split": "2024_01_10T15_33_00.629548", "path": ["**/details_harness|hendrycksTest-world_religions|5_2024-01-10T15-33-00.629548.parquet"]}, {"split": "latest", "path": ["**/details_harness|hendrycksTest-world_religions|5_2024-01-10T15-33-00.629548.parquet"]}]}, {"config_name": "harness_truthfulqa_mc_0", "data_files": [{"split": "2024_01_10T15_33_00.629548", "path": ["**/details_harness|truthfulqa:mc|0_2024-01-10T15-33-00.629548.parquet"]}, {"split": "latest", "path": ["**/details_harness|truthfulqa:mc|0_2024-01-10T15-33-00.629548.parquet"]}]}, {"config_name": "harness_winogrande_5", "data_files": [{"split": "2024_01_10T15_33_00.629548", "path": ["**/details_harness|winogrande|5_2024-01-10T15-33-00.629548.parquet"]}, {"split": "latest", "path": ["**/details_harness|winogrande|5_2024-01-10T15-33-00.629548.parquet"]}]}, {"config_name": "results", "data_files": [{"split": "2024_01_10T15_33_00.629548", "path": ["results_2024-01-10T15-33-00.629548.parquet"]}, {"split": "latest", "path": ["results_2024-01-10T15-33-00.629548.parquet"]}]}]} | 2024-01-10T15:35:41+00:00 | [] | [] | TAGS
#region-us
|
# Dataset Card for Evaluation run of abacusai/Fewshot-Metamath-Mistral
Dataset automatically created during the evaluation run of model abacusai/Fewshot-Metamath-Mistral on the Open LLM Leaderboard.
The dataset is composed of 63 configuration, each one coresponding to one of the evaluated task.
The dataset has been created from 1 run(s). Each run can be found as a specific split in each configuration, the split being named using the timestamp of the run.The "train" split is always pointing to the latest results.
An additional configuration "results" store all the aggregated results of the run (and is used to compute and display the aggregated metrics on the Open LLM Leaderboard).
To load the details from a run, you can for instance do the following:
## Latest results
These are the latest results from run 2024-01-10T15:33:00.629548(note that their might be results for other tasks in the repos if successive evals didn't cover the same tasks. You find each in the results and the "latest" split for each eval):
## Dataset Details
### Dataset Description
- Curated by:
- Funded by [optional]:
- Shared by [optional]:
- Language(s) (NLP):
- License:
### Dataset Sources [optional]
- Repository:
- Paper [optional]:
- Demo [optional]:
## Uses
### Direct Use
### Out-of-Scope Use
## Dataset Structure
## Dataset Creation
### Curation Rationale
### Source Data
#### Data Collection and Processing
#### Who are the source data producers?
### Annotations [optional]
#### Annotation process
#### Who are the annotators?
#### Personal and Sensitive Information
## Bias, Risks, and Limitations
### Recommendations
Users should be made aware of the risks, biases and limitations of the dataset. More information needed for further recommendations.
[optional]
BibTeX:
APA:
## Glossary [optional]
## More Information [optional]
## Dataset Card Authors [optional]
## Dataset Card Contact
| [
"# Dataset Card for Evaluation run of abacusai/Fewshot-Metamath-Mistral\n\n\n\nDataset automatically created during the evaluation run of model abacusai/Fewshot-Metamath-Mistral on the Open LLM Leaderboard.\n\nThe dataset is composed of 63 configuration, each one coresponding to one of the evaluated task.\n\nThe dataset has been created from 1 run(s). Each run can be found as a specific split in each configuration, the split being named using the timestamp of the run.The \"train\" split is always pointing to the latest results.\n\nAn additional configuration \"results\" store all the aggregated results of the run (and is used to compute and display the aggregated metrics on the Open LLM Leaderboard).\n\nTo load the details from a run, you can for instance do the following:",
"## Latest results\n\nThese are the latest results from run 2024-01-10T15:33:00.629548(note that their might be results for other tasks in the repos if successive evals didn't cover the same tasks. You find each in the results and the \"latest\" split for each eval):",
"## Dataset Details",
"### Dataset Description\n\n\n\n\n\n- Curated by: \n- Funded by [optional]: \n- Shared by [optional]: \n- Language(s) (NLP): \n- License:",
"### Dataset Sources [optional]\n\n\n\n- Repository: \n- Paper [optional]: \n- Demo [optional]:",
"## Uses",
"### Direct Use",
"### Out-of-Scope Use",
"## Dataset Structure",
"## Dataset Creation",
"### Curation Rationale",
"### Source Data",
"#### Data Collection and Processing",
"#### Who are the source data producers?",
"### Annotations [optional]",
"#### Annotation process",
"#### Who are the annotators?",
"#### Personal and Sensitive Information",
"## Bias, Risks, and Limitations",
"### Recommendations\n\n\n\nUsers should be made aware of the risks, biases and limitations of the dataset. More information needed for further recommendations.\n\n[optional]\n\n\n\nBibTeX:\n\n\n\nAPA:",
"## Glossary [optional]",
"## More Information [optional]",
"## Dataset Card Authors [optional]",
"## Dataset Card Contact"
] | [
"TAGS\n#region-us \n",
"# Dataset Card for Evaluation run of abacusai/Fewshot-Metamath-Mistral\n\n\n\nDataset automatically created during the evaluation run of model abacusai/Fewshot-Metamath-Mistral on the Open LLM Leaderboard.\n\nThe dataset is composed of 63 configuration, each one coresponding to one of the evaluated task.\n\nThe dataset has been created from 1 run(s). Each run can be found as a specific split in each configuration, the split being named using the timestamp of the run.The \"train\" split is always pointing to the latest results.\n\nAn additional configuration \"results\" store all the aggregated results of the run (and is used to compute and display the aggregated metrics on the Open LLM Leaderboard).\n\nTo load the details from a run, you can for instance do the following:",
"## Latest results\n\nThese are the latest results from run 2024-01-10T15:33:00.629548(note that their might be results for other tasks in the repos if successive evals didn't cover the same tasks. You find each in the results and the \"latest\" split for each eval):",
"## Dataset Details",
"### Dataset Description\n\n\n\n\n\n- Curated by: \n- Funded by [optional]: \n- Shared by [optional]: \n- Language(s) (NLP): \n- License:",
"### Dataset Sources [optional]\n\n\n\n- Repository: \n- Paper [optional]: \n- Demo [optional]:",
"## Uses",
"### Direct Use",
"### Out-of-Scope Use",
"## Dataset Structure",
"## Dataset Creation",
"### Curation Rationale",
"### Source Data",
"#### Data Collection and Processing",
"#### Who are the source data producers?",
"### Annotations [optional]",
"#### Annotation process",
"#### Who are the annotators?",
"#### Personal and Sensitive Information",
"## Bias, Risks, and Limitations",
"### Recommendations\n\n\n\nUsers should be made aware of the risks, biases and limitations of the dataset. More information needed for further recommendations.\n\n[optional]\n\n\n\nBibTeX:\n\n\n\nAPA:",
"## Glossary [optional]",
"## More Information [optional]",
"## Dataset Card Authors [optional]",
"## Dataset Card Contact"
] |
71e2d81132b343462442433c0b5828e12a3289d5 |
# Dataset Card for Evaluation run of abacusai/Fewshot-Metamath-OrcaVicuna-Mistral
<!-- Provide a quick summary of the dataset. -->
Dataset automatically created during the evaluation run of model [abacusai/Fewshot-Metamath-OrcaVicuna-Mistral](https://huggingface.co/abacusai/Fewshot-Metamath-OrcaVicuna-Mistral) on the [Open LLM Leaderboard](https://huggingface.co/spaces/HuggingFaceH4/open_llm_leaderboard).
The dataset is composed of 63 configuration, each one coresponding to one of the evaluated task.
The dataset has been created from 1 run(s). Each run can be found as a specific split in each configuration, the split being named using the timestamp of the run.The "train" split is always pointing to the latest results.
An additional configuration "results" store all the aggregated results of the run (and is used to compute and display the aggregated metrics on the [Open LLM Leaderboard](https://huggingface.co/spaces/HuggingFaceH4/open_llm_leaderboard)).
To load the details from a run, you can for instance do the following:
```python
from datasets import load_dataset
data = load_dataset("open-llm-leaderboard/details_abacusai__Fewshot-Metamath-OrcaVicuna-Mistral",
"harness_winogrande_5",
split="train")
```
## Latest results
These are the [latest results from run 2024-01-10T15:33:11.471365](https://huggingface.co/datasets/open-llm-leaderboard/details_abacusai__Fewshot-Metamath-OrcaVicuna-Mistral/blob/main/results_2024-01-10T15-33-11.471365.json)(note that their might be results for other tasks in the repos if successive evals didn't cover the same tasks. You find each in the results and the "latest" split for each eval):
```python
{
"all": {
"acc": 0.6202552921359215,
"acc_stderr": 0.03264933582271829,
"acc_norm": 0.6199993104526068,
"acc_norm_stderr": 0.03332566222099852,
"mc1": 0.3659730722154223,
"mc1_stderr": 0.016862941684088365,
"mc2": 0.5323239517078452,
"mc2_stderr": 0.0151650266597209
},
"harness|arc:challenge|25": {
"acc": 0.568259385665529,
"acc_stderr": 0.014474591427196202,
"acc_norm": 0.5964163822525598,
"acc_norm_stderr": 0.014337158914268443
},
"harness|hellaswag|10": {
"acc": 0.6259709221270663,
"acc_stderr": 0.004828822920915222,
"acc_norm": 0.8181637124078869,
"acc_norm_stderr": 0.0038492126228151682
},
"harness|hendrycksTest-abstract_algebra|5": {
"acc": 0.25,
"acc_stderr": 0.04351941398892446,
"acc_norm": 0.25,
"acc_norm_stderr": 0.04351941398892446
},
"harness|hendrycksTest-anatomy|5": {
"acc": 0.562962962962963,
"acc_stderr": 0.042849586397534015,
"acc_norm": 0.562962962962963,
"acc_norm_stderr": 0.042849586397534015
},
"harness|hendrycksTest-astronomy|5": {
"acc": 0.6644736842105263,
"acc_stderr": 0.03842498559395268,
"acc_norm": 0.6644736842105263,
"acc_norm_stderr": 0.03842498559395268
},
"harness|hendrycksTest-business_ethics|5": {
"acc": 0.6,
"acc_stderr": 0.04923659639173309,
"acc_norm": 0.6,
"acc_norm_stderr": 0.04923659639173309
},
"harness|hendrycksTest-clinical_knowledge|5": {
"acc": 0.6716981132075471,
"acc_stderr": 0.02890159361241178,
"acc_norm": 0.6716981132075471,
"acc_norm_stderr": 0.02890159361241178
},
"harness|hendrycksTest-college_biology|5": {
"acc": 0.6736111111111112,
"acc_stderr": 0.03921067198982266,
"acc_norm": 0.6736111111111112,
"acc_norm_stderr": 0.03921067198982266
},
"harness|hendrycksTest-college_chemistry|5": {
"acc": 0.44,
"acc_stderr": 0.04988876515698589,
"acc_norm": 0.44,
"acc_norm_stderr": 0.04988876515698589
},
"harness|hendrycksTest-college_computer_science|5": {
"acc": 0.53,
"acc_stderr": 0.05016135580465919,
"acc_norm": 0.53,
"acc_norm_stderr": 0.05016135580465919
},
"harness|hendrycksTest-college_mathematics|5": {
"acc": 0.32,
"acc_stderr": 0.046882617226215034,
"acc_norm": 0.32,
"acc_norm_stderr": 0.046882617226215034
},
"harness|hendrycksTest-college_medicine|5": {
"acc": 0.6416184971098265,
"acc_stderr": 0.03656343653353159,
"acc_norm": 0.6416184971098265,
"acc_norm_stderr": 0.03656343653353159
},
"harness|hendrycksTest-college_physics|5": {
"acc": 0.35294117647058826,
"acc_stderr": 0.04755129616062946,
"acc_norm": 0.35294117647058826,
"acc_norm_stderr": 0.04755129616062946
},
"harness|hendrycksTest-computer_security|5": {
"acc": 0.77,
"acc_stderr": 0.042295258468165065,
"acc_norm": 0.77,
"acc_norm_stderr": 0.042295258468165065
},
"harness|hendrycksTest-conceptual_physics|5": {
"acc": 0.5191489361702127,
"acc_stderr": 0.03266204299064678,
"acc_norm": 0.5191489361702127,
"acc_norm_stderr": 0.03266204299064678
},
"harness|hendrycksTest-econometrics|5": {
"acc": 0.37719298245614036,
"acc_stderr": 0.04559522141958216,
"acc_norm": 0.37719298245614036,
"acc_norm_stderr": 0.04559522141958216
},
"harness|hendrycksTest-electrical_engineering|5": {
"acc": 0.5517241379310345,
"acc_stderr": 0.04144311810878152,
"acc_norm": 0.5517241379310345,
"acc_norm_stderr": 0.04144311810878152
},
"harness|hendrycksTest-elementary_mathematics|5": {
"acc": 0.3862433862433862,
"acc_stderr": 0.02507598176760168,
"acc_norm": 0.3862433862433862,
"acc_norm_stderr": 0.02507598176760168
},
"harness|hendrycksTest-formal_logic|5": {
"acc": 0.4603174603174603,
"acc_stderr": 0.04458029125470973,
"acc_norm": 0.4603174603174603,
"acc_norm_stderr": 0.04458029125470973
},
"harness|hendrycksTest-global_facts|5": {
"acc": 0.29,
"acc_stderr": 0.045604802157206845,
"acc_norm": 0.29,
"acc_norm_stderr": 0.045604802157206845
},
"harness|hendrycksTest-high_school_biology|5": {
"acc": 0.7677419354838709,
"acc_stderr": 0.024022256130308235,
"acc_norm": 0.7677419354838709,
"acc_norm_stderr": 0.024022256130308235
},
"harness|hendrycksTest-high_school_chemistry|5": {
"acc": 0.4876847290640394,
"acc_stderr": 0.035169204442208966,
"acc_norm": 0.4876847290640394,
"acc_norm_stderr": 0.035169204442208966
},
"harness|hendrycksTest-high_school_computer_science|5": {
"acc": 0.61,
"acc_stderr": 0.04902071300001975,
"acc_norm": 0.61,
"acc_norm_stderr": 0.04902071300001975
},
"harness|hendrycksTest-high_school_european_history|5": {
"acc": 0.7393939393939394,
"acc_stderr": 0.034277431758165236,
"acc_norm": 0.7393939393939394,
"acc_norm_stderr": 0.034277431758165236
},
"harness|hendrycksTest-high_school_geography|5": {
"acc": 0.7878787878787878,
"acc_stderr": 0.029126522834586815,
"acc_norm": 0.7878787878787878,
"acc_norm_stderr": 0.029126522834586815
},
"harness|hendrycksTest-high_school_government_and_politics|5": {
"acc": 0.8393782383419689,
"acc_stderr": 0.02649905770139744,
"acc_norm": 0.8393782383419689,
"acc_norm_stderr": 0.02649905770139744
},
"harness|hendrycksTest-high_school_macroeconomics|5": {
"acc": 0.6230769230769231,
"acc_stderr": 0.024570975364225995,
"acc_norm": 0.6230769230769231,
"acc_norm_stderr": 0.024570975364225995
},
"harness|hendrycksTest-high_school_mathematics|5": {
"acc": 0.3,
"acc_stderr": 0.027940457136228412,
"acc_norm": 0.3,
"acc_norm_stderr": 0.027940457136228412
},
"harness|hendrycksTest-high_school_microeconomics|5": {
"acc": 0.6134453781512605,
"acc_stderr": 0.03163145807552378,
"acc_norm": 0.6134453781512605,
"acc_norm_stderr": 0.03163145807552378
},
"harness|hendrycksTest-high_school_physics|5": {
"acc": 0.3509933774834437,
"acc_stderr": 0.03896981964257375,
"acc_norm": 0.3509933774834437,
"acc_norm_stderr": 0.03896981964257375
},
"harness|hendrycksTest-high_school_psychology|5": {
"acc": 0.818348623853211,
"acc_stderr": 0.016530617409266854,
"acc_norm": 0.818348623853211,
"acc_norm_stderr": 0.016530617409266854
},
"harness|hendrycksTest-high_school_statistics|5": {
"acc": 0.4861111111111111,
"acc_stderr": 0.03408655867977748,
"acc_norm": 0.4861111111111111,
"acc_norm_stderr": 0.03408655867977748
},
"harness|hendrycksTest-high_school_us_history|5": {
"acc": 0.7794117647058824,
"acc_stderr": 0.02910225438967408,
"acc_norm": 0.7794117647058824,
"acc_norm_stderr": 0.02910225438967408
},
"harness|hendrycksTest-high_school_world_history|5": {
"acc": 0.7763713080168776,
"acc_stderr": 0.027123298205229962,
"acc_norm": 0.7763713080168776,
"acc_norm_stderr": 0.027123298205229962
},
"harness|hendrycksTest-human_aging|5": {
"acc": 0.672645739910314,
"acc_stderr": 0.03149384670994131,
"acc_norm": 0.672645739910314,
"acc_norm_stderr": 0.03149384670994131
},
"harness|hendrycksTest-human_sexuality|5": {
"acc": 0.7480916030534351,
"acc_stderr": 0.038073871163060866,
"acc_norm": 0.7480916030534351,
"acc_norm_stderr": 0.038073871163060866
},
"harness|hendrycksTest-international_law|5": {
"acc": 0.768595041322314,
"acc_stderr": 0.03849856098794089,
"acc_norm": 0.768595041322314,
"acc_norm_stderr": 0.03849856098794089
},
"harness|hendrycksTest-jurisprudence|5": {
"acc": 0.7222222222222222,
"acc_stderr": 0.043300437496507437,
"acc_norm": 0.7222222222222222,
"acc_norm_stderr": 0.043300437496507437
},
"harness|hendrycksTest-logical_fallacies|5": {
"acc": 0.7607361963190185,
"acc_stderr": 0.03351953879521269,
"acc_norm": 0.7607361963190185,
"acc_norm_stderr": 0.03351953879521269
},
"harness|hendrycksTest-machine_learning|5": {
"acc": 0.5089285714285714,
"acc_stderr": 0.04745033255489123,
"acc_norm": 0.5089285714285714,
"acc_norm_stderr": 0.04745033255489123
},
"harness|hendrycksTest-management|5": {
"acc": 0.7766990291262136,
"acc_stderr": 0.04123553189891431,
"acc_norm": 0.7766990291262136,
"acc_norm_stderr": 0.04123553189891431
},
"harness|hendrycksTest-marketing|5": {
"acc": 0.8717948717948718,
"acc_stderr": 0.021901905115073325,
"acc_norm": 0.8717948717948718,
"acc_norm_stderr": 0.021901905115073325
},
"harness|hendrycksTest-medical_genetics|5": {
"acc": 0.72,
"acc_stderr": 0.04512608598542128,
"acc_norm": 0.72,
"acc_norm_stderr": 0.04512608598542128
},
"harness|hendrycksTest-miscellaneous|5": {
"acc": 0.8045977011494253,
"acc_stderr": 0.014179171373424384,
"acc_norm": 0.8045977011494253,
"acc_norm_stderr": 0.014179171373424384
},
"harness|hendrycksTest-moral_disputes|5": {
"acc": 0.6907514450867052,
"acc_stderr": 0.02488314057007176,
"acc_norm": 0.6907514450867052,
"acc_norm_stderr": 0.02488314057007176
},
"harness|hendrycksTest-moral_scenarios|5": {
"acc": 0.41787709497206704,
"acc_stderr": 0.016495400635820084,
"acc_norm": 0.41787709497206704,
"acc_norm_stderr": 0.016495400635820084
},
"harness|hendrycksTest-nutrition|5": {
"acc": 0.6895424836601307,
"acc_stderr": 0.026493033225145898,
"acc_norm": 0.6895424836601307,
"acc_norm_stderr": 0.026493033225145898
},
"harness|hendrycksTest-philosophy|5": {
"acc": 0.7106109324758842,
"acc_stderr": 0.02575586592263295,
"acc_norm": 0.7106109324758842,
"acc_norm_stderr": 0.02575586592263295
},
"harness|hendrycksTest-prehistory|5": {
"acc": 0.7037037037037037,
"acc_stderr": 0.025407197798890155,
"acc_norm": 0.7037037037037037,
"acc_norm_stderr": 0.025407197798890155
},
"harness|hendrycksTest-professional_accounting|5": {
"acc": 0.46808510638297873,
"acc_stderr": 0.029766675075873866,
"acc_norm": 0.46808510638297873,
"acc_norm_stderr": 0.029766675075873866
},
"harness|hendrycksTest-professional_law|5": {
"acc": 0.44002607561929596,
"acc_stderr": 0.012678037478574513,
"acc_norm": 0.44002607561929596,
"acc_norm_stderr": 0.012678037478574513
},
"harness|hendrycksTest-professional_medicine|5": {
"acc": 0.6433823529411765,
"acc_stderr": 0.029097209568411952,
"acc_norm": 0.6433823529411765,
"acc_norm_stderr": 0.029097209568411952
},
"harness|hendrycksTest-professional_psychology|5": {
"acc": 0.6241830065359477,
"acc_stderr": 0.01959402113657744,
"acc_norm": 0.6241830065359477,
"acc_norm_stderr": 0.01959402113657744
},
"harness|hendrycksTest-public_relations|5": {
"acc": 0.6363636363636364,
"acc_stderr": 0.046075820907199756,
"acc_norm": 0.6363636363636364,
"acc_norm_stderr": 0.046075820907199756
},
"harness|hendrycksTest-security_studies|5": {
"acc": 0.7020408163265306,
"acc_stderr": 0.029279567411065677,
"acc_norm": 0.7020408163265306,
"acc_norm_stderr": 0.029279567411065677
},
"harness|hendrycksTest-sociology|5": {
"acc": 0.845771144278607,
"acc_stderr": 0.02553843336857833,
"acc_norm": 0.845771144278607,
"acc_norm_stderr": 0.02553843336857833
},
"harness|hendrycksTest-us_foreign_policy|5": {
"acc": 0.86,
"acc_stderr": 0.034873508801977704,
"acc_norm": 0.86,
"acc_norm_stderr": 0.034873508801977704
},
"harness|hendrycksTest-virology|5": {
"acc": 0.5240963855421686,
"acc_stderr": 0.03887971849597264,
"acc_norm": 0.5240963855421686,
"acc_norm_stderr": 0.03887971849597264
},
"harness|hendrycksTest-world_religions|5": {
"acc": 0.8128654970760234,
"acc_stderr": 0.02991312723236804,
"acc_norm": 0.8128654970760234,
"acc_norm_stderr": 0.02991312723236804
},
"harness|truthfulqa:mc|0": {
"mc1": 0.3659730722154223,
"mc1_stderr": 0.016862941684088365,
"mc2": 0.5323239517078452,
"mc2_stderr": 0.0151650266597209
},
"harness|winogrande|5": {
"acc": 0.7845303867403315,
"acc_stderr": 0.011555295286059279
},
"harness|gsm8k|5": {
"acc": 0.6914329037149356,
"acc_stderr": 0.012723076049815896
}
}
```
## Dataset Details
### Dataset Description
<!-- Provide a longer summary of what this dataset is. -->
- **Curated by:** [More Information Needed]
- **Funded by [optional]:** [More Information Needed]
- **Shared by [optional]:** [More Information Needed]
- **Language(s) (NLP):** [More Information Needed]
- **License:** [More Information Needed]
### Dataset Sources [optional]
<!-- Provide the basic links for the dataset. -->
- **Repository:** [More Information Needed]
- **Paper [optional]:** [More Information Needed]
- **Demo [optional]:** [More Information Needed]
## Uses
<!-- Address questions around how the dataset is intended to be used. -->
### Direct Use
<!-- This section describes suitable use cases for the dataset. -->
[More Information Needed]
### Out-of-Scope Use
<!-- This section addresses misuse, malicious use, and uses that the dataset will not work well for. -->
[More Information Needed]
## Dataset Structure
<!-- This section provides a description of the dataset fields, and additional information about the dataset structure such as criteria used to create the splits, relationships between data points, etc. -->
[More Information Needed]
## Dataset Creation
### Curation Rationale
<!-- Motivation for the creation of this dataset. -->
[More Information Needed]
### Source Data
<!-- This section describes the source data (e.g. news text and headlines, social media posts, translated sentences, ...). -->
#### Data Collection and Processing
<!-- This section describes the data collection and processing process such as data selection criteria, filtering and normalization methods, tools and libraries used, etc. -->
[More Information Needed]
#### Who are the source data producers?
<!-- This section describes the people or systems who originally created the data. It should also include self-reported demographic or identity information for the source data creators if this information is available. -->
[More Information Needed]
### Annotations [optional]
<!-- If the dataset contains annotations which are not part of the initial data collection, use this section to describe them. -->
#### Annotation process
<!-- This section describes the annotation process such as annotation tools used in the process, the amount of data annotated, annotation guidelines provided to the annotators, interannotator statistics, annotation validation, etc. -->
[More Information Needed]
#### Who are the annotators?
<!-- This section describes the people or systems who created the annotations. -->
[More Information Needed]
#### Personal and Sensitive Information
<!-- State whether the dataset contains data that might be considered personal, sensitive, or private (e.g., data that reveals addresses, uniquely identifiable names or aliases, racial or ethnic origins, sexual orientations, religious beliefs, political opinions, financial or health data, etc.). If efforts were made to anonymize the data, describe the anonymization process. -->
[More Information Needed]
## Bias, Risks, and Limitations
<!-- This section is meant to convey both technical and sociotechnical limitations. -->
[More Information Needed]
### Recommendations
<!-- This section is meant to convey recommendations with respect to the bias, risk, and technical limitations. -->
Users should be made aware of the risks, biases and limitations of the dataset. More information needed for further recommendations.
## Citation [optional]
<!-- If there is a paper or blog post introducing the dataset, the APA and Bibtex information for that should go in this section. -->
**BibTeX:**
[More Information Needed]
**APA:**
[More Information Needed]
## Glossary [optional]
<!-- If relevant, include terms and calculations in this section that can help readers understand the dataset or dataset card. -->
[More Information Needed]
## More Information [optional]
[More Information Needed]
## Dataset Card Authors [optional]
[More Information Needed]
## Dataset Card Contact
[More Information Needed] | open-llm-leaderboard/details_abacusai__Fewshot-Metamath-OrcaVicuna-Mistral | [
"region:us"
] | 2024-01-10T15:35:32+00:00 | {"pretty_name": "Evaluation run of abacusai/Fewshot-Metamath-OrcaVicuna-Mistral", "dataset_summary": "Dataset automatically created during the evaluation run of model [abacusai/Fewshot-Metamath-OrcaVicuna-Mistral](https://huggingface.co/abacusai/Fewshot-Metamath-OrcaVicuna-Mistral) on the [Open LLM Leaderboard](https://huggingface.co/spaces/HuggingFaceH4/open_llm_leaderboard).\n\nThe dataset is composed of 63 configuration, each one coresponding to one of the evaluated task.\n\nThe dataset has been created from 1 run(s). Each run can be found as a specific split in each configuration, the split being named using the timestamp of the run.The \"train\" split is always pointing to the latest results.\n\nAn additional configuration \"results\" store all the aggregated results of the run (and is used to compute and display the aggregated metrics on the [Open LLM Leaderboard](https://huggingface.co/spaces/HuggingFaceH4/open_llm_leaderboard)).\n\nTo load the details from a run, you can for instance do the following:\n```python\nfrom datasets import load_dataset\ndata = load_dataset(\"open-llm-leaderboard/details_abacusai__Fewshot-Metamath-OrcaVicuna-Mistral\",\n\t\"harness_winogrande_5\",\n\tsplit=\"train\")\n```\n\n## Latest results\n\nThese are the [latest results from run 2024-01-10T15:33:11.471365](https://huggingface.co/datasets/open-llm-leaderboard/details_abacusai__Fewshot-Metamath-OrcaVicuna-Mistral/blob/main/results_2024-01-10T15-33-11.471365.json)(note that their might be results for other tasks in the repos if successive evals didn't cover the same tasks. You find each in the results and the \"latest\" split for each eval):\n\n```python\n{\n \"all\": {\n \"acc\": 0.6202552921359215,\n \"acc_stderr\": 0.03264933582271829,\n \"acc_norm\": 0.6199993104526068,\n \"acc_norm_stderr\": 0.03332566222099852,\n \"mc1\": 0.3659730722154223,\n \"mc1_stderr\": 0.016862941684088365,\n \"mc2\": 0.5323239517078452,\n \"mc2_stderr\": 0.0151650266597209\n },\n \"harness|arc:challenge|25\": {\n \"acc\": 0.568259385665529,\n \"acc_stderr\": 0.014474591427196202,\n \"acc_norm\": 0.5964163822525598,\n \"acc_norm_stderr\": 0.014337158914268443\n },\n \"harness|hellaswag|10\": {\n \"acc\": 0.6259709221270663,\n \"acc_stderr\": 0.004828822920915222,\n \"acc_norm\": 0.8181637124078869,\n \"acc_norm_stderr\": 0.0038492126228151682\n },\n \"harness|hendrycksTest-abstract_algebra|5\": {\n \"acc\": 0.25,\n \"acc_stderr\": 0.04351941398892446,\n \"acc_norm\": 0.25,\n \"acc_norm_stderr\": 0.04351941398892446\n },\n \"harness|hendrycksTest-anatomy|5\": {\n \"acc\": 0.562962962962963,\n \"acc_stderr\": 0.042849586397534015,\n \"acc_norm\": 0.562962962962963,\n \"acc_norm_stderr\": 0.042849586397534015\n },\n \"harness|hendrycksTest-astronomy|5\": {\n \"acc\": 0.6644736842105263,\n \"acc_stderr\": 0.03842498559395268,\n \"acc_norm\": 0.6644736842105263,\n \"acc_norm_stderr\": 0.03842498559395268\n },\n \"harness|hendrycksTest-business_ethics|5\": {\n \"acc\": 0.6,\n \"acc_stderr\": 0.04923659639173309,\n \"acc_norm\": 0.6,\n \"acc_norm_stderr\": 0.04923659639173309\n },\n \"harness|hendrycksTest-clinical_knowledge|5\": {\n \"acc\": 0.6716981132075471,\n \"acc_stderr\": 0.02890159361241178,\n \"acc_norm\": 0.6716981132075471,\n \"acc_norm_stderr\": 0.02890159361241178\n },\n \"harness|hendrycksTest-college_biology|5\": {\n \"acc\": 0.6736111111111112,\n \"acc_stderr\": 0.03921067198982266,\n \"acc_norm\": 0.6736111111111112,\n \"acc_norm_stderr\": 0.03921067198982266\n },\n \"harness|hendrycksTest-college_chemistry|5\": {\n \"acc\": 0.44,\n \"acc_stderr\": 0.04988876515698589,\n \"acc_norm\": 0.44,\n \"acc_norm_stderr\": 0.04988876515698589\n },\n \"harness|hendrycksTest-college_computer_science|5\": {\n \"acc\": 0.53,\n \"acc_stderr\": 0.05016135580465919,\n \"acc_norm\": 0.53,\n \"acc_norm_stderr\": 0.05016135580465919\n },\n \"harness|hendrycksTest-college_mathematics|5\": {\n \"acc\": 0.32,\n \"acc_stderr\": 0.046882617226215034,\n \"acc_norm\": 0.32,\n \"acc_norm_stderr\": 0.046882617226215034\n },\n \"harness|hendrycksTest-college_medicine|5\": {\n \"acc\": 0.6416184971098265,\n \"acc_stderr\": 0.03656343653353159,\n \"acc_norm\": 0.6416184971098265,\n \"acc_norm_stderr\": 0.03656343653353159\n },\n \"harness|hendrycksTest-college_physics|5\": {\n \"acc\": 0.35294117647058826,\n \"acc_stderr\": 0.04755129616062946,\n \"acc_norm\": 0.35294117647058826,\n \"acc_norm_stderr\": 0.04755129616062946\n },\n \"harness|hendrycksTest-computer_security|5\": {\n \"acc\": 0.77,\n \"acc_stderr\": 0.042295258468165065,\n \"acc_norm\": 0.77,\n \"acc_norm_stderr\": 0.042295258468165065\n },\n \"harness|hendrycksTest-conceptual_physics|5\": {\n \"acc\": 0.5191489361702127,\n \"acc_stderr\": 0.03266204299064678,\n \"acc_norm\": 0.5191489361702127,\n \"acc_norm_stderr\": 0.03266204299064678\n },\n \"harness|hendrycksTest-econometrics|5\": {\n \"acc\": 0.37719298245614036,\n \"acc_stderr\": 0.04559522141958216,\n \"acc_norm\": 0.37719298245614036,\n \"acc_norm_stderr\": 0.04559522141958216\n },\n \"harness|hendrycksTest-electrical_engineering|5\": {\n \"acc\": 0.5517241379310345,\n \"acc_stderr\": 0.04144311810878152,\n \"acc_norm\": 0.5517241379310345,\n \"acc_norm_stderr\": 0.04144311810878152\n },\n \"harness|hendrycksTest-elementary_mathematics|5\": {\n \"acc\": 0.3862433862433862,\n \"acc_stderr\": 0.02507598176760168,\n \"acc_norm\": 0.3862433862433862,\n \"acc_norm_stderr\": 0.02507598176760168\n },\n \"harness|hendrycksTest-formal_logic|5\": {\n \"acc\": 0.4603174603174603,\n \"acc_stderr\": 0.04458029125470973,\n \"acc_norm\": 0.4603174603174603,\n \"acc_norm_stderr\": 0.04458029125470973\n },\n \"harness|hendrycksTest-global_facts|5\": {\n \"acc\": 0.29,\n \"acc_stderr\": 0.045604802157206845,\n \"acc_norm\": 0.29,\n \"acc_norm_stderr\": 0.045604802157206845\n },\n \"harness|hendrycksTest-high_school_biology|5\": {\n \"acc\": 0.7677419354838709,\n \"acc_stderr\": 0.024022256130308235,\n \"acc_norm\": 0.7677419354838709,\n \"acc_norm_stderr\": 0.024022256130308235\n },\n \"harness|hendrycksTest-high_school_chemistry|5\": {\n \"acc\": 0.4876847290640394,\n \"acc_stderr\": 0.035169204442208966,\n \"acc_norm\": 0.4876847290640394,\n \"acc_norm_stderr\": 0.035169204442208966\n },\n \"harness|hendrycksTest-high_school_computer_science|5\": {\n \"acc\": 0.61,\n \"acc_stderr\": 0.04902071300001975,\n \"acc_norm\": 0.61,\n \"acc_norm_stderr\": 0.04902071300001975\n },\n \"harness|hendrycksTest-high_school_european_history|5\": {\n \"acc\": 0.7393939393939394,\n \"acc_stderr\": 0.034277431758165236,\n \"acc_norm\": 0.7393939393939394,\n \"acc_norm_stderr\": 0.034277431758165236\n },\n \"harness|hendrycksTest-high_school_geography|5\": {\n \"acc\": 0.7878787878787878,\n \"acc_stderr\": 0.029126522834586815,\n \"acc_norm\": 0.7878787878787878,\n \"acc_norm_stderr\": 0.029126522834586815\n },\n \"harness|hendrycksTest-high_school_government_and_politics|5\": {\n \"acc\": 0.8393782383419689,\n \"acc_stderr\": 0.02649905770139744,\n \"acc_norm\": 0.8393782383419689,\n \"acc_norm_stderr\": 0.02649905770139744\n },\n \"harness|hendrycksTest-high_school_macroeconomics|5\": {\n \"acc\": 0.6230769230769231,\n \"acc_stderr\": 0.024570975364225995,\n \"acc_norm\": 0.6230769230769231,\n \"acc_norm_stderr\": 0.024570975364225995\n },\n \"harness|hendrycksTest-high_school_mathematics|5\": {\n \"acc\": 0.3,\n \"acc_stderr\": 0.027940457136228412,\n \"acc_norm\": 0.3,\n \"acc_norm_stderr\": 0.027940457136228412\n },\n \"harness|hendrycksTest-high_school_microeconomics|5\": {\n \"acc\": 0.6134453781512605,\n \"acc_stderr\": 0.03163145807552378,\n \"acc_norm\": 0.6134453781512605,\n \"acc_norm_stderr\": 0.03163145807552378\n },\n \"harness|hendrycksTest-high_school_physics|5\": {\n \"acc\": 0.3509933774834437,\n \"acc_stderr\": 0.03896981964257375,\n \"acc_norm\": 0.3509933774834437,\n \"acc_norm_stderr\": 0.03896981964257375\n },\n \"harness|hendrycksTest-high_school_psychology|5\": {\n \"acc\": 0.818348623853211,\n \"acc_stderr\": 0.016530617409266854,\n \"acc_norm\": 0.818348623853211,\n \"acc_norm_stderr\": 0.016530617409266854\n },\n \"harness|hendrycksTest-high_school_statistics|5\": {\n \"acc\": 0.4861111111111111,\n \"acc_stderr\": 0.03408655867977748,\n \"acc_norm\": 0.4861111111111111,\n \"acc_norm_stderr\": 0.03408655867977748\n },\n \"harness|hendrycksTest-high_school_us_history|5\": {\n \"acc\": 0.7794117647058824,\n \"acc_stderr\": 0.02910225438967408,\n \"acc_norm\": 0.7794117647058824,\n \"acc_norm_stderr\": 0.02910225438967408\n },\n \"harness|hendrycksTest-high_school_world_history|5\": {\n \"acc\": 0.7763713080168776,\n \"acc_stderr\": 0.027123298205229962,\n \"acc_norm\": 0.7763713080168776,\n \"acc_norm_stderr\": 0.027123298205229962\n },\n \"harness|hendrycksTest-human_aging|5\": {\n \"acc\": 0.672645739910314,\n \"acc_stderr\": 0.03149384670994131,\n \"acc_norm\": 0.672645739910314,\n \"acc_norm_stderr\": 0.03149384670994131\n },\n \"harness|hendrycksTest-human_sexuality|5\": {\n \"acc\": 0.7480916030534351,\n \"acc_stderr\": 0.038073871163060866,\n \"acc_norm\": 0.7480916030534351,\n \"acc_norm_stderr\": 0.038073871163060866\n },\n \"harness|hendrycksTest-international_law|5\": {\n \"acc\": 0.768595041322314,\n \"acc_stderr\": 0.03849856098794089,\n \"acc_norm\": 0.768595041322314,\n \"acc_norm_stderr\": 0.03849856098794089\n },\n \"harness|hendrycksTest-jurisprudence|5\": {\n \"acc\": 0.7222222222222222,\n \"acc_stderr\": 0.043300437496507437,\n \"acc_norm\": 0.7222222222222222,\n \"acc_norm_stderr\": 0.043300437496507437\n },\n \"harness|hendrycksTest-logical_fallacies|5\": {\n \"acc\": 0.7607361963190185,\n \"acc_stderr\": 0.03351953879521269,\n \"acc_norm\": 0.7607361963190185,\n \"acc_norm_stderr\": 0.03351953879521269\n },\n \"harness|hendrycksTest-machine_learning|5\": {\n \"acc\": 0.5089285714285714,\n \"acc_stderr\": 0.04745033255489123,\n \"acc_norm\": 0.5089285714285714,\n \"acc_norm_stderr\": 0.04745033255489123\n },\n \"harness|hendrycksTest-management|5\": {\n \"acc\": 0.7766990291262136,\n \"acc_stderr\": 0.04123553189891431,\n \"acc_norm\": 0.7766990291262136,\n \"acc_norm_stderr\": 0.04123553189891431\n },\n \"harness|hendrycksTest-marketing|5\": {\n \"acc\": 0.8717948717948718,\n \"acc_stderr\": 0.021901905115073325,\n \"acc_norm\": 0.8717948717948718,\n \"acc_norm_stderr\": 0.021901905115073325\n },\n \"harness|hendrycksTest-medical_genetics|5\": {\n \"acc\": 0.72,\n \"acc_stderr\": 0.04512608598542128,\n \"acc_norm\": 0.72,\n \"acc_norm_stderr\": 0.04512608598542128\n },\n \"harness|hendrycksTest-miscellaneous|5\": {\n \"acc\": 0.8045977011494253,\n \"acc_stderr\": 0.014179171373424384,\n \"acc_norm\": 0.8045977011494253,\n \"acc_norm_stderr\": 0.014179171373424384\n },\n \"harness|hendrycksTest-moral_disputes|5\": {\n \"acc\": 0.6907514450867052,\n \"acc_stderr\": 0.02488314057007176,\n \"acc_norm\": 0.6907514450867052,\n \"acc_norm_stderr\": 0.02488314057007176\n },\n \"harness|hendrycksTest-moral_scenarios|5\": {\n \"acc\": 0.41787709497206704,\n \"acc_stderr\": 0.016495400635820084,\n \"acc_norm\": 0.41787709497206704,\n \"acc_norm_stderr\": 0.016495400635820084\n },\n \"harness|hendrycksTest-nutrition|5\": {\n \"acc\": 0.6895424836601307,\n \"acc_stderr\": 0.026493033225145898,\n \"acc_norm\": 0.6895424836601307,\n \"acc_norm_stderr\": 0.026493033225145898\n },\n \"harness|hendrycksTest-philosophy|5\": {\n \"acc\": 0.7106109324758842,\n \"acc_stderr\": 0.02575586592263295,\n \"acc_norm\": 0.7106109324758842,\n \"acc_norm_stderr\": 0.02575586592263295\n },\n \"harness|hendrycksTest-prehistory|5\": {\n \"acc\": 0.7037037037037037,\n \"acc_stderr\": 0.025407197798890155,\n \"acc_norm\": 0.7037037037037037,\n \"acc_norm_stderr\": 0.025407197798890155\n },\n \"harness|hendrycksTest-professional_accounting|5\": {\n \"acc\": 0.46808510638297873,\n \"acc_stderr\": 0.029766675075873866,\n \"acc_norm\": 0.46808510638297873,\n \"acc_norm_stderr\": 0.029766675075873866\n },\n \"harness|hendrycksTest-professional_law|5\": {\n \"acc\": 0.44002607561929596,\n \"acc_stderr\": 0.012678037478574513,\n \"acc_norm\": 0.44002607561929596,\n \"acc_norm_stderr\": 0.012678037478574513\n },\n \"harness|hendrycksTest-professional_medicine|5\": {\n \"acc\": 0.6433823529411765,\n \"acc_stderr\": 0.029097209568411952,\n \"acc_norm\": 0.6433823529411765,\n \"acc_norm_stderr\": 0.029097209568411952\n },\n \"harness|hendrycksTest-professional_psychology|5\": {\n \"acc\": 0.6241830065359477,\n \"acc_stderr\": 0.01959402113657744,\n \"acc_norm\": 0.6241830065359477,\n \"acc_norm_stderr\": 0.01959402113657744\n },\n \"harness|hendrycksTest-public_relations|5\": {\n \"acc\": 0.6363636363636364,\n \"acc_stderr\": 0.046075820907199756,\n \"acc_norm\": 0.6363636363636364,\n \"acc_norm_stderr\": 0.046075820907199756\n },\n \"harness|hendrycksTest-security_studies|5\": {\n \"acc\": 0.7020408163265306,\n \"acc_stderr\": 0.029279567411065677,\n \"acc_norm\": 0.7020408163265306,\n \"acc_norm_stderr\": 0.029279567411065677\n },\n \"harness|hendrycksTest-sociology|5\": {\n \"acc\": 0.845771144278607,\n \"acc_stderr\": 0.02553843336857833,\n \"acc_norm\": 0.845771144278607,\n \"acc_norm_stderr\": 0.02553843336857833\n },\n \"harness|hendrycksTest-us_foreign_policy|5\": {\n \"acc\": 0.86,\n \"acc_stderr\": 0.034873508801977704,\n \"acc_norm\": 0.86,\n \"acc_norm_stderr\": 0.034873508801977704\n },\n \"harness|hendrycksTest-virology|5\": {\n \"acc\": 0.5240963855421686,\n \"acc_stderr\": 0.03887971849597264,\n \"acc_norm\": 0.5240963855421686,\n \"acc_norm_stderr\": 0.03887971849597264\n },\n \"harness|hendrycksTest-world_religions|5\": {\n \"acc\": 0.8128654970760234,\n \"acc_stderr\": 0.02991312723236804,\n \"acc_norm\": 0.8128654970760234,\n \"acc_norm_stderr\": 0.02991312723236804\n },\n \"harness|truthfulqa:mc|0\": {\n \"mc1\": 0.3659730722154223,\n \"mc1_stderr\": 0.016862941684088365,\n \"mc2\": 0.5323239517078452,\n \"mc2_stderr\": 0.0151650266597209\n },\n \"harness|winogrande|5\": {\n \"acc\": 0.7845303867403315,\n \"acc_stderr\": 0.011555295286059279\n },\n \"harness|gsm8k|5\": {\n \"acc\": 0.6914329037149356,\n \"acc_stderr\": 0.012723076049815896\n }\n}\n```", "repo_url": "https://huggingface.co/abacusai/Fewshot-Metamath-OrcaVicuna-Mistral", "leaderboard_url": "https://huggingface.co/spaces/HuggingFaceH4/open_llm_leaderboard", "point_of_contact": "[email protected]", "configs": [{"config_name": "harness_arc_challenge_25", "data_files": [{"split": "2024_01_10T15_33_11.471365", "path": ["**/details_harness|arc:challenge|25_2024-01-10T15-33-11.471365.parquet"]}, {"split": "latest", "path": ["**/details_harness|arc:challenge|25_2024-01-10T15-33-11.471365.parquet"]}]}, {"config_name": "harness_gsm8k_5", "data_files": [{"split": "2024_01_10T15_33_11.471365", "path": ["**/details_harness|gsm8k|5_2024-01-10T15-33-11.471365.parquet"]}, {"split": "latest", "path": ["**/details_harness|gsm8k|5_2024-01-10T15-33-11.471365.parquet"]}]}, {"config_name": "harness_hellaswag_10", "data_files": [{"split": "2024_01_10T15_33_11.471365", "path": ["**/details_harness|hellaswag|10_2024-01-10T15-33-11.471365.parquet"]}, {"split": "latest", "path": ["**/details_harness|hellaswag|10_2024-01-10T15-33-11.471365.parquet"]}]}, {"config_name": "harness_hendrycksTest_5", "data_files": [{"split": "2024_01_10T15_33_11.471365", "path": ["**/details_harness|hendrycksTest-abstract_algebra|5_2024-01-10T15-33-11.471365.parquet", "**/details_harness|hendrycksTest-anatomy|5_2024-01-10T15-33-11.471365.parquet", "**/details_harness|hendrycksTest-astronomy|5_2024-01-10T15-33-11.471365.parquet", "**/details_harness|hendrycksTest-business_ethics|5_2024-01-10T15-33-11.471365.parquet", "**/details_harness|hendrycksTest-clinical_knowledge|5_2024-01-10T15-33-11.471365.parquet", "**/details_harness|hendrycksTest-college_biology|5_2024-01-10T15-33-11.471365.parquet", "**/details_harness|hendrycksTest-college_chemistry|5_2024-01-10T15-33-11.471365.parquet", "**/details_harness|hendrycksTest-college_computer_science|5_2024-01-10T15-33-11.471365.parquet", "**/details_harness|hendrycksTest-college_mathematics|5_2024-01-10T15-33-11.471365.parquet", "**/details_harness|hendrycksTest-college_medicine|5_2024-01-10T15-33-11.471365.parquet", "**/details_harness|hendrycksTest-college_physics|5_2024-01-10T15-33-11.471365.parquet", "**/details_harness|hendrycksTest-computer_security|5_2024-01-10T15-33-11.471365.parquet", "**/details_harness|hendrycksTest-conceptual_physics|5_2024-01-10T15-33-11.471365.parquet", "**/details_harness|hendrycksTest-econometrics|5_2024-01-10T15-33-11.471365.parquet", "**/details_harness|hendrycksTest-electrical_engineering|5_2024-01-10T15-33-11.471365.parquet", "**/details_harness|hendrycksTest-elementary_mathematics|5_2024-01-10T15-33-11.471365.parquet", "**/details_harness|hendrycksTest-formal_logic|5_2024-01-10T15-33-11.471365.parquet", "**/details_harness|hendrycksTest-global_facts|5_2024-01-10T15-33-11.471365.parquet", "**/details_harness|hendrycksTest-high_school_biology|5_2024-01-10T15-33-11.471365.parquet", "**/details_harness|hendrycksTest-high_school_chemistry|5_2024-01-10T15-33-11.471365.parquet", "**/details_harness|hendrycksTest-high_school_computer_science|5_2024-01-10T15-33-11.471365.parquet", "**/details_harness|hendrycksTest-high_school_european_history|5_2024-01-10T15-33-11.471365.parquet", "**/details_harness|hendrycksTest-high_school_geography|5_2024-01-10T15-33-11.471365.parquet", "**/details_harness|hendrycksTest-high_school_government_and_politics|5_2024-01-10T15-33-11.471365.parquet", "**/details_harness|hendrycksTest-high_school_macroeconomics|5_2024-01-10T15-33-11.471365.parquet", "**/details_harness|hendrycksTest-high_school_mathematics|5_2024-01-10T15-33-11.471365.parquet", "**/details_harness|hendrycksTest-high_school_microeconomics|5_2024-01-10T15-33-11.471365.parquet", "**/details_harness|hendrycksTest-high_school_physics|5_2024-01-10T15-33-11.471365.parquet", "**/details_harness|hendrycksTest-high_school_psychology|5_2024-01-10T15-33-11.471365.parquet", "**/details_harness|hendrycksTest-high_school_statistics|5_2024-01-10T15-33-11.471365.parquet", "**/details_harness|hendrycksTest-high_school_us_history|5_2024-01-10T15-33-11.471365.parquet", "**/details_harness|hendrycksTest-high_school_world_history|5_2024-01-10T15-33-11.471365.parquet", "**/details_harness|hendrycksTest-human_aging|5_2024-01-10T15-33-11.471365.parquet", "**/details_harness|hendrycksTest-human_sexuality|5_2024-01-10T15-33-11.471365.parquet", "**/details_harness|hendrycksTest-international_law|5_2024-01-10T15-33-11.471365.parquet", "**/details_harness|hendrycksTest-jurisprudence|5_2024-01-10T15-33-11.471365.parquet", "**/details_harness|hendrycksTest-logical_fallacies|5_2024-01-10T15-33-11.471365.parquet", "**/details_harness|hendrycksTest-machine_learning|5_2024-01-10T15-33-11.471365.parquet", "**/details_harness|hendrycksTest-management|5_2024-01-10T15-33-11.471365.parquet", "**/details_harness|hendrycksTest-marketing|5_2024-01-10T15-33-11.471365.parquet", "**/details_harness|hendrycksTest-medical_genetics|5_2024-01-10T15-33-11.471365.parquet", "**/details_harness|hendrycksTest-miscellaneous|5_2024-01-10T15-33-11.471365.parquet", "**/details_harness|hendrycksTest-moral_disputes|5_2024-01-10T15-33-11.471365.parquet", "**/details_harness|hendrycksTest-moral_scenarios|5_2024-01-10T15-33-11.471365.parquet", "**/details_harness|hendrycksTest-nutrition|5_2024-01-10T15-33-11.471365.parquet", "**/details_harness|hendrycksTest-philosophy|5_2024-01-10T15-33-11.471365.parquet", "**/details_harness|hendrycksTest-prehistory|5_2024-01-10T15-33-11.471365.parquet", "**/details_harness|hendrycksTest-professional_accounting|5_2024-01-10T15-33-11.471365.parquet", "**/details_harness|hendrycksTest-professional_law|5_2024-01-10T15-33-11.471365.parquet", "**/details_harness|hendrycksTest-professional_medicine|5_2024-01-10T15-33-11.471365.parquet", "**/details_harness|hendrycksTest-professional_psychology|5_2024-01-10T15-33-11.471365.parquet", "**/details_harness|hendrycksTest-public_relations|5_2024-01-10T15-33-11.471365.parquet", "**/details_harness|hendrycksTest-security_studies|5_2024-01-10T15-33-11.471365.parquet", "**/details_harness|hendrycksTest-sociology|5_2024-01-10T15-33-11.471365.parquet", "**/details_harness|hendrycksTest-us_foreign_policy|5_2024-01-10T15-33-11.471365.parquet", "**/details_harness|hendrycksTest-virology|5_2024-01-10T15-33-11.471365.parquet", "**/details_harness|hendrycksTest-world_religions|5_2024-01-10T15-33-11.471365.parquet"]}, {"split": "latest", "path": ["**/details_harness|hendrycksTest-abstract_algebra|5_2024-01-10T15-33-11.471365.parquet", "**/details_harness|hendrycksTest-anatomy|5_2024-01-10T15-33-11.471365.parquet", "**/details_harness|hendrycksTest-astronomy|5_2024-01-10T15-33-11.471365.parquet", "**/details_harness|hendrycksTest-business_ethics|5_2024-01-10T15-33-11.471365.parquet", "**/details_harness|hendrycksTest-clinical_knowledge|5_2024-01-10T15-33-11.471365.parquet", "**/details_harness|hendrycksTest-college_biology|5_2024-01-10T15-33-11.471365.parquet", "**/details_harness|hendrycksTest-college_chemistry|5_2024-01-10T15-33-11.471365.parquet", "**/details_harness|hendrycksTest-college_computer_science|5_2024-01-10T15-33-11.471365.parquet", "**/details_harness|hendrycksTest-college_mathematics|5_2024-01-10T15-33-11.471365.parquet", "**/details_harness|hendrycksTest-college_medicine|5_2024-01-10T15-33-11.471365.parquet", "**/details_harness|hendrycksTest-college_physics|5_2024-01-10T15-33-11.471365.parquet", "**/details_harness|hendrycksTest-computer_security|5_2024-01-10T15-33-11.471365.parquet", "**/details_harness|hendrycksTest-conceptual_physics|5_2024-01-10T15-33-11.471365.parquet", "**/details_harness|hendrycksTest-econometrics|5_2024-01-10T15-33-11.471365.parquet", "**/details_harness|hendrycksTest-electrical_engineering|5_2024-01-10T15-33-11.471365.parquet", "**/details_harness|hendrycksTest-elementary_mathematics|5_2024-01-10T15-33-11.471365.parquet", "**/details_harness|hendrycksTest-formal_logic|5_2024-01-10T15-33-11.471365.parquet", "**/details_harness|hendrycksTest-global_facts|5_2024-01-10T15-33-11.471365.parquet", "**/details_harness|hendrycksTest-high_school_biology|5_2024-01-10T15-33-11.471365.parquet", "**/details_harness|hendrycksTest-high_school_chemistry|5_2024-01-10T15-33-11.471365.parquet", "**/details_harness|hendrycksTest-high_school_computer_science|5_2024-01-10T15-33-11.471365.parquet", "**/details_harness|hendrycksTest-high_school_european_history|5_2024-01-10T15-33-11.471365.parquet", "**/details_harness|hendrycksTest-high_school_geography|5_2024-01-10T15-33-11.471365.parquet", "**/details_harness|hendrycksTest-high_school_government_and_politics|5_2024-01-10T15-33-11.471365.parquet", "**/details_harness|hendrycksTest-high_school_macroeconomics|5_2024-01-10T15-33-11.471365.parquet", "**/details_harness|hendrycksTest-high_school_mathematics|5_2024-01-10T15-33-11.471365.parquet", "**/details_harness|hendrycksTest-high_school_microeconomics|5_2024-01-10T15-33-11.471365.parquet", "**/details_harness|hendrycksTest-high_school_physics|5_2024-01-10T15-33-11.471365.parquet", "**/details_harness|hendrycksTest-high_school_psychology|5_2024-01-10T15-33-11.471365.parquet", "**/details_harness|hendrycksTest-high_school_statistics|5_2024-01-10T15-33-11.471365.parquet", "**/details_harness|hendrycksTest-high_school_us_history|5_2024-01-10T15-33-11.471365.parquet", "**/details_harness|hendrycksTest-high_school_world_history|5_2024-01-10T15-33-11.471365.parquet", "**/details_harness|hendrycksTest-human_aging|5_2024-01-10T15-33-11.471365.parquet", "**/details_harness|hendrycksTest-human_sexuality|5_2024-01-10T15-33-11.471365.parquet", "**/details_harness|hendrycksTest-international_law|5_2024-01-10T15-33-11.471365.parquet", "**/details_harness|hendrycksTest-jurisprudence|5_2024-01-10T15-33-11.471365.parquet", "**/details_harness|hendrycksTest-logical_fallacies|5_2024-01-10T15-33-11.471365.parquet", "**/details_harness|hendrycksTest-machine_learning|5_2024-01-10T15-33-11.471365.parquet", "**/details_harness|hendrycksTest-management|5_2024-01-10T15-33-11.471365.parquet", "**/details_harness|hendrycksTest-marketing|5_2024-01-10T15-33-11.471365.parquet", "**/details_harness|hendrycksTest-medical_genetics|5_2024-01-10T15-33-11.471365.parquet", "**/details_harness|hendrycksTest-miscellaneous|5_2024-01-10T15-33-11.471365.parquet", "**/details_harness|hendrycksTest-moral_disputes|5_2024-01-10T15-33-11.471365.parquet", "**/details_harness|hendrycksTest-moral_scenarios|5_2024-01-10T15-33-11.471365.parquet", "**/details_harness|hendrycksTest-nutrition|5_2024-01-10T15-33-11.471365.parquet", "**/details_harness|hendrycksTest-philosophy|5_2024-01-10T15-33-11.471365.parquet", "**/details_harness|hendrycksTest-prehistory|5_2024-01-10T15-33-11.471365.parquet", "**/details_harness|hendrycksTest-professional_accounting|5_2024-01-10T15-33-11.471365.parquet", "**/details_harness|hendrycksTest-professional_law|5_2024-01-10T15-33-11.471365.parquet", "**/details_harness|hendrycksTest-professional_medicine|5_2024-01-10T15-33-11.471365.parquet", "**/details_harness|hendrycksTest-professional_psychology|5_2024-01-10T15-33-11.471365.parquet", "**/details_harness|hendrycksTest-public_relations|5_2024-01-10T15-33-11.471365.parquet", "**/details_harness|hendrycksTest-security_studies|5_2024-01-10T15-33-11.471365.parquet", "**/details_harness|hendrycksTest-sociology|5_2024-01-10T15-33-11.471365.parquet", "**/details_harness|hendrycksTest-us_foreign_policy|5_2024-01-10T15-33-11.471365.parquet", "**/details_harness|hendrycksTest-virology|5_2024-01-10T15-33-11.471365.parquet", "**/details_harness|hendrycksTest-world_religions|5_2024-01-10T15-33-11.471365.parquet"]}]}, {"config_name": "harness_hendrycksTest_abstract_algebra_5", "data_files": [{"split": "2024_01_10T15_33_11.471365", "path": ["**/details_harness|hendrycksTest-abstract_algebra|5_2024-01-10T15-33-11.471365.parquet"]}, {"split": "latest", "path": ["**/details_harness|hendrycksTest-abstract_algebra|5_2024-01-10T15-33-11.471365.parquet"]}]}, {"config_name": "harness_hendrycksTest_anatomy_5", "data_files": [{"split": "2024_01_10T15_33_11.471365", "path": ["**/details_harness|hendrycksTest-anatomy|5_2024-01-10T15-33-11.471365.parquet"]}, {"split": "latest", "path": ["**/details_harness|hendrycksTest-anatomy|5_2024-01-10T15-33-11.471365.parquet"]}]}, {"config_name": "harness_hendrycksTest_astronomy_5", "data_files": [{"split": "2024_01_10T15_33_11.471365", "path": ["**/details_harness|hendrycksTest-astronomy|5_2024-01-10T15-33-11.471365.parquet"]}, {"split": "latest", "path": ["**/details_harness|hendrycksTest-astronomy|5_2024-01-10T15-33-11.471365.parquet"]}]}, {"config_name": "harness_hendrycksTest_business_ethics_5", "data_files": [{"split": "2024_01_10T15_33_11.471365", "path": ["**/details_harness|hendrycksTest-business_ethics|5_2024-01-10T15-33-11.471365.parquet"]}, {"split": "latest", "path": ["**/details_harness|hendrycksTest-business_ethics|5_2024-01-10T15-33-11.471365.parquet"]}]}, {"config_name": "harness_hendrycksTest_clinical_knowledge_5", "data_files": [{"split": "2024_01_10T15_33_11.471365", "path": ["**/details_harness|hendrycksTest-clinical_knowledge|5_2024-01-10T15-33-11.471365.parquet"]}, {"split": "latest", "path": ["**/details_harness|hendrycksTest-clinical_knowledge|5_2024-01-10T15-33-11.471365.parquet"]}]}, {"config_name": "harness_hendrycksTest_college_biology_5", "data_files": [{"split": "2024_01_10T15_33_11.471365", "path": ["**/details_harness|hendrycksTest-college_biology|5_2024-01-10T15-33-11.471365.parquet"]}, {"split": "latest", "path": ["**/details_harness|hendrycksTest-college_biology|5_2024-01-10T15-33-11.471365.parquet"]}]}, {"config_name": "harness_hendrycksTest_college_chemistry_5", "data_files": [{"split": "2024_01_10T15_33_11.471365", "path": ["**/details_harness|hendrycksTest-college_chemistry|5_2024-01-10T15-33-11.471365.parquet"]}, {"split": "latest", "path": ["**/details_harness|hendrycksTest-college_chemistry|5_2024-01-10T15-33-11.471365.parquet"]}]}, {"config_name": "harness_hendrycksTest_college_computer_science_5", "data_files": [{"split": "2024_01_10T15_33_11.471365", "path": ["**/details_harness|hendrycksTest-college_computer_science|5_2024-01-10T15-33-11.471365.parquet"]}, {"split": "latest", "path": ["**/details_harness|hendrycksTest-college_computer_science|5_2024-01-10T15-33-11.471365.parquet"]}]}, {"config_name": "harness_hendrycksTest_college_mathematics_5", "data_files": [{"split": "2024_01_10T15_33_11.471365", "path": ["**/details_harness|hendrycksTest-college_mathematics|5_2024-01-10T15-33-11.471365.parquet"]}, {"split": "latest", "path": ["**/details_harness|hendrycksTest-college_mathematics|5_2024-01-10T15-33-11.471365.parquet"]}]}, {"config_name": "harness_hendrycksTest_college_medicine_5", "data_files": [{"split": "2024_01_10T15_33_11.471365", "path": ["**/details_harness|hendrycksTest-college_medicine|5_2024-01-10T15-33-11.471365.parquet"]}, {"split": "latest", "path": ["**/details_harness|hendrycksTest-college_medicine|5_2024-01-10T15-33-11.471365.parquet"]}]}, {"config_name": "harness_hendrycksTest_college_physics_5", "data_files": [{"split": "2024_01_10T15_33_11.471365", "path": ["**/details_harness|hendrycksTest-college_physics|5_2024-01-10T15-33-11.471365.parquet"]}, {"split": "latest", "path": ["**/details_harness|hendrycksTest-college_physics|5_2024-01-10T15-33-11.471365.parquet"]}]}, {"config_name": "harness_hendrycksTest_computer_security_5", "data_files": [{"split": "2024_01_10T15_33_11.471365", "path": ["**/details_harness|hendrycksTest-computer_security|5_2024-01-10T15-33-11.471365.parquet"]}, {"split": "latest", "path": ["**/details_harness|hendrycksTest-computer_security|5_2024-01-10T15-33-11.471365.parquet"]}]}, {"config_name": "harness_hendrycksTest_conceptual_physics_5", "data_files": [{"split": "2024_01_10T15_33_11.471365", "path": ["**/details_harness|hendrycksTest-conceptual_physics|5_2024-01-10T15-33-11.471365.parquet"]}, {"split": "latest", "path": ["**/details_harness|hendrycksTest-conceptual_physics|5_2024-01-10T15-33-11.471365.parquet"]}]}, {"config_name": "harness_hendrycksTest_econometrics_5", "data_files": [{"split": "2024_01_10T15_33_11.471365", "path": ["**/details_harness|hendrycksTest-econometrics|5_2024-01-10T15-33-11.471365.parquet"]}, {"split": "latest", "path": ["**/details_harness|hendrycksTest-econometrics|5_2024-01-10T15-33-11.471365.parquet"]}]}, {"config_name": "harness_hendrycksTest_electrical_engineering_5", "data_files": [{"split": "2024_01_10T15_33_11.471365", "path": ["**/details_harness|hendrycksTest-electrical_engineering|5_2024-01-10T15-33-11.471365.parquet"]}, {"split": "latest", "path": ["**/details_harness|hendrycksTest-electrical_engineering|5_2024-01-10T15-33-11.471365.parquet"]}]}, {"config_name": "harness_hendrycksTest_elementary_mathematics_5", "data_files": [{"split": "2024_01_10T15_33_11.471365", "path": ["**/details_harness|hendrycksTest-elementary_mathematics|5_2024-01-10T15-33-11.471365.parquet"]}, {"split": "latest", "path": ["**/details_harness|hendrycksTest-elementary_mathematics|5_2024-01-10T15-33-11.471365.parquet"]}]}, {"config_name": "harness_hendrycksTest_formal_logic_5", "data_files": [{"split": "2024_01_10T15_33_11.471365", "path": ["**/details_harness|hendrycksTest-formal_logic|5_2024-01-10T15-33-11.471365.parquet"]}, {"split": "latest", "path": ["**/details_harness|hendrycksTest-formal_logic|5_2024-01-10T15-33-11.471365.parquet"]}]}, {"config_name": "harness_hendrycksTest_global_facts_5", "data_files": [{"split": "2024_01_10T15_33_11.471365", "path": ["**/details_harness|hendrycksTest-global_facts|5_2024-01-10T15-33-11.471365.parquet"]}, {"split": "latest", "path": ["**/details_harness|hendrycksTest-global_facts|5_2024-01-10T15-33-11.471365.parquet"]}]}, {"config_name": "harness_hendrycksTest_high_school_biology_5", "data_files": [{"split": "2024_01_10T15_33_11.471365", "path": ["**/details_harness|hendrycksTest-high_school_biology|5_2024-01-10T15-33-11.471365.parquet"]}, {"split": "latest", "path": ["**/details_harness|hendrycksTest-high_school_biology|5_2024-01-10T15-33-11.471365.parquet"]}]}, {"config_name": "harness_hendrycksTest_high_school_chemistry_5", "data_files": [{"split": "2024_01_10T15_33_11.471365", "path": ["**/details_harness|hendrycksTest-high_school_chemistry|5_2024-01-10T15-33-11.471365.parquet"]}, {"split": "latest", "path": ["**/details_harness|hendrycksTest-high_school_chemistry|5_2024-01-10T15-33-11.471365.parquet"]}]}, {"config_name": "harness_hendrycksTest_high_school_computer_science_5", "data_files": [{"split": "2024_01_10T15_33_11.471365", "path": ["**/details_harness|hendrycksTest-high_school_computer_science|5_2024-01-10T15-33-11.471365.parquet"]}, {"split": "latest", "path": ["**/details_harness|hendrycksTest-high_school_computer_science|5_2024-01-10T15-33-11.471365.parquet"]}]}, {"config_name": "harness_hendrycksTest_high_school_european_history_5", "data_files": [{"split": "2024_01_10T15_33_11.471365", "path": ["**/details_harness|hendrycksTest-high_school_european_history|5_2024-01-10T15-33-11.471365.parquet"]}, {"split": "latest", "path": ["**/details_harness|hendrycksTest-high_school_european_history|5_2024-01-10T15-33-11.471365.parquet"]}]}, {"config_name": "harness_hendrycksTest_high_school_geography_5", "data_files": [{"split": "2024_01_10T15_33_11.471365", "path": ["**/details_harness|hendrycksTest-high_school_geography|5_2024-01-10T15-33-11.471365.parquet"]}, {"split": "latest", "path": ["**/details_harness|hendrycksTest-high_school_geography|5_2024-01-10T15-33-11.471365.parquet"]}]}, {"config_name": "harness_hendrycksTest_high_school_government_and_politics_5", "data_files": [{"split": "2024_01_10T15_33_11.471365", "path": ["**/details_harness|hendrycksTest-high_school_government_and_politics|5_2024-01-10T15-33-11.471365.parquet"]}, {"split": "latest", "path": ["**/details_harness|hendrycksTest-high_school_government_and_politics|5_2024-01-10T15-33-11.471365.parquet"]}]}, {"config_name": "harness_hendrycksTest_high_school_macroeconomics_5", "data_files": [{"split": "2024_01_10T15_33_11.471365", "path": ["**/details_harness|hendrycksTest-high_school_macroeconomics|5_2024-01-10T15-33-11.471365.parquet"]}, {"split": "latest", "path": ["**/details_harness|hendrycksTest-high_school_macroeconomics|5_2024-01-10T15-33-11.471365.parquet"]}]}, {"config_name": "harness_hendrycksTest_high_school_mathematics_5", "data_files": [{"split": "2024_01_10T15_33_11.471365", "path": ["**/details_harness|hendrycksTest-high_school_mathematics|5_2024-01-10T15-33-11.471365.parquet"]}, {"split": "latest", "path": ["**/details_harness|hendrycksTest-high_school_mathematics|5_2024-01-10T15-33-11.471365.parquet"]}]}, {"config_name": "harness_hendrycksTest_high_school_microeconomics_5", "data_files": [{"split": "2024_01_10T15_33_11.471365", "path": ["**/details_harness|hendrycksTest-high_school_microeconomics|5_2024-01-10T15-33-11.471365.parquet"]}, {"split": "latest", "path": ["**/details_harness|hendrycksTest-high_school_microeconomics|5_2024-01-10T15-33-11.471365.parquet"]}]}, {"config_name": "harness_hendrycksTest_high_school_physics_5", "data_files": [{"split": "2024_01_10T15_33_11.471365", "path": ["**/details_harness|hendrycksTest-high_school_physics|5_2024-01-10T15-33-11.471365.parquet"]}, {"split": "latest", "path": ["**/details_harness|hendrycksTest-high_school_physics|5_2024-01-10T15-33-11.471365.parquet"]}]}, {"config_name": "harness_hendrycksTest_high_school_psychology_5", "data_files": [{"split": "2024_01_10T15_33_11.471365", "path": ["**/details_harness|hendrycksTest-high_school_psychology|5_2024-01-10T15-33-11.471365.parquet"]}, {"split": "latest", "path": ["**/details_harness|hendrycksTest-high_school_psychology|5_2024-01-10T15-33-11.471365.parquet"]}]}, {"config_name": "harness_hendrycksTest_high_school_statistics_5", "data_files": [{"split": "2024_01_10T15_33_11.471365", "path": ["**/details_harness|hendrycksTest-high_school_statistics|5_2024-01-10T15-33-11.471365.parquet"]}, {"split": "latest", "path": ["**/details_harness|hendrycksTest-high_school_statistics|5_2024-01-10T15-33-11.471365.parquet"]}]}, {"config_name": "harness_hendrycksTest_high_school_us_history_5", "data_files": [{"split": "2024_01_10T15_33_11.471365", "path": ["**/details_harness|hendrycksTest-high_school_us_history|5_2024-01-10T15-33-11.471365.parquet"]}, {"split": "latest", "path": ["**/details_harness|hendrycksTest-high_school_us_history|5_2024-01-10T15-33-11.471365.parquet"]}]}, {"config_name": "harness_hendrycksTest_high_school_world_history_5", "data_files": [{"split": "2024_01_10T15_33_11.471365", "path": ["**/details_harness|hendrycksTest-high_school_world_history|5_2024-01-10T15-33-11.471365.parquet"]}, {"split": "latest", "path": ["**/details_harness|hendrycksTest-high_school_world_history|5_2024-01-10T15-33-11.471365.parquet"]}]}, {"config_name": "harness_hendrycksTest_human_aging_5", "data_files": [{"split": "2024_01_10T15_33_11.471365", "path": ["**/details_harness|hendrycksTest-human_aging|5_2024-01-10T15-33-11.471365.parquet"]}, {"split": "latest", "path": ["**/details_harness|hendrycksTest-human_aging|5_2024-01-10T15-33-11.471365.parquet"]}]}, {"config_name": "harness_hendrycksTest_human_sexuality_5", "data_files": [{"split": "2024_01_10T15_33_11.471365", "path": ["**/details_harness|hendrycksTest-human_sexuality|5_2024-01-10T15-33-11.471365.parquet"]}, {"split": "latest", "path": ["**/details_harness|hendrycksTest-human_sexuality|5_2024-01-10T15-33-11.471365.parquet"]}]}, {"config_name": "harness_hendrycksTest_international_law_5", "data_files": [{"split": "2024_01_10T15_33_11.471365", "path": ["**/details_harness|hendrycksTest-international_law|5_2024-01-10T15-33-11.471365.parquet"]}, {"split": "latest", "path": ["**/details_harness|hendrycksTest-international_law|5_2024-01-10T15-33-11.471365.parquet"]}]}, {"config_name": "harness_hendrycksTest_jurisprudence_5", "data_files": [{"split": "2024_01_10T15_33_11.471365", "path": ["**/details_harness|hendrycksTest-jurisprudence|5_2024-01-10T15-33-11.471365.parquet"]}, {"split": "latest", "path": ["**/details_harness|hendrycksTest-jurisprudence|5_2024-01-10T15-33-11.471365.parquet"]}]}, {"config_name": "harness_hendrycksTest_logical_fallacies_5", "data_files": [{"split": "2024_01_10T15_33_11.471365", "path": ["**/details_harness|hendrycksTest-logical_fallacies|5_2024-01-10T15-33-11.471365.parquet"]}, {"split": "latest", "path": ["**/details_harness|hendrycksTest-logical_fallacies|5_2024-01-10T15-33-11.471365.parquet"]}]}, {"config_name": "harness_hendrycksTest_machine_learning_5", "data_files": [{"split": "2024_01_10T15_33_11.471365", "path": ["**/details_harness|hendrycksTest-machine_learning|5_2024-01-10T15-33-11.471365.parquet"]}, {"split": "latest", "path": ["**/details_harness|hendrycksTest-machine_learning|5_2024-01-10T15-33-11.471365.parquet"]}]}, {"config_name": "harness_hendrycksTest_management_5", "data_files": [{"split": "2024_01_10T15_33_11.471365", "path": ["**/details_harness|hendrycksTest-management|5_2024-01-10T15-33-11.471365.parquet"]}, {"split": "latest", "path": ["**/details_harness|hendrycksTest-management|5_2024-01-10T15-33-11.471365.parquet"]}]}, {"config_name": "harness_hendrycksTest_marketing_5", "data_files": [{"split": "2024_01_10T15_33_11.471365", "path": ["**/details_harness|hendrycksTest-marketing|5_2024-01-10T15-33-11.471365.parquet"]}, {"split": "latest", "path": ["**/details_harness|hendrycksTest-marketing|5_2024-01-10T15-33-11.471365.parquet"]}]}, {"config_name": "harness_hendrycksTest_medical_genetics_5", "data_files": [{"split": "2024_01_10T15_33_11.471365", "path": ["**/details_harness|hendrycksTest-medical_genetics|5_2024-01-10T15-33-11.471365.parquet"]}, {"split": "latest", "path": ["**/details_harness|hendrycksTest-medical_genetics|5_2024-01-10T15-33-11.471365.parquet"]}]}, {"config_name": "harness_hendrycksTest_miscellaneous_5", "data_files": [{"split": "2024_01_10T15_33_11.471365", "path": ["**/details_harness|hendrycksTest-miscellaneous|5_2024-01-10T15-33-11.471365.parquet"]}, {"split": "latest", "path": ["**/details_harness|hendrycksTest-miscellaneous|5_2024-01-10T15-33-11.471365.parquet"]}]}, {"config_name": "harness_hendrycksTest_moral_disputes_5", "data_files": [{"split": "2024_01_10T15_33_11.471365", "path": ["**/details_harness|hendrycksTest-moral_disputes|5_2024-01-10T15-33-11.471365.parquet"]}, {"split": "latest", "path": ["**/details_harness|hendrycksTest-moral_disputes|5_2024-01-10T15-33-11.471365.parquet"]}]}, {"config_name": "harness_hendrycksTest_moral_scenarios_5", "data_files": [{"split": "2024_01_10T15_33_11.471365", "path": ["**/details_harness|hendrycksTest-moral_scenarios|5_2024-01-10T15-33-11.471365.parquet"]}, {"split": "latest", "path": ["**/details_harness|hendrycksTest-moral_scenarios|5_2024-01-10T15-33-11.471365.parquet"]}]}, {"config_name": "harness_hendrycksTest_nutrition_5", "data_files": [{"split": "2024_01_10T15_33_11.471365", "path": ["**/details_harness|hendrycksTest-nutrition|5_2024-01-10T15-33-11.471365.parquet"]}, {"split": "latest", "path": ["**/details_harness|hendrycksTest-nutrition|5_2024-01-10T15-33-11.471365.parquet"]}]}, {"config_name": "harness_hendrycksTest_philosophy_5", "data_files": [{"split": "2024_01_10T15_33_11.471365", "path": ["**/details_harness|hendrycksTest-philosophy|5_2024-01-10T15-33-11.471365.parquet"]}, {"split": "latest", "path": ["**/details_harness|hendrycksTest-philosophy|5_2024-01-10T15-33-11.471365.parquet"]}]}, {"config_name": "harness_hendrycksTest_prehistory_5", "data_files": [{"split": "2024_01_10T15_33_11.471365", "path": ["**/details_harness|hendrycksTest-prehistory|5_2024-01-10T15-33-11.471365.parquet"]}, {"split": "latest", "path": ["**/details_harness|hendrycksTest-prehistory|5_2024-01-10T15-33-11.471365.parquet"]}]}, {"config_name": "harness_hendrycksTest_professional_accounting_5", "data_files": [{"split": "2024_01_10T15_33_11.471365", "path": ["**/details_harness|hendrycksTest-professional_accounting|5_2024-01-10T15-33-11.471365.parquet"]}, {"split": "latest", "path": ["**/details_harness|hendrycksTest-professional_accounting|5_2024-01-10T15-33-11.471365.parquet"]}]}, {"config_name": "harness_hendrycksTest_professional_law_5", "data_files": [{"split": "2024_01_10T15_33_11.471365", "path": ["**/details_harness|hendrycksTest-professional_law|5_2024-01-10T15-33-11.471365.parquet"]}, {"split": "latest", "path": ["**/details_harness|hendrycksTest-professional_law|5_2024-01-10T15-33-11.471365.parquet"]}]}, {"config_name": "harness_hendrycksTest_professional_medicine_5", "data_files": [{"split": "2024_01_10T15_33_11.471365", "path": ["**/details_harness|hendrycksTest-professional_medicine|5_2024-01-10T15-33-11.471365.parquet"]}, {"split": "latest", "path": ["**/details_harness|hendrycksTest-professional_medicine|5_2024-01-10T15-33-11.471365.parquet"]}]}, {"config_name": "harness_hendrycksTest_professional_psychology_5", "data_files": [{"split": "2024_01_10T15_33_11.471365", "path": ["**/details_harness|hendrycksTest-professional_psychology|5_2024-01-10T15-33-11.471365.parquet"]}, {"split": "latest", "path": ["**/details_harness|hendrycksTest-professional_psychology|5_2024-01-10T15-33-11.471365.parquet"]}]}, {"config_name": "harness_hendrycksTest_public_relations_5", "data_files": [{"split": "2024_01_10T15_33_11.471365", "path": ["**/details_harness|hendrycksTest-public_relations|5_2024-01-10T15-33-11.471365.parquet"]}, {"split": "latest", "path": ["**/details_harness|hendrycksTest-public_relations|5_2024-01-10T15-33-11.471365.parquet"]}]}, {"config_name": "harness_hendrycksTest_security_studies_5", "data_files": [{"split": "2024_01_10T15_33_11.471365", "path": ["**/details_harness|hendrycksTest-security_studies|5_2024-01-10T15-33-11.471365.parquet"]}, {"split": "latest", "path": ["**/details_harness|hendrycksTest-security_studies|5_2024-01-10T15-33-11.471365.parquet"]}]}, {"config_name": "harness_hendrycksTest_sociology_5", "data_files": [{"split": "2024_01_10T15_33_11.471365", "path": ["**/details_harness|hendrycksTest-sociology|5_2024-01-10T15-33-11.471365.parquet"]}, {"split": "latest", "path": ["**/details_harness|hendrycksTest-sociology|5_2024-01-10T15-33-11.471365.parquet"]}]}, {"config_name": "harness_hendrycksTest_us_foreign_policy_5", "data_files": [{"split": "2024_01_10T15_33_11.471365", "path": ["**/details_harness|hendrycksTest-us_foreign_policy|5_2024-01-10T15-33-11.471365.parquet"]}, {"split": "latest", "path": ["**/details_harness|hendrycksTest-us_foreign_policy|5_2024-01-10T15-33-11.471365.parquet"]}]}, {"config_name": "harness_hendrycksTest_virology_5", "data_files": [{"split": "2024_01_10T15_33_11.471365", "path": ["**/details_harness|hendrycksTest-virology|5_2024-01-10T15-33-11.471365.parquet"]}, {"split": "latest", "path": ["**/details_harness|hendrycksTest-virology|5_2024-01-10T15-33-11.471365.parquet"]}]}, {"config_name": "harness_hendrycksTest_world_religions_5", "data_files": [{"split": "2024_01_10T15_33_11.471365", "path": ["**/details_harness|hendrycksTest-world_religions|5_2024-01-10T15-33-11.471365.parquet"]}, {"split": "latest", "path": ["**/details_harness|hendrycksTest-world_religions|5_2024-01-10T15-33-11.471365.parquet"]}]}, {"config_name": "harness_truthfulqa_mc_0", "data_files": [{"split": "2024_01_10T15_33_11.471365", "path": ["**/details_harness|truthfulqa:mc|0_2024-01-10T15-33-11.471365.parquet"]}, {"split": "latest", "path": ["**/details_harness|truthfulqa:mc|0_2024-01-10T15-33-11.471365.parquet"]}]}, {"config_name": "harness_winogrande_5", "data_files": [{"split": "2024_01_10T15_33_11.471365", "path": ["**/details_harness|winogrande|5_2024-01-10T15-33-11.471365.parquet"]}, {"split": "latest", "path": ["**/details_harness|winogrande|5_2024-01-10T15-33-11.471365.parquet"]}]}, {"config_name": "results", "data_files": [{"split": "2024_01_10T15_33_11.471365", "path": ["results_2024-01-10T15-33-11.471365.parquet"]}, {"split": "latest", "path": ["results_2024-01-10T15-33-11.471365.parquet"]}]}]} | 2024-01-10T15:35:55+00:00 | [] | [] | TAGS
#region-us
|
# Dataset Card for Evaluation run of abacusai/Fewshot-Metamath-OrcaVicuna-Mistral
Dataset automatically created during the evaluation run of model abacusai/Fewshot-Metamath-OrcaVicuna-Mistral on the Open LLM Leaderboard.
The dataset is composed of 63 configuration, each one coresponding to one of the evaluated task.
The dataset has been created from 1 run(s). Each run can be found as a specific split in each configuration, the split being named using the timestamp of the run.The "train" split is always pointing to the latest results.
An additional configuration "results" store all the aggregated results of the run (and is used to compute and display the aggregated metrics on the Open LLM Leaderboard).
To load the details from a run, you can for instance do the following:
## Latest results
These are the latest results from run 2024-01-10T15:33:11.471365(note that their might be results for other tasks in the repos if successive evals didn't cover the same tasks. You find each in the results and the "latest" split for each eval):
## Dataset Details
### Dataset Description
- Curated by:
- Funded by [optional]:
- Shared by [optional]:
- Language(s) (NLP):
- License:
### Dataset Sources [optional]
- Repository:
- Paper [optional]:
- Demo [optional]:
## Uses
### Direct Use
### Out-of-Scope Use
## Dataset Structure
## Dataset Creation
### Curation Rationale
### Source Data
#### Data Collection and Processing
#### Who are the source data producers?
### Annotations [optional]
#### Annotation process
#### Who are the annotators?
#### Personal and Sensitive Information
## Bias, Risks, and Limitations
### Recommendations
Users should be made aware of the risks, biases and limitations of the dataset. More information needed for further recommendations.
[optional]
BibTeX:
APA:
## Glossary [optional]
## More Information [optional]
## Dataset Card Authors [optional]
## Dataset Card Contact
| [
"# Dataset Card for Evaluation run of abacusai/Fewshot-Metamath-OrcaVicuna-Mistral\n\n\n\nDataset automatically created during the evaluation run of model abacusai/Fewshot-Metamath-OrcaVicuna-Mistral on the Open LLM Leaderboard.\n\nThe dataset is composed of 63 configuration, each one coresponding to one of the evaluated task.\n\nThe dataset has been created from 1 run(s). Each run can be found as a specific split in each configuration, the split being named using the timestamp of the run.The \"train\" split is always pointing to the latest results.\n\nAn additional configuration \"results\" store all the aggregated results of the run (and is used to compute and display the aggregated metrics on the Open LLM Leaderboard).\n\nTo load the details from a run, you can for instance do the following:",
"## Latest results\n\nThese are the latest results from run 2024-01-10T15:33:11.471365(note that their might be results for other tasks in the repos if successive evals didn't cover the same tasks. You find each in the results and the \"latest\" split for each eval):",
"## Dataset Details",
"### Dataset Description\n\n\n\n\n\n- Curated by: \n- Funded by [optional]: \n- Shared by [optional]: \n- Language(s) (NLP): \n- License:",
"### Dataset Sources [optional]\n\n\n\n- Repository: \n- Paper [optional]: \n- Demo [optional]:",
"## Uses",
"### Direct Use",
"### Out-of-Scope Use",
"## Dataset Structure",
"## Dataset Creation",
"### Curation Rationale",
"### Source Data",
"#### Data Collection and Processing",
"#### Who are the source data producers?",
"### Annotations [optional]",
"#### Annotation process",
"#### Who are the annotators?",
"#### Personal and Sensitive Information",
"## Bias, Risks, and Limitations",
"### Recommendations\n\n\n\nUsers should be made aware of the risks, biases and limitations of the dataset. More information needed for further recommendations.\n\n[optional]\n\n\n\nBibTeX:\n\n\n\nAPA:",
"## Glossary [optional]",
"## More Information [optional]",
"## Dataset Card Authors [optional]",
"## Dataset Card Contact"
] | [
"TAGS\n#region-us \n",
"# Dataset Card for Evaluation run of abacusai/Fewshot-Metamath-OrcaVicuna-Mistral\n\n\n\nDataset automatically created during the evaluation run of model abacusai/Fewshot-Metamath-OrcaVicuna-Mistral on the Open LLM Leaderboard.\n\nThe dataset is composed of 63 configuration, each one coresponding to one of the evaluated task.\n\nThe dataset has been created from 1 run(s). Each run can be found as a specific split in each configuration, the split being named using the timestamp of the run.The \"train\" split is always pointing to the latest results.\n\nAn additional configuration \"results\" store all the aggregated results of the run (and is used to compute and display the aggregated metrics on the Open LLM Leaderboard).\n\nTo load the details from a run, you can for instance do the following:",
"## Latest results\n\nThese are the latest results from run 2024-01-10T15:33:11.471365(note that their might be results for other tasks in the repos if successive evals didn't cover the same tasks. You find each in the results and the \"latest\" split for each eval):",
"## Dataset Details",
"### Dataset Description\n\n\n\n\n\n- Curated by: \n- Funded by [optional]: \n- Shared by [optional]: \n- Language(s) (NLP): \n- License:",
"### Dataset Sources [optional]\n\n\n\n- Repository: \n- Paper [optional]: \n- Demo [optional]:",
"## Uses",
"### Direct Use",
"### Out-of-Scope Use",
"## Dataset Structure",
"## Dataset Creation",
"### Curation Rationale",
"### Source Data",
"#### Data Collection and Processing",
"#### Who are the source data producers?",
"### Annotations [optional]",
"#### Annotation process",
"#### Who are the annotators?",
"#### Personal and Sensitive Information",
"## Bias, Risks, and Limitations",
"### Recommendations\n\n\n\nUsers should be made aware of the risks, biases and limitations of the dataset. More information needed for further recommendations.\n\n[optional]\n\n\n\nBibTeX:\n\n\n\nAPA:",
"## Glossary [optional]",
"## More Information [optional]",
"## Dataset Card Authors [optional]",
"## Dataset Card Contact"
] |
d41045feeafde01febb94405e7454a766a46f6c5 |
# Dataset Card for Evaluation run of bn22/DolphinMini-Mistral-7B
<!-- Provide a quick summary of the dataset. -->
Dataset automatically created during the evaluation run of model [bn22/DolphinMini-Mistral-7B](https://huggingface.co/bn22/DolphinMini-Mistral-7B) on the [Open LLM Leaderboard](https://huggingface.co/spaces/HuggingFaceH4/open_llm_leaderboard).
The dataset is composed of 63 configuration, each one coresponding to one of the evaluated task.
The dataset has been created from 1 run(s). Each run can be found as a specific split in each configuration, the split being named using the timestamp of the run.The "train" split is always pointing to the latest results.
An additional configuration "results" store all the aggregated results of the run (and is used to compute and display the aggregated metrics on the [Open LLM Leaderboard](https://huggingface.co/spaces/HuggingFaceH4/open_llm_leaderboard)).
To load the details from a run, you can for instance do the following:
```python
from datasets import load_dataset
data = load_dataset("open-llm-leaderboard/details_bn22__DolphinMini-Mistral-7B",
"harness_winogrande_5",
split="train")
```
## Latest results
These are the [latest results from run 2024-01-10T15:33:59.144282](https://huggingface.co/datasets/open-llm-leaderboard/details_bn22__DolphinMini-Mistral-7B/blob/main/results_2024-01-10T15-33-59.144282.json)(note that their might be results for other tasks in the repos if successive evals didn't cover the same tasks. You find each in the results and the "latest" split for each eval):
```python
{
"all": {
"acc": 0.6114573200122365,
"acc_stderr": 0.03242214874357647,
"acc_norm": 0.6230238923554481,
"acc_norm_stderr": 0.03328607344560772,
"mc1": 0.36474908200734396,
"mc1_stderr": 0.016850961061720116,
"mc2": 0.523396497177615,
"mc2_stderr": 0.015013938550542574
},
"harness|arc:challenge|25": {
"acc": 0.560580204778157,
"acc_stderr": 0.014503747823580122,
"acc_norm": 0.6117747440273038,
"acc_norm_stderr": 0.01424161420741405
},
"harness|hellaswag|10": {
"acc": 0.6394144592710616,
"acc_stderr": 0.0047918906258341935,
"acc_norm": 0.8424616610237005,
"acc_norm_stderr": 0.0036356303524759065
},
"harness|hendrycksTest-abstract_algebra|5": {
"acc": 0.32,
"acc_stderr": 0.046882617226215034,
"acc_norm": 0.32,
"acc_norm_stderr": 0.046882617226215034
},
"harness|hendrycksTest-anatomy|5": {
"acc": 0.5925925925925926,
"acc_stderr": 0.04244633238353227,
"acc_norm": 0.5925925925925926,
"acc_norm_stderr": 0.04244633238353227
},
"harness|hendrycksTest-astronomy|5": {
"acc": 0.618421052631579,
"acc_stderr": 0.03953173377749194,
"acc_norm": 0.618421052631579,
"acc_norm_stderr": 0.03953173377749194
},
"harness|hendrycksTest-business_ethics|5": {
"acc": 0.58,
"acc_stderr": 0.049604496374885836,
"acc_norm": 0.58,
"acc_norm_stderr": 0.049604496374885836
},
"harness|hendrycksTest-clinical_knowledge|5": {
"acc": 0.6754716981132075,
"acc_stderr": 0.02881561571343211,
"acc_norm": 0.6754716981132075,
"acc_norm_stderr": 0.02881561571343211
},
"harness|hendrycksTest-college_biology|5": {
"acc": 0.7222222222222222,
"acc_stderr": 0.03745554791462456,
"acc_norm": 0.7222222222222222,
"acc_norm_stderr": 0.03745554791462456
},
"harness|hendrycksTest-college_chemistry|5": {
"acc": 0.45,
"acc_stderr": 0.05,
"acc_norm": 0.45,
"acc_norm_stderr": 0.05
},
"harness|hendrycksTest-college_computer_science|5": {
"acc": 0.48,
"acc_stderr": 0.050211673156867795,
"acc_norm": 0.48,
"acc_norm_stderr": 0.050211673156867795
},
"harness|hendrycksTest-college_mathematics|5": {
"acc": 0.35,
"acc_stderr": 0.047937248544110196,
"acc_norm": 0.35,
"acc_norm_stderr": 0.047937248544110196
},
"harness|hendrycksTest-college_medicine|5": {
"acc": 0.653179190751445,
"acc_stderr": 0.036291466701596636,
"acc_norm": 0.653179190751445,
"acc_norm_stderr": 0.036291466701596636
},
"harness|hendrycksTest-college_physics|5": {
"acc": 0.46078431372549017,
"acc_stderr": 0.049598599663841815,
"acc_norm": 0.46078431372549017,
"acc_norm_stderr": 0.049598599663841815
},
"harness|hendrycksTest-computer_security|5": {
"acc": 0.78,
"acc_stderr": 0.04163331998932263,
"acc_norm": 0.78,
"acc_norm_stderr": 0.04163331998932263
},
"harness|hendrycksTest-conceptual_physics|5": {
"acc": 0.5531914893617021,
"acc_stderr": 0.0325005368436584,
"acc_norm": 0.5531914893617021,
"acc_norm_stderr": 0.0325005368436584
},
"harness|hendrycksTest-econometrics|5": {
"acc": 0.45614035087719296,
"acc_stderr": 0.04685473041907789,
"acc_norm": 0.45614035087719296,
"acc_norm_stderr": 0.04685473041907789
},
"harness|hendrycksTest-electrical_engineering|5": {
"acc": 0.5172413793103449,
"acc_stderr": 0.04164188720169375,
"acc_norm": 0.5172413793103449,
"acc_norm_stderr": 0.04164188720169375
},
"harness|hendrycksTest-elementary_mathematics|5": {
"acc": 0.36772486772486773,
"acc_stderr": 0.024833839825562427,
"acc_norm": 0.36772486772486773,
"acc_norm_stderr": 0.024833839825562427
},
"harness|hendrycksTest-formal_logic|5": {
"acc": 0.373015873015873,
"acc_stderr": 0.04325506042017086,
"acc_norm": 0.373015873015873,
"acc_norm_stderr": 0.04325506042017086
},
"harness|hendrycksTest-global_facts|5": {
"acc": 0.33,
"acc_stderr": 0.047258156262526045,
"acc_norm": 0.33,
"acc_norm_stderr": 0.047258156262526045
},
"harness|hendrycksTest-high_school_biology|5": {
"acc": 0.7387096774193549,
"acc_stderr": 0.024993053397764812,
"acc_norm": 0.7387096774193549,
"acc_norm_stderr": 0.024993053397764812
},
"harness|hendrycksTest-high_school_chemistry|5": {
"acc": 0.4975369458128079,
"acc_stderr": 0.03517945038691063,
"acc_norm": 0.4975369458128079,
"acc_norm_stderr": 0.03517945038691063
},
"harness|hendrycksTest-high_school_computer_science|5": {
"acc": 0.68,
"acc_stderr": 0.04688261722621505,
"acc_norm": 0.68,
"acc_norm_stderr": 0.04688261722621505
},
"harness|hendrycksTest-high_school_european_history|5": {
"acc": 0.7212121212121212,
"acc_stderr": 0.035014387062967806,
"acc_norm": 0.7212121212121212,
"acc_norm_stderr": 0.035014387062967806
},
"harness|hendrycksTest-high_school_geography|5": {
"acc": 0.7878787878787878,
"acc_stderr": 0.029126522834586815,
"acc_norm": 0.7878787878787878,
"acc_norm_stderr": 0.029126522834586815
},
"harness|hendrycksTest-high_school_government_and_politics|5": {
"acc": 0.8652849740932642,
"acc_stderr": 0.02463978909770944,
"acc_norm": 0.8652849740932642,
"acc_norm_stderr": 0.02463978909770944
},
"harness|hendrycksTest-high_school_macroeconomics|5": {
"acc": 0.6512820512820513,
"acc_stderr": 0.02416278028401772,
"acc_norm": 0.6512820512820513,
"acc_norm_stderr": 0.02416278028401772
},
"harness|hendrycksTest-high_school_mathematics|5": {
"acc": 0.34814814814814815,
"acc_stderr": 0.029045600290616258,
"acc_norm": 0.34814814814814815,
"acc_norm_stderr": 0.029045600290616258
},
"harness|hendrycksTest-high_school_microeconomics|5": {
"acc": 0.6512605042016807,
"acc_stderr": 0.030956636328566548,
"acc_norm": 0.6512605042016807,
"acc_norm_stderr": 0.030956636328566548
},
"harness|hendrycksTest-high_school_physics|5": {
"acc": 0.33112582781456956,
"acc_stderr": 0.038425817186598696,
"acc_norm": 0.33112582781456956,
"acc_norm_stderr": 0.038425817186598696
},
"harness|hendrycksTest-high_school_psychology|5": {
"acc": 0.8091743119266055,
"acc_stderr": 0.0168476764000911,
"acc_norm": 0.8091743119266055,
"acc_norm_stderr": 0.0168476764000911
},
"harness|hendrycksTest-high_school_statistics|5": {
"acc": 0.42592592592592593,
"acc_stderr": 0.03372343271653063,
"acc_norm": 0.42592592592592593,
"acc_norm_stderr": 0.03372343271653063
},
"harness|hendrycksTest-high_school_us_history|5": {
"acc": 0.7794117647058824,
"acc_stderr": 0.02910225438967408,
"acc_norm": 0.7794117647058824,
"acc_norm_stderr": 0.02910225438967408
},
"harness|hendrycksTest-high_school_world_history|5": {
"acc": 0.7763713080168776,
"acc_stderr": 0.027123298205229966,
"acc_norm": 0.7763713080168776,
"acc_norm_stderr": 0.027123298205229966
},
"harness|hendrycksTest-human_aging|5": {
"acc": 0.6771300448430493,
"acc_stderr": 0.03138147637575499,
"acc_norm": 0.6771300448430493,
"acc_norm_stderr": 0.03138147637575499
},
"harness|hendrycksTest-human_sexuality|5": {
"acc": 0.7633587786259542,
"acc_stderr": 0.03727673575596913,
"acc_norm": 0.7633587786259542,
"acc_norm_stderr": 0.03727673575596913
},
"harness|hendrycksTest-international_law|5": {
"acc": 0.7603305785123967,
"acc_stderr": 0.03896878985070417,
"acc_norm": 0.7603305785123967,
"acc_norm_stderr": 0.03896878985070417
},
"harness|hendrycksTest-jurisprudence|5": {
"acc": 0.7407407407407407,
"acc_stderr": 0.04236511258094633,
"acc_norm": 0.7407407407407407,
"acc_norm_stderr": 0.04236511258094633
},
"harness|hendrycksTest-logical_fallacies|5": {
"acc": 0.7791411042944786,
"acc_stderr": 0.03259177392742179,
"acc_norm": 0.7791411042944786,
"acc_norm_stderr": 0.03259177392742179
},
"harness|hendrycksTest-machine_learning|5": {
"acc": 0.42857142857142855,
"acc_stderr": 0.04697113923010212,
"acc_norm": 0.42857142857142855,
"acc_norm_stderr": 0.04697113923010212
},
"harness|hendrycksTest-management|5": {
"acc": 0.7864077669902912,
"acc_stderr": 0.040580420156460344,
"acc_norm": 0.7864077669902912,
"acc_norm_stderr": 0.040580420156460344
},
"harness|hendrycksTest-marketing|5": {
"acc": 0.8717948717948718,
"acc_stderr": 0.021901905115073325,
"acc_norm": 0.8717948717948718,
"acc_norm_stderr": 0.021901905115073325
},
"harness|hendrycksTest-medical_genetics|5": {
"acc": 0.7,
"acc_stderr": 0.046056618647183814,
"acc_norm": 0.7,
"acc_norm_stderr": 0.046056618647183814
},
"harness|hendrycksTest-miscellaneous|5": {
"acc": 0.8084291187739464,
"acc_stderr": 0.014072859310451949,
"acc_norm": 0.8084291187739464,
"acc_norm_stderr": 0.014072859310451949
},
"harness|hendrycksTest-moral_disputes|5": {
"acc": 0.7023121387283237,
"acc_stderr": 0.024617055388677,
"acc_norm": 0.7023121387283237,
"acc_norm_stderr": 0.024617055388677
},
"harness|hendrycksTest-moral_scenarios|5": {
"acc": 0.2636871508379888,
"acc_stderr": 0.014736926383761985,
"acc_norm": 0.2636871508379888,
"acc_norm_stderr": 0.014736926383761985
},
"harness|hendrycksTest-nutrition|5": {
"acc": 0.7189542483660131,
"acc_stderr": 0.025738854797818737,
"acc_norm": 0.7189542483660131,
"acc_norm_stderr": 0.025738854797818737
},
"harness|hendrycksTest-philosophy|5": {
"acc": 0.7041800643086816,
"acc_stderr": 0.02592237178881876,
"acc_norm": 0.7041800643086816,
"acc_norm_stderr": 0.02592237178881876
},
"harness|hendrycksTest-prehistory|5": {
"acc": 0.7253086419753086,
"acc_stderr": 0.024836057868294677,
"acc_norm": 0.7253086419753086,
"acc_norm_stderr": 0.024836057868294677
},
"harness|hendrycksTest-professional_accounting|5": {
"acc": 0.42907801418439717,
"acc_stderr": 0.02952591430255856,
"acc_norm": 0.42907801418439717,
"acc_norm_stderr": 0.02952591430255856
},
"harness|hendrycksTest-professional_law|5": {
"acc": 0.4198174706649283,
"acc_stderr": 0.012604960816087364,
"acc_norm": 0.4198174706649283,
"acc_norm_stderr": 0.012604960816087364
},
"harness|hendrycksTest-professional_medicine|5": {
"acc": 0.6507352941176471,
"acc_stderr": 0.028959755196824866,
"acc_norm": 0.6507352941176471,
"acc_norm_stderr": 0.028959755196824866
},
"harness|hendrycksTest-professional_psychology|5": {
"acc": 0.630718954248366,
"acc_stderr": 0.01952431674486635,
"acc_norm": 0.630718954248366,
"acc_norm_stderr": 0.01952431674486635
},
"harness|hendrycksTest-public_relations|5": {
"acc": 0.6727272727272727,
"acc_stderr": 0.04494290866252089,
"acc_norm": 0.6727272727272727,
"acc_norm_stderr": 0.04494290866252089
},
"harness|hendrycksTest-security_studies|5": {
"acc": 0.6857142857142857,
"acc_stderr": 0.02971932942241748,
"acc_norm": 0.6857142857142857,
"acc_norm_stderr": 0.02971932942241748
},
"harness|hendrycksTest-sociology|5": {
"acc": 0.8308457711442786,
"acc_stderr": 0.026508590656233257,
"acc_norm": 0.8308457711442786,
"acc_norm_stderr": 0.026508590656233257
},
"harness|hendrycksTest-us_foreign_policy|5": {
"acc": 0.85,
"acc_stderr": 0.03588702812826371,
"acc_norm": 0.85,
"acc_norm_stderr": 0.03588702812826371
},
"harness|hendrycksTest-virology|5": {
"acc": 0.5421686746987951,
"acc_stderr": 0.0387862677100236,
"acc_norm": 0.5421686746987951,
"acc_norm_stderr": 0.0387862677100236
},
"harness|hendrycksTest-world_religions|5": {
"acc": 0.8187134502923976,
"acc_stderr": 0.029547741687640044,
"acc_norm": 0.8187134502923976,
"acc_norm_stderr": 0.029547741687640044
},
"harness|truthfulqa:mc|0": {
"mc1": 0.36474908200734396,
"mc1_stderr": 0.016850961061720116,
"mc2": 0.523396497177615,
"mc2_stderr": 0.015013938550542574
},
"harness|winogrande|5": {
"acc": 0.7932123125493291,
"acc_stderr": 0.0113825668292358
},
"harness|gsm8k|5": {
"acc": 0.001516300227445034,
"acc_stderr": 0.0010717793485492634
}
}
```
## Dataset Details
### Dataset Description
<!-- Provide a longer summary of what this dataset is. -->
- **Curated by:** [More Information Needed]
- **Funded by [optional]:** [More Information Needed]
- **Shared by [optional]:** [More Information Needed]
- **Language(s) (NLP):** [More Information Needed]
- **License:** [More Information Needed]
### Dataset Sources [optional]
<!-- Provide the basic links for the dataset. -->
- **Repository:** [More Information Needed]
- **Paper [optional]:** [More Information Needed]
- **Demo [optional]:** [More Information Needed]
## Uses
<!-- Address questions around how the dataset is intended to be used. -->
### Direct Use
<!-- This section describes suitable use cases for the dataset. -->
[More Information Needed]
### Out-of-Scope Use
<!-- This section addresses misuse, malicious use, and uses that the dataset will not work well for. -->
[More Information Needed]
## Dataset Structure
<!-- This section provides a description of the dataset fields, and additional information about the dataset structure such as criteria used to create the splits, relationships between data points, etc. -->
[More Information Needed]
## Dataset Creation
### Curation Rationale
<!-- Motivation for the creation of this dataset. -->
[More Information Needed]
### Source Data
<!-- This section describes the source data (e.g. news text and headlines, social media posts, translated sentences, ...). -->
#### Data Collection and Processing
<!-- This section describes the data collection and processing process such as data selection criteria, filtering and normalization methods, tools and libraries used, etc. -->
[More Information Needed]
#### Who are the source data producers?
<!-- This section describes the people or systems who originally created the data. It should also include self-reported demographic or identity information for the source data creators if this information is available. -->
[More Information Needed]
### Annotations [optional]
<!-- If the dataset contains annotations which are not part of the initial data collection, use this section to describe them. -->
#### Annotation process
<!-- This section describes the annotation process such as annotation tools used in the process, the amount of data annotated, annotation guidelines provided to the annotators, interannotator statistics, annotation validation, etc. -->
[More Information Needed]
#### Who are the annotators?
<!-- This section describes the people or systems who created the annotations. -->
[More Information Needed]
#### Personal and Sensitive Information
<!-- State whether the dataset contains data that might be considered personal, sensitive, or private (e.g., data that reveals addresses, uniquely identifiable names or aliases, racial or ethnic origins, sexual orientations, religious beliefs, political opinions, financial or health data, etc.). If efforts were made to anonymize the data, describe the anonymization process. -->
[More Information Needed]
## Bias, Risks, and Limitations
<!-- This section is meant to convey both technical and sociotechnical limitations. -->
[More Information Needed]
### Recommendations
<!-- This section is meant to convey recommendations with respect to the bias, risk, and technical limitations. -->
Users should be made aware of the risks, biases and limitations of the dataset. More information needed for further recommendations.
## Citation [optional]
<!-- If there is a paper or blog post introducing the dataset, the APA and Bibtex information for that should go in this section. -->
**BibTeX:**
[More Information Needed]
**APA:**
[More Information Needed]
## Glossary [optional]
<!-- If relevant, include terms and calculations in this section that can help readers understand the dataset or dataset card. -->
[More Information Needed]
## More Information [optional]
[More Information Needed]
## Dataset Card Authors [optional]
[More Information Needed]
## Dataset Card Contact
[More Information Needed] | open-llm-leaderboard/details_bn22__DolphinMini-Mistral-7B | [
"region:us"
] | 2024-01-10T15:36:23+00:00 | {"pretty_name": "Evaluation run of bn22/DolphinMini-Mistral-7B", "dataset_summary": "Dataset automatically created during the evaluation run of model [bn22/DolphinMini-Mistral-7B](https://huggingface.co/bn22/DolphinMini-Mistral-7B) on the [Open LLM Leaderboard](https://huggingface.co/spaces/HuggingFaceH4/open_llm_leaderboard).\n\nThe dataset is composed of 63 configuration, each one coresponding to one of the evaluated task.\n\nThe dataset has been created from 1 run(s). Each run can be found as a specific split in each configuration, the split being named using the timestamp of the run.The \"train\" split is always pointing to the latest results.\n\nAn additional configuration \"results\" store all the aggregated results of the run (and is used to compute and display the aggregated metrics on the [Open LLM Leaderboard](https://huggingface.co/spaces/HuggingFaceH4/open_llm_leaderboard)).\n\nTo load the details from a run, you can for instance do the following:\n```python\nfrom datasets import load_dataset\ndata = load_dataset(\"open-llm-leaderboard/details_bn22__DolphinMini-Mistral-7B\",\n\t\"harness_winogrande_5\",\n\tsplit=\"train\")\n```\n\n## Latest results\n\nThese are the [latest results from run 2024-01-10T15:33:59.144282](https://huggingface.co/datasets/open-llm-leaderboard/details_bn22__DolphinMini-Mistral-7B/blob/main/results_2024-01-10T15-33-59.144282.json)(note that their might be results for other tasks in the repos if successive evals didn't cover the same tasks. You find each in the results and the \"latest\" split for each eval):\n\n```python\n{\n \"all\": {\n \"acc\": 0.6114573200122365,\n \"acc_stderr\": 0.03242214874357647,\n \"acc_norm\": 0.6230238923554481,\n \"acc_norm_stderr\": 0.03328607344560772,\n \"mc1\": 0.36474908200734396,\n \"mc1_stderr\": 0.016850961061720116,\n \"mc2\": 0.523396497177615,\n \"mc2_stderr\": 0.015013938550542574\n },\n \"harness|arc:challenge|25\": {\n \"acc\": 0.560580204778157,\n \"acc_stderr\": 0.014503747823580122,\n \"acc_norm\": 0.6117747440273038,\n \"acc_norm_stderr\": 0.01424161420741405\n },\n \"harness|hellaswag|10\": {\n \"acc\": 0.6394144592710616,\n \"acc_stderr\": 0.0047918906258341935,\n \"acc_norm\": 0.8424616610237005,\n \"acc_norm_stderr\": 0.0036356303524759065\n },\n \"harness|hendrycksTest-abstract_algebra|5\": {\n \"acc\": 0.32,\n \"acc_stderr\": 0.046882617226215034,\n \"acc_norm\": 0.32,\n \"acc_norm_stderr\": 0.046882617226215034\n },\n \"harness|hendrycksTest-anatomy|5\": {\n \"acc\": 0.5925925925925926,\n \"acc_stderr\": 0.04244633238353227,\n \"acc_norm\": 0.5925925925925926,\n \"acc_norm_stderr\": 0.04244633238353227\n },\n \"harness|hendrycksTest-astronomy|5\": {\n \"acc\": 0.618421052631579,\n \"acc_stderr\": 0.03953173377749194,\n \"acc_norm\": 0.618421052631579,\n \"acc_norm_stderr\": 0.03953173377749194\n },\n \"harness|hendrycksTest-business_ethics|5\": {\n \"acc\": 0.58,\n \"acc_stderr\": 0.049604496374885836,\n \"acc_norm\": 0.58,\n \"acc_norm_stderr\": 0.049604496374885836\n },\n \"harness|hendrycksTest-clinical_knowledge|5\": {\n \"acc\": 0.6754716981132075,\n \"acc_stderr\": 0.02881561571343211,\n \"acc_norm\": 0.6754716981132075,\n \"acc_norm_stderr\": 0.02881561571343211\n },\n \"harness|hendrycksTest-college_biology|5\": {\n \"acc\": 0.7222222222222222,\n \"acc_stderr\": 0.03745554791462456,\n \"acc_norm\": 0.7222222222222222,\n \"acc_norm_stderr\": 0.03745554791462456\n },\n \"harness|hendrycksTest-college_chemistry|5\": {\n \"acc\": 0.45,\n \"acc_stderr\": 0.05,\n \"acc_norm\": 0.45,\n \"acc_norm_stderr\": 0.05\n },\n \"harness|hendrycksTest-college_computer_science|5\": {\n \"acc\": 0.48,\n \"acc_stderr\": 0.050211673156867795,\n \"acc_norm\": 0.48,\n \"acc_norm_stderr\": 0.050211673156867795\n },\n \"harness|hendrycksTest-college_mathematics|5\": {\n \"acc\": 0.35,\n \"acc_stderr\": 0.047937248544110196,\n \"acc_norm\": 0.35,\n \"acc_norm_stderr\": 0.047937248544110196\n },\n \"harness|hendrycksTest-college_medicine|5\": {\n \"acc\": 0.653179190751445,\n \"acc_stderr\": 0.036291466701596636,\n \"acc_norm\": 0.653179190751445,\n \"acc_norm_stderr\": 0.036291466701596636\n },\n \"harness|hendrycksTest-college_physics|5\": {\n \"acc\": 0.46078431372549017,\n \"acc_stderr\": 0.049598599663841815,\n \"acc_norm\": 0.46078431372549017,\n \"acc_norm_stderr\": 0.049598599663841815\n },\n \"harness|hendrycksTest-computer_security|5\": {\n \"acc\": 0.78,\n \"acc_stderr\": 0.04163331998932263,\n \"acc_norm\": 0.78,\n \"acc_norm_stderr\": 0.04163331998932263\n },\n \"harness|hendrycksTest-conceptual_physics|5\": {\n \"acc\": 0.5531914893617021,\n \"acc_stderr\": 0.0325005368436584,\n \"acc_norm\": 0.5531914893617021,\n \"acc_norm_stderr\": 0.0325005368436584\n },\n \"harness|hendrycksTest-econometrics|5\": {\n \"acc\": 0.45614035087719296,\n \"acc_stderr\": 0.04685473041907789,\n \"acc_norm\": 0.45614035087719296,\n \"acc_norm_stderr\": 0.04685473041907789\n },\n \"harness|hendrycksTest-electrical_engineering|5\": {\n \"acc\": 0.5172413793103449,\n \"acc_stderr\": 0.04164188720169375,\n \"acc_norm\": 0.5172413793103449,\n \"acc_norm_stderr\": 0.04164188720169375\n },\n \"harness|hendrycksTest-elementary_mathematics|5\": {\n \"acc\": 0.36772486772486773,\n \"acc_stderr\": 0.024833839825562427,\n \"acc_norm\": 0.36772486772486773,\n \"acc_norm_stderr\": 0.024833839825562427\n },\n \"harness|hendrycksTest-formal_logic|5\": {\n \"acc\": 0.373015873015873,\n \"acc_stderr\": 0.04325506042017086,\n \"acc_norm\": 0.373015873015873,\n \"acc_norm_stderr\": 0.04325506042017086\n },\n \"harness|hendrycksTest-global_facts|5\": {\n \"acc\": 0.33,\n \"acc_stderr\": 0.047258156262526045,\n \"acc_norm\": 0.33,\n \"acc_norm_stderr\": 0.047258156262526045\n },\n \"harness|hendrycksTest-high_school_biology|5\": {\n \"acc\": 0.7387096774193549,\n \"acc_stderr\": 0.024993053397764812,\n \"acc_norm\": 0.7387096774193549,\n \"acc_norm_stderr\": 0.024993053397764812\n },\n \"harness|hendrycksTest-high_school_chemistry|5\": {\n \"acc\": 0.4975369458128079,\n \"acc_stderr\": 0.03517945038691063,\n \"acc_norm\": 0.4975369458128079,\n \"acc_norm_stderr\": 0.03517945038691063\n },\n \"harness|hendrycksTest-high_school_computer_science|5\": {\n \"acc\": 0.68,\n \"acc_stderr\": 0.04688261722621505,\n \"acc_norm\": 0.68,\n \"acc_norm_stderr\": 0.04688261722621505\n },\n \"harness|hendrycksTest-high_school_european_history|5\": {\n \"acc\": 0.7212121212121212,\n \"acc_stderr\": 0.035014387062967806,\n \"acc_norm\": 0.7212121212121212,\n \"acc_norm_stderr\": 0.035014387062967806\n },\n \"harness|hendrycksTest-high_school_geography|5\": {\n \"acc\": 0.7878787878787878,\n \"acc_stderr\": 0.029126522834586815,\n \"acc_norm\": 0.7878787878787878,\n \"acc_norm_stderr\": 0.029126522834586815\n },\n \"harness|hendrycksTest-high_school_government_and_politics|5\": {\n \"acc\": 0.8652849740932642,\n \"acc_stderr\": 0.02463978909770944,\n \"acc_norm\": 0.8652849740932642,\n \"acc_norm_stderr\": 0.02463978909770944\n },\n \"harness|hendrycksTest-high_school_macroeconomics|5\": {\n \"acc\": 0.6512820512820513,\n \"acc_stderr\": 0.02416278028401772,\n \"acc_norm\": 0.6512820512820513,\n \"acc_norm_stderr\": 0.02416278028401772\n },\n \"harness|hendrycksTest-high_school_mathematics|5\": {\n \"acc\": 0.34814814814814815,\n \"acc_stderr\": 0.029045600290616258,\n \"acc_norm\": 0.34814814814814815,\n \"acc_norm_stderr\": 0.029045600290616258\n },\n \"harness|hendrycksTest-high_school_microeconomics|5\": {\n \"acc\": 0.6512605042016807,\n \"acc_stderr\": 0.030956636328566548,\n \"acc_norm\": 0.6512605042016807,\n \"acc_norm_stderr\": 0.030956636328566548\n },\n \"harness|hendrycksTest-high_school_physics|5\": {\n \"acc\": 0.33112582781456956,\n \"acc_stderr\": 0.038425817186598696,\n \"acc_norm\": 0.33112582781456956,\n \"acc_norm_stderr\": 0.038425817186598696\n },\n \"harness|hendrycksTest-high_school_psychology|5\": {\n \"acc\": 0.8091743119266055,\n \"acc_stderr\": 0.0168476764000911,\n \"acc_norm\": 0.8091743119266055,\n \"acc_norm_stderr\": 0.0168476764000911\n },\n \"harness|hendrycksTest-high_school_statistics|5\": {\n \"acc\": 0.42592592592592593,\n \"acc_stderr\": 0.03372343271653063,\n \"acc_norm\": 0.42592592592592593,\n \"acc_norm_stderr\": 0.03372343271653063\n },\n \"harness|hendrycksTest-high_school_us_history|5\": {\n \"acc\": 0.7794117647058824,\n \"acc_stderr\": 0.02910225438967408,\n \"acc_norm\": 0.7794117647058824,\n \"acc_norm_stderr\": 0.02910225438967408\n },\n \"harness|hendrycksTest-high_school_world_history|5\": {\n \"acc\": 0.7763713080168776,\n \"acc_stderr\": 0.027123298205229966,\n \"acc_norm\": 0.7763713080168776,\n \"acc_norm_stderr\": 0.027123298205229966\n },\n \"harness|hendrycksTest-human_aging|5\": {\n \"acc\": 0.6771300448430493,\n \"acc_stderr\": 0.03138147637575499,\n \"acc_norm\": 0.6771300448430493,\n \"acc_norm_stderr\": 0.03138147637575499\n },\n \"harness|hendrycksTest-human_sexuality|5\": {\n \"acc\": 0.7633587786259542,\n \"acc_stderr\": 0.03727673575596913,\n \"acc_norm\": 0.7633587786259542,\n \"acc_norm_stderr\": 0.03727673575596913\n },\n \"harness|hendrycksTest-international_law|5\": {\n \"acc\": 0.7603305785123967,\n \"acc_stderr\": 0.03896878985070417,\n \"acc_norm\": 0.7603305785123967,\n \"acc_norm_stderr\": 0.03896878985070417\n },\n \"harness|hendrycksTest-jurisprudence|5\": {\n \"acc\": 0.7407407407407407,\n \"acc_stderr\": 0.04236511258094633,\n \"acc_norm\": 0.7407407407407407,\n \"acc_norm_stderr\": 0.04236511258094633\n },\n \"harness|hendrycksTest-logical_fallacies|5\": {\n \"acc\": 0.7791411042944786,\n \"acc_stderr\": 0.03259177392742179,\n \"acc_norm\": 0.7791411042944786,\n \"acc_norm_stderr\": 0.03259177392742179\n },\n \"harness|hendrycksTest-machine_learning|5\": {\n \"acc\": 0.42857142857142855,\n \"acc_stderr\": 0.04697113923010212,\n \"acc_norm\": 0.42857142857142855,\n \"acc_norm_stderr\": 0.04697113923010212\n },\n \"harness|hendrycksTest-management|5\": {\n \"acc\": 0.7864077669902912,\n \"acc_stderr\": 0.040580420156460344,\n \"acc_norm\": 0.7864077669902912,\n \"acc_norm_stderr\": 0.040580420156460344\n },\n \"harness|hendrycksTest-marketing|5\": {\n \"acc\": 0.8717948717948718,\n \"acc_stderr\": 0.021901905115073325,\n \"acc_norm\": 0.8717948717948718,\n \"acc_norm_stderr\": 0.021901905115073325\n },\n \"harness|hendrycksTest-medical_genetics|5\": {\n \"acc\": 0.7,\n \"acc_stderr\": 0.046056618647183814,\n \"acc_norm\": 0.7,\n \"acc_norm_stderr\": 0.046056618647183814\n },\n \"harness|hendrycksTest-miscellaneous|5\": {\n \"acc\": 0.8084291187739464,\n \"acc_stderr\": 0.014072859310451949,\n \"acc_norm\": 0.8084291187739464,\n \"acc_norm_stderr\": 0.014072859310451949\n },\n \"harness|hendrycksTest-moral_disputes|5\": {\n \"acc\": 0.7023121387283237,\n \"acc_stderr\": 0.024617055388677,\n \"acc_norm\": 0.7023121387283237,\n \"acc_norm_stderr\": 0.024617055388677\n },\n \"harness|hendrycksTest-moral_scenarios|5\": {\n \"acc\": 0.2636871508379888,\n \"acc_stderr\": 0.014736926383761985,\n \"acc_norm\": 0.2636871508379888,\n \"acc_norm_stderr\": 0.014736926383761985\n },\n \"harness|hendrycksTest-nutrition|5\": {\n \"acc\": 0.7189542483660131,\n \"acc_stderr\": 0.025738854797818737,\n \"acc_norm\": 0.7189542483660131,\n \"acc_norm_stderr\": 0.025738854797818737\n },\n \"harness|hendrycksTest-philosophy|5\": {\n \"acc\": 0.7041800643086816,\n \"acc_stderr\": 0.02592237178881876,\n \"acc_norm\": 0.7041800643086816,\n \"acc_norm_stderr\": 0.02592237178881876\n },\n \"harness|hendrycksTest-prehistory|5\": {\n \"acc\": 0.7253086419753086,\n \"acc_stderr\": 0.024836057868294677,\n \"acc_norm\": 0.7253086419753086,\n \"acc_norm_stderr\": 0.024836057868294677\n },\n \"harness|hendrycksTest-professional_accounting|5\": {\n \"acc\": 0.42907801418439717,\n \"acc_stderr\": 0.02952591430255856,\n \"acc_norm\": 0.42907801418439717,\n \"acc_norm_stderr\": 0.02952591430255856\n },\n \"harness|hendrycksTest-professional_law|5\": {\n \"acc\": 0.4198174706649283,\n \"acc_stderr\": 0.012604960816087364,\n \"acc_norm\": 0.4198174706649283,\n \"acc_norm_stderr\": 0.012604960816087364\n },\n \"harness|hendrycksTest-professional_medicine|5\": {\n \"acc\": 0.6507352941176471,\n \"acc_stderr\": 0.028959755196824866,\n \"acc_norm\": 0.6507352941176471,\n \"acc_norm_stderr\": 0.028959755196824866\n },\n \"harness|hendrycksTest-professional_psychology|5\": {\n \"acc\": 0.630718954248366,\n \"acc_stderr\": 0.01952431674486635,\n \"acc_norm\": 0.630718954248366,\n \"acc_norm_stderr\": 0.01952431674486635\n },\n \"harness|hendrycksTest-public_relations|5\": {\n \"acc\": 0.6727272727272727,\n \"acc_stderr\": 0.04494290866252089,\n \"acc_norm\": 0.6727272727272727,\n \"acc_norm_stderr\": 0.04494290866252089\n },\n \"harness|hendrycksTest-security_studies|5\": {\n \"acc\": 0.6857142857142857,\n \"acc_stderr\": 0.02971932942241748,\n \"acc_norm\": 0.6857142857142857,\n \"acc_norm_stderr\": 0.02971932942241748\n },\n \"harness|hendrycksTest-sociology|5\": {\n \"acc\": 0.8308457711442786,\n \"acc_stderr\": 0.026508590656233257,\n \"acc_norm\": 0.8308457711442786,\n \"acc_norm_stderr\": 0.026508590656233257\n },\n \"harness|hendrycksTest-us_foreign_policy|5\": {\n \"acc\": 0.85,\n \"acc_stderr\": 0.03588702812826371,\n \"acc_norm\": 0.85,\n \"acc_norm_stderr\": 0.03588702812826371\n },\n \"harness|hendrycksTest-virology|5\": {\n \"acc\": 0.5421686746987951,\n \"acc_stderr\": 0.0387862677100236,\n \"acc_norm\": 0.5421686746987951,\n \"acc_norm_stderr\": 0.0387862677100236\n },\n \"harness|hendrycksTest-world_religions|5\": {\n \"acc\": 0.8187134502923976,\n \"acc_stderr\": 0.029547741687640044,\n \"acc_norm\": 0.8187134502923976,\n \"acc_norm_stderr\": 0.029547741687640044\n },\n \"harness|truthfulqa:mc|0\": {\n \"mc1\": 0.36474908200734396,\n \"mc1_stderr\": 0.016850961061720116,\n \"mc2\": 0.523396497177615,\n \"mc2_stderr\": 0.015013938550542574\n },\n \"harness|winogrande|5\": {\n \"acc\": 0.7932123125493291,\n \"acc_stderr\": 0.0113825668292358\n },\n \"harness|gsm8k|5\": {\n \"acc\": 0.001516300227445034,\n \"acc_stderr\": 0.0010717793485492634\n }\n}\n```", "repo_url": "https://huggingface.co/bn22/DolphinMini-Mistral-7B", "leaderboard_url": "https://huggingface.co/spaces/HuggingFaceH4/open_llm_leaderboard", "point_of_contact": "[email protected]", "configs": [{"config_name": "harness_arc_challenge_25", "data_files": [{"split": "2024_01_10T15_33_59.144282", "path": ["**/details_harness|arc:challenge|25_2024-01-10T15-33-59.144282.parquet"]}, {"split": "latest", "path": ["**/details_harness|arc:challenge|25_2024-01-10T15-33-59.144282.parquet"]}]}, {"config_name": "harness_gsm8k_5", "data_files": [{"split": "2024_01_10T15_33_59.144282", "path": ["**/details_harness|gsm8k|5_2024-01-10T15-33-59.144282.parquet"]}, {"split": "latest", "path": ["**/details_harness|gsm8k|5_2024-01-10T15-33-59.144282.parquet"]}]}, {"config_name": "harness_hellaswag_10", "data_files": [{"split": "2024_01_10T15_33_59.144282", "path": ["**/details_harness|hellaswag|10_2024-01-10T15-33-59.144282.parquet"]}, {"split": "latest", "path": ["**/details_harness|hellaswag|10_2024-01-10T15-33-59.144282.parquet"]}]}, {"config_name": "harness_hendrycksTest_5", "data_files": [{"split": "2024_01_10T15_33_59.144282", "path": ["**/details_harness|hendrycksTest-abstract_algebra|5_2024-01-10T15-33-59.144282.parquet", "**/details_harness|hendrycksTest-anatomy|5_2024-01-10T15-33-59.144282.parquet", "**/details_harness|hendrycksTest-astronomy|5_2024-01-10T15-33-59.144282.parquet", "**/details_harness|hendrycksTest-business_ethics|5_2024-01-10T15-33-59.144282.parquet", "**/details_harness|hendrycksTest-clinical_knowledge|5_2024-01-10T15-33-59.144282.parquet", "**/details_harness|hendrycksTest-college_biology|5_2024-01-10T15-33-59.144282.parquet", "**/details_harness|hendrycksTest-college_chemistry|5_2024-01-10T15-33-59.144282.parquet", "**/details_harness|hendrycksTest-college_computer_science|5_2024-01-10T15-33-59.144282.parquet", "**/details_harness|hendrycksTest-college_mathematics|5_2024-01-10T15-33-59.144282.parquet", "**/details_harness|hendrycksTest-college_medicine|5_2024-01-10T15-33-59.144282.parquet", "**/details_harness|hendrycksTest-college_physics|5_2024-01-10T15-33-59.144282.parquet", "**/details_harness|hendrycksTest-computer_security|5_2024-01-10T15-33-59.144282.parquet", "**/details_harness|hendrycksTest-conceptual_physics|5_2024-01-10T15-33-59.144282.parquet", "**/details_harness|hendrycksTest-econometrics|5_2024-01-10T15-33-59.144282.parquet", "**/details_harness|hendrycksTest-electrical_engineering|5_2024-01-10T15-33-59.144282.parquet", "**/details_harness|hendrycksTest-elementary_mathematics|5_2024-01-10T15-33-59.144282.parquet", "**/details_harness|hendrycksTest-formal_logic|5_2024-01-10T15-33-59.144282.parquet", "**/details_harness|hendrycksTest-global_facts|5_2024-01-10T15-33-59.144282.parquet", "**/details_harness|hendrycksTest-high_school_biology|5_2024-01-10T15-33-59.144282.parquet", "**/details_harness|hendrycksTest-high_school_chemistry|5_2024-01-10T15-33-59.144282.parquet", "**/details_harness|hendrycksTest-high_school_computer_science|5_2024-01-10T15-33-59.144282.parquet", "**/details_harness|hendrycksTest-high_school_european_history|5_2024-01-10T15-33-59.144282.parquet", "**/details_harness|hendrycksTest-high_school_geography|5_2024-01-10T15-33-59.144282.parquet", "**/details_harness|hendrycksTest-high_school_government_and_politics|5_2024-01-10T15-33-59.144282.parquet", "**/details_harness|hendrycksTest-high_school_macroeconomics|5_2024-01-10T15-33-59.144282.parquet", "**/details_harness|hendrycksTest-high_school_mathematics|5_2024-01-10T15-33-59.144282.parquet", "**/details_harness|hendrycksTest-high_school_microeconomics|5_2024-01-10T15-33-59.144282.parquet", "**/details_harness|hendrycksTest-high_school_physics|5_2024-01-10T15-33-59.144282.parquet", "**/details_harness|hendrycksTest-high_school_psychology|5_2024-01-10T15-33-59.144282.parquet", "**/details_harness|hendrycksTest-high_school_statistics|5_2024-01-10T15-33-59.144282.parquet", "**/details_harness|hendrycksTest-high_school_us_history|5_2024-01-10T15-33-59.144282.parquet", "**/details_harness|hendrycksTest-high_school_world_history|5_2024-01-10T15-33-59.144282.parquet", "**/details_harness|hendrycksTest-human_aging|5_2024-01-10T15-33-59.144282.parquet", "**/details_harness|hendrycksTest-human_sexuality|5_2024-01-10T15-33-59.144282.parquet", "**/details_harness|hendrycksTest-international_law|5_2024-01-10T15-33-59.144282.parquet", "**/details_harness|hendrycksTest-jurisprudence|5_2024-01-10T15-33-59.144282.parquet", "**/details_harness|hendrycksTest-logical_fallacies|5_2024-01-10T15-33-59.144282.parquet", "**/details_harness|hendrycksTest-machine_learning|5_2024-01-10T15-33-59.144282.parquet", "**/details_harness|hendrycksTest-management|5_2024-01-10T15-33-59.144282.parquet", "**/details_harness|hendrycksTest-marketing|5_2024-01-10T15-33-59.144282.parquet", "**/details_harness|hendrycksTest-medical_genetics|5_2024-01-10T15-33-59.144282.parquet", "**/details_harness|hendrycksTest-miscellaneous|5_2024-01-10T15-33-59.144282.parquet", "**/details_harness|hendrycksTest-moral_disputes|5_2024-01-10T15-33-59.144282.parquet", "**/details_harness|hendrycksTest-moral_scenarios|5_2024-01-10T15-33-59.144282.parquet", "**/details_harness|hendrycksTest-nutrition|5_2024-01-10T15-33-59.144282.parquet", "**/details_harness|hendrycksTest-philosophy|5_2024-01-10T15-33-59.144282.parquet", "**/details_harness|hendrycksTest-prehistory|5_2024-01-10T15-33-59.144282.parquet", "**/details_harness|hendrycksTest-professional_accounting|5_2024-01-10T15-33-59.144282.parquet", "**/details_harness|hendrycksTest-professional_law|5_2024-01-10T15-33-59.144282.parquet", "**/details_harness|hendrycksTest-professional_medicine|5_2024-01-10T15-33-59.144282.parquet", "**/details_harness|hendrycksTest-professional_psychology|5_2024-01-10T15-33-59.144282.parquet", "**/details_harness|hendrycksTest-public_relations|5_2024-01-10T15-33-59.144282.parquet", "**/details_harness|hendrycksTest-security_studies|5_2024-01-10T15-33-59.144282.parquet", "**/details_harness|hendrycksTest-sociology|5_2024-01-10T15-33-59.144282.parquet", "**/details_harness|hendrycksTest-us_foreign_policy|5_2024-01-10T15-33-59.144282.parquet", "**/details_harness|hendrycksTest-virology|5_2024-01-10T15-33-59.144282.parquet", "**/details_harness|hendrycksTest-world_religions|5_2024-01-10T15-33-59.144282.parquet"]}, {"split": "latest", "path": ["**/details_harness|hendrycksTest-abstract_algebra|5_2024-01-10T15-33-59.144282.parquet", "**/details_harness|hendrycksTest-anatomy|5_2024-01-10T15-33-59.144282.parquet", "**/details_harness|hendrycksTest-astronomy|5_2024-01-10T15-33-59.144282.parquet", "**/details_harness|hendrycksTest-business_ethics|5_2024-01-10T15-33-59.144282.parquet", "**/details_harness|hendrycksTest-clinical_knowledge|5_2024-01-10T15-33-59.144282.parquet", "**/details_harness|hendrycksTest-college_biology|5_2024-01-10T15-33-59.144282.parquet", "**/details_harness|hendrycksTest-college_chemistry|5_2024-01-10T15-33-59.144282.parquet", "**/details_harness|hendrycksTest-college_computer_science|5_2024-01-10T15-33-59.144282.parquet", "**/details_harness|hendrycksTest-college_mathematics|5_2024-01-10T15-33-59.144282.parquet", "**/details_harness|hendrycksTest-college_medicine|5_2024-01-10T15-33-59.144282.parquet", "**/details_harness|hendrycksTest-college_physics|5_2024-01-10T15-33-59.144282.parquet", "**/details_harness|hendrycksTest-computer_security|5_2024-01-10T15-33-59.144282.parquet", "**/details_harness|hendrycksTest-conceptual_physics|5_2024-01-10T15-33-59.144282.parquet", "**/details_harness|hendrycksTest-econometrics|5_2024-01-10T15-33-59.144282.parquet", "**/details_harness|hendrycksTest-electrical_engineering|5_2024-01-10T15-33-59.144282.parquet", "**/details_harness|hendrycksTest-elementary_mathematics|5_2024-01-10T15-33-59.144282.parquet", "**/details_harness|hendrycksTest-formal_logic|5_2024-01-10T15-33-59.144282.parquet", "**/details_harness|hendrycksTest-global_facts|5_2024-01-10T15-33-59.144282.parquet", "**/details_harness|hendrycksTest-high_school_biology|5_2024-01-10T15-33-59.144282.parquet", "**/details_harness|hendrycksTest-high_school_chemistry|5_2024-01-10T15-33-59.144282.parquet", "**/details_harness|hendrycksTest-high_school_computer_science|5_2024-01-10T15-33-59.144282.parquet", "**/details_harness|hendrycksTest-high_school_european_history|5_2024-01-10T15-33-59.144282.parquet", "**/details_harness|hendrycksTest-high_school_geography|5_2024-01-10T15-33-59.144282.parquet", "**/details_harness|hendrycksTest-high_school_government_and_politics|5_2024-01-10T15-33-59.144282.parquet", "**/details_harness|hendrycksTest-high_school_macroeconomics|5_2024-01-10T15-33-59.144282.parquet", "**/details_harness|hendrycksTest-high_school_mathematics|5_2024-01-10T15-33-59.144282.parquet", "**/details_harness|hendrycksTest-high_school_microeconomics|5_2024-01-10T15-33-59.144282.parquet", "**/details_harness|hendrycksTest-high_school_physics|5_2024-01-10T15-33-59.144282.parquet", "**/details_harness|hendrycksTest-high_school_psychology|5_2024-01-10T15-33-59.144282.parquet", "**/details_harness|hendrycksTest-high_school_statistics|5_2024-01-10T15-33-59.144282.parquet", "**/details_harness|hendrycksTest-high_school_us_history|5_2024-01-10T15-33-59.144282.parquet", "**/details_harness|hendrycksTest-high_school_world_history|5_2024-01-10T15-33-59.144282.parquet", "**/details_harness|hendrycksTest-human_aging|5_2024-01-10T15-33-59.144282.parquet", "**/details_harness|hendrycksTest-human_sexuality|5_2024-01-10T15-33-59.144282.parquet", "**/details_harness|hendrycksTest-international_law|5_2024-01-10T15-33-59.144282.parquet", "**/details_harness|hendrycksTest-jurisprudence|5_2024-01-10T15-33-59.144282.parquet", "**/details_harness|hendrycksTest-logical_fallacies|5_2024-01-10T15-33-59.144282.parquet", "**/details_harness|hendrycksTest-machine_learning|5_2024-01-10T15-33-59.144282.parquet", "**/details_harness|hendrycksTest-management|5_2024-01-10T15-33-59.144282.parquet", "**/details_harness|hendrycksTest-marketing|5_2024-01-10T15-33-59.144282.parquet", "**/details_harness|hendrycksTest-medical_genetics|5_2024-01-10T15-33-59.144282.parquet", "**/details_harness|hendrycksTest-miscellaneous|5_2024-01-10T15-33-59.144282.parquet", "**/details_harness|hendrycksTest-moral_disputes|5_2024-01-10T15-33-59.144282.parquet", "**/details_harness|hendrycksTest-moral_scenarios|5_2024-01-10T15-33-59.144282.parquet", "**/details_harness|hendrycksTest-nutrition|5_2024-01-10T15-33-59.144282.parquet", "**/details_harness|hendrycksTest-philosophy|5_2024-01-10T15-33-59.144282.parquet", "**/details_harness|hendrycksTest-prehistory|5_2024-01-10T15-33-59.144282.parquet", "**/details_harness|hendrycksTest-professional_accounting|5_2024-01-10T15-33-59.144282.parquet", "**/details_harness|hendrycksTest-professional_law|5_2024-01-10T15-33-59.144282.parquet", "**/details_harness|hendrycksTest-professional_medicine|5_2024-01-10T15-33-59.144282.parquet", "**/details_harness|hendrycksTest-professional_psychology|5_2024-01-10T15-33-59.144282.parquet", "**/details_harness|hendrycksTest-public_relations|5_2024-01-10T15-33-59.144282.parquet", "**/details_harness|hendrycksTest-security_studies|5_2024-01-10T15-33-59.144282.parquet", "**/details_harness|hendrycksTest-sociology|5_2024-01-10T15-33-59.144282.parquet", "**/details_harness|hendrycksTest-us_foreign_policy|5_2024-01-10T15-33-59.144282.parquet", "**/details_harness|hendrycksTest-virology|5_2024-01-10T15-33-59.144282.parquet", "**/details_harness|hendrycksTest-world_religions|5_2024-01-10T15-33-59.144282.parquet"]}]}, {"config_name": "harness_hendrycksTest_abstract_algebra_5", "data_files": [{"split": "2024_01_10T15_33_59.144282", "path": ["**/details_harness|hendrycksTest-abstract_algebra|5_2024-01-10T15-33-59.144282.parquet"]}, {"split": "latest", "path": ["**/details_harness|hendrycksTest-abstract_algebra|5_2024-01-10T15-33-59.144282.parquet"]}]}, {"config_name": "harness_hendrycksTest_anatomy_5", "data_files": [{"split": "2024_01_10T15_33_59.144282", "path": ["**/details_harness|hendrycksTest-anatomy|5_2024-01-10T15-33-59.144282.parquet"]}, {"split": "latest", "path": ["**/details_harness|hendrycksTest-anatomy|5_2024-01-10T15-33-59.144282.parquet"]}]}, {"config_name": "harness_hendrycksTest_astronomy_5", "data_files": [{"split": "2024_01_10T15_33_59.144282", "path": ["**/details_harness|hendrycksTest-astronomy|5_2024-01-10T15-33-59.144282.parquet"]}, {"split": "latest", "path": ["**/details_harness|hendrycksTest-astronomy|5_2024-01-10T15-33-59.144282.parquet"]}]}, {"config_name": "harness_hendrycksTest_business_ethics_5", "data_files": [{"split": "2024_01_10T15_33_59.144282", "path": ["**/details_harness|hendrycksTest-business_ethics|5_2024-01-10T15-33-59.144282.parquet"]}, {"split": "latest", "path": ["**/details_harness|hendrycksTest-business_ethics|5_2024-01-10T15-33-59.144282.parquet"]}]}, {"config_name": "harness_hendrycksTest_clinical_knowledge_5", "data_files": [{"split": "2024_01_10T15_33_59.144282", "path": ["**/details_harness|hendrycksTest-clinical_knowledge|5_2024-01-10T15-33-59.144282.parquet"]}, {"split": "latest", "path": ["**/details_harness|hendrycksTest-clinical_knowledge|5_2024-01-10T15-33-59.144282.parquet"]}]}, {"config_name": "harness_hendrycksTest_college_biology_5", "data_files": [{"split": "2024_01_10T15_33_59.144282", "path": ["**/details_harness|hendrycksTest-college_biology|5_2024-01-10T15-33-59.144282.parquet"]}, {"split": "latest", "path": ["**/details_harness|hendrycksTest-college_biology|5_2024-01-10T15-33-59.144282.parquet"]}]}, {"config_name": "harness_hendrycksTest_college_chemistry_5", "data_files": [{"split": "2024_01_10T15_33_59.144282", "path": ["**/details_harness|hendrycksTest-college_chemistry|5_2024-01-10T15-33-59.144282.parquet"]}, {"split": "latest", "path": ["**/details_harness|hendrycksTest-college_chemistry|5_2024-01-10T15-33-59.144282.parquet"]}]}, {"config_name": "harness_hendrycksTest_college_computer_science_5", "data_files": [{"split": "2024_01_10T15_33_59.144282", "path": ["**/details_harness|hendrycksTest-college_computer_science|5_2024-01-10T15-33-59.144282.parquet"]}, {"split": "latest", "path": ["**/details_harness|hendrycksTest-college_computer_science|5_2024-01-10T15-33-59.144282.parquet"]}]}, {"config_name": "harness_hendrycksTest_college_mathematics_5", "data_files": [{"split": "2024_01_10T15_33_59.144282", "path": ["**/details_harness|hendrycksTest-college_mathematics|5_2024-01-10T15-33-59.144282.parquet"]}, {"split": "latest", "path": ["**/details_harness|hendrycksTest-college_mathematics|5_2024-01-10T15-33-59.144282.parquet"]}]}, {"config_name": "harness_hendrycksTest_college_medicine_5", "data_files": [{"split": "2024_01_10T15_33_59.144282", "path": ["**/details_harness|hendrycksTest-college_medicine|5_2024-01-10T15-33-59.144282.parquet"]}, {"split": "latest", "path": ["**/details_harness|hendrycksTest-college_medicine|5_2024-01-10T15-33-59.144282.parquet"]}]}, {"config_name": "harness_hendrycksTest_college_physics_5", "data_files": [{"split": "2024_01_10T15_33_59.144282", "path": ["**/details_harness|hendrycksTest-college_physics|5_2024-01-10T15-33-59.144282.parquet"]}, {"split": "latest", "path": ["**/details_harness|hendrycksTest-college_physics|5_2024-01-10T15-33-59.144282.parquet"]}]}, {"config_name": "harness_hendrycksTest_computer_security_5", "data_files": [{"split": "2024_01_10T15_33_59.144282", "path": ["**/details_harness|hendrycksTest-computer_security|5_2024-01-10T15-33-59.144282.parquet"]}, {"split": "latest", "path": ["**/details_harness|hendrycksTest-computer_security|5_2024-01-10T15-33-59.144282.parquet"]}]}, {"config_name": "harness_hendrycksTest_conceptual_physics_5", "data_files": [{"split": "2024_01_10T15_33_59.144282", "path": ["**/details_harness|hendrycksTest-conceptual_physics|5_2024-01-10T15-33-59.144282.parquet"]}, {"split": "latest", "path": ["**/details_harness|hendrycksTest-conceptual_physics|5_2024-01-10T15-33-59.144282.parquet"]}]}, {"config_name": "harness_hendrycksTest_econometrics_5", "data_files": [{"split": "2024_01_10T15_33_59.144282", "path": ["**/details_harness|hendrycksTest-econometrics|5_2024-01-10T15-33-59.144282.parquet"]}, {"split": "latest", "path": ["**/details_harness|hendrycksTest-econometrics|5_2024-01-10T15-33-59.144282.parquet"]}]}, {"config_name": "harness_hendrycksTest_electrical_engineering_5", "data_files": [{"split": "2024_01_10T15_33_59.144282", "path": ["**/details_harness|hendrycksTest-electrical_engineering|5_2024-01-10T15-33-59.144282.parquet"]}, {"split": "latest", "path": ["**/details_harness|hendrycksTest-electrical_engineering|5_2024-01-10T15-33-59.144282.parquet"]}]}, {"config_name": "harness_hendrycksTest_elementary_mathematics_5", "data_files": [{"split": "2024_01_10T15_33_59.144282", "path": ["**/details_harness|hendrycksTest-elementary_mathematics|5_2024-01-10T15-33-59.144282.parquet"]}, {"split": "latest", "path": ["**/details_harness|hendrycksTest-elementary_mathematics|5_2024-01-10T15-33-59.144282.parquet"]}]}, {"config_name": "harness_hendrycksTest_formal_logic_5", "data_files": [{"split": "2024_01_10T15_33_59.144282", "path": ["**/details_harness|hendrycksTest-formal_logic|5_2024-01-10T15-33-59.144282.parquet"]}, {"split": "latest", "path": ["**/details_harness|hendrycksTest-formal_logic|5_2024-01-10T15-33-59.144282.parquet"]}]}, {"config_name": "harness_hendrycksTest_global_facts_5", "data_files": [{"split": "2024_01_10T15_33_59.144282", "path": ["**/details_harness|hendrycksTest-global_facts|5_2024-01-10T15-33-59.144282.parquet"]}, {"split": "latest", "path": ["**/details_harness|hendrycksTest-global_facts|5_2024-01-10T15-33-59.144282.parquet"]}]}, {"config_name": "harness_hendrycksTest_high_school_biology_5", "data_files": [{"split": "2024_01_10T15_33_59.144282", "path": ["**/details_harness|hendrycksTest-high_school_biology|5_2024-01-10T15-33-59.144282.parquet"]}, {"split": "latest", "path": ["**/details_harness|hendrycksTest-high_school_biology|5_2024-01-10T15-33-59.144282.parquet"]}]}, {"config_name": "harness_hendrycksTest_high_school_chemistry_5", "data_files": [{"split": "2024_01_10T15_33_59.144282", "path": ["**/details_harness|hendrycksTest-high_school_chemistry|5_2024-01-10T15-33-59.144282.parquet"]}, {"split": "latest", "path": ["**/details_harness|hendrycksTest-high_school_chemistry|5_2024-01-10T15-33-59.144282.parquet"]}]}, {"config_name": "harness_hendrycksTest_high_school_computer_science_5", "data_files": [{"split": "2024_01_10T15_33_59.144282", "path": ["**/details_harness|hendrycksTest-high_school_computer_science|5_2024-01-10T15-33-59.144282.parquet"]}, {"split": "latest", "path": ["**/details_harness|hendrycksTest-high_school_computer_science|5_2024-01-10T15-33-59.144282.parquet"]}]}, {"config_name": "harness_hendrycksTest_high_school_european_history_5", "data_files": [{"split": "2024_01_10T15_33_59.144282", "path": ["**/details_harness|hendrycksTest-high_school_european_history|5_2024-01-10T15-33-59.144282.parquet"]}, {"split": "latest", "path": ["**/details_harness|hendrycksTest-high_school_european_history|5_2024-01-10T15-33-59.144282.parquet"]}]}, {"config_name": "harness_hendrycksTest_high_school_geography_5", "data_files": [{"split": "2024_01_10T15_33_59.144282", "path": ["**/details_harness|hendrycksTest-high_school_geography|5_2024-01-10T15-33-59.144282.parquet"]}, {"split": "latest", "path": ["**/details_harness|hendrycksTest-high_school_geography|5_2024-01-10T15-33-59.144282.parquet"]}]}, {"config_name": "harness_hendrycksTest_high_school_government_and_politics_5", "data_files": [{"split": "2024_01_10T15_33_59.144282", "path": ["**/details_harness|hendrycksTest-high_school_government_and_politics|5_2024-01-10T15-33-59.144282.parquet"]}, {"split": "latest", "path": ["**/details_harness|hendrycksTest-high_school_government_and_politics|5_2024-01-10T15-33-59.144282.parquet"]}]}, {"config_name": "harness_hendrycksTest_high_school_macroeconomics_5", "data_files": [{"split": "2024_01_10T15_33_59.144282", "path": ["**/details_harness|hendrycksTest-high_school_macroeconomics|5_2024-01-10T15-33-59.144282.parquet"]}, {"split": "latest", "path": ["**/details_harness|hendrycksTest-high_school_macroeconomics|5_2024-01-10T15-33-59.144282.parquet"]}]}, {"config_name": "harness_hendrycksTest_high_school_mathematics_5", "data_files": [{"split": "2024_01_10T15_33_59.144282", "path": ["**/details_harness|hendrycksTest-high_school_mathematics|5_2024-01-10T15-33-59.144282.parquet"]}, {"split": "latest", "path": ["**/details_harness|hendrycksTest-high_school_mathematics|5_2024-01-10T15-33-59.144282.parquet"]}]}, {"config_name": "harness_hendrycksTest_high_school_microeconomics_5", "data_files": [{"split": "2024_01_10T15_33_59.144282", "path": ["**/details_harness|hendrycksTest-high_school_microeconomics|5_2024-01-10T15-33-59.144282.parquet"]}, {"split": "latest", "path": ["**/details_harness|hendrycksTest-high_school_microeconomics|5_2024-01-10T15-33-59.144282.parquet"]}]}, {"config_name": "harness_hendrycksTest_high_school_physics_5", "data_files": [{"split": "2024_01_10T15_33_59.144282", "path": ["**/details_harness|hendrycksTest-high_school_physics|5_2024-01-10T15-33-59.144282.parquet"]}, {"split": "latest", "path": ["**/details_harness|hendrycksTest-high_school_physics|5_2024-01-10T15-33-59.144282.parquet"]}]}, {"config_name": "harness_hendrycksTest_high_school_psychology_5", "data_files": [{"split": "2024_01_10T15_33_59.144282", "path": ["**/details_harness|hendrycksTest-high_school_psychology|5_2024-01-10T15-33-59.144282.parquet"]}, {"split": "latest", "path": ["**/details_harness|hendrycksTest-high_school_psychology|5_2024-01-10T15-33-59.144282.parquet"]}]}, {"config_name": "harness_hendrycksTest_high_school_statistics_5", "data_files": [{"split": "2024_01_10T15_33_59.144282", "path": ["**/details_harness|hendrycksTest-high_school_statistics|5_2024-01-10T15-33-59.144282.parquet"]}, {"split": "latest", "path": ["**/details_harness|hendrycksTest-high_school_statistics|5_2024-01-10T15-33-59.144282.parquet"]}]}, {"config_name": "harness_hendrycksTest_high_school_us_history_5", "data_files": [{"split": "2024_01_10T15_33_59.144282", "path": ["**/details_harness|hendrycksTest-high_school_us_history|5_2024-01-10T15-33-59.144282.parquet"]}, {"split": "latest", "path": ["**/details_harness|hendrycksTest-high_school_us_history|5_2024-01-10T15-33-59.144282.parquet"]}]}, {"config_name": "harness_hendrycksTest_high_school_world_history_5", "data_files": [{"split": "2024_01_10T15_33_59.144282", "path": ["**/details_harness|hendrycksTest-high_school_world_history|5_2024-01-10T15-33-59.144282.parquet"]}, {"split": "latest", "path": ["**/details_harness|hendrycksTest-high_school_world_history|5_2024-01-10T15-33-59.144282.parquet"]}]}, {"config_name": "harness_hendrycksTest_human_aging_5", "data_files": [{"split": "2024_01_10T15_33_59.144282", "path": ["**/details_harness|hendrycksTest-human_aging|5_2024-01-10T15-33-59.144282.parquet"]}, {"split": "latest", "path": ["**/details_harness|hendrycksTest-human_aging|5_2024-01-10T15-33-59.144282.parquet"]}]}, {"config_name": "harness_hendrycksTest_human_sexuality_5", "data_files": [{"split": "2024_01_10T15_33_59.144282", "path": ["**/details_harness|hendrycksTest-human_sexuality|5_2024-01-10T15-33-59.144282.parquet"]}, {"split": "latest", "path": ["**/details_harness|hendrycksTest-human_sexuality|5_2024-01-10T15-33-59.144282.parquet"]}]}, {"config_name": "harness_hendrycksTest_international_law_5", "data_files": [{"split": "2024_01_10T15_33_59.144282", "path": ["**/details_harness|hendrycksTest-international_law|5_2024-01-10T15-33-59.144282.parquet"]}, {"split": "latest", "path": ["**/details_harness|hendrycksTest-international_law|5_2024-01-10T15-33-59.144282.parquet"]}]}, {"config_name": "harness_hendrycksTest_jurisprudence_5", "data_files": [{"split": "2024_01_10T15_33_59.144282", "path": ["**/details_harness|hendrycksTest-jurisprudence|5_2024-01-10T15-33-59.144282.parquet"]}, {"split": "latest", "path": ["**/details_harness|hendrycksTest-jurisprudence|5_2024-01-10T15-33-59.144282.parquet"]}]}, {"config_name": "harness_hendrycksTest_logical_fallacies_5", "data_files": [{"split": "2024_01_10T15_33_59.144282", "path": ["**/details_harness|hendrycksTest-logical_fallacies|5_2024-01-10T15-33-59.144282.parquet"]}, {"split": "latest", "path": ["**/details_harness|hendrycksTest-logical_fallacies|5_2024-01-10T15-33-59.144282.parquet"]}]}, {"config_name": "harness_hendrycksTest_machine_learning_5", "data_files": [{"split": "2024_01_10T15_33_59.144282", "path": ["**/details_harness|hendrycksTest-machine_learning|5_2024-01-10T15-33-59.144282.parquet"]}, {"split": "latest", "path": ["**/details_harness|hendrycksTest-machine_learning|5_2024-01-10T15-33-59.144282.parquet"]}]}, {"config_name": "harness_hendrycksTest_management_5", "data_files": [{"split": "2024_01_10T15_33_59.144282", "path": ["**/details_harness|hendrycksTest-management|5_2024-01-10T15-33-59.144282.parquet"]}, {"split": "latest", "path": ["**/details_harness|hendrycksTest-management|5_2024-01-10T15-33-59.144282.parquet"]}]}, {"config_name": "harness_hendrycksTest_marketing_5", "data_files": [{"split": "2024_01_10T15_33_59.144282", "path": ["**/details_harness|hendrycksTest-marketing|5_2024-01-10T15-33-59.144282.parquet"]}, {"split": "latest", "path": ["**/details_harness|hendrycksTest-marketing|5_2024-01-10T15-33-59.144282.parquet"]}]}, {"config_name": "harness_hendrycksTest_medical_genetics_5", "data_files": [{"split": "2024_01_10T15_33_59.144282", "path": ["**/details_harness|hendrycksTest-medical_genetics|5_2024-01-10T15-33-59.144282.parquet"]}, {"split": "latest", "path": ["**/details_harness|hendrycksTest-medical_genetics|5_2024-01-10T15-33-59.144282.parquet"]}]}, {"config_name": "harness_hendrycksTest_miscellaneous_5", "data_files": [{"split": "2024_01_10T15_33_59.144282", "path": ["**/details_harness|hendrycksTest-miscellaneous|5_2024-01-10T15-33-59.144282.parquet"]}, {"split": "latest", "path": ["**/details_harness|hendrycksTest-miscellaneous|5_2024-01-10T15-33-59.144282.parquet"]}]}, {"config_name": "harness_hendrycksTest_moral_disputes_5", "data_files": [{"split": "2024_01_10T15_33_59.144282", "path": ["**/details_harness|hendrycksTest-moral_disputes|5_2024-01-10T15-33-59.144282.parquet"]}, {"split": "latest", "path": ["**/details_harness|hendrycksTest-moral_disputes|5_2024-01-10T15-33-59.144282.parquet"]}]}, {"config_name": "harness_hendrycksTest_moral_scenarios_5", "data_files": [{"split": "2024_01_10T15_33_59.144282", "path": ["**/details_harness|hendrycksTest-moral_scenarios|5_2024-01-10T15-33-59.144282.parquet"]}, {"split": "latest", "path": ["**/details_harness|hendrycksTest-moral_scenarios|5_2024-01-10T15-33-59.144282.parquet"]}]}, {"config_name": "harness_hendrycksTest_nutrition_5", "data_files": [{"split": "2024_01_10T15_33_59.144282", "path": ["**/details_harness|hendrycksTest-nutrition|5_2024-01-10T15-33-59.144282.parquet"]}, {"split": "latest", "path": ["**/details_harness|hendrycksTest-nutrition|5_2024-01-10T15-33-59.144282.parquet"]}]}, {"config_name": "harness_hendrycksTest_philosophy_5", "data_files": [{"split": "2024_01_10T15_33_59.144282", "path": ["**/details_harness|hendrycksTest-philosophy|5_2024-01-10T15-33-59.144282.parquet"]}, {"split": "latest", "path": ["**/details_harness|hendrycksTest-philosophy|5_2024-01-10T15-33-59.144282.parquet"]}]}, {"config_name": "harness_hendrycksTest_prehistory_5", "data_files": [{"split": "2024_01_10T15_33_59.144282", "path": ["**/details_harness|hendrycksTest-prehistory|5_2024-01-10T15-33-59.144282.parquet"]}, {"split": "latest", "path": ["**/details_harness|hendrycksTest-prehistory|5_2024-01-10T15-33-59.144282.parquet"]}]}, {"config_name": "harness_hendrycksTest_professional_accounting_5", "data_files": [{"split": "2024_01_10T15_33_59.144282", "path": ["**/details_harness|hendrycksTest-professional_accounting|5_2024-01-10T15-33-59.144282.parquet"]}, {"split": "latest", "path": ["**/details_harness|hendrycksTest-professional_accounting|5_2024-01-10T15-33-59.144282.parquet"]}]}, {"config_name": "harness_hendrycksTest_professional_law_5", "data_files": [{"split": "2024_01_10T15_33_59.144282", "path": ["**/details_harness|hendrycksTest-professional_law|5_2024-01-10T15-33-59.144282.parquet"]}, {"split": "latest", "path": ["**/details_harness|hendrycksTest-professional_law|5_2024-01-10T15-33-59.144282.parquet"]}]}, {"config_name": "harness_hendrycksTest_professional_medicine_5", "data_files": [{"split": "2024_01_10T15_33_59.144282", "path": ["**/details_harness|hendrycksTest-professional_medicine|5_2024-01-10T15-33-59.144282.parquet"]}, {"split": "latest", "path": ["**/details_harness|hendrycksTest-professional_medicine|5_2024-01-10T15-33-59.144282.parquet"]}]}, {"config_name": "harness_hendrycksTest_professional_psychology_5", "data_files": [{"split": "2024_01_10T15_33_59.144282", "path": ["**/details_harness|hendrycksTest-professional_psychology|5_2024-01-10T15-33-59.144282.parquet"]}, {"split": "latest", "path": ["**/details_harness|hendrycksTest-professional_psychology|5_2024-01-10T15-33-59.144282.parquet"]}]}, {"config_name": "harness_hendrycksTest_public_relations_5", "data_files": [{"split": "2024_01_10T15_33_59.144282", "path": ["**/details_harness|hendrycksTest-public_relations|5_2024-01-10T15-33-59.144282.parquet"]}, {"split": "latest", "path": ["**/details_harness|hendrycksTest-public_relations|5_2024-01-10T15-33-59.144282.parquet"]}]}, {"config_name": "harness_hendrycksTest_security_studies_5", "data_files": [{"split": "2024_01_10T15_33_59.144282", "path": ["**/details_harness|hendrycksTest-security_studies|5_2024-01-10T15-33-59.144282.parquet"]}, {"split": "latest", "path": ["**/details_harness|hendrycksTest-security_studies|5_2024-01-10T15-33-59.144282.parquet"]}]}, {"config_name": "harness_hendrycksTest_sociology_5", "data_files": [{"split": "2024_01_10T15_33_59.144282", "path": ["**/details_harness|hendrycksTest-sociology|5_2024-01-10T15-33-59.144282.parquet"]}, {"split": "latest", "path": ["**/details_harness|hendrycksTest-sociology|5_2024-01-10T15-33-59.144282.parquet"]}]}, {"config_name": "harness_hendrycksTest_us_foreign_policy_5", "data_files": [{"split": "2024_01_10T15_33_59.144282", "path": ["**/details_harness|hendrycksTest-us_foreign_policy|5_2024-01-10T15-33-59.144282.parquet"]}, {"split": "latest", "path": ["**/details_harness|hendrycksTest-us_foreign_policy|5_2024-01-10T15-33-59.144282.parquet"]}]}, {"config_name": "harness_hendrycksTest_virology_5", "data_files": [{"split": "2024_01_10T15_33_59.144282", "path": ["**/details_harness|hendrycksTest-virology|5_2024-01-10T15-33-59.144282.parquet"]}, {"split": "latest", "path": ["**/details_harness|hendrycksTest-virology|5_2024-01-10T15-33-59.144282.parquet"]}]}, {"config_name": "harness_hendrycksTest_world_religions_5", "data_files": [{"split": "2024_01_10T15_33_59.144282", "path": ["**/details_harness|hendrycksTest-world_religions|5_2024-01-10T15-33-59.144282.parquet"]}, {"split": "latest", "path": ["**/details_harness|hendrycksTest-world_religions|5_2024-01-10T15-33-59.144282.parquet"]}]}, {"config_name": "harness_truthfulqa_mc_0", "data_files": [{"split": "2024_01_10T15_33_59.144282", "path": ["**/details_harness|truthfulqa:mc|0_2024-01-10T15-33-59.144282.parquet"]}, {"split": "latest", "path": ["**/details_harness|truthfulqa:mc|0_2024-01-10T15-33-59.144282.parquet"]}]}, {"config_name": "harness_winogrande_5", "data_files": [{"split": "2024_01_10T15_33_59.144282", "path": ["**/details_harness|winogrande|5_2024-01-10T15-33-59.144282.parquet"]}, {"split": "latest", "path": ["**/details_harness|winogrande|5_2024-01-10T15-33-59.144282.parquet"]}]}, {"config_name": "results", "data_files": [{"split": "2024_01_10T15_33_59.144282", "path": ["results_2024-01-10T15-33-59.144282.parquet"]}, {"split": "latest", "path": ["results_2024-01-10T15-33-59.144282.parquet"]}]}]} | 2024-01-10T15:36:46+00:00 | [] | [] | TAGS
#region-us
|
# Dataset Card for Evaluation run of bn22/DolphinMini-Mistral-7B
Dataset automatically created during the evaluation run of model bn22/DolphinMini-Mistral-7B on the Open LLM Leaderboard.
The dataset is composed of 63 configuration, each one coresponding to one of the evaluated task.
The dataset has been created from 1 run(s). Each run can be found as a specific split in each configuration, the split being named using the timestamp of the run.The "train" split is always pointing to the latest results.
An additional configuration "results" store all the aggregated results of the run (and is used to compute and display the aggregated metrics on the Open LLM Leaderboard).
To load the details from a run, you can for instance do the following:
## Latest results
These are the latest results from run 2024-01-10T15:33:59.144282(note that their might be results for other tasks in the repos if successive evals didn't cover the same tasks. You find each in the results and the "latest" split for each eval):
## Dataset Details
### Dataset Description
- Curated by:
- Funded by [optional]:
- Shared by [optional]:
- Language(s) (NLP):
- License:
### Dataset Sources [optional]
- Repository:
- Paper [optional]:
- Demo [optional]:
## Uses
### Direct Use
### Out-of-Scope Use
## Dataset Structure
## Dataset Creation
### Curation Rationale
### Source Data
#### Data Collection and Processing
#### Who are the source data producers?
### Annotations [optional]
#### Annotation process
#### Who are the annotators?
#### Personal and Sensitive Information
## Bias, Risks, and Limitations
### Recommendations
Users should be made aware of the risks, biases and limitations of the dataset. More information needed for further recommendations.
[optional]
BibTeX:
APA:
## Glossary [optional]
## More Information [optional]
## Dataset Card Authors [optional]
## Dataset Card Contact
| [
"# Dataset Card for Evaluation run of bn22/DolphinMini-Mistral-7B\n\n\n\nDataset automatically created during the evaluation run of model bn22/DolphinMini-Mistral-7B on the Open LLM Leaderboard.\n\nThe dataset is composed of 63 configuration, each one coresponding to one of the evaluated task.\n\nThe dataset has been created from 1 run(s). Each run can be found as a specific split in each configuration, the split being named using the timestamp of the run.The \"train\" split is always pointing to the latest results.\n\nAn additional configuration \"results\" store all the aggregated results of the run (and is used to compute and display the aggregated metrics on the Open LLM Leaderboard).\n\nTo load the details from a run, you can for instance do the following:",
"## Latest results\n\nThese are the latest results from run 2024-01-10T15:33:59.144282(note that their might be results for other tasks in the repos if successive evals didn't cover the same tasks. You find each in the results and the \"latest\" split for each eval):",
"## Dataset Details",
"### Dataset Description\n\n\n\n\n\n- Curated by: \n- Funded by [optional]: \n- Shared by [optional]: \n- Language(s) (NLP): \n- License:",
"### Dataset Sources [optional]\n\n\n\n- Repository: \n- Paper [optional]: \n- Demo [optional]:",
"## Uses",
"### Direct Use",
"### Out-of-Scope Use",
"## Dataset Structure",
"## Dataset Creation",
"### Curation Rationale",
"### Source Data",
"#### Data Collection and Processing",
"#### Who are the source data producers?",
"### Annotations [optional]",
"#### Annotation process",
"#### Who are the annotators?",
"#### Personal and Sensitive Information",
"## Bias, Risks, and Limitations",
"### Recommendations\n\n\n\nUsers should be made aware of the risks, biases and limitations of the dataset. More information needed for further recommendations.\n\n[optional]\n\n\n\nBibTeX:\n\n\n\nAPA:",
"## Glossary [optional]",
"## More Information [optional]",
"## Dataset Card Authors [optional]",
"## Dataset Card Contact"
] | [
"TAGS\n#region-us \n",
"# Dataset Card for Evaluation run of bn22/DolphinMini-Mistral-7B\n\n\n\nDataset automatically created during the evaluation run of model bn22/DolphinMini-Mistral-7B on the Open LLM Leaderboard.\n\nThe dataset is composed of 63 configuration, each one coresponding to one of the evaluated task.\n\nThe dataset has been created from 1 run(s). Each run can be found as a specific split in each configuration, the split being named using the timestamp of the run.The \"train\" split is always pointing to the latest results.\n\nAn additional configuration \"results\" store all the aggregated results of the run (and is used to compute and display the aggregated metrics on the Open LLM Leaderboard).\n\nTo load the details from a run, you can for instance do the following:",
"## Latest results\n\nThese are the latest results from run 2024-01-10T15:33:59.144282(note that their might be results for other tasks in the repos if successive evals didn't cover the same tasks. You find each in the results and the \"latest\" split for each eval):",
"## Dataset Details",
"### Dataset Description\n\n\n\n\n\n- Curated by: \n- Funded by [optional]: \n- Shared by [optional]: \n- Language(s) (NLP): \n- License:",
"### Dataset Sources [optional]\n\n\n\n- Repository: \n- Paper [optional]: \n- Demo [optional]:",
"## Uses",
"### Direct Use",
"### Out-of-Scope Use",
"## Dataset Structure",
"## Dataset Creation",
"### Curation Rationale",
"### Source Data",
"#### Data Collection and Processing",
"#### Who are the source data producers?",
"### Annotations [optional]",
"#### Annotation process",
"#### Who are the annotators?",
"#### Personal and Sensitive Information",
"## Bias, Risks, and Limitations",
"### Recommendations\n\n\n\nUsers should be made aware of the risks, biases and limitations of the dataset. More information needed for further recommendations.\n\n[optional]\n\n\n\nBibTeX:\n\n\n\nAPA:",
"## Glossary [optional]",
"## More Information [optional]",
"## Dataset Card Authors [optional]",
"## Dataset Card Contact"
] |
78831b0c4fbe82b0f63d1d102108044d5378137d | # Dataset Card for "agieval-gaokao-biology"
Dataset taken from https://github.com/microsoft/AGIEval and processed as in that repo, following dmayhem93/agieval-* datasets on the HF hub.
This dataset contains the contents of the Gaokao Biology subtask of AGIEval, as accessed in https://github.com/ruixiangcui/AGIEval/commit/5c77d073fda993f1652eaae3cf5d04cc5fd21d40 .
Citation:
```
@misc{zhong2023agieval,
title={AGIEval: A Human-Centric Benchmark for Evaluating Foundation Models},
author={Wanjun Zhong and Ruixiang Cui and Yiduo Guo and Yaobo Liang and Shuai Lu and Yanlin Wang and Amin Saied and Weizhu Chen and Nan Duan},
year={2023},
eprint={2304.06364},
archivePrefix={arXiv},
primaryClass={cs.CL}
}
```
Please make sure to cite all the individual datasets in your paper when you use them. We provide the relevant citation information below:
```
@inproceedings{ling-etal-2017-program,
title = "Program Induction by Rationale Generation: Learning to Solve and Explain Algebraic Word Problems",
author = "Ling, Wang and
Yogatama, Dani and
Dyer, Chris and
Blunsom, Phil",
booktitle = "Proceedings of the 55th Annual Meeting of the Association for Computational Linguistics (Volume 1: Long Papers)",
month = jul,
year = "2017",
address = "Vancouver, Canada",
publisher = "Association for Computational Linguistics",
url = "https://aclanthology.org/P17-1015",
doi = "10.18653/v1/P17-1015",
pages = "158--167",
abstract = "Solving algebraic word problems requires executing a series of arithmetic operations{---}a program{---}to obtain a final answer. However, since programs can be arbitrarily complicated, inducing them directly from question-answer pairs is a formidable challenge. To make this task more feasible, we solve these problems by generating answer rationales, sequences of natural language and human-readable mathematical expressions that derive the final answer through a series of small steps. Although rationales do not explicitly specify programs, they provide a scaffolding for their structure via intermediate milestones. To evaluate our approach, we have created a new 100,000-sample dataset of questions, answers and rationales. Experimental results show that indirect supervision of program learning via answer rationales is a promising strategy for inducing arithmetic programs.",
}
@inproceedings{hendrycksmath2021,
title={Measuring Mathematical Problem Solving With the MATH Dataset},
author={Dan Hendrycks and Collin Burns and Saurav Kadavath and Akul Arora and Steven Basart and Eric Tang and Dawn Song and Jacob Steinhardt},
journal={NeurIPS},
year={2021}
}
@inproceedings{Liu2020LogiQAAC,
title={LogiQA: A Challenge Dataset for Machine Reading Comprehension with Logical Reasoning},
author={Jian Liu and Leyang Cui and Hanmeng Liu and Dandan Huang and Yile Wang and Yue Zhang},
booktitle={International Joint Conference on Artificial Intelligence},
year={2020}
}
@inproceedings{zhong2019jec,
title={JEC-QA: A Legal-Domain Question Answering Dataset},
author={Zhong, Haoxi and Xiao, Chaojun and Tu, Cunchao and Zhang, Tianyang and Liu, Zhiyuan and Sun, Maosong},
booktitle={Proceedings of AAAI},
year={2020},
}
@article{Wang2021FromLT,
title={From LSAT: The Progress and Challenges of Complex Reasoning},
author={Siyuan Wang and Zhongkun Liu and Wanjun Zhong and Ming Zhou and Zhongyu Wei and Zhumin Chen and Nan Duan},
journal={IEEE/ACM Transactions on Audio, Speech, and Language Processing},
year={2021},
volume={30},
pages={2201-2216}
}
``` | hails/agieval-gaokao-biology | [
"arxiv:2304.06364",
"region:us"
] | 2024-01-10T15:40:21+00:00 | {"dataset_info": {"features": [{"name": "query", "dtype": "string"}, {"name": "choices", "sequence": "string"}, {"name": "gold", "sequence": "int64"}], "splits": [{"name": "test", "num_bytes": 159178, "num_examples": 210}], "download_size": 94294, "dataset_size": 159178}, "configs": [{"config_name": "default", "data_files": [{"split": "test", "path": "data/test-*"}]}]} | 2024-01-26T18:36:41+00:00 | [
"2304.06364"
] | [] | TAGS
#arxiv-2304.06364 #region-us
| # Dataset Card for "agieval-gaokao-biology"
Dataset taken from URL and processed as in that repo, following dmayhem93/agieval-* datasets on the HF hub.
This dataset contains the contents of the Gaokao Biology subtask of AGIEval, as accessed in URL .
Citation:
Please make sure to cite all the individual datasets in your paper when you use them. We provide the relevant citation information below:
| [
"# Dataset Card for \"agieval-gaokao-biology\"\n\n\nDataset taken from URL and processed as in that repo, following dmayhem93/agieval-* datasets on the HF hub.\n\nThis dataset contains the contents of the Gaokao Biology subtask of AGIEval, as accessed in URL .\n\n\nCitation:\n\n\nPlease make sure to cite all the individual datasets in your paper when you use them. We provide the relevant citation information below:"
] | [
"TAGS\n#arxiv-2304.06364 #region-us \n",
"# Dataset Card for \"agieval-gaokao-biology\"\n\n\nDataset taken from URL and processed as in that repo, following dmayhem93/agieval-* datasets on the HF hub.\n\nThis dataset contains the contents of the Gaokao Biology subtask of AGIEval, as accessed in URL .\n\n\nCitation:\n\n\nPlease make sure to cite all the individual datasets in your paper when you use them. We provide the relevant citation information below:"
] |
f2a53909de37b3b7c084863fe4ea8fa691aeeae8 |
# Dataset Card for Evaluation run of quantumaikr/quantum-v0.01
<!-- Provide a quick summary of the dataset. -->
Dataset automatically created during the evaluation run of model [quantumaikr/quantum-v0.01](https://huggingface.co/quantumaikr/quantum-v0.01) on the [Open LLM Leaderboard](https://huggingface.co/spaces/HuggingFaceH4/open_llm_leaderboard).
The dataset is composed of 63 configuration, each one coresponding to one of the evaluated task.
The dataset has been created from 1 run(s). Each run can be found as a specific split in each configuration, the split being named using the timestamp of the run.The "train" split is always pointing to the latest results.
An additional configuration "results" store all the aggregated results of the run (and is used to compute and display the aggregated metrics on the [Open LLM Leaderboard](https://huggingface.co/spaces/HuggingFaceH4/open_llm_leaderboard)).
To load the details from a run, you can for instance do the following:
```python
from datasets import load_dataset
data = load_dataset("open-llm-leaderboard/details_quantumaikr__quantum-v0.01",
"harness_winogrande_5",
split="train")
```
## Latest results
These are the [latest results from run 2024-01-10T15:38:18.408039](https://huggingface.co/datasets/open-llm-leaderboard/details_quantumaikr__quantum-v0.01/blob/main/results_2024-01-10T15-38-18.408039.json)(note that their might be results for other tasks in the repos if successive evals didn't cover the same tasks. You find each in the results and the "latest" split for each eval):
```python
{
"all": {
"acc": 0.6572995040063592,
"acc_stderr": 0.03199963347273244,
"acc_norm": 0.6571345413469432,
"acc_norm_stderr": 0.032660707489366475,
"mc1": 0.5495716034271726,
"mc1_stderr": 0.01741726437196764,
"mc2": 0.6927526472916785,
"mc2_stderr": 0.015028880570718646
},
"harness|arc:challenge|25": {
"acc": 0.6936860068259386,
"acc_stderr": 0.013470584417276513,
"acc_norm": 0.7252559726962458,
"acc_norm_stderr": 0.013044617212771227
},
"harness|hellaswag|10": {
"acc": 0.7102170882294364,
"acc_stderr": 0.004527343651130798,
"acc_norm": 0.882692690699064,
"acc_norm_stderr": 0.0032112847607016636
},
"harness|hendrycksTest-abstract_algebra|5": {
"acc": 0.35,
"acc_stderr": 0.0479372485441102,
"acc_norm": 0.35,
"acc_norm_stderr": 0.0479372485441102
},
"harness|hendrycksTest-anatomy|5": {
"acc": 0.6444444444444445,
"acc_stderr": 0.04135176749720386,
"acc_norm": 0.6444444444444445,
"acc_norm_stderr": 0.04135176749720386
},
"harness|hendrycksTest-astronomy|5": {
"acc": 0.6907894736842105,
"acc_stderr": 0.037610708698674805,
"acc_norm": 0.6907894736842105,
"acc_norm_stderr": 0.037610708698674805
},
"harness|hendrycksTest-business_ethics|5": {
"acc": 0.61,
"acc_stderr": 0.04902071300001975,
"acc_norm": 0.61,
"acc_norm_stderr": 0.04902071300001975
},
"harness|hendrycksTest-clinical_knowledge|5": {
"acc": 0.720754716981132,
"acc_stderr": 0.027611163402399715,
"acc_norm": 0.720754716981132,
"acc_norm_stderr": 0.027611163402399715
},
"harness|hendrycksTest-college_biology|5": {
"acc": 0.7708333333333334,
"acc_stderr": 0.03514697467862388,
"acc_norm": 0.7708333333333334,
"acc_norm_stderr": 0.03514697467862388
},
"harness|hendrycksTest-college_chemistry|5": {
"acc": 0.47,
"acc_stderr": 0.050161355804659205,
"acc_norm": 0.47,
"acc_norm_stderr": 0.050161355804659205
},
"harness|hendrycksTest-college_computer_science|5": {
"acc": 0.55,
"acc_stderr": 0.05,
"acc_norm": 0.55,
"acc_norm_stderr": 0.05
},
"harness|hendrycksTest-college_mathematics|5": {
"acc": 0.31,
"acc_stderr": 0.04648231987117316,
"acc_norm": 0.31,
"acc_norm_stderr": 0.04648231987117316
},
"harness|hendrycksTest-college_medicine|5": {
"acc": 0.6936416184971098,
"acc_stderr": 0.035149425512674394,
"acc_norm": 0.6936416184971098,
"acc_norm_stderr": 0.035149425512674394
},
"harness|hendrycksTest-college_physics|5": {
"acc": 0.46078431372549017,
"acc_stderr": 0.04959859966384181,
"acc_norm": 0.46078431372549017,
"acc_norm_stderr": 0.04959859966384181
},
"harness|hendrycksTest-computer_security|5": {
"acc": 0.77,
"acc_stderr": 0.042295258468165065,
"acc_norm": 0.77,
"acc_norm_stderr": 0.042295258468165065
},
"harness|hendrycksTest-conceptual_physics|5": {
"acc": 0.5829787234042553,
"acc_stderr": 0.03223276266711712,
"acc_norm": 0.5829787234042553,
"acc_norm_stderr": 0.03223276266711712
},
"harness|hendrycksTest-econometrics|5": {
"acc": 0.5,
"acc_stderr": 0.047036043419179864,
"acc_norm": 0.5,
"acc_norm_stderr": 0.047036043419179864
},
"harness|hendrycksTest-electrical_engineering|5": {
"acc": 0.5793103448275863,
"acc_stderr": 0.0411391498118926,
"acc_norm": 0.5793103448275863,
"acc_norm_stderr": 0.0411391498118926
},
"harness|hendrycksTest-elementary_mathematics|5": {
"acc": 0.42063492063492064,
"acc_stderr": 0.025424835086923996,
"acc_norm": 0.42063492063492064,
"acc_norm_stderr": 0.025424835086923996
},
"harness|hendrycksTest-formal_logic|5": {
"acc": 0.46825396825396826,
"acc_stderr": 0.04463112720677172,
"acc_norm": 0.46825396825396826,
"acc_norm_stderr": 0.04463112720677172
},
"harness|hendrycksTest-global_facts|5": {
"acc": 0.34,
"acc_stderr": 0.04760952285695235,
"acc_norm": 0.34,
"acc_norm_stderr": 0.04760952285695235
},
"harness|hendrycksTest-high_school_biology|5": {
"acc": 0.7806451612903226,
"acc_stderr": 0.023540799358723295,
"acc_norm": 0.7806451612903226,
"acc_norm_stderr": 0.023540799358723295
},
"harness|hendrycksTest-high_school_chemistry|5": {
"acc": 0.5221674876847291,
"acc_stderr": 0.03514528562175007,
"acc_norm": 0.5221674876847291,
"acc_norm_stderr": 0.03514528562175007
},
"harness|hendrycksTest-high_school_computer_science|5": {
"acc": 0.71,
"acc_stderr": 0.045604802157206845,
"acc_norm": 0.71,
"acc_norm_stderr": 0.045604802157206845
},
"harness|hendrycksTest-high_school_european_history|5": {
"acc": 0.7757575757575758,
"acc_stderr": 0.03256866661681102,
"acc_norm": 0.7757575757575758,
"acc_norm_stderr": 0.03256866661681102
},
"harness|hendrycksTest-high_school_geography|5": {
"acc": 0.7929292929292929,
"acc_stderr": 0.028869778460267042,
"acc_norm": 0.7929292929292929,
"acc_norm_stderr": 0.028869778460267042
},
"harness|hendrycksTest-high_school_government_and_politics|5": {
"acc": 0.8963730569948186,
"acc_stderr": 0.02199531196364424,
"acc_norm": 0.8963730569948186,
"acc_norm_stderr": 0.02199531196364424
},
"harness|hendrycksTest-high_school_macroeconomics|5": {
"acc": 0.6666666666666666,
"acc_stderr": 0.023901157979402538,
"acc_norm": 0.6666666666666666,
"acc_norm_stderr": 0.023901157979402538
},
"harness|hendrycksTest-high_school_mathematics|5": {
"acc": 0.3296296296296296,
"acc_stderr": 0.028661201116524565,
"acc_norm": 0.3296296296296296,
"acc_norm_stderr": 0.028661201116524565
},
"harness|hendrycksTest-high_school_microeconomics|5": {
"acc": 0.6722689075630253,
"acc_stderr": 0.03048991141767323,
"acc_norm": 0.6722689075630253,
"acc_norm_stderr": 0.03048991141767323
},
"harness|hendrycksTest-high_school_physics|5": {
"acc": 0.3443708609271523,
"acc_stderr": 0.038796870240733264,
"acc_norm": 0.3443708609271523,
"acc_norm_stderr": 0.038796870240733264
},
"harness|hendrycksTest-high_school_psychology|5": {
"acc": 0.8550458715596331,
"acc_stderr": 0.01509421569970048,
"acc_norm": 0.8550458715596331,
"acc_norm_stderr": 0.01509421569970048
},
"harness|hendrycksTest-high_school_statistics|5": {
"acc": 0.5509259259259259,
"acc_stderr": 0.03392238405321617,
"acc_norm": 0.5509259259259259,
"acc_norm_stderr": 0.03392238405321617
},
"harness|hendrycksTest-high_school_us_history|5": {
"acc": 0.8284313725490197,
"acc_stderr": 0.026460569561240644,
"acc_norm": 0.8284313725490197,
"acc_norm_stderr": 0.026460569561240644
},
"harness|hendrycksTest-high_school_world_history|5": {
"acc": 0.8059071729957806,
"acc_stderr": 0.025744902532290916,
"acc_norm": 0.8059071729957806,
"acc_norm_stderr": 0.025744902532290916
},
"harness|hendrycksTest-human_aging|5": {
"acc": 0.6905829596412556,
"acc_stderr": 0.031024411740572213,
"acc_norm": 0.6905829596412556,
"acc_norm_stderr": 0.031024411740572213
},
"harness|hendrycksTest-human_sexuality|5": {
"acc": 0.8244274809160306,
"acc_stderr": 0.03336820338476074,
"acc_norm": 0.8244274809160306,
"acc_norm_stderr": 0.03336820338476074
},
"harness|hendrycksTest-international_law|5": {
"acc": 0.8099173553719008,
"acc_stderr": 0.03581796951709282,
"acc_norm": 0.8099173553719008,
"acc_norm_stderr": 0.03581796951709282
},
"harness|hendrycksTest-jurisprudence|5": {
"acc": 0.7777777777777778,
"acc_stderr": 0.040191074725573483,
"acc_norm": 0.7777777777777778,
"acc_norm_stderr": 0.040191074725573483
},
"harness|hendrycksTest-logical_fallacies|5": {
"acc": 0.7852760736196319,
"acc_stderr": 0.03226219377286775,
"acc_norm": 0.7852760736196319,
"acc_norm_stderr": 0.03226219377286775
},
"harness|hendrycksTest-machine_learning|5": {
"acc": 0.42857142857142855,
"acc_stderr": 0.04697113923010212,
"acc_norm": 0.42857142857142855,
"acc_norm_stderr": 0.04697113923010212
},
"harness|hendrycksTest-management|5": {
"acc": 0.7572815533980582,
"acc_stderr": 0.04245022486384495,
"acc_norm": 0.7572815533980582,
"acc_norm_stderr": 0.04245022486384495
},
"harness|hendrycksTest-marketing|5": {
"acc": 0.8803418803418803,
"acc_stderr": 0.021262719400406957,
"acc_norm": 0.8803418803418803,
"acc_norm_stderr": 0.021262719400406957
},
"harness|hendrycksTest-medical_genetics|5": {
"acc": 0.71,
"acc_stderr": 0.045604802157206845,
"acc_norm": 0.71,
"acc_norm_stderr": 0.045604802157206845
},
"harness|hendrycksTest-miscellaneous|5": {
"acc": 0.8365261813537676,
"acc_stderr": 0.013223928616741622,
"acc_norm": 0.8365261813537676,
"acc_norm_stderr": 0.013223928616741622
},
"harness|hendrycksTest-moral_disputes|5": {
"acc": 0.7398843930635838,
"acc_stderr": 0.023618678310069356,
"acc_norm": 0.7398843930635838,
"acc_norm_stderr": 0.023618678310069356
},
"harness|hendrycksTest-moral_scenarios|5": {
"acc": 0.4782122905027933,
"acc_stderr": 0.016706617522176132,
"acc_norm": 0.4782122905027933,
"acc_norm_stderr": 0.016706617522176132
},
"harness|hendrycksTest-nutrition|5": {
"acc": 0.7254901960784313,
"acc_stderr": 0.025553169991826528,
"acc_norm": 0.7254901960784313,
"acc_norm_stderr": 0.025553169991826528
},
"harness|hendrycksTest-philosophy|5": {
"acc": 0.7138263665594855,
"acc_stderr": 0.02567025924218893,
"acc_norm": 0.7138263665594855,
"acc_norm_stderr": 0.02567025924218893
},
"harness|hendrycksTest-prehistory|5": {
"acc": 0.7407407407407407,
"acc_stderr": 0.02438366553103545,
"acc_norm": 0.7407407407407407,
"acc_norm_stderr": 0.02438366553103545
},
"harness|hendrycksTest-professional_accounting|5": {
"acc": 0.4929078014184397,
"acc_stderr": 0.02982449855912901,
"acc_norm": 0.4929078014184397,
"acc_norm_stderr": 0.02982449855912901
},
"harness|hendrycksTest-professional_law|5": {
"acc": 0.46936114732724904,
"acc_stderr": 0.012746237711716634,
"acc_norm": 0.46936114732724904,
"acc_norm_stderr": 0.012746237711716634
},
"harness|hendrycksTest-professional_medicine|5": {
"acc": 0.6875,
"acc_stderr": 0.02815637344037142,
"acc_norm": 0.6875,
"acc_norm_stderr": 0.02815637344037142
},
"harness|hendrycksTest-professional_psychology|5": {
"acc": 0.6699346405228758,
"acc_stderr": 0.019023726160724553,
"acc_norm": 0.6699346405228758,
"acc_norm_stderr": 0.019023726160724553
},
"harness|hendrycksTest-public_relations|5": {
"acc": 0.6909090909090909,
"acc_stderr": 0.044262946482000985,
"acc_norm": 0.6909090909090909,
"acc_norm_stderr": 0.044262946482000985
},
"harness|hendrycksTest-security_studies|5": {
"acc": 0.7224489795918367,
"acc_stderr": 0.028666857790274648,
"acc_norm": 0.7224489795918367,
"acc_norm_stderr": 0.028666857790274648
},
"harness|hendrycksTest-sociology|5": {
"acc": 0.8407960199004975,
"acc_stderr": 0.025870646766169136,
"acc_norm": 0.8407960199004975,
"acc_norm_stderr": 0.025870646766169136
},
"harness|hendrycksTest-us_foreign_policy|5": {
"acc": 0.86,
"acc_stderr": 0.0348735088019777,
"acc_norm": 0.86,
"acc_norm_stderr": 0.0348735088019777
},
"harness|hendrycksTest-virology|5": {
"acc": 0.5421686746987951,
"acc_stderr": 0.038786267710023595,
"acc_norm": 0.5421686746987951,
"acc_norm_stderr": 0.038786267710023595
},
"harness|hendrycksTest-world_religions|5": {
"acc": 0.8245614035087719,
"acc_stderr": 0.029170885500727665,
"acc_norm": 0.8245614035087719,
"acc_norm_stderr": 0.029170885500727665
},
"harness|truthfulqa:mc|0": {
"mc1": 0.5495716034271726,
"mc1_stderr": 0.01741726437196764,
"mc2": 0.6927526472916785,
"mc2_stderr": 0.015028880570718646
},
"harness|winogrande|5": {
"acc": 0.8255722178374112,
"acc_stderr": 0.010665187902498428
},
"harness|gsm8k|5": {
"acc": 0.7028051554207733,
"acc_stderr": 0.012588685966624179
}
}
```
## Dataset Details
### Dataset Description
<!-- Provide a longer summary of what this dataset is. -->
- **Curated by:** [More Information Needed]
- **Funded by [optional]:** [More Information Needed]
- **Shared by [optional]:** [More Information Needed]
- **Language(s) (NLP):** [More Information Needed]
- **License:** [More Information Needed]
### Dataset Sources [optional]
<!-- Provide the basic links for the dataset. -->
- **Repository:** [More Information Needed]
- **Paper [optional]:** [More Information Needed]
- **Demo [optional]:** [More Information Needed]
## Uses
<!-- Address questions around how the dataset is intended to be used. -->
### Direct Use
<!-- This section describes suitable use cases for the dataset. -->
[More Information Needed]
### Out-of-Scope Use
<!-- This section addresses misuse, malicious use, and uses that the dataset will not work well for. -->
[More Information Needed]
## Dataset Structure
<!-- This section provides a description of the dataset fields, and additional information about the dataset structure such as criteria used to create the splits, relationships between data points, etc. -->
[More Information Needed]
## Dataset Creation
### Curation Rationale
<!-- Motivation for the creation of this dataset. -->
[More Information Needed]
### Source Data
<!-- This section describes the source data (e.g. news text and headlines, social media posts, translated sentences, ...). -->
#### Data Collection and Processing
<!-- This section describes the data collection and processing process such as data selection criteria, filtering and normalization methods, tools and libraries used, etc. -->
[More Information Needed]
#### Who are the source data producers?
<!-- This section describes the people or systems who originally created the data. It should also include self-reported demographic or identity information for the source data creators if this information is available. -->
[More Information Needed]
### Annotations [optional]
<!-- If the dataset contains annotations which are not part of the initial data collection, use this section to describe them. -->
#### Annotation process
<!-- This section describes the annotation process such as annotation tools used in the process, the amount of data annotated, annotation guidelines provided to the annotators, interannotator statistics, annotation validation, etc. -->
[More Information Needed]
#### Who are the annotators?
<!-- This section describes the people or systems who created the annotations. -->
[More Information Needed]
#### Personal and Sensitive Information
<!-- State whether the dataset contains data that might be considered personal, sensitive, or private (e.g., data that reveals addresses, uniquely identifiable names or aliases, racial or ethnic origins, sexual orientations, religious beliefs, political opinions, financial or health data, etc.). If efforts were made to anonymize the data, describe the anonymization process. -->
[More Information Needed]
## Bias, Risks, and Limitations
<!-- This section is meant to convey both technical and sociotechnical limitations. -->
[More Information Needed]
### Recommendations
<!-- This section is meant to convey recommendations with respect to the bias, risk, and technical limitations. -->
Users should be made aware of the risks, biases and limitations of the dataset. More information needed for further recommendations.
## Citation [optional]
<!-- If there is a paper or blog post introducing the dataset, the APA and Bibtex information for that should go in this section. -->
**BibTeX:**
[More Information Needed]
**APA:**
[More Information Needed]
## Glossary [optional]
<!-- If relevant, include terms and calculations in this section that can help readers understand the dataset or dataset card. -->
[More Information Needed]
## More Information [optional]
[More Information Needed]
## Dataset Card Authors [optional]
[More Information Needed]
## Dataset Card Contact
[More Information Needed] | open-llm-leaderboard/details_quantumaikr__quantum-v0.01 | [
"region:us"
] | 2024-01-10T15:40:35+00:00 | {"pretty_name": "Evaluation run of quantumaikr/quantum-v0.01", "dataset_summary": "Dataset automatically created during the evaluation run of model [quantumaikr/quantum-v0.01](https://huggingface.co/quantumaikr/quantum-v0.01) on the [Open LLM Leaderboard](https://huggingface.co/spaces/HuggingFaceH4/open_llm_leaderboard).\n\nThe dataset is composed of 63 configuration, each one coresponding to one of the evaluated task.\n\nThe dataset has been created from 1 run(s). Each run can be found as a specific split in each configuration, the split being named using the timestamp of the run.The \"train\" split is always pointing to the latest results.\n\nAn additional configuration \"results\" store all the aggregated results of the run (and is used to compute and display the aggregated metrics on the [Open LLM Leaderboard](https://huggingface.co/spaces/HuggingFaceH4/open_llm_leaderboard)).\n\nTo load the details from a run, you can for instance do the following:\n```python\nfrom datasets import load_dataset\ndata = load_dataset(\"open-llm-leaderboard/details_quantumaikr__quantum-v0.01\",\n\t\"harness_winogrande_5\",\n\tsplit=\"train\")\n```\n\n## Latest results\n\nThese are the [latest results from run 2024-01-10T15:38:18.408039](https://huggingface.co/datasets/open-llm-leaderboard/details_quantumaikr__quantum-v0.01/blob/main/results_2024-01-10T15-38-18.408039.json)(note that their might be results for other tasks in the repos if successive evals didn't cover the same tasks. You find each in the results and the \"latest\" split for each eval):\n\n```python\n{\n \"all\": {\n \"acc\": 0.6572995040063592,\n \"acc_stderr\": 0.03199963347273244,\n \"acc_norm\": 0.6571345413469432,\n \"acc_norm_stderr\": 0.032660707489366475,\n \"mc1\": 0.5495716034271726,\n \"mc1_stderr\": 0.01741726437196764,\n \"mc2\": 0.6927526472916785,\n \"mc2_stderr\": 0.015028880570718646\n },\n \"harness|arc:challenge|25\": {\n \"acc\": 0.6936860068259386,\n \"acc_stderr\": 0.013470584417276513,\n \"acc_norm\": 0.7252559726962458,\n \"acc_norm_stderr\": 0.013044617212771227\n },\n \"harness|hellaswag|10\": {\n \"acc\": 0.7102170882294364,\n \"acc_stderr\": 0.004527343651130798,\n \"acc_norm\": 0.882692690699064,\n \"acc_norm_stderr\": 0.0032112847607016636\n },\n \"harness|hendrycksTest-abstract_algebra|5\": {\n \"acc\": 0.35,\n \"acc_stderr\": 0.0479372485441102,\n \"acc_norm\": 0.35,\n \"acc_norm_stderr\": 0.0479372485441102\n },\n \"harness|hendrycksTest-anatomy|5\": {\n \"acc\": 0.6444444444444445,\n \"acc_stderr\": 0.04135176749720386,\n \"acc_norm\": 0.6444444444444445,\n \"acc_norm_stderr\": 0.04135176749720386\n },\n \"harness|hendrycksTest-astronomy|5\": {\n \"acc\": 0.6907894736842105,\n \"acc_stderr\": 0.037610708698674805,\n \"acc_norm\": 0.6907894736842105,\n \"acc_norm_stderr\": 0.037610708698674805\n },\n \"harness|hendrycksTest-business_ethics|5\": {\n \"acc\": 0.61,\n \"acc_stderr\": 0.04902071300001975,\n \"acc_norm\": 0.61,\n \"acc_norm_stderr\": 0.04902071300001975\n },\n \"harness|hendrycksTest-clinical_knowledge|5\": {\n \"acc\": 0.720754716981132,\n \"acc_stderr\": 0.027611163402399715,\n \"acc_norm\": 0.720754716981132,\n \"acc_norm_stderr\": 0.027611163402399715\n },\n \"harness|hendrycksTest-college_biology|5\": {\n \"acc\": 0.7708333333333334,\n \"acc_stderr\": 0.03514697467862388,\n \"acc_norm\": 0.7708333333333334,\n \"acc_norm_stderr\": 0.03514697467862388\n },\n \"harness|hendrycksTest-college_chemistry|5\": {\n \"acc\": 0.47,\n \"acc_stderr\": 0.050161355804659205,\n \"acc_norm\": 0.47,\n \"acc_norm_stderr\": 0.050161355804659205\n },\n \"harness|hendrycksTest-college_computer_science|5\": {\n \"acc\": 0.55,\n \"acc_stderr\": 0.05,\n \"acc_norm\": 0.55,\n \"acc_norm_stderr\": 0.05\n },\n \"harness|hendrycksTest-college_mathematics|5\": {\n \"acc\": 0.31,\n \"acc_stderr\": 0.04648231987117316,\n \"acc_norm\": 0.31,\n \"acc_norm_stderr\": 0.04648231987117316\n },\n \"harness|hendrycksTest-college_medicine|5\": {\n \"acc\": 0.6936416184971098,\n \"acc_stderr\": 0.035149425512674394,\n \"acc_norm\": 0.6936416184971098,\n \"acc_norm_stderr\": 0.035149425512674394\n },\n \"harness|hendrycksTest-college_physics|5\": {\n \"acc\": 0.46078431372549017,\n \"acc_stderr\": 0.04959859966384181,\n \"acc_norm\": 0.46078431372549017,\n \"acc_norm_stderr\": 0.04959859966384181\n },\n \"harness|hendrycksTest-computer_security|5\": {\n \"acc\": 0.77,\n \"acc_stderr\": 0.042295258468165065,\n \"acc_norm\": 0.77,\n \"acc_norm_stderr\": 0.042295258468165065\n },\n \"harness|hendrycksTest-conceptual_physics|5\": {\n \"acc\": 0.5829787234042553,\n \"acc_stderr\": 0.03223276266711712,\n \"acc_norm\": 0.5829787234042553,\n \"acc_norm_stderr\": 0.03223276266711712\n },\n \"harness|hendrycksTest-econometrics|5\": {\n \"acc\": 0.5,\n \"acc_stderr\": 0.047036043419179864,\n \"acc_norm\": 0.5,\n \"acc_norm_stderr\": 0.047036043419179864\n },\n \"harness|hendrycksTest-electrical_engineering|5\": {\n \"acc\": 0.5793103448275863,\n \"acc_stderr\": 0.0411391498118926,\n \"acc_norm\": 0.5793103448275863,\n \"acc_norm_stderr\": 0.0411391498118926\n },\n \"harness|hendrycksTest-elementary_mathematics|5\": {\n \"acc\": 0.42063492063492064,\n \"acc_stderr\": 0.025424835086923996,\n \"acc_norm\": 0.42063492063492064,\n \"acc_norm_stderr\": 0.025424835086923996\n },\n \"harness|hendrycksTest-formal_logic|5\": {\n \"acc\": 0.46825396825396826,\n \"acc_stderr\": 0.04463112720677172,\n \"acc_norm\": 0.46825396825396826,\n \"acc_norm_stderr\": 0.04463112720677172\n },\n \"harness|hendrycksTest-global_facts|5\": {\n \"acc\": 0.34,\n \"acc_stderr\": 0.04760952285695235,\n \"acc_norm\": 0.34,\n \"acc_norm_stderr\": 0.04760952285695235\n },\n \"harness|hendrycksTest-high_school_biology|5\": {\n \"acc\": 0.7806451612903226,\n \"acc_stderr\": 0.023540799358723295,\n \"acc_norm\": 0.7806451612903226,\n \"acc_norm_stderr\": 0.023540799358723295\n },\n \"harness|hendrycksTest-high_school_chemistry|5\": {\n \"acc\": 0.5221674876847291,\n \"acc_stderr\": 0.03514528562175007,\n \"acc_norm\": 0.5221674876847291,\n \"acc_norm_stderr\": 0.03514528562175007\n },\n \"harness|hendrycksTest-high_school_computer_science|5\": {\n \"acc\": 0.71,\n \"acc_stderr\": 0.045604802157206845,\n \"acc_norm\": 0.71,\n \"acc_norm_stderr\": 0.045604802157206845\n },\n \"harness|hendrycksTest-high_school_european_history|5\": {\n \"acc\": 0.7757575757575758,\n \"acc_stderr\": 0.03256866661681102,\n \"acc_norm\": 0.7757575757575758,\n \"acc_norm_stderr\": 0.03256866661681102\n },\n \"harness|hendrycksTest-high_school_geography|5\": {\n \"acc\": 0.7929292929292929,\n \"acc_stderr\": 0.028869778460267042,\n \"acc_norm\": 0.7929292929292929,\n \"acc_norm_stderr\": 0.028869778460267042\n },\n \"harness|hendrycksTest-high_school_government_and_politics|5\": {\n \"acc\": 0.8963730569948186,\n \"acc_stderr\": 0.02199531196364424,\n \"acc_norm\": 0.8963730569948186,\n \"acc_norm_stderr\": 0.02199531196364424\n },\n \"harness|hendrycksTest-high_school_macroeconomics|5\": {\n \"acc\": 0.6666666666666666,\n \"acc_stderr\": 0.023901157979402538,\n \"acc_norm\": 0.6666666666666666,\n \"acc_norm_stderr\": 0.023901157979402538\n },\n \"harness|hendrycksTest-high_school_mathematics|5\": {\n \"acc\": 0.3296296296296296,\n \"acc_stderr\": 0.028661201116524565,\n \"acc_norm\": 0.3296296296296296,\n \"acc_norm_stderr\": 0.028661201116524565\n },\n \"harness|hendrycksTest-high_school_microeconomics|5\": {\n \"acc\": 0.6722689075630253,\n \"acc_stderr\": 0.03048991141767323,\n \"acc_norm\": 0.6722689075630253,\n \"acc_norm_stderr\": 0.03048991141767323\n },\n \"harness|hendrycksTest-high_school_physics|5\": {\n \"acc\": 0.3443708609271523,\n \"acc_stderr\": 0.038796870240733264,\n \"acc_norm\": 0.3443708609271523,\n \"acc_norm_stderr\": 0.038796870240733264\n },\n \"harness|hendrycksTest-high_school_psychology|5\": {\n \"acc\": 0.8550458715596331,\n \"acc_stderr\": 0.01509421569970048,\n \"acc_norm\": 0.8550458715596331,\n \"acc_norm_stderr\": 0.01509421569970048\n },\n \"harness|hendrycksTest-high_school_statistics|5\": {\n \"acc\": 0.5509259259259259,\n \"acc_stderr\": 0.03392238405321617,\n \"acc_norm\": 0.5509259259259259,\n \"acc_norm_stderr\": 0.03392238405321617\n },\n \"harness|hendrycksTest-high_school_us_history|5\": {\n \"acc\": 0.8284313725490197,\n \"acc_stderr\": 0.026460569561240644,\n \"acc_norm\": 0.8284313725490197,\n \"acc_norm_stderr\": 0.026460569561240644\n },\n \"harness|hendrycksTest-high_school_world_history|5\": {\n \"acc\": 0.8059071729957806,\n \"acc_stderr\": 0.025744902532290916,\n \"acc_norm\": 0.8059071729957806,\n \"acc_norm_stderr\": 0.025744902532290916\n },\n \"harness|hendrycksTest-human_aging|5\": {\n \"acc\": 0.6905829596412556,\n \"acc_stderr\": 0.031024411740572213,\n \"acc_norm\": 0.6905829596412556,\n \"acc_norm_stderr\": 0.031024411740572213\n },\n \"harness|hendrycksTest-human_sexuality|5\": {\n \"acc\": 0.8244274809160306,\n \"acc_stderr\": 0.03336820338476074,\n \"acc_norm\": 0.8244274809160306,\n \"acc_norm_stderr\": 0.03336820338476074\n },\n \"harness|hendrycksTest-international_law|5\": {\n \"acc\": 0.8099173553719008,\n \"acc_stderr\": 0.03581796951709282,\n \"acc_norm\": 0.8099173553719008,\n \"acc_norm_stderr\": 0.03581796951709282\n },\n \"harness|hendrycksTest-jurisprudence|5\": {\n \"acc\": 0.7777777777777778,\n \"acc_stderr\": 0.040191074725573483,\n \"acc_norm\": 0.7777777777777778,\n \"acc_norm_stderr\": 0.040191074725573483\n },\n \"harness|hendrycksTest-logical_fallacies|5\": {\n \"acc\": 0.7852760736196319,\n \"acc_stderr\": 0.03226219377286775,\n \"acc_norm\": 0.7852760736196319,\n \"acc_norm_stderr\": 0.03226219377286775\n },\n \"harness|hendrycksTest-machine_learning|5\": {\n \"acc\": 0.42857142857142855,\n \"acc_stderr\": 0.04697113923010212,\n \"acc_norm\": 0.42857142857142855,\n \"acc_norm_stderr\": 0.04697113923010212\n },\n \"harness|hendrycksTest-management|5\": {\n \"acc\": 0.7572815533980582,\n \"acc_stderr\": 0.04245022486384495,\n \"acc_norm\": 0.7572815533980582,\n \"acc_norm_stderr\": 0.04245022486384495\n },\n \"harness|hendrycksTest-marketing|5\": {\n \"acc\": 0.8803418803418803,\n \"acc_stderr\": 0.021262719400406957,\n \"acc_norm\": 0.8803418803418803,\n \"acc_norm_stderr\": 0.021262719400406957\n },\n \"harness|hendrycksTest-medical_genetics|5\": {\n \"acc\": 0.71,\n \"acc_stderr\": 0.045604802157206845,\n \"acc_norm\": 0.71,\n \"acc_norm_stderr\": 0.045604802157206845\n },\n \"harness|hendrycksTest-miscellaneous|5\": {\n \"acc\": 0.8365261813537676,\n \"acc_stderr\": 0.013223928616741622,\n \"acc_norm\": 0.8365261813537676,\n \"acc_norm_stderr\": 0.013223928616741622\n },\n \"harness|hendrycksTest-moral_disputes|5\": {\n \"acc\": 0.7398843930635838,\n \"acc_stderr\": 0.023618678310069356,\n \"acc_norm\": 0.7398843930635838,\n \"acc_norm_stderr\": 0.023618678310069356\n },\n \"harness|hendrycksTest-moral_scenarios|5\": {\n \"acc\": 0.4782122905027933,\n \"acc_stderr\": 0.016706617522176132,\n \"acc_norm\": 0.4782122905027933,\n \"acc_norm_stderr\": 0.016706617522176132\n },\n \"harness|hendrycksTest-nutrition|5\": {\n \"acc\": 0.7254901960784313,\n \"acc_stderr\": 0.025553169991826528,\n \"acc_norm\": 0.7254901960784313,\n \"acc_norm_stderr\": 0.025553169991826528\n },\n \"harness|hendrycksTest-philosophy|5\": {\n \"acc\": 0.7138263665594855,\n \"acc_stderr\": 0.02567025924218893,\n \"acc_norm\": 0.7138263665594855,\n \"acc_norm_stderr\": 0.02567025924218893\n },\n \"harness|hendrycksTest-prehistory|5\": {\n \"acc\": 0.7407407407407407,\n \"acc_stderr\": 0.02438366553103545,\n \"acc_norm\": 0.7407407407407407,\n \"acc_norm_stderr\": 0.02438366553103545\n },\n \"harness|hendrycksTest-professional_accounting|5\": {\n \"acc\": 0.4929078014184397,\n \"acc_stderr\": 0.02982449855912901,\n \"acc_norm\": 0.4929078014184397,\n \"acc_norm_stderr\": 0.02982449855912901\n },\n \"harness|hendrycksTest-professional_law|5\": {\n \"acc\": 0.46936114732724904,\n \"acc_stderr\": 0.012746237711716634,\n \"acc_norm\": 0.46936114732724904,\n \"acc_norm_stderr\": 0.012746237711716634\n },\n \"harness|hendrycksTest-professional_medicine|5\": {\n \"acc\": 0.6875,\n \"acc_stderr\": 0.02815637344037142,\n \"acc_norm\": 0.6875,\n \"acc_norm_stderr\": 0.02815637344037142\n },\n \"harness|hendrycksTest-professional_psychology|5\": {\n \"acc\": 0.6699346405228758,\n \"acc_stderr\": 0.019023726160724553,\n \"acc_norm\": 0.6699346405228758,\n \"acc_norm_stderr\": 0.019023726160724553\n },\n \"harness|hendrycksTest-public_relations|5\": {\n \"acc\": 0.6909090909090909,\n \"acc_stderr\": 0.044262946482000985,\n \"acc_norm\": 0.6909090909090909,\n \"acc_norm_stderr\": 0.044262946482000985\n },\n \"harness|hendrycksTest-security_studies|5\": {\n \"acc\": 0.7224489795918367,\n \"acc_stderr\": 0.028666857790274648,\n \"acc_norm\": 0.7224489795918367,\n \"acc_norm_stderr\": 0.028666857790274648\n },\n \"harness|hendrycksTest-sociology|5\": {\n \"acc\": 0.8407960199004975,\n \"acc_stderr\": 0.025870646766169136,\n \"acc_norm\": 0.8407960199004975,\n \"acc_norm_stderr\": 0.025870646766169136\n },\n \"harness|hendrycksTest-us_foreign_policy|5\": {\n \"acc\": 0.86,\n \"acc_stderr\": 0.0348735088019777,\n \"acc_norm\": 0.86,\n \"acc_norm_stderr\": 0.0348735088019777\n },\n \"harness|hendrycksTest-virology|5\": {\n \"acc\": 0.5421686746987951,\n \"acc_stderr\": 0.038786267710023595,\n \"acc_norm\": 0.5421686746987951,\n \"acc_norm_stderr\": 0.038786267710023595\n },\n \"harness|hendrycksTest-world_religions|5\": {\n \"acc\": 0.8245614035087719,\n \"acc_stderr\": 0.029170885500727665,\n \"acc_norm\": 0.8245614035087719,\n \"acc_norm_stderr\": 0.029170885500727665\n },\n \"harness|truthfulqa:mc|0\": {\n \"mc1\": 0.5495716034271726,\n \"mc1_stderr\": 0.01741726437196764,\n \"mc2\": 0.6927526472916785,\n \"mc2_stderr\": 0.015028880570718646\n },\n \"harness|winogrande|5\": {\n \"acc\": 0.8255722178374112,\n \"acc_stderr\": 0.010665187902498428\n },\n \"harness|gsm8k|5\": {\n \"acc\": 0.7028051554207733,\n \"acc_stderr\": 0.012588685966624179\n }\n}\n```", "repo_url": "https://huggingface.co/quantumaikr/quantum-v0.01", "leaderboard_url": "https://huggingface.co/spaces/HuggingFaceH4/open_llm_leaderboard", "point_of_contact": "[email protected]", "configs": [{"config_name": "harness_arc_challenge_25", "data_files": [{"split": "2024_01_10T15_38_18.408039", "path": ["**/details_harness|arc:challenge|25_2024-01-10T15-38-18.408039.parquet"]}, {"split": "latest", "path": ["**/details_harness|arc:challenge|25_2024-01-10T15-38-18.408039.parquet"]}]}, {"config_name": "harness_gsm8k_5", "data_files": [{"split": "2024_01_10T15_38_18.408039", "path": ["**/details_harness|gsm8k|5_2024-01-10T15-38-18.408039.parquet"]}, {"split": "latest", "path": ["**/details_harness|gsm8k|5_2024-01-10T15-38-18.408039.parquet"]}]}, {"config_name": "harness_hellaswag_10", "data_files": [{"split": "2024_01_10T15_38_18.408039", "path": ["**/details_harness|hellaswag|10_2024-01-10T15-38-18.408039.parquet"]}, {"split": "latest", "path": ["**/details_harness|hellaswag|10_2024-01-10T15-38-18.408039.parquet"]}]}, {"config_name": "harness_hendrycksTest_5", "data_files": [{"split": "2024_01_10T15_38_18.408039", "path": ["**/details_harness|hendrycksTest-abstract_algebra|5_2024-01-10T15-38-18.408039.parquet", "**/details_harness|hendrycksTest-anatomy|5_2024-01-10T15-38-18.408039.parquet", "**/details_harness|hendrycksTest-astronomy|5_2024-01-10T15-38-18.408039.parquet", "**/details_harness|hendrycksTest-business_ethics|5_2024-01-10T15-38-18.408039.parquet", "**/details_harness|hendrycksTest-clinical_knowledge|5_2024-01-10T15-38-18.408039.parquet", "**/details_harness|hendrycksTest-college_biology|5_2024-01-10T15-38-18.408039.parquet", "**/details_harness|hendrycksTest-college_chemistry|5_2024-01-10T15-38-18.408039.parquet", "**/details_harness|hendrycksTest-college_computer_science|5_2024-01-10T15-38-18.408039.parquet", "**/details_harness|hendrycksTest-college_mathematics|5_2024-01-10T15-38-18.408039.parquet", "**/details_harness|hendrycksTest-college_medicine|5_2024-01-10T15-38-18.408039.parquet", "**/details_harness|hendrycksTest-college_physics|5_2024-01-10T15-38-18.408039.parquet", "**/details_harness|hendrycksTest-computer_security|5_2024-01-10T15-38-18.408039.parquet", "**/details_harness|hendrycksTest-conceptual_physics|5_2024-01-10T15-38-18.408039.parquet", "**/details_harness|hendrycksTest-econometrics|5_2024-01-10T15-38-18.408039.parquet", "**/details_harness|hendrycksTest-electrical_engineering|5_2024-01-10T15-38-18.408039.parquet", "**/details_harness|hendrycksTest-elementary_mathematics|5_2024-01-10T15-38-18.408039.parquet", "**/details_harness|hendrycksTest-formal_logic|5_2024-01-10T15-38-18.408039.parquet", "**/details_harness|hendrycksTest-global_facts|5_2024-01-10T15-38-18.408039.parquet", "**/details_harness|hendrycksTest-high_school_biology|5_2024-01-10T15-38-18.408039.parquet", "**/details_harness|hendrycksTest-high_school_chemistry|5_2024-01-10T15-38-18.408039.parquet", "**/details_harness|hendrycksTest-high_school_computer_science|5_2024-01-10T15-38-18.408039.parquet", "**/details_harness|hendrycksTest-high_school_european_history|5_2024-01-10T15-38-18.408039.parquet", "**/details_harness|hendrycksTest-high_school_geography|5_2024-01-10T15-38-18.408039.parquet", "**/details_harness|hendrycksTest-high_school_government_and_politics|5_2024-01-10T15-38-18.408039.parquet", "**/details_harness|hendrycksTest-high_school_macroeconomics|5_2024-01-10T15-38-18.408039.parquet", "**/details_harness|hendrycksTest-high_school_mathematics|5_2024-01-10T15-38-18.408039.parquet", "**/details_harness|hendrycksTest-high_school_microeconomics|5_2024-01-10T15-38-18.408039.parquet", "**/details_harness|hendrycksTest-high_school_physics|5_2024-01-10T15-38-18.408039.parquet", "**/details_harness|hendrycksTest-high_school_psychology|5_2024-01-10T15-38-18.408039.parquet", "**/details_harness|hendrycksTest-high_school_statistics|5_2024-01-10T15-38-18.408039.parquet", "**/details_harness|hendrycksTest-high_school_us_history|5_2024-01-10T15-38-18.408039.parquet", "**/details_harness|hendrycksTest-high_school_world_history|5_2024-01-10T15-38-18.408039.parquet", "**/details_harness|hendrycksTest-human_aging|5_2024-01-10T15-38-18.408039.parquet", "**/details_harness|hendrycksTest-human_sexuality|5_2024-01-10T15-38-18.408039.parquet", "**/details_harness|hendrycksTest-international_law|5_2024-01-10T15-38-18.408039.parquet", "**/details_harness|hendrycksTest-jurisprudence|5_2024-01-10T15-38-18.408039.parquet", "**/details_harness|hendrycksTest-logical_fallacies|5_2024-01-10T15-38-18.408039.parquet", "**/details_harness|hendrycksTest-machine_learning|5_2024-01-10T15-38-18.408039.parquet", "**/details_harness|hendrycksTest-management|5_2024-01-10T15-38-18.408039.parquet", "**/details_harness|hendrycksTest-marketing|5_2024-01-10T15-38-18.408039.parquet", "**/details_harness|hendrycksTest-medical_genetics|5_2024-01-10T15-38-18.408039.parquet", "**/details_harness|hendrycksTest-miscellaneous|5_2024-01-10T15-38-18.408039.parquet", "**/details_harness|hendrycksTest-moral_disputes|5_2024-01-10T15-38-18.408039.parquet", "**/details_harness|hendrycksTest-moral_scenarios|5_2024-01-10T15-38-18.408039.parquet", "**/details_harness|hendrycksTest-nutrition|5_2024-01-10T15-38-18.408039.parquet", "**/details_harness|hendrycksTest-philosophy|5_2024-01-10T15-38-18.408039.parquet", "**/details_harness|hendrycksTest-prehistory|5_2024-01-10T15-38-18.408039.parquet", "**/details_harness|hendrycksTest-professional_accounting|5_2024-01-10T15-38-18.408039.parquet", "**/details_harness|hendrycksTest-professional_law|5_2024-01-10T15-38-18.408039.parquet", "**/details_harness|hendrycksTest-professional_medicine|5_2024-01-10T15-38-18.408039.parquet", "**/details_harness|hendrycksTest-professional_psychology|5_2024-01-10T15-38-18.408039.parquet", "**/details_harness|hendrycksTest-public_relations|5_2024-01-10T15-38-18.408039.parquet", "**/details_harness|hendrycksTest-security_studies|5_2024-01-10T15-38-18.408039.parquet", "**/details_harness|hendrycksTest-sociology|5_2024-01-10T15-38-18.408039.parquet", "**/details_harness|hendrycksTest-us_foreign_policy|5_2024-01-10T15-38-18.408039.parquet", "**/details_harness|hendrycksTest-virology|5_2024-01-10T15-38-18.408039.parquet", "**/details_harness|hendrycksTest-world_religions|5_2024-01-10T15-38-18.408039.parquet"]}, {"split": "latest", "path": ["**/details_harness|hendrycksTest-abstract_algebra|5_2024-01-10T15-38-18.408039.parquet", "**/details_harness|hendrycksTest-anatomy|5_2024-01-10T15-38-18.408039.parquet", "**/details_harness|hendrycksTest-astronomy|5_2024-01-10T15-38-18.408039.parquet", "**/details_harness|hendrycksTest-business_ethics|5_2024-01-10T15-38-18.408039.parquet", "**/details_harness|hendrycksTest-clinical_knowledge|5_2024-01-10T15-38-18.408039.parquet", "**/details_harness|hendrycksTest-college_biology|5_2024-01-10T15-38-18.408039.parquet", "**/details_harness|hendrycksTest-college_chemistry|5_2024-01-10T15-38-18.408039.parquet", "**/details_harness|hendrycksTest-college_computer_science|5_2024-01-10T15-38-18.408039.parquet", "**/details_harness|hendrycksTest-college_mathematics|5_2024-01-10T15-38-18.408039.parquet", "**/details_harness|hendrycksTest-college_medicine|5_2024-01-10T15-38-18.408039.parquet", "**/details_harness|hendrycksTest-college_physics|5_2024-01-10T15-38-18.408039.parquet", "**/details_harness|hendrycksTest-computer_security|5_2024-01-10T15-38-18.408039.parquet", "**/details_harness|hendrycksTest-conceptual_physics|5_2024-01-10T15-38-18.408039.parquet", "**/details_harness|hendrycksTest-econometrics|5_2024-01-10T15-38-18.408039.parquet", "**/details_harness|hendrycksTest-electrical_engineering|5_2024-01-10T15-38-18.408039.parquet", "**/details_harness|hendrycksTest-elementary_mathematics|5_2024-01-10T15-38-18.408039.parquet", "**/details_harness|hendrycksTest-formal_logic|5_2024-01-10T15-38-18.408039.parquet", "**/details_harness|hendrycksTest-global_facts|5_2024-01-10T15-38-18.408039.parquet", "**/details_harness|hendrycksTest-high_school_biology|5_2024-01-10T15-38-18.408039.parquet", "**/details_harness|hendrycksTest-high_school_chemistry|5_2024-01-10T15-38-18.408039.parquet", "**/details_harness|hendrycksTest-high_school_computer_science|5_2024-01-10T15-38-18.408039.parquet", "**/details_harness|hendrycksTest-high_school_european_history|5_2024-01-10T15-38-18.408039.parquet", "**/details_harness|hendrycksTest-high_school_geography|5_2024-01-10T15-38-18.408039.parquet", "**/details_harness|hendrycksTest-high_school_government_and_politics|5_2024-01-10T15-38-18.408039.parquet", "**/details_harness|hendrycksTest-high_school_macroeconomics|5_2024-01-10T15-38-18.408039.parquet", "**/details_harness|hendrycksTest-high_school_mathematics|5_2024-01-10T15-38-18.408039.parquet", "**/details_harness|hendrycksTest-high_school_microeconomics|5_2024-01-10T15-38-18.408039.parquet", "**/details_harness|hendrycksTest-high_school_physics|5_2024-01-10T15-38-18.408039.parquet", "**/details_harness|hendrycksTest-high_school_psychology|5_2024-01-10T15-38-18.408039.parquet", "**/details_harness|hendrycksTest-high_school_statistics|5_2024-01-10T15-38-18.408039.parquet", "**/details_harness|hendrycksTest-high_school_us_history|5_2024-01-10T15-38-18.408039.parquet", "**/details_harness|hendrycksTest-high_school_world_history|5_2024-01-10T15-38-18.408039.parquet", "**/details_harness|hendrycksTest-human_aging|5_2024-01-10T15-38-18.408039.parquet", "**/details_harness|hendrycksTest-human_sexuality|5_2024-01-10T15-38-18.408039.parquet", "**/details_harness|hendrycksTest-international_law|5_2024-01-10T15-38-18.408039.parquet", "**/details_harness|hendrycksTest-jurisprudence|5_2024-01-10T15-38-18.408039.parquet", "**/details_harness|hendrycksTest-logical_fallacies|5_2024-01-10T15-38-18.408039.parquet", "**/details_harness|hendrycksTest-machine_learning|5_2024-01-10T15-38-18.408039.parquet", "**/details_harness|hendrycksTest-management|5_2024-01-10T15-38-18.408039.parquet", "**/details_harness|hendrycksTest-marketing|5_2024-01-10T15-38-18.408039.parquet", "**/details_harness|hendrycksTest-medical_genetics|5_2024-01-10T15-38-18.408039.parquet", "**/details_harness|hendrycksTest-miscellaneous|5_2024-01-10T15-38-18.408039.parquet", "**/details_harness|hendrycksTest-moral_disputes|5_2024-01-10T15-38-18.408039.parquet", "**/details_harness|hendrycksTest-moral_scenarios|5_2024-01-10T15-38-18.408039.parquet", "**/details_harness|hendrycksTest-nutrition|5_2024-01-10T15-38-18.408039.parquet", "**/details_harness|hendrycksTest-philosophy|5_2024-01-10T15-38-18.408039.parquet", "**/details_harness|hendrycksTest-prehistory|5_2024-01-10T15-38-18.408039.parquet", "**/details_harness|hendrycksTest-professional_accounting|5_2024-01-10T15-38-18.408039.parquet", "**/details_harness|hendrycksTest-professional_law|5_2024-01-10T15-38-18.408039.parquet", "**/details_harness|hendrycksTest-professional_medicine|5_2024-01-10T15-38-18.408039.parquet", "**/details_harness|hendrycksTest-professional_psychology|5_2024-01-10T15-38-18.408039.parquet", "**/details_harness|hendrycksTest-public_relations|5_2024-01-10T15-38-18.408039.parquet", "**/details_harness|hendrycksTest-security_studies|5_2024-01-10T15-38-18.408039.parquet", "**/details_harness|hendrycksTest-sociology|5_2024-01-10T15-38-18.408039.parquet", "**/details_harness|hendrycksTest-us_foreign_policy|5_2024-01-10T15-38-18.408039.parquet", "**/details_harness|hendrycksTest-virology|5_2024-01-10T15-38-18.408039.parquet", "**/details_harness|hendrycksTest-world_religions|5_2024-01-10T15-38-18.408039.parquet"]}]}, {"config_name": "harness_hendrycksTest_abstract_algebra_5", "data_files": [{"split": "2024_01_10T15_38_18.408039", "path": ["**/details_harness|hendrycksTest-abstract_algebra|5_2024-01-10T15-38-18.408039.parquet"]}, {"split": "latest", "path": ["**/details_harness|hendrycksTest-abstract_algebra|5_2024-01-10T15-38-18.408039.parquet"]}]}, {"config_name": "harness_hendrycksTest_anatomy_5", "data_files": [{"split": "2024_01_10T15_38_18.408039", "path": ["**/details_harness|hendrycksTest-anatomy|5_2024-01-10T15-38-18.408039.parquet"]}, {"split": "latest", "path": ["**/details_harness|hendrycksTest-anatomy|5_2024-01-10T15-38-18.408039.parquet"]}]}, {"config_name": "harness_hendrycksTest_astronomy_5", "data_files": [{"split": "2024_01_10T15_38_18.408039", "path": ["**/details_harness|hendrycksTest-astronomy|5_2024-01-10T15-38-18.408039.parquet"]}, {"split": "latest", "path": ["**/details_harness|hendrycksTest-astronomy|5_2024-01-10T15-38-18.408039.parquet"]}]}, {"config_name": "harness_hendrycksTest_business_ethics_5", "data_files": [{"split": "2024_01_10T15_38_18.408039", "path": ["**/details_harness|hendrycksTest-business_ethics|5_2024-01-10T15-38-18.408039.parquet"]}, {"split": "latest", "path": ["**/details_harness|hendrycksTest-business_ethics|5_2024-01-10T15-38-18.408039.parquet"]}]}, {"config_name": "harness_hendrycksTest_clinical_knowledge_5", "data_files": [{"split": "2024_01_10T15_38_18.408039", "path": ["**/details_harness|hendrycksTest-clinical_knowledge|5_2024-01-10T15-38-18.408039.parquet"]}, {"split": "latest", "path": ["**/details_harness|hendrycksTest-clinical_knowledge|5_2024-01-10T15-38-18.408039.parquet"]}]}, {"config_name": "harness_hendrycksTest_college_biology_5", "data_files": [{"split": "2024_01_10T15_38_18.408039", "path": ["**/details_harness|hendrycksTest-college_biology|5_2024-01-10T15-38-18.408039.parquet"]}, {"split": "latest", "path": ["**/details_harness|hendrycksTest-college_biology|5_2024-01-10T15-38-18.408039.parquet"]}]}, {"config_name": "harness_hendrycksTest_college_chemistry_5", "data_files": [{"split": "2024_01_10T15_38_18.408039", "path": ["**/details_harness|hendrycksTest-college_chemistry|5_2024-01-10T15-38-18.408039.parquet"]}, {"split": "latest", "path": ["**/details_harness|hendrycksTest-college_chemistry|5_2024-01-10T15-38-18.408039.parquet"]}]}, {"config_name": "harness_hendrycksTest_college_computer_science_5", "data_files": [{"split": "2024_01_10T15_38_18.408039", "path": ["**/details_harness|hendrycksTest-college_computer_science|5_2024-01-10T15-38-18.408039.parquet"]}, {"split": "latest", "path": ["**/details_harness|hendrycksTest-college_computer_science|5_2024-01-10T15-38-18.408039.parquet"]}]}, {"config_name": "harness_hendrycksTest_college_mathematics_5", "data_files": [{"split": "2024_01_10T15_38_18.408039", "path": ["**/details_harness|hendrycksTest-college_mathematics|5_2024-01-10T15-38-18.408039.parquet"]}, {"split": "latest", "path": ["**/details_harness|hendrycksTest-college_mathematics|5_2024-01-10T15-38-18.408039.parquet"]}]}, {"config_name": "harness_hendrycksTest_college_medicine_5", "data_files": [{"split": "2024_01_10T15_38_18.408039", "path": ["**/details_harness|hendrycksTest-college_medicine|5_2024-01-10T15-38-18.408039.parquet"]}, {"split": "latest", "path": ["**/details_harness|hendrycksTest-college_medicine|5_2024-01-10T15-38-18.408039.parquet"]}]}, {"config_name": "harness_hendrycksTest_college_physics_5", "data_files": [{"split": "2024_01_10T15_38_18.408039", "path": ["**/details_harness|hendrycksTest-college_physics|5_2024-01-10T15-38-18.408039.parquet"]}, {"split": "latest", "path": ["**/details_harness|hendrycksTest-college_physics|5_2024-01-10T15-38-18.408039.parquet"]}]}, {"config_name": "harness_hendrycksTest_computer_security_5", "data_files": [{"split": "2024_01_10T15_38_18.408039", "path": ["**/details_harness|hendrycksTest-computer_security|5_2024-01-10T15-38-18.408039.parquet"]}, {"split": "latest", "path": ["**/details_harness|hendrycksTest-computer_security|5_2024-01-10T15-38-18.408039.parquet"]}]}, {"config_name": "harness_hendrycksTest_conceptual_physics_5", "data_files": [{"split": "2024_01_10T15_38_18.408039", "path": ["**/details_harness|hendrycksTest-conceptual_physics|5_2024-01-10T15-38-18.408039.parquet"]}, {"split": "latest", "path": ["**/details_harness|hendrycksTest-conceptual_physics|5_2024-01-10T15-38-18.408039.parquet"]}]}, {"config_name": "harness_hendrycksTest_econometrics_5", "data_files": [{"split": "2024_01_10T15_38_18.408039", "path": ["**/details_harness|hendrycksTest-econometrics|5_2024-01-10T15-38-18.408039.parquet"]}, {"split": "latest", "path": ["**/details_harness|hendrycksTest-econometrics|5_2024-01-10T15-38-18.408039.parquet"]}]}, {"config_name": "harness_hendrycksTest_electrical_engineering_5", "data_files": [{"split": "2024_01_10T15_38_18.408039", "path": ["**/details_harness|hendrycksTest-electrical_engineering|5_2024-01-10T15-38-18.408039.parquet"]}, {"split": "latest", "path": ["**/details_harness|hendrycksTest-electrical_engineering|5_2024-01-10T15-38-18.408039.parquet"]}]}, {"config_name": "harness_hendrycksTest_elementary_mathematics_5", "data_files": [{"split": "2024_01_10T15_38_18.408039", "path": ["**/details_harness|hendrycksTest-elementary_mathematics|5_2024-01-10T15-38-18.408039.parquet"]}, {"split": "latest", "path": ["**/details_harness|hendrycksTest-elementary_mathematics|5_2024-01-10T15-38-18.408039.parquet"]}]}, {"config_name": "harness_hendrycksTest_formal_logic_5", "data_files": [{"split": "2024_01_10T15_38_18.408039", "path": ["**/details_harness|hendrycksTest-formal_logic|5_2024-01-10T15-38-18.408039.parquet"]}, {"split": "latest", "path": ["**/details_harness|hendrycksTest-formal_logic|5_2024-01-10T15-38-18.408039.parquet"]}]}, {"config_name": "harness_hendrycksTest_global_facts_5", "data_files": [{"split": "2024_01_10T15_38_18.408039", "path": ["**/details_harness|hendrycksTest-global_facts|5_2024-01-10T15-38-18.408039.parquet"]}, {"split": "latest", "path": ["**/details_harness|hendrycksTest-global_facts|5_2024-01-10T15-38-18.408039.parquet"]}]}, {"config_name": "harness_hendrycksTest_high_school_biology_5", "data_files": [{"split": "2024_01_10T15_38_18.408039", "path": ["**/details_harness|hendrycksTest-high_school_biology|5_2024-01-10T15-38-18.408039.parquet"]}, {"split": "latest", "path": ["**/details_harness|hendrycksTest-high_school_biology|5_2024-01-10T15-38-18.408039.parquet"]}]}, {"config_name": "harness_hendrycksTest_high_school_chemistry_5", "data_files": [{"split": "2024_01_10T15_38_18.408039", "path": ["**/details_harness|hendrycksTest-high_school_chemistry|5_2024-01-10T15-38-18.408039.parquet"]}, {"split": "latest", "path": ["**/details_harness|hendrycksTest-high_school_chemistry|5_2024-01-10T15-38-18.408039.parquet"]}]}, {"config_name": "harness_hendrycksTest_high_school_computer_science_5", "data_files": [{"split": "2024_01_10T15_38_18.408039", "path": ["**/details_harness|hendrycksTest-high_school_computer_science|5_2024-01-10T15-38-18.408039.parquet"]}, {"split": "latest", "path": ["**/details_harness|hendrycksTest-high_school_computer_science|5_2024-01-10T15-38-18.408039.parquet"]}]}, {"config_name": "harness_hendrycksTest_high_school_european_history_5", "data_files": [{"split": "2024_01_10T15_38_18.408039", "path": ["**/details_harness|hendrycksTest-high_school_european_history|5_2024-01-10T15-38-18.408039.parquet"]}, {"split": "latest", "path": ["**/details_harness|hendrycksTest-high_school_european_history|5_2024-01-10T15-38-18.408039.parquet"]}]}, {"config_name": "harness_hendrycksTest_high_school_geography_5", "data_files": [{"split": "2024_01_10T15_38_18.408039", "path": ["**/details_harness|hendrycksTest-high_school_geography|5_2024-01-10T15-38-18.408039.parquet"]}, {"split": "latest", "path": ["**/details_harness|hendrycksTest-high_school_geography|5_2024-01-10T15-38-18.408039.parquet"]}]}, {"config_name": "harness_hendrycksTest_high_school_government_and_politics_5", "data_files": [{"split": "2024_01_10T15_38_18.408039", "path": ["**/details_harness|hendrycksTest-high_school_government_and_politics|5_2024-01-10T15-38-18.408039.parquet"]}, {"split": "latest", "path": ["**/details_harness|hendrycksTest-high_school_government_and_politics|5_2024-01-10T15-38-18.408039.parquet"]}]}, {"config_name": "harness_hendrycksTest_high_school_macroeconomics_5", "data_files": [{"split": "2024_01_10T15_38_18.408039", "path": ["**/details_harness|hendrycksTest-high_school_macroeconomics|5_2024-01-10T15-38-18.408039.parquet"]}, {"split": "latest", "path": ["**/details_harness|hendrycksTest-high_school_macroeconomics|5_2024-01-10T15-38-18.408039.parquet"]}]}, {"config_name": "harness_hendrycksTest_high_school_mathematics_5", "data_files": [{"split": "2024_01_10T15_38_18.408039", "path": ["**/details_harness|hendrycksTest-high_school_mathematics|5_2024-01-10T15-38-18.408039.parquet"]}, {"split": "latest", "path": ["**/details_harness|hendrycksTest-high_school_mathematics|5_2024-01-10T15-38-18.408039.parquet"]}]}, {"config_name": "harness_hendrycksTest_high_school_microeconomics_5", "data_files": [{"split": "2024_01_10T15_38_18.408039", "path": ["**/details_harness|hendrycksTest-high_school_microeconomics|5_2024-01-10T15-38-18.408039.parquet"]}, {"split": "latest", "path": ["**/details_harness|hendrycksTest-high_school_microeconomics|5_2024-01-10T15-38-18.408039.parquet"]}]}, {"config_name": "harness_hendrycksTest_high_school_physics_5", "data_files": [{"split": "2024_01_10T15_38_18.408039", "path": ["**/details_harness|hendrycksTest-high_school_physics|5_2024-01-10T15-38-18.408039.parquet"]}, {"split": "latest", "path": ["**/details_harness|hendrycksTest-high_school_physics|5_2024-01-10T15-38-18.408039.parquet"]}]}, {"config_name": "harness_hendrycksTest_high_school_psychology_5", "data_files": [{"split": "2024_01_10T15_38_18.408039", "path": ["**/details_harness|hendrycksTest-high_school_psychology|5_2024-01-10T15-38-18.408039.parquet"]}, {"split": "latest", "path": ["**/details_harness|hendrycksTest-high_school_psychology|5_2024-01-10T15-38-18.408039.parquet"]}]}, {"config_name": "harness_hendrycksTest_high_school_statistics_5", "data_files": [{"split": "2024_01_10T15_38_18.408039", "path": ["**/details_harness|hendrycksTest-high_school_statistics|5_2024-01-10T15-38-18.408039.parquet"]}, {"split": "latest", "path": ["**/details_harness|hendrycksTest-high_school_statistics|5_2024-01-10T15-38-18.408039.parquet"]}]}, {"config_name": "harness_hendrycksTest_high_school_us_history_5", "data_files": [{"split": "2024_01_10T15_38_18.408039", "path": ["**/details_harness|hendrycksTest-high_school_us_history|5_2024-01-10T15-38-18.408039.parquet"]}, {"split": "latest", "path": ["**/details_harness|hendrycksTest-high_school_us_history|5_2024-01-10T15-38-18.408039.parquet"]}]}, {"config_name": "harness_hendrycksTest_high_school_world_history_5", "data_files": [{"split": "2024_01_10T15_38_18.408039", "path": ["**/details_harness|hendrycksTest-high_school_world_history|5_2024-01-10T15-38-18.408039.parquet"]}, {"split": "latest", "path": ["**/details_harness|hendrycksTest-high_school_world_history|5_2024-01-10T15-38-18.408039.parquet"]}]}, {"config_name": "harness_hendrycksTest_human_aging_5", "data_files": [{"split": "2024_01_10T15_38_18.408039", "path": ["**/details_harness|hendrycksTest-human_aging|5_2024-01-10T15-38-18.408039.parquet"]}, {"split": "latest", "path": ["**/details_harness|hendrycksTest-human_aging|5_2024-01-10T15-38-18.408039.parquet"]}]}, {"config_name": "harness_hendrycksTest_human_sexuality_5", "data_files": [{"split": "2024_01_10T15_38_18.408039", "path": ["**/details_harness|hendrycksTest-human_sexuality|5_2024-01-10T15-38-18.408039.parquet"]}, {"split": "latest", "path": ["**/details_harness|hendrycksTest-human_sexuality|5_2024-01-10T15-38-18.408039.parquet"]}]}, {"config_name": "harness_hendrycksTest_international_law_5", "data_files": [{"split": "2024_01_10T15_38_18.408039", "path": ["**/details_harness|hendrycksTest-international_law|5_2024-01-10T15-38-18.408039.parquet"]}, {"split": "latest", "path": ["**/details_harness|hendrycksTest-international_law|5_2024-01-10T15-38-18.408039.parquet"]}]}, {"config_name": "harness_hendrycksTest_jurisprudence_5", "data_files": [{"split": "2024_01_10T15_38_18.408039", "path": ["**/details_harness|hendrycksTest-jurisprudence|5_2024-01-10T15-38-18.408039.parquet"]}, {"split": "latest", "path": ["**/details_harness|hendrycksTest-jurisprudence|5_2024-01-10T15-38-18.408039.parquet"]}]}, {"config_name": "harness_hendrycksTest_logical_fallacies_5", "data_files": [{"split": "2024_01_10T15_38_18.408039", "path": ["**/details_harness|hendrycksTest-logical_fallacies|5_2024-01-10T15-38-18.408039.parquet"]}, {"split": "latest", "path": ["**/details_harness|hendrycksTest-logical_fallacies|5_2024-01-10T15-38-18.408039.parquet"]}]}, {"config_name": "harness_hendrycksTest_machine_learning_5", "data_files": [{"split": "2024_01_10T15_38_18.408039", "path": ["**/details_harness|hendrycksTest-machine_learning|5_2024-01-10T15-38-18.408039.parquet"]}, {"split": "latest", "path": ["**/details_harness|hendrycksTest-machine_learning|5_2024-01-10T15-38-18.408039.parquet"]}]}, {"config_name": "harness_hendrycksTest_management_5", "data_files": [{"split": "2024_01_10T15_38_18.408039", "path": ["**/details_harness|hendrycksTest-management|5_2024-01-10T15-38-18.408039.parquet"]}, {"split": "latest", "path": ["**/details_harness|hendrycksTest-management|5_2024-01-10T15-38-18.408039.parquet"]}]}, {"config_name": "harness_hendrycksTest_marketing_5", "data_files": [{"split": "2024_01_10T15_38_18.408039", "path": ["**/details_harness|hendrycksTest-marketing|5_2024-01-10T15-38-18.408039.parquet"]}, {"split": "latest", "path": ["**/details_harness|hendrycksTest-marketing|5_2024-01-10T15-38-18.408039.parquet"]}]}, {"config_name": "harness_hendrycksTest_medical_genetics_5", "data_files": [{"split": "2024_01_10T15_38_18.408039", "path": ["**/details_harness|hendrycksTest-medical_genetics|5_2024-01-10T15-38-18.408039.parquet"]}, {"split": "latest", "path": ["**/details_harness|hendrycksTest-medical_genetics|5_2024-01-10T15-38-18.408039.parquet"]}]}, {"config_name": "harness_hendrycksTest_miscellaneous_5", "data_files": [{"split": "2024_01_10T15_38_18.408039", "path": ["**/details_harness|hendrycksTest-miscellaneous|5_2024-01-10T15-38-18.408039.parquet"]}, {"split": "latest", "path": ["**/details_harness|hendrycksTest-miscellaneous|5_2024-01-10T15-38-18.408039.parquet"]}]}, {"config_name": "harness_hendrycksTest_moral_disputes_5", "data_files": [{"split": "2024_01_10T15_38_18.408039", "path": ["**/details_harness|hendrycksTest-moral_disputes|5_2024-01-10T15-38-18.408039.parquet"]}, {"split": "latest", "path": ["**/details_harness|hendrycksTest-moral_disputes|5_2024-01-10T15-38-18.408039.parquet"]}]}, {"config_name": "harness_hendrycksTest_moral_scenarios_5", "data_files": [{"split": "2024_01_10T15_38_18.408039", "path": ["**/details_harness|hendrycksTest-moral_scenarios|5_2024-01-10T15-38-18.408039.parquet"]}, {"split": "latest", "path": ["**/details_harness|hendrycksTest-moral_scenarios|5_2024-01-10T15-38-18.408039.parquet"]}]}, {"config_name": "harness_hendrycksTest_nutrition_5", "data_files": [{"split": "2024_01_10T15_38_18.408039", "path": ["**/details_harness|hendrycksTest-nutrition|5_2024-01-10T15-38-18.408039.parquet"]}, {"split": "latest", "path": ["**/details_harness|hendrycksTest-nutrition|5_2024-01-10T15-38-18.408039.parquet"]}]}, {"config_name": "harness_hendrycksTest_philosophy_5", "data_files": [{"split": "2024_01_10T15_38_18.408039", "path": ["**/details_harness|hendrycksTest-philosophy|5_2024-01-10T15-38-18.408039.parquet"]}, {"split": "latest", "path": ["**/details_harness|hendrycksTest-philosophy|5_2024-01-10T15-38-18.408039.parquet"]}]}, {"config_name": "harness_hendrycksTest_prehistory_5", "data_files": [{"split": "2024_01_10T15_38_18.408039", "path": ["**/details_harness|hendrycksTest-prehistory|5_2024-01-10T15-38-18.408039.parquet"]}, {"split": "latest", "path": ["**/details_harness|hendrycksTest-prehistory|5_2024-01-10T15-38-18.408039.parquet"]}]}, {"config_name": "harness_hendrycksTest_professional_accounting_5", "data_files": [{"split": "2024_01_10T15_38_18.408039", "path": ["**/details_harness|hendrycksTest-professional_accounting|5_2024-01-10T15-38-18.408039.parquet"]}, {"split": "latest", "path": ["**/details_harness|hendrycksTest-professional_accounting|5_2024-01-10T15-38-18.408039.parquet"]}]}, {"config_name": "harness_hendrycksTest_professional_law_5", "data_files": [{"split": "2024_01_10T15_38_18.408039", "path": ["**/details_harness|hendrycksTest-professional_law|5_2024-01-10T15-38-18.408039.parquet"]}, {"split": "latest", "path": ["**/details_harness|hendrycksTest-professional_law|5_2024-01-10T15-38-18.408039.parquet"]}]}, {"config_name": "harness_hendrycksTest_professional_medicine_5", "data_files": [{"split": "2024_01_10T15_38_18.408039", "path": ["**/details_harness|hendrycksTest-professional_medicine|5_2024-01-10T15-38-18.408039.parquet"]}, {"split": "latest", "path": ["**/details_harness|hendrycksTest-professional_medicine|5_2024-01-10T15-38-18.408039.parquet"]}]}, {"config_name": "harness_hendrycksTest_professional_psychology_5", "data_files": [{"split": "2024_01_10T15_38_18.408039", "path": ["**/details_harness|hendrycksTest-professional_psychology|5_2024-01-10T15-38-18.408039.parquet"]}, {"split": "latest", "path": ["**/details_harness|hendrycksTest-professional_psychology|5_2024-01-10T15-38-18.408039.parquet"]}]}, {"config_name": "harness_hendrycksTest_public_relations_5", "data_files": [{"split": "2024_01_10T15_38_18.408039", "path": ["**/details_harness|hendrycksTest-public_relations|5_2024-01-10T15-38-18.408039.parquet"]}, {"split": "latest", "path": ["**/details_harness|hendrycksTest-public_relations|5_2024-01-10T15-38-18.408039.parquet"]}]}, {"config_name": "harness_hendrycksTest_security_studies_5", "data_files": [{"split": "2024_01_10T15_38_18.408039", "path": ["**/details_harness|hendrycksTest-security_studies|5_2024-01-10T15-38-18.408039.parquet"]}, {"split": "latest", "path": ["**/details_harness|hendrycksTest-security_studies|5_2024-01-10T15-38-18.408039.parquet"]}]}, {"config_name": "harness_hendrycksTest_sociology_5", "data_files": [{"split": "2024_01_10T15_38_18.408039", "path": ["**/details_harness|hendrycksTest-sociology|5_2024-01-10T15-38-18.408039.parquet"]}, {"split": "latest", "path": ["**/details_harness|hendrycksTest-sociology|5_2024-01-10T15-38-18.408039.parquet"]}]}, {"config_name": "harness_hendrycksTest_us_foreign_policy_5", "data_files": [{"split": "2024_01_10T15_38_18.408039", "path": ["**/details_harness|hendrycksTest-us_foreign_policy|5_2024-01-10T15-38-18.408039.parquet"]}, {"split": "latest", "path": ["**/details_harness|hendrycksTest-us_foreign_policy|5_2024-01-10T15-38-18.408039.parquet"]}]}, {"config_name": "harness_hendrycksTest_virology_5", "data_files": [{"split": "2024_01_10T15_38_18.408039", "path": ["**/details_harness|hendrycksTest-virology|5_2024-01-10T15-38-18.408039.parquet"]}, {"split": "latest", "path": ["**/details_harness|hendrycksTest-virology|5_2024-01-10T15-38-18.408039.parquet"]}]}, {"config_name": "harness_hendrycksTest_world_religions_5", "data_files": [{"split": "2024_01_10T15_38_18.408039", "path": ["**/details_harness|hendrycksTest-world_religions|5_2024-01-10T15-38-18.408039.parquet"]}, {"split": "latest", "path": ["**/details_harness|hendrycksTest-world_religions|5_2024-01-10T15-38-18.408039.parquet"]}]}, {"config_name": "harness_truthfulqa_mc_0", "data_files": [{"split": "2024_01_10T15_38_18.408039", "path": ["**/details_harness|truthfulqa:mc|0_2024-01-10T15-38-18.408039.parquet"]}, {"split": "latest", "path": ["**/details_harness|truthfulqa:mc|0_2024-01-10T15-38-18.408039.parquet"]}]}, {"config_name": "harness_winogrande_5", "data_files": [{"split": "2024_01_10T15_38_18.408039", "path": ["**/details_harness|winogrande|5_2024-01-10T15-38-18.408039.parquet"]}, {"split": "latest", "path": ["**/details_harness|winogrande|5_2024-01-10T15-38-18.408039.parquet"]}]}, {"config_name": "results", "data_files": [{"split": "2024_01_10T15_38_18.408039", "path": ["results_2024-01-10T15-38-18.408039.parquet"]}, {"split": "latest", "path": ["results_2024-01-10T15-38-18.408039.parquet"]}]}]} | 2024-01-10T15:40:57+00:00 | [] | [] | TAGS
#region-us
|
# Dataset Card for Evaluation run of quantumaikr/quantum-v0.01
Dataset automatically created during the evaluation run of model quantumaikr/quantum-v0.01 on the Open LLM Leaderboard.
The dataset is composed of 63 configuration, each one coresponding to one of the evaluated task.
The dataset has been created from 1 run(s). Each run can be found as a specific split in each configuration, the split being named using the timestamp of the run.The "train" split is always pointing to the latest results.
An additional configuration "results" store all the aggregated results of the run (and is used to compute and display the aggregated metrics on the Open LLM Leaderboard).
To load the details from a run, you can for instance do the following:
## Latest results
These are the latest results from run 2024-01-10T15:38:18.408039(note that their might be results for other tasks in the repos if successive evals didn't cover the same tasks. You find each in the results and the "latest" split for each eval):
## Dataset Details
### Dataset Description
- Curated by:
- Funded by [optional]:
- Shared by [optional]:
- Language(s) (NLP):
- License:
### Dataset Sources [optional]
- Repository:
- Paper [optional]:
- Demo [optional]:
## Uses
### Direct Use
### Out-of-Scope Use
## Dataset Structure
## Dataset Creation
### Curation Rationale
### Source Data
#### Data Collection and Processing
#### Who are the source data producers?
### Annotations [optional]
#### Annotation process
#### Who are the annotators?
#### Personal and Sensitive Information
## Bias, Risks, and Limitations
### Recommendations
Users should be made aware of the risks, biases and limitations of the dataset. More information needed for further recommendations.
[optional]
BibTeX:
APA:
## Glossary [optional]
## More Information [optional]
## Dataset Card Authors [optional]
## Dataset Card Contact
| [
"# Dataset Card for Evaluation run of quantumaikr/quantum-v0.01\n\n\n\nDataset automatically created during the evaluation run of model quantumaikr/quantum-v0.01 on the Open LLM Leaderboard.\n\nThe dataset is composed of 63 configuration, each one coresponding to one of the evaluated task.\n\nThe dataset has been created from 1 run(s). Each run can be found as a specific split in each configuration, the split being named using the timestamp of the run.The \"train\" split is always pointing to the latest results.\n\nAn additional configuration \"results\" store all the aggregated results of the run (and is used to compute and display the aggregated metrics on the Open LLM Leaderboard).\n\nTo load the details from a run, you can for instance do the following:",
"## Latest results\n\nThese are the latest results from run 2024-01-10T15:38:18.408039(note that their might be results for other tasks in the repos if successive evals didn't cover the same tasks. You find each in the results and the \"latest\" split for each eval):",
"## Dataset Details",
"### Dataset Description\n\n\n\n\n\n- Curated by: \n- Funded by [optional]: \n- Shared by [optional]: \n- Language(s) (NLP): \n- License:",
"### Dataset Sources [optional]\n\n\n\n- Repository: \n- Paper [optional]: \n- Demo [optional]:",
"## Uses",
"### Direct Use",
"### Out-of-Scope Use",
"## Dataset Structure",
"## Dataset Creation",
"### Curation Rationale",
"### Source Data",
"#### Data Collection and Processing",
"#### Who are the source data producers?",
"### Annotations [optional]",
"#### Annotation process",
"#### Who are the annotators?",
"#### Personal and Sensitive Information",
"## Bias, Risks, and Limitations",
"### Recommendations\n\n\n\nUsers should be made aware of the risks, biases and limitations of the dataset. More information needed for further recommendations.\n\n[optional]\n\n\n\nBibTeX:\n\n\n\nAPA:",
"## Glossary [optional]",
"## More Information [optional]",
"## Dataset Card Authors [optional]",
"## Dataset Card Contact"
] | [
"TAGS\n#region-us \n",
"# Dataset Card for Evaluation run of quantumaikr/quantum-v0.01\n\n\n\nDataset automatically created during the evaluation run of model quantumaikr/quantum-v0.01 on the Open LLM Leaderboard.\n\nThe dataset is composed of 63 configuration, each one coresponding to one of the evaluated task.\n\nThe dataset has been created from 1 run(s). Each run can be found as a specific split in each configuration, the split being named using the timestamp of the run.The \"train\" split is always pointing to the latest results.\n\nAn additional configuration \"results\" store all the aggregated results of the run (and is used to compute and display the aggregated metrics on the Open LLM Leaderboard).\n\nTo load the details from a run, you can for instance do the following:",
"## Latest results\n\nThese are the latest results from run 2024-01-10T15:38:18.408039(note that their might be results for other tasks in the repos if successive evals didn't cover the same tasks. You find each in the results and the \"latest\" split for each eval):",
"## Dataset Details",
"### Dataset Description\n\n\n\n\n\n- Curated by: \n- Funded by [optional]: \n- Shared by [optional]: \n- Language(s) (NLP): \n- License:",
"### Dataset Sources [optional]\n\n\n\n- Repository: \n- Paper [optional]: \n- Demo [optional]:",
"## Uses",
"### Direct Use",
"### Out-of-Scope Use",
"## Dataset Structure",
"## Dataset Creation",
"### Curation Rationale",
"### Source Data",
"#### Data Collection and Processing",
"#### Who are the source data producers?",
"### Annotations [optional]",
"#### Annotation process",
"#### Who are the annotators?",
"#### Personal and Sensitive Information",
"## Bias, Risks, and Limitations",
"### Recommendations\n\n\n\nUsers should be made aware of the risks, biases and limitations of the dataset. More information needed for further recommendations.\n\n[optional]\n\n\n\nBibTeX:\n\n\n\nAPA:",
"## Glossary [optional]",
"## More Information [optional]",
"## Dataset Card Authors [optional]",
"## Dataset Card Contact"
] |
d99e2dadde49b2ae64c060837011b8f825e74911 |
# Dataset Card for the Alignement Internship Exercise
## Dataset Description
<!-- Provide a longer summary of what this dataset is. -->
This dataset provides a list of questions accompanied by Phi-2's best answer to them, as ranked by OpenAssitant's reward model.
## Dataset Creation
The questions were handpicked from the LDJnr/Capybara, Open-Orca/OpenOrca and truthful_qa datasets, the coding exercise is from LeetCode's top 100 liked questions and I found the last prompt on a blog and modified it. I have chosen these prompts specifically to evaluate the model on different domains of knowledge (STEM, coding, humanities), different tasks (reasoning, writing, summarization, question-answering), different levels of complexity, different lengths of prompts as well as its safety and alignment with human values and ability to defend itself against adversarial prompts.
Then each prompt was generated using the following logic:
"""\<USER>: You are a helpful, respectful and honest assistant. Always answer as helpfully as possible, while being safe. Your \
answers should not include any harmful, unethical, racist, sexist, toxic, dangerous, or illegal content. Please ensure\
that your responses are socially unbiased and positive in nature.
If a question does not make any sense, or is not factually coherent, explain why instead of answering something not \
correct. If you don't know the answer to a question, please don't share false information.
Here is my question: {question}
\<ASSISTANT>:"""
After that, for each question we generate K=8 answers with Phi-2 by setting the maximum number of new tokens to 300, stopping if the end of text token is generated, doing sampling, and setting the temperature to some predefined value.
We then rank each answer using OpenAssitant's reward model and take the best one.
Finally, we perform a small temperature hyperparameter scan and found that the best answers according to the reward model were given using a temperature value of 0.4. So these are the answers that are in the dataset.
## Dataset Sources
<!-- Provide the basic links for the dataset. -->
- **Capybara Dataset:** [link](https://huggingface.co/datasets/LDJnr/Capybara)
- **OpenOrca Dataset:** [link](https://huggingface.co/datasets/Open-Orca/OpenOrca)
- **Truthful QA Dataset:** [link](https://huggingface.co/datasets/truthful_qa)
- **LeetCode's "Subsets" problem:** [link](https://leetcode.com/problem-list/top-100-liked-questions/)
- **DAN prompt:** [link](https://www.promptingguide.ai/risks/adversarial)
- **Llama's system prompt:** [link](https://github.com/huggingface/transformers/blob/main/src/transformers/models/llama/tokenization_llama.py)
- **Micrososft's Phi-2:** [link](https://huggingface.co/microsoft/phi-2)
- **OpenAssistant's reward model:** [link](https://huggingface.co/OpenAssistant/reward-model-deberta-v3-large-v2)
| gsoisson/alignment-internship-exercise | [
"task_categories:question-answering",
"task_categories:text-generation",
"task_categories:conversational",
"size_categories:n<1K",
"language:en",
"license:apache-2.0",
"region:us"
] | 2024-01-10T15:41:04+00:00 | {"language": ["en"], "license": "apache-2.0", "size_categories": ["n<1K"], "task_categories": ["question-answering", "text-generation", "conversational"]} | 2024-01-10T17:36:13+00:00 | [] | [
"en"
] | TAGS
#task_categories-question-answering #task_categories-text-generation #task_categories-conversational #size_categories-n<1K #language-English #license-apache-2.0 #region-us
|
# Dataset Card for the Alignement Internship Exercise
## Dataset Description
This dataset provides a list of questions accompanied by Phi-2's best answer to them, as ranked by OpenAssitant's reward model.
## Dataset Creation
The questions were handpicked from the LDJnr/Capybara, Open-Orca/OpenOrca and truthful_qa datasets, the coding exercise is from LeetCode's top 100 liked questions and I found the last prompt on a blog and modified it. I have chosen these prompts specifically to evaluate the model on different domains of knowledge (STEM, coding, humanities), different tasks (reasoning, writing, summarization, question-answering), different levels of complexity, different lengths of prompts as well as its safety and alignment with human values and ability to defend itself against adversarial prompts.
Then each prompt was generated using the following logic:
"""\<USER>: You are a helpful, respectful and honest assistant. Always answer as helpfully as possible, while being safe. Your \
answers should not include any harmful, unethical, racist, sexist, toxic, dangerous, or illegal content. Please ensure\
that your responses are socially unbiased and positive in nature.
If a question does not make any sense, or is not factually coherent, explain why instead of answering something not \
correct. If you don't know the answer to a question, please don't share false information.
Here is my question: {question}
\<ASSISTANT>:"""
After that, for each question we generate K=8 answers with Phi-2 by setting the maximum number of new tokens to 300, stopping if the end of text token is generated, doing sampling, and setting the temperature to some predefined value.
We then rank each answer using OpenAssitant's reward model and take the best one.
Finally, we perform a small temperature hyperparameter scan and found that the best answers according to the reward model were given using a temperature value of 0.4. So these are the answers that are in the dataset.
## Dataset Sources
- Capybara Dataset: link
- OpenOrca Dataset: link
- Truthful QA Dataset: link
- LeetCode's "Subsets" problem: link
- DAN prompt: link
- Llama's system prompt: link
- Micrososft's Phi-2: link
- OpenAssistant's reward model: link
| [
"# Dataset Card for the Alignement Internship Exercise",
"## Dataset Description\n\n\n\nThis dataset provides a list of questions accompanied by Phi-2's best answer to them, as ranked by OpenAssitant's reward model.",
"## Dataset Creation\n\nThe questions were handpicked from the LDJnr/Capybara, Open-Orca/OpenOrca and truthful_qa datasets, the coding exercise is from LeetCode's top 100 liked questions and I found the last prompt on a blog and modified it. I have chosen these prompts specifically to evaluate the model on different domains of knowledge (STEM, coding, humanities), different tasks (reasoning, writing, summarization, question-answering), different levels of complexity, different lengths of prompts as well as its safety and alignment with human values and ability to defend itself against adversarial prompts.\n\n\nThen each prompt was generated using the following logic:\n\n\"\"\"\\<USER>: You are a helpful, respectful and honest assistant. Always answer as helpfully as possible, while being safe. Your \\\nanswers should not include any harmful, unethical, racist, sexist, toxic, dangerous, or illegal content. Please ensure\\\n that your responses are socially unbiased and positive in nature.\n\nIf a question does not make any sense, or is not factually coherent, explain why instead of answering something not \\\ncorrect. If you don't know the answer to a question, please don't share false information.\n\nHere is my question: {question}\n\n\\<ASSISTANT>:\"\"\"\n\n\nAfter that, for each question we generate K=8 answers with Phi-2 by setting the maximum number of new tokens to 300, stopping if the end of text token is generated, doing sampling, and setting the temperature to some predefined value.\n\nWe then rank each answer using OpenAssitant's reward model and take the best one.\n\nFinally, we perform a small temperature hyperparameter scan and found that the best answers according to the reward model were given using a temperature value of 0.4. So these are the answers that are in the dataset.",
"## Dataset Sources\n\n\n\n- Capybara Dataset: link\n- OpenOrca Dataset: link\n- Truthful QA Dataset: link\n- LeetCode's \"Subsets\" problem: link\n- DAN prompt: link\n- Llama's system prompt: link\n- Micrososft's Phi-2: link\n- OpenAssistant's reward model: link"
] | [
"TAGS\n#task_categories-question-answering #task_categories-text-generation #task_categories-conversational #size_categories-n<1K #language-English #license-apache-2.0 #region-us \n",
"# Dataset Card for the Alignement Internship Exercise",
"## Dataset Description\n\n\n\nThis dataset provides a list of questions accompanied by Phi-2's best answer to them, as ranked by OpenAssitant's reward model.",
"## Dataset Creation\n\nThe questions were handpicked from the LDJnr/Capybara, Open-Orca/OpenOrca and truthful_qa datasets, the coding exercise is from LeetCode's top 100 liked questions and I found the last prompt on a blog and modified it. I have chosen these prompts specifically to evaluate the model on different domains of knowledge (STEM, coding, humanities), different tasks (reasoning, writing, summarization, question-answering), different levels of complexity, different lengths of prompts as well as its safety and alignment with human values and ability to defend itself against adversarial prompts.\n\n\nThen each prompt was generated using the following logic:\n\n\"\"\"\\<USER>: You are a helpful, respectful and honest assistant. Always answer as helpfully as possible, while being safe. Your \\\nanswers should not include any harmful, unethical, racist, sexist, toxic, dangerous, or illegal content. Please ensure\\\n that your responses are socially unbiased and positive in nature.\n\nIf a question does not make any sense, or is not factually coherent, explain why instead of answering something not \\\ncorrect. If you don't know the answer to a question, please don't share false information.\n\nHere is my question: {question}\n\n\\<ASSISTANT>:\"\"\"\n\n\nAfter that, for each question we generate K=8 answers with Phi-2 by setting the maximum number of new tokens to 300, stopping if the end of text token is generated, doing sampling, and setting the temperature to some predefined value.\n\nWe then rank each answer using OpenAssitant's reward model and take the best one.\n\nFinally, we perform a small temperature hyperparameter scan and found that the best answers according to the reward model were given using a temperature value of 0.4. So these are the answers that are in the dataset.",
"## Dataset Sources\n\n\n\n- Capybara Dataset: link\n- OpenOrca Dataset: link\n- Truthful QA Dataset: link\n- LeetCode's \"Subsets\" problem: link\n- DAN prompt: link\n- Llama's system prompt: link\n- Micrososft's Phi-2: link\n- OpenAssistant's reward model: link"
] |
864bf8d91bac750f047062947088373d29e4bfb1 |
# Dataset Card for Evaluation run of cookinai/OpenCM-14
<!-- Provide a quick summary of the dataset. -->
Dataset automatically created during the evaluation run of model [cookinai/OpenCM-14](https://huggingface.co/cookinai/OpenCM-14) on the [Open LLM Leaderboard](https://huggingface.co/spaces/HuggingFaceH4/open_llm_leaderboard).
The dataset is composed of 63 configuration, each one coresponding to one of the evaluated task.
The dataset has been created from 1 run(s). Each run can be found as a specific split in each configuration, the split being named using the timestamp of the run.The "train" split is always pointing to the latest results.
An additional configuration "results" store all the aggregated results of the run (and is used to compute and display the aggregated metrics on the [Open LLM Leaderboard](https://huggingface.co/spaces/HuggingFaceH4/open_llm_leaderboard)).
To load the details from a run, you can for instance do the following:
```python
from datasets import load_dataset
data = load_dataset("open-llm-leaderboard/details_cookinai__OpenCM-14",
"harness_winogrande_5",
split="train")
```
## Latest results
These are the [latest results from run 2024-01-10T15:40:02.112197](https://huggingface.co/datasets/open-llm-leaderboard/details_cookinai__OpenCM-14/blob/main/results_2024-01-10T15-40-02.112197.json)(note that their might be results for other tasks in the repos if successive evals didn't cover the same tasks. You find each in the results and the "latest" split for each eval):
```python
{
"all": {
"acc": 0.6548270636916859,
"acc_stderr": 0.032081620223238315,
"acc_norm": 0.6545436968641555,
"acc_norm_stderr": 0.03274896423189078,
"mc1": 0.4430844553243574,
"mc1_stderr": 0.01738973034687711,
"mc2": 0.6107353876145338,
"mc2_stderr": 0.015128822743739728
},
"harness|arc:challenge|25": {
"acc": 0.6655290102389079,
"acc_stderr": 0.013787460322441372,
"acc_norm": 0.6928327645051194,
"acc_norm_stderr": 0.013481034054980943
},
"harness|hellaswag|10": {
"acc": 0.6802429794861581,
"acc_stderr": 0.0046542916612559064,
"acc_norm": 0.8688508265285799,
"acc_norm_stderr": 0.003368735434161384
},
"harness|hendrycksTest-abstract_algebra|5": {
"acc": 0.37,
"acc_stderr": 0.04852365870939099,
"acc_norm": 0.37,
"acc_norm_stderr": 0.04852365870939099
},
"harness|hendrycksTest-anatomy|5": {
"acc": 0.6370370370370371,
"acc_stderr": 0.041539484047423976,
"acc_norm": 0.6370370370370371,
"acc_norm_stderr": 0.041539484047423976
},
"harness|hendrycksTest-astronomy|5": {
"acc": 0.6907894736842105,
"acc_stderr": 0.037610708698674805,
"acc_norm": 0.6907894736842105,
"acc_norm_stderr": 0.037610708698674805
},
"harness|hendrycksTest-business_ethics|5": {
"acc": 0.64,
"acc_stderr": 0.04824181513244218,
"acc_norm": 0.64,
"acc_norm_stderr": 0.04824181513244218
},
"harness|hendrycksTest-clinical_knowledge|5": {
"acc": 0.7132075471698113,
"acc_stderr": 0.02783491252754406,
"acc_norm": 0.7132075471698113,
"acc_norm_stderr": 0.02783491252754406
},
"harness|hendrycksTest-college_biology|5": {
"acc": 0.7847222222222222,
"acc_stderr": 0.03437079344106135,
"acc_norm": 0.7847222222222222,
"acc_norm_stderr": 0.03437079344106135
},
"harness|hendrycksTest-college_chemistry|5": {
"acc": 0.46,
"acc_stderr": 0.05009082659620333,
"acc_norm": 0.46,
"acc_norm_stderr": 0.05009082659620333
},
"harness|hendrycksTest-college_computer_science|5": {
"acc": 0.56,
"acc_stderr": 0.04988876515698589,
"acc_norm": 0.56,
"acc_norm_stderr": 0.04988876515698589
},
"harness|hendrycksTest-college_mathematics|5": {
"acc": 0.34,
"acc_stderr": 0.04760952285695235,
"acc_norm": 0.34,
"acc_norm_stderr": 0.04760952285695235
},
"harness|hendrycksTest-college_medicine|5": {
"acc": 0.6763005780346821,
"acc_stderr": 0.035676037996391706,
"acc_norm": 0.6763005780346821,
"acc_norm_stderr": 0.035676037996391706
},
"harness|hendrycksTest-college_physics|5": {
"acc": 0.43137254901960786,
"acc_stderr": 0.04928099597287534,
"acc_norm": 0.43137254901960786,
"acc_norm_stderr": 0.04928099597287534
},
"harness|hendrycksTest-computer_security|5": {
"acc": 0.72,
"acc_stderr": 0.04512608598542127,
"acc_norm": 0.72,
"acc_norm_stderr": 0.04512608598542127
},
"harness|hendrycksTest-conceptual_physics|5": {
"acc": 0.5914893617021276,
"acc_stderr": 0.032134180267015755,
"acc_norm": 0.5914893617021276,
"acc_norm_stderr": 0.032134180267015755
},
"harness|hendrycksTest-econometrics|5": {
"acc": 0.47368421052631576,
"acc_stderr": 0.046970851366478626,
"acc_norm": 0.47368421052631576,
"acc_norm_stderr": 0.046970851366478626
},
"harness|hendrycksTest-electrical_engineering|5": {
"acc": 0.5517241379310345,
"acc_stderr": 0.04144311810878152,
"acc_norm": 0.5517241379310345,
"acc_norm_stderr": 0.04144311810878152
},
"harness|hendrycksTest-elementary_mathematics|5": {
"acc": 0.42592592592592593,
"acc_stderr": 0.025467149045469553,
"acc_norm": 0.42592592592592593,
"acc_norm_stderr": 0.025467149045469553
},
"harness|hendrycksTest-formal_logic|5": {
"acc": 0.4523809523809524,
"acc_stderr": 0.044518079590553275,
"acc_norm": 0.4523809523809524,
"acc_norm_stderr": 0.044518079590553275
},
"harness|hendrycksTest-global_facts|5": {
"acc": 0.35,
"acc_stderr": 0.047937248544110196,
"acc_norm": 0.35,
"acc_norm_stderr": 0.047937248544110196
},
"harness|hendrycksTest-high_school_biology|5": {
"acc": 0.7741935483870968,
"acc_stderr": 0.023785577884181015,
"acc_norm": 0.7741935483870968,
"acc_norm_stderr": 0.023785577884181015
},
"harness|hendrycksTest-high_school_chemistry|5": {
"acc": 0.4827586206896552,
"acc_stderr": 0.035158955511656986,
"acc_norm": 0.4827586206896552,
"acc_norm_stderr": 0.035158955511656986
},
"harness|hendrycksTest-high_school_computer_science|5": {
"acc": 0.7,
"acc_stderr": 0.046056618647183814,
"acc_norm": 0.7,
"acc_norm_stderr": 0.046056618647183814
},
"harness|hendrycksTest-high_school_european_history|5": {
"acc": 0.7818181818181819,
"acc_stderr": 0.03225078108306289,
"acc_norm": 0.7818181818181819,
"acc_norm_stderr": 0.03225078108306289
},
"harness|hendrycksTest-high_school_geography|5": {
"acc": 0.7929292929292929,
"acc_stderr": 0.028869778460267045,
"acc_norm": 0.7929292929292929,
"acc_norm_stderr": 0.028869778460267045
},
"harness|hendrycksTest-high_school_government_and_politics|5": {
"acc": 0.9015544041450777,
"acc_stderr": 0.021500249576033456,
"acc_norm": 0.9015544041450777,
"acc_norm_stderr": 0.021500249576033456
},
"harness|hendrycksTest-high_school_macroeconomics|5": {
"acc": 0.6717948717948717,
"acc_stderr": 0.023807633198657266,
"acc_norm": 0.6717948717948717,
"acc_norm_stderr": 0.023807633198657266
},
"harness|hendrycksTest-high_school_mathematics|5": {
"acc": 0.37407407407407406,
"acc_stderr": 0.02950286112895529,
"acc_norm": 0.37407407407407406,
"acc_norm_stderr": 0.02950286112895529
},
"harness|hendrycksTest-high_school_microeconomics|5": {
"acc": 0.6764705882352942,
"acc_stderr": 0.030388353551886793,
"acc_norm": 0.6764705882352942,
"acc_norm_stderr": 0.030388353551886793
},
"harness|hendrycksTest-high_school_physics|5": {
"acc": 0.3443708609271523,
"acc_stderr": 0.038796870240733264,
"acc_norm": 0.3443708609271523,
"acc_norm_stderr": 0.038796870240733264
},
"harness|hendrycksTest-high_school_psychology|5": {
"acc": 0.8422018348623853,
"acc_stderr": 0.01563002297009244,
"acc_norm": 0.8422018348623853,
"acc_norm_stderr": 0.01563002297009244
},
"harness|hendrycksTest-high_school_statistics|5": {
"acc": 0.5370370370370371,
"acc_stderr": 0.03400603625538271,
"acc_norm": 0.5370370370370371,
"acc_norm_stderr": 0.03400603625538271
},
"harness|hendrycksTest-high_school_us_history|5": {
"acc": 0.8333333333333334,
"acc_stderr": 0.026156867523931045,
"acc_norm": 0.8333333333333334,
"acc_norm_stderr": 0.026156867523931045
},
"harness|hendrycksTest-high_school_world_history|5": {
"acc": 0.8185654008438819,
"acc_stderr": 0.025085961144579654,
"acc_norm": 0.8185654008438819,
"acc_norm_stderr": 0.025085961144579654
},
"harness|hendrycksTest-human_aging|5": {
"acc": 0.6860986547085202,
"acc_stderr": 0.031146796482972465,
"acc_norm": 0.6860986547085202,
"acc_norm_stderr": 0.031146796482972465
},
"harness|hendrycksTest-human_sexuality|5": {
"acc": 0.7786259541984732,
"acc_stderr": 0.03641297081313729,
"acc_norm": 0.7786259541984732,
"acc_norm_stderr": 0.03641297081313729
},
"harness|hendrycksTest-international_law|5": {
"acc": 0.7851239669421488,
"acc_stderr": 0.03749492448709695,
"acc_norm": 0.7851239669421488,
"acc_norm_stderr": 0.03749492448709695
},
"harness|hendrycksTest-jurisprudence|5": {
"acc": 0.7870370370370371,
"acc_stderr": 0.0395783547198098,
"acc_norm": 0.7870370370370371,
"acc_norm_stderr": 0.0395783547198098
},
"harness|hendrycksTest-logical_fallacies|5": {
"acc": 0.7730061349693251,
"acc_stderr": 0.03291099578615769,
"acc_norm": 0.7730061349693251,
"acc_norm_stderr": 0.03291099578615769
},
"harness|hendrycksTest-machine_learning|5": {
"acc": 0.45535714285714285,
"acc_stderr": 0.047268355537191,
"acc_norm": 0.45535714285714285,
"acc_norm_stderr": 0.047268355537191
},
"harness|hendrycksTest-management|5": {
"acc": 0.7961165048543689,
"acc_stderr": 0.039891398595317706,
"acc_norm": 0.7961165048543689,
"acc_norm_stderr": 0.039891398595317706
},
"harness|hendrycksTest-marketing|5": {
"acc": 0.8803418803418803,
"acc_stderr": 0.021262719400406964,
"acc_norm": 0.8803418803418803,
"acc_norm_stderr": 0.021262719400406964
},
"harness|hendrycksTest-medical_genetics|5": {
"acc": 0.72,
"acc_stderr": 0.04512608598542128,
"acc_norm": 0.72,
"acc_norm_stderr": 0.04512608598542128
},
"harness|hendrycksTest-miscellaneous|5": {
"acc": 0.8365261813537676,
"acc_stderr": 0.013223928616741622,
"acc_norm": 0.8365261813537676,
"acc_norm_stderr": 0.013223928616741622
},
"harness|hendrycksTest-moral_disputes|5": {
"acc": 0.7369942196531792,
"acc_stderr": 0.023703099525258172,
"acc_norm": 0.7369942196531792,
"acc_norm_stderr": 0.023703099525258172
},
"harness|hendrycksTest-moral_scenarios|5": {
"acc": 0.4033519553072626,
"acc_stderr": 0.016407123032195246,
"acc_norm": 0.4033519553072626,
"acc_norm_stderr": 0.016407123032195246
},
"harness|hendrycksTest-nutrition|5": {
"acc": 0.7189542483660131,
"acc_stderr": 0.025738854797818737,
"acc_norm": 0.7189542483660131,
"acc_norm_stderr": 0.025738854797818737
},
"harness|hendrycksTest-philosophy|5": {
"acc": 0.7138263665594855,
"acc_stderr": 0.02567025924218893,
"acc_norm": 0.7138263665594855,
"acc_norm_stderr": 0.02567025924218893
},
"harness|hendrycksTest-prehistory|5": {
"acc": 0.7438271604938271,
"acc_stderr": 0.024288533637726095,
"acc_norm": 0.7438271604938271,
"acc_norm_stderr": 0.024288533637726095
},
"harness|hendrycksTest-professional_accounting|5": {
"acc": 0.4787234042553192,
"acc_stderr": 0.029800481645628693,
"acc_norm": 0.4787234042553192,
"acc_norm_stderr": 0.029800481645628693
},
"harness|hendrycksTest-professional_law|5": {
"acc": 0.46740547588005216,
"acc_stderr": 0.012743072942653345,
"acc_norm": 0.46740547588005216,
"acc_norm_stderr": 0.012743072942653345
},
"harness|hendrycksTest-professional_medicine|5": {
"acc": 0.6875,
"acc_stderr": 0.02815637344037142,
"acc_norm": 0.6875,
"acc_norm_stderr": 0.02815637344037142
},
"harness|hendrycksTest-professional_psychology|5": {
"acc": 0.6862745098039216,
"acc_stderr": 0.018771683893528183,
"acc_norm": 0.6862745098039216,
"acc_norm_stderr": 0.018771683893528183
},
"harness|hendrycksTest-public_relations|5": {
"acc": 0.6818181818181818,
"acc_stderr": 0.04461272175910509,
"acc_norm": 0.6818181818181818,
"acc_norm_stderr": 0.04461272175910509
},
"harness|hendrycksTest-security_studies|5": {
"acc": 0.726530612244898,
"acc_stderr": 0.02853556033712844,
"acc_norm": 0.726530612244898,
"acc_norm_stderr": 0.02853556033712844
},
"harness|hendrycksTest-sociology|5": {
"acc": 0.8606965174129353,
"acc_stderr": 0.024484487162913973,
"acc_norm": 0.8606965174129353,
"acc_norm_stderr": 0.024484487162913973
},
"harness|hendrycksTest-us_foreign_policy|5": {
"acc": 0.85,
"acc_stderr": 0.0358870281282637,
"acc_norm": 0.85,
"acc_norm_stderr": 0.0358870281282637
},
"harness|hendrycksTest-virology|5": {
"acc": 0.5662650602409639,
"acc_stderr": 0.03858158940685516,
"acc_norm": 0.5662650602409639,
"acc_norm_stderr": 0.03858158940685516
},
"harness|hendrycksTest-world_religions|5": {
"acc": 0.8362573099415205,
"acc_stderr": 0.028380919596145866,
"acc_norm": 0.8362573099415205,
"acc_norm_stderr": 0.028380919596145866
},
"harness|truthfulqa:mc|0": {
"mc1": 0.4430844553243574,
"mc1_stderr": 0.01738973034687711,
"mc2": 0.6107353876145338,
"mc2_stderr": 0.015128822743739728
},
"harness|winogrande|5": {
"acc": 0.8129439621152328,
"acc_stderr": 0.010959716435242912
},
"harness|gsm8k|5": {
"acc": 0.7293404094010614,
"acc_stderr": 0.012238245006183408
}
}
```
## Dataset Details
### Dataset Description
<!-- Provide a longer summary of what this dataset is. -->
- **Curated by:** [More Information Needed]
- **Funded by [optional]:** [More Information Needed]
- **Shared by [optional]:** [More Information Needed]
- **Language(s) (NLP):** [More Information Needed]
- **License:** [More Information Needed]
### Dataset Sources [optional]
<!-- Provide the basic links for the dataset. -->
- **Repository:** [More Information Needed]
- **Paper [optional]:** [More Information Needed]
- **Demo [optional]:** [More Information Needed]
## Uses
<!-- Address questions around how the dataset is intended to be used. -->
### Direct Use
<!-- This section describes suitable use cases for the dataset. -->
[More Information Needed]
### Out-of-Scope Use
<!-- This section addresses misuse, malicious use, and uses that the dataset will not work well for. -->
[More Information Needed]
## Dataset Structure
<!-- This section provides a description of the dataset fields, and additional information about the dataset structure such as criteria used to create the splits, relationships between data points, etc. -->
[More Information Needed]
## Dataset Creation
### Curation Rationale
<!-- Motivation for the creation of this dataset. -->
[More Information Needed]
### Source Data
<!-- This section describes the source data (e.g. news text and headlines, social media posts, translated sentences, ...). -->
#### Data Collection and Processing
<!-- This section describes the data collection and processing process such as data selection criteria, filtering and normalization methods, tools and libraries used, etc. -->
[More Information Needed]
#### Who are the source data producers?
<!-- This section describes the people or systems who originally created the data. It should also include self-reported demographic or identity information for the source data creators if this information is available. -->
[More Information Needed]
### Annotations [optional]
<!-- If the dataset contains annotations which are not part of the initial data collection, use this section to describe them. -->
#### Annotation process
<!-- This section describes the annotation process such as annotation tools used in the process, the amount of data annotated, annotation guidelines provided to the annotators, interannotator statistics, annotation validation, etc. -->
[More Information Needed]
#### Who are the annotators?
<!-- This section describes the people or systems who created the annotations. -->
[More Information Needed]
#### Personal and Sensitive Information
<!-- State whether the dataset contains data that might be considered personal, sensitive, or private (e.g., data that reveals addresses, uniquely identifiable names or aliases, racial or ethnic origins, sexual orientations, religious beliefs, political opinions, financial or health data, etc.). If efforts were made to anonymize the data, describe the anonymization process. -->
[More Information Needed]
## Bias, Risks, and Limitations
<!-- This section is meant to convey both technical and sociotechnical limitations. -->
[More Information Needed]
### Recommendations
<!-- This section is meant to convey recommendations with respect to the bias, risk, and technical limitations. -->
Users should be made aware of the risks, biases and limitations of the dataset. More information needed for further recommendations.
## Citation [optional]
<!-- If there is a paper or blog post introducing the dataset, the APA and Bibtex information for that should go in this section. -->
**BibTeX:**
[More Information Needed]
**APA:**
[More Information Needed]
## Glossary [optional]
<!-- If relevant, include terms and calculations in this section that can help readers understand the dataset or dataset card. -->
[More Information Needed]
## More Information [optional]
[More Information Needed]
## Dataset Card Authors [optional]
[More Information Needed]
## Dataset Card Contact
[More Information Needed] | open-llm-leaderboard/details_cookinai__OpenCM-14 | [
"region:us"
] | 2024-01-10T15:42:23+00:00 | {"pretty_name": "Evaluation run of cookinai/OpenCM-14", "dataset_summary": "Dataset automatically created during the evaluation run of model [cookinai/OpenCM-14](https://huggingface.co/cookinai/OpenCM-14) on the [Open LLM Leaderboard](https://huggingface.co/spaces/HuggingFaceH4/open_llm_leaderboard).\n\nThe dataset is composed of 63 configuration, each one coresponding to one of the evaluated task.\n\nThe dataset has been created from 1 run(s). Each run can be found as a specific split in each configuration, the split being named using the timestamp of the run.The \"train\" split is always pointing to the latest results.\n\nAn additional configuration \"results\" store all the aggregated results of the run (and is used to compute and display the aggregated metrics on the [Open LLM Leaderboard](https://huggingface.co/spaces/HuggingFaceH4/open_llm_leaderboard)).\n\nTo load the details from a run, you can for instance do the following:\n```python\nfrom datasets import load_dataset\ndata = load_dataset(\"open-llm-leaderboard/details_cookinai__OpenCM-14\",\n\t\"harness_winogrande_5\",\n\tsplit=\"train\")\n```\n\n## Latest results\n\nThese are the [latest results from run 2024-01-10T15:40:02.112197](https://huggingface.co/datasets/open-llm-leaderboard/details_cookinai__OpenCM-14/blob/main/results_2024-01-10T15-40-02.112197.json)(note that their might be results for other tasks in the repos if successive evals didn't cover the same tasks. You find each in the results and the \"latest\" split for each eval):\n\n```python\n{\n \"all\": {\n \"acc\": 0.6548270636916859,\n \"acc_stderr\": 0.032081620223238315,\n \"acc_norm\": 0.6545436968641555,\n \"acc_norm_stderr\": 0.03274896423189078,\n \"mc1\": 0.4430844553243574,\n \"mc1_stderr\": 0.01738973034687711,\n \"mc2\": 0.6107353876145338,\n \"mc2_stderr\": 0.015128822743739728\n },\n \"harness|arc:challenge|25\": {\n \"acc\": 0.6655290102389079,\n \"acc_stderr\": 0.013787460322441372,\n \"acc_norm\": 0.6928327645051194,\n \"acc_norm_stderr\": 0.013481034054980943\n },\n \"harness|hellaswag|10\": {\n \"acc\": 0.6802429794861581,\n \"acc_stderr\": 0.0046542916612559064,\n \"acc_norm\": 0.8688508265285799,\n \"acc_norm_stderr\": 0.003368735434161384\n },\n \"harness|hendrycksTest-abstract_algebra|5\": {\n \"acc\": 0.37,\n \"acc_stderr\": 0.04852365870939099,\n \"acc_norm\": 0.37,\n \"acc_norm_stderr\": 0.04852365870939099\n },\n \"harness|hendrycksTest-anatomy|5\": {\n \"acc\": 0.6370370370370371,\n \"acc_stderr\": 0.041539484047423976,\n \"acc_norm\": 0.6370370370370371,\n \"acc_norm_stderr\": 0.041539484047423976\n },\n \"harness|hendrycksTest-astronomy|5\": {\n \"acc\": 0.6907894736842105,\n \"acc_stderr\": 0.037610708698674805,\n \"acc_norm\": 0.6907894736842105,\n \"acc_norm_stderr\": 0.037610708698674805\n },\n \"harness|hendrycksTest-business_ethics|5\": {\n \"acc\": 0.64,\n \"acc_stderr\": 0.04824181513244218,\n \"acc_norm\": 0.64,\n \"acc_norm_stderr\": 0.04824181513244218\n },\n \"harness|hendrycksTest-clinical_knowledge|5\": {\n \"acc\": 0.7132075471698113,\n \"acc_stderr\": 0.02783491252754406,\n \"acc_norm\": 0.7132075471698113,\n \"acc_norm_stderr\": 0.02783491252754406\n },\n \"harness|hendrycksTest-college_biology|5\": {\n \"acc\": 0.7847222222222222,\n \"acc_stderr\": 0.03437079344106135,\n \"acc_norm\": 0.7847222222222222,\n \"acc_norm_stderr\": 0.03437079344106135\n },\n \"harness|hendrycksTest-college_chemistry|5\": {\n \"acc\": 0.46,\n \"acc_stderr\": 0.05009082659620333,\n \"acc_norm\": 0.46,\n \"acc_norm_stderr\": 0.05009082659620333\n },\n \"harness|hendrycksTest-college_computer_science|5\": {\n \"acc\": 0.56,\n \"acc_stderr\": 0.04988876515698589,\n \"acc_norm\": 0.56,\n \"acc_norm_stderr\": 0.04988876515698589\n },\n \"harness|hendrycksTest-college_mathematics|5\": {\n \"acc\": 0.34,\n \"acc_stderr\": 0.04760952285695235,\n \"acc_norm\": 0.34,\n \"acc_norm_stderr\": 0.04760952285695235\n },\n \"harness|hendrycksTest-college_medicine|5\": {\n \"acc\": 0.6763005780346821,\n \"acc_stderr\": 0.035676037996391706,\n \"acc_norm\": 0.6763005780346821,\n \"acc_norm_stderr\": 0.035676037996391706\n },\n \"harness|hendrycksTest-college_physics|5\": {\n \"acc\": 0.43137254901960786,\n \"acc_stderr\": 0.04928099597287534,\n \"acc_norm\": 0.43137254901960786,\n \"acc_norm_stderr\": 0.04928099597287534\n },\n \"harness|hendrycksTest-computer_security|5\": {\n \"acc\": 0.72,\n \"acc_stderr\": 0.04512608598542127,\n \"acc_norm\": 0.72,\n \"acc_norm_stderr\": 0.04512608598542127\n },\n \"harness|hendrycksTest-conceptual_physics|5\": {\n \"acc\": 0.5914893617021276,\n \"acc_stderr\": 0.032134180267015755,\n \"acc_norm\": 0.5914893617021276,\n \"acc_norm_stderr\": 0.032134180267015755\n },\n \"harness|hendrycksTest-econometrics|5\": {\n \"acc\": 0.47368421052631576,\n \"acc_stderr\": 0.046970851366478626,\n \"acc_norm\": 0.47368421052631576,\n \"acc_norm_stderr\": 0.046970851366478626\n },\n \"harness|hendrycksTest-electrical_engineering|5\": {\n \"acc\": 0.5517241379310345,\n \"acc_stderr\": 0.04144311810878152,\n \"acc_norm\": 0.5517241379310345,\n \"acc_norm_stderr\": 0.04144311810878152\n },\n \"harness|hendrycksTest-elementary_mathematics|5\": {\n \"acc\": 0.42592592592592593,\n \"acc_stderr\": 0.025467149045469553,\n \"acc_norm\": 0.42592592592592593,\n \"acc_norm_stderr\": 0.025467149045469553\n },\n \"harness|hendrycksTest-formal_logic|5\": {\n \"acc\": 0.4523809523809524,\n \"acc_stderr\": 0.044518079590553275,\n \"acc_norm\": 0.4523809523809524,\n \"acc_norm_stderr\": 0.044518079590553275\n },\n \"harness|hendrycksTest-global_facts|5\": {\n \"acc\": 0.35,\n \"acc_stderr\": 0.047937248544110196,\n \"acc_norm\": 0.35,\n \"acc_norm_stderr\": 0.047937248544110196\n },\n \"harness|hendrycksTest-high_school_biology|5\": {\n \"acc\": 0.7741935483870968,\n \"acc_stderr\": 0.023785577884181015,\n \"acc_norm\": 0.7741935483870968,\n \"acc_norm_stderr\": 0.023785577884181015\n },\n \"harness|hendrycksTest-high_school_chemistry|5\": {\n \"acc\": 0.4827586206896552,\n \"acc_stderr\": 0.035158955511656986,\n \"acc_norm\": 0.4827586206896552,\n \"acc_norm_stderr\": 0.035158955511656986\n },\n \"harness|hendrycksTest-high_school_computer_science|5\": {\n \"acc\": 0.7,\n \"acc_stderr\": 0.046056618647183814,\n \"acc_norm\": 0.7,\n \"acc_norm_stderr\": 0.046056618647183814\n },\n \"harness|hendrycksTest-high_school_european_history|5\": {\n \"acc\": 0.7818181818181819,\n \"acc_stderr\": 0.03225078108306289,\n \"acc_norm\": 0.7818181818181819,\n \"acc_norm_stderr\": 0.03225078108306289\n },\n \"harness|hendrycksTest-high_school_geography|5\": {\n \"acc\": 0.7929292929292929,\n \"acc_stderr\": 0.028869778460267045,\n \"acc_norm\": 0.7929292929292929,\n \"acc_norm_stderr\": 0.028869778460267045\n },\n \"harness|hendrycksTest-high_school_government_and_politics|5\": {\n \"acc\": 0.9015544041450777,\n \"acc_stderr\": 0.021500249576033456,\n \"acc_norm\": 0.9015544041450777,\n \"acc_norm_stderr\": 0.021500249576033456\n },\n \"harness|hendrycksTest-high_school_macroeconomics|5\": {\n \"acc\": 0.6717948717948717,\n \"acc_stderr\": 0.023807633198657266,\n \"acc_norm\": 0.6717948717948717,\n \"acc_norm_stderr\": 0.023807633198657266\n },\n \"harness|hendrycksTest-high_school_mathematics|5\": {\n \"acc\": 0.37407407407407406,\n \"acc_stderr\": 0.02950286112895529,\n \"acc_norm\": 0.37407407407407406,\n \"acc_norm_stderr\": 0.02950286112895529\n },\n \"harness|hendrycksTest-high_school_microeconomics|5\": {\n \"acc\": 0.6764705882352942,\n \"acc_stderr\": 0.030388353551886793,\n \"acc_norm\": 0.6764705882352942,\n \"acc_norm_stderr\": 0.030388353551886793\n },\n \"harness|hendrycksTest-high_school_physics|5\": {\n \"acc\": 0.3443708609271523,\n \"acc_stderr\": 0.038796870240733264,\n \"acc_norm\": 0.3443708609271523,\n \"acc_norm_stderr\": 0.038796870240733264\n },\n \"harness|hendrycksTest-high_school_psychology|5\": {\n \"acc\": 0.8422018348623853,\n \"acc_stderr\": 0.01563002297009244,\n \"acc_norm\": 0.8422018348623853,\n \"acc_norm_stderr\": 0.01563002297009244\n },\n \"harness|hendrycksTest-high_school_statistics|5\": {\n \"acc\": 0.5370370370370371,\n \"acc_stderr\": 0.03400603625538271,\n \"acc_norm\": 0.5370370370370371,\n \"acc_norm_stderr\": 0.03400603625538271\n },\n \"harness|hendrycksTest-high_school_us_history|5\": {\n \"acc\": 0.8333333333333334,\n \"acc_stderr\": 0.026156867523931045,\n \"acc_norm\": 0.8333333333333334,\n \"acc_norm_stderr\": 0.026156867523931045\n },\n \"harness|hendrycksTest-high_school_world_history|5\": {\n \"acc\": 0.8185654008438819,\n \"acc_stderr\": 0.025085961144579654,\n \"acc_norm\": 0.8185654008438819,\n \"acc_norm_stderr\": 0.025085961144579654\n },\n \"harness|hendrycksTest-human_aging|5\": {\n \"acc\": 0.6860986547085202,\n \"acc_stderr\": 0.031146796482972465,\n \"acc_norm\": 0.6860986547085202,\n \"acc_norm_stderr\": 0.031146796482972465\n },\n \"harness|hendrycksTest-human_sexuality|5\": {\n \"acc\": 0.7786259541984732,\n \"acc_stderr\": 0.03641297081313729,\n \"acc_norm\": 0.7786259541984732,\n \"acc_norm_stderr\": 0.03641297081313729\n },\n \"harness|hendrycksTest-international_law|5\": {\n \"acc\": 0.7851239669421488,\n \"acc_stderr\": 0.03749492448709695,\n \"acc_norm\": 0.7851239669421488,\n \"acc_norm_stderr\": 0.03749492448709695\n },\n \"harness|hendrycksTest-jurisprudence|5\": {\n \"acc\": 0.7870370370370371,\n \"acc_stderr\": 0.0395783547198098,\n \"acc_norm\": 0.7870370370370371,\n \"acc_norm_stderr\": 0.0395783547198098\n },\n \"harness|hendrycksTest-logical_fallacies|5\": {\n \"acc\": 0.7730061349693251,\n \"acc_stderr\": 0.03291099578615769,\n \"acc_norm\": 0.7730061349693251,\n \"acc_norm_stderr\": 0.03291099578615769\n },\n \"harness|hendrycksTest-machine_learning|5\": {\n \"acc\": 0.45535714285714285,\n \"acc_stderr\": 0.047268355537191,\n \"acc_norm\": 0.45535714285714285,\n \"acc_norm_stderr\": 0.047268355537191\n },\n \"harness|hendrycksTest-management|5\": {\n \"acc\": 0.7961165048543689,\n \"acc_stderr\": 0.039891398595317706,\n \"acc_norm\": 0.7961165048543689,\n \"acc_norm_stderr\": 0.039891398595317706\n },\n \"harness|hendrycksTest-marketing|5\": {\n \"acc\": 0.8803418803418803,\n \"acc_stderr\": 0.021262719400406964,\n \"acc_norm\": 0.8803418803418803,\n \"acc_norm_stderr\": 0.021262719400406964\n },\n \"harness|hendrycksTest-medical_genetics|5\": {\n \"acc\": 0.72,\n \"acc_stderr\": 0.04512608598542128,\n \"acc_norm\": 0.72,\n \"acc_norm_stderr\": 0.04512608598542128\n },\n \"harness|hendrycksTest-miscellaneous|5\": {\n \"acc\": 0.8365261813537676,\n \"acc_stderr\": 0.013223928616741622,\n \"acc_norm\": 0.8365261813537676,\n \"acc_norm_stderr\": 0.013223928616741622\n },\n \"harness|hendrycksTest-moral_disputes|5\": {\n \"acc\": 0.7369942196531792,\n \"acc_stderr\": 0.023703099525258172,\n \"acc_norm\": 0.7369942196531792,\n \"acc_norm_stderr\": 0.023703099525258172\n },\n \"harness|hendrycksTest-moral_scenarios|5\": {\n \"acc\": 0.4033519553072626,\n \"acc_stderr\": 0.016407123032195246,\n \"acc_norm\": 0.4033519553072626,\n \"acc_norm_stderr\": 0.016407123032195246\n },\n \"harness|hendrycksTest-nutrition|5\": {\n \"acc\": 0.7189542483660131,\n \"acc_stderr\": 0.025738854797818737,\n \"acc_norm\": 0.7189542483660131,\n \"acc_norm_stderr\": 0.025738854797818737\n },\n \"harness|hendrycksTest-philosophy|5\": {\n \"acc\": 0.7138263665594855,\n \"acc_stderr\": 0.02567025924218893,\n \"acc_norm\": 0.7138263665594855,\n \"acc_norm_stderr\": 0.02567025924218893\n },\n \"harness|hendrycksTest-prehistory|5\": {\n \"acc\": 0.7438271604938271,\n \"acc_stderr\": 0.024288533637726095,\n \"acc_norm\": 0.7438271604938271,\n \"acc_norm_stderr\": 0.024288533637726095\n },\n \"harness|hendrycksTest-professional_accounting|5\": {\n \"acc\": 0.4787234042553192,\n \"acc_stderr\": 0.029800481645628693,\n \"acc_norm\": 0.4787234042553192,\n \"acc_norm_stderr\": 0.029800481645628693\n },\n \"harness|hendrycksTest-professional_law|5\": {\n \"acc\": 0.46740547588005216,\n \"acc_stderr\": 0.012743072942653345,\n \"acc_norm\": 0.46740547588005216,\n \"acc_norm_stderr\": 0.012743072942653345\n },\n \"harness|hendrycksTest-professional_medicine|5\": {\n \"acc\": 0.6875,\n \"acc_stderr\": 0.02815637344037142,\n \"acc_norm\": 0.6875,\n \"acc_norm_stderr\": 0.02815637344037142\n },\n \"harness|hendrycksTest-professional_psychology|5\": {\n \"acc\": 0.6862745098039216,\n \"acc_stderr\": 0.018771683893528183,\n \"acc_norm\": 0.6862745098039216,\n \"acc_norm_stderr\": 0.018771683893528183\n },\n \"harness|hendrycksTest-public_relations|5\": {\n \"acc\": 0.6818181818181818,\n \"acc_stderr\": 0.04461272175910509,\n \"acc_norm\": 0.6818181818181818,\n \"acc_norm_stderr\": 0.04461272175910509\n },\n \"harness|hendrycksTest-security_studies|5\": {\n \"acc\": 0.726530612244898,\n \"acc_stderr\": 0.02853556033712844,\n \"acc_norm\": 0.726530612244898,\n \"acc_norm_stderr\": 0.02853556033712844\n },\n \"harness|hendrycksTest-sociology|5\": {\n \"acc\": 0.8606965174129353,\n \"acc_stderr\": 0.024484487162913973,\n \"acc_norm\": 0.8606965174129353,\n \"acc_norm_stderr\": 0.024484487162913973\n },\n \"harness|hendrycksTest-us_foreign_policy|5\": {\n \"acc\": 0.85,\n \"acc_stderr\": 0.0358870281282637,\n \"acc_norm\": 0.85,\n \"acc_norm_stderr\": 0.0358870281282637\n },\n \"harness|hendrycksTest-virology|5\": {\n \"acc\": 0.5662650602409639,\n \"acc_stderr\": 0.03858158940685516,\n \"acc_norm\": 0.5662650602409639,\n \"acc_norm_stderr\": 0.03858158940685516\n },\n \"harness|hendrycksTest-world_religions|5\": {\n \"acc\": 0.8362573099415205,\n \"acc_stderr\": 0.028380919596145866,\n \"acc_norm\": 0.8362573099415205,\n \"acc_norm_stderr\": 0.028380919596145866\n },\n \"harness|truthfulqa:mc|0\": {\n \"mc1\": 0.4430844553243574,\n \"mc1_stderr\": 0.01738973034687711,\n \"mc2\": 0.6107353876145338,\n \"mc2_stderr\": 0.015128822743739728\n },\n \"harness|winogrande|5\": {\n \"acc\": 0.8129439621152328,\n \"acc_stderr\": 0.010959716435242912\n },\n \"harness|gsm8k|5\": {\n \"acc\": 0.7293404094010614,\n \"acc_stderr\": 0.012238245006183408\n }\n}\n```", "repo_url": "https://huggingface.co/cookinai/OpenCM-14", "leaderboard_url": "https://huggingface.co/spaces/HuggingFaceH4/open_llm_leaderboard", "point_of_contact": "[email protected]", "configs": [{"config_name": "harness_arc_challenge_25", "data_files": [{"split": "2024_01_10T15_40_02.112197", "path": ["**/details_harness|arc:challenge|25_2024-01-10T15-40-02.112197.parquet"]}, {"split": "latest", "path": ["**/details_harness|arc:challenge|25_2024-01-10T15-40-02.112197.parquet"]}]}, {"config_name": "harness_gsm8k_5", "data_files": [{"split": "2024_01_10T15_40_02.112197", "path": ["**/details_harness|gsm8k|5_2024-01-10T15-40-02.112197.parquet"]}, {"split": "latest", "path": ["**/details_harness|gsm8k|5_2024-01-10T15-40-02.112197.parquet"]}]}, {"config_name": "harness_hellaswag_10", "data_files": [{"split": "2024_01_10T15_40_02.112197", "path": ["**/details_harness|hellaswag|10_2024-01-10T15-40-02.112197.parquet"]}, {"split": "latest", "path": ["**/details_harness|hellaswag|10_2024-01-10T15-40-02.112197.parquet"]}]}, {"config_name": "harness_hendrycksTest_5", "data_files": [{"split": "2024_01_10T15_40_02.112197", "path": ["**/details_harness|hendrycksTest-abstract_algebra|5_2024-01-10T15-40-02.112197.parquet", "**/details_harness|hendrycksTest-anatomy|5_2024-01-10T15-40-02.112197.parquet", "**/details_harness|hendrycksTest-astronomy|5_2024-01-10T15-40-02.112197.parquet", "**/details_harness|hendrycksTest-business_ethics|5_2024-01-10T15-40-02.112197.parquet", "**/details_harness|hendrycksTest-clinical_knowledge|5_2024-01-10T15-40-02.112197.parquet", "**/details_harness|hendrycksTest-college_biology|5_2024-01-10T15-40-02.112197.parquet", "**/details_harness|hendrycksTest-college_chemistry|5_2024-01-10T15-40-02.112197.parquet", "**/details_harness|hendrycksTest-college_computer_science|5_2024-01-10T15-40-02.112197.parquet", "**/details_harness|hendrycksTest-college_mathematics|5_2024-01-10T15-40-02.112197.parquet", "**/details_harness|hendrycksTest-college_medicine|5_2024-01-10T15-40-02.112197.parquet", "**/details_harness|hendrycksTest-college_physics|5_2024-01-10T15-40-02.112197.parquet", "**/details_harness|hendrycksTest-computer_security|5_2024-01-10T15-40-02.112197.parquet", "**/details_harness|hendrycksTest-conceptual_physics|5_2024-01-10T15-40-02.112197.parquet", "**/details_harness|hendrycksTest-econometrics|5_2024-01-10T15-40-02.112197.parquet", "**/details_harness|hendrycksTest-electrical_engineering|5_2024-01-10T15-40-02.112197.parquet", "**/details_harness|hendrycksTest-elementary_mathematics|5_2024-01-10T15-40-02.112197.parquet", "**/details_harness|hendrycksTest-formal_logic|5_2024-01-10T15-40-02.112197.parquet", "**/details_harness|hendrycksTest-global_facts|5_2024-01-10T15-40-02.112197.parquet", "**/details_harness|hendrycksTest-high_school_biology|5_2024-01-10T15-40-02.112197.parquet", "**/details_harness|hendrycksTest-high_school_chemistry|5_2024-01-10T15-40-02.112197.parquet", "**/details_harness|hendrycksTest-high_school_computer_science|5_2024-01-10T15-40-02.112197.parquet", "**/details_harness|hendrycksTest-high_school_european_history|5_2024-01-10T15-40-02.112197.parquet", "**/details_harness|hendrycksTest-high_school_geography|5_2024-01-10T15-40-02.112197.parquet", "**/details_harness|hendrycksTest-high_school_government_and_politics|5_2024-01-10T15-40-02.112197.parquet", "**/details_harness|hendrycksTest-high_school_macroeconomics|5_2024-01-10T15-40-02.112197.parquet", "**/details_harness|hendrycksTest-high_school_mathematics|5_2024-01-10T15-40-02.112197.parquet", "**/details_harness|hendrycksTest-high_school_microeconomics|5_2024-01-10T15-40-02.112197.parquet", "**/details_harness|hendrycksTest-high_school_physics|5_2024-01-10T15-40-02.112197.parquet", "**/details_harness|hendrycksTest-high_school_psychology|5_2024-01-10T15-40-02.112197.parquet", "**/details_harness|hendrycksTest-high_school_statistics|5_2024-01-10T15-40-02.112197.parquet", "**/details_harness|hendrycksTest-high_school_us_history|5_2024-01-10T15-40-02.112197.parquet", "**/details_harness|hendrycksTest-high_school_world_history|5_2024-01-10T15-40-02.112197.parquet", "**/details_harness|hendrycksTest-human_aging|5_2024-01-10T15-40-02.112197.parquet", "**/details_harness|hendrycksTest-human_sexuality|5_2024-01-10T15-40-02.112197.parquet", "**/details_harness|hendrycksTest-international_law|5_2024-01-10T15-40-02.112197.parquet", "**/details_harness|hendrycksTest-jurisprudence|5_2024-01-10T15-40-02.112197.parquet", "**/details_harness|hendrycksTest-logical_fallacies|5_2024-01-10T15-40-02.112197.parquet", "**/details_harness|hendrycksTest-machine_learning|5_2024-01-10T15-40-02.112197.parquet", "**/details_harness|hendrycksTest-management|5_2024-01-10T15-40-02.112197.parquet", "**/details_harness|hendrycksTest-marketing|5_2024-01-10T15-40-02.112197.parquet", "**/details_harness|hendrycksTest-medical_genetics|5_2024-01-10T15-40-02.112197.parquet", "**/details_harness|hendrycksTest-miscellaneous|5_2024-01-10T15-40-02.112197.parquet", "**/details_harness|hendrycksTest-moral_disputes|5_2024-01-10T15-40-02.112197.parquet", "**/details_harness|hendrycksTest-moral_scenarios|5_2024-01-10T15-40-02.112197.parquet", "**/details_harness|hendrycksTest-nutrition|5_2024-01-10T15-40-02.112197.parquet", "**/details_harness|hendrycksTest-philosophy|5_2024-01-10T15-40-02.112197.parquet", "**/details_harness|hendrycksTest-prehistory|5_2024-01-10T15-40-02.112197.parquet", "**/details_harness|hendrycksTest-professional_accounting|5_2024-01-10T15-40-02.112197.parquet", "**/details_harness|hendrycksTest-professional_law|5_2024-01-10T15-40-02.112197.parquet", "**/details_harness|hendrycksTest-professional_medicine|5_2024-01-10T15-40-02.112197.parquet", "**/details_harness|hendrycksTest-professional_psychology|5_2024-01-10T15-40-02.112197.parquet", "**/details_harness|hendrycksTest-public_relations|5_2024-01-10T15-40-02.112197.parquet", "**/details_harness|hendrycksTest-security_studies|5_2024-01-10T15-40-02.112197.parquet", "**/details_harness|hendrycksTest-sociology|5_2024-01-10T15-40-02.112197.parquet", "**/details_harness|hendrycksTest-us_foreign_policy|5_2024-01-10T15-40-02.112197.parquet", "**/details_harness|hendrycksTest-virology|5_2024-01-10T15-40-02.112197.parquet", "**/details_harness|hendrycksTest-world_religions|5_2024-01-10T15-40-02.112197.parquet"]}, {"split": "latest", "path": ["**/details_harness|hendrycksTest-abstract_algebra|5_2024-01-10T15-40-02.112197.parquet", "**/details_harness|hendrycksTest-anatomy|5_2024-01-10T15-40-02.112197.parquet", "**/details_harness|hendrycksTest-astronomy|5_2024-01-10T15-40-02.112197.parquet", "**/details_harness|hendrycksTest-business_ethics|5_2024-01-10T15-40-02.112197.parquet", "**/details_harness|hendrycksTest-clinical_knowledge|5_2024-01-10T15-40-02.112197.parquet", "**/details_harness|hendrycksTest-college_biology|5_2024-01-10T15-40-02.112197.parquet", "**/details_harness|hendrycksTest-college_chemistry|5_2024-01-10T15-40-02.112197.parquet", "**/details_harness|hendrycksTest-college_computer_science|5_2024-01-10T15-40-02.112197.parquet", "**/details_harness|hendrycksTest-college_mathematics|5_2024-01-10T15-40-02.112197.parquet", "**/details_harness|hendrycksTest-college_medicine|5_2024-01-10T15-40-02.112197.parquet", "**/details_harness|hendrycksTest-college_physics|5_2024-01-10T15-40-02.112197.parquet", "**/details_harness|hendrycksTest-computer_security|5_2024-01-10T15-40-02.112197.parquet", "**/details_harness|hendrycksTest-conceptual_physics|5_2024-01-10T15-40-02.112197.parquet", "**/details_harness|hendrycksTest-econometrics|5_2024-01-10T15-40-02.112197.parquet", "**/details_harness|hendrycksTest-electrical_engineering|5_2024-01-10T15-40-02.112197.parquet", "**/details_harness|hendrycksTest-elementary_mathematics|5_2024-01-10T15-40-02.112197.parquet", "**/details_harness|hendrycksTest-formal_logic|5_2024-01-10T15-40-02.112197.parquet", "**/details_harness|hendrycksTest-global_facts|5_2024-01-10T15-40-02.112197.parquet", "**/details_harness|hendrycksTest-high_school_biology|5_2024-01-10T15-40-02.112197.parquet", "**/details_harness|hendrycksTest-high_school_chemistry|5_2024-01-10T15-40-02.112197.parquet", "**/details_harness|hendrycksTest-high_school_computer_science|5_2024-01-10T15-40-02.112197.parquet", "**/details_harness|hendrycksTest-high_school_european_history|5_2024-01-10T15-40-02.112197.parquet", "**/details_harness|hendrycksTest-high_school_geography|5_2024-01-10T15-40-02.112197.parquet", "**/details_harness|hendrycksTest-high_school_government_and_politics|5_2024-01-10T15-40-02.112197.parquet", "**/details_harness|hendrycksTest-high_school_macroeconomics|5_2024-01-10T15-40-02.112197.parquet", "**/details_harness|hendrycksTest-high_school_mathematics|5_2024-01-10T15-40-02.112197.parquet", "**/details_harness|hendrycksTest-high_school_microeconomics|5_2024-01-10T15-40-02.112197.parquet", "**/details_harness|hendrycksTest-high_school_physics|5_2024-01-10T15-40-02.112197.parquet", "**/details_harness|hendrycksTest-high_school_psychology|5_2024-01-10T15-40-02.112197.parquet", "**/details_harness|hendrycksTest-high_school_statistics|5_2024-01-10T15-40-02.112197.parquet", "**/details_harness|hendrycksTest-high_school_us_history|5_2024-01-10T15-40-02.112197.parquet", "**/details_harness|hendrycksTest-high_school_world_history|5_2024-01-10T15-40-02.112197.parquet", "**/details_harness|hendrycksTest-human_aging|5_2024-01-10T15-40-02.112197.parquet", "**/details_harness|hendrycksTest-human_sexuality|5_2024-01-10T15-40-02.112197.parquet", "**/details_harness|hendrycksTest-international_law|5_2024-01-10T15-40-02.112197.parquet", "**/details_harness|hendrycksTest-jurisprudence|5_2024-01-10T15-40-02.112197.parquet", "**/details_harness|hendrycksTest-logical_fallacies|5_2024-01-10T15-40-02.112197.parquet", "**/details_harness|hendrycksTest-machine_learning|5_2024-01-10T15-40-02.112197.parquet", "**/details_harness|hendrycksTest-management|5_2024-01-10T15-40-02.112197.parquet", "**/details_harness|hendrycksTest-marketing|5_2024-01-10T15-40-02.112197.parquet", "**/details_harness|hendrycksTest-medical_genetics|5_2024-01-10T15-40-02.112197.parquet", "**/details_harness|hendrycksTest-miscellaneous|5_2024-01-10T15-40-02.112197.parquet", "**/details_harness|hendrycksTest-moral_disputes|5_2024-01-10T15-40-02.112197.parquet", "**/details_harness|hendrycksTest-moral_scenarios|5_2024-01-10T15-40-02.112197.parquet", "**/details_harness|hendrycksTest-nutrition|5_2024-01-10T15-40-02.112197.parquet", "**/details_harness|hendrycksTest-philosophy|5_2024-01-10T15-40-02.112197.parquet", "**/details_harness|hendrycksTest-prehistory|5_2024-01-10T15-40-02.112197.parquet", "**/details_harness|hendrycksTest-professional_accounting|5_2024-01-10T15-40-02.112197.parquet", "**/details_harness|hendrycksTest-professional_law|5_2024-01-10T15-40-02.112197.parquet", "**/details_harness|hendrycksTest-professional_medicine|5_2024-01-10T15-40-02.112197.parquet", "**/details_harness|hendrycksTest-professional_psychology|5_2024-01-10T15-40-02.112197.parquet", "**/details_harness|hendrycksTest-public_relations|5_2024-01-10T15-40-02.112197.parquet", "**/details_harness|hendrycksTest-security_studies|5_2024-01-10T15-40-02.112197.parquet", "**/details_harness|hendrycksTest-sociology|5_2024-01-10T15-40-02.112197.parquet", "**/details_harness|hendrycksTest-us_foreign_policy|5_2024-01-10T15-40-02.112197.parquet", "**/details_harness|hendrycksTest-virology|5_2024-01-10T15-40-02.112197.parquet", "**/details_harness|hendrycksTest-world_religions|5_2024-01-10T15-40-02.112197.parquet"]}]}, {"config_name": "harness_hendrycksTest_abstract_algebra_5", "data_files": [{"split": "2024_01_10T15_40_02.112197", "path": ["**/details_harness|hendrycksTest-abstract_algebra|5_2024-01-10T15-40-02.112197.parquet"]}, {"split": "latest", "path": ["**/details_harness|hendrycksTest-abstract_algebra|5_2024-01-10T15-40-02.112197.parquet"]}]}, {"config_name": "harness_hendrycksTest_anatomy_5", "data_files": [{"split": "2024_01_10T15_40_02.112197", "path": ["**/details_harness|hendrycksTest-anatomy|5_2024-01-10T15-40-02.112197.parquet"]}, {"split": "latest", "path": ["**/details_harness|hendrycksTest-anatomy|5_2024-01-10T15-40-02.112197.parquet"]}]}, {"config_name": "harness_hendrycksTest_astronomy_5", "data_files": [{"split": "2024_01_10T15_40_02.112197", "path": ["**/details_harness|hendrycksTest-astronomy|5_2024-01-10T15-40-02.112197.parquet"]}, {"split": "latest", "path": ["**/details_harness|hendrycksTest-astronomy|5_2024-01-10T15-40-02.112197.parquet"]}]}, {"config_name": "harness_hendrycksTest_business_ethics_5", "data_files": [{"split": "2024_01_10T15_40_02.112197", "path": ["**/details_harness|hendrycksTest-business_ethics|5_2024-01-10T15-40-02.112197.parquet"]}, {"split": "latest", "path": ["**/details_harness|hendrycksTest-business_ethics|5_2024-01-10T15-40-02.112197.parquet"]}]}, {"config_name": "harness_hendrycksTest_clinical_knowledge_5", "data_files": [{"split": "2024_01_10T15_40_02.112197", "path": ["**/details_harness|hendrycksTest-clinical_knowledge|5_2024-01-10T15-40-02.112197.parquet"]}, {"split": "latest", "path": ["**/details_harness|hendrycksTest-clinical_knowledge|5_2024-01-10T15-40-02.112197.parquet"]}]}, {"config_name": "harness_hendrycksTest_college_biology_5", "data_files": [{"split": "2024_01_10T15_40_02.112197", "path": ["**/details_harness|hendrycksTest-college_biology|5_2024-01-10T15-40-02.112197.parquet"]}, {"split": "latest", "path": ["**/details_harness|hendrycksTest-college_biology|5_2024-01-10T15-40-02.112197.parquet"]}]}, {"config_name": "harness_hendrycksTest_college_chemistry_5", "data_files": [{"split": "2024_01_10T15_40_02.112197", "path": ["**/details_harness|hendrycksTest-college_chemistry|5_2024-01-10T15-40-02.112197.parquet"]}, {"split": "latest", "path": ["**/details_harness|hendrycksTest-college_chemistry|5_2024-01-10T15-40-02.112197.parquet"]}]}, {"config_name": "harness_hendrycksTest_college_computer_science_5", "data_files": [{"split": "2024_01_10T15_40_02.112197", "path": ["**/details_harness|hendrycksTest-college_computer_science|5_2024-01-10T15-40-02.112197.parquet"]}, {"split": "latest", "path": ["**/details_harness|hendrycksTest-college_computer_science|5_2024-01-10T15-40-02.112197.parquet"]}]}, {"config_name": "harness_hendrycksTest_college_mathematics_5", "data_files": [{"split": "2024_01_10T15_40_02.112197", "path": ["**/details_harness|hendrycksTest-college_mathematics|5_2024-01-10T15-40-02.112197.parquet"]}, {"split": "latest", "path": ["**/details_harness|hendrycksTest-college_mathematics|5_2024-01-10T15-40-02.112197.parquet"]}]}, {"config_name": "harness_hendrycksTest_college_medicine_5", "data_files": [{"split": "2024_01_10T15_40_02.112197", "path": ["**/details_harness|hendrycksTest-college_medicine|5_2024-01-10T15-40-02.112197.parquet"]}, {"split": "latest", "path": ["**/details_harness|hendrycksTest-college_medicine|5_2024-01-10T15-40-02.112197.parquet"]}]}, {"config_name": "harness_hendrycksTest_college_physics_5", "data_files": [{"split": "2024_01_10T15_40_02.112197", "path": ["**/details_harness|hendrycksTest-college_physics|5_2024-01-10T15-40-02.112197.parquet"]}, {"split": "latest", "path": ["**/details_harness|hendrycksTest-college_physics|5_2024-01-10T15-40-02.112197.parquet"]}]}, {"config_name": "harness_hendrycksTest_computer_security_5", "data_files": [{"split": "2024_01_10T15_40_02.112197", "path": ["**/details_harness|hendrycksTest-computer_security|5_2024-01-10T15-40-02.112197.parquet"]}, {"split": "latest", "path": ["**/details_harness|hendrycksTest-computer_security|5_2024-01-10T15-40-02.112197.parquet"]}]}, {"config_name": "harness_hendrycksTest_conceptual_physics_5", "data_files": [{"split": "2024_01_10T15_40_02.112197", "path": ["**/details_harness|hendrycksTest-conceptual_physics|5_2024-01-10T15-40-02.112197.parquet"]}, {"split": "latest", "path": ["**/details_harness|hendrycksTest-conceptual_physics|5_2024-01-10T15-40-02.112197.parquet"]}]}, {"config_name": "harness_hendrycksTest_econometrics_5", "data_files": [{"split": "2024_01_10T15_40_02.112197", "path": ["**/details_harness|hendrycksTest-econometrics|5_2024-01-10T15-40-02.112197.parquet"]}, {"split": "latest", "path": ["**/details_harness|hendrycksTest-econometrics|5_2024-01-10T15-40-02.112197.parquet"]}]}, {"config_name": "harness_hendrycksTest_electrical_engineering_5", "data_files": [{"split": "2024_01_10T15_40_02.112197", "path": ["**/details_harness|hendrycksTest-electrical_engineering|5_2024-01-10T15-40-02.112197.parquet"]}, {"split": "latest", "path": ["**/details_harness|hendrycksTest-electrical_engineering|5_2024-01-10T15-40-02.112197.parquet"]}]}, {"config_name": "harness_hendrycksTest_elementary_mathematics_5", "data_files": [{"split": "2024_01_10T15_40_02.112197", "path": ["**/details_harness|hendrycksTest-elementary_mathematics|5_2024-01-10T15-40-02.112197.parquet"]}, {"split": "latest", "path": ["**/details_harness|hendrycksTest-elementary_mathematics|5_2024-01-10T15-40-02.112197.parquet"]}]}, {"config_name": "harness_hendrycksTest_formal_logic_5", "data_files": [{"split": "2024_01_10T15_40_02.112197", "path": ["**/details_harness|hendrycksTest-formal_logic|5_2024-01-10T15-40-02.112197.parquet"]}, {"split": "latest", "path": ["**/details_harness|hendrycksTest-formal_logic|5_2024-01-10T15-40-02.112197.parquet"]}]}, {"config_name": "harness_hendrycksTest_global_facts_5", "data_files": [{"split": "2024_01_10T15_40_02.112197", "path": ["**/details_harness|hendrycksTest-global_facts|5_2024-01-10T15-40-02.112197.parquet"]}, {"split": "latest", "path": ["**/details_harness|hendrycksTest-global_facts|5_2024-01-10T15-40-02.112197.parquet"]}]}, {"config_name": "harness_hendrycksTest_high_school_biology_5", "data_files": [{"split": "2024_01_10T15_40_02.112197", "path": ["**/details_harness|hendrycksTest-high_school_biology|5_2024-01-10T15-40-02.112197.parquet"]}, {"split": "latest", "path": ["**/details_harness|hendrycksTest-high_school_biology|5_2024-01-10T15-40-02.112197.parquet"]}]}, {"config_name": "harness_hendrycksTest_high_school_chemistry_5", "data_files": [{"split": "2024_01_10T15_40_02.112197", "path": ["**/details_harness|hendrycksTest-high_school_chemistry|5_2024-01-10T15-40-02.112197.parquet"]}, {"split": "latest", "path": ["**/details_harness|hendrycksTest-high_school_chemistry|5_2024-01-10T15-40-02.112197.parquet"]}]}, {"config_name": "harness_hendrycksTest_high_school_computer_science_5", "data_files": [{"split": "2024_01_10T15_40_02.112197", "path": ["**/details_harness|hendrycksTest-high_school_computer_science|5_2024-01-10T15-40-02.112197.parquet"]}, {"split": "latest", "path": ["**/details_harness|hendrycksTest-high_school_computer_science|5_2024-01-10T15-40-02.112197.parquet"]}]}, {"config_name": "harness_hendrycksTest_high_school_european_history_5", "data_files": [{"split": "2024_01_10T15_40_02.112197", "path": ["**/details_harness|hendrycksTest-high_school_european_history|5_2024-01-10T15-40-02.112197.parquet"]}, {"split": "latest", "path": ["**/details_harness|hendrycksTest-high_school_european_history|5_2024-01-10T15-40-02.112197.parquet"]}]}, {"config_name": "harness_hendrycksTest_high_school_geography_5", "data_files": [{"split": "2024_01_10T15_40_02.112197", "path": ["**/details_harness|hendrycksTest-high_school_geography|5_2024-01-10T15-40-02.112197.parquet"]}, {"split": "latest", "path": ["**/details_harness|hendrycksTest-high_school_geography|5_2024-01-10T15-40-02.112197.parquet"]}]}, {"config_name": "harness_hendrycksTest_high_school_government_and_politics_5", "data_files": [{"split": "2024_01_10T15_40_02.112197", "path": ["**/details_harness|hendrycksTest-high_school_government_and_politics|5_2024-01-10T15-40-02.112197.parquet"]}, {"split": "latest", "path": ["**/details_harness|hendrycksTest-high_school_government_and_politics|5_2024-01-10T15-40-02.112197.parquet"]}]}, {"config_name": "harness_hendrycksTest_high_school_macroeconomics_5", "data_files": [{"split": "2024_01_10T15_40_02.112197", "path": ["**/details_harness|hendrycksTest-high_school_macroeconomics|5_2024-01-10T15-40-02.112197.parquet"]}, {"split": "latest", "path": ["**/details_harness|hendrycksTest-high_school_macroeconomics|5_2024-01-10T15-40-02.112197.parquet"]}]}, {"config_name": "harness_hendrycksTest_high_school_mathematics_5", "data_files": [{"split": "2024_01_10T15_40_02.112197", "path": ["**/details_harness|hendrycksTest-high_school_mathematics|5_2024-01-10T15-40-02.112197.parquet"]}, {"split": "latest", "path": ["**/details_harness|hendrycksTest-high_school_mathematics|5_2024-01-10T15-40-02.112197.parquet"]}]}, {"config_name": "harness_hendrycksTest_high_school_microeconomics_5", "data_files": [{"split": "2024_01_10T15_40_02.112197", "path": ["**/details_harness|hendrycksTest-high_school_microeconomics|5_2024-01-10T15-40-02.112197.parquet"]}, {"split": "latest", "path": ["**/details_harness|hendrycksTest-high_school_microeconomics|5_2024-01-10T15-40-02.112197.parquet"]}]}, {"config_name": "harness_hendrycksTest_high_school_physics_5", "data_files": [{"split": "2024_01_10T15_40_02.112197", "path": ["**/details_harness|hendrycksTest-high_school_physics|5_2024-01-10T15-40-02.112197.parquet"]}, {"split": "latest", "path": ["**/details_harness|hendrycksTest-high_school_physics|5_2024-01-10T15-40-02.112197.parquet"]}]}, {"config_name": "harness_hendrycksTest_high_school_psychology_5", "data_files": [{"split": "2024_01_10T15_40_02.112197", "path": ["**/details_harness|hendrycksTest-high_school_psychology|5_2024-01-10T15-40-02.112197.parquet"]}, {"split": "latest", "path": ["**/details_harness|hendrycksTest-high_school_psychology|5_2024-01-10T15-40-02.112197.parquet"]}]}, {"config_name": "harness_hendrycksTest_high_school_statistics_5", "data_files": [{"split": "2024_01_10T15_40_02.112197", "path": ["**/details_harness|hendrycksTest-high_school_statistics|5_2024-01-10T15-40-02.112197.parquet"]}, {"split": "latest", "path": ["**/details_harness|hendrycksTest-high_school_statistics|5_2024-01-10T15-40-02.112197.parquet"]}]}, {"config_name": "harness_hendrycksTest_high_school_us_history_5", "data_files": [{"split": "2024_01_10T15_40_02.112197", "path": ["**/details_harness|hendrycksTest-high_school_us_history|5_2024-01-10T15-40-02.112197.parquet"]}, {"split": "latest", "path": ["**/details_harness|hendrycksTest-high_school_us_history|5_2024-01-10T15-40-02.112197.parquet"]}]}, {"config_name": "harness_hendrycksTest_high_school_world_history_5", "data_files": [{"split": "2024_01_10T15_40_02.112197", "path": ["**/details_harness|hendrycksTest-high_school_world_history|5_2024-01-10T15-40-02.112197.parquet"]}, {"split": "latest", "path": ["**/details_harness|hendrycksTest-high_school_world_history|5_2024-01-10T15-40-02.112197.parquet"]}]}, {"config_name": "harness_hendrycksTest_human_aging_5", "data_files": [{"split": "2024_01_10T15_40_02.112197", "path": ["**/details_harness|hendrycksTest-human_aging|5_2024-01-10T15-40-02.112197.parquet"]}, {"split": "latest", "path": ["**/details_harness|hendrycksTest-human_aging|5_2024-01-10T15-40-02.112197.parquet"]}]}, {"config_name": "harness_hendrycksTest_human_sexuality_5", "data_files": [{"split": "2024_01_10T15_40_02.112197", "path": ["**/details_harness|hendrycksTest-human_sexuality|5_2024-01-10T15-40-02.112197.parquet"]}, {"split": "latest", "path": ["**/details_harness|hendrycksTest-human_sexuality|5_2024-01-10T15-40-02.112197.parquet"]}]}, {"config_name": "harness_hendrycksTest_international_law_5", "data_files": [{"split": "2024_01_10T15_40_02.112197", "path": ["**/details_harness|hendrycksTest-international_law|5_2024-01-10T15-40-02.112197.parquet"]}, {"split": "latest", "path": ["**/details_harness|hendrycksTest-international_law|5_2024-01-10T15-40-02.112197.parquet"]}]}, {"config_name": "harness_hendrycksTest_jurisprudence_5", "data_files": [{"split": "2024_01_10T15_40_02.112197", "path": ["**/details_harness|hendrycksTest-jurisprudence|5_2024-01-10T15-40-02.112197.parquet"]}, {"split": "latest", "path": ["**/details_harness|hendrycksTest-jurisprudence|5_2024-01-10T15-40-02.112197.parquet"]}]}, {"config_name": "harness_hendrycksTest_logical_fallacies_5", "data_files": [{"split": "2024_01_10T15_40_02.112197", "path": ["**/details_harness|hendrycksTest-logical_fallacies|5_2024-01-10T15-40-02.112197.parquet"]}, {"split": "latest", "path": ["**/details_harness|hendrycksTest-logical_fallacies|5_2024-01-10T15-40-02.112197.parquet"]}]}, {"config_name": "harness_hendrycksTest_machine_learning_5", "data_files": [{"split": "2024_01_10T15_40_02.112197", "path": ["**/details_harness|hendrycksTest-machine_learning|5_2024-01-10T15-40-02.112197.parquet"]}, {"split": "latest", "path": ["**/details_harness|hendrycksTest-machine_learning|5_2024-01-10T15-40-02.112197.parquet"]}]}, {"config_name": "harness_hendrycksTest_management_5", "data_files": [{"split": "2024_01_10T15_40_02.112197", "path": ["**/details_harness|hendrycksTest-management|5_2024-01-10T15-40-02.112197.parquet"]}, {"split": "latest", "path": ["**/details_harness|hendrycksTest-management|5_2024-01-10T15-40-02.112197.parquet"]}]}, {"config_name": "harness_hendrycksTest_marketing_5", "data_files": [{"split": "2024_01_10T15_40_02.112197", "path": ["**/details_harness|hendrycksTest-marketing|5_2024-01-10T15-40-02.112197.parquet"]}, {"split": "latest", "path": ["**/details_harness|hendrycksTest-marketing|5_2024-01-10T15-40-02.112197.parquet"]}]}, {"config_name": "harness_hendrycksTest_medical_genetics_5", "data_files": [{"split": "2024_01_10T15_40_02.112197", "path": ["**/details_harness|hendrycksTest-medical_genetics|5_2024-01-10T15-40-02.112197.parquet"]}, {"split": "latest", "path": ["**/details_harness|hendrycksTest-medical_genetics|5_2024-01-10T15-40-02.112197.parquet"]}]}, {"config_name": "harness_hendrycksTest_miscellaneous_5", "data_files": [{"split": "2024_01_10T15_40_02.112197", "path": ["**/details_harness|hendrycksTest-miscellaneous|5_2024-01-10T15-40-02.112197.parquet"]}, {"split": "latest", "path": ["**/details_harness|hendrycksTest-miscellaneous|5_2024-01-10T15-40-02.112197.parquet"]}]}, {"config_name": "harness_hendrycksTest_moral_disputes_5", "data_files": [{"split": "2024_01_10T15_40_02.112197", "path": ["**/details_harness|hendrycksTest-moral_disputes|5_2024-01-10T15-40-02.112197.parquet"]}, {"split": "latest", "path": ["**/details_harness|hendrycksTest-moral_disputes|5_2024-01-10T15-40-02.112197.parquet"]}]}, {"config_name": "harness_hendrycksTest_moral_scenarios_5", "data_files": [{"split": "2024_01_10T15_40_02.112197", "path": ["**/details_harness|hendrycksTest-moral_scenarios|5_2024-01-10T15-40-02.112197.parquet"]}, {"split": "latest", "path": ["**/details_harness|hendrycksTest-moral_scenarios|5_2024-01-10T15-40-02.112197.parquet"]}]}, {"config_name": "harness_hendrycksTest_nutrition_5", "data_files": [{"split": "2024_01_10T15_40_02.112197", "path": ["**/details_harness|hendrycksTest-nutrition|5_2024-01-10T15-40-02.112197.parquet"]}, {"split": "latest", "path": ["**/details_harness|hendrycksTest-nutrition|5_2024-01-10T15-40-02.112197.parquet"]}]}, {"config_name": "harness_hendrycksTest_philosophy_5", "data_files": [{"split": "2024_01_10T15_40_02.112197", "path": ["**/details_harness|hendrycksTest-philosophy|5_2024-01-10T15-40-02.112197.parquet"]}, {"split": "latest", "path": ["**/details_harness|hendrycksTest-philosophy|5_2024-01-10T15-40-02.112197.parquet"]}]}, {"config_name": "harness_hendrycksTest_prehistory_5", "data_files": [{"split": "2024_01_10T15_40_02.112197", "path": ["**/details_harness|hendrycksTest-prehistory|5_2024-01-10T15-40-02.112197.parquet"]}, {"split": "latest", "path": ["**/details_harness|hendrycksTest-prehistory|5_2024-01-10T15-40-02.112197.parquet"]}]}, {"config_name": "harness_hendrycksTest_professional_accounting_5", "data_files": [{"split": "2024_01_10T15_40_02.112197", "path": ["**/details_harness|hendrycksTest-professional_accounting|5_2024-01-10T15-40-02.112197.parquet"]}, {"split": "latest", "path": ["**/details_harness|hendrycksTest-professional_accounting|5_2024-01-10T15-40-02.112197.parquet"]}]}, {"config_name": "harness_hendrycksTest_professional_law_5", "data_files": [{"split": "2024_01_10T15_40_02.112197", "path": ["**/details_harness|hendrycksTest-professional_law|5_2024-01-10T15-40-02.112197.parquet"]}, {"split": "latest", "path": ["**/details_harness|hendrycksTest-professional_law|5_2024-01-10T15-40-02.112197.parquet"]}]}, {"config_name": "harness_hendrycksTest_professional_medicine_5", "data_files": [{"split": "2024_01_10T15_40_02.112197", "path": ["**/details_harness|hendrycksTest-professional_medicine|5_2024-01-10T15-40-02.112197.parquet"]}, {"split": "latest", "path": ["**/details_harness|hendrycksTest-professional_medicine|5_2024-01-10T15-40-02.112197.parquet"]}]}, {"config_name": "harness_hendrycksTest_professional_psychology_5", "data_files": [{"split": "2024_01_10T15_40_02.112197", "path": ["**/details_harness|hendrycksTest-professional_psychology|5_2024-01-10T15-40-02.112197.parquet"]}, {"split": "latest", "path": ["**/details_harness|hendrycksTest-professional_psychology|5_2024-01-10T15-40-02.112197.parquet"]}]}, {"config_name": "harness_hendrycksTest_public_relations_5", "data_files": [{"split": "2024_01_10T15_40_02.112197", "path": ["**/details_harness|hendrycksTest-public_relations|5_2024-01-10T15-40-02.112197.parquet"]}, {"split": "latest", "path": ["**/details_harness|hendrycksTest-public_relations|5_2024-01-10T15-40-02.112197.parquet"]}]}, {"config_name": "harness_hendrycksTest_security_studies_5", "data_files": [{"split": "2024_01_10T15_40_02.112197", "path": ["**/details_harness|hendrycksTest-security_studies|5_2024-01-10T15-40-02.112197.parquet"]}, {"split": "latest", "path": ["**/details_harness|hendrycksTest-security_studies|5_2024-01-10T15-40-02.112197.parquet"]}]}, {"config_name": "harness_hendrycksTest_sociology_5", "data_files": [{"split": "2024_01_10T15_40_02.112197", "path": ["**/details_harness|hendrycksTest-sociology|5_2024-01-10T15-40-02.112197.parquet"]}, {"split": "latest", "path": ["**/details_harness|hendrycksTest-sociology|5_2024-01-10T15-40-02.112197.parquet"]}]}, {"config_name": "harness_hendrycksTest_us_foreign_policy_5", "data_files": [{"split": "2024_01_10T15_40_02.112197", "path": ["**/details_harness|hendrycksTest-us_foreign_policy|5_2024-01-10T15-40-02.112197.parquet"]}, {"split": "latest", "path": ["**/details_harness|hendrycksTest-us_foreign_policy|5_2024-01-10T15-40-02.112197.parquet"]}]}, {"config_name": "harness_hendrycksTest_virology_5", "data_files": [{"split": "2024_01_10T15_40_02.112197", "path": ["**/details_harness|hendrycksTest-virology|5_2024-01-10T15-40-02.112197.parquet"]}, {"split": "latest", "path": ["**/details_harness|hendrycksTest-virology|5_2024-01-10T15-40-02.112197.parquet"]}]}, {"config_name": "harness_hendrycksTest_world_religions_5", "data_files": [{"split": "2024_01_10T15_40_02.112197", "path": ["**/details_harness|hendrycksTest-world_religions|5_2024-01-10T15-40-02.112197.parquet"]}, {"split": "latest", "path": ["**/details_harness|hendrycksTest-world_religions|5_2024-01-10T15-40-02.112197.parquet"]}]}, {"config_name": "harness_truthfulqa_mc_0", "data_files": [{"split": "2024_01_10T15_40_02.112197", "path": ["**/details_harness|truthfulqa:mc|0_2024-01-10T15-40-02.112197.parquet"]}, {"split": "latest", "path": ["**/details_harness|truthfulqa:mc|0_2024-01-10T15-40-02.112197.parquet"]}]}, {"config_name": "harness_winogrande_5", "data_files": [{"split": "2024_01_10T15_40_02.112197", "path": ["**/details_harness|winogrande|5_2024-01-10T15-40-02.112197.parquet"]}, {"split": "latest", "path": ["**/details_harness|winogrande|5_2024-01-10T15-40-02.112197.parquet"]}]}, {"config_name": "results", "data_files": [{"split": "2024_01_10T15_40_02.112197", "path": ["results_2024-01-10T15-40-02.112197.parquet"]}, {"split": "latest", "path": ["results_2024-01-10T15-40-02.112197.parquet"]}]}]} | 2024-01-10T15:42:46+00:00 | [] | [] | TAGS
#region-us
|
# Dataset Card for Evaluation run of cookinai/OpenCM-14
Dataset automatically created during the evaluation run of model cookinai/OpenCM-14 on the Open LLM Leaderboard.
The dataset is composed of 63 configuration, each one coresponding to one of the evaluated task.
The dataset has been created from 1 run(s). Each run can be found as a specific split in each configuration, the split being named using the timestamp of the run.The "train" split is always pointing to the latest results.
An additional configuration "results" store all the aggregated results of the run (and is used to compute and display the aggregated metrics on the Open LLM Leaderboard).
To load the details from a run, you can for instance do the following:
## Latest results
These are the latest results from run 2024-01-10T15:40:02.112197(note that their might be results for other tasks in the repos if successive evals didn't cover the same tasks. You find each in the results and the "latest" split for each eval):
## Dataset Details
### Dataset Description
- Curated by:
- Funded by [optional]:
- Shared by [optional]:
- Language(s) (NLP):
- License:
### Dataset Sources [optional]
- Repository:
- Paper [optional]:
- Demo [optional]:
## Uses
### Direct Use
### Out-of-Scope Use
## Dataset Structure
## Dataset Creation
### Curation Rationale
### Source Data
#### Data Collection and Processing
#### Who are the source data producers?
### Annotations [optional]
#### Annotation process
#### Who are the annotators?
#### Personal and Sensitive Information
## Bias, Risks, and Limitations
### Recommendations
Users should be made aware of the risks, biases and limitations of the dataset. More information needed for further recommendations.
[optional]
BibTeX:
APA:
## Glossary [optional]
## More Information [optional]
## Dataset Card Authors [optional]
## Dataset Card Contact
| [
"# Dataset Card for Evaluation run of cookinai/OpenCM-14\n\n\n\nDataset automatically created during the evaluation run of model cookinai/OpenCM-14 on the Open LLM Leaderboard.\n\nThe dataset is composed of 63 configuration, each one coresponding to one of the evaluated task.\n\nThe dataset has been created from 1 run(s). Each run can be found as a specific split in each configuration, the split being named using the timestamp of the run.The \"train\" split is always pointing to the latest results.\n\nAn additional configuration \"results\" store all the aggregated results of the run (and is used to compute and display the aggregated metrics on the Open LLM Leaderboard).\n\nTo load the details from a run, you can for instance do the following:",
"## Latest results\n\nThese are the latest results from run 2024-01-10T15:40:02.112197(note that their might be results for other tasks in the repos if successive evals didn't cover the same tasks. You find each in the results and the \"latest\" split for each eval):",
"## Dataset Details",
"### Dataset Description\n\n\n\n\n\n- Curated by: \n- Funded by [optional]: \n- Shared by [optional]: \n- Language(s) (NLP): \n- License:",
"### Dataset Sources [optional]\n\n\n\n- Repository: \n- Paper [optional]: \n- Demo [optional]:",
"## Uses",
"### Direct Use",
"### Out-of-Scope Use",
"## Dataset Structure",
"## Dataset Creation",
"### Curation Rationale",
"### Source Data",
"#### Data Collection and Processing",
"#### Who are the source data producers?",
"### Annotations [optional]",
"#### Annotation process",
"#### Who are the annotators?",
"#### Personal and Sensitive Information",
"## Bias, Risks, and Limitations",
"### Recommendations\n\n\n\nUsers should be made aware of the risks, biases and limitations of the dataset. More information needed for further recommendations.\n\n[optional]\n\n\n\nBibTeX:\n\n\n\nAPA:",
"## Glossary [optional]",
"## More Information [optional]",
"## Dataset Card Authors [optional]",
"## Dataset Card Contact"
] | [
"TAGS\n#region-us \n",
"# Dataset Card for Evaluation run of cookinai/OpenCM-14\n\n\n\nDataset automatically created during the evaluation run of model cookinai/OpenCM-14 on the Open LLM Leaderboard.\n\nThe dataset is composed of 63 configuration, each one coresponding to one of the evaluated task.\n\nThe dataset has been created from 1 run(s). Each run can be found as a specific split in each configuration, the split being named using the timestamp of the run.The \"train\" split is always pointing to the latest results.\n\nAn additional configuration \"results\" store all the aggregated results of the run (and is used to compute and display the aggregated metrics on the Open LLM Leaderboard).\n\nTo load the details from a run, you can for instance do the following:",
"## Latest results\n\nThese are the latest results from run 2024-01-10T15:40:02.112197(note that their might be results for other tasks in the repos if successive evals didn't cover the same tasks. You find each in the results and the \"latest\" split for each eval):",
"## Dataset Details",
"### Dataset Description\n\n\n\n\n\n- Curated by: \n- Funded by [optional]: \n- Shared by [optional]: \n- Language(s) (NLP): \n- License:",
"### Dataset Sources [optional]\n\n\n\n- Repository: \n- Paper [optional]: \n- Demo [optional]:",
"## Uses",
"### Direct Use",
"### Out-of-Scope Use",
"## Dataset Structure",
"## Dataset Creation",
"### Curation Rationale",
"### Source Data",
"#### Data Collection and Processing",
"#### Who are the source data producers?",
"### Annotations [optional]",
"#### Annotation process",
"#### Who are the annotators?",
"#### Personal and Sensitive Information",
"## Bias, Risks, and Limitations",
"### Recommendations\n\n\n\nUsers should be made aware of the risks, biases and limitations of the dataset. More information needed for further recommendations.\n\n[optional]\n\n\n\nBibTeX:\n\n\n\nAPA:",
"## Glossary [optional]",
"## More Information [optional]",
"## Dataset Card Authors [optional]",
"## Dataset Card Contact"
] |
319aaf9ec6f75ca6624b3b4833ca96812ee20859 |
# Dataset Card for Evaluation run of cookinai/CM-14
<!-- Provide a quick summary of the dataset. -->
Dataset automatically created during the evaluation run of model [cookinai/CM-14](https://huggingface.co/cookinai/CM-14) on the [Open LLM Leaderboard](https://huggingface.co/spaces/HuggingFaceH4/open_llm_leaderboard).
The dataset is composed of 63 configuration, each one coresponding to one of the evaluated task.
The dataset has been created from 1 run(s). Each run can be found as a specific split in each configuration, the split being named using the timestamp of the run.The "train" split is always pointing to the latest results.
An additional configuration "results" store all the aggregated results of the run (and is used to compute and display the aggregated metrics on the [Open LLM Leaderboard](https://huggingface.co/spaces/HuggingFaceH4/open_llm_leaderboard)).
To load the details from a run, you can for instance do the following:
```python
from datasets import load_dataset
data = load_dataset("open-llm-leaderboard/details_cookinai__CM-14",
"harness_winogrande_5",
split="train")
```
## Latest results
These are the [latest results from run 2024-01-10T15:39:56.317779](https://huggingface.co/datasets/open-llm-leaderboard/details_cookinai__CM-14/blob/main/results_2024-01-10T15-39-56.317779.json)(note that their might be results for other tasks in the repos if successive evals didn't cover the same tasks. You find each in the results and the "latest" split for each eval):
```python
{
"all": {
"acc": 0.6580185798671865,
"acc_stderr": 0.03192368037476644,
"acc_norm": 0.6580152943319993,
"acc_norm_stderr": 0.03258224944124306,
"mc1": 0.45532435740514077,
"mc1_stderr": 0.017433490102538765,
"mc2": 0.6190301844108673,
"mc2_stderr": 0.015232563824973148
},
"harness|arc:challenge|25": {
"acc": 0.659556313993174,
"acc_stderr": 0.013847460518892978,
"acc_norm": 0.6936860068259386,
"acc_norm_stderr": 0.013470584417276513
},
"harness|hellaswag|10": {
"acc": 0.6870145389364668,
"acc_stderr": 0.004627607991626914,
"acc_norm": 0.8697470623381797,
"acc_norm_stderr": 0.003358936279867257
},
"harness|hendrycksTest-abstract_algebra|5": {
"acc": 0.38,
"acc_stderr": 0.048783173121456316,
"acc_norm": 0.38,
"acc_norm_stderr": 0.048783173121456316
},
"harness|hendrycksTest-anatomy|5": {
"acc": 0.6666666666666666,
"acc_stderr": 0.04072314811876837,
"acc_norm": 0.6666666666666666,
"acc_norm_stderr": 0.04072314811876837
},
"harness|hendrycksTest-astronomy|5": {
"acc": 0.6973684210526315,
"acc_stderr": 0.037385206761196686,
"acc_norm": 0.6973684210526315,
"acc_norm_stderr": 0.037385206761196686
},
"harness|hendrycksTest-business_ethics|5": {
"acc": 0.63,
"acc_stderr": 0.04852365870939099,
"acc_norm": 0.63,
"acc_norm_stderr": 0.04852365870939099
},
"harness|hendrycksTest-clinical_knowledge|5": {
"acc": 0.720754716981132,
"acc_stderr": 0.027611163402399715,
"acc_norm": 0.720754716981132,
"acc_norm_stderr": 0.027611163402399715
},
"harness|hendrycksTest-college_biology|5": {
"acc": 0.7777777777777778,
"acc_stderr": 0.03476590104304134,
"acc_norm": 0.7777777777777778,
"acc_norm_stderr": 0.03476590104304134
},
"harness|hendrycksTest-college_chemistry|5": {
"acc": 0.46,
"acc_stderr": 0.05009082659620333,
"acc_norm": 0.46,
"acc_norm_stderr": 0.05009082659620333
},
"harness|hendrycksTest-college_computer_science|5": {
"acc": 0.55,
"acc_stderr": 0.05,
"acc_norm": 0.55,
"acc_norm_stderr": 0.05
},
"harness|hendrycksTest-college_mathematics|5": {
"acc": 0.29,
"acc_stderr": 0.04560480215720684,
"acc_norm": 0.29,
"acc_norm_stderr": 0.04560480215720684
},
"harness|hendrycksTest-college_medicine|5": {
"acc": 0.6705202312138728,
"acc_stderr": 0.03583901754736412,
"acc_norm": 0.6705202312138728,
"acc_norm_stderr": 0.03583901754736412
},
"harness|hendrycksTest-college_physics|5": {
"acc": 0.45098039215686275,
"acc_stderr": 0.049512182523962625,
"acc_norm": 0.45098039215686275,
"acc_norm_stderr": 0.049512182523962625
},
"harness|hendrycksTest-computer_security|5": {
"acc": 0.77,
"acc_stderr": 0.04229525846816508,
"acc_norm": 0.77,
"acc_norm_stderr": 0.04229525846816508
},
"harness|hendrycksTest-conceptual_physics|5": {
"acc": 0.5872340425531914,
"acc_stderr": 0.03218471141400351,
"acc_norm": 0.5872340425531914,
"acc_norm_stderr": 0.03218471141400351
},
"harness|hendrycksTest-econometrics|5": {
"acc": 0.4824561403508772,
"acc_stderr": 0.04700708033551038,
"acc_norm": 0.4824561403508772,
"acc_norm_stderr": 0.04700708033551038
},
"harness|hendrycksTest-electrical_engineering|5": {
"acc": 0.5655172413793104,
"acc_stderr": 0.04130740879555498,
"acc_norm": 0.5655172413793104,
"acc_norm_stderr": 0.04130740879555498
},
"harness|hendrycksTest-elementary_mathematics|5": {
"acc": 0.42328042328042326,
"acc_stderr": 0.025446365634406783,
"acc_norm": 0.42328042328042326,
"acc_norm_stderr": 0.025446365634406783
},
"harness|hendrycksTest-formal_logic|5": {
"acc": 0.48412698412698413,
"acc_stderr": 0.04469881854072606,
"acc_norm": 0.48412698412698413,
"acc_norm_stderr": 0.04469881854072606
},
"harness|hendrycksTest-global_facts|5": {
"acc": 0.34,
"acc_stderr": 0.04760952285695235,
"acc_norm": 0.34,
"acc_norm_stderr": 0.04760952285695235
},
"harness|hendrycksTest-high_school_biology|5": {
"acc": 0.7870967741935484,
"acc_stderr": 0.023287665127268552,
"acc_norm": 0.7870967741935484,
"acc_norm_stderr": 0.023287665127268552
},
"harness|hendrycksTest-high_school_chemistry|5": {
"acc": 0.49261083743842365,
"acc_stderr": 0.035176035403610084,
"acc_norm": 0.49261083743842365,
"acc_norm_stderr": 0.035176035403610084
},
"harness|hendrycksTest-high_school_computer_science|5": {
"acc": 0.72,
"acc_stderr": 0.04512608598542127,
"acc_norm": 0.72,
"acc_norm_stderr": 0.04512608598542127
},
"harness|hendrycksTest-high_school_european_history|5": {
"acc": 0.7818181818181819,
"acc_stderr": 0.03225078108306289,
"acc_norm": 0.7818181818181819,
"acc_norm_stderr": 0.03225078108306289
},
"harness|hendrycksTest-high_school_geography|5": {
"acc": 0.7878787878787878,
"acc_stderr": 0.029126522834586815,
"acc_norm": 0.7878787878787878,
"acc_norm_stderr": 0.029126522834586815
},
"harness|hendrycksTest-high_school_government_and_politics|5": {
"acc": 0.8963730569948186,
"acc_stderr": 0.02199531196364424,
"acc_norm": 0.8963730569948186,
"acc_norm_stderr": 0.02199531196364424
},
"harness|hendrycksTest-high_school_macroeconomics|5": {
"acc": 0.6717948717948717,
"acc_stderr": 0.023807633198657266,
"acc_norm": 0.6717948717948717,
"acc_norm_stderr": 0.023807633198657266
},
"harness|hendrycksTest-high_school_mathematics|5": {
"acc": 0.337037037037037,
"acc_stderr": 0.02882088466625326,
"acc_norm": 0.337037037037037,
"acc_norm_stderr": 0.02882088466625326
},
"harness|hendrycksTest-high_school_microeconomics|5": {
"acc": 0.6890756302521008,
"acc_stderr": 0.03006676158297793,
"acc_norm": 0.6890756302521008,
"acc_norm_stderr": 0.03006676158297793
},
"harness|hendrycksTest-high_school_physics|5": {
"acc": 0.3509933774834437,
"acc_stderr": 0.03896981964257375,
"acc_norm": 0.3509933774834437,
"acc_norm_stderr": 0.03896981964257375
},
"harness|hendrycksTest-high_school_psychology|5": {
"acc": 0.8513761467889909,
"acc_stderr": 0.015251253773660836,
"acc_norm": 0.8513761467889909,
"acc_norm_stderr": 0.015251253773660836
},
"harness|hendrycksTest-high_school_statistics|5": {
"acc": 0.5277777777777778,
"acc_stderr": 0.0340470532865388,
"acc_norm": 0.5277777777777778,
"acc_norm_stderr": 0.0340470532865388
},
"harness|hendrycksTest-high_school_us_history|5": {
"acc": 0.8382352941176471,
"acc_stderr": 0.02584501798692692,
"acc_norm": 0.8382352941176471,
"acc_norm_stderr": 0.02584501798692692
},
"harness|hendrycksTest-high_school_world_history|5": {
"acc": 0.810126582278481,
"acc_stderr": 0.025530100460233494,
"acc_norm": 0.810126582278481,
"acc_norm_stderr": 0.025530100460233494
},
"harness|hendrycksTest-human_aging|5": {
"acc": 0.6905829596412556,
"acc_stderr": 0.031024411740572213,
"acc_norm": 0.6905829596412556,
"acc_norm_stderr": 0.031024411740572213
},
"harness|hendrycksTest-human_sexuality|5": {
"acc": 0.8091603053435115,
"acc_stderr": 0.034465133507525995,
"acc_norm": 0.8091603053435115,
"acc_norm_stderr": 0.034465133507525995
},
"harness|hendrycksTest-international_law|5": {
"acc": 0.8016528925619835,
"acc_stderr": 0.03640118271990947,
"acc_norm": 0.8016528925619835,
"acc_norm_stderr": 0.03640118271990947
},
"harness|hendrycksTest-jurisprudence|5": {
"acc": 0.7962962962962963,
"acc_stderr": 0.03893542518824847,
"acc_norm": 0.7962962962962963,
"acc_norm_stderr": 0.03893542518824847
},
"harness|hendrycksTest-logical_fallacies|5": {
"acc": 0.7852760736196319,
"acc_stderr": 0.03226219377286775,
"acc_norm": 0.7852760736196319,
"acc_norm_stderr": 0.03226219377286775
},
"harness|hendrycksTest-machine_learning|5": {
"acc": 0.4642857142857143,
"acc_stderr": 0.04733667890053756,
"acc_norm": 0.4642857142857143,
"acc_norm_stderr": 0.04733667890053756
},
"harness|hendrycksTest-management|5": {
"acc": 0.7766990291262136,
"acc_stderr": 0.04123553189891431,
"acc_norm": 0.7766990291262136,
"acc_norm_stderr": 0.04123553189891431
},
"harness|hendrycksTest-marketing|5": {
"acc": 0.8803418803418803,
"acc_stderr": 0.021262719400406964,
"acc_norm": 0.8803418803418803,
"acc_norm_stderr": 0.021262719400406964
},
"harness|hendrycksTest-medical_genetics|5": {
"acc": 0.72,
"acc_stderr": 0.045126085985421276,
"acc_norm": 0.72,
"acc_norm_stderr": 0.045126085985421276
},
"harness|hendrycksTest-miscellaneous|5": {
"acc": 0.8352490421455939,
"acc_stderr": 0.013265346261323788,
"acc_norm": 0.8352490421455939,
"acc_norm_stderr": 0.013265346261323788
},
"harness|hendrycksTest-moral_disputes|5": {
"acc": 0.7514450867052023,
"acc_stderr": 0.023267528432100174,
"acc_norm": 0.7514450867052023,
"acc_norm_stderr": 0.023267528432100174
},
"harness|hendrycksTest-moral_scenarios|5": {
"acc": 0.43687150837988825,
"acc_stderr": 0.01658868086453063,
"acc_norm": 0.43687150837988825,
"acc_norm_stderr": 0.01658868086453063
},
"harness|hendrycksTest-nutrition|5": {
"acc": 0.7320261437908496,
"acc_stderr": 0.025360603796242553,
"acc_norm": 0.7320261437908496,
"acc_norm_stderr": 0.025360603796242553
},
"harness|hendrycksTest-philosophy|5": {
"acc": 0.7106109324758842,
"acc_stderr": 0.025755865922632945,
"acc_norm": 0.7106109324758842,
"acc_norm_stderr": 0.025755865922632945
},
"harness|hendrycksTest-prehistory|5": {
"acc": 0.75,
"acc_stderr": 0.02409347123262133,
"acc_norm": 0.75,
"acc_norm_stderr": 0.02409347123262133
},
"harness|hendrycksTest-professional_accounting|5": {
"acc": 0.4929078014184397,
"acc_stderr": 0.02982449855912901,
"acc_norm": 0.4929078014184397,
"acc_norm_stderr": 0.02982449855912901
},
"harness|hendrycksTest-professional_law|5": {
"acc": 0.4641460234680574,
"acc_stderr": 0.012737361318730581,
"acc_norm": 0.4641460234680574,
"acc_norm_stderr": 0.012737361318730581
},
"harness|hendrycksTest-professional_medicine|5": {
"acc": 0.6948529411764706,
"acc_stderr": 0.0279715413701706,
"acc_norm": 0.6948529411764706,
"acc_norm_stderr": 0.0279715413701706
},
"harness|hendrycksTest-professional_psychology|5": {
"acc": 0.6928104575163399,
"acc_stderr": 0.01866335967146367,
"acc_norm": 0.6928104575163399,
"acc_norm_stderr": 0.01866335967146367
},
"harness|hendrycksTest-public_relations|5": {
"acc": 0.6727272727272727,
"acc_stderr": 0.0449429086625209,
"acc_norm": 0.6727272727272727,
"acc_norm_stderr": 0.0449429086625209
},
"harness|hendrycksTest-security_studies|5": {
"acc": 0.726530612244898,
"acc_stderr": 0.028535560337128445,
"acc_norm": 0.726530612244898,
"acc_norm_stderr": 0.028535560337128445
},
"harness|hendrycksTest-sociology|5": {
"acc": 0.835820895522388,
"acc_stderr": 0.026193923544454132,
"acc_norm": 0.835820895522388,
"acc_norm_stderr": 0.026193923544454132
},
"harness|hendrycksTest-us_foreign_policy|5": {
"acc": 0.86,
"acc_stderr": 0.03487350880197769,
"acc_norm": 0.86,
"acc_norm_stderr": 0.03487350880197769
},
"harness|hendrycksTest-virology|5": {
"acc": 0.5481927710843374,
"acc_stderr": 0.03874371556587953,
"acc_norm": 0.5481927710843374,
"acc_norm_stderr": 0.03874371556587953
},
"harness|hendrycksTest-world_religions|5": {
"acc": 0.8421052631578947,
"acc_stderr": 0.027966785859160893,
"acc_norm": 0.8421052631578947,
"acc_norm_stderr": 0.027966785859160893
},
"harness|truthfulqa:mc|0": {
"mc1": 0.45532435740514077,
"mc1_stderr": 0.017433490102538765,
"mc2": 0.6190301844108673,
"mc2_stderr": 0.015232563824973148
},
"harness|winogrande|5": {
"acc": 0.8105761641673244,
"acc_stderr": 0.011012790432989245
},
"harness|gsm8k|5": {
"acc": 0.7225170583775588,
"acc_stderr": 0.012333447581047539
}
}
```
## Dataset Details
### Dataset Description
<!-- Provide a longer summary of what this dataset is. -->
- **Curated by:** [More Information Needed]
- **Funded by [optional]:** [More Information Needed]
- **Shared by [optional]:** [More Information Needed]
- **Language(s) (NLP):** [More Information Needed]
- **License:** [More Information Needed]
### Dataset Sources [optional]
<!-- Provide the basic links for the dataset. -->
- **Repository:** [More Information Needed]
- **Paper [optional]:** [More Information Needed]
- **Demo [optional]:** [More Information Needed]
## Uses
<!-- Address questions around how the dataset is intended to be used. -->
### Direct Use
<!-- This section describes suitable use cases for the dataset. -->
[More Information Needed]
### Out-of-Scope Use
<!-- This section addresses misuse, malicious use, and uses that the dataset will not work well for. -->
[More Information Needed]
## Dataset Structure
<!-- This section provides a description of the dataset fields, and additional information about the dataset structure such as criteria used to create the splits, relationships between data points, etc. -->
[More Information Needed]
## Dataset Creation
### Curation Rationale
<!-- Motivation for the creation of this dataset. -->
[More Information Needed]
### Source Data
<!-- This section describes the source data (e.g. news text and headlines, social media posts, translated sentences, ...). -->
#### Data Collection and Processing
<!-- This section describes the data collection and processing process such as data selection criteria, filtering and normalization methods, tools and libraries used, etc. -->
[More Information Needed]
#### Who are the source data producers?
<!-- This section describes the people or systems who originally created the data. It should also include self-reported demographic or identity information for the source data creators if this information is available. -->
[More Information Needed]
### Annotations [optional]
<!-- If the dataset contains annotations which are not part of the initial data collection, use this section to describe them. -->
#### Annotation process
<!-- This section describes the annotation process such as annotation tools used in the process, the amount of data annotated, annotation guidelines provided to the annotators, interannotator statistics, annotation validation, etc. -->
[More Information Needed]
#### Who are the annotators?
<!-- This section describes the people or systems who created the annotations. -->
[More Information Needed]
#### Personal and Sensitive Information
<!-- State whether the dataset contains data that might be considered personal, sensitive, or private (e.g., data that reveals addresses, uniquely identifiable names or aliases, racial or ethnic origins, sexual orientations, religious beliefs, political opinions, financial or health data, etc.). If efforts were made to anonymize the data, describe the anonymization process. -->
[More Information Needed]
## Bias, Risks, and Limitations
<!-- This section is meant to convey both technical and sociotechnical limitations. -->
[More Information Needed]
### Recommendations
<!-- This section is meant to convey recommendations with respect to the bias, risk, and technical limitations. -->
Users should be made aware of the risks, biases and limitations of the dataset. More information needed for further recommendations.
## Citation [optional]
<!-- If there is a paper or blog post introducing the dataset, the APA and Bibtex information for that should go in this section. -->
**BibTeX:**
[More Information Needed]
**APA:**
[More Information Needed]
## Glossary [optional]
<!-- If relevant, include terms and calculations in this section that can help readers understand the dataset or dataset card. -->
[More Information Needed]
## More Information [optional]
[More Information Needed]
## Dataset Card Authors [optional]
[More Information Needed]
## Dataset Card Contact
[More Information Needed] | open-llm-leaderboard/details_cookinai__CM-14 | [
"region:us"
] | 2024-01-10T15:42:24+00:00 | {"pretty_name": "Evaluation run of cookinai/CM-14", "dataset_summary": "Dataset automatically created during the evaluation run of model [cookinai/CM-14](https://huggingface.co/cookinai/CM-14) on the [Open LLM Leaderboard](https://huggingface.co/spaces/HuggingFaceH4/open_llm_leaderboard).\n\nThe dataset is composed of 63 configuration, each one coresponding to one of the evaluated task.\n\nThe dataset has been created from 1 run(s). Each run can be found as a specific split in each configuration, the split being named using the timestamp of the run.The \"train\" split is always pointing to the latest results.\n\nAn additional configuration \"results\" store all the aggregated results of the run (and is used to compute and display the aggregated metrics on the [Open LLM Leaderboard](https://huggingface.co/spaces/HuggingFaceH4/open_llm_leaderboard)).\n\nTo load the details from a run, you can for instance do the following:\n```python\nfrom datasets import load_dataset\ndata = load_dataset(\"open-llm-leaderboard/details_cookinai__CM-14\",\n\t\"harness_winogrande_5\",\n\tsplit=\"train\")\n```\n\n## Latest results\n\nThese are the [latest results from run 2024-01-10T15:39:56.317779](https://huggingface.co/datasets/open-llm-leaderboard/details_cookinai__CM-14/blob/main/results_2024-01-10T15-39-56.317779.json)(note that their might be results for other tasks in the repos if successive evals didn't cover the same tasks. You find each in the results and the \"latest\" split for each eval):\n\n```python\n{\n \"all\": {\n \"acc\": 0.6580185798671865,\n \"acc_stderr\": 0.03192368037476644,\n \"acc_norm\": 0.6580152943319993,\n \"acc_norm_stderr\": 0.03258224944124306,\n \"mc1\": 0.45532435740514077,\n \"mc1_stderr\": 0.017433490102538765,\n \"mc2\": 0.6190301844108673,\n \"mc2_stderr\": 0.015232563824973148\n },\n \"harness|arc:challenge|25\": {\n \"acc\": 0.659556313993174,\n \"acc_stderr\": 0.013847460518892978,\n \"acc_norm\": 0.6936860068259386,\n \"acc_norm_stderr\": 0.013470584417276513\n },\n \"harness|hellaswag|10\": {\n \"acc\": 0.6870145389364668,\n \"acc_stderr\": 0.004627607991626914,\n \"acc_norm\": 0.8697470623381797,\n \"acc_norm_stderr\": 0.003358936279867257\n },\n \"harness|hendrycksTest-abstract_algebra|5\": {\n \"acc\": 0.38,\n \"acc_stderr\": 0.048783173121456316,\n \"acc_norm\": 0.38,\n \"acc_norm_stderr\": 0.048783173121456316\n },\n \"harness|hendrycksTest-anatomy|5\": {\n \"acc\": 0.6666666666666666,\n \"acc_stderr\": 0.04072314811876837,\n \"acc_norm\": 0.6666666666666666,\n \"acc_norm_stderr\": 0.04072314811876837\n },\n \"harness|hendrycksTest-astronomy|5\": {\n \"acc\": 0.6973684210526315,\n \"acc_stderr\": 0.037385206761196686,\n \"acc_norm\": 0.6973684210526315,\n \"acc_norm_stderr\": 0.037385206761196686\n },\n \"harness|hendrycksTest-business_ethics|5\": {\n \"acc\": 0.63,\n \"acc_stderr\": 0.04852365870939099,\n \"acc_norm\": 0.63,\n \"acc_norm_stderr\": 0.04852365870939099\n },\n \"harness|hendrycksTest-clinical_knowledge|5\": {\n \"acc\": 0.720754716981132,\n \"acc_stderr\": 0.027611163402399715,\n \"acc_norm\": 0.720754716981132,\n \"acc_norm_stderr\": 0.027611163402399715\n },\n \"harness|hendrycksTest-college_biology|5\": {\n \"acc\": 0.7777777777777778,\n \"acc_stderr\": 0.03476590104304134,\n \"acc_norm\": 0.7777777777777778,\n \"acc_norm_stderr\": 0.03476590104304134\n },\n \"harness|hendrycksTest-college_chemistry|5\": {\n \"acc\": 0.46,\n \"acc_stderr\": 0.05009082659620333,\n \"acc_norm\": 0.46,\n \"acc_norm_stderr\": 0.05009082659620333\n },\n \"harness|hendrycksTest-college_computer_science|5\": {\n \"acc\": 0.55,\n \"acc_stderr\": 0.05,\n \"acc_norm\": 0.55,\n \"acc_norm_stderr\": 0.05\n },\n \"harness|hendrycksTest-college_mathematics|5\": {\n \"acc\": 0.29,\n \"acc_stderr\": 0.04560480215720684,\n \"acc_norm\": 0.29,\n \"acc_norm_stderr\": 0.04560480215720684\n },\n \"harness|hendrycksTest-college_medicine|5\": {\n \"acc\": 0.6705202312138728,\n \"acc_stderr\": 0.03583901754736412,\n \"acc_norm\": 0.6705202312138728,\n \"acc_norm_stderr\": 0.03583901754736412\n },\n \"harness|hendrycksTest-college_physics|5\": {\n \"acc\": 0.45098039215686275,\n \"acc_stderr\": 0.049512182523962625,\n \"acc_norm\": 0.45098039215686275,\n \"acc_norm_stderr\": 0.049512182523962625\n },\n \"harness|hendrycksTest-computer_security|5\": {\n \"acc\": 0.77,\n \"acc_stderr\": 0.04229525846816508,\n \"acc_norm\": 0.77,\n \"acc_norm_stderr\": 0.04229525846816508\n },\n \"harness|hendrycksTest-conceptual_physics|5\": {\n \"acc\": 0.5872340425531914,\n \"acc_stderr\": 0.03218471141400351,\n \"acc_norm\": 0.5872340425531914,\n \"acc_norm_stderr\": 0.03218471141400351\n },\n \"harness|hendrycksTest-econometrics|5\": {\n \"acc\": 0.4824561403508772,\n \"acc_stderr\": 0.04700708033551038,\n \"acc_norm\": 0.4824561403508772,\n \"acc_norm_stderr\": 0.04700708033551038\n },\n \"harness|hendrycksTest-electrical_engineering|5\": {\n \"acc\": 0.5655172413793104,\n \"acc_stderr\": 0.04130740879555498,\n \"acc_norm\": 0.5655172413793104,\n \"acc_norm_stderr\": 0.04130740879555498\n },\n \"harness|hendrycksTest-elementary_mathematics|5\": {\n \"acc\": 0.42328042328042326,\n \"acc_stderr\": 0.025446365634406783,\n \"acc_norm\": 0.42328042328042326,\n \"acc_norm_stderr\": 0.025446365634406783\n },\n \"harness|hendrycksTest-formal_logic|5\": {\n \"acc\": 0.48412698412698413,\n \"acc_stderr\": 0.04469881854072606,\n \"acc_norm\": 0.48412698412698413,\n \"acc_norm_stderr\": 0.04469881854072606\n },\n \"harness|hendrycksTest-global_facts|5\": {\n \"acc\": 0.34,\n \"acc_stderr\": 0.04760952285695235,\n \"acc_norm\": 0.34,\n \"acc_norm_stderr\": 0.04760952285695235\n },\n \"harness|hendrycksTest-high_school_biology|5\": {\n \"acc\": 0.7870967741935484,\n \"acc_stderr\": 0.023287665127268552,\n \"acc_norm\": 0.7870967741935484,\n \"acc_norm_stderr\": 0.023287665127268552\n },\n \"harness|hendrycksTest-high_school_chemistry|5\": {\n \"acc\": 0.49261083743842365,\n \"acc_stderr\": 0.035176035403610084,\n \"acc_norm\": 0.49261083743842365,\n \"acc_norm_stderr\": 0.035176035403610084\n },\n \"harness|hendrycksTest-high_school_computer_science|5\": {\n \"acc\": 0.72,\n \"acc_stderr\": 0.04512608598542127,\n \"acc_norm\": 0.72,\n \"acc_norm_stderr\": 0.04512608598542127\n },\n \"harness|hendrycksTest-high_school_european_history|5\": {\n \"acc\": 0.7818181818181819,\n \"acc_stderr\": 0.03225078108306289,\n \"acc_norm\": 0.7818181818181819,\n \"acc_norm_stderr\": 0.03225078108306289\n },\n \"harness|hendrycksTest-high_school_geography|5\": {\n \"acc\": 0.7878787878787878,\n \"acc_stderr\": 0.029126522834586815,\n \"acc_norm\": 0.7878787878787878,\n \"acc_norm_stderr\": 0.029126522834586815\n },\n \"harness|hendrycksTest-high_school_government_and_politics|5\": {\n \"acc\": 0.8963730569948186,\n \"acc_stderr\": 0.02199531196364424,\n \"acc_norm\": 0.8963730569948186,\n \"acc_norm_stderr\": 0.02199531196364424\n },\n \"harness|hendrycksTest-high_school_macroeconomics|5\": {\n \"acc\": 0.6717948717948717,\n \"acc_stderr\": 0.023807633198657266,\n \"acc_norm\": 0.6717948717948717,\n \"acc_norm_stderr\": 0.023807633198657266\n },\n \"harness|hendrycksTest-high_school_mathematics|5\": {\n \"acc\": 0.337037037037037,\n \"acc_stderr\": 0.02882088466625326,\n \"acc_norm\": 0.337037037037037,\n \"acc_norm_stderr\": 0.02882088466625326\n },\n \"harness|hendrycksTest-high_school_microeconomics|5\": {\n \"acc\": 0.6890756302521008,\n \"acc_stderr\": 0.03006676158297793,\n \"acc_norm\": 0.6890756302521008,\n \"acc_norm_stderr\": 0.03006676158297793\n },\n \"harness|hendrycksTest-high_school_physics|5\": {\n \"acc\": 0.3509933774834437,\n \"acc_stderr\": 0.03896981964257375,\n \"acc_norm\": 0.3509933774834437,\n \"acc_norm_stderr\": 0.03896981964257375\n },\n \"harness|hendrycksTest-high_school_psychology|5\": {\n \"acc\": 0.8513761467889909,\n \"acc_stderr\": 0.015251253773660836,\n \"acc_norm\": 0.8513761467889909,\n \"acc_norm_stderr\": 0.015251253773660836\n },\n \"harness|hendrycksTest-high_school_statistics|5\": {\n \"acc\": 0.5277777777777778,\n \"acc_stderr\": 0.0340470532865388,\n \"acc_norm\": 0.5277777777777778,\n \"acc_norm_stderr\": 0.0340470532865388\n },\n \"harness|hendrycksTest-high_school_us_history|5\": {\n \"acc\": 0.8382352941176471,\n \"acc_stderr\": 0.02584501798692692,\n \"acc_norm\": 0.8382352941176471,\n \"acc_norm_stderr\": 0.02584501798692692\n },\n \"harness|hendrycksTest-high_school_world_history|5\": {\n \"acc\": 0.810126582278481,\n \"acc_stderr\": 0.025530100460233494,\n \"acc_norm\": 0.810126582278481,\n \"acc_norm_stderr\": 0.025530100460233494\n },\n \"harness|hendrycksTest-human_aging|5\": {\n \"acc\": 0.6905829596412556,\n \"acc_stderr\": 0.031024411740572213,\n \"acc_norm\": 0.6905829596412556,\n \"acc_norm_stderr\": 0.031024411740572213\n },\n \"harness|hendrycksTest-human_sexuality|5\": {\n \"acc\": 0.8091603053435115,\n \"acc_stderr\": 0.034465133507525995,\n \"acc_norm\": 0.8091603053435115,\n \"acc_norm_stderr\": 0.034465133507525995\n },\n \"harness|hendrycksTest-international_law|5\": {\n \"acc\": 0.8016528925619835,\n \"acc_stderr\": 0.03640118271990947,\n \"acc_norm\": 0.8016528925619835,\n \"acc_norm_stderr\": 0.03640118271990947\n },\n \"harness|hendrycksTest-jurisprudence|5\": {\n \"acc\": 0.7962962962962963,\n \"acc_stderr\": 0.03893542518824847,\n \"acc_norm\": 0.7962962962962963,\n \"acc_norm_stderr\": 0.03893542518824847\n },\n \"harness|hendrycksTest-logical_fallacies|5\": {\n \"acc\": 0.7852760736196319,\n \"acc_stderr\": 0.03226219377286775,\n \"acc_norm\": 0.7852760736196319,\n \"acc_norm_stderr\": 0.03226219377286775\n },\n \"harness|hendrycksTest-machine_learning|5\": {\n \"acc\": 0.4642857142857143,\n \"acc_stderr\": 0.04733667890053756,\n \"acc_norm\": 0.4642857142857143,\n \"acc_norm_stderr\": 0.04733667890053756\n },\n \"harness|hendrycksTest-management|5\": {\n \"acc\": 0.7766990291262136,\n \"acc_stderr\": 0.04123553189891431,\n \"acc_norm\": 0.7766990291262136,\n \"acc_norm_stderr\": 0.04123553189891431\n },\n \"harness|hendrycksTest-marketing|5\": {\n \"acc\": 0.8803418803418803,\n \"acc_stderr\": 0.021262719400406964,\n \"acc_norm\": 0.8803418803418803,\n \"acc_norm_stderr\": 0.021262719400406964\n },\n \"harness|hendrycksTest-medical_genetics|5\": {\n \"acc\": 0.72,\n \"acc_stderr\": 0.045126085985421276,\n \"acc_norm\": 0.72,\n \"acc_norm_stderr\": 0.045126085985421276\n },\n \"harness|hendrycksTest-miscellaneous|5\": {\n \"acc\": 0.8352490421455939,\n \"acc_stderr\": 0.013265346261323788,\n \"acc_norm\": 0.8352490421455939,\n \"acc_norm_stderr\": 0.013265346261323788\n },\n \"harness|hendrycksTest-moral_disputes|5\": {\n \"acc\": 0.7514450867052023,\n \"acc_stderr\": 0.023267528432100174,\n \"acc_norm\": 0.7514450867052023,\n \"acc_norm_stderr\": 0.023267528432100174\n },\n \"harness|hendrycksTest-moral_scenarios|5\": {\n \"acc\": 0.43687150837988825,\n \"acc_stderr\": 0.01658868086453063,\n \"acc_norm\": 0.43687150837988825,\n \"acc_norm_stderr\": 0.01658868086453063\n },\n \"harness|hendrycksTest-nutrition|5\": {\n \"acc\": 0.7320261437908496,\n \"acc_stderr\": 0.025360603796242553,\n \"acc_norm\": 0.7320261437908496,\n \"acc_norm_stderr\": 0.025360603796242553\n },\n \"harness|hendrycksTest-philosophy|5\": {\n \"acc\": 0.7106109324758842,\n \"acc_stderr\": 0.025755865922632945,\n \"acc_norm\": 0.7106109324758842,\n \"acc_norm_stderr\": 0.025755865922632945\n },\n \"harness|hendrycksTest-prehistory|5\": {\n \"acc\": 0.75,\n \"acc_stderr\": 0.02409347123262133,\n \"acc_norm\": 0.75,\n \"acc_norm_stderr\": 0.02409347123262133\n },\n \"harness|hendrycksTest-professional_accounting|5\": {\n \"acc\": 0.4929078014184397,\n \"acc_stderr\": 0.02982449855912901,\n \"acc_norm\": 0.4929078014184397,\n \"acc_norm_stderr\": 0.02982449855912901\n },\n \"harness|hendrycksTest-professional_law|5\": {\n \"acc\": 0.4641460234680574,\n \"acc_stderr\": 0.012737361318730581,\n \"acc_norm\": 0.4641460234680574,\n \"acc_norm_stderr\": 0.012737361318730581\n },\n \"harness|hendrycksTest-professional_medicine|5\": {\n \"acc\": 0.6948529411764706,\n \"acc_stderr\": 0.0279715413701706,\n \"acc_norm\": 0.6948529411764706,\n \"acc_norm_stderr\": 0.0279715413701706\n },\n \"harness|hendrycksTest-professional_psychology|5\": {\n \"acc\": 0.6928104575163399,\n \"acc_stderr\": 0.01866335967146367,\n \"acc_norm\": 0.6928104575163399,\n \"acc_norm_stderr\": 0.01866335967146367\n },\n \"harness|hendrycksTest-public_relations|5\": {\n \"acc\": 0.6727272727272727,\n \"acc_stderr\": 0.0449429086625209,\n \"acc_norm\": 0.6727272727272727,\n \"acc_norm_stderr\": 0.0449429086625209\n },\n \"harness|hendrycksTest-security_studies|5\": {\n \"acc\": 0.726530612244898,\n \"acc_stderr\": 0.028535560337128445,\n \"acc_norm\": 0.726530612244898,\n \"acc_norm_stderr\": 0.028535560337128445\n },\n \"harness|hendrycksTest-sociology|5\": {\n \"acc\": 0.835820895522388,\n \"acc_stderr\": 0.026193923544454132,\n \"acc_norm\": 0.835820895522388,\n \"acc_norm_stderr\": 0.026193923544454132\n },\n \"harness|hendrycksTest-us_foreign_policy|5\": {\n \"acc\": 0.86,\n \"acc_stderr\": 0.03487350880197769,\n \"acc_norm\": 0.86,\n \"acc_norm_stderr\": 0.03487350880197769\n },\n \"harness|hendrycksTest-virology|5\": {\n \"acc\": 0.5481927710843374,\n \"acc_stderr\": 0.03874371556587953,\n \"acc_norm\": 0.5481927710843374,\n \"acc_norm_stderr\": 0.03874371556587953\n },\n \"harness|hendrycksTest-world_religions|5\": {\n \"acc\": 0.8421052631578947,\n \"acc_stderr\": 0.027966785859160893,\n \"acc_norm\": 0.8421052631578947,\n \"acc_norm_stderr\": 0.027966785859160893\n },\n \"harness|truthfulqa:mc|0\": {\n \"mc1\": 0.45532435740514077,\n \"mc1_stderr\": 0.017433490102538765,\n \"mc2\": 0.6190301844108673,\n \"mc2_stderr\": 0.015232563824973148\n },\n \"harness|winogrande|5\": {\n \"acc\": 0.8105761641673244,\n \"acc_stderr\": 0.011012790432989245\n },\n \"harness|gsm8k|5\": {\n \"acc\": 0.7225170583775588,\n \"acc_stderr\": 0.012333447581047539\n }\n}\n```", "repo_url": "https://huggingface.co/cookinai/CM-14", "leaderboard_url": "https://huggingface.co/spaces/HuggingFaceH4/open_llm_leaderboard", "point_of_contact": "[email protected]", "configs": [{"config_name": "harness_arc_challenge_25", "data_files": [{"split": "2024_01_10T15_39_56.317779", "path": ["**/details_harness|arc:challenge|25_2024-01-10T15-39-56.317779.parquet"]}, {"split": "latest", "path": ["**/details_harness|arc:challenge|25_2024-01-10T15-39-56.317779.parquet"]}]}, {"config_name": "harness_gsm8k_5", "data_files": [{"split": "2024_01_10T15_39_56.317779", "path": ["**/details_harness|gsm8k|5_2024-01-10T15-39-56.317779.parquet"]}, {"split": "latest", "path": ["**/details_harness|gsm8k|5_2024-01-10T15-39-56.317779.parquet"]}]}, {"config_name": "harness_hellaswag_10", "data_files": [{"split": "2024_01_10T15_39_56.317779", "path": ["**/details_harness|hellaswag|10_2024-01-10T15-39-56.317779.parquet"]}, {"split": "latest", "path": ["**/details_harness|hellaswag|10_2024-01-10T15-39-56.317779.parquet"]}]}, {"config_name": "harness_hendrycksTest_5", "data_files": [{"split": "2024_01_10T15_39_56.317779", "path": ["**/details_harness|hendrycksTest-abstract_algebra|5_2024-01-10T15-39-56.317779.parquet", "**/details_harness|hendrycksTest-anatomy|5_2024-01-10T15-39-56.317779.parquet", "**/details_harness|hendrycksTest-astronomy|5_2024-01-10T15-39-56.317779.parquet", "**/details_harness|hendrycksTest-business_ethics|5_2024-01-10T15-39-56.317779.parquet", "**/details_harness|hendrycksTest-clinical_knowledge|5_2024-01-10T15-39-56.317779.parquet", "**/details_harness|hendrycksTest-college_biology|5_2024-01-10T15-39-56.317779.parquet", "**/details_harness|hendrycksTest-college_chemistry|5_2024-01-10T15-39-56.317779.parquet", "**/details_harness|hendrycksTest-college_computer_science|5_2024-01-10T15-39-56.317779.parquet", "**/details_harness|hendrycksTest-college_mathematics|5_2024-01-10T15-39-56.317779.parquet", "**/details_harness|hendrycksTest-college_medicine|5_2024-01-10T15-39-56.317779.parquet", "**/details_harness|hendrycksTest-college_physics|5_2024-01-10T15-39-56.317779.parquet", "**/details_harness|hendrycksTest-computer_security|5_2024-01-10T15-39-56.317779.parquet", "**/details_harness|hendrycksTest-conceptual_physics|5_2024-01-10T15-39-56.317779.parquet", "**/details_harness|hendrycksTest-econometrics|5_2024-01-10T15-39-56.317779.parquet", "**/details_harness|hendrycksTest-electrical_engineering|5_2024-01-10T15-39-56.317779.parquet", "**/details_harness|hendrycksTest-elementary_mathematics|5_2024-01-10T15-39-56.317779.parquet", "**/details_harness|hendrycksTest-formal_logic|5_2024-01-10T15-39-56.317779.parquet", "**/details_harness|hendrycksTest-global_facts|5_2024-01-10T15-39-56.317779.parquet", "**/details_harness|hendrycksTest-high_school_biology|5_2024-01-10T15-39-56.317779.parquet", "**/details_harness|hendrycksTest-high_school_chemistry|5_2024-01-10T15-39-56.317779.parquet", "**/details_harness|hendrycksTest-high_school_computer_science|5_2024-01-10T15-39-56.317779.parquet", "**/details_harness|hendrycksTest-high_school_european_history|5_2024-01-10T15-39-56.317779.parquet", "**/details_harness|hendrycksTest-high_school_geography|5_2024-01-10T15-39-56.317779.parquet", "**/details_harness|hendrycksTest-high_school_government_and_politics|5_2024-01-10T15-39-56.317779.parquet", "**/details_harness|hendrycksTest-high_school_macroeconomics|5_2024-01-10T15-39-56.317779.parquet", "**/details_harness|hendrycksTest-high_school_mathematics|5_2024-01-10T15-39-56.317779.parquet", "**/details_harness|hendrycksTest-high_school_microeconomics|5_2024-01-10T15-39-56.317779.parquet", "**/details_harness|hendrycksTest-high_school_physics|5_2024-01-10T15-39-56.317779.parquet", "**/details_harness|hendrycksTest-high_school_psychology|5_2024-01-10T15-39-56.317779.parquet", "**/details_harness|hendrycksTest-high_school_statistics|5_2024-01-10T15-39-56.317779.parquet", "**/details_harness|hendrycksTest-high_school_us_history|5_2024-01-10T15-39-56.317779.parquet", "**/details_harness|hendrycksTest-high_school_world_history|5_2024-01-10T15-39-56.317779.parquet", "**/details_harness|hendrycksTest-human_aging|5_2024-01-10T15-39-56.317779.parquet", "**/details_harness|hendrycksTest-human_sexuality|5_2024-01-10T15-39-56.317779.parquet", "**/details_harness|hendrycksTest-international_law|5_2024-01-10T15-39-56.317779.parquet", "**/details_harness|hendrycksTest-jurisprudence|5_2024-01-10T15-39-56.317779.parquet", "**/details_harness|hendrycksTest-logical_fallacies|5_2024-01-10T15-39-56.317779.parquet", "**/details_harness|hendrycksTest-machine_learning|5_2024-01-10T15-39-56.317779.parquet", "**/details_harness|hendrycksTest-management|5_2024-01-10T15-39-56.317779.parquet", "**/details_harness|hendrycksTest-marketing|5_2024-01-10T15-39-56.317779.parquet", "**/details_harness|hendrycksTest-medical_genetics|5_2024-01-10T15-39-56.317779.parquet", "**/details_harness|hendrycksTest-miscellaneous|5_2024-01-10T15-39-56.317779.parquet", "**/details_harness|hendrycksTest-moral_disputes|5_2024-01-10T15-39-56.317779.parquet", "**/details_harness|hendrycksTest-moral_scenarios|5_2024-01-10T15-39-56.317779.parquet", "**/details_harness|hendrycksTest-nutrition|5_2024-01-10T15-39-56.317779.parquet", "**/details_harness|hendrycksTest-philosophy|5_2024-01-10T15-39-56.317779.parquet", "**/details_harness|hendrycksTest-prehistory|5_2024-01-10T15-39-56.317779.parquet", "**/details_harness|hendrycksTest-professional_accounting|5_2024-01-10T15-39-56.317779.parquet", "**/details_harness|hendrycksTest-professional_law|5_2024-01-10T15-39-56.317779.parquet", "**/details_harness|hendrycksTest-professional_medicine|5_2024-01-10T15-39-56.317779.parquet", "**/details_harness|hendrycksTest-professional_psychology|5_2024-01-10T15-39-56.317779.parquet", "**/details_harness|hendrycksTest-public_relations|5_2024-01-10T15-39-56.317779.parquet", "**/details_harness|hendrycksTest-security_studies|5_2024-01-10T15-39-56.317779.parquet", "**/details_harness|hendrycksTest-sociology|5_2024-01-10T15-39-56.317779.parquet", "**/details_harness|hendrycksTest-us_foreign_policy|5_2024-01-10T15-39-56.317779.parquet", "**/details_harness|hendrycksTest-virology|5_2024-01-10T15-39-56.317779.parquet", "**/details_harness|hendrycksTest-world_religions|5_2024-01-10T15-39-56.317779.parquet"]}, {"split": "latest", "path": ["**/details_harness|hendrycksTest-abstract_algebra|5_2024-01-10T15-39-56.317779.parquet", "**/details_harness|hendrycksTest-anatomy|5_2024-01-10T15-39-56.317779.parquet", "**/details_harness|hendrycksTest-astronomy|5_2024-01-10T15-39-56.317779.parquet", "**/details_harness|hendrycksTest-business_ethics|5_2024-01-10T15-39-56.317779.parquet", "**/details_harness|hendrycksTest-clinical_knowledge|5_2024-01-10T15-39-56.317779.parquet", "**/details_harness|hendrycksTest-college_biology|5_2024-01-10T15-39-56.317779.parquet", "**/details_harness|hendrycksTest-college_chemistry|5_2024-01-10T15-39-56.317779.parquet", "**/details_harness|hendrycksTest-college_computer_science|5_2024-01-10T15-39-56.317779.parquet", "**/details_harness|hendrycksTest-college_mathematics|5_2024-01-10T15-39-56.317779.parquet", "**/details_harness|hendrycksTest-college_medicine|5_2024-01-10T15-39-56.317779.parquet", "**/details_harness|hendrycksTest-college_physics|5_2024-01-10T15-39-56.317779.parquet", "**/details_harness|hendrycksTest-computer_security|5_2024-01-10T15-39-56.317779.parquet", "**/details_harness|hendrycksTest-conceptual_physics|5_2024-01-10T15-39-56.317779.parquet", "**/details_harness|hendrycksTest-econometrics|5_2024-01-10T15-39-56.317779.parquet", "**/details_harness|hendrycksTest-electrical_engineering|5_2024-01-10T15-39-56.317779.parquet", "**/details_harness|hendrycksTest-elementary_mathematics|5_2024-01-10T15-39-56.317779.parquet", "**/details_harness|hendrycksTest-formal_logic|5_2024-01-10T15-39-56.317779.parquet", "**/details_harness|hendrycksTest-global_facts|5_2024-01-10T15-39-56.317779.parquet", "**/details_harness|hendrycksTest-high_school_biology|5_2024-01-10T15-39-56.317779.parquet", "**/details_harness|hendrycksTest-high_school_chemistry|5_2024-01-10T15-39-56.317779.parquet", "**/details_harness|hendrycksTest-high_school_computer_science|5_2024-01-10T15-39-56.317779.parquet", "**/details_harness|hendrycksTest-high_school_european_history|5_2024-01-10T15-39-56.317779.parquet", "**/details_harness|hendrycksTest-high_school_geography|5_2024-01-10T15-39-56.317779.parquet", "**/details_harness|hendrycksTest-high_school_government_and_politics|5_2024-01-10T15-39-56.317779.parquet", "**/details_harness|hendrycksTest-high_school_macroeconomics|5_2024-01-10T15-39-56.317779.parquet", "**/details_harness|hendrycksTest-high_school_mathematics|5_2024-01-10T15-39-56.317779.parquet", "**/details_harness|hendrycksTest-high_school_microeconomics|5_2024-01-10T15-39-56.317779.parquet", "**/details_harness|hendrycksTest-high_school_physics|5_2024-01-10T15-39-56.317779.parquet", "**/details_harness|hendrycksTest-high_school_psychology|5_2024-01-10T15-39-56.317779.parquet", "**/details_harness|hendrycksTest-high_school_statistics|5_2024-01-10T15-39-56.317779.parquet", "**/details_harness|hendrycksTest-high_school_us_history|5_2024-01-10T15-39-56.317779.parquet", "**/details_harness|hendrycksTest-high_school_world_history|5_2024-01-10T15-39-56.317779.parquet", "**/details_harness|hendrycksTest-human_aging|5_2024-01-10T15-39-56.317779.parquet", "**/details_harness|hendrycksTest-human_sexuality|5_2024-01-10T15-39-56.317779.parquet", "**/details_harness|hendrycksTest-international_law|5_2024-01-10T15-39-56.317779.parquet", "**/details_harness|hendrycksTest-jurisprudence|5_2024-01-10T15-39-56.317779.parquet", "**/details_harness|hendrycksTest-logical_fallacies|5_2024-01-10T15-39-56.317779.parquet", "**/details_harness|hendrycksTest-machine_learning|5_2024-01-10T15-39-56.317779.parquet", "**/details_harness|hendrycksTest-management|5_2024-01-10T15-39-56.317779.parquet", "**/details_harness|hendrycksTest-marketing|5_2024-01-10T15-39-56.317779.parquet", "**/details_harness|hendrycksTest-medical_genetics|5_2024-01-10T15-39-56.317779.parquet", "**/details_harness|hendrycksTest-miscellaneous|5_2024-01-10T15-39-56.317779.parquet", "**/details_harness|hendrycksTest-moral_disputes|5_2024-01-10T15-39-56.317779.parquet", "**/details_harness|hendrycksTest-moral_scenarios|5_2024-01-10T15-39-56.317779.parquet", "**/details_harness|hendrycksTest-nutrition|5_2024-01-10T15-39-56.317779.parquet", "**/details_harness|hendrycksTest-philosophy|5_2024-01-10T15-39-56.317779.parquet", "**/details_harness|hendrycksTest-prehistory|5_2024-01-10T15-39-56.317779.parquet", "**/details_harness|hendrycksTest-professional_accounting|5_2024-01-10T15-39-56.317779.parquet", "**/details_harness|hendrycksTest-professional_law|5_2024-01-10T15-39-56.317779.parquet", "**/details_harness|hendrycksTest-professional_medicine|5_2024-01-10T15-39-56.317779.parquet", "**/details_harness|hendrycksTest-professional_psychology|5_2024-01-10T15-39-56.317779.parquet", "**/details_harness|hendrycksTest-public_relations|5_2024-01-10T15-39-56.317779.parquet", "**/details_harness|hendrycksTest-security_studies|5_2024-01-10T15-39-56.317779.parquet", "**/details_harness|hendrycksTest-sociology|5_2024-01-10T15-39-56.317779.parquet", "**/details_harness|hendrycksTest-us_foreign_policy|5_2024-01-10T15-39-56.317779.parquet", "**/details_harness|hendrycksTest-virology|5_2024-01-10T15-39-56.317779.parquet", "**/details_harness|hendrycksTest-world_religions|5_2024-01-10T15-39-56.317779.parquet"]}]}, {"config_name": "harness_hendrycksTest_abstract_algebra_5", "data_files": [{"split": "2024_01_10T15_39_56.317779", "path": ["**/details_harness|hendrycksTest-abstract_algebra|5_2024-01-10T15-39-56.317779.parquet"]}, {"split": "latest", "path": ["**/details_harness|hendrycksTest-abstract_algebra|5_2024-01-10T15-39-56.317779.parquet"]}]}, {"config_name": "harness_hendrycksTest_anatomy_5", "data_files": [{"split": "2024_01_10T15_39_56.317779", "path": ["**/details_harness|hendrycksTest-anatomy|5_2024-01-10T15-39-56.317779.parquet"]}, {"split": "latest", "path": ["**/details_harness|hendrycksTest-anatomy|5_2024-01-10T15-39-56.317779.parquet"]}]}, {"config_name": "harness_hendrycksTest_astronomy_5", "data_files": [{"split": "2024_01_10T15_39_56.317779", "path": ["**/details_harness|hendrycksTest-astronomy|5_2024-01-10T15-39-56.317779.parquet"]}, {"split": "latest", "path": ["**/details_harness|hendrycksTest-astronomy|5_2024-01-10T15-39-56.317779.parquet"]}]}, {"config_name": "harness_hendrycksTest_business_ethics_5", "data_files": [{"split": "2024_01_10T15_39_56.317779", "path": ["**/details_harness|hendrycksTest-business_ethics|5_2024-01-10T15-39-56.317779.parquet"]}, {"split": "latest", "path": ["**/details_harness|hendrycksTest-business_ethics|5_2024-01-10T15-39-56.317779.parquet"]}]}, {"config_name": "harness_hendrycksTest_clinical_knowledge_5", "data_files": [{"split": "2024_01_10T15_39_56.317779", "path": ["**/details_harness|hendrycksTest-clinical_knowledge|5_2024-01-10T15-39-56.317779.parquet"]}, {"split": "latest", "path": ["**/details_harness|hendrycksTest-clinical_knowledge|5_2024-01-10T15-39-56.317779.parquet"]}]}, {"config_name": "harness_hendrycksTest_college_biology_5", "data_files": [{"split": "2024_01_10T15_39_56.317779", "path": ["**/details_harness|hendrycksTest-college_biology|5_2024-01-10T15-39-56.317779.parquet"]}, {"split": "latest", "path": ["**/details_harness|hendrycksTest-college_biology|5_2024-01-10T15-39-56.317779.parquet"]}]}, {"config_name": "harness_hendrycksTest_college_chemistry_5", "data_files": [{"split": "2024_01_10T15_39_56.317779", "path": ["**/details_harness|hendrycksTest-college_chemistry|5_2024-01-10T15-39-56.317779.parquet"]}, {"split": "latest", "path": ["**/details_harness|hendrycksTest-college_chemistry|5_2024-01-10T15-39-56.317779.parquet"]}]}, {"config_name": "harness_hendrycksTest_college_computer_science_5", "data_files": [{"split": "2024_01_10T15_39_56.317779", "path": ["**/details_harness|hendrycksTest-college_computer_science|5_2024-01-10T15-39-56.317779.parquet"]}, {"split": "latest", "path": ["**/details_harness|hendrycksTest-college_computer_science|5_2024-01-10T15-39-56.317779.parquet"]}]}, {"config_name": "harness_hendrycksTest_college_mathematics_5", "data_files": [{"split": "2024_01_10T15_39_56.317779", "path": ["**/details_harness|hendrycksTest-college_mathematics|5_2024-01-10T15-39-56.317779.parquet"]}, {"split": "latest", "path": ["**/details_harness|hendrycksTest-college_mathematics|5_2024-01-10T15-39-56.317779.parquet"]}]}, {"config_name": "harness_hendrycksTest_college_medicine_5", "data_files": [{"split": "2024_01_10T15_39_56.317779", "path": ["**/details_harness|hendrycksTest-college_medicine|5_2024-01-10T15-39-56.317779.parquet"]}, {"split": "latest", "path": ["**/details_harness|hendrycksTest-college_medicine|5_2024-01-10T15-39-56.317779.parquet"]}]}, {"config_name": "harness_hendrycksTest_college_physics_5", "data_files": [{"split": "2024_01_10T15_39_56.317779", "path": ["**/details_harness|hendrycksTest-college_physics|5_2024-01-10T15-39-56.317779.parquet"]}, {"split": "latest", "path": ["**/details_harness|hendrycksTest-college_physics|5_2024-01-10T15-39-56.317779.parquet"]}]}, {"config_name": "harness_hendrycksTest_computer_security_5", "data_files": [{"split": "2024_01_10T15_39_56.317779", "path": ["**/details_harness|hendrycksTest-computer_security|5_2024-01-10T15-39-56.317779.parquet"]}, {"split": "latest", "path": ["**/details_harness|hendrycksTest-computer_security|5_2024-01-10T15-39-56.317779.parquet"]}]}, {"config_name": "harness_hendrycksTest_conceptual_physics_5", "data_files": [{"split": "2024_01_10T15_39_56.317779", "path": ["**/details_harness|hendrycksTest-conceptual_physics|5_2024-01-10T15-39-56.317779.parquet"]}, {"split": "latest", "path": ["**/details_harness|hendrycksTest-conceptual_physics|5_2024-01-10T15-39-56.317779.parquet"]}]}, {"config_name": "harness_hendrycksTest_econometrics_5", "data_files": [{"split": "2024_01_10T15_39_56.317779", "path": ["**/details_harness|hendrycksTest-econometrics|5_2024-01-10T15-39-56.317779.parquet"]}, {"split": "latest", "path": ["**/details_harness|hendrycksTest-econometrics|5_2024-01-10T15-39-56.317779.parquet"]}]}, {"config_name": "harness_hendrycksTest_electrical_engineering_5", "data_files": [{"split": "2024_01_10T15_39_56.317779", "path": ["**/details_harness|hendrycksTest-electrical_engineering|5_2024-01-10T15-39-56.317779.parquet"]}, {"split": "latest", "path": ["**/details_harness|hendrycksTest-electrical_engineering|5_2024-01-10T15-39-56.317779.parquet"]}]}, {"config_name": "harness_hendrycksTest_elementary_mathematics_5", "data_files": [{"split": "2024_01_10T15_39_56.317779", "path": ["**/details_harness|hendrycksTest-elementary_mathematics|5_2024-01-10T15-39-56.317779.parquet"]}, {"split": "latest", "path": ["**/details_harness|hendrycksTest-elementary_mathematics|5_2024-01-10T15-39-56.317779.parquet"]}]}, {"config_name": "harness_hendrycksTest_formal_logic_5", "data_files": [{"split": "2024_01_10T15_39_56.317779", "path": ["**/details_harness|hendrycksTest-formal_logic|5_2024-01-10T15-39-56.317779.parquet"]}, {"split": "latest", "path": ["**/details_harness|hendrycksTest-formal_logic|5_2024-01-10T15-39-56.317779.parquet"]}]}, {"config_name": "harness_hendrycksTest_global_facts_5", "data_files": [{"split": "2024_01_10T15_39_56.317779", "path": ["**/details_harness|hendrycksTest-global_facts|5_2024-01-10T15-39-56.317779.parquet"]}, {"split": "latest", "path": ["**/details_harness|hendrycksTest-global_facts|5_2024-01-10T15-39-56.317779.parquet"]}]}, {"config_name": "harness_hendrycksTest_high_school_biology_5", "data_files": [{"split": "2024_01_10T15_39_56.317779", "path": ["**/details_harness|hendrycksTest-high_school_biology|5_2024-01-10T15-39-56.317779.parquet"]}, {"split": "latest", "path": ["**/details_harness|hendrycksTest-high_school_biology|5_2024-01-10T15-39-56.317779.parquet"]}]}, {"config_name": "harness_hendrycksTest_high_school_chemistry_5", "data_files": [{"split": "2024_01_10T15_39_56.317779", "path": ["**/details_harness|hendrycksTest-high_school_chemistry|5_2024-01-10T15-39-56.317779.parquet"]}, {"split": "latest", "path": ["**/details_harness|hendrycksTest-high_school_chemistry|5_2024-01-10T15-39-56.317779.parquet"]}]}, {"config_name": "harness_hendrycksTest_high_school_computer_science_5", "data_files": [{"split": "2024_01_10T15_39_56.317779", "path": ["**/details_harness|hendrycksTest-high_school_computer_science|5_2024-01-10T15-39-56.317779.parquet"]}, {"split": "latest", "path": ["**/details_harness|hendrycksTest-high_school_computer_science|5_2024-01-10T15-39-56.317779.parquet"]}]}, {"config_name": "harness_hendrycksTest_high_school_european_history_5", "data_files": [{"split": "2024_01_10T15_39_56.317779", "path": ["**/details_harness|hendrycksTest-high_school_european_history|5_2024-01-10T15-39-56.317779.parquet"]}, {"split": "latest", "path": ["**/details_harness|hendrycksTest-high_school_european_history|5_2024-01-10T15-39-56.317779.parquet"]}]}, {"config_name": "harness_hendrycksTest_high_school_geography_5", "data_files": [{"split": "2024_01_10T15_39_56.317779", "path": ["**/details_harness|hendrycksTest-high_school_geography|5_2024-01-10T15-39-56.317779.parquet"]}, {"split": "latest", "path": ["**/details_harness|hendrycksTest-high_school_geography|5_2024-01-10T15-39-56.317779.parquet"]}]}, {"config_name": "harness_hendrycksTest_high_school_government_and_politics_5", "data_files": [{"split": "2024_01_10T15_39_56.317779", "path": ["**/details_harness|hendrycksTest-high_school_government_and_politics|5_2024-01-10T15-39-56.317779.parquet"]}, {"split": "latest", "path": ["**/details_harness|hendrycksTest-high_school_government_and_politics|5_2024-01-10T15-39-56.317779.parquet"]}]}, {"config_name": "harness_hendrycksTest_high_school_macroeconomics_5", "data_files": [{"split": "2024_01_10T15_39_56.317779", "path": ["**/details_harness|hendrycksTest-high_school_macroeconomics|5_2024-01-10T15-39-56.317779.parquet"]}, {"split": "latest", "path": ["**/details_harness|hendrycksTest-high_school_macroeconomics|5_2024-01-10T15-39-56.317779.parquet"]}]}, {"config_name": "harness_hendrycksTest_high_school_mathematics_5", "data_files": [{"split": "2024_01_10T15_39_56.317779", "path": ["**/details_harness|hendrycksTest-high_school_mathematics|5_2024-01-10T15-39-56.317779.parquet"]}, {"split": "latest", "path": ["**/details_harness|hendrycksTest-high_school_mathematics|5_2024-01-10T15-39-56.317779.parquet"]}]}, {"config_name": "harness_hendrycksTest_high_school_microeconomics_5", "data_files": [{"split": "2024_01_10T15_39_56.317779", "path": ["**/details_harness|hendrycksTest-high_school_microeconomics|5_2024-01-10T15-39-56.317779.parquet"]}, {"split": "latest", "path": ["**/details_harness|hendrycksTest-high_school_microeconomics|5_2024-01-10T15-39-56.317779.parquet"]}]}, {"config_name": "harness_hendrycksTest_high_school_physics_5", "data_files": [{"split": "2024_01_10T15_39_56.317779", "path": ["**/details_harness|hendrycksTest-high_school_physics|5_2024-01-10T15-39-56.317779.parquet"]}, {"split": "latest", "path": ["**/details_harness|hendrycksTest-high_school_physics|5_2024-01-10T15-39-56.317779.parquet"]}]}, {"config_name": "harness_hendrycksTest_high_school_psychology_5", "data_files": [{"split": "2024_01_10T15_39_56.317779", "path": ["**/details_harness|hendrycksTest-high_school_psychology|5_2024-01-10T15-39-56.317779.parquet"]}, {"split": "latest", "path": ["**/details_harness|hendrycksTest-high_school_psychology|5_2024-01-10T15-39-56.317779.parquet"]}]}, {"config_name": "harness_hendrycksTest_high_school_statistics_5", "data_files": [{"split": "2024_01_10T15_39_56.317779", "path": ["**/details_harness|hendrycksTest-high_school_statistics|5_2024-01-10T15-39-56.317779.parquet"]}, {"split": "latest", "path": ["**/details_harness|hendrycksTest-high_school_statistics|5_2024-01-10T15-39-56.317779.parquet"]}]}, {"config_name": "harness_hendrycksTest_high_school_us_history_5", "data_files": [{"split": "2024_01_10T15_39_56.317779", "path": ["**/details_harness|hendrycksTest-high_school_us_history|5_2024-01-10T15-39-56.317779.parquet"]}, {"split": "latest", "path": ["**/details_harness|hendrycksTest-high_school_us_history|5_2024-01-10T15-39-56.317779.parquet"]}]}, {"config_name": "harness_hendrycksTest_high_school_world_history_5", "data_files": [{"split": "2024_01_10T15_39_56.317779", "path": ["**/details_harness|hendrycksTest-high_school_world_history|5_2024-01-10T15-39-56.317779.parquet"]}, {"split": "latest", "path": ["**/details_harness|hendrycksTest-high_school_world_history|5_2024-01-10T15-39-56.317779.parquet"]}]}, {"config_name": "harness_hendrycksTest_human_aging_5", "data_files": [{"split": "2024_01_10T15_39_56.317779", "path": ["**/details_harness|hendrycksTest-human_aging|5_2024-01-10T15-39-56.317779.parquet"]}, {"split": "latest", "path": ["**/details_harness|hendrycksTest-human_aging|5_2024-01-10T15-39-56.317779.parquet"]}]}, {"config_name": "harness_hendrycksTest_human_sexuality_5", "data_files": [{"split": "2024_01_10T15_39_56.317779", "path": ["**/details_harness|hendrycksTest-human_sexuality|5_2024-01-10T15-39-56.317779.parquet"]}, {"split": "latest", "path": ["**/details_harness|hendrycksTest-human_sexuality|5_2024-01-10T15-39-56.317779.parquet"]}]}, {"config_name": "harness_hendrycksTest_international_law_5", "data_files": [{"split": "2024_01_10T15_39_56.317779", "path": ["**/details_harness|hendrycksTest-international_law|5_2024-01-10T15-39-56.317779.parquet"]}, {"split": "latest", "path": ["**/details_harness|hendrycksTest-international_law|5_2024-01-10T15-39-56.317779.parquet"]}]}, {"config_name": "harness_hendrycksTest_jurisprudence_5", "data_files": [{"split": "2024_01_10T15_39_56.317779", "path": ["**/details_harness|hendrycksTest-jurisprudence|5_2024-01-10T15-39-56.317779.parquet"]}, {"split": "latest", "path": ["**/details_harness|hendrycksTest-jurisprudence|5_2024-01-10T15-39-56.317779.parquet"]}]}, {"config_name": "harness_hendrycksTest_logical_fallacies_5", "data_files": [{"split": "2024_01_10T15_39_56.317779", "path": ["**/details_harness|hendrycksTest-logical_fallacies|5_2024-01-10T15-39-56.317779.parquet"]}, {"split": "latest", "path": ["**/details_harness|hendrycksTest-logical_fallacies|5_2024-01-10T15-39-56.317779.parquet"]}]}, {"config_name": "harness_hendrycksTest_machine_learning_5", "data_files": [{"split": "2024_01_10T15_39_56.317779", "path": ["**/details_harness|hendrycksTest-machine_learning|5_2024-01-10T15-39-56.317779.parquet"]}, {"split": "latest", "path": ["**/details_harness|hendrycksTest-machine_learning|5_2024-01-10T15-39-56.317779.parquet"]}]}, {"config_name": "harness_hendrycksTest_management_5", "data_files": [{"split": "2024_01_10T15_39_56.317779", "path": ["**/details_harness|hendrycksTest-management|5_2024-01-10T15-39-56.317779.parquet"]}, {"split": "latest", "path": ["**/details_harness|hendrycksTest-management|5_2024-01-10T15-39-56.317779.parquet"]}]}, {"config_name": "harness_hendrycksTest_marketing_5", "data_files": [{"split": "2024_01_10T15_39_56.317779", "path": ["**/details_harness|hendrycksTest-marketing|5_2024-01-10T15-39-56.317779.parquet"]}, {"split": "latest", "path": ["**/details_harness|hendrycksTest-marketing|5_2024-01-10T15-39-56.317779.parquet"]}]}, {"config_name": "harness_hendrycksTest_medical_genetics_5", "data_files": [{"split": "2024_01_10T15_39_56.317779", "path": ["**/details_harness|hendrycksTest-medical_genetics|5_2024-01-10T15-39-56.317779.parquet"]}, {"split": "latest", "path": ["**/details_harness|hendrycksTest-medical_genetics|5_2024-01-10T15-39-56.317779.parquet"]}]}, {"config_name": "harness_hendrycksTest_miscellaneous_5", "data_files": [{"split": "2024_01_10T15_39_56.317779", "path": ["**/details_harness|hendrycksTest-miscellaneous|5_2024-01-10T15-39-56.317779.parquet"]}, {"split": "latest", "path": ["**/details_harness|hendrycksTest-miscellaneous|5_2024-01-10T15-39-56.317779.parquet"]}]}, {"config_name": "harness_hendrycksTest_moral_disputes_5", "data_files": [{"split": "2024_01_10T15_39_56.317779", "path": ["**/details_harness|hendrycksTest-moral_disputes|5_2024-01-10T15-39-56.317779.parquet"]}, {"split": "latest", "path": ["**/details_harness|hendrycksTest-moral_disputes|5_2024-01-10T15-39-56.317779.parquet"]}]}, {"config_name": "harness_hendrycksTest_moral_scenarios_5", "data_files": [{"split": "2024_01_10T15_39_56.317779", "path": ["**/details_harness|hendrycksTest-moral_scenarios|5_2024-01-10T15-39-56.317779.parquet"]}, {"split": "latest", "path": ["**/details_harness|hendrycksTest-moral_scenarios|5_2024-01-10T15-39-56.317779.parquet"]}]}, {"config_name": "harness_hendrycksTest_nutrition_5", "data_files": [{"split": "2024_01_10T15_39_56.317779", "path": ["**/details_harness|hendrycksTest-nutrition|5_2024-01-10T15-39-56.317779.parquet"]}, {"split": "latest", "path": ["**/details_harness|hendrycksTest-nutrition|5_2024-01-10T15-39-56.317779.parquet"]}]}, {"config_name": "harness_hendrycksTest_philosophy_5", "data_files": [{"split": "2024_01_10T15_39_56.317779", "path": ["**/details_harness|hendrycksTest-philosophy|5_2024-01-10T15-39-56.317779.parquet"]}, {"split": "latest", "path": ["**/details_harness|hendrycksTest-philosophy|5_2024-01-10T15-39-56.317779.parquet"]}]}, {"config_name": "harness_hendrycksTest_prehistory_5", "data_files": [{"split": "2024_01_10T15_39_56.317779", "path": ["**/details_harness|hendrycksTest-prehistory|5_2024-01-10T15-39-56.317779.parquet"]}, {"split": "latest", "path": ["**/details_harness|hendrycksTest-prehistory|5_2024-01-10T15-39-56.317779.parquet"]}]}, {"config_name": "harness_hendrycksTest_professional_accounting_5", "data_files": [{"split": "2024_01_10T15_39_56.317779", "path": ["**/details_harness|hendrycksTest-professional_accounting|5_2024-01-10T15-39-56.317779.parquet"]}, {"split": "latest", "path": ["**/details_harness|hendrycksTest-professional_accounting|5_2024-01-10T15-39-56.317779.parquet"]}]}, {"config_name": "harness_hendrycksTest_professional_law_5", "data_files": [{"split": "2024_01_10T15_39_56.317779", "path": ["**/details_harness|hendrycksTest-professional_law|5_2024-01-10T15-39-56.317779.parquet"]}, {"split": "latest", "path": ["**/details_harness|hendrycksTest-professional_law|5_2024-01-10T15-39-56.317779.parquet"]}]}, {"config_name": "harness_hendrycksTest_professional_medicine_5", "data_files": [{"split": "2024_01_10T15_39_56.317779", "path": ["**/details_harness|hendrycksTest-professional_medicine|5_2024-01-10T15-39-56.317779.parquet"]}, {"split": "latest", "path": ["**/details_harness|hendrycksTest-professional_medicine|5_2024-01-10T15-39-56.317779.parquet"]}]}, {"config_name": "harness_hendrycksTest_professional_psychology_5", "data_files": [{"split": "2024_01_10T15_39_56.317779", "path": ["**/details_harness|hendrycksTest-professional_psychology|5_2024-01-10T15-39-56.317779.parquet"]}, {"split": "latest", "path": ["**/details_harness|hendrycksTest-professional_psychology|5_2024-01-10T15-39-56.317779.parquet"]}]}, {"config_name": "harness_hendrycksTest_public_relations_5", "data_files": [{"split": "2024_01_10T15_39_56.317779", "path": ["**/details_harness|hendrycksTest-public_relations|5_2024-01-10T15-39-56.317779.parquet"]}, {"split": "latest", "path": ["**/details_harness|hendrycksTest-public_relations|5_2024-01-10T15-39-56.317779.parquet"]}]}, {"config_name": "harness_hendrycksTest_security_studies_5", "data_files": [{"split": "2024_01_10T15_39_56.317779", "path": ["**/details_harness|hendrycksTest-security_studies|5_2024-01-10T15-39-56.317779.parquet"]}, {"split": "latest", "path": ["**/details_harness|hendrycksTest-security_studies|5_2024-01-10T15-39-56.317779.parquet"]}]}, {"config_name": "harness_hendrycksTest_sociology_5", "data_files": [{"split": "2024_01_10T15_39_56.317779", "path": ["**/details_harness|hendrycksTest-sociology|5_2024-01-10T15-39-56.317779.parquet"]}, {"split": "latest", "path": ["**/details_harness|hendrycksTest-sociology|5_2024-01-10T15-39-56.317779.parquet"]}]}, {"config_name": "harness_hendrycksTest_us_foreign_policy_5", "data_files": [{"split": "2024_01_10T15_39_56.317779", "path": ["**/details_harness|hendrycksTest-us_foreign_policy|5_2024-01-10T15-39-56.317779.parquet"]}, {"split": "latest", "path": ["**/details_harness|hendrycksTest-us_foreign_policy|5_2024-01-10T15-39-56.317779.parquet"]}]}, {"config_name": "harness_hendrycksTest_virology_5", "data_files": [{"split": "2024_01_10T15_39_56.317779", "path": ["**/details_harness|hendrycksTest-virology|5_2024-01-10T15-39-56.317779.parquet"]}, {"split": "latest", "path": ["**/details_harness|hendrycksTest-virology|5_2024-01-10T15-39-56.317779.parquet"]}]}, {"config_name": "harness_hendrycksTest_world_religions_5", "data_files": [{"split": "2024_01_10T15_39_56.317779", "path": ["**/details_harness|hendrycksTest-world_religions|5_2024-01-10T15-39-56.317779.parquet"]}, {"split": "latest", "path": ["**/details_harness|hendrycksTest-world_religions|5_2024-01-10T15-39-56.317779.parquet"]}]}, {"config_name": "harness_truthfulqa_mc_0", "data_files": [{"split": "2024_01_10T15_39_56.317779", "path": ["**/details_harness|truthfulqa:mc|0_2024-01-10T15-39-56.317779.parquet"]}, {"split": "latest", "path": ["**/details_harness|truthfulqa:mc|0_2024-01-10T15-39-56.317779.parquet"]}]}, {"config_name": "harness_winogrande_5", "data_files": [{"split": "2024_01_10T15_39_56.317779", "path": ["**/details_harness|winogrande|5_2024-01-10T15-39-56.317779.parquet"]}, {"split": "latest", "path": ["**/details_harness|winogrande|5_2024-01-10T15-39-56.317779.parquet"]}]}, {"config_name": "results", "data_files": [{"split": "2024_01_10T15_39_56.317779", "path": ["results_2024-01-10T15-39-56.317779.parquet"]}, {"split": "latest", "path": ["results_2024-01-10T15-39-56.317779.parquet"]}]}]} | 2024-01-10T15:42:46+00:00 | [] | [] | TAGS
#region-us
|
# Dataset Card for Evaluation run of cookinai/CM-14
Dataset automatically created during the evaluation run of model cookinai/CM-14 on the Open LLM Leaderboard.
The dataset is composed of 63 configuration, each one coresponding to one of the evaluated task.
The dataset has been created from 1 run(s). Each run can be found as a specific split in each configuration, the split being named using the timestamp of the run.The "train" split is always pointing to the latest results.
An additional configuration "results" store all the aggregated results of the run (and is used to compute and display the aggregated metrics on the Open LLM Leaderboard).
To load the details from a run, you can for instance do the following:
## Latest results
These are the latest results from run 2024-01-10T15:39:56.317779(note that their might be results for other tasks in the repos if successive evals didn't cover the same tasks. You find each in the results and the "latest" split for each eval):
## Dataset Details
### Dataset Description
- Curated by:
- Funded by [optional]:
- Shared by [optional]:
- Language(s) (NLP):
- License:
### Dataset Sources [optional]
- Repository:
- Paper [optional]:
- Demo [optional]:
## Uses
### Direct Use
### Out-of-Scope Use
## Dataset Structure
## Dataset Creation
### Curation Rationale
### Source Data
#### Data Collection and Processing
#### Who are the source data producers?
### Annotations [optional]
#### Annotation process
#### Who are the annotators?
#### Personal and Sensitive Information
## Bias, Risks, and Limitations
### Recommendations
Users should be made aware of the risks, biases and limitations of the dataset. More information needed for further recommendations.
[optional]
BibTeX:
APA:
## Glossary [optional]
## More Information [optional]
## Dataset Card Authors [optional]
## Dataset Card Contact
| [
"# Dataset Card for Evaluation run of cookinai/CM-14\n\n\n\nDataset automatically created during the evaluation run of model cookinai/CM-14 on the Open LLM Leaderboard.\n\nThe dataset is composed of 63 configuration, each one coresponding to one of the evaluated task.\n\nThe dataset has been created from 1 run(s). Each run can be found as a specific split in each configuration, the split being named using the timestamp of the run.The \"train\" split is always pointing to the latest results.\n\nAn additional configuration \"results\" store all the aggregated results of the run (and is used to compute and display the aggregated metrics on the Open LLM Leaderboard).\n\nTo load the details from a run, you can for instance do the following:",
"## Latest results\n\nThese are the latest results from run 2024-01-10T15:39:56.317779(note that their might be results for other tasks in the repos if successive evals didn't cover the same tasks. You find each in the results and the \"latest\" split for each eval):",
"## Dataset Details",
"### Dataset Description\n\n\n\n\n\n- Curated by: \n- Funded by [optional]: \n- Shared by [optional]: \n- Language(s) (NLP): \n- License:",
"### Dataset Sources [optional]\n\n\n\n- Repository: \n- Paper [optional]: \n- Demo [optional]:",
"## Uses",
"### Direct Use",
"### Out-of-Scope Use",
"## Dataset Structure",
"## Dataset Creation",
"### Curation Rationale",
"### Source Data",
"#### Data Collection and Processing",
"#### Who are the source data producers?",
"### Annotations [optional]",
"#### Annotation process",
"#### Who are the annotators?",
"#### Personal and Sensitive Information",
"## Bias, Risks, and Limitations",
"### Recommendations\n\n\n\nUsers should be made aware of the risks, biases and limitations of the dataset. More information needed for further recommendations.\n\n[optional]\n\n\n\nBibTeX:\n\n\n\nAPA:",
"## Glossary [optional]",
"## More Information [optional]",
"## Dataset Card Authors [optional]",
"## Dataset Card Contact"
] | [
"TAGS\n#region-us \n",
"# Dataset Card for Evaluation run of cookinai/CM-14\n\n\n\nDataset automatically created during the evaluation run of model cookinai/CM-14 on the Open LLM Leaderboard.\n\nThe dataset is composed of 63 configuration, each one coresponding to one of the evaluated task.\n\nThe dataset has been created from 1 run(s). Each run can be found as a specific split in each configuration, the split being named using the timestamp of the run.The \"train\" split is always pointing to the latest results.\n\nAn additional configuration \"results\" store all the aggregated results of the run (and is used to compute and display the aggregated metrics on the Open LLM Leaderboard).\n\nTo load the details from a run, you can for instance do the following:",
"## Latest results\n\nThese are the latest results from run 2024-01-10T15:39:56.317779(note that their might be results for other tasks in the repos if successive evals didn't cover the same tasks. You find each in the results and the \"latest\" split for each eval):",
"## Dataset Details",
"### Dataset Description\n\n\n\n\n\n- Curated by: \n- Funded by [optional]: \n- Shared by [optional]: \n- Language(s) (NLP): \n- License:",
"### Dataset Sources [optional]\n\n\n\n- Repository: \n- Paper [optional]: \n- Demo [optional]:",
"## Uses",
"### Direct Use",
"### Out-of-Scope Use",
"## Dataset Structure",
"## Dataset Creation",
"### Curation Rationale",
"### Source Data",
"#### Data Collection and Processing",
"#### Who are the source data producers?",
"### Annotations [optional]",
"#### Annotation process",
"#### Who are the annotators?",
"#### Personal and Sensitive Information",
"## Bias, Risks, and Limitations",
"### Recommendations\n\n\n\nUsers should be made aware of the risks, biases and limitations of the dataset. More information needed for further recommendations.\n\n[optional]\n\n\n\nBibTeX:\n\n\n\nAPA:",
"## Glossary [optional]",
"## More Information [optional]",
"## Dataset Card Authors [optional]",
"## Dataset Card Contact"
] |
96d28b642bd7495ab090ba210554770edc94513a | # Dataset Card for "agieval-gaokao-chemistry"
Dataset taken from https://github.com/microsoft/AGIEval and processed as in that repo, following dmayhem93/agieval-* datasets on the HF hub.
This dataset contains the contents of the Gaokao Chemistry subtask of AGIEval, as accessed in https://github.com/ruixiangcui/AGIEval/commit/5c77d073fda993f1652eaae3cf5d04cc5fd21d40 .
Citation:
```
@misc{zhong2023agieval,
title={AGIEval: A Human-Centric Benchmark for Evaluating Foundation Models},
author={Wanjun Zhong and Ruixiang Cui and Yiduo Guo and Yaobo Liang and Shuai Lu and Yanlin Wang and Amin Saied and Weizhu Chen and Nan Duan},
year={2023},
eprint={2304.06364},
archivePrefix={arXiv},
primaryClass={cs.CL}
}
```
Please make sure to cite all the individual datasets in your paper when you use them. We provide the relevant citation information below:
```
@inproceedings{ling-etal-2017-program,
title = "Program Induction by Rationale Generation: Learning to Solve and Explain Algebraic Word Problems",
author = "Ling, Wang and
Yogatama, Dani and
Dyer, Chris and
Blunsom, Phil",
booktitle = "Proceedings of the 55th Annual Meeting of the Association for Computational Linguistics (Volume 1: Long Papers)",
month = jul,
year = "2017",
address = "Vancouver, Canada",
publisher = "Association for Computational Linguistics",
url = "https://aclanthology.org/P17-1015",
doi = "10.18653/v1/P17-1015",
pages = "158--167",
abstract = "Solving algebraic word problems requires executing a series of arithmetic operations{---}a program{---}to obtain a final answer. However, since programs can be arbitrarily complicated, inducing them directly from question-answer pairs is a formidable challenge. To make this task more feasible, we solve these problems by generating answer rationales, sequences of natural language and human-readable mathematical expressions that derive the final answer through a series of small steps. Although rationales do not explicitly specify programs, they provide a scaffolding for their structure via intermediate milestones. To evaluate our approach, we have created a new 100,000-sample dataset of questions, answers and rationales. Experimental results show that indirect supervision of program learning via answer rationales is a promising strategy for inducing arithmetic programs.",
}
@inproceedings{hendrycksmath2021,
title={Measuring Mathematical Problem Solving With the MATH Dataset},
author={Dan Hendrycks and Collin Burns and Saurav Kadavath and Akul Arora and Steven Basart and Eric Tang and Dawn Song and Jacob Steinhardt},
journal={NeurIPS},
year={2021}
}
@inproceedings{Liu2020LogiQAAC,
title={LogiQA: A Challenge Dataset for Machine Reading Comprehension with Logical Reasoning},
author={Jian Liu and Leyang Cui and Hanmeng Liu and Dandan Huang and Yile Wang and Yue Zhang},
booktitle={International Joint Conference on Artificial Intelligence},
year={2020}
}
@inproceedings{zhong2019jec,
title={JEC-QA: A Legal-Domain Question Answering Dataset},
author={Zhong, Haoxi and Xiao, Chaojun and Tu, Cunchao and Zhang, Tianyang and Liu, Zhiyuan and Sun, Maosong},
booktitle={Proceedings of AAAI},
year={2020},
}
@article{Wang2021FromLT,
title={From LSAT: The Progress and Challenges of Complex Reasoning},
author={Siyuan Wang and Zhongkun Liu and Wanjun Zhong and Ming Zhou and Zhongyu Wei and Zhumin Chen and Nan Duan},
journal={IEEE/ACM Transactions on Audio, Speech, and Language Processing},
year={2021},
volume={30},
pages={2201-2216}
}
``` | hails/agieval-gaokao-chemistry | [
"arxiv:2304.06364",
"region:us"
] | 2024-01-10T15:42:46+00:00 | {"dataset_info": {"features": [{"name": "query", "dtype": "string"}, {"name": "choices", "sequence": "string"}, {"name": "gold", "sequence": "int64"}], "splits": [{"name": "test", "num_bytes": 171130, "num_examples": 207}], "download_size": 77487, "dataset_size": 171130}, "configs": [{"config_name": "default", "data_files": [{"split": "test", "path": "data/test-*"}]}]} | 2024-01-26T18:37:13+00:00 | [
"2304.06364"
] | [] | TAGS
#arxiv-2304.06364 #region-us
| # Dataset Card for "agieval-gaokao-chemistry"
Dataset taken from URL and processed as in that repo, following dmayhem93/agieval-* datasets on the HF hub.
This dataset contains the contents of the Gaokao Chemistry subtask of AGIEval, as accessed in URL .
Citation:
Please make sure to cite all the individual datasets in your paper when you use them. We provide the relevant citation information below:
| [
"# Dataset Card for \"agieval-gaokao-chemistry\"\n\n\nDataset taken from URL and processed as in that repo, following dmayhem93/agieval-* datasets on the HF hub.\n\nThis dataset contains the contents of the Gaokao Chemistry subtask of AGIEval, as accessed in URL .\n\n\nCitation:\n\n\nPlease make sure to cite all the individual datasets in your paper when you use them. We provide the relevant citation information below:"
] | [
"TAGS\n#arxiv-2304.06364 #region-us \n",
"# Dataset Card for \"agieval-gaokao-chemistry\"\n\n\nDataset taken from URL and processed as in that repo, following dmayhem93/agieval-* datasets on the HF hub.\n\nThis dataset contains the contents of the Gaokao Chemistry subtask of AGIEval, as accessed in URL .\n\n\nCitation:\n\n\nPlease make sure to cite all the individual datasets in your paper when you use them. We provide the relevant citation information below:"
] |
bf8f870cd9b02f545a1efbea36920ce343f7029d |
# Dataset Card for "agieval-gaokao-chinese"
Dataset taken from https://github.com/microsoft/AGIEval and processed as in that repo, following dmayhem93/agieval-* datasets on the HF hub.
This dataset contains the contents of the Gaokao Chinese subtask of AGIEval, as accessed in https://github.com/ruixiangcui/AGIEval/commit/5c77d073fda993f1652eaae3cf5d04cc5fd21d40 .
Citation:
```
@misc{zhong2023agieval,
title={AGIEval: A Human-Centric Benchmark for Evaluating Foundation Models},
author={Wanjun Zhong and Ruixiang Cui and Yiduo Guo and Yaobo Liang and Shuai Lu and Yanlin Wang and Amin Saied and Weizhu Chen and Nan Duan},
year={2023},
eprint={2304.06364},
archivePrefix={arXiv},
primaryClass={cs.CL}
}
```
Please make sure to cite all the individual datasets in your paper when you use them. We provide the relevant citation information below:
```
@inproceedings{ling-etal-2017-program,
title = "Program Induction by Rationale Generation: Learning to Solve and Explain Algebraic Word Problems",
author = "Ling, Wang and
Yogatama, Dani and
Dyer, Chris and
Blunsom, Phil",
booktitle = "Proceedings of the 55th Annual Meeting of the Association for Computational Linguistics (Volume 1: Long Papers)",
month = jul,
year = "2017",
address = "Vancouver, Canada",
publisher = "Association for Computational Linguistics",
url = "https://aclanthology.org/P17-1015",
doi = "10.18653/v1/P17-1015",
pages = "158--167",
abstract = "Solving algebraic word problems requires executing a series of arithmetic operations{---}a program{---}to obtain a final answer. However, since programs can be arbitrarily complicated, inducing them directly from question-answer pairs is a formidable challenge. To make this task more feasible, we solve these problems by generating answer rationales, sequences of natural language and human-readable mathematical expressions that derive the final answer through a series of small steps. Although rationales do not explicitly specify programs, they provide a scaffolding for their structure via intermediate milestones. To evaluate our approach, we have created a new 100,000-sample dataset of questions, answers and rationales. Experimental results show that indirect supervision of program learning via answer rationales is a promising strategy for inducing arithmetic programs.",
}
@inproceedings{hendrycksmath2021,
title={Measuring Mathematical Problem Solving With the MATH Dataset},
author={Dan Hendrycks and Collin Burns and Saurav Kadavath and Akul Arora and Steven Basart and Eric Tang and Dawn Song and Jacob Steinhardt},
journal={NeurIPS},
year={2021}
}
@inproceedings{Liu2020LogiQAAC,
title={LogiQA: A Challenge Dataset for Machine Reading Comprehension with Logical Reasoning},
author={Jian Liu and Leyang Cui and Hanmeng Liu and Dandan Huang and Yile Wang and Yue Zhang},
booktitle={International Joint Conference on Artificial Intelligence},
year={2020}
}
@inproceedings{zhong2019jec,
title={JEC-QA: A Legal-Domain Question Answering Dataset},
author={Zhong, Haoxi and Xiao, Chaojun and Tu, Cunchao and Zhang, Tianyang and Liu, Zhiyuan and Sun, Maosong},
booktitle={Proceedings of AAAI},
year={2020},
}
@article{Wang2021FromLT,
title={From LSAT: The Progress and Challenges of Complex Reasoning},
author={Siyuan Wang and Zhongkun Liu and Wanjun Zhong and Ming Zhou and Zhongyu Wei and Zhumin Chen and Nan Duan},
journal={IEEE/ACM Transactions on Audio, Speech, and Language Processing},
year={2021},
volume={30},
pages={2201-2216}
}
``` | hails/agieval-gaokao-chinese | [
"arxiv:2304.06364",
"region:us"
] | 2024-01-10T15:42:48+00:00 | {"dataset_info": {"features": [{"name": "query", "dtype": "string"}, {"name": "choices", "sequence": "string"}, {"name": "gold", "sequence": "int64"}], "splits": [{"name": "test", "num_bytes": 843664, "num_examples": 246}], "download_size": 387530, "dataset_size": 843664}, "configs": [{"config_name": "default", "data_files": [{"split": "test", "path": "data/test-*"}]}]} | 2024-01-26T18:37:40+00:00 | [
"2304.06364"
] | [] | TAGS
#arxiv-2304.06364 #region-us
|
# Dataset Card for "agieval-gaokao-chinese"
Dataset taken from URL and processed as in that repo, following dmayhem93/agieval-* datasets on the HF hub.
This dataset contains the contents of the Gaokao Chinese subtask of AGIEval, as accessed in URL .
Citation:
Please make sure to cite all the individual datasets in your paper when you use them. We provide the relevant citation information below:
| [
"# Dataset Card for \"agieval-gaokao-chinese\"\n\n\nDataset taken from URL and processed as in that repo, following dmayhem93/agieval-* datasets on the HF hub.\n\nThis dataset contains the contents of the Gaokao Chinese subtask of AGIEval, as accessed in URL .\n\n\nCitation:\n\n\nPlease make sure to cite all the individual datasets in your paper when you use them. We provide the relevant citation information below:"
] | [
"TAGS\n#arxiv-2304.06364 #region-us \n",
"# Dataset Card for \"agieval-gaokao-chinese\"\n\n\nDataset taken from URL and processed as in that repo, following dmayhem93/agieval-* datasets on the HF hub.\n\nThis dataset contains the contents of the Gaokao Chinese subtask of AGIEval, as accessed in URL .\n\n\nCitation:\n\n\nPlease make sure to cite all the individual datasets in your paper when you use them. We provide the relevant citation information below:"
] |
329cd190757896ac3051a0eb6e59ecfef1a81401 |
# Dataset Card for "agieval-gaokao-english"
Dataset taken from https://github.com/microsoft/AGIEval and processed as in that repo, following dmayhem93/agieval-* datasets on the HF hub.
This dataset contains the contents of the Gaokao-English subtask of AGIEval, as accessed in https://github.com/ruixiangcui/AGIEval/commit/5c77d073fda993f1652eaae3cf5d04cc5fd21d40 .
Citation:
```
@misc{zhong2023agieval,
title={AGIEval: A Human-Centric Benchmark for Evaluating Foundation Models},
author={Wanjun Zhong and Ruixiang Cui and Yiduo Guo and Yaobo Liang and Shuai Lu and Yanlin Wang and Amin Saied and Weizhu Chen and Nan Duan},
year={2023},
eprint={2304.06364},
archivePrefix={arXiv},
primaryClass={cs.CL}
}
```
Please make sure to cite all the individual datasets in your paper when you use them. We provide the relevant citation information below:
```
@inproceedings{ling-etal-2017-program,
title = "Program Induction by Rationale Generation: Learning to Solve and Explain Algebraic Word Problems",
author = "Ling, Wang and
Yogatama, Dani and
Dyer, Chris and
Blunsom, Phil",
booktitle = "Proceedings of the 55th Annual Meeting of the Association for Computational Linguistics (Volume 1: Long Papers)",
month = jul,
year = "2017",
address = "Vancouver, Canada",
publisher = "Association for Computational Linguistics",
url = "https://aclanthology.org/P17-1015",
doi = "10.18653/v1/P17-1015",
pages = "158--167",
abstract = "Solving algebraic word problems requires executing a series of arithmetic operations{---}a program{---}to obtain a final answer. However, since programs can be arbitrarily complicated, inducing them directly from question-answer pairs is a formidable challenge. To make this task more feasible, we solve these problems by generating answer rationales, sequences of natural language and human-readable mathematical expressions that derive the final answer through a series of small steps. Although rationales do not explicitly specify programs, they provide a scaffolding for their structure via intermediate milestones. To evaluate our approach, we have created a new 100,000-sample dataset of questions, answers and rationales. Experimental results show that indirect supervision of program learning via answer rationales is a promising strategy for inducing arithmetic programs.",
}
@inproceedings{hendrycksmath2021,
title={Measuring Mathematical Problem Solving With the MATH Dataset},
author={Dan Hendrycks and Collin Burns and Saurav Kadavath and Akul Arora and Steven Basart and Eric Tang and Dawn Song and Jacob Steinhardt},
journal={NeurIPS},
year={2021}
}
@inproceedings{Liu2020LogiQAAC,
title={LogiQA: A Challenge Dataset for Machine Reading Comprehension with Logical Reasoning},
author={Jian Liu and Leyang Cui and Hanmeng Liu and Dandan Huang and Yile Wang and Yue Zhang},
booktitle={International Joint Conference on Artificial Intelligence},
year={2020}
}
@inproceedings{zhong2019jec,
title={JEC-QA: A Legal-Domain Question Answering Dataset},
author={Zhong, Haoxi and Xiao, Chaojun and Tu, Cunchao and Zhang, Tianyang and Liu, Zhiyuan and Sun, Maosong},
booktitle={Proceedings of AAAI},
year={2020},
}
@article{Wang2021FromLT,
title={From LSAT: The Progress and Challenges of Complex Reasoning},
author={Siyuan Wang and Zhongkun Liu and Wanjun Zhong and Ming Zhou and Zhongyu Wei and Zhumin Chen and Nan Duan},
journal={IEEE/ACM Transactions on Audio, Speech, and Language Processing},
year={2021},
volume={30},
pages={2201-2216}
}
``` | hails/agieval-gaokao-english | [
"arxiv:2304.06364",
"region:us"
] | 2024-01-10T15:42:49+00:00 | {"dataset_info": {"features": [{"name": "query", "dtype": "string"}, {"name": "choices", "sequence": "string"}, {"name": "gold", "sequence": "int64"}], "splits": [{"name": "test", "num_bytes": 688986, "num_examples": 306}], "download_size": 200861, "dataset_size": 688986}, "configs": [{"config_name": "default", "data_files": [{"split": "test", "path": "data/test-*"}]}]} | 2024-01-26T18:38:04+00:00 | [
"2304.06364"
] | [] | TAGS
#arxiv-2304.06364 #region-us
|
# Dataset Card for "agieval-gaokao-english"
Dataset taken from URL and processed as in that repo, following dmayhem93/agieval-* datasets on the HF hub.
This dataset contains the contents of the Gaokao-English subtask of AGIEval, as accessed in URL .
Citation:
Please make sure to cite all the individual datasets in your paper when you use them. We provide the relevant citation information below:
| [
"# Dataset Card for \"agieval-gaokao-english\"\n\n\nDataset taken from URL and processed as in that repo, following dmayhem93/agieval-* datasets on the HF hub.\n\nThis dataset contains the contents of the Gaokao-English subtask of AGIEval, as accessed in URL .\n\n\nCitation:\n\n\nPlease make sure to cite all the individual datasets in your paper when you use them. We provide the relevant citation information below:"
] | [
"TAGS\n#arxiv-2304.06364 #region-us \n",
"# Dataset Card for \"agieval-gaokao-english\"\n\n\nDataset taken from URL and processed as in that repo, following dmayhem93/agieval-* datasets on the HF hub.\n\nThis dataset contains the contents of the Gaokao-English subtask of AGIEval, as accessed in URL .\n\n\nCitation:\n\n\nPlease make sure to cite all the individual datasets in your paper when you use them. We provide the relevant citation information below:"
] |
6f583bbd010f36cb1168bd9d2389d8d82f9dabbc |
# Dataset Card for "agieval-gaokao-geography"
Dataset taken from https://github.com/microsoft/AGIEval and processed as in that repo, following dmayhem93/agieval-* datasets on the HF hub.
This dataset contains the contents of the Gaokao Geography subtask of AGIEval, as accessed in https://github.com/ruixiangcui/AGIEval/commit/5c77d073fda993f1652eaae3cf5d04cc5fd21d40 .
Citation:
```
@misc{zhong2023agieval,
title={AGIEval: A Human-Centric Benchmark for Evaluating Foundation Models},
author={Wanjun Zhong and Ruixiang Cui and Yiduo Guo and Yaobo Liang and Shuai Lu and Yanlin Wang and Amin Saied and Weizhu Chen and Nan Duan},
year={2023},
eprint={2304.06364},
archivePrefix={arXiv},
primaryClass={cs.CL}
}
```
Please make sure to cite all the individual datasets in your paper when you use them. We provide the relevant citation information below:
```
@inproceedings{ling-etal-2017-program,
title = "Program Induction by Rationale Generation: Learning to Solve and Explain Algebraic Word Problems",
author = "Ling, Wang and
Yogatama, Dani and
Dyer, Chris and
Blunsom, Phil",
booktitle = "Proceedings of the 55th Annual Meeting of the Association for Computational Linguistics (Volume 1: Long Papers)",
month = jul,
year = "2017",
address = "Vancouver, Canada",
publisher = "Association for Computational Linguistics",
url = "https://aclanthology.org/P17-1015",
doi = "10.18653/v1/P17-1015",
pages = "158--167",
abstract = "Solving algebraic word problems requires executing a series of arithmetic operations{---}a program{---}to obtain a final answer. However, since programs can be arbitrarily complicated, inducing them directly from question-answer pairs is a formidable challenge. To make this task more feasible, we solve these problems by generating answer rationales, sequences of natural language and human-readable mathematical expressions that derive the final answer through a series of small steps. Although rationales do not explicitly specify programs, they provide a scaffolding for their structure via intermediate milestones. To evaluate our approach, we have created a new 100,000-sample dataset of questions, answers and rationales. Experimental results show that indirect supervision of program learning via answer rationales is a promising strategy for inducing arithmetic programs.",
}
@inproceedings{hendrycksmath2021,
title={Measuring Mathematical Problem Solving With the MATH Dataset},
author={Dan Hendrycks and Collin Burns and Saurav Kadavath and Akul Arora and Steven Basart and Eric Tang and Dawn Song and Jacob Steinhardt},
journal={NeurIPS},
year={2021}
}
@inproceedings{Liu2020LogiQAAC,
title={LogiQA: A Challenge Dataset for Machine Reading Comprehension with Logical Reasoning},
author={Jian Liu and Leyang Cui and Hanmeng Liu and Dandan Huang and Yile Wang and Yue Zhang},
booktitle={International Joint Conference on Artificial Intelligence},
year={2020}
}
@inproceedings{zhong2019jec,
title={JEC-QA: A Legal-Domain Question Answering Dataset},
author={Zhong, Haoxi and Xiao, Chaojun and Tu, Cunchao and Zhang, Tianyang and Liu, Zhiyuan and Sun, Maosong},
booktitle={Proceedings of AAAI},
year={2020},
}
@article{Wang2021FromLT,
title={From LSAT: The Progress and Challenges of Complex Reasoning},
author={Siyuan Wang and Zhongkun Liu and Wanjun Zhong and Ming Zhou and Zhongyu Wei and Zhumin Chen and Nan Duan},
journal={IEEE/ACM Transactions on Audio, Speech, and Language Processing},
year={2021},
volume={30},
pages={2201-2216}
}
``` | hails/agieval-gaokao-geography | [
"arxiv:2304.06364",
"region:us"
] | 2024-01-10T15:42:50+00:00 | {"dataset_info": {"features": [{"name": "query", "dtype": "string"}, {"name": "choices", "sequence": "string"}, {"name": "gold", "sequence": "int64"}], "splits": [{"name": "test", "num_bytes": 116612, "num_examples": 199}], "download_size": 52886, "dataset_size": 116612}, "configs": [{"config_name": "default", "data_files": [{"split": "test", "path": "data/test-*"}]}]} | 2024-01-26T18:38:35+00:00 | [
"2304.06364"
] | [] | TAGS
#arxiv-2304.06364 #region-us
|
# Dataset Card for "agieval-gaokao-geography"
Dataset taken from URL and processed as in that repo, following dmayhem93/agieval-* datasets on the HF hub.
This dataset contains the contents of the Gaokao Geography subtask of AGIEval, as accessed in URL .
Citation:
Please make sure to cite all the individual datasets in your paper when you use them. We provide the relevant citation information below:
| [
"# Dataset Card for \"agieval-gaokao-geography\"\n\n\nDataset taken from URL and processed as in that repo, following dmayhem93/agieval-* datasets on the HF hub.\n\nThis dataset contains the contents of the Gaokao Geography subtask of AGIEval, as accessed in URL .\n\n\nCitation:\n\n\nPlease make sure to cite all the individual datasets in your paper when you use them. We provide the relevant citation information below:"
] | [
"TAGS\n#arxiv-2304.06364 #region-us \n",
"# Dataset Card for \"agieval-gaokao-geography\"\n\n\nDataset taken from URL and processed as in that repo, following dmayhem93/agieval-* datasets on the HF hub.\n\nThis dataset contains the contents of the Gaokao Geography subtask of AGIEval, as accessed in URL .\n\n\nCitation:\n\n\nPlease make sure to cite all the individual datasets in your paper when you use them. We provide the relevant citation information below:"
] |
a72efceb8dfa4ba905506a1b82e6716fed782dfd |
# Dataset Card for "agieval-gaokao-history"
Dataset taken from https://github.com/microsoft/AGIEval and processed as in that repo, following dmayhem93/agieval-* datasets on the HF hub.
This dataset contains the contents of the Gaokao History subtask of AGIEval, as accessed in https://github.com/ruixiangcui/AGIEval/commit/5c77d073fda993f1652eaae3cf5d04cc5fd21d40 .
Citation:
```
@misc{zhong2023agieval,
title={AGIEval: A Human-Centric Benchmark for Evaluating Foundation Models},
author={Wanjun Zhong and Ruixiang Cui and Yiduo Guo and Yaobo Liang and Shuai Lu and Yanlin Wang and Amin Saied and Weizhu Chen and Nan Duan},
year={2023},
eprint={2304.06364},
archivePrefix={arXiv},
primaryClass={cs.CL}
}
```
Please make sure to cite all the individual datasets in your paper when you use them. We provide the relevant citation information below:
```
@inproceedings{ling-etal-2017-program,
title = "Program Induction by Rationale Generation: Learning to Solve and Explain Algebraic Word Problems",
author = "Ling, Wang and
Yogatama, Dani and
Dyer, Chris and
Blunsom, Phil",
booktitle = "Proceedings of the 55th Annual Meeting of the Association for Computational Linguistics (Volume 1: Long Papers)",
month = jul,
year = "2017",
address = "Vancouver, Canada",
publisher = "Association for Computational Linguistics",
url = "https://aclanthology.org/P17-1015",
doi = "10.18653/v1/P17-1015",
pages = "158--167",
abstract = "Solving algebraic word problems requires executing a series of arithmetic operations{---}a program{---}to obtain a final answer. However, since programs can be arbitrarily complicated, inducing them directly from question-answer pairs is a formidable challenge. To make this task more feasible, we solve these problems by generating answer rationales, sequences of natural language and human-readable mathematical expressions that derive the final answer through a series of small steps. Although rationales do not explicitly specify programs, they provide a scaffolding for their structure via intermediate milestones. To evaluate our approach, we have created a new 100,000-sample dataset of questions, answers and rationales. Experimental results show that indirect supervision of program learning via answer rationales is a promising strategy for inducing arithmetic programs.",
}
@inproceedings{hendrycksmath2021,
title={Measuring Mathematical Problem Solving With the MATH Dataset},
author={Dan Hendrycks and Collin Burns and Saurav Kadavath and Akul Arora and Steven Basart and Eric Tang and Dawn Song and Jacob Steinhardt},
journal={NeurIPS},
year={2021}
}
@inproceedings{Liu2020LogiQAAC,
title={LogiQA: A Challenge Dataset for Machine Reading Comprehension with Logical Reasoning},
author={Jian Liu and Leyang Cui and Hanmeng Liu and Dandan Huang and Yile Wang and Yue Zhang},
booktitle={International Joint Conference on Artificial Intelligence},
year={2020}
}
@inproceedings{zhong2019jec,
title={JEC-QA: A Legal-Domain Question Answering Dataset},
author={Zhong, Haoxi and Xiao, Chaojun and Tu, Cunchao and Zhang, Tianyang and Liu, Zhiyuan and Sun, Maosong},
booktitle={Proceedings of AAAI},
year={2020},
}
@article{Wang2021FromLT,
title={From LSAT: The Progress and Challenges of Complex Reasoning},
author={Siyuan Wang and Zhongkun Liu and Wanjun Zhong and Ming Zhou and Zhongyu Wei and Zhumin Chen and Nan Duan},
journal={IEEE/ACM Transactions on Audio, Speech, and Language Processing},
year={2021},
volume={30},
pages={2201-2216}
}
``` | hails/agieval-gaokao-history | [
"arxiv:2304.06364",
"region:us"
] | 2024-01-10T15:42:51+00:00 | {"dataset_info": {"features": [{"name": "query", "dtype": "string"}, {"name": "choices", "sequence": "string"}, {"name": "gold", "sequence": "int64"}], "splits": [{"name": "test", "num_bytes": 120008, "num_examples": 235}], "download_size": 78999, "dataset_size": 120008}, "configs": [{"config_name": "default", "data_files": [{"split": "test", "path": "data/test-*"}]}]} | 2024-01-26T18:39:29+00:00 | [
"2304.06364"
] | [] | TAGS
#arxiv-2304.06364 #region-us
|
# Dataset Card for "agieval-gaokao-history"
Dataset taken from URL and processed as in that repo, following dmayhem93/agieval-* datasets on the HF hub.
This dataset contains the contents of the Gaokao History subtask of AGIEval, as accessed in URL .
Citation:
Please make sure to cite all the individual datasets in your paper when you use them. We provide the relevant citation information below:
| [
"# Dataset Card for \"agieval-gaokao-history\"\n\n\nDataset taken from URL and processed as in that repo, following dmayhem93/agieval-* datasets on the HF hub.\n\nThis dataset contains the contents of the Gaokao History subtask of AGIEval, as accessed in URL .\n\n\nCitation:\n\n\nPlease make sure to cite all the individual datasets in your paper when you use them. We provide the relevant citation information below:"
] | [
"TAGS\n#arxiv-2304.06364 #region-us \n",
"# Dataset Card for \"agieval-gaokao-history\"\n\n\nDataset taken from URL and processed as in that repo, following dmayhem93/agieval-* datasets on the HF hub.\n\nThis dataset contains the contents of the Gaokao History subtask of AGIEval, as accessed in URL .\n\n\nCitation:\n\n\nPlease make sure to cite all the individual datasets in your paper when you use them. We provide the relevant citation information below:"
] |
c7810f0856cd8ac59bb9102bf4f10139849b70d7 |
# Dataset Card for "agieval-gaokao-mathqa"
Dataset taken from https://github.com/microsoft/AGIEval and processed as in that repo, following dmayhem93/agieval-* datasets on the HF hub.
This dataset contains the contents of the Gaokao MathQA subtask of AGIEval, as accessed in https://github.com/ruixiangcui/AGIEval/commit/5c77d073fda993f1652eaae3cf5d04cc5fd21d40 .
Citation:
```
@misc{zhong2023agieval,
title={AGIEval: A Human-Centric Benchmark for Evaluating Foundation Models},
author={Wanjun Zhong and Ruixiang Cui and Yiduo Guo and Yaobo Liang and Shuai Lu and Yanlin Wang and Amin Saied and Weizhu Chen and Nan Duan},
year={2023},
eprint={2304.06364},
archivePrefix={arXiv},
primaryClass={cs.CL}
}
```
Please make sure to cite all the individual datasets in your paper when you use them. We provide the relevant citation information below:
```
@inproceedings{ling-etal-2017-program,
title = "Program Induction by Rationale Generation: Learning to Solve and Explain Algebraic Word Problems",
author = "Ling, Wang and
Yogatama, Dani and
Dyer, Chris and
Blunsom, Phil",
booktitle = "Proceedings of the 55th Annual Meeting of the Association for Computational Linguistics (Volume 1: Long Papers)",
month = jul,
year = "2017",
address = "Vancouver, Canada",
publisher = "Association for Computational Linguistics",
url = "https://aclanthology.org/P17-1015",
doi = "10.18653/v1/P17-1015",
pages = "158--167",
abstract = "Solving algebraic word problems requires executing a series of arithmetic operations{---}a program{---}to obtain a final answer. However, since programs can be arbitrarily complicated, inducing them directly from question-answer pairs is a formidable challenge. To make this task more feasible, we solve these problems by generating answer rationales, sequences of natural language and human-readable mathematical expressions that derive the final answer through a series of small steps. Although rationales do not explicitly specify programs, they provide a scaffolding for their structure via intermediate milestones. To evaluate our approach, we have created a new 100,000-sample dataset of questions, answers and rationales. Experimental results show that indirect supervision of program learning via answer rationales is a promising strategy for inducing arithmetic programs.",
}
@inproceedings{hendrycksmath2021,
title={Measuring Mathematical Problem Solving With the MATH Dataset},
author={Dan Hendrycks and Collin Burns and Saurav Kadavath and Akul Arora and Steven Basart and Eric Tang and Dawn Song and Jacob Steinhardt},
journal={NeurIPS},
year={2021}
}
@inproceedings{Liu2020LogiQAAC,
title={LogiQA: A Challenge Dataset for Machine Reading Comprehension with Logical Reasoning},
author={Jian Liu and Leyang Cui and Hanmeng Liu and Dandan Huang and Yile Wang and Yue Zhang},
booktitle={International Joint Conference on Artificial Intelligence},
year={2020}
}
@inproceedings{zhong2019jec,
title={JEC-QA: A Legal-Domain Question Answering Dataset},
author={Zhong, Haoxi and Xiao, Chaojun and Tu, Cunchao and Zhang, Tianyang and Liu, Zhiyuan and Sun, Maosong},
booktitle={Proceedings of AAAI},
year={2020},
}
@article{Wang2021FromLT,
title={From LSAT: The Progress and Challenges of Complex Reasoning},
author={Siyuan Wang and Zhongkun Liu and Wanjun Zhong and Ming Zhou and Zhongyu Wei and Zhumin Chen and Nan Duan},
journal={IEEE/ACM Transactions on Audio, Speech, and Language Processing},
year={2021},
volume={30},
pages={2201-2216}
}
```
| hails/agieval-gaokao-mathqa | [
"arxiv:2304.06364",
"region:us"
] | 2024-01-10T15:42:52+00:00 | {"dataset_info": {"features": [{"name": "query", "dtype": "string"}, {"name": "choices", "sequence": "string"}, {"name": "gold", "sequence": "int64"}], "splits": [{"name": "test", "num_bytes": 140041, "num_examples": 351}], "download_size": 62490, "dataset_size": 140041}, "configs": [{"config_name": "default", "data_files": [{"split": "test", "path": "data/test-*"}]}]} | 2024-01-26T18:39:02+00:00 | [
"2304.06364"
] | [] | TAGS
#arxiv-2304.06364 #region-us
|
# Dataset Card for "agieval-gaokao-mathqa"
Dataset taken from URL and processed as in that repo, following dmayhem93/agieval-* datasets on the HF hub.
This dataset contains the contents of the Gaokao MathQA subtask of AGIEval, as accessed in URL .
Citation:
Please make sure to cite all the individual datasets in your paper when you use them. We provide the relevant citation information below:
| [
"# Dataset Card for \"agieval-gaokao-mathqa\"\n\n\nDataset taken from URL and processed as in that repo, following dmayhem93/agieval-* datasets on the HF hub.\n\nThis dataset contains the contents of the Gaokao MathQA subtask of AGIEval, as accessed in URL .\n\n\nCitation:\n\n\nPlease make sure to cite all the individual datasets in your paper when you use them. We provide the relevant citation information below:"
] | [
"TAGS\n#arxiv-2304.06364 #region-us \n",
"# Dataset Card for \"agieval-gaokao-mathqa\"\n\n\nDataset taken from URL and processed as in that repo, following dmayhem93/agieval-* datasets on the HF hub.\n\nThis dataset contains the contents of the Gaokao MathQA subtask of AGIEval, as accessed in URL .\n\n\nCitation:\n\n\nPlease make sure to cite all the individual datasets in your paper when you use them. We provide the relevant citation information below:"
] |
Subsets and Splits
No community queries yet
The top public SQL queries from the community will appear here once available.