sha
stringlengths 40
40
| text
stringlengths 1
13.4M
| id
stringlengths 2
117
| tags
sequencelengths 1
7.91k
| created_at
stringlengths 25
25
| metadata
stringlengths 2
875k
| last_modified
stringlengths 25
25
| arxiv
sequencelengths 0
25
| languages
sequencelengths 0
7.91k
| tags_str
stringlengths 17
159k
| text_str
stringlengths 1
447k
| text_lists
sequencelengths 0
352
| processed_texts
sequencelengths 1
353
| tokens_length
sequencelengths 1
353
| input_texts
sequencelengths 1
40
|
---|---|---|---|---|---|---|---|---|---|---|---|---|---|---|
4217838c01c04186ef75ebb624a2adf02d460b23 | ---
annotations_creators:
- expert-generated
language:
- en
language_creators:
- found
license:
- mit
multilinguality:
- monolingual
paperswithcode_id: acronym-identification
pretty_name: Acronym Identification Dataset
size_categories:
- 10K<n<100K
source_datasets:
- original
task_categories:
- token-classification
task_ids:
- token-classification-other-acronym-identification
train-eval-index:
- col_mapping:
labels: tags
tokens: tokens
config: default
splits:
eval_split: test
task: token-classification
task_id: entity_extraction---
# Dataset Card for [Dataset Name]
## Table of Contents
- [Table of Contents](#table-of-contents)
- [Dataset Description](#dataset-description)
- [Dataset Summary](#dataset-summary)
- [Supported Tasks and Leaderboards](#supported-tasks-and-leaderboards)
- [Languages](#languages)
- [Dataset Structure](#dataset-structure)
- [Data Instances](#data-instances)
- [Data Fields](#data-fields)
- [Data Splits](#data-splits)
- [Dataset Creation](#dataset-creation)
- [Curation Rationale](#curation-rationale)
- [Source Data](#source-data)
- [Annotations](#annotations)
- [Personal and Sensitive Information](#personal-and-sensitive-information)
- [Considerations for Using the Data](#considerations-for-using-the-data)
- [Social Impact of Dataset](#social-impact-of-dataset)
- [Discussion of Biases](#discussion-of-biases)
- [Other Known Limitations](#other-known-limitations)
- [Additional Information](#additional-information)
- [Dataset Curators](#dataset-curators)
- [Licensing Information](#licensing-information)
- [Citation Information](#citation-information)
- [Contributions](#contributions)
## Dataset Description
- **Homepage:**
- **Repository:**
- **Paper:**
- **Leaderboard:**
- **Point of Contact:**
### Dataset Summary
[More Information Needed]
### Supported Tasks and Leaderboards
[More Information Needed]
### Languages
[More Information Needed]
## Dataset Structure
### Data Instances
[More Information Needed]
### Data Fields
[More Information Needed]
### Data Splits
[More Information Needed]
## Dataset Creation
### Curation Rationale
[More Information Needed]
### Source Data
#### Initial Data Collection and Normalization
[More Information Needed]
#### Who are the source language producers?
[More Information Needed]
### Annotations
#### Annotation process
[More Information Needed]
#### Who are the annotators?
[More Information Needed]
### Personal and Sensitive Information
[More Information Needed]
## Considerations for Using the Data
### Social Impact of Dataset
[More Information Needed]
### Discussion of Biases
[More Information Needed]
### Other Known Limitations
[More Information Needed]
## Additional Information
### Dataset Curators
[More Information Needed]
### Licensing Information
[More Information Needed]
### Citation Information
[More Information Needed]
### Contributions
Thanks to [@github-username](https://github.com/<github-username>) for adding this dataset.
| DTU54DL/commo-test1k-whisper-proc | [
"region:us"
] | 2022-11-21T13:08:42+00:00 | {} | 2022-11-21T13:30:24+00:00 | [] | [] | TAGS
#region-us
| ---
annotations_creators:
- expert-generated
language:
- en
language_creators:
- found
license:
- mit
multilinguality:
- monolingual
paperswithcode_id: acronym-identification
pretty_name: Acronym Identification Dataset
size_categories:
- 10K<n<100K
source_datasets:
- original
task_categories:
- token-classification
task_ids:
- token-classification-other-acronym-identification
train-eval-index:
- col_mapping:
labels: tags
tokens: tokens
config: default
splits:
eval_split: test
task: token-classification
task_id: entity_extraction---
# Dataset Card for [Dataset Name]
## Table of Contents
- Table of Contents
- Dataset Description
- Dataset Summary
- Supported Tasks and Leaderboards
- Languages
- Dataset Structure
- Data Instances
- Data Fields
- Data Splits
- Dataset Creation
- Curation Rationale
- Source Data
- Annotations
- Personal and Sensitive Information
- Considerations for Using the Data
- Social Impact of Dataset
- Discussion of Biases
- Other Known Limitations
- Additional Information
- Dataset Curators
- Licensing Information
- Citation Information
- Contributions
## Dataset Description
- Homepage:
- Repository:
- Paper:
- Leaderboard:
- Point of Contact:
### Dataset Summary
### Supported Tasks and Leaderboards
### Languages
## Dataset Structure
### Data Instances
### Data Fields
### Data Splits
## Dataset Creation
### Curation Rationale
### Source Data
#### Initial Data Collection and Normalization
#### Who are the source language producers?
### Annotations
#### Annotation process
#### Who are the annotators?
### Personal and Sensitive Information
## Considerations for Using the Data
### Social Impact of Dataset
### Discussion of Biases
### Other Known Limitations
## Additional Information
### Dataset Curators
### Licensing Information
### Contributions
Thanks to @github-username for adding this dataset.
| [
"# Dataset Card for [Dataset Name]",
"## Table of Contents\n- Table of Contents\n- Dataset Description\n - Dataset Summary\n - Supported Tasks and Leaderboards\n - Languages\n- Dataset Structure\n - Data Instances\n - Data Fields\n - Data Splits\n- Dataset Creation\n - Curation Rationale\n - Source Data\n - Annotations\n - Personal and Sensitive Information\n- Considerations for Using the Data\n - Social Impact of Dataset\n - Discussion of Biases\n - Other Known Limitations\n- Additional Information\n - Dataset Curators\n - Licensing Information\n - Citation Information\n - Contributions",
"## Dataset Description\n\n- Homepage:\n- Repository:\n- Paper:\n- Leaderboard:\n- Point of Contact:",
"### Dataset Summary",
"### Supported Tasks and Leaderboards",
"### Languages",
"## Dataset Structure",
"### Data Instances",
"### Data Fields",
"### Data Splits",
"## Dataset Creation",
"### Curation Rationale",
"### Source Data",
"#### Initial Data Collection and Normalization",
"#### Who are the source language producers?",
"### Annotations",
"#### Annotation process",
"#### Who are the annotators?",
"### Personal and Sensitive Information",
"## Considerations for Using the Data",
"### Social Impact of Dataset",
"### Discussion of Biases",
"### Other Known Limitations",
"## Additional Information",
"### Dataset Curators",
"### Licensing Information",
"### Contributions\n\nThanks to @github-username for adding this dataset."
] | [
"TAGS\n#region-us \n",
"# Dataset Card for [Dataset Name]",
"## Table of Contents\n- Table of Contents\n- Dataset Description\n - Dataset Summary\n - Supported Tasks and Leaderboards\n - Languages\n- Dataset Structure\n - Data Instances\n - Data Fields\n - Data Splits\n- Dataset Creation\n - Curation Rationale\n - Source Data\n - Annotations\n - Personal and Sensitive Information\n- Considerations for Using the Data\n - Social Impact of Dataset\n - Discussion of Biases\n - Other Known Limitations\n- Additional Information\n - Dataset Curators\n - Licensing Information\n - Citation Information\n - Contributions",
"## Dataset Description\n\n- Homepage:\n- Repository:\n- Paper:\n- Leaderboard:\n- Point of Contact:",
"### Dataset Summary",
"### Supported Tasks and Leaderboards",
"### Languages",
"## Dataset Structure",
"### Data Instances",
"### Data Fields",
"### Data Splits",
"## Dataset Creation",
"### Curation Rationale",
"### Source Data",
"#### Initial Data Collection and Normalization",
"#### Who are the source language producers?",
"### Annotations",
"#### Annotation process",
"#### Who are the annotators?",
"### Personal and Sensitive Information",
"## Considerations for Using the Data",
"### Social Impact of Dataset",
"### Discussion of Biases",
"### Other Known Limitations",
"## Additional Information",
"### Dataset Curators",
"### Licensing Information",
"### Contributions\n\nThanks to @github-username for adding this dataset."
] | [
6,
10,
125,
24,
6,
10,
4,
6,
6,
5,
5,
5,
7,
4,
10,
10,
5,
5,
9,
8,
8,
7,
8,
7,
5,
6,
6,
19
] | [
"passage: TAGS\n#region-us \n# Dataset Card for [Dataset Name]## Table of Contents\n- Table of Contents\n- Dataset Description\n - Dataset Summary\n - Supported Tasks and Leaderboards\n - Languages\n- Dataset Structure\n - Data Instances\n - Data Fields\n - Data Splits\n- Dataset Creation\n - Curation Rationale\n - Source Data\n - Annotations\n - Personal and Sensitive Information\n- Considerations for Using the Data\n - Social Impact of Dataset\n - Discussion of Biases\n - Other Known Limitations\n- Additional Information\n - Dataset Curators\n - Licensing Information\n - Citation Information\n - Contributions## Dataset Description\n\n- Homepage:\n- Repository:\n- Paper:\n- Leaderboard:\n- Point of Contact:### Dataset Summary### Supported Tasks and Leaderboards### Languages## Dataset Structure### Data Instances### Data Fields### Data Splits## Dataset Creation### Curation Rationale### Source Data#### Initial Data Collection and Normalization#### Who are the source language producers?### Annotations#### Annotation process#### Who are the annotators?### Personal and Sensitive Information## Considerations for Using the Data### Social Impact of Dataset### Discussion of Biases### Other Known Limitations## Additional Information### Dataset Curators### Licensing Information### Contributions\n\nThanks to @github-username for adding this dataset."
] |
b49866491c9f9bf9baa1c6c61ac7a9292b2efbc9 | # Dataset Card for AutoTrain Evaluator
This repository contains model predictions generated by [AutoTrain](https://huggingface.co/autotrain) for the following task and dataset:
* Task: Multi-class Text Classification
* Model: autoevaluate/multi-class-classification
* Dataset: emotion
* Config: default
* Split: test
To run new evaluation jobs, visit Hugging Face's [automatic model evaluator](https://huggingface.co/spaces/autoevaluate/model-evaluator).
## Contributions
Thanks to [@lewtun](https://huggingface.co/lewtun) for evaluating this model. | autoevaluate/autoeval-staging-eval-project-b2b60f7a-3ccf-4daf-af67-2833dd712c28-5048 | [
"autotrain",
"evaluation",
"region:us"
] | 2022-11-21T13:08:52+00:00 | {"type": "predictions", "tags": ["autotrain", "evaluation"], "datasets": ["emotion"], "eval_info": {"task": "multi_class_classification", "model": "autoevaluate/multi-class-classification", "metrics": ["matthews_correlation"], "dataset_name": "emotion", "dataset_config": "default", "dataset_split": "test", "col_mapping": {"text": "text", "target": "label"}}} | 2022-11-21T13:09:34+00:00 | [] | [] | TAGS
#autotrain #evaluation #region-us
| # Dataset Card for AutoTrain Evaluator
This repository contains model predictions generated by AutoTrain for the following task and dataset:
* Task: Multi-class Text Classification
* Model: autoevaluate/multi-class-classification
* Dataset: emotion
* Config: default
* Split: test
To run new evaluation jobs, visit Hugging Face's automatic model evaluator.
## Contributions
Thanks to @lewtun for evaluating this model. | [
"# Dataset Card for AutoTrain Evaluator\n\nThis repository contains model predictions generated by AutoTrain for the following task and dataset:\n\n* Task: Multi-class Text Classification\n* Model: autoevaluate/multi-class-classification\n* Dataset: emotion\n* Config: default\n* Split: test\n\nTo run new evaluation jobs, visit Hugging Face's automatic model evaluator.",
"## Contributions\n\nThanks to @lewtun for evaluating this model."
] | [
"TAGS\n#autotrain #evaluation #region-us \n",
"# Dataset Card for AutoTrain Evaluator\n\nThis repository contains model predictions generated by AutoTrain for the following task and dataset:\n\n* Task: Multi-class Text Classification\n* Model: autoevaluate/multi-class-classification\n* Dataset: emotion\n* Config: default\n* Split: test\n\nTo run new evaluation jobs, visit Hugging Face's automatic model evaluator.",
"## Contributions\n\nThanks to @lewtun for evaluating this model."
] | [
13,
87,
15
] | [
"passage: TAGS\n#autotrain #evaluation #region-us \n# Dataset Card for AutoTrain Evaluator\n\nThis repository contains model predictions generated by AutoTrain for the following task and dataset:\n\n* Task: Multi-class Text Classification\n* Model: autoevaluate/multi-class-classification\n* Dataset: emotion\n* Config: default\n* Split: test\n\nTo run new evaluation jobs, visit Hugging Face's automatic model evaluator.## Contributions\n\nThanks to @lewtun for evaluating this model."
] |
5895f5d10bbdba5b66f6baa23360a7b3dcd9f902 | # Dataset Card for AutoTrain Evaluator
This repository contains model predictions generated by [AutoTrain](https://huggingface.co/autotrain) for the following task and dataset:
* Task: Multi-class Text Classification
* Model: autoevaluate/multi-class-classification
* Dataset: emotion
* Config: default
* Split: test
To run new evaluation jobs, visit Hugging Face's [automatic model evaluator](https://huggingface.co/spaces/autoevaluate/model-evaluator).
## Contributions
Thanks to [@lewtun](https://huggingface.co/lewtun) for evaluating this model. | autoevaluate/autoeval-staging-eval-project-2302ec60-cb56-482a-8d70-ce549b14fd54-5149 | [
"autotrain",
"evaluation",
"region:us"
] | 2022-11-21T13:14:01+00:00 | {"type": "predictions", "tags": ["autotrain", "evaluation"], "datasets": ["emotion"], "eval_info": {"task": "multi_class_classification", "model": "autoevaluate/multi-class-classification", "metrics": ["matthews_correlation"], "dataset_name": "emotion", "dataset_config": "default", "dataset_split": "test", "col_mapping": {"text": "text", "target": "label"}}} | 2022-11-21T13:14:43+00:00 | [] | [] | TAGS
#autotrain #evaluation #region-us
| # Dataset Card for AutoTrain Evaluator
This repository contains model predictions generated by AutoTrain for the following task and dataset:
* Task: Multi-class Text Classification
* Model: autoevaluate/multi-class-classification
* Dataset: emotion
* Config: default
* Split: test
To run new evaluation jobs, visit Hugging Face's automatic model evaluator.
## Contributions
Thanks to @lewtun for evaluating this model. | [
"# Dataset Card for AutoTrain Evaluator\n\nThis repository contains model predictions generated by AutoTrain for the following task and dataset:\n\n* Task: Multi-class Text Classification\n* Model: autoevaluate/multi-class-classification\n* Dataset: emotion\n* Config: default\n* Split: test\n\nTo run new evaluation jobs, visit Hugging Face's automatic model evaluator.",
"## Contributions\n\nThanks to @lewtun for evaluating this model."
] | [
"TAGS\n#autotrain #evaluation #region-us \n",
"# Dataset Card for AutoTrain Evaluator\n\nThis repository contains model predictions generated by AutoTrain for the following task and dataset:\n\n* Task: Multi-class Text Classification\n* Model: autoevaluate/multi-class-classification\n* Dataset: emotion\n* Config: default\n* Split: test\n\nTo run new evaluation jobs, visit Hugging Face's automatic model evaluator.",
"## Contributions\n\nThanks to @lewtun for evaluating this model."
] | [
13,
87,
15
] | [
"passage: TAGS\n#autotrain #evaluation #region-us \n# Dataset Card for AutoTrain Evaluator\n\nThis repository contains model predictions generated by AutoTrain for the following task and dataset:\n\n* Task: Multi-class Text Classification\n* Model: autoevaluate/multi-class-classification\n* Dataset: emotion\n* Config: default\n* Split: test\n\nTo run new evaluation jobs, visit Hugging Face's automatic model evaluator.## Contributions\n\nThanks to @lewtun for evaluating this model."
] |
dbbb28c914a180071f9fe5e95b4c409459bae379 | # Dataset Card for AutoTrain Evaluator
This repository contains model predictions generated by [AutoTrain](https://huggingface.co/autotrain) for the following task and dataset:
* Task: Multi-class Text Classification
* Model: autoevaluate/multi-class-classification
* Dataset: emotion
* Config: default
* Split: test
To run new evaluation jobs, visit Hugging Face's [automatic model evaluator](https://huggingface.co/spaces/autoevaluate/model-evaluator).
## Contributions
Thanks to [@lewtun](https://huggingface.co/lewtun) for evaluating this model. | autoevaluate/autoeval-staging-eval-project-318525f4-cdf7-4888-965c-d4d9dfeeca48-5250 | [
"autotrain",
"evaluation",
"region:us"
] | 2022-11-21T13:19:56+00:00 | {"type": "predictions", "tags": ["autotrain", "evaluation"], "datasets": ["emotion"], "eval_info": {"task": "multi_class_classification", "model": "autoevaluate/multi-class-classification", "metrics": ["matthews_correlation"], "dataset_name": "emotion", "dataset_config": "default", "dataset_split": "test", "col_mapping": {"text": "text", "target": "label"}}} | 2022-11-21T13:20:39+00:00 | [] | [] | TAGS
#autotrain #evaluation #region-us
| # Dataset Card for AutoTrain Evaluator
This repository contains model predictions generated by AutoTrain for the following task and dataset:
* Task: Multi-class Text Classification
* Model: autoevaluate/multi-class-classification
* Dataset: emotion
* Config: default
* Split: test
To run new evaluation jobs, visit Hugging Face's automatic model evaluator.
## Contributions
Thanks to @lewtun for evaluating this model. | [
"# Dataset Card for AutoTrain Evaluator\n\nThis repository contains model predictions generated by AutoTrain for the following task and dataset:\n\n* Task: Multi-class Text Classification\n* Model: autoevaluate/multi-class-classification\n* Dataset: emotion\n* Config: default\n* Split: test\n\nTo run new evaluation jobs, visit Hugging Face's automatic model evaluator.",
"## Contributions\n\nThanks to @lewtun for evaluating this model."
] | [
"TAGS\n#autotrain #evaluation #region-us \n",
"# Dataset Card for AutoTrain Evaluator\n\nThis repository contains model predictions generated by AutoTrain for the following task and dataset:\n\n* Task: Multi-class Text Classification\n* Model: autoevaluate/multi-class-classification\n* Dataset: emotion\n* Config: default\n* Split: test\n\nTo run new evaluation jobs, visit Hugging Face's automatic model evaluator.",
"## Contributions\n\nThanks to @lewtun for evaluating this model."
] | [
13,
87,
15
] | [
"passage: TAGS\n#autotrain #evaluation #region-us \n# Dataset Card for AutoTrain Evaluator\n\nThis repository contains model predictions generated by AutoTrain for the following task and dataset:\n\n* Task: Multi-class Text Classification\n* Model: autoevaluate/multi-class-classification\n* Dataset: emotion\n* Config: default\n* Split: test\n\nTo run new evaluation jobs, visit Hugging Face's automatic model evaluator.## Contributions\n\nThanks to @lewtun for evaluating this model."
] |
e74d373ce6454eedc53107b793b08ec0d6d7b8a3 | # Dataset Card for AutoTrain Evaluator
This repository contains model predictions generated by [AutoTrain](https://huggingface.co/autotrain) for the following task and dataset:
* Task: Binary Text Classification
* Model: autoevaluate/binary-classification
* Dataset: glue
* Config: sst2
* Split: validation
To run new evaluation jobs, visit Hugging Face's [automatic model evaluator](https://huggingface.co/spaces/autoevaluate/model-evaluator).
## Contributions
Thanks to [@lewtun](https://huggingface.co/lewtun) for evaluating this model. | autoevaluate/autoeval-staging-eval-project-5c51f1de-f5e2-46a7-861f-b1b7c80db774-5351 | [
"autotrain",
"evaluation",
"region:us"
] | 2022-11-21T13:25:52+00:00 | {"type": "predictions", "tags": ["autotrain", "evaluation"], "datasets": ["glue"], "eval_info": {"task": "binary_classification", "model": "autoevaluate/binary-classification", "metrics": ["matthews_correlation"], "dataset_name": "glue", "dataset_config": "sst2", "dataset_split": "validation", "col_mapping": {"text": "sentence", "target": "label"}}} | 2022-11-21T13:26:28+00:00 | [] | [] | TAGS
#autotrain #evaluation #region-us
| # Dataset Card for AutoTrain Evaluator
This repository contains model predictions generated by AutoTrain for the following task and dataset:
* Task: Binary Text Classification
* Model: autoevaluate/binary-classification
* Dataset: glue
* Config: sst2
* Split: validation
To run new evaluation jobs, visit Hugging Face's automatic model evaluator.
## Contributions
Thanks to @lewtun for evaluating this model. | [
"# Dataset Card for AutoTrain Evaluator\n\nThis repository contains model predictions generated by AutoTrain for the following task and dataset:\n\n* Task: Binary Text Classification\n* Model: autoevaluate/binary-classification\n* Dataset: glue\n* Config: sst2\n* Split: validation\n\nTo run new evaluation jobs, visit Hugging Face's automatic model evaluator.",
"## Contributions\n\nThanks to @lewtun for evaluating this model."
] | [
"TAGS\n#autotrain #evaluation #region-us \n",
"# Dataset Card for AutoTrain Evaluator\n\nThis repository contains model predictions generated by AutoTrain for the following task and dataset:\n\n* Task: Binary Text Classification\n* Model: autoevaluate/binary-classification\n* Dataset: glue\n* Config: sst2\n* Split: validation\n\nTo run new evaluation jobs, visit Hugging Face's automatic model evaluator.",
"## Contributions\n\nThanks to @lewtun for evaluating this model."
] | [
13,
89,
15
] | [
"passage: TAGS\n#autotrain #evaluation #region-us \n# Dataset Card for AutoTrain Evaluator\n\nThis repository contains model predictions generated by AutoTrain for the following task and dataset:\n\n* Task: Binary Text Classification\n* Model: autoevaluate/binary-classification\n* Dataset: glue\n* Config: sst2\n* Split: validation\n\nTo run new evaluation jobs, visit Hugging Face's automatic model evaluator.## Contributions\n\nThanks to @lewtun for evaluating this model."
] |
da173b9242eb26134365c4b17e69d4a2ded03b8f | # Dataset Card for AutoTrain Evaluator
This repository contains model predictions generated by [AutoTrain](https://huggingface.co/autotrain) for the following task and dataset:
* Task: Zero-Shot Text Classification
* Model: autoevaluate/zero-shot-classification
* Dataset: autoevaluate/zero-shot-classification-sample
* Config: autoevaluate--zero-shot-classification-sample
* Split: test
To run new evaluation jobs, visit Hugging Face's [automatic model evaluator](https://huggingface.co/spaces/autoevaluate/model-evaluator).
## Contributions
Thanks to [@lewtun](https://huggingface.co/lewtun) for evaluating this model. | autoevaluate/autoeval-staging-eval-project-a02353d8-c94a-4476-bd14-15028ee3f918-5452 | [
"autotrain",
"evaluation",
"region:us"
] | 2022-11-21T13:32:44+00:00 | {"type": "predictions", "tags": ["autotrain", "evaluation"], "datasets": ["autoevaluate/zero-shot-classification-sample"], "eval_info": {"task": "text_zero_shot_classification", "model": "autoevaluate/zero-shot-classification", "metrics": [], "dataset_name": "autoevaluate/zero-shot-classification-sample", "dataset_config": "autoevaluate--zero-shot-classification-sample", "dataset_split": "test", "col_mapping": {"text": "text", "classes": "classes", "target": "target"}}} | 2022-11-21T13:33:16+00:00 | [] | [] | TAGS
#autotrain #evaluation #region-us
| # Dataset Card for AutoTrain Evaluator
This repository contains model predictions generated by AutoTrain for the following task and dataset:
* Task: Zero-Shot Text Classification
* Model: autoevaluate/zero-shot-classification
* Dataset: autoevaluate/zero-shot-classification-sample
* Config: autoevaluate--zero-shot-classification-sample
* Split: test
To run new evaluation jobs, visit Hugging Face's automatic model evaluator.
## Contributions
Thanks to @lewtun for evaluating this model. | [
"# Dataset Card for AutoTrain Evaluator\n\nThis repository contains model predictions generated by AutoTrain for the following task and dataset:\n\n* Task: Zero-Shot Text Classification\n* Model: autoevaluate/zero-shot-classification\n* Dataset: autoevaluate/zero-shot-classification-sample\n* Config: autoevaluate--zero-shot-classification-sample\n* Split: test\n\nTo run new evaluation jobs, visit Hugging Face's automatic model evaluator.",
"## Contributions\n\nThanks to @lewtun for evaluating this model."
] | [
"TAGS\n#autotrain #evaluation #region-us \n",
"# Dataset Card for AutoTrain Evaluator\n\nThis repository contains model predictions generated by AutoTrain for the following task and dataset:\n\n* Task: Zero-Shot Text Classification\n* Model: autoevaluate/zero-shot-classification\n* Dataset: autoevaluate/zero-shot-classification-sample\n* Config: autoevaluate--zero-shot-classification-sample\n* Split: test\n\nTo run new evaluation jobs, visit Hugging Face's automatic model evaluator.",
"## Contributions\n\nThanks to @lewtun for evaluating this model."
] | [
13,
113,
15
] | [
"passage: TAGS\n#autotrain #evaluation #region-us \n# Dataset Card for AutoTrain Evaluator\n\nThis repository contains model predictions generated by AutoTrain for the following task and dataset:\n\n* Task: Zero-Shot Text Classification\n* Model: autoevaluate/zero-shot-classification\n* Dataset: autoevaluate/zero-shot-classification-sample\n* Config: autoevaluate--zero-shot-classification-sample\n* Split: test\n\nTo run new evaluation jobs, visit Hugging Face's automatic model evaluator.## Contributions\n\nThanks to @lewtun for evaluating this model."
] |
d14d164ed864c6702a44a2384c2b04f0737e8df1 | # Dataset Card for AutoTrain Evaluator
This repository contains model predictions generated by [AutoTrain](https://huggingface.co/autotrain) for the following task and dataset:
* Task: Natural Language Inference
* Model: autoevaluate/natural-language-inference
* Dataset: glue
* Config: mrpc
* Split: validation
To run new evaluation jobs, visit Hugging Face's [automatic model evaluator](https://huggingface.co/spaces/autoevaluate/model-evaluator).
## Contributions
Thanks to [@lewtun](https://huggingface.co/lewtun) for evaluating this model. | autoevaluate/autoeval-staging-eval-project-31466167-6d47-4d63-9ebd-59fe66b62d96-5553 | [
"autotrain",
"evaluation",
"region:us"
] | 2022-11-21T13:38:58+00:00 | {"type": "predictions", "tags": ["autotrain", "evaluation"], "datasets": ["glue"], "eval_info": {"task": "natural_language_inference", "model": "autoevaluate/natural-language-inference", "metrics": [], "dataset_name": "glue", "dataset_config": "mrpc", "dataset_split": "validation", "col_mapping": {"text1": "sentence1", "text2": "sentence2", "target": "label"}}} | 2022-11-21T13:39:35+00:00 | [] | [] | TAGS
#autotrain #evaluation #region-us
| # Dataset Card for AutoTrain Evaluator
This repository contains model predictions generated by AutoTrain for the following task and dataset:
* Task: Natural Language Inference
* Model: autoevaluate/natural-language-inference
* Dataset: glue
* Config: mrpc
* Split: validation
To run new evaluation jobs, visit Hugging Face's automatic model evaluator.
## Contributions
Thanks to @lewtun for evaluating this model. | [
"# Dataset Card for AutoTrain Evaluator\n\nThis repository contains model predictions generated by AutoTrain for the following task and dataset:\n\n* Task: Natural Language Inference\n* Model: autoevaluate/natural-language-inference\n* Dataset: glue\n* Config: mrpc\n* Split: validation\n\nTo run new evaluation jobs, visit Hugging Face's automatic model evaluator.",
"## Contributions\n\nThanks to @lewtun for evaluating this model."
] | [
"TAGS\n#autotrain #evaluation #region-us \n",
"# Dataset Card for AutoTrain Evaluator\n\nThis repository contains model predictions generated by AutoTrain for the following task and dataset:\n\n* Task: Natural Language Inference\n* Model: autoevaluate/natural-language-inference\n* Dataset: glue\n* Config: mrpc\n* Split: validation\n\nTo run new evaluation jobs, visit Hugging Face's automatic model evaluator.",
"## Contributions\n\nThanks to @lewtun for evaluating this model."
] | [
13,
90,
15
] | [
"passage: TAGS\n#autotrain #evaluation #region-us \n# Dataset Card for AutoTrain Evaluator\n\nThis repository contains model predictions generated by AutoTrain for the following task and dataset:\n\n* Task: Natural Language Inference\n* Model: autoevaluate/natural-language-inference\n* Dataset: glue\n* Config: mrpc\n* Split: validation\n\nTo run new evaluation jobs, visit Hugging Face's automatic model evaluator.## Contributions\n\nThanks to @lewtun for evaluating this model."
] |
635f1f6b24ac85800a7c0b94c644fd888a5c8e4e | # Dataset Card for GoEmotions
## Table of Contents
- [Dataset Description](#dataset-description)
- [Dataset Summary](#dataset-summary)
- [Supported Tasks and Leaderboards](#supported-tasks-and-leaderboards)
- [Languages](#languages)
- [Dataset Structure](#dataset-structure)
- [Data Instances](#data-instances)
- [Data Fields](#data-fields)
- [Data Splits](#data-splits)
- [Dataset Creation](#dataset-creation)
- [Curation Rationale](#curation-rationale)
- [Source Data](#source-data)
- [Annotations](#annotations)
- [Personal and Sensitive Information](#personal-and-sensitive-information)
- [Considerations for Using the Data](#considerations-for-using-the-data)
- [Social Impact of Dataset](#social-impact-of-dataset)
- [Discussion of Biases](#discussion-of-biases)
- [Other Known Limitations](#other-known-limitations)
- [Additional Information](#additional-information)
- [Dataset Curators](#dataset-curators)
- [Licensing Information](#licensing-information)
- [Citation Information](#citation-information)
- [Contributions](#contributions)
## Dataset Description
- **Homepage:** https://github.com/google-research/google-research/tree/master/goemotions
- **Repository:** https://github.com/google-research/google-research/tree/master/goemotions
- **Paper:** https://arxiv.org/abs/2005.00547
- **Leaderboard:**
- **Point of Contact:** [Dora Demszky](https://nlp.stanford.edu/~ddemszky/index.html)
### Dataset Summary
The GoEmotions dataset contains 58k carefully curated Reddit comments labeled for 27 emotion categories or Neutral.
The raw data is included as well as the smaller, simplified version of the dataset with predefined train/val/test
splits.
### Supported Tasks and Leaderboards
This dataset is intended for multi-class, multi-label emotion classification.
### Languages
The data is in English and Brazilian Portuguese (translated by Google Translator).
## Dataset Structure
### Data Instances
Each instance is a reddit comment with a corresponding ID and one or more emotion annotations (or neutral).
### Data Fields
The simplified configuration includes:
- `text`: the reddit comment
- `texto`: the reddit comment in portuguese
- `labels`: the emotion annotations
- `comment_id`: unique identifier of the comment (can be used to look up the entry in the raw dataset)
In addition to the above, the raw data includes:
* `author`: The Reddit username of the comment's author.
* `subreddit`: The subreddit that the comment belongs to.
* `link_id`: The link id of the comment.
* `parent_id`: The parent id of the comment.
* `created_utc`: The timestamp of the comment.
* `rater_id`: The unique id of the annotator.
* `example_very_unclear`: Whether the annotator marked the example as being very unclear or difficult to label (in this
case they did not choose any emotion labels).
In the raw data, labels are listed as their own columns with binary 0/1 entries rather than a list of ids as in the
simplified data.
### Data Splits
The simplified data includes a set of train/val/test splits with 43,410, 5426, and 5427 examples respectively.
## Dataset Creation
### Curation Rationale
From the paper abstract:
> Understanding emotion expressed in language has a wide range of applications, from building empathetic chatbots to
detecting harmful online behavior. Advancement in this area can be improved using large-scale datasets with a
fine-grained typology, adaptable to multiple downstream tasks.
### Source Data
#### Initial Data Collection and Normalization
Data was collected from Reddit comments via a variety of automated methods discussed in 3.1 of the paper.
#### Who are the source language producers?
English-speaking Reddit users.
### Annotations
#### Annotation process
[More Information Needed]
#### Who are the annotators?
Annotations were produced by 3 English-speaking crowdworkers in India.
### Personal and Sensitive Information
This dataset includes the original usernames of the Reddit users who posted each comment. Although Reddit usernames
are typically disasociated from personal real-world identities, this is not always the case. It may therefore be
possible to discover the identities of the individuals who created this content in some cases.
## Considerations for Using the Data
### Social Impact of Dataset
Emotion detection is a worthwhile problem which can potentially lead to improvements such as better human/computer
interaction. However, emotion detection algorithms (particularly in computer vision) have been abused in some cases
to make erroneous inferences in human monitoring and assessment applications such as hiring decisions, insurance
pricing, and student attentiveness (see
[this article](https://www.unite.ai/ai-now-institute-warns-about-misuse-of-emotion-detection-software-and-other-ethical-issues/)).
### Discussion of Biases
From the authors' github page:
> Potential biases in the data include: Inherent biases in Reddit and user base biases, the offensive/vulgar word lists used for data filtering, inherent or unconscious bias in assessment of offensive identity labels, annotators were all native English speakers from India. All these likely affect labelling, precision, and recall for a trained model. Anyone using this dataset should be aware of these limitations of the dataset.
### Other Known Limitations
[More Information Needed]
## Additional Information
### Dataset Curators
Researchers at Amazon Alexa, Google Research, and Stanford. See the [author list](https://arxiv.org/abs/2005.00547).
### Licensing Information
The GitHub repository which houses this dataset has an
[Apache License 2.0](https://github.com/google-research/google-research/blob/master/LICENSE).
### Citation Information
@inproceedings{demszky2020goemotions,
author = {Demszky, Dorottya and Movshovitz-Attias, Dana and Ko, Jeongwoo and Cowen, Alan and Nemade, Gaurav and Ravi, Sujith},
booktitle = {58th Annual Meeting of the Association for Computational Linguistics (ACL)},
title = {{GoEmotions: A Dataset of Fine-Grained Emotions}},
year = {2020}
}
### Contributions
Thanks to [@joeddav](https://github.com/joeddav) for adding this dataset.
Thanks to [@antoniomenezes](https://github.com/antoniomenezes) for extending this dataset. | antoniomenezes/go_emotions_ptbr | [
"task_categories:text-classification",
"task_ids:multi-class-classification",
"task_ids:multi-label-classification",
"annotations_creators:crowdsourced",
"language_creators:found",
"multilinguality:2 languages",
"size_categories:100K<n<1M",
"size_categories:10K<n<100K",
"source_datasets:modified",
"language:en",
"language:pt",
"license:apache-2.0",
"emotion",
"arxiv:2005.00547",
"region:us"
] | 2022-11-21T13:38:59+00:00 | {"annotations_creators": ["crowdsourced"], "language_creators": ["found"], "language": ["en", "pt"], "license": ["apache-2.0"], "multilinguality": ["2 languages"], "size_categories": ["100K<n<1M", "10K<n<100K"], "source_datasets": ["modified"], "task_categories": ["text-classification"], "task_ids": ["multi-class-classification", "multi-label-classification"], "paperswithcode_id": "goemotions", "pretty_name": "GoEmotions", "configs": ["raw", "simplified"], "tags": ["emotion"], "dataset_info": [{"config_name": "raw", "features": [{"name": "text", "dtype": "string"}, {"name": "id", "dtype": "string"}, {"name": "author", "dtype": "string"}, {"name": "subreddit", "dtype": "string"}, {"name": "link_id", "dtype": "string"}, {"name": "parent_id", "dtype": "string"}, {"name": "created_utc", "dtype": "float32"}, {"name": "rater_id", "dtype": "int32"}, {"name": "example_very_unclear", "dtype": "bool"}, {"name": "admiration", "dtype": "int32"}, {"name": "amusement", "dtype": "int32"}, {"name": "anger", "dtype": "int32"}, {"name": "annoyance", "dtype": "int32"}, {"name": "approval", "dtype": "int32"}, {"name": "caring", "dtype": "int32"}, {"name": "confusion", "dtype": "int32"}, {"name": "curiosity", "dtype": "int32"}, {"name": "desire", "dtype": "int32"}, {"name": "disappointment", "dtype": "int32"}, {"name": "disapproval", "dtype": "int32"}, {"name": "disgust", "dtype": "int32"}, {"name": "embarrassment", "dtype": "int32"}, {"name": "excitement", "dtype": "int32"}, {"name": "fear", "dtype": "int32"}, {"name": "gratitude", "dtype": "int32"}, {"name": "grief", "dtype": "int32"}, {"name": "joy", "dtype": "int32"}, {"name": "love", "dtype": "int32"}, {"name": "nervousness", "dtype": "int32"}, {"name": "optimism", "dtype": "int32"}, {"name": "pride", "dtype": "int32"}, {"name": "realization", "dtype": "int32"}, {"name": "relief", "dtype": "int32"}, {"name": "remorse", "dtype": "int32"}, {"name": "sadness", "dtype": "int32"}, {"name": "surprise", "dtype": "int32"}, {"name": "neutral", "dtype": "int32"}, {"name": "texto", "dtype": "string"}], "splits": [{"name": "train", "num_bytes": 55343630, "num_examples": 211225}], "download_size": 42742918, "dataset_size": 55343630}, {"config_name": "simplified", "features": [{"name": "text", "dtype": "string"}, {"name": "labels", "sequence": {"class_label": {"names": {"0": "admiration", "1": "amusement", "2": "anger", "3": "annoyance", "4": "approval", "5": "caring", "6": "confusion", "7": "curiosity", "8": "desire", "9": "disappointment", "10": "disapproval", "11": "disgust", "12": "embarrassment", "13": "excitement", "14": "fear", "15": "gratitude", "16": "grief", "17": "joy", "18": "love", "19": "nervousness", "20": "optimism", "21": "pride", "22": "realization", "23": "relief", "24": "remorse", "25": "sadness", "26": "surprise", "27": "neutral"}}}}, {"name": "id", "dtype": "string"}], "splits": [{"name": "train", "num_bytes": 4224198, "num_examples": 43410}, {"name": "validation", "num_bytes": 527131, "num_examples": 5426}, {"name": "test", "num_bytes": 524455, "num_examples": 5427}], "download_size": 4394818, "dataset_size": 5275784}]} | 2022-11-21T14:27:31+00:00 | [
"2005.00547"
] | [
"en",
"pt"
] | TAGS
#task_categories-text-classification #task_ids-multi-class-classification #task_ids-multi-label-classification #annotations_creators-crowdsourced #language_creators-found #multilinguality-2 languages #size_categories-100K<n<1M #size_categories-10K<n<100K #source_datasets-modified #language-English #language-Portuguese #license-apache-2.0 #emotion #arxiv-2005.00547 #region-us
| # Dataset Card for GoEmotions
## Table of Contents
- Dataset Description
- Dataset Summary
- Supported Tasks and Leaderboards
- Languages
- Dataset Structure
- Data Instances
- Data Fields
- Data Splits
- Dataset Creation
- Curation Rationale
- Source Data
- Annotations
- Personal and Sensitive Information
- Considerations for Using the Data
- Social Impact of Dataset
- Discussion of Biases
- Other Known Limitations
- Additional Information
- Dataset Curators
- Licensing Information
- Citation Information
- Contributions
## Dataset Description
- Homepage: URL
- Repository: URL
- Paper: URL
- Leaderboard:
- Point of Contact: Dora Demszky
### Dataset Summary
The GoEmotions dataset contains 58k carefully curated Reddit comments labeled for 27 emotion categories or Neutral.
The raw data is included as well as the smaller, simplified version of the dataset with predefined train/val/test
splits.
### Supported Tasks and Leaderboards
This dataset is intended for multi-class, multi-label emotion classification.
### Languages
The data is in English and Brazilian Portuguese (translated by Google Translator).
## Dataset Structure
### Data Instances
Each instance is a reddit comment with a corresponding ID and one or more emotion annotations (or neutral).
### Data Fields
The simplified configuration includes:
- 'text': the reddit comment
- 'texto': the reddit comment in portuguese
- 'labels': the emotion annotations
- 'comment_id': unique identifier of the comment (can be used to look up the entry in the raw dataset)
In addition to the above, the raw data includes:
* 'author': The Reddit username of the comment's author.
* 'subreddit': The subreddit that the comment belongs to.
* 'link_id': The link id of the comment.
* 'parent_id': The parent id of the comment.
* 'created_utc': The timestamp of the comment.
* 'rater_id': The unique id of the annotator.
* 'example_very_unclear': Whether the annotator marked the example as being very unclear or difficult to label (in this
case they did not choose any emotion labels).
In the raw data, labels are listed as their own columns with binary 0/1 entries rather than a list of ids as in the
simplified data.
### Data Splits
The simplified data includes a set of train/val/test splits with 43,410, 5426, and 5427 examples respectively.
## Dataset Creation
### Curation Rationale
From the paper abstract:
> Understanding emotion expressed in language has a wide range of applications, from building empathetic chatbots to
detecting harmful online behavior. Advancement in this area can be improved using large-scale datasets with a
fine-grained typology, adaptable to multiple downstream tasks.
### Source Data
#### Initial Data Collection and Normalization
Data was collected from Reddit comments via a variety of automated methods discussed in 3.1 of the paper.
#### Who are the source language producers?
English-speaking Reddit users.
### Annotations
#### Annotation process
#### Who are the annotators?
Annotations were produced by 3 English-speaking crowdworkers in India.
### Personal and Sensitive Information
This dataset includes the original usernames of the Reddit users who posted each comment. Although Reddit usernames
are typically disasociated from personal real-world identities, this is not always the case. It may therefore be
possible to discover the identities of the individuals who created this content in some cases.
## Considerations for Using the Data
### Social Impact of Dataset
Emotion detection is a worthwhile problem which can potentially lead to improvements such as better human/computer
interaction. However, emotion detection algorithms (particularly in computer vision) have been abused in some cases
to make erroneous inferences in human monitoring and assessment applications such as hiring decisions, insurance
pricing, and student attentiveness (see
this article).
### Discussion of Biases
From the authors' github page:
> Potential biases in the data include: Inherent biases in Reddit and user base biases, the offensive/vulgar word lists used for data filtering, inherent or unconscious bias in assessment of offensive identity labels, annotators were all native English speakers from India. All these likely affect labelling, precision, and recall for a trained model. Anyone using this dataset should be aware of these limitations of the dataset.
### Other Known Limitations
## Additional Information
### Dataset Curators
Researchers at Amazon Alexa, Google Research, and Stanford. See the author list.
### Licensing Information
The GitHub repository which houses this dataset has an
Apache License 2.0.
@inproceedings{demszky2020goemotions,
author = {Demszky, Dorottya and Movshovitz-Attias, Dana and Ko, Jeongwoo and Cowen, Alan and Nemade, Gaurav and Ravi, Sujith},
booktitle = {58th Annual Meeting of the Association for Computational Linguistics (ACL)},
title = {{GoEmotions: A Dataset of Fine-Grained Emotions}},
year = {2020}
}
### Contributions
Thanks to @joeddav for adding this dataset.
Thanks to @antoniomenezes for extending this dataset. | [
"# Dataset Card for GoEmotions",
"## Table of Contents\n- Dataset Description\n - Dataset Summary\n - Supported Tasks and Leaderboards\n - Languages\n- Dataset Structure\n - Data Instances\n - Data Fields\n - Data Splits\n- Dataset Creation\n - Curation Rationale\n - Source Data\n - Annotations\n - Personal and Sensitive Information\n- Considerations for Using the Data\n - Social Impact of Dataset\n - Discussion of Biases\n - Other Known Limitations\n- Additional Information\n - Dataset Curators\n - Licensing Information\n - Citation Information\n - Contributions",
"## Dataset Description\n- Homepage: URL\n- Repository: URL\n- Paper: URL\n- Leaderboard:\n- Point of Contact: Dora Demszky",
"### Dataset Summary\nThe GoEmotions dataset contains 58k carefully curated Reddit comments labeled for 27 emotion categories or Neutral.\nThe raw data is included as well as the smaller, simplified version of the dataset with predefined train/val/test\nsplits.",
"### Supported Tasks and Leaderboards\nThis dataset is intended for multi-class, multi-label emotion classification.",
"### Languages\nThe data is in English and Brazilian Portuguese (translated by Google Translator).",
"## Dataset Structure",
"### Data Instances\nEach instance is a reddit comment with a corresponding ID and one or more emotion annotations (or neutral).",
"### Data Fields\nThe simplified configuration includes:\n- 'text': the reddit comment\n- 'texto': the reddit comment in portuguese\n- 'labels': the emotion annotations\n- 'comment_id': unique identifier of the comment (can be used to look up the entry in the raw dataset)\nIn addition to the above, the raw data includes:\n* 'author': The Reddit username of the comment's author.\n* 'subreddit': The subreddit that the comment belongs to.\n* 'link_id': The link id of the comment.\n* 'parent_id': The parent id of the comment.\n* 'created_utc': The timestamp of the comment.\n* 'rater_id': The unique id of the annotator.\n* 'example_very_unclear': Whether the annotator marked the example as being very unclear or difficult to label (in this\ncase they did not choose any emotion labels).\nIn the raw data, labels are listed as their own columns with binary 0/1 entries rather than a list of ids as in the\nsimplified data.",
"### Data Splits\nThe simplified data includes a set of train/val/test splits with 43,410, 5426, and 5427 examples respectively.",
"## Dataset Creation",
"### Curation Rationale\nFrom the paper abstract:\n> Understanding emotion expressed in language has a wide range of applications, from building empathetic chatbots to\ndetecting harmful online behavior. Advancement in this area can be improved using large-scale datasets with a\nfine-grained typology, adaptable to multiple downstream tasks.",
"### Source Data",
"#### Initial Data Collection and Normalization\nData was collected from Reddit comments via a variety of automated methods discussed in 3.1 of the paper.",
"#### Who are the source language producers?\nEnglish-speaking Reddit users.",
"### Annotations",
"#### Annotation process",
"#### Who are the annotators?\nAnnotations were produced by 3 English-speaking crowdworkers in India.",
"### Personal and Sensitive Information\nThis dataset includes the original usernames of the Reddit users who posted each comment. Although Reddit usernames\nare typically disasociated from personal real-world identities, this is not always the case. It may therefore be\npossible to discover the identities of the individuals who created this content in some cases.",
"## Considerations for Using the Data",
"### Social Impact of Dataset\nEmotion detection is a worthwhile problem which can potentially lead to improvements such as better human/computer\ninteraction. However, emotion detection algorithms (particularly in computer vision) have been abused in some cases\nto make erroneous inferences in human monitoring and assessment applications such as hiring decisions, insurance\npricing, and student attentiveness (see\nthis article).",
"### Discussion of Biases\nFrom the authors' github page:\n> Potential biases in the data include: Inherent biases in Reddit and user base biases, the offensive/vulgar word lists used for data filtering, inherent or unconscious bias in assessment of offensive identity labels, annotators were all native English speakers from India. All these likely affect labelling, precision, and recall for a trained model. Anyone using this dataset should be aware of these limitations of the dataset.",
"### Other Known Limitations",
"## Additional Information",
"### Dataset Curators\nResearchers at Amazon Alexa, Google Research, and Stanford. See the author list.",
"### Licensing Information\nThe GitHub repository which houses this dataset has an\nApache License 2.0.\n\n@inproceedings{demszky2020goemotions,\n author = {Demszky, Dorottya and Movshovitz-Attias, Dana and Ko, Jeongwoo and Cowen, Alan and Nemade, Gaurav and Ravi, Sujith},\n booktitle = {58th Annual Meeting of the Association for Computational Linguistics (ACL)},\n title = {{GoEmotions: A Dataset of Fine-Grained Emotions}},\n year = {2020}\n}",
"### Contributions\nThanks to @joeddav for adding this dataset.\nThanks to @antoniomenezes for extending this dataset."
] | [
"TAGS\n#task_categories-text-classification #task_ids-multi-class-classification #task_ids-multi-label-classification #annotations_creators-crowdsourced #language_creators-found #multilinguality-2 languages #size_categories-100K<n<1M #size_categories-10K<n<100K #source_datasets-modified #language-English #language-Portuguese #license-apache-2.0 #emotion #arxiv-2005.00547 #region-us \n",
"# Dataset Card for GoEmotions",
"## Table of Contents\n- Dataset Description\n - Dataset Summary\n - Supported Tasks and Leaderboards\n - Languages\n- Dataset Structure\n - Data Instances\n - Data Fields\n - Data Splits\n- Dataset Creation\n - Curation Rationale\n - Source Data\n - Annotations\n - Personal and Sensitive Information\n- Considerations for Using the Data\n - Social Impact of Dataset\n - Discussion of Biases\n - Other Known Limitations\n- Additional Information\n - Dataset Curators\n - Licensing Information\n - Citation Information\n - Contributions",
"## Dataset Description\n- Homepage: URL\n- Repository: URL\n- Paper: URL\n- Leaderboard:\n- Point of Contact: Dora Demszky",
"### Dataset Summary\nThe GoEmotions dataset contains 58k carefully curated Reddit comments labeled for 27 emotion categories or Neutral.\nThe raw data is included as well as the smaller, simplified version of the dataset with predefined train/val/test\nsplits.",
"### Supported Tasks and Leaderboards\nThis dataset is intended for multi-class, multi-label emotion classification.",
"### Languages\nThe data is in English and Brazilian Portuguese (translated by Google Translator).",
"## Dataset Structure",
"### Data Instances\nEach instance is a reddit comment with a corresponding ID and one or more emotion annotations (or neutral).",
"### Data Fields\nThe simplified configuration includes:\n- 'text': the reddit comment\n- 'texto': the reddit comment in portuguese\n- 'labels': the emotion annotations\n- 'comment_id': unique identifier of the comment (can be used to look up the entry in the raw dataset)\nIn addition to the above, the raw data includes:\n* 'author': The Reddit username of the comment's author.\n* 'subreddit': The subreddit that the comment belongs to.\n* 'link_id': The link id of the comment.\n* 'parent_id': The parent id of the comment.\n* 'created_utc': The timestamp of the comment.\n* 'rater_id': The unique id of the annotator.\n* 'example_very_unclear': Whether the annotator marked the example as being very unclear or difficult to label (in this\ncase they did not choose any emotion labels).\nIn the raw data, labels are listed as their own columns with binary 0/1 entries rather than a list of ids as in the\nsimplified data.",
"### Data Splits\nThe simplified data includes a set of train/val/test splits with 43,410, 5426, and 5427 examples respectively.",
"## Dataset Creation",
"### Curation Rationale\nFrom the paper abstract:\n> Understanding emotion expressed in language has a wide range of applications, from building empathetic chatbots to\ndetecting harmful online behavior. Advancement in this area can be improved using large-scale datasets with a\nfine-grained typology, adaptable to multiple downstream tasks.",
"### Source Data",
"#### Initial Data Collection and Normalization\nData was collected from Reddit comments via a variety of automated methods discussed in 3.1 of the paper.",
"#### Who are the source language producers?\nEnglish-speaking Reddit users.",
"### Annotations",
"#### Annotation process",
"#### Who are the annotators?\nAnnotations were produced by 3 English-speaking crowdworkers in India.",
"### Personal and Sensitive Information\nThis dataset includes the original usernames of the Reddit users who posted each comment. Although Reddit usernames\nare typically disasociated from personal real-world identities, this is not always the case. It may therefore be\npossible to discover the identities of the individuals who created this content in some cases.",
"## Considerations for Using the Data",
"### Social Impact of Dataset\nEmotion detection is a worthwhile problem which can potentially lead to improvements such as better human/computer\ninteraction. However, emotion detection algorithms (particularly in computer vision) have been abused in some cases\nto make erroneous inferences in human monitoring and assessment applications such as hiring decisions, insurance\npricing, and student attentiveness (see\nthis article).",
"### Discussion of Biases\nFrom the authors' github page:\n> Potential biases in the data include: Inherent biases in Reddit and user base biases, the offensive/vulgar word lists used for data filtering, inherent or unconscious bias in assessment of offensive identity labels, annotators were all native English speakers from India. All these likely affect labelling, precision, and recall for a trained model. Anyone using this dataset should be aware of these limitations of the dataset.",
"### Other Known Limitations",
"## Additional Information",
"### Dataset Curators\nResearchers at Amazon Alexa, Google Research, and Stanford. See the author list.",
"### Licensing Information\nThe GitHub repository which houses this dataset has an\nApache License 2.0.\n\n@inproceedings{demszky2020goemotions,\n author = {Demszky, Dorottya and Movshovitz-Attias, Dana and Ko, Jeongwoo and Cowen, Alan and Nemade, Gaurav and Ravi, Sujith},\n booktitle = {58th Annual Meeting of the Association for Computational Linguistics (ACL)},\n title = {{GoEmotions: A Dataset of Fine-Grained Emotions}},\n year = {2020}\n}",
"### Contributions\nThanks to @joeddav for adding this dataset.\nThanks to @antoniomenezes for extending this dataset."
] | [
130,
9,
120,
31,
65,
27,
25,
6,
29,
263,
37,
5,
79,
4,
32,
18,
5,
5,
27,
72,
8,
87,
121,
7,
5,
24,
140,
31
] | [
"passage: TAGS\n#task_categories-text-classification #task_ids-multi-class-classification #task_ids-multi-label-classification #annotations_creators-crowdsourced #language_creators-found #multilinguality-2 languages #size_categories-100K<n<1M #size_categories-10K<n<100K #source_datasets-modified #language-English #language-Portuguese #license-apache-2.0 #emotion #arxiv-2005.00547 #region-us \n# Dataset Card for GoEmotions## Table of Contents\n- Dataset Description\n - Dataset Summary\n - Supported Tasks and Leaderboards\n - Languages\n- Dataset Structure\n - Data Instances\n - Data Fields\n - Data Splits\n- Dataset Creation\n - Curation Rationale\n - Source Data\n - Annotations\n - Personal and Sensitive Information\n- Considerations for Using the Data\n - Social Impact of Dataset\n - Discussion of Biases\n - Other Known Limitations\n- Additional Information\n - Dataset Curators\n - Licensing Information\n - Citation Information\n - Contributions## Dataset Description\n- Homepage: URL\n- Repository: URL\n- Paper: URL\n- Leaderboard:\n- Point of Contact: Dora Demszky### Dataset Summary\nThe GoEmotions dataset contains 58k carefully curated Reddit comments labeled for 27 emotion categories or Neutral.\nThe raw data is included as well as the smaller, simplified version of the dataset with predefined train/val/test\nsplits.### Supported Tasks and Leaderboards\nThis dataset is intended for multi-class, multi-label emotion classification.### Languages\nThe data is in English and Brazilian Portuguese (translated by Google Translator).## Dataset Structure### Data Instances\nEach instance is a reddit comment with a corresponding ID and one or more emotion annotations (or neutral).",
"passage: ### Data Fields\nThe simplified configuration includes:\n- 'text': the reddit comment\n- 'texto': the reddit comment in portuguese\n- 'labels': the emotion annotations\n- 'comment_id': unique identifier of the comment (can be used to look up the entry in the raw dataset)\nIn addition to the above, the raw data includes:\n* 'author': The Reddit username of the comment's author.\n* 'subreddit': The subreddit that the comment belongs to.\n* 'link_id': The link id of the comment.\n* 'parent_id': The parent id of the comment.\n* 'created_utc': The timestamp of the comment.\n* 'rater_id': The unique id of the annotator.\n* 'example_very_unclear': Whether the annotator marked the example as being very unclear or difficult to label (in this\ncase they did not choose any emotion labels).\nIn the raw data, labels are listed as their own columns with binary 0/1 entries rather than a list of ids as in the\nsimplified data.### Data Splits\nThe simplified data includes a set of train/val/test splits with 43,410, 5426, and 5427 examples respectively.## Dataset Creation### Curation Rationale\nFrom the paper abstract:\n> Understanding emotion expressed in language has a wide range of applications, from building empathetic chatbots to\ndetecting harmful online behavior. Advancement in this area can be improved using large-scale datasets with a\nfine-grained typology, adaptable to multiple downstream tasks.### Source Data#### Initial Data Collection and Normalization\nData was collected from Reddit comments via a variety of automated methods discussed in 3.1 of the paper.#### Who are the source language producers?\nEnglish-speaking Reddit users.### Annotations#### Annotation process#### Who are the annotators?\nAnnotations were produced by 3 English-speaking crowdworkers in India.### Personal and Sensitive Information\nThis dataset includes the original usernames of the Reddit users who posted each comment. Although Reddit usernames\nare typically disasociated from personal real-world identities, this is not always the case. It may therefore be\npossible to discover the identities of the individuals who created this content in some cases.## Considerations for Using the Data### Social Impact of Dataset\nEmotion detection is a worthwhile problem which can potentially lead to improvements such as better human/computer\ninteraction. However, emotion detection algorithms (particularly in computer vision) have been abused in some cases\nto make erroneous inferences in human monitoring and assessment applications such as hiring decisions, insurance\npricing, and student attentiveness (see\nthis article).### Discussion of Biases\nFrom the authors' github page:\n> Potential biases in the data include: Inherent biases in Reddit and user base biases, the offensive/vulgar word lists used for data filtering, inherent or unconscious bias in assessment of offensive identity labels, annotators were all native English speakers from India. All these likely affect labelling, precision, and recall for a trained model. Anyone using this dataset should be aware of these limitations of the dataset.### Other Known Limitations"
] |
467f37cd03184724d1eed773bd22e1ef43df859e | # Dataset Card for AutoTrain Evaluator
This repository contains model predictions generated by [AutoTrain](https://huggingface.co/autotrain) for the following task and dataset:
* Task: Natural Language Inference
* Model: autoevaluate/natural-language-inference
* Dataset: glue
* Config: mrpc
* Split: validation
To run new evaluation jobs, visit Hugging Face's [automatic model evaluator](https://huggingface.co/spaces/autoevaluate/model-evaluator).
## Contributions
Thanks to [@lewtun](https://huggingface.co/lewtun) for evaluating this model. | autoevaluate/autoeval-staging-eval-project-fa97c361-989b-438c-bd2b-73aa1588c214-5654 | [
"autotrain",
"evaluation",
"region:us"
] | 2022-11-21T13:45:42+00:00 | {"type": "predictions", "tags": ["autotrain", "evaluation"], "datasets": ["glue"], "eval_info": {"task": "natural_language_inference", "model": "autoevaluate/natural-language-inference", "metrics": [], "dataset_name": "glue", "dataset_config": "mrpc", "dataset_split": "validation", "col_mapping": {"text1": "sentence1", "text2": "sentence2", "target": "label"}}} | 2022-11-21T13:46:15+00:00 | [] | [] | TAGS
#autotrain #evaluation #region-us
| # Dataset Card for AutoTrain Evaluator
This repository contains model predictions generated by AutoTrain for the following task and dataset:
* Task: Natural Language Inference
* Model: autoevaluate/natural-language-inference
* Dataset: glue
* Config: mrpc
* Split: validation
To run new evaluation jobs, visit Hugging Face's automatic model evaluator.
## Contributions
Thanks to @lewtun for evaluating this model. | [
"# Dataset Card for AutoTrain Evaluator\n\nThis repository contains model predictions generated by AutoTrain for the following task and dataset:\n\n* Task: Natural Language Inference\n* Model: autoevaluate/natural-language-inference\n* Dataset: glue\n* Config: mrpc\n* Split: validation\n\nTo run new evaluation jobs, visit Hugging Face's automatic model evaluator.",
"## Contributions\n\nThanks to @lewtun for evaluating this model."
] | [
"TAGS\n#autotrain #evaluation #region-us \n",
"# Dataset Card for AutoTrain Evaluator\n\nThis repository contains model predictions generated by AutoTrain for the following task and dataset:\n\n* Task: Natural Language Inference\n* Model: autoevaluate/natural-language-inference\n* Dataset: glue\n* Config: mrpc\n* Split: validation\n\nTo run new evaluation jobs, visit Hugging Face's automatic model evaluator.",
"## Contributions\n\nThanks to @lewtun for evaluating this model."
] | [
13,
90,
15
] | [
"passage: TAGS\n#autotrain #evaluation #region-us \n# Dataset Card for AutoTrain Evaluator\n\nThis repository contains model predictions generated by AutoTrain for the following task and dataset:\n\n* Task: Natural Language Inference\n* Model: autoevaluate/natural-language-inference\n* Dataset: glue\n* Config: mrpc\n* Split: validation\n\nTo run new evaluation jobs, visit Hugging Face's automatic model evaluator.## Contributions\n\nThanks to @lewtun for evaluating this model."
] |
6d71faac5cd426ecd355ddafd2967db90635b9ea | # Dataset Card for AutoTrain Evaluator
This repository contains model predictions generated by [AutoTrain](https://huggingface.co/autotrain) for the following task and dataset:
* Task: Natural Language Inference
* Model: autoevaluate/natural-language-inference
* Dataset: glue
* Config: mrpc
* Split: validation
To run new evaluation jobs, visit Hugging Face's [automatic model evaluator](https://huggingface.co/spaces/autoevaluate/model-evaluator).
## Contributions
Thanks to [@lewtun](https://huggingface.co/lewtun) for evaluating this model. | autoevaluate/autoeval-staging-eval-project-03e83e3b-2528-4e84-b075-34edd28549da-5755 | [
"autotrain",
"evaluation",
"region:us"
] | 2022-11-21T13:53:36+00:00 | {"type": "predictions", "tags": ["autotrain", "evaluation"], "datasets": ["glue"], "eval_info": {"task": "natural_language_inference", "model": "autoevaluate/natural-language-inference", "metrics": [], "dataset_name": "glue", "dataset_config": "mrpc", "dataset_split": "validation", "col_mapping": {"text1": "sentence1", "text2": "sentence2", "target": "label"}}} | 2022-11-21T13:54:09+00:00 | [] | [] | TAGS
#autotrain #evaluation #region-us
| # Dataset Card for AutoTrain Evaluator
This repository contains model predictions generated by AutoTrain for the following task and dataset:
* Task: Natural Language Inference
* Model: autoevaluate/natural-language-inference
* Dataset: glue
* Config: mrpc
* Split: validation
To run new evaluation jobs, visit Hugging Face's automatic model evaluator.
## Contributions
Thanks to @lewtun for evaluating this model. | [
"# Dataset Card for AutoTrain Evaluator\n\nThis repository contains model predictions generated by AutoTrain for the following task and dataset:\n\n* Task: Natural Language Inference\n* Model: autoevaluate/natural-language-inference\n* Dataset: glue\n* Config: mrpc\n* Split: validation\n\nTo run new evaluation jobs, visit Hugging Face's automatic model evaluator.",
"## Contributions\n\nThanks to @lewtun for evaluating this model."
] | [
"TAGS\n#autotrain #evaluation #region-us \n",
"# Dataset Card for AutoTrain Evaluator\n\nThis repository contains model predictions generated by AutoTrain for the following task and dataset:\n\n* Task: Natural Language Inference\n* Model: autoevaluate/natural-language-inference\n* Dataset: glue\n* Config: mrpc\n* Split: validation\n\nTo run new evaluation jobs, visit Hugging Face's automatic model evaluator.",
"## Contributions\n\nThanks to @lewtun for evaluating this model."
] | [
13,
90,
15
] | [
"passage: TAGS\n#autotrain #evaluation #region-us \n# Dataset Card for AutoTrain Evaluator\n\nThis repository contains model predictions generated by AutoTrain for the following task and dataset:\n\n* Task: Natural Language Inference\n* Model: autoevaluate/natural-language-inference\n* Dataset: glue\n* Config: mrpc\n* Split: validation\n\nTo run new evaluation jobs, visit Hugging Face's automatic model evaluator.## Contributions\n\nThanks to @lewtun for evaluating this model."
] |
7b042f2d8fa83b6c30cd8077fb5ce1e0840d2600 | # Dataset Card for AutoTrain Evaluator
This repository contains model predictions generated by [AutoTrain](https://huggingface.co/autotrain) for the following task and dataset:
* Task: Natural Language Inference
* Model: autoevaluate/natural-language-inference
* Dataset: glue
* Config: mrpc
* Split: validation
To run new evaluation jobs, visit Hugging Face's [automatic model evaluator](https://huggingface.co/spaces/autoevaluate/model-evaluator).
## Contributions
Thanks to [@lewtun](https://huggingface.co/lewtun) for evaluating this model. | autoevaluate/autoeval-staging-eval-project-6ba515cb-e9ef-49fe-9bb8-a4281c03605e-5856 | [
"autotrain",
"evaluation",
"region:us"
] | 2022-11-21T14:04:10+00:00 | {"type": "predictions", "tags": ["autotrain", "evaluation"], "datasets": ["glue"], "eval_info": {"task": "natural_language_inference", "model": "autoevaluate/natural-language-inference", "metrics": [], "dataset_name": "glue", "dataset_config": "mrpc", "dataset_split": "validation", "col_mapping": {"text1": "sentence1", "text2": "sentence2", "target": "label"}}} | 2022-11-21T14:04:47+00:00 | [] | [] | TAGS
#autotrain #evaluation #region-us
| # Dataset Card for AutoTrain Evaluator
This repository contains model predictions generated by AutoTrain for the following task and dataset:
* Task: Natural Language Inference
* Model: autoevaluate/natural-language-inference
* Dataset: glue
* Config: mrpc
* Split: validation
To run new evaluation jobs, visit Hugging Face's automatic model evaluator.
## Contributions
Thanks to @lewtun for evaluating this model. | [
"# Dataset Card for AutoTrain Evaluator\n\nThis repository contains model predictions generated by AutoTrain for the following task and dataset:\n\n* Task: Natural Language Inference\n* Model: autoevaluate/natural-language-inference\n* Dataset: glue\n* Config: mrpc\n* Split: validation\n\nTo run new evaluation jobs, visit Hugging Face's automatic model evaluator.",
"## Contributions\n\nThanks to @lewtun for evaluating this model."
] | [
"TAGS\n#autotrain #evaluation #region-us \n",
"# Dataset Card for AutoTrain Evaluator\n\nThis repository contains model predictions generated by AutoTrain for the following task and dataset:\n\n* Task: Natural Language Inference\n* Model: autoevaluate/natural-language-inference\n* Dataset: glue\n* Config: mrpc\n* Split: validation\n\nTo run new evaluation jobs, visit Hugging Face's automatic model evaluator.",
"## Contributions\n\nThanks to @lewtun for evaluating this model."
] | [
13,
90,
15
] | [
"passage: TAGS\n#autotrain #evaluation #region-us \n# Dataset Card for AutoTrain Evaluator\n\nThis repository contains model predictions generated by AutoTrain for the following task and dataset:\n\n* Task: Natural Language Inference\n* Model: autoevaluate/natural-language-inference\n* Dataset: glue\n* Config: mrpc\n* Split: validation\n\nTo run new evaluation jobs, visit Hugging Face's automatic model evaluator.## Contributions\n\nThanks to @lewtun for evaluating this model."
] |
4705079801edd2cacb314c86ce69c593934a1ab3 | # Dataset Card for AutoTrain Evaluator
This repository contains model predictions generated by [AutoTrain](https://huggingface.co/autotrain) for the following task and dataset:
* Task: Natural Language Inference
* Model: autoevaluate/natural-language-inference
* Dataset: glue
* Config: mrpc
* Split: validation
To run new evaluation jobs, visit Hugging Face's [automatic model evaluator](https://huggingface.co/spaces/autoevaluate/model-evaluator).
## Contributions
Thanks to [@lewtun](https://huggingface.co/lewtun) for evaluating this model. | autoevaluate/autoeval-staging-eval-project-371fafb5-556b-4565-a7bf-530b1396895e-5957 | [
"autotrain",
"evaluation",
"region:us"
] | 2022-11-21T14:10:27+00:00 | {"type": "predictions", "tags": ["autotrain", "evaluation"], "datasets": ["glue"], "eval_info": {"task": "natural_language_inference", "model": "autoevaluate/natural-language-inference", "metrics": [], "dataset_name": "glue", "dataset_config": "mrpc", "dataset_split": "validation", "col_mapping": {"text1": "sentence1", "text2": "sentence2", "target": "label"}}} | 2022-11-21T14:11:01+00:00 | [] | [] | TAGS
#autotrain #evaluation #region-us
| # Dataset Card for AutoTrain Evaluator
This repository contains model predictions generated by AutoTrain for the following task and dataset:
* Task: Natural Language Inference
* Model: autoevaluate/natural-language-inference
* Dataset: glue
* Config: mrpc
* Split: validation
To run new evaluation jobs, visit Hugging Face's automatic model evaluator.
## Contributions
Thanks to @lewtun for evaluating this model. | [
"# Dataset Card for AutoTrain Evaluator\n\nThis repository contains model predictions generated by AutoTrain for the following task and dataset:\n\n* Task: Natural Language Inference\n* Model: autoevaluate/natural-language-inference\n* Dataset: glue\n* Config: mrpc\n* Split: validation\n\nTo run new evaluation jobs, visit Hugging Face's automatic model evaluator.",
"## Contributions\n\nThanks to @lewtun for evaluating this model."
] | [
"TAGS\n#autotrain #evaluation #region-us \n",
"# Dataset Card for AutoTrain Evaluator\n\nThis repository contains model predictions generated by AutoTrain for the following task and dataset:\n\n* Task: Natural Language Inference\n* Model: autoevaluate/natural-language-inference\n* Dataset: glue\n* Config: mrpc\n* Split: validation\n\nTo run new evaluation jobs, visit Hugging Face's automatic model evaluator.",
"## Contributions\n\nThanks to @lewtun for evaluating this model."
] | [
13,
90,
15
] | [
"passage: TAGS\n#autotrain #evaluation #region-us \n# Dataset Card for AutoTrain Evaluator\n\nThis repository contains model predictions generated by AutoTrain for the following task and dataset:\n\n* Task: Natural Language Inference\n* Model: autoevaluate/natural-language-inference\n* Dataset: glue\n* Config: mrpc\n* Split: validation\n\nTo run new evaluation jobs, visit Hugging Face's automatic model evaluator.## Contributions\n\nThanks to @lewtun for evaluating this model."
] |
418264ca3343afbed3297ae861a96d352032d339 | # Dataset Card for AutoTrain Evaluator
This repository contains model predictions generated by [AutoTrain](https://huggingface.co/autotrain) for the following task and dataset:
* Task: Token Classification
* Model: autoevaluate/entity-extraction
* Dataset: autoevaluate/conll2003-sample
* Config: autoevaluate--conll2003-sample
* Split: test
To run new evaluation jobs, visit Hugging Face's [automatic model evaluator](https://huggingface.co/spaces/autoevaluate/model-evaluator).
## Contributions
Thanks to [@lewtun](https://huggingface.co/lewtun) for evaluating this model. | autoevaluate/autoeval-staging-eval-project-e2e28e52-014f-41d6-a473-008f1f5e4d3d-6058 | [
"autotrain",
"evaluation",
"region:us"
] | 2022-11-21T14:15:02+00:00 | {"type": "predictions", "tags": ["autotrain", "evaluation"], "datasets": ["autoevaluate/conll2003-sample"], "eval_info": {"task": "entity_extraction", "model": "autoevaluate/entity-extraction", "metrics": [], "dataset_name": "autoevaluate/conll2003-sample", "dataset_config": "autoevaluate--conll2003-sample", "dataset_split": "test", "col_mapping": {"tokens": "tokens", "tags": "ner_tags"}}} | 2022-11-21T14:15:39+00:00 | [] | [] | TAGS
#autotrain #evaluation #region-us
| # Dataset Card for AutoTrain Evaluator
This repository contains model predictions generated by AutoTrain for the following task and dataset:
* Task: Token Classification
* Model: autoevaluate/entity-extraction
* Dataset: autoevaluate/conll2003-sample
* Config: autoevaluate--conll2003-sample
* Split: test
To run new evaluation jobs, visit Hugging Face's automatic model evaluator.
## Contributions
Thanks to @lewtun for evaluating this model. | [
"# Dataset Card for AutoTrain Evaluator\n\nThis repository contains model predictions generated by AutoTrain for the following task and dataset:\n\n* Task: Token Classification\n* Model: autoevaluate/entity-extraction\n* Dataset: autoevaluate/conll2003-sample\n* Config: autoevaluate--conll2003-sample\n* Split: test\n\nTo run new evaluation jobs, visit Hugging Face's automatic model evaluator.",
"## Contributions\n\nThanks to @lewtun for evaluating this model."
] | [
"TAGS\n#autotrain #evaluation #region-us \n",
"# Dataset Card for AutoTrain Evaluator\n\nThis repository contains model predictions generated by AutoTrain for the following task and dataset:\n\n* Task: Token Classification\n* Model: autoevaluate/entity-extraction\n* Dataset: autoevaluate/conll2003-sample\n* Config: autoevaluate--conll2003-sample\n* Split: test\n\nTo run new evaluation jobs, visit Hugging Face's automatic model evaluator.",
"## Contributions\n\nThanks to @lewtun for evaluating this model."
] | [
13,
103,
15
] | [
"passage: TAGS\n#autotrain #evaluation #region-us \n# Dataset Card for AutoTrain Evaluator\n\nThis repository contains model predictions generated by AutoTrain for the following task and dataset:\n\n* Task: Token Classification\n* Model: autoevaluate/entity-extraction\n* Dataset: autoevaluate/conll2003-sample\n* Config: autoevaluate--conll2003-sample\n* Split: test\n\nTo run new evaluation jobs, visit Hugging Face's automatic model evaluator.## Contributions\n\nThanks to @lewtun for evaluating this model."
] |
cd348cda88fc5bf04f04e1cf878a6093fadb96ee | # Dataset Card for AutoTrain Evaluator
This repository contains model predictions generated by [AutoTrain](https://huggingface.co/autotrain) for the following task and dataset:
* Task: Token Classification
* Model: autoevaluate/entity-extraction
* Dataset: autoevaluate/conll2003-sample
* Config: autoevaluate--conll2003-sample
* Split: test
To run new evaluation jobs, visit Hugging Face's [automatic model evaluator](https://huggingface.co/spaces/autoevaluate/model-evaluator).
## Contributions
Thanks to [@lewtun](https://huggingface.co/lewtun) for evaluating this model. | autoevaluate/autoeval-staging-eval-project-19f625bb-a07b-4f3a-bec2-d734d6029176-6159 | [
"autotrain",
"evaluation",
"region:us"
] | 2022-11-21T14:18:39+00:00 | {"type": "predictions", "tags": ["autotrain", "evaluation"], "datasets": ["autoevaluate/conll2003-sample"], "eval_info": {"task": "entity_extraction", "model": "autoevaluate/entity-extraction", "metrics": [], "dataset_name": "autoevaluate/conll2003-sample", "dataset_config": "autoevaluate--conll2003-sample", "dataset_split": "test", "col_mapping": {"tokens": "tokens", "tags": "ner_tags"}}} | 2022-11-21T14:19:12+00:00 | [] | [] | TAGS
#autotrain #evaluation #region-us
| # Dataset Card for AutoTrain Evaluator
This repository contains model predictions generated by AutoTrain for the following task and dataset:
* Task: Token Classification
* Model: autoevaluate/entity-extraction
* Dataset: autoevaluate/conll2003-sample
* Config: autoevaluate--conll2003-sample
* Split: test
To run new evaluation jobs, visit Hugging Face's automatic model evaluator.
## Contributions
Thanks to @lewtun for evaluating this model. | [
"# Dataset Card for AutoTrain Evaluator\n\nThis repository contains model predictions generated by AutoTrain for the following task and dataset:\n\n* Task: Token Classification\n* Model: autoevaluate/entity-extraction\n* Dataset: autoevaluate/conll2003-sample\n* Config: autoevaluate--conll2003-sample\n* Split: test\n\nTo run new evaluation jobs, visit Hugging Face's automatic model evaluator.",
"## Contributions\n\nThanks to @lewtun for evaluating this model."
] | [
"TAGS\n#autotrain #evaluation #region-us \n",
"# Dataset Card for AutoTrain Evaluator\n\nThis repository contains model predictions generated by AutoTrain for the following task and dataset:\n\n* Task: Token Classification\n* Model: autoevaluate/entity-extraction\n* Dataset: autoevaluate/conll2003-sample\n* Config: autoevaluate--conll2003-sample\n* Split: test\n\nTo run new evaluation jobs, visit Hugging Face's automatic model evaluator.",
"## Contributions\n\nThanks to @lewtun for evaluating this model."
] | [
13,
103,
15
] | [
"passage: TAGS\n#autotrain #evaluation #region-us \n# Dataset Card for AutoTrain Evaluator\n\nThis repository contains model predictions generated by AutoTrain for the following task and dataset:\n\n* Task: Token Classification\n* Model: autoevaluate/entity-extraction\n* Dataset: autoevaluate/conll2003-sample\n* Config: autoevaluate--conll2003-sample\n* Split: test\n\nTo run new evaluation jobs, visit Hugging Face's automatic model evaluator.## Contributions\n\nThanks to @lewtun for evaluating this model."
] |
54f67708bcbadd0e7197a5a1381583eb4939bbd7 |
These word embeddings were computed using the POLAR technique to reproject 'common' word embeddings into roundabout 700 interpretable dimensions of polar opposites (i.e. good/bad).
I just used their scripts here:
https://github.com/Sandipan99/POLAR
I applied those on the wikidata5m embeddings, 5 million knowledge graph embeddings (SimplE).
https://graphvite.io/docs/latest/pretrained_model.html
As the model became too huge, I further filtered it for overlap with fasttext embedding tokens.
Not all dimensions make sense, this is a work in progress.
I intend to remove dimensions which turn out to not make sense, when using them. | KnutJaegersberg/interpretable_word_embeddings | [
"license:mit",
"region:us"
] | 2022-11-21T14:21:38+00:00 | {"license": "mit"} | 2022-11-25T12:10:11+00:00 | [] | [] | TAGS
#license-mit #region-us
|
These word embeddings were computed using the POLAR technique to reproject 'common' word embeddings into roundabout 700 interpretable dimensions of polar opposites (i.e. good/bad).
I just used their scripts here:
URL
I applied those on the wikidata5m embeddings, 5 million knowledge graph embeddings (SimplE).
URL
As the model became too huge, I further filtered it for overlap with fasttext embedding tokens.
Not all dimensions make sense, this is a work in progress.
I intend to remove dimensions which turn out to not make sense, when using them. | [] | [
"TAGS\n#license-mit #region-us \n"
] | [
11
] | [
"passage: TAGS\n#license-mit #region-us \n"
] |
1625be97f42d20fac451bac1daa4f69cb1374594 | # Dataset Card for AutoTrain Evaluator
This repository contains model predictions generated by [AutoTrain](https://huggingface.co/autotrain) for the following task and dataset:
* Task: Question Answering
* Model: autoevaluate/extractive-question-answering
* Dataset: autoevaluate/squad-sample
* Config: autoevaluate--squad-sample
* Split: test
To run new evaluation jobs, visit Hugging Face's [automatic model evaluator](https://huggingface.co/spaces/autoevaluate/model-evaluator).
## Contributions
Thanks to [@lewtun](https://huggingface.co/lewtun) for evaluating this model. | autoevaluate/autoeval-staging-eval-project-e438add5-1e56-41ec-9c26-2ad4182383b0-6260 | [
"autotrain",
"evaluation",
"region:us"
] | 2022-11-21T14:27:41+00:00 | {"type": "predictions", "tags": ["autotrain", "evaluation"], "datasets": ["autoevaluate/squad-sample"], "eval_info": {"task": "extractive_question_answering", "model": "autoevaluate/extractive-question-answering", "metrics": [], "dataset_name": "autoevaluate/squad-sample", "dataset_config": "autoevaluate--squad-sample", "dataset_split": "test", "col_mapping": {"context": "context", "question": "question", "answers-text": "answers.text", "answers-answer_start": "answers.answer_start"}}} | 2022-11-21T14:28:17+00:00 | [] | [] | TAGS
#autotrain #evaluation #region-us
| # Dataset Card for AutoTrain Evaluator
This repository contains model predictions generated by AutoTrain for the following task and dataset:
* Task: Question Answering
* Model: autoevaluate/extractive-question-answering
* Dataset: autoevaluate/squad-sample
* Config: autoevaluate--squad-sample
* Split: test
To run new evaluation jobs, visit Hugging Face's automatic model evaluator.
## Contributions
Thanks to @lewtun for evaluating this model. | [
"# Dataset Card for AutoTrain Evaluator\n\nThis repository contains model predictions generated by AutoTrain for the following task and dataset:\n\n* Task: Question Answering\n* Model: autoevaluate/extractive-question-answering\n* Dataset: autoevaluate/squad-sample\n* Config: autoevaluate--squad-sample\n* Split: test\n\nTo run new evaluation jobs, visit Hugging Face's automatic model evaluator.",
"## Contributions\n\nThanks to @lewtun for evaluating this model."
] | [
"TAGS\n#autotrain #evaluation #region-us \n",
"# Dataset Card for AutoTrain Evaluator\n\nThis repository contains model predictions generated by AutoTrain for the following task and dataset:\n\n* Task: Question Answering\n* Model: autoevaluate/extractive-question-answering\n* Dataset: autoevaluate/squad-sample\n* Config: autoevaluate--squad-sample\n* Split: test\n\nTo run new evaluation jobs, visit Hugging Face's automatic model evaluator.",
"## Contributions\n\nThanks to @lewtun for evaluating this model."
] | [
13,
104,
15
] | [
"passage: TAGS\n#autotrain #evaluation #region-us \n# Dataset Card for AutoTrain Evaluator\n\nThis repository contains model predictions generated by AutoTrain for the following task and dataset:\n\n* Task: Question Answering\n* Model: autoevaluate/extractive-question-answering\n* Dataset: autoevaluate/squad-sample\n* Config: autoevaluate--squad-sample\n* Split: test\n\nTo run new evaluation jobs, visit Hugging Face's automatic model evaluator.## Contributions\n\nThanks to @lewtun for evaluating this model."
] |
29eb805ff0abb2bc32f0b8404f59c4c1d071b909 | # Dataset Card for AutoTrain Evaluator
This repository contains model predictions generated by [AutoTrain](https://huggingface.co/autotrain) for the following task and dataset:
* Task: Summarization
* Model: autoevaluate/summarization
* Dataset: autoevaluate/xsum-sample
* Config: autoevaluate--xsum-sample
* Split: test
To run new evaluation jobs, visit Hugging Face's [automatic model evaluator](https://huggingface.co/spaces/autoevaluate/model-evaluator).
## Contributions
Thanks to [@lewtun](https://huggingface.co/lewtun) for evaluating this model. | autoevaluate/autoeval-staging-eval-project-b40c7dea-3c58-4f26-a941-b0221649edda-6362 | [
"autotrain",
"evaluation",
"region:us"
] | 2022-11-21T14:34:51+00:00 | {"type": "predictions", "tags": ["autotrain", "evaluation"], "datasets": ["autoevaluate/xsum-sample"], "eval_info": {"task": "summarization", "model": "autoevaluate/summarization", "metrics": [], "dataset_name": "autoevaluate/xsum-sample", "dataset_config": "autoevaluate--xsum-sample", "dataset_split": "test", "col_mapping": {"text": "document", "target": "summary"}}} | 2022-11-21T14:35:26+00:00 | [] | [] | TAGS
#autotrain #evaluation #region-us
| # Dataset Card for AutoTrain Evaluator
This repository contains model predictions generated by AutoTrain for the following task and dataset:
* Task: Summarization
* Model: autoevaluate/summarization
* Dataset: autoevaluate/xsum-sample
* Config: autoevaluate--xsum-sample
* Split: test
To run new evaluation jobs, visit Hugging Face's automatic model evaluator.
## Contributions
Thanks to @lewtun for evaluating this model. | [
"# Dataset Card for AutoTrain Evaluator\n\nThis repository contains model predictions generated by AutoTrain for the following task and dataset:\n\n* Task: Summarization\n* Model: autoevaluate/summarization\n* Dataset: autoevaluate/xsum-sample\n* Config: autoevaluate--xsum-sample\n* Split: test\n\nTo run new evaluation jobs, visit Hugging Face's automatic model evaluator.",
"## Contributions\n\nThanks to @lewtun for evaluating this model."
] | [
"TAGS\n#autotrain #evaluation #region-us \n",
"# Dataset Card for AutoTrain Evaluator\n\nThis repository contains model predictions generated by AutoTrain for the following task and dataset:\n\n* Task: Summarization\n* Model: autoevaluate/summarization\n* Dataset: autoevaluate/xsum-sample\n* Config: autoevaluate--xsum-sample\n* Split: test\n\nTo run new evaluation jobs, visit Hugging Face's automatic model evaluator.",
"## Contributions\n\nThanks to @lewtun for evaluating this model."
] | [
13,
98,
15
] | [
"passage: TAGS\n#autotrain #evaluation #region-us \n# Dataset Card for AutoTrain Evaluator\n\nThis repository contains model predictions generated by AutoTrain for the following task and dataset:\n\n* Task: Summarization\n* Model: autoevaluate/summarization\n* Dataset: autoevaluate/xsum-sample\n* Config: autoevaluate--xsum-sample\n* Split: test\n\nTo run new evaluation jobs, visit Hugging Face's automatic model evaluator.## Contributions\n\nThanks to @lewtun for evaluating this model."
] |
46db4b474918671e3a3a724a45cbed26d2c29e11 | # Dataset Card for "un_dataset"
[More Information needed](https://github.com/huggingface/datasets/blob/main/CONTRIBUTING.md#how-to-contribute-to-the-dataset-cards) | MikCil/un_dataset | [
"region:us"
] | 2022-11-21T14:44:54+00:00 | {"dataset_info": {"features": [{"name": "image", "dtype": "image"}, {"name": "caption", "dtype": "string"}], "splits": [{"name": "train", "num_bytes": 462328883.0, "num_examples": 904}, {"name": "validation", "num_bytes": 462328883.0, "num_examples": 904}], "download_size": 924654154, "dataset_size": 924657766.0}} | 2022-11-21T14:45:38+00:00 | [] | [] | TAGS
#region-us
| # Dataset Card for "un_dataset"
More Information needed | [
"# Dataset Card for \"un_dataset\"\n\nMore Information needed"
] | [
"TAGS\n#region-us \n",
"# Dataset Card for \"un_dataset\"\n\nMore Information needed"
] | [
6,
14
] | [
"passage: TAGS\n#region-us \n# Dataset Card for \"un_dataset\"\n\nMore Information needed"
] |
d69cafc5e82166290baea1a5a500a679d52d136a | # Dataset Card for AutoTrain Evaluator
This repository contains model predictions generated by [AutoTrain](https://huggingface.co/autotrain) for the following task and dataset:
* Task: Summarization
* Model: autoevaluate/summarization
* Dataset: autoevaluate/xsum-sample
* Config: autoevaluate--xsum-sample
* Split: test
To run new evaluation jobs, visit Hugging Face's [automatic model evaluator](https://huggingface.co/spaces/autoevaluate/model-evaluator).
## Contributions
Thanks to [@lewtun](https://huggingface.co/lewtun) for evaluating this model. | autoevaluate/autoeval-staging-eval-project-71db21e5-84e8-4c03-8b5c-360b48e9252b-6463 | [
"autotrain",
"evaluation",
"region:us"
] | 2022-11-21T14:45:33+00:00 | {"type": "predictions", "tags": ["autotrain", "evaluation"], "datasets": ["autoevaluate/xsum-sample"], "eval_info": {"task": "summarization", "model": "autoevaluate/summarization", "metrics": [], "dataset_name": "autoevaluate/xsum-sample", "dataset_config": "autoevaluate--xsum-sample", "dataset_split": "test", "col_mapping": {"text": "document", "target": "summary"}}} | 2022-11-21T14:46:06+00:00 | [] | [] | TAGS
#autotrain #evaluation #region-us
| # Dataset Card for AutoTrain Evaluator
This repository contains model predictions generated by AutoTrain for the following task and dataset:
* Task: Summarization
* Model: autoevaluate/summarization
* Dataset: autoevaluate/xsum-sample
* Config: autoevaluate--xsum-sample
* Split: test
To run new evaluation jobs, visit Hugging Face's automatic model evaluator.
## Contributions
Thanks to @lewtun for evaluating this model. | [
"# Dataset Card for AutoTrain Evaluator\n\nThis repository contains model predictions generated by AutoTrain for the following task and dataset:\n\n* Task: Summarization\n* Model: autoevaluate/summarization\n* Dataset: autoevaluate/xsum-sample\n* Config: autoevaluate--xsum-sample\n* Split: test\n\nTo run new evaluation jobs, visit Hugging Face's automatic model evaluator.",
"## Contributions\n\nThanks to @lewtun for evaluating this model."
] | [
"TAGS\n#autotrain #evaluation #region-us \n",
"# Dataset Card for AutoTrain Evaluator\n\nThis repository contains model predictions generated by AutoTrain for the following task and dataset:\n\n* Task: Summarization\n* Model: autoevaluate/summarization\n* Dataset: autoevaluate/xsum-sample\n* Config: autoevaluate--xsum-sample\n* Split: test\n\nTo run new evaluation jobs, visit Hugging Face's automatic model evaluator.",
"## Contributions\n\nThanks to @lewtun for evaluating this model."
] | [
13,
98,
15
] | [
"passage: TAGS\n#autotrain #evaluation #region-us \n# Dataset Card for AutoTrain Evaluator\n\nThis repository contains model predictions generated by AutoTrain for the following task and dataset:\n\n* Task: Summarization\n* Model: autoevaluate/summarization\n* Dataset: autoevaluate/xsum-sample\n* Config: autoevaluate--xsum-sample\n* Split: test\n\nTo run new evaluation jobs, visit Hugging Face's automatic model evaluator.## Contributions\n\nThanks to @lewtun for evaluating this model."
] |
d61906525b859c05cae3fce0e2e7f4a6727de7fb | # Dataset Card for AutoTrain Evaluator
This repository contains model predictions generated by [AutoTrain](https://huggingface.co/autotrain) for the following task and dataset:
* Task: Translation
* Model: autoevaluate/translation
* Dataset: autoevaluate/wmt16-ro-en-sample
* Config: autoevaluate--wmt16-ro-en-sample
* Split: test
To run new evaluation jobs, visit Hugging Face's [automatic model evaluator](https://huggingface.co/spaces/autoevaluate/model-evaluator).
## Contributions
Thanks to [@lewtun](https://huggingface.co/lewtun) for evaluating this model. | autoevaluate/autoeval-staging-eval-project-b07c55ed-3514-4853-9004-f2d3a7737d92-6564 | [
"autotrain",
"evaluation",
"region:us"
] | 2022-11-21T14:48:30+00:00 | {"type": "predictions", "tags": ["autotrain", "evaluation"], "datasets": ["autoevaluate/wmt16-ro-en-sample"], "eval_info": {"task": "translation", "model": "autoevaluate/translation", "metrics": [], "dataset_name": "autoevaluate/wmt16-ro-en-sample", "dataset_config": "autoevaluate--wmt16-ro-en-sample", "dataset_split": "test", "col_mapping": {"source": "translation.ro", "target": "translation.en"}}} | 2022-11-21T14:49:16+00:00 | [] | [] | TAGS
#autotrain #evaluation #region-us
| # Dataset Card for AutoTrain Evaluator
This repository contains model predictions generated by AutoTrain for the following task and dataset:
* Task: Translation
* Model: autoevaluate/translation
* Dataset: autoevaluate/wmt16-ro-en-sample
* Config: autoevaluate--wmt16-ro-en-sample
* Split: test
To run new evaluation jobs, visit Hugging Face's automatic model evaluator.
## Contributions
Thanks to @lewtun for evaluating this model. | [
"# Dataset Card for AutoTrain Evaluator\n\nThis repository contains model predictions generated by AutoTrain for the following task and dataset:\n\n* Task: Translation\n* Model: autoevaluate/translation\n* Dataset: autoevaluate/wmt16-ro-en-sample\n* Config: autoevaluate--wmt16-ro-en-sample\n* Split: test\n\nTo run new evaluation jobs, visit Hugging Face's automatic model evaluator.",
"## Contributions\n\nThanks to @lewtun for evaluating this model."
] | [
"TAGS\n#autotrain #evaluation #region-us \n",
"# Dataset Card for AutoTrain Evaluator\n\nThis repository contains model predictions generated by AutoTrain for the following task and dataset:\n\n* Task: Translation\n* Model: autoevaluate/translation\n* Dataset: autoevaluate/wmt16-ro-en-sample\n* Config: autoevaluate--wmt16-ro-en-sample\n* Split: test\n\nTo run new evaluation jobs, visit Hugging Face's automatic model evaluator.",
"## Contributions\n\nThanks to @lewtun for evaluating this model."
] | [
13,
105,
15
] | [
"passage: TAGS\n#autotrain #evaluation #region-us \n# Dataset Card for AutoTrain Evaluator\n\nThis repository contains model predictions generated by AutoTrain for the following task and dataset:\n\n* Task: Translation\n* Model: autoevaluate/translation\n* Dataset: autoevaluate/wmt16-ro-en-sample\n* Config: autoevaluate--wmt16-ro-en-sample\n* Split: test\n\nTo run new evaluation jobs, visit Hugging Face's automatic model evaluator.## Contributions\n\nThanks to @lewtun for evaluating this model."
] |
875de01ed5e2bbf6860b847bd91cc01ef198eb74 |
# Dataset Card for Brazilian Portuguese Sentiment Analysis Dataset
## Table of Contents
- [Table of Contents](#table-of-contents)
- [Dataset Description](#dataset-description)
- [Dataset Summary](#dataset-summary)
- [Supported Tasks and Leaderboards](#supported-tasks-and-leaderboards)
- [Languages](#languages)
- [Dataset Structure](#dataset-structure)
- [Data Instances](#data-instances)
- [Data Fields](#data-fields)
- [Data Splits](#data-splits)
- [Dataset Creation](#dataset-creation)
- [Curation Rationale](#curation-rationale)
- [Source Data](#source-data)
- [Annotations](#annotations)
- [Personal and Sensitive Information](#personal-and-sensitive-information)
- [Considerations for Using the Data](#considerations-for-using-the-data)
- [Social Impact of Dataset](#social-impact-of-dataset)
- [Discussion of Biases](#discussion-of-biases)
- [Other Known Limitations](#other-known-limitations)
- [Additional Information](#additional-information)
- [Dataset Curators](#dataset-curators)
- [Licensing Information](#licensing-information)
- [Citation Information](#citation-information)
- [Contributions](#contributions)
## Dataset Description
- **Homepage:** [Kaggle Dataset](https://www.kaggle.com/datasets/fredericods/ptbr-sentiment-analysis-datasets)
- **Paper:** [Sentiment Analysis on Brazilian Portuguese User Reviews](https://ieeexplore.ieee.org/abstract/document/9769838)
- **Point of Contact:** [Frederico Dias Souza]([email protected])
### Dataset Summary
**Disclaimer:** *The team releasing the dataset did not write a dataset card
for this dataset so this dataset card has been written by the contributors.*
The Brazilian Portuguese Sentiment Analysis Dataset (BPSAD) is composed
by the concatenation of 5 differents sources (Olist, B2W Digital, Buscapé,
UTLC-Apps and UTLC-Movies), each one is composed by evaluation sentences
classified according to the polarity (0: negative; 1: positive) and ratings
(1, 2, 3, 4 and 5 stars).
This dataset requires manual download:
1. Download the `concatenated` file from dataset homepage.
2. Extract the file inside `<path/to/manual/data>`.
3. Load the dataset using the command:
```python
datasets.load_dataset(
path="lm4pt/bpsad",
name='<polarity|rating>',
data_dir='<path/to/manual/data>')
```
A detailed description about the dataset and the processing steps can be
found at the [dataset homepage](https://www.kaggle.com/datasets/fredericods/ptbr-sentiment-analysis-datasets).
### Supported Tasks and Leaderboards
The dataset contains two configurations that represents the possible tasks
related to sentiment analysis. The polarity classification is a binary
classification problem where the sentences must be classified as positive (1)
or negative (0) reviews. The rating prediction is a multiclass problem
with values ranging from 1 to 5 stars.
### Languages
The texts are in Brazilian Portuguese, as spoken by users of different e-commerces and Filmow social network.
## Dataset Structure
### Data Instances
#### polarity
```
{
"review_text": "Bem macio e felpudo...recomendo. Preço imbatível e entrega rápida. Compraria outro quando precisar",
"polarity": 1
}
```
#### rating
```
{
"review_text": "Bem macio e felpudo...recomendo. Preço imbatível e entrega rápida. Compraria outro quando precisar",
"rating": 4
}
```
### Data Fields
#### polarity
- `review_text`: a `string` feature with product or movie review.
- `polarity`: an `integer` value that represents positive (1) or negative (0) reviews.
#### rating
- `review_text`: a `string` feature with product or movie review.
- `rating`: an `integer` value that represents the number of stars given by the reviewer. Possible values are 1, 2, 3, 4 and 5.
### Data Splits
Data splits are created based on the original `kfold` column of each configuration, following the original authors recomendations:
- train: folds 1 to 8
- validation: fold 9
- test: fold 10
| | train | validation | test |
|----------|--------:|-----------:|-------:|
| polarity | 1908937 | 238614 | 238613 |
| rating | 2228877 | 278608 | 278607 |
More information about sentence size and label distribution can be found in the [dataset homepage](https://www.kaggle.com/datasets/fredericods/ptbr-sentiment-analysis-datasets).
## Dataset Creation
### Curation Rationale
[More Information Needed]
### Source Data
#### Initial Data Collection and Normalization
[More Information Needed]
#### Who are the source language producers?
[More Information Needed]
### Annotations
#### Annotation process
[More Information Needed]
#### Who are the annotators?
[More Information Needed]
### Personal and Sensitive Information
[More Information Needed]
## Considerations for Using the Data
### Social Impact of Dataset
[More Information Needed]
### Discussion of Biases
[More Information Needed]
### Other Known Limitations
[More Information Needed]
## Additional Information
### Dataset Curators
[More Information Needed]
### Licensing Information
[More Information Needed]
### Citation Information
```
@inproceedings{souza2021sentiment,
author={
Souza, Frederico Dias and
Baptista de Oliveira e Souza Filho, João},
booktitle={
2021 IEEE Latin American Conference on
Computational Intelligence (LA-CCI)},
title={
Sentiment Analysis on Brazilian Portuguese User Reviews},
year={2021},
pages={1-6},
doi={10.1109/LA-CCI48322.2021.9769838}
}
```
### Contributions
Thanks to [@guilhermelmello](https://huggingface.co/guilhermelmello) and [@DominguesPH](https://huggingface.co/DominguesPH) for adding this dataset. | lm4pt/bpsad | [
"task_categories:text-classification",
"task_ids:multi-class-classification",
"task_ids:sentiment-classification",
"task_ids:sentiment-scoring",
"task_ids:sentiment-analysis",
"language_creators:other",
"multilinguality:monolingual",
"size_categories:1M<n<10M",
"language:pt",
"license:unknown",
"region:us"
] | 2022-11-21T15:37:12+00:00 | {"annotations_creators": [], "language_creators": ["other"], "language": ["pt"], "license": ["unknown"], "multilinguality": ["monolingual"], "size_categories": ["1M<n<10M"], "source_datasets": [], "task_categories": ["text-classification"], "task_ids": ["multi-class-classification", "sentiment-classification", "sentiment-scoring", "sentiment-analysis"], "pretty_name": "bpsad", "tags": []} | 2022-11-23T19:20:11+00:00 | [] | [
"pt"
] | TAGS
#task_categories-text-classification #task_ids-multi-class-classification #task_ids-sentiment-classification #task_ids-sentiment-scoring #task_ids-sentiment-analysis #language_creators-other #multilinguality-monolingual #size_categories-1M<n<10M #language-Portuguese #license-unknown #region-us
| Dataset Card for Brazilian Portuguese Sentiment Analysis Dataset
================================================================
Table of Contents
-----------------
* Table of Contents
* Dataset Description
+ Dataset Summary
+ Supported Tasks and Leaderboards
+ Languages
* Dataset Structure
+ Data Instances
+ Data Fields
+ Data Splits
* Dataset Creation
+ Curation Rationale
+ Source Data
+ Annotations
+ Personal and Sensitive Information
* Considerations for Using the Data
+ Social Impact of Dataset
+ Discussion of Biases
+ Other Known Limitations
* Additional Information
+ Dataset Curators
+ Licensing Information
+ Citation Information
+ Contributions
Dataset Description
-------------------
* Homepage: Kaggle Dataset
* Paper: Sentiment Analysis on Brazilian Portuguese User Reviews
* Point of Contact: Frederico Dias Souza
### Dataset Summary
Disclaimer: *The team releasing the dataset did not write a dataset card
for this dataset so this dataset card has been written by the contributors.*
The Brazilian Portuguese Sentiment Analysis Dataset (BPSAD) is composed
by the concatenation of 5 differents sources (Olist, B2W Digital, Buscapé,
UTLC-Apps and UTLC-Movies), each one is composed by evaluation sentences
classified according to the polarity (0: negative; 1: positive) and ratings
(1, 2, 3, 4 and 5 stars).
This dataset requires manual download:
1. Download the 'concatenated' file from dataset homepage.
2. Extract the file inside '<path/to/manual/data>'.
3. Load the dataset using the command:
A detailed description about the dataset and the processing steps can be
found at the dataset homepage.
### Supported Tasks and Leaderboards
The dataset contains two configurations that represents the possible tasks
related to sentiment analysis. The polarity classification is a binary
classification problem where the sentences must be classified as positive (1)
or negative (0) reviews. The rating prediction is a multiclass problem
with values ranging from 1 to 5 stars.
### Languages
The texts are in Brazilian Portuguese, as spoken by users of different e-commerces and Filmow social network.
Dataset Structure
-----------------
### Data Instances
#### polarity
#### rating
### Data Fields
#### polarity
* 'review\_text': a 'string' feature with product or movie review.
* 'polarity': an 'integer' value that represents positive (1) or negative (0) reviews.
#### rating
* 'review\_text': a 'string' feature with product or movie review.
* 'rating': an 'integer' value that represents the number of stars given by the reviewer. Possible values are 1, 2, 3, 4 and 5.
### Data Splits
Data splits are created based on the original 'kfold' column of each configuration, following the original authors recomendations:
* train: folds 1 to 8
* validation: fold 9
* test: fold 10
More information about sentence size and label distribution can be found in the dataset homepage.
Dataset Creation
----------------
### Curation Rationale
### Source Data
#### Initial Data Collection and Normalization
#### Who are the source language producers?
### Annotations
#### Annotation process
#### Who are the annotators?
### Personal and Sensitive Information
Considerations for Using the Data
---------------------------------
### Social Impact of Dataset
### Discussion of Biases
### Other Known Limitations
Additional Information
----------------------
### Dataset Curators
### Licensing Information
### Contributions
Thanks to @guilhermelmello and @DominguesPH for adding this dataset.
| [
"### Dataset Summary\n\n\nDisclaimer: *The team releasing the dataset did not write a dataset card\nfor this dataset so this dataset card has been written by the contributors.*\n\n\nThe Brazilian Portuguese Sentiment Analysis Dataset (BPSAD) is composed\nby the concatenation of 5 differents sources (Olist, B2W Digital, Buscapé,\nUTLC-Apps and UTLC-Movies), each one is composed by evaluation sentences\nclassified according to the polarity (0: negative; 1: positive) and ratings\n(1, 2, 3, 4 and 5 stars).\n\n\nThis dataset requires manual download:\n\n\n1. Download the 'concatenated' file from dataset homepage.\n2. Extract the file inside '<path/to/manual/data>'.\n3. Load the dataset using the command:\n\n\nA detailed description about the dataset and the processing steps can be\nfound at the dataset homepage.",
"### Supported Tasks and Leaderboards\n\n\nThe dataset contains two configurations that represents the possible tasks\nrelated to sentiment analysis. The polarity classification is a binary\nclassification problem where the sentences must be classified as positive (1)\nor negative (0) reviews. The rating prediction is a multiclass problem\nwith values ranging from 1 to 5 stars.",
"### Languages\n\n\nThe texts are in Brazilian Portuguese, as spoken by users of different e-commerces and Filmow social network.\n\n\nDataset Structure\n-----------------",
"### Data Instances",
"#### polarity",
"#### rating",
"### Data Fields",
"#### polarity\n\n\n* 'review\\_text': a 'string' feature with product or movie review.\n* 'polarity': an 'integer' value that represents positive (1) or negative (0) reviews.",
"#### rating\n\n\n* 'review\\_text': a 'string' feature with product or movie review.\n* 'rating': an 'integer' value that represents the number of stars given by the reviewer. Possible values are 1, 2, 3, 4 and 5.",
"### Data Splits\n\n\nData splits are created based on the original 'kfold' column of each configuration, following the original authors recomendations:\n\n\n* train: folds 1 to 8\n* validation: fold 9\n* test: fold 10\n\n\n\nMore information about sentence size and label distribution can be found in the dataset homepage.\n\n\nDataset Creation\n----------------",
"### Curation Rationale",
"### Source Data",
"#### Initial Data Collection and Normalization",
"#### Who are the source language producers?",
"### Annotations",
"#### Annotation process",
"#### Who are the annotators?",
"### Personal and Sensitive Information\n\n\nConsiderations for Using the Data\n---------------------------------",
"### Social Impact of Dataset",
"### Discussion of Biases",
"### Other Known Limitations\n\n\nAdditional Information\n----------------------",
"### Dataset Curators",
"### Licensing Information",
"### Contributions\n\n\nThanks to @guilhermelmello and @DominguesPH for adding this dataset."
] | [
"TAGS\n#task_categories-text-classification #task_ids-multi-class-classification #task_ids-sentiment-classification #task_ids-sentiment-scoring #task_ids-sentiment-analysis #language_creators-other #multilinguality-monolingual #size_categories-1M<n<10M #language-Portuguese #license-unknown #region-us \n",
"### Dataset Summary\n\n\nDisclaimer: *The team releasing the dataset did not write a dataset card\nfor this dataset so this dataset card has been written by the contributors.*\n\n\nThe Brazilian Portuguese Sentiment Analysis Dataset (BPSAD) is composed\nby the concatenation of 5 differents sources (Olist, B2W Digital, Buscapé,\nUTLC-Apps and UTLC-Movies), each one is composed by evaluation sentences\nclassified according to the polarity (0: negative; 1: positive) and ratings\n(1, 2, 3, 4 and 5 stars).\n\n\nThis dataset requires manual download:\n\n\n1. Download the 'concatenated' file from dataset homepage.\n2. Extract the file inside '<path/to/manual/data>'.\n3. Load the dataset using the command:\n\n\nA detailed description about the dataset and the processing steps can be\nfound at the dataset homepage.",
"### Supported Tasks and Leaderboards\n\n\nThe dataset contains two configurations that represents the possible tasks\nrelated to sentiment analysis. The polarity classification is a binary\nclassification problem where the sentences must be classified as positive (1)\nor negative (0) reviews. The rating prediction is a multiclass problem\nwith values ranging from 1 to 5 stars.",
"### Languages\n\n\nThe texts are in Brazilian Portuguese, as spoken by users of different e-commerces and Filmow social network.\n\n\nDataset Structure\n-----------------",
"### Data Instances",
"#### polarity",
"#### rating",
"### Data Fields",
"#### polarity\n\n\n* 'review\\_text': a 'string' feature with product or movie review.\n* 'polarity': an 'integer' value that represents positive (1) or negative (0) reviews.",
"#### rating\n\n\n* 'review\\_text': a 'string' feature with product or movie review.\n* 'rating': an 'integer' value that represents the number of stars given by the reviewer. Possible values are 1, 2, 3, 4 and 5.",
"### Data Splits\n\n\nData splits are created based on the original 'kfold' column of each configuration, following the original authors recomendations:\n\n\n* train: folds 1 to 8\n* validation: fold 9\n* test: fold 10\n\n\n\nMore information about sentence size and label distribution can be found in the dataset homepage.\n\n\nDataset Creation\n----------------",
"### Curation Rationale",
"### Source Data",
"#### Initial Data Collection and Normalization",
"#### Who are the source language producers?",
"### Annotations",
"#### Annotation process",
"#### Who are the annotators?",
"### Personal and Sensitive Information\n\n\nConsiderations for Using the Data\n---------------------------------",
"### Social Impact of Dataset",
"### Discussion of Biases",
"### Other Known Limitations\n\n\nAdditional Information\n----------------------",
"### Dataset Curators",
"### Licensing Information",
"### Contributions\n\n\nThanks to @guilhermelmello and @DominguesPH for adding this dataset."
] | [
103,
210,
79,
39,
6,
5,
3,
5,
47,
60,
79,
7,
4,
10,
10,
5,
5,
9,
18,
7,
8,
14,
6,
6,
26
] | [
"passage: TAGS\n#task_categories-text-classification #task_ids-multi-class-classification #task_ids-sentiment-classification #task_ids-sentiment-scoring #task_ids-sentiment-analysis #language_creators-other #multilinguality-monolingual #size_categories-1M<n<10M #language-Portuguese #license-unknown #region-us \n### Dataset Summary\n\n\nDisclaimer: *The team releasing the dataset did not write a dataset card\nfor this dataset so this dataset card has been written by the contributors.*\n\n\nThe Brazilian Portuguese Sentiment Analysis Dataset (BPSAD) is composed\nby the concatenation of 5 differents sources (Olist, B2W Digital, Buscapé,\nUTLC-Apps and UTLC-Movies), each one is composed by evaluation sentences\nclassified according to the polarity (0: negative; 1: positive) and ratings\n(1, 2, 3, 4 and 5 stars).\n\n\nThis dataset requires manual download:\n\n\n1. Download the 'concatenated' file from dataset homepage.\n2. Extract the file inside '<path/to/manual/data>'.\n3. Load the dataset using the command:\n\n\nA detailed description about the dataset and the processing steps can be\nfound at the dataset homepage.### Supported Tasks and Leaderboards\n\n\nThe dataset contains two configurations that represents the possible tasks\nrelated to sentiment analysis. The polarity classification is a binary\nclassification problem where the sentences must be classified as positive (1)\nor negative (0) reviews. The rating prediction is a multiclass problem\nwith values ranging from 1 to 5 stars.### Languages\n\n\nThe texts are in Brazilian Portuguese, as spoken by users of different e-commerces and Filmow social network.\n\n\nDataset Structure\n-----------------### Data Instances#### polarity#### rating### Data Fields#### polarity\n\n\n* 'review\\_text': a 'string' feature with product or movie review.\n* 'polarity': an 'integer' value that represents positive (1) or negative (0) reviews."
] |
5aba6e36e57ad9618d0643c71876a1680b33aaa6 | # Dataset Card for "auto-mpg-split"
[More Information needed](https://github.com/huggingface/datasets/blob/main/CONTRIBUTING.md#how-to-contribute-to-the-dataset-cards) | dvgodoy/auto-mpg-split | [
"region:us"
] | 2022-11-21T16:28:39+00:00 | {"dataset_info": {"features": [{"name": "mpg", "dtype": "float64"}, {"name": "cylinders", "dtype": "int64"}, {"name": "displacement", "dtype": "float64"}, {"name": "horsepower", "dtype": "float64"}, {"name": "weight", "dtype": "int64"}, {"name": "acceleration", "dtype": "float64"}, {"name": "model year", "dtype": "int64"}, {"name": "origin", "dtype": "int64"}, {"name": "car name", "dtype": "string"}], "splits": [{"name": "train", "num_bytes": 26742.361809045226, "num_examples": 318}, {"name": "test", "num_bytes": 3363.819095477387, "num_examples": 40}, {"name": "valid", "num_bytes": 3363.819095477387, "num_examples": 40}], "download_size": 22370, "dataset_size": 33470.0}} | 2022-11-21T16:46:31+00:00 | [] | [] | TAGS
#region-us
| # Dataset Card for "auto-mpg-split"
More Information needed | [
"# Dataset Card for \"auto-mpg-split\"\n\nMore Information needed"
] | [
"TAGS\n#region-us \n",
"# Dataset Card for \"auto-mpg-split\"\n\nMore Information needed"
] | [
6,
17
] | [
"passage: TAGS\n#region-us \n# Dataset Card for \"auto-mpg-split\"\n\nMore Information needed"
] |
81b906121415bf962f67cec454b5ff5b56ae390a | # Dataset Card for "auto-mpg"
[More Information needed](https://github.com/huggingface/datasets/blob/main/CONTRIBUTING.md#how-to-contribute-to-the-dataset-cards) | dvgodoy/auto-mpg | [
"region:us"
] | 2022-11-21T16:37:13+00:00 | {"dataset_info": {"features": [{"name": "mpg", "dtype": "float64"}, {"name": "cylinders", "dtype": "int64"}, {"name": "displacement", "dtype": "float64"}, {"name": "horsepower", "dtype": "float64"}, {"name": "weight", "dtype": "int64"}, {"name": "acceleration", "dtype": "float64"}, {"name": "model year", "dtype": "int64"}, {"name": "origin", "dtype": "int64"}, {"name": "car name", "dtype": "string"}], "splits": [{"name": "train", "num_bytes": 33470, "num_examples": 398}], "download_size": 13036, "dataset_size": 33470}} | 2022-11-21T16:45:37+00:00 | [] | [] | TAGS
#region-us
| # Dataset Card for "auto-mpg"
More Information needed | [
"# Dataset Card for \"auto-mpg\"\n\nMore Information needed"
] | [
"TAGS\n#region-us \n",
"# Dataset Card for \"auto-mpg\"\n\nMore Information needed"
] | [
6,
14
] | [
"passage: TAGS\n#region-us \n# Dataset Card for \"auto-mpg\"\n\nMore Information needed"
] |
c04e1e124fc85ce94aa3956f9a82c630c58c6e96 |
# Dataset Card for [Dataset Name]
## Table of Contents
- [Table of Contents](#table-of-contents)
- [Dataset Description](#dataset-description)
- [Dataset Summary](#dataset-summary)
- [Supported Tasks and Leaderboards](#supported-tasks-and-leaderboards)
- [Languages](#languages)
- [Dataset Structure](#dataset-structure)
- [Data Instances](#data-instances)
- [Data Fields](#data-fields)
- [Data Splits](#data-splits)
- [Dataset Creation](#dataset-creation)
- [Curation Rationale](#curation-rationale)
- [Source Data](#source-data)
- [Annotations](#annotations)
- [Personal and Sensitive Information](#personal-and-sensitive-information)
- [Considerations for Using the Data](#considerations-for-using-the-data)
- [Social Impact of Dataset](#social-impact-of-dataset)
- [Discussion of Biases](#discussion-of-biases)
- [Other Known Limitations](#other-known-limitations)
- [Additional Information](#additional-information)
- [Dataset Curators](#dataset-curators)
- [Licensing Information](#licensing-information)
- [Citation Information](#citation-information)
- [Contributions](#contributions)
## Dataset Description
- **Homepage:**
- **Repository:**
- **Paper:**
- **Leaderboard:**
- **Point of Contact:**
### Dataset Summary
[More Information Needed]
### Supported Tasks and Leaderboards
[More Information Needed]
### Languages
[More Information Needed]
## Dataset Structure
### Data Instances
[More Information Needed]
### Data Fields
[More Information Needed]
### Data Splits
[More Information Needed]
## Dataset Creation
### Curation Rationale
[More Information Needed]
### Source Data
#### Initial Data Collection and Normalization
[More Information Needed]
#### Who are the source language producers?
[More Information Needed]
### Annotations
#### Annotation process
[More Information Needed]
#### Who are the annotators?
[More Information Needed]
### Personal and Sensitive Information
[More Information Needed]
## Considerations for Using the Data
### Social Impact of Dataset
[More Information Needed]
### Discussion of Biases
[More Information Needed]
### Other Known Limitations
[More Information Needed]
## Additional Information
### Dataset Curators
[More Information Needed]
### Licensing Information
[More Information Needed]
### Citation Information
[More Information Needed]
### Contributions
Thanks to [@github-username](https://github.com/<github-username>) for adding this dataset.
| DTU54DL/common-voice | [
"task_categories:token-classification",
"annotations_creators:expert-generated",
"language_creators:found",
"multilinguality:monolingual",
"size_categories:10K<n<100K",
"source_datasets:original",
"language:en",
"license:mit",
"region:us"
] | 2022-11-21T17:32:49+00:00 | {"annotations_creators": ["expert-generated"], "language_creators": ["found"], "language": ["en"], "license": ["mit"], "multilinguality": ["monolingual"], "size_categories": ["10K<n<100K"], "source_datasets": ["original"], "task_categories": ["token-classification"], "task_ids": ["token-classification-other-acronym-identification"], "paperswithcode_id": "acronym-identification", "pretty_name": "Acronym Identification Dataset", "train-eval-index": [{"col_mapping": {"labels": "tags", "tokens": "tokens"}, "config": "default", "splits": {"eval_split": "test"}, "task": "token-classification", "task_id": "entity_extraction"}]} | 2022-11-21T22:28:56+00:00 | [] | [
"en"
] | TAGS
#task_categories-token-classification #annotations_creators-expert-generated #language_creators-found #multilinguality-monolingual #size_categories-10K<n<100K #source_datasets-original #language-English #license-mit #region-us
|
# Dataset Card for [Dataset Name]
## Table of Contents
- Table of Contents
- Dataset Description
- Dataset Summary
- Supported Tasks and Leaderboards
- Languages
- Dataset Structure
- Data Instances
- Data Fields
- Data Splits
- Dataset Creation
- Curation Rationale
- Source Data
- Annotations
- Personal and Sensitive Information
- Considerations for Using the Data
- Social Impact of Dataset
- Discussion of Biases
- Other Known Limitations
- Additional Information
- Dataset Curators
- Licensing Information
- Citation Information
- Contributions
## Dataset Description
- Homepage:
- Repository:
- Paper:
- Leaderboard:
- Point of Contact:
### Dataset Summary
### Supported Tasks and Leaderboards
### Languages
## Dataset Structure
### Data Instances
### Data Fields
### Data Splits
## Dataset Creation
### Curation Rationale
### Source Data
#### Initial Data Collection and Normalization
#### Who are the source language producers?
### Annotations
#### Annotation process
#### Who are the annotators?
### Personal and Sensitive Information
## Considerations for Using the Data
### Social Impact of Dataset
### Discussion of Biases
### Other Known Limitations
## Additional Information
### Dataset Curators
### Licensing Information
### Contributions
Thanks to @github-username for adding this dataset.
| [
"# Dataset Card for [Dataset Name]",
"## Table of Contents\n- Table of Contents\n- Dataset Description\n - Dataset Summary\n - Supported Tasks and Leaderboards\n - Languages\n- Dataset Structure\n - Data Instances\n - Data Fields\n - Data Splits\n- Dataset Creation\n - Curation Rationale\n - Source Data\n - Annotations\n - Personal and Sensitive Information\n- Considerations for Using the Data\n - Social Impact of Dataset\n - Discussion of Biases\n - Other Known Limitations\n- Additional Information\n - Dataset Curators\n - Licensing Information\n - Citation Information\n - Contributions",
"## Dataset Description\n\n- Homepage:\n- Repository:\n- Paper:\n- Leaderboard:\n- Point of Contact:",
"### Dataset Summary",
"### Supported Tasks and Leaderboards",
"### Languages",
"## Dataset Structure",
"### Data Instances",
"### Data Fields",
"### Data Splits",
"## Dataset Creation",
"### Curation Rationale",
"### Source Data",
"#### Initial Data Collection and Normalization",
"#### Who are the source language producers?",
"### Annotations",
"#### Annotation process",
"#### Who are the annotators?",
"### Personal and Sensitive Information",
"## Considerations for Using the Data",
"### Social Impact of Dataset",
"### Discussion of Biases",
"### Other Known Limitations",
"## Additional Information",
"### Dataset Curators",
"### Licensing Information",
"### Contributions\n\nThanks to @github-username for adding this dataset."
] | [
"TAGS\n#task_categories-token-classification #annotations_creators-expert-generated #language_creators-found #multilinguality-monolingual #size_categories-10K<n<100K #source_datasets-original #language-English #license-mit #region-us \n",
"# Dataset Card for [Dataset Name]",
"## Table of Contents\n- Table of Contents\n- Dataset Description\n - Dataset Summary\n - Supported Tasks and Leaderboards\n - Languages\n- Dataset Structure\n - Data Instances\n - Data Fields\n - Data Splits\n- Dataset Creation\n - Curation Rationale\n - Source Data\n - Annotations\n - Personal and Sensitive Information\n- Considerations for Using the Data\n - Social Impact of Dataset\n - Discussion of Biases\n - Other Known Limitations\n- Additional Information\n - Dataset Curators\n - Licensing Information\n - Citation Information\n - Contributions",
"## Dataset Description\n\n- Homepage:\n- Repository:\n- Paper:\n- Leaderboard:\n- Point of Contact:",
"### Dataset Summary",
"### Supported Tasks and Leaderboards",
"### Languages",
"## Dataset Structure",
"### Data Instances",
"### Data Fields",
"### Data Splits",
"## Dataset Creation",
"### Curation Rationale",
"### Source Data",
"#### Initial Data Collection and Normalization",
"#### Who are the source language producers?",
"### Annotations",
"#### Annotation process",
"#### Who are the annotators?",
"### Personal and Sensitive Information",
"## Considerations for Using the Data",
"### Social Impact of Dataset",
"### Discussion of Biases",
"### Other Known Limitations",
"## Additional Information",
"### Dataset Curators",
"### Licensing Information",
"### Contributions\n\nThanks to @github-username for adding this dataset."
] | [
76,
10,
125,
24,
6,
10,
4,
6,
6,
5,
5,
5,
7,
4,
10,
10,
5,
5,
9,
8,
8,
7,
8,
7,
5,
6,
6,
19
] | [
"passage: TAGS\n#task_categories-token-classification #annotations_creators-expert-generated #language_creators-found #multilinguality-monolingual #size_categories-10K<n<100K #source_datasets-original #language-English #license-mit #region-us \n# Dataset Card for [Dataset Name]## Table of Contents\n- Table of Contents\n- Dataset Description\n - Dataset Summary\n - Supported Tasks and Leaderboards\n - Languages\n- Dataset Structure\n - Data Instances\n - Data Fields\n - Data Splits\n- Dataset Creation\n - Curation Rationale\n - Source Data\n - Annotations\n - Personal and Sensitive Information\n- Considerations for Using the Data\n - Social Impact of Dataset\n - Discussion of Biases\n - Other Known Limitations\n- Additional Information\n - Dataset Curators\n - Licensing Information\n - Citation Information\n - Contributions## Dataset Description\n\n- Homepage:\n- Repository:\n- Paper:\n- Leaderboard:\n- Point of Contact:### Dataset Summary### Supported Tasks and Leaderboards### Languages## Dataset Structure### Data Instances### Data Fields### Data Splits## Dataset Creation### Curation Rationale### Source Data#### Initial Data Collection and Normalization#### Who are the source language producers?### Annotations#### Annotation process#### Who are the annotators?### Personal and Sensitive Information## Considerations for Using the Data### Social Impact of Dataset### Discussion of Biases### Other Known Limitations## Additional Information### Dataset Curators### Licensing Information### Contributions\n\nThanks to @github-username for adding this dataset."
] |
c36b48c2cbb4ff5da614340e614fdc73086d963e | # Dataset Card for Counterfactually Augmented SNLI
## Table of Contents
- [Dataset Description](#dataset-description)
- [Dataset Summary](#dataset-summary)
- [Supported Tasks and Leaderboards](#supported-tasks-and-leaderboards)
- [Languages](#languages)
- [Dataset Structure](#dataset-structure)
- [Data Instances](#data-instances)
- [Data Fields](#data-fields)
- [Data Splits](#data-splits)
## Dataset Description
- **Repository:** [Learning the Difference that Makes a Difference with Counterfactually-Augmented Data](https://github.com/acmi-lab/counterfactually-augmented-data)
- **Paper:** [Learning the Difference that Makes a Difference with Counterfactually-Augmented Data](https://openreview.net/forum?id=Sklgs0NFvr)
- **Point of Contact:** [Sagnik Ray Choudhury](mailto:[email protected])
### Dataset Summary
The SNLI corpus (version 1.0) is a collection of 570k human-written English sentence pairs manually labeled for balanced classification with the labels entailment, contradiction, and neutral, supporting the task of natural language inference (NLI), also known as recognizing textual entailment (RTE). In the ICLR 2020 paper [Learning the Difference that Makes a Difference with Counterfactually-Augmented Data](https://openreview.net/forum?id=Sklgs0NFvr), Kaushik et. al. provided a dataset with counterfactual perturbations on the SNLI and IMDB data. This repository contains the original and counterfactual perturbations for the SNLI data, which was generated after processing the original data from [here](https://github.com/acmi-lab/counterfactually-augmented-data).
### Languages
The language in the dataset is English as spoken by users of the website Flickr and as spoken by crowdworkers from Amazon Mechanical Turk. The BCP-47 code for English is en.
## Dataset Structure
### Data Instances
For each instance, there is:
- a string for the premise,
- a string for the hypothesis,
- a label: (entailment, contradiction, neutral)
- a type: this tells whether the data point is the original SNLI data point or a counterfactual perturbation.
- an idx. The ids correspond to the original id in the SNLI data. For example, if the original SNLI instance was `4626192243.jpg#3r1e`, there wil be 5 data points as follows:
```json lines
{
"idx": "4626192243.jpg#3r1e-orig",
"premise": "A man with a beard is talking on the cellphone and standing next to someone who is lying down on the street.",
"hypothesis": "A man is prone on the street while another man stands next to him.",
"label": "entailment",
"type": "original"
}
{
"idx": "4626192243.jpg#3r1e-cf-0",
"premise": "A man with a beard is talking on the cellphone and standing next to someone who is lying down on the street.",
"hypothesis": "A man is talking to his wife on the cellphone.",
"label": "neutral",
"type": "cf"
}
{
"idx": "4626192243.jpg#3r1e-cf-1",
"premise": "A man with a beard is talking on the cellphone and standing next to someone who is on the street.",
"hypothesis": "A man is prone on the street while another man stands next to him.",
"label": "neutral",
"type": "cf"
}
{
"idx": "4626192243.jpg#3r1e-cf-2",
"premise": "A man with a beard is talking on the cellphone and standing next to someone who is sitting on the street.",
"hypothesis": "A man is prone on the street while another man stands next to him.",
"label": "contradiction",
"_type": "cf"
}
{
"idx": "4626192243.jpg#3r1e-cf-3",
"premise": "A man with a beard is talking on the cellphone and standing next to someone who is lying down on the street.",
"hypothesis": "A man is alone on the street.",
"label": "contradiction",
"type": "cf"
}
```
### Data Splits
Following SNLI, this dataset also has 3 splits: _train_, _validation_, and _test_. The original paper says this:
```aidl
RP and RH, each comprised of 3332 pairs in train, 400 in validation, and 800 in test, leading to a total of 6664 pairs in train, 800 in validation, and 1600 in test in the revised dataset.
```
This means for _train_, there are 1666 original SNLI instances, and each has 4 counterfactual perturbations (from premise and hypothesis edit), leading to a total of 1666*5 = 8330 _train_ data points in this dataset. Similarly, _validation_ and _test_ has 200 and 400 original SNLI instances respectively, consequently 1000 and 2000 instances in total.
| Dataset Split | Number of Instances in Split |
|---------------|------------------------------|
| Train | 8,330 |
| Validation | 1,000 |
| Test | 2,000 |
| sagnikrayc/snli-cf-kaushik | [
"task_categories:text-classification",
"task_ids:natural-language-inference",
"task_ids:multi-input-text-classification",
"annotations_creators:crowdsourced",
"language_creators:crowdsourced",
"multilinguality:monolingual",
"size_categories:1K<n<10K",
"source_datasets:extended|snli",
"language:en",
"license:cc-by-4.0",
"region:us"
] | 2022-11-21T18:28:12+00:00 | {"annotations_creators": ["crowdsourced"], "language_creators": ["crowdsourced"], "language": ["en"], "license": ["cc-by-4.0"], "multilinguality": ["monolingual"], "size_categories": ["1K<n<10K"], "source_datasets": ["extended|snli"], "task_categories": ["text-classification"], "task_ids": ["natural-language-inference", "multi-input-text-classification"], "pretty_name": "Counterfactual Instances for Stanford Natural Language Inference", "dataset_info": {"features": [{"name": "premise", "dtype": "string"}, {"name": "hypothesis", "dtype": "string"}, {"name": "label", "dtype": "string"}], "splits": [{"name": "train", "num_bytes": 1771712, "num_examples": 8300}, {"name": "validation", "num_bytes": 217479, "num_examples": 1000}, {"name": "test", "num_bytes": 437468, "num_examples": 2000}]}} | 2022-11-21T22:34:23+00:00 | [] | [
"en"
] | TAGS
#task_categories-text-classification #task_ids-natural-language-inference #task_ids-multi-input-text-classification #annotations_creators-crowdsourced #language_creators-crowdsourced #multilinguality-monolingual #size_categories-1K<n<10K #source_datasets-extended|snli #language-English #license-cc-by-4.0 #region-us
| Dataset Card for Counterfactually Augmented SNLI
================================================
Table of Contents
-----------------
* Dataset Description
+ Dataset Summary
+ Supported Tasks and Leaderboards
+ Languages
* Dataset Structure
+ Data Instances
+ Data Fields
+ Data Splits
Dataset Description
-------------------
* Repository: Learning the Difference that Makes a Difference with Counterfactually-Augmented Data
* Paper: Learning the Difference that Makes a Difference with Counterfactually-Augmented Data
* Point of Contact: Sagnik Ray Choudhury
### Dataset Summary
The SNLI corpus (version 1.0) is a collection of 570k human-written English sentence pairs manually labeled for balanced classification with the labels entailment, contradiction, and neutral, supporting the task of natural language inference (NLI), also known as recognizing textual entailment (RTE). In the ICLR 2020 paper Learning the Difference that Makes a Difference with Counterfactually-Augmented Data, Kaushik et. al. provided a dataset with counterfactual perturbations on the SNLI and IMDB data. This repository contains the original and counterfactual perturbations for the SNLI data, which was generated after processing the original data from here.
### Languages
The language in the dataset is English as spoken by users of the website Flickr and as spoken by crowdworkers from Amazon Mechanical Turk. The BCP-47 code for English is en.
Dataset Structure
-----------------
### Data Instances
For each instance, there is:
* a string for the premise,
* a string for the hypothesis,
* a label: (entailment, contradiction, neutral)
* a type: this tells whether the data point is the original SNLI data point or a counterfactual perturbation.
* an idx. The ids correspond to the original id in the SNLI data. For example, if the original SNLI instance was 'URL#3r1e', there wil be 5 data points as follows:
### Data Splits
Following SNLI, this dataset also has 3 splits: *train*, *validation*, and *test*. The original paper says this:
This means for *train*, there are 1666 original SNLI instances, and each has 4 counterfactual perturbations (from premise and hypothesis edit), leading to a total of 1666\*5 = 8330 *train* data points in this dataset. Similarly, *validation* and *test* has 200 and 400 original SNLI instances respectively, consequently 1000 and 2000 instances in total.
| [
"### Dataset Summary\n\n\nThe SNLI corpus (version 1.0) is a collection of 570k human-written English sentence pairs manually labeled for balanced classification with the labels entailment, contradiction, and neutral, supporting the task of natural language inference (NLI), also known as recognizing textual entailment (RTE). In the ICLR 2020 paper Learning the Difference that Makes a Difference with Counterfactually-Augmented Data, Kaushik et. al. provided a dataset with counterfactual perturbations on the SNLI and IMDB data. This repository contains the original and counterfactual perturbations for the SNLI data, which was generated after processing the original data from here.",
"### Languages\n\n\nThe language in the dataset is English as spoken by users of the website Flickr and as spoken by crowdworkers from Amazon Mechanical Turk. The BCP-47 code for English is en.\n\n\nDataset Structure\n-----------------",
"### Data Instances\n\n\nFor each instance, there is:\n\n\n* a string for the premise,\n* a string for the hypothesis,\n* a label: (entailment, contradiction, neutral)\n* a type: this tells whether the data point is the original SNLI data point or a counterfactual perturbation.\n* an idx. The ids correspond to the original id in the SNLI data. For example, if the original SNLI instance was 'URL#3r1e', there wil be 5 data points as follows:",
"### Data Splits\n\n\nFollowing SNLI, this dataset also has 3 splits: *train*, *validation*, and *test*. The original paper says this:\n\n\nThis means for *train*, there are 1666 original SNLI instances, and each has 4 counterfactual perturbations (from premise and hypothesis edit), leading to a total of 1666\\*5 = 8330 *train* data points in this dataset. Similarly, *validation* and *test* has 200 and 400 original SNLI instances respectively, consequently 1000 and 2000 instances in total."
] | [
"TAGS\n#task_categories-text-classification #task_ids-natural-language-inference #task_ids-multi-input-text-classification #annotations_creators-crowdsourced #language_creators-crowdsourced #multilinguality-monolingual #size_categories-1K<n<10K #source_datasets-extended|snli #language-English #license-cc-by-4.0 #region-us \n",
"### Dataset Summary\n\n\nThe SNLI corpus (version 1.0) is a collection of 570k human-written English sentence pairs manually labeled for balanced classification with the labels entailment, contradiction, and neutral, supporting the task of natural language inference (NLI), also known as recognizing textual entailment (RTE). In the ICLR 2020 paper Learning the Difference that Makes a Difference with Counterfactually-Augmented Data, Kaushik et. al. provided a dataset with counterfactual perturbations on the SNLI and IMDB data. This repository contains the original and counterfactual perturbations for the SNLI data, which was generated after processing the original data from here.",
"### Languages\n\n\nThe language in the dataset is English as spoken by users of the website Flickr and as spoken by crowdworkers from Amazon Mechanical Turk. The BCP-47 code for English is en.\n\n\nDataset Structure\n-----------------",
"### Data Instances\n\n\nFor each instance, there is:\n\n\n* a string for the premise,\n* a string for the hypothesis,\n* a label: (entailment, contradiction, neutral)\n* a type: this tells whether the data point is the original SNLI data point or a counterfactual perturbation.\n* an idx. The ids correspond to the original id in the SNLI data. For example, if the original SNLI instance was 'URL#3r1e', there wil be 5 data points as follows:",
"### Data Splits\n\n\nFollowing SNLI, this dataset also has 3 splits: *train*, *validation*, and *test*. The original paper says this:\n\n\nThis means for *train*, there are 1666 original SNLI instances, and each has 4 counterfactual perturbations (from premise and hypothesis edit), leading to a total of 1666\\*5 = 8330 *train* data points in this dataset. Similarly, *validation* and *test* has 200 and 400 original SNLI instances respectively, consequently 1000 and 2000 instances in total."
] | [
116,
168,
52,
118,
135
] | [
"passage: TAGS\n#task_categories-text-classification #task_ids-natural-language-inference #task_ids-multi-input-text-classification #annotations_creators-crowdsourced #language_creators-crowdsourced #multilinguality-monolingual #size_categories-1K<n<10K #source_datasets-extended|snli #language-English #license-cc-by-4.0 #region-us \n### Dataset Summary\n\n\nThe SNLI corpus (version 1.0) is a collection of 570k human-written English sentence pairs manually labeled for balanced classification with the labels entailment, contradiction, and neutral, supporting the task of natural language inference (NLI), also known as recognizing textual entailment (RTE). In the ICLR 2020 paper Learning the Difference that Makes a Difference with Counterfactually-Augmented Data, Kaushik et. al. provided a dataset with counterfactual perturbations on the SNLI and IMDB data. This repository contains the original and counterfactual perturbations for the SNLI data, which was generated after processing the original data from here.### Languages\n\n\nThe language in the dataset is English as spoken by users of the website Flickr and as spoken by crowdworkers from Amazon Mechanical Turk. The BCP-47 code for English is en.\n\n\nDataset Structure\n-----------------### Data Instances\n\n\nFor each instance, there is:\n\n\n* a string for the premise,\n* a string for the hypothesis,\n* a label: (entailment, contradiction, neutral)\n* a type: this tells whether the data point is the original SNLI data point or a counterfactual perturbation.\n* an idx. The ids correspond to the original id in the SNLI data. For example, if the original SNLI instance was 'URL#3r1e', there wil be 5 data points as follows:"
] |
c134fff8e3358fe542ea22384356d32634fb9b69 |
# Dataset Card for ciempiess_test
## Table of Contents
- [Dataset Description](#dataset-description)
- [Dataset Summary](#dataset-summary)
- [Supported Tasks](#supported-tasks-and-leaderboards)
- [Languages](#languages)
- [Dataset Structure](#dataset-structure)
- [Data Instances](#data-instances)
- [Data Fields](#data-fields)
- [Data Splits](#data-splits)
- [Dataset Creation](#dataset-creation)
- [Curation Rationale](#curation-rationale)
- [Source Data](#source-data)
- [Annotations](#annotations)
- [Personal and Sensitive Information](#personal-and-sensitive-information)
- [Considerations for Using the Data](#considerations-for-using-the-data)
- [Social Impact of Dataset](#social-impact-of-dataset)
- [Discussion of Biases](#discussion-of-biases)
- [Other Known Limitations](#other-known-limitations)
- [Additional Information](#additional-information)
- [Dataset Curators](#dataset-curators)
- [Licensing Information](#licensing-information)
- [Citation Information](#citation-information)
- [Contributions](#contributions)
## Dataset Description
- **Homepage:** [CIEMPIESS-UNAM Project](https://ciempiess.org/)
- **Repository:** [CIEMPIESS-TEST is part of LDC2019S07](https://catalog.ldc.upenn.edu/LDC2019S07)
- **Paper:** [Creating Mexican Spanish Language Resources through the Social Service Program](https://aclanthology.org/2022.nidcp-1.4.pdf)
- **Point of Contact:** [Carlos Mena](mailto:[email protected])
### Dataset Summary
When developing automatic speech recognition engines and any other machine learning system is a good practice to separate the test from the training data and never combined them. So, the CIEMPIESS TEST Corpus was created by this necessity of having an standard test set destined to measure the advances of the community of users of the CIEMPIESS datasets and we strongly recommend not to use the CIEMPIESS TEST for any other purpose.
The CIEMPIESS TEST Corpus is a gender balanced corpus designed to test acoustic models for the speech recognition task. It was created by recordings and human transcripts of 10 male and 10 female speakers.
The CIEMPIESS TEST Corpus is considered a CIEMPIESS dataset because it only contains audio from the same source of the first [CIEMPIESS Corpus](https://catalog.ldc.upenn.edu/LDC2015S07) and it has the word "TEST" in its name, obviously because it is recommended for test purposes only.
This corpus is part of the [CIEMPIESS Experimentation](https://catalog.ldc.upenn.edu/LDC2019S07), which is a set of three different datasets, specifically [CIEMPIESS COMPLEMENTARY](https://huggingface.co/datasets/ciempiess/ciempiess_complementary), [CIEMPIESS FEM](https://huggingface.co/datasets/ciempiess/ciempiess_fem) and [CIEMPIESS TEST](https://huggingface.co/datasets/ciempiess/ciempiess_test).
CIEMPIESS is the acronym for:
"Corpus de Investigación en Español de México del Posgrado de Ingeniería Eléctrica y Servicio Social".
### Example Usage
The CIEMPIESS TEST contains only the test split:
```python
from datasets import load_dataset
ciempiess_test = load_dataset("ciempiess/ciempiess_test")
```
It is also valid to do:
```python
from datasets import load_dataset
ciempiess_test = load_dataset("ciempiess/ciempiess_test",split="test")
```
### Supported Tasks
automatic-speech-recognition: The dataset can be used to test a model for Automatic Speech Recognition (ASR). The model is presented with an audio file and asked to transcribe the audio file to written text. The most common evaluation metric is the word error rate (WER).
### Languages
The language of the corpus is Spanish with the accent of Central Mexico except for the speaker M_09 that comes from El Salvador.
## Dataset Structure
### Data Instances
```python
{
'audio_id': 'CMPT_M_07_0074',
'audio': {
'path': '/home/carlos/.cache/HuggingFace/datasets/downloads/extracted/86a30fdc762ba3fad1e38fbe6900ea4940d6f0070af8d56aa483701faa050d51/test/male/M_07/CMPT_M_07_0074.flac',
'array': array([-0.00192261, -0.00234985, -0.00158691, ..., -0.00839233,
-0.00900269, -0.00698853], dtype=float32),
'sampling_rate': 16000
},
'speaker_id': 'M_07',
'gender': 'male',
'duration': 7.510000228881836,
'normalized_text': 'pues está la libertá de las posiciones de a ver quién es pasivo quién es activo blablablá muchas cosas no pero'
}
```
### Data Fields
* `audio_id` (string) - id of audio segment
* `audio` (datasets.Audio) - a dictionary containing the path to the audio, the decoded audio array, and the sampling rate. In non-streaming mode (default), the path points to the locally extracted audio. In streaming mode, the path is the relative path of an audio inside its archive (as files are not downloaded and extracted locally).
* `speaker_id` (string) - id of speaker
* `gender` (string) - gender of speaker (male or female)
* `duration` (float32) - duration of the audio file in seconds.
* `normalized_text` (string) - normalized audio segment transcription
### Data Splits
The corpus counts just with the test split which has a total of 3558 speech files from 10 male speakers and 10 female speakers with a total duration of 8 hours and 8 minutes.
## Dataset Creation
### Curation Rationale
The CIEMPIESS TEST (CT) Corpus has the following characteristics:
* The CT has a total of 3558 audio files of 10 male speakers and 10 female speakers. It has a total duration of 8 hours and 8 minutes.
* The total number of audio files that come from male speakers is 1694 with a total duration of 4 hours and 3 minutes. The total number of audio files that come from female speakers is 1864 with a total duration of 4 hours and 4 minutes. So CT is perfectly balanced in gender.
* All of the speakers in the CT come from Mexico, except for the speaker M_09 that comes from El Salvador.
* Every audio file in the CT has a duration between 5 and 10 seconds approximately.
* Data in CT is classified by gender and also by speaker, so one can easily select audios from a particular set of speakers to do experiments.
* Audio files in the CT and the first [CIEMPIESS](https://catalog.ldc.upenn.edu/LDC2015S07) are all of the same type. In both, speakers talk about legal and lawyer issues. They also talk about things related to the [UNAM University](https://www.unam.mx/) and the ["Facultad de Derecho de la UNAM"](https://www.derecho.unam.mx/).
* As in the first CIEMPIESS Corpus, transcriptions in the CT were made by humans.
* Speakers in the CT are not present in any other CIEMPIESS dataset.
* Audio files in the CT are distributed in a 16khz@16bit mono format.
### Source Data
#### Initial Data Collection and Normalization
The CIEMPIESS TEST is a Radio Corpus designed to test acoustic models of automatic speech recognition and it is made out of recordings of spontaneous conversations in Spanish between a radio moderator and his guests. Most of the speech in these conversations has the accent of Central Mexico.
All the recordings that constitute the CIEMPIESS TEST come from ["RADIO-IUS"](http://www.derecho.unam.mx/cultura-juridica/radio.php), a radio station belonging to UNAM. Recordings were donated by Lic. Cesar Gabriel Alanis Merchand and Mtro. Ricardo Rojas Arevalo from the "Facultad de Derecho de la UNAM" with the condition that they have to be used for academic and research purposes only.
### Annotations
#### Annotation process
The annotation process is at follows:
* 1. A whole podcast is manually segmented keeping just the portions containing good quality speech.
* 2. A second pass os segmentation is performed; this time to separate speakers and put them in different folders.
* 3. The resulting speech files between 5 and 10 seconds are transcribed by students from different departments (computing, engineering, linguistics). Most of them are native speakers but not with a particular training as transcribers.
#### Who are the annotators?
The CIEMPIESS TEST Corpus was created by the social service program ["Desarrollo de Tecnologías del Habla"](http://profesores.fi-b.unam.mx/carlos_mena/servicio.html) of the ["Facultad de Ingeniería"](https://www.ingenieria.unam.mx/) (FI) in the ["Universidad Nacional Autónoma de México"](https://www.unam.mx/) (UNAM) between 2016 and 2018 by Carlos Daniel Hernández Mena, head of the program.
### Personal and Sensitive Information
The dataset could contain names revealing the identity of some speakers; on the other side, the recordings come from publicly available podcasts, so, there is not a real intent of the participants to be anonymized. Anyway, you agree to not attempt to determine the identity of speakers in this dataset.
## Considerations for Using the Data
### Social Impact of Dataset
This dataset is challenging because it contains spontaneous speech; so, it will be helpful for the ASR community to evaluate their acoustic models in Spanish with it.
### Discussion of Biases
The dataset intents to be gender balanced. It is comprised of 10 male speakers and 10 female speakers. On the other hand the vocabulary is limited to legal issues.
### Other Known Limitations
The transcriptions in this dataset were revised by Mónica Alejandra Ruiz López during 2022 and they are slightly different from the transcriptions found at [LDC](https://catalog.ldc.upenn.edu/LDC2019S07) or at the [CIEMPIESS-UNAM Project](http://www.ciempiess.org/) official website. We strongly recommend to use these updated transcriptions; we will soon update the transcriptions in the rest of the repositories.
### Dataset Curators
The dataset was collected by students belonging to the social service program ["Desarrollo de Tecnologías del Habla"](http://profesores.fi-b.unam.mx/carlos_mena/servicio.html), it was curated by Carlos Daniel Hernández Mena and its transcriptions were manually verified by Mónica Alejandra Ruiz López during 2022.
### Licensing Information
[CC-BY-SA-4.0](https://creativecommons.org/licenses/by-sa/4.0/)
### Citation Information
```
@misc{carlosmenaciempiesstest2019,
title={CIEMPIESS TEST CORPUS: Audio and Transcripts of Mexican Spanish Broadcast Conversations.},
ldc_catalog_no={LDC2019S07},
DOI={https://doi.org/10.35111/xdx5-n815},
author={Hernandez Mena, Carlos Daniel},
journal={Linguistic Data Consortium, Philadelphia},
year={2019},
url={https://catalog.ldc.upenn.edu/LDC2019S07},
}
```
### Contributions
The authors want to thank to Alejandro V. Mena, Elena Vera and Angélica Gutiérrez for their support to the social service program: "Desarrollo de Tecnologías del Habla." We also thank to the social service students for all the hard work.
We also thank to Lic. Cesar Gabriel Alanis Merchand and Mtro. Ricardo Rojas Arevalo from the "Facultad de Derecho de la UNAM" for donating all the recordings that constitute the CIEMPIESS TEST Corpus.
Special thanks to Mónica Alejandra Ruiz López who performed a meticulous verification of the transcriptions of this dataset during 2022.
| ciempiess/ciempiess_test | [
"task_categories:automatic-speech-recognition",
"annotations_creators:expert-generated",
"language_creators:other",
"multilinguality:monolingual",
"size_categories:1K<n<10K",
"source_datasets:original",
"language:es",
"license:cc-by-sa-4.0",
"ciempiess",
"spanish",
"mexican spanish",
"test set",
"ciempiess project",
"ciempiess-unam project",
"ciempiess test",
"region:us"
] | 2022-11-21T18:29:31+00:00 | {"annotations_creators": ["expert-generated"], "language_creators": ["other"], "language": ["es"], "license": ["cc-by-sa-4.0"], "multilinguality": ["monolingual"], "size_categories": ["1K<n<10K"], "source_datasets": ["original"], "task_categories": ["automatic-speech-recognition"], "task_ids": [], "pretty_name": "CIEMPIESS TEST CORPUS: Audio and Transcripts of Mexican Spanish Broadcast Conversations.", "tags": ["ciempiess", "spanish", "mexican spanish", "test set", "ciempiess project", "ciempiess-unam project", "ciempiess test"]} | 2023-08-11T18:19:33+00:00 | [] | [
"es"
] | TAGS
#task_categories-automatic-speech-recognition #annotations_creators-expert-generated #language_creators-other #multilinguality-monolingual #size_categories-1K<n<10K #source_datasets-original #language-Spanish #license-cc-by-sa-4.0 #ciempiess #spanish #mexican spanish #test set #ciempiess project #ciempiess-unam project #ciempiess test #region-us
|
# Dataset Card for ciempiess_test
## Table of Contents
- Dataset Description
- Dataset Summary
- Supported Tasks
- Languages
- Dataset Structure
- Data Instances
- Data Fields
- Data Splits
- Dataset Creation
- Curation Rationale
- Source Data
- Annotations
- Personal and Sensitive Information
- Considerations for Using the Data
- Social Impact of Dataset
- Discussion of Biases
- Other Known Limitations
- Additional Information
- Dataset Curators
- Licensing Information
- Citation Information
- Contributions
## Dataset Description
- Homepage: CIEMPIESS-UNAM Project
- Repository: CIEMPIESS-TEST is part of LDC2019S07
- Paper: Creating Mexican Spanish Language Resources through the Social Service Program
- Point of Contact: Carlos Mena
### Dataset Summary
When developing automatic speech recognition engines and any other machine learning system is a good practice to separate the test from the training data and never combined them. So, the CIEMPIESS TEST Corpus was created by this necessity of having an standard test set destined to measure the advances of the community of users of the CIEMPIESS datasets and we strongly recommend not to use the CIEMPIESS TEST for any other purpose.
The CIEMPIESS TEST Corpus is a gender balanced corpus designed to test acoustic models for the speech recognition task. It was created by recordings and human transcripts of 10 male and 10 female speakers.
The CIEMPIESS TEST Corpus is considered a CIEMPIESS dataset because it only contains audio from the same source of the first CIEMPIESS Corpus and it has the word "TEST" in its name, obviously because it is recommended for test purposes only.
This corpus is part of the CIEMPIESS Experimentation, which is a set of three different datasets, specifically CIEMPIESS COMPLEMENTARY, CIEMPIESS FEM and CIEMPIESS TEST.
CIEMPIESS is the acronym for:
"Corpus de Investigación en Español de México del Posgrado de Ingeniería Eléctrica y Servicio Social".
### Example Usage
The CIEMPIESS TEST contains only the test split:
It is also valid to do:
### Supported Tasks
automatic-speech-recognition: The dataset can be used to test a model for Automatic Speech Recognition (ASR). The model is presented with an audio file and asked to transcribe the audio file to written text. The most common evaluation metric is the word error rate (WER).
### Languages
The language of the corpus is Spanish with the accent of Central Mexico except for the speaker M_09 that comes from El Salvador.
## Dataset Structure
### Data Instances
### Data Fields
* 'audio_id' (string) - id of audio segment
* 'audio' (datasets.Audio) - a dictionary containing the path to the audio, the decoded audio array, and the sampling rate. In non-streaming mode (default), the path points to the locally extracted audio. In streaming mode, the path is the relative path of an audio inside its archive (as files are not downloaded and extracted locally).
* 'speaker_id' (string) - id of speaker
* 'gender' (string) - gender of speaker (male or female)
* 'duration' (float32) - duration of the audio file in seconds.
* 'normalized_text' (string) - normalized audio segment transcription
### Data Splits
The corpus counts just with the test split which has a total of 3558 speech files from 10 male speakers and 10 female speakers with a total duration of 8 hours and 8 minutes.
## Dataset Creation
### Curation Rationale
The CIEMPIESS TEST (CT) Corpus has the following characteristics:
* The CT has a total of 3558 audio files of 10 male speakers and 10 female speakers. It has a total duration of 8 hours and 8 minutes.
* The total number of audio files that come from male speakers is 1694 with a total duration of 4 hours and 3 minutes. The total number of audio files that come from female speakers is 1864 with a total duration of 4 hours and 4 minutes. So CT is perfectly balanced in gender.
* All of the speakers in the CT come from Mexico, except for the speaker M_09 that comes from El Salvador.
* Every audio file in the CT has a duration between 5 and 10 seconds approximately.
* Data in CT is classified by gender and also by speaker, so one can easily select audios from a particular set of speakers to do experiments.
* Audio files in the CT and the first CIEMPIESS are all of the same type. In both, speakers talk about legal and lawyer issues. They also talk about things related to the UNAM University and the "Facultad de Derecho de la UNAM".
* As in the first CIEMPIESS Corpus, transcriptions in the CT were made by humans.
* Speakers in the CT are not present in any other CIEMPIESS dataset.
* Audio files in the CT are distributed in a 16khz@16bit mono format.
### Source Data
#### Initial Data Collection and Normalization
The CIEMPIESS TEST is a Radio Corpus designed to test acoustic models of automatic speech recognition and it is made out of recordings of spontaneous conversations in Spanish between a radio moderator and his guests. Most of the speech in these conversations has the accent of Central Mexico.
All the recordings that constitute the CIEMPIESS TEST come from "RADIO-IUS", a radio station belonging to UNAM. Recordings were donated by Lic. Cesar Gabriel Alanis Merchand and Mtro. Ricardo Rojas Arevalo from the "Facultad de Derecho de la UNAM" with the condition that they have to be used for academic and research purposes only.
### Annotations
#### Annotation process
The annotation process is at follows:
* 1. A whole podcast is manually segmented keeping just the portions containing good quality speech.
* 2. A second pass os segmentation is performed; this time to separate speakers and put them in different folders.
* 3. The resulting speech files between 5 and 10 seconds are transcribed by students from different departments (computing, engineering, linguistics). Most of them are native speakers but not with a particular training as transcribers.
#### Who are the annotators?
The CIEMPIESS TEST Corpus was created by the social service program "Desarrollo de Tecnologías del Habla" of the "Facultad de Ingeniería" (FI) in the "Universidad Nacional Autónoma de México" (UNAM) between 2016 and 2018 by Carlos Daniel Hernández Mena, head of the program.
### Personal and Sensitive Information
The dataset could contain names revealing the identity of some speakers; on the other side, the recordings come from publicly available podcasts, so, there is not a real intent of the participants to be anonymized. Anyway, you agree to not attempt to determine the identity of speakers in this dataset.
## Considerations for Using the Data
### Social Impact of Dataset
This dataset is challenging because it contains spontaneous speech; so, it will be helpful for the ASR community to evaluate their acoustic models in Spanish with it.
### Discussion of Biases
The dataset intents to be gender balanced. It is comprised of 10 male speakers and 10 female speakers. On the other hand the vocabulary is limited to legal issues.
### Other Known Limitations
The transcriptions in this dataset were revised by Mónica Alejandra Ruiz López during 2022 and they are slightly different from the transcriptions found at LDC or at the CIEMPIESS-UNAM Project official website. We strongly recommend to use these updated transcriptions; we will soon update the transcriptions in the rest of the repositories.
### Dataset Curators
The dataset was collected by students belonging to the social service program "Desarrollo de Tecnologías del Habla", it was curated by Carlos Daniel Hernández Mena and its transcriptions were manually verified by Mónica Alejandra Ruiz López during 2022.
### Licensing Information
CC-BY-SA-4.0
### Contributions
The authors want to thank to Alejandro V. Mena, Elena Vera and Angélica Gutiérrez for their support to the social service program: "Desarrollo de Tecnologías del Habla." We also thank to the social service students for all the hard work.
We also thank to Lic. Cesar Gabriel Alanis Merchand and Mtro. Ricardo Rojas Arevalo from the "Facultad de Derecho de la UNAM" for donating all the recordings that constitute the CIEMPIESS TEST Corpus.
Special thanks to Mónica Alejandra Ruiz López who performed a meticulous verification of the transcriptions of this dataset during 2022.
| [
"# Dataset Card for ciempiess_test",
"## Table of Contents\n- Dataset Description\n - Dataset Summary\n - Supported Tasks\n - Languages\n- Dataset Structure\n - Data Instances\n - Data Fields\n - Data Splits\n- Dataset Creation\n - Curation Rationale\n - Source Data\n - Annotations\n - Personal and Sensitive Information\n- Considerations for Using the Data\n - Social Impact of Dataset\n - Discussion of Biases\n - Other Known Limitations\n- Additional Information\n - Dataset Curators\n - Licensing Information\n - Citation Information\n - Contributions",
"## Dataset Description\n- Homepage: CIEMPIESS-UNAM Project\n- Repository: CIEMPIESS-TEST is part of LDC2019S07\n- Paper: Creating Mexican Spanish Language Resources through the Social Service Program\n- Point of Contact: Carlos Mena",
"### Dataset Summary\n\nWhen developing automatic speech recognition engines and any other machine learning system is a good practice to separate the test from the training data and never combined them. So, the CIEMPIESS TEST Corpus was created by this necessity of having an standard test set destined to measure the advances of the community of users of the CIEMPIESS datasets and we strongly recommend not to use the CIEMPIESS TEST for any other purpose.\n\nThe CIEMPIESS TEST Corpus is a gender balanced corpus designed to test acoustic models for the speech recognition task. It was created by recordings and human transcripts of 10 male and 10 female speakers.\n\nThe CIEMPIESS TEST Corpus is considered a CIEMPIESS dataset because it only contains audio from the same source of the first CIEMPIESS Corpus and it has the word \"TEST\" in its name, obviously because it is recommended for test purposes only. \n\nThis corpus is part of the CIEMPIESS Experimentation, which is a set of three different datasets, specifically CIEMPIESS COMPLEMENTARY, CIEMPIESS FEM and CIEMPIESS TEST.\n\nCIEMPIESS is the acronym for:\n\n\"Corpus de Investigación en Español de México del Posgrado de Ingeniería Eléctrica y Servicio Social\".",
"### Example Usage\nThe CIEMPIESS TEST contains only the test split:\n\nIt is also valid to do:",
"### Supported Tasks\nautomatic-speech-recognition: The dataset can be used to test a model for Automatic Speech Recognition (ASR). The model is presented with an audio file and asked to transcribe the audio file to written text. The most common evaluation metric is the word error rate (WER).",
"### Languages\nThe language of the corpus is Spanish with the accent of Central Mexico except for the speaker M_09 that comes from El Salvador.",
"## Dataset Structure",
"### Data Instances",
"### Data Fields\n* 'audio_id' (string) - id of audio segment\n* 'audio' (datasets.Audio) - a dictionary containing the path to the audio, the decoded audio array, and the sampling rate. In non-streaming mode (default), the path points to the locally extracted audio. In streaming mode, the path is the relative path of an audio inside its archive (as files are not downloaded and extracted locally).\n* 'speaker_id' (string) - id of speaker\n* 'gender' (string) - gender of speaker (male or female)\n* 'duration' (float32) - duration of the audio file in seconds.\n* 'normalized_text' (string) - normalized audio segment transcription",
"### Data Splits\n\nThe corpus counts just with the test split which has a total of 3558 speech files from 10 male speakers and 10 female speakers with a total duration of 8 hours and 8 minutes.",
"## Dataset Creation",
"### Curation Rationale\n\nThe CIEMPIESS TEST (CT) Corpus has the following characteristics:\n\n* The CT has a total of 3558 audio files of 10 male speakers and 10 female speakers. It has a total duration of 8 hours and 8 minutes.\n\n* The total number of audio files that come from male speakers is 1694 with a total duration of 4 hours and 3 minutes. The total number of audio files that come from female speakers is 1864 with a total duration of 4 hours and 4 minutes. So CT is perfectly balanced in gender.\n\n* All of the speakers in the CT come from Mexico, except for the speaker M_09 that comes from El Salvador.\n\n* Every audio file in the CT has a duration between 5 and 10 seconds approximately.\n\n* Data in CT is classified by gender and also by speaker, so one can easily select audios from a particular set of speakers to do experiments.\n\n* Audio files in the CT and the first CIEMPIESS are all of the same type. In both, speakers talk about legal and lawyer issues. They also talk about things related to the UNAM University and the \"Facultad de Derecho de la UNAM\".\n\n* As in the first CIEMPIESS Corpus, transcriptions in the CT were made by humans.\n\n* Speakers in the CT are not present in any other CIEMPIESS dataset.\n\n* Audio files in the CT are distributed in a 16khz@16bit mono format.",
"### Source Data",
"#### Initial Data Collection and Normalization\n\nThe CIEMPIESS TEST is a Radio Corpus designed to test acoustic models of automatic speech recognition and it is made out of recordings of spontaneous conversations in Spanish between a radio moderator and his guests. Most of the speech in these conversations has the accent of Central Mexico.\n\nAll the recordings that constitute the CIEMPIESS TEST come from \"RADIO-IUS\", a radio station belonging to UNAM. Recordings were donated by Lic. Cesar Gabriel Alanis Merchand and Mtro. Ricardo Rojas Arevalo from the \"Facultad de Derecho de la UNAM\" with the condition that they have to be used for academic and research purposes only.",
"### Annotations",
"#### Annotation process\n\nThe annotation process is at follows:\n\n* 1. A whole podcast is manually segmented keeping just the portions containing good quality speech.\n* 2. A second pass os segmentation is performed; this time to separate speakers and put them in different folders.\n* 3. The resulting speech files between 5 and 10 seconds are transcribed by students from different departments (computing, engineering, linguistics). Most of them are native speakers but not with a particular training as transcribers.",
"#### Who are the annotators?\n\nThe CIEMPIESS TEST Corpus was created by the social service program \"Desarrollo de Tecnologías del Habla\" of the \"Facultad de Ingeniería\" (FI) in the \"Universidad Nacional Autónoma de México\" (UNAM) between 2016 and 2018 by Carlos Daniel Hernández Mena, head of the program.",
"### Personal and Sensitive Information\n\nThe dataset could contain names revealing the identity of some speakers; on the other side, the recordings come from publicly available podcasts, so, there is not a real intent of the participants to be anonymized. Anyway, you agree to not attempt to determine the identity of speakers in this dataset.",
"## Considerations for Using the Data",
"### Social Impact of Dataset\n\nThis dataset is challenging because it contains spontaneous speech; so, it will be helpful for the ASR community to evaluate their acoustic models in Spanish with it.",
"### Discussion of Biases\n\nThe dataset intents to be gender balanced. It is comprised of 10 male speakers and 10 female speakers. On the other hand the vocabulary is limited to legal issues.",
"### Other Known Limitations\n\nThe transcriptions in this dataset were revised by Mónica Alejandra Ruiz López during 2022 and they are slightly different from the transcriptions found at LDC or at the CIEMPIESS-UNAM Project official website. We strongly recommend to use these updated transcriptions; we will soon update the transcriptions in the rest of the repositories.",
"### Dataset Curators\n\nThe dataset was collected by students belonging to the social service program \"Desarrollo de Tecnologías del Habla\", it was curated by Carlos Daniel Hernández Mena and its transcriptions were manually verified by Mónica Alejandra Ruiz López during 2022.",
"### Licensing Information\nCC-BY-SA-4.0",
"### Contributions\n\nThe authors want to thank to Alejandro V. Mena, Elena Vera and Angélica Gutiérrez for their support to the social service program: \"Desarrollo de Tecnologías del Habla.\" We also thank to the social service students for all the hard work.\n\nWe also thank to Lic. Cesar Gabriel Alanis Merchand and Mtro. Ricardo Rojas Arevalo from the \"Facultad de Derecho de la UNAM\" for donating all the recordings that constitute the CIEMPIESS TEST Corpus.\n\nSpecial thanks to Mónica Alejandra Ruiz López who performed a meticulous verification of the transcriptions of this dataset during 2022."
] | [
"TAGS\n#task_categories-automatic-speech-recognition #annotations_creators-expert-generated #language_creators-other #multilinguality-monolingual #size_categories-1K<n<10K #source_datasets-original #language-Spanish #license-cc-by-sa-4.0 #ciempiess #spanish #mexican spanish #test set #ciempiess project #ciempiess-unam project #ciempiess test #region-us \n",
"# Dataset Card for ciempiess_test",
"## Table of Contents\n- Dataset Description\n - Dataset Summary\n - Supported Tasks\n - Languages\n- Dataset Structure\n - Data Instances\n - Data Fields\n - Data Splits\n- Dataset Creation\n - Curation Rationale\n - Source Data\n - Annotations\n - Personal and Sensitive Information\n- Considerations for Using the Data\n - Social Impact of Dataset\n - Discussion of Biases\n - Other Known Limitations\n- Additional Information\n - Dataset Curators\n - Licensing Information\n - Citation Information\n - Contributions",
"## Dataset Description\n- Homepage: CIEMPIESS-UNAM Project\n- Repository: CIEMPIESS-TEST is part of LDC2019S07\n- Paper: Creating Mexican Spanish Language Resources through the Social Service Program\n- Point of Contact: Carlos Mena",
"### Dataset Summary\n\nWhen developing automatic speech recognition engines and any other machine learning system is a good practice to separate the test from the training data and never combined them. So, the CIEMPIESS TEST Corpus was created by this necessity of having an standard test set destined to measure the advances of the community of users of the CIEMPIESS datasets and we strongly recommend not to use the CIEMPIESS TEST for any other purpose.\n\nThe CIEMPIESS TEST Corpus is a gender balanced corpus designed to test acoustic models for the speech recognition task. It was created by recordings and human transcripts of 10 male and 10 female speakers.\n\nThe CIEMPIESS TEST Corpus is considered a CIEMPIESS dataset because it only contains audio from the same source of the first CIEMPIESS Corpus and it has the word \"TEST\" in its name, obviously because it is recommended for test purposes only. \n\nThis corpus is part of the CIEMPIESS Experimentation, which is a set of three different datasets, specifically CIEMPIESS COMPLEMENTARY, CIEMPIESS FEM and CIEMPIESS TEST.\n\nCIEMPIESS is the acronym for:\n\n\"Corpus de Investigación en Español de México del Posgrado de Ingeniería Eléctrica y Servicio Social\".",
"### Example Usage\nThe CIEMPIESS TEST contains only the test split:\n\nIt is also valid to do:",
"### Supported Tasks\nautomatic-speech-recognition: The dataset can be used to test a model for Automatic Speech Recognition (ASR). The model is presented with an audio file and asked to transcribe the audio file to written text. The most common evaluation metric is the word error rate (WER).",
"### Languages\nThe language of the corpus is Spanish with the accent of Central Mexico except for the speaker M_09 that comes from El Salvador.",
"## Dataset Structure",
"### Data Instances",
"### Data Fields\n* 'audio_id' (string) - id of audio segment\n* 'audio' (datasets.Audio) - a dictionary containing the path to the audio, the decoded audio array, and the sampling rate. In non-streaming mode (default), the path points to the locally extracted audio. In streaming mode, the path is the relative path of an audio inside its archive (as files are not downloaded and extracted locally).\n* 'speaker_id' (string) - id of speaker\n* 'gender' (string) - gender of speaker (male or female)\n* 'duration' (float32) - duration of the audio file in seconds.\n* 'normalized_text' (string) - normalized audio segment transcription",
"### Data Splits\n\nThe corpus counts just with the test split which has a total of 3558 speech files from 10 male speakers and 10 female speakers with a total duration of 8 hours and 8 minutes.",
"## Dataset Creation",
"### Curation Rationale\n\nThe CIEMPIESS TEST (CT) Corpus has the following characteristics:\n\n* The CT has a total of 3558 audio files of 10 male speakers and 10 female speakers. It has a total duration of 8 hours and 8 minutes.\n\n* The total number of audio files that come from male speakers is 1694 with a total duration of 4 hours and 3 minutes. The total number of audio files that come from female speakers is 1864 with a total duration of 4 hours and 4 minutes. So CT is perfectly balanced in gender.\n\n* All of the speakers in the CT come from Mexico, except for the speaker M_09 that comes from El Salvador.\n\n* Every audio file in the CT has a duration between 5 and 10 seconds approximately.\n\n* Data in CT is classified by gender and also by speaker, so one can easily select audios from a particular set of speakers to do experiments.\n\n* Audio files in the CT and the first CIEMPIESS are all of the same type. In both, speakers talk about legal and lawyer issues. They also talk about things related to the UNAM University and the \"Facultad de Derecho de la UNAM\".\n\n* As in the first CIEMPIESS Corpus, transcriptions in the CT were made by humans.\n\n* Speakers in the CT are not present in any other CIEMPIESS dataset.\n\n* Audio files in the CT are distributed in a 16khz@16bit mono format.",
"### Source Data",
"#### Initial Data Collection and Normalization\n\nThe CIEMPIESS TEST is a Radio Corpus designed to test acoustic models of automatic speech recognition and it is made out of recordings of spontaneous conversations in Spanish between a radio moderator and his guests. Most of the speech in these conversations has the accent of Central Mexico.\n\nAll the recordings that constitute the CIEMPIESS TEST come from \"RADIO-IUS\", a radio station belonging to UNAM. Recordings were donated by Lic. Cesar Gabriel Alanis Merchand and Mtro. Ricardo Rojas Arevalo from the \"Facultad de Derecho de la UNAM\" with the condition that they have to be used for academic and research purposes only.",
"### Annotations",
"#### Annotation process\n\nThe annotation process is at follows:\n\n* 1. A whole podcast is manually segmented keeping just the portions containing good quality speech.\n* 2. A second pass os segmentation is performed; this time to separate speakers and put them in different folders.\n* 3. The resulting speech files between 5 and 10 seconds are transcribed by students from different departments (computing, engineering, linguistics). Most of them are native speakers but not with a particular training as transcribers.",
"#### Who are the annotators?\n\nThe CIEMPIESS TEST Corpus was created by the social service program \"Desarrollo de Tecnologías del Habla\" of the \"Facultad de Ingeniería\" (FI) in the \"Universidad Nacional Autónoma de México\" (UNAM) between 2016 and 2018 by Carlos Daniel Hernández Mena, head of the program.",
"### Personal and Sensitive Information\n\nThe dataset could contain names revealing the identity of some speakers; on the other side, the recordings come from publicly available podcasts, so, there is not a real intent of the participants to be anonymized. Anyway, you agree to not attempt to determine the identity of speakers in this dataset.",
"## Considerations for Using the Data",
"### Social Impact of Dataset\n\nThis dataset is challenging because it contains spontaneous speech; so, it will be helpful for the ASR community to evaluate their acoustic models in Spanish with it.",
"### Discussion of Biases\n\nThe dataset intents to be gender balanced. It is comprised of 10 male speakers and 10 female speakers. On the other hand the vocabulary is limited to legal issues.",
"### Other Known Limitations\n\nThe transcriptions in this dataset were revised by Mónica Alejandra Ruiz López during 2022 and they are slightly different from the transcriptions found at LDC or at the CIEMPIESS-UNAM Project official website. We strongly recommend to use these updated transcriptions; we will soon update the transcriptions in the rest of the repositories.",
"### Dataset Curators\n\nThe dataset was collected by students belonging to the social service program \"Desarrollo de Tecnologías del Habla\", it was curated by Carlos Daniel Hernández Mena and its transcriptions were manually verified by Mónica Alejandra Ruiz López during 2022.",
"### Licensing Information\nCC-BY-SA-4.0",
"### Contributions\n\nThe authors want to thank to Alejandro V. Mena, Elena Vera and Angélica Gutiérrez for their support to the social service program: \"Desarrollo de Tecnologías del Habla.\" We also thank to the social service students for all the hard work.\n\nWe also thank to Lic. Cesar Gabriel Alanis Merchand and Mtro. Ricardo Rojas Arevalo from the \"Facultad de Derecho de la UNAM\" for donating all the recordings that constitute the CIEMPIESS TEST Corpus.\n\nSpecial thanks to Mónica Alejandra Ruiz López who performed a meticulous verification of the transcriptions of this dataset during 2022."
] | [
121,
11,
116,
58,
290,
27,
71,
30,
6,
6,
179,
45,
5,
317,
4,
155,
5,
112,
80,
74,
8,
44,
47,
85,
64,
13,
148
] | [
"passage: TAGS\n#task_categories-automatic-speech-recognition #annotations_creators-expert-generated #language_creators-other #multilinguality-monolingual #size_categories-1K<n<10K #source_datasets-original #language-Spanish #license-cc-by-sa-4.0 #ciempiess #spanish #mexican spanish #test set #ciempiess project #ciempiess-unam project #ciempiess test #region-us \n# Dataset Card for ciempiess_test## Table of Contents\n- Dataset Description\n - Dataset Summary\n - Supported Tasks\n - Languages\n- Dataset Structure\n - Data Instances\n - Data Fields\n - Data Splits\n- Dataset Creation\n - Curation Rationale\n - Source Data\n - Annotations\n - Personal and Sensitive Information\n- Considerations for Using the Data\n - Social Impact of Dataset\n - Discussion of Biases\n - Other Known Limitations\n- Additional Information\n - Dataset Curators\n - Licensing Information\n - Citation Information\n - Contributions## Dataset Description\n- Homepage: CIEMPIESS-UNAM Project\n- Repository: CIEMPIESS-TEST is part of LDC2019S07\n- Paper: Creating Mexican Spanish Language Resources through the Social Service Program\n- Point of Contact: Carlos Mena",
"passage: ### Dataset Summary\n\nWhen developing automatic speech recognition engines and any other machine learning system is a good practice to separate the test from the training data and never combined them. So, the CIEMPIESS TEST Corpus was created by this necessity of having an standard test set destined to measure the advances of the community of users of the CIEMPIESS datasets and we strongly recommend not to use the CIEMPIESS TEST for any other purpose.\n\nThe CIEMPIESS TEST Corpus is a gender balanced corpus designed to test acoustic models for the speech recognition task. It was created by recordings and human transcripts of 10 male and 10 female speakers.\n\nThe CIEMPIESS TEST Corpus is considered a CIEMPIESS dataset because it only contains audio from the same source of the first CIEMPIESS Corpus and it has the word \"TEST\" in its name, obviously because it is recommended for test purposes only. \n\nThis corpus is part of the CIEMPIESS Experimentation, which is a set of three different datasets, specifically CIEMPIESS COMPLEMENTARY, CIEMPIESS FEM and CIEMPIESS TEST.\n\nCIEMPIESS is the acronym for:\n\n\"Corpus de Investigación en Español de México del Posgrado de Ingeniería Eléctrica y Servicio Social\".### Example Usage\nThe CIEMPIESS TEST contains only the test split:\n\nIt is also valid to do:### Supported Tasks\nautomatic-speech-recognition: The dataset can be used to test a model for Automatic Speech Recognition (ASR). The model is presented with an audio file and asked to transcribe the audio file to written text. The most common evaluation metric is the word error rate (WER).### Languages\nThe language of the corpus is Spanish with the accent of Central Mexico except for the speaker M_09 that comes from El Salvador.## Dataset Structure### Data Instances### Data Fields\n* 'audio_id' (string) - id of audio segment\n* 'audio' (datasets.Audio) - a dictionary containing the path to the audio, the decoded audio array, and the sampling rate. In non-streaming mode (default), the path points to the locally extracted audio. In streaming mode, the path is the relative path of an audio inside its archive (as files are not downloaded and extracted locally).\n* 'speaker_id' (string) - id of speaker\n* 'gender' (string) - gender of speaker (male or female)\n* 'duration' (float32) - duration of the audio file in seconds.\n* 'normalized_text' (string) - normalized audio segment transcription### Data Splits\n\nThe corpus counts just with the test split which has a total of 3558 speech files from 10 male speakers and 10 female speakers with a total duration of 8 hours and 8 minutes.## Dataset Creation",
"passage: ### Curation Rationale\n\nThe CIEMPIESS TEST (CT) Corpus has the following characteristics:\n\n* The CT has a total of 3558 audio files of 10 male speakers and 10 female speakers. It has a total duration of 8 hours and 8 minutes.\n\n* The total number of audio files that come from male speakers is 1694 with a total duration of 4 hours and 3 minutes. The total number of audio files that come from female speakers is 1864 with a total duration of 4 hours and 4 minutes. So CT is perfectly balanced in gender.\n\n* All of the speakers in the CT come from Mexico, except for the speaker M_09 that comes from El Salvador.\n\n* Every audio file in the CT has a duration between 5 and 10 seconds approximately.\n\n* Data in CT is classified by gender and also by speaker, so one can easily select audios from a particular set of speakers to do experiments.\n\n* Audio files in the CT and the first CIEMPIESS are all of the same type. In both, speakers talk about legal and lawyer issues. They also talk about things related to the UNAM University and the \"Facultad de Derecho de la UNAM\".\n\n* As in the first CIEMPIESS Corpus, transcriptions in the CT were made by humans.\n\n* Speakers in the CT are not present in any other CIEMPIESS dataset.\n\n* Audio files in the CT are distributed in a 16khz@16bit mono format.### Source Data#### Initial Data Collection and Normalization\n\nThe CIEMPIESS TEST is a Radio Corpus designed to test acoustic models of automatic speech recognition and it is made out of recordings of spontaneous conversations in Spanish between a radio moderator and his guests. Most of the speech in these conversations has the accent of Central Mexico.\n\nAll the recordings that constitute the CIEMPIESS TEST come from \"RADIO-IUS\", a radio station belonging to UNAM. Recordings were donated by Lic. Cesar Gabriel Alanis Merchand and Mtro. Ricardo Rojas Arevalo from the \"Facultad de Derecho de la UNAM\" with the condition that they have to be used for academic and research purposes only.### Annotations#### Annotation process\n\nThe annotation process is at follows:\n\n* 1. A whole podcast is manually segmented keeping just the portions containing good quality speech.\n* 2. A second pass os segmentation is performed; this time to separate speakers and put them in different folders.\n* 3. The resulting speech files between 5 and 10 seconds are transcribed by students from different departments (computing, engineering, linguistics). Most of them are native speakers but not with a particular training as transcribers.#### Who are the annotators?\n\nThe CIEMPIESS TEST Corpus was created by the social service program \"Desarrollo de Tecnologías del Habla\" of the \"Facultad de Ingeniería\" (FI) in the \"Universidad Nacional Autónoma de México\" (UNAM) between 2016 and 2018 by Carlos Daniel Hernández Mena, head of the program.### Personal and Sensitive Information\n\nThe dataset could contain names revealing the identity of some speakers; on the other side, the recordings come from publicly available podcasts, so, there is not a real intent of the participants to be anonymized. Anyway, you agree to not attempt to determine the identity of speakers in this dataset.## Considerations for Using the Data### Social Impact of Dataset\n\nThis dataset is challenging because it contains spontaneous speech; so, it will be helpful for the ASR community to evaluate their acoustic models in Spanish with it."
] |
5eca058a8b613cf6232fb888fbe5198de8549ac4 |
# German Backtranslated Paraphrase Dataset
This is a dataset of more than 21 million German paraphrases.
These are text pairs that have the same meaning but are expressed with different words.
The source of the paraphrases are different parallel German / English text corpora.
The English texts were machine translated back into German to obtain the paraphrases.
This dataset can be used for example to train semantic text embeddings.
To do this, for example, [SentenceTransformers](https://www.sbert.net/)
and the [MultipleNegativesRankingLoss](https://www.sbert.net/docs/package_reference/losses.html#multiplenegativesrankingloss)
can be used.
## Creator
This data set was compiled and open sourced by [Philip May](https://may.la/)
of [Deutsche Telekom](https://www.telekom.de/).
## Our pre-processing
Apart from the back translation, we have added more columns (for details see below). We have carried out the following pre-processing and filtering:
- We dropped text pairs where one text was longer than 499 characters.
- In the [GlobalVoices v2018q4](https://opus.nlpl.eu/GlobalVoices-v2018q4.php) texts we have removed the `" · Global Voices"` suffix.
## Your post-processing
You probably don't want to use the dataset as it is, but filter it further.
This is what the additional columns of the dataset are for.
For us it has proven useful to delete the following pairs of sentences:
- `min_char_len` less than 15
- `jaccard_similarity` greater than 0.3
- `de_token_count` greater than 30
- `en_de_token_count` greater than 30
- `cos_sim` less than 0.85
## Columns description
- **`uuid`**: a uuid calculated with Python `uuid.uuid4()`
- **`en`**: the original English texts from the corpus
- **`de`**: the original German texts from the corpus
- **`en_de`**: the German texts translated back from English (from `en`)
- **`corpus`**: the name of the corpus
- **`min_char_len`**: the number of characters of the shortest text
- **`jaccard_similarity`**: the [Jaccard similarity coefficient](https://en.wikipedia.org/wiki/Jaccard_index) of both sentences - see below for more details
- **`de_token_count`**: number of tokens of the `de` text, tokenized with [deepset/gbert-large](https://huggingface.co/deepset/gbert-large)
- **`en_de_token_count`**: number of tokens of the `de` text, tokenized with [deepset/gbert-large](https://huggingface.co/deepset/gbert-large)
- **`cos_sim`**: the [cosine similarity](https://en.wikipedia.org/wiki/Cosine_similarity) of both sentences measured with [sentence-transformers/paraphrase-multilingual-mpnet-base-v2](https://huggingface.co/sentence-transformers/paraphrase-multilingual-mpnet-base-v2)
## Anomalies in the texts
It is noticeable that the [OpenSubtitles](https://opus.nlpl.eu/OpenSubtitles-v2018.php) texts have weird dash prefixes. This looks like this:
```
- Hast du was draufgetan?
```
To remove them you could apply this function:
```python
import re
def clean_text(text):
text = re.sub("^[-\s]*", "", text)
text = re.sub("[-\s]*$", "", text)
return text
df["de"] = df["de"].apply(clean_text)
df["en_de"] = df["en_de"].apply(clean_text)
```
## Parallel text corpora used
| Corpus name & link | Number of paraphrases |
|-----------------------------------------------------------------------|----------------------:|
| [OpenSubtitles](https://opus.nlpl.eu/OpenSubtitles-v2018.php) | 18,764,810 |
| [WikiMatrix v1](https://opus.nlpl.eu/WikiMatrix-v1.php) | 1,569,231 |
| [Tatoeba v2022-03-03](https://opus.nlpl.eu/Tatoeba-v2022-03-03.php) | 313,105 |
| [TED2020 v1](https://opus.nlpl.eu/TED2020-v1.php) | 289,374 |
| [News-Commentary v16](https://opus.nlpl.eu/News-Commentary-v16.php) | 285,722 |
| [GlobalVoices v2018q4](https://opus.nlpl.eu/GlobalVoices-v2018q4.php) | 70,547 |
| **sum** |. **21,292,789** |
## Back translation
We have made the back translation from English to German with the help of [Fairseq](https://github.com/facebookresearch/fairseq).
We used the `transformer.wmt19.en-de` model for this purpose:
```python
en2de = torch.hub.load(
"pytorch/fairseq",
"transformer.wmt19.en-de",
checkpoint_file="model1.pt:model2.pt:model3.pt:model4.pt",
tokenizer="moses",
bpe="fastbpe",
)
```
## How the Jaccard similarity was calculated
To calculate the [Jaccard similarity coefficient](https://en.wikipedia.org/wiki/Jaccard_index)
we are using the [SoMaJo tokenizer](https://github.com/tsproisl/SoMaJo)
to split the texts into tokens.
We then `lower()` the tokens so that upper and lower case letters no longer make a difference. Below you can find a code snippet with the details:
```python
from somajo import SoMaJo
LANGUAGE = "de_CMC"
somajo_tokenizer = SoMaJo(LANGUAGE)
def get_token_set(text, somajo_tokenizer):
sentences = somajo_tokenizer.tokenize_text([text])
tokens = [t.text.lower() for sentence in sentences for t in sentence]
token_set = set(tokens)
return token_set
def jaccard_similarity(text1, text2, somajo_tokenizer):
token_set1 = get_token_set(text1, somajo_tokenizer=somajo_tokenizer)
token_set2 = get_token_set(text2, somajo_tokenizer=somajo_tokenizer)
intersection = token_set1.intersection(token_set2)
union = token_set1.union(token_set2)
jaccard_similarity = float(len(intersection)) / len(union)
return jaccard_similarity
```
## Load this dataset
### With Hugging Face Datasets
```python
# pip install datasets
from datasets import load_dataset
dataset = load_dataset("deutsche-telekom/ger-backtrans-paraphrase")
train_dataset = dataset["train"]
```
### With Pandas
If you want to download the csv file and then load it with Pandas you can do it like this:
```python
df = pd.read_csv("train.csv")
```
## Citations, Acknowledgements and Licenses
**OpenSubtitles**
- citation: P. Lison and J. Tiedemann, 2016, [OpenSubtitles2016: Extracting Large Parallel Corpora from Movie and TV Subtitles](http://www.lrec-conf.org/proceedings/lrec2016/pdf/947_Paper.pdf). In Proceedings of the 10th International Conference on Language Resources and Evaluation (LREC 2016)
- also see http://www.opensubtitles.org/
- license: no special license has been provided at OPUS for this dataset
**WikiMatrix v1**
- citation: Holger Schwenk, Vishrav Chaudhary, Shuo Sun, Hongyu Gong and Paco Guzman, [WikiMatrix: Mining 135M Parallel Sentences in 1620 Language Pairs from Wikipedia](https://arxiv.org/abs/1907.05791), arXiv, July 11 2019
- license: [CC-BY-SA 4.0](https://creativecommons.org/licenses/by-sa/4.0/)
**Tatoeba v2022-03-03**
- citation: J. Tiedemann, 2012, [Parallel Data, Tools and Interfaces in OPUS](https://opus.nlpl.eu/Tatoeba-v2022-03-03.php). In Proceedings of the 8th International Conference on Language Resources and Evaluation (LREC 2012)
- license: [CC BY 2.0 FR](https://creativecommons.org/licenses/by/2.0/fr/)
- copyright: https://tatoeba.org/eng/terms_of_use
**TED2020 v1**
- citation: Reimers, Nils and Gurevych, Iryna, [Making Monolingual Sentence Embeddings Multilingual using Knowledge Distillation](https://arxiv.org/abs/2004.09813), In Proceedings of the 2020 Conference on Empirical Methods in Natural Language Processing, November 2020
- acknowledgements to [OPUS](https://opus.nlpl.eu/) for this service
- license: please respect the [TED Talks Usage Policy](https://www.ted.com/about/our-organization/our-policies-terms/ted-talks-usage-policy)
**News-Commentary v16**
- citation: J. Tiedemann, 2012, [Parallel Data, Tools and Interfaces in OPUS](https://opus.nlpl.eu/Tatoeba-v2022-03-03.php). In Proceedings of the 8th International Conference on Language Resources and Evaluation (LREC 2012)
- license: no special license has been provided at OPUS for this dataset
**GlobalVoices v2018q4**
- citation: J. Tiedemann, 2012, [Parallel Data, Tools and Interfaces in OPUS](https://opus.nlpl.eu/Tatoeba-v2022-03-03.php). In Proceedings of the 8th International Conference on Language Resources and Evaluation (LREC 2012)
- license: no special license has been provided at OPUS for this dataset
## Citation
```latex
@misc{ger-backtrans-paraphrase,
title={Deutsche-Telekom/ger-backtrans-paraphrase - dataset at Hugging Face},
url={https://huggingface.co/datasets/deutsche-telekom/ger-backtrans-paraphrase},
year={2022},
author={May, Philip}
}
```
## Licensing
Copyright (c) 2022 [Philip May](https://may.la/),
[Deutsche Telekom AG](https://www.telekom.com/)
This work is licensed under [CC-BY-SA 4.0](https://creativecommons.org/licenses/by-sa/4.0/).
| deutsche-telekom/ger-backtrans-paraphrase | [
"task_categories:sentence-similarity",
"multilinguality:monolingual",
"size_categories:10M<n<100M",
"language:de",
"license:cc-by-sa-4.0",
"arxiv:1907.05791",
"arxiv:2004.09813",
"region:us"
] | 2022-11-21T19:24:43+00:00 | {"language": ["de"], "license": ["cc-by-sa-4.0"], "multilinguality": ["monolingual"], "size_categories": ["10M<n<100M"], "task_categories": ["sentence-similarity"]} | 2023-12-17T17:42:02+00:00 | [
"1907.05791",
"2004.09813"
] | [
"de"
] | TAGS
#task_categories-sentence-similarity #multilinguality-monolingual #size_categories-10M<n<100M #language-German #license-cc-by-sa-4.0 #arxiv-1907.05791 #arxiv-2004.09813 #region-us
| German Backtranslated Paraphrase Dataset
========================================
This is a dataset of more than 21 million German paraphrases.
These are text pairs that have the same meaning but are expressed with different words.
The source of the paraphrases are different parallel German / English text corpora.
The English texts were machine translated back into German to obtain the paraphrases.
This dataset can be used for example to train semantic text embeddings.
To do this, for example, SentenceTransformers
and the MultipleNegativesRankingLoss
can be used.
Creator
-------
This data set was compiled and open sourced by Philip May
of Deutsche Telekom.
Our pre-processing
------------------
Apart from the back translation, we have added more columns (for details see below). We have carried out the following pre-processing and filtering:
* We dropped text pairs where one text was longer than 499 characters.
* In the GlobalVoices v2018q4 texts we have removed the '" · Global Voices"' suffix.
Your post-processing
--------------------
You probably don't want to use the dataset as it is, but filter it further.
This is what the additional columns of the dataset are for.
For us it has proven useful to delete the following pairs of sentences:
* 'min\_char\_len' less than 15
* 'jaccard\_similarity' greater than 0.3
* 'de\_token\_count' greater than 30
* 'en\_de\_token\_count' greater than 30
* 'cos\_sim' less than 0.85
Columns description
-------------------
* 'uuid': a uuid calculated with Python 'uuid.uuid4()'
* 'en': the original English texts from the corpus
* 'de': the original German texts from the corpus
* 'en\_de': the German texts translated back from English (from 'en')
* 'corpus': the name of the corpus
* 'min\_char\_len': the number of characters of the shortest text
* 'jaccard\_similarity': the Jaccard similarity coefficient of both sentences - see below for more details
* 'de\_token\_count': number of tokens of the 'de' text, tokenized with deepset/gbert-large
* 'en\_de\_token\_count': number of tokens of the 'de' text, tokenized with deepset/gbert-large
* 'cos\_sim': the cosine similarity of both sentences measured with sentence-transformers/paraphrase-multilingual-mpnet-base-v2
Anomalies in the texts
----------------------
It is noticeable that the OpenSubtitles texts have weird dash prefixes. This looks like this:
To remove them you could apply this function:
Parallel text corpora used
--------------------------
Back translation
----------------
We have made the back translation from English to German with the help of Fairseq.
We used the 'URL-de' model for this purpose:
How the Jaccard similarity was calculated
-----------------------------------------
To calculate the Jaccard similarity coefficient
we are using the SoMaJo tokenizer
to split the texts into tokens.
We then 'lower()' the tokens so that upper and lower case letters no longer make a difference. Below you can find a code snippet with the details:
Load this dataset
-----------------
### With Hugging Face Datasets
### With Pandas
If you want to download the csv file and then load it with Pandas you can do it like this:
s, Acknowledgements and Licenses
OpenSubtitles
* citation: P. Lison and J. Tiedemann, 2016, OpenSubtitles2016: Extracting Large Parallel Corpora from Movie and TV Subtitles. In Proceedings of the 10th International Conference on Language Resources and Evaluation (LREC 2016)
* also see URL
* license: no special license has been provided at OPUS for this dataset
WikiMatrix v1
* citation: Holger Schwenk, Vishrav Chaudhary, Shuo Sun, Hongyu Gong and Paco Guzman, WikiMatrix: Mining 135M Parallel Sentences in 1620 Language Pairs from Wikipedia, arXiv, July 11 2019
* license: CC-BY-SA 4.0
Tatoeba v2022-03-03
* citation: J. Tiedemann, 2012, Parallel Data, Tools and Interfaces in OPUS. In Proceedings of the 8th International Conference on Language Resources and Evaluation (LREC 2012)
* license: CC BY 2.0 FR
* copyright: URL
TED2020 v1
* citation: Reimers, Nils and Gurevych, Iryna, Making Monolingual Sentence Embeddings Multilingual using Knowledge Distillation, In Proceedings of the 2020 Conference on Empirical Methods in Natural Language Processing, November 2020
* acknowledgements to OPUS for this service
* license: please respect the TED Talks Usage Policy
News-Commentary v16
* citation: J. Tiedemann, 2012, Parallel Data, Tools and Interfaces in OPUS. In Proceedings of the 8th International Conference on Language Resources and Evaluation (LREC 2012)
* license: no special license has been provided at OPUS for this dataset
GlobalVoices v2018q4
* citation: J. Tiedemann, 2012, Parallel Data, Tools and Interfaces in OPUS. In Proceedings of the 8th International Conference on Language Resources and Evaluation (LREC 2012)
* license: no special license has been provided at OPUS for this dataset
Licensing
---------
Copyright (c) 2022 Philip May,
Deutsche Telekom AG
This work is licensed under CC-BY-SA 4.0.
| [
"### With Hugging Face Datasets",
"### With Pandas\n\n\nIf you want to download the csv file and then load it with Pandas you can do it like this:\n\n\ns, Acknowledgements and Licenses\n\n\nOpenSubtitles\n\n\n* citation: P. Lison and J. Tiedemann, 2016, OpenSubtitles2016: Extracting Large Parallel Corpora from Movie and TV Subtitles. In Proceedings of the 10th International Conference on Language Resources and Evaluation (LREC 2016)\n* also see URL\n* license: no special license has been provided at OPUS for this dataset\n\n\nWikiMatrix v1\n\n\n* citation: Holger Schwenk, Vishrav Chaudhary, Shuo Sun, Hongyu Gong and Paco Guzman, WikiMatrix: Mining 135M Parallel Sentences in 1620 Language Pairs from Wikipedia, arXiv, July 11 2019\n* license: CC-BY-SA 4.0\n\n\nTatoeba v2022-03-03\n\n\n* citation: J. Tiedemann, 2012, Parallel Data, Tools and Interfaces in OPUS. In Proceedings of the 8th International Conference on Language Resources and Evaluation (LREC 2012)\n* license: CC BY 2.0 FR\n* copyright: URL\n\n\nTED2020 v1\n\n\n* citation: Reimers, Nils and Gurevych, Iryna, Making Monolingual Sentence Embeddings Multilingual using Knowledge Distillation, In Proceedings of the 2020 Conference on Empirical Methods in Natural Language Processing, November 2020\n* acknowledgements to OPUS for this service\n* license: please respect the TED Talks Usage Policy\n\n\nNews-Commentary v16\n\n\n* citation: J. Tiedemann, 2012, Parallel Data, Tools and Interfaces in OPUS. In Proceedings of the 8th International Conference on Language Resources and Evaluation (LREC 2012)\n* license: no special license has been provided at OPUS for this dataset\n\n\nGlobalVoices v2018q4\n\n\n* citation: J. Tiedemann, 2012, Parallel Data, Tools and Interfaces in OPUS. In Proceedings of the 8th International Conference on Language Resources and Evaluation (LREC 2012)\n* license: no special license has been provided at OPUS for this dataset\n\n\nLicensing\n---------\n\n\nCopyright (c) 2022 Philip May,\nDeutsche Telekom AG\n\n\nThis work is licensed under CC-BY-SA 4.0."
] | [
"TAGS\n#task_categories-sentence-similarity #multilinguality-monolingual #size_categories-10M<n<100M #language-German #license-cc-by-sa-4.0 #arxiv-1907.05791 #arxiv-2004.09813 #region-us \n",
"### With Hugging Face Datasets",
"### With Pandas\n\n\nIf you want to download the csv file and then load it with Pandas you can do it like this:\n\n\ns, Acknowledgements and Licenses\n\n\nOpenSubtitles\n\n\n* citation: P. Lison and J. Tiedemann, 2016, OpenSubtitles2016: Extracting Large Parallel Corpora from Movie and TV Subtitles. In Proceedings of the 10th International Conference on Language Resources and Evaluation (LREC 2016)\n* also see URL\n* license: no special license has been provided at OPUS for this dataset\n\n\nWikiMatrix v1\n\n\n* citation: Holger Schwenk, Vishrav Chaudhary, Shuo Sun, Hongyu Gong and Paco Guzman, WikiMatrix: Mining 135M Parallel Sentences in 1620 Language Pairs from Wikipedia, arXiv, July 11 2019\n* license: CC-BY-SA 4.0\n\n\nTatoeba v2022-03-03\n\n\n* citation: J. Tiedemann, 2012, Parallel Data, Tools and Interfaces in OPUS. In Proceedings of the 8th International Conference on Language Resources and Evaluation (LREC 2012)\n* license: CC BY 2.0 FR\n* copyright: URL\n\n\nTED2020 v1\n\n\n* citation: Reimers, Nils and Gurevych, Iryna, Making Monolingual Sentence Embeddings Multilingual using Knowledge Distillation, In Proceedings of the 2020 Conference on Empirical Methods in Natural Language Processing, November 2020\n* acknowledgements to OPUS for this service\n* license: please respect the TED Talks Usage Policy\n\n\nNews-Commentary v16\n\n\n* citation: J. Tiedemann, 2012, Parallel Data, Tools and Interfaces in OPUS. In Proceedings of the 8th International Conference on Language Resources and Evaluation (LREC 2012)\n* license: no special license has been provided at OPUS for this dataset\n\n\nGlobalVoices v2018q4\n\n\n* citation: J. Tiedemann, 2012, Parallel Data, Tools and Interfaces in OPUS. In Proceedings of the 8th International Conference on Language Resources and Evaluation (LREC 2012)\n* license: no special license has been provided at OPUS for this dataset\n\n\nLicensing\n---------\n\n\nCopyright (c) 2022 Philip May,\nDeutsche Telekom AG\n\n\nThis work is licensed under CC-BY-SA 4.0."
] | [
71,
9,
497
] | [
"passage: TAGS\n#task_categories-sentence-similarity #multilinguality-monolingual #size_categories-10M<n<100M #language-German #license-cc-by-sa-4.0 #arxiv-1907.05791 #arxiv-2004.09813 #region-us \n### With Hugging Face Datasets"
] |
4c425aef1761ba70d12263586adc06e43c1de3fc | # Learning Stance Embeddings from Signed Social Graphs
[](http://makeapullrequest.com)
[](https://arxiv.org/abs/2201.11675)
This repo contains the datasets from our paper [Learning Stance Embeddings from Signed Social Graphs](https://arxiv.org/abs/2201.11675). <br />
[[PDF]](https://arxiv.org/pdf/2201.11675.pdf)
[[HuggingFace Datasets]](https://huggingface.co/Twitter)
<a rel="license" href="http://creativecommons.org/licenses/by/4.0/"><img alt="Creative Commons License" style="border-width:0" src="https://i.creativecommons.org/l/by/4.0/88x31.png" /></a><br />This work is licensed under a <a rel="license" href="http://creativecommons.org/licenses/by/4.0/">Creative Commons Attribution 4.0 International License</a>.
## Overview
A key challenge in social network analysis is understanding the position, or stance, of people in the graph on a large set of topics. In such social graphs, modeling (dis)agreement patterns across a range of correlated topics may be beneficial. For example, disagreement on one topic may make disagreement (or agreement) more likely for related topics.
We open source **two Twitter signed, topical graph datasets**. One dataset, **TwitterSG**, labels (dis)agreements using engagements between users via tweets to derive topic-informed, signed edges. The other, **BirdwatchSG**,leverages community reports on misinformation and misleading content.
## Datasets
### TwitterSG
Twitter Signed Graph, or TwitterSG, is a signed, directed, edge-attributed graph of users, drawn from Twitter interactions. TwitterSG contains 753,944 nodes (users), 200 topics and 12,848,093 edges. It is the largest publicly available user-to-user signed social graph (∼6x larger than the Epinions graph).
A positive edge exists from user 𝐴 to user 𝐵 if user 𝐴 liked a tweet posted by user 𝐵. A negative edge exists from user 𝐴 to user 𝐵 if user 𝐴 expressed opposition towards user 𝐵’s tweet, e.g., by replying *I disagree with you*. The full list of opposition keywords is specified [here](https://github.com/lejohnyjohn/learning-stance-embeddings-from-signed-social-graphs/tree/main/datasets). The topic of an edge from user 𝐴 to user 𝐵 is determined by the topic of user 𝐵’s tweet.
Tweets' topics were inferred with a topic classifier used in production by Twitter. The topics provided in the dataset are all related to sports (e.g., sports teams, players, managers, or events), and the tweets related to these interactions were published between 20th May (Ice Hockey World Championships) and 8th August 2021 (closing date of the 2020 Tokyo Olympic Games).
9.6\% of edges are negative (opposition) and 90.4\% are positive. There may be several edges between two nodes (several interactions, several topics). The data format is displayed below.
| source_idx | target_idx | topic_idx | topic | rating |
| ------------- | ------------- | ---------- | ------ | ---- |
| 1 | 6 | 19 | Copa America | +1 |
| 1 | 6 | 97 | NFL | -1 |
| 4 | 5 | 23 |Kylian Mbappe | +1 |
### BirdwatchSG
Birdwatch Signed Graph, or BirdwatchSG, is a signed, directed, edge-attributed graph of users, drawn from note ratings on the Birdwatch pilot. The graph contains 2,987 nodes (users), 1,020 topics and 441,986 edges.
[Birdwatch pilot](https://blog.twitter.com/en_us/topics/product/2021/introducing-birdwatch-a-community-based-approach-to-misinformation) was launched by Twitter in January 2021 in the USA to address misleading information on the platform, in a community-driven fashion: the Birdwatch participants can identify information they believe is misleading in tweets and write notes that provide informative context. They can also rate the helpfulness (either *helpful*, *somewhat helpful*, or *not helpful*) of notes added by other contributors. All Birdwatch contributions are publicly available on the [Birdwatch site](https://twitter.github.io/birdwatch/) for anyone in the USA.
Using Birdwatch data from January to July 2021, a positive (negative) edge is created from participant 𝑈1 to 𝑈2 if participant 𝑈1 rated a note written by participant 𝑈2 as *helpful* (*not helpful*). The *somewhat helpful* ratings were filtered out. The topic associated with an edge is the topic inferred from the tweet the note refers to.
36.9% of edges are negative (opposition) and 63.1% are positive. There may be several edges between two nodes (several interactions, several topics).
| source_idx | target_idx | topic_idx | topic | rating |
| ------------- | ------------- | ---------- | ------ | ---- |
| 10 | 6 | 443 | US Politics | +1 |
| 7 | 14 | 12 | Ted Cruz | -1 |
| 1 | 11 | 1003 | COVID-19 | +1 |
## Citation
If you use our datasets in your work, please cite the following:
```bib
@article{pougue2022learning,
title={Learning Stance Embeddings from Signed Social Graphs},
author={Pougu{\'e}-Biyong, John and Gupta, Akshay and Haghighi, Aria and El-Kishky, Ahmed},
journal={arXiv preprint arXiv:2201.11675},
year={2022}
}
``` | Twitter/SignedGraphs | [
"license:cc-by-4.0",
"arxiv:2201.11675",
"region:us"
] | 2022-11-21T20:08:09+00:00 | {"license": "cc-by-4.0"} | 2022-11-22T03:32:19+00:00 | [
"2201.11675"
] | [] | TAGS
#license-cc-by-4.0 #arxiv-2201.11675 #region-us
| Learning Stance Embeddings from Signed Social Graphs
====================================================

agreement patterns across a range of correlated topics may be beneficial. For example, disagreement on one topic may make disagreement (or agreement) more likely for related topics.
We open source two Twitter signed, topical graph datasets. One dataset, TwitterSG, labels (dis)agreements using engagements between users via tweets to derive topic-informed, signed edges. The other, BirdwatchSG,leverages community reports on misinformation and misleading content.
Datasets
--------
### TwitterSG
Twitter Signed Graph, or TwitterSG, is a signed, directed, edge-attributed graph of users, drawn from Twitter interactions. TwitterSG contains 753,944 nodes (users), 200 topics and 12,848,093 edges. It is the largest publicly available user-to-user signed social graph (∼6x larger than the Epinions graph).
A positive edge exists from user 𝐴 to user 𝐵 if user 𝐴 liked a tweet posted by user 𝐵. A negative edge exists from user 𝐴 to user 𝐵 if user 𝐴 expressed opposition towards user 𝐵’s tweet, e.g., by replying *I disagree with you*. The full list of opposition keywords is specified here. The topic of an edge from user 𝐴 to user 𝐵 is determined by the topic of user 𝐵’s tweet.
Tweets' topics were inferred with a topic classifier used in production by Twitter. The topics provided in the dataset are all related to sports (e.g., sports teams, players, managers, or events), and the tweets related to these interactions were published between 20th May (Ice Hockey World Championships) and 8th August 2021 (closing date of the 2020 Tokyo Olympic Games).
9.6% of edges are negative (opposition) and 90.4% are positive. There may be several edges between two nodes (several interactions, several topics). The data format is displayed below.
### BirdwatchSG
Birdwatch Signed Graph, or BirdwatchSG, is a signed, directed, edge-attributed graph of users, drawn from note ratings on the Birdwatch pilot. The graph contains 2,987 nodes (users), 1,020 topics and 441,986 edges.
Birdwatch pilot was launched by Twitter in January 2021 in the USA to address misleading information on the platform, in a community-driven fashion: the Birdwatch participants can identify information they believe is misleading in tweets and write notes that provide informative context. They can also rate the helpfulness (either *helpful*, *somewhat helpful*, or *not helpful*) of notes added by other contributors. All Birdwatch contributions are publicly available on the Birdwatch site for anyone in the USA.
Using Birdwatch data from January to July 2021, a positive (negative) edge is created from participant 𝑈1 to 𝑈2 if participant 𝑈1 rated a note written by participant 𝑈2 as *helpful* (*not helpful*). The *somewhat helpful* ratings were filtered out. The topic associated with an edge is the topic inferred from the tweet the note refers to.
36.9% of edges are negative (opposition) and 63.1% are positive. There may be several edges between two nodes (several interactions, several topics).
If you use our datasets in your work, please cite the following:
| [
"### TwitterSG\n\n\nTwitter Signed Graph, or TwitterSG, is a signed, directed, edge-attributed graph of users, drawn from Twitter interactions. TwitterSG contains 753,944 nodes (users), 200 topics and 12,848,093 edges. It is the largest publicly available user-to-user signed social graph (∼6x larger than the Epinions graph).\n\n\nA positive edge exists from user 𝐴 to user 𝐵 if user 𝐴 liked a tweet posted by user 𝐵. A negative edge exists from user 𝐴 to user 𝐵 if user 𝐴 expressed opposition towards user 𝐵’s tweet, e.g., by replying *I disagree with you*. The full list of opposition keywords is specified here. The topic of an edge from user 𝐴 to user 𝐵 is determined by the topic of user 𝐵’s tweet.\n\n\nTweets' topics were inferred with a topic classifier used in production by Twitter. The topics provided in the dataset are all related to sports (e.g., sports teams, players, managers, or events), and the tweets related to these interactions were published between 20th May (Ice Hockey World Championships) and 8th August 2021 (closing date of the 2020 Tokyo Olympic Games).\n\n\n9.6% of edges are negative (opposition) and 90.4% are positive. There may be several edges between two nodes (several interactions, several topics). The data format is displayed below.",
"### BirdwatchSG\n\n\nBirdwatch Signed Graph, or BirdwatchSG, is a signed, directed, edge-attributed graph of users, drawn from note ratings on the Birdwatch pilot. The graph contains 2,987 nodes (users), 1,020 topics and 441,986 edges.\n\n\nBirdwatch pilot was launched by Twitter in January 2021 in the USA to address misleading information on the platform, in a community-driven fashion: the Birdwatch participants can identify information they believe is misleading in tweets and write notes that provide informative context. They can also rate the helpfulness (either *helpful*, *somewhat helpful*, or *not helpful*) of notes added by other contributors. All Birdwatch contributions are publicly available on the Birdwatch site for anyone in the USA.\n\n\nUsing Birdwatch data from January to July 2021, a positive (negative) edge is created from participant 𝑈1 to 𝑈2 if participant 𝑈1 rated a note written by participant 𝑈2 as *helpful* (*not helpful*). The *somewhat helpful* ratings were filtered out. The topic associated with an edge is the topic inferred from the tweet the note refers to.\n\n\n36.9% of edges are negative (opposition) and 63.1% are positive. There may be several edges between two nodes (several interactions, several topics).\n\n\n\nIf you use our datasets in your work, please cite the following:"
] | [
"TAGS\n#license-cc-by-4.0 #arxiv-2201.11675 #region-us \n",
"### TwitterSG\n\n\nTwitter Signed Graph, or TwitterSG, is a signed, directed, edge-attributed graph of users, drawn from Twitter interactions. TwitterSG contains 753,944 nodes (users), 200 topics and 12,848,093 edges. It is the largest publicly available user-to-user signed social graph (∼6x larger than the Epinions graph).\n\n\nA positive edge exists from user 𝐴 to user 𝐵 if user 𝐴 liked a tweet posted by user 𝐵. A negative edge exists from user 𝐴 to user 𝐵 if user 𝐴 expressed opposition towards user 𝐵’s tweet, e.g., by replying *I disagree with you*. The full list of opposition keywords is specified here. The topic of an edge from user 𝐴 to user 𝐵 is determined by the topic of user 𝐵’s tweet.\n\n\nTweets' topics were inferred with a topic classifier used in production by Twitter. The topics provided in the dataset are all related to sports (e.g., sports teams, players, managers, or events), and the tweets related to these interactions were published between 20th May (Ice Hockey World Championships) and 8th August 2021 (closing date of the 2020 Tokyo Olympic Games).\n\n\n9.6% of edges are negative (opposition) and 90.4% are positive. There may be several edges between two nodes (several interactions, several topics). The data format is displayed below.",
"### BirdwatchSG\n\n\nBirdwatch Signed Graph, or BirdwatchSG, is a signed, directed, edge-attributed graph of users, drawn from note ratings on the Birdwatch pilot. The graph contains 2,987 nodes (users), 1,020 topics and 441,986 edges.\n\n\nBirdwatch pilot was launched by Twitter in January 2021 in the USA to address misleading information on the platform, in a community-driven fashion: the Birdwatch participants can identify information they believe is misleading in tweets and write notes that provide informative context. They can also rate the helpfulness (either *helpful*, *somewhat helpful*, or *not helpful*) of notes added by other contributors. All Birdwatch contributions are publicly available on the Birdwatch site for anyone in the USA.\n\n\nUsing Birdwatch data from January to July 2021, a positive (negative) edge is created from participant 𝑈1 to 𝑈2 if participant 𝑈1 rated a note written by participant 𝑈2 as *helpful* (*not helpful*). The *somewhat helpful* ratings were filtered out. The topic associated with an edge is the topic inferred from the tweet the note refers to.\n\n\n36.9% of edges are negative (opposition) and 63.1% are positive. There may be several edges between two nodes (several interactions, several topics).\n\n\n\nIf you use our datasets in your work, please cite the following:"
] | [
24,
325,
319
] | [
"passage: TAGS\n#license-cc-by-4.0 #arxiv-2201.11675 #region-us \n### TwitterSG\n\n\nTwitter Signed Graph, or TwitterSG, is a signed, directed, edge-attributed graph of users, drawn from Twitter interactions. TwitterSG contains 753,944 nodes (users), 200 topics and 12,848,093 edges. It is the largest publicly available user-to-user signed social graph (∼6x larger than the Epinions graph).\n\n\nA positive edge exists from user 𝐴 to user 𝐵 if user 𝐴 liked a tweet posted by user 𝐵. A negative edge exists from user 𝐴 to user 𝐵 if user 𝐴 expressed opposition towards user 𝐵’s tweet, e.g., by replying *I disagree with you*. The full list of opposition keywords is specified here. The topic of an edge from user 𝐴 to user 𝐵 is determined by the topic of user 𝐵’s tweet.\n\n\nTweets' topics were inferred with a topic classifier used in production by Twitter. The topics provided in the dataset are all related to sports (e.g., sports teams, players, managers, or events), and the tweets related to these interactions were published between 20th May (Ice Hockey World Championships) and 8th August 2021 (closing date of the 2020 Tokyo Olympic Games).\n\n\n9.6% of edges are negative (opposition) and 90.4% are positive. There may be several edges between two nodes (several interactions, several topics). The data format is displayed below."
] |
38540c67529371fa1677be845251bcbd8105a82a |
# Dataset Card for Dead by Daylight perks
## Table of Contents
- [Table of Contents](#table-of-contents)
- [Dataset Description](#dataset-description)
- [Dataset Summary](#dataset-summary)
- [Dataset Creation](#dataset-creation)
- [Source Data](#source-data)
- [Additional Information](#additional-information)
- [Licensing Information](#licensing-information)
- [Contributions](#contributions)
### Dataset Summary
This dataset contains all images (on black background and upscaled to 512x512) of perks from the video game [Dead by Daylight](https://deadbydaylight.com/) with type, name and description (the first sentence) in english.
## Dataset Creation
### Source Data
All images and text have been found online, mainly on the [Dead by Daylight wiki](https://deadbydaylight.fandom.com/wiki/Dead_by_Daylight_Wiki).
## Additional Information
### Licensing Information
All images belong to [Dead by Daylight](https://deadbydaylight.com/).
### Contributions
Thanks to [@GabrielVidal1](https://github.com/GabrielVidal1) for adding this dataset. | GabrielVidal/dead-by-daylight-perks | [
"task_categories:image-classification",
"task_categories:text-to-image",
"task_ids:multi-class-image-classification",
"annotations_creators:found",
"language_creators:found",
"multilinguality:monolingual",
"size_categories:n<1K",
"source_datasets:original",
"language:en",
"license:openrail",
"dead by daylight",
"region:us"
] | 2022-11-21T20:42:24+00:00 | {"annotations_creators": ["found"], "language_creators": ["found"], "language": ["en"], "license": "openrail", "multilinguality": ["monolingual"], "size_categories": ["n<1K"], "source_datasets": ["original"], "task_categories": ["image-classification", "text-to-image"], "task_ids": ["multi-class-image-classification"], "pretty_name": "Dead by daylight video game perks", "dataset_info": {"features": [{"name": "image", "dtype": "image"}, {"name": "name", "dtype": "string"}, {"name": "type", "dtype": "string"}, {"name": "description", "dtype": "string"}], "splits": [{"name": "train", "num_bytes": 22392351.0, "num_examples": 219}], "download_size": 22365600, "dataset_size": 22392351.0}, "tags": ["dead by daylight"]} | 2022-11-27T16:06:46+00:00 | [] | [
"en"
] | TAGS
#task_categories-image-classification #task_categories-text-to-image #task_ids-multi-class-image-classification #annotations_creators-found #language_creators-found #multilinguality-monolingual #size_categories-n<1K #source_datasets-original #language-English #license-openrail #dead by daylight #region-us
|
# Dataset Card for Dead by Daylight perks
## Table of Contents
- Table of Contents
- Dataset Description
- Dataset Summary
- Dataset Creation
- Source Data
- Additional Information
- Licensing Information
- Contributions
### Dataset Summary
This dataset contains all images (on black background and upscaled to 512x512) of perks from the video game Dead by Daylight with type, name and description (the first sentence) in english.
## Dataset Creation
### Source Data
All images and text have been found online, mainly on the Dead by Daylight wiki.
## Additional Information
### Licensing Information
All images belong to Dead by Daylight.
### Contributions
Thanks to @GabrielVidal1 for adding this dataset. | [
"# Dataset Card for Dead by Daylight perks",
"## Table of Contents\n- Table of Contents\n- Dataset Description\n - Dataset Summary\n- Dataset Creation\n - Source Data\n- Additional Information\n - Licensing Information\n - Contributions",
"### Dataset Summary\n\nThis dataset contains all images (on black background and upscaled to 512x512) of perks from the video game Dead by Daylight with type, name and description (the first sentence) in english.",
"## Dataset Creation",
"### Source Data\n\nAll images and text have been found online, mainly on the Dead by Daylight wiki.",
"## Additional Information",
"### Licensing Information\n\nAll images belong to Dead by Daylight.",
"### Contributions\n\nThanks to @GabrielVidal1 for adding this dataset."
] | [
"TAGS\n#task_categories-image-classification #task_categories-text-to-image #task_ids-multi-class-image-classification #annotations_creators-found #language_creators-found #multilinguality-monolingual #size_categories-n<1K #source_datasets-original #language-English #license-openrail #dead by daylight #region-us \n",
"# Dataset Card for Dead by Daylight perks",
"## Table of Contents\n- Table of Contents\n- Dataset Description\n - Dataset Summary\n- Dataset Creation\n - Source Data\n- Additional Information\n - Licensing Information\n - Contributions",
"### Dataset Summary\n\nThis dataset contains all images (on black background and upscaled to 512x512) of perks from the video game Dead by Daylight with type, name and description (the first sentence) in english.",
"## Dataset Creation",
"### Source Data\n\nAll images and text have been found online, mainly on the Dead by Daylight wiki.",
"## Additional Information",
"### Licensing Information\n\nAll images belong to Dead by Daylight.",
"### Contributions\n\nThanks to @GabrielVidal1 for adding this dataset."
] | [
103,
11,
41,
51,
5,
23,
5,
16,
20
] | [
"passage: TAGS\n#task_categories-image-classification #task_categories-text-to-image #task_ids-multi-class-image-classification #annotations_creators-found #language_creators-found #multilinguality-monolingual #size_categories-n<1K #source_datasets-original #language-English #license-openrail #dead by daylight #region-us \n# Dataset Card for Dead by Daylight perks## Table of Contents\n- Table of Contents\n- Dataset Description\n - Dataset Summary\n- Dataset Creation\n - Source Data\n- Additional Information\n - Licensing Information\n - Contributions### Dataset Summary\n\nThis dataset contains all images (on black background and upscaled to 512x512) of perks from the video game Dead by Daylight with type, name and description (the first sentence) in english.## Dataset Creation### Source Data\n\nAll images and text have been found online, mainly on the Dead by Daylight wiki.## Additional Information### Licensing Information\n\nAll images belong to Dead by Daylight.### Contributions\n\nThanks to @GabrielVidal1 for adding this dataset."
] |
aa8060833dacf80e78e83b3666ccf4e39802ec9a | # Dataset Card for "mintaka-qa-en"
[More Information needed](https://github.com/huggingface/datasets/blob/main/CONTRIBUTING.md#how-to-contribute-to-the-dataset-cards) | Rexhaif/mintaka-qa-en | [
"region:us"
] | 2022-11-21T21:35:40+00:00 | {"dataset_info": {"features": [{"name": "question", "dtype": "string"}, {"name": "answer", "dtype": "string"}], "splits": [{"name": "train", "num_bytes": 1047958, "num_examples": 14000}, {"name": "dev", "num_bytes": 150348, "num_examples": 2000}, {"name": "test", "num_bytes": 298101, "num_examples": 4000}], "download_size": 787307, "dataset_size": 1496407}} | 2022-11-21T21:36:47+00:00 | [] | [] | TAGS
#region-us
| # Dataset Card for "mintaka-qa-en"
More Information needed | [
"# Dataset Card for \"mintaka-qa-en\"\n\nMore Information needed"
] | [
"TAGS\n#region-us \n",
"# Dataset Card for \"mintaka-qa-en\"\n\nMore Information needed"
] | [
6,
16
] | [
"passage: TAGS\n#region-us \n# Dataset Card for \"mintaka-qa-en\"\n\nMore Information needed"
] |
38018509896c4f2282b37b76fa90789b356130af | Dummy dataset to check reward model training is learning correctly. Score is the number of question marks in Ray's response. | Jellywibble/dummy_dalio_questions_score | [
"region:us"
] | 2022-11-21T21:56:04+00:00 | {} | 2022-11-21T22:36:32+00:00 | [] | [] | TAGS
#region-us
| Dummy dataset to check reward model training is learning correctly. Score is the number of question marks in Ray's response. | [] | [
"TAGS\n#region-us \n"
] | [
6
] | [
"passage: TAGS\n#region-us \n"
] |
1f109ee9a8cf3c3c33fbfc5c4c3766382785d0ab | # Dataset Card for "VMMRdb_make_model_train"
[More Information needed](https://github.com/huggingface/datasets/blob/main/CONTRIBUTING.md#how-to-contribute-to-the-dataset-cards) | venetis/VMMRdb_make_model_train | [
"region:us"
] | 2022-11-21T22:46:11+00:00 | {"dataset_info": {"features": [{"name": "image", "dtype": "image"}, {"name": "label", "dtype": {"class_label": {"names": {"0": "acura_cl", "1": "acura_integra", "2": "acura_legend", "3": "acura_mdx", "4": "acura_rdx", "5": "acura_rl", "6": "acura_rsx", "7": "acura_tl", "8": "acura_tsx", "9": "audi_a3", "10": "audi_a4", "11": "audi_a6", "12": "audi_a8", "13": "audi_s4", "14": "audi_tt", "15": "bmw_323i", "16": "bmw_325i", "17": "bmw_328i", "18": "bmw_330ci", "19": "bmw_330i", "20": "bmw_335i", "21": "bmw_525i", "22": "bmw_528i", "23": "bmw_530i", "24": "bmw_535i", "25": "bmw_540i", "26": "bmw_545i", "27": "bmw_550i", "28": "bmw_740i", "29": "bmw_745i", "30": "bmw_750i", "31": "bmw_m3", "32": "bmw_m5", "33": "bmw_x3", "34": "bmw_x5", "35": "bmw_z3", "36": "bmw_z4", "37": "buick_century", "38": "buick_enclave", "39": "buick_lacrosse", "40": "buick_lesabre", "41": "buick_lucerne", "42": "buick_parkavenue", "43": "buick_regal", "44": "buick_rendezvous", "45": "buick_riviera", "46": "cadillac_catera", "47": "cadillac_cts", "48": "cadillac_deville", "49": "cadillac_eldorado", "50": "cadillac_escalade", "51": "cadillac_seville", "52": "cadillac_srx", "53": "cadillac_sts", "54": "chevrolet_astro", "55": "chevrolet_avalanche", "56": "chevrolet_aveo", "57": "chevrolet_bel air", "58": "chevrolet_blazer", "59": "chevrolet_c-k1500", "60": "chevrolet_c10", "61": "chevrolet_camaro", "62": "chevrolet_caprice", "63": "chevrolet_cavalier", "64": "chevrolet_chevelle", "65": "chevrolet_cobalt", "66": "chevrolet_colorado", "67": "chevrolet_corvette", "68": "chevrolet_cruze", "69": "chevrolet_el camino", "70": "chevrolet_equinox", "71": "chevrolet_express", "72": "chevrolet_hhr", "73": "chevrolet_impala", "74": "chevrolet_lumina", "75": "chevrolet_malibu", "76": "chevrolet_montecarlo", "77": "chevrolet_nova", "78": "chevrolet_prizm", "79": "chevrolet_s10", "80": "chevrolet_silverado", "81": "chevrolet_sonic", "82": "chevrolet_suburban", "83": "chevrolet_tahoe", "84": "chevrolet_tracker", "85": "chevrolet_trailblazer", "86": "chevrolet_traverse", "87": "chevrolet_uplander", "88": "chevrolet_venture", "89": "chrysler_200", "90": "chrysler_300", "91": "chrysler_concorde", "92": "chrysler_crossfire", "93": "chrysler_pacifica", "94": "chrysler_pt cruiser", "95": "chrysler_sebring", "96": "chrysler_town&country", "97": "chrysler_voyager", "98": "dodge_avenger", "99": "dodge_caliber", "100": "dodge_challenger", "101": "dodge_charger", "102": "dodge_dakota", "103": "dodge_dart", "104": "dodge_durango", "105": "dodge_grand caravan", "106": "dodge_intrepid", "107": "dodge_journey", "108": "dodge_magnum", "109": "dodge_neon", "110": "dodge_nitro", "111": "dodge_ram", "112": "dodge_stratus", "113": "fiat_five hundred", "114": "ford_bronco", "115": "ford_contour", "116": "ford_crown victoria", "117": "ford_e150", "118": "ford_e250", "119": "ford_e350", "120": "ford_edge", "121": "ford_escape", "122": "ford_escort", "123": "ford_excursion", "124": "ford_expedition", "125": "ford_explorer", "126": "ford_f100", "127": "ford_f150", "128": "ford_f250", "129": "ford_f350", "130": "ford_f450", "131": "ford_fiesta", "132": "ford_five hundred", "133": "ford_focus", "134": "ford_freestar", "135": "ford_fusion", "136": "ford_mustang", "137": "ford_ranger", "138": "ford_taurus", "139": "ford_thunderbird", "140": "ford_windstar", "141": "gmc_acadia", "142": "gmc_canyon", "143": "gmc_envoy", "144": "gmc_jimmy", "145": "gmc_sierra", "146": "gmc_sonoma", "147": "gmc_suburban", "148": "gmc_terrain", "149": "gmc_yukon", "150": "honda_accord", "151": "honda_civic", "152": "honda_cr-v", "153": "honda_delsol", "154": "honda_element", "155": "honda_fit", "156": "honda_odyssey", "157": "honda_passport", "158": "honda_pilot", "159": "honda_prelude", "160": "honda_ridgeline", "161": "honda_s2000", "162": "hummer_h2", "163": "hummer_h3", "164": "hyundai_accent", "165": "hyundai_azera", "166": "hyundai_elantra", "167": "hyundai_genesis", "168": "hyundai_santafe", "169": "hyundai_sonata", "170": "hyundai_tiburon", "171": "hyundai_tucson", "172": "infiniti_fx35", "173": "infiniti_g35", "174": "infiniti_g37", "175": "infiniti_i30", "176": "infiniti_i35", "177": "infiniti_m35", "178": "infiniti_q45", "179": "infiniti_qx4", "180": "infiniti_qx56", "181": "isuzu_rodeo", "182": "isuzu_trooper", "183": "jaguar_s-type", "184": "jaguar_x-type", "185": "jaguar_xj", "186": "jeep_cherokee", "187": "jeep_cj5", "188": "jeep_cj7", "189": "jeep_commander", "190": "jeep_compass", "191": "jeep_grand", "192": "jeep_liberty", "193": "jeep_patriot", "194": "jeep_wrangler", "195": "kia_amanti", "196": "kia_forte", "197": "kia_optima", "198": "kia_rio", "199": "kia_sedona", "200": "kia_sephia", "201": "kia_sorento", "202": "kia_soul", "203": "kia_spectra", "204": "kia_sportage", "205": "landrover_discovery", "206": "landrover_rangerover", "207": "lexus_es300", "208": "lexus_es330", "209": "lexus_es350", "210": "lexus_gs300", "211": "lexus_gx470", "212": "lexus_is250", "213": "lexus_is300", "214": "lexus_is350", "215": "lexus_ls400", "216": "lexus_ls430", "217": "lexus_rx300", "218": "lexus_rx330", "219": "lexus_sc430", "220": "lincoln_aviator", "221": "lincoln_continental", "222": "lincoln_ls", "223": "lincoln_mark", "224": "lincoln_mkx", "225": "lincoln_mkz", "226": "lincoln_navigator", "227": "lincoln_towncar", "228": "mazda_3", "229": "mazda_5", "230": "mazda_6", "231": "mazda_626", "232": "mazda_millenia", "233": "mazda_mpv", "234": "mazda_mx5", "235": "mazda_protege", "236": "mazda_rx7", "237": "mazda_rx8", "238": "mazda_tribute", "239": "mercedes benz_c230", "240": "mercedes benz_c240", "241": "mercedes benz_c280", "242": "mercedes benz_c300", "243": "mercedes benz_c320", "244": "mercedes benz_clk320", "245": "mercedes benz_e320", "246": "mercedes benz_e350", "247": "mercedes benz_e500", "248": "mercedes benz_ml320", "249": "mercedes benz_ml350", "250": "mercedes benz_ml500", "251": "mercedes benz_s430", "252": "mercedes benz_s500", "253": "mercedes benz_s550", "254": "mercedes benz_sl500", "255": "mercury_cougar", "256": "mercury_grandmarquis", "257": "mercury_mariner", "258": "mercury_milan", "259": "mercury_mountaineer", "260": "mercury_sable", "261": "mercury_villager", "262": "mini_cooper", "263": "mitsubishi_3000gt", "264": "mitsubishi_eclipse", "265": "mitsubishi_endeavor", "266": "mitsubishi_galant", "267": "mitsubishi_lancer", "268": "mitsubishi_mirage", "269": "mitsubishi_montero", "270": "mitsubishi_outlander", "271": "nissan_240sx", "272": "nissan_300zx", "273": "nissan_350z", "274": "nissan_altima", "275": "nissan_armada", "276": "nissan_frontier", "277": "nissan_maxima", "278": "nissan_murano", "279": "nissan_pathfinder", "280": "nissan_quest", "281": "nissan_rogue", "282": "nissan_sentra", "283": "nissan_titan", "284": "nissan_versa", "285": "nissan_xterra", "286": "oldsmobile_alero", "287": "oldsmobile_aurora", "288": "oldsmobile_bravada", "289": "oldsmobile_cutlass", "290": "oldsmobile_intrigue", "291": "oldsmobile_silhouette", "292": "plymouth_neon", "293": "plymouth_voyager", "294": "pontiac_bonneville", "295": "pontiac_firebird", "296": "pontiac_g5", "297": "pontiac_g6", "298": "pontiac_grandam", "299": "pontiac_grandprix", "300": "pontiac_gto", "301": "pontiac_montana", "302": "pontiac_sunfire", "303": "pontiac_torrent", "304": "pontiac_transam", "305": "pontiac_vibe", "306": "porsche_911", "307": "porsche_boxster", "308": "porsche_cayenne", "309": "ram_1500", "310": "saab_9-3", "311": "saab_9-5", "312": "saturn_aura", "313": "saturn_ion", "314": "saturn_l200", "315": "saturn_l300", "316": "saturn_sl1", "317": "saturn_sl2", "318": "saturn_vue", "319": "scion_tc", "320": "scion_xa", "321": "scion_xb", "322": "scion_xd", "323": "smart_fortwo", "324": "subaru_forester", "325": "subaru_impreza", "326": "subaru_legacy", "327": "subaru_outback", "328": "subaru_wrx", "329": "suzuki_forenza", "330": "suzuki_sx4", "331": "suzuki_xl7", "332": "toyota_4runner", "333": "toyota_avalon", "334": "toyota_camry", "335": "toyota_celica", "336": "toyota_corolla", "337": "toyota_echo", "338": "toyota_fjcruiser", "339": "toyota_highlander", "340": "toyota_landcruiser", "341": "toyota_matrix", "342": "toyota_mr2", "343": "toyota_pickup", "344": "toyota_prius", "345": "toyota_rav4", "346": "toyota_sequoia", "347": "toyota_sienna", "348": "toyota_solara", "349": "toyota_supra", "350": "toyota_t100", "351": "toyota_tacoma", "352": "toyota_tercel", "353": "toyota_tundra", "354": "toyota_yaris", "355": "volkswagen_beetle", "356": "volkswagen_bug", "357": "volkswagen_cc", "358": "volkswagen_eos", "359": "volkswagen_golf", "360": "volkswagen_gti", "361": "volkswagen_jetta", "362": "volkswagen_newbeetle", "363": "volkswagen_passat", "364": "volkswagen_rabbit", "365": "volkswagen_touareg", "366": "volvo_850", "367": "volvo_c70", "368": "volvo_s40", "369": "volvo_s60", "370": "volvo_s70", "371": "volvo_s80", "372": "volvo_v70", "373": "volvo_xc70", "374": "volvo_xc90"}}}}], "splits": [{"name": "train", "num_bytes": 4490369111.482906, "num_examples": 241664}], "download_size": 4489644227, "dataset_size": 4490369111.482906}} | 2022-11-24T04:01:45+00:00 | [] | [] | TAGS
#region-us
| # Dataset Card for "VMMRdb_make_model_train"
More Information needed | [
"# Dataset Card for \"VMMRdb_make_model_train\"\n\nMore Information needed"
] | [
"TAGS\n#region-us \n",
"# Dataset Card for \"VMMRdb_make_model_train\"\n\nMore Information needed"
] | [
6,
20
] | [
"passage: TAGS\n#region-us \n# Dataset Card for \"VMMRdb_make_model_train\"\n\nMore Information needed"
] |
3f53bee1e3478473040f74e11f5d4bea7811bc83 | # NYT News Dataset, 2008-2021 from [Kaggle](https://www.kaggle.com/datasets/brendanmiles/nyt-news-dataset-20082021)
Includes Title, Date, Topics in the News, Abstract, and Keywords
[Github repo with source code](https://github.com/jaimebw/nyt_hugginface) | jaimebw/nyt_dataset | [
"license:cc0-1.0",
"region:us"
] | 2022-11-21T23:42:25+00:00 | {"license": "cc0-1.0"} | 2022-12-16T05:51:38+00:00 | [] | [] | TAGS
#license-cc0-1.0 #region-us
| # NYT News Dataset, 2008-2021 from Kaggle
Includes Title, Date, Topics in the News, Abstract, and Keywords
Github repo with source code | [
"# NYT News Dataset, 2008-2021 from Kaggle\nIncludes Title, Date, Topics in the News, Abstract, and Keywords\n\nGithub repo with source code"
] | [
"TAGS\n#license-cc0-1.0 #region-us \n",
"# NYT News Dataset, 2008-2021 from Kaggle\nIncludes Title, Date, Topics in the News, Abstract, and Keywords\n\nGithub repo with source code"
] | [
14,
38
] | [
"passage: TAGS\n#license-cc0-1.0 #region-us \n# NYT News Dataset, 2008-2021 from Kaggle\nIncludes Title, Date, Topics in the News, Abstract, and Keywords\n\nGithub repo with source code"
] |
af74d12be027242907185d62930b1bbe619db355 | # Dataset Card for "VMMRdb_make_model_val"
[More Information needed](https://github.com/huggingface/datasets/blob/main/CONTRIBUTING.md#how-to-contribute-to-the-dataset-cards) | venetis/VMMRdb_make_model_val | [
"region:us"
] | 2022-11-21T23:46:18+00:00 | {"dataset_info": {"features": [{"name": "image", "dtype": "image"}, {"name": "label", "dtype": {"class_label": {"names": {"0": "acura_cl", "1": "acura_integra", "2": "acura_legend", "3": "acura_mdx", "4": "acura_rdx", "5": "acura_rl", "6": "acura_rsx", "7": "acura_tl", "8": "acura_tsx", "9": "audi_a3", "10": "audi_a4", "11": "audi_a6", "12": "audi_a8", "13": "audi_s4", "14": "audi_tt", "15": "bmw_323i", "16": "bmw_325i", "17": "bmw_328i", "18": "bmw_330ci", "19": "bmw_330i", "20": "bmw_335i", "21": "bmw_525i", "22": "bmw_528i", "23": "bmw_530i", "24": "bmw_535i", "25": "bmw_540i", "26": "bmw_545i", "27": "bmw_550i", "28": "bmw_740i", "29": "bmw_745i", "30": "bmw_750i", "31": "bmw_m3", "32": "bmw_m5", "33": "bmw_x3", "34": "bmw_x5", "35": "bmw_z3", "36": "bmw_z4", "37": "buick_century", "38": "buick_enclave", "39": "buick_lacrosse", "40": "buick_lesabre", "41": "buick_lucerne", "42": "buick_parkavenue", "43": "buick_regal", "44": "buick_rendezvous", "45": "buick_riviera", "46": "cadillac_catera", "47": "cadillac_cts", "48": "cadillac_deville", "49": "cadillac_eldorado", "50": "cadillac_escalade", "51": "cadillac_seville", "52": "cadillac_srx", "53": "cadillac_sts", "54": "chevrolet_astro", "55": "chevrolet_avalanche", "56": "chevrolet_aveo", "57": "chevrolet_bel air", "58": "chevrolet_blazer", "59": "chevrolet_c-k1500", "60": "chevrolet_c10", "61": "chevrolet_camaro", "62": "chevrolet_caprice", "63": "chevrolet_cavalier", "64": "chevrolet_chevelle", "65": "chevrolet_cobalt", "66": "chevrolet_colorado", "67": "chevrolet_corvette", "68": "chevrolet_cruze", "69": "chevrolet_el camino", "70": "chevrolet_equinox", "71": "chevrolet_express", "72": "chevrolet_hhr", "73": "chevrolet_impala", "74": "chevrolet_lumina", "75": "chevrolet_malibu", "76": "chevrolet_montecarlo", "77": "chevrolet_nova", "78": "chevrolet_prizm", "79": "chevrolet_s10", "80": "chevrolet_silverado", "81": "chevrolet_sonic", "82": "chevrolet_suburban", "83": "chevrolet_tahoe", "84": "chevrolet_tracker", "85": "chevrolet_trailblazer", "86": "chevrolet_traverse", "87": "chevrolet_uplander", "88": "chevrolet_venture", "89": "chrysler_200", "90": "chrysler_300", "91": "chrysler_concorde", "92": "chrysler_crossfire", "93": "chrysler_pacifica", "94": "chrysler_pt cruiser", "95": "chrysler_sebring", "96": "chrysler_town&country", "97": "chrysler_voyager", "98": "dodge_avenger", "99": "dodge_caliber", "100": "dodge_challenger", "101": "dodge_charger", "102": "dodge_dakota", "103": "dodge_dart", "104": "dodge_durango", "105": "dodge_grand caravan", "106": "dodge_intrepid", "107": "dodge_journey", "108": "dodge_magnum", "109": "dodge_neon", "110": "dodge_nitro", "111": "dodge_ram", "112": "dodge_stratus", "113": "fiat_five hundred", "114": "ford_bronco", "115": "ford_contour", "116": "ford_crown victoria", "117": "ford_e150", "118": "ford_e250", "119": "ford_e350", "120": "ford_edge", "121": "ford_escape", "122": "ford_escort", "123": "ford_excursion", "124": "ford_expedition", "125": "ford_explorer", "126": "ford_f100", "127": "ford_f150", "128": "ford_f250", "129": "ford_f350", "130": "ford_f450", "131": "ford_fiesta", "132": "ford_five hundred", "133": "ford_focus", "134": "ford_freestar", "135": "ford_fusion", "136": "ford_mustang", "137": "ford_ranger", "138": "ford_taurus", "139": "ford_thunderbird", "140": "ford_windstar", "141": "gmc_acadia", "142": "gmc_canyon", "143": "gmc_envoy", "144": "gmc_jimmy", "145": "gmc_sierra", "146": "gmc_sonoma", "147": "gmc_suburban", "148": "gmc_terrain", "149": "gmc_yukon", "150": "honda_accord", "151": "honda_civic", "152": "honda_cr-v", "153": "honda_delsol", "154": "honda_element", "155": "honda_fit", "156": "honda_odyssey", "157": "honda_passport", "158": "honda_pilot", "159": "honda_prelude", "160": "honda_ridgeline", "161": "honda_s2000", "162": "hummer_h2", "163": "hummer_h3", "164": "hyundai_accent", "165": "hyundai_azera", "166": "hyundai_elantra", "167": "hyundai_genesis", "168": "hyundai_santafe", "169": "hyundai_sonata", "170": "hyundai_tiburon", "171": "hyundai_tucson", "172": "infiniti_fx35", "173": "infiniti_g35", "174": "infiniti_g37", "175": "infiniti_i30", "176": "infiniti_i35", "177": "infiniti_m35", "178": "infiniti_q45", "179": "infiniti_qx4", "180": "infiniti_qx56", "181": "isuzu_rodeo", "182": "isuzu_trooper", "183": "jaguar_s-type", "184": "jaguar_x-type", "185": "jaguar_xj", "186": "jeep_cherokee", "187": "jeep_cj5", "188": "jeep_cj7", "189": "jeep_commander", "190": "jeep_compass", "191": "jeep_grand", "192": "jeep_liberty", "193": "jeep_patriot", "194": "jeep_wrangler", "195": "kia_amanti", "196": "kia_forte", "197": "kia_optima", "198": "kia_rio", "199": "kia_sedona", "200": "kia_sephia", "201": "kia_sorento", "202": "kia_soul", "203": "kia_spectra", "204": "kia_sportage", "205": "landrover_discovery", "206": "landrover_rangerover", "207": "lexus_es300", "208": "lexus_es330", "209": "lexus_es350", "210": "lexus_gs300", "211": "lexus_gx470", "212": "lexus_is250", "213": "lexus_is300", "214": "lexus_is350", "215": "lexus_ls400", "216": "lexus_ls430", "217": "lexus_rx300", "218": "lexus_rx330", "219": "lexus_sc430", "220": "lincoln_aviator", "221": "lincoln_continental", "222": "lincoln_ls", "223": "lincoln_mark", "224": "lincoln_mkx", "225": "lincoln_mkz", "226": "lincoln_navigator", "227": "lincoln_towncar", "228": "mazda_3", "229": "mazda_5", "230": "mazda_6", "231": "mazda_626", "232": "mazda_millenia", "233": "mazda_mpv", "234": "mazda_mx5", "235": "mazda_protege", "236": "mazda_rx7", "237": "mazda_rx8", "238": "mazda_tribute", "239": "mercedes benz_c230", "240": "mercedes benz_c240", "241": "mercedes benz_c280", "242": "mercedes benz_c300", "243": "mercedes benz_c320", "244": "mercedes benz_clk320", "245": "mercedes benz_e320", "246": "mercedes benz_e350", "247": "mercedes benz_e500", "248": "mercedes benz_ml320", "249": "mercedes benz_ml350", "250": "mercedes benz_ml500", "251": "mercedes benz_s430", "252": "mercedes benz_s500", "253": "mercedes benz_s550", "254": "mercedes benz_sl500", "255": "mercury_cougar", "256": "mercury_grandmarquis", "257": "mercury_mariner", "258": "mercury_milan", "259": "mercury_mountaineer", "260": "mercury_sable", "261": "mercury_villager", "262": "mini_cooper", "263": "mitsubishi_3000gt", "264": "mitsubishi_eclipse", "265": "mitsubishi_endeavor", "266": "mitsubishi_galant", "267": "mitsubishi_lancer", "268": "mitsubishi_mirage", "269": "mitsubishi_montero", "270": "mitsubishi_outlander", "271": "nissan_240sx", "272": "nissan_300zx", "273": "nissan_350z", "274": "nissan_altima", "275": "nissan_armada", "276": "nissan_frontier", "277": "nissan_maxima", "278": "nissan_murano", "279": "nissan_pathfinder", "280": "nissan_quest", "281": "nissan_rogue", "282": "nissan_sentra", "283": "nissan_titan", "284": "nissan_versa", "285": "nissan_xterra", "286": "oldsmobile_alero", "287": "oldsmobile_aurora", "288": "oldsmobile_bravada", "289": "oldsmobile_cutlass", "290": "oldsmobile_intrigue", "291": "oldsmobile_silhouette", "292": "plymouth_neon", "293": "plymouth_voyager", "294": "pontiac_bonneville", "295": "pontiac_firebird", "296": "pontiac_g5", "297": "pontiac_g6", "298": "pontiac_grandam", "299": "pontiac_grandprix", "300": "pontiac_gto", "301": "pontiac_montana", "302": "pontiac_sunfire", "303": "pontiac_torrent", "304": "pontiac_transam", "305": "pontiac_vibe", "306": "porsche_911", "307": "porsche_boxster", "308": "porsche_cayenne", "309": "ram_1500", "310": "saab_9-3", "311": "saab_9-5", "312": "saturn_aura", "313": "saturn_ion", "314": "saturn_l200", "315": "saturn_l300", "316": "saturn_sl1", "317": "saturn_sl2", "318": "saturn_vue", "319": "scion_tc", "320": "scion_xa", "321": "scion_xb", "322": "scion_xd", "323": "smart_fortwo", "324": "subaru_forester", "325": "subaru_impreza", "326": "subaru_legacy", "327": "subaru_outback", "328": "subaru_wrx", "329": "suzuki_forenza", "330": "suzuki_sx4", "331": "suzuki_xl7", "332": "toyota_4runner", "333": "toyota_avalon", "334": "toyota_camry", "335": "toyota_celica", "336": "toyota_corolla", "337": "toyota_echo", "338": "toyota_fjcruiser", "339": "toyota_highlander", "340": "toyota_landcruiser", "341": "toyota_matrix", "342": "toyota_mr2", "343": "toyota_pickup", "344": "toyota_prius", "345": "toyota_rav4", "346": "toyota_sequoia", "347": "toyota_sienna", "348": "toyota_solara", "349": "toyota_supra", "350": "toyota_t100", "351": "toyota_tacoma", "352": "toyota_tercel", "353": "toyota_tundra", "354": "toyota_yaris", "355": "volkswagen_beetle", "356": "volkswagen_bug", "357": "volkswagen_cc", "358": "volkswagen_eos", "359": "volkswagen_golf", "360": "volkswagen_gti", "361": "volkswagen_jetta", "362": "volkswagen_newbeetle", "363": "volkswagen_passat", "364": "volkswagen_rabbit", "365": "volkswagen_touareg", "366": "volvo_850", "367": "volvo_c70", "368": "volvo_s40", "369": "volvo_s60", "370": "volvo_s70", "371": "volvo_s80", "372": "volvo_v70", "373": "volvo_xc70", "374": "volvo_xc90"}}}}], "splits": [{"name": "train", "num_bytes": 498938159.51709396, "num_examples": 26852}], "download_size": 498718383, "dataset_size": 498938159.51709396}} | 2022-11-24T04:04:10+00:00 | [] | [] | TAGS
#region-us
| # Dataset Card for "VMMRdb_make_model_val"
More Information needed | [
"# Dataset Card for \"VMMRdb_make_model_val\"\n\nMore Information needed"
] | [
"TAGS\n#region-us \n",
"# Dataset Card for \"VMMRdb_make_model_val\"\n\nMore Information needed"
] | [
6,
19
] | [
"passage: TAGS\n#region-us \n# Dataset Card for \"VMMRdb_make_model_val\"\n\nMore Information needed"
] |
4cebbd89a736a190e77ee3d131109e9a029c2d83 | # Dataset Card for "VMMRdb_make_model_test"
[More Information needed](https://github.com/huggingface/datasets/blob/main/CONTRIBUTING.md#how-to-contribute-to-the-dataset-cards) | venetis/VMMRdb_make_model_test | [
"region:us"
] | 2022-11-22T00:53:42+00:00 | {"dataset_info": {"features": [{"name": "image", "dtype": "image"}, {"name": "label", "dtype": {"class_label": {"names": {"0": "acura_cl", "1": "acura_integra", "2": "acura_legend", "3": "acura_mdx", "4": "acura_rdx", "5": "acura_rl", "6": "acura_rsx", "7": "acura_tl", "8": "acura_tsx", "9": "audi_a3", "10": "audi_a4", "11": "audi_a6", "12": "audi_a8", "13": "audi_s4", "14": "audi_tt", "15": "bmw_323i", "16": "bmw_325i", "17": "bmw_328i", "18": "bmw_330ci", "19": "bmw_330i", "20": "bmw_335i", "21": "bmw_525i", "22": "bmw_528i", "23": "bmw_530i", "24": "bmw_535i", "25": "bmw_540i", "26": "bmw_545i", "27": "bmw_550i", "28": "bmw_740i", "29": "bmw_745i", "30": "bmw_750i", "31": "bmw_m3", "32": "bmw_m5", "33": "bmw_x3", "34": "bmw_x5", "35": "bmw_z3", "36": "bmw_z4", "37": "buick_century", "38": "buick_enclave", "39": "buick_lacrosse", "40": "buick_lesabre", "41": "buick_lucerne", "42": "buick_parkavenue", "43": "buick_regal", "44": "buick_rendezvous", "45": "buick_riviera", "46": "cadillac_catera", "47": "cadillac_cts", "48": "cadillac_deville", "49": "cadillac_eldorado", "50": "cadillac_escalade", "51": "cadillac_seville", "52": "cadillac_srx", "53": "cadillac_sts", "54": "chevrolet_astro", "55": "chevrolet_avalanche", "56": "chevrolet_aveo", "57": "chevrolet_bel air", "58": "chevrolet_blazer", "59": "chevrolet_c-k1500", "60": "chevrolet_c10", "61": "chevrolet_camaro", "62": "chevrolet_caprice", "63": "chevrolet_cavalier", "64": "chevrolet_chevelle", "65": "chevrolet_cobalt", "66": "chevrolet_colorado", "67": "chevrolet_corvette", "68": "chevrolet_cruze", "69": "chevrolet_el camino", "70": "chevrolet_equinox", "71": "chevrolet_express", "72": "chevrolet_hhr", "73": "chevrolet_impala", "74": "chevrolet_lumina", "75": "chevrolet_malibu", "76": "chevrolet_montecarlo", "77": "chevrolet_nova", "78": "chevrolet_prizm", "79": "chevrolet_s10", "80": "chevrolet_silverado", "81": "chevrolet_sonic", "82": "chevrolet_suburban", "83": "chevrolet_tahoe", "84": "chevrolet_tracker", "85": "chevrolet_trailblazer", "86": "chevrolet_traverse", "87": "chevrolet_uplander", "88": "chevrolet_venture", "89": "chrysler_200", "90": "chrysler_300", "91": "chrysler_concorde", "92": "chrysler_crossfire", "93": "chrysler_pacifica", "94": "chrysler_pt cruiser", "95": "chrysler_sebring", "96": "chrysler_town&country", "97": "chrysler_voyager", "98": "dodge_avenger", "99": "dodge_caliber", "100": "dodge_challenger", "101": "dodge_charger", "102": "dodge_dakota", "103": "dodge_dart", "104": "dodge_durango", "105": "dodge_grand caravan", "106": "dodge_intrepid", "107": "dodge_journey", "108": "dodge_magnum", "109": "dodge_neon", "110": "dodge_nitro", "111": "dodge_ram", "112": "dodge_stratus", "113": "fiat_five hundred", "114": "ford_bronco", "115": "ford_contour", "116": "ford_crown victoria", "117": "ford_e150", "118": "ford_e250", "119": "ford_e350", "120": "ford_edge", "121": "ford_escape", "122": "ford_escort", "123": "ford_excursion", "124": "ford_expedition", "125": "ford_explorer", "126": "ford_f100", "127": "ford_f150", "128": "ford_f250", "129": "ford_f350", "130": "ford_f450", "131": "ford_fiesta", "132": "ford_five hundred", "133": "ford_focus", "134": "ford_freestar", "135": "ford_fusion", "136": "ford_mustang", "137": "ford_ranger", "138": "ford_taurus", "139": "ford_thunderbird", "140": "ford_windstar", "141": "gmc_acadia", "142": "gmc_canyon", "143": "gmc_envoy", "144": "gmc_jimmy", "145": "gmc_sierra", "146": "gmc_sonoma", "147": "gmc_suburban", "148": "gmc_terrain", "149": "gmc_yukon", "150": "honda_accord", "151": "honda_civic", "152": "honda_cr-v", "153": "honda_delsol", "154": "honda_element", "155": "honda_fit", "156": "honda_odyssey", "157": "honda_passport", "158": "honda_pilot", "159": "honda_prelude", "160": "honda_ridgeline", "161": "honda_s2000", "162": "hummer_h2", "163": "hummer_h3", "164": "hyundai_accent", "165": "hyundai_azera", "166": "hyundai_elantra", "167": "hyundai_genesis", "168": "hyundai_santafe", "169": "hyundai_sonata", "170": "hyundai_tiburon", "171": "hyundai_tucson", "172": "infiniti_fx35", "173": "infiniti_g35", "174": "infiniti_g37", "175": "infiniti_i30", "176": "infiniti_i35", "177": "infiniti_m35", "178": "infiniti_q45", "179": "infiniti_qx4", "180": "infiniti_qx56", "181": "isuzu_rodeo", "182": "isuzu_trooper", "183": "jaguar_s-type", "184": "jaguar_x-type", "185": "jaguar_xj", "186": "jeep_cherokee", "187": "jeep_cj5", "188": "jeep_cj7", "189": "jeep_commander", "190": "jeep_compass", "191": "jeep_grand", "192": "jeep_liberty", "193": "jeep_patriot", "194": "jeep_wrangler", "195": "kia_amanti", "196": "kia_forte", "197": "kia_optima", "198": "kia_rio", "199": "kia_sedona", "200": "kia_sephia", "201": "kia_sorento", "202": "kia_soul", "203": "kia_spectra", "204": "kia_sportage", "205": "landrover_discovery", "206": "landrover_rangerover", "207": "lexus_es300", "208": "lexus_es330", "209": "lexus_es350", "210": "lexus_gs300", "211": "lexus_gx470", "212": "lexus_is250", "213": "lexus_is300", "214": "lexus_is350", "215": "lexus_ls400", "216": "lexus_ls430", "217": "lexus_rx300", "218": "lexus_rx330", "219": "lexus_sc430", "220": "lincoln_aviator", "221": "lincoln_continental", "222": "lincoln_ls", "223": "lincoln_mark", "224": "lincoln_mkx", "225": "lincoln_mkz", "226": "lincoln_navigator", "227": "lincoln_towncar", "228": "mazda_3", "229": "mazda_5", "230": "mazda_6", "231": "mazda_626", "232": "mazda_millenia", "233": "mazda_mpv", "234": "mazda_mx5", "235": "mazda_protege", "236": "mazda_rx7", "237": "mazda_rx8", "238": "mazda_tribute", "239": "mercedes benz_c230", "240": "mercedes benz_c240", "241": "mercedes benz_c280", "242": "mercedes benz_c300", "243": "mercedes benz_c320", "244": "mercedes benz_clk320", "245": "mercedes benz_e320", "246": "mercedes benz_e350", "247": "mercedes benz_e500", "248": "mercedes benz_ml320", "249": "mercedes benz_ml350", "250": "mercedes benz_ml500", "251": "mercedes benz_s430", "252": "mercedes benz_s500", "253": "mercedes benz_s550", "254": "mercedes benz_sl500", "255": "mercury_cougar", "256": "mercury_grandmarquis", "257": "mercury_mariner", "258": "mercury_milan", "259": "mercury_mountaineer", "260": "mercury_sable", "261": "mercury_villager", "262": "mini_cooper", "263": "mitsubishi_3000gt", "264": "mitsubishi_eclipse", "265": "mitsubishi_endeavor", "266": "mitsubishi_galant", "267": "mitsubishi_lancer", "268": "mitsubishi_mirage", "269": "mitsubishi_montero", "270": "mitsubishi_outlander", "271": "nissan_240sx", "272": "nissan_300zx", "273": "nissan_350z", "274": "nissan_altima", "275": "nissan_armada", "276": "nissan_frontier", "277": "nissan_maxima", "278": "nissan_murano", "279": "nissan_pathfinder", "280": "nissan_quest", "281": "nissan_rogue", "282": "nissan_sentra", "283": "nissan_titan", "284": "nissan_versa", "285": "nissan_xterra", "286": "oldsmobile_alero", "287": "oldsmobile_aurora", "288": "oldsmobile_bravada", "289": "oldsmobile_cutlass", "290": "oldsmobile_intrigue", "291": "oldsmobile_silhouette", "292": "plymouth_neon", "293": "plymouth_voyager", "294": "pontiac_bonneville", "295": "pontiac_firebird", "296": "pontiac_g5", "297": "pontiac_g6", "298": "pontiac_grandam", "299": "pontiac_grandprix", "300": "pontiac_gto", "301": "pontiac_montana", "302": "pontiac_sunfire", "303": "pontiac_torrent", "304": "pontiac_transam", "305": "pontiac_vibe", "306": "porsche_911", "307": "porsche_boxster", "308": "porsche_cayenne", "309": "ram_1500", "310": "saab_9-3", "311": "saab_9-5", "312": "saturn_aura", "313": "saturn_ion", "314": "saturn_l200", "315": "saturn_l300", "316": "saturn_sl1", "317": "saturn_sl2", "318": "saturn_vue", "319": "scion_tc", "320": "scion_xa", "321": "scion_xb", "322": "scion_xd", "323": "smart_fortwo", "324": "subaru_forester", "325": "subaru_impreza", "326": "subaru_legacy", "327": "subaru_outback", "328": "subaru_wrx", "329": "suzuki_forenza", "330": "suzuki_sx4", "331": "suzuki_xl7", "332": "toyota_4runner", "333": "toyota_avalon", "334": "toyota_camry", "335": "toyota_celica", "336": "toyota_corolla", "337": "toyota_echo", "338": "toyota_fjcruiser", "339": "toyota_highlander", "340": "toyota_landcruiser", "341": "toyota_matrix", "342": "toyota_mr2", "343": "toyota_pickup", "344": "toyota_prius", "345": "toyota_rav4", "346": "toyota_sequoia", "347": "toyota_sienna", "348": "toyota_solara", "349": "toyota_supra", "350": "toyota_t100", "351": "toyota_tacoma", "352": "toyota_tercel", "353": "toyota_tundra", "354": "toyota_yaris", "355": "volkswagen_beetle", "356": "volkswagen_bug", "357": "volkswagen_cc", "358": "volkswagen_eos", "359": "volkswagen_golf", "360": "volkswagen_gti", "361": "volkswagen_jetta", "362": "volkswagen_newbeetle", "363": "volkswagen_passat", "364": "volkswagen_rabbit", "365": "volkswagen_touareg", "366": "volvo_850", "367": "volvo_c70", "368": "volvo_s40", "369": "volvo_s60", "370": "volvo_s70", "371": "volvo_s80", "372": "volvo_v70", "373": "volvo_xc70", "374": "volvo_xc90"}}}}], "splits": [{"name": "train", "num_bytes": 498938159.51709396, "num_examples": 26852}], "download_size": 498718383, "dataset_size": 498938159.51709396}} | 2022-11-24T04:03:01+00:00 | [] | [] | TAGS
#region-us
| # Dataset Card for "VMMRdb_make_model_test"
More Information needed | [
"# Dataset Card for \"VMMRdb_make_model_test\"\n\nMore Information needed"
] | [
"TAGS\n#region-us \n",
"# Dataset Card for \"VMMRdb_make_model_test\"\n\nMore Information needed"
] | [
6,
19
] | [
"passage: TAGS\n#region-us \n# Dataset Card for \"VMMRdb_make_model_test\"\n\nMore Information needed"
] |
9830e299776fffa4e820f0be310f3dcea14a4fcf | Used Rob's model: ChaiML/opt_6.7b_dalio_training_topic_epoch_20_lr_3e-7 to generate 100 quality question/answer pairs that are scored.
MSE of random guess is 0.245
MSE of mean is ~0.09
MSE of TR hand scoring is 0.05 | Jellywibble/100_qa_scored_pairs | [
"region:us"
] | 2022-11-22T01:07:44+00:00 | {} | 2022-11-22T19:58:50+00:00 | [] | [] | TAGS
#region-us
| Used Rob's model: ChaiML/opt_6.7b_dalio_training_topic_epoch_20_lr_3e-7 to generate 100 quality question/answer pairs that are scored.
MSE of random guess is 0.245
MSE of mean is ~0.09
MSE of TR hand scoring is 0.05 | [] | [
"TAGS\n#region-us \n"
] | [
6
] | [
"passage: TAGS\n#region-us \n"
] |
a1068d0abd8477a0ab7674740c23c2bb1f02341a |
Preprocessed training and evaluation data from KiloGram.
KiloGram dataset and code repo: https://github.com/lil-lab/kilogram
---
# File Formats
## Training Set
Texts: `train_*.json` are all in the format of `{tangramName: list(annotations)}`.
Images: Colored images with parts (under `/color`) are named in the format of `tangramName_{idx}.png`, where `idx` corresponds to the index of the annotation in the text file.
## Validation, Development, Heldout Set
Texts: `{whole, part}_{black, color}.json` are in the format of `{"targets": list(imageFileNames), "images": list(imageFileNames), "texts": list(annotations)}`. We flattened all the contexts and concatenated them into one list for each entry.
E.g. the first 10 elements in `"targets"` are the image file name of the target of the first context repeated 10 times; the first 10 of `"images"` are the image file names in that context; and the first 10 of `"texts"` are the corresponding 10 annotations in that context.
`/controlled` contains experiments with constrained contexts controlled for number of parts, and `/random` contains ones without. (See Appendix A.8 in paper)
`/development/texts/augmented/aug_dev.json` and `images/augmented.tar.bz2` are experiments in the same format as above used to evaluate the effect of adding part information.
Intermediate files:
`*/text/controlled/eval_batch_data.json` are in the format of
`{tangramName: {numOfParts: list({"target": [tangramName_{idx}, annotation], "distractors": list(list([tangramName_{idx}, annotation]))})}}`, used to generate controlled experiment jsons. Note: annotations are descriptions concatenated by "#" instead of in natural English. | lil-lab/kilogram | [
"annotations_creators:crowdsourced",
"multilinguality:monolingual",
"size_categories:1K<n<10K",
"source_datasets:original",
"language:en",
"tangrams",
"reference-games",
"vision-language",
"region:us"
] | 2022-11-22T03:27:12+00:00 | {"annotations_creators": ["crowdsourced"], "language": ["en"], "multilinguality": ["monolingual"], "size_categories": ["1K<n<10K"], "source_datasets": ["original"], "pretty_name": "KiloGram", "tags": ["tangrams", "reference-games", "vision-language"], "viewer": false} | 2023-08-24T21:05:28+00:00 | [] | [
"en"
] | TAGS
#annotations_creators-crowdsourced #multilinguality-monolingual #size_categories-1K<n<10K #source_datasets-original #language-English #tangrams #reference-games #vision-language #region-us
|
Preprocessed training and evaluation data from KiloGram.
KiloGram dataset and code repo: URL
---
# File Formats
## Training Set
Texts: 'train_*.json' are all in the format of '{tangramName: list(annotations)}'.
Images: Colored images with parts (under '/color') are named in the format of 'tangramName_{idx}.png', where 'idx' corresponds to the index of the annotation in the text file.
## Validation, Development, Heldout Set
Texts: '{whole, part}_{black, color}.json' are in the format of '{"targets": list(imageFileNames), "images": list(imageFileNames), "texts": list(annotations)}'. We flattened all the contexts and concatenated them into one list for each entry.
E.g. the first 10 elements in '"targets"' are the image file name of the target of the first context repeated 10 times; the first 10 of '"images"' are the image file names in that context; and the first 10 of '"texts"' are the corresponding 10 annotations in that context.
'/controlled' contains experiments with constrained contexts controlled for number of parts, and '/random' contains ones without. (See Appendix A.8 in paper)
'/development/texts/augmented/aug_dev.json' and 'images/URL.bz2' are experiments in the same format as above used to evaluate the effect of adding part information.
Intermediate files:
'*/text/controlled/eval_batch_data.json' are in the format of
'{tangramName: {numOfParts: list({"target": [tangramName_{idx}, annotation], "distractors": list(list([tangramName_{idx}, annotation]))})}}', used to generate controlled experiment jsons. Note: annotations are descriptions concatenated by "#" instead of in natural English. | [
"# File Formats",
"## Training Set\nTexts: 'train_*.json' are all in the format of '{tangramName: list(annotations)}'.\n\nImages: Colored images with parts (under '/color') are named in the format of 'tangramName_{idx}.png', where 'idx' corresponds to the index of the annotation in the text file.",
"## Validation, Development, Heldout Set\n\nTexts: '{whole, part}_{black, color}.json' are in the format of '{\"targets\": list(imageFileNames), \"images\": list(imageFileNames), \"texts\": list(annotations)}'. We flattened all the contexts and concatenated them into one list for each entry. \n\nE.g. the first 10 elements in '\"targets\"' are the image file name of the target of the first context repeated 10 times; the first 10 of '\"images\"' are the image file names in that context; and the first 10 of '\"texts\"' are the corresponding 10 annotations in that context.\n\n'/controlled' contains experiments with constrained contexts controlled for number of parts, and '/random' contains ones without. (See Appendix A.8 in paper)\n\n'/development/texts/augmented/aug_dev.json' and 'images/URL.bz2' are experiments in the same format as above used to evaluate the effect of adding part information. \n\n\nIntermediate files:\n\n'*/text/controlled/eval_batch_data.json' are in the format of \n'{tangramName: {numOfParts: list({\"target\": [tangramName_{idx}, annotation], \"distractors\": list(list([tangramName_{idx}, annotation]))})}}', used to generate controlled experiment jsons. Note: annotations are descriptions concatenated by \"#\" instead of in natural English."
] | [
"TAGS\n#annotations_creators-crowdsourced #multilinguality-monolingual #size_categories-1K<n<10K #source_datasets-original #language-English #tangrams #reference-games #vision-language #region-us \n",
"# File Formats",
"## Training Set\nTexts: 'train_*.json' are all in the format of '{tangramName: list(annotations)}'.\n\nImages: Colored images with parts (under '/color') are named in the format of 'tangramName_{idx}.png', where 'idx' corresponds to the index of the annotation in the text file.",
"## Validation, Development, Heldout Set\n\nTexts: '{whole, part}_{black, color}.json' are in the format of '{\"targets\": list(imageFileNames), \"images\": list(imageFileNames), \"texts\": list(annotations)}'. We flattened all the contexts and concatenated them into one list for each entry. \n\nE.g. the first 10 elements in '\"targets\"' are the image file name of the target of the first context repeated 10 times; the first 10 of '\"images\"' are the image file names in that context; and the first 10 of '\"texts\"' are the corresponding 10 annotations in that context.\n\n'/controlled' contains experiments with constrained contexts controlled for number of parts, and '/random' contains ones without. (See Appendix A.8 in paper)\n\n'/development/texts/augmented/aug_dev.json' and 'images/URL.bz2' are experiments in the same format as above used to evaluate the effect of adding part information. \n\n\nIntermediate files:\n\n'*/text/controlled/eval_batch_data.json' are in the format of \n'{tangramName: {numOfParts: list({\"target\": [tangramName_{idx}, annotation], \"distractors\": list(list([tangramName_{idx}, annotation]))})}}', used to generate controlled experiment jsons. Note: annotations are descriptions concatenated by \"#\" instead of in natural English."
] | [
63,
4,
89,
391
] | [
"passage: TAGS\n#annotations_creators-crowdsourced #multilinguality-monolingual #size_categories-1K<n<10K #source_datasets-original #language-English #tangrams #reference-games #vision-language #region-us \n# File Formats## Training Set\nTexts: 'train_*.json' are all in the format of '{tangramName: list(annotations)}'.\n\nImages: Colored images with parts (under '/color') are named in the format of 'tangramName_{idx}.png', where 'idx' corresponds to the index of the annotation in the text file."
] |
f236a4e89fe6d8064fe89715a3566fee97f0c51a | # AutoTrain Dataset for project: model_me
## Dataset Description
This dataset has been automatically processed by AutoTrain for project model_me.
### Languages
The BCP-47 code for the dataset's language is unk.
## Dataset Structure
### Data Instances
A sample from this dataset looks as follows:
```json
[
{
"image": "<600x1100 RGB PIL image>",
"target": 7
},
{
"image": "<1000x750 RGBA PIL image>",
"target": 0
}
]
```
### Dataset Fields
The dataset has the following fields (also called "features"):
```json
{
"image": "Image(decode=True, id=None)",
"target": "ClassLabel(num_classes=8, names=['3d', 'above', 'front', 'game', 'ilustration', 'inside', 'object', 'pixel art'], id=None)"
}
```
### Dataset Splits
This dataset is split into a train and validation split. The split sizes are as follow:
| Split name | Num samples |
| ------------ | ------------------- |
| train | 468 |
| valid | 121 |
| antonmk/ispmetric | [
"task_categories:image-classification",
"region:us"
] | 2022-11-22T04:10:25+00:00 | {"task_categories": ["image-classification"]} | 2022-11-22T18:38:26+00:00 | [] | [] | TAGS
#task_categories-image-classification #region-us
| AutoTrain Dataset for project: model\_me
========================================
Dataset Description
-------------------
This dataset has been automatically processed by AutoTrain for project model\_me.
### Languages
The BCP-47 code for the dataset's language is unk.
Dataset Structure
-----------------
### Data Instances
A sample from this dataset looks as follows:
### Dataset Fields
The dataset has the following fields (also called "features"):
### Dataset Splits
This dataset is split into a train and validation split. The split sizes are as follow:
| [
"### Languages\n\n\nThe BCP-47 code for the dataset's language is unk.\n\n\nDataset Structure\n-----------------",
"### Data Instances\n\n\nA sample from this dataset looks as follows:",
"### Dataset Fields\n\n\nThe dataset has the following fields (also called \"features\"):",
"### Dataset Splits\n\n\nThis dataset is split into a train and validation split. The split sizes are as follow:"
] | [
"TAGS\n#task_categories-image-classification #region-us \n",
"### Languages\n\n\nThe BCP-47 code for the dataset's language is unk.\n\n\nDataset Structure\n-----------------",
"### Data Instances\n\n\nA sample from this dataset looks as follows:",
"### Dataset Fields\n\n\nThe dataset has the following fields (also called \"features\"):",
"### Dataset Splits\n\n\nThis dataset is split into a train and validation split. The split sizes are as follow:"
] | [
17,
27,
17,
23,
27
] | [
"passage: TAGS\n#task_categories-image-classification #region-us \n### Languages\n\n\nThe BCP-47 code for the dataset's language is unk.\n\n\nDataset Structure\n-----------------### Data Instances\n\n\nA sample from this dataset looks as follows:### Dataset Fields\n\n\nThe dataset has the following fields (also called \"features\"):### Dataset Splits\n\n\nThis dataset is split into a train and validation split. The split sizes are as follow:"
] |
e2c31169b37016ec8f336a62450817424f7bf97e | # Dataset Card for "libri_augmented_train_set"
[More Information needed](https://github.com/huggingface/datasets/blob/main/CONTRIBUTING.md#how-to-contribute-to-the-dataset-cards) | DTU54DL/libri_augmented_train_set | [
"region:us"
] | 2022-11-22T05:49:10+00:00 | {"dataset_info": {"features": [{"name": "file", "dtype": "string"}, {"name": "audio", "dtype": {"audio": {"sampling_rate": 16000}}}, {"name": "text", "dtype": "string"}, {"name": "speaker_id", "dtype": "int64"}, {"name": "chapter_id", "dtype": "int64"}, {"name": "id", "dtype": "string"}], "splits": [{"name": "train.360", "num_bytes": 41931835349.25, "num_examples": 104014}], "download_size": 0, "dataset_size": 41931835349.25}} | 2022-11-22T18:34:11+00:00 | [] | [] | TAGS
#region-us
| # Dataset Card for "libri_augmented_train_set"
More Information needed | [
"# Dataset Card for \"libri_augmented_train_set\"\n\nMore Information needed"
] | [
"TAGS\n#region-us \n",
"# Dataset Card for \"libri_augmented_train_set\"\n\nMore Information needed"
] | [
6,
20
] | [
"passage: TAGS\n#region-us \n# Dataset Card for \"libri_augmented_train_set\"\n\nMore Information needed"
] |
eade7b0caec19ea0bb754db386200359978235ad |
# Dataset Card for [Dataset Name]
## Table of Contents
- [Table of Contents](#table-of-contents)
- [Dataset Description](#dataset-description)
- [Dataset Summary](#dataset-summary)
- [Supported Tasks and Leaderboards](#supported-tasks-and-leaderboards)
- [Languages](#languages)
- [Dataset Structure](#dataset-structure)
- [Data Instances](#data-instances)
- [Data Fields](#data-fields)
- [Data Splits](#data-splits)
- [Dataset Creation](#dataset-creation)
- [Curation Rationale](#curation-rationale)
- [Source Data](#source-data)
- [Annotations](#annotations)
- [Personal and Sensitive Information](#personal-and-sensitive-information)
- [Considerations for Using the Data](#considerations-for-using-the-data)
- [Social Impact of Dataset](#social-impact-of-dataset)
- [Discussion of Biases](#discussion-of-biases)
- [Other Known Limitations](#other-known-limitations)
- [Additional Information](#additional-information)
- [Dataset Curators](#dataset-curators)
- [Licensing Information](#licensing-information)
- [Citation Information](#citation-information)
- [Contributions](#contributions)
## Dataset Description
- **Homepage:**
- **Repository:**
- **Paper:**
- **Leaderboard:**
- **Point of Contact:**
### Dataset Summary
[More Information Needed]
### Supported Tasks and Leaderboards
[More Information Needed]
### Languages
[More Information Needed]
## Dataset Structure
### Data Instances
[More Information Needed]
### Data Fields
[More Information Needed]
### Data Splits
[More Information Needed]
## Dataset Creation
### Curation Rationale
[More Information Needed]
### Source Data
#### Initial Data Collection and Normalization
[More Information Needed]
#### Who are the source language producers?
[More Information Needed]
### Annotations
#### Annotation process
[More Information Needed]
#### Who are the annotators?
[More Information Needed]
### Personal and Sensitive Information
[More Information Needed]
## Considerations for Using the Data
### Social Impact of Dataset
[More Information Needed]
### Discussion of Biases
[More Information Needed]
### Other Known Limitations
[More Information Needed]
## Additional Information
### Dataset Curators
[More Information Needed]
### Licensing Information
[More Information Needed]
### Citation Information
[More Information Needed]
### Contributions
Thanks to [@github-username](https://github.com/<github-username>) for adding this dataset.
| DTU54DL/dmeo | [
"task_categories:token-classification",
"annotations_creators:expert-generated",
"language_creators:found",
"multilinguality:monolingual",
"size_categories:10K<n<100K",
"source_datasets:original",
"language:en",
"license:mit",
"region:us"
] | 2022-11-22T06:47:01+00:00 | {"annotations_creators": ["expert-generated"], "language_creators": ["found"], "language": ["en"], "license": ["mit"], "multilinguality": ["monolingual"], "size_categories": ["10K<n<100K"], "source_datasets": ["original"], "task_categories": ["token-classification"], "task_ids": ["token-classification-other-acronym-identification"], "paperswithcode_id": "acronym-identification", "pretty_name": "Acronym Identification Dataset", "train-eval-index": [{"col_mapping": {"labels": "tags", "tokens": "tokens"}, "config": "default", "splits": {"eval_split": "test"}, "task": "token-classification", "task_id": "entity_extraction"}]} | 2022-11-22T08:32:22+00:00 | [] | [
"en"
] | TAGS
#task_categories-token-classification #annotations_creators-expert-generated #language_creators-found #multilinguality-monolingual #size_categories-10K<n<100K #source_datasets-original #language-English #license-mit #region-us
|
# Dataset Card for [Dataset Name]
## Table of Contents
- Table of Contents
- Dataset Description
- Dataset Summary
- Supported Tasks and Leaderboards
- Languages
- Dataset Structure
- Data Instances
- Data Fields
- Data Splits
- Dataset Creation
- Curation Rationale
- Source Data
- Annotations
- Personal and Sensitive Information
- Considerations for Using the Data
- Social Impact of Dataset
- Discussion of Biases
- Other Known Limitations
- Additional Information
- Dataset Curators
- Licensing Information
- Citation Information
- Contributions
## Dataset Description
- Homepage:
- Repository:
- Paper:
- Leaderboard:
- Point of Contact:
### Dataset Summary
### Supported Tasks and Leaderboards
### Languages
## Dataset Structure
### Data Instances
### Data Fields
### Data Splits
## Dataset Creation
### Curation Rationale
### Source Data
#### Initial Data Collection and Normalization
#### Who are the source language producers?
### Annotations
#### Annotation process
#### Who are the annotators?
### Personal and Sensitive Information
## Considerations for Using the Data
### Social Impact of Dataset
### Discussion of Biases
### Other Known Limitations
## Additional Information
### Dataset Curators
### Licensing Information
### Contributions
Thanks to @github-username for adding this dataset.
| [
"# Dataset Card for [Dataset Name]",
"## Table of Contents\n- Table of Contents\n- Dataset Description\n - Dataset Summary\n - Supported Tasks and Leaderboards\n - Languages\n- Dataset Structure\n - Data Instances\n - Data Fields\n - Data Splits\n- Dataset Creation\n - Curation Rationale\n - Source Data\n - Annotations\n - Personal and Sensitive Information\n- Considerations for Using the Data\n - Social Impact of Dataset\n - Discussion of Biases\n - Other Known Limitations\n- Additional Information\n - Dataset Curators\n - Licensing Information\n - Citation Information\n - Contributions",
"## Dataset Description\n\n- Homepage:\n- Repository:\n- Paper:\n- Leaderboard:\n- Point of Contact:",
"### Dataset Summary",
"### Supported Tasks and Leaderboards",
"### Languages",
"## Dataset Structure",
"### Data Instances",
"### Data Fields",
"### Data Splits",
"## Dataset Creation",
"### Curation Rationale",
"### Source Data",
"#### Initial Data Collection and Normalization",
"#### Who are the source language producers?",
"### Annotations",
"#### Annotation process",
"#### Who are the annotators?",
"### Personal and Sensitive Information",
"## Considerations for Using the Data",
"### Social Impact of Dataset",
"### Discussion of Biases",
"### Other Known Limitations",
"## Additional Information",
"### Dataset Curators",
"### Licensing Information",
"### Contributions\n\nThanks to @github-username for adding this dataset."
] | [
"TAGS\n#task_categories-token-classification #annotations_creators-expert-generated #language_creators-found #multilinguality-monolingual #size_categories-10K<n<100K #source_datasets-original #language-English #license-mit #region-us \n",
"# Dataset Card for [Dataset Name]",
"## Table of Contents\n- Table of Contents\n- Dataset Description\n - Dataset Summary\n - Supported Tasks and Leaderboards\n - Languages\n- Dataset Structure\n - Data Instances\n - Data Fields\n - Data Splits\n- Dataset Creation\n - Curation Rationale\n - Source Data\n - Annotations\n - Personal and Sensitive Information\n- Considerations for Using the Data\n - Social Impact of Dataset\n - Discussion of Biases\n - Other Known Limitations\n- Additional Information\n - Dataset Curators\n - Licensing Information\n - Citation Information\n - Contributions",
"## Dataset Description\n\n- Homepage:\n- Repository:\n- Paper:\n- Leaderboard:\n- Point of Contact:",
"### Dataset Summary",
"### Supported Tasks and Leaderboards",
"### Languages",
"## Dataset Structure",
"### Data Instances",
"### Data Fields",
"### Data Splits",
"## Dataset Creation",
"### Curation Rationale",
"### Source Data",
"#### Initial Data Collection and Normalization",
"#### Who are the source language producers?",
"### Annotations",
"#### Annotation process",
"#### Who are the annotators?",
"### Personal and Sensitive Information",
"## Considerations for Using the Data",
"### Social Impact of Dataset",
"### Discussion of Biases",
"### Other Known Limitations",
"## Additional Information",
"### Dataset Curators",
"### Licensing Information",
"### Contributions\n\nThanks to @github-username for adding this dataset."
] | [
76,
10,
125,
24,
6,
10,
4,
6,
6,
5,
5,
5,
7,
4,
10,
10,
5,
5,
9,
8,
8,
7,
8,
7,
5,
6,
6,
19
] | [
"passage: TAGS\n#task_categories-token-classification #annotations_creators-expert-generated #language_creators-found #multilinguality-monolingual #size_categories-10K<n<100K #source_datasets-original #language-English #license-mit #region-us \n# Dataset Card for [Dataset Name]## Table of Contents\n- Table of Contents\n- Dataset Description\n - Dataset Summary\n - Supported Tasks and Leaderboards\n - Languages\n- Dataset Structure\n - Data Instances\n - Data Fields\n - Data Splits\n- Dataset Creation\n - Curation Rationale\n - Source Data\n - Annotations\n - Personal and Sensitive Information\n- Considerations for Using the Data\n - Social Impact of Dataset\n - Discussion of Biases\n - Other Known Limitations\n- Additional Information\n - Dataset Curators\n - Licensing Information\n - Citation Information\n - Contributions## Dataset Description\n\n- Homepage:\n- Repository:\n- Paper:\n- Leaderboard:\n- Point of Contact:### Dataset Summary### Supported Tasks and Leaderboards### Languages## Dataset Structure### Data Instances### Data Fields### Data Splits## Dataset Creation### Curation Rationale### Source Data#### Initial Data Collection and Normalization#### Who are the source language producers?### Annotations#### Annotation process#### Who are the annotators?### Personal and Sensitive Information## Considerations for Using the Data### Social Impact of Dataset### Discussion of Biases### Other Known Limitations## Additional Information### Dataset Curators### Licensing Information### Contributions\n\nThanks to @github-username for adding this dataset."
] |
33884d1f17bf4c89fba320c7878681630c381d53 |
# Dataset Card for HyperRED
## Description
- **Repository:** https://github.com/declare-lab/HyperRED
- **Paper (EMNLP 2022):** https://arxiv.org/abs/2211.10018
### Summary
HyperRED is a dataset for the new task of hyper-relational extraction, which extracts relation triplets together with qualifier information such as time, quantity or location. For example, the relation triplet (Leonard Parker, Educated At, Harvard University) can be factually enriched by including the qualifier (End Time, 1967). HyperRED contains 44k sentences with 62 relation types and 44 qualifier types.
### Languages
English.
## Dataset Structure
### Data Fields
- **tokens:** Sentence text tokens.
- **entities:** List of each entity span. The span indices correspond to each token in the space-separated text (inclusive-start and exclusive-end index)
- **relations:** List of each relationship label between the head and tail entity spans. Each relation contains a list of qualifiers where each qualifier has the value entity span and qualifier label.
### Data Instances
An example instance of the dataset is shown below:
```
{
"tokens": ['Acadia', 'University', 'is', 'a', 'predominantly', 'undergraduate', 'university', 'located', 'in', 'Wolfville', ',', 'Nova', 'Scotia', ',', 'Canada', 'with', 'some', 'graduate', 'programs', 'at', 'the', 'master', "'", 's', 'level', 'and', 'one', 'at', 'the', 'doctoral', 'level', '.'],
"entities": [
{'span': (0, 2), 'label': 'Entity'},
{'span': (9, 13), 'label': 'Entity'},
{'span': (14, 15), 'label': 'Entity'},
],
"relations": [
{
"head": [0, 2],
"tail": [9, 13],
"label": "headquarters location",
"qualifiers": [
{"span": [14, 15], "label": "country"}
]
}
],
}
```
### Data Splits
The dataset contains 39,840 instances for training, 1,000 instances for validation and 4,000 instances for testing.
### Dataset Creation
The dataset is constructed from distant supervision between Wikipedia and Wikidata, and the human annotation process is detailed in the paper.
## Citation Information
```
@inproceedings{chia2022hyperred,
title={A Dataset for Hyper-Relational Extraction and a Cube-Filling Approach},
author={Yew Ken Chia, Lidong Bing, Sharifah Mahani Aljunied, Luo Si and Soujanya Poria},
booktitle={Proceedings of the 2022 Conference on Empirical Methods in Natural Language Processing},
year={2022}
}
``` | declare-lab/HyperRED | [
"license:cc-by-sa-3.0",
"arxiv:2211.10018",
"region:us"
] | 2022-11-22T07:46:53+00:00 | {"license": "cc-by-sa-3.0"} | 2022-11-23T10:55:14+00:00 | [
"2211.10018"
] | [] | TAGS
#license-cc-by-sa-3.0 #arxiv-2211.10018 #region-us
|
# Dataset Card for HyperRED
## Description
- Repository: URL
- Paper (EMNLP 2022): URL
### Summary
HyperRED is a dataset for the new task of hyper-relational extraction, which extracts relation triplets together with qualifier information such as time, quantity or location. For example, the relation triplet (Leonard Parker, Educated At, Harvard University) can be factually enriched by including the qualifier (End Time, 1967). HyperRED contains 44k sentences with 62 relation types and 44 qualifier types.
### Languages
English.
## Dataset Structure
### Data Fields
- tokens: Sentence text tokens.
- entities: List of each entity span. The span indices correspond to each token in the space-separated text (inclusive-start and exclusive-end index)
- relations: List of each relationship label between the head and tail entity spans. Each relation contains a list of qualifiers where each qualifier has the value entity span and qualifier label.
### Data Instances
An example instance of the dataset is shown below:
### Data Splits
The dataset contains 39,840 instances for training, 1,000 instances for validation and 4,000 instances for testing.
### Dataset Creation
The dataset is constructed from distant supervision between Wikipedia and Wikidata, and the human annotation process is detailed in the paper.
| [
"# Dataset Card for HyperRED",
"## Description\n\n- Repository: URL\n- Paper (EMNLP 2022): URL",
"### Summary\n\nHyperRED is a dataset for the new task of hyper-relational extraction, which extracts relation triplets together with qualifier information such as time, quantity or location. For example, the relation triplet (Leonard Parker, Educated At, Harvard University) can be factually enriched by including the qualifier (End Time, 1967). HyperRED contains 44k sentences with 62 relation types and 44 qualifier types.",
"### Languages\n\nEnglish.",
"## Dataset Structure",
"### Data Fields\n\n- tokens: Sentence text tokens.\n- entities: List of each entity span. The span indices correspond to each token in the space-separated text (inclusive-start and exclusive-end index)\n- relations: List of each relationship label between the head and tail entity spans. Each relation contains a list of qualifiers where each qualifier has the value entity span and qualifier label.",
"### Data Instances\n\nAn example instance of the dataset is shown below:",
"### Data Splits\n\nThe dataset contains 39,840 instances for training, 1,000 instances for validation and 4,000 instances for testing.",
"### Dataset Creation\n\nThe dataset is constructed from distant supervision between Wikipedia and Wikidata, and the human annotation process is detailed in the paper."
] | [
"TAGS\n#license-cc-by-sa-3.0 #arxiv-2211.10018 #region-us \n",
"# Dataset Card for HyperRED",
"## Description\n\n- Repository: URL\n- Paper (EMNLP 2022): URL",
"### Summary\n\nHyperRED is a dataset for the new task of hyper-relational extraction, which extracts relation triplets together with qualifier information such as time, quantity or location. For example, the relation triplet (Leonard Parker, Educated At, Harvard University) can be factually enriched by including the qualifier (End Time, 1967). HyperRED contains 44k sentences with 62 relation types and 44 qualifier types.",
"### Languages\n\nEnglish.",
"## Dataset Structure",
"### Data Fields\n\n- tokens: Sentence text tokens.\n- entities: List of each entity span. The span indices correspond to each token in the space-separated text (inclusive-start and exclusive-end index)\n- relations: List of each relationship label between the head and tail entity spans. Each relation contains a list of qualifiers where each qualifier has the value entity span and qualifier label.",
"### Data Instances\n\nAn example instance of the dataset is shown below:",
"### Data Splits\n\nThe dataset contains 39,840 instances for training, 1,000 instances for validation and 4,000 instances for testing.",
"### Dataset Creation\n\nThe dataset is constructed from distant supervision between Wikipedia and Wikidata, and the human annotation process is detailed in the paper."
] | [
26,
7,
17,
99,
6,
6,
98,
17,
32,
34
] | [
"passage: TAGS\n#license-cc-by-sa-3.0 #arxiv-2211.10018 #region-us \n# Dataset Card for HyperRED## Description\n\n- Repository: URL\n- Paper (EMNLP 2022): URL### Summary\n\nHyperRED is a dataset for the new task of hyper-relational extraction, which extracts relation triplets together with qualifier information such as time, quantity or location. For example, the relation triplet (Leonard Parker, Educated At, Harvard University) can be factually enriched by including the qualifier (End Time, 1967). HyperRED contains 44k sentences with 62 relation types and 44 qualifier types.### Languages\n\nEnglish.## Dataset Structure### Data Fields\n\n- tokens: Sentence text tokens.\n- entities: List of each entity span. The span indices correspond to each token in the space-separated text (inclusive-start and exclusive-end index)\n- relations: List of each relationship label between the head and tail entity spans. Each relation contains a list of qualifiers where each qualifier has the value entity span and qualifier label.### Data Instances\n\nAn example instance of the dataset is shown below:### Data Splits\n\nThe dataset contains 39,840 instances for training, 1,000 instances for validation and 4,000 instances for testing.### Dataset Creation\n\nThe dataset is constructed from distant supervision between Wikipedia and Wikidata, and the human annotation process is detailed in the paper."
] |
02d8400506fe656bfd6016d3a8424e33f1b28916 | # 청와대 국민청원
데이터 출처: https://github.com/lovit/petitions_archive<br/>
크기: 651.8MB
sample
```
{
"category": "반려동물",
"begin": "2017-08-25",
"end": "2017-11-23",
"content": "길고양이들 밥주고있는 사람입니다. 최근에 동네주민과 트러블이 생겨 싸움이 일어났습니다. 길고양이들이 모여든다고 밥주지마라고 윽박지르셨습니다. 쓰레기봉투를 뜯는다거나 사람에게 해끼치거나 하지 않았습니다. 단순히 고양이가 모여드는게 싫답니다. 그럼 애들은 굶어죽어야하나요? 길고양이들이 맘놓고 쉬고 밥먹을 수 있는 환경이 전혀 없는데 무작정 밥안주고 물 안주면 얘네는 어떻게 하나요? 안그래도 수명도 짧은데다가 길고양이를 상대로 학대하는 사람들도 많은데 너무 가엾습니다. 강동구청은 고양이 급식소라고 만들어주셨던데 동네마다 한개씩이라도 만들어 주셨으면좋겠어요.. 밥에다가 이상한짓 하는 사람 있을 수 있으니까 cctv도 설치도 해주셨으면 합니다.. (급식소에 쥐약을 뿌려 고양이가 죽은 사례가 있습니다) 지구가 사람껀 아니잖아요 동물과도 더불어 살줄 알아야죠 문대통령님께서 동물복지 관련 공략을 내셨지만 나아진게 전혀 없는거같아요. 공략 꼭 지켜주세요.. 믿고 뽑았는데 전혀 나아지고 바뀐게 없으면 너무 실망스럽잖아요.. 그리고 길고양이뿐만 아니라 다른 동물 학대하는 부분도 처벌 강화 부탁드립니다",
"num_agree": 5,
"petition_idx": "513",
"status": "청원종료",
"title": "길고양이를 도와주세요"
}
``` | heegyu/korean-petitions | [
"license:mit",
"region:us"
] | 2022-11-22T07:56:58+00:00 | {"license": "mit"} | 2023-01-15T09:46:48+00:00 | [] | [] | TAGS
#license-mit #region-us
| # 청와대 국민청원
데이터 출처: URL
크기: 651.8MB
sample
| [
"# 청와대 국민청원\n데이터 출처: URL\n크기: 651.8MB\n\nsample"
] | [
"TAGS\n#license-mit #region-us \n",
"# 청와대 국민청원\n데이터 출처: URL\n크기: 651.8MB\n\nsample"
] | [
11,
16
] | [
"passage: TAGS\n#license-mit #region-us \n# 청와대 국민청원\n데이터 출처: URL\n크기: 651.8MB\n\nsample"
] |
4907bc207705a1da6e08dcd60ee01832a3c012ac |
# Dataset Card for [Dataset Name]
## Table of Contents
- [Table of Contents](#table-of-contents)
- [Dataset Description](#dataset-description)
- [Dataset Summary](#dataset-summary)
- [Supported Tasks and Leaderboards](#supported-tasks-and-leaderboards)
- [Languages](#languages)
- [Dataset Structure](#dataset-structure)
- [Data Instances](#data-instances)
- [Data Fields](#data-fields)
- [Data Splits](#data-splits)
- [Dataset Creation](#dataset-creation)
- [Curation Rationale](#curation-rationale)
- [Source Data](#source-data)
- [Annotations](#annotations)
- [Personal and Sensitive Information](#personal-and-sensitive-information)
- [Considerations for Using the Data](#considerations-for-using-the-data)
- [Social Impact of Dataset](#social-impact-of-dataset)
- [Discussion of Biases](#discussion-of-biases)
- [Other Known Limitations](#other-known-limitations)
- [Additional Information](#additional-information)
- [Dataset Curators](#dataset-curators)
- [Licensing Information](#licensing-information)
- [Citation Information](#citation-information)
- [Contributions](#contributions)
## Dataset Description
- **Homepage:**
- **Repository:**
- **Paper:**
- **Leaderboard:**
- **Point of Contact:**
### Dataset Summary
[More Information Needed]
### Supported Tasks and Leaderboards
[More Information Needed]
### Languages
[More Information Needed]
## Dataset Structure
### Data Instances
[More Information Needed]
### Data Fields
[More Information Needed]
### Data Splits
[More Information Needed]
## Dataset Creation
### Curation Rationale
[More Information Needed]
### Source Data
#### Initial Data Collection and Normalization
[More Information Needed]
#### Who are the source language producers?
[More Information Needed]
### Annotations
#### Annotation process
[More Information Needed]
#### Who are the annotators?
[More Information Needed]
### Personal and Sensitive Information
[More Information Needed]
## Considerations for Using the Data
### Social Impact of Dataset
[More Information Needed]
### Discussion of Biases
[More Information Needed]
### Other Known Limitations
[More Information Needed]
## Additional Information
### Dataset Curators
[More Information Needed]
### Licensing Information
[More Information Needed]
### Citation Information
[More Information Needed]
### Contributions
Thanks to [@github-username](https://github.com/<github-username>) for adding this dataset.
| DTU54DL/demo-common-whisper | [
"task_categories:token-classification",
"annotations_creators:expert-generated",
"language_creators:found",
"multilinguality:monolingual",
"size_categories:10K<n<100K",
"source_datasets:original",
"language:en",
"license:mit",
"region:us"
] | 2022-11-22T08:40:07+00:00 | {"annotations_creators": ["expert-generated"], "language_creators": ["found"], "language": ["en"], "license": ["mit"], "multilinguality": ["monolingual"], "size_categories": ["10K<n<100K"], "source_datasets": ["original"], "task_categories": ["token-classification"], "task_ids": ["token-classification-other-acronym-identification"], "paperswithcode_id": "acronym-identification", "pretty_name": "Acronym Identification Dataset", "train-eval-index": [{"col_mapping": {"labels": "tags", "tokens": "tokens"}, "config": "default", "splits": {"eval_split": "test"}, "task": "token-classification", "task_id": "entity_extraction"}]} | 2022-11-22T08:43:39+00:00 | [] | [
"en"
] | TAGS
#task_categories-token-classification #annotations_creators-expert-generated #language_creators-found #multilinguality-monolingual #size_categories-10K<n<100K #source_datasets-original #language-English #license-mit #region-us
|
# Dataset Card for [Dataset Name]
## Table of Contents
- Table of Contents
- Dataset Description
- Dataset Summary
- Supported Tasks and Leaderboards
- Languages
- Dataset Structure
- Data Instances
- Data Fields
- Data Splits
- Dataset Creation
- Curation Rationale
- Source Data
- Annotations
- Personal and Sensitive Information
- Considerations for Using the Data
- Social Impact of Dataset
- Discussion of Biases
- Other Known Limitations
- Additional Information
- Dataset Curators
- Licensing Information
- Citation Information
- Contributions
## Dataset Description
- Homepage:
- Repository:
- Paper:
- Leaderboard:
- Point of Contact:
### Dataset Summary
### Supported Tasks and Leaderboards
### Languages
## Dataset Structure
### Data Instances
### Data Fields
### Data Splits
## Dataset Creation
### Curation Rationale
### Source Data
#### Initial Data Collection and Normalization
#### Who are the source language producers?
### Annotations
#### Annotation process
#### Who are the annotators?
### Personal and Sensitive Information
## Considerations for Using the Data
### Social Impact of Dataset
### Discussion of Biases
### Other Known Limitations
## Additional Information
### Dataset Curators
### Licensing Information
### Contributions
Thanks to @github-username for adding this dataset.
| [
"# Dataset Card for [Dataset Name]",
"## Table of Contents\n- Table of Contents\n- Dataset Description\n - Dataset Summary\n - Supported Tasks and Leaderboards\n - Languages\n- Dataset Structure\n - Data Instances\n - Data Fields\n - Data Splits\n- Dataset Creation\n - Curation Rationale\n - Source Data\n - Annotations\n - Personal and Sensitive Information\n- Considerations for Using the Data\n - Social Impact of Dataset\n - Discussion of Biases\n - Other Known Limitations\n- Additional Information\n - Dataset Curators\n - Licensing Information\n - Citation Information\n - Contributions",
"## Dataset Description\n\n- Homepage:\n- Repository:\n- Paper:\n- Leaderboard:\n- Point of Contact:",
"### Dataset Summary",
"### Supported Tasks and Leaderboards",
"### Languages",
"## Dataset Structure",
"### Data Instances",
"### Data Fields",
"### Data Splits",
"## Dataset Creation",
"### Curation Rationale",
"### Source Data",
"#### Initial Data Collection and Normalization",
"#### Who are the source language producers?",
"### Annotations",
"#### Annotation process",
"#### Who are the annotators?",
"### Personal and Sensitive Information",
"## Considerations for Using the Data",
"### Social Impact of Dataset",
"### Discussion of Biases",
"### Other Known Limitations",
"## Additional Information",
"### Dataset Curators",
"### Licensing Information",
"### Contributions\n\nThanks to @github-username for adding this dataset."
] | [
"TAGS\n#task_categories-token-classification #annotations_creators-expert-generated #language_creators-found #multilinguality-monolingual #size_categories-10K<n<100K #source_datasets-original #language-English #license-mit #region-us \n",
"# Dataset Card for [Dataset Name]",
"## Table of Contents\n- Table of Contents\n- Dataset Description\n - Dataset Summary\n - Supported Tasks and Leaderboards\n - Languages\n- Dataset Structure\n - Data Instances\n - Data Fields\n - Data Splits\n- Dataset Creation\n - Curation Rationale\n - Source Data\n - Annotations\n - Personal and Sensitive Information\n- Considerations for Using the Data\n - Social Impact of Dataset\n - Discussion of Biases\n - Other Known Limitations\n- Additional Information\n - Dataset Curators\n - Licensing Information\n - Citation Information\n - Contributions",
"## Dataset Description\n\n- Homepage:\n- Repository:\n- Paper:\n- Leaderboard:\n- Point of Contact:",
"### Dataset Summary",
"### Supported Tasks and Leaderboards",
"### Languages",
"## Dataset Structure",
"### Data Instances",
"### Data Fields",
"### Data Splits",
"## Dataset Creation",
"### Curation Rationale",
"### Source Data",
"#### Initial Data Collection and Normalization",
"#### Who are the source language producers?",
"### Annotations",
"#### Annotation process",
"#### Who are the annotators?",
"### Personal and Sensitive Information",
"## Considerations for Using the Data",
"### Social Impact of Dataset",
"### Discussion of Biases",
"### Other Known Limitations",
"## Additional Information",
"### Dataset Curators",
"### Licensing Information",
"### Contributions\n\nThanks to @github-username for adding this dataset."
] | [
76,
10,
125,
24,
6,
10,
4,
6,
6,
5,
5,
5,
7,
4,
10,
10,
5,
5,
9,
8,
8,
7,
8,
7,
5,
6,
6,
19
] | [
"passage: TAGS\n#task_categories-token-classification #annotations_creators-expert-generated #language_creators-found #multilinguality-monolingual #size_categories-10K<n<100K #source_datasets-original #language-English #license-mit #region-us \n# Dataset Card for [Dataset Name]## Table of Contents\n- Table of Contents\n- Dataset Description\n - Dataset Summary\n - Supported Tasks and Leaderboards\n - Languages\n- Dataset Structure\n - Data Instances\n - Data Fields\n - Data Splits\n- Dataset Creation\n - Curation Rationale\n - Source Data\n - Annotations\n - Personal and Sensitive Information\n- Considerations for Using the Data\n - Social Impact of Dataset\n - Discussion of Biases\n - Other Known Limitations\n- Additional Information\n - Dataset Curators\n - Licensing Information\n - Citation Information\n - Contributions## Dataset Description\n\n- Homepage:\n- Repository:\n- Paper:\n- Leaderboard:\n- Point of Contact:### Dataset Summary### Supported Tasks and Leaderboards### Languages## Dataset Structure### Data Instances### Data Fields### Data Splits## Dataset Creation### Curation Rationale### Source Data#### Initial Data Collection and Normalization#### Who are the source language producers?### Annotations#### Annotation process#### Who are the annotators?### Personal and Sensitive Information## Considerations for Using the Data### Social Impact of Dataset### Discussion of Biases### Other Known Limitations## Additional Information### Dataset Curators### Licensing Information### Contributions\n\nThanks to @github-username for adding this dataset."
] |
5f03051ef2617af00983a6961529505e2f9d994a | # Dataset Card for "librispeech_unit_speech"
[More Information needed](https://github.com/huggingface/datasets/blob/main/CONTRIBUTING.md#how-to-contribute-to-the-dataset-cards) | voidful/librispeech_unit_speech | [
"region:us"
] | 2022-11-22T08:45:06+00:00 | {"dataset_info": {"features": [{"name": "text", "dtype": "string"}, {"name": "speaker_id", "dtype": "int64"}, {"name": "chapter_id", "dtype": "int64"}, {"name": "id", "dtype": "string"}, {"name": "audio_0", "dtype": "audio"}, {"name": "audio_1", "dtype": "audio"}, {"name": "audio_2", "dtype": "audio"}, {"name": "audio_3", "dtype": "audio"}, {"name": "audio_4", "dtype": "audio"}, {"name": "audio_5", "dtype": "audio"}, {"name": "audio_6", "dtype": "audio"}, {"name": "audio_7", "dtype": "audio"}, {"name": "audio_8", "dtype": "audio"}, {"name": "audio_9", "dtype": "audio"}, {"name": "audio_10", "dtype": "audio"}], "splits": [{"name": "test.clean", "num_bytes": 6819850294.02, "num_examples": 2620}, {"name": "train.clean.100", "num_bytes": 126297137700.482, "num_examples": 28539}], "download_size": 87782578496, "dataset_size": 133116987994.502}} | 2022-11-22T16:37:07+00:00 | [] | [] | TAGS
#region-us
| # Dataset Card for "librispeech_unit_speech"
More Information needed | [
"# Dataset Card for \"librispeech_unit_speech\"\n\nMore Information needed"
] | [
"TAGS\n#region-us \n",
"# Dataset Card for \"librispeech_unit_speech\"\n\nMore Information needed"
] | [
6,
19
] | [
"passage: TAGS\n#region-us \n# Dataset Card for \"librispeech_unit_speech\"\n\nMore Information needed"
] |
f6e4371203ca415c9b0a0f456927ccfc4a5bdba7 | # Dataset Card for "sroie"
[More Information needed](https://github.com/huggingface/datasets/blob/main/CONTRIBUTING.md#how-to-contribute-to-the-dataset-cards) | Rahul-2022/sroie | [
"region:us"
] | 2022-11-22T09:12:42+00:00 | {"dataset_info": {"features": [{"name": "pixel_values", "sequence": {"sequence": {"sequence": "float32"}}}, {"name": "labels", "sequence": "int64"}, {"name": "target_sequence", "dtype": "string"}], "splits": [{"name": "train", "num_bytes": 4678664586, "num_examples": 563}, {"name": "test", "num_bytes": 523545183, "num_examples": 63}], "download_size": 381412353, "dataset_size": 5202209769}} | 2022-11-25T05:24:35+00:00 | [] | [] | TAGS
#region-us
| # Dataset Card for "sroie"
More Information needed | [
"# Dataset Card for \"sroie\"\n\nMore Information needed"
] | [
"TAGS\n#region-us \n",
"# Dataset Card for \"sroie\"\n\nMore Information needed"
] | [
6,
13
] | [
"passage: TAGS\n#region-us \n# Dataset Card for \"sroie\"\n\nMore Information needed"
] |
d418abf63dff59d65b3d4e7a8ba4fee05eb91ca2 |
# Dataset Card for Czech Named Entity Corpus 2.0
## Dataset Description
The dataset contains Czech sentences and annotated named entities. Total number of sentences is around 9,000 and total number of entities is around 34,000. (Total means train + validation + test)
## Dataset Features
Each sample contains:
- `text`: source sentence
- `entities`: list of selected entities. Each entity contains:
- `category_id`: string identifier of the entity category
- `category_str`: human-friendly category name in Czech (verbalizer)
- `start`: index on which the entity starts in the source sentence
- `end`: index on which the entity ends in the source sentence
- `content`: entity content, it was created as `text[start:end]`
- `entity_id`: unique entity string identifier
- `parent_id`: If entity was selected inside another entity (e.g. house number inside address), `parent_id` is the identifier of the parent entity. None otherwise.
The `entity_id` field was checked to be globally unique (across data samples and dataset splits.)
## Entity categories
The list of the recognized entities (`category_id`, `category_str` pairs):
```python3
{
'A': 'číslo v adrese / kontaktním údaji',
'ah': 'číslo domu',
'at': 'telefonní číslo / fax',
'az': 'PSČ (poštovní směrovací číslo)',
'C': 'reference/bibliografie',
'f': 'cizí výraz',
'g_': 'geografický název - jiný',
'gc': 'stát/země',
'gh': 'jméno vodstva',
'gl': 'přírodní oblast/útvar',
'gq': 'městská čtvrť',
'gr': 'území',
'gs': 'ulice/náměstí',
'gt': 'kontinent',
'gu': 'město/zámek',
'i_': 'instituce - jiná',
'ia': 'konference/soutěž',
'ic': 'kulturní/vzdělávací/vědecká instituce',
'if': 'komerční instituce',
'io': 'vládní/politická instituce',
'me': 'emailová adresa',
'mi': 'URL / internetový odkaz',
'mn': 'časopis',
'ms': 'radio/televizní stanice',
'n_': 'číselný výraz - jiný',
'na': 'věk',
'nb': 'číslo stránky/kapitoly/sekce/objektu',
'nc': 'množství/počet',
'ni': 'číslo položky',
'no': 'pořadí',
'ns': 'sportovní skóre',
'o_': 'artefakt - jiný',
'oa': 'umělecké dílo / kulturní artefakt',
'oe': 'jednotka',
'om': 'měna',
'op': 'produkt/výrobek',
'or': 'zákon/směrnice/listina',
'P': 'celé jméno',
'p_': 'jméno - jiné',
'pc': 'národnost',
'pd': '(akademický) titul',
'pf': 'křestní jméno',
'pm': 'prostřední jméno',
'pp': 'mýtická/historická postava',
'ps': 'příjmení',
's': 'zkratka',
'T': 'čas/datum',
'td': 'den',
'tf': 'svátky',
'th': 'hodiny/minuty',
'tm': 'měsíc',
'ty': 'rok',
}
```
## Dataset Source
The dataset is a preprocessed adaptation of existing CNEC 2.0 dataset [project info](https://ufal.mff.cuni.cz/cnec/cnec2.0), [link to data](https://lindat.mff.cuni.cz/repository/xmlui/handle/11858/00-097C-0000-0023-1B22-8). This adaptation contains (almost) same data, but converted to a convenient format. In addition, we inspected and decided to remove entity categories: `?`, `segm`, `cap`, `lower`, `upper`, which were either undocumented and/or carried little semantic meaning.
The category names (verbalizers) are not in the original dataset. They were added by a Czech native speaker using the available [documentation](https://ufal.mff.cuni.cz/cnec/cnec2.0) and by looking at several occurrences in the data.
## Citation
Cite authors of the [original dataset](https://lindat.mff.cuni.cz/repository/xmlui/handle/11858/00-097C-0000-0023-1B22-8):
```bibtex
@misc{11858/00-097C-0000-0023-1B22-8,
title = {Czech Named Entity Corpus 2.0},
author = {{\v S}ev{\v c}{\'{\i}}kov{\'a}, Magda and {\v Z}abokrtsk{\'y}, Zden{\v e}k and Strakov{\'a}, Jana and Straka, Milan},
url = {http://hdl.handle.net/11858/00-097C-0000-0023-1B22-8},
note = {{LINDAT}/{CLARIAH}-{CZ} digital library at the Institute of Formal and Applied Linguistics ({{\'U}FAL}), Faculty of Mathematics and Physics, Charles University},
copyright = {Attribution-{NonCommercial}-{ShareAlike} 3.0 Unported ({CC} {BY}-{NC}-{SA} 3.0)},
year = {2014}
}
```
| fewshot-goes-multilingual/cs_czech-named-entity-corpus_2.0 | [
"task_categories:token-classification",
"task_ids:named-entity-recognition",
"annotations_creators:expert-generated",
"language_creators:found",
"multilinguality:monolingual",
"size_categories:1K<n<10K",
"source_datasets:original",
"language:cs",
"license:cc-by-nc-sa-3.0",
"czech NER",
"CNEC",
"region:us"
] | 2022-11-22T09:21:00+00:00 | {"annotations_creators": ["expert-generated"], "language_creators": ["found"], "language": ["cs"], "license": ["cc-by-nc-sa-3.0"], "multilinguality": ["monolingual"], "size_categories": ["1K<n<10K"], "source_datasets": ["original"], "task_categories": ["token-classification"], "task_ids": ["named-entity-recognition"], "pretty_name": "Czech Named Entity Corpus 2.0", "tags": ["czech NER", "CNEC"]} | 2022-12-05T22:44:28+00:00 | [] | [
"cs"
] | TAGS
#task_categories-token-classification #task_ids-named-entity-recognition #annotations_creators-expert-generated #language_creators-found #multilinguality-monolingual #size_categories-1K<n<10K #source_datasets-original #language-Czech #license-cc-by-nc-sa-3.0 #czech NER #CNEC #region-us
|
# Dataset Card for Czech Named Entity Corpus 2.0
## Dataset Description
The dataset contains Czech sentences and annotated named entities. Total number of sentences is around 9,000 and total number of entities is around 34,000. (Total means train + validation + test)
## Dataset Features
Each sample contains:
- 'text': source sentence
- 'entities': list of selected entities. Each entity contains:
- 'category_id': string identifier of the entity category
- 'category_str': human-friendly category name in Czech (verbalizer)
- 'start': index on which the entity starts in the source sentence
- 'end': index on which the entity ends in the source sentence
- 'content': entity content, it was created as 'text[start:end]'
- 'entity_id': unique entity string identifier
- 'parent_id': If entity was selected inside another entity (e.g. house number inside address), 'parent_id' is the identifier of the parent entity. None otherwise.
The 'entity_id' field was checked to be globally unique (across data samples and dataset splits.)
## Entity categories
The list of the recognized entities ('category_id', 'category_str' pairs):
## Dataset Source
The dataset is a preprocessed adaptation of existing CNEC 2.0 dataset project info, link to data. This adaptation contains (almost) same data, but converted to a convenient format. In addition, we inspected and decided to remove entity categories: '?', 'segm', 'cap', 'lower', 'upper', which were either undocumented and/or carried little semantic meaning.
The category names (verbalizers) are not in the original dataset. They were added by a Czech native speaker using the available documentation and by looking at several occurrences in the data.
Cite authors of the original dataset:
| [
"# Dataset Card for Czech Named Entity Corpus 2.0",
"## Dataset Description\nThe dataset contains Czech sentences and annotated named entities. Total number of sentences is around 9,000 and total number of entities is around 34,000. (Total means train + validation + test)",
"## Dataset Features\nEach sample contains:\n- 'text': source sentence\n- 'entities': list of selected entities. Each entity contains:\n - 'category_id': string identifier of the entity category\n - 'category_str': human-friendly category name in Czech (verbalizer)\n - 'start': index on which the entity starts in the source sentence\n - 'end': index on which the entity ends in the source sentence\n - 'content': entity content, it was created as 'text[start:end]'\n - 'entity_id': unique entity string identifier\n - 'parent_id': If entity was selected inside another entity (e.g. house number inside address), 'parent_id' is the identifier of the parent entity. None otherwise.\n\nThe 'entity_id' field was checked to be globally unique (across data samples and dataset splits.)",
"## Entity categories\nThe list of the recognized entities ('category_id', 'category_str' pairs):",
"## Dataset Source\n\nThe dataset is a preprocessed adaptation of existing CNEC 2.0 dataset project info, link to data. This adaptation contains (almost) same data, but converted to a convenient format. In addition, we inspected and decided to remove entity categories: '?', 'segm', 'cap', 'lower', 'upper', which were either undocumented and/or carried little semantic meaning.\n\nThe category names (verbalizers) are not in the original dataset. They were added by a Czech native speaker using the available documentation and by looking at several occurrences in the data.\n\n\nCite authors of the original dataset:"
] | [
"TAGS\n#task_categories-token-classification #task_ids-named-entity-recognition #annotations_creators-expert-generated #language_creators-found #multilinguality-monolingual #size_categories-1K<n<10K #source_datasets-original #language-Czech #license-cc-by-nc-sa-3.0 #czech NER #CNEC #region-us \n",
"# Dataset Card for Czech Named Entity Corpus 2.0",
"## Dataset Description\nThe dataset contains Czech sentences and annotated named entities. Total number of sentences is around 9,000 and total number of entities is around 34,000. (Total means train + validation + test)",
"## Dataset Features\nEach sample contains:\n- 'text': source sentence\n- 'entities': list of selected entities. Each entity contains:\n - 'category_id': string identifier of the entity category\n - 'category_str': human-friendly category name in Czech (verbalizer)\n - 'start': index on which the entity starts in the source sentence\n - 'end': index on which the entity ends in the source sentence\n - 'content': entity content, it was created as 'text[start:end]'\n - 'entity_id': unique entity string identifier\n - 'parent_id': If entity was selected inside another entity (e.g. house number inside address), 'parent_id' is the identifier of the parent entity. None otherwise.\n\nThe 'entity_id' field was checked to be globally unique (across data samples and dataset splits.)",
"## Entity categories\nThe list of the recognized entities ('category_id', 'category_str' pairs):",
"## Dataset Source\n\nThe dataset is a preprocessed adaptation of existing CNEC 2.0 dataset project info, link to data. This adaptation contains (almost) same data, but converted to a convenient format. In addition, we inspected and decided to remove entity categories: '?', 'segm', 'cap', 'lower', 'upper', which were either undocumented and/or carried little semantic meaning.\n\nThe category names (verbalizers) are not in the original dataset. They were added by a Czech native speaker using the available documentation and by looking at several occurrences in the data.\n\n\nCite authors of the original dataset:"
] | [
109,
12,
51,
219,
32,
154
] | [
"passage: TAGS\n#task_categories-token-classification #task_ids-named-entity-recognition #annotations_creators-expert-generated #language_creators-found #multilinguality-monolingual #size_categories-1K<n<10K #source_datasets-original #language-Czech #license-cc-by-nc-sa-3.0 #czech NER #CNEC #region-us \n# Dataset Card for Czech Named Entity Corpus 2.0## Dataset Description\nThe dataset contains Czech sentences and annotated named entities. Total number of sentences is around 9,000 and total number of entities is around 34,000. (Total means train + validation + test)## Dataset Features\nEach sample contains:\n- 'text': source sentence\n- 'entities': list of selected entities. Each entity contains:\n - 'category_id': string identifier of the entity category\n - 'category_str': human-friendly category name in Czech (verbalizer)\n - 'start': index on which the entity starts in the source sentence\n - 'end': index on which the entity ends in the source sentence\n - 'content': entity content, it was created as 'text[start:end]'\n - 'entity_id': unique entity string identifier\n - 'parent_id': If entity was selected inside another entity (e.g. house number inside address), 'parent_id' is the identifier of the parent entity. None otherwise.\n\nThe 'entity_id' field was checked to be globally unique (across data samples and dataset splits.)## Entity categories\nThe list of the recognized entities ('category_id', 'category_str' pairs):"
] |
8bd6d4f5906c414ebb27cb13ff28091fc72eeba0 | # Dataset Card for "scottishdirectories"
[More Information needed](https://github.com/huggingface/datasets/blob/main/CONTRIBUTING.md#how-to-contribute-to-the-dataset-cards) | davanstrien/scottishdirectories | [
"region:us"
] | 2022-11-22T10:30:50+00:00 | {"dataset_info": {"features": [{"name": "id", "dtype": "string"}, {"name": "image", "dtype": "string"}, {"name": "iiif_manifest", "dtype": "string"}], "splits": [{"name": "train", "num_bytes": 166317574, "num_examples": 700906}], "download_size": 13810640, "dataset_size": 166317574}} | 2022-11-22T10:30:56+00:00 | [] | [] | TAGS
#region-us
| # Dataset Card for "scottishdirectories"
More Information needed | [
"# Dataset Card for \"scottishdirectories\"\n\nMore Information needed"
] | [
"TAGS\n#region-us \n",
"# Dataset Card for \"scottishdirectories\"\n\nMore Information needed"
] | [
6,
15
] | [
"passage: TAGS\n#region-us \n# Dataset Card for \"scottishdirectories\"\n\nMore Information needed"
] |
a1dec2756f321216385d62503aa7a14e07f03e0e | # Dataset Card for "unbalanced-motifs-50K"
[More Information needed](https://github.com/huggingface/datasets/blob/main/CONTRIBUTING.md#how-to-contribute-to-the-dataset-cards) | PGT/unbalanced-motifs-50K | [
"region:us"
] | 2022-11-22T10:34:18+00:00 | {"dataset_info": {"features": [{"name": "motif_G13", "sequence": "float64"}, {"name": "motif_G14", "sequence": "float64"}, {"name": "motif_G15", "sequence": "float64"}, {"name": "motif_G16", "sequence": "float64"}, {"name": "motif_G17", "sequence": "float64"}, {"name": "motif_G18", "sequence": "float64"}, {"name": "class_label", "sequence": "int64"}, {"name": "num_nodes", "dtype": "int64"}, {"name": "num_bridges", "sequence": "float64"}, {"name": "num_cycles", "sequence": "float64"}, {"name": "avg_shortest_path_len", "sequence": "float64"}, {"name": "edge_attr", "sequence": {"sequence": "int64"}}, {"name": "node_feat", "sequence": {"sequence": "int64"}}, {"name": "edge_index", "sequence": {"sequence": "int64"}}], "splits": [{"name": "train", "num_bytes": 88732557, "num_examples": 49986}], "download_size": 10269466, "dataset_size": 88732557}} | 2022-12-07T15:32:59+00:00 | [] | [] | TAGS
#region-us
| # Dataset Card for "unbalanced-motifs-50K"
More Information needed | [
"# Dataset Card for \"unbalanced-motifs-50K\"\n\nMore Information needed"
] | [
"TAGS\n#region-us \n",
"# Dataset Card for \"unbalanced-motifs-50K\"\n\nMore Information needed"
] | [
6,
18
] | [
"passage: TAGS\n#region-us \n# Dataset Card for \"unbalanced-motifs-50K\"\n\nMore Information needed"
] |
c40e9aa6521fcc3b91423e476ab453f769b95436 | # Dataset Card for "unbalanced-motifs-500K"
[More Information needed](https://github.com/huggingface/datasets/blob/main/CONTRIBUTING.md#how-to-contribute-to-the-dataset-cards) | PGT/unbalanced-motifs-500K | [
"region:us"
] | 2022-11-22T10:47:25+00:00 | {"dataset_info": {"features": [{"name": "motif_G13", "sequence": "float64"}, {"name": "motif_G14", "sequence": "float64"}, {"name": "motif_G15", "sequence": "float64"}, {"name": "motif_G16", "sequence": "float64"}, {"name": "motif_G17", "sequence": "float64"}, {"name": "motif_G18", "sequence": "float64"}, {"name": "class_label", "sequence": "int64"}, {"name": "num_nodes", "dtype": "int64"}, {"name": "num_bridges", "sequence": "float64"}, {"name": "num_cycles", "sequence": "float64"}, {"name": "avg_shortest_path_len", "sequence": "float64"}, {"name": "edge_attr", "sequence": {"sequence": "int64"}}, {"name": "node_feat", "sequence": {"sequence": "int64"}}, {"name": "edge_index", "sequence": {"sequence": "int64"}}], "splits": [{"name": "train", "num_bytes": 893024211, "num_examples": 499986}], "download_size": 102623567, "dataset_size": 893024211}} | 2022-12-07T15:30:52+00:00 | [] | [] | TAGS
#region-us
| # Dataset Card for "unbalanced-motifs-500K"
More Information needed | [
"# Dataset Card for \"unbalanced-motifs-500K\"\n\nMore Information needed"
] | [
"TAGS\n#region-us \n",
"# Dataset Card for \"unbalanced-motifs-500K\"\n\nMore Information needed"
] | [
6,
18
] | [
"passage: TAGS\n#region-us \n# Dataset Card for \"unbalanced-motifs-500K\"\n\nMore Information needed"
] |
50579a0b591445f91bf7269b28611ad1cc6a05d2 |
# Dataset Card for NYU Depth V2
## Table of Contents
- [Table of Contents](#table-of-contents)
- [Dataset Description](#dataset-description)
- [Dataset Summary](#dataset-summary)
- [Supported Tasks](#supported-tasks)
- [Languages](#languages)
- [Dataset Structure](#dataset-structure)
- [Data Instances](#data-instances)
- [Data Fields](#data-fields)
- [Data Splits](#data-splits)
- [Visualization](#visualization)
- [Dataset Creation](#dataset-creation)
- [Curation Rationale](#curation-rationale)
- [Source Data](#source-data)
- [Annotations](#annotations)
- [Personal and Sensitive Information](#personal-and-sensitive-information)
- [Considerations for Using the Data](#considerations-for-using-the-data)
- [Social Impact of Dataset](#social-impact-of-dataset)
- [Discussion of Biases](#discussion-of-biases)
- [Other Known Limitations](#other-known-limitations)
- [Additional Information](#additional-information)
- [Dataset Curators](#dataset-curators)
- [Licensing Information](#licensing-information)
- [Citation Information](#citation-information)
- [Contributions](#contributions)
## Dataset Description
- **Homepage:** [NYU Depth Dataset V2 homepage](https://cs.nyu.edu/~silberman/datasets/nyu_depth_v2.html)
- **Repository:** Fast Depth [repository](https://github.com/dwofk/fast-depth) which was used to source the dataset in this repository. It is a preprocessed version of the original NYU Depth V2 dataset linked above. It is also used in [TensorFlow Datasets](https://www.tensorflow.org/datasets/catalog/nyu_depth_v2).
- **Papers:** [Indoor Segmentation and Support Inference from RGBD Images](http://cs.nyu.edu/~silberman/papers/indoor_seg_support.pdf) and [FastDepth: Fast Monocular Depth Estimation on Embedded Systems](https://arxiv.org/abs/1903.03273)
- **Point of Contact:** [Nathan Silberman](mailto:silberman@@cs.nyu.edu) and [Diana Wofk](mailto:[email protected])
### Dataset Summary
As per the [dataset homepage](https://cs.nyu.edu/~silberman/datasets/nyu_depth_v2.html):
The NYU-Depth V2 data set is comprised of video sequences from a variety of indoor scenes as recorded by both the RGB and Depth cameras from the Microsoft [Kinect](http://www.xbox.com/kinect). It features:
* 1449 densely labeled pairs of aligned RGB and depth images
* 464 new scenes taken from 3 cities
* 407,024 new unlabeled frames
* Each object is labeled with a class and an instance number (cup1, cup2, cup3, etc)
The dataset has several components:
* Labeled: A subset of the video data accompanied by dense multi-class labels. This data has also been preprocessed to fill in missing depth labels.
* Raw: The raw rgb, depth and accelerometer data as provided by the Kinect.
* Toolbox: Useful functions for manipulating the data and labels.
### Supported Tasks
- `depth-estimation`: Depth estimation is the task of approximating the perceived depth of a given image. In other words, it's about measuring the distance of each image pixel from the camera.
- `semantic-segmentation`: Semantic segmentation is the task of associating every pixel of an image to a class label.
There are other tasks supported by this dataset as well. You can find more about them by referring to [this resource](https://paperswithcode.com/dataset/nyuv2).
### Languages
English.
## Dataset Structure
### Data Instances
A data point comprises an image and its annotation depth map for both the `train` and `validation` splits.
```
{
'image': <PIL.PngImagePlugin.PngImageFile image mode=RGB at 0x1FF32A3EDA0>,
'depth_map': <PIL.PngImagePlugin.PngImageFile image mode=L at 0x1FF32E5B978>,
}
```
### Data Fields
- `image`: A `PIL.Image.Image` object containing the image. Note that when accessing the image column: `dataset[0]["image"]` the image file is automatically decoded. Decoding of a large number of image files might take a significant amount of time. Thus it is important to first query the sample index before the `"image"` column, *i.e.* `dataset[0]["image"]` should **always** be preferred over `dataset["image"][0]`.
- `depth_map`: A `PIL.Image.Image` object containing the annotation depth map.
### Data Splits
The data is split into training, and validation splits. The training data contains 47584 images, and the validation data contains 654 images.
## Visualization
You can use the following code snippet to visualize samples from the dataset:
```py
from datasets import load_dataset
import numpy as np
import matplotlib.pyplot as plt
cmap = plt.cm.viridis
ds = load_dataset("sayakpaul/nyu_depth_v2")
def colored_depthmap(depth, d_min=None, d_max=None):
if d_min is None:
d_min = np.min(depth)
if d_max is None:
d_max = np.max(depth)
depth_relative = (depth - d_min) / (d_max - d_min)
return 255 * cmap(depth_relative)[:,:,:3] # H, W, C
def merge_into_row(input, depth_target):
input = np.array(input)
depth_target = np.squeeze(np.array(depth_target))
d_min = np.min(depth_target)
d_max = np.max(depth_target)
depth_target_col = colored_depthmap(depth_target, d_min, d_max)
img_merge = np.hstack([input, depth_target_col])
return img_merge
random_indices = np.random.choice(len(ds["train"]), 9).tolist()
train_set = ds["train"]
plt.figure(figsize=(15, 6))
for i, idx in enumerate(random_indices):
ax = plt.subplot(3, 3, i + 1)
image_viz = merge_into_row(
train_set[idx]["image"], train_set[idx]["depth_map"]
)
plt.imshow(image_viz.astype("uint8"))
plt.axis("off")
```
## Dataset Creation
### Curation Rationale
The rationale from [the paper](http://cs.nyu.edu/~silberman/papers/indoor_seg_support.pdf) that introduced the NYU Depth V2 dataset:
> We present an approach to interpret the major surfaces, objects, and support relations of an indoor scene from an RGBD image. Most existing work ignores physical interactions or is applied only to tidy rooms and hallways. Our goal is to parse typical, often messy, indoor scenes into floor, walls, supporting surfaces, and object regions, and to recover support relationships. One of our main interests is to better understand how 3D cues can best inform a structured 3D interpretation.
### Source Data
#### Initial Data Collection
> The dataset consists of 1449 RGBD images, gathered from a wide range
of commercial and residential buildings in three different US cities, comprising
464 different indoor scenes across 26 scene classes.A dense per-pixel labeling was
obtained for each image using Amazon Mechanical Turk.
### Annotations
#### Annotation process
This is an involved process. Interested readers are referred to Sections 2, 3, and 4 of the [original paper](http://cs.nyu.edu/~silberman/papers/indoor_seg_support.pdf).
#### Who are the annotators?
AMT annotators.
### Personal and Sensitive Information
[More Information Needed]
## Considerations for Using the Data
### Social Impact of Dataset
[More Information Needed]
### Discussion of Biases
[More Information Needed]
### Other Known Limitations
[More Information Needed]
## Additional Information
### Dataset Curators
* Original NYU Depth V2 dataset: Nathan Silberman, Derek Hoiem, Pushmeet Kohli, Rob Fergus
* Preprocessed version: Diana Wofk, Fangchang Ma, Tien-Ju Yang, Sertac Karaman, Vivienne Sze
### Licensing Information
The preprocessed NYU Depth V2 dataset is licensed under a [MIT License](https://github.com/dwofk/fast-depth/blob/master/LICENSE).
### Citation Information
```bibtex
@inproceedings{Silberman:ECCV12,
author = {Nathan Silberman, Derek Hoiem, Pushmeet Kohli and Rob Fergus},
title = {Indoor Segmentation and Support Inference from RGBD Images},
booktitle = {ECCV},
year = {2012}
}
@inproceedings{icra_2019_fastdepth,
author = {{Wofk, Diana and Ma, Fangchang and Yang, Tien-Ju and Karaman, Sertac and Sze, Vivienne}},
title = {{FastDepth: Fast Monocular Depth Estimation on Embedded Systems}},
booktitle = {{IEEE International Conference on Robotics and Automation (ICRA)}},
year = {{2019}}
}
```
### Contributions
Thanks to [@sayakpaul](https://huggingface.co/sayakpaul) for adding this dataset. | sayakpaul/nyu_depth_v2 | [
"task_categories:depth-estimation",
"multilinguality:monolingual",
"size_categories:10K<n<100K",
"language:en",
"license:apache-2.0",
"depth-estimation",
"arxiv:1903.03273",
"region:us"
] | 2022-11-22T10:51:08+00:00 | {"language": ["en"], "license": "apache-2.0", "multilinguality": ["monolingual"], "size_categories": ["10K<n<100K"], "task_categories": ["depth-estimation"], "task_ids": [], "paperswithcode_id": "nyuv2", "pretty_name": "NYU Depth V2", "tags": ["depth-estimation"], "dataset_info": {"features": [{"name": "image", "dtype": "image"}, {"name": "depth_map", "dtype": "image"}], "splits": [{"name": "train", "num_bytes": 20212097551, "num_examples": 47584}, {"name": "validation", "num_bytes": 240785762, "num_examples": 654}], "download_size": 35151124480, "dataset_size": 20452883313}} | 2022-12-12T13:35:31+00:00 | [
"1903.03273"
] | [
"en"
] | TAGS
#task_categories-depth-estimation #multilinguality-monolingual #size_categories-10K<n<100K #language-English #license-apache-2.0 #depth-estimation #arxiv-1903.03273 #region-us
|
# Dataset Card for NYU Depth V2
## Table of Contents
- Table of Contents
- Dataset Description
- Dataset Summary
- Supported Tasks
- Languages
- Dataset Structure
- Data Instances
- Data Fields
- Data Splits
- Visualization
- Dataset Creation
- Curation Rationale
- Source Data
- Annotations
- Personal and Sensitive Information
- Considerations for Using the Data
- Social Impact of Dataset
- Discussion of Biases
- Other Known Limitations
- Additional Information
- Dataset Curators
- Licensing Information
- Citation Information
- Contributions
## Dataset Description
- Homepage: NYU Depth Dataset V2 homepage
- Repository: Fast Depth repository which was used to source the dataset in this repository. It is a preprocessed version of the original NYU Depth V2 dataset linked above. It is also used in TensorFlow Datasets.
- Papers: Indoor Segmentation and Support Inference from RGBD Images and FastDepth: Fast Monocular Depth Estimation on Embedded Systems
- Point of Contact: Nathan Silberman and Diana Wofk
### Dataset Summary
As per the dataset homepage:
The NYU-Depth V2 data set is comprised of video sequences from a variety of indoor scenes as recorded by both the RGB and Depth cameras from the Microsoft Kinect. It features:
* 1449 densely labeled pairs of aligned RGB and depth images
* 464 new scenes taken from 3 cities
* 407,024 new unlabeled frames
* Each object is labeled with a class and an instance number (cup1, cup2, cup3, etc)
The dataset has several components:
* Labeled: A subset of the video data accompanied by dense multi-class labels. This data has also been preprocessed to fill in missing depth labels.
* Raw: The raw rgb, depth and accelerometer data as provided by the Kinect.
* Toolbox: Useful functions for manipulating the data and labels.
### Supported Tasks
- 'depth-estimation': Depth estimation is the task of approximating the perceived depth of a given image. In other words, it's about measuring the distance of each image pixel from the camera.
- 'semantic-segmentation': Semantic segmentation is the task of associating every pixel of an image to a class label.
There are other tasks supported by this dataset as well. You can find more about them by referring to this resource.
### Languages
English.
## Dataset Structure
### Data Instances
A data point comprises an image and its annotation depth map for both the 'train' and 'validation' splits.
### Data Fields
- 'image': A 'PIL.Image.Image' object containing the image. Note that when accessing the image column: 'dataset[0]["image"]' the image file is automatically decoded. Decoding of a large number of image files might take a significant amount of time. Thus it is important to first query the sample index before the '"image"' column, *i.e.* 'dataset[0]["image"]' should always be preferred over 'dataset["image"][0]'.
- 'depth_map': A 'PIL.Image.Image' object containing the annotation depth map.
### Data Splits
The data is split into training, and validation splits. The training data contains 47584 images, and the validation data contains 654 images.
## Visualization
You can use the following code snippet to visualize samples from the dataset:
## Dataset Creation
### Curation Rationale
The rationale from the paper that introduced the NYU Depth V2 dataset:
> We present an approach to interpret the major surfaces, objects, and support relations of an indoor scene from an RGBD image. Most existing work ignores physical interactions or is applied only to tidy rooms and hallways. Our goal is to parse typical, often messy, indoor scenes into floor, walls, supporting surfaces, and object regions, and to recover support relationships. One of our main interests is to better understand how 3D cues can best inform a structured 3D interpretation.
### Source Data
#### Initial Data Collection
> The dataset consists of 1449 RGBD images, gathered from a wide range
of commercial and residential buildings in three different US cities, comprising
464 different indoor scenes across 26 scene classes.A dense per-pixel labeling was
obtained for each image using Amazon Mechanical Turk.
### Annotations
#### Annotation process
This is an involved process. Interested readers are referred to Sections 2, 3, and 4 of the original paper.
#### Who are the annotators?
AMT annotators.
### Personal and Sensitive Information
## Considerations for Using the Data
### Social Impact of Dataset
### Discussion of Biases
### Other Known Limitations
## Additional Information
### Dataset Curators
* Original NYU Depth V2 dataset: Nathan Silberman, Derek Hoiem, Pushmeet Kohli, Rob Fergus
* Preprocessed version: Diana Wofk, Fangchang Ma, Tien-Ju Yang, Sertac Karaman, Vivienne Sze
### Licensing Information
The preprocessed NYU Depth V2 dataset is licensed under a MIT License.
### Contributions
Thanks to @sayakpaul for adding this dataset. | [
"# Dataset Card for NYU Depth V2",
"## Table of Contents\n- Table of Contents\n- Dataset Description\n - Dataset Summary\n - Supported Tasks\n - Languages\n- Dataset Structure\n - Data Instances\n - Data Fields\n - Data Splits\n- Visualization\n- Dataset Creation\n - Curation Rationale\n - Source Data\n - Annotations\n - Personal and Sensitive Information\n- Considerations for Using the Data\n - Social Impact of Dataset\n - Discussion of Biases\n - Other Known Limitations\n- Additional Information\n - Dataset Curators\n - Licensing Information\n - Citation Information\n - Contributions",
"## Dataset Description\n\n- Homepage: NYU Depth Dataset V2 homepage\n- Repository: Fast Depth repository which was used to source the dataset in this repository. It is a preprocessed version of the original NYU Depth V2 dataset linked above. It is also used in TensorFlow Datasets.\n- Papers: Indoor Segmentation and Support Inference from RGBD Images and FastDepth: Fast Monocular Depth Estimation on Embedded Systems\n- Point of Contact: Nathan Silberman and Diana Wofk",
"### Dataset Summary\n\nAs per the dataset homepage:\n\nThe NYU-Depth V2 data set is comprised of video sequences from a variety of indoor scenes as recorded by both the RGB and Depth cameras from the Microsoft Kinect. It features:\n\n* 1449 densely labeled pairs of aligned RGB and depth images\n* 464 new scenes taken from 3 cities\n* 407,024 new unlabeled frames\n* Each object is labeled with a class and an instance number (cup1, cup2, cup3, etc)\n\nThe dataset has several components:\n\n* Labeled: A subset of the video data accompanied by dense multi-class labels. This data has also been preprocessed to fill in missing depth labels.\n* Raw: The raw rgb, depth and accelerometer data as provided by the Kinect.\n* Toolbox: Useful functions for manipulating the data and labels.",
"### Supported Tasks\n\n- 'depth-estimation': Depth estimation is the task of approximating the perceived depth of a given image. In other words, it's about measuring the distance of each image pixel from the camera.\n- 'semantic-segmentation': Semantic segmentation is the task of associating every pixel of an image to a class label. \n\nThere are other tasks supported by this dataset as well. You can find more about them by referring to this resource.",
"### Languages\n\nEnglish.",
"## Dataset Structure",
"### Data Instances\n\nA data point comprises an image and its annotation depth map for both the 'train' and 'validation' splits.",
"### Data Fields\n\n- 'image': A 'PIL.Image.Image' object containing the image. Note that when accessing the image column: 'dataset[0][\"image\"]' the image file is automatically decoded. Decoding of a large number of image files might take a significant amount of time. Thus it is important to first query the sample index before the '\"image\"' column, *i.e.* 'dataset[0][\"image\"]' should always be preferred over 'dataset[\"image\"][0]'.\n- 'depth_map': A 'PIL.Image.Image' object containing the annotation depth map.",
"### Data Splits\n\nThe data is split into training, and validation splits. The training data contains 47584 images, and the validation data contains 654 images.",
"## Visualization\n\nYou can use the following code snippet to visualize samples from the dataset:",
"## Dataset Creation",
"### Curation Rationale\n\nThe rationale from the paper that introduced the NYU Depth V2 dataset:\n\n> We present an approach to interpret the major surfaces, objects, and support relations of an indoor scene from an RGBD image. Most existing work ignores physical interactions or is applied only to tidy rooms and hallways. Our goal is to parse typical, often messy, indoor scenes into floor, walls, supporting surfaces, and object regions, and to recover support relationships. One of our main interests is to better understand how 3D cues can best inform a structured 3D interpretation.",
"### Source Data",
"#### Initial Data Collection \n\n> The dataset consists of 1449 RGBD images, gathered from a wide range\nof commercial and residential buildings in three different US cities, comprising\n464 different indoor scenes across 26 scene classes.A dense per-pixel labeling was\nobtained for each image using Amazon Mechanical Turk.",
"### Annotations",
"#### Annotation process\n\nThis is an involved process. Interested readers are referred to Sections 2, 3, and 4 of the original paper.",
"#### Who are the annotators?\n\nAMT annotators.",
"### Personal and Sensitive Information",
"## Considerations for Using the Data",
"### Social Impact of Dataset",
"### Discussion of Biases",
"### Other Known Limitations",
"## Additional Information",
"### Dataset Curators\n\n* Original NYU Depth V2 dataset: Nathan Silberman, Derek Hoiem, Pushmeet Kohli, Rob Fergus\n* Preprocessed version: Diana Wofk, Fangchang Ma, Tien-Ju Yang, Sertac Karaman, Vivienne Sze",
"### Licensing Information\n\nThe preprocessed NYU Depth V2 dataset is licensed under a MIT License.",
"### Contributions\n\nThanks to @sayakpaul for adding this dataset."
] | [
"TAGS\n#task_categories-depth-estimation #multilinguality-monolingual #size_categories-10K<n<100K #language-English #license-apache-2.0 #depth-estimation #arxiv-1903.03273 #region-us \n",
"# Dataset Card for NYU Depth V2",
"## Table of Contents\n- Table of Contents\n- Dataset Description\n - Dataset Summary\n - Supported Tasks\n - Languages\n- Dataset Structure\n - Data Instances\n - Data Fields\n - Data Splits\n- Visualization\n- Dataset Creation\n - Curation Rationale\n - Source Data\n - Annotations\n - Personal and Sensitive Information\n- Considerations for Using the Data\n - Social Impact of Dataset\n - Discussion of Biases\n - Other Known Limitations\n- Additional Information\n - Dataset Curators\n - Licensing Information\n - Citation Information\n - Contributions",
"## Dataset Description\n\n- Homepage: NYU Depth Dataset V2 homepage\n- Repository: Fast Depth repository which was used to source the dataset in this repository. It is a preprocessed version of the original NYU Depth V2 dataset linked above. It is also used in TensorFlow Datasets.\n- Papers: Indoor Segmentation and Support Inference from RGBD Images and FastDepth: Fast Monocular Depth Estimation on Embedded Systems\n- Point of Contact: Nathan Silberman and Diana Wofk",
"### Dataset Summary\n\nAs per the dataset homepage:\n\nThe NYU-Depth V2 data set is comprised of video sequences from a variety of indoor scenes as recorded by both the RGB and Depth cameras from the Microsoft Kinect. It features:\n\n* 1449 densely labeled pairs of aligned RGB and depth images\n* 464 new scenes taken from 3 cities\n* 407,024 new unlabeled frames\n* Each object is labeled with a class and an instance number (cup1, cup2, cup3, etc)\n\nThe dataset has several components:\n\n* Labeled: A subset of the video data accompanied by dense multi-class labels. This data has also been preprocessed to fill in missing depth labels.\n* Raw: The raw rgb, depth and accelerometer data as provided by the Kinect.\n* Toolbox: Useful functions for manipulating the data and labels.",
"### Supported Tasks\n\n- 'depth-estimation': Depth estimation is the task of approximating the perceived depth of a given image. In other words, it's about measuring the distance of each image pixel from the camera.\n- 'semantic-segmentation': Semantic segmentation is the task of associating every pixel of an image to a class label. \n\nThere are other tasks supported by this dataset as well. You can find more about them by referring to this resource.",
"### Languages\n\nEnglish.",
"## Dataset Structure",
"### Data Instances\n\nA data point comprises an image and its annotation depth map for both the 'train' and 'validation' splits.",
"### Data Fields\n\n- 'image': A 'PIL.Image.Image' object containing the image. Note that when accessing the image column: 'dataset[0][\"image\"]' the image file is automatically decoded. Decoding of a large number of image files might take a significant amount of time. Thus it is important to first query the sample index before the '\"image\"' column, *i.e.* 'dataset[0][\"image\"]' should always be preferred over 'dataset[\"image\"][0]'.\n- 'depth_map': A 'PIL.Image.Image' object containing the annotation depth map.",
"### Data Splits\n\nThe data is split into training, and validation splits. The training data contains 47584 images, and the validation data contains 654 images.",
"## Visualization\n\nYou can use the following code snippet to visualize samples from the dataset:",
"## Dataset Creation",
"### Curation Rationale\n\nThe rationale from the paper that introduced the NYU Depth V2 dataset:\n\n> We present an approach to interpret the major surfaces, objects, and support relations of an indoor scene from an RGBD image. Most existing work ignores physical interactions or is applied only to tidy rooms and hallways. Our goal is to parse typical, often messy, indoor scenes into floor, walls, supporting surfaces, and object regions, and to recover support relationships. One of our main interests is to better understand how 3D cues can best inform a structured 3D interpretation.",
"### Source Data",
"#### Initial Data Collection \n\n> The dataset consists of 1449 RGBD images, gathered from a wide range\nof commercial and residential buildings in three different US cities, comprising\n464 different indoor scenes across 26 scene classes.A dense per-pixel labeling was\nobtained for each image using Amazon Mechanical Turk.",
"### Annotations",
"#### Annotation process\n\nThis is an involved process. Interested readers are referred to Sections 2, 3, and 4 of the original paper.",
"#### Who are the annotators?\n\nAMT annotators.",
"### Personal and Sensitive Information",
"## Considerations for Using the Data",
"### Social Impact of Dataset",
"### Discussion of Biases",
"### Other Known Limitations",
"## Additional Information",
"### Dataset Curators\n\n* Original NYU Depth V2 dataset: Nathan Silberman, Derek Hoiem, Pushmeet Kohli, Rob Fergus\n* Preprocessed version: Diana Wofk, Fangchang Ma, Tien-Ju Yang, Sertac Karaman, Vivienne Sze",
"### Licensing Information\n\nThe preprocessed NYU Depth V2 dataset is licensed under a MIT License.",
"### Contributions\n\nThanks to @sayakpaul for adding this dataset."
] | [
63,
12,
124,
132,
216,
115,
6,
6,
36,
156,
39,
22,
5,
139,
4,
75,
5,
31,
15,
8,
8,
7,
8,
7,
5,
67,
27,
18
] | [
"passage: TAGS\n#task_categories-depth-estimation #multilinguality-monolingual #size_categories-10K<n<100K #language-English #license-apache-2.0 #depth-estimation #arxiv-1903.03273 #region-us \n# Dataset Card for NYU Depth V2## Table of Contents\n- Table of Contents\n- Dataset Description\n - Dataset Summary\n - Supported Tasks\n - Languages\n- Dataset Structure\n - Data Instances\n - Data Fields\n - Data Splits\n- Visualization\n- Dataset Creation\n - Curation Rationale\n - Source Data\n - Annotations\n - Personal and Sensitive Information\n- Considerations for Using the Data\n - Social Impact of Dataset\n - Discussion of Biases\n - Other Known Limitations\n- Additional Information\n - Dataset Curators\n - Licensing Information\n - Citation Information\n - Contributions## Dataset Description\n\n- Homepage: NYU Depth Dataset V2 homepage\n- Repository: Fast Depth repository which was used to source the dataset in this repository. It is a preprocessed version of the original NYU Depth V2 dataset linked above. It is also used in TensorFlow Datasets.\n- Papers: Indoor Segmentation and Support Inference from RGBD Images and FastDepth: Fast Monocular Depth Estimation on Embedded Systems\n- Point of Contact: Nathan Silberman and Diana Wofk",
"passage: ### Dataset Summary\n\nAs per the dataset homepage:\n\nThe NYU-Depth V2 data set is comprised of video sequences from a variety of indoor scenes as recorded by both the RGB and Depth cameras from the Microsoft Kinect. It features:\n\n* 1449 densely labeled pairs of aligned RGB and depth images\n* 464 new scenes taken from 3 cities\n* 407,024 new unlabeled frames\n* Each object is labeled with a class and an instance number (cup1, cup2, cup3, etc)\n\nThe dataset has several components:\n\n* Labeled: A subset of the video data accompanied by dense multi-class labels. This data has also been preprocessed to fill in missing depth labels.\n* Raw: The raw rgb, depth and accelerometer data as provided by the Kinect.\n* Toolbox: Useful functions for manipulating the data and labels.### Supported Tasks\n\n- 'depth-estimation': Depth estimation is the task of approximating the perceived depth of a given image. In other words, it's about measuring the distance of each image pixel from the camera.\n- 'semantic-segmentation': Semantic segmentation is the task of associating every pixel of an image to a class label. \n\nThere are other tasks supported by this dataset as well. You can find more about them by referring to this resource.### Languages\n\nEnglish.## Dataset Structure### Data Instances\n\nA data point comprises an image and its annotation depth map for both the 'train' and 'validation' splits.### Data Fields\n\n- 'image': A 'PIL.Image.Image' object containing the image. Note that when accessing the image column: 'dataset[0][\"image\"]' the image file is automatically decoded. Decoding of a large number of image files might take a significant amount of time. Thus it is important to first query the sample index before the '\"image\"' column, *i.e.* 'dataset[0][\"image\"]' should always be preferred over 'dataset[\"image\"][0]'.\n- 'depth_map': A 'PIL.Image.Image' object containing the annotation depth map.### Data Splits\n\nThe data is split into training, and validation splits. The training data contains 47584 images, and the validation data contains 654 images.## Visualization\n\nYou can use the following code snippet to visualize samples from the dataset:## Dataset Creation"
] |
04fe748e33751339a4cd78f9015c585c31b3962b | # Dataset Card for "docvqa_dataset"
[More Information needed](https://github.com/huggingface/datasets/blob/main/CONTRIBUTING.md#how-to-contribute-to-the-dataset-cards) | Lokibabu/docvqa_dataset | [
"region:us"
] | 2022-11-22T12:34:00+00:00 | {"dataset_info": {"features": [{"name": "id", "dtype": "string"}, {"name": "ground_truth", "dtype": "string"}, {"name": "image", "dtype": "string"}, {"name": "data_split", "dtype": "string"}], "splits": [{"name": "train", "num_bytes": 2579, "num_examples": 4}, {"name": "test", "num_bytes": 2579, "num_examples": 4}], "download_size": 12068, "dataset_size": 5158}} | 2022-11-23T05:05:43+00:00 | [] | [] | TAGS
#region-us
| # Dataset Card for "docvqa_dataset"
More Information needed | [
"# Dataset Card for \"docvqa_dataset\"\n\nMore Information needed"
] | [
"TAGS\n#region-us \n",
"# Dataset Card for \"docvqa_dataset\"\n\nMore Information needed"
] | [
6,
16
] | [
"passage: TAGS\n#region-us \n# Dataset Card for \"docvqa_dataset\"\n\nMore Information needed"
] |
178ea56d53d9f17ef76df5ccd752be88ce7c74e0 |
# Dataset Card for SGD-X v1
- **Repository:** https://github.com/google-research-datasets/dstc8-schema-guided-dialogue/tree/master/sgd_x
- **Paper:** https://arxiv.org/pdf/2110.06800.pdf
- **Leaderboard:** None
- **Who transforms the dataset:** Qi Zhu(zhuq96 at gmail dot com)
To use this dataset, you need to install [ConvLab-3](https://github.com/ConvLab/ConvLab-3) platform first. Then you can load the dataset via:
```
from convlab.util import load_dataset, load_ontology, load_database
dataset = load_dataset('sgd1')
ontology = load_ontology('sgd1')
database = load_database('sgd1')
```
For more usage please refer to [here](https://github.com/ConvLab/ConvLab-3/tree/master/data/unified_datasets).
### Dataset Summary
The **Schema-Guided Dialogue (SGD)** dataset consists of over 20k annotated multi-domain, task-oriented conversations between a human and a virtual assistant. These conversations involve interactions with services and APIs spanning 20 domains, such as banks, events, media, calendar, travel, and weather. For most of these domains, the dataset contains multiple different APIs, many of which have overlapping functionalities but different interfaces, which reflects common real-world scenarios. The wide range of available annotations can be used for intent prediction, slot filling, dialogue state tracking, policy imitation learning, language generation, and user simulation learning, among other tasks for developing large-scale virtual assistants. Additionally, the dataset contains unseen domains and services in the evaluation set to quantify the performance in zero-shot or few-shot settings.
The **SGD-X** dataset consists of 5 linguistic variants of every schema in the original SGD dataset. Linguistic variants were written by hundreds of paid crowd-workers. In the SGD-X directory, v1 represents the variant closest to the original schemas and v5 the farthest in terms of linguistic distance. To evaluate model performance on SGD-X schemas, dialogues must be converted using the script generate_sgdx_dialogues.py.
- **How to get the transformed data from original data:**
- Download [dstc8-schema-guided-dialogue-master.zip](https://github.com/google-research-datasets/dstc8-schema-guided-dialogue/archive/refs/heads/master.zip).
- Modified `sgd_x/generate_sgdx_dialogues.py` as https://github.com/google-research-datasets/dstc8-schema-guided-dialogue/issues/57
- Run `python -m sgd_x.generate_sgdx_dialogues` under `dstc8-schema-guided-dialogue-master` dir which need tensorflow installed.
- Run `python preprocess.py` in the current directory.
- **Main changes of the transformation:**
- Lower case original `act` as `intent`.
- Add `count` slot for each domain, non-categorical, find span by text matching.
- Categorize `dialogue acts` according to the `intent`.
- Concatenate multiple values using `|`.
- Retain `active_intent`, `requested_slots`, `service_call`.
- **Annotations:**
- dialogue acts, state, db_results, service_call, active_intent, requested_slots.
### Supported Tasks and Leaderboards
NLU, DST, Policy, NLG, E2E
### Languages
English
### Data Splits
| split | dialogues | utterances | avg_utt | avg_tokens | avg_domains | cat slot match(state) | cat slot match(goal) | cat slot match(dialogue act) | non-cat slot span(dialogue act) |
|------------|-------------|--------------|-----------|--------------|---------------|-------------------------|------------------------|--------------------------------|-----------------------------------|
| train | 16142 | 329964 | 20.44 | 9.75 | 1.84 | 100 | - | 100 | 100 |
| validation | 2482 | 48726 | 19.63 | 9.66 | 1.84 | 100 | - | 100 | 100 |
| test | 4201 | 84594 | 20.14 | 10.4 | 2.02 | 100 | - | 100 | 100 |
| all | 22825 | 463284 | 20.3 | 9.86 | 1.87 | 100 | - | 100 | 100 |
45 domains: ['Banks_11', 'Buses_11', 'Buses_21', 'Calendar_11', 'Events_11', 'Events_21', 'Flights_11', 'Flights_21', 'Homes_11', 'Hotels_11', 'Hotels_21', 'Hotels_31', 'Media_11', 'Movies_11', 'Music_11', 'Music_21', 'RentalCars_11', 'RentalCars_21', 'Restaurants_11', 'RideSharing_11', 'RideSharing_21', 'Services_11', 'Services_21', 'Services_31', 'Travel_11', 'Weather_11', 'Alarm_11', 'Banks_21', 'Flights_31', 'Hotels_41', 'Media_21', 'Movies_21', 'Restaurants_21', 'Services_41', 'Buses_31', 'Events_31', 'Flights_41', 'Homes_21', 'Media_31', 'Messaging_11', 'Movies_31', 'Music_31', 'Payment_11', 'RentalCars_31', 'Trains_11']
- **cat slot match**: how many values of categorical slots are in the possible values of ontology in percentage.
- **non-cat slot span**: how many values of non-categorical slots have span annotation in percentage.
### Citation
```
@inproceedings{lee2022sgd,
title={SGD-X: A Benchmark for Robust Generalization in Schema-Guided Dialogue Systems},
author={Lee, Harrison and Gupta, Raghav and Rastogi, Abhinav and Cao, Yuan and Zhang, Bin and Wu, Yonghui},
booktitle={Proceedings of the AAAI Conference on Artificial Intelligence},
volume={36},
number={10},
pages={10938--10946},
year={2022}
}
```
### Licensing Information
[**CC BY-SA 4.0**](https://creativecommons.org/licenses/by-sa/4.0/) | ConvLab/sgd1 | [
"task_categories:conversational",
"multilinguality:monolingual",
"size_categories:10K<n<100K",
"language:en",
"license:cc-by-sa-4.0",
"arxiv:2110.06800",
"region:us"
] | 2022-11-22T12:37:54+00:00 | {"language": ["en"], "license": ["cc-by-sa-4.0"], "multilinguality": ["monolingual"], "size_categories": ["10K<n<100K"], "task_categories": ["conversational"], "pretty_name": "SGD-X v1"} | 2022-11-25T08:53:59+00:00 | [
"2110.06800"
] | [
"en"
] | TAGS
#task_categories-conversational #multilinguality-monolingual #size_categories-10K<n<100K #language-English #license-cc-by-sa-4.0 #arxiv-2110.06800 #region-us
| Dataset Card for SGD-X v1
=========================
* Repository: URL
* Paper: URL
* Leaderboard: None
* Who transforms the dataset: Qi Zhu(zhuq96 at gmail dot com)
To use this dataset, you need to install ConvLab-3 platform first. Then you can load the dataset via:
For more usage please refer to here.
### Dataset Summary
The Schema-Guided Dialogue (SGD) dataset consists of over 20k annotated multi-domain, task-oriented conversations between a human and a virtual assistant. These conversations involve interactions with services and APIs spanning 20 domains, such as banks, events, media, calendar, travel, and weather. For most of these domains, the dataset contains multiple different APIs, many of which have overlapping functionalities but different interfaces, which reflects common real-world scenarios. The wide range of available annotations can be used for intent prediction, slot filling, dialogue state tracking, policy imitation learning, language generation, and user simulation learning, among other tasks for developing large-scale virtual assistants. Additionally, the dataset contains unseen domains and services in the evaluation set to quantify the performance in zero-shot or few-shot settings.
The SGD-X dataset consists of 5 linguistic variants of every schema in the original SGD dataset. Linguistic variants were written by hundreds of paid crowd-workers. In the SGD-X directory, v1 represents the variant closest to the original schemas and v5 the farthest in terms of linguistic distance. To evaluate model performance on SGD-X schemas, dialogues must be converted using the script generate\_sgdx\_dialogues.py.
* How to get the transformed data from original data:
+ Download URL.
+ Modified 'sgd\_x/generate\_sgdx\_dialogues.py' as URL
+ Run 'python -m sgd\_x.generate\_sgdx\_dialogues' under 'dstc8-schema-guided-dialogue-master' dir which need tensorflow installed.
+ Run 'python URL' in the current directory.
* Main changes of the transformation:
+ Lower case original 'act' as 'intent'.
+ Add 'count' slot for each domain, non-categorical, find span by text matching.
+ Categorize 'dialogue acts' according to the 'intent'.
+ Concatenate multiple values using '|'.
+ Retain 'active\_intent', 'requested\_slots', 'service\_call'.
* Annotations:
+ dialogue acts, state, db\_results, service\_call, active\_intent, requested\_slots.
### Supported Tasks and Leaderboards
NLU, DST, Policy, NLG, E2E
### Languages
English
### Data Splits
45 domains: ['Banks\_11', 'Buses\_11', 'Buses\_21', 'Calendar\_11', 'Events\_11', 'Events\_21', 'Flights\_11', 'Flights\_21', 'Homes\_11', 'Hotels\_11', 'Hotels\_21', 'Hotels\_31', 'Media\_11', 'Movies\_11', 'Music\_11', 'Music\_21', 'RentalCars\_11', 'RentalCars\_21', 'Restaurants\_11', 'RideSharing\_11', 'RideSharing\_21', 'Services\_11', 'Services\_21', 'Services\_31', 'Travel\_11', 'Weather\_11', 'Alarm\_11', 'Banks\_21', 'Flights\_31', 'Hotels\_41', 'Media\_21', 'Movies\_21', 'Restaurants\_21', 'Services\_41', 'Buses\_31', 'Events\_31', 'Flights\_41', 'Homes\_21', 'Media\_31', 'Messaging\_11', 'Movies\_31', 'Music\_31', 'Payment\_11', 'RentalCars\_31', 'Trains\_11']
* cat slot match: how many values of categorical slots are in the possible values of ontology in percentage.
* non-cat slot span: how many values of non-categorical slots have span annotation in percentage.
### Licensing Information
CC BY-SA 4.0
| [
"### Dataset Summary\n\n\nThe Schema-Guided Dialogue (SGD) dataset consists of over 20k annotated multi-domain, task-oriented conversations between a human and a virtual assistant. These conversations involve interactions with services and APIs spanning 20 domains, such as banks, events, media, calendar, travel, and weather. For most of these domains, the dataset contains multiple different APIs, many of which have overlapping functionalities but different interfaces, which reflects common real-world scenarios. The wide range of available annotations can be used for intent prediction, slot filling, dialogue state tracking, policy imitation learning, language generation, and user simulation learning, among other tasks for developing large-scale virtual assistants. Additionally, the dataset contains unseen domains and services in the evaluation set to quantify the performance in zero-shot or few-shot settings.\n\n\nThe SGD-X dataset consists of 5 linguistic variants of every schema in the original SGD dataset. Linguistic variants were written by hundreds of paid crowd-workers. In the SGD-X directory, v1 represents the variant closest to the original schemas and v5 the farthest in terms of linguistic distance. To evaluate model performance on SGD-X schemas, dialogues must be converted using the script generate\\_sgdx\\_dialogues.py.\n\n\n* How to get the transformed data from original data:\n\t+ Download URL.\n\t+ Modified 'sgd\\_x/generate\\_sgdx\\_dialogues.py' as URL\n\t+ Run 'python -m sgd\\_x.generate\\_sgdx\\_dialogues' under 'dstc8-schema-guided-dialogue-master' dir which need tensorflow installed.\n\t+ Run 'python URL' in the current directory.\n* Main changes of the transformation:\n\t+ Lower case original 'act' as 'intent'.\n\t+ Add 'count' slot for each domain, non-categorical, find span by text matching.\n\t+ Categorize 'dialogue acts' according to the 'intent'.\n\t+ Concatenate multiple values using '|'.\n\t+ Retain 'active\\_intent', 'requested\\_slots', 'service\\_call'.\n* Annotations:\n\t+ dialogue acts, state, db\\_results, service\\_call, active\\_intent, requested\\_slots.",
"### Supported Tasks and Leaderboards\n\n\nNLU, DST, Policy, NLG, E2E",
"### Languages\n\n\nEnglish",
"### Data Splits\n\n\n\n45 domains: ['Banks\\_11', 'Buses\\_11', 'Buses\\_21', 'Calendar\\_11', 'Events\\_11', 'Events\\_21', 'Flights\\_11', 'Flights\\_21', 'Homes\\_11', 'Hotels\\_11', 'Hotels\\_21', 'Hotels\\_31', 'Media\\_11', 'Movies\\_11', 'Music\\_11', 'Music\\_21', 'RentalCars\\_11', 'RentalCars\\_21', 'Restaurants\\_11', 'RideSharing\\_11', 'RideSharing\\_21', 'Services\\_11', 'Services\\_21', 'Services\\_31', 'Travel\\_11', 'Weather\\_11', 'Alarm\\_11', 'Banks\\_21', 'Flights\\_31', 'Hotels\\_41', 'Media\\_21', 'Movies\\_21', 'Restaurants\\_21', 'Services\\_41', 'Buses\\_31', 'Events\\_31', 'Flights\\_41', 'Homes\\_21', 'Media\\_31', 'Messaging\\_11', 'Movies\\_31', 'Music\\_31', 'Payment\\_11', 'RentalCars\\_31', 'Trains\\_11']\n\n\n* cat slot match: how many values of categorical slots are in the possible values of ontology in percentage.\n* non-cat slot span: how many values of non-categorical slots have span annotation in percentage.",
"### Licensing Information\n\n\nCC BY-SA 4.0"
] | [
"TAGS\n#task_categories-conversational #multilinguality-monolingual #size_categories-10K<n<100K #language-English #license-cc-by-sa-4.0 #arxiv-2110.06800 #region-us \n",
"### Dataset Summary\n\n\nThe Schema-Guided Dialogue (SGD) dataset consists of over 20k annotated multi-domain, task-oriented conversations between a human and a virtual assistant. These conversations involve interactions with services and APIs spanning 20 domains, such as banks, events, media, calendar, travel, and weather. For most of these domains, the dataset contains multiple different APIs, many of which have overlapping functionalities but different interfaces, which reflects common real-world scenarios. The wide range of available annotations can be used for intent prediction, slot filling, dialogue state tracking, policy imitation learning, language generation, and user simulation learning, among other tasks for developing large-scale virtual assistants. Additionally, the dataset contains unseen domains and services in the evaluation set to quantify the performance in zero-shot or few-shot settings.\n\n\nThe SGD-X dataset consists of 5 linguistic variants of every schema in the original SGD dataset. Linguistic variants were written by hundreds of paid crowd-workers. In the SGD-X directory, v1 represents the variant closest to the original schemas and v5 the farthest in terms of linguistic distance. To evaluate model performance on SGD-X schemas, dialogues must be converted using the script generate\\_sgdx\\_dialogues.py.\n\n\n* How to get the transformed data from original data:\n\t+ Download URL.\n\t+ Modified 'sgd\\_x/generate\\_sgdx\\_dialogues.py' as URL\n\t+ Run 'python -m sgd\\_x.generate\\_sgdx\\_dialogues' under 'dstc8-schema-guided-dialogue-master' dir which need tensorflow installed.\n\t+ Run 'python URL' in the current directory.\n* Main changes of the transformation:\n\t+ Lower case original 'act' as 'intent'.\n\t+ Add 'count' slot for each domain, non-categorical, find span by text matching.\n\t+ Categorize 'dialogue acts' according to the 'intent'.\n\t+ Concatenate multiple values using '|'.\n\t+ Retain 'active\\_intent', 'requested\\_slots', 'service\\_call'.\n* Annotations:\n\t+ dialogue acts, state, db\\_results, service\\_call, active\\_intent, requested\\_slots.",
"### Supported Tasks and Leaderboards\n\n\nNLU, DST, Policy, NLG, E2E",
"### Languages\n\n\nEnglish",
"### Data Splits\n\n\n\n45 domains: ['Banks\\_11', 'Buses\\_11', 'Buses\\_21', 'Calendar\\_11', 'Events\\_11', 'Events\\_21', 'Flights\\_11', 'Flights\\_21', 'Homes\\_11', 'Hotels\\_11', 'Hotels\\_21', 'Hotels\\_31', 'Media\\_11', 'Movies\\_11', 'Music\\_11', 'Music\\_21', 'RentalCars\\_11', 'RentalCars\\_21', 'Restaurants\\_11', 'RideSharing\\_11', 'RideSharing\\_21', 'Services\\_11', 'Services\\_21', 'Services\\_31', 'Travel\\_11', 'Weather\\_11', 'Alarm\\_11', 'Banks\\_21', 'Flights\\_31', 'Hotels\\_41', 'Media\\_21', 'Movies\\_21', 'Restaurants\\_21', 'Services\\_41', 'Buses\\_31', 'Events\\_31', 'Flights\\_41', 'Homes\\_21', 'Media\\_31', 'Messaging\\_11', 'Movies\\_31', 'Music\\_31', 'Payment\\_11', 'RentalCars\\_31', 'Trains\\_11']\n\n\n* cat slot match: how many values of categorical slots are in the possible values of ontology in percentage.\n* non-cat slot span: how many values of non-categorical slots have span annotation in percentage.",
"### Licensing Information\n\n\nCC BY-SA 4.0"
] | [
60,
582,
24,
5,
431,
11
] | [
"passage: TAGS\n#task_categories-conversational #multilinguality-monolingual #size_categories-10K<n<100K #language-English #license-cc-by-sa-4.0 #arxiv-2110.06800 #region-us \n"
] |
8d1e54efae8a5adcf75e3de29d4d512f5f45a821 |
# Dataset Card for SGD-X v2
- **Repository:** https://github.com/google-research-datasets/dstc8-schema-guided-dialogue/tree/master/sgd_x
- **Paper:** https://arxiv.org/pdf/2110.06800.pdf
- **Leaderboard:** None
- **Who transforms the dataset:** Qi Zhu(zhuq96 at gmail dot com)
To use this dataset, you need to install [ConvLab-3](https://github.com/ConvLab/ConvLab-3) platform first. Then you can load the dataset via:
```
from convlab.util import load_dataset, load_ontology, load_database
dataset = load_dataset('sgd2')
ontology = load_ontology('sgd2')
database = load_database('sgd2')
```
For more usage please refer to [here](https://github.com/ConvLab/ConvLab-3/tree/master/data/unified_datasets).
### Dataset Summary
The **Schema-Guided Dialogue (SGD)** dataset consists of over 20k annotated multi-domain, task-oriented conversations between a human and a virtual assistant. These conversations involve interactions with services and APIs spanning 20 domains, such as banks, events, media, calendar, travel, and weather. For most of these domains, the dataset contains multiple different APIs, many of which have overlapping functionalities but different interfaces, which reflects common real-world scenarios. The wide range of available annotations can be used for intent prediction, slot filling, dialogue state tracking, policy imitation learning, language generation, and user simulation learning, among other tasks for developing large-scale virtual assistants. Additionally, the dataset contains unseen domains and services in the evaluation set to quantify the performance in zero-shot or few-shot settings.
The **SGD-X** dataset consists of 5 linguistic variants of every schema in the original SGD dataset. Linguistic variants were written by hundreds of paid crowd-workers. In the SGD-X directory, v1 represents the variant closest to the original schemas and v5 the farthest in terms of linguistic distance. To evaluate model performance on SGD-X schemas, dialogues must be converted using the script generate_sgdx_dialogues.py.
- **How to get the transformed data from original data:**
- Download [dstc8-schema-guided-dialogue-master.zip](https://github.com/google-research-datasets/dstc8-schema-guided-dialogue/archive/refs/heads/master.zip).
- Modified `sgd_x/generate_sgdx_dialogues.py` as https://github.com/google-research-datasets/dstc8-schema-guided-dialogue/issues/57
- Run `python -m sgd_x.generate_sgdx_dialogues` under `dstc8-schema-guided-dialogue-master` dir which need tensorflow installed.
- Run `python preprocess.py` in the current directory.
- **Main changes of the transformation:**
- Lower case original `act` as `intent`.
- Add `count` slot for each domain, non-categorical, find span by text matching.
- Categorize `dialogue acts` according to the `intent`.
- Concatenate multiple values using `|`.
- Retain `active_intent`, `requested_slots`, `service_call`.
- **Annotations:**
- dialogue acts, state, db_results, service_call, active_intent, requested_slots.
### Supported Tasks and Leaderboards
NLU, DST, Policy, NLG, E2E
### Languages
English
### Data Splits
| split | dialogues | utterances | avg_utt | avg_tokens | avg_domains | cat slot match(state) | cat slot match(goal) | cat slot match(dialogue act) | non-cat slot span(dialogue act) |
|------------|-------------|--------------|-----------|--------------|---------------|-------------------------|------------------------|--------------------------------|-----------------------------------|
| train | 16142 | 329964 | 20.44 | 9.75 | 1.84 | 100 | - | 100 | 100 |
| validation | 2482 | 48726 | 19.63 | 9.66 | 1.84 | 100 | - | 100 | 100 |
| test | 4201 | 84594 | 20.14 | 10.4 | 2.02 | 100 | - | 100 | 100 |
| all | 22825 | 463284 | 20.3 | 9.86 | 1.87 | 100 | - | 100 | 100 |
45 domains: ['Banks_12', 'Buses_12', 'Buses_22', 'Calendar_12', 'Events_12', 'Events_22', 'Flights_12', 'Flights_22', 'Homes_12', 'Hotels_12', 'Hotels_22', 'Hotels_32', 'Media_12', 'Movies_12', 'Music_12', 'Music_22', 'RentalCars_12', 'RentalCars_22', 'Restaurants_12', 'RideSharing_12', 'RideSharing_22', 'Services_12', 'Services_22', 'Services_32', 'Travel_12', 'Weather_12', 'Alarm_12', 'Banks_22', 'Flights_32', 'Hotels_42', 'Media_22', 'Movies_22', 'Restaurants_22', 'Services_42', 'Buses_32', 'Events_32', 'Flights_42', 'Homes_22', 'Media_32', 'Messaging_12', 'Movies_32', 'Music_32', 'Payment_12', 'RentalCars_32', 'Trains_12']
- **cat slot match**: how many values of categorical slots are in the possible values of ontology in percentage.
- **non-cat slot span**: how many values of non-categorical slots have span annotation in percentage.
### Citation
```
@inproceedings{lee2022sgd,
title={SGD-X: A Benchmark for Robust Generalization in Schema-Guided Dialogue Systems},
author={Lee, Harrison and Gupta, Raghav and Rastogi, Abhinav and Cao, Yuan and Zhang, Bin and Wu, Yonghui},
booktitle={Proceedings of the AAAI Conference on Artificial Intelligence},
volume={36},
number={10},
pages={10938--10946},
year={2022}
}
```
### Licensing Information
[**CC BY-SA 4.0**](https://creativecommons.org/licenses/by-sa/4.0/) | ConvLab/sgd2 | [
"task_categories:conversational",
"multilinguality:monolingual",
"size_categories:10K<n<100K",
"language:en",
"license:cc-by-sa-4.0",
"arxiv:2110.06800",
"region:us"
] | 2022-11-22T12:41:47+00:00 | {"language": ["en"], "license": ["cc-by-sa-4.0"], "multilinguality": ["monolingual"], "size_categories": ["10K<n<100K"], "task_categories": ["conversational"], "pretty_name": "SGD-X v2"} | 2022-11-25T08:53:46+00:00 | [
"2110.06800"
] | [
"en"
] | TAGS
#task_categories-conversational #multilinguality-monolingual #size_categories-10K<n<100K #language-English #license-cc-by-sa-4.0 #arxiv-2110.06800 #region-us
| Dataset Card for SGD-X v2
=========================
* Repository: URL
* Paper: URL
* Leaderboard: None
* Who transforms the dataset: Qi Zhu(zhuq96 at gmail dot com)
To use this dataset, you need to install ConvLab-3 platform first. Then you can load the dataset via:
For more usage please refer to here.
### Dataset Summary
The Schema-Guided Dialogue (SGD) dataset consists of over 20k annotated multi-domain, task-oriented conversations between a human and a virtual assistant. These conversations involve interactions with services and APIs spanning 20 domains, such as banks, events, media, calendar, travel, and weather. For most of these domains, the dataset contains multiple different APIs, many of which have overlapping functionalities but different interfaces, which reflects common real-world scenarios. The wide range of available annotations can be used for intent prediction, slot filling, dialogue state tracking, policy imitation learning, language generation, and user simulation learning, among other tasks for developing large-scale virtual assistants. Additionally, the dataset contains unseen domains and services in the evaluation set to quantify the performance in zero-shot or few-shot settings.
The SGD-X dataset consists of 5 linguistic variants of every schema in the original SGD dataset. Linguistic variants were written by hundreds of paid crowd-workers. In the SGD-X directory, v1 represents the variant closest to the original schemas and v5 the farthest in terms of linguistic distance. To evaluate model performance on SGD-X schemas, dialogues must be converted using the script generate\_sgdx\_dialogues.py.
* How to get the transformed data from original data:
+ Download URL.
+ Modified 'sgd\_x/generate\_sgdx\_dialogues.py' as URL
+ Run 'python -m sgd\_x.generate\_sgdx\_dialogues' under 'dstc8-schema-guided-dialogue-master' dir which need tensorflow installed.
+ Run 'python URL' in the current directory.
* Main changes of the transformation:
+ Lower case original 'act' as 'intent'.
+ Add 'count' slot for each domain, non-categorical, find span by text matching.
+ Categorize 'dialogue acts' according to the 'intent'.
+ Concatenate multiple values using '|'.
+ Retain 'active\_intent', 'requested\_slots', 'service\_call'.
* Annotations:
+ dialogue acts, state, db\_results, service\_call, active\_intent, requested\_slots.
### Supported Tasks and Leaderboards
NLU, DST, Policy, NLG, E2E
### Languages
English
### Data Splits
45 domains: ['Banks\_12', 'Buses\_12', 'Buses\_22', 'Calendar\_12', 'Events\_12', 'Events\_22', 'Flights\_12', 'Flights\_22', 'Homes\_12', 'Hotels\_12', 'Hotels\_22', 'Hotels\_32', 'Media\_12', 'Movies\_12', 'Music\_12', 'Music\_22', 'RentalCars\_12', 'RentalCars\_22', 'Restaurants\_12', 'RideSharing\_12', 'RideSharing\_22', 'Services\_12', 'Services\_22', 'Services\_32', 'Travel\_12', 'Weather\_12', 'Alarm\_12', 'Banks\_22', 'Flights\_32', 'Hotels\_42', 'Media\_22', 'Movies\_22', 'Restaurants\_22', 'Services\_42', 'Buses\_32', 'Events\_32', 'Flights\_42', 'Homes\_22', 'Media\_32', 'Messaging\_12', 'Movies\_32', 'Music\_32', 'Payment\_12', 'RentalCars\_32', 'Trains\_12']
* cat slot match: how many values of categorical slots are in the possible values of ontology in percentage.
* non-cat slot span: how many values of non-categorical slots have span annotation in percentage.
### Licensing Information
CC BY-SA 4.0
| [
"### Dataset Summary\n\n\nThe Schema-Guided Dialogue (SGD) dataset consists of over 20k annotated multi-domain, task-oriented conversations between a human and a virtual assistant. These conversations involve interactions with services and APIs spanning 20 domains, such as banks, events, media, calendar, travel, and weather. For most of these domains, the dataset contains multiple different APIs, many of which have overlapping functionalities but different interfaces, which reflects common real-world scenarios. The wide range of available annotations can be used for intent prediction, slot filling, dialogue state tracking, policy imitation learning, language generation, and user simulation learning, among other tasks for developing large-scale virtual assistants. Additionally, the dataset contains unseen domains and services in the evaluation set to quantify the performance in zero-shot or few-shot settings.\n\n\nThe SGD-X dataset consists of 5 linguistic variants of every schema in the original SGD dataset. Linguistic variants were written by hundreds of paid crowd-workers. In the SGD-X directory, v1 represents the variant closest to the original schemas and v5 the farthest in terms of linguistic distance. To evaluate model performance on SGD-X schemas, dialogues must be converted using the script generate\\_sgdx\\_dialogues.py.\n\n\n* How to get the transformed data from original data:\n\t+ Download URL.\n\t+ Modified 'sgd\\_x/generate\\_sgdx\\_dialogues.py' as URL\n\t+ Run 'python -m sgd\\_x.generate\\_sgdx\\_dialogues' under 'dstc8-schema-guided-dialogue-master' dir which need tensorflow installed.\n\t+ Run 'python URL' in the current directory.\n* Main changes of the transformation:\n\t+ Lower case original 'act' as 'intent'.\n\t+ Add 'count' slot for each domain, non-categorical, find span by text matching.\n\t+ Categorize 'dialogue acts' according to the 'intent'.\n\t+ Concatenate multiple values using '|'.\n\t+ Retain 'active\\_intent', 'requested\\_slots', 'service\\_call'.\n* Annotations:\n\t+ dialogue acts, state, db\\_results, service\\_call, active\\_intent, requested\\_slots.",
"### Supported Tasks and Leaderboards\n\n\nNLU, DST, Policy, NLG, E2E",
"### Languages\n\n\nEnglish",
"### Data Splits\n\n\n\n45 domains: ['Banks\\_12', 'Buses\\_12', 'Buses\\_22', 'Calendar\\_12', 'Events\\_12', 'Events\\_22', 'Flights\\_12', 'Flights\\_22', 'Homes\\_12', 'Hotels\\_12', 'Hotels\\_22', 'Hotels\\_32', 'Media\\_12', 'Movies\\_12', 'Music\\_12', 'Music\\_22', 'RentalCars\\_12', 'RentalCars\\_22', 'Restaurants\\_12', 'RideSharing\\_12', 'RideSharing\\_22', 'Services\\_12', 'Services\\_22', 'Services\\_32', 'Travel\\_12', 'Weather\\_12', 'Alarm\\_12', 'Banks\\_22', 'Flights\\_32', 'Hotels\\_42', 'Media\\_22', 'Movies\\_22', 'Restaurants\\_22', 'Services\\_42', 'Buses\\_32', 'Events\\_32', 'Flights\\_42', 'Homes\\_22', 'Media\\_32', 'Messaging\\_12', 'Movies\\_32', 'Music\\_32', 'Payment\\_12', 'RentalCars\\_32', 'Trains\\_12']\n\n\n* cat slot match: how many values of categorical slots are in the possible values of ontology in percentage.\n* non-cat slot span: how many values of non-categorical slots have span annotation in percentage.",
"### Licensing Information\n\n\nCC BY-SA 4.0"
] | [
"TAGS\n#task_categories-conversational #multilinguality-monolingual #size_categories-10K<n<100K #language-English #license-cc-by-sa-4.0 #arxiv-2110.06800 #region-us \n",
"### Dataset Summary\n\n\nThe Schema-Guided Dialogue (SGD) dataset consists of over 20k annotated multi-domain, task-oriented conversations between a human and a virtual assistant. These conversations involve interactions with services and APIs spanning 20 domains, such as banks, events, media, calendar, travel, and weather. For most of these domains, the dataset contains multiple different APIs, many of which have overlapping functionalities but different interfaces, which reflects common real-world scenarios. The wide range of available annotations can be used for intent prediction, slot filling, dialogue state tracking, policy imitation learning, language generation, and user simulation learning, among other tasks for developing large-scale virtual assistants. Additionally, the dataset contains unseen domains and services in the evaluation set to quantify the performance in zero-shot or few-shot settings.\n\n\nThe SGD-X dataset consists of 5 linguistic variants of every schema in the original SGD dataset. Linguistic variants were written by hundreds of paid crowd-workers. In the SGD-X directory, v1 represents the variant closest to the original schemas and v5 the farthest in terms of linguistic distance. To evaluate model performance on SGD-X schemas, dialogues must be converted using the script generate\\_sgdx\\_dialogues.py.\n\n\n* How to get the transformed data from original data:\n\t+ Download URL.\n\t+ Modified 'sgd\\_x/generate\\_sgdx\\_dialogues.py' as URL\n\t+ Run 'python -m sgd\\_x.generate\\_sgdx\\_dialogues' under 'dstc8-schema-guided-dialogue-master' dir which need tensorflow installed.\n\t+ Run 'python URL' in the current directory.\n* Main changes of the transformation:\n\t+ Lower case original 'act' as 'intent'.\n\t+ Add 'count' slot for each domain, non-categorical, find span by text matching.\n\t+ Categorize 'dialogue acts' according to the 'intent'.\n\t+ Concatenate multiple values using '|'.\n\t+ Retain 'active\\_intent', 'requested\\_slots', 'service\\_call'.\n* Annotations:\n\t+ dialogue acts, state, db\\_results, service\\_call, active\\_intent, requested\\_slots.",
"### Supported Tasks and Leaderboards\n\n\nNLU, DST, Policy, NLG, E2E",
"### Languages\n\n\nEnglish",
"### Data Splits\n\n\n\n45 domains: ['Banks\\_12', 'Buses\\_12', 'Buses\\_22', 'Calendar\\_12', 'Events\\_12', 'Events\\_22', 'Flights\\_12', 'Flights\\_22', 'Homes\\_12', 'Hotels\\_12', 'Hotels\\_22', 'Hotels\\_32', 'Media\\_12', 'Movies\\_12', 'Music\\_12', 'Music\\_22', 'RentalCars\\_12', 'RentalCars\\_22', 'Restaurants\\_12', 'RideSharing\\_12', 'RideSharing\\_22', 'Services\\_12', 'Services\\_22', 'Services\\_32', 'Travel\\_12', 'Weather\\_12', 'Alarm\\_12', 'Banks\\_22', 'Flights\\_32', 'Hotels\\_42', 'Media\\_22', 'Movies\\_22', 'Restaurants\\_22', 'Services\\_42', 'Buses\\_32', 'Events\\_32', 'Flights\\_42', 'Homes\\_22', 'Media\\_32', 'Messaging\\_12', 'Movies\\_32', 'Music\\_32', 'Payment\\_12', 'RentalCars\\_32', 'Trains\\_12']\n\n\n* cat slot match: how many values of categorical slots are in the possible values of ontology in percentage.\n* non-cat slot span: how many values of non-categorical slots have span annotation in percentage.",
"### Licensing Information\n\n\nCC BY-SA 4.0"
] | [
60,
582,
24,
5,
431,
11
] | [
"passage: TAGS\n#task_categories-conversational #multilinguality-monolingual #size_categories-10K<n<100K #language-English #license-cc-by-sa-4.0 #arxiv-2110.06800 #region-us \n"
] |
582068e58b58287e3246a7b1402f4580fbabe5fe | # Dataset Card for "vietnamese-wiki-segmented"
[More Information needed](https://github.com/huggingface/datasets/blob/main/CONTRIBUTING.md#how-to-contribute-to-the-dataset-cards) | EddieChen372/vietnamese-wiki-segmented | [
"region:us"
] | 2022-11-22T12:43:48+00:00 | {"dataset_info": {"features": [{"name": "segmented_text", "dtype": "string"}, {"name": "segmented_title", "dtype": "string"}], "splits": [{"name": "train", "num_bytes": 1302569257, "num_examples": 1273469}], "download_size": 604393096, "dataset_size": 1302569257}} | 2022-11-22T12:45:58+00:00 | [] | [] | TAGS
#region-us
| # Dataset Card for "vietnamese-wiki-segmented"
More Information needed | [
"# Dataset Card for \"vietnamese-wiki-segmented\"\n\nMore Information needed"
] | [
"TAGS\n#region-us \n",
"# Dataset Card for \"vietnamese-wiki-segmented\"\n\nMore Information needed"
] | [
6,
19
] | [
"passage: TAGS\n#region-us \n# Dataset Card for \"vietnamese-wiki-segmented\"\n\nMore Information needed"
] |
0e884c21027f7cd35489d8b559ecedc7b42e1859 |
# Dataset Card for SGD-X v3
- **Repository:** https://github.com/google-research-datasets/dstc8-schema-guided-dialogue/tree/master/sgd_x
- **Paper:** https://arxiv.org/pdf/2110.06800.pdf
- **Leaderboard:** None
- **Who transforms the dataset:** Qi Zhu(zhuq96 at gmail dot com)
To use this dataset, you need to install [ConvLab-3](https://github.com/ConvLab/ConvLab-3) platform first. Then you can load the dataset via:
```
from convlab.util import load_dataset, load_ontology, load_database
dataset = load_dataset('sgd3')
ontology = load_ontology('sgd3')
database = load_database('sgd3')
```
For more usage please refer to [here](https://github.com/ConvLab/ConvLab-3/tree/master/data/unified_datasets).
### Dataset Summary
The **Schema-Guided Dialogue (SGD)** dataset consists of over 20k annotated multi-domain, task-oriented conversations between a human and a virtual assistant. These conversations involve interactions with services and APIs spanning 20 domains, such as banks, events, media, calendar, travel, and weather. For most of these domains, the dataset contains multiple different APIs, many of which have overlapping functionalities but different interfaces, which reflects common real-world scenarios. The wide range of available annotations can be used for intent prediction, slot filling, dialogue state tracking, policy imitation learning, language generation, and user simulation learning, among other tasks for developing large-scale virtual assistants. Additionally, the dataset contains unseen domains and services in the evaluation set to quantify the performance in zero-shot or few-shot settings.
The **SGD-X** dataset consists of 5 linguistic variants of every schema in the original SGD dataset. Linguistic variants were written by hundreds of paid crowd-workers. In the SGD-X directory, v1 represents the variant closest to the original schemas and v5 the farthest in terms of linguistic distance. To evaluate model performance on SGD-X schemas, dialogues must be converted using the script generate_sgdx_dialogues.py.
- **How to get the transformed data from original data:**
- Download [dstc8-schema-guided-dialogue-master.zip](https://github.com/google-research-datasets/dstc8-schema-guided-dialogue/archive/refs/heads/master.zip).
- Modified `sgd_x/generate_sgdx_dialogues.py` as https://github.com/google-research-datasets/dstc8-schema-guided-dialogue/issues/57
- Run `python -m sgd_x.generate_sgdx_dialogues` under `dstc8-schema-guided-dialogue-master` dir which need tensorflow installed.
- Run `python preprocess.py` in the current directory.
- **Main changes of the transformation:**
- Lower case original `act` as `intent`.
- Add `count` slot for each domain, non-categorical, find span by text matching.
- Categorize `dialogue acts` according to the `intent`.
- Concatenate multiple values using `|`.
- Retain `active_intent`, `requested_slots`, `service_call`.
- **Annotations:**
- dialogue acts, state, db_results, service_call, active_intent, requested_slots.
### Supported Tasks and Leaderboards
NLU, DST, Policy, NLG, E2E
### Languages
English
### Data Splits
| split | dialogues | utterances | avg_utt | avg_tokens | avg_domains | cat slot match(state) | cat slot match(goal) | cat slot match(dialogue act) | non-cat slot span(dialogue act) |
|------------|-------------|--------------|-----------|--------------|---------------|-------------------------|------------------------|--------------------------------|-----------------------------------|
| train | 16142 | 329964 | 20.44 | 9.75 | 1.84 | 100 | - | 100 | 100 |
| validation | 2482 | 48726 | 19.63 | 9.66 | 1.84 | 100 | - | 100 | 100 |
| test | 4201 | 84594 | 20.14 | 10.4 | 2.02 | 100 | - | 100 | 100 |
| all | 22825 | 463284 | 20.3 | 9.86 | 1.87 | 100 | - | 100 | 100 |
45 domains: ['Banks_13', 'Buses_13', 'Buses_23', 'Calendar_13', 'Events_13', 'Events_23', 'Flights_13', 'Flights_23', 'Homes_13', 'Hotels_13', 'Hotels_23', 'Hotels_33', 'Media_13', 'Movies_13', 'Music_13', 'Music_23', 'RentalCars_13', 'RentalCars_23', 'Restaurants_13', 'RideSharing_13', 'RideSharing_23', 'Services_13', 'Services_23', 'Services_33', 'Travel_13', 'Weather_13', 'Alarm_13', 'Banks_23', 'Flights_33', 'Hotels_43', 'Media_23', 'Movies_23', 'Restaurants_23', 'Services_43', 'Buses_33', 'Events_33', 'Flights_43', 'Homes_23', 'Media_33', 'Messaging_13', 'Movies_33', 'Music_33', 'Payment_13', 'RentalCars_33', 'Trains_13']
- **cat slot match**: how many values of categorical slots are in the possible values of ontology in percentage.
- **non-cat slot span**: how many values of non-categorical slots have span annotation in percentage.
### Citation
```
@inproceedings{lee2022sgd,
title={SGD-X: A Benchmark for Robust Generalization in Schema-Guided Dialogue Systems},
author={Lee, Harrison and Gupta, Raghav and Rastogi, Abhinav and Cao, Yuan and Zhang, Bin and Wu, Yonghui},
booktitle={Proceedings of the AAAI Conference on Artificial Intelligence},
volume={36},
number={10},
pages={10938--10946},
year={2022}
}
```
### Licensing Information
[**CC BY-SA 4.0**](https://creativecommons.org/licenses/by-sa/4.0/) | ConvLab/sgd3 | [
"task_categories:conversational",
"multilinguality:monolingual",
"size_categories:10K<n<100K",
"language:en",
"license:cc-by-sa-4.0",
"arxiv:2110.06800",
"region:us"
] | 2022-11-22T12:47:43+00:00 | {"language": ["en"], "license": ["cc-by-sa-4.0"], "multilinguality": ["monolingual"], "size_categories": ["10K<n<100K"], "task_categories": ["conversational"], "pretty_name": "SGD-X v3"} | 2022-11-25T08:52:36+00:00 | [
"2110.06800"
] | [
"en"
] | TAGS
#task_categories-conversational #multilinguality-monolingual #size_categories-10K<n<100K #language-English #license-cc-by-sa-4.0 #arxiv-2110.06800 #region-us
| Dataset Card for SGD-X v3
=========================
* Repository: URL
* Paper: URL
* Leaderboard: None
* Who transforms the dataset: Qi Zhu(zhuq96 at gmail dot com)
To use this dataset, you need to install ConvLab-3 platform first. Then you can load the dataset via:
For more usage please refer to here.
### Dataset Summary
The Schema-Guided Dialogue (SGD) dataset consists of over 20k annotated multi-domain, task-oriented conversations between a human and a virtual assistant. These conversations involve interactions with services and APIs spanning 20 domains, such as banks, events, media, calendar, travel, and weather. For most of these domains, the dataset contains multiple different APIs, many of which have overlapping functionalities but different interfaces, which reflects common real-world scenarios. The wide range of available annotations can be used for intent prediction, slot filling, dialogue state tracking, policy imitation learning, language generation, and user simulation learning, among other tasks for developing large-scale virtual assistants. Additionally, the dataset contains unseen domains and services in the evaluation set to quantify the performance in zero-shot or few-shot settings.
The SGD-X dataset consists of 5 linguistic variants of every schema in the original SGD dataset. Linguistic variants were written by hundreds of paid crowd-workers. In the SGD-X directory, v1 represents the variant closest to the original schemas and v5 the farthest in terms of linguistic distance. To evaluate model performance on SGD-X schemas, dialogues must be converted using the script generate\_sgdx\_dialogues.py.
* How to get the transformed data from original data:
+ Download URL.
+ Modified 'sgd\_x/generate\_sgdx\_dialogues.py' as URL
+ Run 'python -m sgd\_x.generate\_sgdx\_dialogues' under 'dstc8-schema-guided-dialogue-master' dir which need tensorflow installed.
+ Run 'python URL' in the current directory.
* Main changes of the transformation:
+ Lower case original 'act' as 'intent'.
+ Add 'count' slot for each domain, non-categorical, find span by text matching.
+ Categorize 'dialogue acts' according to the 'intent'.
+ Concatenate multiple values using '|'.
+ Retain 'active\_intent', 'requested\_slots', 'service\_call'.
* Annotations:
+ dialogue acts, state, db\_results, service\_call, active\_intent, requested\_slots.
### Supported Tasks and Leaderboards
NLU, DST, Policy, NLG, E2E
### Languages
English
### Data Splits
45 domains: ['Banks\_13', 'Buses\_13', 'Buses\_23', 'Calendar\_13', 'Events\_13', 'Events\_23', 'Flights\_13', 'Flights\_23', 'Homes\_13', 'Hotels\_13', 'Hotels\_23', 'Hotels\_33', 'Media\_13', 'Movies\_13', 'Music\_13', 'Music\_23', 'RentalCars\_13', 'RentalCars\_23', 'Restaurants\_13', 'RideSharing\_13', 'RideSharing\_23', 'Services\_13', 'Services\_23', 'Services\_33', 'Travel\_13', 'Weather\_13', 'Alarm\_13', 'Banks\_23', 'Flights\_33', 'Hotels\_43', 'Media\_23', 'Movies\_23', 'Restaurants\_23', 'Services\_43', 'Buses\_33', 'Events\_33', 'Flights\_43', 'Homes\_23', 'Media\_33', 'Messaging\_13', 'Movies\_33', 'Music\_33', 'Payment\_13', 'RentalCars\_33', 'Trains\_13']
* cat slot match: how many values of categorical slots are in the possible values of ontology in percentage.
* non-cat slot span: how many values of non-categorical slots have span annotation in percentage.
### Licensing Information
CC BY-SA 4.0
| [
"### Dataset Summary\n\n\nThe Schema-Guided Dialogue (SGD) dataset consists of over 20k annotated multi-domain, task-oriented conversations between a human and a virtual assistant. These conversations involve interactions with services and APIs spanning 20 domains, such as banks, events, media, calendar, travel, and weather. For most of these domains, the dataset contains multiple different APIs, many of which have overlapping functionalities but different interfaces, which reflects common real-world scenarios. The wide range of available annotations can be used for intent prediction, slot filling, dialogue state tracking, policy imitation learning, language generation, and user simulation learning, among other tasks for developing large-scale virtual assistants. Additionally, the dataset contains unseen domains and services in the evaluation set to quantify the performance in zero-shot or few-shot settings.\n\n\nThe SGD-X dataset consists of 5 linguistic variants of every schema in the original SGD dataset. Linguistic variants were written by hundreds of paid crowd-workers. In the SGD-X directory, v1 represents the variant closest to the original schemas and v5 the farthest in terms of linguistic distance. To evaluate model performance on SGD-X schemas, dialogues must be converted using the script generate\\_sgdx\\_dialogues.py.\n\n\n* How to get the transformed data from original data:\n\t+ Download URL.\n\t+ Modified 'sgd\\_x/generate\\_sgdx\\_dialogues.py' as URL\n\t+ Run 'python -m sgd\\_x.generate\\_sgdx\\_dialogues' under 'dstc8-schema-guided-dialogue-master' dir which need tensorflow installed.\n\t+ Run 'python URL' in the current directory.\n* Main changes of the transformation:\n\t+ Lower case original 'act' as 'intent'.\n\t+ Add 'count' slot for each domain, non-categorical, find span by text matching.\n\t+ Categorize 'dialogue acts' according to the 'intent'.\n\t+ Concatenate multiple values using '|'.\n\t+ Retain 'active\\_intent', 'requested\\_slots', 'service\\_call'.\n* Annotations:\n\t+ dialogue acts, state, db\\_results, service\\_call, active\\_intent, requested\\_slots.",
"### Supported Tasks and Leaderboards\n\n\nNLU, DST, Policy, NLG, E2E",
"### Languages\n\n\nEnglish",
"### Data Splits\n\n\n\n45 domains: ['Banks\\_13', 'Buses\\_13', 'Buses\\_23', 'Calendar\\_13', 'Events\\_13', 'Events\\_23', 'Flights\\_13', 'Flights\\_23', 'Homes\\_13', 'Hotels\\_13', 'Hotels\\_23', 'Hotels\\_33', 'Media\\_13', 'Movies\\_13', 'Music\\_13', 'Music\\_23', 'RentalCars\\_13', 'RentalCars\\_23', 'Restaurants\\_13', 'RideSharing\\_13', 'RideSharing\\_23', 'Services\\_13', 'Services\\_23', 'Services\\_33', 'Travel\\_13', 'Weather\\_13', 'Alarm\\_13', 'Banks\\_23', 'Flights\\_33', 'Hotels\\_43', 'Media\\_23', 'Movies\\_23', 'Restaurants\\_23', 'Services\\_43', 'Buses\\_33', 'Events\\_33', 'Flights\\_43', 'Homes\\_23', 'Media\\_33', 'Messaging\\_13', 'Movies\\_33', 'Music\\_33', 'Payment\\_13', 'RentalCars\\_33', 'Trains\\_13']\n\n\n* cat slot match: how many values of categorical slots are in the possible values of ontology in percentage.\n* non-cat slot span: how many values of non-categorical slots have span annotation in percentage.",
"### Licensing Information\n\n\nCC BY-SA 4.0"
] | [
"TAGS\n#task_categories-conversational #multilinguality-monolingual #size_categories-10K<n<100K #language-English #license-cc-by-sa-4.0 #arxiv-2110.06800 #region-us \n",
"### Dataset Summary\n\n\nThe Schema-Guided Dialogue (SGD) dataset consists of over 20k annotated multi-domain, task-oriented conversations between a human and a virtual assistant. These conversations involve interactions with services and APIs spanning 20 domains, such as banks, events, media, calendar, travel, and weather. For most of these domains, the dataset contains multiple different APIs, many of which have overlapping functionalities but different interfaces, which reflects common real-world scenarios. The wide range of available annotations can be used for intent prediction, slot filling, dialogue state tracking, policy imitation learning, language generation, and user simulation learning, among other tasks for developing large-scale virtual assistants. Additionally, the dataset contains unseen domains and services in the evaluation set to quantify the performance in zero-shot or few-shot settings.\n\n\nThe SGD-X dataset consists of 5 linguistic variants of every schema in the original SGD dataset. Linguistic variants were written by hundreds of paid crowd-workers. In the SGD-X directory, v1 represents the variant closest to the original schemas and v5 the farthest in terms of linguistic distance. To evaluate model performance on SGD-X schemas, dialogues must be converted using the script generate\\_sgdx\\_dialogues.py.\n\n\n* How to get the transformed data from original data:\n\t+ Download URL.\n\t+ Modified 'sgd\\_x/generate\\_sgdx\\_dialogues.py' as URL\n\t+ Run 'python -m sgd\\_x.generate\\_sgdx\\_dialogues' under 'dstc8-schema-guided-dialogue-master' dir which need tensorflow installed.\n\t+ Run 'python URL' in the current directory.\n* Main changes of the transformation:\n\t+ Lower case original 'act' as 'intent'.\n\t+ Add 'count' slot for each domain, non-categorical, find span by text matching.\n\t+ Categorize 'dialogue acts' according to the 'intent'.\n\t+ Concatenate multiple values using '|'.\n\t+ Retain 'active\\_intent', 'requested\\_slots', 'service\\_call'.\n* Annotations:\n\t+ dialogue acts, state, db\\_results, service\\_call, active\\_intent, requested\\_slots.",
"### Supported Tasks and Leaderboards\n\n\nNLU, DST, Policy, NLG, E2E",
"### Languages\n\n\nEnglish",
"### Data Splits\n\n\n\n45 domains: ['Banks\\_13', 'Buses\\_13', 'Buses\\_23', 'Calendar\\_13', 'Events\\_13', 'Events\\_23', 'Flights\\_13', 'Flights\\_23', 'Homes\\_13', 'Hotels\\_13', 'Hotels\\_23', 'Hotels\\_33', 'Media\\_13', 'Movies\\_13', 'Music\\_13', 'Music\\_23', 'RentalCars\\_13', 'RentalCars\\_23', 'Restaurants\\_13', 'RideSharing\\_13', 'RideSharing\\_23', 'Services\\_13', 'Services\\_23', 'Services\\_33', 'Travel\\_13', 'Weather\\_13', 'Alarm\\_13', 'Banks\\_23', 'Flights\\_33', 'Hotels\\_43', 'Media\\_23', 'Movies\\_23', 'Restaurants\\_23', 'Services\\_43', 'Buses\\_33', 'Events\\_33', 'Flights\\_43', 'Homes\\_23', 'Media\\_33', 'Messaging\\_13', 'Movies\\_33', 'Music\\_33', 'Payment\\_13', 'RentalCars\\_33', 'Trains\\_13']\n\n\n* cat slot match: how many values of categorical slots are in the possible values of ontology in percentage.\n* non-cat slot span: how many values of non-categorical slots have span annotation in percentage.",
"### Licensing Information\n\n\nCC BY-SA 4.0"
] | [
60,
582,
24,
5,
431,
11
] | [
"passage: TAGS\n#task_categories-conversational #multilinguality-monolingual #size_categories-10K<n<100K #language-English #license-cc-by-sa-4.0 #arxiv-2110.06800 #region-us \n"
] |
7783b67a6f0ff97d66e69713a96a1fcd99a7a369 |
# Dataset Card for SGD-X v4
- **Repository:** https://github.com/google-research-datasets/dstc8-schema-guided-dialogue/tree/master/sgd_x
- **Paper:** https://arxiv.org/pdf/2110.06800.pdf
- **Leaderboard:** None
- **Who transforms the dataset:** Qi Zhu(zhuq96 at gmail dot com)
To use this dataset, you need to install [ConvLab-3](https://github.com/ConvLab/ConvLab-3) platform first. Then you can load the dataset via:
```
from convlab.util import load_dataset, load_ontology, load_database
dataset = load_dataset('sgd4')
ontology = load_ontology('sgd4')
database = load_database('sgd4')
```
For more usage please refer to [here](https://github.com/ConvLab/ConvLab-3/tree/master/data/unified_datasets).
### Dataset Summary
The **Schema-Guided Dialogue (SGD)** dataset consists of over 20k annotated multi-domain, task-oriented conversations between a human and a virtual assistant. These conversations involve interactions with services and APIs spanning 20 domains, such as banks, events, media, calendar, travel, and weather. For most of these domains, the dataset contains multiple different APIs, many of which have overlapping functionalities but different interfaces, which reflects common real-world scenarios. The wide range of available annotations can be used for intent prediction, slot filling, dialogue state tracking, policy imitation learning, language generation, and user simulation learning, among other tasks for developing large-scale virtual assistants. Additionally, the dataset contains unseen domains and services in the evaluation set to quantify the performance in zero-shot or few-shot settings.
The **SGD-X** dataset consists of 5 linguistic variants of every schema in the original SGD dataset. Linguistic variants were written by hundreds of paid crowd-workers. In the SGD-X directory, v1 represents the variant closest to the original schemas and v5 the farthest in terms of linguistic distance. To evaluate model performance on SGD-X schemas, dialogues must be converted using the script generate_sgdx_dialogues.py.
- **How to get the transformed data from original data:**
- Download [dstc8-schema-guided-dialogue-master.zip](https://github.com/google-research-datasets/dstc8-schema-guided-dialogue/archive/refs/heads/master.zip).
- Modified `sgd_x/generate_sgdx_dialogues.py` as https://github.com/google-research-datasets/dstc8-schema-guided-dialogue/issues/57
- Run `python -m sgd_x.generate_sgdx_dialogues` under `dstc8-schema-guided-dialogue-master` dir which need tensorflow installed.
- Run `python preprocess.py` in the current directory.
- **Main changes of the transformation:**
- Lower case original `act` as `intent`.
- Add `count` slot for each domain, non-categorical, find span by text matching.
- Categorize `dialogue acts` according to the `intent`.
- Concatenate multiple values using `|`.
- Retain `active_intent`, `requested_slots`, `service_call`.
- **Annotations:**
- dialogue acts, state, db_results, service_call, active_intent, requested_slots.
### Supported Tasks and Leaderboards
NLU, DST, Policy, NLG, E2E
### Languages
English
### Data Splits
| split | dialogues | utterances | avg_utt | avg_tokens | avg_domains | cat slot match(state) | cat slot match(goal) | cat slot match(dialogue act) | non-cat slot span(dialogue act) |
|------------|-------------|--------------|-----------|--------------|---------------|-------------------------|------------------------|--------------------------------|-----------------------------------|
| train | 16142 | 329964 | 20.44 | 9.75 | 1.84 | 100 | - | 100 | 100 |
| validation | 2482 | 48726 | 19.63 | 9.66 | 1.84 | 100 | - | 100 | 100 |
| test | 4201 | 84594 | 20.14 | 10.4 | 2.02 | 100 | - | 100 | 100 |
| all | 22825 | 463284 | 20.3 | 9.86 | 1.87 | 100 | - | 100 | 100 |
45 domains: ['Banks_14', 'Buses_14', 'Buses_24', 'Calendar_14', 'Events_14', 'Events_24', 'Flights_14', 'Flights_24', 'Homes_14', 'Hotels_14', 'Hotels_24', 'Hotels_34', 'Media_14', 'Movies_14', 'Music_14', 'Music_24', 'RentalCars_14', 'RentalCars_24', 'Restaurants_14', 'RideSharing_14', 'RideSharing_24', 'Services_14', 'Services_24', 'Services_34', 'Travel_14', 'Weather_14', 'Alarm_14', 'Banks_24', 'Flights_34', 'Hotels_44', 'Media_24', 'Movies_24', 'Restaurants_24', 'Services_44', 'Buses_34', 'Events_34', 'Flights_44', 'Homes_24', 'Media_34', 'Messaging_14', 'Movies_34', 'Music_34', 'Payment_14', 'RentalCars_34', 'Trains_14']
- **cat slot match**: how many values of categorical slots are in the possible values of ontology in percentage.
- **non-cat slot span**: how many values of non-categorical slots have span annotation in percentage.
### Citation
```
@inproceedings{lee2022sgd,
title={SGD-X: A Benchmark for Robust Generalization in Schema-Guided Dialogue Systems},
author={Lee, Harrison and Gupta, Raghav and Rastogi, Abhinav and Cao, Yuan and Zhang, Bin and Wu, Yonghui},
booktitle={Proceedings of the AAAI Conference on Artificial Intelligence},
volume={36},
number={10},
pages={10938--10946},
year={2022}
}
```
### Licensing Information
[**CC BY-SA 4.0**](https://creativecommons.org/licenses/by-sa/4.0/) | ConvLab/sgd4 | [
"task_categories:conversational",
"multilinguality:monolingual",
"size_categories:10K<n<100K",
"language:en",
"license:cc-by-sa-4.0",
"arxiv:2110.06800",
"region:us"
] | 2022-11-22T12:47:58+00:00 | {"language": ["en"], "license": ["cc-by-sa-4.0"], "multilinguality": ["monolingual"], "size_categories": ["10K<n<100K"], "task_categories": ["conversational"], "pretty_name": "SGD-X v4"} | 2022-11-25T08:51:35+00:00 | [
"2110.06800"
] | [
"en"
] | TAGS
#task_categories-conversational #multilinguality-monolingual #size_categories-10K<n<100K #language-English #license-cc-by-sa-4.0 #arxiv-2110.06800 #region-us
| Dataset Card for SGD-X v4
=========================
* Repository: URL
* Paper: URL
* Leaderboard: None
* Who transforms the dataset: Qi Zhu(zhuq96 at gmail dot com)
To use this dataset, you need to install ConvLab-3 platform first. Then you can load the dataset via:
For more usage please refer to here.
### Dataset Summary
The Schema-Guided Dialogue (SGD) dataset consists of over 20k annotated multi-domain, task-oriented conversations between a human and a virtual assistant. These conversations involve interactions with services and APIs spanning 20 domains, such as banks, events, media, calendar, travel, and weather. For most of these domains, the dataset contains multiple different APIs, many of which have overlapping functionalities but different interfaces, which reflects common real-world scenarios. The wide range of available annotations can be used for intent prediction, slot filling, dialogue state tracking, policy imitation learning, language generation, and user simulation learning, among other tasks for developing large-scale virtual assistants. Additionally, the dataset contains unseen domains and services in the evaluation set to quantify the performance in zero-shot or few-shot settings.
The SGD-X dataset consists of 5 linguistic variants of every schema in the original SGD dataset. Linguistic variants were written by hundreds of paid crowd-workers. In the SGD-X directory, v1 represents the variant closest to the original schemas and v5 the farthest in terms of linguistic distance. To evaluate model performance on SGD-X schemas, dialogues must be converted using the script generate\_sgdx\_dialogues.py.
* How to get the transformed data from original data:
+ Download URL.
+ Modified 'sgd\_x/generate\_sgdx\_dialogues.py' as URL
+ Run 'python -m sgd\_x.generate\_sgdx\_dialogues' under 'dstc8-schema-guided-dialogue-master' dir which need tensorflow installed.
+ Run 'python URL' in the current directory.
* Main changes of the transformation:
+ Lower case original 'act' as 'intent'.
+ Add 'count' slot for each domain, non-categorical, find span by text matching.
+ Categorize 'dialogue acts' according to the 'intent'.
+ Concatenate multiple values using '|'.
+ Retain 'active\_intent', 'requested\_slots', 'service\_call'.
* Annotations:
+ dialogue acts, state, db\_results, service\_call, active\_intent, requested\_slots.
### Supported Tasks and Leaderboards
NLU, DST, Policy, NLG, E2E
### Languages
English
### Data Splits
45 domains: ['Banks\_14', 'Buses\_14', 'Buses\_24', 'Calendar\_14', 'Events\_14', 'Events\_24', 'Flights\_14', 'Flights\_24', 'Homes\_14', 'Hotels\_14', 'Hotels\_24', 'Hotels\_34', 'Media\_14', 'Movies\_14', 'Music\_14', 'Music\_24', 'RentalCars\_14', 'RentalCars\_24', 'Restaurants\_14', 'RideSharing\_14', 'RideSharing\_24', 'Services\_14', 'Services\_24', 'Services\_34', 'Travel\_14', 'Weather\_14', 'Alarm\_14', 'Banks\_24', 'Flights\_34', 'Hotels\_44', 'Media\_24', 'Movies\_24', 'Restaurants\_24', 'Services\_44', 'Buses\_34', 'Events\_34', 'Flights\_44', 'Homes\_24', 'Media\_34', 'Messaging\_14', 'Movies\_34', 'Music\_34', 'Payment\_14', 'RentalCars\_34', 'Trains\_14']
* cat slot match: how many values of categorical slots are in the possible values of ontology in percentage.
* non-cat slot span: how many values of non-categorical slots have span annotation in percentage.
### Licensing Information
CC BY-SA 4.0
| [
"### Dataset Summary\n\n\nThe Schema-Guided Dialogue (SGD) dataset consists of over 20k annotated multi-domain, task-oriented conversations between a human and a virtual assistant. These conversations involve interactions with services and APIs spanning 20 domains, such as banks, events, media, calendar, travel, and weather. For most of these domains, the dataset contains multiple different APIs, many of which have overlapping functionalities but different interfaces, which reflects common real-world scenarios. The wide range of available annotations can be used for intent prediction, slot filling, dialogue state tracking, policy imitation learning, language generation, and user simulation learning, among other tasks for developing large-scale virtual assistants. Additionally, the dataset contains unseen domains and services in the evaluation set to quantify the performance in zero-shot or few-shot settings.\n\n\nThe SGD-X dataset consists of 5 linguistic variants of every schema in the original SGD dataset. Linguistic variants were written by hundreds of paid crowd-workers. In the SGD-X directory, v1 represents the variant closest to the original schemas and v5 the farthest in terms of linguistic distance. To evaluate model performance on SGD-X schemas, dialogues must be converted using the script generate\\_sgdx\\_dialogues.py.\n\n\n* How to get the transformed data from original data:\n\t+ Download URL.\n\t+ Modified 'sgd\\_x/generate\\_sgdx\\_dialogues.py' as URL\n\t+ Run 'python -m sgd\\_x.generate\\_sgdx\\_dialogues' under 'dstc8-schema-guided-dialogue-master' dir which need tensorflow installed.\n\t+ Run 'python URL' in the current directory.\n* Main changes of the transformation:\n\t+ Lower case original 'act' as 'intent'.\n\t+ Add 'count' slot for each domain, non-categorical, find span by text matching.\n\t+ Categorize 'dialogue acts' according to the 'intent'.\n\t+ Concatenate multiple values using '|'.\n\t+ Retain 'active\\_intent', 'requested\\_slots', 'service\\_call'.\n* Annotations:\n\t+ dialogue acts, state, db\\_results, service\\_call, active\\_intent, requested\\_slots.",
"### Supported Tasks and Leaderboards\n\n\nNLU, DST, Policy, NLG, E2E",
"### Languages\n\n\nEnglish",
"### Data Splits\n\n\n\n45 domains: ['Banks\\_14', 'Buses\\_14', 'Buses\\_24', 'Calendar\\_14', 'Events\\_14', 'Events\\_24', 'Flights\\_14', 'Flights\\_24', 'Homes\\_14', 'Hotels\\_14', 'Hotels\\_24', 'Hotels\\_34', 'Media\\_14', 'Movies\\_14', 'Music\\_14', 'Music\\_24', 'RentalCars\\_14', 'RentalCars\\_24', 'Restaurants\\_14', 'RideSharing\\_14', 'RideSharing\\_24', 'Services\\_14', 'Services\\_24', 'Services\\_34', 'Travel\\_14', 'Weather\\_14', 'Alarm\\_14', 'Banks\\_24', 'Flights\\_34', 'Hotels\\_44', 'Media\\_24', 'Movies\\_24', 'Restaurants\\_24', 'Services\\_44', 'Buses\\_34', 'Events\\_34', 'Flights\\_44', 'Homes\\_24', 'Media\\_34', 'Messaging\\_14', 'Movies\\_34', 'Music\\_34', 'Payment\\_14', 'RentalCars\\_34', 'Trains\\_14']\n\n\n* cat slot match: how many values of categorical slots are in the possible values of ontology in percentage.\n* non-cat slot span: how many values of non-categorical slots have span annotation in percentage.",
"### Licensing Information\n\n\nCC BY-SA 4.0"
] | [
"TAGS\n#task_categories-conversational #multilinguality-monolingual #size_categories-10K<n<100K #language-English #license-cc-by-sa-4.0 #arxiv-2110.06800 #region-us \n",
"### Dataset Summary\n\n\nThe Schema-Guided Dialogue (SGD) dataset consists of over 20k annotated multi-domain, task-oriented conversations between a human and a virtual assistant. These conversations involve interactions with services and APIs spanning 20 domains, such as banks, events, media, calendar, travel, and weather. For most of these domains, the dataset contains multiple different APIs, many of which have overlapping functionalities but different interfaces, which reflects common real-world scenarios. The wide range of available annotations can be used for intent prediction, slot filling, dialogue state tracking, policy imitation learning, language generation, and user simulation learning, among other tasks for developing large-scale virtual assistants. Additionally, the dataset contains unseen domains and services in the evaluation set to quantify the performance in zero-shot or few-shot settings.\n\n\nThe SGD-X dataset consists of 5 linguistic variants of every schema in the original SGD dataset. Linguistic variants were written by hundreds of paid crowd-workers. In the SGD-X directory, v1 represents the variant closest to the original schemas and v5 the farthest in terms of linguistic distance. To evaluate model performance on SGD-X schemas, dialogues must be converted using the script generate\\_sgdx\\_dialogues.py.\n\n\n* How to get the transformed data from original data:\n\t+ Download URL.\n\t+ Modified 'sgd\\_x/generate\\_sgdx\\_dialogues.py' as URL\n\t+ Run 'python -m sgd\\_x.generate\\_sgdx\\_dialogues' under 'dstc8-schema-guided-dialogue-master' dir which need tensorflow installed.\n\t+ Run 'python URL' in the current directory.\n* Main changes of the transformation:\n\t+ Lower case original 'act' as 'intent'.\n\t+ Add 'count' slot for each domain, non-categorical, find span by text matching.\n\t+ Categorize 'dialogue acts' according to the 'intent'.\n\t+ Concatenate multiple values using '|'.\n\t+ Retain 'active\\_intent', 'requested\\_slots', 'service\\_call'.\n* Annotations:\n\t+ dialogue acts, state, db\\_results, service\\_call, active\\_intent, requested\\_slots.",
"### Supported Tasks and Leaderboards\n\n\nNLU, DST, Policy, NLG, E2E",
"### Languages\n\n\nEnglish",
"### Data Splits\n\n\n\n45 domains: ['Banks\\_14', 'Buses\\_14', 'Buses\\_24', 'Calendar\\_14', 'Events\\_14', 'Events\\_24', 'Flights\\_14', 'Flights\\_24', 'Homes\\_14', 'Hotels\\_14', 'Hotels\\_24', 'Hotels\\_34', 'Media\\_14', 'Movies\\_14', 'Music\\_14', 'Music\\_24', 'RentalCars\\_14', 'RentalCars\\_24', 'Restaurants\\_14', 'RideSharing\\_14', 'RideSharing\\_24', 'Services\\_14', 'Services\\_24', 'Services\\_34', 'Travel\\_14', 'Weather\\_14', 'Alarm\\_14', 'Banks\\_24', 'Flights\\_34', 'Hotels\\_44', 'Media\\_24', 'Movies\\_24', 'Restaurants\\_24', 'Services\\_44', 'Buses\\_34', 'Events\\_34', 'Flights\\_44', 'Homes\\_24', 'Media\\_34', 'Messaging\\_14', 'Movies\\_34', 'Music\\_34', 'Payment\\_14', 'RentalCars\\_34', 'Trains\\_14']\n\n\n* cat slot match: how many values of categorical slots are in the possible values of ontology in percentage.\n* non-cat slot span: how many values of non-categorical slots have span annotation in percentage.",
"### Licensing Information\n\n\nCC BY-SA 4.0"
] | [
60,
582,
24,
5,
431,
11
] | [
"passage: TAGS\n#task_categories-conversational #multilinguality-monolingual #size_categories-10K<n<100K #language-English #license-cc-by-sa-4.0 #arxiv-2110.06800 #region-us \n"
] |
7ce84114b1e52a4e44d8d6310f4b26adecedf73c |
# Dataset Card for SGD-X v5
- **Repository:** https://github.com/google-research-datasets/dstc8-schema-guided-dialogue/tree/master/sgd_x
- **Paper:** https://arxiv.org/pdf/2110.06800.pdf
- **Leaderboard:** None
- **Who transforms the dataset:** Qi Zhu(zhuq96 at gmail dot com)
To use this dataset, you need to install [ConvLab-3](https://github.com/ConvLab/ConvLab-3) platform first. Then you can load the dataset via:
```
from convlab.util import load_dataset, load_ontology, load_database
dataset = load_dataset('sgd5')
ontology = load_ontology('sgd5')
database = load_database('sgd5')
```
For more usage please refer to [here](https://github.com/ConvLab/ConvLab-3/tree/master/data/unified_datasets).
### Dataset Summary
The **Schema-Guided Dialogue (SGD)** dataset consists of over 20k annotated multi-domain, task-oriented conversations between a human and a virtual assistant. These conversations involve interactions with services and APIs spanning 20 domains, such as banks, events, media, calendar, travel, and weather. For most of these domains, the dataset contains multiple different APIs, many of which have overlapping functionalities but different interfaces, which reflects common real-world scenarios. The wide range of available annotations can be used for intent prediction, slot filling, dialogue state tracking, policy imitation learning, language generation, and user simulation learning, among other tasks for developing large-scale virtual assistants. Additionally, the dataset contains unseen domains and services in the evaluation set to quantify the performance in zero-shot or few-shot settings.
The **SGD-X** dataset consists of 5 linguistic variants of every schema in the original SGD dataset. Linguistic variants were written by hundreds of paid crowd-workers. In the SGD-X directory, v1 represents the variant closest to the original schemas and v5 the farthest in terms of linguistic distance. To evaluate model performance on SGD-X schemas, dialogues must be converted using the script generate_sgdx_dialogues.py.
- **How to get the transformed data from original data:**
- Download [dstc8-schema-guided-dialogue-master.zip](https://github.com/google-research-datasets/dstc8-schema-guided-dialogue/archive/refs/heads/master.zip).
- Modified `sgd_x/generate_sgdx_dialogues.py` as https://github.com/google-research-datasets/dstc8-schema-guided-dialogue/issues/57
- Run `python -m sgd_x.generate_sgdx_dialogues` under `dstc8-schema-guided-dialogue-master` dir which need tensorflow installed.
- Run `python preprocess.py` in the current directory.
- **Main changes of the transformation:**
- Lower case original `act` as `intent`.
- Add `count` slot for each domain, non-categorical, find span by text matching.
- Categorize `dialogue acts` according to the `intent`.
- Concatenate multiple values using `|`.
- Retain `active_intent`, `requested_slots`, `service_call`.
- **Annotations:**
- dialogue acts, state, db_results, service_call, active_intent, requested_slots.
### Supported Tasks and Leaderboards
NLU, DST, Policy, NLG, E2E
### Languages
English
### Data Splits
| split | dialogues | utterances | avg_utt | avg_tokens | avg_domains | cat slot match(state) | cat slot match(goal) | cat slot match(dialogue act) | non-cat slot span(dialogue act) |
|------------|-------------|--------------|-----------|--------------|---------------|-------------------------|------------------------|--------------------------------|-----------------------------------|
| train | 16142 | 329964 | 20.44 | 9.75 | 1.84 | 100 | - | 100 | 100 |
| validation | 2482 | 48726 | 19.63 | 9.66 | 1.84 | 100 | - | 100 | 100 |
| test | 4201 | 84594 | 20.14 | 10.4 | 2.02 | 100 | - | 100 | 100 |
| all | 22825 | 463284 | 20.3 | 9.86 | 1.87 | 100 | - | 100 | 100 |
45 domains: ['Banks_15', 'Buses_15', 'Buses_25', 'Calendar_15', 'Events_15', 'Events_25', 'Flights_15', 'Flights_25', 'Homes_15', 'Hotels_15', 'Hotels_25', 'Hotels_35', 'Media_15', 'Movies_15', 'Music_15', 'Music_25', 'RentalCars_15', 'RentalCars_25', 'Restaurants_15', 'RideSharing_15', 'RideSharing_25', 'Services_15', 'Services_25', 'Services_35', 'Travel_15', 'Weather_15', 'Alarm_15', 'Banks_25', 'Flights_35', 'Hotels_45', 'Media_25', 'Movies_25', 'Restaurants_25', 'Services_45', 'Buses_35', 'Events_35', 'Flights_45', 'Homes_25', 'Media_35', 'Messaging_15', 'Movies_35', 'Music_35', 'Payment_15', 'RentalCars_35', 'Trains_15']
- **cat slot match**: how many values of categorical slots are in the possible values of ontology in percentage.
- **non-cat slot span**: how many values of non-categorical slots have span annotation in percentage.
### Citation
```
@inproceedings{lee2022sgd,
title={SGD-X: A Benchmark for Robust Generalization in Schema-Guided Dialogue Systems},
author={Lee, Harrison and Gupta, Raghav and Rastogi, Abhinav and Cao, Yuan and Zhang, Bin and Wu, Yonghui},
booktitle={Proceedings of the AAAI Conference on Artificial Intelligence},
volume={36},
number={10},
pages={10938--10946},
year={2022}
}
```
### Licensing Information
[**CC BY-SA 4.0**](https://creativecommons.org/licenses/by-sa/4.0/) | ConvLab/sgd5 | [
"task_categories:conversational",
"multilinguality:monolingual",
"size_categories:10K<n<100K",
"language:en",
"license:cc-by-sa-4.0",
"arxiv:2110.06800",
"region:us"
] | 2022-11-22T12:48:11+00:00 | {"language": ["en"], "license": ["cc-by-sa-4.0"], "multilinguality": ["monolingual"], "size_categories": ["10K<n<100K"], "task_categories": ["conversational"], "pretty_name": "SGD-X v5"} | 2022-11-25T08:49:21+00:00 | [
"2110.06800"
] | [
"en"
] | TAGS
#task_categories-conversational #multilinguality-monolingual #size_categories-10K<n<100K #language-English #license-cc-by-sa-4.0 #arxiv-2110.06800 #region-us
| Dataset Card for SGD-X v5
=========================
* Repository: URL
* Paper: URL
* Leaderboard: None
* Who transforms the dataset: Qi Zhu(zhuq96 at gmail dot com)
To use this dataset, you need to install ConvLab-3 platform first. Then you can load the dataset via:
For more usage please refer to here.
### Dataset Summary
The Schema-Guided Dialogue (SGD) dataset consists of over 20k annotated multi-domain, task-oriented conversations between a human and a virtual assistant. These conversations involve interactions with services and APIs spanning 20 domains, such as banks, events, media, calendar, travel, and weather. For most of these domains, the dataset contains multiple different APIs, many of which have overlapping functionalities but different interfaces, which reflects common real-world scenarios. The wide range of available annotations can be used for intent prediction, slot filling, dialogue state tracking, policy imitation learning, language generation, and user simulation learning, among other tasks for developing large-scale virtual assistants. Additionally, the dataset contains unseen domains and services in the evaluation set to quantify the performance in zero-shot or few-shot settings.
The SGD-X dataset consists of 5 linguistic variants of every schema in the original SGD dataset. Linguistic variants were written by hundreds of paid crowd-workers. In the SGD-X directory, v1 represents the variant closest to the original schemas and v5 the farthest in terms of linguistic distance. To evaluate model performance on SGD-X schemas, dialogues must be converted using the script generate\_sgdx\_dialogues.py.
* How to get the transformed data from original data:
+ Download URL.
+ Modified 'sgd\_x/generate\_sgdx\_dialogues.py' as URL
+ Run 'python -m sgd\_x.generate\_sgdx\_dialogues' under 'dstc8-schema-guided-dialogue-master' dir which need tensorflow installed.
+ Run 'python URL' in the current directory.
* Main changes of the transformation:
+ Lower case original 'act' as 'intent'.
+ Add 'count' slot for each domain, non-categorical, find span by text matching.
+ Categorize 'dialogue acts' according to the 'intent'.
+ Concatenate multiple values using '|'.
+ Retain 'active\_intent', 'requested\_slots', 'service\_call'.
* Annotations:
+ dialogue acts, state, db\_results, service\_call, active\_intent, requested\_slots.
### Supported Tasks and Leaderboards
NLU, DST, Policy, NLG, E2E
### Languages
English
### Data Splits
45 domains: ['Banks\_15', 'Buses\_15', 'Buses\_25', 'Calendar\_15', 'Events\_15', 'Events\_25', 'Flights\_15', 'Flights\_25', 'Homes\_15', 'Hotels\_15', 'Hotels\_25', 'Hotels\_35', 'Media\_15', 'Movies\_15', 'Music\_15', 'Music\_25', 'RentalCars\_15', 'RentalCars\_25', 'Restaurants\_15', 'RideSharing\_15', 'RideSharing\_25', 'Services\_15', 'Services\_25', 'Services\_35', 'Travel\_15', 'Weather\_15', 'Alarm\_15', 'Banks\_25', 'Flights\_35', 'Hotels\_45', 'Media\_25', 'Movies\_25', 'Restaurants\_25', 'Services\_45', 'Buses\_35', 'Events\_35', 'Flights\_45', 'Homes\_25', 'Media\_35', 'Messaging\_15', 'Movies\_35', 'Music\_35', 'Payment\_15', 'RentalCars\_35', 'Trains\_15']
* cat slot match: how many values of categorical slots are in the possible values of ontology in percentage.
* non-cat slot span: how many values of non-categorical slots have span annotation in percentage.
### Licensing Information
CC BY-SA 4.0
| [
"### Dataset Summary\n\n\nThe Schema-Guided Dialogue (SGD) dataset consists of over 20k annotated multi-domain, task-oriented conversations between a human and a virtual assistant. These conversations involve interactions with services and APIs spanning 20 domains, such as banks, events, media, calendar, travel, and weather. For most of these domains, the dataset contains multiple different APIs, many of which have overlapping functionalities but different interfaces, which reflects common real-world scenarios. The wide range of available annotations can be used for intent prediction, slot filling, dialogue state tracking, policy imitation learning, language generation, and user simulation learning, among other tasks for developing large-scale virtual assistants. Additionally, the dataset contains unseen domains and services in the evaluation set to quantify the performance in zero-shot or few-shot settings.\n\n\nThe SGD-X dataset consists of 5 linguistic variants of every schema in the original SGD dataset. Linguistic variants were written by hundreds of paid crowd-workers. In the SGD-X directory, v1 represents the variant closest to the original schemas and v5 the farthest in terms of linguistic distance. To evaluate model performance on SGD-X schemas, dialogues must be converted using the script generate\\_sgdx\\_dialogues.py.\n\n\n* How to get the transformed data from original data:\n\t+ Download URL.\n\t+ Modified 'sgd\\_x/generate\\_sgdx\\_dialogues.py' as URL\n\t+ Run 'python -m sgd\\_x.generate\\_sgdx\\_dialogues' under 'dstc8-schema-guided-dialogue-master' dir which need tensorflow installed.\n\t+ Run 'python URL' in the current directory.\n* Main changes of the transformation:\n\t+ Lower case original 'act' as 'intent'.\n\t+ Add 'count' slot for each domain, non-categorical, find span by text matching.\n\t+ Categorize 'dialogue acts' according to the 'intent'.\n\t+ Concatenate multiple values using '|'.\n\t+ Retain 'active\\_intent', 'requested\\_slots', 'service\\_call'.\n* Annotations:\n\t+ dialogue acts, state, db\\_results, service\\_call, active\\_intent, requested\\_slots.",
"### Supported Tasks and Leaderboards\n\n\nNLU, DST, Policy, NLG, E2E",
"### Languages\n\n\nEnglish",
"### Data Splits\n\n\n\n45 domains: ['Banks\\_15', 'Buses\\_15', 'Buses\\_25', 'Calendar\\_15', 'Events\\_15', 'Events\\_25', 'Flights\\_15', 'Flights\\_25', 'Homes\\_15', 'Hotels\\_15', 'Hotels\\_25', 'Hotels\\_35', 'Media\\_15', 'Movies\\_15', 'Music\\_15', 'Music\\_25', 'RentalCars\\_15', 'RentalCars\\_25', 'Restaurants\\_15', 'RideSharing\\_15', 'RideSharing\\_25', 'Services\\_15', 'Services\\_25', 'Services\\_35', 'Travel\\_15', 'Weather\\_15', 'Alarm\\_15', 'Banks\\_25', 'Flights\\_35', 'Hotels\\_45', 'Media\\_25', 'Movies\\_25', 'Restaurants\\_25', 'Services\\_45', 'Buses\\_35', 'Events\\_35', 'Flights\\_45', 'Homes\\_25', 'Media\\_35', 'Messaging\\_15', 'Movies\\_35', 'Music\\_35', 'Payment\\_15', 'RentalCars\\_35', 'Trains\\_15']\n\n\n* cat slot match: how many values of categorical slots are in the possible values of ontology in percentage.\n* non-cat slot span: how many values of non-categorical slots have span annotation in percentage.",
"### Licensing Information\n\n\nCC BY-SA 4.0"
] | [
"TAGS\n#task_categories-conversational #multilinguality-monolingual #size_categories-10K<n<100K #language-English #license-cc-by-sa-4.0 #arxiv-2110.06800 #region-us \n",
"### Dataset Summary\n\n\nThe Schema-Guided Dialogue (SGD) dataset consists of over 20k annotated multi-domain, task-oriented conversations between a human and a virtual assistant. These conversations involve interactions with services and APIs spanning 20 domains, such as banks, events, media, calendar, travel, and weather. For most of these domains, the dataset contains multiple different APIs, many of which have overlapping functionalities but different interfaces, which reflects common real-world scenarios. The wide range of available annotations can be used for intent prediction, slot filling, dialogue state tracking, policy imitation learning, language generation, and user simulation learning, among other tasks for developing large-scale virtual assistants. Additionally, the dataset contains unseen domains and services in the evaluation set to quantify the performance in zero-shot or few-shot settings.\n\n\nThe SGD-X dataset consists of 5 linguistic variants of every schema in the original SGD dataset. Linguistic variants were written by hundreds of paid crowd-workers. In the SGD-X directory, v1 represents the variant closest to the original schemas and v5 the farthest in terms of linguistic distance. To evaluate model performance on SGD-X schemas, dialogues must be converted using the script generate\\_sgdx\\_dialogues.py.\n\n\n* How to get the transformed data from original data:\n\t+ Download URL.\n\t+ Modified 'sgd\\_x/generate\\_sgdx\\_dialogues.py' as URL\n\t+ Run 'python -m sgd\\_x.generate\\_sgdx\\_dialogues' under 'dstc8-schema-guided-dialogue-master' dir which need tensorflow installed.\n\t+ Run 'python URL' in the current directory.\n* Main changes of the transformation:\n\t+ Lower case original 'act' as 'intent'.\n\t+ Add 'count' slot for each domain, non-categorical, find span by text matching.\n\t+ Categorize 'dialogue acts' according to the 'intent'.\n\t+ Concatenate multiple values using '|'.\n\t+ Retain 'active\\_intent', 'requested\\_slots', 'service\\_call'.\n* Annotations:\n\t+ dialogue acts, state, db\\_results, service\\_call, active\\_intent, requested\\_slots.",
"### Supported Tasks and Leaderboards\n\n\nNLU, DST, Policy, NLG, E2E",
"### Languages\n\n\nEnglish",
"### Data Splits\n\n\n\n45 domains: ['Banks\\_15', 'Buses\\_15', 'Buses\\_25', 'Calendar\\_15', 'Events\\_15', 'Events\\_25', 'Flights\\_15', 'Flights\\_25', 'Homes\\_15', 'Hotels\\_15', 'Hotels\\_25', 'Hotels\\_35', 'Media\\_15', 'Movies\\_15', 'Music\\_15', 'Music\\_25', 'RentalCars\\_15', 'RentalCars\\_25', 'Restaurants\\_15', 'RideSharing\\_15', 'RideSharing\\_25', 'Services\\_15', 'Services\\_25', 'Services\\_35', 'Travel\\_15', 'Weather\\_15', 'Alarm\\_15', 'Banks\\_25', 'Flights\\_35', 'Hotels\\_45', 'Media\\_25', 'Movies\\_25', 'Restaurants\\_25', 'Services\\_45', 'Buses\\_35', 'Events\\_35', 'Flights\\_45', 'Homes\\_25', 'Media\\_35', 'Messaging\\_15', 'Movies\\_35', 'Music\\_35', 'Payment\\_15', 'RentalCars\\_35', 'Trains\\_15']\n\n\n* cat slot match: how many values of categorical slots are in the possible values of ontology in percentage.\n* non-cat slot span: how many values of non-categorical slots have span annotation in percentage.",
"### Licensing Information\n\n\nCC BY-SA 4.0"
] | [
60,
582,
24,
5,
431,
11
] | [
"passage: TAGS\n#task_categories-conversational #multilinguality-monolingual #size_categories-10K<n<100K #language-English #license-cc-by-sa-4.0 #arxiv-2110.06800 #region-us \n"
] |
014d75d58954ac8e6c6d4651781f582ce1beba9f | # Dataset Card for "scottishdirectories_loaded"
[More Information needed](https://github.com/huggingface/datasets/blob/main/CONTRIBUTING.md#how-to-contribute-to-the-dataset-cards) | davanstrien/scottishdirectories_loaded | [
"region:us"
] | 2022-11-22T14:58:56+00:00 | {"dataset_info": {"features": [{"name": "id", "dtype": "string"}, {"name": "image", "dtype": "string"}, {"name": "iiif_manifest", "dtype": "string"}, {"name": "loaded_image", "dtype": "image"}], "splits": [{"name": "train", "num_bytes": 178900224.0, "num_examples": 10000}], "download_size": 0, "dataset_size": 178900224.0}} | 2022-11-22T15:12:19+00:00 | [] | [] | TAGS
#region-us
| # Dataset Card for "scottishdirectories_loaded"
More Information needed | [
"# Dataset Card for \"scottishdirectories_loaded\"\n\nMore Information needed"
] | [
"TAGS\n#region-us \n",
"# Dataset Card for \"scottishdirectories_loaded\"\n\nMore Information needed"
] | [
6,
18
] | [
"passage: TAGS\n#region-us \n# Dataset Card for \"scottishdirectories_loaded\"\n\nMore Information needed"
] |
b4ae25d408bab7ffbdd9769ee8dc9901bb52f65b | # Dataset Card for "wnli_corpus_trainval"
[More Information needed](https://github.com/huggingface/datasets/blob/main/CONTRIBUTING.md#how-to-contribute-to-the-dataset-cards) | carlosejimenez/wnli_corpus_trainval | [
"region:us"
] | 2022-11-22T15:08:08+00:00 | {"dataset_info": {"features": [{"name": "idx", "dtype": "int64"}, {"name": "text", "dtype": "string"}], "splits": [{"name": "test", "num_bytes": 36867, "num_examples": 146}, {"name": "train", "num_bytes": 114329, "num_examples": 706}, {"name": "validation", "num_bytes": 11665, "num_examples": 71}], "download_size": 0, "dataset_size": 162861}} | 2022-11-22T15:16:36+00:00 | [] | [] | TAGS
#region-us
| # Dataset Card for "wnli_corpus_trainval"
More Information needed | [
"# Dataset Card for \"wnli_corpus_trainval\"\n\nMore Information needed"
] | [
"TAGS\n#region-us \n",
"# Dataset Card for \"wnli_corpus_trainval\"\n\nMore Information needed"
] | [
6,
19
] | [
"passage: TAGS\n#region-us \n# Dataset Card for \"wnli_corpus_trainval\"\n\nMore Information needed"
] |
be87f8e13ad17f06056599afe2f09afd63e235a5 | # Dataset Card for "cv11-it-dummy"
[More Information needed](https://github.com/huggingface/datasets/blob/main/CONTRIBUTING.md#how-to-contribute-to-the-dataset-cards) | nefasto/cv11-it-dummy | [
"region:us"
] | 2022-11-22T16:57:45+00:00 | {"dataset_info": {"features": [{"name": "client_id", "dtype": "string"}, {"name": "path", "dtype": "string"}, {"name": "audio", "dtype": {"audio": {"sampling_rate": 16000}}}, {"name": "sentence", "dtype": "string"}, {"name": "up_votes", "dtype": "int64"}, {"name": "down_votes", "dtype": "int64"}, {"name": "age", "dtype": "string"}, {"name": "gender", "dtype": "string"}, {"name": "accent", "dtype": "string"}, {"name": "locale", "dtype": "string"}, {"name": "segment", "dtype": "string"}], "splits": [{"name": "train", "num_bytes": 87250914.0, "num_examples": 500}, {"name": "validation", "num_bytes": 96093472.0, "num_examples": 500}, {"name": "test", "num_bytes": 59687501.0, "num_examples": 300}], "download_size": 225348877, "dataset_size": 243031887.0}} | 2022-11-23T14:04:07+00:00 | [] | [] | TAGS
#region-us
| # Dataset Card for "cv11-it-dummy"
More Information needed | [
"# Dataset Card for \"cv11-it-dummy\"\n\nMore Information needed"
] | [
"TAGS\n#region-us \n",
"# Dataset Card for \"cv11-it-dummy\"\n\nMore Information needed"
] | [
6,
18
] | [
"passage: TAGS\n#region-us \n# Dataset Card for \"cv11-it-dummy\"\n\nMore Information needed"
] |
b2153ceabfaef23105ad4821b8674e5c37c4bd4e | # Dataset Card for "elsalvadorgram"
[More Information needed](https://github.com/huggingface/datasets/blob/main/CONTRIBUTING.md#how-to-contribute-to-the-dataset-cards) | justinian336/elsalvadorgram | [
"region:us"
] | 2022-11-22T17:08:58+00:00 | {"dataset_info": {"features": [{"name": "image_src", "dtype": "string"}, {"name": "title", "dtype": "string"}, {"name": "content", "dtype": "string"}, {"name": "link", "dtype": "string"}], "splits": [{"name": "train", "num_bytes": 2532132, "num_examples": 1452}], "download_size": 1461097, "dataset_size": 2532132}} | 2022-11-22T17:09:44+00:00 | [] | [] | TAGS
#region-us
| # Dataset Card for "elsalvadorgram"
More Information needed | [
"# Dataset Card for \"elsalvadorgram\"\n\nMore Information needed"
] | [
"TAGS\n#region-us \n",
"# Dataset Card for \"elsalvadorgram\"\n\nMore Information needed"
] | [
6,
15
] | [
"passage: TAGS\n#region-us \n# Dataset Card for \"elsalvadorgram\"\n\nMore Information needed"
] |
08ed5ef9065162c163732712eed91438b31d755e |
# Dataset Card for "LENU - Legal Entity Name Understanding"
---------------
<h1 align="center">
<a href="https://gleif.org">
<img src="http://sdglabs.ai/wp-content/uploads/2022/07/gleif-logo-new.png" width="220px" style="display: inherit">
</a>
</h1><br>
<h3 align="center">in collaboration with</h3>
<h1 align="center">
<a href="https://sociovestix.com">
<img src="https://sociovestix.com/img/svl_logo_centered.svg" width="700px" style="width: 100%">
</a>
</h1><br>
---------------
## Table of Contents
- [Dataset Card Creation Guide](#dataset-card-creation-guide)
- [Table of Contents](#table-of-contents)
- [Dataset Description](#dataset-description)
- [Dataset Summary](#dataset-summary)
- [Languages](#languages)
- [Dataset Structure](#dataset-structure)
- [Data Instances](#data-instances)
- [Data Fields](#data-fields)
- [Data Splits](#data-splits)
- [Licensing Information](#licensing-information)
## Dataset Description
- **Homepage:** [gleif.org](https://gleif.org)
- **Repository:** [The LENU project](https://github.com/Sociovestix/lenu)
- **Point of Contact:** [aarimond](https://huggingface.co/aarimond)
### Dataset Summary
This dataset contains legal entity names from the Global LEI System in which each entity is
assigned with a unique
[Legal Entity Identifier](https://www.gleif.org/en/about-lei/introducing-the-legal-entity-identifier-lei)
(LEI) code (ISO Standard 17441)
along with their corresponding
[Entity Legal Form (ELF) Codes](https://www.gleif.org/en/about-lei/code-lists/iso-20275-entity-legal-forms-code-list)
(ISO Standard 20275),
which specifies the legal form of each entity.
The dataset has been created as part of a collaboration of the [Global Legal Entity Identifier Foundation](https://gleif.org) (GLEIF) and
[Sociovestix Labs](https://sociovestix.com) with the goal to explore how Machine Learning can support in detecting the ELF Code solely based on an entity's legal name and legal jurisdiction.
See also the open source python library [LENU](https://github.com/Sociovestix/lenu), which supports in this task.
The data is created from LEI data downloaded from
[GLEIF's public website](https://www.gleif.org/en/lei-data/gleif-golden-copy/download-the-golden-copy/)
(Date: 2022-11-01 00:00), where it is accessible free of charge.
It is divided into subsets for a selection of legal jurisdictions, whereas each Jurisdiction has its own set of ELF Codes.
The ELF Code reference list can be downloaded [here](https://www.gleif.org/en/about-lei/code-lists/iso-20275-entity-legal-forms-code-list).
### Languages
The data contains several major Jurisdictions (e.g. US-DE (US Delaware), JP (Japan), DE (Germany) and others).
Legal entity names usually follow certain language patterns, depending on which jurisdiction they are located in.
Thus, we apply models that are pre-trained on the corresponding language.
## Dataset Structure
### Data Instances
The data contains of the LEI, the corresponding legal name and ELF Code.
```
{
'LEI': '254900OMZ079O2SDWA75',
'Entity.LegalName': 'Park Reseda Mortgage LLC',
'Entity.LegalForm.EntityLegalFormCode': 0
}
```
### Data Fields
This is just a subset of available fields in the LEI system. All fields are described in detail in GLEIF's
[LEI Common Data Format (CDF)](https://www.gleif.org/en/about-lei/common-data-file-format/current-versions/level-1-data-lei-cdf-3-1-format).
- `LEI`: The [Legal Entity Identifier](https://www.gleif.org/en/about-lei/introducing-the-legal-entity-identifier-lei) Code. Uniquely identifies a Legal Entity.
- `Entity.LegalName`: The official name of the legal entity as registered in the LEI system.
- `Entity.LegalForm.EntityLegalFormCode`: class encoded column which contains the [Entity Legal Form Code](https://www.gleif.org/en/about-lei/code-lists/iso-20275-entity-legal-forms-code-list)
### Data Splits
We have divided each jurisdiction's subset into stratified train (70%), validation (10%) and test (20%) splits.
ELF Codes that appear less than three times in a Jurisdiction have been removed.
## Licensing Information
This dataset, which is based on LEI data, is available under Creative Commons (CC0) license.
See [gleif.org/en/about/open-data](https://gleif.org/en/about/open-data). | Sociovestix/lenu | [
"region:us"
] | 2022-11-22T17:24:58+00:00 | {"dataset_info": [{"config_name": "AT", "features": [{"name": "LEI", "dtype": "string"}, {"name": "Entity.LegalName", "dtype": "string"}, {"name": "Entity.LegalForm.EntityLegalFormCode", "dtype": {"class_label": {"names": {"0": "AXSB", "1": "EQOV", "2": "9999", "3": "ONF1", "4": "8888", "5": "DX6Z", "6": "JTAV", "7": "5WWO", "8": "ECWU", "9": "JJYT", "10": "E9OX", "11": "UI81", "12": "GVPD", "13": "NIJH", "14": "8XDW", "15": "1NOX", "16": "CAQ1", "17": "JQOI", "18": "O65B", "19": "69H1", "20": "G3R6"}}}}], "splits": [{"name": "train", "num_bytes": 1201165, "num_examples": 18373}, {"name": "validation", "num_bytes": 171437, "num_examples": 2625}, {"name": "test", "num_bytes": 343856, "num_examples": 5250}], "download_size": 347845208, "dataset_size": 1716458}, {"config_name": "AU", "features": [{"name": "LEI", "dtype": "string"}, {"name": "Entity.LegalName", "dtype": "string"}, {"name": "Entity.LegalForm.EntityLegalFormCode", "dtype": {"class_label": {"names": {"0": "TXVC", "1": "8888", "2": "ADXG", "3": "R4KK", "4": "7TPC", "5": "LZFR", "6": "BC38", "7": "J4JC", "8": "Q82Q", "9": "6W6X", "10": "XHCV", "11": "PQHL"}}}}], "splits": [{"name": "train", "num_bytes": 744518, "num_examples": 11162}, {"name": "validation", "num_bytes": 106753, "num_examples": 1595}, {"name": "test", "num_bytes": 213394, "num_examples": 3190}], "download_size": 347845208, "dataset_size": 1064665}, {"config_name": "CH", "features": [{"name": "LEI", "dtype": "string"}, {"name": "Entity.LegalName", "dtype": "string"}, {"name": "Entity.LegalForm.EntityLegalFormCode", "dtype": {"class_label": {"names": {"0": "3EKS", "1": "9999", "2": "7MNN", "3": "MVII", "4": "FJG4", "5": "8888", "6": "2JZ4", "7": "XJOT", "8": "FLNB", "9": "H781", "10": "QSI2", "11": "W6A7", "12": "L5DU", "13": "5BEZ", "14": "E0NE", "15": "AZA0", "16": "2B81", "17": "HX77", "18": "CQMY", "19": "MRSY", "20": "GP8M", "21": "FFTN", "22": "M848", "23": "TL87", "24": "2XJA", "25": "DP2E", "26": "BF9N"}}}}], "splits": [{"name": "train", "num_bytes": 615328, "num_examples": 9987}, {"name": "validation", "num_bytes": 88416, "num_examples": 1427}, {"name": "test", "num_bytes": 177116, "num_examples": 2854}], "download_size": 347845208, "dataset_size": 880860}, {"config_name": "CN", "features": [{"name": "LEI", "dtype": "string"}, {"name": "Entity.LegalName", "dtype": "string"}, {"name": "Entity.LegalForm.EntityLegalFormCode", "dtype": {"class_label": {"names": {"0": "ECAK", "1": "8888", "2": "6NSC", "3": "B5UZ", "4": "E4FG", "5": "2M6Y", "6": "1IWK", "7": "UMCR", "8": "I39S", "9": "GGZ5", "10": "9999", "11": "SH05", "12": "RV48", "13": "OH9O", "14": "YXJ5", "15": "CYV6", "16": "V816", "17": "BDTI", "18": "OMUD"}}}}], "splits": [{"name": "train", "num_bytes": 1010787, "num_examples": 13232}, {"name": "validation", "num_bytes": 144514, "num_examples": 1891}, {"name": "test", "num_bytes": 289477, "num_examples": 3781}], "download_size": 347845208, "dataset_size": 1444778}, {"config_name": "CZ", "features": [{"name": "LEI", "dtype": "string"}, {"name": "Entity.LegalName", "dtype": "string"}, {"name": "Entity.LegalForm.EntityLegalFormCode", "dtype": {"class_label": {"names": {"0": "9HLU", "1": "6CQN", "2": "9RVC", "3": "ZQO8", "4": "8888", "5": "QIEL", "6": "95G8", "7": "6D9L", "8": "3G3D", "9": "SNWJ", "10": "J8PB", "11": "JCAD", "12": "CATU", "13": "CIO8", "14": "QS6A", "15": "CD28", "16": "UFDA", "17": "7OZQ", "18": "6FAI", "19": "NI3I", "20": "QQ49", "21": "Q25I", "22": "5KU5", "23": "BL4B", "24": "G2I3", "25": "IQ9O", "26": "QJ0F", "27": "4UB2", "28": "FY1B", "29": "VIE3", "30": "OVKW", "31": "917C", "32": "LJL0", "33": "R2XE", "34": "MAVU", "35": "PFE5", "36": "MBUU", "37": "NQHQ", "38": "D1VK", "39": "HQPK", "40": "XG70", "41": "74W6", "42": "CZUA", "43": "NPH3", "44": "NJ87"}}}}], "splits": [{"name": "train", "num_bytes": 644303, "num_examples": 10922}, {"name": "validation", "num_bytes": 91310, "num_examples": 1561}, {"name": "test", "num_bytes": 183481, "num_examples": 3121}], "download_size": 347845208, "dataset_size": 919094}, {"config_name": "DE", "features": [{"name": "LEI", "dtype": "string"}, {"name": "Entity.LegalName", "dtype": "string"}, {"name": "Entity.LegalForm.EntityLegalFormCode", "dtype": {"class_label": {"names": {"0": "2HBR", "1": "6QQB", "2": "XLWA", "3": "8888", "4": "V2YH", "5": "QZ3L", "6": "63KS", "7": "SQKS", "8": "8Z6G", "9": "YJ4C", "10": "US8E", "11": "T0YJ", "12": "SCE1", "13": "FR3V", "14": "79H0", "15": "AZFE", "16": "40DB", "17": "2YZO", "18": "SGST", "19": "OL20", "20": "13AV", "21": "FEBD", "22": "9999", "23": "9JGX", "24": "D40E", "25": "8CM0", "26": "7J3S", "27": "JNDX", "28": "AMKW", "29": "SUA1", "30": "JMVF", "31": "YA01"}}}}], "splits": [{"name": "train", "num_bytes": 6971438, "num_examples": 104629}, {"name": "validation", "num_bytes": 994254, "num_examples": 14948}, {"name": "test", "num_bytes": 1990012, "num_examples": 29895}], "download_size": 347845208, "dataset_size": 9955704}, {"config_name": "DK", "features": [{"name": "LEI", "dtype": "string"}, {"name": "Entity.LegalName", "dtype": "string"}, {"name": "Entity.LegalForm.EntityLegalFormCode", "dtype": {"class_label": {"names": {"0": "H8VP", "1": "599X", "2": "ZRPO", "3": "D4PU", "4": "40R4", "5": "FUKI", "6": "PMJW", "7": "NUL8", "8": "8888", "9": "9KSX", "10": "7WRN", "11": "PIOI", "12": "PZ6Y", "13": "GFXN", "14": "9999", "15": "F7JY", "16": "37UT", "17": "1MWR", "18": "WU7R", "19": "GULL", "20": "FW7S", "21": "5QS7"}}}}], "splits": [{"name": "train", "num_bytes": 2389321, "num_examples": 41457}, {"name": "validation", "num_bytes": 342629, "num_examples": 5923}, {"name": "test", "num_bytes": 682202, "num_examples": 11846}], "download_size": 347845208, "dataset_size": 3414152}, {"config_name": "EE", "features": [{"name": "LEI", "dtype": "string"}, {"name": "Entity.LegalName", "dtype": "string"}, {"name": "Entity.LegalForm.EntityLegalFormCode", "dtype": {"class_label": {"names": {"0": "9LJA", "1": "JC0Y", "2": "PRTB", "3": "8888", "4": "LVEQ", "5": "1NKP", "6": "VSEV", "7": "BE56", "8": "I1UP", "9": "752Q", "10": "J34T", "11": "LA47", "12": "8ZQE", "13": "3UPJ"}}}}], "splits": [{"name": "train", "num_bytes": 570320, "num_examples": 11034}, {"name": "validation", "num_bytes": 81323, "num_examples": 1577}, {"name": "test", "num_bytes": 162403, "num_examples": 3153}], "download_size": 347845208, "dataset_size": 814046}, {"config_name": "ES", "features": [{"name": "LEI", "dtype": "string"}, {"name": "Entity.LegalName", "dtype": "string"}, {"name": "Entity.LegalForm.EntityLegalFormCode", "dtype": {"class_label": {"names": {"0": "5RDO", "1": "S0Z5", "2": "DP3Q", "3": "8888", "4": "R6UT", "5": "MDOL", "6": "UJ35", "7": "1QU8", "8": "FH4R", "9": "8EHB", "10": "S6MS", "11": "JB2M", "12": "CUIH", "13": "1G29", "14": "K0RI", "15": "GJL1", "16": "QMUM", "17": "956I", "18": "AXS5", "19": "JTV5", "20": "9FPZ", "21": "A0J6", "22": "TUHS", "23": "4SJR", "24": "S6X7", "25": "I2WU", "26": "A97B", "27": "AJ9U", "28": "IAS6", "29": "SS0L", "30": "ARDP", "31": "9999", "32": "7U8O", "33": "1SL4", "34": "1ZHJ", "35": "B0V5", "36": "TDD5", "37": "R2L8", "38": "4S57", "39": "DDES", "40": "IT6N", "41": "TLCJ", "42": "XYGP"}}}}], "splits": [{"name": "train", "num_bytes": 3982800, "num_examples": 64239}, {"name": "validation", "num_bytes": 568242, "num_examples": 9178}, {"name": "test", "num_bytes": 1138748, "num_examples": 18355}], "download_size": 347845208, "dataset_size": 5689790}, {"config_name": "FI", "features": [{"name": "LEI", "dtype": "string"}, {"name": "Entity.LegalName", "dtype": "string"}, {"name": "Entity.LegalForm.EntityLegalFormCode", "dtype": {"class_label": {"names": {"0": "DKUW", "1": "5WI2", "2": "K6VE", "3": "8888", "4": "1AFG", "5": "8WJ7", "6": "HEOB", "7": "V0TJ", "8": "UXEW", "9": "NV7C", "10": "XJH3", "11": "VOTI", "12": "9999", "13": "YK5G", "14": "2RK5", "15": "PPMX", "16": "BKVI", "17": "IYF9", "18": "BKQO", "19": "EE90", "20": "8HGS", "21": "4H61", "22": "DAFV", "23": "MRW9", "24": "ZMTL", "25": "SJL9", "26": "K09E", "27": "R39F", "28": "SDPE", "29": "N3LC", "30": "97PB", "31": "EDZP", "32": "6PEQ", "33": "DMT8", "34": "SKGX", "35": "KHI5", "36": "37GR", "37": "T3K4", "38": "HTT9", "39": "SQS1", "40": "OXLO", "41": "R6UB", "42": "9AUC", "43": "Z38E", "44": "DL9Z", "45": "760X", "46": "V42B", "47": "UMF0", "48": "1YIR"}}}}], "splits": [{"name": "train", "num_bytes": 1490715, "num_examples": 26753}, {"name": "validation", "num_bytes": 212745, "num_examples": 3822}, {"name": "test", "num_bytes": 425636, "num_examples": 7644}], "download_size": 347845208, "dataset_size": 2129096}, {"config_name": "GB", "features": [{"name": "LEI", "dtype": "string"}, {"name": "Entity.LegalName", "dtype": "string"}, {"name": "Entity.LegalForm.EntityLegalFormCode", "dtype": {"class_label": {"names": {"0": "8888", "1": "H0PO", "2": "B6ES", "3": "G12F", "4": "Z0EY", "5": "57V7", "6": "XLZV", "7": "AVYY", "8": "ID30", "9": "WBQU", "10": "VV0W", "11": "7T8N", "12": "STX7", "13": "JTCO", "14": "Q0M5", "15": "9B78", "16": "9999", "17": "17R0", "18": "4GJI", "19": "NBTW", "20": "E12O", "21": "BX6Y", "22": "IYXU", "23": "60IF", "24": "468Q", "25": "8CF0", "26": "ZQ6S", "27": "4A3J", "28": "TT2H"}}}}], "splits": [{"name": "train", "num_bytes": 3461241, "num_examples": 53616}, {"name": "validation", "num_bytes": 494134, "num_examples": 7660}, {"name": "test", "num_bytes": 989291, "num_examples": 15319}], "download_size": 347845208, "dataset_size": 4944666}, {"config_name": "HU", "features": [{"name": "LEI", "dtype": "string"}, {"name": "Entity.LegalName", "dtype": "string"}, {"name": "Entity.LegalForm.EntityLegalFormCode", "dtype": {"class_label": {"names": {"0": "P9F2", "1": "BKUX", "2": "8VH3", "3": "S3DA", "4": "EO9F", "5": "M1DW", "6": "8UEG", "7": "BJ8Q", "8": "BMYJ", "9": "TSVO", "10": "2A44", "11": "8888", "12": "DPY1", "13": "DN6F", "14": "QYV5", "15": "876R", "16": "4QRE", "17": "LNY0", "18": "ESTU", "19": "BSK1", "20": "4C5L", "21": "ZQAQ", "22": "2LB5", "23": "OII5", "24": "V3LT", "25": "4WV7", "26": "J6MO", "27": "XW5U", "28": "Y64R", "29": "995K", "30": "9999", "31": "HTJD"}}}}], "splits": [{"name": "train", "num_bytes": 847438, "num_examples": 8707}, {"name": "validation", "num_bytes": 121494, "num_examples": 1245}, {"name": "test", "num_bytes": 241600, "num_examples": 2489}], "download_size": 347845208, "dataset_size": 1210532}, {"config_name": "IE", "features": [{"name": "LEI", "dtype": "string"}, {"name": "Entity.LegalName", "dtype": "string"}, {"name": "Entity.LegalForm.EntityLegalFormCode", "dtype": {"class_label": {"names": {"0": "LGWG", "1": "8888", "2": "9999", "3": "MNQ7", "4": "VYAX", "5": "JXDX", "6": "KMFX", "7": "2GV9", "8": "LZIC", "9": "C58S", "10": "DWS3", "11": "HNJK", "12": "5AX8", "13": "54SK", "14": "URQH", "15": "9BPE", "16": "FF1D", "17": "ZJS8", "18": "363J"}}}}], "splits": [{"name": "train", "num_bytes": 831521, "num_examples": 11454}, {"name": "validation", "num_bytes": 118793, "num_examples": 1637}, {"name": "test", "num_bytes": 239755, "num_examples": 3273}], "download_size": 347845208, "dataset_size": 1190069}, {"config_name": "JP", "features": [{"name": "LEI", "dtype": "string"}, {"name": "Entity.LegalName", "dtype": "string"}, {"name": "Entity.LegalForm.EntityLegalFormCode", "dtype": {"class_label": {"names": {"0": "T417", "1": "8888", "2": "DYQK", "3": "7QQ0", "4": "N3JU", "5": "R4LR", "6": "9999", "7": "IUVI", "8": "MXMH", "9": "2NRQ", "10": "VQLD", "11": "5MVV"}}}}], "splits": [{"name": "train", "num_bytes": 640114, "num_examples": 7164}, {"name": "validation", "num_bytes": 91864, "num_examples": 1024}, {"name": "test", "num_bytes": 182503, "num_examples": 2047}], "download_size": 347845208, "dataset_size": 914481}, {"config_name": "KY", "features": [{"name": "LEI", "dtype": "string"}, {"name": "Entity.LegalName", "dtype": "string"}, {"name": "Entity.LegalForm.EntityLegalFormCode", "dtype": {"class_label": {"names": {"0": "8888", "1": "OSBR", "2": "XAQA", "3": "6XB7", "4": "9999", "5": "MPUG", "6": "MP7S", "7": "4XP8", "8": "K575", "9": "T5UM", "10": "JDX6", "11": "8HR7", "12": "SNUK"}}}}], "splits": [{"name": "train", "num_bytes": 1033926, "num_examples": 15052}, {"name": "validation", "num_bytes": 147417, "num_examples": 2151}, {"name": "test", "num_bytes": 295878, "num_examples": 4301}], "download_size": 347845208, "dataset_size": 1477221}, {"config_name": "LI", "features": [{"name": "LEI", "dtype": "string"}, {"name": "Entity.LegalName", "dtype": "string"}, {"name": "Entity.LegalForm.EntityLegalFormCode", "dtype": {"class_label": {"names": {"0": "TV8Y", "1": "TMU1", "2": "BSZ8", "3": "7RRP", "4": "1DGT", "5": "8888", "6": "53QF", "7": "WAK8", "8": "Y8LH", "9": "IF49", "10": "9999", "11": "32HC", "12": "EV7F", "13": "ANSR", "14": "1SOY"}}}}], "splits": [{"name": "train", "num_bytes": 368429, "num_examples": 6429}, {"name": "validation", "num_bytes": 52583, "num_examples": 919}, {"name": "test", "num_bytes": 105068, "num_examples": 1837}], "download_size": 347845208, "dataset_size": 526080}, {"config_name": "LU", "features": [{"name": "LEI", "dtype": "string"}, {"name": "Entity.LegalName", "dtype": "string"}, {"name": "Entity.LegalForm.EntityLegalFormCode", "dtype": {"class_label": {"names": {"0": "9999", "1": "DVXS", "2": "8888", "3": "UDY2", "4": "5GGB", "5": "U8KA", "6": "81G5", "7": "63P9", "8": "SQ1A", "9": "AIR5", "10": "WCEP", "11": "2JEI", "12": "HHR4", "13": "V19Y", "14": "BEAN", "15": "BKAB", "16": "STBC", "17": "V5OS", "18": "2S2U", "19": "ZFFA", "20": "ATQY", "21": "9C91", "22": "EUT4", "23": "V7QY", "24": "7SIZ", "25": "68J6", "26": "2IGL"}}}}], "splits": [{"name": "train", "num_bytes": 1590000, "num_examples": 24369}, {"name": "validation", "num_bytes": 226585, "num_examples": 3482}, {"name": "test", "num_bytes": 452662, "num_examples": 6963}], "download_size": 347845208, "dataset_size": 2269247}, {"config_name": "NL", "features": [{"name": "LEI", "dtype": "string"}, {"name": "Entity.LegalName", "dtype": "string"}, {"name": "Entity.LegalForm.EntityLegalFormCode", "dtype": {"class_label": {"names": {"0": "54M6", "1": "V44D", "2": "B5PM", "3": "8888", "4": "EZQW", "5": "JHK5", "6": "CODH", "7": "62Y3", "8": "NFFH", "9": "L7HX", "10": "A0W7", "11": "4QXM", "12": "8VFX", "13": "BBEB", "14": "9999", "15": "33MN", "16": "9AAK", "17": "DEO1", "18": "GNXT", "19": "M1IZ", "20": "UNJ2"}}}}], "splits": [{"name": "train", "num_bytes": 3911489, "num_examples": 66541}, {"name": "validation", "num_bytes": 557828, "num_examples": 9507}, {"name": "test", "num_bytes": 1118145, "num_examples": 19012}], "download_size": 347845208, "dataset_size": 5587462}, {"config_name": "NO", "features": [{"name": "LEI", "dtype": "string"}, {"name": "Entity.LegalName", "dtype": "string"}, {"name": "Entity.LegalForm.EntityLegalFormCode", "dtype": {"class_label": {"names": {"0": "YI42", "1": "O0EU", "2": "8888", "3": "EXD7", "4": "FSBD", "5": "3C7U", "6": "CF5L", "7": "LJJW", "8": "V06W", "9": "IQGE", "10": "KX7D", "11": "K5P8", "12": "8S9H", "13": "3L58", "14": "R71C", "15": "5ZTZ", "16": "BJ65", "17": "326Y", "18": "ZQ0Q", "19": "PB3V", "20": "M9IQ", "21": "9DI1", "22": "AEV1", "23": "50TD", "24": "9999", "25": "YTMC", "26": "Q0Q1"}}}}], "splits": [{"name": "train", "num_bytes": 1278071, "num_examples": 24343}, {"name": "validation", "num_bytes": 182433, "num_examples": 3478}, {"name": "test", "num_bytes": 364545, "num_examples": 6956}], "download_size": 347845208, "dataset_size": 1825049}, {"config_name": "PL", "features": [{"name": "LEI", "dtype": "string"}, {"name": "Entity.LegalName", "dtype": "string"}, {"name": "Entity.LegalForm.EntityLegalFormCode", "dtype": {"class_label": {"names": {"0": "FJ0E", "1": "O7XB", "2": "RBHP", "3": "BSJT", "4": "ZVVM", "5": "OMX0", "6": "96XK", "7": "8888", "8": "629I", "9": "H7OD", "10": "8TOF", "11": "WUJ2", "12": "T7PB", "13": "B21W", "14": "ZZKE", "15": "AL9T", "16": "13ZV", "17": "KM66", "18": "LT9U", "19": "JCKO", "20": "SVA3", "21": "SP4S", "22": "60BG", "23": "J3A3", "24": "3BJG", "25": "WNX1", "26": "QUX1", "27": "FQ5Y", "28": "5F76", "29": "WOK7", "30": "QYL4", "31": "GZE5", "32": "SMIS", "33": "CY1M", "34": "YLZL"}}}}], "splits": [{"name": "train", "num_bytes": 1198058, "num_examples": 14518}, {"name": "validation", "num_bytes": 170422, "num_examples": 2075}, {"name": "test", "num_bytes": 343274, "num_examples": 4149}], "download_size": 347845208, "dataset_size": 1711754}, {"config_name": "SE", "features": [{"name": "LEI", "dtype": "string"}, {"name": "Entity.LegalName", "dtype": "string"}, {"name": "Entity.LegalForm.EntityLegalFormCode", "dtype": {"class_label": {"names": {"0": "XJHM", "1": "BEAY", "2": "CX05", "3": "8888", "4": "E9BI", "5": "9999", "6": "BYQJ", "7": "OJ9I", "8": "1TN0", "9": "C61P", "10": "AZTO", "11": "2UAX", "12": "O1QI", "13": "SSOM", "14": "54P7", "15": "G04R", "16": "M0Y0", "17": "UKOL", "18": "381R", "19": "PDQ0", "20": "44CQ", "21": "WZDB", "22": "27AW"}}}}], "splits": [{"name": "train", "num_bytes": 2359501, "num_examples": 40536}, {"name": "validation", "num_bytes": 336916, "num_examples": 5792}, {"name": "test", "num_bytes": 674485, "num_examples": 11582}], "download_size": 347845208, "dataset_size": 3370902}, {"config_name": "US-CA", "features": [{"name": "LEI", "dtype": "string"}, {"name": "Entity.LegalName", "dtype": "string"}, {"name": "Entity.LegalForm.EntityLegalFormCode", "dtype": {"class_label": {"names": {"0": "8888", "1": "K7YU", "2": "5HQ4", "3": "H1UM", "4": "EI4J", "5": "7CDL", "6": "PZR6", "7": "SQ7B", "8": "CVXK", "9": "G1P6", "10": "KQXA", "11": "LYRX", "12": "9999", "13": "5Y1L", "14": "N295", "15": "BADE", "16": "O6AU"}}}}], "splits": [{"name": "train", "num_bytes": 235894, "num_examples": 3671}, {"name": "validation", "num_bytes": 33865, "num_examples": 525}, {"name": "test", "num_bytes": 67358, "num_examples": 1049}], "download_size": 347845208, "dataset_size": 337117}, {"config_name": "US-DE", "features": [{"name": "LEI", "dtype": "string"}, {"name": "Entity.LegalName", "dtype": "string"}, {"name": "Entity.LegalForm.EntityLegalFormCode", "dtype": {"class_label": {"names": {"0": "HZEH", "1": "4FSX", "2": "8888", "3": "T91T", "4": "XTIQ", "5": "QF4W", "6": "1HXP", "7": "TGMR", "8": "JU79", "9": "12N6", "10": "9999", "11": "9ASJ"}}}}], "splits": [{"name": "train", "num_bytes": 2140696, "num_examples": 33491}, {"name": "validation", "num_bytes": 306366, "num_examples": 4785}, {"name": "test", "num_bytes": 611142, "num_examples": 9570}], "download_size": 347845208, "dataset_size": 3058204}, {"config_name": "US-NY", "features": [{"name": "LEI", "dtype": "string"}, {"name": "Entity.LegalName", "dtype": "string"}, {"name": "Entity.LegalForm.EntityLegalFormCode", "dtype": {"class_label": {"names": {"0": "8888", "1": "51RC", "2": "PJ10", "3": "SDX0", "4": "BO6L", "5": "XIZI", "6": "M0ER", "7": "4VH5", "8": "9999", "9": "D6JI"}}}}], "splits": [{"name": "train", "num_bytes": 184734, "num_examples": 2927}, {"name": "validation", "num_bytes": 26223, "num_examples": 419}, {"name": "test", "num_bytes": 52788, "num_examples": 837}], "download_size": 347845208, "dataset_size": 263745}, {"config_name": "VG", "features": [{"name": "LEI", "dtype": "string"}, {"name": "Entity.LegalName", "dtype": "string"}, {"name": "Entity.LegalForm.EntityLegalFormCode", "dtype": {"class_label": {"names": {"0": "6EH6", "1": "8888", "2": "9999", "3": "YOP9", "4": "KORB", "5": "1GR6", "6": "Q62B", "7": "ZHED", "8": "N28C", "9": "BST2", "10": "GLCI", "11": "JS65"}}}}], "splits": [{"name": "train", "num_bytes": 644228, "num_examples": 10590}, {"name": "validation", "num_bytes": 91943, "num_examples": 1514}, {"name": "test", "num_bytes": 182814, "num_examples": 3026}], "download_size": 347845208, "dataset_size": 918985}, {"config_name": "ZA", "features": [{"name": "LEI", "dtype": "string"}, {"name": "Entity.LegalName", "dtype": "string"}, {"name": "Entity.LegalForm.EntityLegalFormCode", "dtype": {"class_label": {"names": {"0": "GQVQ", "1": "8888", "2": "XE4Z", "3": "3QSR", "4": "4YUU", "5": "R155", "6": "MZT6", "7": "J7L0", "8": "9999"}}}}], "splits": [{"name": "train", "num_bytes": 57638, "num_examples": 882}, {"name": "validation", "num_bytes": 8411, "num_examples": 127}, {"name": "test", "num_bytes": 16984, "num_examples": 253}], "download_size": 347845208, "dataset_size": 83033}]} | 2024-01-09T15:07:37+00:00 | [] | [] | TAGS
#region-us
|
# Dataset Card for "LENU - Legal Entity Name Understanding"
---------------
<h1 align="center">
<a href="URL">
<img src="URL width="220px" style="display: inherit">
</a>
</h1><br>
<h3 align="center">in collaboration with</h3>
<h1 align="center">
<a href="URL">
<img src="URL width="700px" style="width: 100%">
</a>
</h1><br>
---------------
## Table of Contents
- Dataset Card Creation Guide
- Table of Contents
- Dataset Description
- Dataset Summary
- Languages
- Dataset Structure
- Data Instances
- Data Fields
- Data Splits
- Licensing Information
## Dataset Description
- Homepage: URL
- Repository: The LENU project
- Point of Contact: aarimond
### Dataset Summary
This dataset contains legal entity names from the Global LEI System in which each entity is
assigned with a unique
Legal Entity Identifier
(LEI) code (ISO Standard 17441)
along with their corresponding
Entity Legal Form (ELF) Codes
(ISO Standard 20275),
which specifies the legal form of each entity.
The dataset has been created as part of a collaboration of the Global Legal Entity Identifier Foundation (GLEIF) and
Sociovestix Labs with the goal to explore how Machine Learning can support in detecting the ELF Code solely based on an entity's legal name and legal jurisdiction.
See also the open source python library LENU, which supports in this task.
The data is created from LEI data downloaded from
GLEIF's public website
(Date: 2022-11-01 00:00), where it is accessible free of charge.
It is divided into subsets for a selection of legal jurisdictions, whereas each Jurisdiction has its own set of ELF Codes.
The ELF Code reference list can be downloaded here.
### Languages
The data contains several major Jurisdictions (e.g. US-DE (US Delaware), JP (Japan), DE (Germany) and others).
Legal entity names usually follow certain language patterns, depending on which jurisdiction they are located in.
Thus, we apply models that are pre-trained on the corresponding language.
## Dataset Structure
### Data Instances
The data contains of the LEI, the corresponding legal name and ELF Code.
### Data Fields
This is just a subset of available fields in the LEI system. All fields are described in detail in GLEIF's
LEI Common Data Format (CDF).
- 'LEI': The Legal Entity Identifier Code. Uniquely identifies a Legal Entity.
- 'Entity.LegalName': The official name of the legal entity as registered in the LEI system.
- 'Entity.LegalForm.EntityLegalFormCode': class encoded column which contains the Entity Legal Form Code
### Data Splits
We have divided each jurisdiction's subset into stratified train (70%), validation (10%) and test (20%) splits.
ELF Codes that appear less than three times in a Jurisdiction have been removed.
## Licensing Information
This dataset, which is based on LEI data, is available under Creative Commons (CC0) license.
See URL | [
"# Dataset Card for \"LENU - Legal Entity Name Understanding\"\n\n---------------\n\n<h1 align=\"center\">\n<a href=\"URL\">\n<img src=\"URL width=\"220px\" style=\"display: inherit\">\n</a>\n</h1><br>\n<h3 align=\"center\">in collaboration with</h3> \n<h1 align=\"center\">\n<a href=\"URL\">\n<img src=\"URL width=\"700px\" style=\"width: 100%\">\n</a>\n</h1><br>\n\n---------------",
"## Table of Contents\n- Dataset Card Creation Guide\n - Table of Contents\n - Dataset Description\n - Dataset Summary\n - Languages\n - Dataset Structure\n - Data Instances\n - Data Fields\n - Data Splits\n- Licensing Information",
"## Dataset Description\n\n- Homepage: URL\n- Repository: The LENU project\n- Point of Contact: aarimond",
"### Dataset Summary\n\nThis dataset contains legal entity names from the Global LEI System in which each entity is\nassigned with a unique\nLegal Entity Identifier\n(LEI) code (ISO Standard 17441)\nalong with their corresponding\nEntity Legal Form (ELF) Codes\n(ISO Standard 20275),\nwhich specifies the legal form of each entity.\n\nThe dataset has been created as part of a collaboration of the Global Legal Entity Identifier Foundation (GLEIF) and\nSociovestix Labs with the goal to explore how Machine Learning can support in detecting the ELF Code solely based on an entity's legal name and legal jurisdiction.\nSee also the open source python library LENU, which supports in this task.\n\nThe data is created from LEI data downloaded from \nGLEIF's public website\n(Date: 2022-11-01 00:00), where it is accessible free of charge.\nIt is divided into subsets for a selection of legal jurisdictions, whereas each Jurisdiction has its own set of ELF Codes.\nThe ELF Code reference list can be downloaded here.",
"### Languages\n\nThe data contains several major Jurisdictions (e.g. US-DE (US Delaware), JP (Japan), DE (Germany) and others).\nLegal entity names usually follow certain language patterns, depending on which jurisdiction they are located in.\nThus, we apply models that are pre-trained on the corresponding language.",
"## Dataset Structure",
"### Data Instances\n\nThe data contains of the LEI, the corresponding legal name and ELF Code.",
"### Data Fields\n\nThis is just a subset of available fields in the LEI system. All fields are described in detail in GLEIF's \nLEI Common Data Format (CDF).\n\n- 'LEI': The Legal Entity Identifier Code. Uniquely identifies a Legal Entity.\n- 'Entity.LegalName': The official name of the legal entity as registered in the LEI system.\n- 'Entity.LegalForm.EntityLegalFormCode': class encoded column which contains the Entity Legal Form Code",
"### Data Splits\n\nWe have divided each jurisdiction's subset into stratified train (70%), validation (10%) and test (20%) splits.\nELF Codes that appear less than three times in a Jurisdiction have been removed.",
"## Licensing Information\n\nThis dataset, which is based on LEI data, is available under Creative Commons (CC0) license. \nSee URL"
] | [
"TAGS\n#region-us \n",
"# Dataset Card for \"LENU - Legal Entity Name Understanding\"\n\n---------------\n\n<h1 align=\"center\">\n<a href=\"URL\">\n<img src=\"URL width=\"220px\" style=\"display: inherit\">\n</a>\n</h1><br>\n<h3 align=\"center\">in collaboration with</h3> \n<h1 align=\"center\">\n<a href=\"URL\">\n<img src=\"URL width=\"700px\" style=\"width: 100%\">\n</a>\n</h1><br>\n\n---------------",
"## Table of Contents\n- Dataset Card Creation Guide\n - Table of Contents\n - Dataset Description\n - Dataset Summary\n - Languages\n - Dataset Structure\n - Data Instances\n - Data Fields\n - Data Splits\n- Licensing Information",
"## Dataset Description\n\n- Homepage: URL\n- Repository: The LENU project\n- Point of Contact: aarimond",
"### Dataset Summary\n\nThis dataset contains legal entity names from the Global LEI System in which each entity is\nassigned with a unique\nLegal Entity Identifier\n(LEI) code (ISO Standard 17441)\nalong with their corresponding\nEntity Legal Form (ELF) Codes\n(ISO Standard 20275),\nwhich specifies the legal form of each entity.\n\nThe dataset has been created as part of a collaboration of the Global Legal Entity Identifier Foundation (GLEIF) and\nSociovestix Labs with the goal to explore how Machine Learning can support in detecting the ELF Code solely based on an entity's legal name and legal jurisdiction.\nSee also the open source python library LENU, which supports in this task.\n\nThe data is created from LEI data downloaded from \nGLEIF's public website\n(Date: 2022-11-01 00:00), where it is accessible free of charge.\nIt is divided into subsets for a selection of legal jurisdictions, whereas each Jurisdiction has its own set of ELF Codes.\nThe ELF Code reference list can be downloaded here.",
"### Languages\n\nThe data contains several major Jurisdictions (e.g. US-DE (US Delaware), JP (Japan), DE (Germany) and others).\nLegal entity names usually follow certain language patterns, depending on which jurisdiction they are located in.\nThus, we apply models that are pre-trained on the corresponding language.",
"## Dataset Structure",
"### Data Instances\n\nThe data contains of the LEI, the corresponding legal name and ELF Code.",
"### Data Fields\n\nThis is just a subset of available fields in the LEI system. All fields are described in detail in GLEIF's \nLEI Common Data Format (CDF).\n\n- 'LEI': The Legal Entity Identifier Code. Uniquely identifies a Legal Entity.\n- 'Entity.LegalName': The official name of the legal entity as registered in the LEI system.\n- 'Entity.LegalForm.EntityLegalFormCode': class encoded column which contains the Entity Legal Form Code",
"### Data Splits\n\nWe have divided each jurisdiction's subset into stratified train (70%), validation (10%) and test (20%) splits.\nELF Codes that appear less than three times in a Jurisdiction have been removed.",
"## Licensing Information\n\nThis dataset, which is based on LEI data, is available under Creative Commons (CC0) license. \nSee URL"
] | [
6,
130,
53,
25,
241,
80,
6,
25,
129,
54,
29
] | [
"passage: TAGS\n#region-us \n# Dataset Card for \"LENU - Legal Entity Name Understanding\"\n\n---------------\n\n<h1 align=\"center\">\n<a href=\"URL\">\n<img src=\"URL width=\"220px\" style=\"display: inherit\">\n</a>\n</h1><br>\n<h3 align=\"center\">in collaboration with</h3> \n<h1 align=\"center\">\n<a href=\"URL\">\n<img src=\"URL width=\"700px\" style=\"width: 100%\">\n</a>\n</h1><br>\n\n---------------## Table of Contents\n- Dataset Card Creation Guide\n - Table of Contents\n - Dataset Description\n - Dataset Summary\n - Languages\n - Dataset Structure\n - Data Instances\n - Data Fields\n - Data Splits\n- Licensing Information## Dataset Description\n\n- Homepage: URL\n- Repository: The LENU project\n- Point of Contact: aarimond### Dataset Summary\n\nThis dataset contains legal entity names from the Global LEI System in which each entity is\nassigned with a unique\nLegal Entity Identifier\n(LEI) code (ISO Standard 17441)\nalong with their corresponding\nEntity Legal Form (ELF) Codes\n(ISO Standard 20275),\nwhich specifies the legal form of each entity.\n\nThe dataset has been created as part of a collaboration of the Global Legal Entity Identifier Foundation (GLEIF) and\nSociovestix Labs with the goal to explore how Machine Learning can support in detecting the ELF Code solely based on an entity's legal name and legal jurisdiction.\nSee also the open source python library LENU, which supports in this task.\n\nThe data is created from LEI data downloaded from \nGLEIF's public website\n(Date: 2022-11-01 00:00), where it is accessible free of charge.\nIt is divided into subsets for a selection of legal jurisdictions, whereas each Jurisdiction has its own set of ELF Codes.\nThe ELF Code reference list can be downloaded here."
] |
7a8633084e1b17c20ae53788b4421eff7165fbc8 | # Dataset Card for "the_verge-linustechtips-two_min"
[More Information needed](https://github.com/huggingface/datasets/blob/main/CONTRIBUTING.md#how-to-contribute-to-the-dataset-cards) | kpriyanshu256/the_verge-linustechtips-two_min | [
"region:us"
] | 2022-11-22T17:47:50+00:00 | {"dataset_info": {"features": [{"name": "text", "dtype": "string"}], "splits": [{"name": "train", "num_bytes": 109422333, "num_examples": 10489}], "download_size": 61977808, "dataset_size": 109422333}} | 2022-11-22T17:48:07+00:00 | [] | [] | TAGS
#region-us
| # Dataset Card for "the_verge-linustechtips-two_min"
More Information needed | [
"# Dataset Card for \"the_verge-linustechtips-two_min\"\n\nMore Information needed"
] | [
"TAGS\n#region-us \n",
"# Dataset Card for \"the_verge-linustechtips-two_min\"\n\nMore Information needed"
] | [
6,
22
] | [
"passage: TAGS\n#region-us \n# Dataset Card for \"the_verge-linustechtips-two_min\"\n\nMore Information needed"
] |
7b33a71effcedab1f8ddcd4012b9e3547f53eed7 |
# Dataset Card for Rakeffet
| izzy-lazerson/rakeffet | [
"task_categories:automatic-speech-recognition",
"task_categories:audio-classification",
"annotations_creators:expert-generated",
"language_creators:expert-generated",
"multilinguality:monolingual",
"source_datasets:original",
"language:en",
"license:cc-by-nc-4.0",
"region:us"
] | 2022-11-22T18:37:59+00:00 | {"annotations_creators": ["expert-generated"], "language_creators": ["expert-generated"], "language": ["en"], "license": ["cc-by-nc-4.0"], "multilinguality": ["monolingual"], "source_datasets": ["original"], "task_categories": ["automatic-speech-recognition", "audio-classification"], "pretty_name": "Rakeffet"} | 2022-11-22T18:53:48+00:00 | [] | [
"en"
] | TAGS
#task_categories-automatic-speech-recognition #task_categories-audio-classification #annotations_creators-expert-generated #language_creators-expert-generated #multilinguality-monolingual #source_datasets-original #language-English #license-cc-by-nc-4.0 #region-us
|
# Dataset Card for Rakeffet
| [
"# Dataset Card for Rakeffet"
] | [
"TAGS\n#task_categories-automatic-speech-recognition #task_categories-audio-classification #annotations_creators-expert-generated #language_creators-expert-generated #multilinguality-monolingual #source_datasets-original #language-English #license-cc-by-nc-4.0 #region-us \n",
"# Dataset Card for Rakeffet"
] | [
89,
7
] | [
"passage: TAGS\n#task_categories-automatic-speech-recognition #task_categories-audio-classification #annotations_creators-expert-generated #language_creators-expert-generated #multilinguality-monolingual #source_datasets-original #language-English #license-cc-by-nc-4.0 #region-us \n# Dataset Card for Rakeffet"
] |
ae949d5da481b429bb154bffc689e05821f6da27 | # Dataset Card for "SST2_train67k_test1.8k_valid0.8k"
[More Information needed](https://github.com/huggingface/datasets/blob/main/CONTRIBUTING.md#how-to-contribute-to-the-dataset-cards) | pig4431/SST2_train67k_test1.8k_valid0.8k | [
"region:us"
] | 2022-11-22T18:41:35+00:00 | {"dataset_info": {"features": [{"name": "text", "dtype": "string"}, {"name": "label", "dtype": "int64"}, {"name": "label_text", "dtype": "string"}], "splits": [{"name": "validate", "num_bytes": 112712, "num_examples": 872}, {"name": "test", "num_bytes": 231803, "num_examples": 1821}, {"name": "train", "num_bytes": 884164, "num_examples": 6920}, {"name": "validation", "num_bytes": 112712, "num_examples": 872}], "download_size": 795853, "dataset_size": 1341391}} | 2023-03-17T17:06:32+00:00 | [] | [] | TAGS
#region-us
| # Dataset Card for "SST2_train67k_test1.8k_valid0.8k"
More Information needed | [
"# Dataset Card for \"SST2_train67k_test1.8k_valid0.8k\"\n\nMore Information needed"
] | [
"TAGS\n#region-us \n",
"# Dataset Card for \"SST2_train67k_test1.8k_valid0.8k\"\n\nMore Information needed"
] | [
6,
27
] | [
"passage: TAGS\n#region-us \n# Dataset Card for \"SST2_train67k_test1.8k_valid0.8k\"\n\nMore Information needed"
] |
7185c715399d999e7c3ab2d29ff4462ae48feb03 | # Dataset Card for "AmazonPolarity_train25k_test5k_valid5k"
[More Information needed](https://github.com/huggingface/datasets/blob/main/CONTRIBUTING.md#how-to-contribute-to-the-dataset-cards) | pig4431/AmazonPolarity_train25k_test5k_valid5k | [
"region:us"
] | 2022-11-22T18:49:49+00:00 | {"dataset_info": {"features": [{"name": "label", "dtype": {"class_label": {"names": {"0": "negative", "1": "positive"}}}}, {"name": "text", "dtype": "string"}], "splits": [{"name": "validate", "num_bytes": 2047050, "num_examples": 5000}, {"name": "test", "num_bytes": 2061545, "num_examples": 5000}, {"name": "train", "num_bytes": 10447565, "num_examples": 25000}], "download_size": 9629583, "dataset_size": 14556160}} | 2023-03-17T17:11:56+00:00 | [] | [] | TAGS
#region-us
| # Dataset Card for "AmazonPolarity_train25k_test5k_valid5k"
More Information needed | [
"# Dataset Card for \"AmazonPolarity_train25k_test5k_valid5k\"\n\nMore Information needed"
] | [
"TAGS\n#region-us \n",
"# Dataset Card for \"AmazonPolarity_train25k_test5k_valid5k\"\n\nMore Information needed"
] | [
6,
28
] | [
"passage: TAGS\n#region-us \n# Dataset Card for \"AmazonPolarity_train25k_test5k_valid5k\"\n\nMore Information needed"
] |
21ede2a2a6f6b163cd8bcf298f8b07534e20d139 | # Dataset Card for "yelp_train25k_test5k_valid5k"
[More Information needed](https://github.com/huggingface/datasets/blob/main/CONTRIBUTING.md#how-to-contribute-to-the-dataset-cards) | pig4431/yelp_train25k_test5k_valid5k | [
"region:us"
] | 2022-11-22T18:50:45+00:00 | {"dataset_info": {"features": [{"name": "label", "dtype": {"class_label": {"names": {"0": "1 star", "1": "2 star", "2": "3 stars", "3": "4 stars", "4": "5 stars"}}}}, {"name": "text", "dtype": "string"}], "splits": [{"name": "validate", "num_bytes": 3646233, "num_examples": 5000}, {"name": "test", "num_bytes": 3701515, "num_examples": 5000}, {"name": "train", "num_bytes": 18186886, "num_examples": 25000}], "download_size": 16341897, "dataset_size": 25534634}} | 2023-03-17T17:10:18+00:00 | [] | [] | TAGS
#region-us
| # Dataset Card for "yelp_train25k_test5k_valid5k"
More Information needed | [
"# Dataset Card for \"yelp_train25k_test5k_valid5k\"\n\nMore Information needed"
] | [
"TAGS\n#region-us \n",
"# Dataset Card for \"yelp_train25k_test5k_valid5k\"\n\nMore Information needed"
] | [
6,
26
] | [
"passage: TAGS\n#region-us \n# Dataset Card for \"yelp_train25k_test5k_valid5k\"\n\nMore Information needed"
] |
35b1c097807e0b07ec5313879b85956b7b3890db |
# PubTables-1M
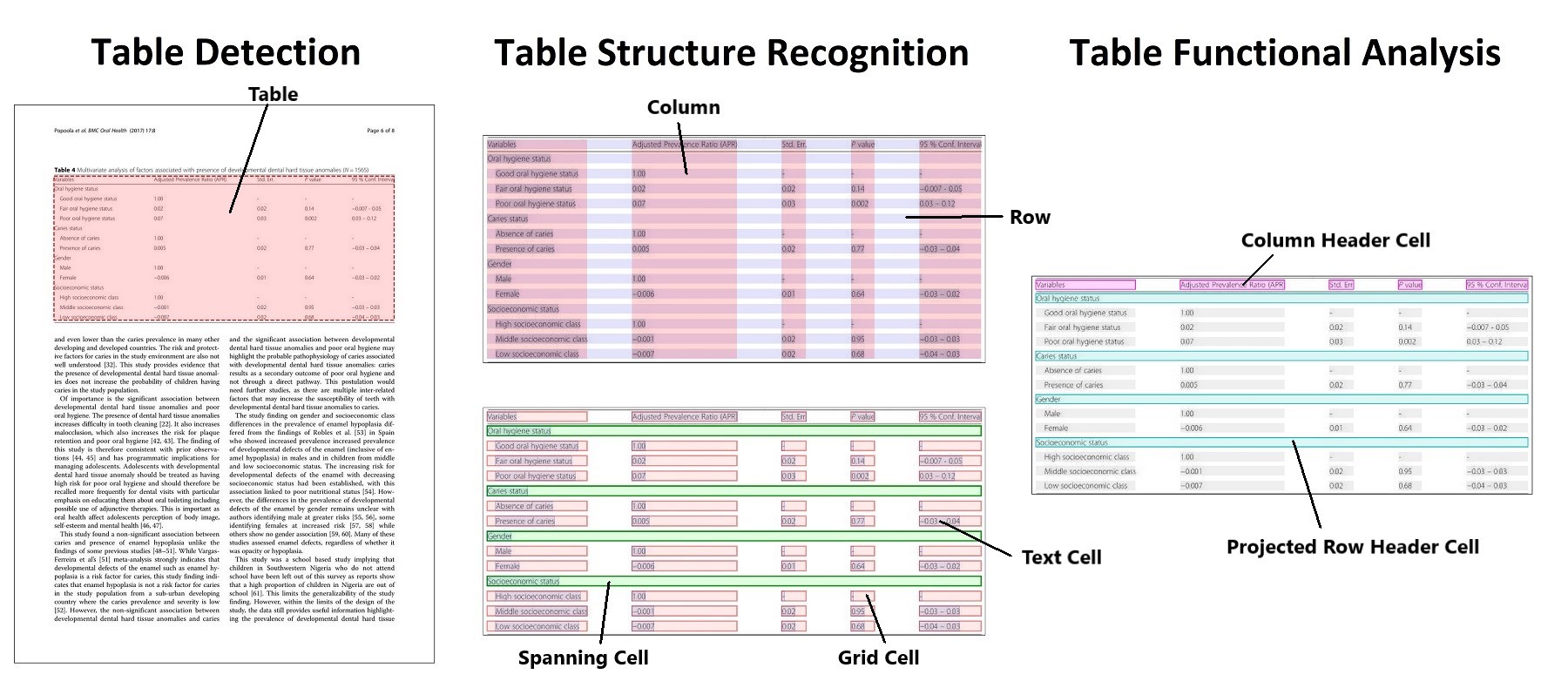
- GitHub: [https://github.com/microsoft/table-transformer](https://github.com/microsoft/table-transformer)
- Paper: ["PubTables-1M: Towards comprehensive table extraction from unstructured documents"](https://openaccess.thecvf.com/content/CVPR2022/html/Smock_PubTables-1M_Towards_Comprehensive_Table_Extraction_From_Unstructured_Documents_CVPR_2022_paper.html)
- Hugging Face:
- [Detection model](https://huggingface.co/microsoft/table-transformer-detection)
- [Structure recognition model](https://huggingface.co/microsoft/table-transformer-structure-recognition)
Currently we only support downloading the dataset as tar.gz files. Integrating with HuggingFace Datasets is something we hope to support in the future!
Please switch to the "Files and versions" tab to download all of the files or use a command such as wget to download from the command line.
Once downloaded, use the included script "extract_structure_dataset.sh" to extract and organize all of the data.
## Files
It comes in 18 tar.gz files:
Training and evaluation data for the structure recognition model (947,642 total cropped table instances):
- PubTables-1M-Structure_Filelists.tar.gz
- PubTables-1M-Structure_Annotations_Test.tar.gz: 93,834 XML files containing bounding boxes in PASCAL VOC format
- PubTables-1M-Structure_Annotations_Train.tar.gz: 758,849 XML files containing bounding boxes in PASCAL VOC format
- PubTables-1M-Structure_Annotations_Val.tar.gz: 94,959 XML files containing bounding boxes in PASCAL VOC format
- PubTables-1M-Structure_Images_Test.tar.gz
- PubTables-1M-Structure_Images_Train.tar.gz
- PubTables-1M-Structure_Images_Val.tar.gz
- PubTables-1M-Structure_Table_Words.tar.gz: Bounding boxes and text content for all of the words in each cropped table image
Training and evaluation data for the detection model (575,305 total document page instances):
- PubTables-1M-Detection_Filelists.tar.gz
- PubTables-1M-Detection_Annotations_Test.tar.gz: 57,125 XML files containing bounding boxes in PASCAL VOC format
- PubTables-1M-Detection_Annotations_Train.tar.gz: 460,589 XML files containing bounding boxes in PASCAL VOC format
- PubTables-1M-Detection_Annotations_Val.tar.gz: 57,591 XML files containing bounding boxes in PASCAL VOC format
- PubTables-1M-Detection_Images_Test.tar.gz
- PubTables-1M-Detection_Images_Train_Part1.tar.gz
- PubTables-1M-Detection_Images_Train_Part2.tar.gz
- PubTables-1M-Detection_Images_Val.tar.gz
- PubTables-1M-Detection_Page_Words.tar.gz: Bounding boxes and text content for all of the words in each page image (plus some unused files)
Full table annotations for the source PDF files:
- PubTables-1M-PDF_Annotations.tar.gz: Detailed annotations for all of the tables appearing in the source PubMed PDFs. All annotations are in PDF coordinates.
- 401,733 JSON files, one per source PDF document | bsmock/pubtables-1m | [
"license:cdla-permissive-2.0",
"region:us"
] | 2022-11-22T18:59:39+00:00 | {"license": "cdla-permissive-2.0"} | 2023-08-08T15:43:14+00:00 | [] | [] | TAGS
#license-cdla-permissive-2.0 #region-us
|
# PubTables-1M
!table_extraction_v2
- GitHub: URL
- Paper: "PubTables-1M: Towards comprehensive table extraction from unstructured documents"
- Hugging Face:
- Detection model
- Structure recognition model
Currently we only support downloading the dataset as URL files. Integrating with HuggingFace Datasets is something we hope to support in the future!
Please switch to the "Files and versions" tab to download all of the files or use a command such as wget to download from the command line.
Once downloaded, use the included script "extract_structure_dataset.sh" to extract and organize all of the data.
## Files
It comes in 18 URL files:
Training and evaluation data for the structure recognition model (947,642 total cropped table instances):
- PubTables-1M-Structure_Filelists.URL
- PubTables-1M-Structure_Annotations_Test.URL: 93,834 XML files containing bounding boxes in PASCAL VOC format
- PubTables-1M-Structure_Annotations_Train.URL: 758,849 XML files containing bounding boxes in PASCAL VOC format
- PubTables-1M-Structure_Annotations_Val.URL: 94,959 XML files containing bounding boxes in PASCAL VOC format
- PubTables-1M-Structure_Images_Test.URL
- PubTables-1M-Structure_Images_Train.URL
- PubTables-1M-Structure_Images_Val.URL
- PubTables-1M-Structure_Table_Words.URL: Bounding boxes and text content for all of the words in each cropped table image
Training and evaluation data for the detection model (575,305 total document page instances):
- PubTables-1M-Detection_Filelists.URL
- PubTables-1M-Detection_Annotations_Test.URL: 57,125 XML files containing bounding boxes in PASCAL VOC format
- PubTables-1M-Detection_Annotations_Train.URL: 460,589 XML files containing bounding boxes in PASCAL VOC format
- PubTables-1M-Detection_Annotations_Val.URL: 57,591 XML files containing bounding boxes in PASCAL VOC format
- PubTables-1M-Detection_Images_Test.URL
- PubTables-1M-Detection_Images_Train_Part1.URL
- PubTables-1M-Detection_Images_Train_Part2.URL
- PubTables-1M-Detection_Images_Val.URL
- PubTables-1M-Detection_Page_Words.URL: Bounding boxes and text content for all of the words in each page image (plus some unused files)
Full table annotations for the source PDF files:
- PubTables-1M-PDF_Annotations.URL: Detailed annotations for all of the tables appearing in the source PubMed PDFs. All annotations are in PDF coordinates.
- 401,733 JSON files, one per source PDF document | [
"# PubTables-1M\n\n!table_extraction_v2\n\n- GitHub: URL\n- Paper: \"PubTables-1M: Towards comprehensive table extraction from unstructured documents\"\n- Hugging Face:\n - Detection model\n - Structure recognition model\n\nCurrently we only support downloading the dataset as URL files. Integrating with HuggingFace Datasets is something we hope to support in the future!\n\nPlease switch to the \"Files and versions\" tab to download all of the files or use a command such as wget to download from the command line.\n\nOnce downloaded, use the included script \"extract_structure_dataset.sh\" to extract and organize all of the data.",
"## Files\n\nIt comes in 18 URL files:\n\nTraining and evaluation data for the structure recognition model (947,642 total cropped table instances):\n- PubTables-1M-Structure_Filelists.URL\n- PubTables-1M-Structure_Annotations_Test.URL: 93,834 XML files containing bounding boxes in PASCAL VOC format\n- PubTables-1M-Structure_Annotations_Train.URL: 758,849 XML files containing bounding boxes in PASCAL VOC format\n- PubTables-1M-Structure_Annotations_Val.URL: 94,959 XML files containing bounding boxes in PASCAL VOC format\n- PubTables-1M-Structure_Images_Test.URL\n- PubTables-1M-Structure_Images_Train.URL\n- PubTables-1M-Structure_Images_Val.URL\n- PubTables-1M-Structure_Table_Words.URL: Bounding boxes and text content for all of the words in each cropped table image\n\nTraining and evaluation data for the detection model (575,305 total document page instances):\n- PubTables-1M-Detection_Filelists.URL\n- PubTables-1M-Detection_Annotations_Test.URL: 57,125 XML files containing bounding boxes in PASCAL VOC format\n- PubTables-1M-Detection_Annotations_Train.URL: 460,589 XML files containing bounding boxes in PASCAL VOC format\n- PubTables-1M-Detection_Annotations_Val.URL: 57,591 XML files containing bounding boxes in PASCAL VOC format\n- PubTables-1M-Detection_Images_Test.URL\n- PubTables-1M-Detection_Images_Train_Part1.URL\n- PubTables-1M-Detection_Images_Train_Part2.URL\n- PubTables-1M-Detection_Images_Val.URL\n- PubTables-1M-Detection_Page_Words.URL: Bounding boxes and text content for all of the words in each page image (plus some unused files)\n\nFull table annotations for the source PDF files:\n- PubTables-1M-PDF_Annotations.URL: Detailed annotations for all of the tables appearing in the source PubMed PDFs. All annotations are in PDF coordinates.\n - 401,733 JSON files, one per source PDF document"
] | [
"TAGS\n#license-cdla-permissive-2.0 #region-us \n",
"# PubTables-1M\n\n!table_extraction_v2\n\n- GitHub: URL\n- Paper: \"PubTables-1M: Towards comprehensive table extraction from unstructured documents\"\n- Hugging Face:\n - Detection model\n - Structure recognition model\n\nCurrently we only support downloading the dataset as URL files. Integrating with HuggingFace Datasets is something we hope to support in the future!\n\nPlease switch to the \"Files and versions\" tab to download all of the files or use a command such as wget to download from the command line.\n\nOnce downloaded, use the included script \"extract_structure_dataset.sh\" to extract and organize all of the data.",
"## Files\n\nIt comes in 18 URL files:\n\nTraining and evaluation data for the structure recognition model (947,642 total cropped table instances):\n- PubTables-1M-Structure_Filelists.URL\n- PubTables-1M-Structure_Annotations_Test.URL: 93,834 XML files containing bounding boxes in PASCAL VOC format\n- PubTables-1M-Structure_Annotations_Train.URL: 758,849 XML files containing bounding boxes in PASCAL VOC format\n- PubTables-1M-Structure_Annotations_Val.URL: 94,959 XML files containing bounding boxes in PASCAL VOC format\n- PubTables-1M-Structure_Images_Test.URL\n- PubTables-1M-Structure_Images_Train.URL\n- PubTables-1M-Structure_Images_Val.URL\n- PubTables-1M-Structure_Table_Words.URL: Bounding boxes and text content for all of the words in each cropped table image\n\nTraining and evaluation data for the detection model (575,305 total document page instances):\n- PubTables-1M-Detection_Filelists.URL\n- PubTables-1M-Detection_Annotations_Test.URL: 57,125 XML files containing bounding boxes in PASCAL VOC format\n- PubTables-1M-Detection_Annotations_Train.URL: 460,589 XML files containing bounding boxes in PASCAL VOC format\n- PubTables-1M-Detection_Annotations_Val.URL: 57,591 XML files containing bounding boxes in PASCAL VOC format\n- PubTables-1M-Detection_Images_Test.URL\n- PubTables-1M-Detection_Images_Train_Part1.URL\n- PubTables-1M-Detection_Images_Train_Part2.URL\n- PubTables-1M-Detection_Images_Val.URL\n- PubTables-1M-Detection_Page_Words.URL: Bounding boxes and text content for all of the words in each page image (plus some unused files)\n\nFull table annotations for the source PDF files:\n- PubTables-1M-PDF_Annotations.URL: Detailed annotations for all of the tables appearing in the source PubMed PDFs. All annotations are in PDF coordinates.\n - 401,733 JSON files, one per source PDF document"
] | [
18,
154,
560
] | [
"passage: TAGS\n#license-cdla-permissive-2.0 #region-us \n# PubTables-1M\n\n!table_extraction_v2\n\n- GitHub: URL\n- Paper: \"PubTables-1M: Towards comprehensive table extraction from unstructured documents\"\n- Hugging Face:\n - Detection model\n - Structure recognition model\n\nCurrently we only support downloading the dataset as URL files. Integrating with HuggingFace Datasets is something we hope to support in the future!\n\nPlease switch to the \"Files and versions\" tab to download all of the files or use a command such as wget to download from the command line.\n\nOnce downloaded, use the included script \"extract_structure_dataset.sh\" to extract and organize all of the data."
] |
dd2ea994543cf19cdefde99ac72be3a4acc058e5 | # AutoTrain Dataset for project: healthcare_summarization_uta
## Dataset Description
This dataset has been automatically processed by AutoTrain for project healthcare_summarization_uta.
### Languages
The BCP-47 code for the dataset's language is en.
## Dataset Structure
### Data Instances
A sample from this dataset looks as follows:
```json
[
{
"text": "We get people to do things that are good for them. And you. | Make the world a healthier place, one person at a time. | Healthcare today is nothing short of amazing. Yet all of it only works when people connect with it. And too often, they dont. Healthcare can be impersonal. Confusing. All elbows. The record scratch at lifes party. Were here to help connect healthcare with the people who need it. Which is everyone. How? By listening. Collaborating. And inspiring. Were pioneering a better way forward. Were making healthcare more human. | In 2020, Revel + NovuHealth joined forces to create Icario because we knew we could do better togethercreating value by uniting pioneering technology, data science, and behavioral insights to make the world a healthier place, one person at a time. | There is an island in the Aegean Sea where people live extremely long lives. Theyre happy, too. Families are close. They eat well. They exercise. And they stay connected with each other, and not just by smartphone. This got us thinking. What if we apply what we learn from the Blue Zone island of Ikaria (our namesake), add pioneering technology and exabytes of data, and help healthcare connect better with everyone? Well have a lot more healthy, happy people, and thats a pretty good thing. | As an organization, were a collaborative team of pioneers, inventors, and systems thinkers. We speak truth, are driven by data, and sweat the details. Were a friendly and easygoing group, but we work hard because we are mission-driven, we know a better way, and were here to make it happen. | The Icario name and brand represent a successful, growing business that deeply understands people and is focused on making healthcare more human through personalized communication. | ",
"target": "We're putting people first in healthcare. In order to create value by fusing cutting-edge technology, data science, and behavioral insights to improve the world's health one person at a time, Revel and NovuHealth partnered to become Icario in 2020. The Icario name and brand speak for a prosperous, expanding company that has a keen understanding of people and is committed to enhancing healthcare through individualized communication."
},
{
"text": "Medicat is the industry leader in College Health Software and serves more college and university campuses than all other college health software companies combined. From the largest universities to the smallest colleges, Medicat specializes in workflow efficiency and has been improving outcomes for campus health centers since 1993. | Over 500 clients, covering 5+ million students across 48 states and three countries, use Medicats total EHR solution to deliver higher quality care more efficiently with the industrys most secure software platform offerings. Medicat offers private cloud hosting with unmatched security and has continued to improve its offering over 29 years, leading the industry in response to client needs. | The industry leader in College Health EHR | Medicat has the leading market share in the college health EHR industry, serving more college and university campuses than all other college health EHR companies combined. From the largest universities to the smallest colleges, Medicat specializes in workflow efficiency and a seamless transition from other EHRs or less efficient manual, paper-based systems to reap the benefits of going digital. | Two kinds of clients are switching from other systems to Medicat: Colleges who are with small niche EHR companies that dont have the capabilities or the security infrastructure; and those who are with larger companies that dont offer Medicats specialized expertise and support in college health. Wherever you fit in, Medicat can help; lets talk! | Medicat is the #1 health management system supporting college health. We support healthcare providers at over 500 universities, from the largest universities to the smallest colleges, covering more than 5 million students. Our software and services are co-created with healthcare professionals to address the unique workflow challenges of medical and mental health practitioners | 303 Perimeter Center North, Suite 450Atlanta, GA 30346 | Toll-Free: (866) 633-4053Phone: (404) 252-2295Fax: (404) 252-2298 | 2022 MEDICAT. | Notifications",
"target": "Medicat is the industry leader in College Health Software and serves more college and university campuses than all other college health software companies combined. From the largest universities to the smallest colleges, Medicat specializes in workflow efficiency and has been improving outcomes for campus health centers since 1993. Over 500 clients, covering 5+ million students across 48 states and three countries, use Medicats total EHR solution to deliver higher quality care more efficiently with the industrys most secure software platform offerings. Medicat offers private cloud hosting with unmatched security and has continued to improve its offering over 29 years, leading the industry in response to client needs. The industry leader in College Health EHR Medicat has the leading market share in the college health EHR industry, serving more college and university campuses than all other college health EHR companies combined."
}
]
```
### Dataset Fields
The dataset has the following fields (also called "features"):
```json
{
"text": "Value(dtype='string', id=None)",
"target": "Value(dtype='string', id=None)"
}
```
### Dataset Splits
This dataset is split into a train and validation split. The split sizes are as follow:
| Split name | Num samples |
| ------------ | ------------------- |
| train | 63 |
| valid | 16 |
| Capstone/autotrain-data-healthcare_summarization_uta | [
"language:en",
"region:us"
] | 2022-11-22T19:40:29+00:00 | {"language": ["en"], "task_categories": ["conditional-text-generation"]} | 2022-11-22T19:40:55+00:00 | [] | [
"en"
] | TAGS
#language-English #region-us
| AutoTrain Dataset for project: healthcare\_summarization\_uta
=============================================================
Dataset Description
-------------------
This dataset has been automatically processed by AutoTrain for project healthcare\_summarization\_uta.
### Languages
The BCP-47 code for the dataset's language is en.
Dataset Structure
-----------------
### Data Instances
A sample from this dataset looks as follows:
### Dataset Fields
The dataset has the following fields (also called "features"):
### Dataset Splits
This dataset is split into a train and validation split. The split sizes are as follow:
| [
"### Languages\n\n\nThe BCP-47 code for the dataset's language is en.\n\n\nDataset Structure\n-----------------",
"### Data Instances\n\n\nA sample from this dataset looks as follows:",
"### Dataset Fields\n\n\nThe dataset has the following fields (also called \"features\"):",
"### Dataset Splits\n\n\nThis dataset is split into a train and validation split. The split sizes are as follow:"
] | [
"TAGS\n#language-English #region-us \n",
"### Languages\n\n\nThe BCP-47 code for the dataset's language is en.\n\n\nDataset Structure\n-----------------",
"### Data Instances\n\n\nA sample from this dataset looks as follows:",
"### Dataset Fields\n\n\nThe dataset has the following fields (also called \"features\"):",
"### Dataset Splits\n\n\nThis dataset is split into a train and validation split. The split sizes are as follow:"
] | [
10,
26,
17,
23,
27
] | [
"passage: TAGS\n#language-English #region-us \n### Languages\n\n\nThe BCP-47 code for the dataset's language is en.\n\n\nDataset Structure\n-----------------### Data Instances\n\n\nA sample from this dataset looks as follows:### Dataset Fields\n\n\nThe dataset has the following fields (also called \"features\"):### Dataset Splits\n\n\nThis dataset is split into a train and validation split. The split sizes are as follow:"
] |
69f921266f77bd6bb3b27a8b65ee112cbc8e944f | # AutoTrain Dataset for project: my-train
## Dataset Description
This dataset has been automatically processed by AutoTrain for project my-train.
### Languages
The BCP-47 code for the dataset's language is en2es.
## Dataset Structure
### Data Instances
A sample from this dataset looks as follows:
```json
[
{
"feat_eng": "eng",
"feat_spa": "spa",
"source": "Can you remember the first time you heard the Beatles?",
"target": "\u00bfRecuerdas la primera vez que escuchaste a los Beatles?",
"feat_Unnamed: 4": null,
"feat_Unnamed: 5": null,
"feat_Unnamed: 6": null,
"feat_Unnamed: 7": null,
"feat_Unnamed: 8": null,
"feat_Unnamed: 9": null,
"feat_Unnamed: 10": null,
"feat_Unnamed: 11": null,
"feat_Unnamed: 12": null,
"feat_Unnamed: 13": null,
"feat_Unnamed: 14": null,
"feat_Unnamed: 15": null,
"feat_Unnamed: 16": null,
"feat_Unnamed: 17": null
},
{
"feat_eng": "eng",
"feat_spa": "spa",
"source": "He is always talking big.",
"target": "\u00c9l siempre est\u00e1 alardeando.",
"feat_Unnamed: 4": null,
"feat_Unnamed: 5": null,
"feat_Unnamed: 6": null,
"feat_Unnamed: 7": null,
"feat_Unnamed: 8": null,
"feat_Unnamed: 9": null,
"feat_Unnamed: 10": null,
"feat_Unnamed: 11": null,
"feat_Unnamed: 12": null,
"feat_Unnamed: 13": null,
"feat_Unnamed: 14": null,
"feat_Unnamed: 15": null,
"feat_Unnamed: 16": null,
"feat_Unnamed: 17": null
}
]
```
### Dataset Fields
The dataset has the following fields (also called "features"):
```json
{
"feat_eng": "Value(dtype='string', id=None)",
"feat_spa": "Value(dtype='string', id=None)",
"source": "Value(dtype='string', id=None)",
"target": "Value(dtype='string', id=None)",
"feat_Unnamed: 4": "Value(dtype='string', id=None)",
"feat_Unnamed: 5": "Value(dtype='string', id=None)",
"feat_Unnamed: 6": "Value(dtype='string', id=None)",
"feat_Unnamed: 7": "Value(dtype='string', id=None)",
"feat_Unnamed: 8": "Value(dtype='string', id=None)",
"feat_Unnamed: 9": "Value(dtype='string', id=None)",
"feat_Unnamed: 10": "Value(dtype='string', id=None)",
"feat_Unnamed: 11": "Value(dtype='string', id=None)",
"feat_Unnamed: 12": "Value(dtype='string', id=None)",
"feat_Unnamed: 13": "Value(dtype='string', id=None)",
"feat_Unnamed: 14": "Value(dtype='string', id=None)",
"feat_Unnamed: 15": "Value(dtype='string', id=None)",
"feat_Unnamed: 16": "Value(dtype='string', id=None)",
"feat_Unnamed: 17": "Value(dtype='string', id=None)"
}
```
### Dataset Splits
This dataset is split into a train and validation split. The split sizes are as follow:
| Split name | Num samples |
| ------------ | ------------------- |
| train | 2028 |
| valid | 507 |
| warrormac/autotrain-data-my-train | [
"task_categories:translation",
"language:en",
"language:es",
"region:us"
] | 2022-11-22T21:30:04+00:00 | {"language": ["en", "es"], "task_categories": ["translation"]} | 2022-11-22T21:54:25+00:00 | [] | [
"en",
"es"
] | TAGS
#task_categories-translation #language-English #language-Spanish #region-us
| AutoTrain Dataset for project: my-train
=======================================
Dataset Description
-------------------
This dataset has been automatically processed by AutoTrain for project my-train.
### Languages
The BCP-47 code for the dataset's language is en2es.
Dataset Structure
-----------------
### Data Instances
A sample from this dataset looks as follows:
### Dataset Fields
The dataset has the following fields (also called "features"):
### Dataset Splits
This dataset is split into a train and validation split. The split sizes are as follow:
| [
"### Languages\n\n\nThe BCP-47 code for the dataset's language is en2es.\n\n\nDataset Structure\n-----------------",
"### Data Instances\n\n\nA sample from this dataset looks as follows:",
"### Dataset Fields\n\n\nThe dataset has the following fields (also called \"features\"):",
"### Dataset Splits\n\n\nThis dataset is split into a train and validation split. The split sizes are as follow:"
] | [
"TAGS\n#task_categories-translation #language-English #language-Spanish #region-us \n",
"### Languages\n\n\nThe BCP-47 code for the dataset's language is en2es.\n\n\nDataset Structure\n-----------------",
"### Data Instances\n\n\nA sample from this dataset looks as follows:",
"### Dataset Fields\n\n\nThe dataset has the following fields (also called \"features\"):",
"### Dataset Splits\n\n\nThis dataset is split into a train and validation split. The split sizes are as follow:"
] | [
24,
28,
17,
23,
27
] | [
"passage: TAGS\n#task_categories-translation #language-English #language-Spanish #region-us \n### Languages\n\n\nThe BCP-47 code for the dataset's language is en2es.\n\n\nDataset Structure\n-----------------### Data Instances\n\n\nA sample from this dataset looks as follows:### Dataset Fields\n\n\nThe dataset has the following fields (also called \"features\"):### Dataset Splits\n\n\nThis dataset is split into a train and validation split. The split sizes are as follow:"
] |
d63437e092dc8c8a77dddfea16132d37816318c9 | # Dataset Card for "biomedical-topic-categorization-validation-cased"
[More Information needed](https://github.com/huggingface/datasets/blob/main/CONTRIBUTING.md#how-to-contribute-to-the-dataset-cards) | Javtor/biomedical-topic-categorization-2022only-cased | [
"region:us"
] | 2022-11-22T22:29:27+00:00 | {"dataset_info": {"features": [{"name": "Title/Abstract", "dtype": "string"}, {"name": "T001", "dtype": "int64"}, {"name": "T002", "dtype": "int64"}, {"name": "T004", "dtype": "int64"}, {"name": "T005", "dtype": "int64"}, {"name": "T007", "dtype": "int64"}, {"name": "T008", "dtype": "int64"}, {"name": "T010", "dtype": "int64"}, {"name": "T011", "dtype": "int64"}, {"name": "T012", "dtype": "int64"}, {"name": "T013", "dtype": "int64"}, {"name": "T014", "dtype": "int64"}, {"name": "T015", "dtype": "int64"}, {"name": "T016", "dtype": "int64"}, {"name": "T017", "dtype": "int64"}, {"name": "T018", "dtype": "int64"}, {"name": "T019", "dtype": "int64"}, {"name": "T020", "dtype": "int64"}, {"name": "T022", "dtype": "int64"}, {"name": "T023", "dtype": "int64"}, {"name": "T024", "dtype": "int64"}, {"name": "T025", "dtype": "int64"}, {"name": "T026", "dtype": "int64"}, {"name": "T028", "dtype": "int64"}, {"name": "T029", "dtype": "int64"}, {"name": "T030", "dtype": "int64"}, {"name": "T031", "dtype": "int64"}, {"name": "T032", "dtype": "int64"}, {"name": "T033", "dtype": "int64"}, {"name": "T034", "dtype": "int64"}, {"name": "T037", "dtype": "int64"}, {"name": "T038", "dtype": "int64"}, {"name": "T039", "dtype": "int64"}, {"name": "T040", "dtype": "int64"}, {"name": "T041", "dtype": "int64"}, {"name": "T042", "dtype": "int64"}, {"name": "T043", "dtype": "int64"}, {"name": "T044", "dtype": "int64"}, {"name": "T045", "dtype": "int64"}, {"name": "T046", "dtype": "int64"}, {"name": "T047", "dtype": "int64"}, {"name": "T048", "dtype": "int64"}, {"name": "T049", "dtype": "int64"}, {"name": "T050", "dtype": "int64"}, {"name": "T051", "dtype": "int64"}, {"name": "T052", "dtype": "int64"}, {"name": "T053", "dtype": "int64"}, {"name": "T054", "dtype": "int64"}, {"name": "T055", "dtype": "int64"}, {"name": "T056", "dtype": "int64"}, {"name": "T057", "dtype": "int64"}, {"name": "T058", "dtype": "int64"}, {"name": "T059", "dtype": "int64"}, {"name": "T060", "dtype": "int64"}, {"name": "T061", "dtype": "int64"}, {"name": "T062", "dtype": "int64"}, {"name": "T063", "dtype": "int64"}, {"name": "T064", "dtype": "int64"}, {"name": "T065", "dtype": "int64"}, {"name": "T066", "dtype": "int64"}, {"name": "T067", "dtype": "int64"}, {"name": "T068", "dtype": "int64"}, {"name": "T069", "dtype": "int64"}, {"name": "T070", "dtype": "int64"}, {"name": "T071", "dtype": "int64"}, {"name": "T072", "dtype": "int64"}, {"name": "T073", "dtype": "int64"}, {"name": "T074", "dtype": "int64"}, {"name": "T075", "dtype": "int64"}, {"name": "T077", "dtype": "int64"}, {"name": "T078", "dtype": "int64"}, {"name": "T079", "dtype": "int64"}, {"name": "T080", "dtype": "int64"}, {"name": "T081", "dtype": "int64"}, {"name": "T082", "dtype": "int64"}, {"name": "T083", "dtype": "int64"}, {"name": "T085", "dtype": "int64"}, {"name": "T086", "dtype": "int64"}, {"name": "T087", "dtype": "int64"}, {"name": "T089", "dtype": "int64"}, {"name": "T090", "dtype": "int64"}, {"name": "T091", "dtype": "int64"}, {"name": "T092", "dtype": "int64"}, {"name": "T093", "dtype": "int64"}, {"name": "T094", "dtype": "int64"}, {"name": "T095", "dtype": "int64"}, {"name": "T096", "dtype": "int64"}, {"name": "T097", "dtype": "int64"}, {"name": "T098", "dtype": "int64"}, {"name": "T099", "dtype": "int64"}, {"name": "T100", "dtype": "int64"}, {"name": "T101", "dtype": "int64"}, {"name": "T102", "dtype": "int64"}, {"name": "T103", "dtype": "int64"}, {"name": "T104", "dtype": "int64"}, {"name": "T109", "dtype": "int64"}, {"name": "T114", "dtype": "int64"}, {"name": "T116", "dtype": "int64"}, {"name": "T120", "dtype": "int64"}, {"name": "T121", "dtype": "int64"}, {"name": "T122", "dtype": "int64"}, {"name": "T123", "dtype": "int64"}, {"name": "T125", "dtype": "int64"}, {"name": "T126", "dtype": "int64"}, {"name": "T127", "dtype": "int64"}, {"name": "T129", "dtype": "int64"}, {"name": "T130", "dtype": "int64"}, {"name": "T131", "dtype": "int64"}, {"name": "T167", "dtype": "int64"}, {"name": "T168", "dtype": "int64"}, {"name": "T169", "dtype": "int64"}, {"name": "T170", "dtype": "int64"}, {"name": "T171", "dtype": "int64"}, {"name": "T184", "dtype": "int64"}, {"name": "T185", "dtype": "int64"}, {"name": "T190", "dtype": "int64"}, {"name": "T191", "dtype": "int64"}, {"name": "T192", "dtype": "int64"}, {"name": "T194", "dtype": "int64"}, {"name": "T195", "dtype": "int64"}, {"name": "T196", "dtype": "int64"}, {"name": "T197", "dtype": "int64"}, {"name": "T200", "dtype": "int64"}, {"name": "T201", "dtype": "int64"}, {"name": "T204", "dtype": "int64"}], "splits": [{"name": "train", "num_bytes": 399873192.8393, "num_examples": 183374}, {"name": "test", "num_bytes": 133291791.16070004, "num_examples": 61125}], "download_size": 178851848, "dataset_size": 533164984.0}} | 2022-12-10T23:56:01+00:00 | [] | [] | TAGS
#region-us
| # Dataset Card for "biomedical-topic-categorization-validation-cased"
More Information needed | [
"# Dataset Card for \"biomedical-topic-categorization-validation-cased\"\n\nMore Information needed"
] | [
"TAGS\n#region-us \n",
"# Dataset Card for \"biomedical-topic-categorization-validation-cased\"\n\nMore Information needed"
] | [
6,
26
] | [
"passage: TAGS\n#region-us \n# Dataset Card for \"biomedical-topic-categorization-validation-cased\"\n\nMore Information needed"
] |
700b52a788a361eff955178767d7ff9f502c73ee |
# Ouroboros Style Embeddings / Textual Inversion
<img alt="Showcase" src="https://huggingface.co/datasets/Nerfgun3/ouroboros_embeddings/resolve/main/ouroboros_showcase.jpg"/>
## Intro
Both embeddings are quiet similar in style, but were trained on a different dataset.
## Usage
To use my embeddings you have to download the file aswell as drop it into the "\stable-diffusion-webui\embeddings" folder
Personally, I would recommend to use my embeddings with a strength of 0.8, like ```"drawn by (filename:0.8)"```
I trained both embeddings two epochs until 8000 steps.
I hope you enjoy the embedding. If you have any questions, you can ask me anything via Discord: "Nerfgun3#7508"
### Dark ouroboros
This embedding was trained on a dataset with dark backgrounds.
To use it in a prompt: ```"drawn by dark_ouroboros"```
### White ouroboros
This embedding was trained on a dataset with white backgrounds.
To use it in a prompt: ```"drawn by white_ouroboros"```
## License
This embedding is open access and available to all, with a CreativeML OpenRAIL-M license further specifying rights and usage.
The CreativeML OpenRAIL License specifies:
1. You can't use the embedding to deliberately produce nor share illegal or harmful outputs or content
2. The authors claims no rights on the outputs you generate, you are free to use them and are accountable for their use which must not go against the provisions set in the license
3. You may re-distribute the weights and use the embedding commercially and/or as a service. If you do, please be aware you have to include the same use restrictions as the ones in the license and share a copy of the CreativeML OpenRAIL-M to all your users (please read the license entirely and carefully)
[Please read the full license here](https://huggingface.co/spaces/CompVis/stable-diffusion-license) | Nerfgun3/ouroboros_embeddings | [
"language:en",
"license:creativeml-openrail-m",
"stable-diffusion",
"text-to-image",
"image-to-image",
"region:us"
] | 2022-11-22T23:28:12+00:00 | {"language": ["en"], "license": "creativeml-openrail-m", "thumbnail": "https://huggingface.co/datasets/Nerfgun3/ouroboros_embeddings/resolve/main/ouroboros_showcase.jpg", "tags": ["stable-diffusion", "text-to-image", "image-to-image"], "inference": false} | 2022-11-22T23:37:12+00:00 | [] | [
"en"
] | TAGS
#language-English #license-creativeml-openrail-m #stable-diffusion #text-to-image #image-to-image #region-us
|
# Ouroboros Style Embeddings / Textual Inversion
<img alt="Showcase" src="URL
## Intro
Both embeddings are quiet similar in style, but were trained on a different dataset.
## Usage
To use my embeddings you have to download the file aswell as drop it into the "\stable-diffusion-webui\embeddings" folder
Personally, I would recommend to use my embeddings with a strength of 0.8, like
I trained both embeddings two epochs until 8000 steps.
I hope you enjoy the embedding. If you have any questions, you can ask me anything via Discord: "Nerfgun3#7508"
### Dark ouroboros
This embedding was trained on a dataset with dark backgrounds.
To use it in a prompt:
### White ouroboros
This embedding was trained on a dataset with white backgrounds.
To use it in a prompt:
## License
This embedding is open access and available to all, with a CreativeML OpenRAIL-M license further specifying rights and usage.
The CreativeML OpenRAIL License specifies:
1. You can't use the embedding to deliberately produce nor share illegal or harmful outputs or content
2. The authors claims no rights on the outputs you generate, you are free to use them and are accountable for their use which must not go against the provisions set in the license
3. You may re-distribute the weights and use the embedding commercially and/or as a service. If you do, please be aware you have to include the same use restrictions as the ones in the license and share a copy of the CreativeML OpenRAIL-M to all your users (please read the license entirely and carefully)
Please read the full license here | [
"# Ouroboros Style Embeddings / Textual Inversion\n\n<img alt=\"Showcase\" src=\"URL",
"## Intro\n\nBoth embeddings are quiet similar in style, but were trained on a different dataset.",
"## Usage\n\nTo use my embeddings you have to download the file aswell as drop it into the \"\\stable-diffusion-webui\\embeddings\" folder\n\nPersonally, I would recommend to use my embeddings with a strength of 0.8, like \n\nI trained both embeddings two epochs until 8000 steps.\n\nI hope you enjoy the embedding. If you have any questions, you can ask me anything via Discord: \"Nerfgun3#7508\"",
"### Dark ouroboros\n\nThis embedding was trained on a dataset with dark backgrounds.\n\nTo use it in a prompt:",
"### White ouroboros\n\nThis embedding was trained on a dataset with white backgrounds.\n\nTo use it in a prompt:",
"## License\n\nThis embedding is open access and available to all, with a CreativeML OpenRAIL-M license further specifying rights and usage.\nThe CreativeML OpenRAIL License specifies: \n\n1. You can't use the embedding to deliberately produce nor share illegal or harmful outputs or content \n2. The authors claims no rights on the outputs you generate, you are free to use them and are accountable for their use which must not go against the provisions set in the license\n3. You may re-distribute the weights and use the embedding commercially and/or as a service. If you do, please be aware you have to include the same use restrictions as the ones in the license and share a copy of the CreativeML OpenRAIL-M to all your users (please read the license entirely and carefully)\nPlease read the full license here"
] | [
"TAGS\n#language-English #license-creativeml-openrail-m #stable-diffusion #text-to-image #image-to-image #region-us \n",
"# Ouroboros Style Embeddings / Textual Inversion\n\n<img alt=\"Showcase\" src=\"URL",
"## Intro\n\nBoth embeddings are quiet similar in style, but were trained on a different dataset.",
"## Usage\n\nTo use my embeddings you have to download the file aswell as drop it into the \"\\stable-diffusion-webui\\embeddings\" folder\n\nPersonally, I would recommend to use my embeddings with a strength of 0.8, like \n\nI trained both embeddings two epochs until 8000 steps.\n\nI hope you enjoy the embedding. If you have any questions, you can ask me anything via Discord: \"Nerfgun3#7508\"",
"### Dark ouroboros\n\nThis embedding was trained on a dataset with dark backgrounds.\n\nTo use it in a prompt:",
"### White ouroboros\n\nThis embedding was trained on a dataset with white backgrounds.\n\nTo use it in a prompt:",
"## License\n\nThis embedding is open access and available to all, with a CreativeML OpenRAIL-M license further specifying rights and usage.\nThe CreativeML OpenRAIL License specifies: \n\n1. You can't use the embedding to deliberately produce nor share illegal or harmful outputs or content \n2. The authors claims no rights on the outputs you generate, you are free to use them and are accountable for their use which must not go against the provisions set in the license\n3. You may re-distribute the weights and use the embedding commercially and/or as a service. If you do, please be aware you have to include the same use restrictions as the ones in the license and share a copy of the CreativeML OpenRAIL-M to all your users (please read the license entirely and carefully)\nPlease read the full license here"
] | [
41,
26,
24,
111,
29,
29,
185
] | [
"passage: TAGS\n#language-English #license-creativeml-openrail-m #stable-diffusion #text-to-image #image-to-image #region-us \n# Ouroboros Style Embeddings / Textual Inversion\n\n<img alt=\"Showcase\" src=\"URL## Intro\n\nBoth embeddings are quiet similar in style, but were trained on a different dataset.## Usage\n\nTo use my embeddings you have to download the file aswell as drop it into the \"\\stable-diffusion-webui\\embeddings\" folder\n\nPersonally, I would recommend to use my embeddings with a strength of 0.8, like \n\nI trained both embeddings two epochs until 8000 steps.\n\nI hope you enjoy the embedding. If you have any questions, you can ask me anything via Discord: \"Nerfgun3#7508\"### Dark ouroboros\n\nThis embedding was trained on a dataset with dark backgrounds.\n\nTo use it in a prompt:### White ouroboros\n\nThis embedding was trained on a dataset with white backgrounds.\n\nTo use it in a prompt:## License\n\nThis embedding is open access and available to all, with a CreativeML OpenRAIL-M license further specifying rights and usage.\nThe CreativeML OpenRAIL License specifies: \n\n1. You can't use the embedding to deliberately produce nor share illegal or harmful outputs or content \n2. The authors claims no rights on the outputs you generate, you are free to use them and are accountable for their use which must not go against the provisions set in the license\n3. You may re-distribute the weights and use the embedding commercially and/or as a service. If you do, please be aware you have to include the same use restrictions as the ones in the license and share a copy of the CreativeML OpenRAIL-M to all your users (please read the license entirely and carefully)\nPlease read the full license here"
] |
ff2c87c90c7e495dd64c481d25aaf65a866d1907 | # Dataset Card for "description2Image"
[More Information needed](https://github.com/huggingface/datasets/blob/main/CONTRIBUTING.md#how-to-contribute-to-the-dataset-cards) | Una713/description2Image | [
"region:us"
] | 2022-11-23T00:54:30+00:00 | {"dataset_info": {"features": [{"name": "image", "dtype": "image"}, {"name": "text", "dtype": "string"}], "splits": [{"name": "train", "num_bytes": 9546659.0, "num_examples": 335}], "download_size": 5446356, "dataset_size": 9546659.0}} | 2022-11-23T23:52:33+00:00 | [] | [] | TAGS
#region-us
| # Dataset Card for "description2Image"
More Information needed | [
"# Dataset Card for \"description2Image\"\n\nMore Information needed"
] | [
"TAGS\n#region-us \n",
"# Dataset Card for \"description2Image\"\n\nMore Information needed"
] | [
6,
14
] | [
"passage: TAGS\n#region-us \n# Dataset Card for \"description2Image\"\n\nMore Information needed"
] |
f2b356c3b8bc53121d6ad47cba1004ea4c7866b1 | # Dataset Card for "ARGEN_title_generation"
[More Information needed](https://github.com/huggingface/datasets/blob/main/CONTRIBUTING.md#how-to-contribute-to-the-dataset-cards) | arbml/ARGEN_title_generation | [
"region:us"
] | 2022-11-23T02:31:19+00:00 | {"dataset_info": {"features": [{"name": "document", "dtype": "string"}, {"name": "title", "dtype": "string"}, {"name": "document_count", "dtype": "string"}, {"name": "title_count", "dtype": "string"}], "splits": [{"name": "train", "num_bytes": 2973709, "num_examples": 1000}, {"name": "validation", "num_bytes": 578378, "num_examples": 200}], "download_size": 1550878, "dataset_size": 3552087}} | 2022-11-23T02:31:30+00:00 | [] | [] | TAGS
#region-us
| # Dataset Card for "ARGEN_title_generation"
More Information needed | [
"# Dataset Card for \"ARGEN_title_generation\"\n\nMore Information needed"
] | [
"TAGS\n#region-us \n",
"# Dataset Card for \"ARGEN_title_generation\"\n\nMore Information needed"
] | [
6,
17
] | [
"passage: TAGS\n#region-us \n# Dataset Card for \"ARGEN_title_generation\"\n\nMore Information needed"
] |
38146e73a34f1b2dbc4f3c6d2c9f5ec40fc4227b | # Dataset Card for "mnli_corpus_trainval"
[More Information needed](https://github.com/huggingface/datasets/blob/main/CONTRIBUTING.md#how-to-contribute-to-the-dataset-cards) | carlosejimenez/mnli_corpus_trainval | [
"region:us"
] | 2022-11-23T02:37:09+00:00 | {"dataset_info": {"features": [{"name": "idx", "dtype": "int64"}, {"name": "text", "dtype": "string"}], "splits": [{"name": "test", "num_bytes": 1780082, "num_examples": 9796}, {"name": "train", "num_bytes": 73635810, "num_examples": 402517}, {"name": "validation", "num_bytes": 1765078, "num_examples": 9815}], "download_size": 28009270, "dataset_size": 77180970}} | 2022-11-23T02:37:49+00:00 | [] | [] | TAGS
#region-us
| # Dataset Card for "mnli_corpus_trainval"
More Information needed | [
"# Dataset Card for \"mnli_corpus_trainval\"\n\nMore Information needed"
] | [
"TAGS\n#region-us \n",
"# Dataset Card for \"mnli_corpus_trainval\"\n\nMore Information needed"
] | [
6,
19
] | [
"passage: TAGS\n#region-us \n# Dataset Card for \"mnli_corpus_trainval\"\n\nMore Information needed"
] |
1027ba1d277e0c685c576633a4d991f6dbad04a9 | # Dataset Card for "the-ai-epiphany"
[More Information needed](https://github.com/huggingface/datasets/blob/main/CONTRIBUTING.md#how-to-contribute-to-the-dataset-cards) | Whispering-GPT/whisper-transcripts-the-ai-epiphany | [
"task_categories:automatic-speech-recognition",
"whisper",
"whispering",
"medium",
"region:us"
] | 2022-11-23T03:11:06+00:00 | {"task_categories": ["automatic-speech-recognition"], "dataset_info": {"features": [{"name": "CHANNEL_NAME", "dtype": "string"}, {"name": "URL", "dtype": "string"}, {"name": "TITLE", "dtype": "string"}, {"name": "DESCRIPTION", "dtype": "string"}, {"name": "TRANSCRIPTION", "dtype": "string"}, {"name": "SEGMENTS", "dtype": "string"}], "splits": [{"name": "train", "num_bytes": 9999269, "num_examples": 108}], "download_size": 4410711, "dataset_size": 9999269}, "tags": ["whisper", "whispering", "medium"]} | 2022-11-23T03:11:10+00:00 | [] | [] | TAGS
#task_categories-automatic-speech-recognition #whisper #whispering #medium #region-us
| # Dataset Card for "the-ai-epiphany"
More Information needed | [
"# Dataset Card for \"the-ai-epiphany\"\n\nMore Information needed"
] | [
"TAGS\n#task_categories-automatic-speech-recognition #whisper #whispering #medium #region-us \n",
"# Dataset Card for \"the-ai-epiphany\"\n\nMore Information needed"
] | [
34,
17
] | [
"passage: TAGS\n#task_categories-automatic-speech-recognition #whisper #whispering #medium #region-us \n# Dataset Card for \"the-ai-epiphany\"\n\nMore Information needed"
] |
a7321254d20061825ed49d8faf00911add3b794f | # Dataset Card for "SemanticScholar_all-distilroberta-v1_Embeddings"
[More Information needed](https://github.com/huggingface/datasets/blob/main/CONTRIBUTING.md#how-to-contribute-to-the-dataset-cards) | KaiserML/SemanticScholar_all-distilroberta-v1_Embeddings | [
"region:us"
] | 2022-11-23T04:19:00+00:00 | {"dataset_info": {"features": [{"name": "corpusid", "dtype": "int64"}, {"name": "openaccessinfo", "struct": [{"name": "externalids", "struct": [{"name": "ACL", "dtype": "string"}, {"name": "ArXiv", "dtype": "string"}, {"name": "DOI", "dtype": "string"}, {"name": "MAG", "dtype": "string"}, {"name": "PubMedCentral", "dtype": "string"}]}, {"name": "license", "dtype": "string"}, {"name": "status", "dtype": "string"}, {"name": "url", "dtype": "string"}]}, {"name": "abstract", "dtype": "string"}, {"name": "updated", "dtype": "string"}, {"name": "embedding", "sequence": "float32"}], "splits": [{"name": "train", "num_bytes": 709059031, "num_examples": 440000}], "download_size": 552056679, "dataset_size": 709059031}} | 2022-11-23T04:58:57+00:00 | [] | [] | TAGS
#region-us
| # Dataset Card for "SemanticScholar_all-distilroberta-v1_Embeddings"
More Information needed | [
"# Dataset Card for \"SemanticScholar_all-distilroberta-v1_Embeddings\"\n\nMore Information needed"
] | [
"TAGS\n#region-us \n",
"# Dataset Card for \"SemanticScholar_all-distilroberta-v1_Embeddings\"\n\nMore Information needed"
] | [
6,
31
] | [
"passage: TAGS\n#region-us \n# Dataset Card for \"SemanticScholar_all-distilroberta-v1_Embeddings\"\n\nMore Information needed"
] |
85d4d861b6a6fde97b27379a96851dab60adf95e | # Dataset Card for "qqp_corpus_trainval"
[More Information needed](https://github.com/huggingface/datasets/blob/main/CONTRIBUTING.md#how-to-contribute-to-the-dataset-cards) | carlosejimenez/qqp_corpus_trainval | [
"region:us"
] | 2022-11-23T05:59:55+00:00 | {"dataset_info": {"features": [{"name": "idx", "dtype": "int64"}, {"name": "text", "dtype": "string"}], "splits": [{"name": "test", "num_bytes": 52434356, "num_examples": 390965}, {"name": "train", "num_bytes": 53724642, "num_examples": 404276}, {"name": "validation", "num_bytes": 5370744, "num_examples": 40430}], "download_size": 50205619, "dataset_size": 111529742}} | 2022-11-23T06:00:08+00:00 | [] | [] | TAGS
#region-us
| # Dataset Card for "qqp_corpus_trainval"
More Information needed | [
"# Dataset Card for \"qqp_corpus_trainval\"\n\nMore Information needed"
] | [
"TAGS\n#region-us \n",
"# Dataset Card for \"qqp_corpus_trainval\"\n\nMore Information needed"
] | [
6,
19
] | [
"passage: TAGS\n#region-us \n# Dataset Card for \"qqp_corpus_trainval\"\n\nMore Information needed"
] |
08904951382c1de8a7591e67687b79469067659d | <a href=”https://www.travomonk.com/blog/can-you-bring-a-razor-on-a-plane/”>Can you bring a shaving razor on plane</a>:- Yes, unfortunately, you are allowed to bring disposable razors in your carry-on luggage. To avoid cutting yourself when reaching into your luggage, you should still make sure these are covered. This is what? Razors that are disposable are allowed in checked baggage. | stellamilos/XYZ | [
"region:us"
] | 2022-11-23T07:51:56+00:00 | {} | 2022-11-23T07:53:19+00:00 | [] | [] | TAGS
#region-us
| <a href=”URL”>Can you bring a shaving razor on plane</a>:- Yes, unfortunately, you are allowed to bring disposable razors in your carry-on luggage. To avoid cutting yourself when reaching into your luggage, you should still make sure these are covered. This is what? Razors that are disposable are allowed in checked baggage. | [] | [
"TAGS\n#region-us \n"
] | [
6
] | [
"passage: TAGS\n#region-us \n"
] |
c04a055b3e4232c93fd99dd6d2419f013adf7edc | # AutoTrain Dataset for project: text_summ
## Dataset Description
This dataset has been automatically processed by AutoTrain for project text_summ.
### Languages
The BCP-47 code for the dataset's language is unk.
## Dataset Structure
### Data Instances
A sample from this dataset looks as follows:
```json
[
{
"feat_guid": "klue-nli-v1_train_06870",
"feat_source": "policy",
"text": "\ub610\ud55c \ub3c5\ub9bd\ucd9c\ud310\ubb3c \uc81c\uc791 \uc6cc\ud06c\uc20d\uc774\ub791 \uae00\uc4f0\uae30 \uc18c\uc124 \uc4f0\uae30 \uc6cc\ud06c\uc20d\ub3c4 \uc9c4\ud589\ud558\uba70 \uc6d0\ub370\uc774 \uc218\uc5c5\uc744 \ud1b5\ud574 \ub2e4\uc591\ud55c \ud65c\ub3d9\uc744 \ud558\ub294 \uc54c\ucc2c \ubcf5\ud569\uc801\uc778 \uacf5\uac04\uc774\ub2e4.",
"target": "\ub3c5\ub9bd\ucd9c\ud310\ubb3c \uc81c\uc791 \uc6cc\ud06c\uc20d\uc740 \uc5b8\ub860\uc0ac\uc758 \ud6c4\uc6d0\ud558\uc5d0 \uc9c4\ud589\ub41c\ub2e4.",
"feat_label": 2
},
{
"feat_guid": "klue-nli-v1_train_02196",
"feat_source": "wikitree",
"text": "\uacf5\uc720\ub41c \uc0ac\uc9c4\uc5d0\ub294 \uc790\uc6b1\ud558\uac8c \uc548\uac1c\uc640 \ubbf8\uc138\uba3c\uc9c0\uac00 \ub0b4\ub824\uc549\uc740 \uc0ac\uc9c4\uc774 \uacf5\uc720\ub410\ub2e4.",
"target": "\uc790\uc6b1\ud55c \uc548\uac1c\uc640 \ubbf8\uc138\uba3c\uc9c0\ub9cc \ub0b4\ub824\uc549\uc558\ub2e4.",
"feat_label": 2
}
]
```
### Dataset Fields
The dataset has the following fields (also called "features"):
```json
{
"feat_guid": "Value(dtype='string', id=None)",
"feat_source": "Value(dtype='string', id=None)",
"text": "Value(dtype='string', id=None)",
"target": "Value(dtype='string', id=None)",
"feat_label": "ClassLabel(num_classes=3, names=['contradiction', 'entailment', 'neutral'], id=None)"
}
```
### Dataset Splits
This dataset is split into a train and validation split. The split sizes are as follow:
| Split name | Num samples |
| ------------ | ------------------- |
| train | 22398 |
| valid | 5600 |
| hreensajin/autotrain-data-text_summ | [
"region:us"
] | 2022-11-23T09:16:48+00:00 | {"task_categories": ["conditional-text-generation"]} | 2022-11-23T09:18:18+00:00 | [] | [] | TAGS
#region-us
| AutoTrain Dataset for project: text\_summ
=========================================
Dataset Description
-------------------
This dataset has been automatically processed by AutoTrain for project text\_summ.
### Languages
The BCP-47 code for the dataset's language is unk.
Dataset Structure
-----------------
### Data Instances
A sample from this dataset looks as follows:
### Dataset Fields
The dataset has the following fields (also called "features"):
### Dataset Splits
This dataset is split into a train and validation split. The split sizes are as follow:
| [
"### Languages\n\n\nThe BCP-47 code for the dataset's language is unk.\n\n\nDataset Structure\n-----------------",
"### Data Instances\n\n\nA sample from this dataset looks as follows:",
"### Dataset Fields\n\n\nThe dataset has the following fields (also called \"features\"):",
"### Dataset Splits\n\n\nThis dataset is split into a train and validation split. The split sizes are as follow:"
] | [
"TAGS\n#region-us \n",
"### Languages\n\n\nThe BCP-47 code for the dataset's language is unk.\n\n\nDataset Structure\n-----------------",
"### Data Instances\n\n\nA sample from this dataset looks as follows:",
"### Dataset Fields\n\n\nThe dataset has the following fields (also called \"features\"):",
"### Dataset Splits\n\n\nThis dataset is split into a train and validation split. The split sizes are as follow:"
] | [
6,
27,
17,
23,
27
] | [
"passage: TAGS\n#region-us \n### Languages\n\n\nThe BCP-47 code for the dataset's language is unk.\n\n\nDataset Structure\n-----------------### Data Instances\n\n\nA sample from this dataset looks as follows:### Dataset Fields\n\n\nThe dataset has the following fields (also called \"features\"):### Dataset Splits\n\n\nThis dataset is split into a train and validation split. The split sizes are as follow:"
] |
7ae013cbc427dceac0cff8db0afbb389ae3a0ebb | # Dataset Card for "test_small_"
[More Information needed](https://github.com/huggingface/datasets/blob/main/CONTRIBUTING.md#how-to-contribute-to-the-dataset-cards) | DTU54DL/test_small_ | [
"region:us"
] | 2022-11-23T09:28:42+00:00 | {"dataset_info": {"features": [{"name": "file", "dtype": "string"}, {"name": "audio", "dtype": {"audio": {"sampling_rate": 16000}}}, {"name": "text", "dtype": "string"}, {"name": "speaker_id", "dtype": "int64"}, {"name": "chapter_id", "dtype": "int64"}, {"name": "id", "dtype": "string"}], "splits": [{"name": "train.360", "num_bytes": 859588.0, "num_examples": 2}], "download_size": 862898, "dataset_size": 859588.0}} | 2022-11-23T11:10:06+00:00 | [] | [] | TAGS
#region-us
| # Dataset Card for "test_small_"
More Information needed | [
"# Dataset Card for \"test_small_\"\n\nMore Information needed"
] | [
"TAGS\n#region-us \n",
"# Dataset Card for \"test_small_\"\n\nMore Information needed"
] | [
6,
15
] | [
"passage: TAGS\n#region-us \n# Dataset Card for \"test_small_\"\n\nMore Information needed"
] |
8feec972043228251eff0458e6052deef93286ad |
# Dataset Card for "tweetyface"
## Table of Contents
- [Table of Contents](#table-of-contents)
- [Dataset Description](#dataset-description)
- [Dataset Summary](#dataset-summary)
- [Supported Tasks and Leaderboards](#supported-tasks-and-leaderboards)
- [Languages](#languages)
- [Dataset Structure](#dataset-structure)
- [Data Instances](#data-instances)
- [Data Fields](#data-fields)
- [Data Splits](#data-splits)
- [Dataset Creation](#dataset-creation)
- [Curation Rationale](#curation-rationale)
- [Source Data](#source-data)
- [Annotations](#annotations)
- [Personal and Sensitive Information](#personal-and-sensitive-information)
- [Considerations for Using the Data](#considerations-for-using-the-data)
- [Social Impact of Dataset](#social-impact-of-dataset)
- [Discussion of Biases](#discussion-of-biases)
- [Other Known Limitations](#other-known-limitations)
- [Additional Information](#additional-information)
- [Dataset Curators](#dataset-curators)
- [Licensing Information](#licensing-information)
- [Citation Information](#citation-information)
- [Contributions](#contributions)
## Dataset Description
- **Homepage:**
- **Repository:** [GitHub](https://github.com/ml-projects-kiel/OpenCampus-ApplicationofTransformers)
### Dataset Summary
Dataset containing Tweets from prominent Twitter Users.
The dataset has been created utilizing a crawler for the Twitter API.
### Supported Tasks and Leaderboards
[More Information Needed]
### Languages
English, German
## Dataset Structure
### Data Instances
#### english
- **Size of downloaded dataset files:** 4.77 MB
- **Size of the generated dataset:** 5.92 MB
- **Total amount of disk used:** 4.77 MB
#### german
- **Size of downloaded dataset files:** 2.58 MB
- **Size of the generated dataset:** 3.10 MB
- **Total amount of disk used:** 2.59 MB
An example of 'validation' looks as follows.
```
{
"text": "@SpaceX @Space_Station About twice as much useful mass to orbit as rest of Earth combined",
"label": elonmusk,
"idx": 1001283
}
```
### Data Fields
The data fields are the same among all splits and languages.
- `text`: a `string` feature.
- `label`: a classification label
- `idx`: an `int64` feature.
### Data Splits
| name | train | validation |
| ------- | ----: | ---------: |
| english | 27857 | 6965 |
| german | 10254 | 2564 |
## Dataset Creation
### Curation Rationale
[More Information Needed]
### Source Data
#### Initial Data Collection and Normalization
[More Information Needed]
#### Who are the source language producers?
[More Information Needed]
### Annotations
#### Annotation process
[More Information Needed]
#### Who are the annotators?
[More Information Needed]
### Personal and Sensitive Information
[More Information Needed]
## Considerations for Using the Data
### Social Impact of Dataset
[More Information Needed]
### Discussion of Biases
[More Information Needed]
### Other Known Limitations
[More Information Needed]
## Additional Information
### Dataset Curators
[More Information Needed]
### Licensing Information
[More Information Needed]
### Citation Information
[More Information Needed]
| ML-Projects-Kiel/tweetyface | [
"task_categories:text-generation",
"annotations_creators:machine-generated",
"language_creators:crowdsourced",
"multilinguality:multilingual",
"size_categories:10K<n<100K",
"language:en",
"language:de",
"license:apache-2.0",
"region:us"
] | 2022-11-23T12:04:44+00:00 | {"annotations_creators": ["machine-generated"], "language_creators": ["crowdsourced"], "language": ["en", "de"], "license": ["apache-2.0"], "multilinguality": ["multilingual"], "size_categories": ["10K<n<100K"], "source_datasets": [], "task_categories": ["text-generation"], "task_ids": [], "pretty_name": "tweetyface_en", "tags": []} | 2022-11-27T20:41:29+00:00 | [] | [
"en",
"de"
] | TAGS
#task_categories-text-generation #annotations_creators-machine-generated #language_creators-crowdsourced #multilinguality-multilingual #size_categories-10K<n<100K #language-English #language-German #license-apache-2.0 #region-us
| Dataset Card for "tweetyface"
=============================
Table of Contents
-----------------
* Table of Contents
* Dataset Description
+ Dataset Summary
+ Supported Tasks and Leaderboards
+ Languages
* Dataset Structure
+ Data Instances
+ Data Fields
+ Data Splits
* Dataset Creation
+ Curation Rationale
+ Source Data
+ Annotations
+ Personal and Sensitive Information
* Considerations for Using the Data
+ Social Impact of Dataset
+ Discussion of Biases
+ Other Known Limitations
* Additional Information
+ Dataset Curators
+ Licensing Information
+ Citation Information
+ Contributions
Dataset Description
-------------------
* Homepage:
* Repository: GitHub
### Dataset Summary
Dataset containing Tweets from prominent Twitter Users.
The dataset has been created utilizing a crawler for the Twitter API.
### Supported Tasks and Leaderboards
### Languages
English, German
Dataset Structure
-----------------
### Data Instances
#### english
* Size of downloaded dataset files: 4.77 MB
* Size of the generated dataset: 5.92 MB
* Total amount of disk used: 4.77 MB
#### german
* Size of downloaded dataset files: 2.58 MB
* Size of the generated dataset: 3.10 MB
* Total amount of disk used: 2.59 MB
An example of 'validation' looks as follows.
### Data Fields
The data fields are the same among all splits and languages.
* 'text': a 'string' feature.
* 'label': a classification label
* 'idx': an 'int64' feature.
### Data Splits
Dataset Creation
----------------
### Curation Rationale
### Source Data
#### Initial Data Collection and Normalization
#### Who are the source language producers?
### Annotations
#### Annotation process
#### Who are the annotators?
### Personal and Sensitive Information
Considerations for Using the Data
---------------------------------
### Social Impact of Dataset
### Discussion of Biases
### Other Known Limitations
Additional Information
----------------------
### Dataset Curators
### Licensing Information
| [
"### Dataset Summary\n\n\nDataset containing Tweets from prominent Twitter Users.\nThe dataset has been created utilizing a crawler for the Twitter API.",
"### Supported Tasks and Leaderboards",
"### Languages\n\n\nEnglish, German\n\n\nDataset Structure\n-----------------",
"### Data Instances",
"#### english\n\n\n* Size of downloaded dataset files: 4.77 MB\n* Size of the generated dataset: 5.92 MB\n* Total amount of disk used: 4.77 MB",
"#### german\n\n\n* Size of downloaded dataset files: 2.58 MB\n* Size of the generated dataset: 3.10 MB\n* Total amount of disk used: 2.59 MB\n\n\nAn example of 'validation' looks as follows.",
"### Data Fields\n\n\nThe data fields are the same among all splits and languages.\n\n\n* 'text': a 'string' feature.\n* 'label': a classification label\n* 'idx': an 'int64' feature.",
"### Data Splits\n\n\n\nDataset Creation\n----------------",
"### Curation Rationale",
"### Source Data",
"#### Initial Data Collection and Normalization",
"#### Who are the source language producers?",
"### Annotations",
"#### Annotation process",
"#### Who are the annotators?",
"### Personal and Sensitive Information\n\n\nConsiderations for Using the Data\n---------------------------------",
"### Social Impact of Dataset",
"### Discussion of Biases",
"### Other Known Limitations\n\n\nAdditional Information\n----------------------",
"### Dataset Curators",
"### Licensing Information"
] | [
"TAGS\n#task_categories-text-generation #annotations_creators-machine-generated #language_creators-crowdsourced #multilinguality-multilingual #size_categories-10K<n<100K #language-English #language-German #license-apache-2.0 #region-us \n",
"### Dataset Summary\n\n\nDataset containing Tweets from prominent Twitter Users.\nThe dataset has been created utilizing a crawler for the Twitter API.",
"### Supported Tasks and Leaderboards",
"### Languages\n\n\nEnglish, German\n\n\nDataset Structure\n-----------------",
"### Data Instances",
"#### english\n\n\n* Size of downloaded dataset files: 4.77 MB\n* Size of the generated dataset: 5.92 MB\n* Total amount of disk used: 4.77 MB",
"#### german\n\n\n* Size of downloaded dataset files: 2.58 MB\n* Size of the generated dataset: 3.10 MB\n* Total amount of disk used: 2.59 MB\n\n\nAn example of 'validation' looks as follows.",
"### Data Fields\n\n\nThe data fields are the same among all splits and languages.\n\n\n* 'text': a 'string' feature.\n* 'label': a classification label\n* 'idx': an 'int64' feature.",
"### Data Splits\n\n\n\nDataset Creation\n----------------",
"### Curation Rationale",
"### Source Data",
"#### Initial Data Collection and Normalization",
"#### Who are the source language producers?",
"### Annotations",
"#### Annotation process",
"#### Who are the annotators?",
"### Personal and Sensitive Information\n\n\nConsiderations for Using the Data\n---------------------------------",
"### Social Impact of Dataset",
"### Discussion of Biases",
"### Other Known Limitations\n\n\nAdditional Information\n----------------------",
"### Dataset Curators",
"### Licensing Information"
] | [
77,
34,
10,
14,
6,
37,
50,
53,
11,
7,
4,
10,
10,
5,
5,
9,
18,
7,
8,
14,
6,
6
] | [
"passage: TAGS\n#task_categories-text-generation #annotations_creators-machine-generated #language_creators-crowdsourced #multilinguality-multilingual #size_categories-10K<n<100K #language-English #language-German #license-apache-2.0 #region-us \n### Dataset Summary\n\n\nDataset containing Tweets from prominent Twitter Users.\nThe dataset has been created utilizing a crawler for the Twitter API.### Supported Tasks and Leaderboards### Languages\n\n\nEnglish, German\n\n\nDataset Structure\n-----------------### Data Instances#### english\n\n\n* Size of downloaded dataset files: 4.77 MB\n* Size of the generated dataset: 5.92 MB\n* Total amount of disk used: 4.77 MB#### german\n\n\n* Size of downloaded dataset files: 2.58 MB\n* Size of the generated dataset: 3.10 MB\n* Total amount of disk used: 2.59 MB\n\n\nAn example of 'validation' looks as follows.### Data Fields\n\n\nThe data fields are the same among all splits and languages.\n\n\n* 'text': a 'string' feature.\n* 'label': a classification label\n* 'idx': an 'int64' feature.### Data Splits\n\n\n\nDataset Creation\n----------------### Curation Rationale### Source Data#### Initial Data Collection and Normalization#### Who are the source language producers?### Annotations#### Annotation process#### Who are the annotators?### Personal and Sensitive Information\n\n\nConsiderations for Using the Data\n---------------------------------### Social Impact of Dataset### Discussion of Biases### Other Known Limitations\n\n\nAdditional Information\n----------------------### Dataset Curators### Licensing Information"
] |
53591de8917f764be40c7ea96541533c6a05fe58 | # Dataset Card for "commonvoice_10_unit"
[More Information needed](https://github.com/huggingface/datasets/blob/main/CONTRIBUTING.md#how-to-contribute-to-the-dataset-cards) | voidful/commonvoice_10_unit | [
"region:us"
] | 2022-11-23T12:36:54+00:00 | {"dataset_info": {"features": [{"name": "audio_name", "dtype": "string"}, {"name": "text", "dtype": "string"}, {"name": "unit", "sequence": "int64"}, {"name": "merged_unit", "sequence": "int64"}], "splits": [{"name": "ab.test", "num_bytes": 30209396, "num_examples": 9121}, {"name": "ab.train", "num_bytes": 72648911, "num_examples": 21026}, {"name": "ab.validation", "num_bytes": 30131267, "num_examples": 9151}, {"name": "ar.test", "num_bytes": 29012726, "num_examples": 10434}, {"name": "ar.train", "num_bytes": 75638635, "num_examples": 28077}, {"name": "ar.validation", "num_bytes": 29372704, "num_examples": 10353}, {"name": "as.test", "num_bytes": 1133404, "num_examples": 306}, {"name": "as.train", "num_bytes": 2208312, "num_examples": 603}, {"name": "as.validation", "num_bytes": 1534601, "num_examples": 447}, {"name": "az.test", "num_bytes": 87257, "num_examples": 21}, {"name": "az.train", "num_bytes": 109199, "num_examples": 38}, {"name": "az.validation", "num_bytes": 83232, "num_examples": 19}, {"name": "ba.test", "num_bytes": 45536865, "num_examples": 14525}, {"name": "ba.train", "num_bytes": 339374593, "num_examples": 118982}, {"name": "ba.validation", "num_bytes": 44893843, "num_examples": 14558}, {"name": "bas.test", "num_bytes": 1279508, "num_examples": 443}, {"name": "bas.train", "num_bytes": 1935038, "num_examples": 762}, {"name": "bas.validation", "num_bytes": 1092622, "num_examples": 456}, {"name": "be.test", "num_bytes": 62070475, "num_examples": 15878}, {"name": "be.train", "num_bytes": 1115771987, "num_examples": 347011}, {"name": "be.validation", "num_bytes": 58976849, "num_examples": 15874}, {"name": "bg.test", "num_bytes": 7042115, "num_examples": 1899}, {"name": "bg.train", "num_bytes": 11263062, "num_examples": 3160}, {"name": "bg.validation", "num_bytes": 3285294, "num_examples": 914}, {"name": "bn.test", "num_bytes": 35583281, "num_examples": 8225}, {"name": "bn.train", "num_bytes": 65887111, "num_examples": 16270}, {"name": "bn.validation", "num_bytes": 34982471, "num_examples": 8225}, {"name": "br.test", "num_bytes": 4236895, "num_examples": 2156}, {"name": "br.train", "num_bytes": 4460359, "num_examples": 2558}, {"name": "br.validation", "num_bytes": 4204891, "num_examples": 2157}, {"name": "ca.test", "num_bytes": 63720907, "num_examples": 16276}, {"name": "ca.train", "num_bytes": 2737728428, "num_examples": 744271}, {"name": "ca.validation", "num_bytes": 63187343, "num_examples": 16276}, {"name": "ckb.test", "num_bytes": 12698796, "num_examples": 4525}, {"name": "ckb.train", "num_bytes": 16204168, "num_examples": 6224}, {"name": "ckb.validation", "num_bytes": 12173721, "num_examples": 4523}, {"name": "cnh.test", "num_bytes": 1928361, "num_examples": 762}, {"name": "cnh.train", "num_bytes": 1565110, "num_examples": 816}, {"name": "cnh.validation", "num_bytes": 1757182, "num_examples": 760}, {"name": "cs.test", "num_bytes": 22404392, "num_examples": 7584}, {"name": "cs.train", "num_bytes": 46227653, "num_examples": 14412}, {"name": "cs.validation", "num_bytes": 21937936, "num_examples": 7256}, {"name": "cv.test", "num_bytes": 4499085, "num_examples": 1266}, {"name": "cv.train", "num_bytes": 5066770, "num_examples": 1565}, {"name": "cv.validation", "num_bytes": 3744163, "num_examples": 1139}, {"name": "cy.test", "num_bytes": 18059256, "num_examples": 5208}, {"name": "cy.train", "num_bytes": 24928101, "num_examples": 7593}, {"name": "cy.validation", "num_bytes": 17998576, "num_examples": 5199}, {"name": "da.test", "num_bytes": 5975319, "num_examples": 1911}, {"name": "da.train", "num_bytes": 6355313, "num_examples": 2272}, {"name": "da.validation", "num_bytes": 6103284, "num_examples": 1904}, {"name": "de.test", "num_bytes": 62855401, "num_examples": 16066}, {"name": "de.train", "num_bytes": 1750155393, "num_examples": 466188}, {"name": "de.validation", "num_bytes": 62697597, "num_examples": 16066}, {"name": "dv.test", "num_bytes": 8037962, "num_examples": 2248}, {"name": "dv.train", "num_bytes": 9183668, "num_examples": 2610}, {"name": "dv.validation", "num_bytes": 7783753, "num_examples": 2252}, {"name": "el.test", "num_bytes": 4664275, "num_examples": 1694}, {"name": "el.train", "num_bytes": 4864676, "num_examples": 1909}, {"name": "el.validation", "num_bytes": 4699991, "num_examples": 1703}, {"name": "en.test", "num_bytes": 60310390, "num_examples": 16344}, {"name": "en.train", "num_bytes": 3351731661, "num_examples": 921403}, {"name": "en.validation", "num_bytes": 61644400, "num_examples": 16344}, {"name": "eo.test", "num_bytes": 56785008, "num_examples": 14906}, {"name": "eo.train", "num_bytes": 576563781, "num_examples": 143987}, {"name": "eo.validation", "num_bytes": 57118641, "num_examples": 14906}, {"name": "es.test", "num_bytes": 60749604, "num_examples": 15458}, {"name": "es.train", "num_bytes": 759531111, "num_examples": 217773}, {"name": "es.validation", "num_bytes": 59592886, "num_examples": 15458}, {"name": "et.test", "num_bytes": 12127522, "num_examples": 2636}, {"name": "et.train", "num_bytes": 14553259, "num_examples": 3135}, {"name": "et.validation", "num_bytes": 12438683, "num_examples": 2636}, {"name": "eu.test", "num_bytes": 24402418, "num_examples": 6559}, {"name": "eu.train", "num_bytes": 37090572, "num_examples": 10828}, {"name": "eu.validation", "num_bytes": 23340492, "num_examples": 6559}, {"name": "fa.test", "num_bytes": 31226040, "num_examples": 9936}, {"name": "fa.train", "num_bytes": 61919562, "num_examples": 24671}, {"name": "fa.validation", "num_bytes": 26791714, "num_examples": 9936}, {"name": "fi.test", "num_bytes": 5488876, "num_examples": 1725}, {"name": "fi.train", "num_bytes": 6849723, "num_examples": 2204}, {"name": "fi.validation", "num_bytes": 4632355, "num_examples": 1583}, {"name": "fr.test", "num_bytes": 58845726, "num_examples": 16057}, {"name": "fr.train", "num_bytes": 1522321574, "num_examples": 458934}, {"name": "fr.validation", "num_bytes": 57656507, "num_examples": 16057}, {"name": "fy_NL.test", "num_bytes": 10077036, "num_examples": 3024}, {"name": "fy_NL.train", "num_bytes": 11581806, "num_examples": 3699}, {"name": "fy_NL.validation", "num_bytes": 9860204, "num_examples": 3024}, {"name": "ga_IE.test", "num_bytes": 1283221, "num_examples": 511}, {"name": "ga_IE.train", "num_bytes": 1292979, "num_examples": 534}, {"name": "ga_IE.validation", "num_bytes": 1213313, "num_examples": 510}, {"name": "gl.test", "num_bytes": 8536372, "num_examples": 2555}, {"name": "gl.train", "num_bytes": 10223468, "num_examples": 3401}, {"name": "gl.validation", "num_bytes": 7720516, "num_examples": 2395}, {"name": "gn.test", "num_bytes": 773371, "num_examples": 266}, {"name": "gn.train", "num_bytes": 883973, "num_examples": 355}, {"name": "gn.validation", "num_bytes": 578736, "num_examples": 200}, {"name": "ha.test", "num_bytes": 1439995, "num_examples": 468}, {"name": "ha.train", "num_bytes": 5219682, "num_examples": 1944}, {"name": "ha.validation", "num_bytes": 1491709, "num_examples": 531}, {"name": "hi.test", "num_bytes": 9231046, "num_examples": 2838}, {"name": "hi.train", "num_bytes": 12571747, "num_examples": 4320}, {"name": "hi.validation", "num_bytes": 6664794, "num_examples": 2177}, {"name": "hsb.test", "num_bytes": 1895142, "num_examples": 439}, {"name": "hsb.train", "num_bytes": 3461122, "num_examples": 807}, {"name": "hsb.validation", "num_bytes": 703934, "num_examples": 171}, {"name": "hu.test", "num_bytes": 15111305, "num_examples": 4626}, {"name": "hu.train", "num_bytes": 22047310, "num_examples": 6869}, {"name": "hu.validation", "num_bytes": 14341281, "num_examples": 4633}, {"name": "hy_AM.test", "num_bytes": 1494820, "num_examples": 381}, {"name": "hy_AM.train", "num_bytes": 2437800, "num_examples": 598}, {"name": "hy_AM.validation", "num_bytes": 1406137, "num_examples": 351}, {"name": "ia.test", "num_bytes": 4713444, "num_examples": 1730}, {"name": "ia.train", "num_bytes": 12051571, "num_examples": 5048}, {"name": "ia.validation", "num_bytes": 5686851, "num_examples": 1788}, {"name": "id.test", "num_bytes": 9757019, "num_examples": 3620}, {"name": "id.train", "num_bytes": 18924100, "num_examples": 5045}, {"name": "id.validation", "num_bytes": 9094996, "num_examples": 3218}, {"name": "ig.test", "num_bytes": 8206, "num_examples": 3}, {"name": "ig.train", "num_bytes": 24440, "num_examples": 7}, {"name": "ig.validation", "num_bytes": 2773, "num_examples": 1}, {"name": "it.test", "num_bytes": 60718136, "num_examples": 14972}, {"name": "it.train", "num_bytes": 527090062, "num_examples": 149589}, {"name": "it.validation", "num_bytes": 58173429, "num_examples": 14963}, {"name": "ja.test", "num_bytes": 15838437, "num_examples": 4488}, {"name": "ja.train", "num_bytes": 21356719, "num_examples": 6351}, {"name": "ja.validation", "num_bytes": 14045298, "num_examples": 4311}, {"name": "ka.test", "num_bytes": 5462388, "num_examples": 1364}, {"name": "ka.train", "num_bytes": 6690702, "num_examples": 1685}, {"name": "ka.validation", "num_bytes": 5262679, "num_examples": 1352}, {"name": "kab.test", "num_bytes": 35069440, "num_examples": 14890}, {"name": "kab.train", "num_bytes": 299230961, "num_examples": 141619}, {"name": "kab.validation", "num_bytes": 33441408, "num_examples": 14888}, {"name": "kk.test", "num_bytes": 1229154, "num_examples": 383}, {"name": "kk.train", "num_bytes": 1271150, "num_examples": 400}, {"name": "kk.validation", "num_bytes": 1130163, "num_examples": 378}, {"name": "kmr.test", "num_bytes": 7085166, "num_examples": 2397}, {"name": "kmr.train", "num_bytes": 7768188, "num_examples": 2837}, {"name": "kmr.validation", "num_bytes": 6911640, "num_examples": 2374}, {"name": "ky.test", "num_bytes": 5183318, "num_examples": 1612}, {"name": "ky.train", "num_bytes": 5546876, "num_examples": 1786}, {"name": "ky.validation", "num_bytes": 5103317, "num_examples": 1612}, {"name": "lg.test", "num_bytes": 49155042, "num_examples": 12716}, {"name": "lg.train", "num_bytes": 214666581, "num_examples": 55019}, {"name": "lg.validation", "num_bytes": 49293157, "num_examples": 12659}, {"name": "lt.test", "num_bytes": 12648419, "num_examples": 3666}, {"name": "lt.train", "num_bytes": 16794757, "num_examples": 5156}, {"name": "lt.validation", "num_bytes": 12329799, "num_examples": 3504}, {"name": "lv.test", "num_bytes": 4997045, "num_examples": 2160}, {"name": "lv.train", "num_bytes": 6381232, "num_examples": 3102}, {"name": "lv.validation", "num_bytes": 4062326, "num_examples": 1856}, {"name": "mdf.test", "num_bytes": 252634, "num_examples": 77}, {"name": "mdf.train", "num_bytes": 449624, "num_examples": 129}, {"name": "mdf.validation", "num_bytes": 159453, "num_examples": 47}, {"name": "mhr.test", "num_bytes": 39489724, "num_examples": 12796}, {"name": "mhr.train", "num_bytes": 182724800, "num_examples": 59241}, {"name": "mhr.validation", "num_bytes": 37852738, "num_examples": 12582}, {"name": "mk.test", "num_bytes": 53230, "num_examples": 14}, {"name": "mk.train", "num_bytes": 376638, "num_examples": 113}, {"name": "ml.test", "num_bytes": 198457, "num_examples": 79}, {"name": "ml.train", "num_bytes": 1166779, "num_examples": 413}, {"name": "mn.test", "num_bytes": 7182840, "num_examples": 1880}, {"name": "mn.train", "num_bytes": 7524846, "num_examples": 2169}, {"name": "mn.validation", "num_bytes": 6796977, "num_examples": 1852}, {"name": "mr.test", "num_bytes": 7482282, "num_examples": 1760}, {"name": "mr.train", "num_bytes": 9450247, "num_examples": 2283}, {"name": "mr.validation", "num_bytes": 7319155, "num_examples": 1688}, {"name": "mt.test", "num_bytes": 5322515, "num_examples": 1635}, {"name": "mt.train", "num_bytes": 5580390, "num_examples": 1947}, {"name": "mt.validation", "num_bytes": 4742117, "num_examples": 1593}, {"name": "myv.test", "num_bytes": 1830381, "num_examples": 487}, {"name": "myv.train", "num_bytes": 2525361, "num_examples": 683}, {"name": "myv.validation", "num_bytes": 1963442, "num_examples": 497}, {"name": "nan_tw.test", "num_bytes": 1509827, "num_examples": 891}, {"name": "nan_tw.train", "num_bytes": 1686917, "num_examples": 1039}, {"name": "nan_tw.validation", "num_bytes": 1478784, "num_examples": 935}, {"name": "ne_NP.train", "num_bytes": 10967, "num_examples": 3}, {"name": "nl.test", "num_bytes": 33301387, "num_examples": 10640}, {"name": "nl.train", "num_bytes": 88347845, "num_examples": 29520}, {"name": "nl.validation", "num_bytes": 33508392, "num_examples": 10633}, {"name": "nn_NO.test", "num_bytes": 566280, "num_examples": 194}, {"name": "nn_NO.train", "num_bytes": 680138, "num_examples": 239}, {"name": "nn_NO.validation", "num_bytes": 551697, "num_examples": 192}, {"name": "or.test", "num_bytes": 785400, "num_examples": 217}, {"name": "or.train", "num_bytes": 1619908, "num_examples": 476}, {"name": "or.validation", "num_bytes": 1145419, "num_examples": 308}, {"name": "pa_IN.test", "num_bytes": 1176962, "num_examples": 398}, {"name": "pa_IN.train", "num_bytes": 2330389, "num_examples": 684}, {"name": "pa_IN.validation", "num_bytes": 772016, "num_examples": 279}, {"name": "pl.test", "num_bytes": 26392639, "num_examples": 8222}, {"name": "pl.train", "num_bytes": 57587437, "num_examples": 16260}, {"name": "pl.validation", "num_bytes": 26735515, "num_examples": 8222}, {"name": "pt.test", "num_bytes": 26106136, "num_examples": 8610}, {"name": "pt.train", "num_bytes": 45965013, "num_examples": 17851}, {"name": "pt.validation", "num_bytes": 24284616, "num_examples": 8605}, {"name": "rm_sursilv.test", "num_bytes": 4553427, "num_examples": 1328}, {"name": "rm_sursilv.train", "num_bytes": 5574989, "num_examples": 1543}, {"name": "rm_sursilv.validation", "num_bytes": 4671325, "num_examples": 1343}, {"name": "rm_vallader.test", "num_bytes": 1709213, "num_examples": 436}, {"name": "rm_vallader.train", "num_bytes": 2669079, "num_examples": 663}, {"name": "rm_vallader.validation", "num_bytes": 1431722, "num_examples": 375}, {"name": "ro.test", "num_bytes": 10578867, "num_examples": 3840}, {"name": "ro.train", "num_bytes": 13352412, "num_examples": 5079}, {"name": "ro.validation", "num_bytes": 9942172, "num_examples": 3791}, {"name": "ru.test", "num_bytes": 35927971, "num_examples": 9493}, {"name": "ru.train", "num_bytes": 77774104, "num_examples": 22116}, {"name": "ru.validation", "num_bytes": 35006533, "num_examples": 9494}, {"name": "rw.test", "num_bytes": 59207119, "num_examples": 16212}, {"name": "rw.train", "num_bytes": 3392931547, "num_examples": 1003020}, {"name": "rw.validation", "num_bytes": 58174363, "num_examples": 15986}, {"name": "sah.test", "num_bytes": 5310737, "num_examples": 1248}, {"name": "sah.train", "num_bytes": 6426768, "num_examples": 1574}, {"name": "sah.validation", "num_bytes": 4062606, "num_examples": 1082}, {"name": "sat.test", "num_bytes": 367424, "num_examples": 117}, {"name": "sat.train", "num_bytes": 827089, "num_examples": 274}, {"name": "sc.test", "num_bytes": 318509, "num_examples": 98}, {"name": "sc.train", "num_bytes": 595029, "num_examples": 200}, {"name": "sc.validation", "num_bytes": 260174, "num_examples": 79}, {"name": "sk.test", "num_bytes": 5986068, "num_examples": 2240}, {"name": "sk.train", "num_bytes": 7414748, "num_examples": 3028}, {"name": "sk.validation", "num_bytes": 5479212, "num_examples": 2239}, {"name": "sl.test", "num_bytes": 3168082, "num_examples": 1232}, {"name": "sl.train", "num_bytes": 3379634, "num_examples": 1459}, {"name": "sl.validation", "num_bytes": 2802642, "num_examples": 1142}, {"name": "sr.test", "num_bytes": 1281008, "num_examples": 658}, {"name": "sr.train", "num_bytes": 1819521, "num_examples": 1036}, {"name": "sr.validation", "num_bytes": 976212, "num_examples": 622}, {"name": "sv_SE.test", "num_bytes": 14311831, "num_examples": 5056}, {"name": "sv_SE.train", "num_bytes": 19307370, "num_examples": 7274}, {"name": "sv_SE.validation", "num_bytes": 12721433, "num_examples": 5054}, {"name": "sw.test", "num_bytes": 33868314, "num_examples": 9287}, {"name": "sw.train", "num_bytes": 79124614, "num_examples": 21140}, {"name": "sw.validation", "num_bytes": 33448485, "num_examples": 9195}, {"name": "ta.test", "num_bytes": 44837399, "num_examples": 11819}, {"name": "ta.train", "num_bytes": 181092274, "num_examples": 40986}, {"name": "ta.validation", "num_bytes": 46758704, "num_examples": 11780}, {"name": "th.test", "num_bytes": 33957673, "num_examples": 10867}, {"name": "th.train", "num_bytes": 86006549, "num_examples": 31330}, {"name": "th.validation", "num_bytes": 32618248, "num_examples": 10867}, {"name": "tig.train", "num_bytes": 23864, "num_examples": 9}, {"name": "tok.test", "num_bytes": 4244202, "num_examples": 1615}, {"name": "tok.train", "num_bytes": 5015342, "num_examples": 2213}, {"name": "tok.validation", "num_bytes": 4100553, "num_examples": 1755}, {"name": "tr.test", "num_bytes": 23798173, "num_examples": 9123}, {"name": "tr.train", "num_bytes": 44100333, "num_examples": 20227}, {"name": "tr.validation", "num_bytes": 21236995, "num_examples": 9094}, {"name": "tt.test", "num_bytes": 13473024, "num_examples": 5118}, {"name": "tt.train", "num_bytes": 24182984, "num_examples": 9782}, {"name": "tt.validation", "num_bytes": 7320766, "num_examples": 3061}, {"name": "ug.test", "num_bytes": 11238325, "num_examples": 2746}, {"name": "ug.train", "num_bytes": 12826453, "num_examples": 3292}, {"name": "ug.validation", "num_bytes": 10680174, "num_examples": 2747}, {"name": "uk.test", "num_bytes": 23136165, "num_examples": 6784}, {"name": "uk.train", "num_bytes": 33020773, "num_examples": 11462}, {"name": "uk.validation", "num_bytes": 22236240, "num_examples": 6785}, {"name": "ur.test", "num_bytes": 8994468, "num_examples": 3297}, {"name": "ur.train", "num_bytes": 11907797, "num_examples": 4127}, {"name": "ur.validation", "num_bytes": 9152835, "num_examples": 3302}, {"name": "uz.test", "num_bytes": 40939367, "num_examples": 12241}, {"name": "uz.train", "num_bytes": 128948587, "num_examples": 47081}, {"name": "uz.validation", "num_bytes": 34820511, "num_examples": 11569}, {"name": "vi.test", "num_bytes": 2874673, "num_examples": 1194}, {"name": "vi.train", "num_bytes": 7073358, "num_examples": 2558}, {"name": "vi.validation", "num_bytes": 501685, "num_examples": 226}, {"name": "vot.test", "num_bytes": 8259, "num_examples": 5}, {"name": "vot.train", "num_bytes": 242122, "num_examples": 95}, {"name": "yue.test", "num_bytes": 6096935, "num_examples": 2167}, {"name": "yue.train", "num_bytes": 6683156, "num_examples": 2546}, {"name": "yue.validation", "num_bytes": 5633352, "num_examples": 2156}, {"name": "zh_CN.test", "num_bytes": 36626532, "num_examples": 9782}, {"name": "zh_CN.train", "num_bytes": 81185061, "num_examples": 23763}, {"name": "zh_CN.validation", "num_bytes": 34695094, "num_examples": 9759}, {"name": "zh_HK.test", "num_bytes": 18198362, "num_examples": 5586}, {"name": "zh_HK.train", "num_bytes": 21935901, "num_examples": 8413}, {"name": "zh_HK.validation", "num_bytes": 16904959, "num_examples": 5586}, {"name": "zh_TW.test", "num_bytes": 10821247, "num_examples": 4669}, {"name": "zh_TW.train", "num_bytes": 13408983, "num_examples": 6493}, {"name": "zh_TW.validation", "num_bytes": 10000814, "num_examples": 4669}], "download_size": 3412816221, "dataset_size": 21642019134}} | 2022-11-23T15:09:06+00:00 | [] | [] | TAGS
#region-us
| # Dataset Card for "commonvoice_10_unit"
More Information needed | [
"# Dataset Card for \"commonvoice_10_unit\"\n\nMore Information needed"
] | [
"TAGS\n#region-us \n",
"# Dataset Card for \"commonvoice_10_unit\"\n\nMore Information needed"
] | [
6,
19
] | [
"passage: TAGS\n#region-us \n# Dataset Card for \"commonvoice_10_unit\"\n\nMore Information needed"
] |
634210fd7538b888b711108a258ef2547d6b259d | # Dataset Card for "uneven-side-walk"
[More Information needed](https://github.com/huggingface/datasets/blob/main/CONTRIBUTING.md#how-to-contribute-to-the-dataset-cards) | omree/uneven-side-walk | [
"region:us"
] | 2022-11-23T13:21:25+00:00 | {"dataset_info": {"features": [{"name": "pixel_values", "dtype": "image"}, {"name": "label", "dtype": "image"}], "splits": [{"name": "train", "num_bytes": 26515652.0, "num_examples": 57}], "download_size": 26512665, "dataset_size": 26515652.0}} | 2022-11-23T13:24:21+00:00 | [] | [] | TAGS
#region-us
| # Dataset Card for "uneven-side-walk"
More Information needed | [
"# Dataset Card for \"uneven-side-walk\"\n\nMore Information needed"
] | [
"TAGS\n#region-us \n",
"# Dataset Card for \"uneven-side-walk\"\n\nMore Information needed"
] | [
6,
16
] | [
"passage: TAGS\n#region-us \n# Dataset Card for \"uneven-side-walk\"\n\nMore Information needed"
] |
1fcfc0db6c20cc37e2b1a37d8ac2169e75c77739 | # Dataset Card for "flowers-dataset"
[More Information needed](https://github.com/huggingface/datasets/blob/main/CONTRIBUTING.md#how-to-contribute-to-the-dataset-cards) | VishwanathanR/flowers-dataset | [
"region:us"
] | 2022-11-23T14:05:29+00:00 | {"dataset_info": {"features": [{"name": "image", "dtype": "image"}], "splits": [{"name": "train", "num_bytes": 347100141.78, "num_examples": 8189}], "download_size": 346573740, "dataset_size": 347100141.78}} | 2022-11-23T14:08:09+00:00 | [] | [] | TAGS
#region-us
| # Dataset Card for "flowers-dataset"
More Information needed | [
"# Dataset Card for \"flowers-dataset\"\n\nMore Information needed"
] | [
"TAGS\n#region-us \n",
"# Dataset Card for \"flowers-dataset\"\n\nMore Information needed"
] | [
6,
15
] | [
"passage: TAGS\n#region-us \n# Dataset Card for \"flowers-dataset\"\n\nMore Information needed"
] |
93247bd2f4b86e91f20979bf775ceeafd9b2dd93 | # Dataset Card for "dalio-book-handwritten-io"
[More Information needed](https://github.com/huggingface/datasets/blob/main/CONTRIBUTING.md#how-to-contribute-to-the-dataset-cards) | AlekseyKorshuk/dalio-book-handwritten-io | [
"region:us"
] | 2022-11-23T14:34:28+00:00 | {"dataset_info": {"features": [{"name": "input_text", "dtype": "string"}, {"name": "output_text", "dtype": "string"}], "splits": [{"name": "train", "num_bytes": 674482, "num_examples": 442}, {"name": "validation", "num_bytes": 519665, "num_examples": 315}, {"name": "test", "num_bytes": 14786, "num_examples": 10}], "download_size": 673908, "dataset_size": 1208933}} | 2022-11-23T14:36:15+00:00 | [] | [] | TAGS
#region-us
| # Dataset Card for "dalio-book-handwritten-io"
More Information needed | [
"# Dataset Card for \"dalio-book-handwritten-io\"\n\nMore Information needed"
] | [
"TAGS\n#region-us \n",
"# Dataset Card for \"dalio-book-handwritten-io\"\n\nMore Information needed"
] | [
6,
20
] | [
"passage: TAGS\n#region-us \n# Dataset Card for \"dalio-book-handwritten-io\"\n\nMore Information needed"
] |
71d57cdd02cc87558350e3fbd4e9d706e5b65123 | # Dataset Card for "dalio-book-handwritten-io-sorted"
[More Information needed](https://github.com/huggingface/datasets/blob/main/CONTRIBUTING.md#how-to-contribute-to-the-dataset-cards) | AlekseyKorshuk/dalio-book-handwritten-io-sorted | [
"region:us"
] | 2022-11-23T14:53:50+00:00 | {"dataset_info": {"features": [{"name": "input_text", "dtype": "string"}, {"name": "output_text", "dtype": "string"}], "splits": [{"name": "train", "num_bytes": 674482.0, "num_examples": 442}, {"name": "validation", "num_bytes": 519665, "num_examples": 315}, {"name": "test", "num_bytes": 14786, "num_examples": 10}], "download_size": 0, "dataset_size": 1208933.0}} | 2022-11-23T15:15:05+00:00 | [] | [] | TAGS
#region-us
| # Dataset Card for "dalio-book-handwritten-io-sorted"
More Information needed | [
"# Dataset Card for \"dalio-book-handwritten-io-sorted\"\n\nMore Information needed"
] | [
"TAGS\n#region-us \n",
"# Dataset Card for \"dalio-book-handwritten-io-sorted\"\n\nMore Information needed"
] | [
6,
23
] | [
"passage: TAGS\n#region-us \n# Dataset Card for \"dalio-book-handwritten-io-sorted\"\n\nMore Information needed"
] |
2defe9c703068134b5dd2a7dc4c306768c4328ee | # Dataset Card for "python_comment_code_ratio_08"
[More Information needed](https://github.com/huggingface/datasets/blob/main/CONTRIBUTING.md#how-to-contribute-to-the-dataset-cards) | loubnabnl/python_comment_code_ratio_08 | [
"region:us"
] | 2022-11-23T16:02:32+00:00 | {"dataset_info": {"features": [{"name": "content", "dtype": "string"}, {"name": "avg_line_length", "dtype": "float64"}, {"name": "max_line_length", "dtype": "int64"}, {"name": "alphanum_fraction", "dtype": "float64"}, {"name": "licenses", "sequence": "string"}, {"name": "repository_name", "dtype": "string"}, {"name": "path", "dtype": "string"}, {"name": "size", "dtype": "int64"}, {"name": "lang", "dtype": "string"}, {"name": "nl_text", "dtype": "string"}, {"name": "nl_size", "dtype": "int64"}, {"name": "nl_ratio", "dtype": "float64"}], "splits": [{"name": "train", "num_bytes": 1272677.3664, "num_examples": 131}], "download_size": 324517, "dataset_size": 1272677.3664}} | 2022-11-23T16:02:40+00:00 | [] | [] | TAGS
#region-us
| # Dataset Card for "python_comment_code_ratio_08"
More Information needed | [
"# Dataset Card for \"python_comment_code_ratio_08\"\n\nMore Information needed"
] | [
"TAGS\n#region-us \n",
"# Dataset Card for \"python_comment_code_ratio_08\"\n\nMore Information needed"
] | [
6,
22
] | [
"passage: TAGS\n#region-us \n# Dataset Card for \"python_comment_code_ratio_08\"\n\nMore Information needed"
] |
cf78db2ab9f1a43fac1c906113ec6d798c971dbc | # Dataset Card for "Georges_Sand"
[More Information needed](https://github.com/huggingface/datasets/blob/main/CONTRIBUTING.md#how-to-contribute-to-the-dataset-cards) | channotte/Georges_Sand | [
"region:us"
] | 2022-11-23T16:35:52+00:00 | {"dataset_info": {"features": [{"name": "text", "dtype": "string"}], "splits": [{"name": "train", "num_bytes": 2455525, "num_examples": 42086}, {"name": "test", "num_bytes": 258701, "num_examples": 5286}], "download_size": 1777131, "dataset_size": 2714226}} | 2022-11-25T13:21:17+00:00 | [] | [] | TAGS
#region-us
| # Dataset Card for "Georges_Sand"
More Information needed | [
"# Dataset Card for \"Georges_Sand\"\n\nMore Information needed"
] | [
"TAGS\n#region-us \n",
"# Dataset Card for \"Georges_Sand\"\n\nMore Information needed"
] | [
6,
15
] | [
"passage: TAGS\n#region-us \n# Dataset Card for \"Georges_Sand\"\n\nMore Information needed"
] |
a1a8e13e8a27b400fc35876baf59013f401976f3 | ---
# booksum short
`BookSum` but all summaries with length greater than 512 `long-t5` tokens are filtered out.
The columns `chapter_length` and `summary_length` **in this dataset** have been updated to reflect the total of Long-T5 tokens in the respective source text.
## Token Length Distribution for inputs
 | pszemraj/booksum-short | [
"task_categories:summarization",
"task_categories:text2text-generation",
"size_categories:10K<n<100K",
"source_datasets:kmfoda/booksum",
"language:en",
"license:bsd-3-clause",
"booksum",
"long-document",
"region:us"
] | 2022-11-23T16:40:45+00:00 | {"language": ["en"], "license": ["bsd-3-clause"], "size_categories": ["10K<n<100K"], "source_datasets": "kmfoda/booksum", "task_categories": ["summarization", "text2text-generation"], "tags": ["booksum", "long-document"], "train-eval-index": [{"config": "pszemraj--booksum_short", "task": "summarization", "task_id": "summarization", "splits": {"eval_split": "test"}, "col_mapping": {"chapter": "text", "summary_text": "target"}}]} | 2023-02-27T08:45:01+00:00 | [] | [
"en"
] | TAGS
#task_categories-summarization #task_categories-text2text-generation #size_categories-10K<n<100K #source_datasets-kmfoda/booksum #language-English #license-bsd-3-clause #booksum #long-document #region-us
| ---
# booksum short
'BookSum' but all summaries with length greater than 512 'long-t5' tokens are filtered out.
The columns 'chapter_length' and 'summary_length' in this dataset have been updated to reflect the total of Long-T5 tokens in the respective source text.
## Token Length Distribution for inputs
!distribution | [
"# booksum short\n\n\n'BookSum' but all summaries with length greater than 512 'long-t5' tokens are filtered out.\n\nThe columns 'chapter_length' and 'summary_length' in this dataset have been updated to reflect the total of Long-T5 tokens in the respective source text.",
"## Token Length Distribution for inputs\n\n!distribution"
] | [
"TAGS\n#task_categories-summarization #task_categories-text2text-generation #size_categories-10K<n<100K #source_datasets-kmfoda/booksum #language-English #license-bsd-3-clause #booksum #long-document #region-us \n",
"# booksum short\n\n\n'BookSum' but all summaries with length greater than 512 'long-t5' tokens are filtered out.\n\nThe columns 'chapter_length' and 'summary_length' in this dataset have been updated to reflect the total of Long-T5 tokens in the respective source text.",
"## Token Length Distribution for inputs\n\n!distribution"
] | [
75,
74,
13
] | [
"passage: TAGS\n#task_categories-summarization #task_categories-text2text-generation #size_categories-10K<n<100K #source_datasets-kmfoda/booksum #language-English #license-bsd-3-clause #booksum #long-document #region-us \n# booksum short\n\n\n'BookSum' but all summaries with length greater than 512 'long-t5' tokens are filtered out.\n\nThe columns 'chapter_length' and 'summary_length' in this dataset have been updated to reflect the total of Long-T5 tokens in the respective source text.## Token Length Distribution for inputs\n\n!distribution"
] |
44258931f74e10d8163031ff7832702a786bbbe9 | # Dataset Card for "word_based_IAM_SROIE_text_recognition"
[More Information needed](https://github.com/huggingface/datasets/blob/main/CONTRIBUTING.md#how-to-contribute-to-the-dataset-cards) | priyank-m/word_based_IAM_SROIE_text_recognition | [
"region:us"
] | 2022-11-23T17:28:23+00:00 | {"dataset_info": {"features": [{"name": "image", "dtype": "image"}, {"name": "text", "dtype": "string"}], "splits": [{"name": "train", "num_bytes": 1683757167.625, "num_examples": 221155}, {"name": "test", "num_bytes": 21328701.375, "num_examples": 3149}, {"name": "val", "num_bytes": 21325608.875, "num_examples": 3145}], "download_size": 1726460218, "dataset_size": 1726411477.875}} | 2022-11-23T17:29:21+00:00 | [] | [] | TAGS
#region-us
| # Dataset Card for "word_based_IAM_SROIE_text_recognition"
More Information needed | [
"# Dataset Card for \"word_based_IAM_SROIE_text_recognition\"\n\nMore Information needed"
] | [
"TAGS\n#region-us \n",
"# Dataset Card for \"word_based_IAM_SROIE_text_recognition\"\n\nMore Information needed"
] | [
6,
26
] | [
"passage: TAGS\n#region-us \n# Dataset Card for \"word_based_IAM_SROIE_text_recognition\"\n\nMore Information needed"
] |
efc90a44393c848c051f794b3d3d53588f1607fc |
# booksum - 1024 tokens max output
**goal:** limit max output length explicitly to prevent partial summaries being generated.
- [notebook](https://colab.research.google.com/gist/pszemraj/7f7b66d535441a9d0e8419fde1e1c98a/booksum-1024.ipynb) to create
## info
 | pszemraj/booksum-1024-output | [
"task_categories:summarization",
"task_categories:text2text-generation",
"size_categories:1K<n<10K",
"source_datasets:kmfoda/booksum",
"license:bsd-3-clause",
"region:us"
] | 2022-11-23T20:02:05+00:00 | {"license": ["bsd-3-clause"], "size_categories": ["1K<n<10K"], "source_datasets": "kmfoda/booksum", "task_categories": ["summarization", "text2text-generation"], "train-eval-index": [{"config": "pszemraj--booksum_1024", "task": "summarization", "task_id": "summarization", "splits": {"eval_split": "test"}, "col_mapping": {"chapter": "text", "summary_text": "target"}}]} | 2023-11-21T13:15:10+00:00 | [] | [] | TAGS
#task_categories-summarization #task_categories-text2text-generation #size_categories-1K<n<10K #source_datasets-kmfoda/booksum #license-bsd-3-clause #region-us
|
# booksum - 1024 tokens max output
goal: limit max output length explicitly to prevent partial summaries being generated.
- notebook to create
## info
!basic eda | [
"# booksum - 1024 tokens max output\n\n\ngoal: limit max output length explicitly to prevent partial summaries being generated.\n\n- notebook to create",
"## info\n\n\n!basic eda"
] | [
"TAGS\n#task_categories-summarization #task_categories-text2text-generation #size_categories-1K<n<10K #source_datasets-kmfoda/booksum #license-bsd-3-clause #region-us \n",
"# booksum - 1024 tokens max output\n\n\ngoal: limit max output length explicitly to prevent partial summaries being generated.\n\n- notebook to create",
"## info\n\n\n!basic eda"
] | [
64,
31,
7
] | [
"passage: TAGS\n#task_categories-summarization #task_categories-text2text-generation #size_categories-1K<n<10K #source_datasets-kmfoda/booksum #license-bsd-3-clause #region-us \n# booksum - 1024 tokens max output\n\n\ngoal: limit max output length explicitly to prevent partial summaries being generated.\n\n- notebook to create## info\n\n\n!basic eda"
] |
2be4d3b7dd319a291e5dce35b74ae61476310156 | # Dataset Card for "test-glue"
[More Information needed](https://github.com/huggingface/datasets/blob/main/CONTRIBUTING.md#how-to-contribute-to-the-dataset-cards) | NimaBoscarino/test-glue | [
"region:us"
] | 2022-11-23T23:14:41+00:00 | {"dataset_info": {"features": [{"name": "sentence", "dtype": "string"}, {"name": "label", "dtype": {"class_label": {"names": {"0": "unacceptable", "1": "acceptable"}}}}, {"name": "idx", "dtype": "int32"}], "splits": [{"name": "train", "num_bytes": 5145, "num_examples": 100}], "download_size": 4268, "dataset_size": 5145}} | 2022-11-23T23:14:47+00:00 | [] | [] | TAGS
#region-us
| # Dataset Card for "test-glue"
More Information needed | [
"# Dataset Card for \"test-glue\"\n\nMore Information needed"
] | [
"TAGS\n#region-us \n",
"# Dataset Card for \"test-glue\"\n\nMore Information needed"
] | [
6,
14
] | [
"passage: TAGS\n#region-us \n# Dataset Card for \"test-glue\"\n\nMore Information needed"
] |
796b60bb950d484c794605f6d962c38ba1043a25 | # Dataset Card for "yannic-aleksa-2min"
[More Information needed](https://github.com/huggingface/datasets/blob/main/CONTRIBUTING.md#how-to-contribute-to-the-dataset-cards) | kpriyanshu256/yannic-aleksa-2min | [
"region:us"
] | 2022-11-24T03:38:29+00:00 | {"dataset_info": {"features": [{"name": "TRANSCRIPTION", "dtype": "string"}, {"name": "text", "dtype": "string"}], "splits": [{"name": "train", "num_bytes": 15401256, "num_examples": 1105}], "download_size": 7783618, "dataset_size": 15401256}} | 2022-11-24T03:38:35+00:00 | [] | [] | TAGS
#region-us
| # Dataset Card for "yannic-aleksa-2min"
More Information needed | [
"# Dataset Card for \"yannic-aleksa-2min\"\n\nMore Information needed"
] | [
"TAGS\n#region-us \n",
"# Dataset Card for \"yannic-aleksa-2min\"\n\nMore Information needed"
] | [
6,
17
] | [
"passage: TAGS\n#region-us \n# Dataset Card for \"yannic-aleksa-2min\"\n\nMore Information needed"
] |
2ab0579ead657f5567b5596e0d859c00d5918878 |
# Dataset Card for Coco
## Table of Contents
- [Table of Contents](#table-of-contents)
- [Dataset Description](#dataset-description)
- [Dataset Summary](#dataset-summary)
- [Supported Tasks and Leaderboards](#supported-tasks-and-leaderboards)
- [Languages](#languages)
- [Dataset Structure](#dataset-structure)
- [Data Instances](#data-instances)
- [Data Fields](#data-fields)
- [Data Splits](#data-splits)
- [Dataset Creation](#dataset-creation)
- [Curation Rationale](#curation-rationale)
- [Source Data](#source-data)
- [Annotations](#annotations)
- [Personal and Sensitive Information](#personal-and-sensitive-information)
- [Considerations for Using the Data](#considerations-for-using-the-data)
- [Social Impact of Dataset](#social-impact-of-dataset)
- [Discussion of Biases](#discussion-of-biases)
- [Other Known Limitations](#other-known-limitations)
- [Additional Information](#additional-information)
- [Dataset Curators](#dataset-curators)
- [Licensing Information](#licensing-information)
- [Citation Information](#citation-information)
- [Contributions](#contributions)
## Dataset Description
- **Homepage:** https://cocodataset.org/#home
- **Repository:** https://huggingface.co/datasets/eto-ai/coco
- **Paper:**
- **Leaderboard:**
- **Point of Contact:** [email protected]
### Dataset Summary
Microsoft COCO (Common Objects in Context) dataset.
Before using this dataset, please make sure [`Huggingface datasets`](https://huggingface.co/docs/datasets/installation)
and [`Lance`](https://github.com/eto-ai/lance) are installed.
```shell
pip install datasets[vision] pylance
```
### Supported Tasks and Leaderboards
[More Information Needed]
### Languages
English
## Dataset Structure
### Data Instances
[More Information Needed]
### Data Fields
[More Information Needed]
### Data Splits
Data is split into `train`, `val` and `test`.
## Dataset Creation
### Curation Rationale
[More Information Needed]
### Source Data
#### Initial Data Collection and Normalization
[More Information Needed]
#### Who are the source language producers?
[More Information Needed]
### Annotations
#### Annotation process
[More Information Needed]
#### Who are the annotators?
[More Information Needed]
### Personal and Sensitive Information
N/A
## Considerations for Using the Data
### Social Impact of Dataset
[More Information Needed]
### Discussion of Biases
[More Information Needed]
### Other Known Limitations
[More Information Needed]
## Additional Information
### Dataset Curators
[More Information Needed]
### Licensing Information
[More Information Needed]
### Citation Information
[More Information Needed]
### Contributions
Thanks to [@eto-ai](https://github.com/eto-ai) for adding this dataset.
| eto-ai/coco | [
"task_categories:object-detection",
"annotations_creators:crowdsourced",
"language_creators:crowdsourced",
"multilinguality:monolingual",
"size_categories:100K<n<1M",
"language:en",
"license:cc-by-4.0",
"object detection",
"ssd",
"lance",
"region:us"
] | 2022-11-24T04:13:45+00:00 | {"annotations_creators": ["crowdsourced"], "language_creators": ["crowdsourced"], "language": ["en"], "license": ["cc-by-4.0"], "multilinguality": ["monolingual"], "size_categories": ["100K<n<1M"], "source_datasets": [], "task_categories": ["object-detection"], "task_ids": [], "pretty_name": "COCO", "tags": ["object detection", "ssd", "lance"]} | 2022-11-24T04:51:34+00:00 | [] | [
"en"
] | TAGS
#task_categories-object-detection #annotations_creators-crowdsourced #language_creators-crowdsourced #multilinguality-monolingual #size_categories-100K<n<1M #language-English #license-cc-by-4.0 #object detection #ssd #lance #region-us
|
# Dataset Card for Coco
## Table of Contents
- Table of Contents
- Dataset Description
- Dataset Summary
- Supported Tasks and Leaderboards
- Languages
- Dataset Structure
- Data Instances
- Data Fields
- Data Splits
- Dataset Creation
- Curation Rationale
- Source Data
- Annotations
- Personal and Sensitive Information
- Considerations for Using the Data
- Social Impact of Dataset
- Discussion of Biases
- Other Known Limitations
- Additional Information
- Dataset Curators
- Licensing Information
- Citation Information
- Contributions
## Dataset Description
- Homepage: URL
- Repository: URL
- Paper:
- Leaderboard:
- Point of Contact: contact@URL
### Dataset Summary
Microsoft COCO (Common Objects in Context) dataset.
Before using this dataset, please make sure 'Huggingface datasets'
and 'Lance' are installed.
### Supported Tasks and Leaderboards
### Languages
English
## Dataset Structure
### Data Instances
### Data Fields
### Data Splits
Data is split into 'train', 'val' and 'test'.
## Dataset Creation
### Curation Rationale
### Source Data
#### Initial Data Collection and Normalization
#### Who are the source language producers?
### Annotations
#### Annotation process
#### Who are the annotators?
### Personal and Sensitive Information
N/A
## Considerations for Using the Data
### Social Impact of Dataset
### Discussion of Biases
### Other Known Limitations
## Additional Information
### Dataset Curators
### Licensing Information
### Contributions
Thanks to @eto-ai for adding this dataset.
| [
"# Dataset Card for Coco",
"## Table of Contents\n- Table of Contents\n- Dataset Description\n - Dataset Summary\n - Supported Tasks and Leaderboards\n - Languages\n- Dataset Structure\n - Data Instances\n - Data Fields\n - Data Splits\n- Dataset Creation\n - Curation Rationale\n - Source Data\n - Annotations\n - Personal and Sensitive Information\n- Considerations for Using the Data\n - Social Impact of Dataset\n - Discussion of Biases\n - Other Known Limitations\n- Additional Information\n - Dataset Curators\n - Licensing Information\n - Citation Information\n - Contributions",
"## Dataset Description\n\n- Homepage: URL\n\n- Repository: URL\n\n- Paper:\n- Leaderboard:\n- Point of Contact: contact@URL",
"### Dataset Summary\n\nMicrosoft COCO (Common Objects in Context) dataset.\n\nBefore using this dataset, please make sure 'Huggingface datasets'\nand 'Lance' are installed.",
"### Supported Tasks and Leaderboards",
"### Languages\n\nEnglish",
"## Dataset Structure",
"### Data Instances",
"### Data Fields",
"### Data Splits\n\nData is split into 'train', 'val' and 'test'.",
"## Dataset Creation",
"### Curation Rationale",
"### Source Data",
"#### Initial Data Collection and Normalization",
"#### Who are the source language producers?",
"### Annotations",
"#### Annotation process",
"#### Who are the annotators?",
"### Personal and Sensitive Information\n\nN/A",
"## Considerations for Using the Data",
"### Social Impact of Dataset",
"### Discussion of Biases",
"### Other Known Limitations",
"## Additional Information",
"### Dataset Curators",
"### Licensing Information",
"### Contributions\n\nThanks to @eto-ai for adding this dataset."
] | [
"TAGS\n#task_categories-object-detection #annotations_creators-crowdsourced #language_creators-crowdsourced #multilinguality-monolingual #size_categories-100K<n<1M #language-English #license-cc-by-4.0 #object detection #ssd #lance #region-us \n",
"# Dataset Card for Coco",
"## Table of Contents\n- Table of Contents\n- Dataset Description\n - Dataset Summary\n - Supported Tasks and Leaderboards\n - Languages\n- Dataset Structure\n - Data Instances\n - Data Fields\n - Data Splits\n- Dataset Creation\n - Curation Rationale\n - Source Data\n - Annotations\n - Personal and Sensitive Information\n- Considerations for Using the Data\n - Social Impact of Dataset\n - Discussion of Biases\n - Other Known Limitations\n- Additional Information\n - Dataset Curators\n - Licensing Information\n - Citation Information\n - Contributions",
"## Dataset Description\n\n- Homepage: URL\n\n- Repository: URL\n\n- Paper:\n- Leaderboard:\n- Point of Contact: contact@URL",
"### Dataset Summary\n\nMicrosoft COCO (Common Objects in Context) dataset.\n\nBefore using this dataset, please make sure 'Huggingface datasets'\nand 'Lance' are installed.",
"### Supported Tasks and Leaderboards",
"### Languages\n\nEnglish",
"## Dataset Structure",
"### Data Instances",
"### Data Fields",
"### Data Splits\n\nData is split into 'train', 'val' and 'test'.",
"## Dataset Creation",
"### Curation Rationale",
"### Source Data",
"#### Initial Data Collection and Normalization",
"#### Who are the source language producers?",
"### Annotations",
"#### Annotation process",
"#### Who are the annotators?",
"### Personal and Sensitive Information\n\nN/A",
"## Considerations for Using the Data",
"### Social Impact of Dataset",
"### Discussion of Biases",
"### Other Known Limitations",
"## Additional Information",
"### Dataset Curators",
"### Licensing Information",
"### Contributions\n\nThanks to @eto-ai for adding this dataset."
] | [
84,
6,
125,
29,
47,
10,
5,
6,
6,
5,
22,
5,
7,
4,
10,
10,
5,
5,
9,
11,
8,
7,
8,
7,
5,
6,
6,
17
] | [
"passage: TAGS\n#task_categories-object-detection #annotations_creators-crowdsourced #language_creators-crowdsourced #multilinguality-monolingual #size_categories-100K<n<1M #language-English #license-cc-by-4.0 #object detection #ssd #lance #region-us \n# Dataset Card for Coco## Table of Contents\n- Table of Contents\n- Dataset Description\n - Dataset Summary\n - Supported Tasks and Leaderboards\n - Languages\n- Dataset Structure\n - Data Instances\n - Data Fields\n - Data Splits\n- Dataset Creation\n - Curation Rationale\n - Source Data\n - Annotations\n - Personal and Sensitive Information\n- Considerations for Using the Data\n - Social Impact of Dataset\n - Discussion of Biases\n - Other Known Limitations\n- Additional Information\n - Dataset Curators\n - Licensing Information\n - Citation Information\n - Contributions## Dataset Description\n\n- Homepage: URL\n\n- Repository: URL\n\n- Paper:\n- Leaderboard:\n- Point of Contact: contact@URL### Dataset Summary\n\nMicrosoft COCO (Common Objects in Context) dataset.\n\nBefore using this dataset, please make sure 'Huggingface datasets'\nand 'Lance' are installed.### Supported Tasks and Leaderboards### Languages\n\nEnglish## Dataset Structure### Data Instances### Data Fields### Data Splits\n\nData is split into 'train', 'val' and 'test'.## Dataset Creation### Curation Rationale### Source Data#### Initial Data Collection and Normalization#### Who are the source language producers?### Annotations#### Annotation process#### Who are the annotators?### Personal and Sensitive Information\n\nN/A## Considerations for Using the Data### Social Impact of Dataset### Discussion of Biases### Other Known Limitations## Additional Information### Dataset Curators### Licensing Information### Contributions\n\nThanks to @eto-ai for adding this dataset."
] |
a2ea2613deecdfc446205e53f57f6b986da4fe4c | # Dataset Card for "oct"
[More Information needed](https://github.com/huggingface/datasets/blob/main/CONTRIBUTING.md#how-to-contribute-to-the-dataset-cards) | pattern90/test4 | [
"region:us"
] | 2022-11-24T04:49:24+00:00 | {"dataset_info": {"features": [{"name": "pixel_values", "dtype": "image"}, {"name": "label", "dtype": "image"}], "splits": [{"name": "train", "num_bytes": 380878.0, "num_examples": 6}], "download_size": 80720, "dataset_size": 380878.0}} | 2022-11-24T05:21:15+00:00 | [] | [] | TAGS
#region-us
| # Dataset Card for "oct"
More Information needed | [
"# Dataset Card for \"oct\"\n\nMore Information needed"
] | [
"TAGS\n#region-us \n",
"# Dataset Card for \"oct\"\n\nMore Information needed"
] | [
6,
12
] | [
"passage: TAGS\n#region-us \n# Dataset Card for \"oct\"\n\nMore Information needed"
] |
b3249bf5ea68ef377867e98ade2b81a62d1bee2d | # Dataset Card for "sidewalk-imagery"
[More Information needed](https://github.com/huggingface/datasets/blob/main/CONTRIBUTING.md#how-to-contribute-to-the-dataset-cards) | pattern90/sidewalk-imagery | [
"region:us"
] | 2022-11-24T05:01:47+00:00 | {"dataset_info": {"features": [{"name": "pixel_values", "dtype": "image"}, {"name": "label", "dtype": "image"}], "splits": [{"name": "train", "num_bytes": 3138386.0, "num_examples": 10}], "download_size": 3139599, "dataset_size": 3138386.0}} | 2022-11-24T05:02:00+00:00 | [] | [] | TAGS
#region-us
| # Dataset Card for "sidewalk-imagery"
More Information needed | [
"# Dataset Card for \"sidewalk-imagery\"\n\nMore Information needed"
] | [
"TAGS\n#region-us \n",
"# Dataset Card for \"sidewalk-imagery\"\n\nMore Information needed"
] | [
6,
15
] | [
"passage: TAGS\n#region-us \n# Dataset Card for \"sidewalk-imagery\"\n\nMore Information needed"
] |
ba9ff7770738383a23406c0e05eae6bf63dd903b | # Dataset Card for "es_en_2999"
[More Information needed](https://github.com/huggingface/datasets/blob/main/CONTRIBUTING.md#how-to-contribute-to-the-dataset-cards) | watermelonhydro/es_en_2999 | [
"region:us"
] | 2022-11-24T05:24:33+00:00 | {"dataset_info": {"features": [{"name": "inputs", "dtype": "string"}, {"name": "targets", "dtype": "string"}], "splits": [{"name": "train", "num_bytes": 1361312, "num_examples": 2999}], "download_size": 770888, "dataset_size": 1361312}} | 2022-11-24T05:40:39+00:00 | [] | [] | TAGS
#region-us
| # Dataset Card for "es_en_2999"
More Information needed | [
"# Dataset Card for \"es_en_2999\"\n\nMore Information needed"
] | [
"TAGS\n#region-us \n",
"# Dataset Card for \"es_en_2999\"\n\nMore Information needed"
] | [
6,
16
] | [
"passage: TAGS\n#region-us \n# Dataset Card for \"es_en_2999\"\n\nMore Information needed"
] |
536229439ec7f920e01c18f70808a17854521797 | # Filipino Storytelling Books
## Dataset Description
The dataset consists of 148 Filipino storytelling books, 5,005 total sentences, 45,792 total tokens, and 5,646 unique tokens.
This NER model only supports the Filipino language and does not include proper nouns, verbs, adjectives, and adverbs as of the moment
The input must undergo preprocessing. Soon I will upload the code to GitHub for preprocessing the input
To replicate the preprocessed input use this example as a guide
Input: "May umaapoy na bahay "
Preprocessed Input: "apoy bahay"
### Languages
The BCP-47 code for the dataset's language is Filipino.
## Dataset Structure
### Data Instances
A sample from this dataset looks as follows:
```json
[
{
"tokens": [
"toot"
],
"tags": [
3
]
},
{
"tokens": [
"hindi",
"ako",
"yun",
"boltu"
],
"tags": [
3,
1,
3,
3
]
}
]
```
### Dataset Fields
The dataset has the following fields (also called "features"):
```json
{
"tokens": "Sequence(feature=Value(dtype='string', id=None), length=-1, id=None)",
"tags": "Sequence(feature=ClassLabel(num_classes=7, names=['Animals', 'Humans_Body', 'Natural_Environment', 'O', 'Objects', 'Transportation', 'Urban_Environment'], id=None), length=-1, id=None)"
}
```
### Dataset Splits
This dataset is split into a train and validation split. The split sizes are as follow:
| Split name | Num samples |
| ------------ | ------------------- |
| train | 3279 |
| valid | 1244 |
| scostiniano/storytelling_books_filipino | [
"doi:10.57967/hf/0202",
"region:us"
] | 2022-11-24T05:57:53+00:00 | {} | 2022-11-24T08:11:51+00:00 | [] | [] | TAGS
#doi-10.57967/hf/0202 #region-us
| Filipino Storytelling Books
===========================
Dataset Description
-------------------
The dataset consists of 148 Filipino storytelling books, 5,005 total sentences, 45,792 total tokens, and 5,646 unique tokens.
This NER model only supports the Filipino language and does not include proper nouns, verbs, adjectives, and adverbs as of the moment
The input must undergo preprocessing. Soon I will upload the code to GitHub for preprocessing the input
To replicate the preprocessed input use this example as a guide
Input: "May umaapoy na bahay "
Preprocessed Input: "apoy bahay"
### Languages
The BCP-47 code for the dataset's language is Filipino.
Dataset Structure
-----------------
### Data Instances
A sample from this dataset looks as follows:
### Dataset Fields
The dataset has the following fields (also called "features"):
### Dataset Splits
This dataset is split into a train and validation split. The split sizes are as follow:
| [
"### Languages\n\n\nThe BCP-47 code for the dataset's language is Filipino.\n\n\nDataset Structure\n-----------------",
"### Data Instances\n\n\nA sample from this dataset looks as follows:",
"### Dataset Fields\n\n\nThe dataset has the following fields (also called \"features\"):",
"### Dataset Splits\n\n\nThis dataset is split into a train and validation split. The split sizes are as follow:"
] | [
"TAGS\n#doi-10.57967/hf/0202 #region-us \n",
"### Languages\n\n\nThe BCP-47 code for the dataset's language is Filipino.\n\n\nDataset Structure\n-----------------",
"### Data Instances\n\n\nA sample from this dataset looks as follows:",
"### Dataset Fields\n\n\nThe dataset has the following fields (also called \"features\"):",
"### Dataset Splits\n\n\nThis dataset is split into a train and validation split. The split sizes are as follow:"
] | [
18,
26,
17,
23,
27
] | [
"passage: TAGS\n#doi-10.57967/hf/0202 #region-us \n### Languages\n\n\nThe BCP-47 code for the dataset's language is Filipino.\n\n\nDataset Structure\n-----------------### Data Instances\n\n\nA sample from this dataset looks as follows:### Dataset Fields\n\n\nThe dataset has the following fields (also called \"features\"):### Dataset Splits\n\n\nThis dataset is split into a train and validation split. The split sizes are as follow:"
] |
c039a9229f045a740a5fb0f4bef8eb5b1814c435 | # _RiddleSense_ ++: Evaluating LLMs' Riddling abilities
Some cleaning and other modifications to make [the riddle_sense dataset](https://huggingface.co/datasets/riddle_sense) more suitable for training language models to generate riddles via standard `text generation`
## Notable changes
- reformatting to use special tags indicating the question and answer components
- normalization: whitespace normalization, etc with `clean-text`
- **spell correction** the original dataset has numerous spelling errors; these are fixed using `BertChecker` from the `neuspell` library **for the questions only**. The answers were left as-is as there is some specific terminology/puns that get changed by SC.
## Notes & Gotchas
- the original dataset has a non-commercial clause, so this does too.
| pszemraj/riddlesense_plusplus | [
"annotations_creators:crowdsourced",
"language_creators:found",
"multilinguality:monolingual",
"size_categories:1K<n<10K",
"source_datasets:riddle_sense",
"language:en",
"license:other",
"riddles",
"riddler",
"non-commercial",
"doi:10.57967/hf/0133",
"region:us"
] | 2022-11-24T07:26:31+00:00 | {"annotations_creators": ["crowdsourced"], "language_creators": ["found"], "language": ["en"], "license": ["other"], "multilinguality": ["monolingual"], "size_categories": ["1K<n<10K"], "source_datasets": ["riddle_sense"], "pretty_name": "RiddleSense", "tags": ["riddles", "riddler", "non-commercial"]} | 2022-11-24T20:00:36+00:00 | [] | [
"en"
] | TAGS
#annotations_creators-crowdsourced #language_creators-found #multilinguality-monolingual #size_categories-1K<n<10K #source_datasets-riddle_sense #language-English #license-other #riddles #riddler #non-commercial #doi-10.57967/hf/0133 #region-us
| # _RiddleSense_ ++: Evaluating LLMs' Riddling abilities
Some cleaning and other modifications to make the riddle_sense dataset more suitable for training language models to generate riddles via standard 'text generation'
## Notable changes
- reformatting to use special tags indicating the question and answer components
- normalization: whitespace normalization, etc with 'clean-text'
- spell correction the original dataset has numerous spelling errors; these are fixed using 'BertChecker' from the 'neuspell' library for the questions only. The answers were left as-is as there is some specific terminology/puns that get changed by SC.
## Notes & Gotchas
- the original dataset has a non-commercial clause, so this does too.
| [
"# _RiddleSense_ ++: Evaluating LLMs' Riddling abilities\n\nSome cleaning and other modifications to make the riddle_sense dataset more suitable for training language models to generate riddles via standard 'text generation'",
"## Notable changes\n\n- reformatting to use special tags indicating the question and answer components\n- normalization: whitespace normalization, etc with 'clean-text'\n- spell correction the original dataset has numerous spelling errors; these are fixed using 'BertChecker' from the 'neuspell' library for the questions only. The answers were left as-is as there is some specific terminology/puns that get changed by SC.",
"## Notes & Gotchas\n\n- the original dataset has a non-commercial clause, so this does too."
] | [
"TAGS\n#annotations_creators-crowdsourced #language_creators-found #multilinguality-monolingual #size_categories-1K<n<10K #source_datasets-riddle_sense #language-English #license-other #riddles #riddler #non-commercial #doi-10.57967/hf/0133 #region-us \n",
"# _RiddleSense_ ++: Evaluating LLMs' Riddling abilities\n\nSome cleaning and other modifications to make the riddle_sense dataset more suitable for training language models to generate riddles via standard 'text generation'",
"## Notable changes\n\n- reformatting to use special tags indicating the question and answer components\n- normalization: whitespace normalization, etc with 'clean-text'\n- spell correction the original dataset has numerous spelling errors; these are fixed using 'BertChecker' from the 'neuspell' library for the questions only. The answers were left as-is as there is some specific terminology/puns that get changed by SC.",
"## Notes & Gotchas\n\n- the original dataset has a non-commercial clause, so this does too."
] | [
93,
54,
99,
26
] | [
"passage: TAGS\n#annotations_creators-crowdsourced #language_creators-found #multilinguality-monolingual #size_categories-1K<n<10K #source_datasets-riddle_sense #language-English #license-other #riddles #riddler #non-commercial #doi-10.57967/hf/0133 #region-us \n# _RiddleSense_ ++: Evaluating LLMs' Riddling abilities\n\nSome cleaning and other modifications to make the riddle_sense dataset more suitable for training language models to generate riddles via standard 'text generation'## Notable changes\n\n- reformatting to use special tags indicating the question and answer components\n- normalization: whitespace normalization, etc with 'clean-text'\n- spell correction the original dataset has numerous spelling errors; these are fixed using 'BertChecker' from the 'neuspell' library for the questions only. The answers were left as-is as there is some specific terminology/puns that get changed by SC.## Notes & Gotchas\n\n- the original dataset has a non-commercial clause, so this does too."
] |
Subsets and Splits
No community queries yet
The top public SQL queries from the community will appear here once available.