sha
stringlengths 40
40
| text
stringlengths 1
13.4M
| id
stringlengths 2
117
| tags
sequencelengths 1
7.91k
| created_at
stringlengths 25
25
| metadata
stringlengths 2
875k
| last_modified
stringlengths 25
25
| arxiv
sequencelengths 0
25
| languages
sequencelengths 0
7.91k
| tags_str
stringlengths 17
159k
| text_str
stringlengths 1
447k
| text_lists
sequencelengths 0
352
| processed_texts
sequencelengths 1
353
| tokens_length
sequencelengths 1
353
| input_texts
sequencelengths 1
40
|
---|---|---|---|---|---|---|---|---|---|---|---|---|---|---|
8950f534f8012eef317e1b90b2a8b13fbec8746d |
# Dataset Card for GAD
## Dataset Description
- **Homepage:** https://geneticassociationdb.nih.gov/
- **Pubmed:** True
- **Public:** True
- **Tasks:** TXTCLASS
A corpus identifying associations between genes and diseases by a semi-automatic
annotation procedure based on the Genetic Association Database.
## Note about homepage
The homepage for this dataset is no longer reachable, but the url is recorded here.
Data for this dataset was originally downloaded from a google drive
folder (the link used in the [BLURB benchmark data download script](https://microsoft.github.io/BLURB/submit.html).
However, we host the data in the huggingface hub for more reliable downloads and access.
## Citation Information
```
@article{Bravo2015,
doi = {10.1186/s12859-015-0472-9},
url = {https://doi.org/10.1186/s12859-015-0472-9},
year = {2015},
month = feb,
publisher = {Springer Science and Business Media {LLC}},
volume = {16},
number = {1},
author = {{\`{A}}lex Bravo and Janet Pi{\~{n}}ero and N{\'{u}}ria Queralt-Rosinach and Michael Rautschka and Laura I Furlong},
title = {Extraction of relations between genes and diseases from text and large-scale data analysis: implications for translational research},
journal = {{BMC} Bioinformatics}
}
```
| bigbio/gad | [
"multilinguality:momolingual",
"language:en",
"license:cc-by-4.0",
"region:us"
] | 2022-09-26T02:36:32+00:00 | {"language": ["en"], "license": "cc-by-4.0", "multilinguality": "momolingual", "paperswithcode_id": "gad", "pretty_name": "GAD", "bigbio_language": ["English"], "bigbio_license_shortname": "CC_BY_4p0", "homepage": "https://geneticassociationdb.nih.gov/", "bigbio_pubmed": true, "bigbio_public": true, "bigbio_tasks": ["TEXT_CLASSIFICATION"]} | 2022-12-22T15:25:28+00:00 | [] | [
"en"
] | TAGS
#multilinguality-momolingual #language-English #license-cc-by-4.0 #region-us
|
# Dataset Card for GAD
## Dataset Description
- Homepage: URL
- Pubmed: True
- Public: True
- Tasks: TXTCLASS
A corpus identifying associations between genes and diseases by a semi-automatic
annotation procedure based on the Genetic Association Database.
## Note about homepage
The homepage for this dataset is no longer reachable, but the url is recorded here.
Data for this dataset was originally downloaded from a google drive
folder (the link used in the BLURB benchmark data download script.
However, we host the data in the huggingface hub for more reliable downloads and access.
| [
"# Dataset Card for GAD",
"## Dataset Description\n\n- Homepage: URL\n- Pubmed: True\n- Public: True\n- Tasks: TXTCLASS\n\n\nA corpus identifying associations between genes and diseases by a semi-automatic\nannotation procedure based on the Genetic Association Database.",
"## Note about homepage\n\nThe homepage for this dataset is no longer reachable, but the url is recorded here.\nData for this dataset was originally downloaded from a google drive\nfolder (the link used in the BLURB benchmark data download script.\nHowever, we host the data in the huggingface hub for more reliable downloads and access."
] | [
"TAGS\n#multilinguality-momolingual #language-English #license-cc-by-4.0 #region-us \n",
"# Dataset Card for GAD",
"## Dataset Description\n\n- Homepage: URL\n- Pubmed: True\n- Public: True\n- Tasks: TXTCLASS\n\n\nA corpus identifying associations between genes and diseases by a semi-automatic\nannotation procedure based on the Genetic Association Database.",
"## Note about homepage\n\nThe homepage for this dataset is no longer reachable, but the url is recorded here.\nData for this dataset was originally downloaded from a google drive\nfolder (the link used in the BLURB benchmark data download script.\nHowever, we host the data in the huggingface hub for more reliable downloads and access."
] | [
27,
7,
53,
76
] | [
"passage: TAGS\n#multilinguality-momolingual #language-English #license-cc-by-4.0 #region-us \n# Dataset Card for GAD## Dataset Description\n\n- Homepage: URL\n- Pubmed: True\n- Public: True\n- Tasks: TXTCLASS\n\n\nA corpus identifying associations between genes and diseases by a semi-automatic\nannotation procedure based on the Genetic Association Database.## Note about homepage\n\nThe homepage for this dataset is no longer reachable, but the url is recorded here.\nData for this dataset was originally downloaded from a google drive\nfolder (the link used in the BLURB benchmark data download script.\nHowever, we host the data in the huggingface hub for more reliable downloads and access."
] |
e0ca639ce1a5f1267ada3f8fae2fdad79737887c |
# Dataset Card for BioASQ Task B
## Dataset Description
- **Homepage:** http://participants-area.bioasq.org/datasets/
- **Pubmed:** True
- **Public:** False
- **Tasks:** QA
The BioASQ corpus contains multiple question
answering tasks annotated by biomedical experts, including yes/no, factoid, list,
and summary questions. Pertaining to our objective of comparing neural language
models, we focus on the the yes/no questions (Task 7b), and leave the inclusion
of other tasks to future work. Each question is paired with a reference text
containing multiple sentences from a PubMed abstract and a yes/no answer. We use
the official train/dev/test split of 670/75/140 questions.
See 'Domain-Specific Language Model Pretraining for Biomedical
Natural Language Processing'
## Citation Information
```
@article{tsatsaronis2015overview,
title = {
An overview of the BIOASQ large-scale biomedical semantic indexing and
question answering competition
},
author = {
Tsatsaronis, George and Balikas, Georgios and Malakasiotis, Prodromos
and Partalas, Ioannis and Zschunke, Matthias and Alvers, Michael R and
Weissenborn, Dirk and Krithara, Anastasia and Petridis, Sergios and
Polychronopoulos, Dimitris and others
},
year = 2015,
journal = {BMC bioinformatics},
publisher = {BioMed Central Ltd},
volume = 16,
number = 1,
pages = 138
}
```
| bigbio/bioasq_task_b | [
"multilinguality:monolingual",
"language:en",
"license:other",
"region:us"
] | 2022-09-26T03:05:28+00:00 | {"language": ["en"], "license": "other", "multilinguality": "monolingual", "pretty_name": "BioASQ Task B", "bigbio_language": ["English"], "bigbio_license_shortname": "NLM_LICENSE", "homepage": "http://participants-area.bioasq.org/datasets/", "bigbio_pubmed": true, "bigbio_public": false, "bigbio_tasks": ["QUESTION_ANSWERING"]} | 2022-12-22T15:41:12+00:00 | [] | [
"en"
] | TAGS
#multilinguality-monolingual #language-English #license-other #region-us
|
# Dataset Card for BioASQ Task B
## Dataset Description
- Homepage: URL
- Pubmed: True
- Public: False
- Tasks: QA
The BioASQ corpus contains multiple question
answering tasks annotated by biomedical experts, including yes/no, factoid, list,
and summary questions. Pertaining to our objective of comparing neural language
models, we focus on the the yes/no questions (Task 7b), and leave the inclusion
of other tasks to future work. Each question is paired with a reference text
containing multiple sentences from a PubMed abstract and a yes/no answer. We use
the official train/dev/test split of 670/75/140 questions.
See 'Domain-Specific Language Model Pretraining for Biomedical
Natural Language Processing'
| [
"# Dataset Card for BioASQ Task B",
"## Dataset Description\n\n- Homepage: URL\n- Pubmed: True\n- Public: False\n- Tasks: QA\n\n\nThe BioASQ corpus contains multiple question\nanswering tasks annotated by biomedical experts, including yes/no, factoid, list,\nand summary questions. Pertaining to our objective of comparing neural language\nmodels, we focus on the the yes/no questions (Task 7b), and leave the inclusion\nof other tasks to future work. Each question is paired with a reference text\ncontaining multiple sentences from a PubMed abstract and a yes/no answer. We use\nthe official train/dev/test split of 670/75/140 questions.\n\nSee 'Domain-Specific Language Model Pretraining for Biomedical\nNatural Language Processing'"
] | [
"TAGS\n#multilinguality-monolingual #language-English #license-other #region-us \n",
"# Dataset Card for BioASQ Task B",
"## Dataset Description\n\n- Homepage: URL\n- Pubmed: True\n- Public: False\n- Tasks: QA\n\n\nThe BioASQ corpus contains multiple question\nanswering tasks annotated by biomedical experts, including yes/no, factoid, list,\nand summary questions. Pertaining to our objective of comparing neural language\nmodels, we focus on the the yes/no questions (Task 7b), and leave the inclusion\nof other tasks to future work. Each question is paired with a reference text\ncontaining multiple sentences from a PubMed abstract and a yes/no answer. We use\nthe official train/dev/test split of 670/75/140 questions.\n\nSee 'Domain-Specific Language Model Pretraining for Biomedical\nNatural Language Processing'"
] | [
23,
11,
168
] | [
"passage: TAGS\n#multilinguality-monolingual #language-English #license-other #region-us \n# Dataset Card for BioASQ Task B## Dataset Description\n\n- Homepage: URL\n- Pubmed: True\n- Public: False\n- Tasks: QA\n\n\nThe BioASQ corpus contains multiple question\nanswering tasks annotated by biomedical experts, including yes/no, factoid, list,\nand summary questions. Pertaining to our objective of comparing neural language\nmodels, we focus on the the yes/no questions (Task 7b), and leave the inclusion\nof other tasks to future work. Each question is paired with a reference text\ncontaining multiple sentences from a PubMed abstract and a yes/no answer. We use\nthe official train/dev/test split of 670/75/140 questions.\n\nSee 'Domain-Specific Language Model Pretraining for Biomedical\nNatural Language Processing'"
] |
7f2fb24be7c82a385ee81a1152bc679b6400f41b |  | VirtualJesus/Anthonyface | [
"region:us"
] | 2022-09-26T04:01:42+00:00 | {} | 2022-09-26T07:48:44+00:00 | [] | [] | TAGS
#region-us
| !IMG_20220926_000035_Bokeh.jpg | [] | [
"TAGS\n#region-us \n"
] | [
6
] | [
"passage: TAGS\n#region-us \n"
] |
2be31cb9f5880cbce04b5b68299121992587ace7 | # Dataset Card for AutoTrain Evaluator
This repository contains model predictions generated by [AutoTrain](https://huggingface.co/autotrain) for the following task and dataset:
* Task: Summarization
* Model: Samuel-Fipps/t5-efficient-large-nl36_fine_tune_sum_V2
* Dataset: samsum
* Config: samsum
* Split: test
To run new evaluation jobs, visit Hugging Face's [automatic model evaluator](https://huggingface.co/spaces/autoevaluate/model-evaluator).
## Contributions
Thanks to [@samuel-fipps](https://huggingface.co/samuel-fipps) for evaluating this model. | autoevaluate/autoeval-eval-samsum-samsum-8a4c42-1554855493 | [
"autotrain",
"evaluation",
"region:us"
] | 2022-09-26T05:54:54+00:00 | {"type": "predictions", "tags": ["autotrain", "evaluation"], "datasets": ["samsum"], "eval_info": {"task": "summarization", "model": "Samuel-Fipps/t5-efficient-large-nl36_fine_tune_sum_V2", "metrics": ["mse"], "dataset_name": "samsum", "dataset_config": "samsum", "dataset_split": "test", "col_mapping": {"text": "dialogue", "target": "summary"}}} | 2022-09-26T06:02:52+00:00 | [] | [] | TAGS
#autotrain #evaluation #region-us
| # Dataset Card for AutoTrain Evaluator
This repository contains model predictions generated by AutoTrain for the following task and dataset:
* Task: Summarization
* Model: Samuel-Fipps/t5-efficient-large-nl36_fine_tune_sum_V2
* Dataset: samsum
* Config: samsum
* Split: test
To run new evaluation jobs, visit Hugging Face's automatic model evaluator.
## Contributions
Thanks to @samuel-fipps for evaluating this model. | [
"# Dataset Card for AutoTrain Evaluator\n\nThis repository contains model predictions generated by AutoTrain for the following task and dataset:\n\n* Task: Summarization\n* Model: Samuel-Fipps/t5-efficient-large-nl36_fine_tune_sum_V2\n* Dataset: samsum\n* Config: samsum\n* Split: test\n\nTo run new evaluation jobs, visit Hugging Face's automatic model evaluator.",
"## Contributions\n\nThanks to @samuel-fipps for evaluating this model."
] | [
"TAGS\n#autotrain #evaluation #region-us \n",
"# Dataset Card for AutoTrain Evaluator\n\nThis repository contains model predictions generated by AutoTrain for the following task and dataset:\n\n* Task: Summarization\n* Model: Samuel-Fipps/t5-efficient-large-nl36_fine_tune_sum_V2\n* Dataset: samsum\n* Config: samsum\n* Split: test\n\nTo run new evaluation jobs, visit Hugging Face's automatic model evaluator.",
"## Contributions\n\nThanks to @samuel-fipps for evaluating this model."
] | [
13,
102,
19
] | [
"passage: TAGS\n#autotrain #evaluation #region-us \n# Dataset Card for AutoTrain Evaluator\n\nThis repository contains model predictions generated by AutoTrain for the following task and dataset:\n\n* Task: Summarization\n* Model: Samuel-Fipps/t5-efficient-large-nl36_fine_tune_sum_V2\n* Dataset: samsum\n* Config: samsum\n* Split: test\n\nTo run new evaluation jobs, visit Hugging Face's automatic model evaluator.## Contributions\n\nThanks to @samuel-fipps for evaluating this model."
] |
a35672081af08bf55b7cdcdd8f2864edcb50a2ff | train data | BraimComplexe/train_1 | [
"region:us"
] | 2022-09-26T08:02:31+00:00 | {} | 2022-09-26T08:13:22+00:00 | [] | [] | TAGS
#region-us
| train data | [] | [
"TAGS\n#region-us \n"
] | [
6
] | [
"passage: TAGS\n#region-us \n"
] |
911e1d214162fd11d2c78d3f1428cbfcbe07782c |
# Dataset Card for MultiLegalPile: A Large-Scale Multilingual Corpus for the Legal Domain
## Table of Contents
- [Table of Contents](#table-of-contents)
- [Dataset Description](#dataset-description)
- [Dataset Summary](#dataset-summary)
- [Supported Tasks and Leaderboards](#supported-tasks-and-leaderboards)
- [Languages](#languages)
- [Dataset Structure](#dataset-structure)
- [Data Instances](#data-instances)
- [Data Fields](#data-fields)
- [Data Splits](#data-splits)
- [Dataset Creation](#dataset-creation)
- [Curation Rationale](#curation-rationale)
- [Source Data](#source-data)
- [Annotations](#annotations)
- [Personal and Sensitive Information](#personal-and-sensitive-information)
- [Considerations for Using the Data](#considerations-for-using-the-data)
- [Social Impact of Dataset](#social-impact-of-dataset)
- [Discussion of Biases](#discussion-of-biases)
- [Other Known Limitations](#other-known-limitations)
- [Additional Information](#additional-information)
- [Dataset Curators](#dataset-curators)
- [Licensing Information](#licensing-information)
- [Citation Information](#citation-information)
- [Contributions](#contributions)
## Dataset Description
- **Homepage:**
- **Repository:**
- **Paper:** [MultiLegalPile](https://arxiv.org/abs/2306.02069)
- **Leaderboard:**
- **Point of Contact:** [Joel Niklaus](mailto:[email protected])
### Dataset Summary
The Multi_Legal_Pile is a large-scale multilingual legal dataset suited for pretraining language models.
It spans over 24 languages and five legal text types.
### Supported Tasks and Leaderboards
The dataset supports the tasks of fill-mask.
### Languages
The following languages are supported: bg, cs, da, de, el, en, es, et, fi, fr, ga, hr, hu, it, lt, lv, mt, nl, pl, pt,
ro, sk, sl, sv
## Dataset Structure
It is structured in the following format:
type -> language -> jurisdiction.jsonl.xz
type is one of the following:
- caselaw
- contracts
- legislation
- other
- legal_mc4
`legal_mc4` is a subset of the other type but is listed separately so it can be easily excluded since it is less
permissively licensed than the other types.
Use the dataset like this:
```python
from datasets import load_dataset
config = 'en_contracts' # {language}_{type}
dataset = load_dataset('joelniklaus/Multi_Legal_Pile', config, split='train', streaming=True)
```
'config' is a combination of language and text_type, e.g. 'en_contracts' or 'de_caselaw'.
To load all the languages or all the text_types, use 'all' instead of the language or text_type (e.g., '
all_legislation').
### Data Instances
The file format is jsonl.xz and there is one split available ("train").
The complete dataset (689GB) consists of four large subsets:
- Native Multi Legal Pile (112GB)
- Eurlex Resources (179GB)
- Legal MC4 (106GB)
- Pile of Law (292GB)
#### Native Multilingual Legal Pile data
| | Language | Text Type | Jurisdiction | Source | Size (MB) | Words | Documents | Words/Document | URL | License |
|---:|:-----------|:------------|:---------------|:-----------------------------------|------------:|------------:|------------:|-----------------:|:-----------------------------------------------------------------------------------------------------------------------------------------------------|:----------------------------------------------------------------------------------------------------|
| 0 | bg | legislation | Bulgaria | MARCELL | 8015 | 308946116 | 82777 | 3732 | https://elrc-share.eu/repository/browse/marcell-bulgarian-legislative-subcorpus-v2/946267fe8d8711eb9c1a00155d026706d2c9267e5cdf4d75b5f02168f01906c6/ | [CC0-1.0](https://elrc-share.eu/static/metashare/licences/CC0-1.0.pdf) |
| 1 | cs | caselaw | Czechia | CzCDC Constitutional Court | 11151 | 574336489 | 296652 | 1936 | https://lindat.mff.cuni.cz/repository/xmlui/handle/11372/LRT-3052 | [CC BY-NC 4.0](https://creativecommons.org/licenses/by-nc/4.0/) |
| 2 | cs | caselaw | Czechia | CzCDC Supreme Administrative Court | 11151 | 574336489 | 296652 | 1936 | https://lindat.mff.cuni.cz/repository/xmlui/handle/11372/LRT-3052 | [CC BY-NC 4.0](https://creativecommons.org/licenses/by-nc/4.0/) |
| 3 | cs | caselaw | Czechia | CzCDC Supreme Court | 11151 | 574336489 | 296652 | 1936 | https://lindat.mff.cuni.cz/repository/xmlui/handle/11372/LRT-3052 | [CC BY-NC 4.0](https://creativecommons.org/licenses/by-nc/4.0/) |
| 4 | da | caselaw | Denmark | DDSC | 3469 | 210730560 | 89702 | 2349 | https://huggingface.co/DDSC | [CC BY 4.0 and other, depending on the dataset](https://creativecommons.org/licenses/by-nc/4.0/) |
| 5 | da | legislation | Denmark | DDSC | 10736 | 653153146 | 265868 | 2456 | https://huggingface.co/DDSC | [CC BY 4.0 and other, depending on the dataset](https://creativecommons.org/licenses/by-nc/4.0/) |
| 6 | de | caselaw | Germany | openlegaldata | 31527 | 1785439383 | 596800 | 2991 | https://de.openlegaldata.io/ | [ODbL-1.0](https://opendatacommons.org/licenses/odbl/1-0/) |
| 7 | de | caselaw | Switzerland | entscheidsuche | 31527 | 1785439383 | 596800 | 2991 | https://entscheidsuche.ch/ | [See description](https://entscheidsuche.ch/dataUsage) |
| 8 | de | legislation | Germany | openlegaldata | 8934 | 512840663 | 276034 | 1857 | https://de.openlegaldata.io/ | [ODbL-1.0](https://opendatacommons.org/licenses/odbl/1-0/) |
| 9 | de | legislation | Switzerland | lexfind | 8934 | 512840663 | 276034 | 1857 | https://www.lexfind.ch/fe/de/search | No information provided |
| 10 | fr | caselaw | Switzerland | entscheidsuche | 18313 | 1170335690 | 435569 | 2686 | https://entscheidsuche.ch/ | [See description](https://entscheidsuche.ch/dataUsage) |
| 11 | fr | caselaw | Belgium | jurportal | 18313 | 1170335690 | 435569 | 2686 | https://juportal.be/home/welkom | [See description](https://juportal.be/home/disclaimer) |
| 12 | fr | caselaw | France | CASS | 18313 | 1170335690 | 435569 | 2686 | https://echanges.dila.gouv.fr/OPENDATA/CASS/ | [Open Licence 2.0](https://echanges.dila.gouv.fr/OPENDATA/CASS/DILA_CASS_Presentation_20170824.pdf) |
| 13 | fr | caselaw | Luxembourg | judoc | 18313 | 1170335690 | 435569 | 2686 | https://justice.public.lu/fr.html | [See description](https://justice.public.lu/fr/support/aspects-legaux/conditions-generales.html) |
| 14 | it | caselaw | Switzerland | entscheidsuche | 6483 | 406520336 | 156630 | 2595 | https://entscheidsuche.ch/ | [See description](https://entscheidsuche.ch/dataUsage) |
| 15 | en | legislation | Switzerland | lexfind | 36587 | 2537696894 | 657805 | 3857 | https://www.lexfind.ch/fe/de/search | No information provided |
| 16 | en | legislation | UK | uk-lex | 36587 | 2537696894 | 657805 | 3857 | https://zenodo.org/record/6355465 | [CC BY 4.0](https://creativecommons.org/licenses/by/4.0/legalcode) |
| 17 | fr | legislation | Switzerland | lexfind | 9297 | 600170792 | 243313 | 2466 | https://www.lexfind.ch/fe/fr/search | No information provided |
| 18 | fr | legislation | Belgium | ejustice | 9297 | 600170792 | 243313 | 2466 | https://www.ejustice.just.fgov.be/cgi/welcome.pl | No information provided |
| 19 | it | legislation | Switzerland | lexfind | 8332 | 542579039 | 227968 | 2380 | https://www.lexfind.ch/fe/it/search | No information provided |
| 20 | nl | legislation | Belgium | ejustice | 8484 | 550788527 | 232204 | 2372 | https://www.ejustice.just.fgov.be/cgi/welcome.pl | No information provided |
| 21 | hu | legislation | Hungary | MARCELL | 5744 | 264572303 | 86862 | 3045 | https://elrc-share.eu/repository/browse/marcell-hungarian-legislative-subcorpus-v2/a87295ec8d6511eb9c1a00155d0267065f7e56dc7db34ce5aaae0b48a329daaa/ | [CC0-1.0](https://elrc-share.eu/static/metashare/licences/CC0-1.0.pdf) |
| 22 | pl | legislation | Poland | MARCELL | 5459 | 299334705 | 89264 | 3353 | https://elrc-share.eu/repository/browse/marcell-polish-legislative-subcorpus-v2/dd14fa1c8d6811eb9c1a00155d026706c4718ddc9c6e4a92a88923816ca8b219/ | [CC0-1.0](https://elrc-share.eu/static/metashare/licences/CC0-1.0.pdf) |
| 23 | pt | caselaw | Brazil | RulingBR | 196919 | 12611760973 | 17251236 | 731 | https://github.com/diego-feijo/rulingbr | No information provided |
| 24 | pt | caselaw | Brazil | CRETA | 196919 | 12611760973 | 17251236 | 731 | https://www.kaggle.com/datasets/eliasjacob/brcad5?resource=download&select=language_modeling_texts.parquet | [CC BY-NC-SA 4.0](https://creativecommons.org/licenses/by-nc-sa/4.0/) |
| 25 | pt | caselaw | Brazil | CJPG | 196919 | 12611760973 | 17251236 | 731 | https://esaj.tjsp.jus.br/cjsg/consultaCompleta.do?f=1 | No information provided |
| 26 | ro | legislation | Romania | MARCELL | 10464 | 559092153 | 215694 | 2592 | https://elrc-share.eu/repository/browse/marcell-romanian-legislative-subcorpus-v2/2da548428b9d11eb9c1a00155d026706ce94a6b59ffc4b0e9fb5cd9cebe6889e/ | [CC0-1.0](https://elrc-share.eu/static/metashare/licences/CC0-1.0.pdf) |
| 27 | sk | legislation | Slovakia | MARCELL | 5208 | 280182047 | 76760 | 3650 | https://elrc-share.eu/repository/browse/marcell-slovak-legislative-subcorpus-v2/6bdee1d68c8311eb9c1a00155d0267063398d3f1a3af40e1b728468dcbd6efdd/ | [CC0-1.0](https://elrc-share.eu/static/metashare/licences/CC0-1.0.pdf) |
| 28 | sl | legislation | Slovenia | MARCELL | 6057 | 365513763 | 88651 | 4123 | https://elrc-share.eu/repository/browse/marcell-slovenian-legislative-subcorpus-v2/e2a779868d4611eb9c1a00155d026706983c845a30d741b78e051faf91828b0d/ | [CC-BY-4.0](https://elrc-share.eu/static/metashare/licences/CC-BY-4.0.pdf)
| total | all | all | all | 1297609 | xxx | 81214262514 | 57305071 | 1417 | |
#### Eurlex Resources
See [Eurlex Resources](https://huggingface.co/datasets/joelito/eurlex_resources#data-instances) for more information.
#### Legal-MC4
See [Legal-MC4](https://huggingface.co/datasets/joelito/legal-mc4#data-instances) for more information.
#### Pile-of-Law
See [Pile-of-Law](https://huggingface.co/datasets/pile-of-law/pile-of-law#data-instances) for more information.
| Language | Type | Jurisdiction | Source | Size (MB) | Tokens | Documents | Tokens/Document | Part of Multi_Legal_Pile |
|:-----------|:------------|:---------------|:-------------------------------------|------------:|------------:|------------:|------------------:|:---------------------------|
| en | all | all | all | 503712 | 50547777921 | 9872444 | 5120 | yes |
| en | caselaw | EU | echr | 298 | 28374996 | 8480 | 3346 | yes |
| en | caselaw | Canada | canadian_decisions | 486 | 45438083 | 11343 | 4005 | yes |
| en | caselaw | US | dol_ecab | 942 | 99113541 | 28211 | 3513 | no |
| en | caselaw | US | scotus_oral_arguments | 1092 | 108228951 | 7996 | 13535 | no |
| en | caselaw | US | tax_rulings | 1704 | 166915887 | 54064 | 3087 | no |
| en | caselaw | US | nlrb_decisions | 2652 | 294471818 | 32080 | 9179 | no |
| en | caselaw | US | scotus_filings | 4018 | 593870413 | 63775 | 9311 | yes |
| en | caselaw | US | bva_opinions | 35238 | 4084140080 | 839523 | 4864 | no |
| en | caselaw | US | courtlistener_docket_entry_documents | 139006 | 12713614864 | 1983436 | 6409 | yes |
| en | caselaw | US | courtlistener_opinions | 158110 | 15899704961 | 4518445 | 3518 | yes |
| en | contracts | -- | tos | 4 | 391890 | 50 | 7837 | no |
| en | contracts | US | cfpb_creditcard_contracts | 188 | 25984824 | 2638 | 9850 | yes |
| en | contracts | US | edgar | 28698 | 2936402810 | 987926 | 2972 | yes |
| en | contracts | US | atticus_contracts | 78300 | 7997013703 | 650833 | 12287 | yes |
| en | legislation | US | fre | 2 | 173325 | 68 | 2548 | no |
| en | legislation | US | frcp | 4 | 427614 | 92 | 4647 | no |
| en | legislation | US | eoir | 62 | 6109737 | 2229 | 2741 | no |
| en | legislation | -- | constitutions | 66 | 5984865 | 187 | 32004 | yes |
| en | legislation | US | federal_register | 424 | 39854787 | 5414 | 7361 | yes |
| en | legislation | US | uscode | 716 | 78466325 | 58 | 1352867 | yes |
| en | legislation | EU | euro_parl | 808 | 71344326 | 9672 | 7376 | no |
| en | legislation | US | cfr | 1788 | 160849007 | 243 | 661930 | yes |
| en | legislation | US | us_bills | 3394 | 320723838 | 112483 | 2851 | yes |
| en | legislation | EU | eurlex | 3504 | 401324829 | 142036 | 2825 | no |
| en | legislation | US | state_codes | 18066 | 1858333235 | 217 | 8563747 | yes |
| en | other | -- | bar_exam_outlines | 4 | 346924 | 59 | 5880 | no |
| en | other | US | ftc_advisory_opinions | 4 | 509025 | 145 | 3510 | no |
| en | other | US | olc_memos | 98 | 12764635 | 1384 | 9223 | yes |
| en | other | -- | cc_casebooks | 258 | 24857378 | 73 | 340512 | no |
| en | other | -- | un_debates | 360 | 31152497 | 8481 | 3673 | no |
| en | other | -- | r_legaladvice | 798 | 72605386 | 146671 | 495 | no |
| en | other | US | founding_docs | 1118 | 100390231 | 183664 | 546 | no |
| en | other | US | oig | 5056 | 566782244 | 38954 | 14550 | yes |
| en | other | US | congressional_hearings | 16448 | 1801110892 | 31514 | 57152 | no |
### Data Fields
[More Information Needed]
### Data Splits
[More Information Needed]
## Dataset Creation
### Curation Rationale
[More Information Needed]
### Source Data
#### Initial Data Collection and Normalization
[More Information Needed]
#### Who are the source language producers?
[More Information Needed]
### Annotations
#### Annotation process
[More Information Needed]
#### Who are the annotators?
[More Information Needed]
### Personal and Sensitive Information
[More Information Needed]
## Considerations for Using the Data
### Social Impact of Dataset
[More Information Needed]
### Discussion of Biases
[More Information Needed]
### Other Known Limitations
[More Information Needed]
## Additional Information
### Dataset Curators
[More Information Needed]
### Licensing Information
[More Information Needed]
### Citation Information
```
@misc{niklaus2023multilegalpile,
title={MultiLegalPile: A 689GB Multilingual Legal Corpus},
author={Joel Niklaus and Veton Matoshi and Matthias Stรผrmer and Ilias Chalkidis and Daniel E. Ho},
year={2023},
eprint={2306.02069},
archivePrefix={arXiv},
primaryClass={cs.CL}
}
```
### Contributions
Thanks to [@JoelNiklaus](https://github.com/joelniklaus) for adding this dataset.
| joelniklaus/Multi_Legal_Pile | [
"task_categories:fill-mask",
"annotations_creators:other",
"language_creators:found",
"multilinguality:multilingual",
"size_categories:10M<n<100M",
"source_datasets:original",
"language:bg",
"language:cs",
"language:da",
"language:de",
"language:el",
"language:en",
"language:es",
"language:et",
"language:fi",
"language:fr",
"language:ga",
"language:hr",
"language:hu",
"language:it",
"language:lt",
"language:lv",
"language:mt",
"language:nl",
"language:pl",
"language:pt",
"language:ro",
"language:sk",
"language:sl",
"language:sv",
"license:cc-by-nc-sa-4.0",
"arxiv:2306.02069",
"region:us"
] | 2022-09-26T09:28:06+00:00 | {"annotations_creators": ["other"], "language_creators": ["found"], "language": ["bg", "cs", "da", "de", "el", "en", "es", "et", "fi", "fr", "ga", "hr", "hu", "it", "lt", "lv", "mt", "nl", "pl", "pt", "ro", "sk", "sl", "sv"], "license": ["cc-by-nc-sa-4.0"], "multilinguality": ["multilingual"], "size_categories": ["10M<n<100M"], "source_datasets": ["original"], "task_categories": ["fill-mask"], "pretty_name": "MultiLegalPile: A Large-Scale Multilingual Corpus for the Legal Domain"} | 2024-01-12T08:50:24+00:00 | [
"2306.02069"
] | [
"bg",
"cs",
"da",
"de",
"el",
"en",
"es",
"et",
"fi",
"fr",
"ga",
"hr",
"hu",
"it",
"lt",
"lv",
"mt",
"nl",
"pl",
"pt",
"ro",
"sk",
"sl",
"sv"
] | TAGS
#task_categories-fill-mask #annotations_creators-other #language_creators-found #multilinguality-multilingual #size_categories-10M<n<100M #source_datasets-original #language-Bulgarian #language-Czech #language-Danish #language-German #language-Modern Greek (1453-) #language-English #language-Spanish #language-Estonian #language-Finnish #language-French #language-Irish #language-Croatian #language-Hungarian #language-Italian #language-Lithuanian #language-Latvian #language-Maltese #language-Dutch #language-Polish #language-Portuguese #language-Romanian #language-Slovak #language-Slovenian #language-Swedish #license-cc-by-nc-sa-4.0 #arxiv-2306.02069 #region-us
| Dataset Card for MultiLegalPile: A Large-Scale Multilingual Corpus for the Legal Domain
=======================================================================================
Table of Contents
-----------------
* Table of Contents
* Dataset Description
+ Dataset Summary
+ Supported Tasks and Leaderboards
+ Languages
* Dataset Structure
+ Data Instances
+ Data Fields
+ Data Splits
* Dataset Creation
+ Curation Rationale
+ Source Data
+ Annotations
+ Personal and Sensitive Information
* Considerations for Using the Data
+ Social Impact of Dataset
+ Discussion of Biases
+ Other Known Limitations
* Additional Information
+ Dataset Curators
+ Licensing Information
+ Citation Information
+ Contributions
Dataset Description
-------------------
* Homepage:
* Repository:
* Paper: MultiLegalPile
* Leaderboard:
* Point of Contact: Joel Niklaus
### Dataset Summary
The Multi\_Legal\_Pile is a large-scale multilingual legal dataset suited for pretraining language models.
It spans over 24 languages and five legal text types.
### Supported Tasks and Leaderboards
The dataset supports the tasks of fill-mask.
### Languages
The following languages are supported: bg, cs, da, de, el, en, es, et, fi, fr, ga, hr, hu, it, lt, lv, mt, nl, pl, pt,
ro, sk, sl, sv
Dataset Structure
-----------------
It is structured in the following format:
type -> language -> URL
type is one of the following:
* caselaw
* contracts
* legislation
* other
* legal\_mc4
'legal\_mc4' is a subset of the other type but is listed separately so it can be easily excluded since it is less
permissively licensed than the other types.
Use the dataset like this:
'config' is a combination of language and text\_type, e.g. 'en\_contracts' or 'de\_caselaw'.
To load all the languages or all the text\_types, use 'all' instead of the language or text\_type (e.g., '
all\_legislation').
### Data Instances
The file format is URL and there is one split available ("train").
The complete dataset (689GB) consists of four large subsets:
* Native Multi Legal Pile (112GB)
* Eurlex Resources (179GB)
* Legal MC4 (106GB)
* Pile of Law (292GB)
#### Native Multilingual Legal Pile data
#### Eurlex Resources
See Eurlex Resources for more information.
#### Legal-MC4
See Legal-MC4 for more information.
#### Pile-of-Law
See Pile-of-Law for more information.
### Data Fields
### Data Splits
Dataset Creation
----------------
### Curation Rationale
### Source Data
#### Initial Data Collection and Normalization
#### Who are the source language producers?
### Annotations
#### Annotation process
#### Who are the annotators?
### Personal and Sensitive Information
Considerations for Using the Data
---------------------------------
### Social Impact of Dataset
### Discussion of Biases
### Other Known Limitations
Additional Information
----------------------
### Dataset Curators
### Licensing Information
### Contributions
Thanks to @JoelNiklaus for adding this dataset.
| [
"### Dataset Summary\n\n\nThe Multi\\_Legal\\_Pile is a large-scale multilingual legal dataset suited for pretraining language models.\nIt spans over 24 languages and five legal text types.",
"### Supported Tasks and Leaderboards\n\n\nThe dataset supports the tasks of fill-mask.",
"### Languages\n\n\nThe following languages are supported: bg, cs, da, de, el, en, es, et, fi, fr, ga, hr, hu, it, lt, lv, mt, nl, pl, pt,\nro, sk, sl, sv\n\n\nDataset Structure\n-----------------\n\n\nIt is structured in the following format:\ntype -> language -> URL\n\n\ntype is one of the following:\n\n\n* caselaw\n* contracts\n* legislation\n* other\n* legal\\_mc4\n\n\n'legal\\_mc4' is a subset of the other type but is listed separately so it can be easily excluded since it is less\npermissively licensed than the other types.\n\n\nUse the dataset like this:\n\n\n'config' is a combination of language and text\\_type, e.g. 'en\\_contracts' or 'de\\_caselaw'.\nTo load all the languages or all the text\\_types, use 'all' instead of the language or text\\_type (e.g., '\nall\\_legislation').",
"### Data Instances\n\n\nThe file format is URL and there is one split available (\"train\").\n\n\nThe complete dataset (689GB) consists of four large subsets:\n\n\n* Native Multi Legal Pile (112GB)\n* Eurlex Resources (179GB)\n* Legal MC4 (106GB)\n* Pile of Law (292GB)",
"#### Native Multilingual Legal Pile data",
"#### Eurlex Resources\n\n\nSee Eurlex Resources for more information.",
"#### Legal-MC4\n\n\nSee Legal-MC4 for more information.",
"#### Pile-of-Law\n\n\nSee Pile-of-Law for more information.",
"### Data Fields",
"### Data Splits\n\n\nDataset Creation\n----------------",
"### Curation Rationale",
"### Source Data",
"#### Initial Data Collection and Normalization",
"#### Who are the source language producers?",
"### Annotations",
"#### Annotation process",
"#### Who are the annotators?",
"### Personal and Sensitive Information\n\n\nConsiderations for Using the Data\n---------------------------------",
"### Social Impact of Dataset",
"### Discussion of Biases",
"### Other Known Limitations\n\n\nAdditional Information\n----------------------",
"### Dataset Curators",
"### Licensing Information",
"### Contributions\n\n\nThanks to @JoelNiklaus for adding this dataset."
] | [
"TAGS\n#task_categories-fill-mask #annotations_creators-other #language_creators-found #multilinguality-multilingual #size_categories-10M<n<100M #source_datasets-original #language-Bulgarian #language-Czech #language-Danish #language-German #language-Modern Greek (1453-) #language-English #language-Spanish #language-Estonian #language-Finnish #language-French #language-Irish #language-Croatian #language-Hungarian #language-Italian #language-Lithuanian #language-Latvian #language-Maltese #language-Dutch #language-Polish #language-Portuguese #language-Romanian #language-Slovak #language-Slovenian #language-Swedish #license-cc-by-nc-sa-4.0 #arxiv-2306.02069 #region-us \n",
"### Dataset Summary\n\n\nThe Multi\\_Legal\\_Pile is a large-scale multilingual legal dataset suited for pretraining language models.\nIt spans over 24 languages and five legal text types.",
"### Supported Tasks and Leaderboards\n\n\nThe dataset supports the tasks of fill-mask.",
"### Languages\n\n\nThe following languages are supported: bg, cs, da, de, el, en, es, et, fi, fr, ga, hr, hu, it, lt, lv, mt, nl, pl, pt,\nro, sk, sl, sv\n\n\nDataset Structure\n-----------------\n\n\nIt is structured in the following format:\ntype -> language -> URL\n\n\ntype is one of the following:\n\n\n* caselaw\n* contracts\n* legislation\n* other\n* legal\\_mc4\n\n\n'legal\\_mc4' is a subset of the other type but is listed separately so it can be easily excluded since it is less\npermissively licensed than the other types.\n\n\nUse the dataset like this:\n\n\n'config' is a combination of language and text\\_type, e.g. 'en\\_contracts' or 'de\\_caselaw'.\nTo load all the languages or all the text\\_types, use 'all' instead of the language or text\\_type (e.g., '\nall\\_legislation').",
"### Data Instances\n\n\nThe file format is URL and there is one split available (\"train\").\n\n\nThe complete dataset (689GB) consists of four large subsets:\n\n\n* Native Multi Legal Pile (112GB)\n* Eurlex Resources (179GB)\n* Legal MC4 (106GB)\n* Pile of Law (292GB)",
"#### Native Multilingual Legal Pile data",
"#### Eurlex Resources\n\n\nSee Eurlex Resources for more information.",
"#### Legal-MC4\n\n\nSee Legal-MC4 for more information.",
"#### Pile-of-Law\n\n\nSee Pile-of-Law for more information.",
"### Data Fields",
"### Data Splits\n\n\nDataset Creation\n----------------",
"### Curation Rationale",
"### Source Data",
"#### Initial Data Collection and Normalization",
"#### Who are the source language producers?",
"### Annotations",
"#### Annotation process",
"#### Who are the annotators?",
"### Personal and Sensitive Information\n\n\nConsiderations for Using the Data\n---------------------------------",
"### Social Impact of Dataset",
"### Discussion of Biases",
"### Other Known Limitations\n\n\nAdditional Information\n----------------------",
"### Dataset Curators",
"### Licensing Information",
"### Contributions\n\n\nThanks to @JoelNiklaus for adding this dataset."
] | [
221,
49,
24,
236,
75,
11,
15,
15,
21,
5,
11,
7,
4,
10,
10,
5,
5,
9,
18,
7,
8,
14,
6,
6,
18
] | [
"passage: TAGS\n#task_categories-fill-mask #annotations_creators-other #language_creators-found #multilinguality-multilingual #size_categories-10M<n<100M #source_datasets-original #language-Bulgarian #language-Czech #language-Danish #language-German #language-Modern Greek (1453-) #language-English #language-Spanish #language-Estonian #language-Finnish #language-French #language-Irish #language-Croatian #language-Hungarian #language-Italian #language-Lithuanian #language-Latvian #language-Maltese #language-Dutch #language-Polish #language-Portuguese #language-Romanian #language-Slovak #language-Slovenian #language-Swedish #license-cc-by-nc-sa-4.0 #arxiv-2306.02069 #region-us \n### Dataset Summary\n\n\nThe Multi\\_Legal\\_Pile is a large-scale multilingual legal dataset suited for pretraining language models.\nIt spans over 24 languages and five legal text types.### Supported Tasks and Leaderboards\n\n\nThe dataset supports the tasks of fill-mask."
] |
db3f9a34f0c1c287db91e86861ca8bdff67f5935 |
# Download zenodo dataset files using huggingface datasets
You can download a specific file from the Zenodo dataset using the following code:
Zenodo id : 5172018
File name : FDB-17-fragmentset.smi.gz
```python
from datasets import load_dataset
load_dataset("osbm/zenodo", "5172018_FDB-17-fragmentset.smi.gz")
```
This command will also copy the file into your current directory so that you can use it directly.
Here is an example notebook: https://gist.github.com/osbm/35a499f5756df22de30be20463aa6331
# Contribution
[The huggingface repository](https://huggingface.co/datasets/osbm/zenodo) is actually a mirror of the github repository [osbm/zenodo](https://github.com/osbm/huggingface-zenodo-datasets). If you want to open an issue or PR, please do it on the github repository. I chose to do it this way because I wanted to use github actions. Currently only github action is mirroring the repository to huggingface. ๐
| osbm/zenodo | [
"region:us"
] | 2022-09-26T10:04:40+00:00 | {"pretty_name": "Download Zenodo Dataset files"} | 2023-06-12T10:36:45+00:00 | [] | [] | TAGS
#region-us
|
# Download zenodo dataset files using huggingface datasets
You can download a specific file from the Zenodo dataset using the following code:
Zenodo id : 5172018
File name : URL
This command will also copy the file into your current directory so that you can use it directly.
Here is an example notebook: URL
# Contribution
The huggingface repository is actually a mirror of the github repository osbm/zenodo. If you want to open an issue or PR, please do it on the github repository. I chose to do it this way because I wanted to use github actions. Currently only github action is mirroring the repository to huggingface.
| [
"# Download zenodo dataset files using huggingface datasets\n\nYou can download a specific file from the Zenodo dataset using the following code:\n\nZenodo id : 5172018\nFile name : URL\n\n\n\nThis command will also copy the file into your current directory so that you can use it directly.\n\nHere is an example notebook: URL",
"# Contribution\n\nThe huggingface repository is actually a mirror of the github repository osbm/zenodo. If you want to open an issue or PR, please do it on the github repository. I chose to do it this way because I wanted to use github actions. Currently only github action is mirroring the repository to huggingface."
] | [
"TAGS\n#region-us \n",
"# Download zenodo dataset files using huggingface datasets\n\nYou can download a specific file from the Zenodo dataset using the following code:\n\nZenodo id : 5172018\nFile name : URL\n\n\n\nThis command will also copy the file into your current directory so that you can use it directly.\n\nHere is an example notebook: URL",
"# Contribution\n\nThe huggingface repository is actually a mirror of the github repository osbm/zenodo. If you want to open an issue or PR, please do it on the github repository. I chose to do it this way because I wanted to use github actions. Currently only github action is mirroring the repository to huggingface."
] | [
6,
69,
83
] | [
"passage: TAGS\n#region-us \n# Download zenodo dataset files using huggingface datasets\n\nYou can download a specific file from the Zenodo dataset using the following code:\n\nZenodo id : 5172018\nFile name : URL\n\n\n\nThis command will also copy the file into your current directory so that you can use it directly.\n\nHere is an example notebook: URL# Contribution\n\nThe huggingface repository is actually a mirror of the github repository osbm/zenodo. If you want to open an issue or PR, please do it on the github repository. I chose to do it this way because I wanted to use github actions. Currently only github action is mirroring the repository to huggingface."
] |
05f2b9a2b864e04ec1a969f6d31923a776307c53 | ........ | datascopum/datascopum | [
"region:us"
] | 2022-09-26T13:56:42+00:00 | {} | 2022-09-29T15:33:40+00:00 | [] | [] | TAGS
#region-us
| ........ | [] | [
"TAGS\n#region-us \n"
] | [
6
] | [
"passage: TAGS\n#region-us \n"
] |
68d75d195c960726ab362a157dfa311e075295a8 | # Dataset Card for "model-repos-stats"
[More Information needed](https://github.com/huggingface/datasets/blob/main/CONTRIBUTING.md#how-to-contribute-to-the-dataset-cards) | open-source-metrics/model-repos-stats | [
"region:us"
] | 2022-09-26T14:54:28+00:00 | {"dataset_info": {"features": [{"name": "Unnamed: 0", "dtype": "int64"}, {"name": "repo_id", "dtype": "string"}, {"name": "author", "dtype": "string"}, {"name": "model_type", "dtype": "string"}, {"name": "files_per_repo", "dtype": "int64"}, {"name": "downloads_30d", "dtype": "int64"}, {"name": "library", "dtype": "string"}, {"name": "likes", "dtype": "int64"}, {"name": "pipeline", "dtype": "string"}, {"name": "pytorch", "dtype": "bool"}, {"name": "tensorflow", "dtype": "bool"}, {"name": "jax", "dtype": "bool"}, {"name": "license", "dtype": "string"}, {"name": "languages", "dtype": "string"}, {"name": "datasets", "dtype": "string"}, {"name": "co2", "dtype": "string"}, {"name": "prs_count", "dtype": "int64"}, {"name": "prs_open", "dtype": "int64"}, {"name": "prs_merged", "dtype": "int64"}, {"name": "prs_closed", "dtype": "int64"}, {"name": "discussions_count", "dtype": "int64"}, {"name": "discussions_open", "dtype": "int64"}, {"name": "discussions_closed", "dtype": "int64"}, {"name": "tags", "dtype": "string"}, {"name": "has_model_index", "dtype": "bool"}, {"name": "has_metadata", "dtype": "bool"}, {"name": "has_text", "dtype": "bool"}, {"name": "text_length", "dtype": "int64"}], "splits": [{"name": "train", "num_bytes": 68539081, "num_examples": 245197}], "download_size": 14926618, "dataset_size": 68539081}} | 2023-07-03T00:35:17+00:00 | [] | [] | TAGS
#region-us
| # Dataset Card for "model-repos-stats"
More Information needed | [
"# Dataset Card for \"model-repos-stats\"\n\nMore Information needed"
] | [
"TAGS\n#region-us \n",
"# Dataset Card for \"model-repos-stats\"\n\nMore Information needed"
] | [
6,
17
] | [
"passage: TAGS\n#region-us \n# Dataset Card for \"model-repos-stats\"\n\nMore Information needed"
] |
da31b6c38403a4811b20342486bdf0ec2a724a2a | **Context**
Generating humor is a complex task in the domain of machine learning, and it requires the models to understand the deep semantic meaning of a joke in order to generate new ones. Such problems, however, are difficult to solve due to a number of reasons, one of which is the lack of a database that gives an elaborate list of jokes. Thus, a large corpus of over 0.2 million jokes has been collected by scraping several websites containing funny and short jokes.
You can visit the [Github repository](https://github.com/amoudgl/short-jokes-dataset) from [amoudgl](https://github.com/amoudgl) for more information regarding collection of data and the scripts used.
**Content**
This dataset is in the form of a csv file containing 231,657 jokes. Length of jokes ranges from 10 to 200 characters. Each line in the file contains a unique ID and joke.
**Disclaimer**
It has been attempted to keep the jokes as clean as possible. Since the data has been collected by scraping websites, it is possible that there may be a few jokes that are inappropriate or offensive to some people.
**Note**
This dataset is taken from Kaggle dataset that can be found [here](https://www.kaggle.com/datasets/abhinavmoudgil95/short-jokes). | ysharma/short_jokes | [
"license:mit",
"region:us"
] | 2022-09-26T15:57:00+00:00 | {"license": "mit"} | 2022-09-26T16:11:06+00:00 | [] | [] | TAGS
#license-mit #region-us
| Context
Generating humor is a complex task in the domain of machine learning, and it requires the models to understand the deep semantic meaning of a joke in order to generate new ones. Such problems, however, are difficult to solve due to a number of reasons, one of which is the lack of a database that gives an elaborate list of jokes. Thus, a large corpus of over 0.2 million jokes has been collected by scraping several websites containing funny and short jokes.
You can visit the Github repository from amoudgl for more information regarding collection of data and the scripts used.
Content
This dataset is in the form of a csv file containing 231,657 jokes. Length of jokes ranges from 10 to 200 characters. Each line in the file contains a unique ID and joke.
Disclaimer
It has been attempted to keep the jokes as clean as possible. Since the data has been collected by scraping websites, it is possible that there may be a few jokes that are inappropriate or offensive to some people.
Note
This dataset is taken from Kaggle dataset that can be found here. | [] | [
"TAGS\n#license-mit #region-us \n"
] | [
11
] | [
"passage: TAGS\n#license-mit #region-us \n"
] |
de93f205b1d46c99e45e3da694207776da2bbf63 |
# Dataset Card for CoSimLex
### Dataset Summary
The dataset contains human similarity ratings for pairs of words. The annotators were presented with contexts that contained both of the words in the pair and the dataset features two different contexts per pair. The words were sourced from the English, Croatian, Finnish and Slovenian versions of the original Simlex dataset.
Statistics:
- 340 English pairs (config `en`),
- 112 Croatian pairs (config `hr`),
- 111 Slovenian pairs (config `sl`),
- 24 Finnish pairs (config `fi`).
### Supported Tasks and Leaderboards
Graded word similarity in context.
### Languages
English, Croatian, Slovenian, Finnish.
## Dataset Structure
### Data Instances
A sample instance from the dataset:
```
{
'word1': 'absence',
'word2': 'presence',
'context1': 'African slaves from Angola and Mozambique were also present, but in fewer numbers than in other Brazilian areas, because Paranรก was a poor region that did not need much slave manpower. The immigration grew in the mid-19th century, mostly composed of Italian, German, Polish, Ukrainian, and Japanese peoples. While Poles and Ukrainians are present in Paranรก, their <strong>presence</strong> in the rest of Brazil is almost <strong>absence</strong>.',
'context2': 'The Chinese had become almost impossible to deal with because of the turmoil associated with the cultural revolution. The North Vietnamese <strong>presence</strong> in Eastern Cambodia had grown so large that it was destabilizing Cambodia politically and economically. Further, when the Cambodian left went underground in the late 1960s, Sihanouk had to make concessions to the right in the <strong>absence</strong> of any force that he could play off against them.',
'sim1': 2.2699999809265137,
'sim2': 1.3700000047683716,
'stdev1': 2.890000104904175,
'stdev2': 1.7899999618530273,
'pvalue': 0.2409999966621399,
'word1_context1': 'absence',
'word2_context1': 'presence',
'word1_context2': 'absence',
'word2_context2': 'presence'
}
```
### Data Fields
- `word1`: a string representing the first word in the pair. Uninflected form.
- `word2`: a string representing the second word in the pair. Uninflected form.
- `context1`: a string representing the first context containing the pair of words. The target words are marked with a `<strong></strong>` labels.
- `context2`: a string representing the second context containing the pair of words. The target words are marked with a `<strong></strong>` labels.
- `sim1`: a float representing the mean of the similarity scores within the first context.
- `sim2`: a float representing the mean of the similarity scores within the second context.
- `stdev1`: a float representing the standard Deviation for the scores within the first context.
- `stdev2`: a float representing the standard deviation for the scores within the second context.
- `pvalue`: a float representing the p-value calculated using the Mann-Whitney U test.
- `word1_context1`: a string representing the inflected version of the first word as it appears in the first context.
- `word2_context1`: a string representing the inflected version of the second word as it appears in the first context.
- `word1_context2`: a string representing the inflected version of the first word as it appears in the second context.
- `word2_context2`: a string representing the inflected version of the second word as it appears in the second context.
## Additional Information
### Dataset Curators
Carlos Armendariz; et al. (please see http://hdl.handle.net/11356/1308 for the full list)
### Licensing Information
GNU GPL v3.0.
### Citation Information
```
@inproceedings{armendariz-etal-2020-semeval,
title = "{SemEval-2020} {T}ask 3: Graded Word Similarity in Context ({GWSC})",
author = "Armendariz, Carlos S. and
Purver, Matthew and
Pollak, Senja and
Ljube{\v{s}}i{\'{c}}, Nikola and
Ul{\v{c}}ar, Matej and
Robnik-{\v{S}}ikonja, Marko and
Vuli{\'{c}}, Ivan and
Pilehvar, Mohammad Taher",
booktitle = "Proceedings of the 14th International Workshop on Semantic Evaluation",
year = "2020",
address="Online"
}
```
### Contributions
Thanks to [@matejklemen](https://github.com/matejklemen) for adding this dataset.
| cjvt/cosimlex | [
"task_categories:other",
"annotations_creators:crowdsourced",
"language_creators:found",
"multilinguality:multilingual",
"size_categories:n<1K",
"language:en",
"language:hr",
"language:sl",
"language:fi",
"license:gpl-3.0",
"graded-word-similarity-in-context",
"region:us"
] | 2022-09-26T17:13:05+00:00 | {"annotations_creators": ["crowdsourced"], "language_creators": ["found"], "language": ["en", "hr", "sl", "fi"], "license": ["gpl-3.0"], "multilinguality": ["multilingual"], "size_categories": ["n<1K"], "source_datasets": [], "task_categories": ["other"], "task_ids": [], "pretty_name": "CoSimLex", "tags": ["graded-word-similarity-in-context"]} | 2022-10-21T06:34:58+00:00 | [] | [
"en",
"hr",
"sl",
"fi"
] | TAGS
#task_categories-other #annotations_creators-crowdsourced #language_creators-found #multilinguality-multilingual #size_categories-n<1K #language-English #language-Croatian #language-Slovenian #language-Finnish #license-gpl-3.0 #graded-word-similarity-in-context #region-us
|
# Dataset Card for CoSimLex
### Dataset Summary
The dataset contains human similarity ratings for pairs of words. The annotators were presented with contexts that contained both of the words in the pair and the dataset features two different contexts per pair. The words were sourced from the English, Croatian, Finnish and Slovenian versions of the original Simlex dataset.
Statistics:
- 340 English pairs (config 'en'),
- 112 Croatian pairs (config 'hr'),
- 111 Slovenian pairs (config 'sl'),
- 24 Finnish pairs (config 'fi').
### Supported Tasks and Leaderboards
Graded word similarity in context.
### Languages
English, Croatian, Slovenian, Finnish.
## Dataset Structure
### Data Instances
A sample instance from the dataset:
### Data Fields
- 'word1': a string representing the first word in the pair. Uninflected form.
- 'word2': a string representing the second word in the pair. Uninflected form.
- 'context1': a string representing the first context containing the pair of words. The target words are marked with a '<strong></strong>' labels.
- 'context2': a string representing the second context containing the pair of words. The target words are marked with a '<strong></strong>' labels.
- 'sim1': a float representing the mean of the similarity scores within the first context.
- 'sim2': a float representing the mean of the similarity scores within the second context.
- 'stdev1': a float representing the standard Deviation for the scores within the first context.
- 'stdev2': a float representing the standard deviation for the scores within the second context.
- 'pvalue': a float representing the p-value calculated using the Mann-Whitney U test.
- 'word1_context1': a string representing the inflected version of the first word as it appears in the first context.
- 'word2_context1': a string representing the inflected version of the second word as it appears in the first context.
- 'word1_context2': a string representing the inflected version of the first word as it appears in the second context.
- 'word2_context2': a string representing the inflected version of the second word as it appears in the second context.
## Additional Information
### Dataset Curators
Carlos Armendariz; et al. (please see URL for the full list)
### Licensing Information
GNU GPL v3.0.
### Contributions
Thanks to @matejklemen for adding this dataset.
| [
"# Dataset Card for CoSimLex",
"### Dataset Summary\n\nThe dataset contains human similarity ratings for pairs of words. The annotators were presented with contexts that contained both of the words in the pair and the dataset features two different contexts per pair. The words were sourced from the English, Croatian, Finnish and Slovenian versions of the original Simlex dataset. \nStatistics: \n- 340 English pairs (config 'en'),\n- 112 Croatian pairs (config 'hr'), \n- 111 Slovenian pairs (config 'sl'),\n- 24 Finnish pairs (config 'fi').",
"### Supported Tasks and Leaderboards\n\nGraded word similarity in context.",
"### Languages\n\nEnglish, Croatian, Slovenian, Finnish.",
"## Dataset Structure",
"### Data Instances\n\nA sample instance from the dataset:",
"### Data Fields\n\n- 'word1': a string representing the first word in the pair. Uninflected form.\n- 'word2': a string representing the second word in the pair. Uninflected form.\n- 'context1': a string representing the first context containing the pair of words. The target words are marked with a '<strong></strong>' labels.\n- 'context2': a string representing the second context containing the pair of words. The target words are marked with a '<strong></strong>' labels.\n- 'sim1': a float representing the mean of the similarity scores within the first context.\n- 'sim2': a float representing the mean of the similarity scores within the second context.\n- 'stdev1': a float representing the standard Deviation for the scores within the first context.\n- 'stdev2': a float representing the standard deviation for the scores within the second context.\n- 'pvalue': a float representing the p-value calculated using the Mann-Whitney U test.\n- 'word1_context1': a string representing the inflected version of the first word as it appears in the first context.\n- 'word2_context1': a string representing the inflected version of the second word as it appears in the first context.\n- 'word1_context2': a string representing the inflected version of the first word as it appears in the second context.\n- 'word2_context2': a string representing the inflected version of the second word as it appears in the second context.",
"## Additional Information",
"### Dataset Curators\n\nCarlos Armendariz; et al. (please see URL for the full list)",
"### Licensing Information\n\nGNU GPL v3.0.",
"### Contributions\n\nThanks to @matejklemen for adding this dataset."
] | [
"TAGS\n#task_categories-other #annotations_creators-crowdsourced #language_creators-found #multilinguality-multilingual #size_categories-n<1K #language-English #language-Croatian #language-Slovenian #language-Finnish #license-gpl-3.0 #graded-word-similarity-in-context #region-us \n",
"# Dataset Card for CoSimLex",
"### Dataset Summary\n\nThe dataset contains human similarity ratings for pairs of words. The annotators were presented with contexts that contained both of the words in the pair and the dataset features two different contexts per pair. The words were sourced from the English, Croatian, Finnish and Slovenian versions of the original Simlex dataset. \nStatistics: \n- 340 English pairs (config 'en'),\n- 112 Croatian pairs (config 'hr'), \n- 111 Slovenian pairs (config 'sl'),\n- 24 Finnish pairs (config 'fi').",
"### Supported Tasks and Leaderboards\n\nGraded word similarity in context.",
"### Languages\n\nEnglish, Croatian, Slovenian, Finnish.",
"## Dataset Structure",
"### Data Instances\n\nA sample instance from the dataset:",
"### Data Fields\n\n- 'word1': a string representing the first word in the pair. Uninflected form.\n- 'word2': a string representing the second word in the pair. Uninflected form.\n- 'context1': a string representing the first context containing the pair of words. The target words are marked with a '<strong></strong>' labels.\n- 'context2': a string representing the second context containing the pair of words. The target words are marked with a '<strong></strong>' labels.\n- 'sim1': a float representing the mean of the similarity scores within the first context.\n- 'sim2': a float representing the mean of the similarity scores within the second context.\n- 'stdev1': a float representing the standard Deviation for the scores within the first context.\n- 'stdev2': a float representing the standard deviation for the scores within the second context.\n- 'pvalue': a float representing the p-value calculated using the Mann-Whitney U test.\n- 'word1_context1': a string representing the inflected version of the first word as it appears in the first context.\n- 'word2_context1': a string representing the inflected version of the second word as it appears in the first context.\n- 'word1_context2': a string representing the inflected version of the first word as it appears in the second context.\n- 'word2_context2': a string representing the inflected version of the second word as it appears in the second context.",
"## Additional Information",
"### Dataset Curators\n\nCarlos Armendariz; et al. (please see URL for the full list)",
"### Licensing Information\n\nGNU GPL v3.0.",
"### Contributions\n\nThanks to @matejklemen for adding this dataset."
] | [
97,
9,
131,
18,
15,
6,
14,
382,
5,
24,
12,
18
] | [
"passage: TAGS\n#task_categories-other #annotations_creators-crowdsourced #language_creators-found #multilinguality-multilingual #size_categories-n<1K #language-English #language-Croatian #language-Slovenian #language-Finnish #license-gpl-3.0 #graded-word-similarity-in-context #region-us \n# Dataset Card for CoSimLex### Dataset Summary\n\nThe dataset contains human similarity ratings for pairs of words. The annotators were presented with contexts that contained both of the words in the pair and the dataset features two different contexts per pair. The words were sourced from the English, Croatian, Finnish and Slovenian versions of the original Simlex dataset. \nStatistics: \n- 340 English pairs (config 'en'),\n- 112 Croatian pairs (config 'hr'), \n- 111 Slovenian pairs (config 'sl'),\n- 24 Finnish pairs (config 'fi').### Supported Tasks and Leaderboards\n\nGraded word similarity in context.### Languages\n\nEnglish, Croatian, Slovenian, Finnish.## Dataset Structure### Data Instances\n\nA sample instance from the dataset:"
] |
16e24521436eaf961e62b0406744617666a741ba |
# Dataset Card for Airplane Crashes and Fatalities
## Table of Contents
- [Table of Contents](#table-of-contents)
- [Dataset Description](#dataset-description)
- [Dataset Summary](#dataset-summary)
- [Supported Tasks and Leaderboards](#supported-tasks-and-leaderboards)
- [Languages](#languages)
- [Dataset Structure](#dataset-structure)
- [Data Instances](#data-instances)
- [Data Fields](#data-fields)
- [Data Splits](#data-splits)
- [Dataset Creation](#dataset-creation)
- [Curation Rationale](#curation-rationale)
- [Source Data](#source-data)
- [Annotations](#annotations)
- [Personal and Sensitive Information](#personal-and-sensitive-information)
- [Considerations for Using the Data](#considerations-for-using-the-data)
- [Social Impact of Dataset](#social-impact-of-dataset)
- [Discussion of Biases](#discussion-of-biases)
- [Other Known Limitations](#other-known-limitations)
- [Additional Information](#additional-information)
- [Dataset Curators](#dataset-curators)
- [Licensing Information](#licensing-information)
- [Citation Information](#citation-information)
- [Contributions](#contributions)
## Dataset Description
- **Homepage:** https://kaggle.com/datasets/thedevastator/airplane-crashes-and-fatalities
- **Repository:**
- **Paper:**
- **Leaderboard:**
- **Point of Contact:**
### Dataset Summary
## Airplane Crashes and Fatalities
_____
This dataset showcases Boeing 707 accidents that have occurred since 1948. The data includes information on the date, time, location, operator, flight number, route, type of aircraft, registration number, cn/In number of persons on board, fatalities, ground fatalities, and a summary of the accident
### How to use the dataset
This dataset includes information on over 5,000 airplane crashes around the world.
This is an absolutely essential dataset for anyone interested in aviation safety! Here you will find information on when and where each crash occurred, what type of plane was involved, how many people were killed, and much more.
This dataset is perfect for anyone interested in data visualization or analysis. With so much information available, there are endless possibilities for interesting stories and insights that can be gleaned from this data.
So whether you're a seasoned data pro or just getting started, this dataset is sure to give you plenty to work with. So get started today and see what you can discover!
### Research Ideas
1. Plot a map of all flight routes
2. Analyze what type of aircraft is involved in the most crashes
3. Identify patterns in where/when crashes occur
### Columns
- **index:** the index of the row
- **Date:** the date of the incident
- **Time:** the time of the incident
- **Location:** the location of the incident
- **Operator:** the operator of the aircraft
- **Flight #:** the flight number of the aircraft
- **Route:** the route of the aircraft
- **Type:** the type of aircraft
- **Registration:** the registration of the aircraft
- **cn/In:** the construction number/serial number of the aircraft
- **Aboard:** the number of people on board the aircraft
- **Fatalities:** the number of fatalities in the incident
- **Ground:** the number of people on the ground killed in the incident
- **Summary:** a summary of the incident
### Acknowledgements
This dataset was obtained from the Data Society. If you use this dataset in your research, please credit the Data Society.
Columns: index, Date, Time, Location, Operator, Flight #, Route, Type, Registration, cn/In, Aboard, Fatalities Ground Summary
> [Data Source](https://data.world/data-society)
### Supported Tasks and Leaderboards
[More Information Needed]
### Languages
[More Information Needed]
## Dataset Structure
### Data Instances
[More Information Needed]
### Data Fields
[More Information Needed]
### Data Splits
[More Information Needed]
## Dataset Creation
### Curation Rationale
[More Information Needed]
### Source Data
#### Initial Data Collection and Normalization
[More Information Needed]
#### Who are the source language producers?
[More Information Needed]
### Annotations
#### Annotation process
[More Information Needed]
#### Who are the annotators?
[More Information Needed]
### Personal and Sensitive Information
[More Information Needed]
## Considerations for Using the Data
### Social Impact of Dataset
[More Information Needed]
### Discussion of Biases
[More Information Needed]
### Other Known Limitations
[More Information Needed]
## Additional Information
### Dataset Curators
This dataset was shared by [@thedevastator](https://kaggle.com/thedevastator)
### Licensing Information
The license for this dataset is cc-by-nc-sa-4.0
### Citation Information
```bibtex
[More Information Needed]
```
### Contributions
[More Information Needed] | nateraw/airplane-crashes-and-fatalities | [
"license:cc-by-nc-sa-4.0",
"region:us"
] | 2022-09-26T18:02:55+00:00 | {"license": ["cc-by-nc-sa-4.0"], "converted_from": "kaggle", "kaggle_id": "thedevastator/airplane-crashes-and-fatalities"} | 2022-09-27T16:55:18+00:00 | [] | [] | TAGS
#license-cc-by-nc-sa-4.0 #region-us
|
# Dataset Card for Airplane Crashes and Fatalities
## Table of Contents
- Table of Contents
- Dataset Description
- Dataset Summary
- Supported Tasks and Leaderboards
- Languages
- Dataset Structure
- Data Instances
- Data Fields
- Data Splits
- Dataset Creation
- Curation Rationale
- Source Data
- Annotations
- Personal and Sensitive Information
- Considerations for Using the Data
- Social Impact of Dataset
- Discussion of Biases
- Other Known Limitations
- Additional Information
- Dataset Curators
- Licensing Information
- Citation Information
- Contributions
## Dataset Description
- Homepage: URL
- Repository:
- Paper:
- Leaderboard:
- Point of Contact:
### Dataset Summary
## Airplane Crashes and Fatalities
_____
This dataset showcases Boeing 707 accidents that have occurred since 1948. The data includes information on the date, time, location, operator, flight number, route, type of aircraft, registration number, cn/In number of persons on board, fatalities, ground fatalities, and a summary of the accident
### How to use the dataset
This dataset includes information on over 5,000 airplane crashes around the world.
This is an absolutely essential dataset for anyone interested in aviation safety! Here you will find information on when and where each crash occurred, what type of plane was involved, how many people were killed, and much more.
This dataset is perfect for anyone interested in data visualization or analysis. With so much information available, there are endless possibilities for interesting stories and insights that can be gleaned from this data.
So whether you're a seasoned data pro or just getting started, this dataset is sure to give you plenty to work with. So get started today and see what you can discover!
### Research Ideas
1. Plot a map of all flight routes
2. Analyze what type of aircraft is involved in the most crashes
3. Identify patterns in where/when crashes occur
### Columns
- index: the index of the row
- Date: the date of the incident
- Time: the time of the incident
- Location: the location of the incident
- Operator: the operator of the aircraft
- Flight #: the flight number of the aircraft
- Route: the route of the aircraft
- Type: the type of aircraft
- Registration: the registration of the aircraft
- cn/In: the construction number/serial number of the aircraft
- Aboard: the number of people on board the aircraft
- Fatalities: the number of fatalities in the incident
- Ground: the number of people on the ground killed in the incident
- Summary: a summary of the incident
### Acknowledgements
This dataset was obtained from the Data Society. If you use this dataset in your research, please credit the Data Society.
Columns: index, Date, Time, Location, Operator, Flight #, Route, Type, Registration, cn/In, Aboard, Fatalities Ground Summary
> Data Source
### Supported Tasks and Leaderboards
### Languages
## Dataset Structure
### Data Instances
### Data Fields
### Data Splits
## Dataset Creation
### Curation Rationale
### Source Data
#### Initial Data Collection and Normalization
#### Who are the source language producers?
### Annotations
#### Annotation process
#### Who are the annotators?
### Personal and Sensitive Information
## Considerations for Using the Data
### Social Impact of Dataset
### Discussion of Biases
### Other Known Limitations
## Additional Information
### Dataset Curators
This dataset was shared by @thedevastator
### Licensing Information
The license for this dataset is cc-by-nc-sa-4.0
### Contributions
| [
"# Dataset Card for Airplane Crashes and Fatalities",
"## Table of Contents\n- Table of Contents\n- Dataset Description\n - Dataset Summary\n - Supported Tasks and Leaderboards\n - Languages\n- Dataset Structure\n - Data Instances\n - Data Fields\n - Data Splits\n- Dataset Creation\n - Curation Rationale\n - Source Data\n - Annotations\n - Personal and Sensitive Information\n- Considerations for Using the Data\n - Social Impact of Dataset\n - Discussion of Biases\n - Other Known Limitations\n- Additional Information\n - Dataset Curators\n - Licensing Information\n - Citation Information\n - Contributions",
"## Dataset Description\n\n- Homepage: URL\n- Repository:\n- Paper:\n- Leaderboard:\n- Point of Contact:",
"### Dataset Summary",
"## Airplane Crashes and Fatalities\n_____\n\nThis dataset showcases Boeing 707 accidents that have occurred since 1948. The data includes information on the date, time, location, operator, flight number, route, type of aircraft, registration number, cn/In number of persons on board, fatalities, ground fatalities, and a summary of the accident",
"### How to use the dataset\nThis dataset includes information on over 5,000 airplane crashes around the world.\n\nThis is an absolutely essential dataset for anyone interested in aviation safety! Here you will find information on when and where each crash occurred, what type of plane was involved, how many people were killed, and much more.\n\nThis dataset is perfect for anyone interested in data visualization or analysis. With so much information available, there are endless possibilities for interesting stories and insights that can be gleaned from this data.\n\nSo whether you're a seasoned data pro or just getting started, this dataset is sure to give you plenty to work with. So get started today and see what you can discover!",
"### Research Ideas\n1. Plot a map of all flight routes\n2. Analyze what type of aircraft is involved in the most crashes\n3. Identify patterns in where/when crashes occur",
"### Columns\n- index: the index of the row\n- Date: the date of the incident\n- Time: the time of the incident\n- Location: the location of the incident\n- Operator: the operator of the aircraft\n- Flight #: the flight number of the aircraft\n- Route: the route of the aircraft\n- Type: the type of aircraft\n- Registration: the registration of the aircraft\n- cn/In: the construction number/serial number of the aircraft\n- Aboard: the number of people on board the aircraft\n- Fatalities: the number of fatalities in the incident\n- Ground: the number of people on the ground killed in the incident\n- Summary: a summary of the incident",
"### Acknowledgements\nThis dataset was obtained from the Data Society. If you use this dataset in your research, please credit the Data Society.\n\nColumns: index, Date, Time, Location, Operator, Flight #, Route, Type, Registration, cn/In, Aboard, Fatalities Ground Summary\n\n> Data Source",
"### Supported Tasks and Leaderboards",
"### Languages",
"## Dataset Structure",
"### Data Instances",
"### Data Fields",
"### Data Splits",
"## Dataset Creation",
"### Curation Rationale",
"### Source Data",
"#### Initial Data Collection and Normalization",
"#### Who are the source language producers?",
"### Annotations",
"#### Annotation process",
"#### Who are the annotators?",
"### Personal and Sensitive Information",
"## Considerations for Using the Data",
"### Social Impact of Dataset",
"### Discussion of Biases",
"### Other Known Limitations",
"## Additional Information",
"### Dataset Curators\n\nThis dataset was shared by @thedevastator",
"### Licensing Information\n\nThe license for this dataset is cc-by-nc-sa-4.0",
"### Contributions"
] | [
"TAGS\n#license-cc-by-nc-sa-4.0 #region-us \n",
"# Dataset Card for Airplane Crashes and Fatalities",
"## Table of Contents\n- Table of Contents\n- Dataset Description\n - Dataset Summary\n - Supported Tasks and Leaderboards\n - Languages\n- Dataset Structure\n - Data Instances\n - Data Fields\n - Data Splits\n- Dataset Creation\n - Curation Rationale\n - Source Data\n - Annotations\n - Personal and Sensitive Information\n- Considerations for Using the Data\n - Social Impact of Dataset\n - Discussion of Biases\n - Other Known Limitations\n- Additional Information\n - Dataset Curators\n - Licensing Information\n - Citation Information\n - Contributions",
"## Dataset Description\n\n- Homepage: URL\n- Repository:\n- Paper:\n- Leaderboard:\n- Point of Contact:",
"### Dataset Summary",
"## Airplane Crashes and Fatalities\n_____\n\nThis dataset showcases Boeing 707 accidents that have occurred since 1948. The data includes information on the date, time, location, operator, flight number, route, type of aircraft, registration number, cn/In number of persons on board, fatalities, ground fatalities, and a summary of the accident",
"### How to use the dataset\nThis dataset includes information on over 5,000 airplane crashes around the world.\n\nThis is an absolutely essential dataset for anyone interested in aviation safety! Here you will find information on when and where each crash occurred, what type of plane was involved, how many people were killed, and much more.\n\nThis dataset is perfect for anyone interested in data visualization or analysis. With so much information available, there are endless possibilities for interesting stories and insights that can be gleaned from this data.\n\nSo whether you're a seasoned data pro or just getting started, this dataset is sure to give you plenty to work with. So get started today and see what you can discover!",
"### Research Ideas\n1. Plot a map of all flight routes\n2. Analyze what type of aircraft is involved in the most crashes\n3. Identify patterns in where/when crashes occur",
"### Columns\n- index: the index of the row\n- Date: the date of the incident\n- Time: the time of the incident\n- Location: the location of the incident\n- Operator: the operator of the aircraft\n- Flight #: the flight number of the aircraft\n- Route: the route of the aircraft\n- Type: the type of aircraft\n- Registration: the registration of the aircraft\n- cn/In: the construction number/serial number of the aircraft\n- Aboard: the number of people on board the aircraft\n- Fatalities: the number of fatalities in the incident\n- Ground: the number of people on the ground killed in the incident\n- Summary: a summary of the incident",
"### Acknowledgements\nThis dataset was obtained from the Data Society. If you use this dataset in your research, please credit the Data Society.\n\nColumns: index, Date, Time, Location, Operator, Flight #, Route, Type, Registration, cn/In, Aboard, Fatalities Ground Summary\n\n> Data Source",
"### Supported Tasks and Leaderboards",
"### Languages",
"## Dataset Structure",
"### Data Instances",
"### Data Fields",
"### Data Splits",
"## Dataset Creation",
"### Curation Rationale",
"### Source Data",
"#### Initial Data Collection and Normalization",
"#### Who are the source language producers?",
"### Annotations",
"#### Annotation process",
"#### Who are the annotators?",
"### Personal and Sensitive Information",
"## Considerations for Using the Data",
"### Social Impact of Dataset",
"### Discussion of Biases",
"### Other Known Limitations",
"## Additional Information",
"### Dataset Curators\n\nThis dataset was shared by @thedevastator",
"### Licensing Information\n\nThe license for this dataset is cc-by-nc-sa-4.0",
"### Contributions"
] | [
19,
14,
125,
25,
6,
80,
154,
43,
150,
75,
10,
4,
6,
6,
5,
5,
5,
7,
4,
10,
10,
5,
5,
9,
8,
8,
7,
8,
7,
5,
17,
23,
5
] | [
"passage: TAGS\n#license-cc-by-nc-sa-4.0 #region-us \n# Dataset Card for Airplane Crashes and Fatalities## Table of Contents\n- Table of Contents\n- Dataset Description\n - Dataset Summary\n - Supported Tasks and Leaderboards\n - Languages\n- Dataset Structure\n - Data Instances\n - Data Fields\n - Data Splits\n- Dataset Creation\n - Curation Rationale\n - Source Data\n - Annotations\n - Personal and Sensitive Information\n- Considerations for Using the Data\n - Social Impact of Dataset\n - Discussion of Biases\n - Other Known Limitations\n- Additional Information\n - Dataset Curators\n - Licensing Information\n - Citation Information\n - Contributions## Dataset Description\n\n- Homepage: URL\n- Repository:\n- Paper:\n- Leaderboard:\n- Point of Contact:### Dataset Summary## Airplane Crashes and Fatalities\n_____\n\nThis dataset showcases Boeing 707 accidents that have occurred since 1948. The data includes information on the date, time, location, operator, flight number, route, type of aircraft, registration number, cn/In number of persons on board, fatalities, ground fatalities, and a summary of the accident### How to use the dataset\nThis dataset includes information on over 5,000 airplane crashes around the world.\n\nThis is an absolutely essential dataset for anyone interested in aviation safety! Here you will find information on when and where each crash occurred, what type of plane was involved, how many people were killed, and much more.\n\nThis dataset is perfect for anyone interested in data visualization or analysis. With so much information available, there are endless possibilities for interesting stories and insights that can be gleaned from this data.\n\nSo whether you're a seasoned data pro or just getting started, this dataset is sure to give you plenty to work with. So get started today and see what you can discover!### Research Ideas\n1. Plot a map of all flight routes\n2. Analyze what type of aircraft is involved in the most crashes\n3. Identify patterns in where/when crashes occur"
] |
ad5e82960d05d634773914859e9e47c70614823c | Simple English Wikipedia it has only about 170k articles. We split these articles into paragraphs.
wikipedia_filepath = 'simplewiki-2020-11-01.jsonl.gz'
if not os.path.exists(wikipedia_filepath):
util.http_get('http://sbert.net/datasets/simplewiki-2020-11-01.jsonl.gz', wikipedia_filepath) | gfhayworth/wiki_mini | [
"region:us"
] | 2022-09-26T19:42:59+00:00 | {} | 2023-01-28T23:28:54+00:00 | [] | [] | TAGS
#region-us
| Simple English Wikipedia it has only about 170k articles. We split these articles into paragraphs.
wikipedia_filepath = 'URL'
if not URL(wikipedia_filepath):
util.http_get('URL wikipedia_filepath) | [] | [
"TAGS\n#region-us \n"
] | [
6
] | [
"passage: TAGS\n#region-us \n"
] |
d4548d8a0d713c364d69e6dafeec59d3c7717026 | Tweets containing '#Mets' from early August through late September | Ceetar/MetsTweets | [
"region:us"
] | 2022-09-26T22:22:51+00:00 | {} | 2022-09-26T23:08:51+00:00 | [] | [] | TAGS
#region-us
| Tweets containing '#Mets' from early August through late September | [] | [
"TAGS\n#region-us \n"
] | [
6
] | [
"passage: TAGS\n#region-us \n"
] |
5e26419ab91ed4a212eb945097dfc3b5d0687401 | # Dataset Card for AutoTrain Evaluator
This repository contains model predictions generated by [AutoTrain](https://huggingface.co/autotrain) for the following task and dataset:
* Task: Zero-Shot Text Classification
* Model: Tristan/opt-66b-copy
* Dataset: mathemakitten/winobias_antistereotype_dev
* Config: mathemakitten--winobias_antistereotype_dev
* Split: validation
To run new evaluation jobs, visit Hugging Face's [automatic model evaluator](https://huggingface.co/spaces/autoevaluate/model-evaluator).
## Contributions
Thanks to [@Tristan](https://huggingface.co/Tristan) for evaluating this model. | autoevaluate/autoeval-eval-mathemakitten__winobias_antistereotype_dev-mathemakitte-08a58b-1563555688 | [
"autotrain",
"evaluation",
"region:us"
] | 2022-09-27T00:44:58+00:00 | {"type": "predictions", "tags": ["autotrain", "evaluation"], "datasets": ["mathemakitten/winobias_antistereotype_dev"], "eval_info": {"task": "text_zero_shot_classification", "model": "Tristan/opt-66b-copy", "metrics": [], "dataset_name": "mathemakitten/winobias_antistereotype_dev", "dataset_config": "mathemakitten--winobias_antistereotype_dev", "dataset_split": "validation", "col_mapping": {"text": "text", "classes": "classes", "target": "target"}}} | 2022-09-27T03:26:16+00:00 | [] | [] | TAGS
#autotrain #evaluation #region-us
| # Dataset Card for AutoTrain Evaluator
This repository contains model predictions generated by AutoTrain for the following task and dataset:
* Task: Zero-Shot Text Classification
* Model: Tristan/opt-66b-copy
* Dataset: mathemakitten/winobias_antistereotype_dev
* Config: mathemakitten--winobias_antistereotype_dev
* Split: validation
To run new evaluation jobs, visit Hugging Face's automatic model evaluator.
## Contributions
Thanks to @Tristan for evaluating this model. | [
"# Dataset Card for AutoTrain Evaluator\n\nThis repository contains model predictions generated by AutoTrain for the following task and dataset:\n\n* Task: Zero-Shot Text Classification\n* Model: Tristan/opt-66b-copy\n* Dataset: mathemakitten/winobias_antistereotype_dev\n* Config: mathemakitten--winobias_antistereotype_dev\n* Split: validation\n\nTo run new evaluation jobs, visit Hugging Face's automatic model evaluator.",
"## Contributions\n\nThanks to @Tristan for evaluating this model."
] | [
"TAGS\n#autotrain #evaluation #region-us \n",
"# Dataset Card for AutoTrain Evaluator\n\nThis repository contains model predictions generated by AutoTrain for the following task and dataset:\n\n* Task: Zero-Shot Text Classification\n* Model: Tristan/opt-66b-copy\n* Dataset: mathemakitten/winobias_antistereotype_dev\n* Config: mathemakitten--winobias_antistereotype_dev\n* Split: validation\n\nTo run new evaluation jobs, visit Hugging Face's automatic model evaluator.",
"## Contributions\n\nThanks to @Tristan for evaluating this model."
] | [
13,
118,
15
] | [
"passage: TAGS\n#autotrain #evaluation #region-us \n# Dataset Card for AutoTrain Evaluator\n\nThis repository contains model predictions generated by AutoTrain for the following task and dataset:\n\n* Task: Zero-Shot Text Classification\n* Model: Tristan/opt-66b-copy\n* Dataset: mathemakitten/winobias_antistereotype_dev\n* Config: mathemakitten--winobias_antistereotype_dev\n* Split: validation\n\nTo run new evaluation jobs, visit Hugging Face's automatic model evaluator.## Contributions\n\nThanks to @Tristan for evaluating this model."
] |
5bf51cd1b371b4c8aa0fe48d64123e20b25cdaf7 |
# Aggregated Captcha Images and Text
## Credits
All the images (not the texts) here contained have been downloaded and selected from various datasets on kaggle.com
### What is this?
This is a dataset containing some hundreds of thousands of images taken from real and used captchas (reCaptcha, hCaptcha and various others) and containing an equally big amount of random 4-8 length texts generated each one in 363 different fonts and with different random noise, size, colors and scratches on them.
While the texts part might result difficult to recognize from the models you could train, the images quantity allows the model to offer a significant possibility of recognization of captcha images.
### Disclaimer
This dataset is NOT intended to break any ToS of any website or to execute malicious, illegal or unethical actions. This dataset is distributed with a purely informative and educative finality, namely the study of the weakness or strength of the current protection systems.
You will for example notice how puzzle based captchas are highly resistant to this kind of analysis. | tcsenpai/aggregated_captcha_images_and_text | [
"license:cc-by-nc-4.0",
"region:us"
] | 2022-09-27T01:36:22+00:00 | {"license": "cc-by-nc-4.0"} | 2022-09-27T02:31:17+00:00 | [] | [] | TAGS
#license-cc-by-nc-4.0 #region-us
|
# Aggregated Captcha Images and Text
## Credits
All the images (not the texts) here contained have been downloaded and selected from various datasets on URL
### What is this?
This is a dataset containing some hundreds of thousands of images taken from real and used captchas (reCaptcha, hCaptcha and various others) and containing an equally big amount of random 4-8 length texts generated each one in 363 different fonts and with different random noise, size, colors and scratches on them.
While the texts part might result difficult to recognize from the models you could train, the images quantity allows the model to offer a significant possibility of recognization of captcha images.
### Disclaimer
This dataset is NOT intended to break any ToS of any website or to execute malicious, illegal or unethical actions. This dataset is distributed with a purely informative and educative finality, namely the study of the weakness or strength of the current protection systems.
You will for example notice how puzzle based captchas are highly resistant to this kind of analysis. | [
"# Aggregated Captcha Images and Text",
"## Credits\n\nAll the images (not the texts) here contained have been downloaded and selected from various datasets on URL",
"### What is this?\n\nThis is a dataset containing some hundreds of thousands of images taken from real and used captchas (reCaptcha, hCaptcha and various others) and containing an equally big amount of random 4-8 length texts generated each one in 363 different fonts and with different random noise, size, colors and scratches on them.\n\nWhile the texts part might result difficult to recognize from the models you could train, the images quantity allows the model to offer a significant possibility of recognization of captcha images.",
"### Disclaimer\n\nThis dataset is NOT intended to break any ToS of any website or to execute malicious, illegal or unethical actions. This dataset is distributed with a purely informative and educative finality, namely the study of the weakness or strength of the current protection systems.\nYou will for example notice how puzzle based captchas are highly resistant to this kind of analysis."
] | [
"TAGS\n#license-cc-by-nc-4.0 #region-us \n",
"# Aggregated Captcha Images and Text",
"## Credits\n\nAll the images (not the texts) here contained have been downloaded and selected from various datasets on URL",
"### What is this?\n\nThis is a dataset containing some hundreds of thousands of images taken from real and used captchas (reCaptcha, hCaptcha and various others) and containing an equally big amount of random 4-8 length texts generated each one in 363 different fonts and with different random noise, size, colors and scratches on them.\n\nWhile the texts part might result difficult to recognize from the models you could train, the images quantity allows the model to offer a significant possibility of recognization of captcha images.",
"### Disclaimer\n\nThis dataset is NOT intended to break any ToS of any website or to execute malicious, illegal or unethical actions. This dataset is distributed with a purely informative and educative finality, namely the study of the weakness or strength of the current protection systems.\nYou will for example notice how puzzle based captchas are highly resistant to this kind of analysis."
] | [
17,
10,
28,
122,
86
] | [
"passage: TAGS\n#license-cc-by-nc-4.0 #region-us \n# Aggregated Captcha Images and Text## Credits\n\nAll the images (not the texts) here contained have been downloaded and selected from various datasets on URL### What is this?\n\nThis is a dataset containing some hundreds of thousands of images taken from real and used captchas (reCaptcha, hCaptcha and various others) and containing an equally big amount of random 4-8 length texts generated each one in 363 different fonts and with different random noise, size, colors and scratches on them.\n\nWhile the texts part might result difficult to recognize from the models you could train, the images quantity allows the model to offer a significant possibility of recognization of captcha images.### Disclaimer\n\nThis dataset is NOT intended to break any ToS of any website or to execute malicious, illegal or unethical actions. This dataset is distributed with a purely informative and educative finality, namely the study of the weakness or strength of the current protection systems.\nYou will for example notice how puzzle based captchas are highly resistant to this kind of analysis."
] |
76aeb129b64a67d72998420da80c2e51032c6907 |
# Dataset Card for Lexicap
## Table of Contents
- [Dataset Description](#dataset-description)
- [Dataset Summary](#dataset-summary)
- [Supported Tasks and Leaderboards](#supported-tasks-and-leaderboards)
- [Languages](#languages)
- [Dataset Structure](#dataset-structure)
- [Data Instances](#data-instances)
- [Data Fields](#data-fields)
- [Data Splits](#data-splits)
- [Dataset Creation](#dataset-creation)
- [Curation Rationale](#curation-rationale)
- [Source Data](#source-data)
- [Annotations](#annotations)
- [Personal and Sensitive Information](#personal-and-sensitive-information)
- [Considerations for Using the Data](#considerations-for-using-the-data)
- [Social Impact of Dataset](#social-impact-of-dataset)
- [Discussion of Biases](#discussion-of-biases)
- [Other Known Limitations](#other-known-limitations)
- [Additional Information](#additional-information)
- [Dataset Curators](#dataset-curators)
- [Licensing Information](#licensing-information)
- [Citation Information](#citation-information)
- [Contributions](#contributions)
## Dataset Description
-
## Dataset Structure
### Data Instances
Train and test dataset.
j
### Data Fields
## Dataset Creation
### Curation Rationale
[More Information Needed]
### Source Data
#### Initial Data Collection and Normalization
[More Information Needed]
#### Who are the source language producers?
[More Information Needed]
### Annotations
#### Annotation process
[More Information Needed]
#### Who are the annotators?
[More Information Needed]
### Personal and Sensitive Information
[More Information Needed]
## Considerations for Using the Data
### Social Impact of Dataset
[More Information Needed]
### Discussion of Biases
[More Information Needed]
### Other Known Limitations
[More Information Needed]
## Additional Information
### Dataset Curators
[More Information Needed]
### Licensing Information
[More Information Needed]
### Citation Information
### Contributions
| shubhamg2208/lexicap | [
"task_categories:text-classification",
"task_categories:text-generation",
"task_ids:sentiment-analysis",
"task_ids:dialogue-modeling",
"task_ids:language-modeling",
"language_creators:found",
"multilinguality:monolingual",
"size_categories:n<1K",
"source_datasets:original",
"language:en",
"karpathy,whisper,openai",
"region:us"
] | 2022-09-27T02:59:08+00:00 | {"language_creators": ["found"], "language": ["en"], "license": [], "multilinguality": ["monolingual"], "size_categories": ["n<1K"], "source_datasets": ["original"], "task_categories": ["text-classification", "text-generation"], "task_ids": ["sentiment-analysis", "dialogue-modeling", "language-modeling"], "pretty_name": "Lexicap: Lex Fridman Podcast Whisper captions", "lexicap": ["found"], "tags": ["karpathy,whisper,openai"]} | 2022-09-27T03:41:00+00:00 | [] | [
"en"
] | TAGS
#task_categories-text-classification #task_categories-text-generation #task_ids-sentiment-analysis #task_ids-dialogue-modeling #task_ids-language-modeling #language_creators-found #multilinguality-monolingual #size_categories-n<1K #source_datasets-original #language-English #karpathy,whisper,openai #region-us
|
# Dataset Card for Lexicap
## Table of Contents
- Dataset Description
- Dataset Summary
- Supported Tasks and Leaderboards
- Languages
- Dataset Structure
- Data Instances
- Data Fields
- Data Splits
- Dataset Creation
- Curation Rationale
- Source Data
- Annotations
- Personal and Sensitive Information
- Considerations for Using the Data
- Social Impact of Dataset
- Discussion of Biases
- Other Known Limitations
- Additional Information
- Dataset Curators
- Licensing Information
- Citation Information
- Contributions
## Dataset Description
-
## Dataset Structure
### Data Instances
Train and test dataset.
j
### Data Fields
## Dataset Creation
### Curation Rationale
### Source Data
#### Initial Data Collection and Normalization
#### Who are the source language producers?
### Annotations
#### Annotation process
#### Who are the annotators?
### Personal and Sensitive Information
## Considerations for Using the Data
### Social Impact of Dataset
### Discussion of Biases
### Other Known Limitations
## Additional Information
### Dataset Curators
### Licensing Information
### Contributions
| [
"# Dataset Card for Lexicap",
"## Table of Contents\n- Dataset Description\n - Dataset Summary\n - Supported Tasks and Leaderboards\n - Languages\n- Dataset Structure\n - Data Instances\n - Data Fields\n - Data Splits\n- Dataset Creation\n - Curation Rationale\n - Source Data\n - Annotations\n - Personal and Sensitive Information\n- Considerations for Using the Data\n - Social Impact of Dataset\n - Discussion of Biases\n - Other Known Limitations\n- Additional Information\n - Dataset Curators\n - Licensing Information\n - Citation Information\n - Contributions",
"## Dataset Description\n-",
"## Dataset Structure",
"### Data Instances\nTrain and test dataset.\nj",
"### Data Fields",
"## Dataset Creation",
"### Curation Rationale",
"### Source Data",
"#### Initial Data Collection and Normalization",
"#### Who are the source language producers?",
"### Annotations",
"#### Annotation process",
"#### Who are the annotators?",
"### Personal and Sensitive Information",
"## Considerations for Using the Data",
"### Social Impact of Dataset",
"### Discussion of Biases",
"### Other Known Limitations",
"## Additional Information",
"### Dataset Curators",
"### Licensing Information",
"### Contributions"
] | [
"TAGS\n#task_categories-text-classification #task_categories-text-generation #task_ids-sentiment-analysis #task_ids-dialogue-modeling #task_ids-language-modeling #language_creators-found #multilinguality-monolingual #size_categories-n<1K #source_datasets-original #language-English #karpathy,whisper,openai #region-us \n",
"# Dataset Card for Lexicap",
"## Table of Contents\n- Dataset Description\n - Dataset Summary\n - Supported Tasks and Leaderboards\n - Languages\n- Dataset Structure\n - Data Instances\n - Data Fields\n - Data Splits\n- Dataset Creation\n - Curation Rationale\n - Source Data\n - Annotations\n - Personal and Sensitive Information\n- Considerations for Using the Data\n - Social Impact of Dataset\n - Discussion of Biases\n - Other Known Limitations\n- Additional Information\n - Dataset Curators\n - Licensing Information\n - Citation Information\n - Contributions",
"## Dataset Description\n-",
"## Dataset Structure",
"### Data Instances\nTrain and test dataset.\nj",
"### Data Fields",
"## Dataset Creation",
"### Curation Rationale",
"### Source Data",
"#### Initial Data Collection and Normalization",
"#### Who are the source language producers?",
"### Annotations",
"#### Annotation process",
"#### Who are the annotators?",
"### Personal and Sensitive Information",
"## Considerations for Using the Data",
"### Social Impact of Dataset",
"### Discussion of Biases",
"### Other Known Limitations",
"## Additional Information",
"### Dataset Curators",
"### Licensing Information",
"### Contributions"
] | [
109,
7,
120,
5,
6,
13,
5,
5,
7,
4,
10,
10,
5,
5,
9,
8,
8,
7,
8,
7,
5,
6,
6,
5
] | [
"passage: TAGS\n#task_categories-text-classification #task_categories-text-generation #task_ids-sentiment-analysis #task_ids-dialogue-modeling #task_ids-language-modeling #language_creators-found #multilinguality-monolingual #size_categories-n<1K #source_datasets-original #language-English #karpathy,whisper,openai #region-us \n# Dataset Card for Lexicap## Table of Contents\n- Dataset Description\n - Dataset Summary\n - Supported Tasks and Leaderboards\n - Languages\n- Dataset Structure\n - Data Instances\n - Data Fields\n - Data Splits\n- Dataset Creation\n - Curation Rationale\n - Source Data\n - Annotations\n - Personal and Sensitive Information\n- Considerations for Using the Data\n - Social Impact of Dataset\n - Discussion of Biases\n - Other Known Limitations\n- Additional Information\n - Dataset Curators\n - Licensing Information\n - Citation Information\n - Contributions## Dataset Description\n-## Dataset Structure### Data Instances\nTrain and test dataset.\nj### Data Fields## Dataset Creation### Curation Rationale### Source Data#### Initial Data Collection and Normalization#### Who are the source language producers?### Annotations#### Annotation process#### Who are the annotators?### Personal and Sensitive Information## Considerations for Using the Data### Social Impact of Dataset### Discussion of Biases### Other Known Limitations## Additional Information### Dataset Curators### Licensing Information### Contributions"
] |
d5dfe0d2fdc72e5d881a47cd3e8e8e57c2ca5b1b | # Dataset Card for AutoTrain Evaluator
This repository contains model predictions generated by [AutoTrain](https://huggingface.co/autotrain) for the following task and dataset:
* Task: Summarization
* Model: pszemraj/long-t5-tglobal-large-pubmed-3k-booksum-16384-WIP17
* Dataset: kmfoda/booksum
* Config: kmfoda--booksum
* Split: test
To run new evaluation jobs, visit Hugging Face's [automatic model evaluator](https://huggingface.co/spaces/autoevaluate/model-evaluator).
## Contributions
Thanks to [@pszemraj](https://huggingface.co/pszemraj) for evaluating this model. | autoevaluate/autoeval-eval-kmfoda__booksum-kmfoda__booksum-ba6080-1564655701 | [
"autotrain",
"evaluation",
"region:us"
] | 2022-09-27T07:14:27+00:00 | {"type": "predictions", "tags": ["autotrain", "evaluation"], "datasets": ["kmfoda/booksum"], "eval_info": {"task": "summarization", "model": "pszemraj/long-t5-tglobal-large-pubmed-3k-booksum-16384-WIP17", "metrics": [], "dataset_name": "kmfoda/booksum", "dataset_config": "kmfoda--booksum", "dataset_split": "test", "col_mapping": {"text": "chapter", "target": "summary_text"}}} | 2022-09-28T11:45:02+00:00 | [] | [] | TAGS
#autotrain #evaluation #region-us
| # Dataset Card for AutoTrain Evaluator
This repository contains model predictions generated by AutoTrain for the following task and dataset:
* Task: Summarization
* Model: pszemraj/long-t5-tglobal-large-pubmed-3k-booksum-16384-WIP17
* Dataset: kmfoda/booksum
* Config: kmfoda--booksum
* Split: test
To run new evaluation jobs, visit Hugging Face's automatic model evaluator.
## Contributions
Thanks to @pszemraj for evaluating this model. | [
"# Dataset Card for AutoTrain Evaluator\n\nThis repository contains model predictions generated by AutoTrain for the following task and dataset:\n\n* Task: Summarization\n* Model: pszemraj/long-t5-tglobal-large-pubmed-3k-booksum-16384-WIP17\n* Dataset: kmfoda/booksum\n* Config: kmfoda--booksum\n* Split: test\n\nTo run new evaluation jobs, visit Hugging Face's automatic model evaluator.",
"## Contributions\n\nThanks to @pszemraj for evaluating this model."
] | [
"TAGS\n#autotrain #evaluation #region-us \n",
"# Dataset Card for AutoTrain Evaluator\n\nThis repository contains model predictions generated by AutoTrain for the following task and dataset:\n\n* Task: Summarization\n* Model: pszemraj/long-t5-tglobal-large-pubmed-3k-booksum-16384-WIP17\n* Dataset: kmfoda/booksum\n* Config: kmfoda--booksum\n* Split: test\n\nTo run new evaluation jobs, visit Hugging Face's automatic model evaluator.",
"## Contributions\n\nThanks to @pszemraj for evaluating this model."
] | [
13,
112,
16
] | [
"passage: TAGS\n#autotrain #evaluation #region-us \n# Dataset Card for AutoTrain Evaluator\n\nThis repository contains model predictions generated by AutoTrain for the following task and dataset:\n\n* Task: Summarization\n* Model: pszemraj/long-t5-tglobal-large-pubmed-3k-booksum-16384-WIP17\n* Dataset: kmfoda/booksum\n* Config: kmfoda--booksum\n* Split: test\n\nTo run new evaluation jobs, visit Hugging Face's automatic model evaluator.## Contributions\n\nThanks to @pszemraj for evaluating this model."
] |
5b001451c8a86ecabf3e8aa1486ab7780534b48a | # Dataset Card for AutoTrain Evaluator
This repository contains model predictions generated by [AutoTrain](https://huggingface.co/autotrain) for the following task and dataset:
* Task: Summarization
* Model: pszemraj/long-t5-tglobal-large-pubmed-3k-booksum-16384-WIP17
* Dataset: billsum
* Config: default
* Split: test
To run new evaluation jobs, visit Hugging Face's [automatic model evaluator](https://huggingface.co/spaces/autoevaluate/model-evaluator).
## Contributions
Thanks to [@pszemraj](https://huggingface.co/pszemraj) for evaluating this model. | autoevaluate/autoeval-eval-billsum-default-37bdaa-1564755702 | [
"autotrain",
"evaluation",
"region:us"
] | 2022-09-27T07:14:31+00:00 | {"type": "predictions", "tags": ["autotrain", "evaluation"], "datasets": ["billsum"], "eval_info": {"task": "summarization", "model": "pszemraj/long-t5-tglobal-large-pubmed-3k-booksum-16384-WIP17", "metrics": [], "dataset_name": "billsum", "dataset_config": "default", "dataset_split": "test", "col_mapping": {"text": "text", "target": "summary"}}} | 2022-09-28T13:20:08+00:00 | [] | [] | TAGS
#autotrain #evaluation #region-us
| # Dataset Card for AutoTrain Evaluator
This repository contains model predictions generated by AutoTrain for the following task and dataset:
* Task: Summarization
* Model: pszemraj/long-t5-tglobal-large-pubmed-3k-booksum-16384-WIP17
* Dataset: billsum
* Config: default
* Split: test
To run new evaluation jobs, visit Hugging Face's automatic model evaluator.
## Contributions
Thanks to @pszemraj for evaluating this model. | [
"# Dataset Card for AutoTrain Evaluator\n\nThis repository contains model predictions generated by AutoTrain for the following task and dataset:\n\n* Task: Summarization\n* Model: pszemraj/long-t5-tglobal-large-pubmed-3k-booksum-16384-WIP17\n* Dataset: billsum\n* Config: default\n* Split: test\n\nTo run new evaluation jobs, visit Hugging Face's automatic model evaluator.",
"## Contributions\n\nThanks to @pszemraj for evaluating this model."
] | [
"TAGS\n#autotrain #evaluation #region-us \n",
"# Dataset Card for AutoTrain Evaluator\n\nThis repository contains model predictions generated by AutoTrain for the following task and dataset:\n\n* Task: Summarization\n* Model: pszemraj/long-t5-tglobal-large-pubmed-3k-booksum-16384-WIP17\n* Dataset: billsum\n* Config: default\n* Split: test\n\nTo run new evaluation jobs, visit Hugging Face's automatic model evaluator.",
"## Contributions\n\nThanks to @pszemraj for evaluating this model."
] | [
13,
102,
16
] | [
"passage: TAGS\n#autotrain #evaluation #region-us \n# Dataset Card for AutoTrain Evaluator\n\nThis repository contains model predictions generated by AutoTrain for the following task and dataset:\n\n* Task: Summarization\n* Model: pszemraj/long-t5-tglobal-large-pubmed-3k-booksum-16384-WIP17\n* Dataset: billsum\n* Config: default\n* Split: test\n\nTo run new evaluation jobs, visit Hugging Face's automatic model evaluator.## Contributions\n\nThanks to @pszemraj for evaluating this model."
] |
ee5cf7dc24900b58bd4a0f8c0de335ad4f7bdb4d | # Dataset Card for AutoTrain Evaluator
This repository contains model predictions generated by [AutoTrain](https://huggingface.co/autotrain) for the following task and dataset:
* Task: Summarization
* Model: pszemraj/long-t5-tglobal-large-pubmed-3k-booksum-16384-WIP17
* Dataset: launch/gov_report
* Config: plain_text
* Split: test
To run new evaluation jobs, visit Hugging Face's [automatic model evaluator](https://huggingface.co/spaces/autoevaluate/model-evaluator).
## Contributions
Thanks to [@pszemraj](https://huggingface.co/pszemraj) for evaluating this model. | autoevaluate/autoeval-eval-launch__gov_report-plain_text-45e121-1564955705 | [
"autotrain",
"evaluation",
"region:us"
] | 2022-09-27T07:14:49+00:00 | {"type": "predictions", "tags": ["autotrain", "evaluation"], "datasets": ["launch/gov_report"], "eval_info": {"task": "summarization", "model": "pszemraj/long-t5-tglobal-large-pubmed-3k-booksum-16384-WIP17", "metrics": [], "dataset_name": "launch/gov_report", "dataset_config": "plain_text", "dataset_split": "test", "col_mapping": {"text": "document", "target": "summary"}}} | 2022-09-27T22:02:40+00:00 | [] | [] | TAGS
#autotrain #evaluation #region-us
| # Dataset Card for AutoTrain Evaluator
This repository contains model predictions generated by AutoTrain for the following task and dataset:
* Task: Summarization
* Model: pszemraj/long-t5-tglobal-large-pubmed-3k-booksum-16384-WIP17
* Dataset: launch/gov_report
* Config: plain_text
* Split: test
To run new evaluation jobs, visit Hugging Face's automatic model evaluator.
## Contributions
Thanks to @pszemraj for evaluating this model. | [
"# Dataset Card for AutoTrain Evaluator\n\nThis repository contains model predictions generated by AutoTrain for the following task and dataset:\n\n* Task: Summarization\n* Model: pszemraj/long-t5-tglobal-large-pubmed-3k-booksum-16384-WIP17\n* Dataset: launch/gov_report\n* Config: plain_text\n* Split: test\n\nTo run new evaluation jobs, visit Hugging Face's automatic model evaluator.",
"## Contributions\n\nThanks to @pszemraj for evaluating this model."
] | [
"TAGS\n#autotrain #evaluation #region-us \n",
"# Dataset Card for AutoTrain Evaluator\n\nThis repository contains model predictions generated by AutoTrain for the following task and dataset:\n\n* Task: Summarization\n* Model: pszemraj/long-t5-tglobal-large-pubmed-3k-booksum-16384-WIP17\n* Dataset: launch/gov_report\n* Config: plain_text\n* Split: test\n\nTo run new evaluation jobs, visit Hugging Face's automatic model evaluator.",
"## Contributions\n\nThanks to @pszemraj for evaluating this model."
] | [
13,
107,
16
] | [
"passage: TAGS\n#autotrain #evaluation #region-us \n# Dataset Card for AutoTrain Evaluator\n\nThis repository contains model predictions generated by AutoTrain for the following task and dataset:\n\n* Task: Summarization\n* Model: pszemraj/long-t5-tglobal-large-pubmed-3k-booksum-16384-WIP17\n* Dataset: launch/gov_report\n* Config: plain_text\n* Split: test\n\nTo run new evaluation jobs, visit Hugging Face's automatic model evaluator.## Contributions\n\nThanks to @pszemraj for evaluating this model."
] |
b080eb0ef952f2c8283f6bf0186d2e03bf88b527 | # Dataset Card for AutoTrain Evaluator
This repository contains model predictions generated by [AutoTrain](https://huggingface.co/autotrain) for the following task and dataset:
* Task: Summarization
* Model: pszemraj/long-t5-tglobal-large-pubmed-3k-booksum-16384-WIP15
* Dataset: launch/gov_report
* Config: plain_text
* Split: test
To run new evaluation jobs, visit Hugging Face's [automatic model evaluator](https://huggingface.co/spaces/autoevaluate/model-evaluator).
## Contributions
Thanks to [@pszemraj](https://huggingface.co/pszemraj) for evaluating this model. | autoevaluate/autoeval-eval-launch__gov_report-plain_text-45e121-1564955706 | [
"autotrain",
"evaluation",
"region:us"
] | 2022-09-27T07:14:55+00:00 | {"type": "predictions", "tags": ["autotrain", "evaluation"], "datasets": ["launch/gov_report"], "eval_info": {"task": "summarization", "model": "pszemraj/long-t5-tglobal-large-pubmed-3k-booksum-16384-WIP15", "metrics": [], "dataset_name": "launch/gov_report", "dataset_config": "plain_text", "dataset_split": "test", "col_mapping": {"text": "document", "target": "summary"}}} | 2022-09-27T22:17:35+00:00 | [] | [] | TAGS
#autotrain #evaluation #region-us
| # Dataset Card for AutoTrain Evaluator
This repository contains model predictions generated by AutoTrain for the following task and dataset:
* Task: Summarization
* Model: pszemraj/long-t5-tglobal-large-pubmed-3k-booksum-16384-WIP15
* Dataset: launch/gov_report
* Config: plain_text
* Split: test
To run new evaluation jobs, visit Hugging Face's automatic model evaluator.
## Contributions
Thanks to @pszemraj for evaluating this model. | [
"# Dataset Card for AutoTrain Evaluator\n\nThis repository contains model predictions generated by AutoTrain for the following task and dataset:\n\n* Task: Summarization\n* Model: pszemraj/long-t5-tglobal-large-pubmed-3k-booksum-16384-WIP15\n* Dataset: launch/gov_report\n* Config: plain_text\n* Split: test\n\nTo run new evaluation jobs, visit Hugging Face's automatic model evaluator.",
"## Contributions\n\nThanks to @pszemraj for evaluating this model."
] | [
"TAGS\n#autotrain #evaluation #region-us \n",
"# Dataset Card for AutoTrain Evaluator\n\nThis repository contains model predictions generated by AutoTrain for the following task and dataset:\n\n* Task: Summarization\n* Model: pszemraj/long-t5-tglobal-large-pubmed-3k-booksum-16384-WIP15\n* Dataset: launch/gov_report\n* Config: plain_text\n* Split: test\n\nTo run new evaluation jobs, visit Hugging Face's automatic model evaluator.",
"## Contributions\n\nThanks to @pszemraj for evaluating this model."
] | [
13,
107,
16
] | [
"passage: TAGS\n#autotrain #evaluation #region-us \n# Dataset Card for AutoTrain Evaluator\n\nThis repository contains model predictions generated by AutoTrain for the following task and dataset:\n\n* Task: Summarization\n* Model: pszemraj/long-t5-tglobal-large-pubmed-3k-booksum-16384-WIP15\n* Dataset: launch/gov_report\n* Config: plain_text\n* Split: test\n\nTo run new evaluation jobs, visit Hugging Face's automatic model evaluator.## Contributions\n\nThanks to @pszemraj for evaluating this model."
] |
702c3ff0bee31d2479f7f98a1095210683c3fec0 | #### automatic-dissection
# **HuBMAP + HPA - Hacking the Human Body**
##### **Segment multi-organ functional tissue units in biopsy slides from several different organs.**
### **Overview**
When you think of "life hacks," normally youโd imagine productivity techniques. But how about the kind that helps you understand your body at a molecular level? It may be possible! Researchers must first determine the function and relationships among the 37 trillion cells that make up the human body. A better understanding of our cellular composition could help people live healthier, longer lives.
A previous Kaggle [competition](https://www.kaggle.com/c/hubmap-kidney-segmentation) aimed to annotate cell population neighborhoods that perform an organโs main physiologic function, also called functional tissue units (FTUs). Manually annotating FTUs (e.g., glomeruli in kidney or alveoli in the lung) is a time-consuming process. In the average kidney, there are over 1 million glomeruli FTUs. While there are existing cell and FTU segmentation methods, we want to push the boundaries by building algorithms that generalize across different organs and are robust across different dataset differences.
The [Human BioMolecular Atlas Program](https://hubmapconsortium.org/) (HuBMAP) is working to create a [Human Reference Atlas](https://www.nature.com/articles/s41556-021-00788-6) at the cellular level. Sponsored by the National Institutes of Health (NIH), HuBMAP and Indiana Universityโs Cyberinfrastructure for Network Science Center (CNS) have partnered with institutions across the globe for this endeavor. A major partner is the [Human Protein Atlas](https://www.proteinatlas.org/) (HPA), a Swedish research program aiming to map the protein expression in human cells, tissues, and organs, funded by the Knut and Alice Wallenberg Foundation.
In this repository, we [aim](https://www.kaggle.com/competitions/hubmap-organ-segmentation/) to identify and segment functional tissue units (FTUs) across five human organs. We have to build a model using a dataset of tissue section images, with the best submissions segmenting FTUs as accurately as possible.
If successful, we can help accelerate the worldโs understanding of the relationships between cell and tissue organization. With a better idea of the relationship of cells, researchers will have more insight into the function of cells that impact human health. Further, the Human Reference Atlas constructed by HuBMAP will be freely available for use by researchers and pharmaceutical companies alike, potentially improving and prolonging human life.
### **Dataset Description**
The goal is to identify the locations of each functional tissue unit (FTU) in biopsy slides from several different organs. The underlying data includes imagery from different sources prepared with different protocols at a variety of resolutions, reflecting typical challenges for working with medical data.
This project uses [data](https://huggingface.co/datasets/n1ghtf4l1/automatic-dissection) from two different consortia, the [Human Protein Atlas](https://www.proteinatlas.org/) (HPA) and [Human BioMolecular Atlas Program](https://hubmapconsortium.org/) (HuBMAP). The training dataset consists of data from public HPA data, the public test set is a combination of private HPA data and HuBMAP data, and the private test set contains only HuBMAP data. Adapting models to function properly when presented with data that was prepared using a different protocol will be one of the core challenges of this competition. While this is expected to make the problem more difficult, developing models that generalize is a key goal of this endeavor.
### **Files**
**[train/test].csv** Metadata for the train/test set. Only the first few rows of the test set are available for download.
- ```id``` - The image ID.
- ```organ``` - The organ that the biopsy sample was taken from.
- ```data_source``` - Whether the image was provided by HuBMAP or HPA.
- ```img_height``` - The height of the image in pixels.
- ```img_width``` - The width of the image in pixels.
- ```pixel_size``` - The height/width of a single pixel from this image in micrometers. All HPA images have a pixel size of 0.4 ยตm. For HuBMAP imagery the pixel size is 0.5 ยตm for kidney, 0.2290 ยตm for large intestine, 0.7562 ยตm for lung, 0.4945 ยตm for spleen, and 6.263 ยตm for prostate.
- ```tissue_thickness``` - The thickness of the biopsy sample in micrometers. All HPA images have a thickness of 4 ยตm. The HuBMAP samples have tissue slice thicknesses 10 ยตm for kidney, 8 ยตm for large intestine, 4 ยตm for spleen, 5 ยตm for lung, and 5 ยตm for prostate.
- ```rle``` - The target column. A run length encoded copy of the annotations. Provided for the training set only.
- ```age``` - The patient's age in years. Provided for the training set only.
- ```sex``` - The gender of the patient. Provided for the training set only.
**sample_submission.csv**
- ```id``` - The image ID.
- ```rle``` - A run length encoded mask of the FTUs in the image.
**[train/test]_images/** The images. Expect roughly 550 images in the hidden test set. All HPA images are 3000 x 3000 pixels with a tissue area within the image around 2500 x 2500 pixels. The HuBMAP images range in size from 4500x4500 down to 160x160 pixels. HPA samples were stained with antibodies visualized with 3,3'-diaminobenzidine (DAB) and counterstained with hematoxylin. HuBMAP images were prepared using Periodic acid-Schiff (PAS)/hematoxylin and eosin (H&E) stains. All images used have at least one FTU. All tissue data used in this competition is from healthy donors that pathologists identified as pathologically unremarkable tissue.
**train_annotations/** The annotations provided in the format of points that define the boundaries of the polygon masks of the FTUs.
| n1ghtf4l1/automatic-dissection | [
"license:mit",
"region:us"
] | 2022-09-27T08:45:43+00:00 | {"license": "mit"} | 2022-11-01T07:08:47+00:00 | [] | [] | TAGS
#license-mit #region-us
| #### automatic-dissection
# HuBMAP + HPA - Hacking the Human Body
##### Segment multi-organ functional tissue units in biopsy slides from several different organs.
### Overview
When you think of "life hacks," normally youโd imagine productivity techniques. But how about the kind that helps you understand your body at a molecular level? It may be possible! Researchers must first determine the function and relationships among the 37 trillion cells that make up the human body. A better understanding of our cellular composition could help people live healthier, longer lives.
A previous Kaggle competition aimed to annotate cell population neighborhoods that perform an organโs main physiologic function, also called functional tissue units (FTUs). Manually annotating FTUs (e.g., glomeruli in kidney or alveoli in the lung) is a time-consuming process. In the average kidney, there are over 1 million glomeruli FTUs. While there are existing cell and FTU segmentation methods, we want to push the boundaries by building algorithms that generalize across different organs and are robust across different dataset differences.
The Human BioMolecular Atlas Program (HuBMAP) is working to create a Human Reference Atlas at the cellular level. Sponsored by the National Institutes of Health (NIH), HuBMAP and Indiana Universityโs Cyberinfrastructure for Network Science Center (CNS) have partnered with institutions across the globe for this endeavor. A major partner is the Human Protein Atlas (HPA), a Swedish research program aiming to map the protein expression in human cells, tissues, and organs, funded by the Knut and Alice Wallenberg Foundation.
In this repository, we aim to identify and segment functional tissue units (FTUs) across five human organs. We have to build a model using a dataset of tissue section images, with the best submissions segmenting FTUs as accurately as possible.
If successful, we can help accelerate the worldโs understanding of the relationships between cell and tissue organization. With a better idea of the relationship of cells, researchers will have more insight into the function of cells that impact human health. Further, the Human Reference Atlas constructed by HuBMAP will be freely available for use by researchers and pharmaceutical companies alike, potentially improving and prolonging human life.
### Dataset Description
The goal is to identify the locations of each functional tissue unit (FTU) in biopsy slides from several different organs. The underlying data includes imagery from different sources prepared with different protocols at a variety of resolutions, reflecting typical challenges for working with medical data.
This project uses data from two different consortia, the Human Protein Atlas (HPA) and Human BioMolecular Atlas Program (HuBMAP). The training dataset consists of data from public HPA data, the public test set is a combination of private HPA data and HuBMAP data, and the private test set contains only HuBMAP data. Adapting models to function properly when presented with data that was prepared using a different protocol will be one of the core challenges of this competition. While this is expected to make the problem more difficult, developing models that generalize is a key goal of this endeavor.
### Files
[train/test].csv Metadata for the train/test set. Only the first few rows of the test set are available for download.
- - The image ID.
- - The organ that the biopsy sample was taken from.
- - Whether the image was provided by HuBMAP or HPA.
- - The height of the image in pixels.
- - The width of the image in pixels.
- - The height/width of a single pixel from this image in micrometers. All HPA images have a pixel size of 0.4 ยตm. For HuBMAP imagery the pixel size is 0.5 ยตm for kidney, 0.2290 ยตm for large intestine, 0.7562 ยตm for lung, 0.4945 ยตm for spleen, and 6.263 ยตm for prostate.
- - The thickness of the biopsy sample in micrometers. All HPA images have a thickness of 4 ยตm. The HuBMAP samples have tissue slice thicknesses 10 ยตm for kidney, 8 ยตm for large intestine, 4 ยตm for spleen, 5 ยตm for lung, and 5 ยตm for prostate.
- - The target column. A run length encoded copy of the annotations. Provided for the training set only.
- - The patient's age in years. Provided for the training set only.
- - The gender of the patient. Provided for the training set only.
sample_submission.csv
- - The image ID.
- - A run length encoded mask of the FTUs in the image.
[train/test]_images/ The images. Expect roughly 550 images in the hidden test set. All HPA images are 3000 x 3000 pixels with a tissue area within the image around 2500 x 2500 pixels. The HuBMAP images range in size from 4500x4500 down to 160x160 pixels. HPA samples were stained with antibodies visualized with 3,3'-diaminobenzidine (DAB) and counterstained with hematoxylin. HuBMAP images were prepared using Periodic acid-Schiff (PAS)/hematoxylin and eosin (H&E) stains. All images used have at least one FTU. All tissue data used in this competition is from healthy donors that pathologists identified as pathologically unremarkable tissue.
train_annotations/ The annotations provided in the format of points that define the boundaries of the polygon masks of the FTUs.
| [
"#### automatic-dissection",
"# HuBMAP + HPA - Hacking the Human Body",
"##### Segment multi-organ functional tissue units in biopsy slides from several different organs.",
"### Overview\n\nWhen you think of \"life hacks,\" normally youโd imagine productivity techniques. But how about the kind that helps you understand your body at a molecular level? It may be possible! Researchers must first determine the function and relationships among the 37 trillion cells that make up the human body. A better understanding of our cellular composition could help people live healthier, longer lives.\n\nA previous Kaggle competition aimed to annotate cell population neighborhoods that perform an organโs main physiologic function, also called functional tissue units (FTUs). Manually annotating FTUs (e.g., glomeruli in kidney or alveoli in the lung) is a time-consuming process. In the average kidney, there are over 1 million glomeruli FTUs. While there are existing cell and FTU segmentation methods, we want to push the boundaries by building algorithms that generalize across different organs and are robust across different dataset differences.\n\nThe Human BioMolecular Atlas Program (HuBMAP) is working to create a Human Reference Atlas at the cellular level. Sponsored by the National Institutes of Health (NIH), HuBMAP and Indiana Universityโs Cyberinfrastructure for Network Science Center (CNS) have partnered with institutions across the globe for this endeavor. A major partner is the Human Protein Atlas (HPA), a Swedish research program aiming to map the protein expression in human cells, tissues, and organs, funded by the Knut and Alice Wallenberg Foundation.\n\nIn this repository, we aim to identify and segment functional tissue units (FTUs) across five human organs. We have to build a model using a dataset of tissue section images, with the best submissions segmenting FTUs as accurately as possible.\n\nIf successful, we can help accelerate the worldโs understanding of the relationships between cell and tissue organization. With a better idea of the relationship of cells, researchers will have more insight into the function of cells that impact human health. Further, the Human Reference Atlas constructed by HuBMAP will be freely available for use by researchers and pharmaceutical companies alike, potentially improving and prolonging human life.",
"### Dataset Description\n\nThe goal is to identify the locations of each functional tissue unit (FTU) in biopsy slides from several different organs. The underlying data includes imagery from different sources prepared with different protocols at a variety of resolutions, reflecting typical challenges for working with medical data.\n\nThis project uses data from two different consortia, the Human Protein Atlas (HPA) and Human BioMolecular Atlas Program (HuBMAP). The training dataset consists of data from public HPA data, the public test set is a combination of private HPA data and HuBMAP data, and the private test set contains only HuBMAP data. Adapting models to function properly when presented with data that was prepared using a different protocol will be one of the core challenges of this competition. While this is expected to make the problem more difficult, developing models that generalize is a key goal of this endeavor.",
"### Files\n\n[train/test].csv Metadata for the train/test set. Only the first few rows of the test set are available for download.\n\n- - The image ID.\n- - The organ that the biopsy sample was taken from.\n- - Whether the image was provided by HuBMAP or HPA.\n- - The height of the image in pixels.\n- - The width of the image in pixels.\n- - The height/width of a single pixel from this image in micrometers. All HPA images have a pixel size of 0.4 ยตm. For HuBMAP imagery the pixel size is 0.5 ยตm for kidney, 0.2290 ยตm for large intestine, 0.7562 ยตm for lung, 0.4945 ยตm for spleen, and 6.263 ยตm for prostate.\n- - The thickness of the biopsy sample in micrometers. All HPA images have a thickness of 4 ยตm. The HuBMAP samples have tissue slice thicknesses 10 ยตm for kidney, 8 ยตm for large intestine, 4 ยตm for spleen, 5 ยตm for lung, and 5 ยตm for prostate.\n- - The target column. A run length encoded copy of the annotations. Provided for the training set only.\n- - The patient's age in years. Provided for the training set only.\n- - The gender of the patient. Provided for the training set only.\n\nsample_submission.csv\n\n- - The image ID.\n- - A run length encoded mask of the FTUs in the image.\n\n[train/test]_images/ The images. Expect roughly 550 images in the hidden test set. All HPA images are 3000 x 3000 pixels with a tissue area within the image around 2500 x 2500 pixels. The HuBMAP images range in size from 4500x4500 down to 160x160 pixels. HPA samples were stained with antibodies visualized with 3,3'-diaminobenzidine (DAB) and counterstained with hematoxylin. HuBMAP images were prepared using Periodic acid-Schiff (PAS)/hematoxylin and eosin (H&E) stains. All images used have at least one FTU. All tissue data used in this competition is from healthy donors that pathologists identified as pathologically unremarkable tissue.\n\ntrain_annotations/ The annotations provided in the format of points that define the boundaries of the polygon masks of the FTUs."
] | [
"TAGS\n#license-mit #region-us \n",
"#### automatic-dissection",
"# HuBMAP + HPA - Hacking the Human Body",
"##### Segment multi-organ functional tissue units in biopsy slides from several different organs.",
"### Overview\n\nWhen you think of \"life hacks,\" normally youโd imagine productivity techniques. But how about the kind that helps you understand your body at a molecular level? It may be possible! Researchers must first determine the function and relationships among the 37 trillion cells that make up the human body. A better understanding of our cellular composition could help people live healthier, longer lives.\n\nA previous Kaggle competition aimed to annotate cell population neighborhoods that perform an organโs main physiologic function, also called functional tissue units (FTUs). Manually annotating FTUs (e.g., glomeruli in kidney or alveoli in the lung) is a time-consuming process. In the average kidney, there are over 1 million glomeruli FTUs. While there are existing cell and FTU segmentation methods, we want to push the boundaries by building algorithms that generalize across different organs and are robust across different dataset differences.\n\nThe Human BioMolecular Atlas Program (HuBMAP) is working to create a Human Reference Atlas at the cellular level. Sponsored by the National Institutes of Health (NIH), HuBMAP and Indiana Universityโs Cyberinfrastructure for Network Science Center (CNS) have partnered with institutions across the globe for this endeavor. A major partner is the Human Protein Atlas (HPA), a Swedish research program aiming to map the protein expression in human cells, tissues, and organs, funded by the Knut and Alice Wallenberg Foundation.\n\nIn this repository, we aim to identify and segment functional tissue units (FTUs) across five human organs. We have to build a model using a dataset of tissue section images, with the best submissions segmenting FTUs as accurately as possible.\n\nIf successful, we can help accelerate the worldโs understanding of the relationships between cell and tissue organization. With a better idea of the relationship of cells, researchers will have more insight into the function of cells that impact human health. Further, the Human Reference Atlas constructed by HuBMAP will be freely available for use by researchers and pharmaceutical companies alike, potentially improving and prolonging human life.",
"### Dataset Description\n\nThe goal is to identify the locations of each functional tissue unit (FTU) in biopsy slides from several different organs. The underlying data includes imagery from different sources prepared with different protocols at a variety of resolutions, reflecting typical challenges for working with medical data.\n\nThis project uses data from two different consortia, the Human Protein Atlas (HPA) and Human BioMolecular Atlas Program (HuBMAP). The training dataset consists of data from public HPA data, the public test set is a combination of private HPA data and HuBMAP data, and the private test set contains only HuBMAP data. Adapting models to function properly when presented with data that was prepared using a different protocol will be one of the core challenges of this competition. While this is expected to make the problem more difficult, developing models that generalize is a key goal of this endeavor.",
"### Files\n\n[train/test].csv Metadata for the train/test set. Only the first few rows of the test set are available for download.\n\n- - The image ID.\n- - The organ that the biopsy sample was taken from.\n- - Whether the image was provided by HuBMAP or HPA.\n- - The height of the image in pixels.\n- - The width of the image in pixels.\n- - The height/width of a single pixel from this image in micrometers. All HPA images have a pixel size of 0.4 ยตm. For HuBMAP imagery the pixel size is 0.5 ยตm for kidney, 0.2290 ยตm for large intestine, 0.7562 ยตm for lung, 0.4945 ยตm for spleen, and 6.263 ยตm for prostate.\n- - The thickness of the biopsy sample in micrometers. All HPA images have a thickness of 4 ยตm. The HuBMAP samples have tissue slice thicknesses 10 ยตm for kidney, 8 ยตm for large intestine, 4 ยตm for spleen, 5 ยตm for lung, and 5 ยตm for prostate.\n- - The target column. A run length encoded copy of the annotations. Provided for the training set only.\n- - The patient's age in years. Provided for the training set only.\n- - The gender of the patient. Provided for the training set only.\n\nsample_submission.csv\n\n- - The image ID.\n- - A run length encoded mask of the FTUs in the image.\n\n[train/test]_images/ The images. Expect roughly 550 images in the hidden test set. All HPA images are 3000 x 3000 pixels with a tissue area within the image around 2500 x 2500 pixels. The HuBMAP images range in size from 4500x4500 down to 160x160 pixels. HPA samples were stained with antibodies visualized with 3,3'-diaminobenzidine (DAB) and counterstained with hematoxylin. HuBMAP images were prepared using Periodic acid-Schiff (PAS)/hematoxylin and eosin (H&E) stains. All images used have at least one FTU. All tissue data used in this competition is from healthy donors that pathologists identified as pathologically unremarkable tissue.\n\ntrain_annotations/ The annotations provided in the format of points that define the boundaries of the polygon masks of the FTUs."
] | [
11,
6,
13,
23,
497,
200,
574
] | [
"passage: TAGS\n#license-mit #region-us \n#### automatic-dissection# HuBMAP + HPA - Hacking the Human Body##### Segment multi-organ functional tissue units in biopsy slides from several different organs.",
"passage: ### Overview\n\nWhen you think of \"life hacks,\" normally youโd imagine productivity techniques. But how about the kind that helps you understand your body at a molecular level? It may be possible! Researchers must first determine the function and relationships among the 37 trillion cells that make up the human body. A better understanding of our cellular composition could help people live healthier, longer lives.\n\nA previous Kaggle competition aimed to annotate cell population neighborhoods that perform an organโs main physiologic function, also called functional tissue units (FTUs). Manually annotating FTUs (e.g., glomeruli in kidney or alveoli in the lung) is a time-consuming process. In the average kidney, there are over 1 million glomeruli FTUs. While there are existing cell and FTU segmentation methods, we want to push the boundaries by building algorithms that generalize across different organs and are robust across different dataset differences.\n\nThe Human BioMolecular Atlas Program (HuBMAP) is working to create a Human Reference Atlas at the cellular level. Sponsored by the National Institutes of Health (NIH), HuBMAP and Indiana Universityโs Cyberinfrastructure for Network Science Center (CNS) have partnered with institutions across the globe for this endeavor. A major partner is the Human Protein Atlas (HPA), a Swedish research program aiming to map the protein expression in human cells, tissues, and organs, funded by the Knut and Alice Wallenberg Foundation.\n\nIn this repository, we aim to identify and segment functional tissue units (FTUs) across five human organs. We have to build a model using a dataset of tissue section images, with the best submissions segmenting FTUs as accurately as possible.\n\nIf successful, we can help accelerate the worldโs understanding of the relationships between cell and tissue organization. With a better idea of the relationship of cells, researchers will have more insight into the function of cells that impact human health. Further, the Human Reference Atlas constructed by HuBMAP will be freely available for use by researchers and pharmaceutical companies alike, potentially improving and prolonging human life.### Dataset Description\n\nThe goal is to identify the locations of each functional tissue unit (FTU) in biopsy slides from several different organs. The underlying data includes imagery from different sources prepared with different protocols at a variety of resolutions, reflecting typical challenges for working with medical data.\n\nThis project uses data from two different consortia, the Human Protein Atlas (HPA) and Human BioMolecular Atlas Program (HuBMAP). The training dataset consists of data from public HPA data, the public test set is a combination of private HPA data and HuBMAP data, and the private test set contains only HuBMAP data. Adapting models to function properly when presented with data that was prepared using a different protocol will be one of the core challenges of this competition. While this is expected to make the problem more difficult, developing models that generalize is a key goal of this endeavor."
] |
3b0559e997b2dc1a5eb080364ba2420e29e4dd2d |
Converted to json version of dataset from [Koziev/NLP_Datasets](https://github.com/Koziev/NLP_Datasets/blob/master/Conversations/Data/extract_dialogues_from_anekdots.tar.xz) | artemsnegirev/dialogs_from_jokes | [
"task_categories:conversational",
"task_ids:dialogue-generation",
"multilinguality:monolingual",
"size_categories:100K<n<1M",
"language:ru",
"license:cc0-1.0",
"region:us"
] | 2022-09-27T10:32:40+00:00 | {"language": ["ru"], "license": "cc0-1.0", "multilinguality": ["monolingual"], "size_categories": ["100K<n<1M"], "task_categories": ["conversational"], "task_ids": ["dialogue-generation"], "pretty_name": "Dialogs from Jokes"} | 2022-09-27T10:43:32+00:00 | [] | [
"ru"
] | TAGS
#task_categories-conversational #task_ids-dialogue-generation #multilinguality-monolingual #size_categories-100K<n<1M #language-Russian #license-cc0-1.0 #region-us
|
Converted to json version of dataset from Koziev/NLP_Datasets | [] | [
"TAGS\n#task_categories-conversational #task_ids-dialogue-generation #multilinguality-monolingual #size_categories-100K<n<1M #language-Russian #license-cc0-1.0 #region-us \n"
] | [
61
] | [
"passage: TAGS\n#task_categories-conversational #task_ids-dialogue-generation #multilinguality-monolingual #size_categories-100K<n<1M #language-Russian #license-cc0-1.0 #region-us \n"
] |
5500a07ad0e88dae61f0f78a46f17751d5a95c7f |
```sh
git clone https://github.com/rust-bio/rust-bio-tools
rm -f RustBioGPT-validate.csv && for i in `find . -name "*.rs"`;do paste -d "," <(echo "rust-bio-tools"|perl -pe "s/(.+)/\"\1\"/g") <(echo $i|perl -pe "s/(.+)/\"\1\"/g") <(perl -pe "s/\n/\\\n/g" $i|perl -pe s"/\"/\'/g" |perl -pe "s/(.+)/\"\1\"/g") <(echo "mit"|perl -pe "s/(.+)/\"\1\"/g") >> RustBioGPT-validate.csv; done
sed -i '1i "repo_name","path","content","license"' RustBioGPT-validate.csv
``` | jelber2/RustBioGPT-valid | [
"license:mit",
"region:us"
] | 2022-09-27T10:52:42+00:00 | {"license": "mit"} | 2022-09-27T11:01:37+00:00 | [] | [] | TAGS
#license-mit #region-us
| [] | [
"TAGS\n#license-mit #region-us \n"
] | [
11
] | [
"passage: TAGS\n#license-mit #region-us \n"
] |
|
58684b7a75ae57ed0dbcfcb87bdbd8ff3541aade |
# laion2B-multi-chinese-subset
- Github: [Fengshenbang-LM](https://github.com/IDEA-CCNL/Fengshenbang-LM)
- Docs: [Fengshenbang-Docs](https://fengshenbang-doc.readthedocs.io/)
## ็ฎไป Brief Introduction
ๅ่ชLaion2Bๅค่ฏญ่จๅคๆจกๆๆฐๆฎ้ไธญ็ไธญๆ้จๅ๏ผไธๅ
ฑ143Mไธชๅพๆๅฏนใ
A subset from Laion2B (a multimodal dataset), around 143M image-text pairs (only Chinese).
## ๆฐๆฎ้ไฟกๆฏ Dataset Information
ๅคง็บฆไธๅ
ฑ143Mไธชไธญๆๅพๆๅฏนใๅคง็บฆๅ ็จ19GB็ฉบ้ด๏ผไป
ไป
ๆฏurl็ญๆๆฌไฟกๆฏ๏ผไธๅ
ๅซๅพ็๏ผใ
- Homepage: [laion-5b](https://laion.ai/blog/laion-5b/)
- Huggingface: [laion/laion2B-multi](https://huggingface.co/datasets/laion/laion2B-multi)
## ไธ่ฝฝ Download
```bash
mkdir laion2b_chinese_release && cd laion2b_chinese_release
for i in {00000..00012}; do wget https://huggingface.co/datasets/IDEA-CCNL/laion2B-multi-chinese-subset/resolve/main/data/train-$i-of-00013.parquet; done
cd ..
```
## Lisence
CC-BY-4.0
## ๅผ็จ Citation
ๅฆๆๆจๅจๆจ็ๅทฅไฝไธญไฝฟ็จไบๆไปฌ็ๆจกๅ๏ผๅฏไปฅๅผ็จๆไปฌ็[่ฎบๆ](https://arxiv.org/abs/2209.02970)๏ผ
If you are using the resource for your work, please cite the our [paper](https://arxiv.org/abs/2209.02970):
```text
@article{fengshenbang,
author = {Jiaxing Zhang and Ruyi Gan and Junjie Wang and Yuxiang Zhang and Lin Zhang and Ping Yang and Xinyu Gao and Ziwei Wu and Xiaoqun Dong and Junqing He and Jianheng Zhuo and Qi Yang and Yongfeng Huang and Xiayu Li and Yanghan Wu and Junyu Lu and Xinyu Zhu and Weifeng Chen and Ting Han and Kunhao Pan and Rui Wang and Hao Wang and Xiaojun Wu and Zhongshen Zeng and Chongpei Chen},
title = {Fengshenbang 1.0: Being the Foundation of Chinese Cognitive Intelligence},
journal = {CoRR},
volume = {abs/2209.02970},
year = {2022}
}
```
ไนๅฏไปฅๅผ็จๆไปฌ็[็ฝ็ซ](https://github.com/IDEA-CCNL/Fengshenbang-LM/):
You can also cite our [website](https://github.com/IDEA-CCNL/Fengshenbang-LM/):
```text
@misc{Fengshenbang-LM,
title={Fengshenbang-LM},
author={IDEA-CCNL},
year={2021},
howpublished={\url{https://github.com/IDEA-CCNL/Fengshenbang-LM}},
}
| IDEA-CCNL/laion2B-multi-chinese-subset | [
"task_categories:feature-extraction",
"annotations_creators:crowdsourced",
"language_creators:crowdsourced",
"multilinguality:monolingual",
"language:zh",
"license:cc-by-4.0",
"arxiv:2209.02970",
"region:us"
] | 2022-09-27T11:22:38+00:00 | {"annotations_creators": ["crowdsourced"], "language_creators": ["crowdsourced"], "language": ["zh"], "license": ["cc-by-4.0"], "multilinguality": ["monolingual"], "task_categories": ["feature-extraction"], "pretty_name": "laion2B-multi-chinese-subset"} | 2023-04-06T05:32:18+00:00 | [
"2209.02970"
] | [
"zh"
] | TAGS
#task_categories-feature-extraction #annotations_creators-crowdsourced #language_creators-crowdsourced #multilinguality-monolingual #language-Chinese #license-cc-by-4.0 #arxiv-2209.02970 #region-us
|
# laion2B-multi-chinese-subset
- Github: Fengshenbang-LM
- Docs: Fengshenbang-Docs
## ็ฎไป Brief Introduction
ๅ่ชLaion2Bๅค่ฏญ่จๅคๆจกๆๆฐๆฎ้ไธญ็ไธญๆ้จๅ๏ผไธๅ
ฑ143Mไธชๅพๆๅฏนใ
A subset from Laion2B (a multimodal dataset), around 143M image-text pairs (only Chinese).
## ๆฐๆฎ้ไฟกๆฏ Dataset Information
ๅคง็บฆไธๅ
ฑ143Mไธชไธญๆๅพๆๅฏนใๅคง็บฆๅ ็จ19GB็ฉบ้ด๏ผไป
ไป
ๆฏurl็ญๆๆฌไฟกๆฏ๏ผไธๅ
ๅซๅพ็๏ผใ
- Homepage: laion-5b
- Huggingface: laion/laion2B-multi
## ไธ่ฝฝ Download
## Lisence
CC-BY-4.0
## ๅผ็จ Citation
ๅฆๆๆจๅจๆจ็ๅทฅไฝไธญไฝฟ็จไบๆไปฌ็ๆจกๅ๏ผๅฏไปฅๅผ็จๆไปฌ็่ฎบๆ๏ผ
If you are using the resource for your work, please cite the our paper:
ไนๅฏไปฅๅผ็จๆไปฌ็็ฝ็ซ:
You can also cite our website:
'''text
@misc{Fengshenbang-LM,
title={Fengshenbang-LM},
author={IDEA-CCNL},
year={2021},
howpublished={\url{URL
}
| [
"# laion2B-multi-chinese-subset\n\n- Github: Fengshenbang-LM\n- Docs: Fengshenbang-Docs",
"## ็ฎไป Brief Introduction\n\nๅ่ชLaion2Bๅค่ฏญ่จๅคๆจกๆๆฐๆฎ้ไธญ็ไธญๆ้จๅ๏ผไธๅ
ฑ143Mไธชๅพๆๅฏนใ\n\nA subset from Laion2B (a multimodal dataset), around 143M image-text pairs (only Chinese).",
"## ๆฐๆฎ้ไฟกๆฏ Dataset Information\n\nๅคง็บฆไธๅ
ฑ143Mไธชไธญๆๅพๆๅฏนใๅคง็บฆๅ ็จ19GB็ฉบ้ด๏ผไป
ไป
ๆฏurl็ญๆๆฌไฟกๆฏ๏ผไธๅ
ๅซๅพ็๏ผใ\n\n- Homepage: laion-5b\n- Huggingface: laion/laion2B-multi",
"## ไธ่ฝฝ Download",
"## Lisence\n\nCC-BY-4.0",
"## ๅผ็จ Citation\n\nๅฆๆๆจๅจๆจ็ๅทฅไฝไธญไฝฟ็จไบๆไปฌ็ๆจกๅ๏ผๅฏไปฅๅผ็จๆไปฌ็่ฎบๆ๏ผ\n\nIf you are using the resource for your work, please cite the our paper:\n\n\n\nไนๅฏไปฅๅผ็จๆไปฌ็็ฝ็ซ:\n\nYou can also cite our website:\n\n'''text\n@misc{Fengshenbang-LM,\n title={Fengshenbang-LM},\n author={IDEA-CCNL},\n year={2021},\n howpublished={\\url{URL\n}"
] | [
"TAGS\n#task_categories-feature-extraction #annotations_creators-crowdsourced #language_creators-crowdsourced #multilinguality-monolingual #language-Chinese #license-cc-by-4.0 #arxiv-2209.02970 #region-us \n",
"# laion2B-multi-chinese-subset\n\n- Github: Fengshenbang-LM\n- Docs: Fengshenbang-Docs",
"## ็ฎไป Brief Introduction\n\nๅ่ชLaion2Bๅค่ฏญ่จๅคๆจกๆๆฐๆฎ้ไธญ็ไธญๆ้จๅ๏ผไธๅ
ฑ143Mไธชๅพๆๅฏนใ\n\nA subset from Laion2B (a multimodal dataset), around 143M image-text pairs (only Chinese).",
"## ๆฐๆฎ้ไฟกๆฏ Dataset Information\n\nๅคง็บฆไธๅ
ฑ143Mไธชไธญๆๅพๆๅฏนใๅคง็บฆๅ ็จ19GB็ฉบ้ด๏ผไป
ไป
ๆฏurl็ญๆๆฌไฟกๆฏ๏ผไธๅ
ๅซๅพ็๏ผใ\n\n- Homepage: laion-5b\n- Huggingface: laion/laion2B-multi",
"## ไธ่ฝฝ Download",
"## Lisence\n\nCC-BY-4.0",
"## ๅผ็จ Citation\n\nๅฆๆๆจๅจๆจ็ๅทฅไฝไธญไฝฟ็จไบๆไปฌ็ๆจกๅ๏ผๅฏไปฅๅผ็จๆไปฌ็่ฎบๆ๏ผ\n\nIf you are using the resource for your work, please cite the our paper:\n\n\n\nไนๅฏไปฅๅผ็จๆไปฌ็็ฝ็ซ:\n\nYou can also cite our website:\n\n'''text\n@misc{Fengshenbang-LM,\n title={Fengshenbang-LM},\n author={IDEA-CCNL},\n year={2021},\n howpublished={\\url{URL\n}"
] | [
72,
33,
61,
58,
4,
8,
100
] | [
"passage: TAGS\n#task_categories-feature-extraction #annotations_creators-crowdsourced #language_creators-crowdsourced #multilinguality-monolingual #language-Chinese #license-cc-by-4.0 #arxiv-2209.02970 #region-us \n# laion2B-multi-chinese-subset\n\n- Github: Fengshenbang-LM\n- Docs: Fengshenbang-Docs## ็ฎไป Brief Introduction\n\nๅ่ชLaion2Bๅค่ฏญ่จๅคๆจกๆๆฐๆฎ้ไธญ็ไธญๆ้จๅ๏ผไธๅ
ฑ143Mไธชๅพๆๅฏนใ\n\nA subset from Laion2B (a multimodal dataset), around 143M image-text pairs (only Chinese).## ๆฐๆฎ้ไฟกๆฏ Dataset Information\n\nๅคง็บฆไธๅ
ฑ143Mไธชไธญๆๅพๆๅฏนใๅคง็บฆๅ ็จ19GB็ฉบ้ด๏ผไป
ไป
ๆฏurl็ญๆๆฌไฟกๆฏ๏ผไธๅ
ๅซๅพ็๏ผใ\n\n- Homepage: laion-5b\n- Huggingface: laion/laion2B-multi## ไธ่ฝฝ Download## Lisence\n\nCC-BY-4.0## ๅผ็จ Citation\n\nๅฆๆๆจๅจๆจ็ๅทฅไฝไธญไฝฟ็จไบๆไปฌ็ๆจกๅ๏ผๅฏไปฅๅผ็จๆไปฌ็่ฎบๆ๏ผ\n\nIf you are using the resource for your work, please cite the our paper:\n\n\n\nไนๅฏไปฅๅผ็จๆไปฌ็็ฝ็ซ:\n\nYou can also cite our website:\n\n'''text\n@misc{Fengshenbang-LM,\n title={Fengshenbang-LM},\n author={IDEA-CCNL},\n year={2021},\n howpublished={\\url{URL\n}"
] |
4d17ebae87690692e4ce9f102f35d28fa7ed5b66 |
# Dataset Card for WinoGAViL
- [Dataset Description](#dataset-description)
- [Supported Tasks and Leaderboards](#supported-tasks-and-leaderboards)
- [Colab notebook code for Winogavil evaluation with CLIP](#colab-notebook-code-for-winogavil-evaluation-with-clip)
- [Languages](#languages)
- [Dataset Structure](#dataset-structure)
- [Data Fields](#data-fields)
- [Data Splits](#data-splits)
- [Dataset Creation](#dataset-creation)
- [Considerations for Using the Data](#considerations-for-using-the-data)
- [Licensing Information](#licensing-information)
- [Citation Information](#citation-information)
## Dataset Description
WinoGAViL is a challenging dataset for evaluating vision-and-language commonsense reasoning abilities. Given a set of images, a cue, and a number K, the task is to select the K images that best fits the association. This dataset was collected via the WinoGAViL online game to collect vision-and-language associations, (e.g., werewolves to a full moon). Inspired by the popular card game Codenames, a spymaster gives a textual cue related to several visual candidates, and another player has to identify them. Human players are rewarded for creating associations that are challenging for a rival AI model but still solvable by other human players. We evaluate several state-of-the-art vision-and-language models, finding that they are intuitive for humans (>90% Jaccard index) but challenging for state-of-the-art AI models, where the best model (ViLT) achieves a score of 52%, succeeding mostly where the cue is visually salient. Our analysis as well as the feedback we collect from players indicate that the collected associations require diverse reasoning skills, including general knowledge, common sense, abstraction, and more.
- **Homepage:**
https://winogavil.github.io/
- **Colab**
https://colab.research.google.com/drive/19qcPovniLj2PiLlP75oFgsK-uhTr6SSi
- **Repository:**
https://github.com/WinoGAViL/WinoGAViL-experiments/
- **Paper:**
https://arxiv.org/abs/2207.12576
- **Leaderboard:**
https://winogavil.github.io/leaderboard
- **Point of Contact:**
[email protected]; [email protected]
### Supported Tasks and Leaderboards
https://winogavil.github.io/leaderboard.
https://paperswithcode.com/dataset/winogavil.
## Colab notebook code for Winogavil evaluation with CLIP
https://colab.research.google.com/drive/19qcPovniLj2PiLlP75oFgsK-uhTr6SSi
### Languages
English.
## Dataset Structure
### Data Fields
candidates (list): ["bison", "shelter", "beard", "flea", "cattle", "shave"] - list of image candidates.
cue (string): pogonophile - the generated cue.
associations (string): ["bison", "beard", "shave"] - the images associated with the cue selected by the user.
score_fool_the_ai (int64): 80 - the spymaster score (100 - model score) for fooling the AI, with CLIP RN50 model.
num_associations (int64): 3 - The number of images selected as associative with the cue.
num_candidates (int64): 6 - the number of total candidates.
solvers_jaccard_mean (float64): 1.0 - three solvers scores average on the generated association instance.
solvers_jaccard_std (float64): 1.0 - three solvers scores standard deviation on the generated association instance
ID (int64): 367 - association ID.
### Data Splits
There is a single TEST split. In the accompanied paper and code we sample it to create different training sets, but the intended use is to use winogavil as a test set.
There are different number of candidates, which creates different difficulty levels:
-- With 5 candidates, random model expected score is 38%.
-- With 6 candidates, random model expected score is 34%.
-- With 10 candidates, random model expected score is 24%.
-- With 12 candidates, random model expected score is 19%.
<details>
<summary>Why random chance for success with 5 candidates is 38%?</summary>
It is a binomial distribution probability calculation.
Assuming N=5 candidates, and K=2 associations, there could be three events:
(1) The probability for a random guess is correct in 0 associations is 0.3 (elaborate below), and the Jaccard index is 0 (there is no intersection between the correct labels and the wrong guesses). Therefore the expected random score is 0.
(2) The probability for a random guess is correct in 1 associations is 0.6, and the Jaccard index is 0.33 (intersection=1, union=3, one of the correct guesses, and one of the wrong guesses). Therefore the expected random score is 0.6*0.33 = 0.198.
(3) The probability for a random guess is correct in 2 associations is 0.1, and the Jaccard index is 1 (intersection=2, union=2). Therefore the expected random score is 0.1*1 = 0.1.
* Together, when K=2, the expected score is 0+0.198+0.1 = 0.298.
To calculate (1), the first guess needs to be wrong. There are 3 "wrong" guesses and 5 candidates, so the probability for it is 3/5. The next guess should also be wrong. Now there are only 2 "wrong" guesses, and 4 candidates, so the probability for it is 2/4. Multiplying 3/5 * 2/4 = 0.3.
Same goes for (2) and (3).
Now we can perform the same calculation with K=3 associations.
Assuming N=5 candidates, and K=3 associations, there could be four events:
(4) The probability for a random guess is correct in 0 associations is 0, and the Jaccard index is 0. Therefore the expected random score is 0.
(5) The probability for a random guess is correct in 1 associations is 0.3, and the Jaccard index is 0.2 (intersection=1, union=4). Therefore the expected random score is 0.3*0.2 = 0.06.
(6) The probability for a random guess is correct in 2 associations is 0.6, and the Jaccard index is 0.5 (intersection=2, union=4). Therefore the expected random score is 0.6*5 = 0.3.
(7) The probability for a random guess is correct in 3 associations is 0.1, and the Jaccard index is 1 (intersection=3, union=3). Therefore the expected random score is 0.1*1 = 0.1.
* Together, when K=3, the expected score is 0+0.06+0.3+0.1 = 0.46.
Taking the average of 0.298 and 0.46 we reach 0.379.
Same process can be recalculated with 6 candidates (and K=2,3,4), 10 candidates (and K=2,3,4,5) and 123 candidates (and K=2,3,4,5,6).
</details>
## Dataset Creation
Inspired by the popular card game Codenames, a โspymasterโ gives a textual cue related to several visual candidates, and another player has to identify them. Human players are rewarded for creating
associations that are challenging for a rival AI model but still solvable by other
human players.
### Annotations
#### Annotation process
We paid Amazon Mechanical Turk Workers to play our game.
## Considerations for Using the Data
All associations were obtained with human annotators.
### Licensing Information
CC-By 4.0
### Citation Information
@article{bitton2022winogavil,
title={WinoGAViL: Gamified Association Benchmark to Challenge Vision-and-Language Models},
author={Bitton, Yonatan and Guetta, Nitzan Bitton and Yosef, Ron and Elovici, Yuval and Bansal, Mohit and Stanovsky, Gabriel and Schwartz, Roy},
journal={arXiv preprint arXiv:2207.12576},
year={2022}
| severo/winogavil | [
"annotations_creators:crowdsourced",
"language_creators:found",
"multilinguality:monolingual",
"size_categories:10K<n<100K",
"source_datasets:original",
"language:en",
"license:cc-by-4.0",
"commonsense-reasoning",
"visual-reasoning",
"arxiv:2207.12576",
"region:us"
] | 2022-09-27T13:06:01+00:00 | {"annotations_creators": ["crowdsourced"], "language_creators": ["found"], "language": ["en"], "license": ["cc-by-4.0"], "multilinguality": ["monolingual"], "size_categories": ["10K<n<100K"], "source_datasets": ["original"], "task_ids": [], "paperswithcode_id": "winogavil", "pretty_name": "WinoGAViL", "tags": ["commonsense-reasoning", "visual-reasoning"], "extra_gated_prompt": "By clicking on \u201cAccess repository\u201d below, you also agree that you are using it solely for research purposes. The full license agreement is available in the dataset files."} | 2022-09-27T13:00:32+00:00 | [
"2207.12576"
] | [
"en"
] | TAGS
#annotations_creators-crowdsourced #language_creators-found #multilinguality-monolingual #size_categories-10K<n<100K #source_datasets-original #language-English #license-cc-by-4.0 #commonsense-reasoning #visual-reasoning #arxiv-2207.12576 #region-us
|
# Dataset Card for WinoGAViL
- Dataset Description
- Supported Tasks and Leaderboards
- Colab notebook code for Winogavil evaluation with CLIP
- Languages
- Dataset Structure
- Data Fields
- Data Splits
- Dataset Creation
- Considerations for Using the Data
- Licensing Information
- Citation Information
## Dataset Description
WinoGAViL is a challenging dataset for evaluating vision-and-language commonsense reasoning abilities. Given a set of images, a cue, and a number K, the task is to select the K images that best fits the association. This dataset was collected via the WinoGAViL online game to collect vision-and-language associations, (e.g., werewolves to a full moon). Inspired by the popular card game Codenames, a spymaster gives a textual cue related to several visual candidates, and another player has to identify them. Human players are rewarded for creating associations that are challenging for a rival AI model but still solvable by other human players. We evaluate several state-of-the-art vision-and-language models, finding that they are intuitive for humans (>90% Jaccard index) but challenging for state-of-the-art AI models, where the best model (ViLT) achieves a score of 52%, succeeding mostly where the cue is visually salient. Our analysis as well as the feedback we collect from players indicate that the collected associations require diverse reasoning skills, including general knowledge, common sense, abstraction, and more.
- Homepage:
URL
- Colab
URL
- Repository:
URL
- Paper:
URL
- Leaderboard:
URL
- Point of Contact:
winogavil@URL; yonatanbitton1@URL
### Supported Tasks and Leaderboards
URL
URL
## Colab notebook code for Winogavil evaluation with CLIP
URL
### Languages
English.
## Dataset Structure
### Data Fields
candidates (list): ["bison", "shelter", "beard", "flea", "cattle", "shave"] - list of image candidates.
cue (string): pogonophile - the generated cue.
associations (string): ["bison", "beard", "shave"] - the images associated with the cue selected by the user.
score_fool_the_ai (int64): 80 - the spymaster score (100 - model score) for fooling the AI, with CLIP RN50 model.
num_associations (int64): 3 - The number of images selected as associative with the cue.
num_candidates (int64): 6 - the number of total candidates.
solvers_jaccard_mean (float64): 1.0 - three solvers scores average on the generated association instance.
solvers_jaccard_std (float64): 1.0 - three solvers scores standard deviation on the generated association instance
ID (int64): 367 - association ID.
### Data Splits
There is a single TEST split. In the accompanied paper and code we sample it to create different training sets, but the intended use is to use winogavil as a test set.
There are different number of candidates, which creates different difficulty levels:
-- With 5 candidates, random model expected score is 38%.
-- With 6 candidates, random model expected score is 34%.
-- With 10 candidates, random model expected score is 24%.
-- With 12 candidates, random model expected score is 19%.
<details>
<summary>Why random chance for success with 5 candidates is 38%?</summary>
It is a binomial distribution probability calculation.
Assuming N=5 candidates, and K=2 associations, there could be three events:
(1) The probability for a random guess is correct in 0 associations is 0.3 (elaborate below), and the Jaccard index is 0 (there is no intersection between the correct labels and the wrong guesses). Therefore the expected random score is 0.
(2) The probability for a random guess is correct in 1 associations is 0.6, and the Jaccard index is 0.33 (intersection=1, union=3, one of the correct guesses, and one of the wrong guesses). Therefore the expected random score is 0.6*0.33 = 0.198.
(3) The probability for a random guess is correct in 2 associations is 0.1, and the Jaccard index is 1 (intersection=2, union=2). Therefore the expected random score is 0.1*1 = 0.1.
* Together, when K=2, the expected score is 0+0.198+0.1 = 0.298.
To calculate (1), the first guess needs to be wrong. There are 3 "wrong" guesses and 5 candidates, so the probability for it is 3/5. The next guess should also be wrong. Now there are only 2 "wrong" guesses, and 4 candidates, so the probability for it is 2/4. Multiplying 3/5 * 2/4 = 0.3.
Same goes for (2) and (3).
Now we can perform the same calculation with K=3 associations.
Assuming N=5 candidates, and K=3 associations, there could be four events:
(4) The probability for a random guess is correct in 0 associations is 0, and the Jaccard index is 0. Therefore the expected random score is 0.
(5) The probability for a random guess is correct in 1 associations is 0.3, and the Jaccard index is 0.2 (intersection=1, union=4). Therefore the expected random score is 0.3*0.2 = 0.06.
(6) The probability for a random guess is correct in 2 associations is 0.6, and the Jaccard index is 0.5 (intersection=2, union=4). Therefore the expected random score is 0.6*5 = 0.3.
(7) The probability for a random guess is correct in 3 associations is 0.1, and the Jaccard index is 1 (intersection=3, union=3). Therefore the expected random score is 0.1*1 = 0.1.
* Together, when K=3, the expected score is 0+0.06+0.3+0.1 = 0.46.
Taking the average of 0.298 and 0.46 we reach 0.379.
Same process can be recalculated with 6 candidates (and K=2,3,4), 10 candidates (and K=2,3,4,5) and 123 candidates (and K=2,3,4,5,6).
</details>
## Dataset Creation
Inspired by the popular card game Codenames, a โspymasterโ gives a textual cue related to several visual candidates, and another player has to identify them. Human players are rewarded for creating
associations that are challenging for a rival AI model but still solvable by other
human players.
### Annotations
#### Annotation process
We paid Amazon Mechanical Turk Workers to play our game.
## Considerations for Using the Data
All associations were obtained with human annotators.
### Licensing Information
CC-By 4.0
@article{bitton2022winogavil,
title={WinoGAViL: Gamified Association Benchmark to Challenge Vision-and-Language Models},
author={Bitton, Yonatan and Guetta, Nitzan Bitton and Yosef, Ron and Elovici, Yuval and Bansal, Mohit and Stanovsky, Gabriel and Schwartz, Roy},
journal={arXiv preprint arXiv:2207.12576},
year={2022}
| [
"# Dataset Card for WinoGAViL\n\n- Dataset Description\n - Supported Tasks and Leaderboards\n - Colab notebook code for Winogavil evaluation with CLIP\n - Languages\n- Dataset Structure\n - Data Fields\n - Data Splits\n- Dataset Creation\n- Considerations for Using the Data\n - Licensing Information\n - Citation Information",
"## Dataset Description\n\nWinoGAViL is a challenging dataset for evaluating vision-and-language commonsense reasoning abilities. Given a set of images, a cue, and a number K, the task is to select the K images that best fits the association. This dataset was collected via the WinoGAViL online game to collect vision-and-language associations, (e.g., werewolves to a full moon). Inspired by the popular card game Codenames, a spymaster gives a textual cue related to several visual candidates, and another player has to identify them. Human players are rewarded for creating associations that are challenging for a rival AI model but still solvable by other human players. We evaluate several state-of-the-art vision-and-language models, finding that they are intuitive for humans (>90% Jaccard index) but challenging for state-of-the-art AI models, where the best model (ViLT) achieves a score of 52%, succeeding mostly where the cue is visually salient. Our analysis as well as the feedback we collect from players indicate that the collected associations require diverse reasoning skills, including general knowledge, common sense, abstraction, and more. \n\n- Homepage: \nURL\n- Colab\nURL\n- Repository:\nURL\n- Paper:\nURL\n- Leaderboard:\nURL\n- Point of Contact:\nwinogavil@URL; yonatanbitton1@URL",
"### Supported Tasks and Leaderboards\n\nURL \nURL",
"## Colab notebook code for Winogavil evaluation with CLIP\nURL",
"### Languages\n\nEnglish.",
"## Dataset Structure",
"### Data Fields\n\ncandidates (list): [\"bison\", \"shelter\", \"beard\", \"flea\", \"cattle\", \"shave\"] - list of image candidates. \ncue (string): pogonophile - the generated cue. \nassociations (string): [\"bison\", \"beard\", \"shave\"] - the images associated with the cue selected by the user. \nscore_fool_the_ai (int64): 80 - the spymaster score (100 - model score) for fooling the AI, with CLIP RN50 model. \nnum_associations (int64): 3 - The number of images selected as associative with the cue. \nnum_candidates (int64): 6 - the number of total candidates. \nsolvers_jaccard_mean (float64): 1.0 - three solvers scores average on the generated association instance. \nsolvers_jaccard_std (float64): 1.0 - three solvers scores standard deviation on the generated association instance\nID (int64): 367 - association ID.",
"### Data Splits\nThere is a single TEST split. In the accompanied paper and code we sample it to create different training sets, but the intended use is to use winogavil as a test set.\nThere are different number of candidates, which creates different difficulty levels: \n -- With 5 candidates, random model expected score is 38%. \n -- With 6 candidates, random model expected score is 34%. \n -- With 10 candidates, random model expected score is 24%. \n -- With 12 candidates, random model expected score is 19%. \n\n<details>\n <summary>Why random chance for success with 5 candidates is 38%?</summary>\n \n It is a binomial distribution probability calculation. \n \n Assuming N=5 candidates, and K=2 associations, there could be three events: \n (1) The probability for a random guess is correct in 0 associations is 0.3 (elaborate below), and the Jaccard index is 0 (there is no intersection between the correct labels and the wrong guesses). Therefore the expected random score is 0. \n (2) The probability for a random guess is correct in 1 associations is 0.6, and the Jaccard index is 0.33 (intersection=1, union=3, one of the correct guesses, and one of the wrong guesses). Therefore the expected random score is 0.6*0.33 = 0.198. \n (3) The probability for a random guess is correct in 2 associations is 0.1, and the Jaccard index is 1 (intersection=2, union=2). Therefore the expected random score is 0.1*1 = 0.1. \n * Together, when K=2, the expected score is 0+0.198+0.1 = 0.298. \n \n To calculate (1), the first guess needs to be wrong. There are 3 \"wrong\" guesses and 5 candidates, so the probability for it is 3/5. The next guess should also be wrong. Now there are only 2 \"wrong\" guesses, and 4 candidates, so the probability for it is 2/4. Multiplying 3/5 * 2/4 = 0.3. \n Same goes for (2) and (3). \n \n Now we can perform the same calculation with K=3 associations. \n Assuming N=5 candidates, and K=3 associations, there could be four events: \n (4) The probability for a random guess is correct in 0 associations is 0, and the Jaccard index is 0. Therefore the expected random score is 0. \n (5) The probability for a random guess is correct in 1 associations is 0.3, and the Jaccard index is 0.2 (intersection=1, union=4). Therefore the expected random score is 0.3*0.2 = 0.06. \n (6) The probability for a random guess is correct in 2 associations is 0.6, and the Jaccard index is 0.5 (intersection=2, union=4). Therefore the expected random score is 0.6*5 = 0.3. \n (7) The probability for a random guess is correct in 3 associations is 0.1, and the Jaccard index is 1 (intersection=3, union=3). Therefore the expected random score is 0.1*1 = 0.1. \n * Together, when K=3, the expected score is 0+0.06+0.3+0.1 = 0.46. \n \nTaking the average of 0.298 and 0.46 we reach 0.379. \n\nSame process can be recalculated with 6 candidates (and K=2,3,4), 10 candidates (and K=2,3,4,5) and 123 candidates (and K=2,3,4,5,6). \n\n</details>",
"## Dataset Creation\n\nInspired by the popular card game Codenames, a โspymasterโ gives a textual cue related to several visual candidates, and another player has to identify them. Human players are rewarded for creating\nassociations that are challenging for a rival AI model but still solvable by other\nhuman players.",
"### Annotations",
"#### Annotation process\n\nWe paid Amazon Mechanical Turk Workers to play our game.",
"## Considerations for Using the Data\n\nAll associations were obtained with human annotators.",
"### Licensing Information\n\nCC-By 4.0 \n\n\n\n @article{bitton2022winogavil,\n title={WinoGAViL: Gamified Association Benchmark to Challenge Vision-and-Language Models},\n author={Bitton, Yonatan and Guetta, Nitzan Bitton and Yosef, Ron and Elovici, Yuval and Bansal, Mohit and Stanovsky, Gabriel and Schwartz, Roy},\n journal={arXiv preprint arXiv:2207.12576},\n year={2022}"
] | [
"TAGS\n#annotations_creators-crowdsourced #language_creators-found #multilinguality-monolingual #size_categories-10K<n<100K #source_datasets-original #language-English #license-cc-by-4.0 #commonsense-reasoning #visual-reasoning #arxiv-2207.12576 #region-us \n",
"# Dataset Card for WinoGAViL\n\n- Dataset Description\n - Supported Tasks and Leaderboards\n - Colab notebook code for Winogavil evaluation with CLIP\n - Languages\n- Dataset Structure\n - Data Fields\n - Data Splits\n- Dataset Creation\n- Considerations for Using the Data\n - Licensing Information\n - Citation Information",
"## Dataset Description\n\nWinoGAViL is a challenging dataset for evaluating vision-and-language commonsense reasoning abilities. Given a set of images, a cue, and a number K, the task is to select the K images that best fits the association. This dataset was collected via the WinoGAViL online game to collect vision-and-language associations, (e.g., werewolves to a full moon). Inspired by the popular card game Codenames, a spymaster gives a textual cue related to several visual candidates, and another player has to identify them. Human players are rewarded for creating associations that are challenging for a rival AI model but still solvable by other human players. We evaluate several state-of-the-art vision-and-language models, finding that they are intuitive for humans (>90% Jaccard index) but challenging for state-of-the-art AI models, where the best model (ViLT) achieves a score of 52%, succeeding mostly where the cue is visually salient. Our analysis as well as the feedback we collect from players indicate that the collected associations require diverse reasoning skills, including general knowledge, common sense, abstraction, and more. \n\n- Homepage: \nURL\n- Colab\nURL\n- Repository:\nURL\n- Paper:\nURL\n- Leaderboard:\nURL\n- Point of Contact:\nwinogavil@URL; yonatanbitton1@URL",
"### Supported Tasks and Leaderboards\n\nURL \nURL",
"## Colab notebook code for Winogavil evaluation with CLIP\nURL",
"### Languages\n\nEnglish.",
"## Dataset Structure",
"### Data Fields\n\ncandidates (list): [\"bison\", \"shelter\", \"beard\", \"flea\", \"cattle\", \"shave\"] - list of image candidates. \ncue (string): pogonophile - the generated cue. \nassociations (string): [\"bison\", \"beard\", \"shave\"] - the images associated with the cue selected by the user. \nscore_fool_the_ai (int64): 80 - the spymaster score (100 - model score) for fooling the AI, with CLIP RN50 model. \nnum_associations (int64): 3 - The number of images selected as associative with the cue. \nnum_candidates (int64): 6 - the number of total candidates. \nsolvers_jaccard_mean (float64): 1.0 - three solvers scores average on the generated association instance. \nsolvers_jaccard_std (float64): 1.0 - three solvers scores standard deviation on the generated association instance\nID (int64): 367 - association ID.",
"### Data Splits\nThere is a single TEST split. In the accompanied paper and code we sample it to create different training sets, but the intended use is to use winogavil as a test set.\nThere are different number of candidates, which creates different difficulty levels: \n -- With 5 candidates, random model expected score is 38%. \n -- With 6 candidates, random model expected score is 34%. \n -- With 10 candidates, random model expected score is 24%. \n -- With 12 candidates, random model expected score is 19%. \n\n<details>\n <summary>Why random chance for success with 5 candidates is 38%?</summary>\n \n It is a binomial distribution probability calculation. \n \n Assuming N=5 candidates, and K=2 associations, there could be three events: \n (1) The probability for a random guess is correct in 0 associations is 0.3 (elaborate below), and the Jaccard index is 0 (there is no intersection between the correct labels and the wrong guesses). Therefore the expected random score is 0. \n (2) The probability for a random guess is correct in 1 associations is 0.6, and the Jaccard index is 0.33 (intersection=1, union=3, one of the correct guesses, and one of the wrong guesses). Therefore the expected random score is 0.6*0.33 = 0.198. \n (3) The probability for a random guess is correct in 2 associations is 0.1, and the Jaccard index is 1 (intersection=2, union=2). Therefore the expected random score is 0.1*1 = 0.1. \n * Together, when K=2, the expected score is 0+0.198+0.1 = 0.298. \n \n To calculate (1), the first guess needs to be wrong. There are 3 \"wrong\" guesses and 5 candidates, so the probability for it is 3/5. The next guess should also be wrong. Now there are only 2 \"wrong\" guesses, and 4 candidates, so the probability for it is 2/4. Multiplying 3/5 * 2/4 = 0.3. \n Same goes for (2) and (3). \n \n Now we can perform the same calculation with K=3 associations. \n Assuming N=5 candidates, and K=3 associations, there could be four events: \n (4) The probability for a random guess is correct in 0 associations is 0, and the Jaccard index is 0. Therefore the expected random score is 0. \n (5) The probability for a random guess is correct in 1 associations is 0.3, and the Jaccard index is 0.2 (intersection=1, union=4). Therefore the expected random score is 0.3*0.2 = 0.06. \n (6) The probability for a random guess is correct in 2 associations is 0.6, and the Jaccard index is 0.5 (intersection=2, union=4). Therefore the expected random score is 0.6*5 = 0.3. \n (7) The probability for a random guess is correct in 3 associations is 0.1, and the Jaccard index is 1 (intersection=3, union=3). Therefore the expected random score is 0.1*1 = 0.1. \n * Together, when K=3, the expected score is 0+0.06+0.3+0.1 = 0.46. \n \nTaking the average of 0.298 and 0.46 we reach 0.379. \n\nSame process can be recalculated with 6 candidates (and K=2,3,4), 10 candidates (and K=2,3,4,5) and 123 candidates (and K=2,3,4,5,6). \n\n</details>",
"## Dataset Creation\n\nInspired by the popular card game Codenames, a โspymasterโ gives a textual cue related to several visual candidates, and another player has to identify them. Human players are rewarded for creating\nassociations that are challenging for a rival AI model but still solvable by other\nhuman players.",
"### Annotations",
"#### Annotation process\n\nWe paid Amazon Mechanical Turk Workers to play our game.",
"## Considerations for Using the Data\n\nAll associations were obtained with human annotators.",
"### Licensing Information\n\nCC-By 4.0 \n\n\n\n @article{bitton2022winogavil,\n title={WinoGAViL: Gamified Association Benchmark to Challenge Vision-and-Language Models},\n author={Bitton, Yonatan and Guetta, Nitzan Bitton and Yosef, Ron and Elovici, Yuval and Bansal, Mohit and Stanovsky, Gabriel and Schwartz, Roy},\n journal={arXiv preprint arXiv:2207.12576},\n year={2022}"
] | [
91,
75,
312,
12,
14,
6,
6,
239,
746,
68,
5,
18,
19,
121
] | [
"passage: TAGS\n#annotations_creators-crowdsourced #language_creators-found #multilinguality-monolingual #size_categories-10K<n<100K #source_datasets-original #language-English #license-cc-by-4.0 #commonsense-reasoning #visual-reasoning #arxiv-2207.12576 #region-us \n# Dataset Card for WinoGAViL\n\n- Dataset Description\n - Supported Tasks and Leaderboards\n - Colab notebook code for Winogavil evaluation with CLIP\n - Languages\n- Dataset Structure\n - Data Fields\n - Data Splits\n- Dataset Creation\n- Considerations for Using the Data\n - Licensing Information\n - Citation Information## Dataset Description\n\nWinoGAViL is a challenging dataset for evaluating vision-and-language commonsense reasoning abilities. Given a set of images, a cue, and a number K, the task is to select the K images that best fits the association. This dataset was collected via the WinoGAViL online game to collect vision-and-language associations, (e.g., werewolves to a full moon). Inspired by the popular card game Codenames, a spymaster gives a textual cue related to several visual candidates, and another player has to identify them. Human players are rewarded for creating associations that are challenging for a rival AI model but still solvable by other human players. We evaluate several state-of-the-art vision-and-language models, finding that they are intuitive for humans (>90% Jaccard index) but challenging for state-of-the-art AI models, where the best model (ViLT) achieves a score of 52%, succeeding mostly where the cue is visually salient. Our analysis as well as the feedback we collect from players indicate that the collected associations require diverse reasoning skills, including general knowledge, common sense, abstraction, and more. \n\n- Homepage: \nURL\n- Colab\nURL\n- Repository:\nURL\n- Paper:\nURL\n- Leaderboard:\nURL\n- Point of Contact:\nwinogavil@URL; yonatanbitton1@URL### Supported Tasks and Leaderboards\n\nURL \nURL## Colab notebook code for Winogavil evaluation with CLIP\nURL",
"passage: ### Languages\n\nEnglish.## Dataset Structure### Data Fields\n\ncandidates (list): [\"bison\", \"shelter\", \"beard\", \"flea\", \"cattle\", \"shave\"] - list of image candidates. \ncue (string): pogonophile - the generated cue. \nassociations (string): [\"bison\", \"beard\", \"shave\"] - the images associated with the cue selected by the user. \nscore_fool_the_ai (int64): 80 - the spymaster score (100 - model score) for fooling the AI, with CLIP RN50 model. \nnum_associations (int64): 3 - The number of images selected as associative with the cue. \nnum_candidates (int64): 6 - the number of total candidates. \nsolvers_jaccard_mean (float64): 1.0 - three solvers scores average on the generated association instance. \nsolvers_jaccard_std (float64): 1.0 - three solvers scores standard deviation on the generated association instance\nID (int64): 367 - association ID."
] |
c20fb7cdff2c4b197e4c4125f850db01a559b4ab | Dataset for paper: Learning the Solution Operator of Boundary Value Problems using Graph Neural Networks
https://arxiv.org/abs/2206.14092 | winfried/gnn_bvp_solver | [
"license:mit",
"arxiv:2206.14092",
"region:us"
] | 2022-09-27T14:14:07+00:00 | {"license": "mit"} | 2022-09-27T15:52:13+00:00 | [
"2206.14092"
] | [] | TAGS
#license-mit #arxiv-2206.14092 #region-us
| Dataset for paper: Learning the Solution Operator of Boundary Value Problems using Graph Neural Networks
URL | [] | [
"TAGS\n#license-mit #arxiv-2206.14092 #region-us \n"
] | [
19
] | [
"passage: TAGS\n#license-mit #arxiv-2206.14092 #region-us \n"
] |
c219307f7fd35f295dcd0cdf4cc94cd949158b30 | # Dataset Card for AutoTrain Evaluator
This repository contains model predictions generated by [AutoTrain](https://huggingface.co/autotrain) for the following task and dataset:
* Task: Zero-Shot Text Classification
* Model: facebook/opt-6.7b
* Dataset: mathemakitten/winobias_antistereotype_dev
* Config: mathemakitten--winobias_antistereotype_dev
* Split: validation
To run new evaluation jobs, visit Hugging Face's [automatic model evaluator](https://huggingface.co/spaces/autoevaluate/model-evaluator).
## Contributions
Thanks to [@mathemakitten](https://huggingface.co/mathemakitten) for evaluating this model. | autoevaluate/autoeval-eval-mathemakitten__winobias_antistereotype_dev-mathemakitte-7776e8-1573055858 | [
"autotrain",
"evaluation",
"region:us"
] | 2022-09-27T15:14:32+00:00 | {"type": "predictions", "tags": ["autotrain", "evaluation"], "datasets": ["mathemakitten/winobias_antistereotype_dev"], "eval_info": {"task": "text_zero_shot_classification", "model": "facebook/opt-6.7b", "metrics": [], "dataset_name": "mathemakitten/winobias_antistereotype_dev", "dataset_config": "mathemakitten--winobias_antistereotype_dev", "dataset_split": "validation", "col_mapping": {"text": "text", "classes": "classes", "target": "target"}}} | 2022-09-27T15:30:46+00:00 | [] | [] | TAGS
#autotrain #evaluation #region-us
| # Dataset Card for AutoTrain Evaluator
This repository contains model predictions generated by AutoTrain for the following task and dataset:
* Task: Zero-Shot Text Classification
* Model: facebook/opt-6.7b
* Dataset: mathemakitten/winobias_antistereotype_dev
* Config: mathemakitten--winobias_antistereotype_dev
* Split: validation
To run new evaluation jobs, visit Hugging Face's automatic model evaluator.
## Contributions
Thanks to @mathemakitten for evaluating this model. | [
"# Dataset Card for AutoTrain Evaluator\n\nThis repository contains model predictions generated by AutoTrain for the following task and dataset:\n\n* Task: Zero-Shot Text Classification\n* Model: facebook/opt-6.7b\n* Dataset: mathemakitten/winobias_antistereotype_dev\n* Config: mathemakitten--winobias_antistereotype_dev\n* Split: validation\n\nTo run new evaluation jobs, visit Hugging Face's automatic model evaluator.",
"## Contributions\n\nThanks to @mathemakitten for evaluating this model."
] | [
"TAGS\n#autotrain #evaluation #region-us \n",
"# Dataset Card for AutoTrain Evaluator\n\nThis repository contains model predictions generated by AutoTrain for the following task and dataset:\n\n* Task: Zero-Shot Text Classification\n* Model: facebook/opt-6.7b\n* Dataset: mathemakitten/winobias_antistereotype_dev\n* Config: mathemakitten--winobias_antistereotype_dev\n* Split: validation\n\nTo run new evaluation jobs, visit Hugging Face's automatic model evaluator.",
"## Contributions\n\nThanks to @mathemakitten for evaluating this model."
] | [
13,
115,
17
] | [
"passage: TAGS\n#autotrain #evaluation #region-us \n# Dataset Card for AutoTrain Evaluator\n\nThis repository contains model predictions generated by AutoTrain for the following task and dataset:\n\n* Task: Zero-Shot Text Classification\n* Model: facebook/opt-6.7b\n* Dataset: mathemakitten/winobias_antistereotype_dev\n* Config: mathemakitten--winobias_antistereotype_dev\n* Split: validation\n\nTo run new evaluation jobs, visit Hugging Face's automatic model evaluator.## Contributions\n\nThanks to @mathemakitten for evaluating this model."
] |
4596f8cd06aa6f0fc71957d2e6a1f33c8664b643 | # Dataset Card for AutoTrain Evaluator
This repository contains model predictions generated by [AutoTrain](https://huggingface.co/autotrain) for the following task and dataset:
* Task: Zero-Shot Text Classification
* Model: facebook/opt-1.3b
* Dataset: mathemakitten/winobias_antistereotype_dev
* Config: mathemakitten--winobias_antistereotype_dev
* Split: validation
To run new evaluation jobs, visit Hugging Face's [automatic model evaluator](https://huggingface.co/spaces/autoevaluate/model-evaluator).
## Contributions
Thanks to [@mathemakitten](https://huggingface.co/mathemakitten) for evaluating this model. | autoevaluate/autoeval-eval-mathemakitten__winobias_antistereotype_dev-mathemakitte-e92f99-1572955856 | [
"autotrain",
"evaluation",
"region:us"
] | 2022-09-27T15:14:33+00:00 | {"type": "predictions", "tags": ["autotrain", "evaluation"], "datasets": ["mathemakitten/winobias_antistereotype_dev"], "eval_info": {"task": "text_zero_shot_classification", "model": "facebook/opt-1.3b", "metrics": [], "dataset_name": "mathemakitten/winobias_antistereotype_dev", "dataset_config": "mathemakitten--winobias_antistereotype_dev", "dataset_split": "validation", "col_mapping": {"text": "text", "classes": "classes", "target": "target"}}} | 2022-09-27T15:17:41+00:00 | [] | [] | TAGS
#autotrain #evaluation #region-us
| # Dataset Card for AutoTrain Evaluator
This repository contains model predictions generated by AutoTrain for the following task and dataset:
* Task: Zero-Shot Text Classification
* Model: facebook/opt-1.3b
* Dataset: mathemakitten/winobias_antistereotype_dev
* Config: mathemakitten--winobias_antistereotype_dev
* Split: validation
To run new evaluation jobs, visit Hugging Face's automatic model evaluator.
## Contributions
Thanks to @mathemakitten for evaluating this model. | [
"# Dataset Card for AutoTrain Evaluator\n\nThis repository contains model predictions generated by AutoTrain for the following task and dataset:\n\n* Task: Zero-Shot Text Classification\n* Model: facebook/opt-1.3b\n* Dataset: mathemakitten/winobias_antistereotype_dev\n* Config: mathemakitten--winobias_antistereotype_dev\n* Split: validation\n\nTo run new evaluation jobs, visit Hugging Face's automatic model evaluator.",
"## Contributions\n\nThanks to @mathemakitten for evaluating this model."
] | [
"TAGS\n#autotrain #evaluation #region-us \n",
"# Dataset Card for AutoTrain Evaluator\n\nThis repository contains model predictions generated by AutoTrain for the following task and dataset:\n\n* Task: Zero-Shot Text Classification\n* Model: facebook/opt-1.3b\n* Dataset: mathemakitten/winobias_antistereotype_dev\n* Config: mathemakitten--winobias_antistereotype_dev\n* Split: validation\n\nTo run new evaluation jobs, visit Hugging Face's automatic model evaluator.",
"## Contributions\n\nThanks to @mathemakitten for evaluating this model."
] | [
13,
115,
17
] | [
"passage: TAGS\n#autotrain #evaluation #region-us \n# Dataset Card for AutoTrain Evaluator\n\nThis repository contains model predictions generated by AutoTrain for the following task and dataset:\n\n* Task: Zero-Shot Text Classification\n* Model: facebook/opt-1.3b\n* Dataset: mathemakitten/winobias_antistereotype_dev\n* Config: mathemakitten--winobias_antistereotype_dev\n* Split: validation\n\nTo run new evaluation jobs, visit Hugging Face's automatic model evaluator.## Contributions\n\nThanks to @mathemakitten for evaluating this model."
] |
fba43e6d568abcfdab87ffe3068571fd21dca450 | # Dataset Card for AutoTrain Evaluator
This repository contains model predictions generated by [AutoTrain](https://huggingface.co/autotrain) for the following task and dataset:
* Task: Zero-Shot Text Classification
* Model: facebook/opt-13b
* Dataset: mathemakitten/winobias_antistereotype_dev
* Config: mathemakitten--winobias_antistereotype_dev
* Split: validation
To run new evaluation jobs, visit Hugging Face's [automatic model evaluator](https://huggingface.co/spaces/autoevaluate/model-evaluator).
## Contributions
Thanks to [@mathemakitten](https://huggingface.co/mathemakitten) for evaluating this model. | autoevaluate/autoeval-eval-mathemakitten__winobias_antistereotype_dev-mathemakitte-7776e8-1573055859 | [
"autotrain",
"evaluation",
"region:us"
] | 2022-09-27T15:14:34+00:00 | {"type": "predictions", "tags": ["autotrain", "evaluation"], "datasets": ["mathemakitten/winobias_antistereotype_dev"], "eval_info": {"task": "text_zero_shot_classification", "model": "facebook/opt-13b", "metrics": [], "dataset_name": "mathemakitten/winobias_antistereotype_dev", "dataset_config": "mathemakitten--winobias_antistereotype_dev", "dataset_split": "validation", "col_mapping": {"text": "text", "classes": "classes", "target": "target"}}} | 2022-09-27T15:43:28+00:00 | [] | [] | TAGS
#autotrain #evaluation #region-us
| # Dataset Card for AutoTrain Evaluator
This repository contains model predictions generated by AutoTrain for the following task and dataset:
* Task: Zero-Shot Text Classification
* Model: facebook/opt-13b
* Dataset: mathemakitten/winobias_antistereotype_dev
* Config: mathemakitten--winobias_antistereotype_dev
* Split: validation
To run new evaluation jobs, visit Hugging Face's automatic model evaluator.
## Contributions
Thanks to @mathemakitten for evaluating this model. | [
"# Dataset Card for AutoTrain Evaluator\n\nThis repository contains model predictions generated by AutoTrain for the following task and dataset:\n\n* Task: Zero-Shot Text Classification\n* Model: facebook/opt-13b\n* Dataset: mathemakitten/winobias_antistereotype_dev\n* Config: mathemakitten--winobias_antistereotype_dev\n* Split: validation\n\nTo run new evaluation jobs, visit Hugging Face's automatic model evaluator.",
"## Contributions\n\nThanks to @mathemakitten for evaluating this model."
] | [
"TAGS\n#autotrain #evaluation #region-us \n",
"# Dataset Card for AutoTrain Evaluator\n\nThis repository contains model predictions generated by AutoTrain for the following task and dataset:\n\n* Task: Zero-Shot Text Classification\n* Model: facebook/opt-13b\n* Dataset: mathemakitten/winobias_antistereotype_dev\n* Config: mathemakitten--winobias_antistereotype_dev\n* Split: validation\n\nTo run new evaluation jobs, visit Hugging Face's automatic model evaluator.",
"## Contributions\n\nThanks to @mathemakitten for evaluating this model."
] | [
13,
114,
17
] | [
"passage: TAGS\n#autotrain #evaluation #region-us \n# Dataset Card for AutoTrain Evaluator\n\nThis repository contains model predictions generated by AutoTrain for the following task and dataset:\n\n* Task: Zero-Shot Text Classification\n* Model: facebook/opt-13b\n* Dataset: mathemakitten/winobias_antistereotype_dev\n* Config: mathemakitten--winobias_antistereotype_dev\n* Split: validation\n\nTo run new evaluation jobs, visit Hugging Face's automatic model evaluator.## Contributions\n\nThanks to @mathemakitten for evaluating this model."
] |
25a3771e345e9226611b04bc2bd695eaebad972e | # Dataset Card for AutoTrain Evaluator
This repository contains model predictions generated by [AutoTrain](https://huggingface.co/autotrain) for the following task and dataset:
* Task: Zero-Shot Text Classification
* Model: facebook/opt-2.7b
* Dataset: mathemakitten/winobias_antistereotype_dev
* Config: mathemakitten--winobias_antistereotype_dev
* Split: validation
To run new evaluation jobs, visit Hugging Face's [automatic model evaluator](https://huggingface.co/spaces/autoevaluate/model-evaluator).
## Contributions
Thanks to [@mathemakitten](https://huggingface.co/mathemakitten) for evaluating this model. | autoevaluate/autoeval-eval-mathemakitten__winobias_antistereotype_dev-mathemakitte-e92f99-1572955857 | [
"autotrain",
"evaluation",
"region:us"
] | 2022-09-27T15:14:36+00:00 | {"type": "predictions", "tags": ["autotrain", "evaluation"], "datasets": ["mathemakitten/winobias_antistereotype_dev"], "eval_info": {"task": "text_zero_shot_classification", "model": "facebook/opt-2.7b", "metrics": [], "dataset_name": "mathemakitten/winobias_antistereotype_dev", "dataset_config": "mathemakitten--winobias_antistereotype_dev", "dataset_split": "validation", "col_mapping": {"text": "text", "classes": "classes", "target": "target"}}} | 2022-09-27T15:19:55+00:00 | [] | [] | TAGS
#autotrain #evaluation #region-us
| # Dataset Card for AutoTrain Evaluator
This repository contains model predictions generated by AutoTrain for the following task and dataset:
* Task: Zero-Shot Text Classification
* Model: facebook/opt-2.7b
* Dataset: mathemakitten/winobias_antistereotype_dev
* Config: mathemakitten--winobias_antistereotype_dev
* Split: validation
To run new evaluation jobs, visit Hugging Face's automatic model evaluator.
## Contributions
Thanks to @mathemakitten for evaluating this model. | [
"# Dataset Card for AutoTrain Evaluator\n\nThis repository contains model predictions generated by AutoTrain for the following task and dataset:\n\n* Task: Zero-Shot Text Classification\n* Model: facebook/opt-2.7b\n* Dataset: mathemakitten/winobias_antistereotype_dev\n* Config: mathemakitten--winobias_antistereotype_dev\n* Split: validation\n\nTo run new evaluation jobs, visit Hugging Face's automatic model evaluator.",
"## Contributions\n\nThanks to @mathemakitten for evaluating this model."
] | [
"TAGS\n#autotrain #evaluation #region-us \n",
"# Dataset Card for AutoTrain Evaluator\n\nThis repository contains model predictions generated by AutoTrain for the following task and dataset:\n\n* Task: Zero-Shot Text Classification\n* Model: facebook/opt-2.7b\n* Dataset: mathemakitten/winobias_antistereotype_dev\n* Config: mathemakitten--winobias_antistereotype_dev\n* Split: validation\n\nTo run new evaluation jobs, visit Hugging Face's automatic model evaluator.",
"## Contributions\n\nThanks to @mathemakitten for evaluating this model."
] | [
13,
116,
17
] | [
"passage: TAGS\n#autotrain #evaluation #region-us \n# Dataset Card for AutoTrain Evaluator\n\nThis repository contains model predictions generated by AutoTrain for the following task and dataset:\n\n* Task: Zero-Shot Text Classification\n* Model: facebook/opt-2.7b\n* Dataset: mathemakitten/winobias_antistereotype_dev\n* Config: mathemakitten--winobias_antistereotype_dev\n* Split: validation\n\nTo run new evaluation jobs, visit Hugging Face's automatic model evaluator.## Contributions\n\nThanks to @mathemakitten for evaluating this model."
] |
36506bf4050ad3043e111c1812be9c557b238954 | # Dataset Card for AutoTrain Evaluator
This repository contains model predictions generated by [AutoTrain](https://huggingface.co/autotrain) for the following task and dataset:
* Task: Zero-Shot Text Classification
* Model: facebook/opt-30b
* Dataset: mathemakitten/winobias_antistereotype_dev
* Config: mathemakitten--winobias_antistereotype_dev
* Split: validation
To run new evaluation jobs, visit Hugging Face's [automatic model evaluator](https://huggingface.co/spaces/autoevaluate/model-evaluator).
## Contributions
Thanks to [@mathemakitten](https://huggingface.co/mathemakitten) for evaluating this model. | autoevaluate/autoeval-eval-mathemakitten__winobias_antistereotype_dev-mathemakitte-7776e8-1573055860 | [
"autotrain",
"evaluation",
"region:us"
] | 2022-09-27T15:14:36+00:00 | {"type": "predictions", "tags": ["autotrain", "evaluation"], "datasets": ["mathemakitten/winobias_antistereotype_dev"], "eval_info": {"task": "text_zero_shot_classification", "model": "facebook/opt-30b", "metrics": [], "dataset_name": "mathemakitten/winobias_antistereotype_dev", "dataset_config": "mathemakitten--winobias_antistereotype_dev", "dataset_split": "validation", "col_mapping": {"text": "text", "classes": "classes", "target": "target"}}} | 2022-09-27T16:25:03+00:00 | [] | [] | TAGS
#autotrain #evaluation #region-us
| # Dataset Card for AutoTrain Evaluator
This repository contains model predictions generated by AutoTrain for the following task and dataset:
* Task: Zero-Shot Text Classification
* Model: facebook/opt-30b
* Dataset: mathemakitten/winobias_antistereotype_dev
* Config: mathemakitten--winobias_antistereotype_dev
* Split: validation
To run new evaluation jobs, visit Hugging Face's automatic model evaluator.
## Contributions
Thanks to @mathemakitten for evaluating this model. | [
"# Dataset Card for AutoTrain Evaluator\n\nThis repository contains model predictions generated by AutoTrain for the following task and dataset:\n\n* Task: Zero-Shot Text Classification\n* Model: facebook/opt-30b\n* Dataset: mathemakitten/winobias_antistereotype_dev\n* Config: mathemakitten--winobias_antistereotype_dev\n* Split: validation\n\nTo run new evaluation jobs, visit Hugging Face's automatic model evaluator.",
"## Contributions\n\nThanks to @mathemakitten for evaluating this model."
] | [
"TAGS\n#autotrain #evaluation #region-us \n",
"# Dataset Card for AutoTrain Evaluator\n\nThis repository contains model predictions generated by AutoTrain for the following task and dataset:\n\n* Task: Zero-Shot Text Classification\n* Model: facebook/opt-30b\n* Dataset: mathemakitten/winobias_antistereotype_dev\n* Config: mathemakitten--winobias_antistereotype_dev\n* Split: validation\n\nTo run new evaluation jobs, visit Hugging Face's automatic model evaluator.",
"## Contributions\n\nThanks to @mathemakitten for evaluating this model."
] | [
13,
114,
17
] | [
"passage: TAGS\n#autotrain #evaluation #region-us \n# Dataset Card for AutoTrain Evaluator\n\nThis repository contains model predictions generated by AutoTrain for the following task and dataset:\n\n* Task: Zero-Shot Text Classification\n* Model: facebook/opt-30b\n* Dataset: mathemakitten/winobias_antistereotype_dev\n* Config: mathemakitten--winobias_antistereotype_dev\n* Split: validation\n\nTo run new evaluation jobs, visit Hugging Face's automatic model evaluator.## Contributions\n\nThanks to @mathemakitten for evaluating this model."
] |
2afaf26908533ee079a8fe1fb7d36c595b8d7176 | # Dataset Card for AutoTrain Evaluator
This repository contains model predictions generated by [AutoTrain](https://huggingface.co/autotrain) for the following task and dataset:
* Task: Zero-Shot Text Classification
* Model: facebook/opt-350m
* Dataset: mathemakitten/winobias_antistereotype_dev
* Config: mathemakitten--winobias_antistereotype_dev
* Split: validation
To run new evaluation jobs, visit Hugging Face's [automatic model evaluator](https://huggingface.co/spaces/autoevaluate/model-evaluator).
## Contributions
Thanks to [@mathemakitten](https://huggingface.co/mathemakitten) for evaluating this model. | autoevaluate/autoeval-eval-mathemakitten__winobias_antistereotype_dev-mathemakitte-e92f99-1572955855 | [
"autotrain",
"evaluation",
"region:us"
] | 2022-09-27T15:14:38+00:00 | {"type": "predictions", "tags": ["autotrain", "evaluation"], "datasets": ["mathemakitten/winobias_antistereotype_dev"], "eval_info": {"task": "text_zero_shot_classification", "model": "facebook/opt-350m", "metrics": [], "dataset_name": "mathemakitten/winobias_antistereotype_dev", "dataset_config": "mathemakitten--winobias_antistereotype_dev", "dataset_split": "validation", "col_mapping": {"text": "text", "classes": "classes", "target": "target"}}} | 2022-09-27T15:15:50+00:00 | [] | [] | TAGS
#autotrain #evaluation #region-us
| # Dataset Card for AutoTrain Evaluator
This repository contains model predictions generated by AutoTrain for the following task and dataset:
* Task: Zero-Shot Text Classification
* Model: facebook/opt-350m
* Dataset: mathemakitten/winobias_antistereotype_dev
* Config: mathemakitten--winobias_antistereotype_dev
* Split: validation
To run new evaluation jobs, visit Hugging Face's automatic model evaluator.
## Contributions
Thanks to @mathemakitten for evaluating this model. | [
"# Dataset Card for AutoTrain Evaluator\n\nThis repository contains model predictions generated by AutoTrain for the following task and dataset:\n\n* Task: Zero-Shot Text Classification\n* Model: facebook/opt-350m\n* Dataset: mathemakitten/winobias_antistereotype_dev\n* Config: mathemakitten--winobias_antistereotype_dev\n* Split: validation\n\nTo run new evaluation jobs, visit Hugging Face's automatic model evaluator.",
"## Contributions\n\nThanks to @mathemakitten for evaluating this model."
] | [
"TAGS\n#autotrain #evaluation #region-us \n",
"# Dataset Card for AutoTrain Evaluator\n\nThis repository contains model predictions generated by AutoTrain for the following task and dataset:\n\n* Task: Zero-Shot Text Classification\n* Model: facebook/opt-350m\n* Dataset: mathemakitten/winobias_antistereotype_dev\n* Config: mathemakitten--winobias_antistereotype_dev\n* Split: validation\n\nTo run new evaluation jobs, visit Hugging Face's automatic model evaluator.",
"## Contributions\n\nThanks to @mathemakitten for evaluating this model."
] | [
13,
115,
17
] | [
"passage: TAGS\n#autotrain #evaluation #region-us \n# Dataset Card for AutoTrain Evaluator\n\nThis repository contains model predictions generated by AutoTrain for the following task and dataset:\n\n* Task: Zero-Shot Text Classification\n* Model: facebook/opt-350m\n* Dataset: mathemakitten/winobias_antistereotype_dev\n* Config: mathemakitten--winobias_antistereotype_dev\n* Split: validation\n\nTo run new evaluation jobs, visit Hugging Face's automatic model evaluator.## Contributions\n\nThanks to @mathemakitten for evaluating this model."
] |
e17a8195959cef8071410fd7fa8c4130a16a3a72 |
# Dataset Card for "tner/wikiann"
## Dataset Description
- **Repository:** [T-NER](https://github.com/asahi417/tner)
- **Paper:** [https://aclanthology.org/P17-1178/](https://aclanthology.org/P17-1178/)
- **Dataset:** WikiAnn
- **Domain:** Wikipedia
- **Number of Entity:** 3
### Dataset Summary
WikiAnn NER dataset formatted in a part of [TNER](https://github.com/asahi417/tner) project.
- Entity Types: `LOC`, `ORG`, `PER`
## Dataset Structure
### Data Instances
An example of `train` of `ja` looks as follows.
```
{
'tokens': ['#', '#', 'ใฆ', 'ใช', 'ใฆ', 'ใน', 'ใป', 'ใ', 'ใผ', 'ใช', 'ใ', 'ใฏ', '#', '1', '9','9','9'],
'tags': [6, 6, 2, 5, 5, 5, 5, 5, 5, 5, 5, 5, 6, 6, 6, 6, 6]
}
```
### Label ID
The label2id dictionary can be found at [here](https://huggingface.co/datasets/tner/wikiann/raw/main/dataset/label.json).
```python
{
"B-LOC": 0,
"B-ORG": 1,
"B-PER": 2,
"I-LOC": 3,
"I-ORG": 4,
"I-PER": 5,
"O": 6
}
```
### Data Splits
| language | train | validation | test |
|:-------------|--------:|-------------:|-------:|
| ace | 100 | 100 | 100 |
| bg | 20000 | 10000 | 10000 |
| da | 20000 | 10000 | 10000 |
| fur | 100 | 100 | 100 |
| ilo | 100 | 100 | 100 |
| lij | 100 | 100 | 100 |
| mzn | 100 | 100 | 100 |
| qu | 100 | 100 | 100 |
| su | 100 | 100 | 100 |
| vi | 20000 | 10000 | 10000 |
| af | 5000 | 1000 | 1000 |
| bh | 100 | 100 | 100 |
| de | 20000 | 10000 | 10000 |
| fy | 1000 | 1000 | 1000 |
| io | 100 | 100 | 100 |
| lmo | 100 | 100 | 100 |
| nap | 100 | 100 | 100 |
| rm | 100 | 100 | 100 |
| sv | 20000 | 10000 | 10000 |
| vls | 100 | 100 | 100 |
| als | 100 | 100 | 100 |
| bn | 10000 | 1000 | 1000 |
| diq | 100 | 100 | 100 |
| ga | 1000 | 1000 | 1000 |
| is | 1000 | 1000 | 1000 |
| ln | 100 | 100 | 100 |
| nds | 100 | 100 | 100 |
| ro | 20000 | 10000 | 10000 |
| sw | 1000 | 1000 | 1000 |
| vo | 100 | 100 | 100 |
| am | 100 | 100 | 100 |
| bo | 100 | 100 | 100 |
| dv | 100 | 100 | 100 |
| gan | 100 | 100 | 100 |
| it | 20000 | 10000 | 10000 |
| lt | 10000 | 10000 | 10000 |
| ne | 100 | 100 | 100 |
| ru | 20000 | 10000 | 10000 |
| szl | 100 | 100 | 100 |
| wa | 100 | 100 | 100 |
| an | 1000 | 1000 | 1000 |
| br | 1000 | 1000 | 1000 |
| el | 20000 | 10000 | 10000 |
| gd | 100 | 100 | 100 |
| ja | 20000 | 10000 | 10000 |
| lv | 10000 | 10000 | 10000 |
| nl | 20000 | 10000 | 10000 |
| rw | 100 | 100 | 100 |
| ta | 15000 | 1000 | 1000 |
| war | 100 | 100 | 100 |
| ang | 100 | 100 | 100 |
| bs | 15000 | 1000 | 1000 |
| eml | 100 | 100 | 100 |
| gl | 15000 | 10000 | 10000 |
| jbo | 100 | 100 | 100 |
| map-bms | 100 | 100 | 100 |
| nn | 20000 | 1000 | 1000 |
| sa | 100 | 100 | 100 |
| te | 1000 | 1000 | 1000 |
| wuu | 100 | 100 | 100 |
| ar | 20000 | 10000 | 10000 |
| ca | 20000 | 10000 | 10000 |
| en | 20000 | 10000 | 10000 |
| gn | 100 | 100 | 100 |
| jv | 100 | 100 | 100 |
| mg | 100 | 100 | 100 |
| no | 20000 | 10000 | 10000 |
| sah | 100 | 100 | 100 |
| tg | 100 | 100 | 100 |
| xmf | 100 | 100 | 100 |
| arc | 100 | 100 | 100 |
| cbk-zam | 100 | 100 | 100 |
| eo | 15000 | 10000 | 10000 |
| gu | 100 | 100 | 100 |
| ka | 10000 | 10000 | 10000 |
| mhr | 100 | 100 | 100 |
| nov | 100 | 100 | 100 |
| scn | 100 | 100 | 100 |
| th | 20000 | 10000 | 10000 |
| yi | 100 | 100 | 100 |
| arz | 100 | 100 | 100 |
| cdo | 100 | 100 | 100 |
| es | 20000 | 10000 | 10000 |
| hak | 100 | 100 | 100 |
| kk | 1000 | 1000 | 1000 |
| mi | 100 | 100 | 100 |
| oc | 100 | 100 | 100 |
| sco | 100 | 100 | 100 |
| tk | 100 | 100 | 100 |
| yo | 100 | 100 | 100 |
| as | 100 | 100 | 100 |
| ce | 100 | 100 | 100 |
| et | 15000 | 10000 | 10000 |
| he | 20000 | 10000 | 10000 |
| km | 100 | 100 | 100 |
| min | 100 | 100 | 100 |
| or | 100 | 100 | 100 |
| sd | 100 | 100 | 100 |
| tl | 10000 | 1000 | 1000 |
| zea | 100 | 100 | 100 |
| ast | 1000 | 1000 | 1000 |
| ceb | 100 | 100 | 100 |
| eu | 10000 | 10000 | 10000 |
| hi | 5000 | 1000 | 1000 |
| kn | 100 | 100 | 100 |
| mk | 10000 | 1000 | 1000 |
| os | 100 | 100 | 100 |
| sh | 20000 | 10000 | 10000 |
| tr | 20000 | 10000 | 10000 |
| zh-classical | 100 | 100 | 100 |
| ay | 100 | 100 | 100 |
| ckb | 1000 | 1000 | 1000 |
| ext | 100 | 100 | 100 |
| hr | 20000 | 10000 | 10000 |
| ko | 20000 | 10000 | 10000 |
| ml | 10000 | 1000 | 1000 |
| pa | 100 | 100 | 100 |
| si | 100 | 100 | 100 |
| tt | 1000 | 1000 | 1000 |
| zh-min-nan | 100 | 100 | 100 |
| az | 10000 | 1000 | 1000 |
| co | 100 | 100 | 100 |
| fa | 20000 | 10000 | 10000 |
| hsb | 100 | 100 | 100 |
| ksh | 100 | 100 | 100 |
| mn | 100 | 100 | 100 |
| pdc | 100 | 100 | 100 |
| simple | 20000 | 1000 | 1000 |
| ug | 100 | 100 | 100 |
| zh-yue | 20000 | 10000 | 10000 |
| ba | 100 | 100 | 100 |
| crh | 100 | 100 | 100 |
| fi | 20000 | 10000 | 10000 |
| hu | 20000 | 10000 | 10000 |
| ku | 100 | 100 | 100 |
| mr | 5000 | 1000 | 1000 |
| pl | 20000 | 10000 | 10000 |
| sk | 20000 | 10000 | 10000 |
| uk | 20000 | 10000 | 10000 |
| zh | 20000 | 10000 | 10000 |
| bar | 100 | 100 | 100 |
| cs | 20000 | 10000 | 10000 |
| fiu-vro | 100 | 100 | 100 |
| hy | 15000 | 1000 | 1000 |
| ky | 100 | 100 | 100 |
| ms | 20000 | 1000 | 1000 |
| pms | 100 | 100 | 100 |
| sl | 15000 | 10000 | 10000 |
| ur | 20000 | 1000 | 1000 |
| bat-smg | 100 | 100 | 100 |
| csb | 100 | 100 | 100 |
| fo | 100 | 100 | 100 |
| ia | 100 | 100 | 100 |
| la | 5000 | 1000 | 1000 |
| mt | 100 | 100 | 100 |
| pnb | 100 | 100 | 100 |
| so | 100 | 100 | 100 |
| uz | 1000 | 1000 | 1000 |
| be-x-old | 5000 | 1000 | 1000 |
| cv | 100 | 100 | 100 |
| fr | 20000 | 10000 | 10000 |
| id | 20000 | 10000 | 10000 |
| lb | 5000 | 1000 | 1000 |
| mwl | 100 | 100 | 100 |
| ps | 100 | 100 | 100 |
| sq | 5000 | 1000 | 1000 |
| vec | 100 | 100 | 100 |
| be | 15000 | 1000 | 1000 |
| cy | 10000 | 1000 | 1000 |
| frr | 100 | 100 | 100 |
| ig | 100 | 100 | 100 |
| li | 100 | 100 | 100 |
| my | 100 | 100 | 100 |
| pt | 20000 | 10000 | 10000 |
| sr | 20000 | 10000 | 10000 |
| vep | 100 | 100 | 100 |
### Citation Information
```
@inproceedings{pan-etal-2017-cross,
title = "Cross-lingual Name Tagging and Linking for 282 Languages",
author = "Pan, Xiaoman and
Zhang, Boliang and
May, Jonathan and
Nothman, Joel and
Knight, Kevin and
Ji, Heng",
booktitle = "Proceedings of the 55th Annual Meeting of the Association for Computational Linguistics (Volume 1: Long Papers)",
month = jul,
year = "2017",
address = "Vancouver, Canada",
publisher = "Association for Computational Linguistics",
url = "https://aclanthology.org/P17-1178",
doi = "10.18653/v1/P17-1178",
pages = "1946--1958",
abstract = "The ambitious goal of this work is to develop a cross-lingual name tagging and linking framework for 282 languages that exist in Wikipedia. Given a document in any of these languages, our framework is able to identify name mentions, assign a coarse-grained or fine-grained type to each mention, and link it to an English Knowledge Base (KB) if it is linkable. We achieve this goal by performing a series of new KB mining methods: generating {``}silver-standard{''} annotations by transferring annotations from English to other languages through cross-lingual links and KB properties, refining annotations through self-training and topic selection, deriving language-specific morphology features from anchor links, and mining word translation pairs from cross-lingual links. Both name tagging and linking results for 282 languages are promising on Wikipedia data and on-Wikipedia data.",
}
``` | tner/wikiann | [
"task_categories:token-classification",
"task_ids:named-entity-recognition",
"multilinguality:multilingual",
"size_categories:10K<100k",
"language:ace",
"language:bg",
"language:da",
"language:fur",
"language:ilo",
"language:lij",
"language:mzn",
"language:qu",
"language:su",
"language:vi",
"language:af",
"language:bh",
"language:de",
"language:fy",
"language:io",
"language:lmo",
"language:nap",
"language:rm",
"language:sv",
"language:vls",
"language:als",
"language:bn",
"language:diq",
"language:ga",
"language:is",
"language:ln",
"language:nds",
"language:ro",
"language:sw",
"language:vo",
"language:am",
"language:bo",
"language:dv",
"language:gan",
"language:it",
"language:lt",
"language:ne",
"language:ru",
"language:szl",
"language:wa",
"language:an",
"language:br",
"language:el",
"language:gd",
"language:ja",
"language:lv",
"language:nl",
"language:rw",
"language:ta",
"language:war",
"language:ang",
"language:bs",
"language:eml",
"language:gl",
"language:jbo",
"language:nn",
"language:sa",
"language:te",
"language:wuu",
"language:ar",
"language:ca",
"language:en",
"language:gn",
"language:jv",
"language:mg",
"language:no",
"language:sah",
"language:tg",
"language:xmf",
"language:arc",
"language:eo",
"language:gu",
"language:ka",
"language:mhr",
"language:nov",
"language:scn",
"language:th",
"language:yi",
"language:arz",
"language:cdo",
"language:es",
"language:hak",
"language:kk",
"language:mi",
"language:oc",
"language:sco",
"language:tk",
"language:yo",
"language:as",
"language:ce",
"language:et",
"language:he",
"language:km",
"language:min",
"language:or",
"language:sd",
"language:tl",
"language:zea",
"language:ast",
"language:ceb",
"language:eu",
"language:hi",
"language:kn",
"language:mk",
"language:os",
"language:sh",
"language:tr",
"language:ay",
"language:ckb",
"language:ext",
"language:hr",
"language:ko",
"language:ml",
"language:pa",
"language:si",
"language:tt",
"language:az",
"language:co",
"language:fa",
"language:hsb",
"language:ksh",
"language:mn",
"language:pdc",
"language:ug",
"language:ba",
"language:crh",
"language:fi",
"language:hu",
"language:ku",
"language:mr",
"language:pl",
"language:sk",
"language:uk",
"language:zh",
"language:bar",
"language:cs",
"language:hy",
"language:ky",
"language:ms",
"language:pms",
"language:sl",
"language:ur",
"language:csb",
"language:fo",
"language:ia",
"language:la",
"language:mt",
"language:pnb",
"language:so",
"language:uz",
"language:cv",
"language:fr",
"language:id",
"language:lb",
"language:mwl",
"language:ps",
"language:sq",
"language:vec",
"language:be",
"language:cy",
"language:frr",
"language:ig",
"language:li",
"language:my",
"language:pt",
"language:sr",
"region:us"
] | 2022-09-27T15:22:58+00:00 | {"language": ["ace", "bg", "da", "fur", "ilo", "lij", "mzn", "qu", "su", "vi", "af", "bh", "de", "fy", "io", "lmo", "nap", "rm", "sv", "vls", "als", "bn", "diq", "ga", "is", "ln", "nds", "ro", "sw", "vo", "am", "bo", "dv", "gan", "it", "lt", "ne", "ru", "szl", "wa", "an", "br", "el", "gd", "ja", "lv", "nl", "rw", "ta", "war", "ang", "bs", "eml", "gl", "jbo", "nn", "sa", "te", "wuu", "ar", "ca", "en", "gn", "jv", "mg", false, "sah", "tg", "xmf", "arc", "eo", "gu", "ka", "mhr", "nov", "scn", "th", "yi", "arz", "cdo", "es", "hak", "kk", "mi", "oc", "sco", "tk", "yo", "as", "ce", "et", "he", "km", "min", "or", "sd", "tl", "zea", "ast", "ceb", "eu", "hi", "kn", "mk", "os", "sh", "tr", "ay", "ckb", "ext", "hr", "ko", "ml", "pa", "si", "tt", "az", "co", "fa", "hsb", "ksh", "mn", "pdc", "ug", "ba", "crh", "fi", "hu", "ku", "mr", "pl", "sk", "uk", "zh", "bar", "cs", "hy", "ky", "ms", "pms", "sl", "ur", "csb", "fo", "ia", "la", "mt", "pnb", "so", "uz", "cv", "fr", "id", "lb", "mwl", "ps", "sq", "vec", "be", "cy", "frr", "ig", "li", "my", "pt", "sr"], "multilinguality": ["multilingual"], "size_categories": ["10K<100k"], "task_categories": ["token-classification"], "task_ids": ["named-entity-recognition"], "pretty_name": "WikiAnn"} | 2022-09-27T17:39:42+00:00 | [] | [
"ace",
"bg",
"da",
"fur",
"ilo",
"lij",
"mzn",
"qu",
"su",
"vi",
"af",
"bh",
"de",
"fy",
"io",
"lmo",
"nap",
"rm",
"sv",
"vls",
"als",
"bn",
"diq",
"ga",
"is",
"ln",
"nds",
"ro",
"sw",
"vo",
"am",
"bo",
"dv",
"gan",
"it",
"lt",
"ne",
"ru",
"szl",
"wa",
"an",
"br",
"el",
"gd",
"ja",
"lv",
"nl",
"rw",
"ta",
"war",
"ang",
"bs",
"eml",
"gl",
"jbo",
"nn",
"sa",
"te",
"wuu",
"ar",
"ca",
"en",
"gn",
"jv",
"mg",
"no",
"sah",
"tg",
"xmf",
"arc",
"eo",
"gu",
"ka",
"mhr",
"nov",
"scn",
"th",
"yi",
"arz",
"cdo",
"es",
"hak",
"kk",
"mi",
"oc",
"sco",
"tk",
"yo",
"as",
"ce",
"et",
"he",
"km",
"min",
"or",
"sd",
"tl",
"zea",
"ast",
"ceb",
"eu",
"hi",
"kn",
"mk",
"os",
"sh",
"tr",
"ay",
"ckb",
"ext",
"hr",
"ko",
"ml",
"pa",
"si",
"tt",
"az",
"co",
"fa",
"hsb",
"ksh",
"mn",
"pdc",
"ug",
"ba",
"crh",
"fi",
"hu",
"ku",
"mr",
"pl",
"sk",
"uk",
"zh",
"bar",
"cs",
"hy",
"ky",
"ms",
"pms",
"sl",
"ur",
"csb",
"fo",
"ia",
"la",
"mt",
"pnb",
"so",
"uz",
"cv",
"fr",
"id",
"lb",
"mwl",
"ps",
"sq",
"vec",
"be",
"cy",
"frr",
"ig",
"li",
"my",
"pt",
"sr"
] | TAGS
#task_categories-token-classification #task_ids-named-entity-recognition #multilinguality-multilingual #size_categories-10K<100k #language-Achinese #language-Bulgarian #language-Danish #language-Friulian #language-Iloko #language-Ligurian #language-Mazanderani #language-Quechua #language-Sundanese #language-Vietnamese #language-Afrikaans #language-bh #language-German #language-Western Frisian #language-Ido #language-Lombard #language-Neapolitan #language-Romansh #language-Swedish #language-Vlaams #language-Tosk Albanian #language-Bengali #language-Dimli (individual language) #language-Irish #language-Icelandic #language-Lingala #language-Low German #language-Romanian #language-Swahili (macrolanguage) #language-Volapรผk #language-Amharic #language-Tibetan #language-Dhivehi #language-Gan Chinese #language-Italian #language-Lithuanian #language-Nepali (macrolanguage) #language-Russian #language-Silesian #language-Walloon #language-Aragonese #language-Breton #language-Modern Greek (1453-) #language-Scottish Gaelic #language-Japanese #language-Latvian #language-Dutch #language-Kinyarwanda #language-Tamil #language-Waray (Philippines) #language-Old English (ca. 450-1100) #language-Bosnian #language-Emiliano-Romagnolo #language-Galician #language-Lojban #language-Norwegian Nynorsk #language-Sanskrit #language-Telugu #language-Wu Chinese #language-Arabic #language-Catalan #language-English #language-Guarani #language-Javanese #language-Malagasy #language-Norwegian #language-Yakut #language-Tajik #language-Mingrelian #language-Official Aramaic (700-300 BCE) #language-Esperanto #language-Gujarati #language-Georgian #language-Eastern Mari #language-Novial #language-Sicilian #language-Thai #language-Yiddish #language-Egyptian Arabic #language-Min Dong Chinese #language-Spanish #language-Hakka Chinese #language-Kazakh #language-Maori #language-Occitan (post 1500) #language-Scots #language-Turkmen #language-Yoruba #language-Assamese #language-Chechen #language-Estonian #language-Hebrew #language-Khmer #language-Minangkabau #language-Oriya (macrolanguage) #language-Sindhi #language-Tagalog #language-Zeeuws #language-Asturian #language-Cebuano #language-Basque #language-Hindi #language-Kannada #language-Macedonian #language-Ossetian #language-Serbo-Croatian #language-Turkish #language-Aymara #language-Central Kurdish #language-Extremaduran #language-Croatian #language-Korean #language-Malayalam #language-Panjabi #language-Sinhala #language-Tatar #language-Azerbaijani #language-Corsican #language-Persian #language-Upper Sorbian #language-Kรถlsch #language-Mongolian #language-Pennsylvania German #language-Uighur #language-Bashkir #language-Crimean Tatar #language-Finnish #language-Hungarian #language-Kurdish #language-Marathi #language-Polish #language-Slovak #language-Ukrainian #language-Chinese #language-Bavarian #language-Czech #language-Armenian #language-Kirghiz #language-Malay (macrolanguage) #language-Piemontese #language-Slovenian #language-Urdu #language-Kashubian #language-Faroese #language-Interlingua (International Auxiliary Language Association) #language-Latin #language-Maltese #language-Western Panjabi #language-Somali #language-Uzbek #language-Chuvash #language-French #language-Indonesian #language-Luxembourgish #language-Mirandese #language-Pushto #language-Albanian #language-Venetian #language-Belarusian #language-Welsh #language-Northern Frisian #language-Igbo #language-Limburgan #language-Burmese #language-Portuguese #language-Serbian #region-us
| Dataset Card for "tner/wikiann"
===============================
Dataset Description
-------------------
* Repository: T-NER
* Paper: URL
* Dataset: WikiAnn
* Domain: Wikipedia
* Number of Entity: 3
### Dataset Summary
WikiAnn NER dataset formatted in a part of TNER project.
* Entity Types: 'LOC', 'ORG', 'PER'
Dataset Structure
-----------------
### Data Instances
An example of 'train' of 'ja' looks as follows.
### Label ID
The label2id dictionary can be found at here.
### Data Splits
| [
"### Dataset Summary\n\n\nWikiAnn NER dataset formatted in a part of TNER project.\n\n\n* Entity Types: 'LOC', 'ORG', 'PER'\n\n\nDataset Structure\n-----------------",
"### Data Instances\n\n\nAn example of 'train' of 'ja' looks as follows.",
"### Label ID\n\n\nThe label2id dictionary can be found at here.",
"### Data Splits"
] | [
"TAGS\n#task_categories-token-classification #task_ids-named-entity-recognition #multilinguality-multilingual #size_categories-10K<100k #language-Achinese #language-Bulgarian #language-Danish #language-Friulian #language-Iloko #language-Ligurian #language-Mazanderani #language-Quechua #language-Sundanese #language-Vietnamese #language-Afrikaans #language-bh #language-German #language-Western Frisian #language-Ido #language-Lombard #language-Neapolitan #language-Romansh #language-Swedish #language-Vlaams #language-Tosk Albanian #language-Bengali #language-Dimli (individual language) #language-Irish #language-Icelandic #language-Lingala #language-Low German #language-Romanian #language-Swahili (macrolanguage) #language-Volapรผk #language-Amharic #language-Tibetan #language-Dhivehi #language-Gan Chinese #language-Italian #language-Lithuanian #language-Nepali (macrolanguage) #language-Russian #language-Silesian #language-Walloon #language-Aragonese #language-Breton #language-Modern Greek (1453-) #language-Scottish Gaelic #language-Japanese #language-Latvian #language-Dutch #language-Kinyarwanda #language-Tamil #language-Waray (Philippines) #language-Old English (ca. 450-1100) #language-Bosnian #language-Emiliano-Romagnolo #language-Galician #language-Lojban #language-Norwegian Nynorsk #language-Sanskrit #language-Telugu #language-Wu Chinese #language-Arabic #language-Catalan #language-English #language-Guarani #language-Javanese #language-Malagasy #language-Norwegian #language-Yakut #language-Tajik #language-Mingrelian #language-Official Aramaic (700-300 BCE) #language-Esperanto #language-Gujarati #language-Georgian #language-Eastern Mari #language-Novial #language-Sicilian #language-Thai #language-Yiddish #language-Egyptian Arabic #language-Min Dong Chinese #language-Spanish #language-Hakka Chinese #language-Kazakh #language-Maori #language-Occitan (post 1500) #language-Scots #language-Turkmen #language-Yoruba #language-Assamese #language-Chechen #language-Estonian #language-Hebrew #language-Khmer #language-Minangkabau #language-Oriya (macrolanguage) #language-Sindhi #language-Tagalog #language-Zeeuws #language-Asturian #language-Cebuano #language-Basque #language-Hindi #language-Kannada #language-Macedonian #language-Ossetian #language-Serbo-Croatian #language-Turkish #language-Aymara #language-Central Kurdish #language-Extremaduran #language-Croatian #language-Korean #language-Malayalam #language-Panjabi #language-Sinhala #language-Tatar #language-Azerbaijani #language-Corsican #language-Persian #language-Upper Sorbian #language-Kรถlsch #language-Mongolian #language-Pennsylvania German #language-Uighur #language-Bashkir #language-Crimean Tatar #language-Finnish #language-Hungarian #language-Kurdish #language-Marathi #language-Polish #language-Slovak #language-Ukrainian #language-Chinese #language-Bavarian #language-Czech #language-Armenian #language-Kirghiz #language-Malay (macrolanguage) #language-Piemontese #language-Slovenian #language-Urdu #language-Kashubian #language-Faroese #language-Interlingua (International Auxiliary Language Association) #language-Latin #language-Maltese #language-Western Panjabi #language-Somali #language-Uzbek #language-Chuvash #language-French #language-Indonesian #language-Luxembourgish #language-Mirandese #language-Pushto #language-Albanian #language-Venetian #language-Belarusian #language-Welsh #language-Northern Frisian #language-Igbo #language-Limburgan #language-Burmese #language-Portuguese #language-Serbian #region-us \n",
"### Dataset Summary\n\n\nWikiAnn NER dataset formatted in a part of TNER project.\n\n\n* Entity Types: 'LOC', 'ORG', 'PER'\n\n\nDataset Structure\n-----------------",
"### Data Instances\n\n\nAn example of 'train' of 'ja' looks as follows.",
"### Label ID\n\n\nThe label2id dictionary can be found at here.",
"### Data Splits"
] | [
1080,
47,
22,
17,
5
] | [
"passage: "
] |
ce7483a909a7b68ddc02920087462355f7680057 |
# Dataset Card for "tner/wikineural"
## Dataset Description
- **Repository:** [T-NER](https://github.com/asahi417/tner)
- **Paper:** [https://aclanthology.org/2021.findings-emnlp.215/](https://aclanthology.org/2021.findings-emnlp.215/)
- **Dataset:** WikiNeural
- **Domain:** Wikipedia
- **Number of Entity:** 16
### Dataset Summary
WikiAnn NER dataset formatted in a part of [TNER](https://github.com/asahi417/tner) project.
- Entity Types: `PER`, `LOC`, `ORG`, `ANIM`, `BIO`, `CEL`, `DIS`, `EVE`, `FOOD`, `INST`, `MEDIA`, `PLANT`, `MYTH`, `TIME`, `VEHI`, `MISC`
## Dataset Structure
### Data Instances
An example of `train` of `de` looks as follows.
```
{
'tokens': [ "Dieses", "wiederum", "basierte", "auf", "dem", "gleichnamigen", "Roman", "von", "Noรซl", "Calef", "." ],
'tags': [ 0, 0, 0, 0, 0, 0, 0, 0, 1, 2, 0 ]
}
```
### Label ID
The label2id dictionary can be found at [here](https://huggingface.co/datasets/tner/wikineural/raw/main/dataset/label.json).
```python
{
"O": 0,
"B-PER": 1,
"I-PER": 2,
"B-LOC": 3,
"I-LOC": 4,
"B-ORG": 5,
"I-ORG": 6,
"B-ANIM": 7,
"I-ANIM": 8,
"B-BIO": 9,
"I-BIO": 10,
"B-CEL": 11,
"I-CEL": 12,
"B-DIS": 13,
"I-DIS": 14,
"B-EVE": 15,
"I-EVE": 16,
"B-FOOD": 17,
"I-FOOD": 18,
"B-INST": 19,
"I-INST": 20,
"B-MEDIA": 21,
"I-MEDIA": 22,
"B-PLANT": 23,
"I-PLANT": 24,
"B-MYTH": 25,
"I-MYTH": 26,
"B-TIME": 27,
"I-TIME": 28,
"B-VEHI": 29,
"I-VEHI": 30,
"B-MISC": 31,
"I-MISC": 32
}
```
### Data Splits
| language | train | validation | test |
|:-----------|--------:|-------------:|-------:|
| de | 98640 | 12330 | 12372 |
| en | 92720 | 11590 | 11597 |
| es | 76320 | 9540 | 9618 |
| fr | 100800 | 12600 | 12678 |
| it | 88400 | 11050 | 11069 |
| nl | 83680 | 10460 | 10547 |
| pl | 108160 | 13520 | 13585 |
| pt | 80560 | 10070 | 10160 |
| ru | 92320 | 11540 | 11580 |
### Citation Information
```
@inproceedings{tedeschi-etal-2021-wikineural-combined,
title = "{W}iki{NE}u{R}al: {C}ombined Neural and Knowledge-based Silver Data Creation for Multilingual {NER}",
author = "Tedeschi, Simone and
Maiorca, Valentino and
Campolungo, Niccol{\`o} and
Cecconi, Francesco and
Navigli, Roberto",
booktitle = "Findings of the Association for Computational Linguistics: EMNLP 2021",
month = nov,
year = "2021",
address = "Punta Cana, Dominican Republic",
publisher = "Association for Computational Linguistics",
url = "https://aclanthology.org/2021.findings-emnlp.215",
doi = "10.18653/v1/2021.findings-emnlp.215",
pages = "2521--2533",
abstract = "Multilingual Named Entity Recognition (NER) is a key intermediate task which is needed in many areas of NLP. In this paper, we address the well-known issue of data scarcity in NER, especially relevant when moving to a multilingual scenario, and go beyond current approaches to the creation of multilingual silver data for the task. We exploit the texts of Wikipedia and introduce a new methodology based on the effective combination of knowledge-based approaches and neural models, together with a novel domain adaptation technique, to produce high-quality training corpora for NER. We evaluate our datasets extensively on standard benchmarks for NER, yielding substantial improvements up to 6 span-based F1-score points over previous state-of-the-art systems for data creation.",
}
``` | tner/wikineural | [
"task_categories:token-classification",
"task_ids:named-entity-recognition",
"multilinguality:multilingual",
"size_categories:10K<100k",
"language:de",
"language:en",
"language:es",
"language:fr",
"language:it",
"language:nl",
"language:pl",
"language:pt",
"language:ru",
"region:us"
] | 2022-09-27T16:56:40+00:00 | {"language": ["de", "en", "es", "fr", "it", "nl", "pl", "pt", "ru"], "multilinguality": ["multilingual"], "size_categories": ["10K<100k"], "task_categories": ["token-classification"], "task_ids": ["named-entity-recognition"], "pretty_name": "WikiNeural"} | 2022-09-27T18:46:37+00:00 | [] | [
"de",
"en",
"es",
"fr",
"it",
"nl",
"pl",
"pt",
"ru"
] | TAGS
#task_categories-token-classification #task_ids-named-entity-recognition #multilinguality-multilingual #size_categories-10K<100k #language-German #language-English #language-Spanish #language-French #language-Italian #language-Dutch #language-Polish #language-Portuguese #language-Russian #region-us
| Dataset Card for "tner/wikineural"
==================================
Dataset Description
-------------------
* Repository: T-NER
* Paper: URL
* Dataset: WikiNeural
* Domain: Wikipedia
* Number of Entity: 16
### Dataset Summary
WikiAnn NER dataset formatted in a part of TNER project.
* Entity Types: 'PER', 'LOC', 'ORG', 'ANIM', 'BIO', 'CEL', 'DIS', 'EVE', 'FOOD', 'INST', 'MEDIA', 'PLANT', 'MYTH', 'TIME', 'VEHI', 'MISC'
Dataset Structure
-----------------
### Data Instances
An example of 'train' of 'de' looks as follows.
### Label ID
The label2id dictionary can be found at here.
### Data Splits
| [
"### Dataset Summary\n\n\nWikiAnn NER dataset formatted in a part of TNER project.\n\n\n* Entity Types: 'PER', 'LOC', 'ORG', 'ANIM', 'BIO', 'CEL', 'DIS', 'EVE', 'FOOD', 'INST', 'MEDIA', 'PLANT', 'MYTH', 'TIME', 'VEHI', 'MISC'\n\n\nDataset Structure\n-----------------",
"### Data Instances\n\n\nAn example of 'train' of 'de' looks as follows.",
"### Label ID\n\n\nThe label2id dictionary can be found at here.",
"### Data Splits"
] | [
"TAGS\n#task_categories-token-classification #task_ids-named-entity-recognition #multilinguality-multilingual #size_categories-10K<100k #language-German #language-English #language-Spanish #language-French #language-Italian #language-Dutch #language-Polish #language-Portuguese #language-Russian #region-us \n",
"### Dataset Summary\n\n\nWikiAnn NER dataset formatted in a part of TNER project.\n\n\n* Entity Types: 'PER', 'LOC', 'ORG', 'ANIM', 'BIO', 'CEL', 'DIS', 'EVE', 'FOOD', 'INST', 'MEDIA', 'PLANT', 'MYTH', 'TIME', 'VEHI', 'MISC'\n\n\nDataset Structure\n-----------------",
"### Data Instances\n\n\nAn example of 'train' of 'de' looks as follows.",
"### Label ID\n\n\nThe label2id dictionary can be found at here.",
"### Data Splits"
] | [
97,
107,
22,
17,
5
] | [
"passage: TAGS\n#task_categories-token-classification #task_ids-named-entity-recognition #multilinguality-multilingual #size_categories-10K<100k #language-German #language-English #language-Spanish #language-French #language-Italian #language-Dutch #language-Polish #language-Portuguese #language-Russian #region-us \n### Dataset Summary\n\n\nWikiAnn NER dataset formatted in a part of TNER project.\n\n\n* Entity Types: 'PER', 'LOC', 'ORG', 'ANIM', 'BIO', 'CEL', 'DIS', 'EVE', 'FOOD', 'INST', 'MEDIA', 'PLANT', 'MYTH', 'TIME', 'VEHI', 'MISC'\n\n\nDataset Structure\n-----------------### Data Instances\n\n\nAn example of 'train' of 'de' looks as follows.### Label ID\n\n\nThe label2id dictionary can be found at here.### Data Splits"
] |
3c9285ea8a531da6066ac04bb17394bc8e8ca3b6 | # Dataset Card for "pip"
[More Information needed](https://github.com/huggingface/datasets/blob/main/CONTRIBUTING.md#how-to-contribute-to-the-dataset-cards) | open-source-metrics/pip | [
"region:us"
] | 2022-09-27T17:19:45+00:00 | {"dataset_info": {"features": [{"name": "day", "dtype": "string"}, {"name": "num_downloads", "dtype": "int64"}], "splits": [{"name": "gradio", "num_bytes": 27742, "num_examples": 1261}, {"name": "safetensors", "num_bytes": 9812, "num_examples": 446}, {"name": "optimum", "num_bytes": 19360, "num_examples": 880}, {"name": "evaluate", "num_bytes": 16346, "num_examples": 743}, {"name": "huggingface_hub", "num_bytes": 25256, "num_examples": 1148}, {"name": "pytorch_image_models", "num_bytes": 27742, "num_examples": 1261}, {"name": "accelerate", "num_bytes": 24376, "num_examples": 1108}, {"name": "tokenizers", "num_bytes": 27742, "num_examples": 1261}, {"name": "transformers", "num_bytes": 28424, "num_examples": 1292}, {"name": "peft", "num_bytes": 8602, "num_examples": 391}, {"name": "diffusers", "num_bytes": 13750, "num_examples": 625}, {"name": "datasets", "num_bytes": 24376, "num_examples": 1108}], "download_size": 148060, "dataset_size": 253528}, "configs": [{"config_name": "default", "data_files": [{"split": "accelerate", "path": "data/accelerate-*"}, {"split": "datasets", "path": "data/datasets-*"}, {"split": "diffusers", "path": "data/diffusers-*"}, {"split": "evaluate", "path": "data/evaluate-*"}, {"split": "gradio", "path": "data/gradio-*"}, {"split": "huggingface_hub", "path": "data/huggingface_hub-*"}, {"split": "optimum", "path": "data/optimum-*"}, {"split": "peft", "path": "data/peft-*"}, {"split": "pytorch_image_models", "path": "data/pytorch_image_models-*"}, {"split": "safetensors", "path": "data/safetensors-*"}, {"split": "tokenizers", "path": "data/tokenizers-*"}, {"split": "transformers", "path": "data/transformers-*"}]}]} | 2024-02-15T11:18:27+00:00 | [] | [] | TAGS
#region-us
| # Dataset Card for "pip"
More Information needed | [
"# Dataset Card for \"pip\"\n\nMore Information needed"
] | [
"TAGS\n#region-us \n",
"# Dataset Card for \"pip\"\n\nMore Information needed"
] | [
6,
12
] | [
"passage: TAGS\n#region-us \n# Dataset Card for \"pip\"\n\nMore Information needed"
] |
facdfd1c6f139820e44b5dd7b341d056fbe2044e |
# Dataset Card for "tner/multinerd"
## Dataset Description
- **Repository:** [T-NER](https://github.com/asahi417/tner)
- **Paper:** [https://aclanthology.org/2022.findings-naacl.60/](https://aclanthology.org/2022.findings-naacl.60/)
- **Dataset:** MultiNERD
- **Domain:** Wikipedia, WikiNews
- **Number of Entity:** 18
### Dataset Summary
MultiNERD NER benchmark dataset formatted in a part of [TNER](https://github.com/asahi417/tner) project.
- Entity Types: `PER`, `LOC`, `ORG`, `ANIM`, `BIO`, `CEL`, `DIS`, `EVE`, `FOOD`, `INST`, `MEDIA`, `PLANT`, `MYTH`, `TIME`, `VEHI`, `MISC`, `SUPER`, `PHY`
## Dataset Structure
### Data Instances
An example of `train` of `de` looks as follows.
```
{
'tokens': [ "Die", "Blรคtter", "des", "Huflattichs", "sind", "leicht", "mit", "den", "sehr", "รคhnlichen", "Blรคttern", "der", "Weiรen", "Pestwurz", "(", "\"", "Petasites", "albus", "\"", ")", "zu", "verwechseln", "." ],
'tags': [ 0, 0, 0, 0, 0, 0, 0, 0, 0, 0, 0, 0, 0, 0, 0, 0, 0, 0, 0, 0, 0, 0, 0, 0, 0, 0, 0, 0, 0, 0, 0, 0, 0, 0, 0, 0, 0, 0, 0, 0, 0, 0, 0, 1, 2, 0, 0, 0 ]
}
```
### Label ID
The label2id dictionary can be found at [here](https://huggingface.co/datasets/tner/multinerd/raw/main/dataset/label.json).
```python
{
"O": 0,
"B-PER": 1,
"I-PER": 2,
"B-LOC": 3,
"I-LOC": 4,
"B-ORG": 5,
"I-ORG": 6,
"B-ANIM": 7,
"I-ANIM": 8,
"B-BIO": 9,
"I-BIO": 10,
"B-CEL": 11,
"I-CEL": 12,
"B-DIS": 13,
"I-DIS": 14,
"B-EVE": 15,
"I-EVE": 16,
"B-FOOD": 17,
"I-FOOD": 18,
"B-INST": 19,
"I-INST": 20,
"B-MEDIA": 21,
"I-MEDIA": 22,
"B-PLANT": 23,
"I-PLANT": 24,
"B-MYTH": 25,
"I-MYTH": 26,
"B-TIME": 27,
"I-TIME": 28,
"B-VEHI": 29,
"I-VEHI": 30,
"B-SUPER": 31,
"I-SUPER": 32,
"B-PHY": 33,
"I-PHY": 34
}
```
### Data Splits
| language | test |
|:-----------|-------:|
| de | 156792 |
| en | 164144 |
| es | 173189 |
| fr | 176185 |
| it | 181927 |
| nl | 171711 |
| pl | 194965 |
| pt | 177565 |
| ru | 82858 |
### Citation Information
```
@inproceedings{tedeschi-navigli-2022-multinerd,
title = "{M}ulti{NERD}: A Multilingual, Multi-Genre and Fine-Grained Dataset for Named Entity Recognition (and Disambiguation)",
author = "Tedeschi, Simone and
Navigli, Roberto",
booktitle = "Findings of the Association for Computational Linguistics: NAACL 2022",
month = jul,
year = "2022",
address = "Seattle, United States",
publisher = "Association for Computational Linguistics",
url = "https://aclanthology.org/2022.findings-naacl.60",
doi = "10.18653/v1/2022.findings-naacl.60",
pages = "801--812",
abstract = "Named Entity Recognition (NER) is the task of identifying named entities in texts and classifying them through specific semantic categories, a process which is crucial for a wide range of NLP applications. Current datasets for NER focus mainly on coarse-grained entity types, tend to consider a single textual genre and to cover a narrow set of languages, thus limiting the general applicability of NER systems.In this work, we design a new methodology for automatically producing NER annotations, and address the aforementioned limitations by introducing a novel dataset that covers 10 languages, 15 NER categories and 2 textual genres.We also introduce a manually-annotated test set, and extensively evaluate the quality of our novel dataset on both this new test set and standard benchmarks for NER.In addition, in our dataset, we include: i) disambiguation information to enable the development of multilingual entity linking systems, and ii) image URLs to encourage the creation of multimodal systems.We release our dataset at https://github.com/Babelscape/multinerd.",
}
``` | tner/multinerd | [
"task_categories:token-classification",
"task_ids:named-entity-recognition",
"multilinguality:multilingual",
"size_categories:<10K",
"language:de",
"language:en",
"language:es",
"language:fr",
"language:it",
"language:nl",
"language:pl",
"language:pt",
"language:ru",
"region:us"
] | 2022-09-27T18:13:36+00:00 | {"language": ["de", "en", "es", "fr", "it", "nl", "pl", "pt", "ru"], "multilinguality": ["multilingual"], "size_categories": ["<10K"], "task_categories": ["token-classification"], "task_ids": ["named-entity-recognition"], "pretty_name": "MultiNERD"} | 2022-09-27T18:48:40+00:00 | [] | [
"de",
"en",
"es",
"fr",
"it",
"nl",
"pl",
"pt",
"ru"
] | TAGS
#task_categories-token-classification #task_ids-named-entity-recognition #multilinguality-multilingual #size_categories-<10K #language-German #language-English #language-Spanish #language-French #language-Italian #language-Dutch #language-Polish #language-Portuguese #language-Russian #region-us
| Dataset Card for "tner/multinerd"
=================================
Dataset Description
-------------------
* Repository: T-NER
* Paper: URL
* Dataset: MultiNERD
* Domain: Wikipedia, WikiNews
* Number of Entity: 18
### Dataset Summary
MultiNERD NER benchmark dataset formatted in a part of TNER project.
* Entity Types: 'PER', 'LOC', 'ORG', 'ANIM', 'BIO', 'CEL', 'DIS', 'EVE', 'FOOD', 'INST', 'MEDIA', 'PLANT', 'MYTH', 'TIME', 'VEHI', 'MISC', 'SUPER', 'PHY'
Dataset Structure
-----------------
### Data Instances
An example of 'train' of 'de' looks as follows.
### Label ID
The label2id dictionary can be found at here.
### Data Splits
| [
"### Dataset Summary\n\n\nMultiNERD NER benchmark dataset formatted in a part of TNER project.\n\n\n* Entity Types: 'PER', 'LOC', 'ORG', 'ANIM', 'BIO', 'CEL', 'DIS', 'EVE', 'FOOD', 'INST', 'MEDIA', 'PLANT', 'MYTH', 'TIME', 'VEHI', 'MISC', 'SUPER', 'PHY'\n\n\nDataset Structure\n-----------------",
"### Data Instances\n\n\nAn example of 'train' of 'de' looks as follows.",
"### Label ID\n\n\nThe label2id dictionary can be found at here.",
"### Data Splits"
] | [
"TAGS\n#task_categories-token-classification #task_ids-named-entity-recognition #multilinguality-multilingual #size_categories-<10K #language-German #language-English #language-Spanish #language-French #language-Italian #language-Dutch #language-Polish #language-Portuguese #language-Russian #region-us \n",
"### Dataset Summary\n\n\nMultiNERD NER benchmark dataset formatted in a part of TNER project.\n\n\n* Entity Types: 'PER', 'LOC', 'ORG', 'ANIM', 'BIO', 'CEL', 'DIS', 'EVE', 'FOOD', 'INST', 'MEDIA', 'PLANT', 'MYTH', 'TIME', 'VEHI', 'MISC', 'SUPER', 'PHY'\n\n\nDataset Structure\n-----------------",
"### Data Instances\n\n\nAn example of 'train' of 'de' looks as follows.",
"### Label ID\n\n\nThe label2id dictionary can be found at here.",
"### Data Splits"
] | [
96,
118,
22,
17,
5
] | [
"passage: TAGS\n#task_categories-token-classification #task_ids-named-entity-recognition #multilinguality-multilingual #size_categories-<10K #language-German #language-English #language-Spanish #language-French #language-Italian #language-Dutch #language-Polish #language-Portuguese #language-Russian #region-us \n### Dataset Summary\n\n\nMultiNERD NER benchmark dataset formatted in a part of TNER project.\n\n\n* Entity Types: 'PER', 'LOC', 'ORG', 'ANIM', 'BIO', 'CEL', 'DIS', 'EVE', 'FOOD', 'INST', 'MEDIA', 'PLANT', 'MYTH', 'TIME', 'VEHI', 'MISC', 'SUPER', 'PHY'\n\n\nDataset Structure\n-----------------### Data Instances\n\n\nAn example of 'train' of 'de' looks as follows.### Label ID\n\n\nThe label2id dictionary can be found at here.### Data Splits"
] |
ebbb3a2ae953c0a73ab3db40e849c6c23a82542a |
---
Sample
---
- 6900 transcripts
- 44 churches
- timeframe: 2010-2022
- Denomination: Unitarian Universalist, USA
---
Dataset structure
---
- church (church name or website)
- source (mp3 file)
- text
- sentences (count)
- errors (number of sentences skipped because could not understand audio, or just long pauses skipped)
- duration (in seconds)
---
Dataset creation
---
- see notebook in files
| marcmaxmeister/unitarian-universalist-sermons | [
"license:mit",
"region:us"
] | 2022-09-27T21:11:20+00:00 | {"license": "mit"} | 2022-09-28T20:04:16+00:00 | [] | [] | TAGS
#license-mit #region-us
|
---
Sample
---
- 6900 transcripts
- 44 churches
- timeframe: 2010-2022
- Denomination: Unitarian Universalist, USA
---
Dataset structure
---
- church (church name or website)
- source (mp3 file)
- text
- sentences (count)
- errors (number of sentences skipped because could not understand audio, or just long pauses skipped)
- duration (in seconds)
---
Dataset creation
---
- see notebook in files
| [] | [
"TAGS\n#license-mit #region-us \n"
] | [
11
] | [
"passage: TAGS\n#license-mit #region-us \n"
] |
6947305648990c358f904def2a18cc3cc62fd4c0 |
The code is provided under a Attribution-NonCommercial 4.0 International (CC BY-NC 4.0) license. Under the license, the code is provided royalty free for non-commercial purposes only. The code may be covered by patents and if you want to use the code for commercial purposes, please contact us for a different license.
This dataset is a pre-processed small sample of the Waymo Open Motion Dataset intended for illustration purposes only.
| jmercat/risk_biased_dataset | [
"license:cc-by-nc-4.0",
"region:us"
] | 2022-09-27T21:35:21+00:00 | {"license": "cc-by-nc-4.0"} | 2023-08-01T18:08:31+00:00 | [] | [] | TAGS
#license-cc-by-nc-4.0 #region-us
|
The code is provided under a Attribution-NonCommercial 4.0 International (CC BY-NC 4.0) license. Under the license, the code is provided royalty free for non-commercial purposes only. The code may be covered by patents and if you want to use the code for commercial purposes, please contact us for a different license.
This dataset is a pre-processed small sample of the Waymo Open Motion Dataset intended for illustration purposes only.
| [] | [
"TAGS\n#license-cc-by-nc-4.0 #region-us \n"
] | [
17
] | [
"passage: TAGS\n#license-cc-by-nc-4.0 #region-us \n"
] |
5e92c47f62e3a16dc4b38ed70aa8841eacb22514 | ---
annotations_creators:
- expert-generated
language:
- en
language_creators:
- found
license:
- mit
multilinguality:
- monolingual
paperswithcode_id: acronym-identification
pretty_name: datahogyas
size_categories:
- 10K<n<100K
source_datasets:
- original
tags: []
task_categories:
- token-classification
task_ids:
- part-of-speech
train-eval-index:
- col_mapping:
labels: tags
tokens: tokens
config: default
splits:
eval_split: test
task: token-classification
task_id: entity_extraction
--- | dhruvs00/datahogyas | [
"region:us"
] | 2022-09-28T05:47:21+00:00 | {} | 2022-09-28T07:08:02+00:00 | [] | [] | TAGS
#region-us
| ---
annotations_creators:
- expert-generated
language:
- en
language_creators:
- found
license:
- mit
multilinguality:
- monolingual
paperswithcode_id: acronym-identification
pretty_name: datahogyas
size_categories:
- 10K<n<100K
source_datasets:
- original
tags: []
task_categories:
- token-classification
task_ids:
- part-of-speech
train-eval-index:
- col_mapping:
labels: tags
tokens: tokens
config: default
splits:
eval_split: test
task: token-classification
task_id: entity_extraction
--- | [] | [
"TAGS\n#region-us \n"
] | [
6
] | [
"passage: TAGS\n#region-us \n"
] |
5e2a11d9729621f0375b6ccd1114d335c6ee1b94 | # Dummy Dataset for AutoTrain Benchmark
This dataset contains dummy data that's needed to create AutoTrain projects for benchmarks like [RAFT](https://huggingface.co/spaces/ought/raft-leaderboard). See [here](https://github.com/huggingface/hf_benchmarks) for more details. | autoevaluator/benchmark-dummy-data | [
"region:us"
] | 2022-09-28T06:57:08+00:00 | {} | 2022-11-18T13:19:56+00:00 | [] | [] | TAGS
#region-us
| # Dummy Dataset for AutoTrain Benchmark
This dataset contains dummy data that's needed to create AutoTrain projects for benchmarks like RAFT. See here for more details. | [
"# Dummy Dataset for AutoTrain Benchmark\n\nThis dataset contains dummy data that's needed to create AutoTrain projects for benchmarks like RAFT. See here for more details."
] | [
"TAGS\n#region-us \n",
"# Dummy Dataset for AutoTrain Benchmark\n\nThis dataset contains dummy data that's needed to create AutoTrain projects for benchmarks like RAFT. See here for more details."
] | [
6,
43
] | [
"passage: TAGS\n#region-us \n# Dummy Dataset for AutoTrain Benchmark\n\nThis dataset contains dummy data that's needed to create AutoTrain projects for benchmarks like RAFT. See here for more details."
] |
519a29f2934a650967d7c6c99f4c53ed99e083d0 | # dureader
ๆฐๆฎๆฅ่ชDuReader-Retrevalๆฐๆฎ้๏ผ่ฟ้ๆฏ[ๅๅงๅฐๅ](https://github.com/baidu/DuReader/tree/master/DuReader-Retrieval)ใ
> ๆฌๆฐๆฎ้ๅช็จไฝๅญฆๆฏ็ ็ฉถไฝฟ็จใๅฆๆๆฌไปๅบๆถๅไพตๆ่กไธบ๏ผไผ็ซๅณๅ ้คใ
| zyznull/dureader-retrieval-corpus | [
"license:apache-2.0",
"region:us"
] | 2022-09-28T07:03:03+00:00 | {"license": "apache-2.0"} | 2023-01-03T08:05:06+00:00 | [] | [] | TAGS
#license-apache-2.0 #region-us
| # dureader
ๆฐๆฎๆฅ่ชDuReader-Retrevalๆฐๆฎ้๏ผ่ฟ้ๆฏๅๅงๅฐๅใ
> ๆฌๆฐๆฎ้ๅช็จไฝๅญฆๆฏ็ ็ฉถไฝฟ็จใๅฆๆๆฌไปๅบๆถๅไพตๆ่กไธบ๏ผไผ็ซๅณๅ ้คใ
| [
"# dureader\n\nๆฐๆฎๆฅ่ชDuReader-Retrevalๆฐๆฎ้๏ผ่ฟ้ๆฏๅๅงๅฐๅใ\n\n> ๆฌๆฐๆฎ้ๅช็จไฝๅญฆๆฏ็ ็ฉถไฝฟ็จใๅฆๆๆฌไปๅบๆถๅไพตๆ่กไธบ๏ผไผ็ซๅณๅ ้คใ"
] | [
"TAGS\n#license-apache-2.0 #region-us \n",
"# dureader\n\nๆฐๆฎๆฅ่ชDuReader-Retrevalๆฐๆฎ้๏ผ่ฟ้ๆฏๅๅงๅฐๅใ\n\n> ๆฌๆฐๆฎ้ๅช็จไฝๅญฆๆฏ็ ็ฉถไฝฟ็จใๅฆๆๆฌไปๅบๆถๅไพตๆ่กไธบ๏ผไผ็ซๅณๅ ้คใ"
] | [
14,
43
] | [
"passage: TAGS\n#license-apache-2.0 #region-us \n# dureader\n\nๆฐๆฎๆฅ่ชDuReader-Retrevalๆฐๆฎ้๏ผ่ฟ้ๆฏๅๅงๅฐๅใ\n\n> ๆฌๆฐๆฎ้ๅช็จไฝๅญฆๆฏ็ ็ฉถไฝฟ็จใๅฆๆๆฌไปๅบๆถๅไพตๆ่กไธบ๏ผไผ็ซๅณๅ ้คใ"
] |
f33c72ade15f98638f3598a9ca4ac989d21f699e |
All eight of datasets in ESC can be downloaded and prepared in just a single line of code through the Hugging Face Datasets library:
```python
from datasets import load_dataset
librispeech = load_dataset("esc-benchmark/esc-datasets", "librispeech", split="train")
```
- `"esc-benchmark"`: the repository namespace. This is fixed for all ESC datasets.
- `"librispeech"`: the dataset name. This can be changed to any of any one of the eight datasets in ESC to download that dataset.
- `split="train"`: the split. Set this to one of train/validation/test to generate a specific split. Omit the `split` argument to generate all splits for a dataset.
The datasets are full prepared, such that the audio and transcription files can be used directly in training/evaluation scripts.
## Dataset Information
A data point can be accessed by indexing the dataset object loaded through `load_dataset`:
```python
print(librispeech[0])
```
A typical data point comprises the path to the audio file and its transcription. Also included is information of the dataset from which the sample derives and a unique identifier name:
```python
{
'dataset': 'librispeech',
'audio': {'path': '/home/esc-bencher/.cache/huggingface/datasets/downloads/extracted/d2da1969fe9e7d06661b5dc370cf2e3c119a14c35950045bcb76243b264e4f01/374-180298-0000.flac',
'array': array([ 7.01904297e-04, 7.32421875e-04, 7.32421875e-04, ...,
-2.74658203e-04, -1.83105469e-04, -3.05175781e-05]),
'sampling_rate': 16000},
'text': 'chapter sixteen i might have told you of the beginning of this liaison in a few lines but i wanted you to see every step by which we came i to agree to whatever marguerite wished',
'id': '374-180298-0000'
}
```
### Data Fields
- `dataset`: name of the ESC dataset from which the sample is taken.
- `audio`: a dictionary containing the path to the downloaded audio file, the decoded audio array, and the sampling rate.
- `text`: the transcription of the audio file.
- `id`: unique id of the data sample.
### Data Preparation
#### Audio
The audio for all ESC datasets is segmented into sample lengths suitable for training ASR systems. The Hugging Face datasets library decodes audio files on the fly, reading the segments and converting them to a Python arrays. Consequently, no further preparation of the audio is required to be used in training/evaluation scripts.
Note that when accessing the audio column: `dataset[0]["audio"]` the audio file is automatically decoded and resampled to `dataset.features["audio"].sampling_rate`. Decoding and resampling of a large number of audio files might take a significant amount of time. Thus it is important to first query the sample index before the `"audio"` column, i.e. `dataset[0]["audio"]` should always be preferred over `dataset["audio"][0]`.
#### Transcriptions
The transcriptions corresponding to each audio file are provided in their 'error corrected' format. No transcription pre-processing is applied to the text, only necessary 'error correction' steps such as removing junk tokens (_<unk>_) or converting symbolic punctuation to spelled out form (_<comma>_ to _,_). As such, no further preparation of the transcriptions is required to be used in training/evaluation scripts.
Transcriptions are provided for training and validation splits. The transcriptions are **not** provided for the test splits. The ESC benchmark requires you to generate predictions for the test sets and upload them to https://huggingface.co/spaces/esc-benchmark/esc for scoring.
### Access
All eight of the datasets in ESC are accessible and licensing is freely available. Three of the ESC datasets have specific terms of usage that must be agreed to before using the data. To do so, fill in the access forms on the specific datasets' pages:
* Common Voice: https://huggingface.co/datasets/mozilla-foundation/common_voice_9_0
* GigaSpeech: https://huggingface.co/datasets/speechcolab/gigaspeech
* SPGISpeech: https://huggingface.co/datasets/kensho/spgispeech
## LibriSpeech
The LibriSpeech corpus is a standard large-scale corpus for assessing ASR systems. It consists of approximately 1,000 hours of narrated audiobooks from the [LibriVox](https://librivox.org) project. It is licensed under CC-BY-4.0.
Example Usage:
```python
librispeech = load_dataset("esc-benchmark/esc-datasets", "librispeech")
```
Train/validation splits:
- `train` (combination of `train.clean.100`, `train.clean.360` and `train.other.500`)
- `validation.clean`
- `validation.other`
Test splits:
- `test.clean`
- `test.other`
Also available are subsets of the train split, which can be accessed by setting the `subconfig` argument:
```python
librispeech = load_dataset("esc-benchmark/esc-datasets", "librispeech", subconfig="clean.100")
```
- `clean.100`: 100 hours of training data from the 'clean' subset
- `clean.360`: 360 hours of training data from the 'clean' subset
- `other.500`: 500 hours of training data from the 'other' subset
## Common Voice
Common Voice is a series of crowd-sourced open-licensed speech datasets where speakers record text from Wikipedia in various languages. The English subset of contains approximately 1,400 hours of audio data from speakers of various nationalities, accents and different recording conditions. It is licensed under CC0-1.0.
Example usage:
```python
common_voice = load_dataset("esc-benchmark/esc-datasets", "common_voice", use_auth_token=True)
```
Training/validation splits:
- `train`
- `validation`
Test splits:
- `test`
## VoxPopuli
VoxPopuli s a large-scale multilingual speech corpus consisting of political data sourced from 2009-2020 European Parliament event recordings. The English subset contains approximately 550 hours of speech largely from non-native English speakers. It is licensed under CC0.
Example usage:
```python
voxpopuli = load_dataset("esc-benchmark/esc-datasets", "voxpopuli")
```
Training/validation splits:
- `train`
- `validation`
Test splits:
- `test`
## TED-LIUM
TED-LIUM consists of English-language TED Talk conference videos covering a range of different cultural, political, and academic topics. It contains approximately 450 hours of transcribed speech data. It is licensed under CC-BY-NC-ND 3.0.
Example usage:
```python
tedlium = load_dataset("esc-benchmark/esc-datasets", "tedlium")
```
Training/validation splits:
- `train`
- `validation`
Test splits:
- `test`
## GigaSpeech
GigaSpeech is a multi-domain English speech recognition corpus created from audiobooks, podcasts and YouTube. We provide the large train set (2,500 hours) and the standard validation and test splits. It is licensed under apache-2.0.
Example usage:
```python
gigaspeech = load_dataset("esc-benchmark/esc-datasets", "gigaspeech", use_auth_token=True)
```
Training/validation splits:
- `train` (`l` subset of training data (2,500 h))
- `validation`
Test splits:
- `test`
Also available are subsets of the train split, which can be accessed by setting the `subconfig` argument:
```python
gigaspeech = load_dataset("esc-benchmark/esc-datasets", "spgispeech", subconfig="xs", use_auth_token=True)
```
- `xs`: extra-small subset of training data (10 h)
- `s`: small subset of training data (250 h)
- `m`: medium subset of training data (1,000 h)
- `xl`: extra-large subset of training data (10,000 h)
## SPGISpeech
SPGISpeech consists of company earnings calls that have been manually transcribed by S&P Global, Inc according to a professional style guide. We provide the large train set (5,000 hours) and the standard validation and test splits. It is licensed under a Kensho user agreement.
Loading the dataset requires authorization.
Example usage:
```python
spgispeech = load_dataset("esc-benchmark/esc-datasets", "spgispeech", use_auth_token=True)
```
Training/validation splits:
- `train` (`l` subset of training data (~5,000 h))
- `validation`
Test splits:
- `test`
Also available are subsets of the train split, which can be accessed by setting the `subconfig` argument:
```python
spgispeech = load_dataset("esc-benchmark/esc-datasets", "spgispeech", subconfig="s", use_auth_token=True)
```
- `s`: small subset of training data (~200 h)
- `m`: medium subset of training data (~1,000 h)
## Earnings-22
Earnings-22 is a 119-hour corpus of English-language earnings calls collected from global companies, with speakers of many different nationalities and accents. It is licensed under CC-BY-SA-4.0.
Example usage:
```python
earnings22 = load_dataset("esc-benchmark/esc-datasets", "earnings22")
```
Training/validation splits:
- `train`
- `validation`
Test splits:
- `test`
## AMI
The AMI Meeting Corpus consists of 100 hours of meeting recordings from multiple recording devices synced to a common timeline. It is licensed under CC-BY-4.0.
Example usage:
```python
ami = load_dataset("esc-benchmark/esc-datasets", "ami")
```
Training/validation splits:
- `train`
- `validation`
Test splits:
- `test`
| esc-benchmark/esc-datasets | [
"task_categories:automatic-speech-recognition",
"annotations_creators:expert-generated",
"annotations_creators:crowdsourced",
"annotations_creators:machine-generated",
"language_creators:crowdsourced",
"language_creators:expert-generated",
"multilinguality:monolingual",
"size_categories:100K<n<1M",
"size_categories:1M<n<10M",
"source_datasets:original",
"source_datasets:extended|librispeech_asr",
"source_datasets:extended|common_voice",
"language:en",
"license:cc-by-4.0",
"license:apache-2.0",
"license:cc0-1.0",
"license:cc-by-nc-3.0",
"license:other",
"asr",
"benchmark",
"speech",
"esc",
"region:us"
] | 2022-09-28T07:40:04+00:00 | {"annotations_creators": ["expert-generated", "crowdsourced", "machine-generated"], "language_creators": ["crowdsourced", "expert-generated"], "language": ["en"], "license": ["cc-by-4.0", "apache-2.0", "cc0-1.0", "cc-by-nc-3.0", "other"], "multilinguality": ["monolingual"], "size_categories": ["100K<n<1M", "1M<n<10M"], "source_datasets": ["original", "extended|librispeech_asr", "extended|common_voice"], "task_categories": ["automatic-speech-recognition"], "task_ids": [], "pretty_name": "esc-datasets", "tags": ["asr", "benchmark", "speech", "esc"], "extra_gated_prompt": "Three of the ESC datasets have specific terms of usage that must be agreed to before using the data. \nTo do so, fill in the access forms on the specific datasets' pages:\n * Common Voice: https://huggingface.co/datasets/mozilla-foundation/common_voice_9_0\n * GigaSpeech: https://huggingface.co/datasets/speechcolab/gigaspeech\n * SPGISpeech: https://huggingface.co/datasets/kensho/spgispeech", "extra_gated_fields": {"I hereby confirm that I have registered on the original Common Voice page and agree to not attempt to determine the identity of speakers in the Common Voice dataset": "checkbox", "I hereby confirm that I have accepted the terms of usages on GigaSpeech page": "checkbox", "I hereby confirm that I have accepted the terms of usages on SPGISpeech page": "checkbox"}} | 2022-10-14T13:30:30+00:00 | [] | [
"en"
] | TAGS
#task_categories-automatic-speech-recognition #annotations_creators-expert-generated #annotations_creators-crowdsourced #annotations_creators-machine-generated #language_creators-crowdsourced #language_creators-expert-generated #multilinguality-monolingual #size_categories-100K<n<1M #size_categories-1M<n<10M #source_datasets-original #source_datasets-extended|librispeech_asr #source_datasets-extended|common_voice #language-English #license-cc-by-4.0 #license-apache-2.0 #license-cc0-1.0 #license-cc-by-nc-3.0 #license-other #asr #benchmark #speech #esc #region-us
|
All eight of datasets in ESC can be downloaded and prepared in just a single line of code through the Hugging Face Datasets library:
- '"esc-benchmark"': the repository namespace. This is fixed for all ESC datasets.
- '"librispeech"': the dataset name. This can be changed to any of any one of the eight datasets in ESC to download that dataset.
- 'split="train"': the split. Set this to one of train/validation/test to generate a specific split. Omit the 'split' argument to generate all splits for a dataset.
The datasets are full prepared, such that the audio and transcription files can be used directly in training/evaluation scripts.
## Dataset Information
A data point can be accessed by indexing the dataset object loaded through 'load_dataset':
A typical data point comprises the path to the audio file and its transcription. Also included is information of the dataset from which the sample derives and a unique identifier name:
### Data Fields
- 'dataset': name of the ESC dataset from which the sample is taken.
- 'audio': a dictionary containing the path to the downloaded audio file, the decoded audio array, and the sampling rate.
- 'text': the transcription of the audio file.
- 'id': unique id of the data sample.
### Data Preparation
#### Audio
The audio for all ESC datasets is segmented into sample lengths suitable for training ASR systems. The Hugging Face datasets library decodes audio files on the fly, reading the segments and converting them to a Python arrays. Consequently, no further preparation of the audio is required to be used in training/evaluation scripts.
Note that when accessing the audio column: 'dataset[0]["audio"]' the audio file is automatically decoded and resampled to 'dataset.features["audio"].sampling_rate'. Decoding and resampling of a large number of audio files might take a significant amount of time. Thus it is important to first query the sample index before the '"audio"' column, i.e. 'dataset[0]["audio"]' should always be preferred over 'dataset["audio"][0]'.
#### Transcriptions
The transcriptions corresponding to each audio file are provided in their 'error corrected' format. No transcription pre-processing is applied to the text, only necessary 'error correction' steps such as removing junk tokens (_<unk>_) or converting symbolic punctuation to spelled out form (_<comma>_ to _,_). As such, no further preparation of the transcriptions is required to be used in training/evaluation scripts.
Transcriptions are provided for training and validation splits. The transcriptions are not provided for the test splits. The ESC benchmark requires you to generate predictions for the test sets and upload them to URL for scoring.
### Access
All eight of the datasets in ESC are accessible and licensing is freely available. Three of the ESC datasets have specific terms of usage that must be agreed to before using the data. To do so, fill in the access forms on the specific datasets' pages:
* Common Voice: URL
* GigaSpeech: URL
* SPGISpeech: URL
## LibriSpeech
The LibriSpeech corpus is a standard large-scale corpus for assessing ASR systems. It consists of approximately 1,000 hours of narrated audiobooks from the LibriVox project. It is licensed under CC-BY-4.0.
Example Usage:
Train/validation splits:
- 'train' (combination of 'URL.100', 'URL.360' and 'URL.500')
- 'URL'
- 'URL'
Test splits:
- 'URL'
- 'URL'
Also available are subsets of the train split, which can be accessed by setting the 'subconfig' argument:
- 'clean.100': 100 hours of training data from the 'clean' subset
- 'clean.360': 360 hours of training data from the 'clean' subset
- 'other.500': 500 hours of training data from the 'other' subset
## Common Voice
Common Voice is a series of crowd-sourced open-licensed speech datasets where speakers record text from Wikipedia in various languages. The English subset of contains approximately 1,400 hours of audio data from speakers of various nationalities, accents and different recording conditions. It is licensed under CC0-1.0.
Example usage:
Training/validation splits:
- 'train'
- 'validation'
Test splits:
- 'test'
## VoxPopuli
VoxPopuli s a large-scale multilingual speech corpus consisting of political data sourced from 2009-2020 European Parliament event recordings. The English subset contains approximately 550 hours of speech largely from non-native English speakers. It is licensed under CC0.
Example usage:
Training/validation splits:
- 'train'
- 'validation'
Test splits:
- 'test'
## TED-LIUM
TED-LIUM consists of English-language TED Talk conference videos covering a range of different cultural, political, and academic topics. It contains approximately 450 hours of transcribed speech data. It is licensed under CC-BY-NC-ND 3.0.
Example usage:
Training/validation splits:
- 'train'
- 'validation'
Test splits:
- 'test'
## GigaSpeech
GigaSpeech is a multi-domain English speech recognition corpus created from audiobooks, podcasts and YouTube. We provide the large train set (2,500 hours) and the standard validation and test splits. It is licensed under apache-2.0.
Example usage:
Training/validation splits:
- 'train' ('l' subset of training data (2,500 h))
- 'validation'
Test splits:
- 'test'
Also available are subsets of the train split, which can be accessed by setting the 'subconfig' argument:
- 'xs': extra-small subset of training data (10 h)
- 's': small subset of training data (250 h)
- 'm': medium subset of training data (1,000 h)
- 'xl': extra-large subset of training data (10,000 h)
## SPGISpeech
SPGISpeech consists of company earnings calls that have been manually transcribed by S&P Global, Inc according to a professional style guide. We provide the large train set (5,000 hours) and the standard validation and test splits. It is licensed under a Kensho user agreement.
Loading the dataset requires authorization.
Example usage:
Training/validation splits:
- 'train' ('l' subset of training data (~5,000 h))
- 'validation'
Test splits:
- 'test'
Also available are subsets of the train split, which can be accessed by setting the 'subconfig' argument:
- 's': small subset of training data (~200 h)
- 'm': medium subset of training data (~1,000 h)
## Earnings-22
Earnings-22 is a 119-hour corpus of English-language earnings calls collected from global companies, with speakers of many different nationalities and accents. It is licensed under CC-BY-SA-4.0.
Example usage:
Training/validation splits:
- 'train'
- 'validation'
Test splits:
- 'test'
## AMI
The AMI Meeting Corpus consists of 100 hours of meeting recordings from multiple recording devices synced to a common timeline. It is licensed under CC-BY-4.0.
Example usage:
Training/validation splits:
- 'train'
- 'validation'
Test splits:
- 'test'
| [
"## Dataset Information\n\nA data point can be accessed by indexing the dataset object loaded through 'load_dataset':\n\n\n\nA typical data point comprises the path to the audio file and its transcription. Also included is information of the dataset from which the sample derives and a unique identifier name:",
"### Data Fields\n\n- 'dataset': name of the ESC dataset from which the sample is taken.\n\n- 'audio': a dictionary containing the path to the downloaded audio file, the decoded audio array, and the sampling rate.\n\n- 'text': the transcription of the audio file.\n\n- 'id': unique id of the data sample.",
"### Data Preparation",
"#### Audio\nThe audio for all ESC datasets is segmented into sample lengths suitable for training ASR systems. The Hugging Face datasets library decodes audio files on the fly, reading the segments and converting them to a Python arrays. Consequently, no further preparation of the audio is required to be used in training/evaluation scripts.\n\nNote that when accessing the audio column: 'dataset[0][\"audio\"]' the audio file is automatically decoded and resampled to 'dataset.features[\"audio\"].sampling_rate'. Decoding and resampling of a large number of audio files might take a significant amount of time. Thus it is important to first query the sample index before the '\"audio\"' column, i.e. 'dataset[0][\"audio\"]' should always be preferred over 'dataset[\"audio\"][0]'.",
"#### Transcriptions\nThe transcriptions corresponding to each audio file are provided in their 'error corrected' format. No transcription pre-processing is applied to the text, only necessary 'error correction' steps such as removing junk tokens (_<unk>_) or converting symbolic punctuation to spelled out form (_<comma>_ to _,_). As such, no further preparation of the transcriptions is required to be used in training/evaluation scripts.\n\nTranscriptions are provided for training and validation splits. The transcriptions are not provided for the test splits. The ESC benchmark requires you to generate predictions for the test sets and upload them to URL for scoring.",
"### Access\nAll eight of the datasets in ESC are accessible and licensing is freely available. Three of the ESC datasets have specific terms of usage that must be agreed to before using the data. To do so, fill in the access forms on the specific datasets' pages:\n* Common Voice: URL\n* GigaSpeech: URL\n* SPGISpeech: URL",
"## LibriSpeech\n\nThe LibriSpeech corpus is a standard large-scale corpus for assessing ASR systems. It consists of approximately 1,000 hours of narrated audiobooks from the LibriVox project. It is licensed under CC-BY-4.0.\n\nExample Usage:\n\n\n\nTrain/validation splits:\n- 'train' (combination of 'URL.100', 'URL.360' and 'URL.500')\n- 'URL'\n- 'URL'\n\nTest splits:\n- 'URL'\n- 'URL'\n\nAlso available are subsets of the train split, which can be accessed by setting the 'subconfig' argument:\n\n\n- 'clean.100': 100 hours of training data from the 'clean' subset\n- 'clean.360': 360 hours of training data from the 'clean' subset\n- 'other.500': 500 hours of training data from the 'other' subset",
"## Common Voice\nCommon Voice is a series of crowd-sourced open-licensed speech datasets where speakers record text from Wikipedia in various languages. The English subset of contains approximately 1,400 hours of audio data from speakers of various nationalities, accents and different recording conditions. It is licensed under CC0-1.0.\n\nExample usage:\n\n\n\nTraining/validation splits:\n- 'train'\n- 'validation'\n\nTest splits:\n- 'test'",
"## VoxPopuli\nVoxPopuli s a large-scale multilingual speech corpus consisting of political data sourced from 2009-2020 European Parliament event recordings. The English subset contains approximately 550 hours of speech largely from non-native English speakers. It is licensed under CC0.\n\nExample usage:\n\n\n\nTraining/validation splits:\n- 'train'\n- 'validation'\n\nTest splits:\n- 'test'",
"## TED-LIUM\nTED-LIUM consists of English-language TED Talk conference videos covering a range of different cultural, political, and academic topics. It contains approximately 450 hours of transcribed speech data. It is licensed under CC-BY-NC-ND 3.0.\n\nExample usage:\n\n\n\nTraining/validation splits:\n- 'train'\n- 'validation'\n\nTest splits:\n- 'test'",
"## GigaSpeech\nGigaSpeech is a multi-domain English speech recognition corpus created from audiobooks, podcasts and YouTube. We provide the large train set (2,500 hours) and the standard validation and test splits. It is licensed under apache-2.0.\n\nExample usage:\n\n\n\nTraining/validation splits:\n- 'train' ('l' subset of training data (2,500 h))\n- 'validation'\n\nTest splits:\n- 'test'\n\nAlso available are subsets of the train split, which can be accessed by setting the 'subconfig' argument:\n\n- 'xs': extra-small subset of training data (10 h)\n- 's': small subset of training data (250 h)\n- 'm': medium subset of training data (1,000 h)\n- 'xl': extra-large subset of training data (10,000 h)",
"## SPGISpeech\nSPGISpeech consists of company earnings calls that have been manually transcribed by S&P Global, Inc according to a professional style guide. We provide the large train set (5,000 hours) and the standard validation and test splits. It is licensed under a Kensho user agreement.\n\nLoading the dataset requires authorization.\n\nExample usage:\n\n\n\nTraining/validation splits:\n- 'train' ('l' subset of training data (~5,000 h))\n- 'validation'\n\nTest splits:\n- 'test'\n\nAlso available are subsets of the train split, which can be accessed by setting the 'subconfig' argument:\n\n- 's': small subset of training data (~200 h)\n- 'm': medium subset of training data (~1,000 h)",
"## Earnings-22\nEarnings-22 is a 119-hour corpus of English-language earnings calls collected from global companies, with speakers of many different nationalities and accents. It is licensed under CC-BY-SA-4.0. \n\nExample usage:\n\n\n\nTraining/validation splits:\n- 'train'\n- 'validation'\n\nTest splits:\n- 'test'",
"## AMI\nThe AMI Meeting Corpus consists of 100 hours of meeting recordings from multiple recording devices synced to a common timeline. It is licensed under CC-BY-4.0.\n\nExample usage:\n\n\n\nTraining/validation splits:\n- 'train'\n- 'validation'\n\nTest splits:\n- 'test'"
] | [
"TAGS\n#task_categories-automatic-speech-recognition #annotations_creators-expert-generated #annotations_creators-crowdsourced #annotations_creators-machine-generated #language_creators-crowdsourced #language_creators-expert-generated #multilinguality-monolingual #size_categories-100K<n<1M #size_categories-1M<n<10M #source_datasets-original #source_datasets-extended|librispeech_asr #source_datasets-extended|common_voice #language-English #license-cc-by-4.0 #license-apache-2.0 #license-cc0-1.0 #license-cc-by-nc-3.0 #license-other #asr #benchmark #speech #esc #region-us \n",
"## Dataset Information\n\nA data point can be accessed by indexing the dataset object loaded through 'load_dataset':\n\n\n\nA typical data point comprises the path to the audio file and its transcription. Also included is information of the dataset from which the sample derives and a unique identifier name:",
"### Data Fields\n\n- 'dataset': name of the ESC dataset from which the sample is taken.\n\n- 'audio': a dictionary containing the path to the downloaded audio file, the decoded audio array, and the sampling rate.\n\n- 'text': the transcription of the audio file.\n\n- 'id': unique id of the data sample.",
"### Data Preparation",
"#### Audio\nThe audio for all ESC datasets is segmented into sample lengths suitable for training ASR systems. The Hugging Face datasets library decodes audio files on the fly, reading the segments and converting them to a Python arrays. Consequently, no further preparation of the audio is required to be used in training/evaluation scripts.\n\nNote that when accessing the audio column: 'dataset[0][\"audio\"]' the audio file is automatically decoded and resampled to 'dataset.features[\"audio\"].sampling_rate'. Decoding and resampling of a large number of audio files might take a significant amount of time. Thus it is important to first query the sample index before the '\"audio\"' column, i.e. 'dataset[0][\"audio\"]' should always be preferred over 'dataset[\"audio\"][0]'.",
"#### Transcriptions\nThe transcriptions corresponding to each audio file are provided in their 'error corrected' format. No transcription pre-processing is applied to the text, only necessary 'error correction' steps such as removing junk tokens (_<unk>_) or converting symbolic punctuation to spelled out form (_<comma>_ to _,_). As such, no further preparation of the transcriptions is required to be used in training/evaluation scripts.\n\nTranscriptions are provided for training and validation splits. The transcriptions are not provided for the test splits. The ESC benchmark requires you to generate predictions for the test sets and upload them to URL for scoring.",
"### Access\nAll eight of the datasets in ESC are accessible and licensing is freely available. Three of the ESC datasets have specific terms of usage that must be agreed to before using the data. To do so, fill in the access forms on the specific datasets' pages:\n* Common Voice: URL\n* GigaSpeech: URL\n* SPGISpeech: URL",
"## LibriSpeech\n\nThe LibriSpeech corpus is a standard large-scale corpus for assessing ASR systems. It consists of approximately 1,000 hours of narrated audiobooks from the LibriVox project. It is licensed under CC-BY-4.0.\n\nExample Usage:\n\n\n\nTrain/validation splits:\n- 'train' (combination of 'URL.100', 'URL.360' and 'URL.500')\n- 'URL'\n- 'URL'\n\nTest splits:\n- 'URL'\n- 'URL'\n\nAlso available are subsets of the train split, which can be accessed by setting the 'subconfig' argument:\n\n\n- 'clean.100': 100 hours of training data from the 'clean' subset\n- 'clean.360': 360 hours of training data from the 'clean' subset\n- 'other.500': 500 hours of training data from the 'other' subset",
"## Common Voice\nCommon Voice is a series of crowd-sourced open-licensed speech datasets where speakers record text from Wikipedia in various languages. The English subset of contains approximately 1,400 hours of audio data from speakers of various nationalities, accents and different recording conditions. It is licensed under CC0-1.0.\n\nExample usage:\n\n\n\nTraining/validation splits:\n- 'train'\n- 'validation'\n\nTest splits:\n- 'test'",
"## VoxPopuli\nVoxPopuli s a large-scale multilingual speech corpus consisting of political data sourced from 2009-2020 European Parliament event recordings. The English subset contains approximately 550 hours of speech largely from non-native English speakers. It is licensed under CC0.\n\nExample usage:\n\n\n\nTraining/validation splits:\n- 'train'\n- 'validation'\n\nTest splits:\n- 'test'",
"## TED-LIUM\nTED-LIUM consists of English-language TED Talk conference videos covering a range of different cultural, political, and academic topics. It contains approximately 450 hours of transcribed speech data. It is licensed under CC-BY-NC-ND 3.0.\n\nExample usage:\n\n\n\nTraining/validation splits:\n- 'train'\n- 'validation'\n\nTest splits:\n- 'test'",
"## GigaSpeech\nGigaSpeech is a multi-domain English speech recognition corpus created from audiobooks, podcasts and YouTube. We provide the large train set (2,500 hours) and the standard validation and test splits. It is licensed under apache-2.0.\n\nExample usage:\n\n\n\nTraining/validation splits:\n- 'train' ('l' subset of training data (2,500 h))\n- 'validation'\n\nTest splits:\n- 'test'\n\nAlso available are subsets of the train split, which can be accessed by setting the 'subconfig' argument:\n\n- 'xs': extra-small subset of training data (10 h)\n- 's': small subset of training data (250 h)\n- 'm': medium subset of training data (1,000 h)\n- 'xl': extra-large subset of training data (10,000 h)",
"## SPGISpeech\nSPGISpeech consists of company earnings calls that have been manually transcribed by S&P Global, Inc according to a professional style guide. We provide the large train set (5,000 hours) and the standard validation and test splits. It is licensed under a Kensho user agreement.\n\nLoading the dataset requires authorization.\n\nExample usage:\n\n\n\nTraining/validation splits:\n- 'train' ('l' subset of training data (~5,000 h))\n- 'validation'\n\nTest splits:\n- 'test'\n\nAlso available are subsets of the train split, which can be accessed by setting the 'subconfig' argument:\n\n- 's': small subset of training data (~200 h)\n- 'm': medium subset of training data (~1,000 h)",
"## Earnings-22\nEarnings-22 is a 119-hour corpus of English-language earnings calls collected from global companies, with speakers of many different nationalities and accents. It is licensed under CC-BY-SA-4.0. \n\nExample usage:\n\n\n\nTraining/validation splits:\n- 'train'\n- 'validation'\n\nTest splits:\n- 'test'",
"## AMI\nThe AMI Meeting Corpus consists of 100 hours of meeting recordings from multiple recording devices synced to a common timeline. It is licensed under CC-BY-4.0.\n\nExample usage:\n\n\n\nTraining/validation splits:\n- 'train'\n- 'validation'\n\nTest splits:\n- 'test'"
] | [
213,
67,
85,
5,
219,
164,
84,
203,
105,
97,
95,
199,
181,
86,
71
] | [
"passage: TAGS\n#task_categories-automatic-speech-recognition #annotations_creators-expert-generated #annotations_creators-crowdsourced #annotations_creators-machine-generated #language_creators-crowdsourced #language_creators-expert-generated #multilinguality-monolingual #size_categories-100K<n<1M #size_categories-1M<n<10M #source_datasets-original #source_datasets-extended|librispeech_asr #source_datasets-extended|common_voice #language-English #license-cc-by-4.0 #license-apache-2.0 #license-cc0-1.0 #license-cc-by-nc-3.0 #license-other #asr #benchmark #speech #esc #region-us \n## Dataset Information\n\nA data point can be accessed by indexing the dataset object loaded through 'load_dataset':\n\n\n\nA typical data point comprises the path to the audio file and its transcription. Also included is information of the dataset from which the sample derives and a unique identifier name:### Data Fields\n\n- 'dataset': name of the ESC dataset from which the sample is taken.\n\n- 'audio': a dictionary containing the path to the downloaded audio file, the decoded audio array, and the sampling rate.\n\n- 'text': the transcription of the audio file.\n\n- 'id': unique id of the data sample.### Data Preparation",
"passage: #### Audio\nThe audio for all ESC datasets is segmented into sample lengths suitable for training ASR systems. The Hugging Face datasets library decodes audio files on the fly, reading the segments and converting them to a Python arrays. Consequently, no further preparation of the audio is required to be used in training/evaluation scripts.\n\nNote that when accessing the audio column: 'dataset[0][\"audio\"]' the audio file is automatically decoded and resampled to 'dataset.features[\"audio\"].sampling_rate'. Decoding and resampling of a large number of audio files might take a significant amount of time. Thus it is important to first query the sample index before the '\"audio\"' column, i.e. 'dataset[0][\"audio\"]' should always be preferred over 'dataset[\"audio\"][0]'.#### Transcriptions\nThe transcriptions corresponding to each audio file are provided in their 'error corrected' format. No transcription pre-processing is applied to the text, only necessary 'error correction' steps such as removing junk tokens (_<unk>_) or converting symbolic punctuation to spelled out form (_<comma>_ to _,_). As such, no further preparation of the transcriptions is required to be used in training/evaluation scripts.\n\nTranscriptions are provided for training and validation splits. The transcriptions are not provided for the test splits. The ESC benchmark requires you to generate predictions for the test sets and upload them to URL for scoring.### Access\nAll eight of the datasets in ESC are accessible and licensing is freely available. Three of the ESC datasets have specific terms of usage that must be agreed to before using the data. To do so, fill in the access forms on the specific datasets' pages:\n* Common Voice: URL\n* GigaSpeech: URL\n* SPGISpeech: URL## LibriSpeech\n\nThe LibriSpeech corpus is a standard large-scale corpus for assessing ASR systems. It consists of approximately 1,000 hours of narrated audiobooks from the LibriVox project. It is licensed under CC-BY-4.0.\n\nExample Usage:\n\n\n\nTrain/validation splits:\n- 'train' (combination of 'URL.100', 'URL.360' and 'URL.500')\n- 'URL'\n- 'URL'\n\nTest splits:\n- 'URL'\n- 'URL'\n\nAlso available are subsets of the train split, which can be accessed by setting the 'subconfig' argument:\n\n\n- 'clean.100': 100 hours of training data from the 'clean' subset\n- 'clean.360': 360 hours of training data from the 'clean' subset\n- 'other.500': 500 hours of training data from the 'other' subset",
"passage: ## Common Voice\nCommon Voice is a series of crowd-sourced open-licensed speech datasets where speakers record text from Wikipedia in various languages. The English subset of contains approximately 1,400 hours of audio data from speakers of various nationalities, accents and different recording conditions. It is licensed under CC0-1.0.\n\nExample usage:\n\n\n\nTraining/validation splits:\n- 'train'\n- 'validation'\n\nTest splits:\n- 'test'## VoxPopuli\nVoxPopuli s a large-scale multilingual speech corpus consisting of political data sourced from 2009-2020 European Parliament event recordings. The English subset contains approximately 550 hours of speech largely from non-native English speakers. It is licensed under CC0.\n\nExample usage:\n\n\n\nTraining/validation splits:\n- 'train'\n- 'validation'\n\nTest splits:\n- 'test'## TED-LIUM\nTED-LIUM consists of English-language TED Talk conference videos covering a range of different cultural, political, and academic topics. It contains approximately 450 hours of transcribed speech data. It is licensed under CC-BY-NC-ND 3.0.\n\nExample usage:\n\n\n\nTraining/validation splits:\n- 'train'\n- 'validation'\n\nTest splits:\n- 'test'## GigaSpeech\nGigaSpeech is a multi-domain English speech recognition corpus created from audiobooks, podcasts and YouTube. We provide the large train set (2,500 hours) and the standard validation and test splits. It is licensed under apache-2.0.\n\nExample usage:\n\n\n\nTraining/validation splits:\n- 'train' ('l' subset of training data (2,500 h))\n- 'validation'\n\nTest splits:\n- 'test'\n\nAlso available are subsets of the train split, which can be accessed by setting the 'subconfig' argument:\n\n- 'xs': extra-small subset of training data (10 h)\n- 's': small subset of training data (250 h)\n- 'm': medium subset of training data (1,000 h)\n- 'xl': extra-large subset of training data (10,000 h)"
] |
70ae446852c18cf146a29082a2acf66e74609cd8 | # dureader
ๆฐๆฎๆฅ่ชDuReader-Retrevalๆฐๆฎ้๏ผ่ฟ้ๆฏ[ๅๅงๅฐๅ](https://github.com/baidu/DuReader/tree/master/DuReader-Retrieval)ใ
> ๆฌๆฐๆฎ้ๅช็จไฝๅญฆๆฏ็ ็ฉถไฝฟ็จใๅฆๆๆฌไปๅบๆถๅไพตๆ่กไธบ๏ผไผ็ซๅณๅ ้คใ | zyznull/dureader-retrieval-ranking | [
"license:apache-2.0",
"region:us"
] | 2022-09-28T08:00:20+00:00 | {"license": "apache-2.0"} | 2023-01-03T08:05:57+00:00 | [] | [] | TAGS
#license-apache-2.0 #region-us
| # dureader
ๆฐๆฎๆฅ่ชDuReader-Retrevalๆฐๆฎ้๏ผ่ฟ้ๆฏๅๅงๅฐๅใ
> ๆฌๆฐๆฎ้ๅช็จไฝๅญฆๆฏ็ ็ฉถไฝฟ็จใๅฆๆๆฌไปๅบๆถๅไพตๆ่กไธบ๏ผไผ็ซๅณๅ ้คใ | [
"# dureader\n\nๆฐๆฎๆฅ่ชDuReader-Retrevalๆฐๆฎ้๏ผ่ฟ้ๆฏๅๅงๅฐๅใ\n\n> ๆฌๆฐๆฎ้ๅช็จไฝๅญฆๆฏ็ ็ฉถไฝฟ็จใๅฆๆๆฌไปๅบๆถๅไพตๆ่กไธบ๏ผไผ็ซๅณๅ ้คใ"
] | [
"TAGS\n#license-apache-2.0 #region-us \n",
"# dureader\n\nๆฐๆฎๆฅ่ชDuReader-Retrevalๆฐๆฎ้๏ผ่ฟ้ๆฏๅๅงๅฐๅใ\n\n> ๆฌๆฐๆฎ้ๅช็จไฝๅญฆๆฏ็ ็ฉถไฝฟ็จใๅฆๆๆฌไปๅบๆถๅไพตๆ่กไธบ๏ผไผ็ซๅณๅ ้คใ"
] | [
14,
43
] | [
"passage: TAGS\n#license-apache-2.0 #region-us \n# dureader\n\nๆฐๆฎๆฅ่ชDuReader-Retrevalๆฐๆฎ้๏ผ่ฟ้ๆฏๅๅงๅฐๅใ\n\n> ๆฌๆฐๆฎ้ๅช็จไฝๅญฆๆฏ็ ็ฉถไฝฟ็จใๅฆๆๆฌไปๅบๆถๅไพตๆ่กไธบ๏ผไผ็ซๅณๅ ้คใ"
] |
0d792180b9349c544a2ea220de6b72f78611fb17 | # Dataset Card for AutoTrain Evaluator
This repository contains model predictions generated by [AutoTrain](https://huggingface.co/autotrain) for the following task and dataset:
* Task: Summarization
* Model: facebook/bart-large-cnn
* Dataset: big_patent
* Config: g
* Split: validation
To run new evaluation jobs, visit Hugging Face's [automatic model evaluator](https://huggingface.co/spaces/autoevaluate/model-evaluator).
## Contributions
Thanks to [@jonesdaniel](https://huggingface.co/jonesdaniel) for evaluating this model. | autoevaluate/autoeval-eval-big_patent-g-9d42aa-1581555947 | [
"autotrain",
"evaluation",
"region:us"
] | 2022-09-28T08:54:38+00:00 | {"type": "predictions", "tags": ["autotrain", "evaluation"], "datasets": ["big_patent"], "eval_info": {"task": "summarization", "model": "facebook/bart-large-cnn", "metrics": ["perplexity"], "dataset_name": "big_patent", "dataset_config": "g", "dataset_split": "validation", "col_mapping": {"text": "description", "target": "abstract"}}} | 2022-09-28T10:15:24+00:00 | [] | [] | TAGS
#autotrain #evaluation #region-us
| # Dataset Card for AutoTrain Evaluator
This repository contains model predictions generated by AutoTrain for the following task and dataset:
* Task: Summarization
* Model: facebook/bart-large-cnn
* Dataset: big_patent
* Config: g
* Split: validation
To run new evaluation jobs, visit Hugging Face's automatic model evaluator.
## Contributions
Thanks to @jonesdaniel for evaluating this model. | [
"# Dataset Card for AutoTrain Evaluator\n\nThis repository contains model predictions generated by AutoTrain for the following task and dataset:\n\n* Task: Summarization\n* Model: facebook/bart-large-cnn\n* Dataset: big_patent\n* Config: g\n* Split: validation\n\nTo run new evaluation jobs, visit Hugging Face's automatic model evaluator.",
"## Contributions\n\nThanks to @jonesdaniel for evaluating this model."
] | [
"TAGS\n#autotrain #evaluation #region-us \n",
"# Dataset Card for AutoTrain Evaluator\n\nThis repository contains model predictions generated by AutoTrain for the following task and dataset:\n\n* Task: Summarization\n* Model: facebook/bart-large-cnn\n* Dataset: big_patent\n* Config: g\n* Split: validation\n\nTo run new evaluation jobs, visit Hugging Face's automatic model evaluator.",
"## Contributions\n\nThanks to @jonesdaniel for evaluating this model."
] | [
13,
87,
17
] | [
"passage: TAGS\n#autotrain #evaluation #region-us \n# Dataset Card for AutoTrain Evaluator\n\nThis repository contains model predictions generated by AutoTrain for the following task and dataset:\n\n* Task: Summarization\n* Model: facebook/bart-large-cnn\n* Dataset: big_patent\n* Config: g\n* Split: validation\n\nTo run new evaluation jobs, visit Hugging Face's automatic model evaluator.## Contributions\n\nThanks to @jonesdaniel for evaluating this model."
] |
c801dc186b40a532c5820b4662570390da90431b | # Dataset Card for "tacred"
## Table of Contents
- [Table of Contents](#table-of-contents)
- [Dataset Description](#dataset-description)
- [Dataset Summary](#dataset-summary)
- [Supported Tasks and Leaderboards](#supported-tasks-and-leaderboards)
- [Languages](#languages)
- [Dataset Structure](#dataset-structure)
- [Data Instances](#data-instances)
- [Data Fields](#data-fields)
- [Data Splits](#data-splits)
- [Dataset Creation](#dataset-creation)
- [Curation Rationale](#curation-rationale)
- [Source Data](#source-data)
- [Annotations](#annotations)
- [Personal and Sensitive Information](#personal-and-sensitive-information)
- [Considerations for Using the Data](#considerations-for-using-the-data)
- [Social Impact of Dataset](#social-impact-of-dataset)
- [Discussion of Biases](#discussion-of-biases)
- [Other Known Limitations](#other-known-limitations)
- [Additional Information](#additional-information)
- [Dataset Curators](#dataset-curators)
- [Licensing Information](#licensing-information)
- [Citation Information](#citation-information)
- [Contributions](#contributions)
## Dataset Description
- **Homepage:** [https://nlp.stanford.edu/projects/tacred](https://nlp.stanford.edu/projects/tacred)
- **Paper:** [Position-aware Attention and Supervised Data Improve Slot Filling](https://aclanthology.org/D17-1004/)
- **Point of Contact:** See [https://nlp.stanford.edu/projects/tacred/](https://nlp.stanford.edu/projects/tacred/)
- **Size of downloaded dataset files:** 62.3 MB
- **Size of the generated dataset:** 139.2 MB
- **Total amount of disk used:** 201.5 MB
### Dataset Summary
The TAC Relation Extraction Dataset (TACRED) is a large-scale relation extraction dataset with 106,264 examples built over newswire and web text from the corpus used in the yearly TAC Knowledge Base Population (TAC KBP) challenges. Examples in TACRED cover 41 relation types as used in the TAC KBP challenges (e.g., per:schools_attended
and org:members) or are labeled as no_relation if no defined relation is held. These examples are created by combining available human annotations from the TAC
KBP challenges and crowdsourcing. Please see [Stanford's EMNLP paper](https://nlp.stanford.edu/pubs/zhang2017tacred.pdf), or their [EMNLP slides](https://nlp.stanford.edu/projects/tacred/files/position-emnlp2017.pdf) for full details.
Note:
- There is currently a [label-corrected version](https://github.com/DFKI-NLP/tacrev) of the TACRED dataset, which you should consider using instead of
the original version released in 2017. For more details on this new version, see the [TACRED Revisited paper](https://aclanthology.org/2020.acl-main.142/)
published at ACL 2020.
- There is also a [relabeled and pruned version](https://github.com/gstoica27/Re-TACRED) of the TACRED dataset.
For more details on this new version, see the [Re-TACRED paper](https://arxiv.org/abs/2104.08398)
published at ACL 2020.
This repository provides all three versions of the dataset as BuilderConfigs - `'original'`, `'revisited'` and `'re-tacred'`.
Simply set the `name` parameter in the `load_dataset` method in order to choose a specific version. The original TACRED is loaded per default.
### Supported Tasks and Leaderboards
- **Tasks:** Relation Classification
- **Leaderboards:** [https://paperswithcode.com/sota/relation-extraction-on-tacred](https://paperswithcode.com/sota/relation-extraction-on-tacred)
### Languages
The language in the dataset is English.
## Dataset Structure
### Data Instances
- **Size of downloaded dataset files:** 62.3 MB
- **Size of the generated dataset:** 139.2 MB
- **Total amount of disk used:** 201.5 MB
An example of 'train' looks as follows:
```json
{
"id": "61b3a5c8c9a882dcfcd2",
"docid": "AFP_ENG_20070218.0019.LDC2009T13",
"relation": "org:founded_by",
"token": ["Tom", "Thabane", "resigned", "in", "October", "last", "year", "to", "form", "the", "All", "Basotho", "Convention", "-LRB-", "ABC", "-RRB-", ",", "crossing", "the", "floor", "with", "17", "members", "of", "parliament", ",", "causing", "constitutional", "monarch", "King", "Letsie", "III", "to", "dissolve", "parliament", "and", "call", "the", "snap", "election", "."],
"subj_start": 10,
"subj_end": 13,
"obj_start": 0,
"obj_end": 2,
"subj_type": "ORGANIZATION",
"obj_type": "PERSON",
"stanford_pos": ["NNP", "NNP", "VBD", "IN", "NNP", "JJ", "NN", "TO", "VB", "DT", "DT", "NNP", "NNP", "-LRB-", "NNP", "-RRB-", ",", "VBG", "DT", "NN", "IN", "CD", "NNS", "IN", "NN", ",", "VBG", "JJ", "NN", "NNP", "NNP", "NNP", "TO", "VB", "NN", "CC", "VB", "DT", "NN", "NN", "."],
"stanford_ner": ["PERSON", "PERSON", "O", "O", "DATE", "DATE", "DATE", "O", "O", "O", "O", "O", "O", "O", "ORGANIZATION", "O", "O", "O", "O", "O", "O", "NUMBER", "O", "O", "O", "O", "O", "O", "O", "O", "PERSON", "PERSON", "O", "O", "O", "O", "O", "O", "O", "O", "O"],
"stanford_head": [2, 3, 0, 5, 3, 7, 3, 9, 3, 13, 13, 13, 9, 15, 13, 15, 3, 3, 20, 18, 23, 23, 18, 25, 23, 3, 3, 32, 32, 32, 32, 27, 34, 27, 34, 34, 34, 40, 40, 37, 3],
"stanford_deprel": ["compound", "nsubj", "ROOT", "case", "nmod", "amod", "nmod:tmod", "mark", "xcomp", "det", "compound", "compound", "dobj", "punct", "appos", "punct", "punct", "xcomp", "det", "dobj", "case", "nummod", "nmod", "case", "nmod", "punct", "xcomp", "amod", "compound", "compound", "compound", "dobj", "mark", "xcomp", "dobj", "cc", "conj", "det", "compound", "dobj", "punct"]
}
```
### Data Fields
The data fields are the same among all splits.
- `id`: the instance id of this sentence, a `string` feature.
- `docid`: the TAC KBP document id of this sentence, a `string` feature.
- `token`: the list of tokens of this sentence, obtained with the StanfordNLP toolkit, a `list` of `string` features.
- `relation`: the relation label of this instance, a `string` classification label.
- `subj_start`: the 0-based index of the start token of the relation subject mention, an `รฌnt` feature.
- `subj_end`: the 0-based index of the end token of the relation subject mention, exclusive, an `รฌnt` feature.
- `subj_type`: the NER type of the subject mention, among 23 fine-grained types used in the [Stanford NER system](https://stanfordnlp.github.io/CoreNLP/ner.html), a `string` feature.
- `obj_start`: the 0-based index of the start token of the relation object mention, an `รฌnt` feature.
- `obj_end`: the 0-based index of the end token of the relation object mention, exclusive, an `รฌnt` feature.
- `obj_type`: the NER type of the object mention, among 23 fine-grained types used in the [Stanford NER system](https://stanfordnlp.github.io/CoreNLP/ner.html), a `string` feature.
- `stanford_pos`: the part-of-speech tag per token. the NER type of the subject mention, among 23 fine-grained types used in the [Stanford NER system](https://stanfordnlp.github.io/CoreNLP/ner.html), a `list` of `string` features.
- `stanford_ner`: the NER tags of tokens (IO-Scheme), among 23 fine-grained types used in the [Stanford NER system](https://stanfordnlp.github.io/CoreNLP/ner.html), a `list` of `string` features.
- `stanford_deprel`: the Stanford dependency relation tag per token, a `list` of `string` features.
- `stanford_head`: the head (source) token index (0-based) for the dependency relation per token. The root token has a head index of -1, a `list` of `int` features.
### Data Splits
To miminize dataset bias, TACRED is stratified across years in which the TAC KBP challenge was run:
| | Train | Dev | Test |
| ----- | ------ | ----- | ---- |
| TACRED | 68,124 (TAC KBP 2009-2012) | 22,631 (TAC KBP 2013) | 15,509 (TAC KBP 2014) |
| Re-TACRED | 58,465 (TAC KBP 2009-2012) | 19,584 (TAC KBP 2013) | 13,418 (TAC KBP 2014) |
## Dataset Creation
### Curation Rationale
[More Information Needed]
### Source Data
#### Initial Data Collection and Normalization
[More Information Needed]
#### Who are the source language producers?
[More Information Needed]
### Annotations
#### Annotation process
See the Stanford paper and the Tacred Revisited paper, plus their appendices.
To ensure that models trained on TACRED are not biased towards predicting false positives on real-world text,
all sampled sentences where no relation was found between the mention pairs were fully annotated to be negative examples. As a result, 79.5% of the examples
are labeled as no_relation.
#### Who are the annotators?
[More Information Needed]
### Personal and Sensitive Information
[More Information Needed]
## Considerations for Using the Data
### Social Impact of Dataset
[More Information Needed]
### Discussion of Biases
[More Information Needed]
### Other Known Limitations
[More Information Needed]
## Additional Information
### Dataset Curators
[More Information Needed]
### Licensing Information
To respect the copyright of the underlying TAC KBP corpus, TACRED is released via the
Linguistic Data Consortium ([LDC License](https://catalog.ldc.upenn.edu/license/ldc-non-members-agreement.pdf)).
You can download TACRED from the [LDC TACRED webpage](https://catalog.ldc.upenn.edu/LDC2018T24).
If you are an LDC member, the access will be free; otherwise, an access fee of $25 is needed.
### Citation Information
The original dataset:
```
@inproceedings{zhang2017tacred,
author = {Zhang, Yuhao and Zhong, Victor and Chen, Danqi and Angeli, Gabor and Manning, Christopher D.},
booktitle = {Proceedings of the 2017 Conference on Empirical Methods in Natural Language Processing (EMNLP 2017)},
title = {Position-aware Attention and Supervised Data Improve Slot Filling},
url = {https://nlp.stanford.edu/pubs/zhang2017tacred.pdf},
pages = {35--45},
year = {2017}
}
```
For the revised version (`"revisited"`), please also cite:
```
@inproceedings{alt-etal-2020-tacred,
title = "{TACRED} Revisited: A Thorough Evaluation of the {TACRED} Relation Extraction Task",
author = "Alt, Christoph and
Gabryszak, Aleksandra and
Hennig, Leonhard",
booktitle = "Proceedings of the 58th Annual Meeting of the Association for Computational Linguistics",
month = jul,
year = "2020",
address = "Online",
publisher = "Association for Computational Linguistics",
url = "https://www.aclweb.org/anthology/2020.acl-main.142",
doi = "10.18653/v1/2020.acl-main.142",
pages = "1558--1569",
}
```
For the relabeled version (`"re-tacred"`), please also cite:
```
@inproceedings{DBLP:conf/aaai/StoicaPP21,
author = {George Stoica and
Emmanouil Antonios Platanios and
Barnab{\'{a}}s P{\'{o}}czos},
title = {Re-TACRED: Addressing Shortcomings of the {TACRED} Dataset},
booktitle = {Thirty-Fifth {AAAI} Conference on Artificial Intelligence, {AAAI}
2021, Thirty-Third Conference on Innovative Applications of Artificial
Intelligence, {IAAI} 2021, The Eleventh Symposium on Educational Advances
in Artificial Intelligence, {EAAI} 2021, Virtual Event, February 2-9,
2021},
pages = {13843--13850},
publisher = {{AAAI} Press},
year = {2021},
url = {https://ojs.aaai.org/index.php/AAAI/article/view/17631},
}
```
### Contributions
Thanks to [@dfki-nlp](https://github.com/dfki-nlp) and [@phucdev](https://github.com/phucdev) for adding this dataset.
| DFKI-SLT/tacred | [
"task_categories:text-classification",
"task_ids:multi-class-classification",
"annotations_creators:crowdsourced",
"annotations_creators:expert-generated",
"language_creators:found",
"multilinguality:monolingual",
"size_categories:100K<n<1M",
"source_datasets:extended|other",
"language:en",
"license:other",
"relation extraction",
"arxiv:2104.08398",
"region:us"
] | 2022-09-28T09:02:34+00:00 | {"annotations_creators": ["crowdsourced", "expert-generated"], "language_creators": ["found"], "language": ["en"], "license": ["other"], "multilinguality": ["monolingual"], "size_categories": ["100K<n<1M"], "source_datasets": ["extended|other"], "task_categories": ["text-classification"], "task_ids": ["multi-class-classification"], "pretty_name": "The TAC Relation Extraction Dataset, TACRED Revisited and Re-TACRED", "tags": ["relation extraction"]} | 2023-05-17T11:55:00+00:00 | [
"2104.08398"
] | [
"en"
] | TAGS
#task_categories-text-classification #task_ids-multi-class-classification #annotations_creators-crowdsourced #annotations_creators-expert-generated #language_creators-found #multilinguality-monolingual #size_categories-100K<n<1M #source_datasets-extended|other #language-English #license-other #relation extraction #arxiv-2104.08398 #region-us
| Dataset Card for "tacred"
=========================
Table of Contents
-----------------
* Table of Contents
* Dataset Description
+ Dataset Summary
+ Supported Tasks and Leaderboards
+ Languages
* Dataset Structure
+ Data Instances
+ Data Fields
+ Data Splits
* Dataset Creation
+ Curation Rationale
+ Source Data
+ Annotations
+ Personal and Sensitive Information
* Considerations for Using the Data
+ Social Impact of Dataset
+ Discussion of Biases
+ Other Known Limitations
* Additional Information
+ Dataset Curators
+ Licensing Information
+ Citation Information
+ Contributions
Dataset Description
-------------------
* Homepage: URL
* Paper: Position-aware Attention and Supervised Data Improve Slot Filling
* Point of Contact: See URL
* Size of downloaded dataset files: 62.3 MB
* Size of the generated dataset: 139.2 MB
* Total amount of disk used: 201.5 MB
### Dataset Summary
The TAC Relation Extraction Dataset (TACRED) is a large-scale relation extraction dataset with 106,264 examples built over newswire and web text from the corpus used in the yearly TAC Knowledge Base Population (TAC KBP) challenges. Examples in TACRED cover 41 relation types as used in the TAC KBP challenges (e.g., per:schools\_attended
and org:members) or are labeled as no\_relation if no defined relation is held. These examples are created by combining available human annotations from the TAC
KBP challenges and crowdsourcing. Please see Stanford's EMNLP paper, or their EMNLP slides for full details.
Note:
* There is currently a label-corrected version of the TACRED dataset, which you should consider using instead of
the original version released in 2017. For more details on this new version, see the TACRED Revisited paper
published at ACL 2020.
* There is also a relabeled and pruned version of the TACRED dataset.
For more details on this new version, see the Re-TACRED paper
published at ACL 2020.
This repository provides all three versions of the dataset as BuilderConfigs - ''original'', ''revisited'' and ''re-tacred''.
Simply set the 'name' parameter in the 'load\_dataset' method in order to choose a specific version. The original TACRED is loaded per default.
### Supported Tasks and Leaderboards
* Tasks: Relation Classification
* Leaderboards: URL
### Languages
The language in the dataset is English.
Dataset Structure
-----------------
### Data Instances
* Size of downloaded dataset files: 62.3 MB
* Size of the generated dataset: 139.2 MB
* Total amount of disk used: 201.5 MB
An example of 'train' looks as follows:
### Data Fields
The data fields are the same among all splits.
* 'id': the instance id of this sentence, a 'string' feature.
* 'docid': the TAC KBP document id of this sentence, a 'string' feature.
* 'token': the list of tokens of this sentence, obtained with the StanfordNLP toolkit, a 'list' of 'string' features.
* 'relation': the relation label of this instance, a 'string' classification label.
* 'subj\_start': the 0-based index of the start token of the relation subject mention, an 'รฌnt' feature.
* 'subj\_end': the 0-based index of the end token of the relation subject mention, exclusive, an 'รฌnt' feature.
* 'subj\_type': the NER type of the subject mention, among 23 fine-grained types used in the Stanford NER system, a 'string' feature.
* 'obj\_start': the 0-based index of the start token of the relation object mention, an 'รฌnt' feature.
* 'obj\_end': the 0-based index of the end token of the relation object mention, exclusive, an 'รฌnt' feature.
* 'obj\_type': the NER type of the object mention, among 23 fine-grained types used in the Stanford NER system, a 'string' feature.
* 'stanford\_pos': the part-of-speech tag per token. the NER type of the subject mention, among 23 fine-grained types used in the Stanford NER system, a 'list' of 'string' features.
* 'stanford\_ner': the NER tags of tokens (IO-Scheme), among 23 fine-grained types used in the Stanford NER system, a 'list' of 'string' features.
* 'stanford\_deprel': the Stanford dependency relation tag per token, a 'list' of 'string' features.
* 'stanford\_head': the head (source) token index (0-based) for the dependency relation per token. The root token has a head index of -1, a 'list' of 'int' features.
### Data Splits
To miminize dataset bias, TACRED is stratified across years in which the TAC KBP challenge was run:
Dataset Creation
----------------
### Curation Rationale
### Source Data
#### Initial Data Collection and Normalization
#### Who are the source language producers?
### Annotations
#### Annotation process
See the Stanford paper and the Tacred Revisited paper, plus their appendices.
To ensure that models trained on TACRED are not biased towards predicting false positives on real-world text,
all sampled sentences where no relation was found between the mention pairs were fully annotated to be negative examples. As a result, 79.5% of the examples
are labeled as no\_relation.
#### Who are the annotators?
### Personal and Sensitive Information
Considerations for Using the Data
---------------------------------
### Social Impact of Dataset
### Discussion of Biases
### Other Known Limitations
Additional Information
----------------------
### Dataset Curators
### Licensing Information
To respect the copyright of the underlying TAC KBP corpus, TACRED is released via the
Linguistic Data Consortium (LDC License).
You can download TACRED from the LDC TACRED webpage.
If you are an LDC member, the access will be free; otherwise, an access fee of $25 is needed.
The original dataset:
For the revised version ('"revisited"'), please also cite:
For the relabeled version ('"re-tacred"'), please also cite:
### Contributions
Thanks to @dfki-nlp and @phucdev for adding this dataset.
| [
"### Dataset Summary\n\n\nThe TAC Relation Extraction Dataset (TACRED) is a large-scale relation extraction dataset with 106,264 examples built over newswire and web text from the corpus used in the yearly TAC Knowledge Base Population (TAC KBP) challenges. Examples in TACRED cover 41 relation types as used in the TAC KBP challenges (e.g., per:schools\\_attended\nand org:members) or are labeled as no\\_relation if no defined relation is held. These examples are created by combining available human annotations from the TAC\nKBP challenges and crowdsourcing. Please see Stanford's EMNLP paper, or their EMNLP slides for full details.\n\n\nNote:\n\n\n* There is currently a label-corrected version of the TACRED dataset, which you should consider using instead of\nthe original version released in 2017. For more details on this new version, see the TACRED Revisited paper\npublished at ACL 2020.\n* There is also a relabeled and pruned version of the TACRED dataset.\nFor more details on this new version, see the Re-TACRED paper\npublished at ACL 2020.\n\n\nThis repository provides all three versions of the dataset as BuilderConfigs - ''original'', ''revisited'' and ''re-tacred''.\nSimply set the 'name' parameter in the 'load\\_dataset' method in order to choose a specific version. The original TACRED is loaded per default.",
"### Supported Tasks and Leaderboards\n\n\n* Tasks: Relation Classification\n* Leaderboards: URL",
"### Languages\n\n\nThe language in the dataset is English.\n\n\nDataset Structure\n-----------------",
"### Data Instances\n\n\n* Size of downloaded dataset files: 62.3 MB\n* Size of the generated dataset: 139.2 MB\n* Total amount of disk used: 201.5 MB\n\n\nAn example of 'train' looks as follows:",
"### Data Fields\n\n\nThe data fields are the same among all splits.\n\n\n* 'id': the instance id of this sentence, a 'string' feature.\n* 'docid': the TAC KBP document id of this sentence, a 'string' feature.\n* 'token': the list of tokens of this sentence, obtained with the StanfordNLP toolkit, a 'list' of 'string' features.\n* 'relation': the relation label of this instance, a 'string' classification label.\n* 'subj\\_start': the 0-based index of the start token of the relation subject mention, an 'รฌnt' feature.\n* 'subj\\_end': the 0-based index of the end token of the relation subject mention, exclusive, an 'รฌnt' feature.\n* 'subj\\_type': the NER type of the subject mention, among 23 fine-grained types used in the Stanford NER system, a 'string' feature.\n* 'obj\\_start': the 0-based index of the start token of the relation object mention, an 'รฌnt' feature.\n* 'obj\\_end': the 0-based index of the end token of the relation object mention, exclusive, an 'รฌnt' feature.\n* 'obj\\_type': the NER type of the object mention, among 23 fine-grained types used in the Stanford NER system, a 'string' feature.\n* 'stanford\\_pos': the part-of-speech tag per token. the NER type of the subject mention, among 23 fine-grained types used in the Stanford NER system, a 'list' of 'string' features.\n* 'stanford\\_ner': the NER tags of tokens (IO-Scheme), among 23 fine-grained types used in the Stanford NER system, a 'list' of 'string' features.\n* 'stanford\\_deprel': the Stanford dependency relation tag per token, a 'list' of 'string' features.\n* 'stanford\\_head': the head (source) token index (0-based) for the dependency relation per token. The root token has a head index of -1, a 'list' of 'int' features.",
"### Data Splits\n\n\nTo miminize dataset bias, TACRED is stratified across years in which the TAC KBP challenge was run:\n\n\n\nDataset Creation\n----------------",
"### Curation Rationale",
"### Source Data",
"#### Initial Data Collection and Normalization",
"#### Who are the source language producers?",
"### Annotations",
"#### Annotation process\n\n\nSee the Stanford paper and the Tacred Revisited paper, plus their appendices.\n\n\nTo ensure that models trained on TACRED are not biased towards predicting false positives on real-world text,\nall sampled sentences where no relation was found between the mention pairs were fully annotated to be negative examples. As a result, 79.5% of the examples\nare labeled as no\\_relation.",
"#### Who are the annotators?",
"### Personal and Sensitive Information\n\n\nConsiderations for Using the Data\n---------------------------------",
"### Social Impact of Dataset",
"### Discussion of Biases",
"### Other Known Limitations\n\n\nAdditional Information\n----------------------",
"### Dataset Curators",
"### Licensing Information\n\n\nTo respect the copyright of the underlying TAC KBP corpus, TACRED is released via the\nLinguistic Data Consortium (LDC License).\nYou can download TACRED from the LDC TACRED webpage.\nIf you are an LDC member, the access will be free; otherwise, an access fee of $25 is needed.\n\n\nThe original dataset:\n\n\nFor the revised version ('\"revisited\"'), please also cite:\n\n\nFor the relabeled version ('\"re-tacred\"'), please also cite:",
"### Contributions\n\n\nThanks to @dfki-nlp and @phucdev for adding this dataset."
] | [
"TAGS\n#task_categories-text-classification #task_ids-multi-class-classification #annotations_creators-crowdsourced #annotations_creators-expert-generated #language_creators-found #multilinguality-monolingual #size_categories-100K<n<1M #source_datasets-extended|other #language-English #license-other #relation extraction #arxiv-2104.08398 #region-us \n",
"### Dataset Summary\n\n\nThe TAC Relation Extraction Dataset (TACRED) is a large-scale relation extraction dataset with 106,264 examples built over newswire and web text from the corpus used in the yearly TAC Knowledge Base Population (TAC KBP) challenges. Examples in TACRED cover 41 relation types as used in the TAC KBP challenges (e.g., per:schools\\_attended\nand org:members) or are labeled as no\\_relation if no defined relation is held. These examples are created by combining available human annotations from the TAC\nKBP challenges and crowdsourcing. Please see Stanford's EMNLP paper, or their EMNLP slides for full details.\n\n\nNote:\n\n\n* There is currently a label-corrected version of the TACRED dataset, which you should consider using instead of\nthe original version released in 2017. For more details on this new version, see the TACRED Revisited paper\npublished at ACL 2020.\n* There is also a relabeled and pruned version of the TACRED dataset.\nFor more details on this new version, see the Re-TACRED paper\npublished at ACL 2020.\n\n\nThis repository provides all three versions of the dataset as BuilderConfigs - ''original'', ''revisited'' and ''re-tacred''.\nSimply set the 'name' parameter in the 'load\\_dataset' method in order to choose a specific version. The original TACRED is loaded per default.",
"### Supported Tasks and Leaderboards\n\n\n* Tasks: Relation Classification\n* Leaderboards: URL",
"### Languages\n\n\nThe language in the dataset is English.\n\n\nDataset Structure\n-----------------",
"### Data Instances\n\n\n* Size of downloaded dataset files: 62.3 MB\n* Size of the generated dataset: 139.2 MB\n* Total amount of disk used: 201.5 MB\n\n\nAn example of 'train' looks as follows:",
"### Data Fields\n\n\nThe data fields are the same among all splits.\n\n\n* 'id': the instance id of this sentence, a 'string' feature.\n* 'docid': the TAC KBP document id of this sentence, a 'string' feature.\n* 'token': the list of tokens of this sentence, obtained with the StanfordNLP toolkit, a 'list' of 'string' features.\n* 'relation': the relation label of this instance, a 'string' classification label.\n* 'subj\\_start': the 0-based index of the start token of the relation subject mention, an 'รฌnt' feature.\n* 'subj\\_end': the 0-based index of the end token of the relation subject mention, exclusive, an 'รฌnt' feature.\n* 'subj\\_type': the NER type of the subject mention, among 23 fine-grained types used in the Stanford NER system, a 'string' feature.\n* 'obj\\_start': the 0-based index of the start token of the relation object mention, an 'รฌnt' feature.\n* 'obj\\_end': the 0-based index of the end token of the relation object mention, exclusive, an 'รฌnt' feature.\n* 'obj\\_type': the NER type of the object mention, among 23 fine-grained types used in the Stanford NER system, a 'string' feature.\n* 'stanford\\_pos': the part-of-speech tag per token. the NER type of the subject mention, among 23 fine-grained types used in the Stanford NER system, a 'list' of 'string' features.\n* 'stanford\\_ner': the NER tags of tokens (IO-Scheme), among 23 fine-grained types used in the Stanford NER system, a 'list' of 'string' features.\n* 'stanford\\_deprel': the Stanford dependency relation tag per token, a 'list' of 'string' features.\n* 'stanford\\_head': the head (source) token index (0-based) for the dependency relation per token. The root token has a head index of -1, a 'list' of 'int' features.",
"### Data Splits\n\n\nTo miminize dataset bias, TACRED is stratified across years in which the TAC KBP challenge was run:\n\n\n\nDataset Creation\n----------------",
"### Curation Rationale",
"### Source Data",
"#### Initial Data Collection and Normalization",
"#### Who are the source language producers?",
"### Annotations",
"#### Annotation process\n\n\nSee the Stanford paper and the Tacred Revisited paper, plus their appendices.\n\n\nTo ensure that models trained on TACRED are not biased towards predicting false positives on real-world text,\nall sampled sentences where no relation was found between the mention pairs were fully annotated to be negative examples. As a result, 79.5% of the examples\nare labeled as no\\_relation.",
"#### Who are the annotators?",
"### Personal and Sensitive Information\n\n\nConsiderations for Using the Data\n---------------------------------",
"### Social Impact of Dataset",
"### Discussion of Biases",
"### Other Known Limitations\n\n\nAdditional Information\n----------------------",
"### Dataset Curators",
"### Licensing Information\n\n\nTo respect the copyright of the underlying TAC KBP corpus, TACRED is released via the\nLinguistic Data Consortium (LDC License).\nYou can download TACRED from the LDC TACRED webpage.\nIf you are an LDC member, the access will be free; otherwise, an access fee of $25 is needed.\n\n\nThe original dataset:\n\n\nFor the revised version ('\"revisited\"'), please also cite:\n\n\nFor the relabeled version ('\"re-tacred\"'), please also cite:",
"### Contributions\n\n\nThanks to @dfki-nlp and @phucdev for adding this dataset."
] | [
117,
338,
23,
20,
52,
508,
38,
7,
4,
10,
10,
5,
97,
9,
18,
7,
8,
14,
6,
124,
25
] | [
"passage: TAGS\n#task_categories-text-classification #task_ids-multi-class-classification #annotations_creators-crowdsourced #annotations_creators-expert-generated #language_creators-found #multilinguality-monolingual #size_categories-100K<n<1M #source_datasets-extended|other #language-English #license-other #relation extraction #arxiv-2104.08398 #region-us \n### Dataset Summary\n\n\nThe TAC Relation Extraction Dataset (TACRED) is a large-scale relation extraction dataset with 106,264 examples built over newswire and web text from the corpus used in the yearly TAC Knowledge Base Population (TAC KBP) challenges. Examples in TACRED cover 41 relation types as used in the TAC KBP challenges (e.g., per:schools\\_attended\nand org:members) or are labeled as no\\_relation if no defined relation is held. These examples are created by combining available human annotations from the TAC\nKBP challenges and crowdsourcing. Please see Stanford's EMNLP paper, or their EMNLP slides for full details.\n\n\nNote:\n\n\n* There is currently a label-corrected version of the TACRED dataset, which you should consider using instead of\nthe original version released in 2017. For more details on this new version, see the TACRED Revisited paper\npublished at ACL 2020.\n* There is also a relabeled and pruned version of the TACRED dataset.\nFor more details on this new version, see the Re-TACRED paper\npublished at ACL 2020.\n\n\nThis repository provides all three versions of the dataset as BuilderConfigs - ''original'', ''revisited'' and ''re-tacred''.\nSimply set the 'name' parameter in the 'load\\_dataset' method in order to choose a specific version. The original TACRED is loaded per default.### Supported Tasks and Leaderboards\n\n\n* Tasks: Relation Classification\n* Leaderboards: URL### Languages\n\n\nThe language in the dataset is English.\n\n\nDataset Structure\n-----------------",
"passage: ### Data Instances\n\n\n* Size of downloaded dataset files: 62.3 MB\n* Size of the generated dataset: 139.2 MB\n* Total amount of disk used: 201.5 MB\n\n\nAn example of 'train' looks as follows:"
] |
c385a6a9a7c200cde48d6b7ed171e9187db8c99a | ---
annotations_creators:
- found
language_creators:
- found
language:
- ar
license:
- other
multilinguality:
- monolingual
pretty_name: disTD
task_categories:
- token-classification
task_ids:
- disfluency-detection
dataset_info:
features:
- name: tokens
sequence: string
- name: isDisf
sequence:
class_label:
names:
0: O
1: B_RM
2: I_RM
3: B_RP
4: I_RP
5: IP
config_name: disTD
# Dataset Card for myds
## Table of Contents
- [Dataset Description](#dataset-description)
- [Dataset Summary](#dataset-summary)
- [Supported Tasks](#supported-tasks-and-leaderboards)
- [Languages](#languages)
- [Dataset Structure](#dataset-structure)
- [Data Instances](#data-instances)
- [Data Fields](#data-instances)
- [Data Splits](#data-instances)
- [Dataset Creation](#dataset-creation)
- [Curation Rationale](#curation-rationale)
- [Source Data](#source-data)
- [Annotations](#annotations)
- [Personal and Sensitive Information](#personal-and-sensitive-information)
- [Considerations for Using the Data](#considerations-for-using-the-data)
- [Social Impact of Dataset](#social-impact-of-dataset)
- [Discussion of Biases](#discussion-of-biases)
- [Other Known Limitations](#other-known-limitations)
- [Additional Information](#additional-information)
- [Dataset Curators](#dataset-curators)
- [Licensing Information](#licensing-information)
- [Citation Information](#citation-information)
## Dataset Description
- **Homepage:** [Needs More Information]
- **Repository:** [Needs More Information]
- **Paper:** [Needs More Information]
- **Leaderboard:** [Needs More Information]
- **Point of Contact:** [Needs More Information]
### Dataset Summary
dataset for Tunisian dialect
### Supported Tasks and Leaderboards
[Needs More Information]
### Languages
tuanisian arabic dialect
## Dataset Structure
### Data Instances
Size of downloaded dataset files: 4.63 MB
Size of the generated dataset: 9.78 MB
Total amount of disk used: 14.41 MB
### Data Fields
dsfsergrth
### Data Splits
rtsert
## Dataset Creation
### Curation Rationale
link
### Source Data
#### Initial Data Collection and Normalization
kink
#### Who are the source language producers?
link
### Annotations
#### Annotation process
tool
#### Who are the annotators?
me
### Personal and Sensitive Information
ok
## Considerations for Using the Data
### Social Impact of Dataset
[Needs More Information]
### Discussion of Biases
[Needs More Information]
### Other Known Limitations
[Needs More Information]
## Additional Information
### Dataset Curators
[Needs More Information]
### Licensing Information
[Needs More Information]
### Citation Information
[Needs More Information] | EmnaBou/tokenDS | [
"region:us"
] | 2022-09-28T10:34:05+00:00 | {} | 2022-11-30T11:32:39+00:00 | [] | [] | TAGS
#region-us
| ---
annotations_creators:
- found
language_creators:
- found
language:
- ar
license:
- other
multilinguality:
- monolingual
pretty_name: disTD
task_categories:
- token-classification
task_ids:
- disfluency-detection
dataset_info:
features:
- name: tokens
sequence: string
- name: isDisf
sequence:
class_label:
names:
0: O
1: B_RM
2: I_RM
3: B_RP
4: I_RP
5: IP
config_name: disTD
# Dataset Card for myds
## Table of Contents
- Dataset Description
- Dataset Summary
- Supported Tasks
- Languages
- Dataset Structure
- Data Instances
- Data Fields
- Data Splits
- Dataset Creation
- Curation Rationale
- Source Data
- Annotations
- Personal and Sensitive Information
- Considerations for Using the Data
- Social Impact of Dataset
- Discussion of Biases
- Other Known Limitations
- Additional Information
- Dataset Curators
- Licensing Information
- Citation Information
## Dataset Description
- Homepage:
- Repository:
- Paper:
- Leaderboard:
- Point of Contact:
### Dataset Summary
dataset for Tunisian dialect
### Supported Tasks and Leaderboards
### Languages
tuanisian arabic dialect
## Dataset Structure
### Data Instances
Size of downloaded dataset files: 4.63 MB
Size of the generated dataset: 9.78 MB
Total amount of disk used: 14.41 MB
### Data Fields
dsfsergrth
### Data Splits
rtsert
## Dataset Creation
### Curation Rationale
link
### Source Data
#### Initial Data Collection and Normalization
kink
#### Who are the source language producers?
link
### Annotations
#### Annotation process
tool
#### Who are the annotators?
me
### Personal and Sensitive Information
ok
## Considerations for Using the Data
### Social Impact of Dataset
### Discussion of Biases
### Other Known Limitations
## Additional Information
### Dataset Curators
### Licensing Information
| [
"# Dataset Card for myds",
"## Table of Contents\n- Dataset Description\n - Dataset Summary\n - Supported Tasks\n - Languages\n- Dataset Structure\n - Data Instances\n - Data Fields\n - Data Splits\n- Dataset Creation\n - Curation Rationale\n - Source Data\n - Annotations\n - Personal and Sensitive Information\n- Considerations for Using the Data\n - Social Impact of Dataset\n - Discussion of Biases\n - Other Known Limitations\n- Additional Information\n - Dataset Curators\n - Licensing Information\n - Citation Information",
"## Dataset Description\n\n- Homepage: \n- Repository: \n- Paper: \n- Leaderboard: \n- Point of Contact:",
"### Dataset Summary\n\ndataset for Tunisian dialect",
"### Supported Tasks and Leaderboards",
"### Languages\n\ntuanisian arabic dialect",
"## Dataset Structure",
"### Data Instances\n\nSize of downloaded dataset files: 4.63 MB\nSize of the generated dataset: 9.78 MB\nTotal amount of disk used: 14.41 MB",
"### Data Fields\n\ndsfsergrth",
"### Data Splits\n\nrtsert",
"## Dataset Creation",
"### Curation Rationale\n\nlink",
"### Source Data",
"#### Initial Data Collection and Normalization\n\nkink",
"#### Who are the source language producers?\n\nlink",
"### Annotations",
"#### Annotation process\n\ntool",
"#### Who are the annotators?\n\nme",
"### Personal and Sensitive Information\n\nok",
"## Considerations for Using the Data",
"### Social Impact of Dataset",
"### Discussion of Biases",
"### Other Known Limitations",
"## Additional Information",
"### Dataset Curators",
"### Licensing Information"
] | [
"TAGS\n#region-us \n",
"# Dataset Card for myds",
"## Table of Contents\n- Dataset Description\n - Dataset Summary\n - Supported Tasks\n - Languages\n- Dataset Structure\n - Data Instances\n - Data Fields\n - Data Splits\n- Dataset Creation\n - Curation Rationale\n - Source Data\n - Annotations\n - Personal and Sensitive Information\n- Considerations for Using the Data\n - Social Impact of Dataset\n - Discussion of Biases\n - Other Known Limitations\n- Additional Information\n - Dataset Curators\n - Licensing Information\n - Citation Information",
"## Dataset Description\n\n- Homepage: \n- Repository: \n- Paper: \n- Leaderboard: \n- Point of Contact:",
"### Dataset Summary\n\ndataset for Tunisian dialect",
"### Supported Tasks and Leaderboards",
"### Languages\n\ntuanisian arabic dialect",
"## Dataset Structure",
"### Data Instances\n\nSize of downloaded dataset files: 4.63 MB\nSize of the generated dataset: 9.78 MB\nTotal amount of disk used: 14.41 MB",
"### Data Fields\n\ndsfsergrth",
"### Data Splits\n\nrtsert",
"## Dataset Creation",
"### Curation Rationale\n\nlink",
"### Source Data",
"#### Initial Data Collection and Normalization\n\nkink",
"#### Who are the source language producers?\n\nlink",
"### Annotations",
"#### Annotation process\n\ntool",
"#### Who are the annotators?\n\nme",
"### Personal and Sensitive Information\n\nok",
"## Considerations for Using the Data",
"### Social Impact of Dataset",
"### Discussion of Biases",
"### Other Known Limitations",
"## Additional Information",
"### Dataset Curators",
"### Licensing Information"
] | [
6,
7,
112,
24,
12,
10,
10,
6,
37,
11,
8,
5,
8,
4,
12,
11,
5,
6,
10,
9,
8,
7,
8,
7,
5,
6,
6
] | [
"passage: TAGS\n#region-us \n# Dataset Card for myds## Table of Contents\n- Dataset Description\n - Dataset Summary\n - Supported Tasks\n - Languages\n- Dataset Structure\n - Data Instances\n - Data Fields\n - Data Splits\n- Dataset Creation\n - Curation Rationale\n - Source Data\n - Annotations\n - Personal and Sensitive Information\n- Considerations for Using the Data\n - Social Impact of Dataset\n - Discussion of Biases\n - Other Known Limitations\n- Additional Information\n - Dataset Curators\n - Licensing Information\n - Citation Information## Dataset Description\n\n- Homepage: \n- Repository: \n- Paper: \n- Leaderboard: \n- Point of Contact:### Dataset Summary\n\ndataset for Tunisian dialect### Supported Tasks and Leaderboards### Languages\n\ntuanisian arabic dialect## Dataset Structure### Data Instances\n\nSize of downloaded dataset files: 4.63 MB\nSize of the generated dataset: 9.78 MB\nTotal amount of disk used: 14.41 MB### Data Fields\n\ndsfsergrth### Data Splits\n\nrtsert## Dataset Creation### Curation Rationale\n\nlink### Source Data#### Initial Data Collection and Normalization\n\nkink#### Who are the source language producers?\n\nlink### Annotations#### Annotation process\n\ntool#### Who are the annotators?\n\nme### Personal and Sensitive Information\n\nok## Considerations for Using the Data### Social Impact of Dataset### Discussion of Biases### Other Known Limitations## Additional Information### Dataset Curators### Licensing Information"
] |
91e996a3d990bddbd4c554f54ebe821afc978fb9 |
# UD_Catalan-AnCora
## Table of Contents
- [Table of Contents](#table-of-contents)
- [Dataset Description](#dataset-description)
- [Dataset Summary](#dataset-summary)
- [Supported Tasks and Leaderboards](#supported-tasks-and-leaderboards)
- [Languages](#languages)
- [Dataset Structure](#dataset-structure)
- [Data Instances](#data-instances)
- [Data Fields](#data-fields)
- [Data Splits](#data-splits)
- [Dataset Creation](#dataset-creation)
- [Curation Rationale](#curation-rationale)
- [Source Data](#source-data)
- [Annotations](#annotations)
- [Personal and Sensitive Information](#personal-and-sensitive-information)
- [Considerations for Using the Data](#considerations-for-using-the-data)
- [Social Impact of Dataset](#social-impact-of-dataset)
- [Discussion of Biases](#discussion-of-biases)
- [Other Known Limitations](#other-known-limitations)
- [Additional Information](#additional-information)
- [Dataset Curators](#dataset-curators)
- [Licensing Information](#licensing-information)
- [Citation Information](#citation-information)
- [Contributions](#contributions)
## Dataset Description
- **Website:** https://github.com/UniversalDependencies/UD_Catalan-AnCora
- **Point of Contact:** [Daniel Zeman]([email protected])
### Dataset Summary
This dataset is composed of the annotations from the [AnCora corpus](http://clic.ub.edu/corpus/), projected on the [Universal Dependencies treebank](https://universaldependencies.org/). We use the POS annotations of this corpus as part of the [Catalan Language Understanding Benchmark (CLUB)](https://club.aina.bsc.es/).
This work is licensed under a <a rel="license" href="https://creativecommons.org/licenses/by/4.0/">CC Attribution 4.0 International License</a>.
### Supported Tasks and Leaderboards
POS tagging
### Languages
The dataset is in Catalan (`ca-ES`)
## Dataset Structure
### Data Instances
Three conllu files.
Annotations are encoded in plain text files (UTF-8, normalized to NFC, using only the LF character as line break, including an LF character at the end of file) with three types of lines:
1) Word lines containing the annotation of a word/token in 10 fields separated by single tab characters (see below).
2) Blank lines marking sentence boundaries.
3) Comment lines starting with hash (#).
### Data Fields
Word lines contain the following fields:
1) ID: Word index, integer starting at 1 for each new sentence; may be a range for multiword tokens; may be a decimal number for empty nodes (decimal numbers can be lower than 1 but must be greater than 0).
2) FORM: Word form or punctuation symbol.
3) LEMMA: Lemma or stem of word form.
4) UPOS: Universal part-of-speech tag.
5) XPOS: Language-specific part-of-speech tag; underscore if not available.
6) FEATS: List of morphological features from the universal feature inventory or from a defined language-specific extension; underscore if not available.
7) HEAD: Head of the current word, which is either a value of ID or zero (0).
8) DEPREL: Universal dependency relation to the HEAD (root iff HEAD = 0) or a defined language-specific subtype of one.
9) DEPS: Enhanced dependency graph in the form of a list of head-deprel pairs.
10) MISC: Any other annotation.
From: [https://universaldependencies.org](https://universaldependencies.org/guidelines.html)
### Data Splits
- ca_ancora-ud-train.conllu
- ca_ancora-ud-dev.conllu
- ca_ancora-ud-test.conllu
## Dataset Creation
### Curation Rationale
[N/A]
### Source Data
- [UD_Catalan-AnCora](https://github.com/UniversalDependencies/UD_Catalan-AnCora)
#### Initial Data Collection and Normalization
The original annotation was done in a constituency framework as a part of the [AnCora project](http://clic.ub.edu/corpus/) at the University of Barcelona. It was converted to dependencies by the [Universal Dependencies team](https://universaldependencies.org/) and used in the CoNLL 2009 shared task. The CoNLL 2009 version was later converted to HamleDT and to Universal Dependencies.
For more information on the AnCora project, visit the [AnCora site](http://clic.ub.edu/corpus/).
To learn about the Universal Dependences, visit the webpage [https://universaldependencies.org](https://universaldependencies.org)
#### Who are the source language producers?
For more information on the AnCora corpus and its sources, visit the [AnCora site](http://clic.ub.edu/corpus/).
### Annotations
#### Annotation process
For more information on the first AnCora annotation, visit the [AnCora site](http://clic.ub.edu/corpus/).
#### Who are the annotators?
For more information on the AnCora annotation team, visit the [AnCora site](http://clic.ub.edu/corpus/).
### Personal and Sensitive Information
No personal or sensitive information included.
## Considerations for Using the Data
### Social Impact of Dataset
This dataset contributes to the development of language models in Catalan, a low-resource language.
### Discussion of Biases
[N/A]
### Other Known Limitations
[N/A]
## Additional Information
### Dataset Curators
### Licensing Information
This work is licensed under a <a rel="license" href="https://creativecommons.org/licenses/by/4.0/">CC Attribution 4.0 International License</a>.
### Citation Information
The following paper must be cited when using this corpus:
Taulรฉ, M., M.A. Martรญ, M. Recasens (2008) 'Ancora: Multilevel Annotated Corpora for Catalan and Spanish', Proceedings of 6th International Conference on Language Resources and Evaluation. Marrakesh (Morocco).
To cite the Universal Dependencies project:
Rueter, J. (Creator), Erina, O. (Contributor), Klementeva, J. (Contributor), Ryabov, I. (Contributor), Tyers, F. M. (Contributor), Zeman, D. (Contributor), Nivre, J. (Creator) (15 Nov 2020). Universal Dependencies version 2.7 Erzya JR. Universal Dependencies Consortium.
| projecte-aina/UD_Catalan-AnCora | [
"task_categories:token-classification",
"task_ids:part-of-speech",
"annotations_creators:expert-generated",
"language_creators:found",
"multilinguality:monolingual",
"language:ca",
"license:cc-by-4.0",
"region:us"
] | 2022-09-28T10:51:06+00:00 | {"annotations_creators": ["expert-generated"], "language_creators": ["found"], "language": ["ca"], "license": ["cc-by-4.0"], "multilinguality": ["monolingual"], "size_categories": [], "source_datasets": [], "task_categories": ["token-classification"], "task_ids": ["part-of-speech"], "pretty_name": "UD_Catalan-AnCora", "tags": []} | 2023-11-25T06:31:40+00:00 | [] | [
"ca"
] | TAGS
#task_categories-token-classification #task_ids-part-of-speech #annotations_creators-expert-generated #language_creators-found #multilinguality-monolingual #language-Catalan #license-cc-by-4.0 #region-us
|
# UD_Catalan-AnCora
## Table of Contents
- Table of Contents
- Dataset Description
- Dataset Summary
- Supported Tasks and Leaderboards
- Languages
- Dataset Structure
- Data Instances
- Data Fields
- Data Splits
- Dataset Creation
- Curation Rationale
- Source Data
- Annotations
- Personal and Sensitive Information
- Considerations for Using the Data
- Social Impact of Dataset
- Discussion of Biases
- Other Known Limitations
- Additional Information
- Dataset Curators
- Licensing Information
- Citation Information
- Contributions
## Dataset Description
- Website: URL
- Point of Contact: Daniel Zeman
### Dataset Summary
This dataset is composed of the annotations from the AnCora corpus, projected on the Universal Dependencies treebank. We use the POS annotations of this corpus as part of the Catalan Language Understanding Benchmark (CLUB).
This work is licensed under a <a rel="license" href="URL Attribution 4.0 International License</a>.
### Supported Tasks and Leaderboards
POS tagging
### Languages
The dataset is in Catalan ('ca-ES')
## Dataset Structure
### Data Instances
Three conllu files.
Annotations are encoded in plain text files (UTF-8, normalized to NFC, using only the LF character as line break, including an LF character at the end of file) with three types of lines:
1) Word lines containing the annotation of a word/token in 10 fields separated by single tab characters (see below).
2) Blank lines marking sentence boundaries.
3) Comment lines starting with hash (#).
### Data Fields
Word lines contain the following fields:
1) ID: Word index, integer starting at 1 for each new sentence; may be a range for multiword tokens; may be a decimal number for empty nodes (decimal numbers can be lower than 1 but must be greater than 0).
2) FORM: Word form or punctuation symbol.
3) LEMMA: Lemma or stem of word form.
4) UPOS: Universal part-of-speech tag.
5) XPOS: Language-specific part-of-speech tag; underscore if not available.
6) FEATS: List of morphological features from the universal feature inventory or from a defined language-specific extension; underscore if not available.
7) HEAD: Head of the current word, which is either a value of ID or zero (0).
8) DEPREL: Universal dependency relation to the HEAD (root iff HEAD = 0) or a defined language-specific subtype of one.
9) DEPS: Enhanced dependency graph in the form of a list of head-deprel pairs.
10) MISC: Any other annotation.
From: URL
### Data Splits
- ca_ancora-URL
- ca_ancora-URL
- ca_ancora-URL
## Dataset Creation
### Curation Rationale
[N/A]
### Source Data
- UD_Catalan-AnCora
#### Initial Data Collection and Normalization
The original annotation was done in a constituency framework as a part of the AnCora project at the University of Barcelona. It was converted to dependencies by the Universal Dependencies team and used in the CoNLL 2009 shared task. The CoNLL 2009 version was later converted to HamleDT and to Universal Dependencies.
For more information on the AnCora project, visit the AnCora site.
To learn about the Universal Dependences, visit the webpage URL
#### Who are the source language producers?
For more information on the AnCora corpus and its sources, visit the AnCora site.
### Annotations
#### Annotation process
For more information on the first AnCora annotation, visit the AnCora site.
#### Who are the annotators?
For more information on the AnCora annotation team, visit the AnCora site.
### Personal and Sensitive Information
No personal or sensitive information included.
## Considerations for Using the Data
### Social Impact of Dataset
This dataset contributes to the development of language models in Catalan, a low-resource language.
### Discussion of Biases
[N/A]
### Other Known Limitations
[N/A]
## Additional Information
### Dataset Curators
### Licensing Information
This work is licensed under a <a rel="license" href="URL Attribution 4.0 International License</a>.
The following paper must be cited when using this corpus:
Taulรฉ, M., M.A. Martรญ, M. Recasens (2008) 'Ancora: Multilevel Annotated Corpora for Catalan and Spanish', Proceedings of 6th International Conference on Language Resources and Evaluation. Marrakesh (Morocco).
To cite the Universal Dependencies project:
Rueter, J. (Creator), Erina, O. (Contributor), Klementeva, J. (Contributor), Ryabov, I. (Contributor), Tyers, F. M. (Contributor), Zeman, D. (Contributor), Nivre, J. (Creator) (15 Nov 2020). Universal Dependencies version 2.7 Erzya JR. Universal Dependencies Consortium.
| [
"# UD_Catalan-AnCora",
"## Table of Contents\n- Table of Contents\n- Dataset Description\n - Dataset Summary\n - Supported Tasks and Leaderboards\n - Languages\n- Dataset Structure\n - Data Instances\n - Data Fields\n - Data Splits\n- Dataset Creation\n - Curation Rationale\n - Source Data\n - Annotations\n - Personal and Sensitive Information\n- Considerations for Using the Data\n - Social Impact of Dataset\n - Discussion of Biases\n - Other Known Limitations\n- Additional Information\n - Dataset Curators\n - Licensing Information\n - Citation Information\n - Contributions",
"## Dataset Description\n- Website: URL\n- Point of Contact: Daniel Zeman",
"### Dataset Summary\n\nThis dataset is composed of the annotations from the AnCora corpus, projected on the Universal Dependencies treebank. We use the POS annotations of this corpus as part of the Catalan Language Understanding Benchmark (CLUB).\n\nThis work is licensed under a <a rel=\"license\" href=\"URL Attribution 4.0 International License</a>.",
"### Supported Tasks and Leaderboards\n\nPOS tagging",
"### Languages\n\nThe dataset is in Catalan ('ca-ES')",
"## Dataset Structure",
"### Data Instances\n\nThree conllu files.\n\nAnnotations are encoded in plain text files (UTF-8, normalized to NFC, using only the LF character as line break, including an LF character at the end of file) with three types of lines:\n\n1) Word lines containing the annotation of a word/token in 10 fields separated by single tab characters (see below).\n2) Blank lines marking sentence boundaries.\n3) Comment lines starting with hash (#).",
"### Data Fields\nWord lines contain the following fields:\n\n1) ID: Word index, integer starting at 1 for each new sentence; may be a range for multiword tokens; may be a decimal number for empty nodes (decimal numbers can be lower than 1 but must be greater than 0).\n2) FORM: Word form or punctuation symbol.\n3) LEMMA: Lemma or stem of word form.\n4) UPOS: Universal part-of-speech tag.\n5) XPOS: Language-specific part-of-speech tag; underscore if not available.\n6) FEATS: List of morphological features from the universal feature inventory or from a defined language-specific extension; underscore if not available.\n7) HEAD: Head of the current word, which is either a value of ID or zero (0).\n8) DEPREL: Universal dependency relation to the HEAD (root iff HEAD = 0) or a defined language-specific subtype of one.\n9) DEPS: Enhanced dependency graph in the form of a list of head-deprel pairs.\n10) MISC: Any other annotation.\n \nFrom: URL",
"### Data Splits\n\n- ca_ancora-URL\n- ca_ancora-URL\n- ca_ancora-URL",
"## Dataset Creation",
"### Curation Rationale\n[N/A]",
"### Source Data\n\n- UD_Catalan-AnCora",
"#### Initial Data Collection and Normalization\n\nThe original annotation was done in a constituency framework as a part of the AnCora project at the University of Barcelona. It was converted to dependencies by the Universal Dependencies team and used in the CoNLL 2009 shared task. The CoNLL 2009 version was later converted to HamleDT and to Universal Dependencies.\n\nFor more information on the AnCora project, visit the AnCora site.\n\nTo learn about the Universal Dependences, visit the webpage URL",
"#### Who are the source language producers?\n\nFor more information on the AnCora corpus and its sources, visit the AnCora site.",
"### Annotations",
"#### Annotation process\n\nFor more information on the first AnCora annotation, visit the AnCora site.",
"#### Who are the annotators?\n\nFor more information on the AnCora annotation team, visit the AnCora site.",
"### Personal and Sensitive Information\n\nNo personal or sensitive information included.",
"## Considerations for Using the Data",
"### Social Impact of Dataset\n\nThis dataset contributes to the development of language models in Catalan, a low-resource language.",
"### Discussion of Biases\n\n[N/A]",
"### Other Known Limitations\n\n[N/A]",
"## Additional Information",
"### Dataset Curators",
"### Licensing Information\n\nThis work is licensed under a <a rel=\"license\" href=\"URL Attribution 4.0 International License</a>.\n\n\n\nThe following paper must be cited when using this corpus:\n\nTaulรฉ, M., M.A. Martรญ, M. Recasens (2008) 'Ancora: Multilevel Annotated Corpora for Catalan and Spanish', Proceedings of 6th International Conference on Language Resources and Evaluation. Marrakesh (Morocco).\n\nTo cite the Universal Dependencies project:\n\nRueter, J. (Creator), Erina, O. (Contributor), Klementeva, J. (Contributor), Ryabov, I. (Contributor), Tyers, F. M. (Contributor), Zeman, D. (Contributor), Nivre, J. (Creator) (15 Nov 2020). Universal Dependencies version 2.7 Erzya JR. Universal Dependencies Consortium."
] | [
"TAGS\n#task_categories-token-classification #task_ids-part-of-speech #annotations_creators-expert-generated #language_creators-found #multilinguality-monolingual #language-Catalan #license-cc-by-4.0 #region-us \n",
"# UD_Catalan-AnCora",
"## Table of Contents\n- Table of Contents\n- Dataset Description\n - Dataset Summary\n - Supported Tasks and Leaderboards\n - Languages\n- Dataset Structure\n - Data Instances\n - Data Fields\n - Data Splits\n- Dataset Creation\n - Curation Rationale\n - Source Data\n - Annotations\n - Personal and Sensitive Information\n- Considerations for Using the Data\n - Social Impact of Dataset\n - Discussion of Biases\n - Other Known Limitations\n- Additional Information\n - Dataset Curators\n - Licensing Information\n - Citation Information\n - Contributions",
"## Dataset Description\n- Website: URL\n- Point of Contact: Daniel Zeman",
"### Dataset Summary\n\nThis dataset is composed of the annotations from the AnCora corpus, projected on the Universal Dependencies treebank. We use the POS annotations of this corpus as part of the Catalan Language Understanding Benchmark (CLUB).\n\nThis work is licensed under a <a rel=\"license\" href=\"URL Attribution 4.0 International License</a>.",
"### Supported Tasks and Leaderboards\n\nPOS tagging",
"### Languages\n\nThe dataset is in Catalan ('ca-ES')",
"## Dataset Structure",
"### Data Instances\n\nThree conllu files.\n\nAnnotations are encoded in plain text files (UTF-8, normalized to NFC, using only the LF character as line break, including an LF character at the end of file) with three types of lines:\n\n1) Word lines containing the annotation of a word/token in 10 fields separated by single tab characters (see below).\n2) Blank lines marking sentence boundaries.\n3) Comment lines starting with hash (#).",
"### Data Fields\nWord lines contain the following fields:\n\n1) ID: Word index, integer starting at 1 for each new sentence; may be a range for multiword tokens; may be a decimal number for empty nodes (decimal numbers can be lower than 1 but must be greater than 0).\n2) FORM: Word form or punctuation symbol.\n3) LEMMA: Lemma or stem of word form.\n4) UPOS: Universal part-of-speech tag.\n5) XPOS: Language-specific part-of-speech tag; underscore if not available.\n6) FEATS: List of morphological features from the universal feature inventory or from a defined language-specific extension; underscore if not available.\n7) HEAD: Head of the current word, which is either a value of ID or zero (0).\n8) DEPREL: Universal dependency relation to the HEAD (root iff HEAD = 0) or a defined language-specific subtype of one.\n9) DEPS: Enhanced dependency graph in the form of a list of head-deprel pairs.\n10) MISC: Any other annotation.\n \nFrom: URL",
"### Data Splits\n\n- ca_ancora-URL\n- ca_ancora-URL\n- ca_ancora-URL",
"## Dataset Creation",
"### Curation Rationale\n[N/A]",
"### Source Data\n\n- UD_Catalan-AnCora",
"#### Initial Data Collection and Normalization\n\nThe original annotation was done in a constituency framework as a part of the AnCora project at the University of Barcelona. It was converted to dependencies by the Universal Dependencies team and used in the CoNLL 2009 shared task. The CoNLL 2009 version was later converted to HamleDT and to Universal Dependencies.\n\nFor more information on the AnCora project, visit the AnCora site.\n\nTo learn about the Universal Dependences, visit the webpage URL",
"#### Who are the source language producers?\n\nFor more information on the AnCora corpus and its sources, visit the AnCora site.",
"### Annotations",
"#### Annotation process\n\nFor more information on the first AnCora annotation, visit the AnCora site.",
"#### Who are the annotators?\n\nFor more information on the AnCora annotation team, visit the AnCora site.",
"### Personal and Sensitive Information\n\nNo personal or sensitive information included.",
"## Considerations for Using the Data",
"### Social Impact of Dataset\n\nThis dataset contributes to the development of language models in Catalan, a low-resource language.",
"### Discussion of Biases\n\n[N/A]",
"### Other Known Limitations\n\n[N/A]",
"## Additional Information",
"### Dataset Curators",
"### Licensing Information\n\nThis work is licensed under a <a rel=\"license\" href=\"URL Attribution 4.0 International License</a>.\n\n\n\nThe following paper must be cited when using this corpus:\n\nTaulรฉ, M., M.A. Martรญ, M. Recasens (2008) 'Ancora: Multilevel Annotated Corpora for Catalan and Spanish', Proceedings of 6th International Conference on Language Resources and Evaluation. Marrakesh (Morocco).\n\nTo cite the Universal Dependencies project:\n\nRueter, J. (Creator), Erina, O. (Contributor), Klementeva, J. (Contributor), Ryabov, I. (Contributor), Tyers, F. M. (Contributor), Zeman, D. (Contributor), Nivre, J. (Creator) (15 Nov 2020). Universal Dependencies version 2.7 Erzya JR. Universal Dependencies Consortium."
] | [
73,
9,
125,
15,
87,
13,
17,
6,
107,
251,
26,
5,
12,
13,
115,
30,
5,
24,
28,
15,
8,
28,
13,
12,
5,
6,
214
] | [
"passage: TAGS\n#task_categories-token-classification #task_ids-part-of-speech #annotations_creators-expert-generated #language_creators-found #multilinguality-monolingual #language-Catalan #license-cc-by-4.0 #region-us \n# UD_Catalan-AnCora## Table of Contents\n- Table of Contents\n- Dataset Description\n - Dataset Summary\n - Supported Tasks and Leaderboards\n - Languages\n- Dataset Structure\n - Data Instances\n - Data Fields\n - Data Splits\n- Dataset Creation\n - Curation Rationale\n - Source Data\n - Annotations\n - Personal and Sensitive Information\n- Considerations for Using the Data\n - Social Impact of Dataset\n - Discussion of Biases\n - Other Known Limitations\n- Additional Information\n - Dataset Curators\n - Licensing Information\n - Citation Information\n - Contributions## Dataset Description\n- Website: URL\n- Point of Contact: Daniel Zeman### Dataset Summary\n\nThis dataset is composed of the annotations from the AnCora corpus, projected on the Universal Dependencies treebank. We use the POS annotations of this corpus as part of the Catalan Language Understanding Benchmark (CLUB).\n\nThis work is licensed under a <a rel=\"license\" href=\"URL Attribution 4.0 International License</a>.### Supported Tasks and Leaderboards\n\nPOS tagging### Languages\n\nThe dataset is in Catalan ('ca-ES')## Dataset Structure### Data Instances\n\nThree conllu files.\n\nAnnotations are encoded in plain text files (UTF-8, normalized to NFC, using only the LF character as line break, including an LF character at the end of file) with three types of lines:\n\n1) Word lines containing the annotation of a word/token in 10 fields separated by single tab characters (see below).\n2) Blank lines marking sentence boundaries.\n3) Comment lines starting with hash (#).",
"passage: ### Data Fields\nWord lines contain the following fields:\n\n1) ID: Word index, integer starting at 1 for each new sentence; may be a range for multiword tokens; may be a decimal number for empty nodes (decimal numbers can be lower than 1 but must be greater than 0).\n2) FORM: Word form or punctuation symbol.\n3) LEMMA: Lemma or stem of word form.\n4) UPOS: Universal part-of-speech tag.\n5) XPOS: Language-specific part-of-speech tag; underscore if not available.\n6) FEATS: List of morphological features from the universal feature inventory or from a defined language-specific extension; underscore if not available.\n7) HEAD: Head of the current word, which is either a value of ID or zero (0).\n8) DEPREL: Universal dependency relation to the HEAD (root iff HEAD = 0) or a defined language-specific subtype of one.\n9) DEPS: Enhanced dependency graph in the form of a list of head-deprel pairs.\n10) MISC: Any other annotation.\n \nFrom: URL### Data Splits\n\n- ca_ancora-URL\n- ca_ancora-URL\n- ca_ancora-URL## Dataset Creation### Curation Rationale\n[N/A]### Source Data\n\n- UD_Catalan-AnCora#### Initial Data Collection and Normalization\n\nThe original annotation was done in a constituency framework as a part of the AnCora project at the University of Barcelona. It was converted to dependencies by the Universal Dependencies team and used in the CoNLL 2009 shared task. The CoNLL 2009 version was later converted to HamleDT and to Universal Dependencies.\n\nFor more information on the AnCora project, visit the AnCora site.\n\nTo learn about the Universal Dependences, visit the webpage URL#### Who are the source language producers?\n\nFor more information on the AnCora corpus and its sources, visit the AnCora site.### Annotations#### Annotation process\n\nFor more information on the first AnCora annotation, visit the AnCora site.#### Who are the annotators?\n\nFor more information on the AnCora annotation team, visit the AnCora site.### Personal and Sensitive Information\n\nNo personal or sensitive information included.## Considerations for Using the Data### Social Impact of Dataset\n\nThis dataset contributes to the development of language models in Catalan, a low-resource language.### Discussion of Biases\n\n[N/A]### Other Known Limitations\n\n[N/A]## Additional Information### Dataset Curators"
] |
8a5e23f6ffbd1b55efaf0ffe6322f985fe859bf2 |
# Dataset Card for xP3
## Table of Contents
- [Table of Contents](#table-of-contents)
- [Dataset Description](#dataset-description)
- [Dataset Summary](#dataset-summary)
- [Supported Tasks and Leaderboards](#supported-tasks-and-leaderboards)
- [Languages](#languages)
- [Dataset Structure](#dataset-structure)
- [Data Instances](#data-instances)
- [Data Fields](#data-fields)
- [Data Splits](#data-splits)
- [Dataset Creation](#dataset-creation)
- [Curation Rationale](#curation-rationale)
- [Source Data](#source-data)
- [Annotations](#annotations)
- [Additional Information](#additional-information)
- [Licensing Information](#licensing-information)
- [Citation Information](#citation-information)
- [Contributions](#contributions)
## Dataset Description
- **Repository:** https://github.com/bigscience-workshop/xmtf
- **Paper:** [Crosslingual Generalization through Multitask Finetuning](https://arxiv.org/abs/2211.01786)
- **Point of Contact:** [Niklas Muennighoff](mailto:[email protected])
### Dataset Summary
> xP3 (Crosslingual Public Pool of Prompts) is a collection of prompts & datasets across 46 of languages & 16 NLP tasks. It is used for the training of BLOOMZ and mT0, multilingual language models capable of following human instructions in dozens of languages zero-shot.
- **Creation:** The dataset can be recreated using instructions available [here](https://github.com/bigscience-workshop/xmtf#create-xp3). We provide this version to save processing time and ease reproducibility.
- **Languages:** 46 (Can be extended by [recreating with more splits](https://github.com/bigscience-workshop/xmtf#create-xp3))
- **xP3 Dataset Family:**
<table>
<tr>
<th>Name</th>
<th>Explanation</th>
<th>Example models</th>
</tr>
<tr>
<td><a href=https://huggingface.co/datasets/Muennighoff/xP3x>xP3x</a></t>
<td>Mixture of 17 tasks in 277 languages with English prompts</td>
<td>WIP - Join us at Project Aya @<a href=https://cohere.for.ai/>C4AI</a> to help!</td>
</tr>
<tr>
<td><a href=https://huggingface.co/datasets/bigscience/xP3>xP3</a></t>
<td>Mixture of 13 training tasks in 46 languages with English prompts</td>
<td><a href=https://huggingface.co/bigscience/bloomz>bloomz</a> & <a href=https://huggingface.co/bigscience/mt0-xxl>mt0-xxl</a></td>
</tr>
<tr>
<td><a href=https://huggingface.co/datasets/bigscience/xP3mt>xP3mt</a></t>
<td>Mixture of 13 training tasks in 46 languages with prompts in 20 languages (machine-translated from English)</td>
<td><a href=https://huggingface.co/bigscience/bloomz-mt>bloomz-mt</a> & <a href=https://huggingface.co/bigscience/mt0-xxl-mt>mt0-xxl-mt</a></td>
</tr>
<tr>
<td><a href=https://huggingface.co/datasets/bigscience/xP3all>xP3all</a></t>
<td>xP3 + evaluation datasets adding an additional 3 tasks for a total of 16 tasks in 46 languages with English prompts</td>
<td></td>
</tr>
<tr>
<td><a href=https://huggingface.co/datasets/bigscience/xP3megds>xP3megds</a></t>
<td><a href=https://github.com/bigscience-workshop/Megatron-DeepSpeed>Megatron-DeepSpeed</a> processed version of xP3</td>
<td><a href=https://huggingface.co/bigscience/bloomz>bloomz</a></td>
</tr>
<tr>
<td><a href=https://huggingface.co/datasets/Muennighoff/P3>P3</a></t>
<td>Repreprocessed version of the English-only <a href=https://huggingface.co/datasets/bigscience/P3>P3</a> with 8 training tasks</td>
<td><a href=https://huggingface.co/bigscience/bloomz-p3>bloomz-p3</a> & <a href=https://huggingface.co/bigscience/mt0-xxl-p3>mt0-xxl-p3</a></td>
</tr>
</table>
## Dataset Structure
### Data Instances
An example of "train" looks as follows:
```json
{
"inputs": "Oraciรณn 1: Fue acadรฉmico en literatura metafรญsica, teologรญa y ciencias clรกsicas.\Oraciรณn 2: Fue acadรฉmico en literatura metafรญsica, teologรญa y ciencia clรกsica.\nPregunta: ยฟLa oraciรณn 1 parafrasea la oraciรณn 2? ยฟSi o no?",
"targets": "Sรญ"
}
```
### Data Fields
The data fields are the same among all splits:
- `inputs`: the natural language input fed to the model
- `targets`: the natural language target that the model has to generate
### Data Splits
The below table summarizes sizes per language (computed from the `merged_{lang}.jsonl` files). Due to languages like `tw` only being single sentence translation samples from Flores, their byte percentage is significantly lower than their sample percentage. We machine-translated prompts for monolingual datasets, thus languages with only crosslingual datasets (e.g. Translation) do not have non-English prompts. Languages without non-English prompts are equivalent to [xP3](https://huggingface.co/datasets/bigscience/xP3).
|Language|Kilobytes|%|Samples|%|Non-English prompts|
|--------|------:|-:|---:|-:|-:|
|tw|106288|0.11|265071|0.33| |
|bm|107056|0.11|265180|0.33| |
|ak|108096|0.11|265071|0.33| |
|ca|110608|0.11|271191|0.34| |
|eu|113008|0.12|281199|0.35| |
|fon|113072|0.12|265063|0.33| |
|st|114080|0.12|265063|0.33| |
|ki|115040|0.12|265180|0.33| |
|tum|116032|0.12|265063|0.33| |
|wo|122560|0.13|365063|0.46| |
|ln|126304|0.13|365060|0.46| |
|as|156256|0.16|265063|0.33| |
|or|161472|0.17|265063|0.33| |
|kn|165456|0.17|265063|0.33| |
|ml|175040|0.18|265864|0.33| |
|rn|192992|0.2|318189|0.4| |
|nso|229712|0.24|915051|1.14| |
|tn|235536|0.24|915054|1.14| |
|lg|235936|0.24|915021|1.14| |
|rw|249360|0.26|915043|1.14| |
|ts|250256|0.26|915044|1.14| |
|sn|252496|0.26|865056|1.08| |
|xh|254672|0.26|915058|1.14| |
|zu|263712|0.27|915061|1.14| |
|ny|272128|0.28|915063|1.14| |
|ig|325440|0.33|950097|1.19|โ
|
|yo|339664|0.35|913021|1.14|โ
|
|ne|398144|0.41|315754|0.39|โ
|
|pa|529632|0.55|339210|0.42|โ
|
|sw|561392|0.58|1114439|1.39|โ
|
|gu|566576|0.58|347499|0.43|โ
|
|mr|674000|0.69|417269|0.52|โ
|
|bn|854864|0.88|428725|0.54|โ
|
|ta|943440|0.97|410633|0.51|โ
|
|te|1384016|1.42|573354|0.72|โ
|
|ur|1944416|2.0|855756|1.07|โ
|
|vi|3113184|3.2|1667306|2.08|โ
|
|code|4330752|4.46|2707724|3.38| |
|hi|4469712|4.6|1543441|1.93|โ
|
|id|4538768|4.67|2582272|3.22|โ
|
|zh|4604112|4.74|3571636|4.46|โ
|
|ar|4703968|4.84|2148970|2.68|โ
|
|fr|5558912|5.72|5055942|6.31|โ
|
|pt|6130016|6.31|3562772|4.45|โ
|
|es|7579424|7.8|5151349|6.43|โ
|
|en|39252528|40.4|32740750|40.87| |
|total|97150128|100.0|80100816|100.0|โ
|
## Dataset Creation
### Source Data
#### Training datasets
- Code Miscellaneous
- [CodeComplex](https://huggingface.co/datasets/codeparrot/codecomplex)
- [Docstring Corpus](https://huggingface.co/datasets/teven/code_docstring_corpus)
- [GreatCode](https://huggingface.co/datasets/great_code)
- [State Changes](https://huggingface.co/datasets/Fraser/python-state-changes)
- Closed-book QA
- [Hotpot QA](https://huggingface.co/datasets/hotpot_qa)
- [Trivia QA](https://huggingface.co/datasets/trivia_qa)
- [Web Questions](https://huggingface.co/datasets/web_questions)
- [Wiki QA](https://huggingface.co/datasets/wiki_qa)
- Extractive QA
- [Adversarial QA](https://huggingface.co/datasets/adversarial_qa)
- [CMRC2018](https://huggingface.co/datasets/cmrc2018)
- [DRCD](https://huggingface.co/datasets/clue)
- [DuoRC](https://huggingface.co/datasets/duorc)
- [MLQA](https://huggingface.co/datasets/mlqa)
- [Quoref](https://huggingface.co/datasets/quoref)
- [ReCoRD](https://huggingface.co/datasets/super_glue)
- [ROPES](https://huggingface.co/datasets/ropes)
- [SQuAD v2](https://huggingface.co/datasets/squad_v2)
- [xQuAD](https://huggingface.co/datasets/xquad)
- TyDI QA
- [Primary](https://huggingface.co/datasets/khalidalt/tydiqa-primary)
- [Goldp](https://huggingface.co/datasets/khalidalt/tydiqa-goldp)
- Multiple-Choice QA
- [ARC](https://huggingface.co/datasets/ai2_arc)
- [C3](https://huggingface.co/datasets/c3)
- [CoS-E](https://huggingface.co/datasets/cos_e)
- [Cosmos](https://huggingface.co/datasets/cosmos)
- [DREAM](https://huggingface.co/datasets/dream)
- [MultiRC](https://huggingface.co/datasets/super_glue)
- [OpenBookQA](https://huggingface.co/datasets/openbookqa)
- [PiQA](https://huggingface.co/datasets/piqa)
- [QUAIL](https://huggingface.co/datasets/quail)
- [QuaRel](https://huggingface.co/datasets/quarel)
- [QuaRTz](https://huggingface.co/datasets/quartz)
- [QASC](https://huggingface.co/datasets/qasc)
- [RACE](https://huggingface.co/datasets/race)
- [SciQ](https://huggingface.co/datasets/sciq)
- [Social IQA](https://huggingface.co/datasets/social_i_qa)
- [Wiki Hop](https://huggingface.co/datasets/wiki_hop)
- [WiQA](https://huggingface.co/datasets/wiqa)
- Paraphrase Identification
- [MRPC](https://huggingface.co/datasets/super_glue)
- [PAWS](https://huggingface.co/datasets/paws)
- [PAWS-X](https://huggingface.co/datasets/paws-x)
- [QQP](https://huggingface.co/datasets/qqp)
- Program Synthesis
- [APPS](https://huggingface.co/datasets/codeparrot/apps)
- [CodeContests](https://huggingface.co/datasets/teven/code_contests)
- [JupyterCodePairs](https://huggingface.co/datasets/codeparrot/github-jupyter-text-code-pairs)
- [MBPP](https://huggingface.co/datasets/Muennighoff/mbpp)
- [NeuralCodeSearch](https://huggingface.co/datasets/neural_code_search)
- [XLCoST](https://huggingface.co/datasets/codeparrot/xlcost-text-to-code)
- Structure-to-text
- [Common Gen](https://huggingface.co/datasets/common_gen)
- [Wiki Bio](https://huggingface.co/datasets/wiki_bio)
- Sentiment
- [Amazon](https://huggingface.co/datasets/amazon_polarity)
- [App Reviews](https://huggingface.co/datasets/app_reviews)
- [IMDB](https://huggingface.co/datasets/imdb)
- [Rotten Tomatoes](https://huggingface.co/datasets/rotten_tomatoes)
- [Yelp](https://huggingface.co/datasets/yelp_review_full)
- Simplification
- [BiSECT](https://huggingface.co/datasets/GEM/BiSECT)
- Summarization
- [CNN Daily Mail](https://huggingface.co/datasets/cnn_dailymail)
- [Gigaword](https://huggingface.co/datasets/gigaword)
- [MultiNews](https://huggingface.co/datasets/multi_news)
- [SamSum](https://huggingface.co/datasets/samsum)
- [Wiki-Lingua](https://huggingface.co/datasets/GEM/wiki_lingua)
- [XLSum](https://huggingface.co/datasets/GEM/xlsum)
- [XSum](https://huggingface.co/datasets/xsum)
- Topic Classification
- [AG News](https://huggingface.co/datasets/ag_news)
- [DBPedia](https://huggingface.co/datasets/dbpedia_14)
- [TNEWS](https://huggingface.co/datasets/clue)
- [TREC](https://huggingface.co/datasets/trec)
- [CSL](https://huggingface.co/datasets/clue)
- Translation
- [Flores-200](https://huggingface.co/datasets/Muennighoff/flores200)
- [Tatoeba](https://huggingface.co/datasets/Helsinki-NLP/tatoeba_mt)
- Word Sense disambiguation
- [WiC](https://huggingface.co/datasets/super_glue)
- [XL-WiC](https://huggingface.co/datasets/pasinit/xlwic)
#### Evaluation datasets (included in [xP3all](https://huggingface.co/datasets/bigscience/xP3all) except for NLI & HumanEval)
- Natural Language Inference (NLI)
- [ANLI](https://huggingface.co/datasets/anli)
- [CB](https://huggingface.co/datasets/super_glue)
- [RTE](https://huggingface.co/datasets/super_glue)
- [XNLI](https://huggingface.co/datasets/xnli)
- Coreference Resolution
- [Winogrande](https://huggingface.co/datasets/winogrande)
- [XWinograd](https://huggingface.co/datasets/Muennighoff/xwinograd)
- Program Synthesis
- [HumanEval](https://huggingface.co/datasets/openai_humaneval)
- Sentence Completion
- [COPA](https://huggingface.co/datasets/super_glue)
- [Story Cloze](https://huggingface.co/datasets/story_cloze)
- [XCOPA](https://huggingface.co/datasets/xcopa)
- [XStoryCloze](https://huggingface.co/datasets/Muennighoff/xstory_cloze)
## Additional Information
### Licensing Information
The dataset is released under Apache 2.0.
### Citation Information
```bibtex
@misc{muennighoff2022crosslingual,
title={Crosslingual Generalization through Multitask Finetuning},
author={Niklas Muennighoff and Thomas Wang and Lintang Sutawika and Adam Roberts and Stella Biderman and Teven Le Scao and M Saiful Bari and Sheng Shen and Zheng-Xin Yong and Hailey Schoelkopf and Xiangru Tang and Dragomir Radev and Alham Fikri Aji and Khalid Almubarak and Samuel Albanie and Zaid Alyafeai and Albert Webson and Edward Raff and Colin Raffel},
year={2022},
eprint={2211.01786},
archivePrefix={arXiv},
primaryClass={cs.CL}
}
```
### Contributions
Thanks to the contributors of [promptsource](https://github.com/bigscience-workshop/promptsource/graphs/contributors) for adding many prompts used in this dataset. | bigscience/xP3mt | [
"task_categories:other",
"annotations_creators:expert-generated",
"annotations_creators:crowdsourced",
"multilinguality:multilingual",
"size_categories:100M<n<1B",
"language:ak",
"language:ar",
"language:as",
"language:bm",
"language:bn",
"language:ca",
"language:code",
"language:en",
"language:es",
"language:eu",
"language:fon",
"language:fr",
"language:gu",
"language:hi",
"language:id",
"language:ig",
"language:ki",
"language:kn",
"language:lg",
"language:ln",
"language:ml",
"language:mr",
"language:ne",
"language:nso",
"language:ny",
"language:or",
"language:pa",
"language:pt",
"language:rn",
"language:rw",
"language:sn",
"language:st",
"language:sw",
"language:ta",
"language:te",
"language:tn",
"language:ts",
"language:tum",
"language:tw",
"language:ur",
"language:vi",
"language:wo",
"language:xh",
"language:yo",
"language:zh",
"language:zu",
"license:apache-2.0",
"arxiv:2211.01786",
"region:us"
] | 2022-09-28T11:36:00+00:00 | {"annotations_creators": ["expert-generated", "crowdsourced"], "language": ["ak", "ar", "as", "bm", "bn", "ca", "code", "en", "es", "eu", "fon", "fr", "gu", "hi", "id", "ig", "ki", "kn", "lg", "ln", "ml", "mr", "ne", "nso", "ny", "or", "pa", "pt", "rn", "rw", "sn", "st", "sw", "ta", "te", "tn", "ts", "tum", "tw", "ur", "vi", "wo", "xh", "yo", "zh", "zu"], "license": ["apache-2.0"], "multilinguality": ["multilingual"], "size_categories": ["100M<n<1B"], "task_categories": ["other"], "pretty_name": "xP3", "programming_language": ["C", "C++", "C#", "Go", "Java", "JavaScript", "Lua", "PHP", "Python", "Ruby", "Rust", "Scala", "TypeScript"]} | 2023-05-30T14:50:57+00:00 | [
"2211.01786"
] | [
"ak",
"ar",
"as",
"bm",
"bn",
"ca",
"code",
"en",
"es",
"eu",
"fon",
"fr",
"gu",
"hi",
"id",
"ig",
"ki",
"kn",
"lg",
"ln",
"ml",
"mr",
"ne",
"nso",
"ny",
"or",
"pa",
"pt",
"rn",
"rw",
"sn",
"st",
"sw",
"ta",
"te",
"tn",
"ts",
"tum",
"tw",
"ur",
"vi",
"wo",
"xh",
"yo",
"zh",
"zu"
] | TAGS
#task_categories-other #annotations_creators-expert-generated #annotations_creators-crowdsourced #multilinguality-multilingual #size_categories-100M<n<1B #language-Akan #language-Arabic #language-Assamese #language-Bambara #language-Bengali #language-Catalan #language-code #language-English #language-Spanish #language-Basque #language-Fon #language-French #language-Gujarati #language-Hindi #language-Indonesian #language-Igbo #language-Kikuyu #language-Kannada #language-Ganda #language-Lingala #language-Malayalam #language-Marathi #language-Nepali (macrolanguage) #language-Pedi #language-Nyanja #language-Oriya (macrolanguage) #language-Panjabi #language-Portuguese #language-Rundi #language-Kinyarwanda #language-Shona #language-Southern Sotho #language-Swahili (macrolanguage) #language-Tamil #language-Telugu #language-Tswana #language-Tsonga #language-Tumbuka #language-Twi #language-Urdu #language-Vietnamese #language-Wolof #language-Xhosa #language-Yoruba #language-Chinese #language-Zulu #license-apache-2.0 #arxiv-2211.01786 #region-us
| Dataset Card for xP3
====================
Table of Contents
-----------------
* Table of Contents
* Dataset Description
+ Dataset Summary
+ Supported Tasks and Leaderboards
+ Languages
* Dataset Structure
+ Data Instances
+ Data Fields
+ Data Splits
* Dataset Creation
+ Curation Rationale
+ Source Data
+ Annotations
* Additional Information
+ Licensing Information
+ Citation Information
+ Contributions
Dataset Description
-------------------
* Repository: URL
* Paper: Crosslingual Generalization through Multitask Finetuning
* Point of Contact: Niklas Muennighoff
### Dataset Summary
>
> xP3 (Crosslingual Public Pool of Prompts) is a collection of prompts & datasets across 46 of languages & 16 NLP tasks. It is used for the training of BLOOMZ and mT0, multilingual language models capable of following human instructions in dozens of languages zero-shot.
>
>
>
* Creation: The dataset can be recreated using instructions available here. We provide this version to save processing time and ease reproducibility.
* Languages: 46 (Can be extended by recreating with more splits)
* xP3 Dataset Family:
Dataset Structure
-----------------
### Data Instances
An example of "train" looks as follows:
### Data Fields
The data fields are the same among all splits:
* 'inputs': the natural language input fed to the model
* 'targets': the natural language target that the model has to generate
### Data Splits
The below table summarizes sizes per language (computed from the 'merged\_{lang}.jsonl' files). Due to languages like 'tw' only being single sentence translation samples from Flores, their byte percentage is significantly lower than their sample percentage. We machine-translated prompts for monolingual datasets, thus languages with only crosslingual datasets (e.g. Translation) do not have non-English prompts. Languages without non-English prompts are equivalent to xP3.
Dataset Creation
----------------
### Source Data
#### Training datasets
* Code Miscellaneous
+ CodeComplex
+ Docstring Corpus
+ GreatCode
+ State Changes
* Closed-book QA
+ Hotpot QA
+ Trivia QA
+ Web Questions
+ Wiki QA
* Extractive QA
+ Adversarial QA
+ CMRC2018
+ DRCD
+ DuoRC
+ MLQA
+ Quoref
+ ReCoRD
+ ROPES
+ SQuAD v2
+ xQuAD
+ TyDI QA
- Primary
- Goldp
* Multiple-Choice QA
+ ARC
+ C3
+ CoS-E
+ Cosmos
+ DREAM
+ MultiRC
+ OpenBookQA
+ PiQA
+ QUAIL
+ QuaRel
+ QuaRTz
+ QASC
+ RACE
+ SciQ
+ Social IQA
+ Wiki Hop
+ WiQA
* Paraphrase Identification
+ MRPC
+ PAWS
+ PAWS-X
+ QQP
* Program Synthesis
+ APPS
+ CodeContests
+ JupyterCodePairs
+ MBPP
+ NeuralCodeSearch
+ XLCoST
* Structure-to-text
+ Common Gen
+ Wiki Bio
* Sentiment
+ Amazon
+ App Reviews
+ IMDB
+ Rotten Tomatoes
+ Yelp
* Simplification
+ BiSECT
* Summarization
+ CNN Daily Mail
+ Gigaword
+ MultiNews
+ SamSum
+ Wiki-Lingua
+ XLSum
+ XSum
* Topic Classification
+ AG News
+ DBPedia
+ TNEWS
+ TREC
+ CSL
* Translation
+ Flores-200
+ Tatoeba
* Word Sense disambiguation
+ WiC
+ XL-WiC
#### Evaluation datasets (included in xP3all except for NLI & HumanEval)
* Natural Language Inference (NLI)
+ ANLI
+ CB
+ RTE
+ XNLI
* Coreference Resolution
+ Winogrande
+ XWinograd
* Program Synthesis
+ HumanEval
* Sentence Completion
+ COPA
+ Story Cloze
+ XCOPA
+ XStoryCloze
Additional Information
----------------------
### Licensing Information
The dataset is released under Apache 2.0.
### Contributions
Thanks to the contributors of promptsource for adding many prompts used in this dataset.
| [
"### Dataset Summary\n\n\n\n> \n> xP3 (Crosslingual Public Pool of Prompts) is a collection of prompts & datasets across 46 of languages & 16 NLP tasks. It is used for the training of BLOOMZ and mT0, multilingual language models capable of following human instructions in dozens of languages zero-shot.\n> \n> \n> \n\n\n* Creation: The dataset can be recreated using instructions available here. We provide this version to save processing time and ease reproducibility.\n* Languages: 46 (Can be extended by recreating with more splits)\n* xP3 Dataset Family:\n\n\n\nDataset Structure\n-----------------",
"### Data Instances\n\n\nAn example of \"train\" looks as follows:",
"### Data Fields\n\n\nThe data fields are the same among all splits:\n\n\n* 'inputs': the natural language input fed to the model\n* 'targets': the natural language target that the model has to generate",
"### Data Splits\n\n\nThe below table summarizes sizes per language (computed from the 'merged\\_{lang}.jsonl' files). Due to languages like 'tw' only being single sentence translation samples from Flores, their byte percentage is significantly lower than their sample percentage. We machine-translated prompts for monolingual datasets, thus languages with only crosslingual datasets (e.g. Translation) do not have non-English prompts. Languages without non-English prompts are equivalent to xP3.\n\n\n\nDataset Creation\n----------------",
"### Source Data",
"#### Training datasets\n\n\n* Code Miscellaneous\n\t+ CodeComplex\n\t+ Docstring Corpus\n\t+ GreatCode\n\t+ State Changes\n* Closed-book QA\n\t+ Hotpot QA\n\t+ Trivia QA\n\t+ Web Questions\n\t+ Wiki QA\n* Extractive QA\n\t+ Adversarial QA\n\t+ CMRC2018\n\t+ DRCD\n\t+ DuoRC\n\t+ MLQA\n\t+ Quoref\n\t+ ReCoRD\n\t+ ROPES\n\t+ SQuAD v2\n\t+ xQuAD\n\t+ TyDI QA\n\t\t- Primary\n\t\t- Goldp\n* Multiple-Choice QA\n\t+ ARC\n\t+ C3\n\t+ CoS-E\n\t+ Cosmos\n\t+ DREAM\n\t+ MultiRC\n\t+ OpenBookQA\n\t+ PiQA\n\t+ QUAIL\n\t+ QuaRel\n\t+ QuaRTz\n\t+ QASC\n\t+ RACE\n\t+ SciQ\n\t+ Social IQA\n\t+ Wiki Hop\n\t+ WiQA\n* Paraphrase Identification\n\t+ MRPC\n\t+ PAWS\n\t+ PAWS-X\n\t+ QQP\n* Program Synthesis\n\t+ APPS\n\t+ CodeContests\n\t+ JupyterCodePairs\n\t+ MBPP\n\t+ NeuralCodeSearch\n\t+ XLCoST\n* Structure-to-text\n\t+ Common Gen\n\t+ Wiki Bio\n* Sentiment\n\t+ Amazon\n\t+ App Reviews\n\t+ IMDB\n\t+ Rotten Tomatoes\n\t+ Yelp\n* Simplification\n\t+ BiSECT\n* Summarization\n\t+ CNN Daily Mail\n\t+ Gigaword\n\t+ MultiNews\n\t+ SamSum\n\t+ Wiki-Lingua\n\t+ XLSum\n\t+ XSum\n* Topic Classification\n\t+ AG News\n\t+ DBPedia\n\t+ TNEWS\n\t+ TREC\n\t+ CSL\n* Translation\n\t+ Flores-200\n\t+ Tatoeba\n* Word Sense disambiguation\n\t+ WiC\n\t+ XL-WiC",
"#### Evaluation datasets (included in xP3all except for NLI & HumanEval)\n\n\n* Natural Language Inference (NLI)\n\t+ ANLI\n\t+ CB\n\t+ RTE\n\t+ XNLI\n* Coreference Resolution\n\t+ Winogrande\n\t+ XWinograd\n* Program Synthesis\n\t+ HumanEval\n* Sentence Completion\n\t+ COPA\n\t+ Story Cloze\n\t+ XCOPA\n\t+ XStoryCloze\n\n\nAdditional Information\n----------------------",
"### Licensing Information\n\n\nThe dataset is released under Apache 2.0.",
"### Contributions\n\n\nThanks to the contributors of promptsource for adding many prompts used in this dataset."
] | [
"TAGS\n#task_categories-other #annotations_creators-expert-generated #annotations_creators-crowdsourced #multilinguality-multilingual #size_categories-100M<n<1B #language-Akan #language-Arabic #language-Assamese #language-Bambara #language-Bengali #language-Catalan #language-code #language-English #language-Spanish #language-Basque #language-Fon #language-French #language-Gujarati #language-Hindi #language-Indonesian #language-Igbo #language-Kikuyu #language-Kannada #language-Ganda #language-Lingala #language-Malayalam #language-Marathi #language-Nepali (macrolanguage) #language-Pedi #language-Nyanja #language-Oriya (macrolanguage) #language-Panjabi #language-Portuguese #language-Rundi #language-Kinyarwanda #language-Shona #language-Southern Sotho #language-Swahili (macrolanguage) #language-Tamil #language-Telugu #language-Tswana #language-Tsonga #language-Tumbuka #language-Twi #language-Urdu #language-Vietnamese #language-Wolof #language-Xhosa #language-Yoruba #language-Chinese #language-Zulu #license-apache-2.0 #arxiv-2211.01786 #region-us \n",
"### Dataset Summary\n\n\n\n> \n> xP3 (Crosslingual Public Pool of Prompts) is a collection of prompts & datasets across 46 of languages & 16 NLP tasks. It is used for the training of BLOOMZ and mT0, multilingual language models capable of following human instructions in dozens of languages zero-shot.\n> \n> \n> \n\n\n* Creation: The dataset can be recreated using instructions available here. We provide this version to save processing time and ease reproducibility.\n* Languages: 46 (Can be extended by recreating with more splits)\n* xP3 Dataset Family:\n\n\n\nDataset Structure\n-----------------",
"### Data Instances\n\n\nAn example of \"train\" looks as follows:",
"### Data Fields\n\n\nThe data fields are the same among all splits:\n\n\n* 'inputs': the natural language input fed to the model\n* 'targets': the natural language target that the model has to generate",
"### Data Splits\n\n\nThe below table summarizes sizes per language (computed from the 'merged\\_{lang}.jsonl' files). Due to languages like 'tw' only being single sentence translation samples from Flores, their byte percentage is significantly lower than their sample percentage. We machine-translated prompts for monolingual datasets, thus languages with only crosslingual datasets (e.g. Translation) do not have non-English prompts. Languages without non-English prompts are equivalent to xP3.\n\n\n\nDataset Creation\n----------------",
"### Source Data",
"#### Training datasets\n\n\n* Code Miscellaneous\n\t+ CodeComplex\n\t+ Docstring Corpus\n\t+ GreatCode\n\t+ State Changes\n* Closed-book QA\n\t+ Hotpot QA\n\t+ Trivia QA\n\t+ Web Questions\n\t+ Wiki QA\n* Extractive QA\n\t+ Adversarial QA\n\t+ CMRC2018\n\t+ DRCD\n\t+ DuoRC\n\t+ MLQA\n\t+ Quoref\n\t+ ReCoRD\n\t+ ROPES\n\t+ SQuAD v2\n\t+ xQuAD\n\t+ TyDI QA\n\t\t- Primary\n\t\t- Goldp\n* Multiple-Choice QA\n\t+ ARC\n\t+ C3\n\t+ CoS-E\n\t+ Cosmos\n\t+ DREAM\n\t+ MultiRC\n\t+ OpenBookQA\n\t+ PiQA\n\t+ QUAIL\n\t+ QuaRel\n\t+ QuaRTz\n\t+ QASC\n\t+ RACE\n\t+ SciQ\n\t+ Social IQA\n\t+ Wiki Hop\n\t+ WiQA\n* Paraphrase Identification\n\t+ MRPC\n\t+ PAWS\n\t+ PAWS-X\n\t+ QQP\n* Program Synthesis\n\t+ APPS\n\t+ CodeContests\n\t+ JupyterCodePairs\n\t+ MBPP\n\t+ NeuralCodeSearch\n\t+ XLCoST\n* Structure-to-text\n\t+ Common Gen\n\t+ Wiki Bio\n* Sentiment\n\t+ Amazon\n\t+ App Reviews\n\t+ IMDB\n\t+ Rotten Tomatoes\n\t+ Yelp\n* Simplification\n\t+ BiSECT\n* Summarization\n\t+ CNN Daily Mail\n\t+ Gigaword\n\t+ MultiNews\n\t+ SamSum\n\t+ Wiki-Lingua\n\t+ XLSum\n\t+ XSum\n* Topic Classification\n\t+ AG News\n\t+ DBPedia\n\t+ TNEWS\n\t+ TREC\n\t+ CSL\n* Translation\n\t+ Flores-200\n\t+ Tatoeba\n* Word Sense disambiguation\n\t+ WiC\n\t+ XL-WiC",
"#### Evaluation datasets (included in xP3all except for NLI & HumanEval)\n\n\n* Natural Language Inference (NLI)\n\t+ ANLI\n\t+ CB\n\t+ RTE\n\t+ XNLI\n* Coreference Resolution\n\t+ Winogrande\n\t+ XWinograd\n* Program Synthesis\n\t+ HumanEval\n* Sentence Completion\n\t+ COPA\n\t+ Story Cloze\n\t+ XCOPA\n\t+ XStoryCloze\n\n\nAdditional Information\n----------------------",
"### Licensing Information\n\n\nThe dataset is released under Apache 2.0.",
"### Contributions\n\n\nThanks to the contributors of promptsource for adding many prompts used in this dataset."
] | [
338,
149,
18,
49,
132,
4,
351,
98,
16,
24
] | [
"passage: TAGS\n#task_categories-other #annotations_creators-expert-generated #annotations_creators-crowdsourced #multilinguality-multilingual #size_categories-100M<n<1B #language-Akan #language-Arabic #language-Assamese #language-Bambara #language-Bengali #language-Catalan #language-code #language-English #language-Spanish #language-Basque #language-Fon #language-French #language-Gujarati #language-Hindi #language-Indonesian #language-Igbo #language-Kikuyu #language-Kannada #language-Ganda #language-Lingala #language-Malayalam #language-Marathi #language-Nepali (macrolanguage) #language-Pedi #language-Nyanja #language-Oriya (macrolanguage) #language-Panjabi #language-Portuguese #language-Rundi #language-Kinyarwanda #language-Shona #language-Southern Sotho #language-Swahili (macrolanguage) #language-Tamil #language-Telugu #language-Tswana #language-Tsonga #language-Tumbuka #language-Twi #language-Urdu #language-Vietnamese #language-Wolof #language-Xhosa #language-Yoruba #language-Chinese #language-Zulu #license-apache-2.0 #arxiv-2211.01786 #region-us \n### Dataset Summary\n\n\n\n> \n> xP3 (Crosslingual Public Pool of Prompts) is a collection of prompts & datasets across 46 of languages & 16 NLP tasks. It is used for the training of BLOOMZ and mT0, multilingual language models capable of following human instructions in dozens of languages zero-shot.\n> \n> \n> \n\n\n* Creation: The dataset can be recreated using instructions available here. We provide this version to save processing time and ease reproducibility.\n* Languages: 46 (Can be extended by recreating with more splits)\n* xP3 Dataset Family:\n\n\n\nDataset Structure\n-----------------### Data Instances\n\n\nAn example of \"train\" looks as follows:",
"passage: ### Data Fields\n\n\nThe data fields are the same among all splits:\n\n\n* 'inputs': the natural language input fed to the model\n* 'targets': the natural language target that the model has to generate### Data Splits\n\n\nThe below table summarizes sizes per language (computed from the 'merged\\_{lang}.jsonl' files). Due to languages like 'tw' only being single sentence translation samples from Flores, their byte percentage is significantly lower than their sample percentage. We machine-translated prompts for monolingual datasets, thus languages with only crosslingual datasets (e.g. Translation) do not have non-English prompts. Languages without non-English prompts are equivalent to xP3.\n\n\n\nDataset Creation\n----------------### Source Data#### Training datasets\n\n\n* Code Miscellaneous\n\t+ CodeComplex\n\t+ Docstring Corpus\n\t+ GreatCode\n\t+ State Changes\n* Closed-book QA\n\t+ Hotpot QA\n\t+ Trivia QA\n\t+ Web Questions\n\t+ Wiki QA\n* Extractive QA\n\t+ Adversarial QA\n\t+ CMRC2018\n\t+ DRCD\n\t+ DuoRC\n\t+ MLQA\n\t+ Quoref\n\t+ ReCoRD\n\t+ ROPES\n\t+ SQuAD v2\n\t+ xQuAD\n\t+ TyDI QA\n\t\t- Primary\n\t\t- Goldp\n* Multiple-Choice QA\n\t+ ARC\n\t+ C3\n\t+ CoS-E\n\t+ Cosmos\n\t+ DREAM\n\t+ MultiRC\n\t+ OpenBookQA\n\t+ PiQA\n\t+ QUAIL\n\t+ QuaRel\n\t+ QuaRTz\n\t+ QASC\n\t+ RACE\n\t+ SciQ\n\t+ Social IQA\n\t+ Wiki Hop\n\t+ WiQA\n* Paraphrase Identification\n\t+ MRPC\n\t+ PAWS\n\t+ PAWS-X\n\t+ QQP\n* Program Synthesis\n\t+ APPS\n\t+ CodeContests\n\t+ JupyterCodePairs\n\t+ MBPP\n\t+ NeuralCodeSearch\n\t+ XLCoST\n* Structure-to-text\n\t+ Common Gen\n\t+ Wiki Bio\n* Sentiment\n\t+ Amazon\n\t+ App Reviews\n\t+ IMDB\n\t+ Rotten Tomatoes\n\t+ Yelp\n* Simplification\n\t+ BiSECT\n* Summarization\n\t+ CNN Daily Mail\n\t+ Gigaword\n\t+ MultiNews\n\t+ SamSum\n\t+ Wiki-Lingua\n\t+ XLSum\n\t+ XSum\n* Topic Classification\n\t+ AG News\n\t+ DBPedia\n\t+ TNEWS\n\t+ TREC\n\t+ CSL\n* Translation\n\t+ Flores-200\n\t+ Tatoeba\n* Word Sense disambiguation\n\t+ WiC\n\t+ XL-WiC"
] |
cc06d31cd266a978219b212ba00e72eb0ad14d4c | a | CANUTO/images | [
"region:us"
] | 2022-09-28T14:54:45+00:00 | {} | 2022-09-28T15:00:43+00:00 | [] | [] | TAGS
#region-us
| a | [] | [
"TAGS\n#region-us \n"
] | [
6
] | [
"passage: TAGS\n#region-us \n"
] |
e91596d78fb16f41a5b993e2db7d4345bca01d77 | #Training IA Model
Here are the images that i used to train an a SD model with "tiomonkey" concept | EltioMonkey/MonkeyTrain | [
"region:us"
] | 2022-09-28T17:52:43+00:00 | {} | 2022-09-29T16:44:43+00:00 | [] | [] | TAGS
#region-us
| #Training IA Model
Here are the images that i used to train an a SD model with "tiomonkey" concept | [] | [
"TAGS\n#region-us \n"
] | [
6
] | [
"passage: TAGS\n#region-us \n"
] |
d23b094346c5dbda1080a74bb2a24c18adbf7409 |
# Dataset Card for MultiPL-E
## Dataset Description
- **Homepage:** https://nuprl.github.io/MultiPL-E/
- **Repository:** https://github.com/nuprl/MultiPL-E
- **Paper:** https://ieeexplore.ieee.org/abstract/document/10103177
- **Point of Contact:** [email protected], [email protected], [email protected]
## Dataset Summary
MultiPL-E is a dataset for evaluating large language models for code
generation that supports 18 programming languages. It takes the OpenAI
"HumanEval" and the MBPP Python benchmarks and uses little compilers to
translate them to other languages. It is easy to add support for new languages
and benchmarks.
## Subsets
For most purposes, you should use the variations called *SRCDATA-LANG*, where
*SRCDATA* is either "humaneval" or "mbpp" and *LANG* is one of the supported
languages. We use the canonical file extension for each language to identify
the language, e.g., "py" for Python, "cpp" for C++, "lua" for Lua, and so on.
We also provide a few other variations:
- *SRCDATA-LANG-keep* is the same as *SRCDATA-LANG*, but the text of the prompt
is totally unchanged. If the original prompt had Python doctests, they remain
as Python instead of being translated to *LANG*. If the original prompt had
Python-specific terminology, e.g., "list", it remains "list", instead of
being translated, e.g., to "vector" for C++.
- *SRCDATA-LANG-transform* transforms the doctests to *LANG* but leaves
the natural language text of the prompt unchanged.
- *SRCDATA-LANG-removed* removes the doctests from the prompt.
Note that MBPP does not have any doctests, so the "removed" and "transform"
variations are not available for MBPP.
## Example
The following script uses the Salesforce/codegen model to generate Lua
and MultiPL-E to produce a script with unit tests for luaunit.
```python
import datasets
from transformers import AutoTokenizer, AutoModelForCausalLM
LANG = "lua"
MODEL_NAME = "Salesforce/codegen-350M-multi"
tokenizer = AutoTokenizer.from_pretrained(MODEL_NAME)
model = AutoModelForCausalLM.from_pretrained(MODEL_NAME).half().cuda()
problems = datasets.load_dataset("nuprl/MultiPL-E", f"humaneval-{LANG}")
def stop_at_stop_token(decoded_string, problem):
"""
Truncates the output at stop tokens, taking care to skip the prompt
which may have stop tokens.
"""
min_stop_index = len(decoded_string)
for stop_token in problem["stop_tokens"]:
stop_index = decoded_string.find(stop_token)
if stop_index != -1 and stop_index > len(problem["prompt"]) and stop_index < min_stop_index:
min_stop_index = stop_index
return decoded_string[:min_stop_index]
for problem in problems["test"]:
input_ids = tokenizer(
problem["prompt"],
return_tensors="pt",
).input_ids.cuda()
generated_ids = model.generate(
input_ids, max_length=512, pad_token_id=tokenizer.eos_token_id + 2
)
truncated_string = stop_at_stop_token(tokenizer.decode(generated_ids[0]), problem)
filename = problem["name"] + "." + LANG
with open(filename, "w") as f:
print(f"Created {filename}")
f.write(truncated_string)
f.write("\n")
f.write(problem["tests"])
``` | nuprl/MultiPL-E | [
"annotations_creators:machine-generated",
"language_creators:machine-generated",
"language_creators:expert-generated",
"multilinguality:monolingual",
"size_categories:1K<n<10K",
"source_datasets:original",
"source_datasets:extended|openai_humaneval",
"source_datasets:extended|mbpp",
"language:en",
"license:mit",
"region:us"
] | 2022-09-28T18:20:07+00:00 | {"annotations_creators": ["machine-generated"], "language_creators": ["machine-generated", "expert-generated"], "language": ["en"], "license": ["mit"], "multilinguality": ["monolingual"], "size_categories": ["1K<n<10K"], "source_datasets": ["original", "extended|openai_humaneval", "extended|mbpp"], "task_categories": [], "task_ids": [], "pretty_name": "MultiPLE-E", "tags": [], "dataset_info": [{"config_name": "cpp-keep", "features": [{"name": "name", "dtype": "string"}, {"name": "language", "dtype": "string"}, {"name": "prompt", "dtype": "string"}, {"name": "doctests", "dtype": "string"}, {"name": "original", "dtype": "string"}, {"name": "prompt_terminology", "dtype": "string"}, {"name": "tests", "dtype": "string"}, {"name": "stop_tokens", "sequence": "string"}], "splits": [{"name": "test", "num_bytes": 217792, "num_examples": 161}], "download_size": 248493, "dataset_size": 217792}, {"config_name": "cpp-transform", "features": [{"name": "name", "dtype": "string"}, {"name": "language", "dtype": "string"}, {"name": "prompt", "dtype": "string"}, {"name": "doctests", "dtype": "string"}, {"name": "original", "dtype": "string"}, {"name": "prompt_terminology", "dtype": "string"}, {"name": "tests", "dtype": "string"}, {"name": "stop_tokens", "sequence": "string"}], "splits": [{"name": "test", "num_bytes": 239517, "num_examples": 161}], "download_size": 270773, "dataset_size": 239517}, {"config_name": "cpp-reworded", "features": [{"name": "name", "dtype": "string"}, {"name": "language", "dtype": "string"}, {"name": "prompt", "dtype": "string"}, {"name": "doctests", "dtype": "string"}, {"name": "original", "dtype": "string"}, {"name": "prompt_terminology", "dtype": "string"}, {"name": "tests", "dtype": "string"}, {"name": "stop_tokens", "sequence": "string"}], "splits": [{"name": "test", "num_bytes": 239767, "num_examples": 161}], "download_size": 271023, "dataset_size": 239767}, {"config_name": "cpp-remove", "features": [{"name": "name", "dtype": "string"}, {"name": "language", "dtype": "string"}, {"name": "prompt", "dtype": "string"}, {"name": "doctests", "dtype": "string"}, {"name": "original", "dtype": "string"}, {"name": "prompt_terminology", "dtype": "string"}, {"name": "tests", "dtype": "string"}, {"name": "stop_tokens", "sequence": "string"}], "splits": [{"name": "test", "num_bytes": 198566, "num_examples": 158}], "download_size": 227555, "dataset_size": 198566}, {"config_name": "cs-keep", "features": [{"name": "name", "dtype": "string"}, {"name": "language", "dtype": "string"}, {"name": "prompt", "dtype": "string"}, {"name": "doctests", "dtype": "string"}, {"name": "original", "dtype": "string"}, {"name": "prompt_terminology", "dtype": "string"}, {"name": "tests", "dtype": "string"}, {"name": "stop_tokens", "sequence": "string"}], "splits": [{"name": "test", "num_bytes": 259874, "num_examples": 158}], "download_size": 291137, "dataset_size": 259874}, {"config_name": "cs-transform", "features": [{"name": "name", "dtype": "string"}, {"name": "language", "dtype": "string"}, {"name": "prompt", "dtype": "string"}, {"name": "doctests", "dtype": "string"}, {"name": "original", "dtype": "string"}, {"name": "prompt_terminology", "dtype": "string"}, {"name": "tests", "dtype": "string"}, {"name": "stop_tokens", "sequence": "string"}], "splits": [{"name": "test", "num_bytes": 283738, "num_examples": 158}], "download_size": 315563, "dataset_size": 283738}, {"config_name": "cs-reworded", "features": [{"name": "name", "dtype": "string"}, {"name": "language", "dtype": "string"}, {"name": "prompt", "dtype": "string"}, {"name": "doctests", "dtype": "string"}, {"name": "original", "dtype": "string"}, {"name": "prompt_terminology", "dtype": "string"}, {"name": "tests", "dtype": "string"}, {"name": "stop_tokens", "sequence": "string"}], "splits": [{"name": "test", "num_bytes": 283673, "num_examples": 158}], "download_size": 315498, "dataset_size": 283673}, {"config_name": "cs-remove", "features": [{"name": "name", "dtype": "string"}, {"name": "language", "dtype": "string"}, {"name": "prompt", "dtype": "string"}, {"name": "doctests", "dtype": "string"}, {"name": "original", "dtype": "string"}, {"name": "prompt_terminology", "dtype": "string"}, {"name": "tests", "dtype": "string"}, {"name": "stop_tokens", "sequence": "string"}], "splits": [{"name": "test", "num_bytes": 237663, "num_examples": 155}], "download_size": 267251, "dataset_size": 237663}, {"config_name": "d-keep", "features": [{"name": "name", "dtype": "string"}, {"name": "language", "dtype": "string"}, {"name": "prompt", "dtype": "string"}, {"name": "doctests", "dtype": "string"}, {"name": "original", "dtype": "string"}, {"name": "prompt_terminology", "dtype": "string"}, {"name": "tests", "dtype": "string"}, {"name": "stop_tokens", "sequence": "string"}], "splits": [{"name": "test", "num_bytes": 175592, "num_examples": 156}], "download_size": 209568, "dataset_size": 175592}, {"config_name": "d-transform", "features": [{"name": "name", "dtype": "string"}, {"name": "language", "dtype": "string"}, {"name": "prompt", "dtype": "string"}, {"name": "doctests", "dtype": "string"}, {"name": "original", "dtype": "string"}, {"name": "prompt_terminology", "dtype": "string"}, {"name": "tests", "dtype": "string"}, {"name": "stop_tokens", "sequence": "string"}], "splits": [{"name": "test", "num_bytes": 181121, "num_examples": 156}], "download_size": 215649, "dataset_size": 181121}, {"config_name": "d-reworded", "features": [{"name": "name", "dtype": "string"}, {"name": "language", "dtype": "string"}, {"name": "prompt", "dtype": "string"}, {"name": "doctests", "dtype": "string"}, {"name": "original", "dtype": "string"}, {"name": "prompt_terminology", "dtype": "string"}, {"name": "tests", "dtype": "string"}, {"name": "stop_tokens", "sequence": "string"}], "splits": [{"name": "test", "num_bytes": 181296, "num_examples": 156}], "download_size": 215824, "dataset_size": 181296}, {"config_name": "d-remove", "features": [{"name": "name", "dtype": "string"}, {"name": "language", "dtype": "string"}, {"name": "prompt", "dtype": "string"}, {"name": "doctests", "dtype": "string"}, {"name": "original", "dtype": "string"}, {"name": "prompt_terminology", "dtype": "string"}, {"name": "tests", "dtype": "string"}, {"name": "stop_tokens", "sequence": "string"}], "splits": [{"name": "test", "num_bytes": 157938, "num_examples": 153}], "download_size": 190211, "dataset_size": 157938}, {"config_name": "go-keep", "features": [{"name": "name", "dtype": "string"}, {"name": "language", "dtype": "string"}, {"name": "prompt", "dtype": "string"}, {"name": "doctests", "dtype": "string"}, {"name": "original", "dtype": "string"}, {"name": "prompt_terminology", "dtype": "string"}, {"name": "tests", "dtype": "string"}, {"name": "stop_tokens", "sequence": "string"}], "splits": [{"name": "test", "num_bytes": 241130, "num_examples": 154}], "download_size": 280424, "dataset_size": 241130}, {"config_name": "go-transform", "features": [{"name": "name", "dtype": "string"}, {"name": "language", "dtype": "string"}, {"name": "prompt", "dtype": "string"}, {"name": "doctests", "dtype": "string"}, {"name": "original", "dtype": "string"}, {"name": "prompt_terminology", "dtype": "string"}, {"name": "tests", "dtype": "string"}, {"name": "stop_tokens", "sequence": "string"}], "splits": [{"name": "test", "num_bytes": 247448, "num_examples": 154}], "download_size": 287275, "dataset_size": 247448}, {"config_name": "go-reworded", "features": [{"name": "name", "dtype": "string"}, {"name": "language", "dtype": "string"}, {"name": "prompt", "dtype": "string"}, {"name": "doctests", "dtype": "string"}, {"name": "original", "dtype": "string"}, {"name": "prompt_terminology", "dtype": "string"}, {"name": "tests", "dtype": "string"}, {"name": "stop_tokens", "sequence": "string"}], "splits": [{"name": "test", "num_bytes": 247354, "num_examples": 154}], "download_size": 287181, "dataset_size": 247354}, {"config_name": "go-remove", "features": [{"name": "name", "dtype": "string"}, {"name": "language", "dtype": "string"}, {"name": "prompt", "dtype": "string"}, {"name": "doctests", "dtype": "string"}, {"name": "original", "dtype": "string"}, {"name": "prompt_terminology", "dtype": "string"}, {"name": "tests", "dtype": "string"}, {"name": "stop_tokens", "sequence": "string"}], "splits": [{"name": "test", "num_bytes": 221519, "num_examples": 151}], "download_size": 258980, "dataset_size": 221519}, {"config_name": "java-keep", "features": [{"name": "name", "dtype": "string"}, {"name": "language", "dtype": "string"}, {"name": "prompt", "dtype": "string"}, {"name": "doctests", "dtype": "string"}, {"name": "original", "dtype": "string"}, {"name": "prompt_terminology", "dtype": "string"}, {"name": "tests", "dtype": "string"}, {"name": "stop_tokens", "sequence": "string"}], "splits": [{"name": "test", "num_bytes": 259836, "num_examples": 158}], "download_size": 291099, "dataset_size": 259836}, {"config_name": "java-transform", "features": [{"name": "name", "dtype": "string"}, {"name": "language", "dtype": "string"}, {"name": "prompt", "dtype": "string"}, {"name": "doctests", "dtype": "string"}, {"name": "original", "dtype": "string"}, {"name": "prompt_terminology", "dtype": "string"}, {"name": "tests", "dtype": "string"}, {"name": "stop_tokens", "sequence": "string"}], "splits": [{"name": "test", "num_bytes": 286548, "num_examples": 158}], "download_size": 318373, "dataset_size": 286548}, {"config_name": "java-reworded", "features": [{"name": "name", "dtype": "string"}, {"name": "language", "dtype": "string"}, {"name": "prompt", "dtype": "string"}, {"name": "doctests", "dtype": "string"}, {"name": "original", "dtype": "string"}, {"name": "prompt_terminology", "dtype": "string"}, {"name": "tests", "dtype": "string"}, {"name": "stop_tokens", "sequence": "string"}], "splits": [{"name": "test", "num_bytes": 288031, "num_examples": 158}], "download_size": 319856, "dataset_size": 288031}, {"config_name": "java-remove", "features": [{"name": "name", "dtype": "string"}, {"name": "language", "dtype": "string"}, {"name": "prompt", "dtype": "string"}, {"name": "doctests", "dtype": "string"}, {"name": "original", "dtype": "string"}, {"name": "prompt_terminology", "dtype": "string"}, {"name": "tests", "dtype": "string"}, {"name": "stop_tokens", "sequence": "string"}], "splits": [{"name": "test", "num_bytes": 237672, "num_examples": 155}], "download_size": 267260, "dataset_size": 237672}, {"config_name": "jl-keep", "features": [{"name": "name", "dtype": "string"}, {"name": "language", "dtype": "string"}, {"name": "prompt", "dtype": "string"}, {"name": "doctests", "dtype": "string"}, {"name": "original", "dtype": "string"}, {"name": "prompt_terminology", "dtype": "string"}, {"name": "tests", "dtype": "string"}, {"name": "stop_tokens", "sequence": "string"}], "splits": [{"name": "test", "num_bytes": 163708, "num_examples": 159}], "download_size": 198696, "dataset_size": 163708}, {"config_name": "jl-transform", "features": [{"name": "name", "dtype": "string"}, {"name": "language", "dtype": "string"}, {"name": "prompt", "dtype": "string"}, {"name": "doctests", "dtype": "string"}, {"name": "original", "dtype": "string"}, {"name": "prompt_terminology", "dtype": "string"}, {"name": "tests", "dtype": "string"}, {"name": "stop_tokens", "sequence": "string"}], "splits": [{"name": "test", "num_bytes": 167969, "num_examples": 159}], "download_size": 203514, "dataset_size": 167969}, {"config_name": "jl-reworded", "features": [{"name": "name", "dtype": "string"}, {"name": "language", "dtype": "string"}, {"name": "prompt", "dtype": "string"}, {"name": "doctests", "dtype": "string"}, {"name": "original", "dtype": "string"}, {"name": "prompt_terminology", "dtype": "string"}, {"name": "tests", "dtype": "string"}, {"name": "stop_tokens", "sequence": "string"}], "splits": [{"name": "test", "num_bytes": 168251, "num_examples": 159}], "download_size": 203796, "dataset_size": 168251}, {"config_name": "jl-remove", "features": [{"name": "name", "dtype": "string"}, {"name": "language", "dtype": "string"}, {"name": "prompt", "dtype": "string"}, {"name": "doctests", "dtype": "string"}, {"name": "original", "dtype": "string"}, {"name": "prompt_terminology", "dtype": "string"}, {"name": "tests", "dtype": "string"}, {"name": "stop_tokens", "sequence": "string"}], "splits": [{"name": "test", "num_bytes": 145913, "num_examples": 156}], "download_size": 179158, "dataset_size": 145913}, {"config_name": "js-keep", "features": [{"name": "name", "dtype": "string"}, {"name": "language", "dtype": "string"}, {"name": "prompt", "dtype": "string"}, {"name": "doctests", "dtype": "string"}, {"name": "original", "dtype": "string"}, {"name": "prompt_terminology", "dtype": "string"}, {"name": "tests", "dtype": "string"}, {"name": "stop_tokens", "sequence": "string"}], "splits": [{"name": "test", "num_bytes": 177635, "num_examples": 161}], "download_size": 211822, "dataset_size": 177635}, {"config_name": "js-transform", "features": [{"name": "name", "dtype": "string"}, {"name": "language", "dtype": "string"}, {"name": "prompt", "dtype": "string"}, {"name": "doctests", "dtype": "string"}, {"name": "original", "dtype": "string"}, {"name": "prompt_terminology", "dtype": "string"}, {"name": "tests", "dtype": "string"}, {"name": "stop_tokens", "sequence": "string"}], "splits": [{"name": "test", "num_bytes": 181987, "num_examples": 161}], "download_size": 216729, "dataset_size": 181987}, {"config_name": "js-reworded", "features": [{"name": "name", "dtype": "string"}, {"name": "language", "dtype": "string"}, {"name": "prompt", "dtype": "string"}, {"name": "doctests", "dtype": "string"}, {"name": "original", "dtype": "string"}, {"name": "prompt_terminology", "dtype": "string"}, {"name": "tests", "dtype": "string"}, {"name": "stop_tokens", "sequence": "string"}], "splits": [{"name": "test", "num_bytes": 182171, "num_examples": 161}], "download_size": 216913, "dataset_size": 182171}, {"config_name": "js-remove", "features": [{"name": "name", "dtype": "string"}, {"name": "language", "dtype": "string"}, {"name": "prompt", "dtype": "string"}, {"name": "doctests", "dtype": "string"}, {"name": "original", "dtype": "string"}, {"name": "prompt_terminology", "dtype": "string"}, {"name": "tests", "dtype": "string"}, {"name": "stop_tokens", "sequence": "string"}], "splits": [{"name": "test", "num_bytes": 158619, "num_examples": 158}], "download_size": 191028, "dataset_size": 158619}, {"config_name": "lua-keep", "features": [{"name": "name", "dtype": "string"}, {"name": "language", "dtype": "string"}, {"name": "prompt", "dtype": "string"}, {"name": "doctests", "dtype": "string"}, {"name": "original", "dtype": "string"}, {"name": "prompt_terminology", "dtype": "string"}, {"name": "tests", "dtype": "string"}, {"name": "stop_tokens", "sequence": "string"}], "splits": [{"name": "test", "num_bytes": 180398, "num_examples": 161}], "download_size": 212511, "dataset_size": 180398}, {"config_name": "lua-transform", "features": [{"name": "name", "dtype": "string"}, {"name": "language", "dtype": "string"}, {"name": "prompt", "dtype": "string"}, {"name": "doctests", "dtype": "string"}, {"name": "original", "dtype": "string"}, {"name": "prompt_terminology", "dtype": "string"}, {"name": "tests", "dtype": "string"}, {"name": "stop_tokens", "sequence": "string"}], "splits": [{"name": "test", "num_bytes": 184763, "num_examples": 161}], "download_size": 216595, "dataset_size": 184763}, {"config_name": "lua-reworded", "features": [{"name": "name", "dtype": "string"}, {"name": "language", "dtype": "string"}, {"name": "prompt", "dtype": "string"}, {"name": "doctests", "dtype": "string"}, {"name": "original", "dtype": "string"}, {"name": "prompt_terminology", "dtype": "string"}, {"name": "tests", "dtype": "string"}, {"name": "stop_tokens", "sequence": "string"}], "splits": [{"name": "test", "num_bytes": 184853, "num_examples": 161}], "download_size": 216685, "dataset_size": 184853}, {"config_name": "lua-remove", "features": [{"name": "name", "dtype": "string"}, {"name": "language", "dtype": "string"}, {"name": "prompt", "dtype": "string"}, {"name": "doctests", "dtype": "string"}, {"name": "original", "dtype": "string"}, {"name": "prompt_terminology", "dtype": "string"}, {"name": "tests", "dtype": "string"}, {"name": "stop_tokens", "sequence": "string"}], "splits": [{"name": "test", "num_bytes": 161339, "num_examples": 158}], "download_size": 191690, "dataset_size": 161339}, {"config_name": "php-keep", "features": [{"name": "name", "dtype": "string"}, {"name": "language", "dtype": "string"}, {"name": "prompt", "dtype": "string"}, {"name": "doctests", "dtype": "string"}, {"name": "original", "dtype": "string"}, {"name": "prompt_terminology", "dtype": "string"}, {"name": "tests", "dtype": "string"}, {"name": "stop_tokens", "sequence": "string"}], "splits": [{"name": "test", "num_bytes": 219526, "num_examples": 161}], "download_size": 256134, "dataset_size": 219526}, {"config_name": "php-transform", "features": [{"name": "name", "dtype": "string"}, {"name": "language", "dtype": "string"}, {"name": "prompt", "dtype": "string"}, {"name": "doctests", "dtype": "string"}, {"name": "original", "dtype": "string"}, {"name": "prompt_terminology", "dtype": "string"}, {"name": "tests", "dtype": "string"}, {"name": "stop_tokens", "sequence": "string"}], "splits": [{"name": "test", "num_bytes": 225575, "num_examples": 161}], "download_size": 262738, "dataset_size": 225575}, {"config_name": "php-reworded", "features": [{"name": "name", "dtype": "string"}, {"name": "language", "dtype": "string"}, {"name": "prompt", "dtype": "string"}, {"name": "doctests", "dtype": "string"}, {"name": "original", "dtype": "string"}, {"name": "prompt_terminology", "dtype": "string"}, {"name": "tests", "dtype": "string"}, {"name": "stop_tokens", "sequence": "string"}], "splits": [{"name": "test", "num_bytes": 225730, "num_examples": 161}], "download_size": 262893, "dataset_size": 225730}, {"config_name": "php-remove", "features": [{"name": "name", "dtype": "string"}, {"name": "language", "dtype": "string"}, {"name": "prompt", "dtype": "string"}, {"name": "doctests", "dtype": "string"}, {"name": "original", "dtype": "string"}, {"name": "prompt_terminology", "dtype": "string"}, {"name": "tests", "dtype": "string"}, {"name": "stop_tokens", "sequence": "string"}], "splits": [{"name": "test", "num_bytes": 200047, "num_examples": 158}], "download_size": 234848, "dataset_size": 200047}, {"config_name": "pl-keep", "features": [{"name": "name", "dtype": "string"}, {"name": "language", "dtype": "string"}, {"name": "prompt", "dtype": "string"}, {"name": "doctests", "dtype": "string"}, {"name": "original", "dtype": "string"}, {"name": "prompt_terminology", "dtype": "string"}, {"name": "tests", "dtype": "string"}, {"name": "stop_tokens", "sequence": "string"}], "splits": [{"name": "test", "num_bytes": 239874, "num_examples": 161}], "download_size": 279351, "dataset_size": 239874}, {"config_name": "pl-transform", "features": [{"name": "name", "dtype": "string"}, {"name": "language", "dtype": "string"}, {"name": "prompt", "dtype": "string"}, {"name": "doctests", "dtype": "string"}, {"name": "original", "dtype": "string"}, {"name": "prompt_terminology", "dtype": "string"}, {"name": "tests", "dtype": "string"}, {"name": "stop_tokens", "sequence": "string"}], "splits": [{"name": "test", "num_bytes": 243611, "num_examples": 161}], "download_size": 283767, "dataset_size": 243611}, {"config_name": "pl-reworded", "features": [{"name": "name", "dtype": "string"}, {"name": "language", "dtype": "string"}, {"name": "prompt", "dtype": "string"}, {"name": "doctests", "dtype": "string"}, {"name": "original", "dtype": "string"}, {"name": "prompt_terminology", "dtype": "string"}, {"name": "tests", "dtype": "string"}, {"name": "stop_tokens", "sequence": "string"}], "splits": [{"name": "test", "num_bytes": 243661, "num_examples": 161}], "download_size": 283817, "dataset_size": 243661}, {"config_name": "pl-remove", "features": [{"name": "name", "dtype": "string"}, {"name": "language", "dtype": "string"}, {"name": "prompt", "dtype": "string"}, {"name": "doctests", "dtype": "string"}, {"name": "original", "dtype": "string"}, {"name": "prompt_terminology", "dtype": "string"}, {"name": "tests", "dtype": "string"}, {"name": "stop_tokens", "sequence": "string"}], "splits": [{"name": "test", "num_bytes": 220817, "num_examples": 158}], "download_size": 258463, "dataset_size": 220817}, {"config_name": "py-keep", "features": [{"name": "name", "dtype": "string"}, {"name": "language", "dtype": "string"}, {"name": "prompt", "dtype": "string"}, {"name": "doctests", "dtype": "string"}, {"name": "original", "dtype": "string"}, {"name": "prompt_terminology", "dtype": "string"}, {"name": "tests", "dtype": "string"}, {"name": "stop_tokens", "sequence": "string"}], "splits": [{"name": "test", "num_bytes": 173537, "num_examples": 161}], "download_size": 207009, "dataset_size": 173537}, {"config_name": "py-transform", "features": [{"name": "name", "dtype": "string"}, {"name": "language", "dtype": "string"}, {"name": "prompt", "dtype": "string"}, {"name": "doctests", "dtype": "string"}, {"name": "original", "dtype": "string"}, {"name": "prompt_terminology", "dtype": "string"}, {"name": "tests", "dtype": "string"}, {"name": "stop_tokens", "sequence": "string"}], "splits": [{"name": "test", "num_bytes": 177787, "num_examples": 161}], "download_size": 210975, "dataset_size": 177787}, {"config_name": "py-reworded", "features": [{"name": "name", "dtype": "string"}, {"name": "language", "dtype": "string"}, {"name": "prompt", "dtype": "string"}, {"name": "doctests", "dtype": "string"}, {"name": "original", "dtype": "string"}, {"name": "prompt_terminology", "dtype": "string"}, {"name": "tests", "dtype": "string"}, {"name": "stop_tokens", "sequence": "string"}], "splits": [{"name": "test", "num_bytes": 177787, "num_examples": 161}], "download_size": 210975, "dataset_size": 177787}, {"config_name": "py-remove", "features": [{"name": "name", "dtype": "string"}, {"name": "language", "dtype": "string"}, {"name": "prompt", "dtype": "string"}, {"name": "doctests", "dtype": "string"}, {"name": "original", "dtype": "string"}, {"name": "prompt_terminology", "dtype": "string"}, {"name": "tests", "dtype": "string"}, {"name": "stop_tokens", "sequence": "string"}], "splits": [{"name": "test", "num_bytes": 155389, "num_examples": 158}], "download_size": 187068, "dataset_size": 155389}, {"config_name": "r-keep", "features": [{"name": "name", "dtype": "string"}, {"name": "language", "dtype": "string"}, {"name": "prompt", "dtype": "string"}, {"name": "doctests", "dtype": "string"}, {"name": "original", "dtype": "string"}, {"name": "prompt_terminology", "dtype": "string"}, {"name": "tests", "dtype": "string"}, {"name": "stop_tokens", "sequence": "string"}], "splits": [{"name": "test", "num_bytes": 186803, "num_examples": 161}], "download_size": 215857, "dataset_size": 186803}, {"config_name": "r-transform", "features": [{"name": "name", "dtype": "string"}, {"name": "language", "dtype": "string"}, {"name": "prompt", "dtype": "string"}, {"name": "doctests", "dtype": "string"}, {"name": "original", "dtype": "string"}, {"name": "prompt_terminology", "dtype": "string"}, {"name": "tests", "dtype": "string"}, {"name": "stop_tokens", "sequence": "string"}], "splits": [{"name": "test", "num_bytes": 191732, "num_examples": 161}], "download_size": 220505, "dataset_size": 191732}, {"config_name": "r-reworded", "features": [{"name": "name", "dtype": "string"}, {"name": "language", "dtype": "string"}, {"name": "prompt", "dtype": "string"}, {"name": "doctests", "dtype": "string"}, {"name": "original", "dtype": "string"}, {"name": "prompt_terminology", "dtype": "string"}, {"name": "tests", "dtype": "string"}, {"name": "stop_tokens", "sequence": "string"}], "splits": [{"name": "test", "num_bytes": 191747, "num_examples": 161}], "download_size": 220520, "dataset_size": 191747}, {"config_name": "r-remove", "features": [{"name": "name", "dtype": "string"}, {"name": "language", "dtype": "string"}, {"name": "prompt", "dtype": "string"}, {"name": "doctests", "dtype": "string"}, {"name": "original", "dtype": "string"}, {"name": "prompt_terminology", "dtype": "string"}, {"name": "tests", "dtype": "string"}, {"name": "stop_tokens", "sequence": "string"}], "splits": [{"name": "test", "num_bytes": 168422, "num_examples": 158}], "download_size": 195771, "dataset_size": 168422}, {"config_name": "rb-keep", "features": [{"name": "name", "dtype": "string"}, {"name": "language", "dtype": "string"}, {"name": "prompt", "dtype": "string"}, {"name": "doctests", "dtype": "string"}, {"name": "original", "dtype": "string"}, {"name": "prompt_terminology", "dtype": "string"}, {"name": "tests", "dtype": "string"}, {"name": "stop_tokens", "sequence": "string"}], "splits": [{"name": "test", "num_bytes": 181999, "num_examples": 161}], "download_size": 216186, "dataset_size": 181999}, {"config_name": "rb-transform", "features": [{"name": "name", "dtype": "string"}, {"name": "language", "dtype": "string"}, {"name": "prompt", "dtype": "string"}, {"name": "doctests", "dtype": "string"}, {"name": "original", "dtype": "string"}, {"name": "prompt_terminology", "dtype": "string"}, {"name": "tests", "dtype": "string"}, {"name": "stop_tokens", "sequence": "string"}], "splits": [{"name": "test", "num_bytes": 188317, "num_examples": 161}], "download_size": 223059, "dataset_size": 188317}, {"config_name": "rb-reworded", "features": [{"name": "name", "dtype": "string"}, {"name": "language", "dtype": "string"}, {"name": "prompt", "dtype": "string"}, {"name": "doctests", "dtype": "string"}, {"name": "original", "dtype": "string"}, {"name": "prompt_terminology", "dtype": "string"}, {"name": "tests", "dtype": "string"}, {"name": "stop_tokens", "sequence": "string"}], "splits": [{"name": "test", "num_bytes": 188457, "num_examples": 161}], "download_size": 223199, "dataset_size": 188457}, {"config_name": "rb-remove", "features": [{"name": "name", "dtype": "string"}, {"name": "language", "dtype": "string"}, {"name": "prompt", "dtype": "string"}, {"name": "doctests", "dtype": "string"}, {"name": "original", "dtype": "string"}, {"name": "prompt_terminology", "dtype": "string"}, {"name": "tests", "dtype": "string"}, {"name": "stop_tokens", "sequence": "string"}], "splits": [{"name": "test", "num_bytes": 163569, "num_examples": 158}], "download_size": 195978, "dataset_size": 163569}, {"config_name": "rkt-keep", "features": [{"name": "name", "dtype": "string"}, {"name": "language", "dtype": "string"}, {"name": "prompt", "dtype": "string"}, {"name": "doctests", "dtype": "string"}, {"name": "original", "dtype": "string"}, {"name": "prompt_terminology", "dtype": "string"}, {"name": "tests", "dtype": "string"}, {"name": "stop_tokens", "sequence": "string"}], "splits": [{"name": "test", "num_bytes": 177757, "num_examples": 161}], "download_size": 212266, "dataset_size": 177757}, {"config_name": "rkt-transform", "features": [{"name": "name", "dtype": "string"}, {"name": "language", "dtype": "string"}, {"name": "prompt", "dtype": "string"}, {"name": "doctests", "dtype": "string"}, {"name": "original", "dtype": "string"}, {"name": "prompt_terminology", "dtype": "string"}, {"name": "tests", "dtype": "string"}, {"name": "stop_tokens", "sequence": "string"}], "splits": [{"name": "test", "num_bytes": 182937, "num_examples": 161}], "download_size": 218001, "dataset_size": 182937}, {"config_name": "rkt-reworded", "features": [{"name": "name", "dtype": "string"}, {"name": "language", "dtype": "string"}, {"name": "prompt", "dtype": "string"}, {"name": "doctests", "dtype": "string"}, {"name": "original", "dtype": "string"}, {"name": "prompt_terminology", "dtype": "string"}, {"name": "tests", "dtype": "string"}, {"name": "stop_tokens", "sequence": "string"}], "splits": [{"name": "test", "num_bytes": 182754, "num_examples": 161}], "download_size": 217818, "dataset_size": 182754}, {"config_name": "rkt-remove", "features": [{"name": "name", "dtype": "string"}, {"name": "language", "dtype": "string"}, {"name": "prompt", "dtype": "string"}, {"name": "doctests", "dtype": "string"}, {"name": "original", "dtype": "string"}, {"name": "prompt_terminology", "dtype": "string"}, {"name": "tests", "dtype": "string"}, {"name": "stop_tokens", "sequence": "string"}], "splits": [{"name": "test", "num_bytes": 158729, "num_examples": 158}], "download_size": 191454, "dataset_size": 158729}, {"config_name": "rs-keep", "features": [{"name": "name", "dtype": "string"}, {"name": "language", "dtype": "string"}, {"name": "prompt", "dtype": "string"}, {"name": "doctests", "dtype": "string"}, {"name": "original", "dtype": "string"}, {"name": "prompt_terminology", "dtype": "string"}, {"name": "tests", "dtype": "string"}, {"name": "stop_tokens", "sequence": "string"}], "splits": [{"name": "test", "num_bytes": 177191, "num_examples": 156}], "download_size": 206604, "dataset_size": 177191}, {"config_name": "rs-transform", "features": [{"name": "name", "dtype": "string"}, {"name": "language", "dtype": "string"}, {"name": "prompt", "dtype": "string"}, {"name": "doctests", "dtype": "string"}, {"name": "original", "dtype": "string"}, {"name": "prompt_terminology", "dtype": "string"}, {"name": "tests", "dtype": "string"}, {"name": "stop_tokens", "sequence": "string"}], "splits": [{"name": "test", "num_bytes": 188587, "num_examples": 156}], "download_size": 218555, "dataset_size": 188587}, {"config_name": "rs-reworded", "features": [{"name": "name", "dtype": "string"}, {"name": "language", "dtype": "string"}, {"name": "prompt", "dtype": "string"}, {"name": "doctests", "dtype": "string"}, {"name": "original", "dtype": "string"}, {"name": "prompt_terminology", "dtype": "string"}, {"name": "tests", "dtype": "string"}, {"name": "stop_tokens", "sequence": "string"}], "splits": [{"name": "test", "num_bytes": 188841, "num_examples": 156}], "download_size": 218809, "dataset_size": 188841}, {"config_name": "rs-remove", "features": [{"name": "name", "dtype": "string"}, {"name": "language", "dtype": "string"}, {"name": "prompt", "dtype": "string"}, {"name": "doctests", "dtype": "string"}, {"name": "original", "dtype": "string"}, {"name": "prompt_terminology", "dtype": "string"}, {"name": "tests", "dtype": "string"}, {"name": "stop_tokens", "sequence": "string"}], "splits": [{"name": "test", "num_bytes": 158191, "num_examples": 153}], "download_size": 185991, "dataset_size": 158191}, {"config_name": "scala-keep", "features": [{"name": "name", "dtype": "string"}, {"name": "language", "dtype": "string"}, {"name": "prompt", "dtype": "string"}, {"name": "doctests", "dtype": "string"}, {"name": "original", "dtype": "string"}, {"name": "prompt_terminology", "dtype": "string"}, {"name": "tests", "dtype": "string"}, {"name": "stop_tokens", "sequence": "string"}], "splits": [{"name": "test", "num_bytes": 222118, "num_examples": 160}], "download_size": 253027, "dataset_size": 222118}, {"config_name": "scala-transform", "features": [{"name": "name", "dtype": "string"}, {"name": "language", "dtype": "string"}, {"name": "prompt", "dtype": "string"}, {"name": "doctests", "dtype": "string"}, {"name": "original", "dtype": "string"}, {"name": "prompt_terminology", "dtype": "string"}, {"name": "tests", "dtype": "string"}, {"name": "stop_tokens", "sequence": "string"}], "splits": [{"name": "test", "num_bytes": 240540, "num_examples": 160}], "download_size": 272012, "dataset_size": 240540}, {"config_name": "scala-reworded", "features": [{"name": "name", "dtype": "string"}, {"name": "language", "dtype": "string"}, {"name": "prompt", "dtype": "string"}, {"name": "doctests", "dtype": "string"}, {"name": "original", "dtype": "string"}, {"name": "prompt_terminology", "dtype": "string"}, {"name": "tests", "dtype": "string"}, {"name": "stop_tokens", "sequence": "string"}], "splits": [{"name": "test", "num_bytes": 240466, "num_examples": 160}], "download_size": 271938, "dataset_size": 240466}, {"config_name": "scala-remove", "features": [{"name": "name", "dtype": "string"}, {"name": "language", "dtype": "string"}, {"name": "prompt", "dtype": "string"}, {"name": "doctests", "dtype": "string"}, {"name": "original", "dtype": "string"}, {"name": "prompt_terminology", "dtype": "string"}, {"name": "tests", "dtype": "string"}, {"name": "stop_tokens", "sequence": "string"}], "splits": [{"name": "test", "num_bytes": 200261, "num_examples": 157}], "download_size": 229477, "dataset_size": 200261}, {"config_name": "sh-keep", "features": [{"name": "name", "dtype": "string"}, {"name": "language", "dtype": "string"}, {"name": "prompt", "dtype": "string"}, {"name": "doctests", "dtype": "string"}, {"name": "original", "dtype": "string"}, {"name": "prompt_terminology", "dtype": "string"}, {"name": "tests", "dtype": "string"}, {"name": "stop_tokens", "sequence": "string"}], "splits": [{"name": "test", "num_bytes": 158460, "num_examples": 158}], "download_size": 193268, "dataset_size": 158460}, {"config_name": "sh-transform", "features": [{"name": "name", "dtype": "string"}, {"name": "language", "dtype": "string"}, {"name": "prompt", "dtype": "string"}, {"name": "doctests", "dtype": "string"}, {"name": "original", "dtype": "string"}, {"name": "prompt_terminology", "dtype": "string"}, {"name": "tests", "dtype": "string"}, {"name": "stop_tokens", "sequence": "string"}], "splits": [{"name": "test", "num_bytes": 164552, "num_examples": 158}], "download_size": 201631, "dataset_size": 164552}, {"config_name": "sh-reworded", "features": [{"name": "name", "dtype": "string"}, {"name": "language", "dtype": "string"}, {"name": "prompt", "dtype": "string"}, {"name": "doctests", "dtype": "string"}, {"name": "original", "dtype": "string"}, {"name": "prompt_terminology", "dtype": "string"}, {"name": "tests", "dtype": "string"}, {"name": "stop_tokens", "sequence": "string"}], "splits": [{"name": "test", "num_bytes": 164521, "num_examples": 158}], "download_size": 201600, "dataset_size": 164521}, {"config_name": "sh-remove", "features": [{"name": "name", "dtype": "string"}, {"name": "language", "dtype": "string"}, {"name": "prompt", "dtype": "string"}, {"name": "doctests", "dtype": "string"}, {"name": "original", "dtype": "string"}, {"name": "prompt_terminology", "dtype": "string"}, {"name": "tests", "dtype": "string"}, {"name": "stop_tokens", "sequence": "string"}], "splits": [{"name": "test", "num_bytes": 140720, "num_examples": 155}], "download_size": 173767, "dataset_size": 140720}, {"config_name": "swift-keep", "features": [{"name": "name", "dtype": "string"}, {"name": "language", "dtype": "string"}, {"name": "prompt", "dtype": "string"}, {"name": "doctests", "dtype": "string"}, {"name": "original", "dtype": "string"}, {"name": "prompt_terminology", "dtype": "string"}, {"name": "tests", "dtype": "string"}, {"name": "stop_tokens", "sequence": "string"}], "splits": [{"name": "test", "num_bytes": 201798, "num_examples": 161}], "download_size": 233903, "dataset_size": 201798}, {"config_name": "swift-transform", "features": [{"name": "name", "dtype": "string"}, {"name": "language", "dtype": "string"}, {"name": "prompt", "dtype": "string"}, {"name": "doctests", "dtype": "string"}, {"name": "original", "dtype": "string"}, {"name": "prompt_terminology", "dtype": "string"}, {"name": "tests", "dtype": "string"}, {"name": "stop_tokens", "sequence": "string"}], "splits": [{"name": "test", "num_bytes": 204760, "num_examples": 158}], "download_size": 236660, "dataset_size": 204760}, {"config_name": "swift-reworded", "features": [{"name": "name", "dtype": "string"}, {"name": "language", "dtype": "string"}, {"name": "prompt", "dtype": "string"}, {"name": "doctests", "dtype": "string"}, {"name": "original", "dtype": "string"}, {"name": "prompt_terminology", "dtype": "string"}, {"name": "tests", "dtype": "string"}, {"name": "stop_tokens", "sequence": "string"}], "splits": [{"name": "test", "num_bytes": 204920, "num_examples": 158}], "download_size": 236820, "dataset_size": 204920}, {"config_name": "swift-remove", "features": [{"name": "name", "dtype": "string"}, {"name": "language", "dtype": "string"}, {"name": "prompt", "dtype": "string"}, {"name": "doctests", "dtype": "string"}, {"name": "original", "dtype": "string"}, {"name": "prompt_terminology", "dtype": "string"}, {"name": "tests", "dtype": "string"}, {"name": "stop_tokens", "sequence": "string"}], "splits": [{"name": "test", "num_bytes": 181681, "num_examples": 158}], "download_size": 212047, "dataset_size": 181681}, {"config_name": "ts-keep", "features": [{"name": "name", "dtype": "string"}, {"name": "language", "dtype": "string"}, {"name": "prompt", "dtype": "string"}, {"name": "doctests", "dtype": "string"}, {"name": "original", "dtype": "string"}, {"name": "prompt_terminology", "dtype": "string"}, {"name": "tests", "dtype": "string"}, {"name": "stop_tokens", "sequence": "string"}], "splits": [{"name": "test", "num_bytes": 181763, "num_examples": 159}], "download_size": 215589, "dataset_size": 181763}, {"config_name": "ts-transform", "features": [{"name": "name", "dtype": "string"}, {"name": "language", "dtype": "string"}, {"name": "prompt", "dtype": "string"}, {"name": "doctests", "dtype": "string"}, {"name": "original", "dtype": "string"}, {"name": "prompt_terminology", "dtype": "string"}, {"name": "tests", "dtype": "string"}, {"name": "stop_tokens", "sequence": "string"}], "splits": [{"name": "test", "num_bytes": 186037, "num_examples": 159}], "download_size": 220423, "dataset_size": 186037}, {"config_name": "ts-reworded", "features": [{"name": "name", "dtype": "string"}, {"name": "language", "dtype": "string"}, {"name": "prompt", "dtype": "string"}, {"name": "doctests", "dtype": "string"}, {"name": "original", "dtype": "string"}, {"name": "prompt_terminology", "dtype": "string"}, {"name": "tests", "dtype": "string"}, {"name": "stop_tokens", "sequence": "string"}], "splits": [{"name": "test", "num_bytes": 186215, "num_examples": 159}], "download_size": 220601, "dataset_size": 186215}, {"config_name": "ts-remove", "features": [{"name": "name", "dtype": "string"}, {"name": "language", "dtype": "string"}, {"name": "prompt", "dtype": "string"}, {"name": "doctests", "dtype": "string"}, {"name": "original", "dtype": "string"}, {"name": "prompt_terminology", "dtype": "string"}, {"name": "tests", "dtype": "string"}, {"name": "stop_tokens", "sequence": "string"}], "splits": [{"name": "test", "num_bytes": 162881, "num_examples": 156}], "download_size": 194985, "dataset_size": 162881}, {"config_name": "cpp", "features": [{"name": "name", "dtype": "string"}, {"name": "language", "dtype": "string"}, {"name": "prompt", "dtype": "string"}, {"name": "doctests", "dtype": "string"}, {"name": "original", "dtype": "string"}, {"name": "prompt_terminology", "dtype": "string"}, {"name": "tests", "dtype": "string"}, {"name": "stop_tokens", "sequence": "string"}], "splits": [{"name": "test", "num_bytes": 239767, "num_examples": 161}], "download_size": 271023, "dataset_size": 239767}, {"config_name": "cs", "features": [{"name": "name", "dtype": "string"}, {"name": "language", "dtype": "string"}, {"name": "prompt", "dtype": "string"}, {"name": "doctests", "dtype": "string"}, {"name": "original", "dtype": "string"}, {"name": "prompt_terminology", "dtype": "string"}, {"name": "tests", "dtype": "string"}, {"name": "stop_tokens", "sequence": "string"}], "splits": [{"name": "test", "num_bytes": 283673, "num_examples": 158}], "download_size": 315498, "dataset_size": 283673}, {"config_name": "d", "features": [{"name": "name", "dtype": "string"}, {"name": "language", "dtype": "string"}, {"name": "prompt", "dtype": "string"}, {"name": "doctests", "dtype": "string"}, {"name": "original", "dtype": "string"}, {"name": "prompt_terminology", "dtype": "string"}, {"name": "tests", "dtype": "string"}, {"name": "stop_tokens", "sequence": "string"}], "splits": [{"name": "test", "num_bytes": 181296, "num_examples": 156}], "download_size": 215824, "dataset_size": 181296}, {"config_name": "go", "features": [{"name": "name", "dtype": "string"}, {"name": "language", "dtype": "string"}, {"name": "prompt", "dtype": "string"}, {"name": "doctests", "dtype": "string"}, {"name": "original", "dtype": "string"}, {"name": "prompt_terminology", "dtype": "string"}, {"name": "tests", "dtype": "string"}, {"name": "stop_tokens", "sequence": "string"}], "splits": [{"name": "test", "num_bytes": 247354, "num_examples": 154}], "download_size": 287181, "dataset_size": 247354}, {"config_name": "java", "features": [{"name": "name", "dtype": "string"}, {"name": "language", "dtype": "string"}, {"name": "prompt", "dtype": "string"}, {"name": "doctests", "dtype": "string"}, {"name": "original", "dtype": "string"}, {"name": "prompt_terminology", "dtype": "string"}, {"name": "tests", "dtype": "string"}, {"name": "stop_tokens", "sequence": "string"}], "splits": [{"name": "test", "num_bytes": 288031, "num_examples": 158}], "download_size": 319856, "dataset_size": 288031}, {"config_name": "jl", "features": [{"name": "name", "dtype": "string"}, {"name": "language", "dtype": "string"}, {"name": "prompt", "dtype": "string"}, {"name": "doctests", "dtype": "string"}, {"name": "original", "dtype": "string"}, {"name": "prompt_terminology", "dtype": "string"}, {"name": "tests", "dtype": "string"}, {"name": "stop_tokens", "sequence": "string"}], "splits": [{"name": "test", "num_bytes": 168251, "num_examples": 159}], "download_size": 203796, "dataset_size": 168251}, {"config_name": "js", "features": [{"name": "name", "dtype": "string"}, {"name": "language", "dtype": "string"}, {"name": "prompt", "dtype": "string"}, {"name": "doctests", "dtype": "string"}, {"name": "original", "dtype": "string"}, {"name": "prompt_terminology", "dtype": "string"}, {"name": "tests", "dtype": "string"}, {"name": "stop_tokens", "sequence": "string"}], "splits": [{"name": "test", "num_bytes": 182171, "num_examples": 161}], "download_size": 216913, "dataset_size": 182171}, {"config_name": "lua", "features": [{"name": "name", "dtype": "string"}, {"name": "language", "dtype": "string"}, {"name": "prompt", "dtype": "string"}, {"name": "doctests", "dtype": "string"}, {"name": "original", "dtype": "string"}, {"name": "prompt_terminology", "dtype": "string"}, {"name": "tests", "dtype": "string"}, {"name": "stop_tokens", "sequence": "string"}], "splits": [{"name": "test", "num_bytes": 184853, "num_examples": 161}], "download_size": 216685, "dataset_size": 184853}, {"config_name": "php", "features": [{"name": "name", "dtype": "string"}, {"name": "language", "dtype": "string"}, {"name": "prompt", "dtype": "string"}, {"name": "doctests", "dtype": "string"}, {"name": "original", "dtype": "string"}, {"name": "prompt_terminology", "dtype": "string"}, {"name": "tests", "dtype": "string"}, {"name": "stop_tokens", "sequence": "string"}], "splits": [{"name": "test", "num_bytes": 225730, "num_examples": 161}], "download_size": 262893, "dataset_size": 225730}, {"config_name": "pl", "features": [{"name": "name", "dtype": "string"}, {"name": "language", "dtype": "string"}, {"name": "prompt", "dtype": "string"}, {"name": "doctests", "dtype": "string"}, {"name": "original", "dtype": "string"}, {"name": "prompt_terminology", "dtype": "string"}, {"name": "tests", "dtype": "string"}, {"name": "stop_tokens", "sequence": "string"}], "splits": [{"name": "test", "num_bytes": 243661, "num_examples": 161}], "download_size": 283817, "dataset_size": 243661}, {"config_name": "py", "features": [{"name": "name", "dtype": "string"}, {"name": "language", "dtype": "string"}, {"name": "prompt", "dtype": "string"}, {"name": "doctests", "dtype": "string"}, {"name": "original", "dtype": "string"}, {"name": "prompt_terminology", "dtype": "string"}, {"name": "tests", "dtype": "string"}, {"name": "stop_tokens", "sequence": "string"}], "splits": [{"name": "test", "num_bytes": 177787, "num_examples": 161}], "download_size": 210975, "dataset_size": 177787}, {"config_name": "r", "features": [{"name": "name", "dtype": "string"}, {"name": "language", "dtype": "string"}, {"name": "prompt", "dtype": "string"}, {"name": "doctests", "dtype": "string"}, {"name": "original", "dtype": "string"}, {"name": "prompt_terminology", "dtype": "string"}, {"name": "tests", "dtype": "string"}, {"name": "stop_tokens", "sequence": "string"}], "splits": [{"name": "test", "num_bytes": 191747, "num_examples": 161}], "download_size": 220520, "dataset_size": 191747}, {"config_name": "rb", "features": [{"name": "name", "dtype": "string"}, {"name": "language", "dtype": "string"}, {"name": "prompt", "dtype": "string"}, {"name": "doctests", "dtype": "string"}, {"name": "original", "dtype": "string"}, {"name": "prompt_terminology", "dtype": "string"}, {"name": "tests", "dtype": "string"}, {"name": "stop_tokens", "sequence": "string"}], "splits": [{"name": "test", "num_bytes": 188457, "num_examples": 161}], "download_size": 223199, "dataset_size": 188457}, {"config_name": "rkt", "features": [{"name": "name", "dtype": "string"}, {"name": "language", "dtype": "string"}, {"name": "prompt", "dtype": "string"}, {"name": "doctests", "dtype": "string"}, {"name": "original", "dtype": "string"}, {"name": "prompt_terminology", "dtype": "string"}, {"name": "tests", "dtype": "string"}, {"name": "stop_tokens", "sequence": "string"}], "splits": [{"name": "test", "num_bytes": 182754, "num_examples": 161}], "download_size": 217818, "dataset_size": 182754}, {"config_name": "rs", "features": [{"name": "name", "dtype": "string"}, {"name": "language", "dtype": "string"}, {"name": "prompt", "dtype": "string"}, {"name": "doctests", "dtype": "string"}, {"name": "original", "dtype": "string"}, {"name": "prompt_terminology", "dtype": "string"}, {"name": "tests", "dtype": "string"}, {"name": "stop_tokens", "sequence": "string"}], "splits": [{"name": "test", "num_bytes": 188841, "num_examples": 156}], "download_size": 218809, "dataset_size": 188841}, {"config_name": "scala", "features": [{"name": "name", "dtype": "string"}, {"name": "language", "dtype": "string"}, {"name": "prompt", "dtype": "string"}, {"name": "doctests", "dtype": "string"}, {"name": "original", "dtype": "string"}, {"name": "prompt_terminology", "dtype": "string"}, {"name": "tests", "dtype": "string"}, {"name": "stop_tokens", "sequence": "string"}], "splits": [{"name": "test", "num_bytes": 240466, "num_examples": 160}], "download_size": 271938, "dataset_size": 240466}, {"config_name": "sh", "features": [{"name": "name", "dtype": "string"}, {"name": "language", "dtype": "string"}, {"name": "prompt", "dtype": "string"}, {"name": "doctests", "dtype": "string"}, {"name": "original", "dtype": "string"}, {"name": "prompt_terminology", "dtype": "string"}, {"name": "tests", "dtype": "string"}, {"name": "stop_tokens", "sequence": "string"}], "splits": [{"name": "test", "num_bytes": 164521, "num_examples": 158}], "download_size": 201600, "dataset_size": 164521}, {"config_name": "swift", "features": [{"name": "name", "dtype": "string"}, {"name": "language", "dtype": "string"}, {"name": "prompt", "dtype": "string"}, {"name": "doctests", "dtype": "string"}, {"name": "original", "dtype": "string"}, {"name": "prompt_terminology", "dtype": "string"}, {"name": "tests", "dtype": "string"}, {"name": "stop_tokens", "sequence": "string"}], "splits": [{"name": "test", "num_bytes": 204920, "num_examples": 158}], "download_size": 236820, "dataset_size": 204920}, {"config_name": "ts", "features": [{"name": "name", "dtype": "string"}, {"name": "language", "dtype": "string"}, {"name": "prompt", "dtype": "string"}, {"name": "doctests", "dtype": "string"}, {"name": "original", "dtype": "string"}, {"name": "prompt_terminology", "dtype": "string"}, {"name": "tests", "dtype": "string"}, {"name": "stop_tokens", "sequence": "string"}], "splits": [{"name": "test", "num_bytes": 186215, "num_examples": 159}], "download_size": 220601, "dataset_size": 186215}, {"config_name": "humaneval-cpp-keep", "features": [{"name": "name", "dtype": "string"}, {"name": "language", "dtype": "string"}, {"name": "prompt", "dtype": "string"}, {"name": "doctests", "dtype": "string"}, {"name": "original", "dtype": "string"}, {"name": "prompt_terminology", "dtype": "string"}, {"name": "tests", "dtype": "string"}, {"name": "stop_tokens", "sequence": "string"}], "splits": [{"name": "test", "num_bytes": 218990, "num_examples": 161}], "download_size": 249691, "dataset_size": 218990}, {"config_name": "humaneval-cpp-transform", "features": [{"name": "name", "dtype": "string"}, {"name": "language", "dtype": "string"}, {"name": "prompt", "dtype": "string"}, {"name": "doctests", "dtype": "string"}, {"name": "original", "dtype": "string"}, {"name": "prompt_terminology", "dtype": "string"}, {"name": "tests", "dtype": "string"}, {"name": "stop_tokens", "sequence": "string"}], "splits": [{"name": "test", "num_bytes": 240786, "num_examples": 161}], "download_size": 272042, "dataset_size": 240786}, {"config_name": "humaneval-cpp", "features": [{"name": "name", "dtype": "string"}, {"name": "language", "dtype": "string"}, {"name": "prompt", "dtype": "string"}, {"name": "doctests", "dtype": "string"}, {"name": "original", "dtype": "string"}, {"name": "prompt_terminology", "dtype": "string"}, {"name": "tests", "dtype": "string"}, {"name": "stop_tokens", "sequence": "string"}], "splits": [{"name": "test", "num_bytes": 241036, "num_examples": 161}], "download_size": 272292, "dataset_size": 241036}, {"config_name": "humaneval-cpp-remove", "features": [{"name": "name", "dtype": "string"}, {"name": "language", "dtype": "string"}, {"name": "prompt", "dtype": "string"}, {"name": "doctests", "dtype": "string"}, {"name": "original", "dtype": "string"}, {"name": "prompt_terminology", "dtype": "string"}, {"name": "tests", "dtype": "string"}, {"name": "stop_tokens", "sequence": "string"}], "splits": [{"name": "test", "num_bytes": 199746, "num_examples": 158}], "download_size": 228735, "dataset_size": 199746}, {"config_name": "humaneval-cs-keep", "features": [{"name": "name", "dtype": "string"}, {"name": "language", "dtype": "string"}, {"name": "prompt", "dtype": "string"}, {"name": "doctests", "dtype": "string"}, {"name": "original", "dtype": "string"}, {"name": "prompt_terminology", "dtype": "string"}, {"name": "tests", "dtype": "string"}, {"name": "stop_tokens", "sequence": "string"}], "splits": [{"name": "test", "num_bytes": 260822, "num_examples": 158}], "download_size": 292085, "dataset_size": 260822}, {"config_name": "humaneval-cs-transform", "features": [{"name": "name", "dtype": "string"}, {"name": "language", "dtype": "string"}, {"name": "prompt", "dtype": "string"}, {"name": "doctests", "dtype": "string"}, {"name": "original", "dtype": "string"}, {"name": "prompt_terminology", "dtype": "string"}, {"name": "tests", "dtype": "string"}, {"name": "stop_tokens", "sequence": "string"}], "splits": [{"name": "test", "num_bytes": 284686, "num_examples": 158}], "download_size": 316511, "dataset_size": 284686}, {"config_name": "humaneval-cs", "features": [{"name": "name", "dtype": "string"}, {"name": "language", "dtype": "string"}, {"name": "prompt", "dtype": "string"}, {"name": "doctests", "dtype": "string"}, {"name": "original", "dtype": "string"}, {"name": "prompt_terminology", "dtype": "string"}, {"name": "tests", "dtype": "string"}, {"name": "stop_tokens", "sequence": "string"}], "splits": [{"name": "test", "num_bytes": 284621, "num_examples": 158}], "download_size": 316446, "dataset_size": 284621}, {"config_name": "humaneval-cs-remove", "features": [{"name": "name", "dtype": "string"}, {"name": "language", "dtype": "string"}, {"name": "prompt", "dtype": "string"}, {"name": "doctests", "dtype": "string"}, {"name": "original", "dtype": "string"}, {"name": "prompt_terminology", "dtype": "string"}, {"name": "tests", "dtype": "string"}, {"name": "stop_tokens", "sequence": "string"}], "splits": [{"name": "test", "num_bytes": 238593, "num_examples": 155}], "download_size": 268181, "dataset_size": 238593}, {"config_name": "humaneval-d-keep", "features": [{"name": "name", "dtype": "string"}, {"name": "language", "dtype": "string"}, {"name": "prompt", "dtype": "string"}, {"name": "doctests", "dtype": "string"}, {"name": "original", "dtype": "string"}, {"name": "prompt_terminology", "dtype": "string"}, {"name": "tests", "dtype": "string"}, {"name": "stop_tokens", "sequence": "string"}], "splits": [{"name": "test", "num_bytes": 176864, "num_examples": 156}], "download_size": 210856, "dataset_size": 176864}, {"config_name": "humaneval-d-transform", "features": [{"name": "name", "dtype": "string"}, {"name": "language", "dtype": "string"}, {"name": "prompt", "dtype": "string"}, {"name": "doctests", "dtype": "string"}, {"name": "original", "dtype": "string"}, {"name": "prompt_terminology", "dtype": "string"}, {"name": "tests", "dtype": "string"}, {"name": "stop_tokens", "sequence": "string"}], "splits": [{"name": "test", "num_bytes": 182057, "num_examples": 156}], "download_size": 216585, "dataset_size": 182057}, {"config_name": "humaneval-d", "features": [{"name": "name", "dtype": "string"}, {"name": "language", "dtype": "string"}, {"name": "prompt", "dtype": "string"}, {"name": "doctests", "dtype": "string"}, {"name": "original", "dtype": "string"}, {"name": "prompt_terminology", "dtype": "string"}, {"name": "tests", "dtype": "string"}, {"name": "stop_tokens", "sequence": "string"}], "splits": [{"name": "test", "num_bytes": 182232, "num_examples": 156}], "download_size": 216760, "dataset_size": 182232}, {"config_name": "humaneval-d-remove", "features": [{"name": "name", "dtype": "string"}, {"name": "language", "dtype": "string"}, {"name": "prompt", "dtype": "string"}, {"name": "doctests", "dtype": "string"}, {"name": "original", "dtype": "string"}, {"name": "prompt_terminology", "dtype": "string"}, {"name": "tests", "dtype": "string"}, {"name": "stop_tokens", "sequence": "string"}], "splits": [{"name": "test", "num_bytes": 158856, "num_examples": 153}], "download_size": 191129, "dataset_size": 158856}, {"config_name": "humaneval-go-keep", "features": [{"name": "name", "dtype": "string"}, {"name": "language", "dtype": "string"}, {"name": "prompt", "dtype": "string"}, {"name": "doctests", "dtype": "string"}, {"name": "original", "dtype": "string"}, {"name": "prompt_terminology", "dtype": "string"}, {"name": "tests", "dtype": "string"}, {"name": "stop_tokens", "sequence": "string"}], "splits": [{"name": "test", "num_bytes": 242054, "num_examples": 154}], "download_size": 281348, "dataset_size": 242054}, {"config_name": "humaneval-go-transform", "features": [{"name": "name", "dtype": "string"}, {"name": "language", "dtype": "string"}, {"name": "prompt", "dtype": "string"}, {"name": "doctests", "dtype": "string"}, {"name": "original", "dtype": "string"}, {"name": "prompt_terminology", "dtype": "string"}, {"name": "tests", "dtype": "string"}, {"name": "stop_tokens", "sequence": "string"}], "splits": [{"name": "test", "num_bytes": 248372, "num_examples": 154}], "download_size": 288199, "dataset_size": 248372}, {"config_name": "humaneval-go", "features": [{"name": "name", "dtype": "string"}, {"name": "language", "dtype": "string"}, {"name": "prompt", "dtype": "string"}, {"name": "doctests", "dtype": "string"}, {"name": "original", "dtype": "string"}, {"name": "prompt_terminology", "dtype": "string"}, {"name": "tests", "dtype": "string"}, {"name": "stop_tokens", "sequence": "string"}], "splits": [{"name": "test", "num_bytes": 248278, "num_examples": 154}], "download_size": 288105, "dataset_size": 248278}, {"config_name": "humaneval-go-remove", "features": [{"name": "name", "dtype": "string"}, {"name": "language", "dtype": "string"}, {"name": "prompt", "dtype": "string"}, {"name": "doctests", "dtype": "string"}, {"name": "original", "dtype": "string"}, {"name": "prompt_terminology", "dtype": "string"}, {"name": "tests", "dtype": "string"}, {"name": "stop_tokens", "sequence": "string"}], "splits": [{"name": "test", "num_bytes": 222425, "num_examples": 151}], "download_size": 259886, "dataset_size": 222425}, {"config_name": "humaneval-java-keep", "features": [{"name": "name", "dtype": "string"}, {"name": "language", "dtype": "string"}, {"name": "prompt", "dtype": "string"}, {"name": "doctests", "dtype": "string"}, {"name": "original", "dtype": "string"}, {"name": "prompt_terminology", "dtype": "string"}, {"name": "tests", "dtype": "string"}, {"name": "stop_tokens", "sequence": "string"}], "splits": [{"name": "test", "num_bytes": 261057, "num_examples": 158}], "download_size": 292320, "dataset_size": 261057}, {"config_name": "humaneval-java-transform", "features": [{"name": "name", "dtype": "string"}, {"name": "language", "dtype": "string"}, {"name": "prompt", "dtype": "string"}, {"name": "doctests", "dtype": "string"}, {"name": "original", "dtype": "string"}, {"name": "prompt_terminology", "dtype": "string"}, {"name": "tests", "dtype": "string"}, {"name": "stop_tokens", "sequence": "string"}], "splits": [{"name": "test", "num_bytes": 287860, "num_examples": 158}], "download_size": 319685, "dataset_size": 287860}, {"config_name": "humaneval-java", "features": [{"name": "name", "dtype": "string"}, {"name": "language", "dtype": "string"}, {"name": "prompt", "dtype": "string"}, {"name": "doctests", "dtype": "string"}, {"name": "original", "dtype": "string"}, {"name": "prompt_terminology", "dtype": "string"}, {"name": "tests", "dtype": "string"}, {"name": "stop_tokens", "sequence": "string"}], "splits": [{"name": "test", "num_bytes": 289343, "num_examples": 158}], "download_size": 321168, "dataset_size": 289343}, {"config_name": "humaneval-java-remove", "features": [{"name": "name", "dtype": "string"}, {"name": "language", "dtype": "string"}, {"name": "prompt", "dtype": "string"}, {"name": "doctests", "dtype": "string"}, {"name": "original", "dtype": "string"}, {"name": "prompt_terminology", "dtype": "string"}, {"name": "tests", "dtype": "string"}, {"name": "stop_tokens", "sequence": "string"}], "splits": [{"name": "test", "num_bytes": 238875, "num_examples": 155}], "download_size": 268463, "dataset_size": 238875}, {"config_name": "humaneval-jl-keep", "features": [{"name": "name", "dtype": "string"}, {"name": "language", "dtype": "string"}, {"name": "prompt", "dtype": "string"}, {"name": "doctests", "dtype": "string"}, {"name": "original", "dtype": "string"}, {"name": "prompt_terminology", "dtype": "string"}, {"name": "tests", "dtype": "string"}, {"name": "stop_tokens", "sequence": "string"}], "splits": [{"name": "test", "num_bytes": 164664, "num_examples": 159}], "download_size": 199654, "dataset_size": 164664}, {"config_name": "humaneval-jl-transform", "features": [{"name": "name", "dtype": "string"}, {"name": "language", "dtype": "string"}, {"name": "prompt", "dtype": "string"}, {"name": "doctests", "dtype": "string"}, {"name": "original", "dtype": "string"}, {"name": "prompt_terminology", "dtype": "string"}, {"name": "tests", "dtype": "string"}, {"name": "stop_tokens", "sequence": "string"}], "splits": [{"name": "test", "num_bytes": 168925, "num_examples": 159}], "download_size": 204472, "dataset_size": 168925}, {"config_name": "humaneval-jl", "features": [{"name": "name", "dtype": "string"}, {"name": "language", "dtype": "string"}, {"name": "prompt", "dtype": "string"}, {"name": "doctests", "dtype": "string"}, {"name": "original", "dtype": "string"}, {"name": "prompt_terminology", "dtype": "string"}, {"name": "tests", "dtype": "string"}, {"name": "stop_tokens", "sequence": "string"}], "splits": [{"name": "test", "num_bytes": 169207, "num_examples": 159}], "download_size": 204754, "dataset_size": 169207}, {"config_name": "humaneval-jl-remove", "features": [{"name": "name", "dtype": "string"}, {"name": "language", "dtype": "string"}, {"name": "prompt", "dtype": "string"}, {"name": "doctests", "dtype": "string"}, {"name": "original", "dtype": "string"}, {"name": "prompt_terminology", "dtype": "string"}, {"name": "tests", "dtype": "string"}, {"name": "stop_tokens", "sequence": "string"}], "splits": [{"name": "test", "num_bytes": 146851, "num_examples": 156}], "download_size": 180098, "dataset_size": 146851}, {"config_name": "humaneval-js-keep", "features": [{"name": "name", "dtype": "string"}, {"name": "language", "dtype": "string"}, {"name": "prompt", "dtype": "string"}, {"name": "doctests", "dtype": "string"}, {"name": "original", "dtype": "string"}, {"name": "prompt_terminology", "dtype": "string"}, {"name": "tests", "dtype": "string"}, {"name": "stop_tokens", "sequence": "string"}], "splits": [{"name": "test", "num_bytes": 178601, "num_examples": 161}], "download_size": 212788, "dataset_size": 178601}, {"config_name": "humaneval-js-transform", "features": [{"name": "name", "dtype": "string"}, {"name": "language", "dtype": "string"}, {"name": "prompt", "dtype": "string"}, {"name": "doctests", "dtype": "string"}, {"name": "original", "dtype": "string"}, {"name": "prompt_terminology", "dtype": "string"}, {"name": "tests", "dtype": "string"}, {"name": "stop_tokens", "sequence": "string"}], "splits": [{"name": "test", "num_bytes": 182953, "num_examples": 161}], "download_size": 217695, "dataset_size": 182953}, {"config_name": "humaneval-js", "features": [{"name": "name", "dtype": "string"}, {"name": "language", "dtype": "string"}, {"name": "prompt", "dtype": "string"}, {"name": "doctests", "dtype": "string"}, {"name": "original", "dtype": "string"}, {"name": "prompt_terminology", "dtype": "string"}, {"name": "tests", "dtype": "string"}, {"name": "stop_tokens", "sequence": "string"}], "splits": [{"name": "test", "num_bytes": 183137, "num_examples": 161}], "download_size": 217879, "dataset_size": 183137}, {"config_name": "humaneval-js-remove", "features": [{"name": "name", "dtype": "string"}, {"name": "language", "dtype": "string"}, {"name": "prompt", "dtype": "string"}, {"name": "doctests", "dtype": "string"}, {"name": "original", "dtype": "string"}, {"name": "prompt_terminology", "dtype": "string"}, {"name": "tests", "dtype": "string"}, {"name": "stop_tokens", "sequence": "string"}], "splits": [{"name": "test", "num_bytes": 159567, "num_examples": 158}], "download_size": 191976, "dataset_size": 159567}, {"config_name": "humaneval-lua-keep", "features": [{"name": "name", "dtype": "string"}, {"name": "language", "dtype": "string"}, {"name": "prompt", "dtype": "string"}, {"name": "doctests", "dtype": "string"}, {"name": "original", "dtype": "string"}, {"name": "prompt_terminology", "dtype": "string"}, {"name": "tests", "dtype": "string"}, {"name": "stop_tokens", "sequence": "string"}], "splits": [{"name": "test", "num_bytes": 181364, "num_examples": 161}], "download_size": 213477, "dataset_size": 181364}, {"config_name": "humaneval-lua-transform", "features": [{"name": "name", "dtype": "string"}, {"name": "language", "dtype": "string"}, {"name": "prompt", "dtype": "string"}, {"name": "doctests", "dtype": "string"}, {"name": "original", "dtype": "string"}, {"name": "prompt_terminology", "dtype": "string"}, {"name": "tests", "dtype": "string"}, {"name": "stop_tokens", "sequence": "string"}], "splits": [{"name": "test", "num_bytes": 185729, "num_examples": 161}], "download_size": 217561, "dataset_size": 185729}, {"config_name": "humaneval-lua", "features": [{"name": "name", "dtype": "string"}, {"name": "language", "dtype": "string"}, {"name": "prompt", "dtype": "string"}, {"name": "doctests", "dtype": "string"}, {"name": "original", "dtype": "string"}, {"name": "prompt_terminology", "dtype": "string"}, {"name": "tests", "dtype": "string"}, {"name": "stop_tokens", "sequence": "string"}], "splits": [{"name": "test", "num_bytes": 185819, "num_examples": 161}], "download_size": 217651, "dataset_size": 185819}, {"config_name": "humaneval-lua-remove", "features": [{"name": "name", "dtype": "string"}, {"name": "language", "dtype": "string"}, {"name": "prompt", "dtype": "string"}, {"name": "doctests", "dtype": "string"}, {"name": "original", "dtype": "string"}, {"name": "prompt_terminology", "dtype": "string"}, {"name": "tests", "dtype": "string"}, {"name": "stop_tokens", "sequence": "string"}], "splits": [{"name": "test", "num_bytes": 162287, "num_examples": 158}], "download_size": 192638, "dataset_size": 162287}, {"config_name": "humaneval-php-keep", "features": [{"name": "name", "dtype": "string"}, {"name": "language", "dtype": "string"}, {"name": "prompt", "dtype": "string"}, {"name": "doctests", "dtype": "string"}, {"name": "original", "dtype": "string"}, {"name": "prompt_terminology", "dtype": "string"}, {"name": "tests", "dtype": "string"}, {"name": "stop_tokens", "sequence": "string"}], "splits": [{"name": "test", "num_bytes": 220492, "num_examples": 161}], "download_size": 257100, "dataset_size": 220492}, {"config_name": "humaneval-php-transform", "features": [{"name": "name", "dtype": "string"}, {"name": "language", "dtype": "string"}, {"name": "prompt", "dtype": "string"}, {"name": "doctests", "dtype": "string"}, {"name": "original", "dtype": "string"}, {"name": "prompt_terminology", "dtype": "string"}, {"name": "tests", "dtype": "string"}, {"name": "stop_tokens", "sequence": "string"}], "splits": [{"name": "test", "num_bytes": 226541, "num_examples": 161}], "download_size": 263704, "dataset_size": 226541}, {"config_name": "humaneval-php", "features": [{"name": "name", "dtype": "string"}, {"name": "language", "dtype": "string"}, {"name": "prompt", "dtype": "string"}, {"name": "doctests", "dtype": "string"}, {"name": "original", "dtype": "string"}, {"name": "prompt_terminology", "dtype": "string"}, {"name": "tests", "dtype": "string"}, {"name": "stop_tokens", "sequence": "string"}], "splits": [{"name": "test", "num_bytes": 226696, "num_examples": 161}], "download_size": 263859, "dataset_size": 226696}, {"config_name": "humaneval-php-remove", "features": [{"name": "name", "dtype": "string"}, {"name": "language", "dtype": "string"}, {"name": "prompt", "dtype": "string"}, {"name": "doctests", "dtype": "string"}, {"name": "original", "dtype": "string"}, {"name": "prompt_terminology", "dtype": "string"}, {"name": "tests", "dtype": "string"}, {"name": "stop_tokens", "sequence": "string"}], "splits": [{"name": "test", "num_bytes": 200995, "num_examples": 158}], "download_size": 235796, "dataset_size": 200995}, {"config_name": "humaneval-pl-keep", "features": [{"name": "name", "dtype": "string"}, {"name": "language", "dtype": "string"}, {"name": "prompt", "dtype": "string"}, {"name": "doctests", "dtype": "string"}, {"name": "original", "dtype": "string"}, {"name": "prompt_terminology", "dtype": "string"}, {"name": "tests", "dtype": "string"}, {"name": "stop_tokens", "sequence": "string"}], "splits": [{"name": "test", "num_bytes": 240840, "num_examples": 161}], "download_size": 280317, "dataset_size": 240840}, {"config_name": "humaneval-pl-transform", "features": [{"name": "name", "dtype": "string"}, {"name": "language", "dtype": "string"}, {"name": "prompt", "dtype": "string"}, {"name": "doctests", "dtype": "string"}, {"name": "original", "dtype": "string"}, {"name": "prompt_terminology", "dtype": "string"}, {"name": "tests", "dtype": "string"}, {"name": "stop_tokens", "sequence": "string"}], "splits": [{"name": "test", "num_bytes": 244577, "num_examples": 161}], "download_size": 284733, "dataset_size": 244577}, {"config_name": "humaneval-pl", "features": [{"name": "name", "dtype": "string"}, {"name": "language", "dtype": "string"}, {"name": "prompt", "dtype": "string"}, {"name": "doctests", "dtype": "string"}, {"name": "original", "dtype": "string"}, {"name": "prompt_terminology", "dtype": "string"}, {"name": "tests", "dtype": "string"}, {"name": "stop_tokens", "sequence": "string"}], "splits": [{"name": "test", "num_bytes": 244627, "num_examples": 161}], "download_size": 284783, "dataset_size": 244627}, {"config_name": "humaneval-pl-remove", "features": [{"name": "name", "dtype": "string"}, {"name": "language", "dtype": "string"}, {"name": "prompt", "dtype": "string"}, {"name": "doctests", "dtype": "string"}, {"name": "original", "dtype": "string"}, {"name": "prompt_terminology", "dtype": "string"}, {"name": "tests", "dtype": "string"}, {"name": "stop_tokens", "sequence": "string"}], "splits": [{"name": "test", "num_bytes": 221765, "num_examples": 158}], "download_size": 259411, "dataset_size": 221765}, {"config_name": "humaneval-py-keep", "features": [{"name": "name", "dtype": "string"}, {"name": "language", "dtype": "string"}, {"name": "prompt", "dtype": "string"}, {"name": "doctests", "dtype": "string"}, {"name": "original", "dtype": "string"}, {"name": "prompt_terminology", "dtype": "string"}, {"name": "tests", "dtype": "string"}, {"name": "stop_tokens", "sequence": "string"}], "splits": [{"name": "test", "num_bytes": 174503, "num_examples": 161}], "download_size": 207975, "dataset_size": 174503}, {"config_name": "humaneval-py-transform", "features": [{"name": "name", "dtype": "string"}, {"name": "language", "dtype": "string"}, {"name": "prompt", "dtype": "string"}, {"name": "doctests", "dtype": "string"}, {"name": "original", "dtype": "string"}, {"name": "prompt_terminology", "dtype": "string"}, {"name": "tests", "dtype": "string"}, {"name": "stop_tokens", "sequence": "string"}], "splits": [{"name": "test", "num_bytes": 178753, "num_examples": 161}], "download_size": 211941, "dataset_size": 178753}, {"config_name": "humaneval-py", "features": [{"name": "name", "dtype": "string"}, {"name": "language", "dtype": "string"}, {"name": "prompt", "dtype": "string"}, {"name": "doctests", "dtype": "string"}, {"name": "original", "dtype": "string"}, {"name": "prompt_terminology", "dtype": "string"}, {"name": "tests", "dtype": "string"}, {"name": "stop_tokens", "sequence": "string"}], "splits": [{"name": "test", "num_bytes": 178753, "num_examples": 161}], "download_size": 211941, "dataset_size": 178753}, {"config_name": "humaneval-py-remove", "features": [{"name": "name", "dtype": "string"}, {"name": "language", "dtype": "string"}, {"name": "prompt", "dtype": "string"}, {"name": "doctests", "dtype": "string"}, {"name": "original", "dtype": "string"}, {"name": "prompt_terminology", "dtype": "string"}, {"name": "tests", "dtype": "string"}, {"name": "stop_tokens", "sequence": "string"}], "splits": [{"name": "test", "num_bytes": 156337, "num_examples": 158}], "download_size": 188016, "dataset_size": 156337}, {"config_name": "humaneval-r-keep", "features": [{"name": "name", "dtype": "string"}, {"name": "language", "dtype": "string"}, {"name": "prompt", "dtype": "string"}, {"name": "doctests", "dtype": "string"}, {"name": "original", "dtype": "string"}, {"name": "prompt_terminology", "dtype": "string"}, {"name": "tests", "dtype": "string"}, {"name": "stop_tokens", "sequence": "string"}], "splits": [{"name": "test", "num_bytes": 186140, "num_examples": 161}], "download_size": 215194, "dataset_size": 186140}, {"config_name": "humaneval-r-transform", "features": [{"name": "name", "dtype": "string"}, {"name": "language", "dtype": "string"}, {"name": "prompt", "dtype": "string"}, {"name": "doctests", "dtype": "string"}, {"name": "original", "dtype": "string"}, {"name": "prompt_terminology", "dtype": "string"}, {"name": "tests", "dtype": "string"}, {"name": "stop_tokens", "sequence": "string"}], "splits": [{"name": "test", "num_bytes": 190637, "num_examples": 161}], "download_size": 219410, "dataset_size": 190637}, {"config_name": "humaneval-r", "features": [{"name": "name", "dtype": "string"}, {"name": "language", "dtype": "string"}, {"name": "prompt", "dtype": "string"}, {"name": "doctests", "dtype": "string"}, {"name": "original", "dtype": "string"}, {"name": "prompt_terminology", "dtype": "string"}, {"name": "tests", "dtype": "string"}, {"name": "stop_tokens", "sequence": "string"}], "splits": [{"name": "test", "num_bytes": 190652, "num_examples": 161}], "download_size": 219425, "dataset_size": 190652}, {"config_name": "humaneval-r-remove", "features": [{"name": "name", "dtype": "string"}, {"name": "language", "dtype": "string"}, {"name": "prompt", "dtype": "string"}, {"name": "doctests", "dtype": "string"}, {"name": "original", "dtype": "string"}, {"name": "prompt_terminology", "dtype": "string"}, {"name": "tests", "dtype": "string"}, {"name": "stop_tokens", "sequence": "string"}], "splits": [{"name": "test", "num_bytes": 167742, "num_examples": 158}], "download_size": 195091, "dataset_size": 167742}, {"config_name": "humaneval-rb-keep", "features": [{"name": "name", "dtype": "string"}, {"name": "language", "dtype": "string"}, {"name": "prompt", "dtype": "string"}, {"name": "doctests", "dtype": "string"}, {"name": "original", "dtype": "string"}, {"name": "prompt_terminology", "dtype": "string"}, {"name": "tests", "dtype": "string"}, {"name": "stop_tokens", "sequence": "string"}], "splits": [{"name": "test", "num_bytes": 182965, "num_examples": 161}], "download_size": 217152, "dataset_size": 182965}, {"config_name": "humaneval-rb-transform", "features": [{"name": "name", "dtype": "string"}, {"name": "language", "dtype": "string"}, {"name": "prompt", "dtype": "string"}, {"name": "doctests", "dtype": "string"}, {"name": "original", "dtype": "string"}, {"name": "prompt_terminology", "dtype": "string"}, {"name": "tests", "dtype": "string"}, {"name": "stop_tokens", "sequence": "string"}], "splits": [{"name": "test", "num_bytes": 189283, "num_examples": 161}], "download_size": 224025, "dataset_size": 189283}, {"config_name": "humaneval-rb", "features": [{"name": "name", "dtype": "string"}, {"name": "language", "dtype": "string"}, {"name": "prompt", "dtype": "string"}, {"name": "doctests", "dtype": "string"}, {"name": "original", "dtype": "string"}, {"name": "prompt_terminology", "dtype": "string"}, {"name": "tests", "dtype": "string"}, {"name": "stop_tokens", "sequence": "string"}], "splits": [{"name": "test", "num_bytes": 189423, "num_examples": 161}], "download_size": 224165, "dataset_size": 189423}, {"config_name": "humaneval-rb-remove", "features": [{"name": "name", "dtype": "string"}, {"name": "language", "dtype": "string"}, {"name": "prompt", "dtype": "string"}, {"name": "doctests", "dtype": "string"}, {"name": "original", "dtype": "string"}, {"name": "prompt_terminology", "dtype": "string"}, {"name": "tests", "dtype": "string"}, {"name": "stop_tokens", "sequence": "string"}], "splits": [{"name": "test", "num_bytes": 164517, "num_examples": 158}], "download_size": 196926, "dataset_size": 164517}, {"config_name": "humaneval-rkt-keep", "features": [{"name": "name", "dtype": "string"}, {"name": "language", "dtype": "string"}, {"name": "prompt", "dtype": "string"}, {"name": "doctests", "dtype": "string"}, {"name": "original", "dtype": "string"}, {"name": "prompt_terminology", "dtype": "string"}, {"name": "tests", "dtype": "string"}, {"name": "stop_tokens", "sequence": "string"}], "splits": [{"name": "test", "num_bytes": 185503, "num_examples": 161}], "download_size": 220012, "dataset_size": 185503}, {"config_name": "humaneval-rkt-transform", "features": [{"name": "name", "dtype": "string"}, {"name": "language", "dtype": "string"}, {"name": "prompt", "dtype": "string"}, {"name": "doctests", "dtype": "string"}, {"name": "original", "dtype": "string"}, {"name": "prompt_terminology", "dtype": "string"}, {"name": "tests", "dtype": "string"}, {"name": "stop_tokens", "sequence": "string"}], "splits": [{"name": "test", "num_bytes": 190683, "num_examples": 161}], "download_size": 225747, "dataset_size": 190683}, {"config_name": "humaneval-rkt", "features": [{"name": "name", "dtype": "string"}, {"name": "language", "dtype": "string"}, {"name": "prompt", "dtype": "string"}, {"name": "doctests", "dtype": "string"}, {"name": "original", "dtype": "string"}, {"name": "prompt_terminology", "dtype": "string"}, {"name": "tests", "dtype": "string"}, {"name": "stop_tokens", "sequence": "string"}], "splits": [{"name": "test", "num_bytes": 190500, "num_examples": 161}], "download_size": 225564, "dataset_size": 190500}, {"config_name": "humaneval-rkt-remove", "features": [{"name": "name", "dtype": "string"}, {"name": "language", "dtype": "string"}, {"name": "prompt", "dtype": "string"}, {"name": "doctests", "dtype": "string"}, {"name": "original", "dtype": "string"}, {"name": "prompt_terminology", "dtype": "string"}, {"name": "tests", "dtype": "string"}, {"name": "stop_tokens", "sequence": "string"}], "splits": [{"name": "test", "num_bytes": 166379, "num_examples": 158}], "download_size": 199104, "dataset_size": 166379}, {"config_name": "humaneval-rs-keep", "features": [{"name": "name", "dtype": "string"}, {"name": "language", "dtype": "string"}, {"name": "prompt", "dtype": "string"}, {"name": "doctests", "dtype": "string"}, {"name": "original", "dtype": "string"}, {"name": "prompt_terminology", "dtype": "string"}, {"name": "tests", "dtype": "string"}, {"name": "stop_tokens", "sequence": "string"}], "splits": [{"name": "test", "num_bytes": 178127, "num_examples": 156}], "download_size": 207540, "dataset_size": 178127}, {"config_name": "humaneval-rs-transform", "features": [{"name": "name", "dtype": "string"}, {"name": "language", "dtype": "string"}, {"name": "prompt", "dtype": "string"}, {"name": "doctests", "dtype": "string"}, {"name": "original", "dtype": "string"}, {"name": "prompt_terminology", "dtype": "string"}, {"name": "tests", "dtype": "string"}, {"name": "stop_tokens", "sequence": "string"}], "splits": [{"name": "test", "num_bytes": 189523, "num_examples": 156}], "download_size": 219491, "dataset_size": 189523}, {"config_name": "humaneval-rs", "features": [{"name": "name", "dtype": "string"}, {"name": "language", "dtype": "string"}, {"name": "prompt", "dtype": "string"}, {"name": "doctests", "dtype": "string"}, {"name": "original", "dtype": "string"}, {"name": "prompt_terminology", "dtype": "string"}, {"name": "tests", "dtype": "string"}, {"name": "stop_tokens", "sequence": "string"}], "splits": [{"name": "test", "num_bytes": 189777, "num_examples": 156}], "download_size": 219745, "dataset_size": 189777}, {"config_name": "humaneval-rs-remove", "features": [{"name": "name", "dtype": "string"}, {"name": "language", "dtype": "string"}, {"name": "prompt", "dtype": "string"}, {"name": "doctests", "dtype": "string"}, {"name": "original", "dtype": "string"}, {"name": "prompt_terminology", "dtype": "string"}, {"name": "tests", "dtype": "string"}, {"name": "stop_tokens", "sequence": "string"}], "splits": [{"name": "test", "num_bytes": 159109, "num_examples": 153}], "download_size": 186909, "dataset_size": 159109}, {"config_name": "humaneval-scala-keep", "features": [{"name": "name", "dtype": "string"}, {"name": "language", "dtype": "string"}, {"name": "prompt", "dtype": "string"}, {"name": "doctests", "dtype": "string"}, {"name": "original", "dtype": "string"}, {"name": "prompt_terminology", "dtype": "string"}, {"name": "tests", "dtype": "string"}, {"name": "stop_tokens", "sequence": "string"}], "splits": [{"name": "test", "num_bytes": 223078, "num_examples": 160}], "download_size": 253987, "dataset_size": 223078}, {"config_name": "humaneval-scala-transform", "features": [{"name": "name", "dtype": "string"}, {"name": "language", "dtype": "string"}, {"name": "prompt", "dtype": "string"}, {"name": "doctests", "dtype": "string"}, {"name": "original", "dtype": "string"}, {"name": "prompt_terminology", "dtype": "string"}, {"name": "tests", "dtype": "string"}, {"name": "stop_tokens", "sequence": "string"}], "splits": [{"name": "test", "num_bytes": 241500, "num_examples": 160}], "download_size": 272972, "dataset_size": 241500}, {"config_name": "humaneval-scala", "features": [{"name": "name", "dtype": "string"}, {"name": "language", "dtype": "string"}, {"name": "prompt", "dtype": "string"}, {"name": "doctests", "dtype": "string"}, {"name": "original", "dtype": "string"}, {"name": "prompt_terminology", "dtype": "string"}, {"name": "tests", "dtype": "string"}, {"name": "stop_tokens", "sequence": "string"}], "splits": [{"name": "test", "num_bytes": 241426, "num_examples": 160}], "download_size": 272898, "dataset_size": 241426}, {"config_name": "humaneval-scala-remove", "features": [{"name": "name", "dtype": "string"}, {"name": "language", "dtype": "string"}, {"name": "prompt", "dtype": "string"}, {"name": "doctests", "dtype": "string"}, {"name": "original", "dtype": "string"}, {"name": "prompt_terminology", "dtype": "string"}, {"name": "tests", "dtype": "string"}, {"name": "stop_tokens", "sequence": "string"}], "splits": [{"name": "test", "num_bytes": 201203, "num_examples": 157}], "download_size": 230419, "dataset_size": 201203}, {"config_name": "humaneval-sh-keep", "features": [{"name": "name", "dtype": "string"}, {"name": "language", "dtype": "string"}, {"name": "prompt", "dtype": "string"}, {"name": "doctests", "dtype": "string"}, {"name": "original", "dtype": "string"}, {"name": "prompt_terminology", "dtype": "string"}, {"name": "tests", "dtype": "string"}, {"name": "stop_tokens", "sequence": "string"}], "splits": [{"name": "test", "num_bytes": 159408, "num_examples": 158}], "download_size": 194216, "dataset_size": 159408}, {"config_name": "humaneval-sh-transform", "features": [{"name": "name", "dtype": "string"}, {"name": "language", "dtype": "string"}, {"name": "prompt", "dtype": "string"}, {"name": "doctests", "dtype": "string"}, {"name": "original", "dtype": "string"}, {"name": "prompt_terminology", "dtype": "string"}, {"name": "tests", "dtype": "string"}, {"name": "stop_tokens", "sequence": "string"}], "splits": [{"name": "test", "num_bytes": 165500, "num_examples": 158}], "download_size": 202579, "dataset_size": 165500}, {"config_name": "humaneval-sh", "features": [{"name": "name", "dtype": "string"}, {"name": "language", "dtype": "string"}, {"name": "prompt", "dtype": "string"}, {"name": "doctests", "dtype": "string"}, {"name": "original", "dtype": "string"}, {"name": "prompt_terminology", "dtype": "string"}, {"name": "tests", "dtype": "string"}, {"name": "stop_tokens", "sequence": "string"}], "splits": [{"name": "test", "num_bytes": 165469, "num_examples": 158}], "download_size": 202548, "dataset_size": 165469}, {"config_name": "humaneval-sh-remove", "features": [{"name": "name", "dtype": "string"}, {"name": "language", "dtype": "string"}, {"name": "prompt", "dtype": "string"}, {"name": "doctests", "dtype": "string"}, {"name": "original", "dtype": "string"}, {"name": "prompt_terminology", "dtype": "string"}, {"name": "tests", "dtype": "string"}, {"name": "stop_tokens", "sequence": "string"}], "splits": [{"name": "test", "num_bytes": 141650, "num_examples": 155}], "download_size": 174697, "dataset_size": 141650}, {"config_name": "humaneval-swift-keep", "features": [{"name": "name", "dtype": "string"}, {"name": "language", "dtype": "string"}, {"name": "prompt", "dtype": "string"}, {"name": "doctests", "dtype": "string"}, {"name": "original", "dtype": "string"}, {"name": "prompt_terminology", "dtype": "string"}, {"name": "tests", "dtype": "string"}, {"name": "stop_tokens", "sequence": "string"}], "splits": [{"name": "test", "num_bytes": 202764, "num_examples": 161}], "download_size": 234869, "dataset_size": 202764}, {"config_name": "humaneval-swift-transform", "features": [{"name": "name", "dtype": "string"}, {"name": "language", "dtype": "string"}, {"name": "prompt", "dtype": "string"}, {"name": "doctests", "dtype": "string"}, {"name": "original", "dtype": "string"}, {"name": "prompt_terminology", "dtype": "string"}, {"name": "tests", "dtype": "string"}, {"name": "stop_tokens", "sequence": "string"}], "splits": [{"name": "test", "num_bytes": 205708, "num_examples": 158}], "download_size": 237608, "dataset_size": 205708}, {"config_name": "humaneval-swift", "features": [{"name": "name", "dtype": "string"}, {"name": "language", "dtype": "string"}, {"name": "prompt", "dtype": "string"}, {"name": "doctests", "dtype": "string"}, {"name": "original", "dtype": "string"}, {"name": "prompt_terminology", "dtype": "string"}, {"name": "tests", "dtype": "string"}, {"name": "stop_tokens", "sequence": "string"}], "splits": [{"name": "test", "num_bytes": 205868, "num_examples": 158}], "download_size": 237768, "dataset_size": 205868}, {"config_name": "humaneval-swift-remove", "features": [{"name": "name", "dtype": "string"}, {"name": "language", "dtype": "string"}, {"name": "prompt", "dtype": "string"}, {"name": "doctests", "dtype": "string"}, {"name": "original", "dtype": "string"}, {"name": "prompt_terminology", "dtype": "string"}, {"name": "tests", "dtype": "string"}, {"name": "stop_tokens", "sequence": "string"}], "splits": [{"name": "test", "num_bytes": 182629, "num_examples": 158}], "download_size": 212995, "dataset_size": 182629}, {"config_name": "humaneval-ts-keep", "features": [{"name": "name", "dtype": "string"}, {"name": "language", "dtype": "string"}, {"name": "prompt", "dtype": "string"}, {"name": "doctests", "dtype": "string"}, {"name": "original", "dtype": "string"}, {"name": "prompt_terminology", "dtype": "string"}, {"name": "tests", "dtype": "string"}, {"name": "stop_tokens", "sequence": "string"}], "splits": [{"name": "test", "num_bytes": 182717, "num_examples": 159}], "download_size": 216543, "dataset_size": 182717}, {"config_name": "humaneval-ts-transform", "features": [{"name": "name", "dtype": "string"}, {"name": "language", "dtype": "string"}, {"name": "prompt", "dtype": "string"}, {"name": "doctests", "dtype": "string"}, {"name": "original", "dtype": "string"}, {"name": "prompt_terminology", "dtype": "string"}, {"name": "tests", "dtype": "string"}, {"name": "stop_tokens", "sequence": "string"}], "splits": [{"name": "test", "num_bytes": 186991, "num_examples": 159}], "download_size": 221377, "dataset_size": 186991}, {"config_name": "humaneval-ts", "features": [{"name": "name", "dtype": "string"}, {"name": "language", "dtype": "string"}, {"name": "prompt", "dtype": "string"}, {"name": "doctests", "dtype": "string"}, {"name": "original", "dtype": "string"}, {"name": "prompt_terminology", "dtype": "string"}, {"name": "tests", "dtype": "string"}, {"name": "stop_tokens", "sequence": "string"}], "splits": [{"name": "test", "num_bytes": 187169, "num_examples": 159}], "download_size": 221555, "dataset_size": 187169}, {"config_name": "humaneval-ts-remove", "features": [{"name": "name", "dtype": "string"}, {"name": "language", "dtype": "string"}, {"name": "prompt", "dtype": "string"}, {"name": "doctests", "dtype": "string"}, {"name": "original", "dtype": "string"}, {"name": "prompt_terminology", "dtype": "string"}, {"name": "tests", "dtype": "string"}, {"name": "stop_tokens", "sequence": "string"}], "splits": [{"name": "test", "num_bytes": 163817, "num_examples": 156}], "download_size": 195921, "dataset_size": 163817}, {"config_name": "mbpp-cpp-keep", "features": [{"name": "name", "dtype": "string"}, {"name": "language", "dtype": "string"}, {"name": "prompt", "dtype": "string"}, {"name": "doctests", "dtype": "string"}, {"name": "original", "dtype": "string"}, {"name": "prompt_terminology", "dtype": "string"}, {"name": "tests", "dtype": "string"}, {"name": "stop_tokens", "sequence": "string"}], "splits": [{"name": "test", "num_bytes": 360057, "num_examples": 397}], "download_size": 428174, "dataset_size": 360057}, {"config_name": "mbpp-cpp", "features": [{"name": "name", "dtype": "string"}, {"name": "language", "dtype": "string"}, {"name": "prompt", "dtype": "string"}, {"name": "doctests", "dtype": "string"}, {"name": "original", "dtype": "string"}, {"name": "prompt_terminology", "dtype": "string"}, {"name": "tests", "dtype": "string"}, {"name": "stop_tokens", "sequence": "string"}], "splits": [{"name": "test", "num_bytes": 362541, "num_examples": 397}], "download_size": 430658, "dataset_size": 362541}, {"config_name": "mbpp-cs-keep", "features": [{"name": "name", "dtype": "string"}, {"name": "language", "dtype": "string"}, {"name": "prompt", "dtype": "string"}, {"name": "doctests", "dtype": "string"}, {"name": "original", "dtype": "string"}, {"name": "prompt_terminology", "dtype": "string"}, {"name": "tests", "dtype": "string"}, {"name": "stop_tokens", "sequence": "string"}], "splits": [{"name": "test", "num_bytes": 416276, "num_examples": 386}], "download_size": 484875, "dataset_size": 416276}, {"config_name": "mbpp-cs", "features": [{"name": "name", "dtype": "string"}, {"name": "language", "dtype": "string"}, {"name": "prompt", "dtype": "string"}, {"name": "doctests", "dtype": "string"}, {"name": "original", "dtype": "string"}, {"name": "prompt_terminology", "dtype": "string"}, {"name": "tests", "dtype": "string"}, {"name": "stop_tokens", "sequence": "string"}], "splits": [{"name": "test", "num_bytes": 418156, "num_examples": 386}], "download_size": 486755, "dataset_size": 418156}, {"config_name": "mbpp-d-keep", "features": [{"name": "name", "dtype": "string"}, {"name": "language", "dtype": "string"}, {"name": "prompt", "dtype": "string"}, {"name": "doctests", "dtype": "string"}, {"name": "original", "dtype": "string"}, {"name": "prompt_terminology", "dtype": "string"}, {"name": "tests", "dtype": "string"}, {"name": "stop_tokens", "sequence": "string"}], "splits": [{"name": "test", "num_bytes": 232820, "num_examples": 358}], "download_size": 303807, "dataset_size": 232820}, {"config_name": "mbpp-d", "features": [{"name": "name", "dtype": "string"}, {"name": "language", "dtype": "string"}, {"name": "prompt", "dtype": "string"}, {"name": "doctests", "dtype": "string"}, {"name": "original", "dtype": "string"}, {"name": "prompt_terminology", "dtype": "string"}, {"name": "tests", "dtype": "string"}, {"name": "stop_tokens", "sequence": "string"}], "splits": [{"name": "test", "num_bytes": 234776, "num_examples": 358}], "download_size": 305763, "dataset_size": 234776}, {"config_name": "mbpp-go-keep", "features": [{"name": "name", "dtype": "string"}, {"name": "language", "dtype": "string"}, {"name": "prompt", "dtype": "string"}, {"name": "doctests", "dtype": "string"}, {"name": "original", "dtype": "string"}, {"name": "prompt_terminology", "dtype": "string"}, {"name": "tests", "dtype": "string"}, {"name": "stop_tokens", "sequence": "string"}], "splits": [{"name": "test", "num_bytes": 399157, "num_examples": 374}], "download_size": 486803, "dataset_size": 399157}, {"config_name": "mbpp-go", "features": [{"name": "name", "dtype": "string"}, {"name": "language", "dtype": "string"}, {"name": "prompt", "dtype": "string"}, {"name": "doctests", "dtype": "string"}, {"name": "original", "dtype": "string"}, {"name": "prompt_terminology", "dtype": "string"}, {"name": "tests", "dtype": "string"}, {"name": "stop_tokens", "sequence": "string"}], "splits": [{"name": "test", "num_bytes": 400841, "num_examples": 374}], "download_size": 488487, "dataset_size": 400841}, {"config_name": "mbpp-java-keep", "features": [{"name": "name", "dtype": "string"}, {"name": "language", "dtype": "string"}, {"name": "prompt", "dtype": "string"}, {"name": "doctests", "dtype": "string"}, {"name": "original", "dtype": "string"}, {"name": "prompt_terminology", "dtype": "string"}, {"name": "tests", "dtype": "string"}, {"name": "stop_tokens", "sequence": "string"}], "splits": [{"name": "test", "num_bytes": 419406, "num_examples": 386}], "download_size": 488005, "dataset_size": 419406}, {"config_name": "mbpp-java", "features": [{"name": "name", "dtype": "string"}, {"name": "language", "dtype": "string"}, {"name": "prompt", "dtype": "string"}, {"name": "doctests", "dtype": "string"}, {"name": "original", "dtype": "string"}, {"name": "prompt_terminology", "dtype": "string"}, {"name": "tests", "dtype": "string"}, {"name": "stop_tokens", "sequence": "string"}], "splits": [{"name": "test", "num_bytes": 423652, "num_examples": 386}], "download_size": 492251, "dataset_size": 423652}, {"config_name": "mbpp-jl-keep", "features": [{"name": "name", "dtype": "string"}, {"name": "language", "dtype": "string"}, {"name": "prompt", "dtype": "string"}, {"name": "doctests", "dtype": "string"}, {"name": "original", "dtype": "string"}, {"name": "prompt_terminology", "dtype": "string"}, {"name": "tests", "dtype": "string"}, {"name": "stop_tokens", "sequence": "string"}], "splits": [{"name": "test", "num_bytes": 228259, "num_examples": 390}], "download_size": 305322, "dataset_size": 228259}, {"config_name": "mbpp-jl", "features": [{"name": "name", "dtype": "string"}, {"name": "language", "dtype": "string"}, {"name": "prompt", "dtype": "string"}, {"name": "doctests", "dtype": "string"}, {"name": "original", "dtype": "string"}, {"name": "prompt_terminology", "dtype": "string"}, {"name": "tests", "dtype": "string"}, {"name": "stop_tokens", "sequence": "string"}], "splits": [{"name": "test", "num_bytes": 230672, "num_examples": 390}], "download_size": 307735, "dataset_size": 230672}, {"config_name": "mbpp-js-keep", "features": [{"name": "name", "dtype": "string"}, {"name": "language", "dtype": "string"}, {"name": "prompt", "dtype": "string"}, {"name": "doctests", "dtype": "string"}, {"name": "original", "dtype": "string"}, {"name": "prompt_terminology", "dtype": "string"}, {"name": "tests", "dtype": "string"}, {"name": "stop_tokens", "sequence": "string"}], "splits": [{"name": "test", "num_bytes": 256499, "num_examples": 397}], "download_size": 333225, "dataset_size": 256499}, {"config_name": "mbpp-js", "features": [{"name": "name", "dtype": "string"}, {"name": "language", "dtype": "string"}, {"name": "prompt", "dtype": "string"}, {"name": "doctests", "dtype": "string"}, {"name": "original", "dtype": "string"}, {"name": "prompt_terminology", "dtype": "string"}, {"name": "tests", "dtype": "string"}, {"name": "stop_tokens", "sequence": "string"}], "splits": [{"name": "test", "num_bytes": 258734, "num_examples": 397}], "download_size": 335460, "dataset_size": 258734}, {"config_name": "mbpp-lua-keep", "features": [{"name": "name", "dtype": "string"}, {"name": "language", "dtype": "string"}, {"name": "prompt", "dtype": "string"}, {"name": "doctests", "dtype": "string"}, {"name": "original", "dtype": "string"}, {"name": "prompt_terminology", "dtype": "string"}, {"name": "tests", "dtype": "string"}, {"name": "stop_tokens", "sequence": "string"}], "splits": [{"name": "test", "num_bytes": 262378, "num_examples": 397}], "download_size": 335520, "dataset_size": 262378}, {"config_name": "mbpp-lua", "features": [{"name": "name", "dtype": "string"}, {"name": "language", "dtype": "string"}, {"name": "prompt", "dtype": "string"}, {"name": "doctests", "dtype": "string"}, {"name": "original", "dtype": "string"}, {"name": "prompt_terminology", "dtype": "string"}, {"name": "tests", "dtype": "string"}, {"name": "stop_tokens", "sequence": "string"}], "splits": [{"name": "test", "num_bytes": 264635, "num_examples": 397}], "download_size": 337777, "dataset_size": 264635}, {"config_name": "mbpp-php-keep", "features": [{"name": "name", "dtype": "string"}, {"name": "language", "dtype": "string"}, {"name": "prompt", "dtype": "string"}, {"name": "doctests", "dtype": "string"}, {"name": "original", "dtype": "string"}, {"name": "prompt_terminology", "dtype": "string"}, {"name": "tests", "dtype": "string"}, {"name": "stop_tokens", "sequence": "string"}], "splits": [{"name": "test", "num_bytes": 308918, "num_examples": 397}], "download_size": 388541, "dataset_size": 308918}, {"config_name": "mbpp-php", "features": [{"name": "name", "dtype": "string"}, {"name": "language", "dtype": "string"}, {"name": "prompt", "dtype": "string"}, {"name": "doctests", "dtype": "string"}, {"name": "original", "dtype": "string"}, {"name": "prompt_terminology", "dtype": "string"}, {"name": "tests", "dtype": "string"}, {"name": "stop_tokens", "sequence": "string"}], "splits": [{"name": "test", "num_bytes": 311263, "num_examples": 397}], "download_size": 390886, "dataset_size": 311263}, {"config_name": "mbpp-pl-keep", "features": [{"name": "name", "dtype": "string"}, {"name": "language", "dtype": "string"}, {"name": "prompt", "dtype": "string"}, {"name": "doctests", "dtype": "string"}, {"name": "original", "dtype": "string"}, {"name": "prompt_terminology", "dtype": "string"}, {"name": "tests", "dtype": "string"}, {"name": "stop_tokens", "sequence": "string"}], "splits": [{"name": "test", "num_bytes": 321045, "num_examples": 396}], "download_size": 402353, "dataset_size": 321045}, {"config_name": "mbpp-pl", "features": [{"name": "name", "dtype": "string"}, {"name": "language", "dtype": "string"}, {"name": "prompt", "dtype": "string"}, {"name": "doctests", "dtype": "string"}, {"name": "original", "dtype": "string"}, {"name": "prompt_terminology", "dtype": "string"}, {"name": "tests", "dtype": "string"}, {"name": "stop_tokens", "sequence": "string"}], "splits": [{"name": "test", "num_bytes": 323224, "num_examples": 396}], "download_size": 404532, "dataset_size": 323224}, {"config_name": "mbpp-py-keep", "features": [{"name": "name", "dtype": "string"}, {"name": "language", "dtype": "string"}, {"name": "prompt", "dtype": "string"}, {"name": "doctests", "dtype": "string"}, {"name": "original", "dtype": "string"}, {"name": "prompt_terminology", "dtype": "string"}, {"name": "tests", "dtype": "string"}, {"name": "stop_tokens", "sequence": "string"}], "splits": [{"name": "test", "num_bytes": 253037, "num_examples": 397}], "download_size": 330230, "dataset_size": 253037}, {"config_name": "mbpp-py", "features": [{"name": "name", "dtype": "string"}, {"name": "language", "dtype": "string"}, {"name": "prompt", "dtype": "string"}, {"name": "doctests", "dtype": "string"}, {"name": "original", "dtype": "string"}, {"name": "prompt_terminology", "dtype": "string"}, {"name": "tests", "dtype": "string"}, {"name": "stop_tokens", "sequence": "string"}], "splits": [{"name": "test", "num_bytes": 255022, "num_examples": 397}], "download_size": 332215, "dataset_size": 255022}, {"config_name": "mbpp-r-keep", "features": [{"name": "name", "dtype": "string"}, {"name": "language", "dtype": "string"}, {"name": "prompt", "dtype": "string"}, {"name": "doctests", "dtype": "string"}, {"name": "original", "dtype": "string"}, {"name": "prompt_terminology", "dtype": "string"}, {"name": "tests", "dtype": "string"}, {"name": "stop_tokens", "sequence": "string"}], "splits": [{"name": "test", "num_bytes": 257698, "num_examples": 397}], "download_size": 323297, "dataset_size": 257698}, {"config_name": "mbpp-r", "features": [{"name": "name", "dtype": "string"}, {"name": "language", "dtype": "string"}, {"name": "prompt", "dtype": "string"}, {"name": "doctests", "dtype": "string"}, {"name": "original", "dtype": "string"}, {"name": "prompt_terminology", "dtype": "string"}, {"name": "tests", "dtype": "string"}, {"name": "stop_tokens", "sequence": "string"}], "splits": [{"name": "test", "num_bytes": 259514, "num_examples": 397}], "download_size": 325113, "dataset_size": 259514}, {"config_name": "mbpp-rb-keep", "features": [{"name": "name", "dtype": "string"}, {"name": "language", "dtype": "string"}, {"name": "prompt", "dtype": "string"}, {"name": "doctests", "dtype": "string"}, {"name": "original", "dtype": "string"}, {"name": "prompt_terminology", "dtype": "string"}, {"name": "tests", "dtype": "string"}, {"name": "stop_tokens", "sequence": "string"}], "splits": [{"name": "test", "num_bytes": 266702, "num_examples": 397}], "download_size": 343428, "dataset_size": 266702}, {"config_name": "mbpp-rb", "features": [{"name": "name", "dtype": "string"}, {"name": "language", "dtype": "string"}, {"name": "prompt", "dtype": "string"}, {"name": "doctests", "dtype": "string"}, {"name": "original", "dtype": "string"}, {"name": "prompt_terminology", "dtype": "string"}, {"name": "tests", "dtype": "string"}, {"name": "stop_tokens", "sequence": "string"}], "splits": [{"name": "test", "num_bytes": 268881, "num_examples": 397}], "download_size": 345607, "dataset_size": 268881}, {"config_name": "mbpp-rkt-keep", "features": [{"name": "name", "dtype": "string"}, {"name": "language", "dtype": "string"}, {"name": "prompt", "dtype": "string"}, {"name": "doctests", "dtype": "string"}, {"name": "original", "dtype": "string"}, {"name": "prompt_terminology", "dtype": "string"}, {"name": "tests", "dtype": "string"}, {"name": "stop_tokens", "sequence": "string"}], "splits": [{"name": "test", "num_bytes": 269019, "num_examples": 397}], "download_size": 346539, "dataset_size": 269019}, {"config_name": "mbpp-rkt", "features": [{"name": "name", "dtype": "string"}, {"name": "language", "dtype": "string"}, {"name": "prompt", "dtype": "string"}, {"name": "doctests", "dtype": "string"}, {"name": "original", "dtype": "string"}, {"name": "prompt_terminology", "dtype": "string"}, {"name": "tests", "dtype": "string"}, {"name": "stop_tokens", "sequence": "string"}], "splits": [{"name": "test", "num_bytes": 270933, "num_examples": 397}], "download_size": 348453, "dataset_size": 270933}, {"config_name": "mbpp-rs-keep", "features": [{"name": "name", "dtype": "string"}, {"name": "language", "dtype": "string"}, {"name": "prompt", "dtype": "string"}, {"name": "doctests", "dtype": "string"}, {"name": "original", "dtype": "string"}, {"name": "prompt_terminology", "dtype": "string"}, {"name": "tests", "dtype": "string"}, {"name": "stop_tokens", "sequence": "string"}], "splits": [{"name": "test", "num_bytes": 218020, "num_examples": 354}], "download_size": 277268, "dataset_size": 218020}, {"config_name": "mbpp-rs", "features": [{"name": "name", "dtype": "string"}, {"name": "language", "dtype": "string"}, {"name": "prompt", "dtype": "string"}, {"name": "doctests", "dtype": "string"}, {"name": "original", "dtype": "string"}, {"name": "prompt_terminology", "dtype": "string"}, {"name": "tests", "dtype": "string"}, {"name": "stop_tokens", "sequence": "string"}], "splits": [{"name": "test", "num_bytes": 220113, "num_examples": 354}], "download_size": 279361, "dataset_size": 220113}, {"config_name": "mbpp-scala-keep", "features": [{"name": "name", "dtype": "string"}, {"name": "language", "dtype": "string"}, {"name": "prompt", "dtype": "string"}, {"name": "doctests", "dtype": "string"}, {"name": "original", "dtype": "string"}, {"name": "prompt_terminology", "dtype": "string"}, {"name": "tests", "dtype": "string"}, {"name": "stop_tokens", "sequence": "string"}], "splits": [{"name": "test", "num_bytes": 330435, "num_examples": 396}], "download_size": 399451, "dataset_size": 330435}, {"config_name": "mbpp-scala", "features": [{"name": "name", "dtype": "string"}, {"name": "language", "dtype": "string"}, {"name": "prompt", "dtype": "string"}, {"name": "doctests", "dtype": "string"}, {"name": "original", "dtype": "string"}, {"name": "prompt_terminology", "dtype": "string"}, {"name": "tests", "dtype": "string"}, {"name": "stop_tokens", "sequence": "string"}], "splits": [{"name": "test", "num_bytes": 332677, "num_examples": 396}], "download_size": 401693, "dataset_size": 332677}, {"config_name": "mbpp-sh-keep", "features": [{"name": "name", "dtype": "string"}, {"name": "language", "dtype": "string"}, {"name": "prompt", "dtype": "string"}, {"name": "doctests", "dtype": "string"}, {"name": "original", "dtype": "string"}, {"name": "prompt_terminology", "dtype": "string"}, {"name": "tests", "dtype": "string"}, {"name": "stop_tokens", "sequence": "string"}], "splits": [{"name": "test", "num_bytes": 217246, "num_examples": 382}], "download_size": 289241, "dataset_size": 217246}, {"config_name": "mbpp-sh", "features": [{"name": "name", "dtype": "string"}, {"name": "language", "dtype": "string"}, {"name": "prompt", "dtype": "string"}, {"name": "doctests", "dtype": "string"}, {"name": "original", "dtype": "string"}, {"name": "prompt_terminology", "dtype": "string"}, {"name": "tests", "dtype": "string"}, {"name": "stop_tokens", "sequence": "string"}], "splits": [{"name": "test", "num_bytes": 219035, "num_examples": 382}], "download_size": 291030, "dataset_size": 219035}, {"config_name": "mbpp-swift-keep", "features": [{"name": "name", "dtype": "string"}, {"name": "language", "dtype": "string"}, {"name": "prompt", "dtype": "string"}, {"name": "doctests", "dtype": "string"}, {"name": "original", "dtype": "string"}, {"name": "prompt_terminology", "dtype": "string"}, {"name": "tests", "dtype": "string"}, {"name": "stop_tokens", "sequence": "string"}], "splits": [{"name": "test", "num_bytes": 317271, "num_examples": 396}], "download_size": 388726, "dataset_size": 317271}, {"config_name": "mbpp-swift", "features": [{"name": "name", "dtype": "string"}, {"name": "language", "dtype": "string"}, {"name": "prompt", "dtype": "string"}, {"name": "doctests", "dtype": "string"}, {"name": "original", "dtype": "string"}, {"name": "prompt_terminology", "dtype": "string"}, {"name": "tests", "dtype": "string"}, {"name": "stop_tokens", "sequence": "string"}], "splits": [{"name": "test", "num_bytes": 319946, "num_examples": 396}], "download_size": 391401, "dataset_size": 319946}, {"config_name": "mbpp-ts-keep", "features": [{"name": "name", "dtype": "string"}, {"name": "language", "dtype": "string"}, {"name": "prompt", "dtype": "string"}, {"name": "doctests", "dtype": "string"}, {"name": "original", "dtype": "string"}, {"name": "prompt_terminology", "dtype": "string"}, {"name": "tests", "dtype": "string"}, {"name": "stop_tokens", "sequence": "string"}], "splits": [{"name": "test", "num_bytes": 265973, "num_examples": 390}], "download_size": 341007, "dataset_size": 265973}, {"config_name": "mbpp-ts", "features": [{"name": "name", "dtype": "string"}, {"name": "language", "dtype": "string"}, {"name": "prompt", "dtype": "string"}, {"name": "doctests", "dtype": "string"}, {"name": "original", "dtype": "string"}, {"name": "prompt_terminology", "dtype": "string"}, {"name": "tests", "dtype": "string"}, {"name": "stop_tokens", "sequence": "string"}], "splits": [{"name": "test", "num_bytes": 268179, "num_examples": 390}], "download_size": 343213, "dataset_size": 268179}]} | 2023-06-15T23:08:57+00:00 | [] | [
"en"
] | TAGS
#annotations_creators-machine-generated #language_creators-machine-generated #language_creators-expert-generated #multilinguality-monolingual #size_categories-1K<n<10K #source_datasets-original #source_datasets-extended|openai_humaneval #source_datasets-extended|mbpp #language-English #license-mit #region-us
|
# Dataset Card for MultiPL-E
## Dataset Description
- Homepage: URL
- Repository: URL
- Paper: URL
- Point of Contact: carolyn.anderson@URL, mfeldman@URL, a.guha@URL
## Dataset Summary
MultiPL-E is a dataset for evaluating large language models for code
generation that supports 18 programming languages. It takes the OpenAI
"HumanEval" and the MBPP Python benchmarks and uses little compilers to
translate them to other languages. It is easy to add support for new languages
and benchmarks.
## Subsets
For most purposes, you should use the variations called *SRCDATA-LANG*, where
*SRCDATA* is either "humaneval" or "mbpp" and *LANG* is one of the supported
languages. We use the canonical file extension for each language to identify
the language, e.g., "py" for Python, "cpp" for C++, "lua" for Lua, and so on.
We also provide a few other variations:
- *SRCDATA-LANG-keep* is the same as *SRCDATA-LANG*, but the text of the prompt
is totally unchanged. If the original prompt had Python doctests, they remain
as Python instead of being translated to *LANG*. If the original prompt had
Python-specific terminology, e.g., "list", it remains "list", instead of
being translated, e.g., to "vector" for C++.
- *SRCDATA-LANG-transform* transforms the doctests to *LANG* but leaves
the natural language text of the prompt unchanged.
- *SRCDATA-LANG-removed* removes the doctests from the prompt.
Note that MBPP does not have any doctests, so the "removed" and "transform"
variations are not available for MBPP.
## Example
The following script uses the Salesforce/codegen model to generate Lua
and MultiPL-E to produce a script with unit tests for luaunit.
| [
"# Dataset Card for MultiPL-E",
"## Dataset Description\n\n- Homepage: URL\n- Repository: URL\n- Paper: URL\n- Point of Contact: carolyn.anderson@URL, mfeldman@URL, a.guha@URL",
"## Dataset Summary\n\nMultiPL-E is a dataset for evaluating large language models for code\ngeneration that supports 18 programming languages. It takes the OpenAI \n\"HumanEval\" and the MBPP Python benchmarks and uses little compilers to\ntranslate them to other languages. It is easy to add support for new languages \nand benchmarks.",
"## Subsets\n\nFor most purposes, you should use the variations called *SRCDATA-LANG*, where\n*SRCDATA* is either \"humaneval\" or \"mbpp\" and *LANG* is one of the supported\nlanguages. We use the canonical file extension for each language to identify\nthe language, e.g., \"py\" for Python, \"cpp\" for C++, \"lua\" for Lua, and so on.\n\nWe also provide a few other variations:\n\n- *SRCDATA-LANG-keep* is the same as *SRCDATA-LANG*, but the text of the prompt\n is totally unchanged. If the original prompt had Python doctests, they remain\n as Python instead of being translated to *LANG*. If the original prompt had \n Python-specific terminology, e.g., \"list\", it remains \"list\", instead of \n being translated, e.g., to \"vector\" for C++.\n\n- *SRCDATA-LANG-transform* transforms the doctests to *LANG* but leaves\n the natural language text of the prompt unchanged.\n\n- *SRCDATA-LANG-removed* removes the doctests from the prompt.\n\nNote that MBPP does not have any doctests, so the \"removed\" and \"transform\"\nvariations are not available for MBPP.",
"## Example\n\nThe following script uses the Salesforce/codegen model to generate Lua\nand MultiPL-E to produce a script with unit tests for luaunit."
] | [
"TAGS\n#annotations_creators-machine-generated #language_creators-machine-generated #language_creators-expert-generated #multilinguality-monolingual #size_categories-1K<n<10K #source_datasets-original #source_datasets-extended|openai_humaneval #source_datasets-extended|mbpp #language-English #license-mit #region-us \n",
"# Dataset Card for MultiPL-E",
"## Dataset Description\n\n- Homepage: URL\n- Repository: URL\n- Paper: URL\n- Point of Contact: carolyn.anderson@URL, mfeldman@URL, a.guha@URL",
"## Dataset Summary\n\nMultiPL-E is a dataset for evaluating large language models for code\ngeneration that supports 18 programming languages. It takes the OpenAI \n\"HumanEval\" and the MBPP Python benchmarks and uses little compilers to\ntranslate them to other languages. It is easy to add support for new languages \nand benchmarks.",
"## Subsets\n\nFor most purposes, you should use the variations called *SRCDATA-LANG*, where\n*SRCDATA* is either \"humaneval\" or \"mbpp\" and *LANG* is one of the supported\nlanguages. We use the canonical file extension for each language to identify\nthe language, e.g., \"py\" for Python, \"cpp\" for C++, \"lua\" for Lua, and so on.\n\nWe also provide a few other variations:\n\n- *SRCDATA-LANG-keep* is the same as *SRCDATA-LANG*, but the text of the prompt\n is totally unchanged. If the original prompt had Python doctests, they remain\n as Python instead of being translated to *LANG*. If the original prompt had \n Python-specific terminology, e.g., \"list\", it remains \"list\", instead of \n being translated, e.g., to \"vector\" for C++.\n\n- *SRCDATA-LANG-transform* transforms the doctests to *LANG* but leaves\n the natural language text of the prompt unchanged.\n\n- *SRCDATA-LANG-removed* removes the doctests from the prompt.\n\nNote that MBPP does not have any doctests, so the \"removed\" and \"transform\"\nvariations are not available for MBPP.",
"## Example\n\nThe following script uses the Salesforce/codegen model to generate Lua\nand MultiPL-E to produce a script with unit tests for luaunit."
] | [
108,
9,
43,
78,
302,
36
] | [
"passage: TAGS\n#annotations_creators-machine-generated #language_creators-machine-generated #language_creators-expert-generated #multilinguality-monolingual #size_categories-1K<n<10K #source_datasets-original #source_datasets-extended|openai_humaneval #source_datasets-extended|mbpp #language-English #license-mit #region-us \n# Dataset Card for MultiPL-E## Dataset Description\n\n- Homepage: URL\n- Repository: URL\n- Paper: URL\n- Point of Contact: carolyn.anderson@URL, mfeldman@URL, a.guha@URL## Dataset Summary\n\nMultiPL-E is a dataset for evaluating large language models for code\ngeneration that supports 18 programming languages. It takes the OpenAI \n\"HumanEval\" and the MBPP Python benchmarks and uses little compilers to\ntranslate them to other languages. It is easy to add support for new languages \nand benchmarks."
] |
5c9e80ea311d9ab56264265b77ed06a1d32bcef0 |
# Cannabis Licenses
<!-- FIXME:
<div align="center" style="text-align:center; margin-top:1rem; margin-bottom: 1rem;">
<img style="max-height:365px;width:100%;max-width:720px;" alt="" src="analysis/figures/cannabis-licenses-map.png">
</div> -->
## Table of Contents
- [Table of Contents](#table-of-contents)
- [Dataset Description](#dataset-description)
- [Dataset Summary](#dataset-summary)
- [Dataset Structure](#dataset-structure)
- [Data Instances](#data-instances)
- [Data Fields](#data-fields)
- [Data Splits](#data-splits)
- [Dataset Creation](#dataset-creation)
- [Curation Rationale](#curation-rationale)
- [Source Data](#source-data)
- [Data Collection and Normalization](#data-collection-and-normalization)
- [Personal and Sensitive Information](#personal-and-sensitive-information)
- [Considerations for Using the Data](#considerations-for-using-the-data)
- [Social Impact of Dataset](#social-impact-of-dataset)
- [Discussion of Biases](#discussion-of-biases)
- [Other Known Limitations](#other-known-limitations)
- [Additional Information](#additional-information)
- [Dataset Curators](#dataset-curators)
- [License](#license)
- [Citation](#citation)
- [Contributions](#contributions)
## Dataset Description
- **Homepage:** <https://github.com/cannlytics/cannlytics>
- **Repository:** <https://huggingface.co/datasets/cannlytics/cannabis_licenses>
- **Point of Contact:** <[email protected]>
### Dataset Summary
**Cannabis Licenses** is a collection of cannabis license data for each state with permitted adult-use cannabis. The dataset also includes a sub-dataset, `all`, that includes all licenses.
## Dataset Structure
The dataset is partitioned into 18 subsets for each state and the aggregate.
| State | Code | Status |
|-------|------|--------|
| [All](https://huggingface.co/datasets/cannlytics/cannabis_licenses/tree/main/data/all) | `all` | โ
|
| [Alaska](https://huggingface.co/datasets/cannlytics/cannabis_licenses/tree/main/data/ak) | `ak` | โ
|
| [Arizona](https://huggingface.co/datasets/cannlytics/cannabis_licenses/tree/main/data/az) | `az` | โ
|
| [California](https://huggingface.co/datasets/cannlytics/cannabis_licenses/tree/main/data/ca) | `ca` | โ
|
| [Colorado](https://huggingface.co/datasets/cannlytics/cannabis_licenses/tree/main/data/co) | `co` | โ
|
| [Connecticut](https://huggingface.co/datasets/cannlytics/cannabis_licenses/tree/main/data/ct) | `ct` | โ
|
| [Delaware](https://huggingface.co/datasets/cannlytics/cannabis_licenses/tree/main/data/de) | `md` | โ
|
| [Illinois](https://huggingface.co/datasets/cannlytics/cannabis_licenses/tree/main/data/il) | `il` | โ
|
| [Maine](https://huggingface.co/datasets/cannlytics/cannabis_licenses/tree/main/data/me) | `me` | โ
|
| [Maryland](https://huggingface.co/datasets/cannlytics/cannabis_licenses/tree/main/data/md) | `md` | โ
|
| [Massachusetts](https://huggingface.co/datasets/cannlytics/cannabis_licenses/tree/main/data/ma) | `ma` | โ
|
| [Michigan](https://huggingface.co/datasets/cannlytics/cannabis_licenses/tree/main/data/mi) | `mi` | โ
|
| [Missouri](https://huggingface.co/datasets/cannlytics/cannabis_licenses/tree/main/data/mo) | `mo` | โ
|
| [Montana](https://huggingface.co/datasets/cannlytics/cannabis_licenses/tree/main/data/mt) | `mt` | โ
|
| [Nevada](https://huggingface.co/datasets/cannlytics/cannabis_licenses/tree/main/data/nv) | `nv` | โ
|
| [New Jersey](https://huggingface.co/datasets/cannlytics/cannabis_licenses/tree/main/data/nj) | `nj` | โ
|
| [New Mexico](https://huggingface.co/datasets/cannlytics/cannabis_licenses/tree/main/data/nm) | `nm` | โ
|
| [New York](https://huggingface.co/datasets/cannlytics/cannabis_licenses/tree/main/data/ny) | `ny` | โ
|
| [Oregon](https://huggingface.co/datasets/cannlytics/cannabis_licenses/tree/main/data/or) | `or` | โ
|
| [Rhode Island](https://huggingface.co/datasets/cannlytics/cannabis_licenses/tree/main/data/ri) | `ri` | โ
|
| [Vermont](https://huggingface.co/datasets/cannlytics/cannabis_licenses/tree/main/data/vt) | `vt` | โ
|
| Virginia | `va` | โณ Expected 2024 |
| [Washington](https://huggingface.co/datasets/cannlytics/cannabis_licenses/tree/main/data/wa) | `wa` | โ
|
The following states have issued medical cannabis licenses, but are not (yet) included in the dataset:
- Alabama
- Arkansas
- District of Columbia (D.C.)
- Florida
- Kentucky (2024)
- Louisiana
- Minnesota
- Mississippi
- New Hampshire
- North Dakota
- Ohio
- Oklahoma
- Pennsylvania
- South Dakota
- Utah
- West Virginia
### Data Instances
You can load the licenses for each state. For example:
```py
from datasets import load_dataset
# Get the licenses for a specific state.
dataset = load_dataset('cannlytics/cannabis_licenses', 'all')
data = dataset['data']
```
### Data Fields
Below is a non-exhaustive list of fields, used to standardize the various data that are encountered, that you may expect to find for each observation.
| Field | Example | Description |
|-------|-----|-------------|
| `id` | `"1046"` | A state-unique ID for the license. |
| `license_number` | `"C10-0000423-LIC"` | A unique license number. |
| `license_status` | `"Active"` | The status of the license. Only licenses that are active are included. |
| `license_status_date` | `"2022-04-20T00:00"` | The date the status was assigned, an ISO-formatted date if present. |
| `license_term` | `"Provisional"` | The term for the license. |
| `license_type` | `"Commercial - Retailer"` | The type of business license. |
| `license_designation` | `"Adult-Use and Medicinal"` | A state-specific classification for the license. |
| `issue_date` | `"2019-07-15T00:00:00"` | An issue date for the license, an ISO-formatted date if present. |
| `expiration_date` | `"2023-07-14T00:00:00"` | An expiration date for the license, an ISO-formatted date if present. |
| `licensing_authority_id` | `"BCC"` | A unique ID for the state licensing authority. |
| `licensing_authority` | `"Bureau of Cannabis Control (BCC)"` | The state licensing authority. |
| `business_legal_name` | `"Movocan"` | The legal name of the business that owns the license. |
| `business_dba_name` | `"Movocan"` | The name the license is doing business as. |
| `business_owner_name` | `"redacted"` | The name of the owner of the license. |
| `business_structure` | `"Corporation"` | The structure of the business that owns the license. |
| `activity` | `"Pending Inspection"` | Any relevant license activity. |
| `premise_street_address` | `"1632 Gateway Rd"` | The street address of the business. |
| `premise_city` | `"Calexico"` | The city of the business. |
| `premise_state` | `"CA"` | The state abbreviation of the business. |
| `premise_county` | `"Imperial"` | The county of the business. |
| `premise_zip_code` | `"92231"` | The zip code of the business. |
| `business_email` | `"[email protected]"` | The business email of the license. |
| `business_phone` | `"(555) 555-5555"` | The business phone of the license. |
| `business_website` | `"cannlytics.com"` | The business website of the license. |
| `parcel_number` | `"A42"` | An ID for the business location. |
| `premise_latitude` | `32.69035693` | The latitude of the business. |
| `premise_longitude` | `-115.38987552` | The longitude of the business. |
| `data_refreshed_date` | `"2022-09-21T12:16:33.3866667"` | An ISO-formatted time when the license data was updated. |
### Data Splits
The data is split into subsets by state. You can retrieve all licenses by requesting the `all` subset.
```py
from datasets import load_dataset
# Get all cannabis licenses.
dataset = load_dataset('cannlytics/cannabis_licenses', 'all')
data = dataset['data']
```
## Dataset Creation
### Curation Rationale
Data about organizations operating in the cannabis industry for each state is valuable for research.
### Source Data
| State | Data Source URL |
|-------|-----------------|
| Alaska | <https://www.commerce.alaska.gov/abc/marijuana/Home/licensesearch> |
| Arizona | <https://azcarecheck.azdhs.gov/s/?licenseType=null> |
| California | <https://search.cannabis.ca.gov/> |
| Colorado | <https://sbg.colorado.gov/med/licensed-facilities> |
| Connecticut | <https://portal.ct.gov/DCP/Medical-Marijuana-Program/Connecticut-Medical-Marijuana-Dispensary-Facilities> |
| Delaware | <https://dhss.delaware.gov/dhss/dph/hsp/medmarcc.html> |
| Illinois | <https://www.idfpr.com/LicenseLookup/AdultUseDispensaries.pdf> |
| Maine | <https://www.maine.gov/dafs/ocp/open-data/adult-use> |
| Maryland | <https://mmcc.maryland.gov/Pages/Dispensaries.aspx> |
| Massachusetts | <https://masscannabiscontrol.com/open-data/data-catalog/> |
| Michigan | <https://michigan.maps.arcgis.com/apps/webappviewer/index.html?id=cd5a1a76daaf470b823a382691c0ff60> |
| Missouri | <https://health.mo.gov/safety/cannabis/licensed-facilities.php> |
| Montana | <https://mtrevenue.gov/cannabis/#CannabisLicenses> |
| Nevada | <https://ccb.nv.gov/list-of-licensees/> |
| New Jersey | <https://data.nj.gov/stories/s/ggm4-mprw> |
| New Mexico | <https://nmrldlpi.force.com/bcd/s/public-search-license?division=CCD&language=en_US> |
| New York | <https://cannabis.ny.gov/licensing> |
| Oregon | <https://www.oregon.gov/olcc/marijuana/pages/recreational-marijuana-licensing.aspx> |
| Rhode Island | <https://dbr.ri.gov/office-cannabis-regulation/compassion-centers/licensed-compassion-centers> |
| Vermont | <https://ccb.vermont.gov/licenses> |
| Washington | <https://lcb.wa.gov/records/frequently-requested-lists> |
### Data Collection and Normalization
In the `algorithms` directory, you can find the algorithms used for data collection. You can use these algorithms to recreate the dataset. First, you will need to clone the repository:
```
git clone https://huggingface.co/datasets/cannlytics/cannabis_licenses
```
You can then install the algorithm Python (3.9+) requirements:
```
cd cannabis_licenses
pip install -r requirements.txt
```
Then you can run all of the data-collection algorithms:
```
python algorithms/main.py
```
Or you can run each algorithm individually. For example:
```
python algorithms/get_licenses_ny.py
```
### Personal and Sensitive Information
This dataset includes names of individuals, public addresses, and contact information for cannabis licensees. It is important to take care to use these data points in a legal manner.
## Considerations for Using the Data
### Social Impact of Dataset
Arguably, there is substantial social impact that could result from the study of permitted adult-use cannabis, therefore, researchers and data consumers alike should take the utmost care in the use of this dataset.
### Discussion of Biases
Cannlytics is a for-profit data and analytics company that primarily serves cannabis businesses. The data are not randomly collected and thus sampling bias should be taken into consideration.
### Other Known Limitations
The data is for adult-use cannabis licenses. It would be valuable to include medical cannabis licenses too.
## Additional Information
### Dataset Curators
Curated by [๐ฅCannlytics](https://cannlytics.com)<br>
<[email protected]>
### License
```
Copyright (c) 2022-2023 Cannlytics and the Cannabis Data Science Team
The files associated with this dataset are licensed under a
Creative Commons Attribution 4.0 International license.
You can share, copy and modify this dataset so long as you give
appropriate credit, provide a link to the CC BY license, and
indicate if changes were made, but you may not do so in a way
that suggests the rights holder has endorsed you or your use of
the dataset. Note that further permission may be required for
any content within the dataset that is identified as belonging
to a third party.
```
### Citation
Please cite the following if you use the code examples in your research:
```bibtex
@misc{cannlytics2023,
title={Cannabis Data Science},
author={Skeate, Keegan and O'Sullivan-Sutherland, Candace},
journal={https://github.com/cannlytics/cannabis-data-science},
year={2023}
}
```
### Contributions
Thanks to [๐ฅCannlytics](https://cannlytics.com), [@candy-o](https://github.com/candy-o), [@hcadeaux](https://huggingface.co/hcadeaux), [@keeganskeate](https://github.com/keeganskeate), and the entire [Cannabis Data Science Team](https://meetup.com/cannabis-data-science/members) for their contributions.
| cannlytics/cannabis_licenses | [
"annotations_creators:expert-generated",
"language_creators:expert-generated",
"license:cc-by-4.0",
"cannabis",
"licenses",
"region:us"
] | 2022-09-28T18:52:23+00:00 | {"annotations_creators": ["expert-generated"], "language_creators": ["expert-generated"], "license": ["cc-by-4.0"], "pretty_name": "cannabis_licenses", "tags": ["cannabis", "licenses"]} | 2023-09-30T13:23:05+00:00 | [] | [] | TAGS
#annotations_creators-expert-generated #language_creators-expert-generated #license-cc-by-4.0 #cannabis #licenses #region-us
| Cannabis Licenses
=================
Table of Contents
-----------------
* Table of Contents
* Dataset Description
+ Dataset Summary
* Dataset Structure
+ Data Instances
+ Data Fields
+ Data Splits
* Dataset Creation
+ Curation Rationale
+ Source Data
+ Data Collection and Normalization
+ Personal and Sensitive Information
* Considerations for Using the Data
+ Social Impact of Dataset
+ Discussion of Biases
+ Other Known Limitations
* Additional Information
+ Dataset Curators
+ License
+ Citation
+ Contributions
Dataset Description
-------------------
* Homepage: <URL
* Repository: <URL
* Point of Contact: [dev@URL](mailto:dev@URL)
### Dataset Summary
Cannabis Licenses is a collection of cannabis license data for each state with permitted adult-use cannabis. The dataset also includes a sub-dataset, 'all', that includes all licenses.
Dataset Structure
-----------------
The dataset is partitioned into 18 subsets for each state and the aggregate.
State: All, Code: 'all', Status:
State: Alaska, Code: 'ak', Status:
State: Arizona, Code: 'az', Status:
State: California, Code: 'ca', Status:
State: Colorado, Code: 'co', Status:
State: Connecticut, Code: 'ct', Status:
State: Delaware, Code: 'md', Status:
State: Illinois, Code: 'il', Status:
State: Maine, Code: 'me', Status:
State: Maryland, Code: 'md', Status:
State: Massachusetts, Code: 'ma', Status:
State: Michigan, Code: 'mi', Status:
State: Missouri, Code: 'mo', Status:
State: Montana, Code: 'mt', Status:
State: Nevada, Code: 'nv', Status:
State: New Jersey, Code: 'nj', Status:
State: New Mexico, Code: 'nm', Status:
State: New York, Code: 'ny', Status:
State: Oregon, Code: 'or', Status:
State: Rhode Island, Code: 'ri', Status:
State: Vermont, Code: 'vt', Status:
State: Virginia, Code: 'va', Status: โณ Expected 2024
State: Washington, Code: 'wa', Status:
The following states have issued medical cannabis licenses, but are not (yet) included in the dataset:
* Alabama
* Arkansas
* District of Columbia (D.C.)
* Florida
* Kentucky (2024)
* Louisiana
* Minnesota
* Mississippi
* New Hampshire
* North Dakota
* Ohio
* Oklahoma
* Pennsylvania
* South Dakota
* Utah
* West Virginia
### Data Instances
You can load the licenses for each state. For example:
### Data Fields
Below is a non-exhaustive list of fields, used to standardize the various data that are encountered, that you may expect to find for each observation.
Field: 'id', Example: '"1046"', Description: A state-unique ID for the license.
Field: 'license\_number', Example: '"C10-0000423-LIC"', Description: A unique license number.
Field: 'license\_status', Example: '"Active"', Description: The status of the license. Only licenses that are active are included.
Field: 'license\_status\_date', Example: '"2022-04-20T00:00"', Description: The date the status was assigned, an ISO-formatted date if present.
Field: 'license\_term', Example: '"Provisional"', Description: The term for the license.
Field: 'license\_type', Example: '"Commercial - Retailer"', Description: The type of business license.
Field: 'license\_designation', Example: '"Adult-Use and Medicinal"', Description: A state-specific classification for the license.
Field: 'issue\_date', Example: '"2019-07-15T00:00:00"', Description: An issue date for the license, an ISO-formatted date if present.
Field: 'expiration\_date', Example: '"2023-07-14T00:00:00"', Description: An expiration date for the license, an ISO-formatted date if present.
Field: 'licensing\_authority\_id', Example: '"BCC"', Description: A unique ID for the state licensing authority.
Field: 'licensing\_authority', Example: '"Bureau of Cannabis Control (BCC)"', Description: The state licensing authority.
Field: 'business\_legal\_name', Example: '"Movocan"', Description: The legal name of the business that owns the license.
Field: 'business\_dba\_name', Example: '"Movocan"', Description: The name the license is doing business as.
Field: 'business\_owner\_name', Example: '"redacted"', Description: The name of the owner of the license.
Field: 'business\_structure', Example: '"Corporation"', Description: The structure of the business that owns the license.
Field: 'activity', Example: '"Pending Inspection"', Description: Any relevant license activity.
Field: 'premise\_street\_address', Example: '"1632 Gateway Rd"', Description: The street address of the business.
Field: 'premise\_city', Example: '"Calexico"', Description: The city of the business.
Field: 'premise\_state', Example: '"CA"', Description: The state abbreviation of the business.
Field: 'premise\_county', Example: '"Imperial"', Description: The county of the business.
Field: 'premise\_zip\_code', Example: '"92231"', Description: The zip code of the business.
Field: 'business\_email', Example: '"redacted@URL"', Description: The business email of the license.
Field: 'business\_phone', Example: '"(555) 555-5555"', Description: The business phone of the license.
Field: 'business\_website', Example: '"URL"', Description: The business website of the license.
Field: 'parcel\_number', Example: '"A42"', Description: An ID for the business location.
Field: 'premise\_latitude', Example: '32.69035693', Description: The latitude of the business.
Field: 'premise\_longitude', Example: '-115.38987552', Description: The longitude of the business.
Field: 'data\_refreshed\_date', Example: '"2022-09-21T12:16:33.3866667"', Description: An ISO-formatted time when the license data was updated.
### Data Splits
The data is split into subsets by state. You can retrieve all licenses by requesting the 'all' subset.
Dataset Creation
----------------
### Curation Rationale
Data about organizations operating in the cannabis industry for each state is valuable for research.
### Source Data
### Data Collection and Normalization
In the 'algorithms' directory, you can find the algorithms used for data collection. You can use these algorithms to recreate the dataset. First, you will need to clone the repository:
You can then install the algorithm Python (3.9+) requirements:
Then you can run all of the data-collection algorithms:
Or you can run each algorithm individually. For example:
### Personal and Sensitive Information
This dataset includes names of individuals, public addresses, and contact information for cannabis licensees. It is important to take care to use these data points in a legal manner.
Considerations for Using the Data
---------------------------------
### Social Impact of Dataset
Arguably, there is substantial social impact that could result from the study of permitted adult-use cannabis, therefore, researchers and data consumers alike should take the utmost care in the use of this dataset.
### Discussion of Biases
Cannlytics is a for-profit data and analytics company that primarily serves cannabis businesses. The data are not randomly collected and thus sampling bias should be taken into consideration.
### Other Known Limitations
The data is for adult-use cannabis licenses. It would be valuable to include medical cannabis licenses too.
Additional Information
----------------------
### Dataset Curators
Curated by Cannlytics
[contact@URL](mailto:contact@URL)
### License
Please cite the following if you use the code examples in your research:
### Contributions
Thanks to Cannlytics, @candy-o, @hcadeaux, @keeganskeate, and the entire Cannabis Data Science Team for their contributions.
| [
"### Dataset Summary\n\n\nCannabis Licenses is a collection of cannabis license data for each state with permitted adult-use cannabis. The dataset also includes a sub-dataset, 'all', that includes all licenses.\n\n\nDataset Structure\n-----------------\n\n\nThe dataset is partitioned into 18 subsets for each state and the aggregate.\n\n\nState: All, Code: 'all', Status: \nState: Alaska, Code: 'ak', Status: \nState: Arizona, Code: 'az', Status: \nState: California, Code: 'ca', Status: \nState: Colorado, Code: 'co', Status: \nState: Connecticut, Code: 'ct', Status: \nState: Delaware, Code: 'md', Status: \nState: Illinois, Code: 'il', Status: \nState: Maine, Code: 'me', Status: \nState: Maryland, Code: 'md', Status: \nState: Massachusetts, Code: 'ma', Status: \nState: Michigan, Code: 'mi', Status: \nState: Missouri, Code: 'mo', Status: \nState: Montana, Code: 'mt', Status: \nState: Nevada, Code: 'nv', Status: \nState: New Jersey, Code: 'nj', Status: \nState: New Mexico, Code: 'nm', Status: \nState: New York, Code: 'ny', Status: \nState: Oregon, Code: 'or', Status: \nState: Rhode Island, Code: 'ri', Status: \nState: Vermont, Code: 'vt', Status: \nState: Virginia, Code: 'va', Status: โณ Expected 2024\nState: Washington, Code: 'wa', Status: \n\n\nThe following states have issued medical cannabis licenses, but are not (yet) included in the dataset:\n\n\n* Alabama\n* Arkansas\n* District of Columbia (D.C.)\n* Florida\n* Kentucky (2024)\n* Louisiana\n* Minnesota\n* Mississippi\n* New Hampshire\n* North Dakota\n* Ohio\n* Oklahoma\n* Pennsylvania\n* South Dakota\n* Utah\n* West Virginia",
"### Data Instances\n\n\nYou can load the licenses for each state. For example:",
"### Data Fields\n\n\nBelow is a non-exhaustive list of fields, used to standardize the various data that are encountered, that you may expect to find for each observation.\n\n\nField: 'id', Example: '\"1046\"', Description: A state-unique ID for the license.\nField: 'license\\_number', Example: '\"C10-0000423-LIC\"', Description: A unique license number.\nField: 'license\\_status', Example: '\"Active\"', Description: The status of the license. Only licenses that are active are included.\nField: 'license\\_status\\_date', Example: '\"2022-04-20T00:00\"', Description: The date the status was assigned, an ISO-formatted date if present.\nField: 'license\\_term', Example: '\"Provisional\"', Description: The term for the license.\nField: 'license\\_type', Example: '\"Commercial - Retailer\"', Description: The type of business license.\nField: 'license\\_designation', Example: '\"Adult-Use and Medicinal\"', Description: A state-specific classification for the license.\nField: 'issue\\_date', Example: '\"2019-07-15T00:00:00\"', Description: An issue date for the license, an ISO-formatted date if present.\nField: 'expiration\\_date', Example: '\"2023-07-14T00:00:00\"', Description: An expiration date for the license, an ISO-formatted date if present.\nField: 'licensing\\_authority\\_id', Example: '\"BCC\"', Description: A unique ID for the state licensing authority.\nField: 'licensing\\_authority', Example: '\"Bureau of Cannabis Control (BCC)\"', Description: The state licensing authority.\nField: 'business\\_legal\\_name', Example: '\"Movocan\"', Description: The legal name of the business that owns the license.\nField: 'business\\_dba\\_name', Example: '\"Movocan\"', Description: The name the license is doing business as.\nField: 'business\\_owner\\_name', Example: '\"redacted\"', Description: The name of the owner of the license.\nField: 'business\\_structure', Example: '\"Corporation\"', Description: The structure of the business that owns the license.\nField: 'activity', Example: '\"Pending Inspection\"', Description: Any relevant license activity.\nField: 'premise\\_street\\_address', Example: '\"1632 Gateway Rd\"', Description: The street address of the business.\nField: 'premise\\_city', Example: '\"Calexico\"', Description: The city of the business.\nField: 'premise\\_state', Example: '\"CA\"', Description: The state abbreviation of the business.\nField: 'premise\\_county', Example: '\"Imperial\"', Description: The county of the business.\nField: 'premise\\_zip\\_code', Example: '\"92231\"', Description: The zip code of the business.\nField: 'business\\_email', Example: '\"redacted@URL\"', Description: The business email of the license.\nField: 'business\\_phone', Example: '\"(555) 555-5555\"', Description: The business phone of the license.\nField: 'business\\_website', Example: '\"URL\"', Description: The business website of the license.\nField: 'parcel\\_number', Example: '\"A42\"', Description: An ID for the business location.\nField: 'premise\\_latitude', Example: '32.69035693', Description: The latitude of the business.\nField: 'premise\\_longitude', Example: '-115.38987552', Description: The longitude of the business.\nField: 'data\\_refreshed\\_date', Example: '\"2022-09-21T12:16:33.3866667\"', Description: An ISO-formatted time when the license data was updated.",
"### Data Splits\n\n\nThe data is split into subsets by state. You can retrieve all licenses by requesting the 'all' subset.\n\n\nDataset Creation\n----------------",
"### Curation Rationale\n\n\nData about organizations operating in the cannabis industry for each state is valuable for research.",
"### Source Data",
"### Data Collection and Normalization\n\n\nIn the 'algorithms' directory, you can find the algorithms used for data collection. You can use these algorithms to recreate the dataset. First, you will need to clone the repository:\n\n\nYou can then install the algorithm Python (3.9+) requirements:\n\n\nThen you can run all of the data-collection algorithms:\n\n\nOr you can run each algorithm individually. For example:",
"### Personal and Sensitive Information\n\n\nThis dataset includes names of individuals, public addresses, and contact information for cannabis licensees. It is important to take care to use these data points in a legal manner.\n\n\nConsiderations for Using the Data\n---------------------------------",
"### Social Impact of Dataset\n\n\nArguably, there is substantial social impact that could result from the study of permitted adult-use cannabis, therefore, researchers and data consumers alike should take the utmost care in the use of this dataset.",
"### Discussion of Biases\n\n\nCannlytics is a for-profit data and analytics company that primarily serves cannabis businesses. The data are not randomly collected and thus sampling bias should be taken into consideration.",
"### Other Known Limitations\n\n\nThe data is for adult-use cannabis licenses. It would be valuable to include medical cannabis licenses too.\n\n\nAdditional Information\n----------------------",
"### Dataset Curators\n\n\nCurated by Cannlytics \n\n[contact@URL](mailto:contact@URL)",
"### License\n\n\nPlease cite the following if you use the code examples in your research:",
"### Contributions\n\n\nThanks to Cannlytics, @candy-o, @hcadeaux, @keeganskeate, and the entire Cannabis Data Science Team for their contributions."
] | [
"TAGS\n#annotations_creators-expert-generated #language_creators-expert-generated #license-cc-by-4.0 #cannabis #licenses #region-us \n",
"### Dataset Summary\n\n\nCannabis Licenses is a collection of cannabis license data for each state with permitted adult-use cannabis. The dataset also includes a sub-dataset, 'all', that includes all licenses.\n\n\nDataset Structure\n-----------------\n\n\nThe dataset is partitioned into 18 subsets for each state and the aggregate.\n\n\nState: All, Code: 'all', Status: \nState: Alaska, Code: 'ak', Status: \nState: Arizona, Code: 'az', Status: \nState: California, Code: 'ca', Status: \nState: Colorado, Code: 'co', Status: \nState: Connecticut, Code: 'ct', Status: \nState: Delaware, Code: 'md', Status: \nState: Illinois, Code: 'il', Status: \nState: Maine, Code: 'me', Status: \nState: Maryland, Code: 'md', Status: \nState: Massachusetts, Code: 'ma', Status: \nState: Michigan, Code: 'mi', Status: \nState: Missouri, Code: 'mo', Status: \nState: Montana, Code: 'mt', Status: \nState: Nevada, Code: 'nv', Status: \nState: New Jersey, Code: 'nj', Status: \nState: New Mexico, Code: 'nm', Status: \nState: New York, Code: 'ny', Status: \nState: Oregon, Code: 'or', Status: \nState: Rhode Island, Code: 'ri', Status: \nState: Vermont, Code: 'vt', Status: \nState: Virginia, Code: 'va', Status: โณ Expected 2024\nState: Washington, Code: 'wa', Status: \n\n\nThe following states have issued medical cannabis licenses, but are not (yet) included in the dataset:\n\n\n* Alabama\n* Arkansas\n* District of Columbia (D.C.)\n* Florida\n* Kentucky (2024)\n* Louisiana\n* Minnesota\n* Mississippi\n* New Hampshire\n* North Dakota\n* Ohio\n* Oklahoma\n* Pennsylvania\n* South Dakota\n* Utah\n* West Virginia",
"### Data Instances\n\n\nYou can load the licenses for each state. For example:",
"### Data Fields\n\n\nBelow is a non-exhaustive list of fields, used to standardize the various data that are encountered, that you may expect to find for each observation.\n\n\nField: 'id', Example: '\"1046\"', Description: A state-unique ID for the license.\nField: 'license\\_number', Example: '\"C10-0000423-LIC\"', Description: A unique license number.\nField: 'license\\_status', Example: '\"Active\"', Description: The status of the license. Only licenses that are active are included.\nField: 'license\\_status\\_date', Example: '\"2022-04-20T00:00\"', Description: The date the status was assigned, an ISO-formatted date if present.\nField: 'license\\_term', Example: '\"Provisional\"', Description: The term for the license.\nField: 'license\\_type', Example: '\"Commercial - Retailer\"', Description: The type of business license.\nField: 'license\\_designation', Example: '\"Adult-Use and Medicinal\"', Description: A state-specific classification for the license.\nField: 'issue\\_date', Example: '\"2019-07-15T00:00:00\"', Description: An issue date for the license, an ISO-formatted date if present.\nField: 'expiration\\_date', Example: '\"2023-07-14T00:00:00\"', Description: An expiration date for the license, an ISO-formatted date if present.\nField: 'licensing\\_authority\\_id', Example: '\"BCC\"', Description: A unique ID for the state licensing authority.\nField: 'licensing\\_authority', Example: '\"Bureau of Cannabis Control (BCC)\"', Description: The state licensing authority.\nField: 'business\\_legal\\_name', Example: '\"Movocan\"', Description: The legal name of the business that owns the license.\nField: 'business\\_dba\\_name', Example: '\"Movocan\"', Description: The name the license is doing business as.\nField: 'business\\_owner\\_name', Example: '\"redacted\"', Description: The name of the owner of the license.\nField: 'business\\_structure', Example: '\"Corporation\"', Description: The structure of the business that owns the license.\nField: 'activity', Example: '\"Pending Inspection\"', Description: Any relevant license activity.\nField: 'premise\\_street\\_address', Example: '\"1632 Gateway Rd\"', Description: The street address of the business.\nField: 'premise\\_city', Example: '\"Calexico\"', Description: The city of the business.\nField: 'premise\\_state', Example: '\"CA\"', Description: The state abbreviation of the business.\nField: 'premise\\_county', Example: '\"Imperial\"', Description: The county of the business.\nField: 'premise\\_zip\\_code', Example: '\"92231\"', Description: The zip code of the business.\nField: 'business\\_email', Example: '\"redacted@URL\"', Description: The business email of the license.\nField: 'business\\_phone', Example: '\"(555) 555-5555\"', Description: The business phone of the license.\nField: 'business\\_website', Example: '\"URL\"', Description: The business website of the license.\nField: 'parcel\\_number', Example: '\"A42\"', Description: An ID for the business location.\nField: 'premise\\_latitude', Example: '32.69035693', Description: The latitude of the business.\nField: 'premise\\_longitude', Example: '-115.38987552', Description: The longitude of the business.\nField: 'data\\_refreshed\\_date', Example: '\"2022-09-21T12:16:33.3866667\"', Description: An ISO-formatted time when the license data was updated.",
"### Data Splits\n\n\nThe data is split into subsets by state. You can retrieve all licenses by requesting the 'all' subset.\n\n\nDataset Creation\n----------------",
"### Curation Rationale\n\n\nData about organizations operating in the cannabis industry for each state is valuable for research.",
"### Source Data",
"### Data Collection and Normalization\n\n\nIn the 'algorithms' directory, you can find the algorithms used for data collection. You can use these algorithms to recreate the dataset. First, you will need to clone the repository:\n\n\nYou can then install the algorithm Python (3.9+) requirements:\n\n\nThen you can run all of the data-collection algorithms:\n\n\nOr you can run each algorithm individually. For example:",
"### Personal and Sensitive Information\n\n\nThis dataset includes names of individuals, public addresses, and contact information for cannabis licensees. It is important to take care to use these data points in a legal manner.\n\n\nConsiderations for Using the Data\n---------------------------------",
"### Social Impact of Dataset\n\n\nArguably, there is substantial social impact that could result from the study of permitted adult-use cannabis, therefore, researchers and data consumers alike should take the utmost care in the use of this dataset.",
"### Discussion of Biases\n\n\nCannlytics is a for-profit data and analytics company that primarily serves cannabis businesses. The data are not randomly collected and thus sampling bias should be taken into consideration.",
"### Other Known Limitations\n\n\nThe data is for adult-use cannabis licenses. It would be valuable to include medical cannabis licenses too.\n\n\nAdditional Information\n----------------------",
"### Dataset Curators\n\n\nCurated by Cannlytics \n\n[contact@URL](mailto:contact@URL)",
"### License\n\n\nPlease cite the following if you use the code examples in your research:",
"### Contributions\n\n\nThanks to Cannlytics, @candy-o, @hcadeaux, @keeganskeate, and the entire Cannabis Data Science Team for their contributions."
] | [
46,
450,
19,
1011,
40,
24,
4,
93,
54,
55,
53,
37,
26,
18,
41
] | [
"passage: TAGS\n#annotations_creators-expert-generated #language_creators-expert-generated #license-cc-by-4.0 #cannabis #licenses #region-us \n### Dataset Summary\n\n\nCannabis Licenses is a collection of cannabis license data for each state with permitted adult-use cannabis. The dataset also includes a sub-dataset, 'all', that includes all licenses.\n\n\nDataset Structure\n-----------------\n\n\nThe dataset is partitioned into 18 subsets for each state and the aggregate.\n\n\nState: All, Code: 'all', Status: \nState: Alaska, Code: 'ak', Status: \nState: Arizona, Code: 'az', Status: \nState: California, Code: 'ca', Status: \nState: Colorado, Code: 'co', Status: \nState: Connecticut, Code: 'ct', Status: \nState: Delaware, Code: 'md', Status: \nState: Illinois, Code: 'il', Status: \nState: Maine, Code: 'me', Status: \nState: Maryland, Code: 'md', Status: \nState: Massachusetts, Code: 'ma', Status: \nState: Michigan, Code: 'mi', Status: \nState: Missouri, Code: 'mo', Status: \nState: Montana, Code: 'mt', Status: \nState: Nevada, Code: 'nv', Status: \nState: New Jersey, Code: 'nj', Status: \nState: New Mexico, Code: 'nm', Status: \nState: New York, Code: 'ny', Status: \nState: Oregon, Code: 'or', Status: \nState: Rhode Island, Code: 'ri', Status: \nState: Vermont, Code: 'vt', Status: \nState: Virginia, Code: 'va', Status: โณ Expected 2024\nState: Washington, Code: 'wa', Status: \n\n\nThe following states have issued medical cannabis licenses, but are not (yet) included in the dataset:\n\n\n* Alabama\n* Arkansas\n* District of Columbia (D.C.)\n* Florida\n* Kentucky (2024)\n* Louisiana\n* Minnesota\n* Mississippi\n* New Hampshire\n* North Dakota\n* Ohio\n* Oklahoma\n* Pennsylvania\n* South Dakota\n* Utah\n* West Virginia",
"passage: ### Data Instances\n\n\nYou can load the licenses for each state. For example:"
] |
3562204543b81d961ccef05e11e3d69011fe5104 | # ****Dataset Card for tathagata****
# **I-Dataset Summary**
tathagata.txt is a dataset based on summaries of major Buddhist, Hindu and Advaita texts such as:
- Diamond Sutra
- Lankavatara Sutra
- Sri Nisargadatta Maharaj quotes
- Quotes from the Bhagavad Gita
This dataset was used to train this model https://huggingface.co/radm/rugpt3medium-tathagata
# **II-Languages**
The texts in the dataset are in Russian (ru). | radm/tathagata | [
"task_categories:text-generation",
"task_ids:language-modeling",
"annotations_creators:found",
"language_creators:found",
"multilinguality:monolingual",
"size_categories:n<1K",
"source_datasets:original",
"language:ru",
"license:apache-2.0",
"text_generation",
"quotes",
"region:us"
] | 2022-09-28T18:55:18+00:00 | {"annotations_creators": ["found"], "language_creators": ["found"], "language": ["ru"], "license": ["apache-2.0"], "multilinguality": ["monolingual"], "size_categories": ["n<1K"], "source_datasets": ["original"], "task_categories": ["text-generation"], "task_ids": ["language-modeling"], "pretty_name": "tathagata", "tags": ["text_generation", "quotes"]} | 2022-09-28T19:20:13+00:00 | [] | [
"ru"
] | TAGS
#task_categories-text-generation #task_ids-language-modeling #annotations_creators-found #language_creators-found #multilinguality-monolingual #size_categories-n<1K #source_datasets-original #language-Russian #license-apache-2.0 #text_generation #quotes #region-us
| # Dataset Card for tathagata
# I-Dataset Summary
URL is a dataset based on summaries of major Buddhist, Hindu and Advaita texts such as:
- Diamond Sutra
- Lankavatara Sutra
- Sri Nisargadatta Maharaj quotes
- Quotes from the Bhagavad Gita
This dataset was used to train this model URL
# II-Languages
The texts in the dataset are in Russian (ru). | [
"# Dataset Card for tathagata",
"# I-Dataset Summary\nURL is a dataset based on summaries of major Buddhist, Hindu and Advaita texts such as:\n- Diamond Sutra\n- Lankavatara Sutra\n- Sri Nisargadatta Maharaj quotes\n- Quotes from the Bhagavad Gita\n\nThis dataset was used to train this model URL",
"# II-Languages\nThe texts in the dataset are in Russian (ru)."
] | [
"TAGS\n#task_categories-text-generation #task_ids-language-modeling #annotations_creators-found #language_creators-found #multilinguality-monolingual #size_categories-n<1K #source_datasets-original #language-Russian #license-apache-2.0 #text_generation #quotes #region-us \n",
"# Dataset Card for tathagata",
"# I-Dataset Summary\nURL is a dataset based on summaries of major Buddhist, Hindu and Advaita texts such as:\n- Diamond Sutra\n- Lankavatara Sutra\n- Sri Nisargadatta Maharaj quotes\n- Quotes from the Bhagavad Gita\n\nThis dataset was used to train this model URL",
"# II-Languages\nThe texts in the dataset are in Russian (ru)."
] | [
92,
8,
70,
19
] | [
"passage: TAGS\n#task_categories-text-generation #task_ids-language-modeling #annotations_creators-found #language_creators-found #multilinguality-monolingual #size_categories-n<1K #source_datasets-original #language-Russian #license-apache-2.0 #text_generation #quotes #region-us \n# Dataset Card for tathagata# I-Dataset Summary\nURL is a dataset based on summaries of major Buddhist, Hindu and Advaita texts such as:\n- Diamond Sutra\n- Lankavatara Sutra\n- Sri Nisargadatta Maharaj quotes\n- Quotes from the Bhagavad Gita\n\nThis dataset was used to train this model URL# II-Languages\nThe texts in the dataset are in Russian (ru)."
] |
1786207ffebfbe62211179fccbd4d0566ace37a9 | This textual inversion has been trained on WaifuDiffusion v1.2 (`[45dee52b]`). This will probably not work well with the standard Stable Diffusion model.
# How to use (with webui)
- create `embeddings` folder in the root directory of the webui
- paste the .bin in there
**keyword: `<marine>`** | cattoroboto/waifudiffusion-marine-textual-inversion | [
"region:us"
] | 2022-09-28T22:30:57+00:00 | {} | 2022-09-28T23:06:45+00:00 | [] | [] | TAGS
#region-us
| This textual inversion has been trained on WaifuDiffusion v1.2 ('[45dee52b]'). This will probably not work well with the standard Stable Diffusion model.
# How to use (with webui)
- create 'embeddings' folder in the root directory of the webui
- paste the .bin in there
keyword: '<marine>' | [
"# How to use (with webui)\n\n- create 'embeddings' folder in the root directory of the webui\n- paste the .bin in there\n\nkeyword: '<marine>'"
] | [
"TAGS\n#region-us \n",
"# How to use (with webui)\n\n- create 'embeddings' folder in the root directory of the webui\n- paste the .bin in there\n\nkeyword: '<marine>'"
] | [
6,
42
] | [
"passage: TAGS\n#region-us \n# How to use (with webui)\n\n- create 'embeddings' folder in the root directory of the webui\n- paste the .bin in there\n\nkeyword: '<marine>'"
] |
337ec38c58a30812c0944d807f5acdc1f86f4bc3 | # Info
> This is a repository for anime regularization. If you wish to contribute to the dataset, contact me at naotsue#9786. I will add them to the dataset and update it.
# Criteria
> 512x512
> No excessive deformations
> Vaguely resembles an anime artstyle
# Contribution Leaderboard
> 1. bWm_nubby: 5838 images
> 2. naotsue: 888 images
 | waifu-research-department/regularization | [
"license:mit",
"region:us"
] | 2022-09-29T01:09:44+00:00 | {"license": "mit"} | 2022-09-29T21:00:10+00:00 | [] | [] | TAGS
#license-mit #region-us
| # Info
> This is a repository for anime regularization. If you wish to contribute to the dataset, contact me at naotsue#9786. I will add them to the dataset and update it.
# Criteria
> 512x512
> No excessive deformations
> Vaguely resembles an anime artstyle
# Contribution Leaderboard
> 1. bWm_nubby: 5838 images
> 2. naotsue: 888 images
!Sak | [
"# Info\n> This is a repository for anime regularization. If you wish to contribute to the dataset, contact me at naotsue#9786. I will add them to the dataset and update it.",
"# Criteria\n> 512x512\n\n> No excessive deformations\n\n> Vaguely resembles an anime artstyle",
"# Contribution Leaderboard\n> 1. bWm_nubby: 5838 images\n\n> 2. naotsue: 888 images\n\n!Sak"
] | [
"TAGS\n#license-mit #region-us \n",
"# Info\n> This is a repository for anime regularization. If you wish to contribute to the dataset, contact me at naotsue#9786. I will add them to the dataset and update it.",
"# Criteria\n> 512x512\n\n> No excessive deformations\n\n> Vaguely resembles an anime artstyle",
"# Contribution Leaderboard\n> 1. bWm_nubby: 5838 images\n\n> 2. naotsue: 888 images\n\n!Sak"
] | [
11,
47,
25,
30
] | [
"passage: TAGS\n#license-mit #region-us \n# Info\n> This is a repository for anime regularization. If you wish to contribute to the dataset, contact me at naotsue#9786. I will add them to the dataset and update it.# Criteria\n> 512x512\n\n> No excessive deformations\n\n> Vaguely resembles an anime artstyle# Contribution Leaderboard\n> 1. bWm_nubby: 5838 images\n\n> 2. naotsue: 888 images\n\n!Sak"
] |
4d7946ef7f0c5ff5e261e384db8015dfe8e417cb |
# Dataset Card for EurlexResources: A Corpus Covering the Largest EURLEX Resources
## Table of Contents
- [Table of Contents](#table-of-contents)
- [Dataset Description](#dataset-description)
- [Dataset Summary](#dataset-summary)
- [Supported Tasks and Leaderboards](#supported-tasks-and-leaderboards)
- [Languages](#languages)
- [Dataset Structure](#dataset-structure)
- [Data Instances](#data-instances)
- [Data Fields](#data-fields)
- [Data Splits](#data-splits)
- [Dataset Creation](#dataset-creation)
- [Curation Rationale](#curation-rationale)
- [Source Data](#source-data)
- [Annotations](#annotations)
- [Personal and Sensitive Information](#personal-and-sensitive-information)
- [Considerations for Using the Data](#considerations-for-using-the-data)
- [Social Impact of Dataset](#social-impact-of-dataset)
- [Discussion of Biases](#discussion-of-biases)
- [Other Known Limitations](#other-known-limitations)
- [Additional Information](#additional-information)
- [Dataset Curators](#dataset-curators)
- [Licensing Information](#licensing-information)
- [Citation Information](#citation-information)
- [Contributions](#contributions)
## Dataset Description
- **Homepage:**
- **Repository:** [GitHub](https://github.com/JoelNiklaus/LegalDatasets/tree/main/pretrain/eurlex)
- **Paper:**
- **Leaderboard:**
- **Point of Contact:** [Joel Niklaus](mailto:[email protected])
### Dataset Summary
This dataset contains large text resources (~179GB in total) from EURLEX that can be used for pretraining language models.
Use the dataset like this:
```python
from datasets import load_dataset
config = "de_caselaw" # {lang}_{resource}
dataset = load_dataset("joelito/eurlex_resources", config, split='train', streaming=True)
```
### Supported Tasks and Leaderboards
The dataset supports the task of masked language modeling.
### Languages
The following languages are supported: bg, cs, da, de, el, en, es, et, fi, fr, ga, hr, hu, it, lt, lv, mt, nl, pl, pt, ro, sk, sl, sv
## Dataset Structure
### Data Instances
The file format is jsonl.xz and there is one split available ("train").
The following resource types are supported: caselaw, decision, directive, intagr, proposal, recommendation, regulation
More information about the resource types can be found here:
- Caselaw: [EU](https://eur-lex.europa.eu/collection/eu-law/eu-case-law.html)
- Decision: [EU](https://eur-lex.europa.eu/EN/legal-content/summary/european-union-decisions.html), [Wikipedia](https://en.wikipedia.org/wiki/Decision_(European_Union))
- Directive: [EU](https://european-union.europa.eu/institutions-law-budget/law/types-legislation_en), [Wikipedia](https://en.wikipedia.org/wiki/Directive_(European_Union))
- Recommendation: [EU](https://eur-lex.europa.eu/EN/legal-content/glossary/recommendation.html), [Wikipedia](https://en.wikipedia.org/wiki/Recommendation_(European_Union))
- Regulation: [EU](https://european-union.europa.eu/institutions-law-budget/law/types-legislation_en), [Wikipedia](https://en.wikipedia.org/wiki/Regulation_(European_Union))
- Intagr: [EU](https://eur-lex.europa.eu/collection/eu-law/inter-agree.html), [Wikipedia](https://en.wikipedia.org/wiki/Treaties_of_the_European_Union)
- Proposal: No resource found
| Source | Size (MB) | Words | Documents | Words/Document |
|:-------------------|------------:|------------:|------------:|-----------------:|
| all_all | 180668 | 12106556233 | 8306749 | 1457 |
| all_caselaw | 34939 | 3413551598 | 2487794 | 1372 |
| all_decision | 28519 | 1698585620 | 1267402 | 1340 |
| all_directive | 4786 | 368577940 | 104187 | 3537 |
| all_intagr | 11421 | 743271516 | 274485 | 2707 |
| all_proposal | 26526 | 2087989530 | 702392 | 2972 |
| all_recommendation | 1886 | 164979037 | 80277 | 2055 |
| all_regulation | 72590 | 3629600992 | 3390212 | 1070 |
| bg_all | 7819 | 398067053 | 348691 | 1141 |
| bg_caselaw | 1588 | 109749174 | 104434 | 1050 |
| bg_decision | 1248 | 58817972 | 54075 | 1087 |
| bg_directive | 263 | 15731608 | 4388 | 3585 |
| bg_intagr | 603 | 31292848 | 11581 | 2702 |
| bg_proposal | 1083 | 60674956 | 29251 | 2074 |
| bg_recommendation | 89 | 5588991 | 3321 | 1682 |
| bg_regulation | 2943 | 116211504 | 141641 | 820 |
| cs_all | 8360 | 471961631 | 449793 | 1049 |
| cs_caselaw | 1163 | 110005022 | 104519 | 1052 |
| cs_decision | 1102 | 58921128 | 54075 | 1089 |
| cs_directive | 186 | 13951134 | 4388 | 3179 |
| cs_intagr | 449 | 28106332 | 11581 | 2426 |
| cs_proposal | 840 | 61838692 | 29252 | 2113 |
| cs_recommendation | 64 | 5416549 | 3323 | 1630 |
| cs_regulation | 4557 | 193722774 | 242655 | 798 |
| da_all | 8932 | 671484862 | 332500 | 2019 |
| da_caselaw | 1746 | 185589641 | 88234 | 2103 |
| da_decision | 1356 | 89498535 | 54085 | 1654 |
| da_directive | 207 | 17525792 | 4388 | 3994 |
| da_intagr | 506 | 35596169 | 11582 | 3073 |
| da_proposal | 1399 | 119759476 | 29257 | 4093 |
| da_recommendation | 100 | 9463897 | 3352 | 2823 |
| da_regulation | 3618 | 214051352 | 141602 | 1511 |
| de_all | 9607 | 695512401 | 348290 | 1996 |
| de_caselaw | 1930 | 193232441 | 104228 | 1853 |
| de_decision | 1449 | 93688222 | 53980 | 1735 |
| de_directive | 218 | 17337760 | 4385 | 3953 |
| de_intagr | 531 | 36791153 | 11580 | 3177 |
| de_proposal | 1556 | 126987454 | 29219 | 4346 |
| de_recommendation | 109 | 9608034 | 3318 | 2895 |
| de_regulation | 3813 | 217867337 | 141580 | 1538 |
| el_all | 12469 | 696216541 | 349667 | 1991 |
| el_caselaw | 2951 | 202027703 | 105138 | 1921 |
| el_decision | 1823 | 94919886 | 54150 | 1752 |
| el_directive | 321 | 19411959 | 4390 | 4421 |
| el_intagr | 701 | 38965777 | 11584 | 3363 |
| el_proposal | 2085 | 128005737 | 29290 | 4370 |
| el_recommendation | 145 | 9344866 | 3357 | 2783 |
| el_regulation | 4443 | 203540613 | 141758 | 1435 |
| en_all | 9217 | 769465561 | 348641 | 2207 |
| en_caselaw | 1846 | 222891827 | 104422 | 2134 |
| en_decision | 1504 | 114626013 | 54054 | 2120 |
| en_directive | 204 | 18860876 | 4388 | 4298 |
| en_intagr | 499 | 39029843 | 11581 | 3370 |
| en_proposal | 1538 | 140781768 | 29242 | 4814 |
| en_recommendation | 97 | 10091809 | 3320 | 3039 |
| en_regulation | 3530 | 223183425 | 141634 | 1575 |
| es_all | 8588 | 725125274 | 348443 | 2081 |
| es_caselaw | 1870 | 220621730 | 104312 | 2115 |
| es_decision | 1334 | 98163499 | 54001 | 1817 |
| es_directive | 221 | 21484479 | 4385 | 4899 |
| es_intagr | 516 | 41841805 | 11581 | 3612 |
| es_proposal | 1366 | 133674486 | 29224 | 4574 |
| es_recommendation | 82 | 8864018 | 3319 | 2670 |
| es_regulation | 3199 | 200475257 | 141621 | 1415 |
| et_all | 6090 | 328068754 | 349615 | 938 |
| et_caselaw | 1074 | 93096396 | 105111 | 885 |
| et_decision | 1069 | 50752324 | 54159 | 937 |
| et_directive | 177 | 11555930 | 4390 | 2632 |
| et_intagr | 436 | 24018147 | 11584 | 2073 |
| et_proposal | 810 | 51600852 | 29283 | 1762 |
| et_recommendation | 61 | 4451369 | 3355 | 1326 |
| et_regulation | 2464 | 92593736 | 141733 | 653 |
| fi_all | 7346 | 404265224 | 349633 | 1156 |
| fi_caselaw | 1596 | 126525296 | 105119 | 1203 |
| fi_decision | 1227 | 59659475 | 54163 | 1101 |
| fi_directive | 204 | 12766491 | 4389 | 2908 |
| fi_intagr | 463 | 25392311 | 11584 | 2192 |
| fi_proposal | 1075 | 69198401 | 29288 | 2362 |
| fi_recommendation | 73 | 5070392 | 3356 | 1510 |
| fi_regulation | 2707 | 105652858 | 141734 | 745 |
| fr_all | 9937 | 828959218 | 348295 | 2380 |
| fr_caselaw | 2158 | 246262666 | 104228 | 2362 |
| fr_decision | 1473 | 108648744 | 53981 | 2012 |
| fr_directive | 222 | 20308801 | 4385 | 4631 |
| fr_intagr | 536 | 41986012 | 11580 | 3625 |
| fr_proposal | 1592 | 149134298 | 29218 | 5104 |
| fr_recommendation | 112 | 11510415 | 3318 | 3469 |
| fr_regulation | 3845 | 251108282 | 141585 | 1773 |
| ga_all | 1028 | 65030095 | 349778 | 185 |
| ga_caselaw | 11 | 696305 | 105205 | 6 |
| ga_decision | 87 | 4415457 | 54189 | 81 |
| ga_directive | 18 | 1512027 | 4390 | 344 |
| ga_intagr | 19 | 1820723 | 11586 | 157 |
| ga_proposal | 289 | 26106889 | 29298 | 891 |
| ga_recommendation | 10 | 902390 | 3361 | 268 |
| ga_regulation | 594 | 29576304 | 141749 | 208 |
| hr_all | 4594 | 258816068 | 348691 | 742 |
| hr_caselaw | 617 | 62432734 | 104434 | 597 |
| hr_decision | 596 | 31911903 | 54075 | 590 |
| hr_directive | 156 | 10855913 | 4388 | 2474 |
| hr_intagr | 450 | 24962086 | 11581 | 2155 |
| hr_proposal | 552 | 33437815 | 29251 | 1143 |
| hr_recommendation | 40 | 3612247 | 3321 | 1087 |
| hr_regulation | 2183 | 91603370 | 141641 | 646 |
| hu_all | 6653 | 375253894 | 349605 | 1073 |
| hu_caselaw | 1278 | 110179375 | 105144 | 1047 |
| hu_decision | 1147 | 57108172 | 54156 | 1054 |
| hu_directive | 200 | 13568304 | 4389 | 3091 |
| hu_intagr | 470 | 27258501 | 11586 | 2352 |
| hu_proposal | 912 | 60882750 | 29291 | 2078 |
| hu_recommendation | 70 | 5312868 | 3357 | 1582 |
| hu_regulation | 2576 | 100943924 | 141682 | 712 |
| it_all | 9586 | 768605772 | 333631 | 2303 |
| it_caselaw | 1889 | 206117726 | 89560 | 2301 |
| it_decision | 1445 | 102848859 | 53983 | 1905 |
| it_directive | 217 | 19687773 | 4385 | 4489 |
| it_intagr | 528 | 40134330 | 11580 | 3465 |
| it_proposal | 1533 | 140713925 | 29218 | 4816 |
| it_recommendation | 109 | 10923431 | 3318 | 3292 |
| it_regulation | 3865 | 248179728 | 141587 | 1752 |
| lt_all | 6400 | 364361783 | 200565 | 1816 |
| lt_caselaw | 1137 | 101808706 | 105477 | 965 |
| lt_decision | 1096 | 55850308 | 21990 | 2539 |
| lt_directive | 185 | 13078983 | 3239 | 4037 |
| lt_intagr | 452 | 27009631 | 7481 | 3610 |
| lt_proposal | 850 | 58553579 | 29272 | 2000 |
| lt_recommendation | 64 | 5121089 | 3363 | 1522 |
| lt_regulation | 2617 | 102939487 | 29743 | 3460 |
| lv_all | 6349 | 363239195 | 349919 | 1038 |
| lv_caselaw | 1153 | 103456811 | 105242 | 983 |
| lv_decision | 1103 | 55512944 | 54224 | 1023 |
| lv_directive | 186 | 13023024 | 4392 | 2965 |
| lv_intagr | 452 | 26693107 | 11630 | 2295 |
| lv_proposal | 96 | 58176216 | 29298 | 1985 |
| lv_recommendation | 64 | 5074494 | 3361 | 1509 |
| lv_regulation | 2545 | 101302599 | 141772 | 714 |
| mt_all | 6540 | 367834815 | 350292 | 1050 |
| mt_caselaw | 1164 | 100423543 | 105479 | 952 |
| mt_decision | 1109 | 55239141 | 54280 | 1017 |
| mt_directive | 203 | 14355266 | 4392 | 3268 |
| mt_intagr | 470 | 27701991 | 11675 | 2372 |
| mt_proposal | 878 | 59749277 | 29274 | 2041 |
| mt_recommendation | 65 | 5039600 | 3363 | 1498 |
| mt_regulation | 2650 | 105325997 | 141829 | 742 |
| nl_all | 9586 | 770312808 | 349407 | 2204 |
| nl_caselaw | 1847 | 206271837 | 105005 | 1964 |
| nl_decision | 1456 | 104060901 | 54152 | 1921 |
| nl_directive | 217 | 19529361 | 4388 | 4450 |
| nl_intagr | 529 | 40247634 | 11584 | 3474 |
| nl_proposal | 1540 | 141258274 | 29279 | 4824 |
| nl_recommendation | 111 | 11002405 | 3355 | 3279 |
| nl_regulation | 3886 | 247942396 | 141644 | 1750 |
| pl_all | 6677 | 406648795 | 350349 | 1160 |
| pl_caselaw | 1231 | 115824759 | 105479 | 1098 |
| pl_decision | 1125 | 60407576 | 54287 | 1112 |
| pl_directive | 197 | 14672157 | 4392 | 3340 |
| pl_intagr | 466 | 28543668 | 11680 | 2443 |
| pl_proposal | 886 | 64728230 | 29317 | 2207 |
| pl_recommendation | 68 | 5769893 | 3363 | 1715 |
| pl_regulation | 2703 | 116702512 | 141831 | 822 |
| pt_all | 8450 | 675152149 | 348449 | 1937 |
| pt_caselaw | 1763 | 198084937 | 104312 | 1898 |
| pt_decision | 1327 | 93278293 | 54007 | 1727 |
| pt_directive | 217 | 19831549 | 4385 | 4522 |
| pt_intagr | 504 | 37999753 | 11581 | 3281 |
| pt_proposal | 1361 | 127461782 | 29224 | 4361 |
| pt_recommendation | 81 | 8396661 | 3319 | 2529 |
| pt_regulation | 3197 | 190099174 | 141621 | 1342 |
| ro_all | 6315 | 415038571 | 350300 | 1184 |
| ro_caselaw | 1110 | 114780999 | 105516 | 1087 |
| ro_decision | 1047 | 59479553 | 54281 | 1095 |
| ro_directive | 206 | 16101628 | 4392 | 3666 |
| ro_intagr | 481 | 31497000 | 11675 | 2697 |
| ro_proposal | 805 | 62130419 | 29274 | 2122 |
| ro_recommendation | 63 | 5977913 | 3363 | 1777 |
| ro_regulation | 2603 | 125071059 | 141799 | 882 |
| sk_all | 6484 | 392235510 | 350570 | 1118 |
| sk_caselaw | 1160 | 110125141 | 105608 | 1042 |
| sk_decision | 1111 | 59576875 | 54349 | 1096 |
| sk_directive | 188 | 14132755 | 4393 | 3217 |
| sk_intagr | 458 | 28298155 | 11676 | 2423 |
| sk_proposal | 859 | 63726047 | 29290 | 2175 |
| sk_recommendation | 66 | 5654790 | 3364 | 1680 |
| sk_regulation | 2642 | 110721747 | 141890 | 780 |
| sl_all | 6222 | 394814289 | 350574 | 1126 |
| sl_caselaw | 1071 | 111238184 | 105608 | 1053 |
| sl_decision | 1075 | 59454906 | 54349 | 1093 |
| sl_directive | 176 | 13908097 | 4393 | 3165 |
| sl_intagr | 441 | 28239078 | 11676 | 2418 |
| sl_proposal | 812 | 63391970 | 29290 | 2164 |
| sl_recommendation | 62 | 5628775 | 3364 | 1673 |
| sl_regulation | 2585 | 112953279 | 141894 | 796 |
| sv_all | 7419 | 500085970 | 351051 | 1424 |
| sv_caselaw | 1585 | 162108645 | 105980 | 1529 |
| sv_decision | 1213 | 71744934 | 54357 | 1319 |
| sv_directive | 195 | 15386273 | 4393 | 3502 |
| sv_intagr | 463 | 29845462 | 11676 | 2556 |
| sv_proposal | 1059 | 86016237 | 29292 | 2936 |
| sv_recommendation | 79 | 7152141 | 3366 | 2124 |
| sv_regulation | 2825 | 127832278 | 141987 | 900 |
### Data Fields
[More Information Needed]
### Data Splits
[More Information Needed]
## Dataset Creation
### Curation Rationale
[More Information Needed]
### Source Data
#### Initial Data Collection and Normalization
The data has been downloaded using the R package [eurlex](https://cran.r-project.org/web/packages/eurlex/vignettes/eurlexpkg.html) between June and August 2022.
#### Who are the source language producers?
[More Information Needed]
### Annotations
#### Annotation process
[More Information Needed]
#### Who are the annotators?
[More Information Needed]
### Personal and Sensitive Information
[More Information Needed]
## Considerations for Using the Data
### Social Impact of Dataset
[More Information Needed]
### Discussion of Biases
[More Information Needed]
### Other Known Limitations
[More Information Needed]
## Additional Information
### Dataset Curators
[More Information Needed]
### Licensing Information
[CC BY 4.0](https://creativecommons.org/licenses/by/4.0/)
[see also the legal notice](https://eur-lex.europa.eu/content/legal-notice/legal-notice.html)
### Citation Information
[More Information Needed]
### Contributions
Thanks to [@JoelNiklaus](https://github.com/joelniklaus) for adding this dataset.
| joelniklaus/eurlex_resources | [
"task_categories:fill-mask",
"annotations_creators:other",
"language_creators:found",
"multilinguality:multilingual",
"size_categories:1M<n<10M",
"source_datasets:original",
"language:bg",
"language:cs",
"language:da",
"language:de",
"language:el",
"language:en",
"language:es",
"language:et",
"language:fi",
"language:fr",
"language:ga",
"language:hr",
"language:hu",
"language:it",
"language:lt",
"language:lv",
"language:mt",
"language:nl",
"language:pl",
"language:pt",
"language:ro",
"language:sk",
"language:sl",
"language:sv",
"license:cc-by-4.0",
"region:us"
] | 2022-09-29T06:35:34+00:00 | {"annotations_creators": ["other"], "language_creators": ["found"], "language": ["bg", "cs", "da", "de", "el", "en", "es", "et", "fi", "fr", "ga", "hr", "hu", "it", "lt", "lv", "mt", "nl", "pl", "pt", "ro", "sk", "sl", "sv"], "license": ["cc-by-4.0"], "multilinguality": ["multilingual"], "size_categories": ["1M<n<10M"], "source_datasets": ["original"], "task_categories": ["fill-mask"], "pretty_name": "EurlexResources: A Corpus Covering the Largest EURLEX Resources"} | 2023-05-10T07:04:28+00:00 | [] | [
"bg",
"cs",
"da",
"de",
"el",
"en",
"es",
"et",
"fi",
"fr",
"ga",
"hr",
"hu",
"it",
"lt",
"lv",
"mt",
"nl",
"pl",
"pt",
"ro",
"sk",
"sl",
"sv"
] | TAGS
#task_categories-fill-mask #annotations_creators-other #language_creators-found #multilinguality-multilingual #size_categories-1M<n<10M #source_datasets-original #language-Bulgarian #language-Czech #language-Danish #language-German #language-Modern Greek (1453-) #language-English #language-Spanish #language-Estonian #language-Finnish #language-French #language-Irish #language-Croatian #language-Hungarian #language-Italian #language-Lithuanian #language-Latvian #language-Maltese #language-Dutch #language-Polish #language-Portuguese #language-Romanian #language-Slovak #language-Slovenian #language-Swedish #license-cc-by-4.0 #region-us
| Dataset Card for EurlexResources: A Corpus Covering the Largest EURLEX Resources
================================================================================
Table of Contents
-----------------
* Table of Contents
* Dataset Description
+ Dataset Summary
+ Supported Tasks and Leaderboards
+ Languages
* Dataset Structure
+ Data Instances
+ Data Fields
+ Data Splits
* Dataset Creation
+ Curation Rationale
+ Source Data
+ Annotations
+ Personal and Sensitive Information
* Considerations for Using the Data
+ Social Impact of Dataset
+ Discussion of Biases
+ Other Known Limitations
* Additional Information
+ Dataset Curators
+ Licensing Information
+ Citation Information
+ Contributions
Dataset Description
-------------------
* Homepage:
* Repository: GitHub
* Paper:
* Leaderboard:
* Point of Contact: Joel Niklaus
### Dataset Summary
This dataset contains large text resources (~179GB in total) from EURLEX that can be used for pretraining language models.
Use the dataset like this:
### Supported Tasks and Leaderboards
The dataset supports the task of masked language modeling.
### Languages
The following languages are supported: bg, cs, da, de, el, en, es, et, fi, fr, ga, hr, hu, it, lt, lv, mt, nl, pl, pt, ro, sk, sl, sv
Dataset Structure
-----------------
### Data Instances
The file format is URL and there is one split available ("train").
The following resource types are supported: caselaw, decision, directive, intagr, proposal, recommendation, regulation
More information about the resource types can be found here:
* Caselaw: EU
* Decision: EU, Wikipedia)
* Directive: EU, Wikipedia)
* Recommendation: EU, Wikipedia)
* Regulation: EU, Wikipedia)
* Intagr: EU, Wikipedia
* Proposal: No resource found
### Data Fields
### Data Splits
Dataset Creation
----------------
### Curation Rationale
### Source Data
#### Initial Data Collection and Normalization
The data has been downloaded using the R package eurlex between June and August 2022.
#### Who are the source language producers?
### Annotations
#### Annotation process
#### Who are the annotators?
### Personal and Sensitive Information
Considerations for Using the Data
---------------------------------
### Social Impact of Dataset
### Discussion of Biases
### Other Known Limitations
Additional Information
----------------------
### Dataset Curators
### Licensing Information
CC BY 4.0
see also the legal notice
### Contributions
Thanks to @JoelNiklaus for adding this dataset.
| [
"### Dataset Summary\n\n\nThis dataset contains large text resources (~179GB in total) from EURLEX that can be used for pretraining language models.\n\n\nUse the dataset like this:",
"### Supported Tasks and Leaderboards\n\n\nThe dataset supports the task of masked language modeling.",
"### Languages\n\n\nThe following languages are supported: bg, cs, da, de, el, en, es, et, fi, fr, ga, hr, hu, it, lt, lv, mt, nl, pl, pt, ro, sk, sl, sv\n\n\nDataset Structure\n-----------------",
"### Data Instances\n\n\nThe file format is URL and there is one split available (\"train\").\nThe following resource types are supported: caselaw, decision, directive, intagr, proposal, recommendation, regulation\n\n\nMore information about the resource types can be found here:\n\n\n* Caselaw: EU\n* Decision: EU, Wikipedia)\n* Directive: EU, Wikipedia)\n* Recommendation: EU, Wikipedia)\n* Regulation: EU, Wikipedia)\n* Intagr: EU, Wikipedia\n* Proposal: No resource found",
"### Data Fields",
"### Data Splits\n\n\nDataset Creation\n----------------",
"### Curation Rationale",
"### Source Data",
"#### Initial Data Collection and Normalization\n\n\nThe data has been downloaded using the R package eurlex between June and August 2022.",
"#### Who are the source language producers?",
"### Annotations",
"#### Annotation process",
"#### Who are the annotators?",
"### Personal and Sensitive Information\n\n\nConsiderations for Using the Data\n---------------------------------",
"### Social Impact of Dataset",
"### Discussion of Biases",
"### Other Known Limitations\n\n\nAdditional Information\n----------------------",
"### Dataset Curators",
"### Licensing Information\n\n\nCC BY 4.0\nsee also the legal notice",
"### Contributions\n\n\nThanks to @JoelNiklaus for adding this dataset."
] | [
"TAGS\n#task_categories-fill-mask #annotations_creators-other #language_creators-found #multilinguality-multilingual #size_categories-1M<n<10M #source_datasets-original #language-Bulgarian #language-Czech #language-Danish #language-German #language-Modern Greek (1453-) #language-English #language-Spanish #language-Estonian #language-Finnish #language-French #language-Irish #language-Croatian #language-Hungarian #language-Italian #language-Lithuanian #language-Latvian #language-Maltese #language-Dutch #language-Polish #language-Portuguese #language-Romanian #language-Slovak #language-Slovenian #language-Swedish #license-cc-by-4.0 #region-us \n",
"### Dataset Summary\n\n\nThis dataset contains large text resources (~179GB in total) from EURLEX that can be used for pretraining language models.\n\n\nUse the dataset like this:",
"### Supported Tasks and Leaderboards\n\n\nThe dataset supports the task of masked language modeling.",
"### Languages\n\n\nThe following languages are supported: bg, cs, da, de, el, en, es, et, fi, fr, ga, hr, hu, it, lt, lv, mt, nl, pl, pt, ro, sk, sl, sv\n\n\nDataset Structure\n-----------------",
"### Data Instances\n\n\nThe file format is URL and there is one split available (\"train\").\nThe following resource types are supported: caselaw, decision, directive, intagr, proposal, recommendation, regulation\n\n\nMore information about the resource types can be found here:\n\n\n* Caselaw: EU\n* Decision: EU, Wikipedia)\n* Directive: EU, Wikipedia)\n* Recommendation: EU, Wikipedia)\n* Regulation: EU, Wikipedia)\n* Intagr: EU, Wikipedia\n* Proposal: No resource found",
"### Data Fields",
"### Data Splits\n\n\nDataset Creation\n----------------",
"### Curation Rationale",
"### Source Data",
"#### Initial Data Collection and Normalization\n\n\nThe data has been downloaded using the R package eurlex between June and August 2022.",
"#### Who are the source language producers?",
"### Annotations",
"#### Annotation process",
"#### Who are the annotators?",
"### Personal and Sensitive Information\n\n\nConsiderations for Using the Data\n---------------------------------",
"### Social Impact of Dataset",
"### Discussion of Biases",
"### Other Known Limitations\n\n\nAdditional Information\n----------------------",
"### Dataset Curators",
"### Licensing Information\n\n\nCC BY 4.0\nsee also the legal notice",
"### Contributions\n\n\nThanks to @JoelNiklaus for adding this dataset."
] | [
209,
41,
24,
71,
113,
5,
11,
7,
4,
28,
10,
5,
5,
9,
18,
7,
8,
14,
6,
14,
18
] | [
"passage: TAGS\n#task_categories-fill-mask #annotations_creators-other #language_creators-found #multilinguality-multilingual #size_categories-1M<n<10M #source_datasets-original #language-Bulgarian #language-Czech #language-Danish #language-German #language-Modern Greek (1453-) #language-English #language-Spanish #language-Estonian #language-Finnish #language-French #language-Irish #language-Croatian #language-Hungarian #language-Italian #language-Lithuanian #language-Latvian #language-Maltese #language-Dutch #language-Polish #language-Portuguese #language-Romanian #language-Slovak #language-Slovenian #language-Swedish #license-cc-by-4.0 #region-us \n### Dataset Summary\n\n\nThis dataset contains large text resources (~179GB in total) from EURLEX that can be used for pretraining language models.\n\n\nUse the dataset like this:### Supported Tasks and Leaderboards\n\n\nThe dataset supports the task of masked language modeling.### Languages\n\n\nThe following languages are supported: bg, cs, da, de, el, en, es, et, fi, fr, ga, hr, hu, it, lt, lv, mt, nl, pl, pt, ro, sk, sl, sv\n\n\nDataset Structure\n-----------------### Data Instances\n\n\nThe file format is URL and there is one split available (\"train\").\nThe following resource types are supported: caselaw, decision, directive, intagr, proposal, recommendation, regulation\n\n\nMore information about the resource types can be found here:\n\n\n* Caselaw: EU\n* Decision: EU, Wikipedia)\n* Directive: EU, Wikipedia)\n* Recommendation: EU, Wikipedia)\n* Regulation: EU, Wikipedia)\n* Intagr: EU, Wikipedia\n* Proposal: No resource found### Data Fields### Data Splits\n\n\nDataset Creation\n----------------### Curation Rationale### Source Data"
] |
e5f041fc5d507821b395ff746d57f97818bd8db1 |
# Dataset Card for Weakly supervised AG News Dataset
## Table of Contents
- [Table of Contents](#table-of-contents)
- [Dataset Description](#dataset-description)
- [Dataset Summary](#dataset-summary)
- [Supported Tasks and Leaderboards](#supported-tasks-and-leaderboards)
- [Languages](#languages)
- [Dataset Structure](#dataset-structure)
- [Data Instances](#data-instances)
- [Data Fields](#data-fields)
- [Data Splits](#data-splits)
- [Dataset Creation](#dataset-creation)
- [Curation Rationale](#curation-rationale)
- [Source Data](#source-data)
- [Annotations](#annotations)
- [Personal and Sensitive Information](#personal-and-sensitive-information)
- [Considerations for Using the Data](#considerations-for-using-the-data)
- [Social Impact of Dataset](#social-impact-of-dataset)
- [Discussion of Biases](#discussion-of-biases)
- [Other Known Limitations](#other-known-limitations)
- [Additional Information](#additional-information)
- [Dataset Curators](#dataset-curators)
- [Licensing Information](#licensing-information)
- [Citation Information](#citation-information)
- [Contributions](#contributions)
## Dataset Description
### Dataset Summary
AG is a collection of more than 1 million news articles. News articles have been gathered from more than 2000 news sources by ComeToMyHead in more than 1 year of activity. ComeToMyHead is an academic news search engine which has been running since July, 2004. The dataset is provided by the academic comunity for research purposes in data mining (clustering, classification, etc), information retrieval (ranking, search, etc), xml, data compression, data streaming, and any other non-commercial activity. For more information, please refer to the link http://www.di.unipi.it/~gulli/AG_corpus_of_news_articles.html .
The Weakly supervised AG News Dataset was created by Team 44 of FSDL 2022 course with the only purpose of experimenting with weak supervision techniques. It was assumed that only the labels of the original test set and 20% of the training set were available. The labels in the training set were obtained by creating weak labels with LFs and denoising them with Snorkel's label model.
### Supported Tasks and Leaderboards
[More Information Needed]
### Languages
English
## Dataset Structure
### Data Instances
[More Information Needed]
### Data Fields
text: a string feature
label: a classification label, with possible values including World (0), Sports (1), Business (2), Sci/Tech (3).
### Data Splits
- Training set with probabilistic labels from weak supervision: 37340
- Unlabeled data: 58660
- Validation set: 24000
- Test set: 7600
## Dataset Creation
### Curation Rationale
[More Information Needed]
### Source Data
#### Initial Data Collection and Normalization
[More Information Needed]
#### Who are the source language producers?
[More Information Needed]
### Annotations
#### Annotation process
[More Information Needed]
#### Who are the annotators?
[More Information Needed]
### Personal and Sensitive Information
[More Information Needed]
## Considerations for Using the Data
### Social Impact of Dataset
[More Information Needed]
### Discussion of Biases
[More Information Needed]
### Other Known Limitations
[More Information Needed]
## Additional Information
### Dataset Curators
[More Information Needed]
### Licensing Information
[More Information Needed]
### Citation Information
[More Information Needed]
### Contributions
Thanks to Xiang Zhang ([email protected]) for adding this dataset to the HF Dataset Hub. | bergr7/weakly_supervised_ag_news | [
"task_categories:text-classification",
"task_ids:multi-class-classification",
"language_creators:other",
"multilinguality:monolingual",
"size_categories:1K<n<10K",
"source_datasets:extended|ag_news",
"language:en",
"region:us"
] | 2022-09-29T07:43:34+00:00 | {"annotations_creators": [], "language_creators": ["other"], "language": ["en"], "license": [], "multilinguality": ["monolingual"], "size_categories": ["1K<n<10K"], "source_datasets": ["extended|ag_news"], "task_categories": ["text-classification"], "task_ids": ["multi-class-classification"], "pretty_name": "Weakly supervised AG News Dataset", "tags": []} | 2022-10-06T11:51:52+00:00 | [] | [
"en"
] | TAGS
#task_categories-text-classification #task_ids-multi-class-classification #language_creators-other #multilinguality-monolingual #size_categories-1K<n<10K #source_datasets-extended|ag_news #language-English #region-us
|
# Dataset Card for Weakly supervised AG News Dataset
## Table of Contents
- Table of Contents
- Dataset Description
- Dataset Summary
- Supported Tasks and Leaderboards
- Languages
- Dataset Structure
- Data Instances
- Data Fields
- Data Splits
- Dataset Creation
- Curation Rationale
- Source Data
- Annotations
- Personal and Sensitive Information
- Considerations for Using the Data
- Social Impact of Dataset
- Discussion of Biases
- Other Known Limitations
- Additional Information
- Dataset Curators
- Licensing Information
- Citation Information
- Contributions
## Dataset Description
### Dataset Summary
AG is a collection of more than 1 million news articles. News articles have been gathered from more than 2000 news sources by ComeToMyHead in more than 1 year of activity. ComeToMyHead is an academic news search engine which has been running since July, 2004. The dataset is provided by the academic comunity for research purposes in data mining (clustering, classification, etc), information retrieval (ranking, search, etc), xml, data compression, data streaming, and any other non-commercial activity. For more information, please refer to the link URL .
The Weakly supervised AG News Dataset was created by Team 44 of FSDL 2022 course with the only purpose of experimenting with weak supervision techniques. It was assumed that only the labels of the original test set and 20% of the training set were available. The labels in the training set were obtained by creating weak labels with LFs and denoising them with Snorkel's label model.
### Supported Tasks and Leaderboards
### Languages
English
## Dataset Structure
### Data Instances
### Data Fields
text: a string feature
label: a classification label, with possible values including World (0), Sports (1), Business (2), Sci/Tech (3).
### Data Splits
- Training set with probabilistic labels from weak supervision: 37340
- Unlabeled data: 58660
- Validation set: 24000
- Test set: 7600
## Dataset Creation
### Curation Rationale
### Source Data
#### Initial Data Collection and Normalization
#### Who are the source language producers?
### Annotations
#### Annotation process
#### Who are the annotators?
### Personal and Sensitive Information
## Considerations for Using the Data
### Social Impact of Dataset
### Discussion of Biases
### Other Known Limitations
## Additional Information
### Dataset Curators
### Licensing Information
### Contributions
Thanks to Xiang Zhang (URL@URL) for adding this dataset to the HF Dataset Hub. | [
"# Dataset Card for Weakly supervised AG News Dataset",
"## Table of Contents\n- Table of Contents\n- Dataset Description\n - Dataset Summary\n - Supported Tasks and Leaderboards\n - Languages\n- Dataset Structure\n - Data Instances\n - Data Fields\n - Data Splits\n- Dataset Creation\n - Curation Rationale\n - Source Data\n - Annotations\n - Personal and Sensitive Information\n- Considerations for Using the Data\n - Social Impact of Dataset\n - Discussion of Biases\n - Other Known Limitations\n- Additional Information\n - Dataset Curators\n - Licensing Information\n - Citation Information\n - Contributions",
"## Dataset Description",
"### Dataset Summary\n\nAG is a collection of more than 1 million news articles. News articles have been gathered from more than 2000 news sources by ComeToMyHead in more than 1 year of activity. ComeToMyHead is an academic news search engine which has been running since July, 2004. The dataset is provided by the academic comunity for research purposes in data mining (clustering, classification, etc), information retrieval (ranking, search, etc), xml, data compression, data streaming, and any other non-commercial activity. For more information, please refer to the link URL .\n\nThe Weakly supervised AG News Dataset was created by Team 44 of FSDL 2022 course with the only purpose of experimenting with weak supervision techniques. It was assumed that only the labels of the original test set and 20% of the training set were available. The labels in the training set were obtained by creating weak labels with LFs and denoising them with Snorkel's label model.",
"### Supported Tasks and Leaderboards",
"### Languages\n\nEnglish",
"## Dataset Structure",
"### Data Instances",
"### Data Fields\n\ntext: a string feature\nlabel: a classification label, with possible values including World (0), Sports (1), Business (2), Sci/Tech (3).",
"### Data Splits\n\n- Training set with probabilistic labels from weak supervision: 37340\n- Unlabeled data: 58660\n- Validation set: 24000\n- Test set: 7600",
"## Dataset Creation",
"### Curation Rationale",
"### Source Data",
"#### Initial Data Collection and Normalization",
"#### Who are the source language producers?",
"### Annotations",
"#### Annotation process",
"#### Who are the annotators?",
"### Personal and Sensitive Information",
"## Considerations for Using the Data",
"### Social Impact of Dataset",
"### Discussion of Biases",
"### Other Known Limitations",
"## Additional Information",
"### Dataset Curators",
"### Licensing Information",
"### Contributions\n\nThanks to Xiang Zhang (URL@URL) for adding this dataset to the HF Dataset Hub."
] | [
"TAGS\n#task_categories-text-classification #task_ids-multi-class-classification #language_creators-other #multilinguality-monolingual #size_categories-1K<n<10K #source_datasets-extended|ag_news #language-English #region-us \n",
"# Dataset Card for Weakly supervised AG News Dataset",
"## Table of Contents\n- Table of Contents\n- Dataset Description\n - Dataset Summary\n - Supported Tasks and Leaderboards\n - Languages\n- Dataset Structure\n - Data Instances\n - Data Fields\n - Data Splits\n- Dataset Creation\n - Curation Rationale\n - Source Data\n - Annotations\n - Personal and Sensitive Information\n- Considerations for Using the Data\n - Social Impact of Dataset\n - Discussion of Biases\n - Other Known Limitations\n- Additional Information\n - Dataset Curators\n - Licensing Information\n - Citation Information\n - Contributions",
"## Dataset Description",
"### Dataset Summary\n\nAG is a collection of more than 1 million news articles. News articles have been gathered from more than 2000 news sources by ComeToMyHead in more than 1 year of activity. ComeToMyHead is an academic news search engine which has been running since July, 2004. The dataset is provided by the academic comunity for research purposes in data mining (clustering, classification, etc), information retrieval (ranking, search, etc), xml, data compression, data streaming, and any other non-commercial activity. For more information, please refer to the link URL .\n\nThe Weakly supervised AG News Dataset was created by Team 44 of FSDL 2022 course with the only purpose of experimenting with weak supervision techniques. It was assumed that only the labels of the original test set and 20% of the training set were available. The labels in the training set were obtained by creating weak labels with LFs and denoising them with Snorkel's label model.",
"### Supported Tasks and Leaderboards",
"### Languages\n\nEnglish",
"## Dataset Structure",
"### Data Instances",
"### Data Fields\n\ntext: a string feature\nlabel: a classification label, with possible values including World (0), Sports (1), Business (2), Sci/Tech (3).",
"### Data Splits\n\n- Training set with probabilistic labels from weak supervision: 37340\n- Unlabeled data: 58660\n- Validation set: 24000\n- Test set: 7600",
"## Dataset Creation",
"### Curation Rationale",
"### Source Data",
"#### Initial Data Collection and Normalization",
"#### Who are the source language producers?",
"### Annotations",
"#### Annotation process",
"#### Who are the annotators?",
"### Personal and Sensitive Information",
"## Considerations for Using the Data",
"### Social Impact of Dataset",
"### Discussion of Biases",
"### Other Known Limitations",
"## Additional Information",
"### Dataset Curators",
"### Licensing Information",
"### Contributions\n\nThanks to Xiang Zhang (URL@URL) for adding this dataset to the HF Dataset Hub."
] | [
75,
15,
125,
4,
228,
10,
5,
6,
6,
35,
43,
5,
7,
4,
10,
10,
5,
5,
9,
8,
8,
7,
8,
7,
5,
6,
6,
29
] | [
"passage: TAGS\n#task_categories-text-classification #task_ids-multi-class-classification #language_creators-other #multilinguality-monolingual #size_categories-1K<n<10K #source_datasets-extended|ag_news #language-English #region-us \n# Dataset Card for Weakly supervised AG News Dataset## Table of Contents\n- Table of Contents\n- Dataset Description\n - Dataset Summary\n - Supported Tasks and Leaderboards\n - Languages\n- Dataset Structure\n - Data Instances\n - Data Fields\n - Data Splits\n- Dataset Creation\n - Curation Rationale\n - Source Data\n - Annotations\n - Personal and Sensitive Information\n- Considerations for Using the Data\n - Social Impact of Dataset\n - Discussion of Biases\n - Other Known Limitations\n- Additional Information\n - Dataset Curators\n - Licensing Information\n - Citation Information\n - Contributions## Dataset Description### Dataset Summary\n\nAG is a collection of more than 1 million news articles. News articles have been gathered from more than 2000 news sources by ComeToMyHead in more than 1 year of activity. ComeToMyHead is an academic news search engine which has been running since July, 2004. The dataset is provided by the academic comunity for research purposes in data mining (clustering, classification, etc), information retrieval (ranking, search, etc), xml, data compression, data streaming, and any other non-commercial activity. For more information, please refer to the link URL .\n\nThe Weakly supervised AG News Dataset was created by Team 44 of FSDL 2022 course with the only purpose of experimenting with weak supervision techniques. It was assumed that only the labels of the original test set and 20% of the training set were available. The labels in the training set were obtained by creating weak labels with LFs and denoising them with Snorkel's label model.### Supported Tasks and Leaderboards### Languages\n\nEnglish## Dataset Structure### Data Instances"
] |
f6323032886e971c842c7b0b5b9f3592e6e2bd0a | Ces images de nuages sont divisรฉes en 2 classes, les cirrus et les cumulus.
These cloud images are divided into 2 classes, cirrus and cumulus. | Doudou69/Cloud_Recognition | [
"region:us"
] | 2022-09-29T08:48:44+00:00 | {} | 2022-09-29T09:19:04+00:00 | [] | [] | TAGS
#region-us
| Ces images de nuages sont divisรฉes en 2 classes, les cirrus et les cumulus.
These cloud images are divided into 2 classes, cirrus and cumulus. | [] | [
"TAGS\n#region-us \n"
] | [
6
] | [
"passage: TAGS\n#region-us \n"
] |
81b731b90a2a11229c78e6791d0d8c1ccf6833d4 | # Dataset Card for [Dataset Name]
## Table of Contents
- [Table of Contents](#table-of-contents)
- [Dataset Description](#dataset-description)
- [Dataset Summary](#dataset-summary)
- [Supported Tasks and Leaderboards](#supported-tasks-and-leaderboards)
- [Languages](#languages)
- [Dataset Structure](#dataset-structure)
- [Data Instances](#data-instances)
- [Data Fields](#data-fields)
- [Data Splits](#data-splits)
- [Dataset Creation](#dataset-creation)
- [Curation Rationale](#curation-rationale)
- [Source Data](#source-data)
- [Annotations](#annotations)
- [Personal and Sensitive Information](#personal-and-sensitive-information)
- [Considerations for Using the Data](#considerations-for-using-the-data)
- [Social Impact of Dataset](#social-impact-of-dataset)
- [Discussion of Biases](#discussion-of-biases)
- [Other Known Limitations](#other-known-limitations)
- [Additional Information](#additional-information)
- [Dataset Curators](#dataset-curators)
- [Licensing Information](#licensing-information)
- [Citation Information](#citation-information)
- [Contributions](#contributions)
## Dataset Description
- **Homepage:**
- **Repository:**
- **Paper:**
- **Leaderboard:**
- **Point of Contact:**
### Dataset Summary
[More Information Needed]
### Supported Tasks and Leaderboards
[More Information Needed]
### Languages
[More Information Needed]
## Dataset Structure
### Data Instances
[More Information Needed]
### Data Fields
[More Information Needed]
### Data Splits
[More Information Needed]
## Dataset Creation
### Curation Rationale
[More Information Needed]
### Source Data
#### Initial Data Collection and Normalization
[More Information Needed]
#### Who are the source language producers?
[More Information Needed]
### Annotations
#### Annotation process
[More Information Needed]
#### Who are the annotators?
[More Information Needed]
### Personal and Sensitive Information
[More Information Needed]
## Considerations for Using the Data
### Social Impact of Dataset
[More Information Needed]
### Discussion of Biases
[More Information Needed]
### Other Known Limitations
[More Information Needed]
## Additional Information
### Dataset Curators
[More Information Needed]
### Licensing Information
[More Information Needed]
### Citation Information
[More Information Needed]
### Contributions
Thanks to [@github-username](https://github.com/<github-username>) for adding this dataset.
| merkalo-ziri/vsosh2022 | [
"task_categories:text-classification",
"task_ids:sentiment-classification",
"annotations_creators:found",
"language_creators:found",
"multilinguality:monolingual",
"size_categories:1K<n<10K",
"language:ru",
"license:other",
"region:us"
] | 2022-09-29T09:35:38+00:00 | {"annotations_creators": ["found"], "language_creators": ["found"], "language": ["ru"], "license": ["other"], "multilinguality": ["monolingual"], "size_categories": ["1K<n<10K"], "source_datasets": [], "task_categories": ["text-classification"], "task_ids": ["sentiment-classification"], "pretty_name": "vsosh_dataset", "tags": []} | 2022-09-29T10:02:34+00:00 | [] | [
"ru"
] | TAGS
#task_categories-text-classification #task_ids-sentiment-classification #annotations_creators-found #language_creators-found #multilinguality-monolingual #size_categories-1K<n<10K #language-Russian #license-other #region-us
| # Dataset Card for [Dataset Name]
## Table of Contents
- Table of Contents
- Dataset Description
- Dataset Summary
- Supported Tasks and Leaderboards
- Languages
- Dataset Structure
- Data Instances
- Data Fields
- Data Splits
- Dataset Creation
- Curation Rationale
- Source Data
- Annotations
- Personal and Sensitive Information
- Considerations for Using the Data
- Social Impact of Dataset
- Discussion of Biases
- Other Known Limitations
- Additional Information
- Dataset Curators
- Licensing Information
- Citation Information
- Contributions
## Dataset Description
- Homepage:
- Repository:
- Paper:
- Leaderboard:
- Point of Contact:
### Dataset Summary
### Supported Tasks and Leaderboards
### Languages
## Dataset Structure
### Data Instances
### Data Fields
### Data Splits
## Dataset Creation
### Curation Rationale
### Source Data
#### Initial Data Collection and Normalization
#### Who are the source language producers?
### Annotations
#### Annotation process
#### Who are the annotators?
### Personal and Sensitive Information
## Considerations for Using the Data
### Social Impact of Dataset
### Discussion of Biases
### Other Known Limitations
## Additional Information
### Dataset Curators
### Licensing Information
### Contributions
Thanks to @github-username for adding this dataset.
| [
"# Dataset Card for [Dataset Name]",
"## Table of Contents\n- Table of Contents\n- Dataset Description\n - Dataset Summary\n - Supported Tasks and Leaderboards\n - Languages\n- Dataset Structure\n - Data Instances\n - Data Fields\n - Data Splits\n- Dataset Creation\n - Curation Rationale\n - Source Data\n - Annotations\n - Personal and Sensitive Information\n- Considerations for Using the Data\n - Social Impact of Dataset\n - Discussion of Biases\n - Other Known Limitations\n- Additional Information\n - Dataset Curators\n - Licensing Information\n - Citation Information\n - Contributions",
"## Dataset Description\n- Homepage:\n- Repository:\n- Paper:\n- Leaderboard:\n- Point of Contact:",
"### Dataset Summary",
"### Supported Tasks and Leaderboards",
"### Languages",
"## Dataset Structure",
"### Data Instances",
"### Data Fields",
"### Data Splits",
"## Dataset Creation",
"### Curation Rationale",
"### Source Data",
"#### Initial Data Collection and Normalization",
"#### Who are the source language producers?",
"### Annotations",
"#### Annotation process",
"#### Who are the annotators?",
"### Personal and Sensitive Information",
"## Considerations for Using the Data",
"### Social Impact of Dataset",
"### Discussion of Biases",
"### Other Known Limitations",
"## Additional Information",
"### Dataset Curators",
"### Licensing Information",
"### Contributions\nThanks to @github-username for adding this dataset."
] | [
"TAGS\n#task_categories-text-classification #task_ids-sentiment-classification #annotations_creators-found #language_creators-found #multilinguality-monolingual #size_categories-1K<n<10K #language-Russian #license-other #region-us \n",
"# Dataset Card for [Dataset Name]",
"## Table of Contents\n- Table of Contents\n- Dataset Description\n - Dataset Summary\n - Supported Tasks and Leaderboards\n - Languages\n- Dataset Structure\n - Data Instances\n - Data Fields\n - Data Splits\n- Dataset Creation\n - Curation Rationale\n - Source Data\n - Annotations\n - Personal and Sensitive Information\n- Considerations for Using the Data\n - Social Impact of Dataset\n - Discussion of Biases\n - Other Known Limitations\n- Additional Information\n - Dataset Curators\n - Licensing Information\n - Citation Information\n - Contributions",
"## Dataset Description\n- Homepage:\n- Repository:\n- Paper:\n- Leaderboard:\n- Point of Contact:",
"### Dataset Summary",
"### Supported Tasks and Leaderboards",
"### Languages",
"## Dataset Structure",
"### Data Instances",
"### Data Fields",
"### Data Splits",
"## Dataset Creation",
"### Curation Rationale",
"### Source Data",
"#### Initial Data Collection and Normalization",
"#### Who are the source language producers?",
"### Annotations",
"#### Annotation process",
"#### Who are the annotators?",
"### Personal and Sensitive Information",
"## Considerations for Using the Data",
"### Social Impact of Dataset",
"### Discussion of Biases",
"### Other Known Limitations",
"## Additional Information",
"### Dataset Curators",
"### Licensing Information",
"### Contributions\nThanks to @github-username for adding this dataset."
] | [
76,
10,
125,
24,
6,
10,
4,
6,
6,
5,
5,
5,
7,
4,
10,
10,
5,
5,
9,
8,
8,
7,
8,
7,
5,
6,
6,
19
] | [
"passage: TAGS\n#task_categories-text-classification #task_ids-sentiment-classification #annotations_creators-found #language_creators-found #multilinguality-monolingual #size_categories-1K<n<10K #language-Russian #license-other #region-us \n# Dataset Card for [Dataset Name]## Table of Contents\n- Table of Contents\n- Dataset Description\n - Dataset Summary\n - Supported Tasks and Leaderboards\n - Languages\n- Dataset Structure\n - Data Instances\n - Data Fields\n - Data Splits\n- Dataset Creation\n - Curation Rationale\n - Source Data\n - Annotations\n - Personal and Sensitive Information\n- Considerations for Using the Data\n - Social Impact of Dataset\n - Discussion of Biases\n - Other Known Limitations\n- Additional Information\n - Dataset Curators\n - Licensing Information\n - Citation Information\n - Contributions## Dataset Description\n- Homepage:\n- Repository:\n- Paper:\n- Leaderboard:\n- Point of Contact:### Dataset Summary### Supported Tasks and Leaderboards### Languages## Dataset Structure### Data Instances### Data Fields### Data Splits## Dataset Creation### Curation Rationale### Source Data#### Initial Data Collection and Normalization#### Who are the source language producers?### Annotations#### Annotation process#### Who are the annotators?### Personal and Sensitive Information## Considerations for Using the Data### Social Impact of Dataset### Discussion of Biases### Other Known Limitations## Additional Information### Dataset Curators### Licensing Information### Contributions\nThanks to @github-username for adding this dataset."
] |
e214dad7ae9dd678a2f01c9220d45d42c94c8f91 |
# Dataset Card for MC4_Legal: A Corpus Covering the Legal Part of MC4 for European Languages
## Table of Contents
- [Table of Contents](#table-of-contents)
- [Dataset Description](#dataset-description)
- [Dataset Summary](#dataset-summary)
- [Supported Tasks and Leaderboards](#supported-tasks-and-leaderboards)
- [Languages](#languages)
- [Dataset Structure](#dataset-structure)
- [Data Instances](#data-instances)
- [Data Fields](#data-fields)
- [Data Splits](#data-splits)
- [Dataset Creation](#dataset-creation)
- [Curation Rationale](#curation-rationale)
- [Source Data](#source-data)
- [Annotations](#annotations)
- [Personal and Sensitive Information](#personal-and-sensitive-information)
- [Considerations for Using the Data](#considerations-for-using-the-data)
- [Social Impact of Dataset](#social-impact-of-dataset)
- [Discussion of Biases](#discussion-of-biases)
- [Other Known Limitations](#other-known-limitations)
- [Additional Information](#additional-information)
- [Dataset Curators](#dataset-curators)
- [Licensing Information](#licensing-information)
- [Citation Information](#citation-information)
- [Contributions](#contributions)
## Dataset Description
- **Homepage:**
- **Repository:** [GitHub](https://github.com/JoelNiklaus/LegalDatasets/tree/main/pretrain/mc4_legal)
- **Paper:**
- **Leaderboard:**
- **Point of Contact:** [Joel Niklaus](mailto:[email protected])
### Dataset Summary
This dataset contains large text resources (~133GB in total) from mc4 filtered for legal data that can be used for pretraining language models.
Use the dataset like this:
```python
from datasets import load_dataset
dataset = load_dataset("joelito/mc4_legal", "de", split='train', streaming=True)
```
### Supported Tasks and Leaderboards
The dataset supports the task of masked language modeling.
### Languages
The following languages are supported: bg, cs, da, de, el, en, es, et, fi, fr, ga, hu, it, lt, lv, mt, nl, pl, pt, ro, sk, sl, sv
## Dataset Structure
### Data Instances
The file format is jsonl.xz and there is one split available ("train").
| Source | Size (MB) | Words | Documents | Words/Document |
|:---------|------------:|------------:|------------:|-----------------:|
| all | 448980 | 28599300521 | 9873288 | 2896 |
| bg | 57 | 2390349 | 379 | 6306 |
| cs | 31005 | 1840827375 | 677796 | 2715 |
| da | 162 | 10466716 | 3231 | 3239 |
| de | 105739 | 6184578784 | 3164461 | 1954 |
| el | 30 | 1155977 | 307 | 3765 |
| en | 13734 | 966539309 | 359283 | 2690 |
| es | 132053 | 9058939804 | 2281888 | 3969 |
| et | 2059 | 110198368 | 49987 | 2204 |
| fi | 1270 | 62799074 | 44875 | 1399 |
| fr | 30878 | 2117306229 | 598983 | 3534 |
| ga | 1 | 32772 | 8 | 4096 |
| hu | 4677 | 244911748 | 58857 | 4161 |
| it | 46957 | 3053920779 | 990823 | 3082 |
| lt | 156 | 9142223 | 1529 | 5979 |
| lv | 1 | 58702 | 16 | 3668 |
| mt | 65 | 3479869 | 731 | 4760 |
| nl | 326 | 21962633 | 6875 | 3194 |
| pl | 37950 | 2235839721 | 827641 | 2701 |
| pt | 20120 | 1338147828 | 382173 | 3501 |
| ro | 8816 | 551372510 | 136513 | 4038 |
| sk | 5850 | 349265172 | 130701 | 2672 |
| sl | 1742 | 107493024 | 32574 | 3299 |
| sv | 5332 | 328471555 | 123657 | 2656 |
### Data Fields
[More Information Needed]
### Data Splits
[More Information Needed]
## Dataset Creation
The dataset was created by filtering mc4 for legal data.
We used terms indicating legal citations to get the texts.
Note that this dataset can be quite noisy, and the quality is not known.
### Curation Rationale
[More Information Needed]
### Source Data
#### Initial Data Collection and Normalization
[More Information Needed]
#### Who are the source language producers?
[More Information Needed]
### Annotations
#### Annotation process
[More Information Needed]
#### Who are the annotators?
[More Information Needed]
### Personal and Sensitive Information
[More Information Needed]
## Considerations for Using the Data
### Social Impact of Dataset
[More Information Needed]
### Discussion of Biases
[More Information Needed]
### Other Known Limitations
[More Information Needed]
## Additional Information
### Dataset Curators
[More Information Needed]
### Licensing Information
[More Information Needed]
### Citation Information
[More Information Needed]
### Contributions
Thanks to [@JoelNiklaus](https://github.com/joelniklaus) for adding this dataset.
| joelniklaus/mc4_legal | [
"task_categories:fill-mask",
"annotations_creators:other",
"language_creators:found",
"multilinguality:multilingual",
"size_categories:10M<n<100M",
"source_datasets:original",
"language:bg",
"language:cs",
"language:da",
"language:de",
"language:el",
"language:en",
"language:es",
"language:et",
"language:fi",
"language:fr",
"language:ga",
"language:hu",
"language:it",
"language:lt",
"language:lv",
"language:mt",
"language:nl",
"language:pl",
"language:pt",
"language:ro",
"language:sk",
"language:sl",
"language:sv",
"license:cc-by-4.0",
"region:us"
] | 2022-09-29T09:53:01+00:00 | {"annotations_creators": ["other"], "language_creators": ["found"], "language": ["bg", "cs", "da", "de", "el", "en", "es", "et", "fi", "fr", "ga", "hu", "it", "lt", "lv", "mt", "nl", "pl", "pt", "ro", "sk", "sl", "sv"], "license": ["cc-by-4.0"], "multilinguality": ["multilingual"], "size_categories": ["10M<n<100M"], "source_datasets": ["original"], "task_categories": ["fill-mask"], "pretty_name": "MC4_Legal: A Corpus Covering the Legal Part of MC4 for European Languages"} | 2023-03-20T23:24:13+00:00 | [] | [
"bg",
"cs",
"da",
"de",
"el",
"en",
"es",
"et",
"fi",
"fr",
"ga",
"hu",
"it",
"lt",
"lv",
"mt",
"nl",
"pl",
"pt",
"ro",
"sk",
"sl",
"sv"
] | TAGS
#task_categories-fill-mask #annotations_creators-other #language_creators-found #multilinguality-multilingual #size_categories-10M<n<100M #source_datasets-original #language-Bulgarian #language-Czech #language-Danish #language-German #language-Modern Greek (1453-) #language-English #language-Spanish #language-Estonian #language-Finnish #language-French #language-Irish #language-Hungarian #language-Italian #language-Lithuanian #language-Latvian #language-Maltese #language-Dutch #language-Polish #language-Portuguese #language-Romanian #language-Slovak #language-Slovenian #language-Swedish #license-cc-by-4.0 #region-us
| Dataset Card for MC4\_Legal: A Corpus Covering the Legal Part of MC4 for European Languages
===========================================================================================
Table of Contents
-----------------
* Table of Contents
* Dataset Description
+ Dataset Summary
+ Supported Tasks and Leaderboards
+ Languages
* Dataset Structure
+ Data Instances
+ Data Fields
+ Data Splits
* Dataset Creation
+ Curation Rationale
+ Source Data
+ Annotations
+ Personal and Sensitive Information
* Considerations for Using the Data
+ Social Impact of Dataset
+ Discussion of Biases
+ Other Known Limitations
* Additional Information
+ Dataset Curators
+ Licensing Information
+ Citation Information
+ Contributions
Dataset Description
-------------------
* Homepage:
* Repository: GitHub
* Paper:
* Leaderboard:
* Point of Contact: Joel Niklaus
### Dataset Summary
This dataset contains large text resources (~133GB in total) from mc4 filtered for legal data that can be used for pretraining language models.
Use the dataset like this:
### Supported Tasks and Leaderboards
The dataset supports the task of masked language modeling.
### Languages
The following languages are supported: bg, cs, da, de, el, en, es, et, fi, fr, ga, hu, it, lt, lv, mt, nl, pl, pt, ro, sk, sl, sv
Dataset Structure
-----------------
### Data Instances
The file format is URL and there is one split available ("train").
### Data Fields
### Data Splits
Dataset Creation
----------------
The dataset was created by filtering mc4 for legal data.
We used terms indicating legal citations to get the texts.
Note that this dataset can be quite noisy, and the quality is not known.
### Curation Rationale
### Source Data
#### Initial Data Collection and Normalization
#### Who are the source language producers?
### Annotations
#### Annotation process
#### Who are the annotators?
### Personal and Sensitive Information
Considerations for Using the Data
---------------------------------
### Social Impact of Dataset
### Discussion of Biases
### Other Known Limitations
Additional Information
----------------------
### Dataset Curators
### Licensing Information
### Contributions
Thanks to @JoelNiklaus for adding this dataset.
| [
"### Dataset Summary\n\n\nThis dataset contains large text resources (~133GB in total) from mc4 filtered for legal data that can be used for pretraining language models.\n\n\nUse the dataset like this:",
"### Supported Tasks and Leaderboards\n\n\nThe dataset supports the task of masked language modeling.",
"### Languages\n\n\nThe following languages are supported: bg, cs, da, de, el, en, es, et, fi, fr, ga, hu, it, lt, lv, mt, nl, pl, pt, ro, sk, sl, sv\n\n\nDataset Structure\n-----------------",
"### Data Instances\n\n\nThe file format is URL and there is one split available (\"train\").",
"### Data Fields",
"### Data Splits\n\n\nDataset Creation\n----------------\n\n\nThe dataset was created by filtering mc4 for legal data.\nWe used terms indicating legal citations to get the texts.\nNote that this dataset can be quite noisy, and the quality is not known.",
"### Curation Rationale",
"### Source Data",
"#### Initial Data Collection and Normalization",
"#### Who are the source language producers?",
"### Annotations",
"#### Annotation process",
"#### Who are the annotators?",
"### Personal and Sensitive Information\n\n\nConsiderations for Using the Data\n---------------------------------",
"### Social Impact of Dataset",
"### Discussion of Biases",
"### Other Known Limitations\n\n\nAdditional Information\n----------------------",
"### Dataset Curators",
"### Licensing Information",
"### Contributions\n\n\nThanks to @JoelNiklaus for adding this dataset."
] | [
"TAGS\n#task_categories-fill-mask #annotations_creators-other #language_creators-found #multilinguality-multilingual #size_categories-10M<n<100M #source_datasets-original #language-Bulgarian #language-Czech #language-Danish #language-German #language-Modern Greek (1453-) #language-English #language-Spanish #language-Estonian #language-Finnish #language-French #language-Irish #language-Hungarian #language-Italian #language-Lithuanian #language-Latvian #language-Maltese #language-Dutch #language-Polish #language-Portuguese #language-Romanian #language-Slovak #language-Slovenian #language-Swedish #license-cc-by-4.0 #region-us \n",
"### Dataset Summary\n\n\nThis dataset contains large text resources (~133GB in total) from mc4 filtered for legal data that can be used for pretraining language models.\n\n\nUse the dataset like this:",
"### Supported Tasks and Leaderboards\n\n\nThe dataset supports the task of masked language modeling.",
"### Languages\n\n\nThe following languages are supported: bg, cs, da, de, el, en, es, et, fi, fr, ga, hu, it, lt, lv, mt, nl, pl, pt, ro, sk, sl, sv\n\n\nDataset Structure\n-----------------",
"### Data Instances\n\n\nThe file format is URL and there is one split available (\"train\").",
"### Data Fields",
"### Data Splits\n\n\nDataset Creation\n----------------\n\n\nThe dataset was created by filtering mc4 for legal data.\nWe used terms indicating legal citations to get the texts.\nNote that this dataset can be quite noisy, and the quality is not known.",
"### Curation Rationale",
"### Source Data",
"#### Initial Data Collection and Normalization",
"#### Who are the source language producers?",
"### Annotations",
"#### Annotation process",
"#### Who are the annotators?",
"### Personal and Sensitive Information\n\n\nConsiderations for Using the Data\n---------------------------------",
"### Social Impact of Dataset",
"### Discussion of Biases",
"### Other Known Limitations\n\n\nAdditional Information\n----------------------",
"### Dataset Curators",
"### Licensing Information",
"### Contributions\n\n\nThanks to @JoelNiklaus for adding this dataset."
] | [
202,
47,
24,
69,
21,
5,
58,
7,
4,
10,
10,
5,
5,
9,
18,
7,
8,
14,
6,
6,
18
] | [
"passage: TAGS\n#task_categories-fill-mask #annotations_creators-other #language_creators-found #multilinguality-multilingual #size_categories-10M<n<100M #source_datasets-original #language-Bulgarian #language-Czech #language-Danish #language-German #language-Modern Greek (1453-) #language-English #language-Spanish #language-Estonian #language-Finnish #language-French #language-Irish #language-Hungarian #language-Italian #language-Lithuanian #language-Latvian #language-Maltese #language-Dutch #language-Polish #language-Portuguese #language-Romanian #language-Slovak #language-Slovenian #language-Swedish #license-cc-by-4.0 #region-us \n### Dataset Summary\n\n\nThis dataset contains large text resources (~133GB in total) from mc4 filtered for legal data that can be used for pretraining language models.\n\n\nUse the dataset like this:### Supported Tasks and Leaderboards\n\n\nThe dataset supports the task of masked language modeling.### Languages\n\n\nThe following languages are supported: bg, cs, da, de, el, en, es, et, fi, fr, ga, hu, it, lt, lv, mt, nl, pl, pt, ro, sk, sl, sv\n\n\nDataset Structure\n-----------------### Data Instances\n\n\nThe file format is URL and there is one split available (\"train\").### Data Fields### Data Splits\n\n\nDataset Creation\n----------------\n\n\nThe dataset was created by filtering mc4 for legal data.\nWe used terms indicating legal citations to get the texts.\nNote that this dataset can be quite noisy, and the quality is not known.### Curation Rationale### Source Data#### Initial Data Collection and Normalization#### Who are the source language producers?### Annotations#### Annotation process#### Who are the annotators?### Personal and Sensitive Information\n\n\nConsiderations for Using the Data\n---------------------------------### Social Impact of Dataset"
] |
f400ef054edf219b2529b673de34ff6c49f9ac9c |
# Dataset Card for AISegment.cn - Matting Human datasets
## Table of Contents
- [Dataset Card for AISegment.cn - Matting Human datasets](#dataset-card-for-aisegmentcn---matting-human-datasets)
- [Table of Contents](#table-of-contents)
- [Dataset Description](#dataset-description)
- [Dataset Structure](#dataset-structure)
- [Licensing Information](#licensing-information)
## Dataset Description
Quoting the [dataset's github](https://github.com/aisegmentcn/matting_human_datasets) (translated by Apple Translator):
> This dataset is currently the largest portrait matting dataset, containing 34,427 images and corresponding matting results.
> The data set was marked by the high quality of Beijing Play Star Convergence Technology Co. Ltd., and the portrait soft segmentation model trained using this data set has been commercialized.
> The original images in the dataset are from `Flickr`, `Baidu`, and `Taobao`. After face detection and area cropping, a half-length portrait of 600\*800 was generated.
> The clip_img directory is a half-length portrait image in the format jpg; the matting directory is the corresponding matting file (convenient to confirm the matting quality), the format is png, you should first extract the alpha map from the png image before training.
- **Repository:** [aisegmentcn/matting_human_datasets](https://github.com/aisegmentcn/matting_human_datasets)
## Dataset Structure
```text
โโโ data/
โโโ clip_img/
โ โโโ {group-id}/
โ โโโ clip_{subgroup-id}/
โ โโโ {group-id}-{img-id}.jpg
โโโ matting/
โโโ {group-id}/
โโโ matting_{subgroup-id}/
โโโ {group-id}-{img-id}.png
```
The input `data/clip_img/1803151818/clip_00000000/1803151818-00000003.jpg` matches the label `data/matting/1803151818/matting_00000000/1803151818-00000003.png`
### Licensing Information
See authors [Github](https://github.com/aisegmentcn/matting_human_datasets)
| fredguth/aisegmentcn-matting-human | [
"task_categories:image-segmentation",
"task_ids:semantic-segmentation",
"annotations_creators:Beijing Wanxing Convergence Technology Co",
"size_categories:10K<n<100K",
"license:mit",
"binary",
"aisegment.cn",
"region:us"
] | 2022-09-29T12:32:40+00:00 | {"annotations_creators": ["Beijing Wanxing Convergence Technology Co"], "license": ["mit"], "size_categories": ["10K<n<100K"], "task_categories": ["image-segmentation"], "task_ids": ["semantic-segmentation"], "pretty_name": "aisegmentcn-matting-human", "tags": ["binary", "aisegment.cn"]} | 2022-09-29T14:18:42+00:00 | [] | [] | TAGS
#task_categories-image-segmentation #task_ids-semantic-segmentation #annotations_creators-Beijing Wanxing Convergence Technology Co #size_categories-10K<n<100K #license-mit #binary #aisegment.cn #region-us
|
# Dataset Card for URL - Matting Human datasets
## Table of Contents
- Dataset Card for URL - Matting Human datasets
- Table of Contents
- Dataset Description
- Dataset Structure
- Licensing Information
## Dataset Description
Quoting the dataset's github (translated by Apple Translator):
> This dataset is currently the largest portrait matting dataset, containing 34,427 images and corresponding matting results.
> The data set was marked by the high quality of Beijing Play Star Convergence Technology Co. Ltd., and the portrait soft segmentation model trained using this data set has been commercialized.
> The original images in the dataset are from 'Flickr', 'Baidu', and 'Taobao'. After face detection and area cropping, a half-length portrait of 600\*800 was generated.
> The clip_img directory is a half-length portrait image in the format jpg; the matting directory is the corresponding matting file (convenient to confirm the matting quality), the format is png, you should first extract the alpha map from the png image before training.
- Repository: aisegmentcn/matting_human_datasets
## Dataset Structure
The input 'data/clip_img/1803151818/clip_00000000/URL' matches the label 'data/matting/1803151818/matting_00000000/URL'
### Licensing Information
See authors Github
| [
"# Dataset Card for URL - Matting Human datasets",
"## Table of Contents\n\n- Dataset Card for URL - Matting Human datasets\n - Table of Contents\n - Dataset Description\n - Dataset Structure\n - Licensing Information",
"## Dataset Description\n\nQuoting the dataset's github (translated by Apple Translator):\n\n> This dataset is currently the largest portrait matting dataset, containing 34,427 images and corresponding matting results.\n> The data set was marked by the high quality of Beijing Play Star Convergence Technology Co. Ltd., and the portrait soft segmentation model trained using this data set has been commercialized.\n\n> The original images in the dataset are from 'Flickr', 'Baidu', and 'Taobao'. After face detection and area cropping, a half-length portrait of 600\\*800 was generated.\n> The clip_img directory is a half-length portrait image in the format jpg; the matting directory is the corresponding matting file (convenient to confirm the matting quality), the format is png, you should first extract the alpha map from the png image before training.\n\n- Repository: aisegmentcn/matting_human_datasets",
"## Dataset Structure\n\n\n\nThe input 'data/clip_img/1803151818/clip_00000000/URL' matches the label 'data/matting/1803151818/matting_00000000/URL'",
"### Licensing Information\n\nSee authors Github"
] | [
"TAGS\n#task_categories-image-segmentation #task_ids-semantic-segmentation #annotations_creators-Beijing Wanxing Convergence Technology Co #size_categories-10K<n<100K #license-mit #binary #aisegment.cn #region-us \n",
"# Dataset Card for URL - Matting Human datasets",
"## Table of Contents\n\n- Dataset Card for URL - Matting Human datasets\n - Table of Contents\n - Dataset Description\n - Dataset Structure\n - Licensing Information",
"## Dataset Description\n\nQuoting the dataset's github (translated by Apple Translator):\n\n> This dataset is currently the largest portrait matting dataset, containing 34,427 images and corresponding matting results.\n> The data set was marked by the high quality of Beijing Play Star Convergence Technology Co. Ltd., and the portrait soft segmentation model trained using this data set has been commercialized.\n\n> The original images in the dataset are from 'Flickr', 'Baidu', and 'Taobao'. After face detection and area cropping, a half-length portrait of 600\\*800 was generated.\n> The clip_img directory is a half-length portrait image in the format jpg; the matting directory is the corresponding matting file (convenient to confirm the matting quality), the format is png, you should first extract the alpha map from the png image before training.\n\n- Repository: aisegmentcn/matting_human_datasets",
"## Dataset Structure\n\n\n\nThe input 'data/clip_img/1803151818/clip_00000000/URL' matches the label 'data/matting/1803151818/matting_00000000/URL'",
"### Licensing Information\n\nSee authors Github"
] | [
76,
13,
38,
232,
51,
12
] | [
"passage: TAGS\n#task_categories-image-segmentation #task_ids-semantic-segmentation #annotations_creators-Beijing Wanxing Convergence Technology Co #size_categories-10K<n<100K #license-mit #binary #aisegment.cn #region-us \n# Dataset Card for URL - Matting Human datasets## Table of Contents\n\n- Dataset Card for URL - Matting Human datasets\n - Table of Contents\n - Dataset Description\n - Dataset Structure\n - Licensing Information## Dataset Description\n\nQuoting the dataset's github (translated by Apple Translator):\n\n> This dataset is currently the largest portrait matting dataset, containing 34,427 images and corresponding matting results.\n> The data set was marked by the high quality of Beijing Play Star Convergence Technology Co. Ltd., and the portrait soft segmentation model trained using this data set has been commercialized.\n\n> The original images in the dataset are from 'Flickr', 'Baidu', and 'Taobao'. After face detection and area cropping, a half-length portrait of 600\\*800 was generated.\n> The clip_img directory is a half-length portrait image in the format jpg; the matting directory is the corresponding matting file (convenient to confirm the matting quality), the format is png, you should first extract the alpha map from the png image before training.\n\n- Repository: aisegmentcn/matting_human_datasets## Dataset Structure\n\n\n\nThe input 'data/clip_img/1803151818/clip_00000000/URL' matches the label 'data/matting/1803151818/matting_00000000/URL'### Licensing Information\n\nSee authors Github"
] |
d921ec7e349ce0d28daf30b2da9da5ee698bef0d |
# Dataset Card for MIRACL Corpus
## Dataset Description
* **Homepage:** http://miracl.ai
* **Repository:** https://github.com/project-miracl/miracl
* **Paper:** https://arxiv.org/abs/2210.09984
MIRACL ๐๐๐ (Multilingual Information Retrieval Across a Continuum of Languages) is a multilingual retrieval dataset that focuses on search across 18 different languages, which collectively encompass over three billion native speakers around the world.
This dataset contains the collection data of the 16 "known languages". The remaining 2 "surprise languages" will not be released until later.
The corpus for each language is prepared from a Wikipedia dump, where we keep only the plain text and discard images, tables, etc. Each article is segmented into multiple passages using WikiExtractor based on natural discourse units (e.g., `\n\n` in the wiki markup). Each of these passages comprises a "document" or unit of retrieval. We preserve the Wikipedia article title of each passage.
## Dataset Structure
Each retrieval unit contains three fields: `docid`, `title`, and `text`. Consider an example from the English corpus:
```
{
"docid": "39#0",
"title": "Albedo",
"text": "Albedo (meaning 'whiteness') is the measure of the diffuse reflection of solar radiation out of the total solar radiation received by an astronomical body (e.g. a planet like Earth). It is dimensionless and measured on a scale from 0 (corresponding to a black body that absorbs all incident radiation) to 1 (corresponding to a body that reflects all incident radiation)."
}
```
The `docid` has the schema `X#Y`, where all passages with the same `X` come from the same Wikipedia article, whereas `Y` denotes the passage within that article, numbered sequentially. The text field contains the text of the passage. The title field contains the name of the article the passage comes from.
The collection can be loaded using:
```
lang='ar' # or any of the 16 languages
miracl_corpus = datasets.load_dataset('miracl/miracl-corpus', lang)['train']
for doc in miracl_corpus:
docid = doc['docid']
title = doc['title']
text = doc['text']
```
## Dataset Statistics and Links
The following table contains the number of passage and Wikipedia articles in the collection of each language, along with the links to the datasets and raw Wikipedia dumps.
| Language | # of Passages | # of Articles | Links | Raw Wiki Dump |
|:----------------|--------------:|--------------:|:------|:------|
| Arabic (ar) | 2,061,414 | 656,982 | [๐ค](https://huggingface.co/datasets/miracl/miracl-corpus/tree/main/miracl-corpus-v1.0-ar) | [๐](https://archive.org/download/arwiki-20190201/arwiki-20190201-pages-articles-multistream.xml.bz2)
| Bengali (bn) | 297,265 | 63,762 | [๐ค](https://huggingface.co/datasets/miracl/miracl-corpus/tree/main/miracl-corpus-v1.0-bn) | [๐](https://archive.org/download/bnwiki-20190201/bnwiki-20190201-pages-articles-multistream.xml.bz2)
| English (en) | 32,893,221 | 5,758,285 | [๐ค](https://huggingface.co/datasets/miracl/miracl-corpus/tree/main/miracl-corpus-v1.0-en) | [๐](https://archive.org/download/enwiki-20190201/enwiki-20190201-pages-articles-multistream.xml.bz2)
| Spanish (es) | 10,373,953 | 1,669,181 | [๐ค](https://huggingface.co/datasets/miracl/miracl-corpus/tree/main/miracl-corpus-v1.0-es) | [๐](https://archive.org/download/eswiki-20220301/eswiki-20220301-pages-articles-multistream.xml.bz2)
| Persian (fa) | 2,207,172 | 857,827 | [๐ค](https://huggingface.co/datasets/miracl/miracl-corpus/tree/main/miracl-corpus-v1.0-fa) | [๐](https://archive.org/download/fawiki-20220301/fawiki-20220301-pages-articles-multistream.xml.bz2)
| Finnish (fi) | 1,883,509 | 447,815 | [๐ค](https://huggingface.co/datasets/miracl/miracl-corpus/tree/main/miracl-corpus-v1.0-fi) | [๐](https://archive.org/download/fiwiki-20190201/fiwiki-20190201-pages-articles-multistream.xml.bz2)
| French (fr) | 14,636,953 | 2,325,608 | [๐ค](https://huggingface.co/datasets/miracl/miracl-corpus/tree/main/miracl-corpus-v1.0-fr) | [๐](https://archive.org/download/frwiki-20220301/frwiki-20220301-pages-articles-multistream.xml.bz2)
| Hindi (hi) | 506,264 | 148,107 | [๐ค](https://huggingface.co/datasets/miracl/miracl-corpus/tree/main/miracl-corpus-v1.0-hi) | [๐](https://archive.org/download/hiwiki-20220301/hiwiki-20220301-pages-articles-multistream.xml.bz2)
| Indonesian (id) | 1,446,315 | 446,330 | [๐ค](https://huggingface.co/datasets/miracl/miracl-corpus/tree/main/miracl-corpus-v1.0-id) | [๐](https://archive.org/download/idwiki-20190201/idwiki-20190201-pages-articles-multistream.xml.bz2)
| Japanese (ja) | 6,953,614 | 1,133,444 | [๐ค](https://huggingface.co/datasets/miracl/miracl-corpus/tree/main/miracl-corpus-v1.0-ja) | [๐](https://archive.org/download/jawiki-20190201/jawiki-20190201-pages-articles-multistream.xml.bz2)
| Korean (ko) | 1,486,752 | 437,373 | [๐ค](https://huggingface.co/datasets/miracl/miracl-corpus/tree/main/miracl-corpus-v1.0-ko) | [๐](https://archive.org/download/kowiki-20190201/kowiki-20190201-pages-articles-multistream.xml.bz2)
| Russian (ru) | 9,543,918 | 1,476,045 | [๐ค](https://huggingface.co/datasets/miracl/miracl-corpus/tree/main/miracl-corpus-v1.0-ru) | [๐](https://archive.org/download/ruwiki-20190201/ruwiki-20190201-pages-articles-multistream.xml.bz2)
| Swahili (sw) | 131,924 | 47,793 | [๐ค](https://huggingface.co/datasets/miracl/miracl-corpus/tree/main/miracl-corpus-v1.0-sw) | [๐](https://archive.org/download/swwiki-20190201/swwiki-20190201-pages-articles-multistream.xml.bz2)
| Telugu (te) | 518,079 | 66,353 | [๐ค](https://huggingface.co/datasets/miracl/miracl-corpus/tree/main/miracl-corpus-v1.0-te) | [๐](https://archive.org/download/tewiki-20190201/tewiki-20190201-pages-articles-multistream.xml.bz2)
| Thai (th) | 542,166 | 128,179 | [๐ค](https://huggingface.co/datasets/miracl/miracl-corpus/tree/main/miracl-corpus-v1.0-th) | [๐](https://archive.org/download/thwiki-20190101/thwiki-20190101-pages-articles-multistream.xml.bz2)
| Chinese (zh) | 4,934,368 | 1,246,389 | [๐ค](https://huggingface.co/datasets/miracl/miracl-corpus/tree/main/miracl-corpus-v1.0-zh) | [๐](https://archive.org/download/zhwiki-20220301/zhwiki-20220301-pages-articles-multistream.xml.bz2)
| miracl/miracl-corpus | [
"task_categories:text-retrieval",
"task_ids:document-retrieval",
"annotations_creators:expert-generated",
"multilinguality:multilingual",
"language:ar",
"language:bn",
"language:en",
"language:es",
"language:fa",
"language:fi",
"language:fr",
"language:hi",
"language:id",
"language:ja",
"language:ko",
"language:ru",
"language:sw",
"language:te",
"language:th",
"language:zh",
"license:apache-2.0",
"arxiv:2210.09984",
"region:us"
] | 2022-09-29T13:49:58+00:00 | {"annotations_creators": ["expert-generated"], "language": ["ar", "bn", "en", "es", "fa", "fi", "fr", "hi", "id", "ja", "ko", "ru", "sw", "te", "th", "zh"], "license": ["apache-2.0"], "multilinguality": ["multilingual"], "size_categories": [], "source_datasets": [], "task_categories": ["text-retrieval"], "task_ids": ["document-retrieval"], "pretty_name": "MIRACL-corpus", "tags": []} | 2023-01-05T17:28:26+00:00 | [
"2210.09984"
] | [
"ar",
"bn",
"en",
"es",
"fa",
"fi",
"fr",
"hi",
"id",
"ja",
"ko",
"ru",
"sw",
"te",
"th",
"zh"
] | TAGS
#task_categories-text-retrieval #task_ids-document-retrieval #annotations_creators-expert-generated #multilinguality-multilingual #language-Arabic #language-Bengali #language-English #language-Spanish #language-Persian #language-Finnish #language-French #language-Hindi #language-Indonesian #language-Japanese #language-Korean #language-Russian #language-Swahili (macrolanguage) #language-Telugu #language-Thai #language-Chinese #license-apache-2.0 #arxiv-2210.09984 #region-us
| Dataset Card for MIRACL Corpus
==============================
Dataset Description
-------------------
* Homepage: URL
* Repository: URL
* Paper: URL
MIRACL (Multilingual Information Retrieval Across a Continuum of Languages) is a multilingual retrieval dataset that focuses on search across 18 different languages, which collectively encompass over three billion native speakers around the world.
This dataset contains the collection data of the 16 "known languages". The remaining 2 "surprise languages" will not be released until later.
The corpus for each language is prepared from a Wikipedia dump, where we keep only the plain text and discard images, tables, etc. Each article is segmented into multiple passages using WikiExtractor based on natural discourse units (e.g., '\n\n' in the wiki markup). Each of these passages comprises a "document" or unit of retrieval. We preserve the Wikipedia article title of each passage.
Dataset Structure
-----------------
Each retrieval unit contains three fields: 'docid', 'title', and 'text'. Consider an example from the English corpus:
The 'docid' has the schema 'X#Y', where all passages with the same 'X' come from the same Wikipedia article, whereas 'Y' denotes the passage within that article, numbered sequentially. The text field contains the text of the passage. The title field contains the name of the article the passage comes from.
The collection can be loaded using:
Dataset Statistics and Links
----------------------------
The following table contains the number of passage and Wikipedia articles in the collection of each language, along with the links to the datasets and raw Wikipedia dumps.
| [] | [
"TAGS\n#task_categories-text-retrieval #task_ids-document-retrieval #annotations_creators-expert-generated #multilinguality-multilingual #language-Arabic #language-Bengali #language-English #language-Spanish #language-Persian #language-Finnish #language-French #language-Hindi #language-Indonesian #language-Japanese #language-Korean #language-Russian #language-Swahili (macrolanguage) #language-Telugu #language-Thai #language-Chinese #license-apache-2.0 #arxiv-2210.09984 #region-us \n"
] | [
153
] | [
"passage: TAGS\n#task_categories-text-retrieval #task_ids-document-retrieval #annotations_creators-expert-generated #multilinguality-multilingual #language-Arabic #language-Bengali #language-English #language-Spanish #language-Persian #language-Finnish #language-French #language-Hindi #language-Indonesian #language-Japanese #language-Korean #language-Russian #language-Swahili (macrolanguage) #language-Telugu #language-Thai #language-Chinese #license-apache-2.0 #arxiv-2210.09984 #region-us \n"
] |
aa4f6645451098df234769f89af1fcccd16d567f | ---
license: othera
| Shinadayu/test | [
"region:us"
] | 2022-09-29T14:19:40+00:00 | {} | 2022-09-29T14:21:16+00:00 | [] | [] | TAGS
#region-us
| ---
license: othera
| [] | [
"TAGS\n#region-us \n"
] | [
6
] | [
"passage: TAGS\n#region-us \n"
] |
f0f37162e31f17be4a703fc555be1a965b77adf5 | # Dataset Card for AutoTrain Evaluator
This repository contains model predictions generated by [AutoTrain](https://huggingface.co/autotrain) for the following task and dataset:
* Task: Zero-Shot Text Classification
* Model: facebook/opt-66b
* Dataset: mathemakitten/winobias_antistereotype_test
* Config: mathemakitten--winobias_antistereotype_test
* Split: test
To run new evaluation jobs, visit Hugging Face's [automatic model evaluator](https://huggingface.co/spaces/autoevaluate/model-evaluator).
## Contributions
Thanks to [@mathemakitten](https://huggingface.co/mathemakitten) for evaluating this model. | autoevaluate/autoeval-eval-mathemakitten__winobias_antistereotype_test-mathemakitt-c50da3-1597456336 | [
"autotrain",
"evaluation",
"region:us"
] | 2022-09-29T14:31:27+00:00 | {"type": "predictions", "tags": ["autotrain", "evaluation"], "datasets": ["mathemakitten/winobias_antistereotype_test"], "eval_info": {"task": "text_zero_shot_classification", "model": "facebook/opt-66b", "metrics": [], "dataset_name": "mathemakitten/winobias_antistereotype_test", "dataset_config": "mathemakitten--winobias_antistereotype_test", "dataset_split": "test", "col_mapping": {"text": "text", "classes": "classes", "target": "target"}}} | 2022-09-29T17:00:45+00:00 | [] | [] | TAGS
#autotrain #evaluation #region-us
| # Dataset Card for AutoTrain Evaluator
This repository contains model predictions generated by AutoTrain for the following task and dataset:
* Task: Zero-Shot Text Classification
* Model: facebook/opt-66b
* Dataset: mathemakitten/winobias_antistereotype_test
* Config: mathemakitten--winobias_antistereotype_test
* Split: test
To run new evaluation jobs, visit Hugging Face's automatic model evaluator.
## Contributions
Thanks to @mathemakitten for evaluating this model. | [
"# Dataset Card for AutoTrain Evaluator\n\nThis repository contains model predictions generated by AutoTrain for the following task and dataset:\n\n* Task: Zero-Shot Text Classification\n* Model: facebook/opt-66b\n* Dataset: mathemakitten/winobias_antistereotype_test\n* Config: mathemakitten--winobias_antistereotype_test\n* Split: test\n\nTo run new evaluation jobs, visit Hugging Face's automatic model evaluator.",
"## Contributions\n\nThanks to @mathemakitten for evaluating this model."
] | [
"TAGS\n#autotrain #evaluation #region-us \n",
"# Dataset Card for AutoTrain Evaluator\n\nThis repository contains model predictions generated by AutoTrain for the following task and dataset:\n\n* Task: Zero-Shot Text Classification\n* Model: facebook/opt-66b\n* Dataset: mathemakitten/winobias_antistereotype_test\n* Config: mathemakitten--winobias_antistereotype_test\n* Split: test\n\nTo run new evaluation jobs, visit Hugging Face's automatic model evaluator.",
"## Contributions\n\nThanks to @mathemakitten for evaluating this model."
] | [
13,
114,
17
] | [
"passage: TAGS\n#autotrain #evaluation #region-us \n# Dataset Card for AutoTrain Evaluator\n\nThis repository contains model predictions generated by AutoTrain for the following task and dataset:\n\n* Task: Zero-Shot Text Classification\n* Model: facebook/opt-66b\n* Dataset: mathemakitten/winobias_antistereotype_test\n* Config: mathemakitten--winobias_antistereotype_test\n* Split: test\n\nTo run new evaluation jobs, visit Hugging Face's automatic model evaluator.## Contributions\n\nThanks to @mathemakitten for evaluating this model."
] |
4ab783c3e7e2cc5ca9ea75ab922b856f096e6b9e | # Dataset Card for AutoTrain Evaluator
This repository contains model predictions generated by [AutoTrain](https://huggingface.co/autotrain) for the following task and dataset:
* Task: Zero-Shot Text Classification
* Model: facebook/opt-6.7b
* Dataset: mathemakitten/winobias_antistereotype_test
* Config: mathemakitten--winobias_antistereotype_test
* Split: test
To run new evaluation jobs, visit Hugging Face's [automatic model evaluator](https://huggingface.co/spaces/autoevaluate/model-evaluator).
## Contributions
Thanks to [@mathemakitten](https://huggingface.co/mathemakitten) for evaluating this model. | autoevaluate/autoeval-eval-mathemakitten__winobias_antistereotype_test-mathemakitt-c50da3-1597456333 | [
"autotrain",
"evaluation",
"region:us"
] | 2022-09-29T14:31:27+00:00 | {"type": "predictions", "tags": ["autotrain", "evaluation"], "datasets": ["mathemakitten/winobias_antistereotype_test"], "eval_info": {"task": "text_zero_shot_classification", "model": "facebook/opt-6.7b", "metrics": [], "dataset_name": "mathemakitten/winobias_antistereotype_test", "dataset_config": "mathemakitten--winobias_antistereotype_test", "dataset_split": "test", "col_mapping": {"text": "text", "classes": "classes", "target": "target"}}} | 2022-09-29T14:47:19+00:00 | [] | [] | TAGS
#autotrain #evaluation #region-us
| # Dataset Card for AutoTrain Evaluator
This repository contains model predictions generated by AutoTrain for the following task and dataset:
* Task: Zero-Shot Text Classification
* Model: facebook/opt-6.7b
* Dataset: mathemakitten/winobias_antistereotype_test
* Config: mathemakitten--winobias_antistereotype_test
* Split: test
To run new evaluation jobs, visit Hugging Face's automatic model evaluator.
## Contributions
Thanks to @mathemakitten for evaluating this model. | [
"# Dataset Card for AutoTrain Evaluator\n\nThis repository contains model predictions generated by AutoTrain for the following task and dataset:\n\n* Task: Zero-Shot Text Classification\n* Model: facebook/opt-6.7b\n* Dataset: mathemakitten/winobias_antistereotype_test\n* Config: mathemakitten--winobias_antistereotype_test\n* Split: test\n\nTo run new evaluation jobs, visit Hugging Face's automatic model evaluator.",
"## Contributions\n\nThanks to @mathemakitten for evaluating this model."
] | [
"TAGS\n#autotrain #evaluation #region-us \n",
"# Dataset Card for AutoTrain Evaluator\n\nThis repository contains model predictions generated by AutoTrain for the following task and dataset:\n\n* Task: Zero-Shot Text Classification\n* Model: facebook/opt-6.7b\n* Dataset: mathemakitten/winobias_antistereotype_test\n* Config: mathemakitten--winobias_antistereotype_test\n* Split: test\n\nTo run new evaluation jobs, visit Hugging Face's automatic model evaluator.",
"## Contributions\n\nThanks to @mathemakitten for evaluating this model."
] | [
13,
114,
17
] | [
"passage: TAGS\n#autotrain #evaluation #region-us \n# Dataset Card for AutoTrain Evaluator\n\nThis repository contains model predictions generated by AutoTrain for the following task and dataset:\n\n* Task: Zero-Shot Text Classification\n* Model: facebook/opt-6.7b\n* Dataset: mathemakitten/winobias_antistereotype_test\n* Config: mathemakitten--winobias_antistereotype_test\n* Split: test\n\nTo run new evaluation jobs, visit Hugging Face's automatic model evaluator.## Contributions\n\nThanks to @mathemakitten for evaluating this model."
] |
8881f6b4ef7d33351a0e5b73d482b280bf35992e | # Dataset Card for AutoTrain Evaluator
This repository contains model predictions generated by [AutoTrain](https://huggingface.co/autotrain) for the following task and dataset:
* Task: Zero-Shot Text Classification
* Model: facebook/opt-2.7b
* Dataset: mathemakitten/winobias_antistereotype_test
* Config: mathemakitten--winobias_antistereotype_test
* Split: test
To run new evaluation jobs, visit Hugging Face's [automatic model evaluator](https://huggingface.co/spaces/autoevaluate/model-evaluator).
## Contributions
Thanks to [@mathemakitten](https://huggingface.co/mathemakitten) for evaluating this model. | autoevaluate/autoeval-eval-mathemakitten__winobias_antistereotype_test-mathemakitt-c50da3-1597456332 | [
"autotrain",
"evaluation",
"region:us"
] | 2022-09-29T14:31:27+00:00 | {"type": "predictions", "tags": ["autotrain", "evaluation"], "datasets": ["mathemakitten/winobias_antistereotype_test"], "eval_info": {"task": "text_zero_shot_classification", "model": "facebook/opt-2.7b", "metrics": [], "dataset_name": "mathemakitten/winobias_antistereotype_test", "dataset_config": "mathemakitten--winobias_antistereotype_test", "dataset_split": "test", "col_mapping": {"text": "text", "classes": "classes", "target": "target"}}} | 2022-09-29T14:36:34+00:00 | [] | [] | TAGS
#autotrain #evaluation #region-us
| # Dataset Card for AutoTrain Evaluator
This repository contains model predictions generated by AutoTrain for the following task and dataset:
* Task: Zero-Shot Text Classification
* Model: facebook/opt-2.7b
* Dataset: mathemakitten/winobias_antistereotype_test
* Config: mathemakitten--winobias_antistereotype_test
* Split: test
To run new evaluation jobs, visit Hugging Face's automatic model evaluator.
## Contributions
Thanks to @mathemakitten for evaluating this model. | [
"# Dataset Card for AutoTrain Evaluator\n\nThis repository contains model predictions generated by AutoTrain for the following task and dataset:\n\n* Task: Zero-Shot Text Classification\n* Model: facebook/opt-2.7b\n* Dataset: mathemakitten/winobias_antistereotype_test\n* Config: mathemakitten--winobias_antistereotype_test\n* Split: test\n\nTo run new evaluation jobs, visit Hugging Face's automatic model evaluator.",
"## Contributions\n\nThanks to @mathemakitten for evaluating this model."
] | [
"TAGS\n#autotrain #evaluation #region-us \n",
"# Dataset Card for AutoTrain Evaluator\n\nThis repository contains model predictions generated by AutoTrain for the following task and dataset:\n\n* Task: Zero-Shot Text Classification\n* Model: facebook/opt-2.7b\n* Dataset: mathemakitten/winobias_antistereotype_test\n* Config: mathemakitten--winobias_antistereotype_test\n* Split: test\n\nTo run new evaluation jobs, visit Hugging Face's automatic model evaluator.",
"## Contributions\n\nThanks to @mathemakitten for evaluating this model."
] | [
13,
115,
17
] | [
"passage: TAGS\n#autotrain #evaluation #region-us \n# Dataset Card for AutoTrain Evaluator\n\nThis repository contains model predictions generated by AutoTrain for the following task and dataset:\n\n* Task: Zero-Shot Text Classification\n* Model: facebook/opt-2.7b\n* Dataset: mathemakitten/winobias_antistereotype_test\n* Config: mathemakitten--winobias_antistereotype_test\n* Split: test\n\nTo run new evaluation jobs, visit Hugging Face's automatic model evaluator.## Contributions\n\nThanks to @mathemakitten for evaluating this model."
] |
4f0ea713c9fbb0e90fb46605a9d6fa40045c0cb7 | # Dataset Card for AutoTrain Evaluator
This repository contains model predictions generated by [AutoTrain](https://huggingface.co/autotrain) for the following task and dataset:
* Task: Zero-Shot Text Classification
* Model: facebook/opt-125m
* Dataset: mathemakitten/winobias_antistereotype_test
* Config: mathemakitten--winobias_antistereotype_test
* Split: test
To run new evaluation jobs, visit Hugging Face's [automatic model evaluator](https://huggingface.co/spaces/autoevaluate/model-evaluator).
## Contributions
Thanks to [@mathemakitten](https://huggingface.co/mathemakitten) for evaluating this model. | autoevaluate/autoeval-eval-mathemakitten__winobias_antistereotype_test-mathemakitt-c50da3-1597456329 | [
"autotrain",
"evaluation",
"region:us"
] | 2022-09-29T14:31:27+00:00 | {"type": "predictions", "tags": ["autotrain", "evaluation"], "datasets": ["mathemakitten/winobias_antistereotype_test"], "eval_info": {"task": "text_zero_shot_classification", "model": "facebook/opt-125m", "metrics": [], "dataset_name": "mathemakitten/winobias_antistereotype_test", "dataset_config": "mathemakitten--winobias_antistereotype_test", "dataset_split": "test", "col_mapping": {"text": "text", "classes": "classes", "target": "target"}}} | 2022-09-29T14:32:08+00:00 | [] | [] | TAGS
#autotrain #evaluation #region-us
| # Dataset Card for AutoTrain Evaluator
This repository contains model predictions generated by AutoTrain for the following task and dataset:
* Task: Zero-Shot Text Classification
* Model: facebook/opt-125m
* Dataset: mathemakitten/winobias_antistereotype_test
* Config: mathemakitten--winobias_antistereotype_test
* Split: test
To run new evaluation jobs, visit Hugging Face's automatic model evaluator.
## Contributions
Thanks to @mathemakitten for evaluating this model. | [
"# Dataset Card for AutoTrain Evaluator\n\nThis repository contains model predictions generated by AutoTrain for the following task and dataset:\n\n* Task: Zero-Shot Text Classification\n* Model: facebook/opt-125m\n* Dataset: mathemakitten/winobias_antistereotype_test\n* Config: mathemakitten--winobias_antistereotype_test\n* Split: test\n\nTo run new evaluation jobs, visit Hugging Face's automatic model evaluator.",
"## Contributions\n\nThanks to @mathemakitten for evaluating this model."
] | [
"TAGS\n#autotrain #evaluation #region-us \n",
"# Dataset Card for AutoTrain Evaluator\n\nThis repository contains model predictions generated by AutoTrain for the following task and dataset:\n\n* Task: Zero-Shot Text Classification\n* Model: facebook/opt-125m\n* Dataset: mathemakitten/winobias_antistereotype_test\n* Config: mathemakitten--winobias_antistereotype_test\n* Split: test\n\nTo run new evaluation jobs, visit Hugging Face's automatic model evaluator.",
"## Contributions\n\nThanks to @mathemakitten for evaluating this model."
] | [
13,
114,
17
] | [
"passage: TAGS\n#autotrain #evaluation #region-us \n# Dataset Card for AutoTrain Evaluator\n\nThis repository contains model predictions generated by AutoTrain for the following task and dataset:\n\n* Task: Zero-Shot Text Classification\n* Model: facebook/opt-125m\n* Dataset: mathemakitten/winobias_antistereotype_test\n* Config: mathemakitten--winobias_antistereotype_test\n* Split: test\n\nTo run new evaluation jobs, visit Hugging Face's automatic model evaluator.## Contributions\n\nThanks to @mathemakitten for evaluating this model."
] |
26eec3ffb27c97bfd5b123dae4f046a6c6cb2676 | # Dataset Card for AutoTrain Evaluator
This repository contains model predictions generated by [AutoTrain](https://huggingface.co/autotrain) for the following task and dataset:
* Task: Zero-Shot Text Classification
* Model: facebook/opt-1.3b
* Dataset: mathemakitten/winobias_antistereotype_test
* Config: mathemakitten--winobias_antistereotype_test
* Split: test
To run new evaluation jobs, visit Hugging Face's [automatic model evaluator](https://huggingface.co/spaces/autoevaluate/model-evaluator).
## Contributions
Thanks to [@mathemakitten](https://huggingface.co/mathemakitten) for evaluating this model. | autoevaluate/autoeval-eval-mathemakitten__winobias_antistereotype_test-mathemakitt-c50da3-1597456331 | [
"autotrain",
"evaluation",
"region:us"
] | 2022-09-29T14:31:27+00:00 | {"type": "predictions", "tags": ["autotrain", "evaluation"], "datasets": ["mathemakitten/winobias_antistereotype_test"], "eval_info": {"task": "text_zero_shot_classification", "model": "facebook/opt-1.3b", "metrics": [], "dataset_name": "mathemakitten/winobias_antistereotype_test", "dataset_config": "mathemakitten--winobias_antistereotype_test", "dataset_split": "test", "col_mapping": {"text": "text", "classes": "classes", "target": "target"}}} | 2022-09-29T14:34:41+00:00 | [] | [] | TAGS
#autotrain #evaluation #region-us
| # Dataset Card for AutoTrain Evaluator
This repository contains model predictions generated by AutoTrain for the following task and dataset:
* Task: Zero-Shot Text Classification
* Model: facebook/opt-1.3b
* Dataset: mathemakitten/winobias_antistereotype_test
* Config: mathemakitten--winobias_antistereotype_test
* Split: test
To run new evaluation jobs, visit Hugging Face's automatic model evaluator.
## Contributions
Thanks to @mathemakitten for evaluating this model. | [
"# Dataset Card for AutoTrain Evaluator\n\nThis repository contains model predictions generated by AutoTrain for the following task and dataset:\n\n* Task: Zero-Shot Text Classification\n* Model: facebook/opt-1.3b\n* Dataset: mathemakitten/winobias_antistereotype_test\n* Config: mathemakitten--winobias_antistereotype_test\n* Split: test\n\nTo run new evaluation jobs, visit Hugging Face's automatic model evaluator.",
"## Contributions\n\nThanks to @mathemakitten for evaluating this model."
] | [
"TAGS\n#autotrain #evaluation #region-us \n",
"# Dataset Card for AutoTrain Evaluator\n\nThis repository contains model predictions generated by AutoTrain for the following task and dataset:\n\n* Task: Zero-Shot Text Classification\n* Model: facebook/opt-1.3b\n* Dataset: mathemakitten/winobias_antistereotype_test\n* Config: mathemakitten--winobias_antistereotype_test\n* Split: test\n\nTo run new evaluation jobs, visit Hugging Face's automatic model evaluator.",
"## Contributions\n\nThanks to @mathemakitten for evaluating this model."
] | [
13,
114,
17
] | [
"passage: TAGS\n#autotrain #evaluation #region-us \n# Dataset Card for AutoTrain Evaluator\n\nThis repository contains model predictions generated by AutoTrain for the following task and dataset:\n\n* Task: Zero-Shot Text Classification\n* Model: facebook/opt-1.3b\n* Dataset: mathemakitten/winobias_antistereotype_test\n* Config: mathemakitten--winobias_antistereotype_test\n* Split: test\n\nTo run new evaluation jobs, visit Hugging Face's automatic model evaluator.## Contributions\n\nThanks to @mathemakitten for evaluating this model."
] |
4cf687b19fb10893ab4f13a9e2bec3323150897b | # Dataset Card for AutoTrain Evaluator
This repository contains model predictions generated by [AutoTrain](https://huggingface.co/autotrain) for the following task and dataset:
* Task: Zero-Shot Text Classification
* Model: facebook/opt-350m
* Dataset: mathemakitten/winobias_antistereotype_test
* Config: mathemakitten--winobias_antistereotype_test
* Split: test
To run new evaluation jobs, visit Hugging Face's [automatic model evaluator](https://huggingface.co/spaces/autoevaluate/model-evaluator).
## Contributions
Thanks to [@mathemakitten](https://huggingface.co/mathemakitten) for evaluating this model. | autoevaluate/autoeval-eval-mathemakitten__winobias_antistereotype_test-mathemakitt-c50da3-1597456330 | [
"autotrain",
"evaluation",
"region:us"
] | 2022-09-29T14:31:29+00:00 | {"type": "predictions", "tags": ["autotrain", "evaluation"], "datasets": ["mathemakitten/winobias_antistereotype_test"], "eval_info": {"task": "text_zero_shot_classification", "model": "facebook/opt-350m", "metrics": [], "dataset_name": "mathemakitten/winobias_antistereotype_test", "dataset_config": "mathemakitten--winobias_antistereotype_test", "dataset_split": "test", "col_mapping": {"text": "text", "classes": "classes", "target": "target"}}} | 2022-09-29T14:32:36+00:00 | [] | [] | TAGS
#autotrain #evaluation #region-us
| # Dataset Card for AutoTrain Evaluator
This repository contains model predictions generated by AutoTrain for the following task and dataset:
* Task: Zero-Shot Text Classification
* Model: facebook/opt-350m
* Dataset: mathemakitten/winobias_antistereotype_test
* Config: mathemakitten--winobias_antistereotype_test
* Split: test
To run new evaluation jobs, visit Hugging Face's automatic model evaluator.
## Contributions
Thanks to @mathemakitten for evaluating this model. | [
"# Dataset Card for AutoTrain Evaluator\n\nThis repository contains model predictions generated by AutoTrain for the following task and dataset:\n\n* Task: Zero-Shot Text Classification\n* Model: facebook/opt-350m\n* Dataset: mathemakitten/winobias_antistereotype_test\n* Config: mathemakitten--winobias_antistereotype_test\n* Split: test\n\nTo run new evaluation jobs, visit Hugging Face's automatic model evaluator.",
"## Contributions\n\nThanks to @mathemakitten for evaluating this model."
] | [
"TAGS\n#autotrain #evaluation #region-us \n",
"# Dataset Card for AutoTrain Evaluator\n\nThis repository contains model predictions generated by AutoTrain for the following task and dataset:\n\n* Task: Zero-Shot Text Classification\n* Model: facebook/opt-350m\n* Dataset: mathemakitten/winobias_antistereotype_test\n* Config: mathemakitten--winobias_antistereotype_test\n* Split: test\n\nTo run new evaluation jobs, visit Hugging Face's automatic model evaluator.",
"## Contributions\n\nThanks to @mathemakitten for evaluating this model."
] | [
13,
114,
17
] | [
"passage: TAGS\n#autotrain #evaluation #region-us \n# Dataset Card for AutoTrain Evaluator\n\nThis repository contains model predictions generated by AutoTrain for the following task and dataset:\n\n* Task: Zero-Shot Text Classification\n* Model: facebook/opt-350m\n* Dataset: mathemakitten/winobias_antistereotype_test\n* Config: mathemakitten--winobias_antistereotype_test\n* Split: test\n\nTo run new evaluation jobs, visit Hugging Face's automatic model evaluator.## Contributions\n\nThanks to @mathemakitten for evaluating this model."
] |
40811b7d45e7be647accbaad064231273e3d5ff0 | # Dataset Card for AutoTrain Evaluator
This repository contains model predictions generated by [AutoTrain](https://huggingface.co/autotrain) for the following task and dataset:
* Task: Zero-Shot Text Classification
* Model: facebook/opt-30b
* Dataset: mathemakitten/winobias_antistereotype_test
* Config: mathemakitten--winobias_antistereotype_test
* Split: test
To run new evaluation jobs, visit Hugging Face's [automatic model evaluator](https://huggingface.co/spaces/autoevaluate/model-evaluator).
## Contributions
Thanks to [@mathemakitten](https://huggingface.co/mathemakitten) for evaluating this model. | autoevaluate/autoeval-eval-mathemakitten__winobias_antistereotype_test-mathemakitt-c50da3-1597456335 | [
"autotrain",
"evaluation",
"region:us"
] | 2022-09-29T14:31:29+00:00 | {"type": "predictions", "tags": ["autotrain", "evaluation"], "datasets": ["mathemakitten/winobias_antistereotype_test"], "eval_info": {"task": "text_zero_shot_classification", "model": "facebook/opt-30b", "metrics": [], "dataset_name": "mathemakitten/winobias_antistereotype_test", "dataset_config": "mathemakitten--winobias_antistereotype_test", "dataset_split": "test", "col_mapping": {"text": "text", "classes": "classes", "target": "target"}}} | 2022-09-29T15:38:50+00:00 | [] | [] | TAGS
#autotrain #evaluation #region-us
| # Dataset Card for AutoTrain Evaluator
This repository contains model predictions generated by AutoTrain for the following task and dataset:
* Task: Zero-Shot Text Classification
* Model: facebook/opt-30b
* Dataset: mathemakitten/winobias_antistereotype_test
* Config: mathemakitten--winobias_antistereotype_test
* Split: test
To run new evaluation jobs, visit Hugging Face's automatic model evaluator.
## Contributions
Thanks to @mathemakitten for evaluating this model. | [
"# Dataset Card for AutoTrain Evaluator\n\nThis repository contains model predictions generated by AutoTrain for the following task and dataset:\n\n* Task: Zero-Shot Text Classification\n* Model: facebook/opt-30b\n* Dataset: mathemakitten/winobias_antistereotype_test\n* Config: mathemakitten--winobias_antistereotype_test\n* Split: test\n\nTo run new evaluation jobs, visit Hugging Face's automatic model evaluator.",
"## Contributions\n\nThanks to @mathemakitten for evaluating this model."
] | [
"TAGS\n#autotrain #evaluation #region-us \n",
"# Dataset Card for AutoTrain Evaluator\n\nThis repository contains model predictions generated by AutoTrain for the following task and dataset:\n\n* Task: Zero-Shot Text Classification\n* Model: facebook/opt-30b\n* Dataset: mathemakitten/winobias_antistereotype_test\n* Config: mathemakitten--winobias_antistereotype_test\n* Split: test\n\nTo run new evaluation jobs, visit Hugging Face's automatic model evaluator.",
"## Contributions\n\nThanks to @mathemakitten for evaluating this model."
] | [
13,
113,
17
] | [
"passage: TAGS\n#autotrain #evaluation #region-us \n# Dataset Card for AutoTrain Evaluator\n\nThis repository contains model predictions generated by AutoTrain for the following task and dataset:\n\n* Task: Zero-Shot Text Classification\n* Model: facebook/opt-30b\n* Dataset: mathemakitten/winobias_antistereotype_test\n* Config: mathemakitten--winobias_antistereotype_test\n* Split: test\n\nTo run new evaluation jobs, visit Hugging Face's automatic model evaluator.## Contributions\n\nThanks to @mathemakitten for evaluating this model."
] |
1201e7301176c674f1f05bd1d01787c919b1ea76 | # Dataset Card for AutoTrain Evaluator
This repository contains model predictions generated by [AutoTrain](https://huggingface.co/autotrain) for the following task and dataset:
* Task: Zero-Shot Text Classification
* Model: facebook/opt-13b
* Dataset: mathemakitten/winobias_antistereotype_test
* Config: mathemakitten--winobias_antistereotype_test
* Split: test
To run new evaluation jobs, visit Hugging Face's [automatic model evaluator](https://huggingface.co/spaces/autoevaluate/model-evaluator).
## Contributions
Thanks to [@mathemakitten](https://huggingface.co/mathemakitten) for evaluating this model. | autoevaluate/autoeval-eval-mathemakitten__winobias_antistereotype_test-mathemakitt-c50da3-1597456334 | [
"autotrain",
"evaluation",
"region:us"
] | 2022-09-29T14:31:36+00:00 | {"type": "predictions", "tags": ["autotrain", "evaluation"], "datasets": ["mathemakitten/winobias_antistereotype_test"], "eval_info": {"task": "text_zero_shot_classification", "model": "facebook/opt-13b", "metrics": [], "dataset_name": "mathemakitten/winobias_antistereotype_test", "dataset_config": "mathemakitten--winobias_antistereotype_test", "dataset_split": "test", "col_mapping": {"text": "text", "classes": "classes", "target": "target"}}} | 2022-09-29T14:59:04+00:00 | [] | [] | TAGS
#autotrain #evaluation #region-us
| # Dataset Card for AutoTrain Evaluator
This repository contains model predictions generated by AutoTrain for the following task and dataset:
* Task: Zero-Shot Text Classification
* Model: facebook/opt-13b
* Dataset: mathemakitten/winobias_antistereotype_test
* Config: mathemakitten--winobias_antistereotype_test
* Split: test
To run new evaluation jobs, visit Hugging Face's automatic model evaluator.
## Contributions
Thanks to @mathemakitten for evaluating this model. | [
"# Dataset Card for AutoTrain Evaluator\n\nThis repository contains model predictions generated by AutoTrain for the following task and dataset:\n\n* Task: Zero-Shot Text Classification\n* Model: facebook/opt-13b\n* Dataset: mathemakitten/winobias_antistereotype_test\n* Config: mathemakitten--winobias_antistereotype_test\n* Split: test\n\nTo run new evaluation jobs, visit Hugging Face's automatic model evaluator.",
"## Contributions\n\nThanks to @mathemakitten for evaluating this model."
] | [
"TAGS\n#autotrain #evaluation #region-us \n",
"# Dataset Card for AutoTrain Evaluator\n\nThis repository contains model predictions generated by AutoTrain for the following task and dataset:\n\n* Task: Zero-Shot Text Classification\n* Model: facebook/opt-13b\n* Dataset: mathemakitten/winobias_antistereotype_test\n* Config: mathemakitten--winobias_antistereotype_test\n* Split: test\n\nTo run new evaluation jobs, visit Hugging Face's automatic model evaluator.",
"## Contributions\n\nThanks to @mathemakitten for evaluating this model."
] | [
13,
113,
17
] | [
"passage: TAGS\n#autotrain #evaluation #region-us \n# Dataset Card for AutoTrain Evaluator\n\nThis repository contains model predictions generated by AutoTrain for the following task and dataset:\n\n* Task: Zero-Shot Text Classification\n* Model: facebook/opt-13b\n* Dataset: mathemakitten/winobias_antistereotype_test\n* Config: mathemakitten--winobias_antistereotype_test\n* Split: test\n\nTo run new evaluation jobs, visit Hugging Face's automatic model evaluator.## Contributions\n\nThanks to @mathemakitten for evaluating this model."
] |
9704434c1038783fb4eb69ffc76b029e2ea43643 | annotations_creators:
- no-annotation
language:
- en
language_creators:
- other
license:
- artistic-2.0
multilinguality:
- monolingual
pretty_name: m3 dataset (a dataset with my face in it)
size_categories:
- n<1K
source_datasets:
- original
tags: []
task_categories:
- text-to-image
task_ids: [] | Gr3en/m3 | [
"region:us"
] | 2022-09-29T15:07:05+00:00 | {} | 2022-09-29T16:13:42+00:00 | [] | [] | TAGS
#region-us
| annotations_creators:
- no-annotation
language:
- en
language_creators:
- other
license:
- artistic-2.0
multilinguality:
- monolingual
pretty_name: m3 dataset (a dataset with my face in it)
size_categories:
- n<1K
source_datasets:
- original
tags: []
task_categories:
- text-to-image
task_ids: [] | [] | [
"TAGS\n#region-us \n"
] | [
6
] | [
"passage: TAGS\n#region-us \n"
] |
6e20e114326dd6e209339bc47f392d5906aeb931 | yes | Ivanrex/images | [
"region:us"
] | 2022-09-29T16:08:56+00:00 | {} | 2022-09-29T16:12:35+00:00 | [] | [] | TAGS
#region-us
| yes | [] | [
"TAGS\n#region-us \n"
] | [
6
] | [
"passage: TAGS\n#region-us \n"
] |
d81cbab7d0392708d5371d3a4960e69261824db4 |
# Dataset Card for New Yorker Caption Contest Benchmarks
## Table of Contents
- [Table of Contents](#table-of-contents)
- [Dataset Description](#dataset-description)
- [Dataset Summary](#dataset-summary)
- [Supported Tasks and Leaderboards](#supported-tasks-and-leaderboards)
- [Languages](#languages)
- [Dataset Structure](#dataset-structure)
- [Data Instances](#data-instances)
- [Data Fields](#data-fields)
- [Data Splits](#data-splits)
- [Dataset Creation](#dataset-creation)
- [Curation Rationale](#curation-rationale)
- [Source Data](#source-data)
- [Annotations](#annotations)
- [Personal and Sensitive Information](#personal-and-sensitive-information)
- [Considerations for Using the Data](#considerations-for-using-the-data)
- [Social Impact of Dataset](#social-impact-of-dataset)
- [Discussion of Biases](#discussion-of-biases)
- [Other Known Limitations](#other-known-limitations)
- [Additional Information](#additional-information)
- [Dataset Curators](#dataset-curators)
- [Licensing Information](#licensing-information)
- [Citation Information](#citation-information)
- [Contributions](#contributions)
## Dataset Description
- **Homepage:** [capcon.dev](https://www.capcon.dev)
- **Repository:** [https://github.com/jmhessel/caption_contest_corpus](https://github.com/jmhessel/caption_contest_corpus)
- **Paper:** [Do Androids Laugh at Electric Sheep? Humor "Understanding" Benchmarks from The New Yorker Caption Contest](https://arxiv.org/abs/2209.06293)
- **Leaderboard:** https://leaderboard.allenai.org/nycc-matching/
- **Point of Contact:** [email protected]
### Dataset Summary
See [capcon.dev](https://www.capcon.dev) for more!
Data from:
[Do Androids Laugh at Electric Sheep? Humor "Understanding" Benchmarks from The New Yorker Caption Contest](https://arxiv.org/abs/2209.06293)
```
@inproceedings{hessel2023androids,
title={Do Androids Laugh at Electric Sheep? {Humor} ``Understanding''
Benchmarks from {The New Yorker Caption Contest}},
author={Hessel, Jack and Marasovi{\'c}, Ana and Hwang, Jena D. and Lee, Lillian
and Da, Jeff and Zellers, Rowan and Mankoff, Robert and Choi, Yejin},
booktitle={Proceedings of the ACL},
year={2023}
}
```
If you use this dataset, we would appreciate you citing our work, but also -- several other papers that we build this corpus upon. See [Citation Information](#citation-information).
We challenge AI models to "demonstrate understanding" of the
sophisticated multimodal humor of The New Yorker Caption Contest.
Concretely, we develop three carefully circumscribed tasks for which
it suffices (but is not necessary) to grasp potentially complex and
unexpected relationships between image and caption, and similarly
complex and unexpected allusions to the wide varieties of human
experience.
### Supported Tasks and Leaderboards
Three tasks are supported:
- "Matching:" a model must recognize a caption written about a cartoon (vs. options that were not);
- "Quality ranking:" a model must evaluate the quality of a caption by scoring it more highly than a lower quality option from the same contest;
- "Explanation:" a model must explain why a given joke is funny.
There are no official leaderboards (yet).
### Languages
English
## Dataset Structure
Here's an example instance from Matching:
```
{'caption_choices': ['Tell me about your childhood very quickly.',
"Believe me . . . it's what's UNDER the ground that's "
'most interesting.',
"Stop me if you've heard this one.",
'I have trouble saying no.',
'Yes, I see the train but I think we can beat it.'],
'contest_number': 49,
'entities': ['https://en.wikipedia.org/wiki/Rule_of_three_(writing)',
'https://en.wikipedia.org/wiki/Bar_joke',
'https://en.wikipedia.org/wiki/Religious_institute'],
'from_description': 'scene: a bar description: Two priests and a rabbi are '
'walking into a bar, as the bartender and another patron '
'look on. The bartender talks on the phone while looking '
'skeptically at the incoming crew. uncanny: The scene '
'depicts a very stereotypical "bar joke" that would be '
'unlikely to be encountered in real life; the skepticism '
'of the bartender suggests that he is aware he is seeing '
'this trope, and is explaining it to someone on the '
'phone. entities: Rule_of_three_(writing), Bar_joke, '
'Religious_institute. choices A: Tell me about your '
"childhood very quickly. B: Believe me . . . it's what's "
"UNDER the ground that's most interesting. C: Stop me if "
"you've heard this one. D: I have trouble saying no. E: "
'Yes, I see the train but I think we can beat it.',
'image': <PIL.JpegImagePlugin.JpegImageFile image mode=L size=323x231 at 0x7F34F283E9D0>,
'image_description': 'Two priests and a rabbi are walking into a bar, as the '
'bartender and another patron look on. The bartender '
'talks on the phone while looking skeptically at the '
'incoming crew.',
'image_location': 'a bar',
'image_uncanny_description': 'The scene depicts a very stereotypical "bar '
'joke" that would be unlikely to be encountered '
'in real life; the skepticism of the bartender '
'suggests that he is aware he is seeing this '
'trope, and is explaining it to someone on the '
'phone.',
'instance_id': '21125bb8787b4e7e82aa3b0a1cba1571',
'label': 'C',
'n_tokens_label': 1,
'questions': ['What is the bartender saying on the phone in response to the '
'living, breathing, stereotypical bar joke that is unfolding?']}
```
The label "C" indicates that the 3rd choice in the `caption_choices` is correct.
Here's an example instance from Ranking (in the from pixels setting --- though, this is also available in the from description setting)
```
{'caption_choices': ['I guess I misunderstood when you said long bike ride.',
'Does your divorce lawyer have any other cool ideas?'],
'contest_number': 582,
'image': <PIL.JpegImagePlugin.JpegImageFile image mode=L size=600x414 at 0x7F8FF9F96610>,
'instance_id': 'dd1c214a1ca3404aa4e582c9ce50795a',
'label': 'A',
'n_tokens_label': 1,
'winner_source': 'official_winner'}
```
the label indicates that the first caption choice ("A", here) in the `caption_choices` list was more highly rated.
Here's an example instance from Explanation:
```
{'caption_choices': 'The classics can be so intimidating.',
'contest_number': 752,
'entities': ['https://en.wikipedia.org/wiki/Literature',
'https://en.wikipedia.org/wiki/Solicitor'],
'from_description': 'scene: a road description: Two people are walking down a '
'path. A number of giant books have surrounded them. '
'uncanny: There are book people in this world. entities: '
'Literature, Solicitor. caption: The classics can be so '
'intimidating.',
'image': <PIL.JpegImagePlugin.JpegImageFile image mode=L size=800x706 at 0x7F90003D0BB0>,
'image_description': 'Two people are walking down a path. A number of giant '
'books have surrounded them.',
'image_location': 'a road',
'image_uncanny_description': 'There are book people in this world.',
'instance_id': 'eef9baf450e2fab19b96facc128adf80',
'label': 'A play on the word intimidating --- usually if the classics (i.e., '
'classic novels) were to be intimidating, this would mean that they '
'are intimidating to read due to their length, complexity, etc. But '
'here, they are surrounded by anthropomorphic books which look '
'physically intimidating, i.e., they are intimidating because they '
'may try to beat up these people.',
'n_tokens_label': 59,
'questions': ['What do the books want?']}
```
The label is an explanation of the joke, which serves as the autoregressive target.
### Data Instances
See above
### Data Fields
See above
### Data Splits
Data splits can be accessed as:
```
from datasets import load_dataset
dset = load_dataset("jmhessel/newyorker_caption_contest", "matching")
dset = load_dataset("jmhessel/newyorker_caption_contest", "ranking")
dset = load_dataset("jmhessel/newyorker_caption_contest", "explanation")
```
Or, in the from pixels setting, e.g.,
```
from datasets import load_dataset
dset = load_dataset("jmhessel/newyorker_caption_contest", "ranking_from_pixels")
```
Because the dataset is small, we reported in 5-fold cross-validation setting initially. The default splits are split 0. You can access the other splits, e.g.:
```
from datasets import load_dataset
# the 4th data split
dset = load_dataset("jmhessel/newyorker_caption_contest", "explanation_4")
```
## Dataset Creation
Full details are in the paper.
### Curation Rationale
See the paper for rationale/motivation.
### Source Data
See citation below. We combined 3 sources of data, and added significant annotations of our own.
#### Initial Data Collection and Normalization
Full details are in the paper.
#### Who are the source language producers?
We paid crowdworkers $15/hr to annotate the corpus.
In addition, significant annotation efforts were conducted by the authors of this work.
### Annotations
Full details are in the paper.
#### Annotation process
Full details are in the paper.
#### Who are the annotators?
A mix of crowdworks and authors of this paper.
### Personal and Sensitive Information
Has been redacted from the dataset. Images are published in the New Yorker already.
## Considerations for Using the Data
### Social Impact of Dataset
It's plausible that humor could perpetuate negative stereotypes. The jokes in this corpus are a mix of crowdsourced entries that are highly rated, and ones published in the new yorker.
### Discussion of Biases
Humor is subjective, and some of the jokes may be considered offensive. The images may contain adult themes and minor cartoon nudity.
### Other Known Limitations
More details are in the paper
## Additional Information
### Dataset Curators
The dataset was curated by researchers at AI2
### Licensing Information
The annotations we provide are CC-BY-4.0. See www.capcon.dev for more info.
### Citation Information
```
@article{hessel2022androids,
title={Do Androids Laugh at Electric Sheep? Humor "Understanding" Benchmarks from The New Yorker Caption Contest},
author={Hessel, Jack and Marasovi{\'c}, Ana and Hwang, Jena D and Lee, Lillian and Da, Jeff and Zellers, Rowan and Mankoff, Robert and Choi, Yejin},
journal={arXiv preprint arXiv:2209.06293},
year={2022}
}
```
Our data contributions are:
- The cartoon-level annotations;
- The joke explanations;
- and the framing of the tasks
We release these data we contribute under CC-BY (see DATASET_LICENSE). If you find this data useful in your work, in addition to citing our contributions, please also cite the following, from which the cartoons/captions in our corpus are derived:
```
@misc{newyorkernextmldataset,
author={Jain, Lalit and Jamieson, Kevin and Mankoff, Robert and Nowak, Robert and Sievert, Scott},
title={The {N}ew {Y}orker Cartoon Caption Contest Dataset},
year={2020},
url={https://nextml.github.io/caption-contest-data/}
}
@inproceedings{radev-etal-2016-humor,
title = "Humor in Collective Discourse: Unsupervised Funniness Detection in The {New Yorker} Cartoon Caption Contest",
author = "Radev, Dragomir and
Stent, Amanda and
Tetreault, Joel and
Pappu, Aasish and
Iliakopoulou, Aikaterini and
Chanfreau, Agustin and
de Juan, Paloma and
Vallmitjana, Jordi and
Jaimes, Alejandro and
Jha, Rahul and
Mankoff, Robert",
booktitle = "LREC",
year = "2016",
}
@inproceedings{shahaf2015inside,
title={Inside jokes: Identifying humorous cartoon captions},
author={Shahaf, Dafna and Horvitz, Eric and Mankoff, Robert},
booktitle={KDD},
year={2015},
}
``` | jmhessel/newyorker_caption_contest | [
"task_categories:image-to-text",
"task_categories:multiple-choice",
"task_categories:text-classification",
"task_categories:text-generation",
"task_categories:visual-question-answering",
"task_categories:other",
"task_categories:text2text-generation",
"task_ids:multi-class-classification",
"task_ids:language-modeling",
"task_ids:visual-question-answering",
"task_ids:explanation-generation",
"annotations_creators:expert-generated",
"annotations_creators:crowdsourced",
"annotations_creators:found",
"language_creators:crowdsourced",
"language_creators:expert-generated",
"multilinguality:monolingual",
"size_categories:1K<n<10K",
"source_datasets:original",
"language:en",
"license:cc-by-4.0",
"humor",
"caption contest",
"new yorker",
"arxiv:2209.06293",
"region:us"
] | 2022-09-29T16:28:05+00:00 | {"annotations_creators": ["expert-generated", "crowdsourced", "found"], "language_creators": ["crowdsourced", "expert-generated"], "language": ["en"], "license": ["cc-by-4.0"], "multilinguality": ["monolingual"], "size_categories": ["1K<n<10K"], "source_datasets": ["original"], "task_categories": ["image-to-text", "multiple-choice", "text-classification", "text-generation", "visual-question-answering", "other", "text2text-generation"], "task_ids": ["multi-class-classification", "language-modeling", "visual-question-answering", "explanation-generation"], "pretty_name": "newyorker_caption_contest", "tags": ["humor", "caption contest", "new yorker"], "dataset_info": [{"config_name": "explanation", "features": [{"name": "image", "dtype": "image"}, {"name": "contest_number", "dtype": "int32"}, {"name": "image_location", "dtype": "string"}, {"name": "image_description", "dtype": "string"}, {"name": "image_uncanny_description", "dtype": "string"}, {"name": "entities", "sequence": "string"}, {"name": "questions", "sequence": "string"}, {"name": "caption_choices", "dtype": "string"}, {"name": "from_description", "dtype": "string"}, {"name": "label", "dtype": "string"}, {"name": "n_tokens_label", "dtype": "int32"}, {"name": "instance_id", "dtype": "string"}], "splits": [{"name": "train", "num_bytes": 133827514.64, "num_examples": 2340}, {"name": "validation", "num_bytes": 8039885.0, "num_examples": 130}, {"name": "test", "num_bytes": 6863533.0, "num_examples": 131}], "download_size": 139737042, "dataset_size": 148730932.64}, {"config_name": "explanation_1", "features": [{"name": "image", "dtype": "image"}, {"name": "contest_number", "dtype": "int32"}, {"name": "image_location", "dtype": "string"}, {"name": "image_description", "dtype": "string"}, {"name": "image_uncanny_description", "dtype": "string"}, {"name": "entities", "sequence": "string"}, {"name": "questions", "sequence": "string"}, {"name": "caption_choices", "dtype": "string"}, {"name": "from_description", "dtype": "string"}, {"name": "label", "dtype": "string"}, {"name": "n_tokens_label", "dtype": "int32"}, {"name": "instance_id", "dtype": "string"}], "splits": [{"name": "train", "num_bytes": 136614332.45999998, "num_examples": 2358}, {"name": "validation", "num_bytes": 7911995.0, "num_examples": 128}, {"name": "test", "num_bytes": 8039885.0, "num_examples": 130}], "download_size": 134637839, "dataset_size": 152566212.45999998}, {"config_name": "explanation_2", "features": [{"name": "image", "dtype": "image"}, {"name": "contest_number", "dtype": "int32"}, {"name": "image_location", "dtype": "string"}, {"name": "image_description", "dtype": "string"}, {"name": "image_uncanny_description", "dtype": "string"}, {"name": "entities", "sequence": "string"}, {"name": "questions", "sequence": "string"}, {"name": "caption_choices", "dtype": "string"}, {"name": "from_description", "dtype": "string"}, {"name": "label", "dtype": "string"}, {"name": "n_tokens_label", "dtype": "int32"}, {"name": "instance_id", "dtype": "string"}], "splits": [{"name": "train", "num_bytes": 138337491.342, "num_examples": 2346}, {"name": "validation", "num_bytes": 7460490.0, "num_examples": 132}, {"name": "test", "num_bytes": 7911995.0, "num_examples": 128}], "download_size": 138271185, "dataset_size": 153709976.342}, {"config_name": "explanation_3", "features": [{"name": "image", "dtype": "image"}, {"name": "contest_number", "dtype": "int32"}, {"name": "image_location", "dtype": "string"}, {"name": "image_description", "dtype": "string"}, {"name": "image_uncanny_description", "dtype": "string"}, {"name": "entities", "sequence": "string"}, {"name": "questions", "sequence": "string"}, {"name": "caption_choices", "dtype": "string"}, {"name": "from_description", "dtype": "string"}, {"name": "label", "dtype": "string"}, {"name": "n_tokens_label", "dtype": "int32"}, {"name": "instance_id", "dtype": "string"}], "splits": [{"name": "train", "num_bytes": 138247435.342, "num_examples": 2334}, {"name": "validation", "num_bytes": 7911920.0, "num_examples": 130}, {"name": "test", "num_bytes": 7460490.0, "num_examples": 132}], "download_size": 136862726, "dataset_size": 153619845.342}, {"config_name": "explanation_4", "features": [{"name": "image", "dtype": "image"}, {"name": "contest_number", "dtype": "int32"}, {"name": "image_location", "dtype": "string"}, {"name": "image_description", "dtype": "string"}, {"name": "image_uncanny_description", "dtype": "string"}, {"name": "entities", "sequence": "string"}, {"name": "questions", "sequence": "string"}, {"name": "caption_choices", "dtype": "string"}, {"name": "from_description", "dtype": "string"}, {"name": "label", "dtype": "string"}, {"name": "n_tokens_label", "dtype": "int32"}, {"name": "instance_id", "dtype": "string"}], "splits": [{"name": "train", "num_bytes": 141175335.3, "num_examples": 2340}, {"name": "validation", "num_bytes": 6863533.0, "num_examples": 131}, {"name": "test", "num_bytes": 7911920.0, "num_examples": 130}], "download_size": 140501251, "dataset_size": 155950788.3}, {"config_name": "explanation_from_pixels", "features": [{"name": "image", "dtype": "image"}, {"name": "contest_number", "dtype": "int32"}, {"name": "caption_choices", "dtype": "string"}, {"name": "label", "dtype": "string"}, {"name": "n_tokens_label", "dtype": "int32"}, {"name": "instance_id", "dtype": "string"}], "splits": [{"name": "train", "num_bytes": 23039316.0, "num_examples": 390}, {"name": "validation", "num_bytes": 7956182.0, "num_examples": 130}, {"name": "test", "num_bytes": 6778892.0, "num_examples": 131}], "download_size": 37552582, "dataset_size": 37774390.0}, {"config_name": "explanation_from_pixels_1", "features": [{"name": "image", "dtype": "image"}, {"name": "contest_number", "dtype": "int32"}, {"name": "caption_choices", "dtype": "string"}, {"name": "label", "dtype": "string"}, {"name": "n_tokens_label", "dtype": "int32"}, {"name": "instance_id", "dtype": "string"}], "splits": [{"name": "train", "num_bytes": 21986652.0, "num_examples": 393}, {"name": "validation", "num_bytes": 7831556.0, "num_examples": 128}, {"name": "test", "num_bytes": 7956182.0, "num_examples": 130}], "download_size": 37534409, "dataset_size": 37774390.0}, {"config_name": "explanation_from_pixels_2", "features": [{"name": "image", "dtype": "image"}, {"name": "contest_number", "dtype": "int32"}, {"name": "caption_choices", "dtype": "string"}, {"name": "label", "dtype": "string"}, {"name": "n_tokens_label", "dtype": "int32"}, {"name": "instance_id", "dtype": "string"}], "splits": [{"name": "train", "num_bytes": 22566608.0, "num_examples": 391}, {"name": "validation", "num_bytes": 7376225.0, "num_examples": 132}, {"name": "test", "num_bytes": 7831556.0, "num_examples": 128}], "download_size": 37544724, "dataset_size": 37774389.0}, {"config_name": "explanation_from_pixels_3", "features": [{"name": "image", "dtype": "image"}, {"name": "contest_number", "dtype": "int32"}, {"name": "caption_choices", "dtype": "string"}, {"name": "label", "dtype": "string"}, {"name": "n_tokens_label", "dtype": "int32"}, {"name": "instance_id", "dtype": "string"}], "splits": [{"name": "train", "num_bytes": 22566629.0, "num_examples": 389}, {"name": "validation", "num_bytes": 7831536.0, "num_examples": 130}, {"name": "test", "num_bytes": 7376225.0, "num_examples": 132}], "download_size": 37573931, "dataset_size": 37774390.0}, {"config_name": "explanation_from_pixels_4", "features": [{"name": "image", "dtype": "image"}, {"name": "contest_number", "dtype": "int32"}, {"name": "caption_choices", "dtype": "string"}, {"name": "label", "dtype": "string"}, {"name": "n_tokens_label", "dtype": "int32"}, {"name": "instance_id", "dtype": "string"}], "splits": [{"name": "train", "num_bytes": 23163962.0, "num_examples": 390}, {"name": "validation", "num_bytes": 6778892.0, "num_examples": 131}, {"name": "test", "num_bytes": 7831536.0, "num_examples": 130}], "download_size": 37582524, "dataset_size": 37774390.0}, {"config_name": "matching", "features": [{"name": "image", "dtype": "image"}, {"name": "contest_number", "dtype": "int32"}, {"name": "image_location", "dtype": "string"}, {"name": "image_description", "dtype": "string"}, {"name": "image_uncanny_description", "dtype": "string"}, {"name": "entities", "sequence": "string"}, {"name": "questions", "sequence": "string"}, {"name": "caption_choices", "sequence": "string"}, {"name": "from_description", "dtype": "string"}, {"name": "label", "dtype": "string"}, {"name": "n_tokens_label", "dtype": "int32"}, {"name": "instance_id", "dtype": "string"}], "splits": [{"name": "train", "num_bytes": 618272766.36, "num_examples": 9792}, {"name": "validation", "num_bytes": 34157757.0, "num_examples": 531}, {"name": "test", "num_bytes": 29813118.0, "num_examples": 528}], "download_size": 594460072, "dataset_size": 682243641.36}, {"config_name": "matching_1", "features": [{"name": "image", "dtype": "image"}, {"name": "contest_number", "dtype": "int32"}, {"name": "image_location", "dtype": "string"}, {"name": "image_description", "dtype": "string"}, {"name": "image_uncanny_description", "dtype": "string"}, {"name": "entities", "sequence": "string"}, {"name": "questions", "sequence": "string"}, {"name": "caption_choices", "sequence": "string"}, {"name": "from_description", "dtype": "string"}, {"name": "label", "dtype": "string"}, {"name": "n_tokens_label", "dtype": "int32"}, {"name": "instance_id", "dtype": "string"}], "splits": [{"name": "train", "num_bytes": 593200158.116, "num_examples": 9684}, {"name": "validation", "num_bytes": 36712942.0, "num_examples": 546}, {"name": "test", "num_bytes": 34157757.0, "num_examples": 531}], "download_size": 563587231, "dataset_size": 664070857.116}, {"config_name": "matching_2", "features": [{"name": "image", "dtype": "image"}, {"name": "contest_number", "dtype": "int32"}, {"name": "image_location", "dtype": "string"}, {"name": "image_description", "dtype": "string"}, {"name": "image_uncanny_description", "dtype": "string"}, {"name": "entities", "sequence": "string"}, {"name": "questions", "sequence": "string"}, {"name": "caption_choices", "sequence": "string"}, {"name": "from_description", "dtype": "string"}, {"name": "label", "dtype": "string"}, {"name": "n_tokens_label", "dtype": "int32"}, {"name": "instance_id", "dtype": "string"}], "splits": [{"name": "train", "num_bytes": 591676321.09, "num_examples": 9630}, {"name": "validation", "num_bytes": 33697178.0, "num_examples": 540}, {"name": "test", "num_bytes": 36712942.0, "num_examples": 546}], "download_size": 571864348, "dataset_size": 662086441.09}, {"config_name": "matching_3", "features": [{"name": "image", "dtype": "image"}, {"name": "contest_number", "dtype": "int32"}, {"name": "image_location", "dtype": "string"}, {"name": "image_description", "dtype": "string"}, {"name": "image_uncanny_description", "dtype": "string"}, {"name": "entities", "sequence": "string"}, {"name": "questions", "sequence": "string"}, {"name": "caption_choices", "sequence": "string"}, {"name": "from_description", "dtype": "string"}, {"name": "label", "dtype": "string"}, {"name": "n_tokens_label", "dtype": "int32"}, {"name": "instance_id", "dtype": "string"}], "splits": [{"name": "train", "num_bytes": 615620189.53, "num_examples": 9630}, {"name": "validation", "num_bytes": 34829502.0, "num_examples": 546}, {"name": "test", "num_bytes": 33697178.0, "num_examples": 540}], "download_size": 571744845, "dataset_size": 684146869.53}, {"config_name": "matching_4", "features": [{"name": "image", "dtype": "image"}, {"name": "contest_number", "dtype": "int32"}, {"name": "image_location", "dtype": "string"}, {"name": "image_description", "dtype": "string"}, {"name": "image_uncanny_description", "dtype": "string"}, {"name": "entities", "sequence": "string"}, {"name": "questions", "sequence": "string"}, {"name": "caption_choices", "sequence": "string"}, {"name": "from_description", "dtype": "string"}, {"name": "label", "dtype": "string"}, {"name": "n_tokens_label", "dtype": "int32"}, {"name": "instance_id", "dtype": "string"}], "splits": [{"name": "train", "num_bytes": 609696610.648, "num_examples": 9702}, {"name": "validation", "num_bytes": 29813118.0, "num_examples": 528}, {"name": "test", "num_bytes": 34829502.0, "num_examples": 546}], "download_size": 592174904, "dataset_size": 674339230.648}, {"config_name": "matching_from_pixels", "features": [{"name": "image", "dtype": "image"}, {"name": "contest_number", "dtype": "int32"}, {"name": "caption_choices", "sequence": "string"}, {"name": "label", "dtype": "string"}, {"name": "n_tokens_label", "dtype": "int32"}, {"name": "instance_id", "dtype": "string"}], "splits": [{"name": "train", "num_bytes": 101439044.384, "num_examples": 1632}, {"name": "validation", "num_bytes": 33714551.0, "num_examples": 531}, {"name": "test", "num_bytes": 29368704.0, "num_examples": 528}], "download_size": 139733134, "dataset_size": 164522299.384}, {"config_name": "matching_from_pixels_1", "features": [{"name": "image", "dtype": "image"}, {"name": "contest_number", "dtype": "int32"}, {"name": "caption_choices", "sequence": "string"}, {"name": "label", "dtype": "string"}, {"name": "n_tokens_label", "dtype": "int32"}, {"name": "instance_id", "dtype": "string"}], "splits": [{"name": "train", "num_bytes": 94090646.83, "num_examples": 1614}, {"name": "validation", "num_bytes": 36257141.0, "num_examples": 546}, {"name": "test", "num_bytes": 33714551.0, "num_examples": 531}], "download_size": 137278691, "dataset_size": 164062338.82999998}, {"config_name": "matching_from_pixels_2", "features": [{"name": "image", "dtype": "image"}, {"name": "contest_number", "dtype": "int32"}, {"name": "caption_choices", "sequence": "string"}, {"name": "label", "dtype": "string"}, {"name": "n_tokens_label", "dtype": "int32"}, {"name": "instance_id", "dtype": "string"}], "splits": [{"name": "train", "num_bytes": 96253584.505, "num_examples": 1605}, {"name": "validation", "num_bytes": 33236000.0, "num_examples": 540}, {"name": "test", "num_bytes": 36257141.0, "num_examples": 546}], "download_size": 137890850, "dataset_size": 165746725.505}, {"config_name": "matching_from_pixels_3", "features": [{"name": "image", "dtype": "image"}, {"name": "contest_number", "dtype": "int32"}, {"name": "caption_choices", "sequence": "string"}, {"name": "label", "dtype": "string"}, {"name": "n_tokens_label", "dtype": "int32"}, {"name": "instance_id", "dtype": "string"}], "splits": [{"name": "train", "num_bytes": 99928910.28, "num_examples": 1605}, {"name": "validation", "num_bytes": 34380303.0, "num_examples": 546}, {"name": "test", "num_bytes": 33236000.0, "num_examples": 540}], "download_size": 139585876, "dataset_size": 167545213.28}, {"config_name": "matching_from_pixels_4", "features": [{"name": "image", "dtype": "image"}, {"name": "contest_number", "dtype": "int32"}, {"name": "caption_choices", "sequence": "string"}, {"name": "label", "dtype": "string"}, {"name": "n_tokens_label", "dtype": "int32"}, {"name": "instance_id", "dtype": "string"}], "splits": [{"name": "train", "num_bytes": 102509197.79, "num_examples": 1617}, {"name": "validation", "num_bytes": 29368704.0, "num_examples": 528}, {"name": "test", "num_bytes": 34380303.0, "num_examples": 546}], "download_size": 138725891, "dataset_size": 166258204.79000002}, {"config_name": "ranking", "features": [{"name": "image", "dtype": "image"}, {"name": "contest_number", "dtype": "int32"}, {"name": "image_location", "dtype": "string"}, {"name": "image_description", "dtype": "string"}, {"name": "image_uncanny_description", "dtype": "string"}, {"name": "entities", "sequence": "string"}, {"name": "questions", "sequence": "string"}, {"name": "caption_choices", "sequence": "string"}, {"name": "from_description", "dtype": "string"}, {"name": "winner_source", "dtype": "string"}, {"name": "label", "dtype": "string"}, {"name": "n_tokens_label", "dtype": "int32"}, {"name": "instance_id", "dtype": "string"}], "splits": [{"name": "train", "num_bytes": 594615535.632, "num_examples": 9576}, {"name": "validation", "num_bytes": 32624105.0, "num_examples": 507}, {"name": "test", "num_bytes": 28907567.0, "num_examples": 513}], "download_size": 571604579, "dataset_size": 656147207.632}, {"config_name": "ranking_1", "features": [{"name": "image", "dtype": "image"}, {"name": "contest_number", "dtype": "int32"}, {"name": "image_location", "dtype": "string"}, {"name": "image_description", "dtype": "string"}, {"name": "image_uncanny_description", "dtype": "string"}, {"name": "entities", "sequence": "string"}, {"name": "questions", "sequence": "string"}, {"name": "caption_choices", "sequence": "string"}, {"name": "from_description", "dtype": "string"}, {"name": "winner_source", "dtype": "string"}, {"name": "label", "dtype": "string"}, {"name": "n_tokens_label", "dtype": "int32"}, {"name": "instance_id", "dtype": "string"}], "splits": [{"name": "train", "num_bytes": 580099188.9, "num_examples": 9450}, {"name": "validation", "num_bytes": 35332200.0, "num_examples": 534}, {"name": "test", "num_bytes": 32624105.0, "num_examples": 507}], "download_size": 546559254, "dataset_size": 648055493.9}, {"config_name": "ranking_2", "features": [{"name": "image", "dtype": "image"}, {"name": "contest_number", "dtype": "int32"}, {"name": "image_location", "dtype": "string"}, {"name": "image_description", "dtype": "string"}, {"name": "image_uncanny_description", "dtype": "string"}, {"name": "entities", "sequence": "string"}, {"name": "questions", "sequence": "string"}, {"name": "caption_choices", "sequence": "string"}, {"name": "from_description", "dtype": "string"}, {"name": "winner_source", "dtype": "string"}, {"name": "label", "dtype": "string"}, {"name": "n_tokens_label", "dtype": "int32"}, {"name": "instance_id", "dtype": "string"}], "splits": [{"name": "train", "num_bytes": 566811450.504, "num_examples": 9306}, {"name": "validation", "num_bytes": 32519173.0, "num_examples": 531}, {"name": "test", "num_bytes": 35332200.0, "num_examples": 534}], "download_size": 544444097, "dataset_size": 634662823.504}, {"config_name": "ranking_3", "features": [{"name": "image", "dtype": "image"}, {"name": "contest_number", "dtype": "int32"}, {"name": "image_location", "dtype": "string"}, {"name": "image_description", "dtype": "string"}, {"name": "image_uncanny_description", "dtype": "string"}, {"name": "entities", "sequence": "string"}, {"name": "questions", "sequence": "string"}, {"name": "caption_choices", "sequence": "string"}, {"name": "from_description", "dtype": "string"}, {"name": "winner_source", "dtype": "string"}, {"name": "label", "dtype": "string"}, {"name": "n_tokens_label", "dtype": "int32"}, {"name": "instance_id", "dtype": "string"}], "splits": [{"name": "train", "num_bytes": 577828323.272, "num_examples": 9324}, {"name": "validation", "num_bytes": 34072817.0, "num_examples": 531}, {"name": "test", "num_bytes": 32519173.0, "num_examples": 531}], "download_size": 548880699, "dataset_size": 644420313.272}, {"config_name": "ranking_4", "features": [{"name": "image", "dtype": "image"}, {"name": "contest_number", "dtype": "int32"}, {"name": "image_location", "dtype": "string"}, {"name": "image_description", "dtype": "string"}, {"name": "image_uncanny_description", "dtype": "string"}, {"name": "entities", "sequence": "string"}, {"name": "questions", "sequence": "string"}, {"name": "caption_choices", "sequence": "string"}, {"name": "from_description", "dtype": "string"}, {"name": "winner_source", "dtype": "string"}, {"name": "label", "dtype": "string"}, {"name": "n_tokens_label", "dtype": "int32"}, {"name": "instance_id", "dtype": "string"}], "splits": [{"name": "train", "num_bytes": 593388719.232, "num_examples": 9432}, {"name": "validation", "num_bytes": 28907567.0, "num_examples": 513}, {"name": "test", "num_bytes": 34072817.0, "num_examples": 531}], "download_size": 562902941, "dataset_size": 656369103.232}, {"config_name": "ranking_from_pixels", "features": [{"name": "image", "dtype": "image"}, {"name": "contest_number", "dtype": "int32"}, {"name": "caption_choices", "sequence": "string"}, {"name": "winner_source", "dtype": "string"}, {"name": "label", "dtype": "string"}, {"name": "n_tokens_label", "dtype": "int32"}, {"name": "instance_id", "dtype": "string"}], "splits": [{"name": "train", "num_bytes": 101282973.752, "num_examples": 1596}, {"name": "validation", "num_bytes": 32072331.0, "num_examples": 506}, {"name": "test", "num_bytes": 28550057.0, "num_examples": 513}], "download_size": 134283256, "dataset_size": 161905361.752}, {"config_name": "ranking_from_pixels_1", "features": [{"name": "image", "dtype": "image"}, {"name": "contest_number", "dtype": "int32"}, {"name": "caption_choices", "sequence": "string"}, {"name": "winner_source", "dtype": "string"}, {"name": "label", "dtype": "string"}, {"name": "n_tokens_label", "dtype": "int32"}, {"name": "instance_id", "dtype": "string"}], "splits": [{"name": "train", "num_bytes": 93123370.15, "num_examples": 1575}, {"name": "validation", "num_bytes": 34965110.0, "num_examples": 534}, {"name": "test", "num_bytes": 32072331.0, "num_examples": 506}], "download_size": 130879365, "dataset_size": 160160811.15}, {"config_name": "ranking_from_pixels_2", "features": [{"name": "image", "dtype": "image"}, {"name": "contest_number", "dtype": "int32"}, {"name": "caption_choices", "sequence": "string"}, {"name": "winner_source", "dtype": "string"}, {"name": "label", "dtype": "string"}, {"name": "n_tokens_label", "dtype": "int32"}, {"name": "instance_id", "dtype": "string"}], "splits": [{"name": "train", "num_bytes": 93496576.85, "num_examples": 1550}, {"name": "validation", "num_bytes": 32145436.0, "num_examples": 531}, {"name": "test", "num_bytes": 34965110.0, "num_examples": 534}], "download_size": 131637359, "dataset_size": 160607122.85}, {"config_name": "ranking_from_pixels_3", "features": [{"name": "image", "dtype": "image"}, {"name": "contest_number", "dtype": "int32"}, {"name": "caption_choices", "sequence": "string"}, {"name": "winner_source", "dtype": "string"}, {"name": "label", "dtype": "string"}, {"name": "n_tokens_label", "dtype": "int32"}, {"name": "instance_id", "dtype": "string"}], "splits": [{"name": "train", "num_bytes": 93840620.26, "num_examples": 1553}, {"name": "validation", "num_bytes": 33718821.0, "num_examples": 531}, {"name": "test", "num_bytes": 32145436.0, "num_examples": 531}], "download_size": 133214495, "dataset_size": 159704877.26}, {"config_name": "ranking_from_pixels_4", "features": [{"name": "image", "dtype": "image"}, {"name": "contest_number", "dtype": "int32"}, {"name": "caption_choices", "sequence": "string"}, {"name": "winner_source", "dtype": "string"}, {"name": "label", "dtype": "string"}, {"name": "n_tokens_label", "dtype": "int32"}, {"name": "instance_id", "dtype": "string"}], "splits": [{"name": "train", "num_bytes": 99008131.43, "num_examples": 1571}, {"name": "validation", "num_bytes": 28550057.0, "num_examples": 513}, {"name": "test", "num_bytes": 33718821.0, "num_examples": 531}], "download_size": 136230399, "dataset_size": 161277009.43}], "configs": [{"config_name": "explanation", "data_files": [{"split": "train", "path": "explanation/train-*"}, {"split": "validation", "path": "explanation/validation-*"}, {"split": "test", "path": "explanation/test-*"}]}, {"config_name": "explanation_1", "data_files": [{"split": "train", "path": "explanation_1/train-*"}, {"split": "validation", "path": "explanation_1/validation-*"}, {"split": "test", "path": "explanation_1/test-*"}]}, {"config_name": "explanation_2", "data_files": [{"split": "train", "path": "explanation_2/train-*"}, {"split": "validation", "path": "explanation_2/validation-*"}, {"split": "test", "path": "explanation_2/test-*"}]}, {"config_name": "explanation_3", "data_files": [{"split": "train", "path": "explanation_3/train-*"}, {"split": "validation", "path": "explanation_3/validation-*"}, {"split": "test", "path": "explanation_3/test-*"}]}, {"config_name": "explanation_4", "data_files": [{"split": "train", "path": "explanation_4/train-*"}, {"split": "validation", "path": "explanation_4/validation-*"}, {"split": "test", "path": "explanation_4/test-*"}]}, {"config_name": "explanation_from_pixels", "data_files": [{"split": "train", "path": "explanation_from_pixels/train-*"}, {"split": "validation", "path": "explanation_from_pixels/validation-*"}, {"split": "test", "path": "explanation_from_pixels/test-*"}]}, {"config_name": "explanation_from_pixels_1", "data_files": [{"split": "train", "path": "explanation_from_pixels_1/train-*"}, {"split": "validation", "path": "explanation_from_pixels_1/validation-*"}, {"split": "test", "path": "explanation_from_pixels_1/test-*"}]}, {"config_name": "explanation_from_pixels_2", "data_files": [{"split": "train", "path": "explanation_from_pixels_2/train-*"}, {"split": "validation", "path": "explanation_from_pixels_2/validation-*"}, {"split": "test", "path": "explanation_from_pixels_2/test-*"}]}, {"config_name": "explanation_from_pixels_3", "data_files": [{"split": "train", "path": "explanation_from_pixels_3/train-*"}, {"split": "validation", "path": "explanation_from_pixels_3/validation-*"}, {"split": "test", "path": "explanation_from_pixels_3/test-*"}]}, {"config_name": "explanation_from_pixels_4", "data_files": [{"split": "train", "path": "explanation_from_pixels_4/train-*"}, {"split": "validation", "path": "explanation_from_pixels_4/validation-*"}, {"split": "test", "path": "explanation_from_pixels_4/test-*"}]}, {"config_name": "matching", "data_files": [{"split": "train", "path": "matching/train-*"}, {"split": "validation", "path": "matching/validation-*"}, {"split": "test", "path": "matching/test-*"}]}, {"config_name": "matching_1", "data_files": [{"split": "train", "path": "matching_1/train-*"}, {"split": "validation", "path": "matching_1/validation-*"}, {"split": "test", "path": "matching_1/test-*"}]}, {"config_name": "matching_2", "data_files": [{"split": "train", "path": "matching_2/train-*"}, {"split": "validation", "path": "matching_2/validation-*"}, {"split": "test", "path": "matching_2/test-*"}]}, {"config_name": "matching_3", "data_files": [{"split": "train", "path": "matching_3/train-*"}, {"split": "validation", "path": "matching_3/validation-*"}, {"split": "test", "path": "matching_3/test-*"}]}, {"config_name": "matching_4", "data_files": [{"split": "train", "path": "matching_4/train-*"}, {"split": "validation", "path": "matching_4/validation-*"}, {"split": "test", "path": "matching_4/test-*"}]}, {"config_name": "matching_from_pixels", "data_files": [{"split": "train", "path": "matching_from_pixels/train-*"}, {"split": "validation", "path": "matching_from_pixels/validation-*"}, {"split": "test", "path": "matching_from_pixels/test-*"}]}, {"config_name": "matching_from_pixels_1", "data_files": [{"split": "train", "path": "matching_from_pixels_1/train-*"}, {"split": "validation", "path": "matching_from_pixels_1/validation-*"}, {"split": "test", "path": "matching_from_pixels_1/test-*"}]}, {"config_name": "matching_from_pixels_2", "data_files": [{"split": "train", "path": "matching_from_pixels_2/train-*"}, {"split": "validation", "path": "matching_from_pixels_2/validation-*"}, {"split": "test", "path": "matching_from_pixels_2/test-*"}]}, {"config_name": "matching_from_pixels_3", "data_files": [{"split": "train", "path": "matching_from_pixels_3/train-*"}, {"split": "validation", "path": "matching_from_pixels_3/validation-*"}, {"split": "test", "path": "matching_from_pixels_3/test-*"}]}, {"config_name": "matching_from_pixels_4", "data_files": [{"split": "train", "path": "matching_from_pixels_4/train-*"}, {"split": "validation", "path": "matching_from_pixels_4/validation-*"}, {"split": "test", "path": "matching_from_pixels_4/test-*"}]}, {"config_name": "ranking", "data_files": [{"split": "train", "path": "ranking/train-*"}, {"split": "validation", "path": "ranking/validation-*"}, {"split": "test", "path": "ranking/test-*"}]}, {"config_name": "ranking_1", "data_files": [{"split": "train", "path": "ranking_1/train-*"}, {"split": "validation", "path": "ranking_1/validation-*"}, {"split": "test", "path": "ranking_1/test-*"}]}, {"config_name": "ranking_2", "data_files": [{"split": "train", "path": "ranking_2/train-*"}, {"split": "validation", "path": "ranking_2/validation-*"}, {"split": "test", "path": "ranking_2/test-*"}]}, {"config_name": "ranking_3", "data_files": [{"split": "train", "path": "ranking_3/train-*"}, {"split": "validation", "path": "ranking_3/validation-*"}, {"split": "test", "path": "ranking_3/test-*"}]}, {"config_name": "ranking_4", "data_files": [{"split": "train", "path": "ranking_4/train-*"}, {"split": "validation", "path": "ranking_4/validation-*"}, {"split": "test", "path": "ranking_4/test-*"}]}, {"config_name": "ranking_from_pixels", "data_files": [{"split": "train", "path": "ranking_from_pixels/train-*"}, {"split": "validation", "path": "ranking_from_pixels/validation-*"}, {"split": "test", "path": "ranking_from_pixels/test-*"}]}, {"config_name": "ranking_from_pixels_1", "data_files": [{"split": "train", "path": "ranking_from_pixels_1/train-*"}, {"split": "validation", "path": "ranking_from_pixels_1/validation-*"}, {"split": "test", "path": "ranking_from_pixels_1/test-*"}]}, {"config_name": "ranking_from_pixels_2", "data_files": [{"split": "train", "path": "ranking_from_pixels_2/train-*"}, {"split": "validation", "path": "ranking_from_pixels_2/validation-*"}, {"split": "test", "path": "ranking_from_pixels_2/test-*"}]}, {"config_name": "ranking_from_pixels_3", "data_files": [{"split": "train", "path": "ranking_from_pixels_3/train-*"}, {"split": "validation", "path": "ranking_from_pixels_3/validation-*"}, {"split": "test", "path": "ranking_from_pixels_3/test-*"}]}, {"config_name": "ranking_from_pixels_4", "data_files": [{"split": "train", "path": "ranking_from_pixels_4/train-*"}, {"split": "validation", "path": "ranking_from_pixels_4/validation-*"}, {"split": "test", "path": "ranking_from_pixels_4/test-*"}]}]} | 2023-12-22T19:13:58+00:00 | [
"2209.06293"
] | [
"en"
] | TAGS
#task_categories-image-to-text #task_categories-multiple-choice #task_categories-text-classification #task_categories-text-generation #task_categories-visual-question-answering #task_categories-other #task_categories-text2text-generation #task_ids-multi-class-classification #task_ids-language-modeling #task_ids-visual-question-answering #task_ids-explanation-generation #annotations_creators-expert-generated #annotations_creators-crowdsourced #annotations_creators-found #language_creators-crowdsourced #language_creators-expert-generated #multilinguality-monolingual #size_categories-1K<n<10K #source_datasets-original #language-English #license-cc-by-4.0 #humor #caption contest #new yorker #arxiv-2209.06293 #region-us
|
# Dataset Card for New Yorker Caption Contest Benchmarks
## Table of Contents
- Table of Contents
- Dataset Description
- Dataset Summary
- Supported Tasks and Leaderboards
- Languages
- Dataset Structure
- Data Instances
- Data Fields
- Data Splits
- Dataset Creation
- Curation Rationale
- Source Data
- Annotations
- Personal and Sensitive Information
- Considerations for Using the Data
- Social Impact of Dataset
- Discussion of Biases
- Other Known Limitations
- Additional Information
- Dataset Curators
- Licensing Information
- Citation Information
- Contributions
## Dataset Description
- Homepage: URL
- Repository: URL
- Paper: Do Androids Laugh at Electric Sheep? Humor "Understanding" Benchmarks from The New Yorker Caption Contest
- Leaderboard: URL
- Point of Contact: jmhessel@URL
### Dataset Summary
See URL for more!
Data from:
Do Androids Laugh at Electric Sheep? Humor "Understanding" Benchmarks from The New Yorker Caption Contest
If you use this dataset, we would appreciate you citing our work, but also -- several other papers that we build this corpus upon. See Citation Information.
We challenge AI models to "demonstrate understanding" of the
sophisticated multimodal humor of The New Yorker Caption Contest.
Concretely, we develop three carefully circumscribed tasks for which
it suffices (but is not necessary) to grasp potentially complex and
unexpected relationships between image and caption, and similarly
complex and unexpected allusions to the wide varieties of human
experience.
### Supported Tasks and Leaderboards
Three tasks are supported:
- "Matching:" a model must recognize a caption written about a cartoon (vs. options that were not);
- "Quality ranking:" a model must evaluate the quality of a caption by scoring it more highly than a lower quality option from the same contest;
- "Explanation:" a model must explain why a given joke is funny.
There are no official leaderboards (yet).
### Languages
English
## Dataset Structure
Here's an example instance from Matching:
The label "C" indicates that the 3rd choice in the 'caption_choices' is correct.
Here's an example instance from Ranking (in the from pixels setting --- though, this is also available in the from description setting)
the label indicates that the first caption choice ("A", here) in the 'caption_choices' list was more highly rated.
Here's an example instance from Explanation:
The label is an explanation of the joke, which serves as the autoregressive target.
### Data Instances
See above
### Data Fields
See above
### Data Splits
Data splits can be accessed as:
Or, in the from pixels setting, e.g.,
Because the dataset is small, we reported in 5-fold cross-validation setting initially. The default splits are split 0. You can access the other splits, e.g.:
## Dataset Creation
Full details are in the paper.
### Curation Rationale
See the paper for rationale/motivation.
### Source Data
See citation below. We combined 3 sources of data, and added significant annotations of our own.
#### Initial Data Collection and Normalization
Full details are in the paper.
#### Who are the source language producers?
We paid crowdworkers $15/hr to annotate the corpus.
In addition, significant annotation efforts were conducted by the authors of this work.
### Annotations
Full details are in the paper.
#### Annotation process
Full details are in the paper.
#### Who are the annotators?
A mix of crowdworks and authors of this paper.
### Personal and Sensitive Information
Has been redacted from the dataset. Images are published in the New Yorker already.
## Considerations for Using the Data
### Social Impact of Dataset
It's plausible that humor could perpetuate negative stereotypes. The jokes in this corpus are a mix of crowdsourced entries that are highly rated, and ones published in the new yorker.
### Discussion of Biases
Humor is subjective, and some of the jokes may be considered offensive. The images may contain adult themes and minor cartoon nudity.
### Other Known Limitations
More details are in the paper
## Additional Information
### Dataset Curators
The dataset was curated by researchers at AI2
### Licensing Information
The annotations we provide are CC-BY-4.0. See URL for more info.
Our data contributions are:
- The cartoon-level annotations;
- The joke explanations;
- and the framing of the tasks
We release these data we contribute under CC-BY (see DATASET_LICENSE). If you find this data useful in your work, in addition to citing our contributions, please also cite the following, from which the cartoons/captions in our corpus are derived:
| [
"# Dataset Card for New Yorker Caption Contest Benchmarks",
"## Table of Contents\n- Table of Contents\n- Dataset Description\n - Dataset Summary\n - Supported Tasks and Leaderboards\n - Languages\n- Dataset Structure\n - Data Instances\n - Data Fields\n - Data Splits\n- Dataset Creation\n - Curation Rationale\n - Source Data\n - Annotations\n - Personal and Sensitive Information\n- Considerations for Using the Data\n - Social Impact of Dataset\n - Discussion of Biases\n - Other Known Limitations\n- Additional Information\n - Dataset Curators\n - Licensing Information\n - Citation Information\n - Contributions",
"## Dataset Description\n\n- Homepage: URL\n- Repository: URL\n- Paper: Do Androids Laugh at Electric Sheep? Humor \"Understanding\" Benchmarks from The New Yorker Caption Contest\n- Leaderboard: URL\n- Point of Contact: jmhessel@URL",
"### Dataset Summary\n\nSee URL for more!\n\nData from:\nDo Androids Laugh at Electric Sheep? Humor \"Understanding\" Benchmarks from The New Yorker Caption Contest\n\n\n\nIf you use this dataset, we would appreciate you citing our work, but also -- several other papers that we build this corpus upon. See Citation Information.\n\nWe challenge AI models to \"demonstrate understanding\" of the\nsophisticated multimodal humor of The New Yorker Caption Contest.\nConcretely, we develop three carefully circumscribed tasks for which\nit suffices (but is not necessary) to grasp potentially complex and\nunexpected relationships between image and caption, and similarly\ncomplex and unexpected allusions to the wide varieties of human\nexperience.",
"### Supported Tasks and Leaderboards\n\nThree tasks are supported:\n\n- \"Matching:\" a model must recognize a caption written about a cartoon (vs. options that were not);\n- \"Quality ranking:\" a model must evaluate the quality of a caption by scoring it more highly than a lower quality option from the same contest;\n- \"Explanation:\" a model must explain why a given joke is funny.\n\nThere are no official leaderboards (yet).",
"### Languages\n\nEnglish",
"## Dataset Structure\n\nHere's an example instance from Matching:\n\n\nThe label \"C\" indicates that the 3rd choice in the 'caption_choices' is correct.\n\nHere's an example instance from Ranking (in the from pixels setting --- though, this is also available in the from description setting)\n\nthe label indicates that the first caption choice (\"A\", here) in the 'caption_choices' list was more highly rated.\n\n\nHere's an example instance from Explanation:\n\nThe label is an explanation of the joke, which serves as the autoregressive target.",
"### Data Instances\n\nSee above",
"### Data Fields\n\nSee above",
"### Data Splits\n\nData splits can be accessed as:\n\n\nOr, in the from pixels setting, e.g.,\n\n\nBecause the dataset is small, we reported in 5-fold cross-validation setting initially. The default splits are split 0. You can access the other splits, e.g.:",
"## Dataset Creation\n\nFull details are in the paper.",
"### Curation Rationale\n\nSee the paper for rationale/motivation.",
"### Source Data\n\nSee citation below. We combined 3 sources of data, and added significant annotations of our own.",
"#### Initial Data Collection and Normalization\n\nFull details are in the paper.",
"#### Who are the source language producers?\n\nWe paid crowdworkers $15/hr to annotate the corpus.\nIn addition, significant annotation efforts were conducted by the authors of this work.",
"### Annotations\n\nFull details are in the paper.",
"#### Annotation process\n\nFull details are in the paper.",
"#### Who are the annotators?\n\nA mix of crowdworks and authors of this paper.",
"### Personal and Sensitive Information\n\nHas been redacted from the dataset. Images are published in the New Yorker already.",
"## Considerations for Using the Data",
"### Social Impact of Dataset\n\nIt's plausible that humor could perpetuate negative stereotypes. The jokes in this corpus are a mix of crowdsourced entries that are highly rated, and ones published in the new yorker.",
"### Discussion of Biases\n\nHumor is subjective, and some of the jokes may be considered offensive. The images may contain adult themes and minor cartoon nudity.",
"### Other Known Limitations\n\nMore details are in the paper",
"## Additional Information",
"### Dataset Curators\n\nThe dataset was curated by researchers at AI2",
"### Licensing Information\n\nThe annotations we provide are CC-BY-4.0. See URL for more info.\n\n\n\n\n\n\nOur data contributions are:\n\n- The cartoon-level annotations;\n- The joke explanations;\n- and the framing of the tasks\n\nWe release these data we contribute under CC-BY (see DATASET_LICENSE). If you find this data useful in your work, in addition to citing our contributions, please also cite the following, from which the cartoons/captions in our corpus are derived:"
] | [
"TAGS\n#task_categories-image-to-text #task_categories-multiple-choice #task_categories-text-classification #task_categories-text-generation #task_categories-visual-question-answering #task_categories-other #task_categories-text2text-generation #task_ids-multi-class-classification #task_ids-language-modeling #task_ids-visual-question-answering #task_ids-explanation-generation #annotations_creators-expert-generated #annotations_creators-crowdsourced #annotations_creators-found #language_creators-crowdsourced #language_creators-expert-generated #multilinguality-monolingual #size_categories-1K<n<10K #source_datasets-original #language-English #license-cc-by-4.0 #humor #caption contest #new yorker #arxiv-2209.06293 #region-us \n",
"# Dataset Card for New Yorker Caption Contest Benchmarks",
"## Table of Contents\n- Table of Contents\n- Dataset Description\n - Dataset Summary\n - Supported Tasks and Leaderboards\n - Languages\n- Dataset Structure\n - Data Instances\n - Data Fields\n - Data Splits\n- Dataset Creation\n - Curation Rationale\n - Source Data\n - Annotations\n - Personal and Sensitive Information\n- Considerations for Using the Data\n - Social Impact of Dataset\n - Discussion of Biases\n - Other Known Limitations\n- Additional Information\n - Dataset Curators\n - Licensing Information\n - Citation Information\n - Contributions",
"## Dataset Description\n\n- Homepage: URL\n- Repository: URL\n- Paper: Do Androids Laugh at Electric Sheep? Humor \"Understanding\" Benchmarks from The New Yorker Caption Contest\n- Leaderboard: URL\n- Point of Contact: jmhessel@URL",
"### Dataset Summary\n\nSee URL for more!\n\nData from:\nDo Androids Laugh at Electric Sheep? Humor \"Understanding\" Benchmarks from The New Yorker Caption Contest\n\n\n\nIf you use this dataset, we would appreciate you citing our work, but also -- several other papers that we build this corpus upon. See Citation Information.\n\nWe challenge AI models to \"demonstrate understanding\" of the\nsophisticated multimodal humor of The New Yorker Caption Contest.\nConcretely, we develop three carefully circumscribed tasks for which\nit suffices (but is not necessary) to grasp potentially complex and\nunexpected relationships between image and caption, and similarly\ncomplex and unexpected allusions to the wide varieties of human\nexperience.",
"### Supported Tasks and Leaderboards\n\nThree tasks are supported:\n\n- \"Matching:\" a model must recognize a caption written about a cartoon (vs. options that were not);\n- \"Quality ranking:\" a model must evaluate the quality of a caption by scoring it more highly than a lower quality option from the same contest;\n- \"Explanation:\" a model must explain why a given joke is funny.\n\nThere are no official leaderboards (yet).",
"### Languages\n\nEnglish",
"## Dataset Structure\n\nHere's an example instance from Matching:\n\n\nThe label \"C\" indicates that the 3rd choice in the 'caption_choices' is correct.\n\nHere's an example instance from Ranking (in the from pixels setting --- though, this is also available in the from description setting)\n\nthe label indicates that the first caption choice (\"A\", here) in the 'caption_choices' list was more highly rated.\n\n\nHere's an example instance from Explanation:\n\nThe label is an explanation of the joke, which serves as the autoregressive target.",
"### Data Instances\n\nSee above",
"### Data Fields\n\nSee above",
"### Data Splits\n\nData splits can be accessed as:\n\n\nOr, in the from pixels setting, e.g.,\n\n\nBecause the dataset is small, we reported in 5-fold cross-validation setting initially. The default splits are split 0. You can access the other splits, e.g.:",
"## Dataset Creation\n\nFull details are in the paper.",
"### Curation Rationale\n\nSee the paper for rationale/motivation.",
"### Source Data\n\nSee citation below. We combined 3 sources of data, and added significant annotations of our own.",
"#### Initial Data Collection and Normalization\n\nFull details are in the paper.",
"#### Who are the source language producers?\n\nWe paid crowdworkers $15/hr to annotate the corpus.\nIn addition, significant annotation efforts were conducted by the authors of this work.",
"### Annotations\n\nFull details are in the paper.",
"#### Annotation process\n\nFull details are in the paper.",
"#### Who are the annotators?\n\nA mix of crowdworks and authors of this paper.",
"### Personal and Sensitive Information\n\nHas been redacted from the dataset. Images are published in the New Yorker already.",
"## Considerations for Using the Data",
"### Social Impact of Dataset\n\nIt's plausible that humor could perpetuate negative stereotypes. The jokes in this corpus are a mix of crowdsourced entries that are highly rated, and ones published in the new yorker.",
"### Discussion of Biases\n\nHumor is subjective, and some of the jokes may be considered offensive. The images may contain adult themes and minor cartoon nudity.",
"### Other Known Limitations\n\nMore details are in the paper",
"## Additional Information",
"### Dataset Curators\n\nThe dataset was curated by researchers at AI2",
"### Licensing Information\n\nThe annotations we provide are CC-BY-4.0. See URL for more info.\n\n\n\n\n\n\nOur data contributions are:\n\n- The cartoon-level annotations;\n- The joke explanations;\n- and the framing of the tasks\n\nWe release these data we contribute under CC-BY (see DATASET_LICENSE). If you find this data useful in your work, in addition to citing our contributions, please also cite the following, from which the cartoons/captions in our corpus are derived:"
] | [
255,
14,
125,
60,
161,
102,
5,
130,
8,
7,
70,
12,
17,
27,
17,
43,
12,
12,
22,
27,
8,
51,
38,
13,
5,
18,
114
] | [
"passage: TAGS\n#task_categories-image-to-text #task_categories-multiple-choice #task_categories-text-classification #task_categories-text-generation #task_categories-visual-question-answering #task_categories-other #task_categories-text2text-generation #task_ids-multi-class-classification #task_ids-language-modeling #task_ids-visual-question-answering #task_ids-explanation-generation #annotations_creators-expert-generated #annotations_creators-crowdsourced #annotations_creators-found #language_creators-crowdsourced #language_creators-expert-generated #multilinguality-monolingual #size_categories-1K<n<10K #source_datasets-original #language-English #license-cc-by-4.0 #humor #caption contest #new yorker #arxiv-2209.06293 #region-us \n# Dataset Card for New Yorker Caption Contest Benchmarks## Table of Contents\n- Table of Contents\n- Dataset Description\n - Dataset Summary\n - Supported Tasks and Leaderboards\n - Languages\n- Dataset Structure\n - Data Instances\n - Data Fields\n - Data Splits\n- Dataset Creation\n - Curation Rationale\n - Source Data\n - Annotations\n - Personal and Sensitive Information\n- Considerations for Using the Data\n - Social Impact of Dataset\n - Discussion of Biases\n - Other Known Limitations\n- Additional Information\n - Dataset Curators\n - Licensing Information\n - Citation Information\n - Contributions## Dataset Description\n\n- Homepage: URL\n- Repository: URL\n- Paper: Do Androids Laugh at Electric Sheep? Humor \"Understanding\" Benchmarks from The New Yorker Caption Contest\n- Leaderboard: URL\n- Point of Contact: jmhessel@URL",
"passage: ### Dataset Summary\n\nSee URL for more!\n\nData from:\nDo Androids Laugh at Electric Sheep? Humor \"Understanding\" Benchmarks from The New Yorker Caption Contest\n\n\n\nIf you use this dataset, we would appreciate you citing our work, but also -- several other papers that we build this corpus upon. See Citation Information.\n\nWe challenge AI models to \"demonstrate understanding\" of the\nsophisticated multimodal humor of The New Yorker Caption Contest.\nConcretely, we develop three carefully circumscribed tasks for which\nit suffices (but is not necessary) to grasp potentially complex and\nunexpected relationships between image and caption, and similarly\ncomplex and unexpected allusions to the wide varieties of human\nexperience.### Supported Tasks and Leaderboards\n\nThree tasks are supported:\n\n- \"Matching:\" a model must recognize a caption written about a cartoon (vs. options that were not);\n- \"Quality ranking:\" a model must evaluate the quality of a caption by scoring it more highly than a lower quality option from the same contest;\n- \"Explanation:\" a model must explain why a given joke is funny.\n\nThere are no official leaderboards (yet).### Languages\n\nEnglish## Dataset Structure\n\nHere's an example instance from Matching:\n\n\nThe label \"C\" indicates that the 3rd choice in the 'caption_choices' is correct.\n\nHere's an example instance from Ranking (in the from pixels setting --- though, this is also available in the from description setting)\n\nthe label indicates that the first caption choice (\"A\", here) in the 'caption_choices' list was more highly rated.\n\n\nHere's an example instance from Explanation:\n\nThe label is an explanation of the joke, which serves as the autoregressive target.### Data Instances\n\nSee above### Data Fields\n\nSee above### Data Splits\n\nData splits can be accessed as:\n\n\nOr, in the from pixels setting, e.g.,\n\n\nBecause the dataset is small, we reported in 5-fold cross-validation setting initially. The default splits are split 0. You can access the other splits, e.g.:## Dataset Creation\n\nFull details are in the paper.### Curation Rationale\n\nSee the paper for rationale/motivation.### Source Data\n\nSee citation below. We combined 3 sources of data, and added significant annotations of our own.#### Initial Data Collection and Normalization\n\nFull details are in the paper.#### Who are the source language producers?\n\nWe paid crowdworkers $15/hr to annotate the corpus.\nIn addition, significant annotation efforts were conducted by the authors of this work.### Annotations\n\nFull details are in the paper.#### Annotation process\n\nFull details are in the paper.#### Who are the annotators?\n\nA mix of crowdworks and authors of this paper."
] |
d2e593d645e8b7d71ab76738be13269f96b0139b | # AutoTrain Dataset for project: github-emotion-surprise
## Dataset Description
Dataset used in the paper: Imran et al., ["Data Augmentation for Improving Emotion Recognition in Software Engineering Communication"](https://arxiv.org/abs/2208.05573), ASE-2022.
### Languages
The BCP-47 code for the dataset's language is unk.
## Dataset Structure
### Data Instances
A sample from this dataset looks as follows:
```json
[
{
"feat_id": 704844644,
"text": "This change doesn't affect anything but makes the code more clear. If you look at the line about, `currentUrlTree` is set to `urlAfterRedirects`.",
"feat_Anger": 0,
"feat_Love": 0,
"feat_Fear": 0,
"feat_Joy": 1,
"feat_Sadness": 0,
"target": 0
},
{
"feat_id": 886568180,
"text": "Thanks very much for your feedback [USER] Your point is totally fair. My intention was to highlight that camelCase or dash-case class names are perfectly fine to use in Angular templates. Most people, especially beginners, do not know that and end up using the `ngClass` directive. Do you think that rewording the alert towards that direction would make sense?",
"feat_Anger": 0,
"feat_Love": 1,
"feat_Fear": 0,
"feat_Joy": 0,
"feat_Sadness": 0,
"target": 0
}
]
```
### Dataset Fields
The dataset has the following fields (also called "features"):
```json
{
"feat_id": "Value(dtype='int64', id=None)",
"text": "Value(dtype='string', id=None)",
"feat_Anger": "Value(dtype='int64', id=None)",
"feat_Love": "Value(dtype='int64', id=None)",
"feat_Fear": "Value(dtype='int64', id=None)",
"feat_Joy": "Value(dtype='int64', id=None)",
"feat_Sadness": "Value(dtype='int64', id=None)",
"target": "ClassLabel(num_classes=2, names=['0', '1'], id=None)"
}
```
### Dataset Splits
This dataset is split into a train and validation split. The split sizes are as follow:
| Split name | Num samples |
| ------------ | ------------------- |
| train | 1600 |
| valid | 400 |
| imranraad/github-emotion-surprise | [
"task_categories:text-classification",
"arxiv:2208.05573",
"doi:10.57967/hf/0050",
"region:us"
] | 2022-09-29T20:03:25+00:00 | {"task_categories": ["text-classification"]} | 2022-10-20T09:18:22+00:00 | [
"2208.05573"
] | [] | TAGS
#task_categories-text-classification #arxiv-2208.05573 #doi-10.57967/hf/0050 #region-us
| AutoTrain Dataset for project: github-emotion-surprise
======================================================
Dataset Description
-------------------
Dataset used in the paper: Imran et al., "Data Augmentation for Improving Emotion Recognition in Software Engineering Communication", ASE-2022.
### Languages
The BCP-47 code for the dataset's language is unk.
Dataset Structure
-----------------
### Data Instances
A sample from this dataset looks as follows:
### Dataset Fields
The dataset has the following fields (also called "features"):
### Dataset Splits
This dataset is split into a train and validation split. The split sizes are as follow:
| [
"### Languages\n\n\nThe BCP-47 code for the dataset's language is unk.\n\n\nDataset Structure\n-----------------",
"### Data Instances\n\n\nA sample from this dataset looks as follows:",
"### Dataset Fields\n\n\nThe dataset has the following fields (also called \"features\"):",
"### Dataset Splits\n\n\nThis dataset is split into a train and validation split. The split sizes are as follow:"
] | [
"TAGS\n#task_categories-text-classification #arxiv-2208.05573 #doi-10.57967/hf/0050 #region-us \n",
"### Languages\n\n\nThe BCP-47 code for the dataset's language is unk.\n\n\nDataset Structure\n-----------------",
"### Data Instances\n\n\nA sample from this dataset looks as follows:",
"### Dataset Fields\n\n\nThe dataset has the following fields (also called \"features\"):",
"### Dataset Splits\n\n\nThis dataset is split into a train and validation split. The split sizes are as follow:"
] | [
37,
27,
17,
23,
27
] | [
"passage: TAGS\n#task_categories-text-classification #arxiv-2208.05573 #doi-10.57967/hf/0050 #region-us \n### Languages\n\n\nThe BCP-47 code for the dataset's language is unk.\n\n\nDataset Structure\n-----------------### Data Instances\n\n\nA sample from this dataset looks as follows:### Dataset Fields\n\n\nThe dataset has the following fields (also called \"features\"):### Dataset Splits\n\n\nThis dataset is split into a train and validation split. The split sizes are as follow:"
] |
8d818753c4d4b3541433a20d2a7008e4e3cfa427 | pictures | wallyg/Pictures | [
"region:us"
] | 2022-09-29T20:04:21+00:00 | {} | 2022-09-29T20:20:59+00:00 | [] | [] | TAGS
#region-us
| pictures | [] | [
"TAGS\n#region-us \n"
] | [
6
] | [
"passage: TAGS\n#region-us \n"
] |
3d4df382ad4507ff652d99e244bb3e3c1532d0a0 | # AutoTrain Dataset for project: github-emotion-love
## Dataset Description
Dataset used in the paper: Imran et al., ["Data Augmentation for Improving Emotion Recognition in Software Engineering Communication"](https://arxiv.org/abs/2208.05573), ASE-2022.
### Languages
The BCP-47 code for the dataset's language is unk.
## Dataset Structure
### Data Instances
A sample from this dataset looks as follows:
```json
[
{
"feat_id": 704844644,
"text": "This change doesn't affect anything but makes the code more clear. If you look at the line about, `currentUrlTree` is set to `urlAfterRedirects`.",
"feat_Anger": 0,
"target": 0,
"feat_Fear": 0,
"feat_Joy": 1,
"feat_Sadness": 0,
"feat_Surprise": 0
},
{
"feat_id": 886568180,
"text": "Thanks very much for your feedback [USER] Your point is totally fair. My intention was to highlight that camelCase or dash-case class names are perfectly fine to use in Angular templates. Most people, especially beginners, do not know that and end up using the `ngClass` directive. Do you think that rewording the alert towards that direction would make sense?",
"feat_Anger": 0,
"target": 1,
"feat_Fear": 0,
"feat_Joy": 0,
"feat_Sadness": 0,
"feat_Surprise": 0
}
]
```
### Dataset Fields
The dataset has the following fields (also called "features"):
```json
{
"feat_id": "Value(dtype='int64', id=None)",
"text": "Value(dtype='string', id=None)",
"feat_Anger": "Value(dtype='int64', id=None)",
"target": "ClassLabel(num_classes=2, names=['0', '1'], id=None)",
"feat_Fear": "Value(dtype='int64', id=None)",
"feat_Joy": "Value(dtype='int64', id=None)",
"feat_Sadness": "Value(dtype='int64', id=None)",
"feat_Surprise": "Value(dtype='int64', id=None)"
}
```
### Dataset Splits
This dataset is split into a train and validation split. The split sizes are as follow:
| Split name | Num samples |
| ------------ | ------------------- |
| train | 1600 |
| valid | 400 |
| imranraad/github-emotion-love | [
"task_categories:text-classification",
"arxiv:2208.05573",
"doi:10.57967/hf/0049",
"region:us"
] | 2022-09-29T20:10:30+00:00 | {"task_categories": ["text-classification"]} | 2022-10-20T09:18:07+00:00 | [
"2208.05573"
] | [] | TAGS
#task_categories-text-classification #arxiv-2208.05573 #doi-10.57967/hf/0049 #region-us
| AutoTrain Dataset for project: github-emotion-love
==================================================
Dataset Description
-------------------
Dataset used in the paper: Imran et al., "Data Augmentation for Improving Emotion Recognition in Software Engineering Communication", ASE-2022.
### Languages
The BCP-47 code for the dataset's language is unk.
Dataset Structure
-----------------
### Data Instances
A sample from this dataset looks as follows:
### Dataset Fields
The dataset has the following fields (also called "features"):
### Dataset Splits
This dataset is split into a train and validation split. The split sizes are as follow:
| [
"### Languages\n\n\nThe BCP-47 code for the dataset's language is unk.\n\n\nDataset Structure\n-----------------",
"### Data Instances\n\n\nA sample from this dataset looks as follows:",
"### Dataset Fields\n\n\nThe dataset has the following fields (also called \"features\"):",
"### Dataset Splits\n\n\nThis dataset is split into a train and validation split. The split sizes are as follow:"
] | [
"TAGS\n#task_categories-text-classification #arxiv-2208.05573 #doi-10.57967/hf/0049 #region-us \n",
"### Languages\n\n\nThe BCP-47 code for the dataset's language is unk.\n\n\nDataset Structure\n-----------------",
"### Data Instances\n\n\nA sample from this dataset looks as follows:",
"### Dataset Fields\n\n\nThe dataset has the following fields (also called \"features\"):",
"### Dataset Splits\n\n\nThis dataset is split into a train and validation split. The split sizes are as follow:"
] | [
38,
27,
17,
23,
27
] | [
"passage: TAGS\n#task_categories-text-classification #arxiv-2208.05573 #doi-10.57967/hf/0049 #region-us \n### Languages\n\n\nThe BCP-47 code for the dataset's language is unk.\n\n\nDataset Structure\n-----------------### Data Instances\n\n\nA sample from this dataset looks as follows:### Dataset Fields\n\n\nThe dataset has the following fields (also called \"features\"):### Dataset Splits\n\n\nThis dataset is split into a train and validation split. The split sizes are as follow:"
] |
6a151c5d80f3c0d00af267e030daca4f42df9012 | Imagenes:
"https://huggingface.co/datasets/sati93/fotos/resolve/main/me1.jpg",
"https://huggingface.co/datasets/sati93/fotos/resolve/main/me2.jpg",
"https://huggingface.co/datasets/sati93/fotos/resolve/main/me3.jpg",
"https://huggingface.co/datasets/sati93/fotos/resolve/main/me4.jpg",
"https://huggingface.co/datasets/sati93/fotos/resolve/main/me5.jpg",
"https://huggingface.co/datasets/sati93/fotos/resolve/main/me6.jpg",
"https://huggingface.co/datasets/sati93/fotos/resolve/main/me7.jpg",
"https://huggingface.co/datasets/sati93/fotos/resolve/main/me8.jpg",
"https://huggingface.co/datasets/sati93/fotos/resolve/main/me9.jpg",
"https://huggingface.co/datasets/sati93/fotos/resolve/main/me10.jpg",
"https://huggingface.co/datasets/sati93/fotos/resolve/main/me11.jpg",
"https://huggingface.co/datasets/sati93/fotos/resolve/main/me12.jpg",
"https://huggingface.co/datasets/sati93/fotos/resolve/main/me13.jpg",
"https://huggingface.co/datasets/sati93/fotos/resolve/main/me14.jpg",
"https://huggingface.co/datasets/sati93/fotos/resolve/main/me15.jpg",
"https://huggingface.co/datasets/sati93/fotos/resolve/main/me16.jpg",
"https://huggingface.co/datasets/sati93/fotos/resolve/main/me17.jpg",
"https://huggingface.co/datasets/sati93/fotos/resolve/main/me18.jpg",
"https://huggingface.co/datasets/sati93/fotos/resolve/main/me19.jpg",
"https://huggingface.co/datasets/sati93/fotos/resolve/main/me20.jpg",
"https://huggingface.co/datasets/sati93/fotos/resolve/main/me21.jpg",
Configuracion:
instance_prompt: sati
prior_preservation_class_prompt: person
| sati93/fotos | [
"region:us"
] | 2022-09-29T23:28:59+00:00 | {} | 2022-10-02T19:19:26+00:00 | [] | [] | TAGS
#region-us
| Imagenes:
"URL
"URL
"URL
"URL
"URL
"URL
"URL
"URL
"URL
"URL
"URL
"URL
"URL
"URL
"URL
"URL
"URL
"URL
"URL
"URL
"URL
Configuracion:
instance_prompt: sati
prior_preservation_class_prompt: person
| [] | [
"TAGS\n#region-us \n"
] | [
6
] | [
"passage: TAGS\n#region-us \n"
] |
627e5cc137bcd577a9769bbb108ff97c65cd8aac | scratch directory for storing image datasets which are processed through a clip embedding model!
---
license: mit
---
| murphyk/dogs-cats-small-clip-embedding | [
"region:us"
] | 2022-09-30T00:38:19+00:00 | {} | 2022-09-30T02:46:33+00:00 | [] | [] | TAGS
#region-us
| scratch directory for storing image datasets which are processed through a clip embedding model!
---
license: mit
---
| [] | [
"TAGS\n#region-us \n"
] | [
6
] | [
"passage: TAGS\n#region-us \n"
] |
e99e27c90f20307ebbefd7e79e35255a62de3118 |
# Dataset Card for Reflections in Peer Counseling
## Table of Contents
- [Table of Contents](#table-of-contents)
- [Dataset Description](#dataset-description)
- [Dataset Summary](#dataset-summary)
- [Supported Tasks and Leaderboards](#supported-tasks-and-leaderboards)
- [Languages](#languages)
- [Dataset Structure](#dataset-structure)
- [Data Instances](#data-instances)
- [Data Fields](#data-fields)
- [Data Splits](#data-splits)
- [Dataset Creation](#dataset-creation)
- [Curation Rationale](#curation-rationale)
- [Source Data](#source-data)
- [Annotations](#annotations)
- [Personal and Sensitive Information](#personal-and-sensitive-information)
- [Considerations for Using the Data](#considerations-for-using-the-data)
- [Social Impact of Dataset](#social-impact-of-dataset)
- [Discussion of Biases](#discussion-of-biases)
- [Other Known Limitations](#other-known-limitations)
- [Additional Information](#additional-information)
- [Dataset Curators](#dataset-curators)
- [Licensing Information](#licensing-information)
- [Citation Information](#citation-information)
- [Contributions](#contributions)
## Dataset Description
- **Homepage:**
- **Repository:**
- **Paper: Automatic Reflection Generation for Peer-to-Peer Counseling**
- **Point of Contact: [email protected]**
### Dataset Summary
The dataset derives from conversations between clients and counselors on a large peer-to-peer online counseling service. There are a total of 1061 observations across training and testing datasets, with 50 additional randomly sampled examples used in defining the few-shot learning prompt or for validation purposes in tuning hyperparameters, thus totaling 1111 observations across these sets. These observations were sourced from a larger dataset consisting of annotations of several different clinical counseling skills. We thus focus on the annotations of counselor reflections. The counselor reflections were annotated at utterance level with counselor verbal behaviors using the Motivational Interviewing Treatment Integrity 4.2 (MITI) and the Motivational Interviewing Skill Code 2.5 (MISC) manuals. Thus, the entire dataset consists of conversational context-counselor reflection pairs.
### Supported Tasks and Leaderboards
The dataset was used for conditioning and tuning generative models for generating reflection statements in the domain of peer-to-peer counseling.
### Languages
The language in the dataset is English.
## Dataset Structure
### Data Instances
Each instance consists of the chat room id of the conversation in which the dialogue occurred, the prompt which is the conversational context that immediately precedes the counselor reflection (including previous utterances from either the client or counselor up until and including the most recent prior client message that immediately followed a counselorโs message), and the completion which is the counselor reflection.
```
{
'chat_id': "1234567",
'prompt': "Client: I'm 19, he's 25. He's not very considerate of how I feel but says he cares about me and loves me.\nCounselor:",
'completion': " The words are easy, actions are needed. Guys who are 25 just desire to have different experiences.\n\n",
}
```
### Data Fields
* `chat_id`: an integer defining the chat id of the conversation
* `prompt`: a string corresponding to the conversational context preceding the counselor reflection with the messages separated by new line characters and each utterance prepended by 'Client:' or 'Counselor:'. The string ends with 'Counselor:' to indicate that it is followed by the counselor completion described below.
* `completion`: a string corresponding to the counselor reflection
### Data Splits
The dataset is split into training, testing, and a small set of 50 examples used either for designing the few-shot learning prompt or tuning hyperparameters. 911 examples were used for training. 350 of these examples also constitute a reduced training set used in comparative experiments. 150 examples were used for testing. 50 of these testing examples (randomly selected) were used in the human evaluation. We ensured that the chat identifiers for messages in the test set uniquely differed from those included in the training set.
## Dataset Creation
### Curation Rationale
Reflective listening is a critical skill in peer-to-peer counseling that is only effective when tailored to the context. Thus, we wanted to home in on this particular skill and explore the potential of state-of-the-art language models for text generation in this domain.
### Source Data
#### Initial Data Collection and Normalization
The dataset was created by filtering the larger dataset of utterances annotated for many different counseling skills to only those counselor messages annotated as reflections. Then, the prompt instances were created by identifying the preceding messages for each of these counselor reflection instances. After the prompts were initially created, prompts with less than or equal to five words were removed.
The author created reference reflections for each of the 350 training example prompts in the reduced training set and each of the 150 testing example prompts. In creating a reference reflection given each conversational context, the author intended to simulate responding to the client in roughly the same time a counselor would as if this turn was embedded in a conversation the client was having with the author. This gauging of time is based on the authorโs experience in volunteering as a counselor at crisis hotlines. It is possible that the reference reflections were created in roughly even less time than an average counselor response given that there were hundreds of conversational contexts for which reflections needed to be created.
#### Who are the source language producers?
The 'client' messages are utterances of those seeking mental health support on a large online counseling service platform. The 'counselor' messages are utterances of minimally-trained peer counselors of this large online counseling service.
For each of the 350 training example prompts in the reduced training set and each of the 150 testing example prompts, a reference reflection was also created by the author.
### Annotations
#### Annotation process
The human evaluation examined text of generative models fine-tuned on the full training set, a reduced training set, and reference reflections; a few-shot learning model; the actual counselor; and the reference reflection.
We administered a survey through Amazon Mechanical Turk Developer Sandbox. 50 of the testing prompts were provided along with the corresponding six response sources. Provided with the conversational context, the annotators evaluated responses based on three criteria: fluency, resemblance of reflection, and overall preference. Thus, for each context, evaluators measured the fluency, reflection resemblance, and overall preference for all six candidate responses.
We used a variation of Efficient Annotation of Scalar Labels (EASL), a hybrid approach between direct assessment and online pairwise ranking aggregation and rank-based magnitude estimation. Evaluators saw all six responses at once (without knowledge of each responseโs origin) and used a sliding scale from 1 to 5 to rate the responses based on each of the three dimensions. The order of the model responses for each conversational context was randomized. We provided examples of response ratings for ratings of 1 and 5 on the overall fluency and reflection resemblance dimensions. However, we did not include an example for overall preference, noting its subjectivity. The order of the model responses for each conversational context was randomized. We provided examples of response ratings for ratings of 1 and 5 on the overall fluency and reflection resemblance dimensions. However, we did not include an example for overall preference, noting its subjectivity.
Fluency refers to the response's overall fluency and human-likeness. In the instructions, we noted non-capitalized words and colloquial language are acceptable and not to be considered fluency errors. Reflection resemblance refers to whether the response captures and returns to the client something the client has said. Overall preference refers to the extent to which the evaluator likes the response.
Using Krippendorffโs alpha, we measured inter-annotator agreement, obtaining alpha values of -0.0369, 0.557, and 0.358 for overall fluency, reflection resemblance, and overall preference, respectively. Although these agreement values are low, the 0.557 inter-annotator agreement we obtained for reflection resemblance is notably higher than the inter-annotator agreement obtained for reflection likeness in the most relevant prior work.
#### Who are the annotators?
The three annotators recruited for the human evaluation were familiar with counseling reflections. All three annotators have worked with this large online counseling service dataset with IRB approval. They are quite familiar with motivational interviewing codes, annotating messages and using large language models for mass labeling.
### Personal and Sensitive Information
Due to the sensitive nature of this dataset and privacy concerns, we are unable to publicly share the data.
## Considerations for Using the Data
### Social Impact of Dataset
This dataset of reflections in peer-to-peer counseling can be used as a reference point in understanding and evaluating counselor clinical skills and furthering the potential of language technology to be applied in this space. Given the sensitive nature of the mental health care context and the minimal training of these counselors, the use of such data requires care in understanding the limitations of technology defined based on this language.
### Discussion of Biases
Much of the language of conversations on this online counseling service platform is very informal and some client and counselor utterances may also contain pejorative language.
As for the generated text assessed in the human evaluation of this work, it is important to note that GPT-3 was trained on over 45 terabytes of data from the internet and books, and large volumes of data collected from online sources will inevitably contain biases that may be captured. There may thus be inadvertent discrimination against subclasses of particular protected groups. Using generated responses as a source of guidance rather than using generative systems as the counselors themselves may be able to balance the benefits and risks of using artificial intelligence in delicate mental health settings. It is imperative that such systems are not misused by companies seeking to maximize efficiency and minimize cost.
The reference reflections in this work were created by the author, whose experience with counseling and motivational interviewing derives from over one hundred hours of training at a teen-to-teen crisis hotline and textline service and experience through a research fellowship developing and user testing a platform for nurses to practice and grow their motivational interviewing skills. Therefore, the reference reflections may not be as clinically precise as are possible from a medical professional, and the diversity of reflections is inherently limited.
### Other Known Limitations
## Additional Information
### Dataset Curators
Developed by Emma O'Neil, Joรฃo Sedoc, Diyi Yang, Haiyi Zhu, Lyle Ungar.
### Licensing Information
### Citation Information
### Contributions
Thanks to [@emoneil](https://github.com/emoneil) for adding this dataset. | emoneil/reflections-in-peer-counseling | [
"task_categories:summarization",
"task_categories:text-generation",
"task_categories:conversational",
"task_ids:dialogue-generation",
"annotations_creators:expert-generated",
"size_categories:1K<n<10K",
"gpt3",
"natural language processing",
"natural language generation",
"peer counseling",
"region:us"
] | 2022-09-30T03:21:28+00:00 | {"annotations_creators": ["expert-generated"], "language_creators": [], "language": [], "license": [], "size_categories": ["1K<n<10K"], "source_datasets": [], "task_categories": ["summarization", "text-generation", "conversational"], "task_ids": ["dialogue-generation"], "pretty_name": "Reflections in Peer Counseling", "tags": ["gpt3", "natural language processing", "natural language generation", "peer counseling"]} | 2022-10-14T02:59:04+00:00 | [] | [] | TAGS
#task_categories-summarization #task_categories-text-generation #task_categories-conversational #task_ids-dialogue-generation #annotations_creators-expert-generated #size_categories-1K<n<10K #gpt3 #natural language processing #natural language generation #peer counseling #region-us
|
# Dataset Card for Reflections in Peer Counseling
## Table of Contents
- Table of Contents
- Dataset Description
- Dataset Summary
- Supported Tasks and Leaderboards
- Languages
- Dataset Structure
- Data Instances
- Data Fields
- Data Splits
- Dataset Creation
- Curation Rationale
- Source Data
- Annotations
- Personal and Sensitive Information
- Considerations for Using the Data
- Social Impact of Dataset
- Discussion of Biases
- Other Known Limitations
- Additional Information
- Dataset Curators
- Licensing Information
- Citation Information
- Contributions
## Dataset Description
- Homepage:
- Repository:
- Paper: Automatic Reflection Generation for Peer-to-Peer Counseling
- Point of Contact: emoneil@URL
### Dataset Summary
The dataset derives from conversations between clients and counselors on a large peer-to-peer online counseling service. There are a total of 1061 observations across training and testing datasets, with 50 additional randomly sampled examples used in defining the few-shot learning prompt or for validation purposes in tuning hyperparameters, thus totaling 1111 observations across these sets. These observations were sourced from a larger dataset consisting of annotations of several different clinical counseling skills. We thus focus on the annotations of counselor reflections. The counselor reflections were annotated at utterance level with counselor verbal behaviors using the Motivational Interviewing Treatment Integrity 4.2 (MITI) and the Motivational Interviewing Skill Code 2.5 (MISC) manuals. Thus, the entire dataset consists of conversational context-counselor reflection pairs.
### Supported Tasks and Leaderboards
The dataset was used for conditioning and tuning generative models for generating reflection statements in the domain of peer-to-peer counseling.
### Languages
The language in the dataset is English.
## Dataset Structure
### Data Instances
Each instance consists of the chat room id of the conversation in which the dialogue occurred, the prompt which is the conversational context that immediately precedes the counselor reflection (including previous utterances from either the client or counselor up until and including the most recent prior client message that immediately followed a counselorโs message), and the completion which is the counselor reflection.
### Data Fields
* 'chat_id': an integer defining the chat id of the conversation
* 'prompt': a string corresponding to the conversational context preceding the counselor reflection with the messages separated by new line characters and each utterance prepended by 'Client:' or 'Counselor:'. The string ends with 'Counselor:' to indicate that it is followed by the counselor completion described below.
* 'completion': a string corresponding to the counselor reflection
### Data Splits
The dataset is split into training, testing, and a small set of 50 examples used either for designing the few-shot learning prompt or tuning hyperparameters. 911 examples were used for training. 350 of these examples also constitute a reduced training set used in comparative experiments. 150 examples were used for testing. 50 of these testing examples (randomly selected) were used in the human evaluation. We ensured that the chat identifiers for messages in the test set uniquely differed from those included in the training set.
## Dataset Creation
### Curation Rationale
Reflective listening is a critical skill in peer-to-peer counseling that is only effective when tailored to the context. Thus, we wanted to home in on this particular skill and explore the potential of state-of-the-art language models for text generation in this domain.
### Source Data
#### Initial Data Collection and Normalization
The dataset was created by filtering the larger dataset of utterances annotated for many different counseling skills to only those counselor messages annotated as reflections. Then, the prompt instances were created by identifying the preceding messages for each of these counselor reflection instances. After the prompts were initially created, prompts with less than or equal to five words were removed.
The author created reference reflections for each of the 350 training example prompts in the reduced training set and each of the 150 testing example prompts. In creating a reference reflection given each conversational context, the author intended to simulate responding to the client in roughly the same time a counselor would as if this turn was embedded in a conversation the client was having with the author. This gauging of time is based on the authorโs experience in volunteering as a counselor at crisis hotlines. It is possible that the reference reflections were created in roughly even less time than an average counselor response given that there were hundreds of conversational contexts for which reflections needed to be created.
#### Who are the source language producers?
The 'client' messages are utterances of those seeking mental health support on a large online counseling service platform. The 'counselor' messages are utterances of minimally-trained peer counselors of this large online counseling service.
For each of the 350 training example prompts in the reduced training set and each of the 150 testing example prompts, a reference reflection was also created by the author.
### Annotations
#### Annotation process
The human evaluation examined text of generative models fine-tuned on the full training set, a reduced training set, and reference reflections; a few-shot learning model; the actual counselor; and the reference reflection.
We administered a survey through Amazon Mechanical Turk Developer Sandbox. 50 of the testing prompts were provided along with the corresponding six response sources. Provided with the conversational context, the annotators evaluated responses based on three criteria: fluency, resemblance of reflection, and overall preference. Thus, for each context, evaluators measured the fluency, reflection resemblance, and overall preference for all six candidate responses.
We used a variation of Efficient Annotation of Scalar Labels (EASL), a hybrid approach between direct assessment and online pairwise ranking aggregation and rank-based magnitude estimation. Evaluators saw all six responses at once (without knowledge of each responseโs origin) and used a sliding scale from 1 to 5 to rate the responses based on each of the three dimensions. The order of the model responses for each conversational context was randomized. We provided examples of response ratings for ratings of 1 and 5 on the overall fluency and reflection resemblance dimensions. However, we did not include an example for overall preference, noting its subjectivity. The order of the model responses for each conversational context was randomized. We provided examples of response ratings for ratings of 1 and 5 on the overall fluency and reflection resemblance dimensions. However, we did not include an example for overall preference, noting its subjectivity.
Fluency refers to the response's overall fluency and human-likeness. In the instructions, we noted non-capitalized words and colloquial language are acceptable and not to be considered fluency errors. Reflection resemblance refers to whether the response captures and returns to the client something the client has said. Overall preference refers to the extent to which the evaluator likes the response.
Using Krippendorffโs alpha, we measured inter-annotator agreement, obtaining alpha values of -0.0369, 0.557, and 0.358 for overall fluency, reflection resemblance, and overall preference, respectively. Although these agreement values are low, the 0.557 inter-annotator agreement we obtained for reflection resemblance is notably higher than the inter-annotator agreement obtained for reflection likeness in the most relevant prior work.
#### Who are the annotators?
The three annotators recruited for the human evaluation were familiar with counseling reflections. All three annotators have worked with this large online counseling service dataset with IRB approval. They are quite familiar with motivational interviewing codes, annotating messages and using large language models for mass labeling.
### Personal and Sensitive Information
Due to the sensitive nature of this dataset and privacy concerns, we are unable to publicly share the data.
## Considerations for Using the Data
### Social Impact of Dataset
This dataset of reflections in peer-to-peer counseling can be used as a reference point in understanding and evaluating counselor clinical skills and furthering the potential of language technology to be applied in this space. Given the sensitive nature of the mental health care context and the minimal training of these counselors, the use of such data requires care in understanding the limitations of technology defined based on this language.
### Discussion of Biases
Much of the language of conversations on this online counseling service platform is very informal and some client and counselor utterances may also contain pejorative language.
As for the generated text assessed in the human evaluation of this work, it is important to note that GPT-3 was trained on over 45 terabytes of data from the internet and books, and large volumes of data collected from online sources will inevitably contain biases that may be captured. There may thus be inadvertent discrimination against subclasses of particular protected groups. Using generated responses as a source of guidance rather than using generative systems as the counselors themselves may be able to balance the benefits and risks of using artificial intelligence in delicate mental health settings. It is imperative that such systems are not misused by companies seeking to maximize efficiency and minimize cost.
The reference reflections in this work were created by the author, whose experience with counseling and motivational interviewing derives from over one hundred hours of training at a teen-to-teen crisis hotline and textline service and experience through a research fellowship developing and user testing a platform for nurses to practice and grow their motivational interviewing skills. Therefore, the reference reflections may not be as clinically precise as are possible from a medical professional, and the diversity of reflections is inherently limited.
### Other Known Limitations
## Additional Information
### Dataset Curators
Developed by Emma O'Neil, Joรฃo Sedoc, Diyi Yang, Haiyi Zhu, Lyle Ungar.
### Licensing Information
### Contributions
Thanks to @emoneil for adding this dataset. | [
"# Dataset Card for Reflections in Peer Counseling",
"## Table of Contents\n- Table of Contents\n- Dataset Description\n - Dataset Summary\n - Supported Tasks and Leaderboards\n - Languages\n- Dataset Structure\n - Data Instances\n - Data Fields\n - Data Splits\n- Dataset Creation\n - Curation Rationale\n - Source Data\n - Annotations\n - Personal and Sensitive Information\n- Considerations for Using the Data\n - Social Impact of Dataset\n - Discussion of Biases\n - Other Known Limitations\n- Additional Information\n - Dataset Curators\n - Licensing Information\n - Citation Information\n - Contributions",
"## Dataset Description\n- Homepage:\n- Repository:\n- Paper: Automatic Reflection Generation for Peer-to-Peer Counseling\n- Point of Contact: emoneil@URL",
"### Dataset Summary\n\nThe dataset derives from conversations between clients and counselors on a large peer-to-peer online counseling service. There are a total of 1061 observations across training and testing datasets, with 50 additional randomly sampled examples used in defining the few-shot learning prompt or for validation purposes in tuning hyperparameters, thus totaling 1111 observations across these sets. These observations were sourced from a larger dataset consisting of annotations of several different clinical counseling skills. We thus focus on the annotations of counselor reflections. The counselor reflections were annotated at utterance level with counselor verbal behaviors using the Motivational Interviewing Treatment Integrity 4.2 (MITI) and the Motivational Interviewing Skill Code 2.5 (MISC) manuals. Thus, the entire dataset consists of conversational context-counselor reflection pairs.",
"### Supported Tasks and Leaderboards\n\nThe dataset was used for conditioning and tuning generative models for generating reflection statements in the domain of peer-to-peer counseling.",
"### Languages\n\nThe language in the dataset is English.",
"## Dataset Structure",
"### Data Instances\n\nEach instance consists of the chat room id of the conversation in which the dialogue occurred, the prompt which is the conversational context that immediately precedes the counselor reflection (including previous utterances from either the client or counselor up until and including the most recent prior client message that immediately followed a counselorโs message), and the completion which is the counselor reflection.",
"### Data Fields\n\n* 'chat_id': an integer defining the chat id of the conversation\n* 'prompt': a string corresponding to the conversational context preceding the counselor reflection with the messages separated by new line characters and each utterance prepended by 'Client:' or 'Counselor:'. The string ends with 'Counselor:' to indicate that it is followed by the counselor completion described below.\n* 'completion': a string corresponding to the counselor reflection",
"### Data Splits\n\nThe dataset is split into training, testing, and a small set of 50 examples used either for designing the few-shot learning prompt or tuning hyperparameters. 911 examples were used for training. 350 of these examples also constitute a reduced training set used in comparative experiments. 150 examples were used for testing. 50 of these testing examples (randomly selected) were used in the human evaluation. We ensured that the chat identifiers for messages in the test set uniquely differed from those included in the training set.",
"## Dataset Creation",
"### Curation Rationale\n\nReflective listening is a critical skill in peer-to-peer counseling that is only effective when tailored to the context. Thus, we wanted to home in on this particular skill and explore the potential of state-of-the-art language models for text generation in this domain.",
"### Source Data",
"#### Initial Data Collection and Normalization\n\nThe dataset was created by filtering the larger dataset of utterances annotated for many different counseling skills to only those counselor messages annotated as reflections. Then, the prompt instances were created by identifying the preceding messages for each of these counselor reflection instances. After the prompts were initially created, prompts with less than or equal to five words were removed.\n\nThe author created reference reflections for each of the 350 training example prompts in the reduced training set and each of the 150 testing example prompts. In creating a reference reflection given each conversational context, the author intended to simulate responding to the client in roughly the same time a counselor would as if this turn was embedded in a conversation the client was having with the author. This gauging of time is based on the authorโs experience in volunteering as a counselor at crisis hotlines. It is possible that the reference reflections were created in roughly even less time than an average counselor response given that there were hundreds of conversational contexts for which reflections needed to be created.",
"#### Who are the source language producers?\n\nThe 'client' messages are utterances of those seeking mental health support on a large online counseling service platform. The 'counselor' messages are utterances of minimally-trained peer counselors of this large online counseling service.\n\nFor each of the 350 training example prompts in the reduced training set and each of the 150 testing example prompts, a reference reflection was also created by the author.",
"### Annotations",
"#### Annotation process\n\nThe human evaluation examined text of generative models fine-tuned on the full training set, a reduced training set, and reference reflections; a few-shot learning model; the actual counselor; and the reference reflection.\n\nWe administered a survey through Amazon Mechanical Turk Developer Sandbox. 50 of the testing prompts were provided along with the corresponding six response sources. Provided with the conversational context, the annotators evaluated responses based on three criteria: fluency, resemblance of reflection, and overall preference. Thus, for each context, evaluators measured the fluency, reflection resemblance, and overall preference for all six candidate responses. \n\nWe used a variation of Efficient Annotation of Scalar Labels (EASL), a hybrid approach between direct assessment and online pairwise ranking aggregation and rank-based magnitude estimation. Evaluators saw all six responses at once (without knowledge of each responseโs origin) and used a sliding scale from 1 to 5 to rate the responses based on each of the three dimensions. The order of the model responses for each conversational context was randomized. We provided examples of response ratings for ratings of 1 and 5 on the overall fluency and reflection resemblance dimensions. However, we did not include an example for overall preference, noting its subjectivity. The order of the model responses for each conversational context was randomized. We provided examples of response ratings for ratings of 1 and 5 on the overall fluency and reflection resemblance dimensions. However, we did not include an example for overall preference, noting its subjectivity.\n\nFluency refers to the response's overall fluency and human-likeness. In the instructions, we noted non-capitalized words and colloquial language are acceptable and not to be considered fluency errors. Reflection resemblance refers to whether the response captures and returns to the client something the client has said. Overall preference refers to the extent to which the evaluator likes the response.\n\nUsing Krippendorffโs alpha, we measured inter-annotator agreement, obtaining alpha values of -0.0369, 0.557, and 0.358 for overall fluency, reflection resemblance, and overall preference, respectively. Although these agreement values are low, the 0.557 inter-annotator agreement we obtained for reflection resemblance is notably higher than the inter-annotator agreement obtained for reflection likeness in the most relevant prior work.",
"#### Who are the annotators?\n\nThe three annotators recruited for the human evaluation were familiar with counseling reflections. All three annotators have worked with this large online counseling service dataset with IRB approval. They are quite familiar with motivational interviewing codes, annotating messages and using large language models for mass labeling.",
"### Personal and Sensitive Information\n\nDue to the sensitive nature of this dataset and privacy concerns, we are unable to publicly share the data.",
"## Considerations for Using the Data",
"### Social Impact of Dataset\n\nThis dataset of reflections in peer-to-peer counseling can be used as a reference point in understanding and evaluating counselor clinical skills and furthering the potential of language technology to be applied in this space. Given the sensitive nature of the mental health care context and the minimal training of these counselors, the use of such data requires care in understanding the limitations of technology defined based on this language.",
"### Discussion of Biases\n\nMuch of the language of conversations on this online counseling service platform is very informal and some client and counselor utterances may also contain pejorative language. \n\nAs for the generated text assessed in the human evaluation of this work, it is important to note that GPT-3 was trained on over 45 terabytes of data from the internet and books, and large volumes of data collected from online sources will inevitably contain biases that may be captured. There may thus be inadvertent discrimination against subclasses of particular protected groups. Using generated responses as a source of guidance rather than using generative systems as the counselors themselves may be able to balance the benefits and risks of using artificial intelligence in delicate mental health settings. It is imperative that such systems are not misused by companies seeking to maximize efficiency and minimize cost.\n\nThe reference reflections in this work were created by the author, whose experience with counseling and motivational interviewing derives from over one hundred hours of training at a teen-to-teen crisis hotline and textline service and experience through a research fellowship developing and user testing a platform for nurses to practice and grow their motivational interviewing skills. Therefore, the reference reflections may not be as clinically precise as are possible from a medical professional, and the diversity of reflections is inherently limited.",
"### Other Known Limitations",
"## Additional Information",
"### Dataset Curators\n\nDeveloped by Emma O'Neil, Joรฃo Sedoc, Diyi Yang, Haiyi Zhu, Lyle Ungar.",
"### Licensing Information",
"### Contributions\n\nThanks to @emoneil for adding this dataset."
] | [
"TAGS\n#task_categories-summarization #task_categories-text-generation #task_categories-conversational #task_ids-dialogue-generation #annotations_creators-expert-generated #size_categories-1K<n<10K #gpt3 #natural language processing #natural language generation #peer counseling #region-us \n",
"# Dataset Card for Reflections in Peer Counseling",
"## Table of Contents\n- Table of Contents\n- Dataset Description\n - Dataset Summary\n - Supported Tasks and Leaderboards\n - Languages\n- Dataset Structure\n - Data Instances\n - Data Fields\n - Data Splits\n- Dataset Creation\n - Curation Rationale\n - Source Data\n - Annotations\n - Personal and Sensitive Information\n- Considerations for Using the Data\n - Social Impact of Dataset\n - Discussion of Biases\n - Other Known Limitations\n- Additional Information\n - Dataset Curators\n - Licensing Information\n - Citation Information\n - Contributions",
"## Dataset Description\n- Homepage:\n- Repository:\n- Paper: Automatic Reflection Generation for Peer-to-Peer Counseling\n- Point of Contact: emoneil@URL",
"### Dataset Summary\n\nThe dataset derives from conversations between clients and counselors on a large peer-to-peer online counseling service. There are a total of 1061 observations across training and testing datasets, with 50 additional randomly sampled examples used in defining the few-shot learning prompt or for validation purposes in tuning hyperparameters, thus totaling 1111 observations across these sets. These observations were sourced from a larger dataset consisting of annotations of several different clinical counseling skills. We thus focus on the annotations of counselor reflections. The counselor reflections were annotated at utterance level with counselor verbal behaviors using the Motivational Interviewing Treatment Integrity 4.2 (MITI) and the Motivational Interviewing Skill Code 2.5 (MISC) manuals. Thus, the entire dataset consists of conversational context-counselor reflection pairs.",
"### Supported Tasks and Leaderboards\n\nThe dataset was used for conditioning and tuning generative models for generating reflection statements in the domain of peer-to-peer counseling.",
"### Languages\n\nThe language in the dataset is English.",
"## Dataset Structure",
"### Data Instances\n\nEach instance consists of the chat room id of the conversation in which the dialogue occurred, the prompt which is the conversational context that immediately precedes the counselor reflection (including previous utterances from either the client or counselor up until and including the most recent prior client message that immediately followed a counselorโs message), and the completion which is the counselor reflection.",
"### Data Fields\n\n* 'chat_id': an integer defining the chat id of the conversation\n* 'prompt': a string corresponding to the conversational context preceding the counselor reflection with the messages separated by new line characters and each utterance prepended by 'Client:' or 'Counselor:'. The string ends with 'Counselor:' to indicate that it is followed by the counselor completion described below.\n* 'completion': a string corresponding to the counselor reflection",
"### Data Splits\n\nThe dataset is split into training, testing, and a small set of 50 examples used either for designing the few-shot learning prompt or tuning hyperparameters. 911 examples were used for training. 350 of these examples also constitute a reduced training set used in comparative experiments. 150 examples were used for testing. 50 of these testing examples (randomly selected) were used in the human evaluation. We ensured that the chat identifiers for messages in the test set uniquely differed from those included in the training set.",
"## Dataset Creation",
"### Curation Rationale\n\nReflective listening is a critical skill in peer-to-peer counseling that is only effective when tailored to the context. Thus, we wanted to home in on this particular skill and explore the potential of state-of-the-art language models for text generation in this domain.",
"### Source Data",
"#### Initial Data Collection and Normalization\n\nThe dataset was created by filtering the larger dataset of utterances annotated for many different counseling skills to only those counselor messages annotated as reflections. Then, the prompt instances were created by identifying the preceding messages for each of these counselor reflection instances. After the prompts were initially created, prompts with less than or equal to five words were removed.\n\nThe author created reference reflections for each of the 350 training example prompts in the reduced training set and each of the 150 testing example prompts. In creating a reference reflection given each conversational context, the author intended to simulate responding to the client in roughly the same time a counselor would as if this turn was embedded in a conversation the client was having with the author. This gauging of time is based on the authorโs experience in volunteering as a counselor at crisis hotlines. It is possible that the reference reflections were created in roughly even less time than an average counselor response given that there were hundreds of conversational contexts for which reflections needed to be created.",
"#### Who are the source language producers?\n\nThe 'client' messages are utterances of those seeking mental health support on a large online counseling service platform. The 'counselor' messages are utterances of minimally-trained peer counselors of this large online counseling service.\n\nFor each of the 350 training example prompts in the reduced training set and each of the 150 testing example prompts, a reference reflection was also created by the author.",
"### Annotations",
"#### Annotation process\n\nThe human evaluation examined text of generative models fine-tuned on the full training set, a reduced training set, and reference reflections; a few-shot learning model; the actual counselor; and the reference reflection.\n\nWe administered a survey through Amazon Mechanical Turk Developer Sandbox. 50 of the testing prompts were provided along with the corresponding six response sources. Provided with the conversational context, the annotators evaluated responses based on three criteria: fluency, resemblance of reflection, and overall preference. Thus, for each context, evaluators measured the fluency, reflection resemblance, and overall preference for all six candidate responses. \n\nWe used a variation of Efficient Annotation of Scalar Labels (EASL), a hybrid approach between direct assessment and online pairwise ranking aggregation and rank-based magnitude estimation. Evaluators saw all six responses at once (without knowledge of each responseโs origin) and used a sliding scale from 1 to 5 to rate the responses based on each of the three dimensions. The order of the model responses for each conversational context was randomized. We provided examples of response ratings for ratings of 1 and 5 on the overall fluency and reflection resemblance dimensions. However, we did not include an example for overall preference, noting its subjectivity. The order of the model responses for each conversational context was randomized. We provided examples of response ratings for ratings of 1 and 5 on the overall fluency and reflection resemblance dimensions. However, we did not include an example for overall preference, noting its subjectivity.\n\nFluency refers to the response's overall fluency and human-likeness. In the instructions, we noted non-capitalized words and colloquial language are acceptable and not to be considered fluency errors. Reflection resemblance refers to whether the response captures and returns to the client something the client has said. Overall preference refers to the extent to which the evaluator likes the response.\n\nUsing Krippendorffโs alpha, we measured inter-annotator agreement, obtaining alpha values of -0.0369, 0.557, and 0.358 for overall fluency, reflection resemblance, and overall preference, respectively. Although these agreement values are low, the 0.557 inter-annotator agreement we obtained for reflection resemblance is notably higher than the inter-annotator agreement obtained for reflection likeness in the most relevant prior work.",
"#### Who are the annotators?\n\nThe three annotators recruited for the human evaluation were familiar with counseling reflections. All three annotators have worked with this large online counseling service dataset with IRB approval. They are quite familiar with motivational interviewing codes, annotating messages and using large language models for mass labeling.",
"### Personal and Sensitive Information\n\nDue to the sensitive nature of this dataset and privacy concerns, we are unable to publicly share the data.",
"## Considerations for Using the Data",
"### Social Impact of Dataset\n\nThis dataset of reflections in peer-to-peer counseling can be used as a reference point in understanding and evaluating counselor clinical skills and furthering the potential of language technology to be applied in this space. Given the sensitive nature of the mental health care context and the minimal training of these counselors, the use of such data requires care in understanding the limitations of technology defined based on this language.",
"### Discussion of Biases\n\nMuch of the language of conversations on this online counseling service platform is very informal and some client and counselor utterances may also contain pejorative language. \n\nAs for the generated text assessed in the human evaluation of this work, it is important to note that GPT-3 was trained on over 45 terabytes of data from the internet and books, and large volumes of data collected from online sources will inevitably contain biases that may be captured. There may thus be inadvertent discrimination against subclasses of particular protected groups. Using generated responses as a source of guidance rather than using generative systems as the counselors themselves may be able to balance the benefits and risks of using artificial intelligence in delicate mental health settings. It is imperative that such systems are not misused by companies seeking to maximize efficiency and minimize cost.\n\nThe reference reflections in this work were created by the author, whose experience with counseling and motivational interviewing derives from over one hundred hours of training at a teen-to-teen crisis hotline and textline service and experience through a research fellowship developing and user testing a platform for nurses to practice and grow their motivational interviewing skills. Therefore, the reference reflections may not be as clinically precise as are possible from a medical professional, and the diversity of reflections is inherently limited.",
"### Other Known Limitations",
"## Additional Information",
"### Dataset Curators\n\nDeveloped by Emma O'Neil, Joรฃo Sedoc, Diyi Yang, Haiyi Zhu, Lyle Ungar.",
"### Licensing Information",
"### Contributions\n\nThanks to @emoneil for adding this dataset."
] | [
92,
15,
125,
43,
210,
45,
13,
6,
87,
123,
124,
5,
70,
4,
246,
99,
5,
568,
76,
34,
8,
96,
303,
7,
5,
33,
6,
16
] | [
"passage: TAGS\n#task_categories-summarization #task_categories-text-generation #task_categories-conversational #task_ids-dialogue-generation #annotations_creators-expert-generated #size_categories-1K<n<10K #gpt3 #natural language processing #natural language generation #peer counseling #region-us \n# Dataset Card for Reflections in Peer Counseling## Table of Contents\n- Table of Contents\n- Dataset Description\n - Dataset Summary\n - Supported Tasks and Leaderboards\n - Languages\n- Dataset Structure\n - Data Instances\n - Data Fields\n - Data Splits\n- Dataset Creation\n - Curation Rationale\n - Source Data\n - Annotations\n - Personal and Sensitive Information\n- Considerations for Using the Data\n - Social Impact of Dataset\n - Discussion of Biases\n - Other Known Limitations\n- Additional Information\n - Dataset Curators\n - Licensing Information\n - Citation Information\n - Contributions## Dataset Description\n- Homepage:\n- Repository:\n- Paper: Automatic Reflection Generation for Peer-to-Peer Counseling\n- Point of Contact: emoneil@URL### Dataset Summary\n\nThe dataset derives from conversations between clients and counselors on a large peer-to-peer online counseling service. There are a total of 1061 observations across training and testing datasets, with 50 additional randomly sampled examples used in defining the few-shot learning prompt or for validation purposes in tuning hyperparameters, thus totaling 1111 observations across these sets. These observations were sourced from a larger dataset consisting of annotations of several different clinical counseling skills. We thus focus on the annotations of counselor reflections. The counselor reflections were annotated at utterance level with counselor verbal behaviors using the Motivational Interviewing Treatment Integrity 4.2 (MITI) and the Motivational Interviewing Skill Code 2.5 (MISC) manuals. Thus, the entire dataset consists of conversational context-counselor reflection pairs.",
"passage: ### Supported Tasks and Leaderboards\n\nThe dataset was used for conditioning and tuning generative models for generating reflection statements in the domain of peer-to-peer counseling.### Languages\n\nThe language in the dataset is English.## Dataset Structure### Data Instances\n\nEach instance consists of the chat room id of the conversation in which the dialogue occurred, the prompt which is the conversational context that immediately precedes the counselor reflection (including previous utterances from either the client or counselor up until and including the most recent prior client message that immediately followed a counselorโs message), and the completion which is the counselor reflection.### Data Fields\n\n* 'chat_id': an integer defining the chat id of the conversation\n* 'prompt': a string corresponding to the conversational context preceding the counselor reflection with the messages separated by new line characters and each utterance prepended by 'Client:' or 'Counselor:'. The string ends with 'Counselor:' to indicate that it is followed by the counselor completion described below.\n* 'completion': a string corresponding to the counselor reflection### Data Splits\n\nThe dataset is split into training, testing, and a small set of 50 examples used either for designing the few-shot learning prompt or tuning hyperparameters. 911 examples were used for training. 350 of these examples also constitute a reduced training set used in comparative experiments. 150 examples were used for testing. 50 of these testing examples (randomly selected) were used in the human evaluation. We ensured that the chat identifiers for messages in the test set uniquely differed from those included in the training set.## Dataset Creation### Curation Rationale\n\nReflective listening is a critical skill in peer-to-peer counseling that is only effective when tailored to the context. Thus, we wanted to home in on this particular skill and explore the potential of state-of-the-art language models for text generation in this domain.### Source Data",
"passage: #### Initial Data Collection and Normalization\n\nThe dataset was created by filtering the larger dataset of utterances annotated for many different counseling skills to only those counselor messages annotated as reflections. Then, the prompt instances were created by identifying the preceding messages for each of these counselor reflection instances. After the prompts were initially created, prompts with less than or equal to five words were removed.\n\nThe author created reference reflections for each of the 350 training example prompts in the reduced training set and each of the 150 testing example prompts. In creating a reference reflection given each conversational context, the author intended to simulate responding to the client in roughly the same time a counselor would as if this turn was embedded in a conversation the client was having with the author. This gauging of time is based on the authorโs experience in volunteering as a counselor at crisis hotlines. It is possible that the reference reflections were created in roughly even less time than an average counselor response given that there were hundreds of conversational contexts for which reflections needed to be created.#### Who are the source language producers?\n\nThe 'client' messages are utterances of those seeking mental health support on a large online counseling service platform. The 'counselor' messages are utterances of minimally-trained peer counselors of this large online counseling service.\n\nFor each of the 350 training example prompts in the reduced training set and each of the 150 testing example prompts, a reference reflection was also created by the author.### Annotations",
"passage: #### Annotation process\n\nThe human evaluation examined text of generative models fine-tuned on the full training set, a reduced training set, and reference reflections; a few-shot learning model; the actual counselor; and the reference reflection.\n\nWe administered a survey through Amazon Mechanical Turk Developer Sandbox. 50 of the testing prompts were provided along with the corresponding six response sources. Provided with the conversational context, the annotators evaluated responses based on three criteria: fluency, resemblance of reflection, and overall preference. Thus, for each context, evaluators measured the fluency, reflection resemblance, and overall preference for all six candidate responses. \n\nWe used a variation of Efficient Annotation of Scalar Labels (EASL), a hybrid approach between direct assessment and online pairwise ranking aggregation and rank-based magnitude estimation. Evaluators saw all six responses at once (without knowledge of each responseโs origin) and used a sliding scale from 1 to 5 to rate the responses based on each of the three dimensions. The order of the model responses for each conversational context was randomized. We provided examples of response ratings for ratings of 1 and 5 on the overall fluency and reflection resemblance dimensions. However, we did not include an example for overall preference, noting its subjectivity. The order of the model responses for each conversational context was randomized. We provided examples of response ratings for ratings of 1 and 5 on the overall fluency and reflection resemblance dimensions. However, we did not include an example for overall preference, noting its subjectivity.\n\nFluency refers to the response's overall fluency and human-likeness. In the instructions, we noted non-capitalized words and colloquial language are acceptable and not to be considered fluency errors. Reflection resemblance refers to whether the response captures and returns to the client something the client has said. Overall preference refers to the extent to which the evaluator likes the response.\n\nUsing Krippendorffโs alpha, we measured inter-annotator agreement, obtaining alpha values of -0.0369, 0.557, and 0.358 for overall fluency, reflection resemblance, and overall preference, respectively. Although these agreement values are low, the 0.557 inter-annotator agreement we obtained for reflection resemblance is notably higher than the inter-annotator agreement obtained for reflection likeness in the most relevant prior work.#### Who are the annotators?\n\nThe three annotators recruited for the human evaluation were familiar with counseling reflections. All three annotators have worked with this large online counseling service dataset with IRB approval. They are quite familiar with motivational interviewing codes, annotating messages and using large language models for mass labeling.### Personal and Sensitive Information\n\nDue to the sensitive nature of this dataset and privacy concerns, we are unable to publicly share the data.## Considerations for Using the Data### Social Impact of Dataset\n\nThis dataset of reflections in peer-to-peer counseling can be used as a reference point in understanding and evaluating counselor clinical skills and furthering the potential of language technology to be applied in this space. Given the sensitive nature of the mental health care context and the minimal training of these counselors, the use of such data requires care in understanding the limitations of technology defined based on this language."
] |
6685505e1e3c02ac0483398e633922b31de89fb0 |
## Dataset Description
A segmentation dataset for anime character
My project: [anime-segmentation](https://github.com/SkyTNT/anime-segmentation)
### Dataset Summary
| Dir | Description | Format | Images |
| ---- | ---- | ---- | ---- |
| bg | background images | jpg | 8057 |
| fg | foreground images, transparent background | png | 11802 |
| imgs | real images with background and foreground| jpg | 1111 |
| masks| labels for imgs | jpg | 1111 |
Total size: 18GB
### Collection Method
Collect background from [character_bg_seg_data](https://github.com/ShuhongChen/bizarre-pose-estimator#download)
Collect foreground from danbooru website.
Collect imgs and masks from [AniSeg](https://github.com/jerryli27/AniSeg#about-the-models) and danbooru website.
I use [Real-ESRGAN](https://github.com/xinntao/Real-ESRGAN) to restore the background images.
I clean the dataset using [DeepDanbooru](https://github.com/KichangKim/DeepDanbooru) first then manually, to make sue all foreground is anime character.
### Contributions
Thanks to [@SkyTNT](https://github.com/SkyTNT) for adding this dataset.
Thanks to [@ShuhongChen](https://github.com/ShuhongChen) for [character_bg_seg_data](https://github.com/ShuhongChen/bizarre-pose-estimator#download)
Thanks to [@jerryli27](https://github.com/jerryli27) for [AniSeg](https://github.com/jerryli27/AniSeg#about-the-models)
| skytnt/anime-segmentation | [
"task_categories:image-segmentation",
"task_ids:semantic-segmentation",
"size_categories:10K<n<100K",
"source_datasets:original",
"license:cc0-1.0",
"region:us"
] | 2022-09-30T04:27:06+00:00 | {"annotations_creators": [], "language_creators": [], "language": [], "license": ["cc0-1.0"], "multilinguality": [], "size_categories": ["10K<n<100K"], "source_datasets": ["original"], "task_categories": ["image-segmentation"], "task_ids": ["semantic-segmentation"], "pretty_name": "Anime Segmentation", "tags": []} | 2022-10-03T00:35:40+00:00 | [] | [] | TAGS
#task_categories-image-segmentation #task_ids-semantic-segmentation #size_categories-10K<n<100K #source_datasets-original #license-cc0-1.0 #region-us
| Dataset Description
-------------------
A segmentation dataset for anime character
My project: anime-segmentation
### Dataset Summary
Total size: 18GB
### Collection Method
Collect background from character\_bg\_seg\_data
Collect foreground from danbooru website.
Collect imgs and masks from AniSeg and danbooru website.
I use Real-ESRGAN to restore the background images.
I clean the dataset using DeepDanbooru first then manually, to make sue all foreground is anime character.
### Contributions
Thanks to @SkyTNT for adding this dataset.
Thanks to @ShuhongChen for character\_bg\_seg\_data
Thanks to @jerryli27 for AniSeg
| [
"### Dataset Summary\n\n\n\nTotal size: 18GB",
"### Collection Method\n\n\nCollect background from character\\_bg\\_seg\\_data\n\n\nCollect foreground from danbooru website.\n\n\nCollect imgs and masks from AniSeg and danbooru website.\n\n\nI use Real-ESRGAN to restore the background images.\n\n\nI clean the dataset using DeepDanbooru first then manually, to make sue all foreground is anime character.",
"### Contributions\n\n\nThanks to @SkyTNT for adding this dataset.\n\n\nThanks to @ShuhongChen for character\\_bg\\_seg\\_data\n\n\nThanks to @jerryli27 for AniSeg"
] | [
"TAGS\n#task_categories-image-segmentation #task_ids-semantic-segmentation #size_categories-10K<n<100K #source_datasets-original #license-cc0-1.0 #region-us \n",
"### Dataset Summary\n\n\n\nTotal size: 18GB",
"### Collection Method\n\n\nCollect background from character\\_bg\\_seg\\_data\n\n\nCollect foreground from danbooru website.\n\n\nCollect imgs and masks from AniSeg and danbooru website.\n\n\nI use Real-ESRGAN to restore the background images.\n\n\nI clean the dataset using DeepDanbooru first then manually, to make sue all foreground is anime character.",
"### Contributions\n\n\nThanks to @SkyTNT for adding this dataset.\n\n\nThanks to @ShuhongChen for character\\_bg\\_seg\\_data\n\n\nThanks to @jerryli27 for AniSeg"
] | [
58,
11,
83,
47
] | [
"passage: TAGS\n#task_categories-image-segmentation #task_ids-semantic-segmentation #size_categories-10K<n<100K #source_datasets-original #license-cc0-1.0 #region-us \n### Dataset Summary\n\n\n\nTotal size: 18GB### Collection Method\n\n\nCollect background from character\\_bg\\_seg\\_data\n\n\nCollect foreground from danbooru website.\n\n\nCollect imgs and masks from AniSeg and danbooru website.\n\n\nI use Real-ESRGAN to restore the background images.\n\n\nI clean the dataset using DeepDanbooru first then manually, to make sue all foreground is anime character.### Contributions\n\n\nThanks to @SkyTNT for adding this dataset.\n\n\nThanks to @ShuhongChen for character\\_bg\\_seg\\_data\n\n\nThanks to @jerryli27 for AniSeg"
] |
2fc722a09b37bee7ea8bbf850f59f004c7bb5c15 |
All eight of datasets in ESC can be downloaded and prepared in just a single line of code through the Hugging Face Datasets library:
```python
from datasets import load_dataset
librispeech = load_dataset("esc-benchmark/esc-datasets", "librispeech", split="train")
```
- `"esc-benchmark"`: the repository namespace. This is fixed for all ESC datasets.
- `"librispeech"`: the dataset name. This can be changed to any of any one of the eight datasets in ESC to download that dataset.
- `split="train"`: the split. Set this to one of train/validation/test to generate a specific split. Omit the `split` argument to generate all splits for a dataset.
The datasets are full prepared, such that the audio and transcription files can be used directly in training/evaluation scripts.
## Dataset Information
A data point can be accessed by indexing the dataset object loaded through `load_dataset`:
```python
print(librispeech[0])
```
A typical data point comprises the path to the audio file and its transcription. Also included is information of the dataset from which the sample derives and a unique identifier name:
```python
{
'dataset': 'librispeech',
'audio': {'path': '/home/esc-bencher/.cache/huggingface/datasets/downloads/extracted/d2da1969fe9e7d06661b5dc370cf2e3c119a14c35950045bcb76243b264e4f01/374-180298-0000.flac',
'array': array([ 7.01904297e-04, 7.32421875e-04, 7.32421875e-04, ...,
-2.74658203e-04, -1.83105469e-04, -3.05175781e-05]),
'sampling_rate': 16000},
'text': 'chapter sixteen i might have told you of the beginning of this liaison in a few lines but i wanted you to see every step by which we came i to agree to whatever marguerite wished',
'id': '374-180298-0000'
}
```
### Data Fields
- `dataset`: name of the ESC dataset from which the sample is taken.
- `audio`: a dictionary containing the path to the downloaded audio file, the decoded audio array, and the sampling rate.
- `text`: the transcription of the audio file.
- `id`: unique id of the data sample.
### Data Preparation
#### Audio
The audio for all ESC datasets is segmented into sample lengths suitable for training ASR systems. The Hugging Face datasets library decodes audio files on the fly, reading the segments and converting them to a Python arrays. Consequently, no further preparation of the audio is required to be used in training/evaluation scripts.
Note that when accessing the audio column: `dataset[0]["audio"]` the audio file is automatically decoded and resampled to `dataset.features["audio"].sampling_rate`. Decoding and resampling of a large number of audio files might take a significant amount of time. Thus it is important to first query the sample index before the `"audio"` column, i.e. `dataset[0]["audio"]` should always be preferred over `dataset["audio"][0]`.
#### Transcriptions
The transcriptions corresponding to each audio file are provided in their 'error corrected' format. No transcription pre-processing is applied to the text, only necessary 'error correction' steps such as removing junk tokens (_<unk>_) or converting symbolic punctuation to spelled out form (_<comma>_ to _,_). As such, no further preparation of the transcriptions is required to be used in training/evaluation scripts.
Transcriptions are provided for training and validation splits. The transcriptions are **not** provided for the test splits. The ESC benchmark requires you to generate predictions for the test sets and upload them to https://huggingface.co/spaces/esc-benchmark/esc for scoring.
### Access
All eight of the datasets in ESC are accessible and licensing is freely available. Three of the ESC datasets have specific terms of usage that must be agreed to before using the data. To do so, fill in the access forms on the specific datasets' pages:
* Common Voice: https://huggingface.co/datasets/mozilla-foundation/common_voice_9_0
* GigaSpeech: https://huggingface.co/datasets/speechcolab/gigaspeech
* SPGISpeech: https://huggingface.co/datasets/kensho/spgispeech
### Diagnostic Dataset
ESC contains a small, 8h diagnostic dataset of in-domain validation data with newly annotated transcriptions. The audio data is sampled from each of the ESC validation sets, giving a range of different domains and speaking styles. The transcriptions are annotated according to a consistent style guide with two formats: normalised and un-normalised. The dataset is structured in the same way as the ESC dataset, by grouping audio-transcription samples according to the dataset from which they were taken. We encourage participants to use this dataset when evaluating their systems to quickly assess performance on a range of different speech recognition conditions. For more information, visit: [esc-bench/esc-diagnostic-dataset](https://huggingface.co/datasets/esc-bench/esc-diagnostic-datasets).
## LibriSpeech
The LibriSpeech corpus is a standard large-scale corpus for assessing ASR systems. It consists of approximately 1,000 hours of narrated audiobooks from the [LibriVox](https://librivox.org) project. It is licensed under CC-BY-4.0.
Example Usage:
```python
librispeech = load_dataset("esc-benchmark/esc-datasets", "librispeech")
```
Train/validation splits:
- `train` (combination of `train.clean.100`, `train.clean.360` and `train.other.500`)
- `validation.clean`
- `validation.other`
Test splits:
- `test.clean`
- `test.other`
Also available are subsets of the train split, which can be accessed by setting the `subconfig` argument:
```python
librispeech = load_dataset("esc-benchmark/esc-datasets", "librispeech", subconfig="clean.100")
```
- `clean.100`: 100 hours of training data from the 'clean' subset
- `clean.360`: 360 hours of training data from the 'clean' subset
- `other.500`: 500 hours of training data from the 'other' subset
## Common Voice
Common Voice is a series of crowd-sourced open-licensed speech datasets where speakers record text from Wikipedia in various languages. The English subset of contains approximately 1,400 hours of audio data from speakers of various nationalities, accents and different recording conditions. It is licensed under CC0-1.0.
Example usage:
```python
common_voice = load_dataset("esc-benchmark/esc-datasets", "common_voice", use_auth_token=True)
```
Training/validation splits:
- `train`
- `validation`
Test splits:
- `test`
## VoxPopuli
VoxPopuli s a large-scale multilingual speech corpus consisting of political data sourced from 2009-2020 European Parliament event recordings. The English subset contains approximately 550 hours of speech largely from non-native English speakers. It is licensed under CC0.
Example usage:
```python
voxpopuli = load_dataset("esc-benchmark/esc-datasets", "voxpopuli")
```
Training/validation splits:
- `train`
- `validation`
Test splits:
- `test`
## TED-LIUM
TED-LIUM consists of English-language TED Talk conference videos covering a range of different cultural, political, and academic topics. It contains approximately 450 hours of transcribed speech data. It is licensed under CC-BY-NC-ND 3.0.
Example usage:
```python
tedlium = load_dataset("esc-benchmark/esc-datasets", "tedlium")
```
Training/validation splits:
- `train`
- `validation`
Test splits:
- `test`
## GigaSpeech
GigaSpeech is a multi-domain English speech recognition corpus created from audiobooks, podcasts and YouTube. We provide the large train set (2,500 hours) and the standard validation and test splits. It is licensed under apache-2.0.
Example usage:
```python
gigaspeech = load_dataset("esc-benchmark/esc-datasets", "gigaspeech", use_auth_token=True)
```
Training/validation splits:
- `train` (`l` subset of training data (2,500 h))
- `validation`
Test splits:
- `test`
Also available are subsets of the train split, which can be accessed by setting the `subconfig` argument:
```python
gigaspeech = load_dataset("esc-benchmark/esc-datasets", "spgispeech", subconfig="xs", use_auth_token=True)
```
- `xs`: extra-small subset of training data (10 h)
- `s`: small subset of training data (250 h)
- `m`: medium subset of training data (1,000 h)
- `xl`: extra-large subset of training data (10,000 h)
## SPGISpeech
SPGISpeech consists of company earnings calls that have been manually transcribed by S&P Global, Inc according to a professional style guide. We provide the large train set (5,000 hours) and the standard validation and test splits. It is licensed under a Kensho user agreement.
Loading the dataset requires authorization.
Example usage:
```python
spgispeech = load_dataset("esc-benchmark/esc-datasets", "spgispeech", use_auth_token=True)
```
Training/validation splits:
- `train` (`l` subset of training data (~5,000 h))
- `validation`
Test splits:
- `test`
Also available are subsets of the train split, which can be accessed by setting the `subconfig` argument:
```python
spgispeech = load_dataset("esc-benchmark/esc-datasets", "spgispeech", subconfig="s", use_auth_token=True)
```
- `s`: small subset of training data (~200 h)
- `m`: medium subset of training data (~1,000 h)
## Earnings-22
Earnings-22 is a 119-hour corpus of English-language earnings calls collected from global companies, with speakers of many different nationalities and accents. It is licensed under CC-BY-SA-4.0.
Example usage:
```python
earnings22 = load_dataset("esc-benchmark/esc-datasets", "earnings22")
```
Training/validation splits:
- `train`
- `validation`
Test splits:
- `test`
## AMI
The AMI Meeting Corpus consists of 100 hours of meeting recordings from multiple recording devices synced to a common timeline. It is licensed under CC-BY-4.0.
Example usage:
```python
ami = load_dataset("esc-benchmark/esc-datasets", "ami")
```
Training/validation splits:
- `train`
- `validation`
Test splits:
- `test` | esc-bench/esc-datasets | [
"task_categories:automatic-speech-recognition",
"annotations_creators:expert-generated",
"annotations_creators:crowdsourced",
"annotations_creators:machine-generated",
"language_creators:crowdsourced",
"language_creators:expert-generated",
"multilinguality:monolingual",
"size_categories:100K<n<1M",
"size_categories:1M<n<10M",
"source_datasets:original",
"source_datasets:extended|librispeech_asr",
"source_datasets:extended|common_voice",
"language:en",
"license:cc-by-4.0",
"license:apache-2.0",
"license:cc0-1.0",
"license:cc-by-nc-3.0",
"license:other",
"asr",
"benchmark",
"speech",
"esc",
"region:us"
] | 2022-09-30T07:32:42+00:00 | {"annotations_creators": ["expert-generated", "crowdsourced", "machine-generated"], "language_creators": ["crowdsourced", "expert-generated"], "language": ["en"], "license": ["cc-by-4.0", "apache-2.0", "cc0-1.0", "cc-by-nc-3.0", "other"], "multilinguality": ["monolingual"], "size_categories": ["100K<n<1M", "1M<n<10M"], "source_datasets": ["original", "extended|librispeech_asr", "extended|common_voice"], "task_categories": ["automatic-speech-recognition"], "pretty_name": "esc-datasets", "tags": ["asr", "benchmark", "speech", "esc"], "extra_gated_prompt": "Three of the ESC datasets have specific terms of usage that must be agreed to before using the data. \nTo do so, fill in the access forms on the specific datasets' pages:\n * Common Voice: https://huggingface.co/datasets/mozilla-foundation/common_voice_9_0\n * GigaSpeech: https://huggingface.co/datasets/speechcolab/gigaspeech\n * SPGISpeech: https://huggingface.co/datasets/kensho/spgispeech", "extra_gated_fields": {"I hereby confirm that I have registered on the original Common Voice page and agree to not attempt to determine the identity of speakers in the Common Voice dataset": "checkbox", "I hereby confirm that I have accepted the terms of usages on GigaSpeech page": "checkbox", "I hereby confirm that I have accepted the terms of usages on SPGISpeech page": "checkbox"}} | 2022-10-21T13:34:49+00:00 | [] | [
"en"
] | TAGS
#task_categories-automatic-speech-recognition #annotations_creators-expert-generated #annotations_creators-crowdsourced #annotations_creators-machine-generated #language_creators-crowdsourced #language_creators-expert-generated #multilinguality-monolingual #size_categories-100K<n<1M #size_categories-1M<n<10M #source_datasets-original #source_datasets-extended|librispeech_asr #source_datasets-extended|common_voice #language-English #license-cc-by-4.0 #license-apache-2.0 #license-cc0-1.0 #license-cc-by-nc-3.0 #license-other #asr #benchmark #speech #esc #region-us
|
All eight of datasets in ESC can be downloaded and prepared in just a single line of code through the Hugging Face Datasets library:
- '"esc-benchmark"': the repository namespace. This is fixed for all ESC datasets.
- '"librispeech"': the dataset name. This can be changed to any of any one of the eight datasets in ESC to download that dataset.
- 'split="train"': the split. Set this to one of train/validation/test to generate a specific split. Omit the 'split' argument to generate all splits for a dataset.
The datasets are full prepared, such that the audio and transcription files can be used directly in training/evaluation scripts.
## Dataset Information
A data point can be accessed by indexing the dataset object loaded through 'load_dataset':
A typical data point comprises the path to the audio file and its transcription. Also included is information of the dataset from which the sample derives and a unique identifier name:
### Data Fields
- 'dataset': name of the ESC dataset from which the sample is taken.
- 'audio': a dictionary containing the path to the downloaded audio file, the decoded audio array, and the sampling rate.
- 'text': the transcription of the audio file.
- 'id': unique id of the data sample.
### Data Preparation
#### Audio
The audio for all ESC datasets is segmented into sample lengths suitable for training ASR systems. The Hugging Face datasets library decodes audio files on the fly, reading the segments and converting them to a Python arrays. Consequently, no further preparation of the audio is required to be used in training/evaluation scripts.
Note that when accessing the audio column: 'dataset[0]["audio"]' the audio file is automatically decoded and resampled to 'dataset.features["audio"].sampling_rate'. Decoding and resampling of a large number of audio files might take a significant amount of time. Thus it is important to first query the sample index before the '"audio"' column, i.e. 'dataset[0]["audio"]' should always be preferred over 'dataset["audio"][0]'.
#### Transcriptions
The transcriptions corresponding to each audio file are provided in their 'error corrected' format. No transcription pre-processing is applied to the text, only necessary 'error correction' steps such as removing junk tokens (_<unk>_) or converting symbolic punctuation to spelled out form (_<comma>_ to _,_). As such, no further preparation of the transcriptions is required to be used in training/evaluation scripts.
Transcriptions are provided for training and validation splits. The transcriptions are not provided for the test splits. The ESC benchmark requires you to generate predictions for the test sets and upload them to URL for scoring.
### Access
All eight of the datasets in ESC are accessible and licensing is freely available. Three of the ESC datasets have specific terms of usage that must be agreed to before using the data. To do so, fill in the access forms on the specific datasets' pages:
* Common Voice: URL
* GigaSpeech: URL
* SPGISpeech: URL
### Diagnostic Dataset
ESC contains a small, 8h diagnostic dataset of in-domain validation data with newly annotated transcriptions. The audio data is sampled from each of the ESC validation sets, giving a range of different domains and speaking styles. The transcriptions are annotated according to a consistent style guide with two formats: normalised and un-normalised. The dataset is structured in the same way as the ESC dataset, by grouping audio-transcription samples according to the dataset from which they were taken. We encourage participants to use this dataset when evaluating their systems to quickly assess performance on a range of different speech recognition conditions. For more information, visit: esc-bench/esc-diagnostic-dataset.
## LibriSpeech
The LibriSpeech corpus is a standard large-scale corpus for assessing ASR systems. It consists of approximately 1,000 hours of narrated audiobooks from the LibriVox project. It is licensed under CC-BY-4.0.
Example Usage:
Train/validation splits:
- 'train' (combination of 'URL.100', 'URL.360' and 'URL.500')
- 'URL'
- 'URL'
Test splits:
- 'URL'
- 'URL'
Also available are subsets of the train split, which can be accessed by setting the 'subconfig' argument:
- 'clean.100': 100 hours of training data from the 'clean' subset
- 'clean.360': 360 hours of training data from the 'clean' subset
- 'other.500': 500 hours of training data from the 'other' subset
## Common Voice
Common Voice is a series of crowd-sourced open-licensed speech datasets where speakers record text from Wikipedia in various languages. The English subset of contains approximately 1,400 hours of audio data from speakers of various nationalities, accents and different recording conditions. It is licensed under CC0-1.0.
Example usage:
Training/validation splits:
- 'train'
- 'validation'
Test splits:
- 'test'
## VoxPopuli
VoxPopuli s a large-scale multilingual speech corpus consisting of political data sourced from 2009-2020 European Parliament event recordings. The English subset contains approximately 550 hours of speech largely from non-native English speakers. It is licensed under CC0.
Example usage:
Training/validation splits:
- 'train'
- 'validation'
Test splits:
- 'test'
## TED-LIUM
TED-LIUM consists of English-language TED Talk conference videos covering a range of different cultural, political, and academic topics. It contains approximately 450 hours of transcribed speech data. It is licensed under CC-BY-NC-ND 3.0.
Example usage:
Training/validation splits:
- 'train'
- 'validation'
Test splits:
- 'test'
## GigaSpeech
GigaSpeech is a multi-domain English speech recognition corpus created from audiobooks, podcasts and YouTube. We provide the large train set (2,500 hours) and the standard validation and test splits. It is licensed under apache-2.0.
Example usage:
Training/validation splits:
- 'train' ('l' subset of training data (2,500 h))
- 'validation'
Test splits:
- 'test'
Also available are subsets of the train split, which can be accessed by setting the 'subconfig' argument:
- 'xs': extra-small subset of training data (10 h)
- 's': small subset of training data (250 h)
- 'm': medium subset of training data (1,000 h)
- 'xl': extra-large subset of training data (10,000 h)
## SPGISpeech
SPGISpeech consists of company earnings calls that have been manually transcribed by S&P Global, Inc according to a professional style guide. We provide the large train set (5,000 hours) and the standard validation and test splits. It is licensed under a Kensho user agreement.
Loading the dataset requires authorization.
Example usage:
Training/validation splits:
- 'train' ('l' subset of training data (~5,000 h))
- 'validation'
Test splits:
- 'test'
Also available are subsets of the train split, which can be accessed by setting the 'subconfig' argument:
- 's': small subset of training data (~200 h)
- 'm': medium subset of training data (~1,000 h)
## Earnings-22
Earnings-22 is a 119-hour corpus of English-language earnings calls collected from global companies, with speakers of many different nationalities and accents. It is licensed under CC-BY-SA-4.0.
Example usage:
Training/validation splits:
- 'train'
- 'validation'
Test splits:
- 'test'
## AMI
The AMI Meeting Corpus consists of 100 hours of meeting recordings from multiple recording devices synced to a common timeline. It is licensed under CC-BY-4.0.
Example usage:
Training/validation splits:
- 'train'
- 'validation'
Test splits:
- 'test' | [
"## Dataset Information\n\nA data point can be accessed by indexing the dataset object loaded through 'load_dataset':\n\n\n\nA typical data point comprises the path to the audio file and its transcription. Also included is information of the dataset from which the sample derives and a unique identifier name:",
"### Data Fields\n\n- 'dataset': name of the ESC dataset from which the sample is taken.\n\n- 'audio': a dictionary containing the path to the downloaded audio file, the decoded audio array, and the sampling rate.\n\n- 'text': the transcription of the audio file.\n\n- 'id': unique id of the data sample.",
"### Data Preparation",
"#### Audio\nThe audio for all ESC datasets is segmented into sample lengths suitable for training ASR systems. The Hugging Face datasets library decodes audio files on the fly, reading the segments and converting them to a Python arrays. Consequently, no further preparation of the audio is required to be used in training/evaluation scripts.\n\nNote that when accessing the audio column: 'dataset[0][\"audio\"]' the audio file is automatically decoded and resampled to 'dataset.features[\"audio\"].sampling_rate'. Decoding and resampling of a large number of audio files might take a significant amount of time. Thus it is important to first query the sample index before the '\"audio\"' column, i.e. 'dataset[0][\"audio\"]' should always be preferred over 'dataset[\"audio\"][0]'.",
"#### Transcriptions\nThe transcriptions corresponding to each audio file are provided in their 'error corrected' format. No transcription pre-processing is applied to the text, only necessary 'error correction' steps such as removing junk tokens (_<unk>_) or converting symbolic punctuation to spelled out form (_<comma>_ to _,_). As such, no further preparation of the transcriptions is required to be used in training/evaluation scripts.\n\nTranscriptions are provided for training and validation splits. The transcriptions are not provided for the test splits. The ESC benchmark requires you to generate predictions for the test sets and upload them to URL for scoring.",
"### Access\nAll eight of the datasets in ESC are accessible and licensing is freely available. Three of the ESC datasets have specific terms of usage that must be agreed to before using the data. To do so, fill in the access forms on the specific datasets' pages:\n* Common Voice: URL\n* GigaSpeech: URL\n* SPGISpeech: URL",
"### Diagnostic Dataset\nESC contains a small, 8h diagnostic dataset of in-domain validation data with newly annotated transcriptions. The audio data is sampled from each of the ESC validation sets, giving a range of different domains and speaking styles. The transcriptions are annotated according to a consistent style guide with two formats: normalised and un-normalised. The dataset is structured in the same way as the ESC dataset, by grouping audio-transcription samples according to the dataset from which they were taken. We encourage participants to use this dataset when evaluating their systems to quickly assess performance on a range of different speech recognition conditions. For more information, visit: esc-bench/esc-diagnostic-dataset.",
"## LibriSpeech\n\nThe LibriSpeech corpus is a standard large-scale corpus for assessing ASR systems. It consists of approximately 1,000 hours of narrated audiobooks from the LibriVox project. It is licensed under CC-BY-4.0.\n\nExample Usage:\n\n\n\nTrain/validation splits:\n- 'train' (combination of 'URL.100', 'URL.360' and 'URL.500')\n- 'URL'\n- 'URL'\n\nTest splits:\n- 'URL'\n- 'URL'\n\nAlso available are subsets of the train split, which can be accessed by setting the 'subconfig' argument:\n\n\n- 'clean.100': 100 hours of training data from the 'clean' subset\n- 'clean.360': 360 hours of training data from the 'clean' subset\n- 'other.500': 500 hours of training data from the 'other' subset",
"## Common Voice\nCommon Voice is a series of crowd-sourced open-licensed speech datasets where speakers record text from Wikipedia in various languages. The English subset of contains approximately 1,400 hours of audio data from speakers of various nationalities, accents and different recording conditions. It is licensed under CC0-1.0.\n\nExample usage:\n\n\n\nTraining/validation splits:\n- 'train'\n- 'validation'\n\nTest splits:\n- 'test'",
"## VoxPopuli\nVoxPopuli s a large-scale multilingual speech corpus consisting of political data sourced from 2009-2020 European Parliament event recordings. The English subset contains approximately 550 hours of speech largely from non-native English speakers. It is licensed under CC0.\n\nExample usage:\n\n\n\nTraining/validation splits:\n- 'train'\n- 'validation'\n\nTest splits:\n- 'test'",
"## TED-LIUM\nTED-LIUM consists of English-language TED Talk conference videos covering a range of different cultural, political, and academic topics. It contains approximately 450 hours of transcribed speech data. It is licensed under CC-BY-NC-ND 3.0.\n\nExample usage:\n\n\n\nTraining/validation splits:\n- 'train'\n- 'validation'\n\nTest splits:\n- 'test'",
"## GigaSpeech\nGigaSpeech is a multi-domain English speech recognition corpus created from audiobooks, podcasts and YouTube. We provide the large train set (2,500 hours) and the standard validation and test splits. It is licensed under apache-2.0.\n\nExample usage:\n\n\n\nTraining/validation splits:\n- 'train' ('l' subset of training data (2,500 h))\n- 'validation'\n\nTest splits:\n- 'test'\n\nAlso available are subsets of the train split, which can be accessed by setting the 'subconfig' argument:\n\n- 'xs': extra-small subset of training data (10 h)\n- 's': small subset of training data (250 h)\n- 'm': medium subset of training data (1,000 h)\n- 'xl': extra-large subset of training data (10,000 h)",
"## SPGISpeech\nSPGISpeech consists of company earnings calls that have been manually transcribed by S&P Global, Inc according to a professional style guide. We provide the large train set (5,000 hours) and the standard validation and test splits. It is licensed under a Kensho user agreement.\n\nLoading the dataset requires authorization.\n\nExample usage:\n\n\n\nTraining/validation splits:\n- 'train' ('l' subset of training data (~5,000 h))\n- 'validation'\n\nTest splits:\n- 'test'\n\nAlso available are subsets of the train split, which can be accessed by setting the 'subconfig' argument:\n\n- 's': small subset of training data (~200 h)\n- 'm': medium subset of training data (~1,000 h)",
"## Earnings-22\nEarnings-22 is a 119-hour corpus of English-language earnings calls collected from global companies, with speakers of many different nationalities and accents. It is licensed under CC-BY-SA-4.0. \n\nExample usage:\n\n\n\nTraining/validation splits:\n- 'train'\n- 'validation'\n\nTest splits:\n- 'test'",
"## AMI\nThe AMI Meeting Corpus consists of 100 hours of meeting recordings from multiple recording devices synced to a common timeline. It is licensed under CC-BY-4.0.\n\nExample usage:\n\n\n\nTraining/validation splits:\n- 'train'\n- 'validation'\n\nTest splits:\n- 'test'"
] | [
"TAGS\n#task_categories-automatic-speech-recognition #annotations_creators-expert-generated #annotations_creators-crowdsourced #annotations_creators-machine-generated #language_creators-crowdsourced #language_creators-expert-generated #multilinguality-monolingual #size_categories-100K<n<1M #size_categories-1M<n<10M #source_datasets-original #source_datasets-extended|librispeech_asr #source_datasets-extended|common_voice #language-English #license-cc-by-4.0 #license-apache-2.0 #license-cc0-1.0 #license-cc-by-nc-3.0 #license-other #asr #benchmark #speech #esc #region-us \n",
"## Dataset Information\n\nA data point can be accessed by indexing the dataset object loaded through 'load_dataset':\n\n\n\nA typical data point comprises the path to the audio file and its transcription. Also included is information of the dataset from which the sample derives and a unique identifier name:",
"### Data Fields\n\n- 'dataset': name of the ESC dataset from which the sample is taken.\n\n- 'audio': a dictionary containing the path to the downloaded audio file, the decoded audio array, and the sampling rate.\n\n- 'text': the transcription of the audio file.\n\n- 'id': unique id of the data sample.",
"### Data Preparation",
"#### Audio\nThe audio for all ESC datasets is segmented into sample lengths suitable for training ASR systems. The Hugging Face datasets library decodes audio files on the fly, reading the segments and converting them to a Python arrays. Consequently, no further preparation of the audio is required to be used in training/evaluation scripts.\n\nNote that when accessing the audio column: 'dataset[0][\"audio\"]' the audio file is automatically decoded and resampled to 'dataset.features[\"audio\"].sampling_rate'. Decoding and resampling of a large number of audio files might take a significant amount of time. Thus it is important to first query the sample index before the '\"audio\"' column, i.e. 'dataset[0][\"audio\"]' should always be preferred over 'dataset[\"audio\"][0]'.",
"#### Transcriptions\nThe transcriptions corresponding to each audio file are provided in their 'error corrected' format. No transcription pre-processing is applied to the text, only necessary 'error correction' steps such as removing junk tokens (_<unk>_) or converting symbolic punctuation to spelled out form (_<comma>_ to _,_). As such, no further preparation of the transcriptions is required to be used in training/evaluation scripts.\n\nTranscriptions are provided for training and validation splits. The transcriptions are not provided for the test splits. The ESC benchmark requires you to generate predictions for the test sets and upload them to URL for scoring.",
"### Access\nAll eight of the datasets in ESC are accessible and licensing is freely available. Three of the ESC datasets have specific terms of usage that must be agreed to before using the data. To do so, fill in the access forms on the specific datasets' pages:\n* Common Voice: URL\n* GigaSpeech: URL\n* SPGISpeech: URL",
"### Diagnostic Dataset\nESC contains a small, 8h diagnostic dataset of in-domain validation data with newly annotated transcriptions. The audio data is sampled from each of the ESC validation sets, giving a range of different domains and speaking styles. The transcriptions are annotated according to a consistent style guide with two formats: normalised and un-normalised. The dataset is structured in the same way as the ESC dataset, by grouping audio-transcription samples according to the dataset from which they were taken. We encourage participants to use this dataset when evaluating their systems to quickly assess performance on a range of different speech recognition conditions. For more information, visit: esc-bench/esc-diagnostic-dataset.",
"## LibriSpeech\n\nThe LibriSpeech corpus is a standard large-scale corpus for assessing ASR systems. It consists of approximately 1,000 hours of narrated audiobooks from the LibriVox project. It is licensed under CC-BY-4.0.\n\nExample Usage:\n\n\n\nTrain/validation splits:\n- 'train' (combination of 'URL.100', 'URL.360' and 'URL.500')\n- 'URL'\n- 'URL'\n\nTest splits:\n- 'URL'\n- 'URL'\n\nAlso available are subsets of the train split, which can be accessed by setting the 'subconfig' argument:\n\n\n- 'clean.100': 100 hours of training data from the 'clean' subset\n- 'clean.360': 360 hours of training data from the 'clean' subset\n- 'other.500': 500 hours of training data from the 'other' subset",
"## Common Voice\nCommon Voice is a series of crowd-sourced open-licensed speech datasets where speakers record text from Wikipedia in various languages. The English subset of contains approximately 1,400 hours of audio data from speakers of various nationalities, accents and different recording conditions. It is licensed under CC0-1.0.\n\nExample usage:\n\n\n\nTraining/validation splits:\n- 'train'\n- 'validation'\n\nTest splits:\n- 'test'",
"## VoxPopuli\nVoxPopuli s a large-scale multilingual speech corpus consisting of political data sourced from 2009-2020 European Parliament event recordings. The English subset contains approximately 550 hours of speech largely from non-native English speakers. It is licensed under CC0.\n\nExample usage:\n\n\n\nTraining/validation splits:\n- 'train'\n- 'validation'\n\nTest splits:\n- 'test'",
"## TED-LIUM\nTED-LIUM consists of English-language TED Talk conference videos covering a range of different cultural, political, and academic topics. It contains approximately 450 hours of transcribed speech data. It is licensed under CC-BY-NC-ND 3.0.\n\nExample usage:\n\n\n\nTraining/validation splits:\n- 'train'\n- 'validation'\n\nTest splits:\n- 'test'",
"## GigaSpeech\nGigaSpeech is a multi-domain English speech recognition corpus created from audiobooks, podcasts and YouTube. We provide the large train set (2,500 hours) and the standard validation and test splits. It is licensed under apache-2.0.\n\nExample usage:\n\n\n\nTraining/validation splits:\n- 'train' ('l' subset of training data (2,500 h))\n- 'validation'\n\nTest splits:\n- 'test'\n\nAlso available are subsets of the train split, which can be accessed by setting the 'subconfig' argument:\n\n- 'xs': extra-small subset of training data (10 h)\n- 's': small subset of training data (250 h)\n- 'm': medium subset of training data (1,000 h)\n- 'xl': extra-large subset of training data (10,000 h)",
"## SPGISpeech\nSPGISpeech consists of company earnings calls that have been manually transcribed by S&P Global, Inc according to a professional style guide. We provide the large train set (5,000 hours) and the standard validation and test splits. It is licensed under a Kensho user agreement.\n\nLoading the dataset requires authorization.\n\nExample usage:\n\n\n\nTraining/validation splits:\n- 'train' ('l' subset of training data (~5,000 h))\n- 'validation'\n\nTest splits:\n- 'test'\n\nAlso available are subsets of the train split, which can be accessed by setting the 'subconfig' argument:\n\n- 's': small subset of training data (~200 h)\n- 'm': medium subset of training data (~1,000 h)",
"## Earnings-22\nEarnings-22 is a 119-hour corpus of English-language earnings calls collected from global companies, with speakers of many different nationalities and accents. It is licensed under CC-BY-SA-4.0. \n\nExample usage:\n\n\n\nTraining/validation splits:\n- 'train'\n- 'validation'\n\nTest splits:\n- 'test'",
"## AMI\nThe AMI Meeting Corpus consists of 100 hours of meeting recordings from multiple recording devices synced to a common timeline. It is licensed under CC-BY-4.0.\n\nExample usage:\n\n\n\nTraining/validation splits:\n- 'train'\n- 'validation'\n\nTest splits:\n- 'test'"
] | [
213,
67,
85,
5,
219,
164,
84,
173,
203,
105,
97,
95,
199,
181,
86,
71
] | [
"passage: TAGS\n#task_categories-automatic-speech-recognition #annotations_creators-expert-generated #annotations_creators-crowdsourced #annotations_creators-machine-generated #language_creators-crowdsourced #language_creators-expert-generated #multilinguality-monolingual #size_categories-100K<n<1M #size_categories-1M<n<10M #source_datasets-original #source_datasets-extended|librispeech_asr #source_datasets-extended|common_voice #language-English #license-cc-by-4.0 #license-apache-2.0 #license-cc0-1.0 #license-cc-by-nc-3.0 #license-other #asr #benchmark #speech #esc #region-us \n## Dataset Information\n\nA data point can be accessed by indexing the dataset object loaded through 'load_dataset':\n\n\n\nA typical data point comprises the path to the audio file and its transcription. Also included is information of the dataset from which the sample derives and a unique identifier name:### Data Fields\n\n- 'dataset': name of the ESC dataset from which the sample is taken.\n\n- 'audio': a dictionary containing the path to the downloaded audio file, the decoded audio array, and the sampling rate.\n\n- 'text': the transcription of the audio file.\n\n- 'id': unique id of the data sample.### Data Preparation",
"passage: #### Audio\nThe audio for all ESC datasets is segmented into sample lengths suitable for training ASR systems. The Hugging Face datasets library decodes audio files on the fly, reading the segments and converting them to a Python arrays. Consequently, no further preparation of the audio is required to be used in training/evaluation scripts.\n\nNote that when accessing the audio column: 'dataset[0][\"audio\"]' the audio file is automatically decoded and resampled to 'dataset.features[\"audio\"].sampling_rate'. Decoding and resampling of a large number of audio files might take a significant amount of time. Thus it is important to first query the sample index before the '\"audio\"' column, i.e. 'dataset[0][\"audio\"]' should always be preferred over 'dataset[\"audio\"][0]'.#### Transcriptions\nThe transcriptions corresponding to each audio file are provided in their 'error corrected' format. No transcription pre-processing is applied to the text, only necessary 'error correction' steps such as removing junk tokens (_<unk>_) or converting symbolic punctuation to spelled out form (_<comma>_ to _,_). As such, no further preparation of the transcriptions is required to be used in training/evaluation scripts.\n\nTranscriptions are provided for training and validation splits. The transcriptions are not provided for the test splits. The ESC benchmark requires you to generate predictions for the test sets and upload them to URL for scoring.### Access\nAll eight of the datasets in ESC are accessible and licensing is freely available. Three of the ESC datasets have specific terms of usage that must be agreed to before using the data. To do so, fill in the access forms on the specific datasets' pages:\n* Common Voice: URL\n* GigaSpeech: URL\n* SPGISpeech: URL### Diagnostic Dataset\nESC contains a small, 8h diagnostic dataset of in-domain validation data with newly annotated transcriptions. The audio data is sampled from each of the ESC validation sets, giving a range of different domains and speaking styles. The transcriptions are annotated according to a consistent style guide with two formats: normalised and un-normalised. The dataset is structured in the same way as the ESC dataset, by grouping audio-transcription samples according to the dataset from which they were taken. We encourage participants to use this dataset when evaluating their systems to quickly assess performance on a range of different speech recognition conditions. For more information, visit: esc-bench/esc-diagnostic-dataset.",
"passage: ## LibriSpeech\n\nThe LibriSpeech corpus is a standard large-scale corpus for assessing ASR systems. It consists of approximately 1,000 hours of narrated audiobooks from the LibriVox project. It is licensed under CC-BY-4.0.\n\nExample Usage:\n\n\n\nTrain/validation splits:\n- 'train' (combination of 'URL.100', 'URL.360' and 'URL.500')\n- 'URL'\n- 'URL'\n\nTest splits:\n- 'URL'\n- 'URL'\n\nAlso available are subsets of the train split, which can be accessed by setting the 'subconfig' argument:\n\n\n- 'clean.100': 100 hours of training data from the 'clean' subset\n- 'clean.360': 360 hours of training data from the 'clean' subset\n- 'other.500': 500 hours of training data from the 'other' subset## Common Voice\nCommon Voice is a series of crowd-sourced open-licensed speech datasets where speakers record text from Wikipedia in various languages. The English subset of contains approximately 1,400 hours of audio data from speakers of various nationalities, accents and different recording conditions. It is licensed under CC0-1.0.\n\nExample usage:\n\n\n\nTraining/validation splits:\n- 'train'\n- 'validation'\n\nTest splits:\n- 'test'## VoxPopuli\nVoxPopuli s a large-scale multilingual speech corpus consisting of political data sourced from 2009-2020 European Parliament event recordings. The English subset contains approximately 550 hours of speech largely from non-native English speakers. It is licensed under CC0.\n\nExample usage:\n\n\n\nTraining/validation splits:\n- 'train'\n- 'validation'\n\nTest splits:\n- 'test'## TED-LIUM\nTED-LIUM consists of English-language TED Talk conference videos covering a range of different cultural, political, and academic topics. It contains approximately 450 hours of transcribed speech data. It is licensed under CC-BY-NC-ND 3.0.\n\nExample usage:\n\n\n\nTraining/validation splits:\n- 'train'\n- 'validation'\n\nTest splits:\n- 'test'## GigaSpeech\nGigaSpeech is a multi-domain English speech recognition corpus created from audiobooks, podcasts and YouTube. We provide the large train set (2,500 hours) and the standard validation and test splits. It is licensed under apache-2.0.\n\nExample usage:\n\n\n\nTraining/validation splits:\n- 'train' ('l' subset of training data (2,500 h))\n- 'validation'\n\nTest splits:\n- 'test'\n\nAlso available are subsets of the train split, which can be accessed by setting the 'subconfig' argument:\n\n- 'xs': extra-small subset of training data (10 h)\n- 's': small subset of training data (250 h)\n- 'm': medium subset of training data (1,000 h)\n- 'xl': extra-large subset of training data (10,000 h)"
] |
73cb1384b467451a0c32c1851b712a7e90a9bc57 |
# Dataset Card for PP4AV
## Table of Contents
- [Table of Contents](#table-of-contents)
- [Dataset Description](#dataset-description)
- [Dataset Summary](#dataset-summary)
- [Languages](#languages)
- [Dataset Structure](#dataset-structure)
- [Data Instances](#data-instances)
- [Data Fields](#data-fields)
- [Dataset Creation](#dataset-creation)
- [Source Data](#source-data)
- [Annotations](#annotations)
- [Personal and Sensitive Information](#personal-and-sensitive-information)
- [Considerations for Using the Data](#considerations-for-using-the-data)
- [Social Impact of Dataset](#social-impact-of-dataset)
- [Discussion of Biases](#discussion-of-biases)
- [Other Known Limitations](#other-known-limitations)
- [Additional Information](#additional-information)
- [Dataset Curators](#dataset-curators)
- [Licensing Information](#licensing-information)
- [Citation Information](#citation-information)
- [Contributions](#contributions)
## Dataset Description
- **Homepage:** https://github.com/khaclinh/pp4av
- **Repository:**
- **Paper:** [PP4AV: A benchmarking Dataset for Privacy-preserving Autonomous Driving]
- **Point of Contact:** [email protected]
### Dataset Summary
PP4AV is the first public dataset with faces and license plates annotated with driving scenarios. P4AV provides 3,447 annotated driving images for both faces and license plates. For normal camera data, dataset sampled images from the existing videos in which cameras were mounted in moving vehicles, running around the European cities. The images in PP4AV were sampled from 6 European cities at various times of day, including nighttime. This dataset use the fisheye images from the WoodScape dataset to select 244 images from the front, rear, left, and right cameras for fisheye camera data. PP4AV dataset can be used as a benchmark suite (evaluating dataset) for data anonymization models in autonomous driving.
### Languages
English
## Dataset Structure
### Data Instances
A data point comprises an image and its face and license plate annotations.
```
{
'image': <PIL.JpegImagePlugin.JpegImageFile image mode=RGB size=1920x1080 at 0x19FA12186D8>, 'objects': {
'bbox': [
[0 0.230078 0.317081 0.239062 0.331367],
[1 0.5017185 0.0306425 0.5185935 0.0410975],
[1 0.695078 0.0710145 0.7109375 0.0863355],
[1 0.4089065 0.31646 0.414375 0.32764],
[0 0.1843745 0.403416 0.201093 0.414182],
[0 0.7132 0.3393474 0.717922 0.3514285]
]
}
}
```
### Data Fields
- `image`: A `PIL.Image.Image` object containing the image. Note that when accessing the image column: `dataset[0]["image"]` the image file is automatically decoded. Decoding of a large number of image files might take a significant amount of time. Thus it is important to first query the sample index before the `"image"` column, *i.e.* `dataset[0]["image"]` should **always** be preferred over `dataset["image"][0]`
- `objects`: a dictionary of face and license plate bounding boxes present on the image
- `bbox`: the bounding box of each face and license plate (in the [yolo](https://albumentations.ai/docs/getting_started/bounding_boxes_augmentation/#yolo) format). Basically, each row in annotation `.txt` file for each image `.png` file consists of data in format: `<object-class> <x_center> <y_center> <width> <height>`:
- `object-class`: integer number of object from 0 to 1, where 0 indicate face object, and 1 indicate licese plate object
- `x_center`: normalized x-axis coordinate of the center of the bounding box.
`x_center = <absolute_x_center> / <image_width>`
- `y_center`: normalized y-axis coordinate of the center of the bounding box.
`y_center = <absolute_y_center> / <image_height>`
- `width`: normalized width of the bounding box.
`width = <absolute_width> / <image_width>`
- `height`: normalized wheightdth of the bounding box.
`height = <absolute_height> / <image_height>`
- Example lines in YOLO v1.1 format `.txt' annotation file:
` 1 0.716797 0.395833 0.216406 0.147222
0 0.687109 0.379167 0.255469 0.158333
1 0.420312 0.395833 0.140625 0.166667
`
## Dataset Creation
### Source Data
#### Initial Data Collection and Normalization
The objective of PP4AV is to build a benchmark dataset that can be used to evaluate face and license plate detection models for autonomous driving. For normal camera data, we sampled images from the existing videos in which cameras were mounted in moving vehicles, running around the European cities. We focus on sampling data in urban areas rather than highways in order to provide sufficient samples of license plates and pedestrians. The images in PP4AV were sampled from **6** European cities at various times of day, including nighttime. The source data from 6 cities in European was described as follow:
- `Paris`: This subset contains **1450** images of the car driving down a Parisian street during the day. The video frame rate is 30 frames per second. The video is longer than one hour. We cut a shorter video for sampling and annotation. The original video can be found at the following URL:
URL: [paris_youtube_video](https://www.youtube.com/watch?v=nqWtGWymV6c)
- `Netherland day time`: This subset consists of **388** images of Hague, Amsterdam city in day time. The image of this subset are sampled from the bellow original video:
URL: [netherland_youtube_video](https://www.youtube.com/watch?v=Xuo4uCZxNrE)
The frame rate of the video is 30 frames per second. We cut a shorter video for sampling and annotation. The original video was longer than a half hour.
- `Netherland night time`: This subset consists of **824** images of Hague, Amsterdam city in night time sampled by the following original video:
URL: [netherland_youtube_video](https://www.youtube.com/watch?v=eAy9eHsynhM)
The frame rate of the video is 30 frames per second. We cut a shorter video for sampling and annotation. The original video was longer than a half hour.
- `Switzerland`: This subset consists of **372** images of Switzerland sampled by the following video:
URL: [switzerland_youtube_video](https://www.youtube.com/watch?v=0iw5IP94m0Q)
The frame rate of the video is 30 frames per second. We cut a shorter video for sampling and annotation. The original video was longer than one hour.
- `Zurich`: This subset consists of **50** images of Zurich city provided by the Cityscapes training set in package [leftImg8bit_trainvaltest.zip](https://www.cityscapes-dataset.com/file-handling/?packageID=3)
- `Stuttgart`: This subset consists of **69** images of Stuttgart city provided by the Cityscapes training set in package [leftImg8bit_trainvaltest.zip](https://www.cityscapes-dataset.com/file-handling/?packageID=3)
- `Strasbourg`: This subset consists of **50** images of Strasbourg city provided by the Cityscapes training set in package [leftImg8bit_trainvaltest.zip](https://www.cityscapes-dataset.com/file-handling/?packageID=3)
We use the fisheye images from the WoodScape dataset to select **244** images from the front, rear, left, and right cameras for fisheye camera data.
The source of fisheye data for sampling is located at WoodScape's [Fisheye images](https://woodscape.valeo.com/download).
In total, **3,447** images were selected and annotated in PP4AV.
### Annotations
#### Annotation process
Annotators annotate facial and license plate objects in images. For facial objects, bounding boxes are defined by all detectable human faces from the forehead to the chin to the ears. Faces were labelled with diverse sizes, skin tones, and faces partially obscured by a transparent material, such as a car windshield. For license plate objects, bounding boxes consists of all recognizable license plates with high variability, such as different sizes, countries, vehicle types (motorcycle, automobile, bus, truck), and occlusions by other vehicles. License plates were annotated for vehicles involved in moving traffic. To ensure the quality of annotation, there are two-step process for annotation. In the first phase, two teams of annotators will independently annotate identical image sets. After their annotation output is complete, a merging method based on the IoU scores between the two bounding boxes of the two annotations will be applied. Pairs of annotations with IoU scores above a threshold will be merged and saved as a single annotation. Annotated pairs with IoU scores below a threshold will be considered conflicting. In the second phase, two teams of reviewers will inspect the conflicting pairs of annotations for revision before a second merging method similar to the first is applied. The results of these two phases will be combined to form the final annotation. All work is conducted on the CVAT tool https://github.com/openvinotoolkit/cvat.
#### Who are the annotators?
Vantix Data Science team
### Personal and Sensitive Information
[More Information Needed]
## Considerations for Using the Data
### Social Impact of Dataset
[More Information Needed]
### Discussion of Biases
[More Information Needed]
### Other Known Limitations
[More Information Needed]
## Additional Information
### Dataset Curators
Linh Trinh
### Licensing Information
[Creative Commons Attribution-NonCommercial-NoDerivatives 4.0 International (CC BY-NC-ND 4.0)](https://creativecommons.org/licenses/by-nc-nd/4.0/).
### Citation Information
```
@article{PP4AV2022,
title = {PP4AV: A benchmarking Dataset for Privacy-preserving Autonomous Driving},
author = {Linh Trinh, Phuong Pham, Hoang Trinh, Nguyen Bach, Dung Nguyen, Giang Nguyen, Huy Nguyen},
booktitle = {IEEE/CVF Winter Conference on Applications of Computer Vision (WACV)},
year = {2023}
}
```
### Contributions
Thanks to [@khaclinh](https://github.com/khaclinh) for adding this dataset.
| khaclinh/testdata | [
"task_categories:object-detection",
"task_ids:face-detection",
"annotations_creators:expert-generated",
"language_creators:found",
"multilinguality:monolingual",
"size_categories:1K<n<10K",
"source_datasets:extended",
"language:en",
"license:cc-by-nc-nd-4.0",
"region:us"
] | 2022-09-30T08:12:25+00:00 | {"annotations_creators": ["expert-generated"], "language_creators": ["found"], "language": ["en"], "license": ["cc-by-nc-nd-4.0"], "multilinguality": ["monolingual"], "size_categories": ["1K<n<10K"], "source_datasets": ["extended"], "task_categories": ["object-detection"], "task_ids": ["face-detection", "license-plate-detection"], "pretty_name": "PP4AV"} | 2023-11-10T23:16:51+00:00 | [] | [
"en"
] | TAGS
#task_categories-object-detection #task_ids-face-detection #annotations_creators-expert-generated #language_creators-found #multilinguality-monolingual #size_categories-1K<n<10K #source_datasets-extended #language-English #license-cc-by-nc-nd-4.0 #region-us
|
# Dataset Card for PP4AV
## Table of Contents
- Table of Contents
- Dataset Description
- Dataset Summary
- Languages
- Dataset Structure
- Data Instances
- Data Fields
- Dataset Creation
- Source Data
- Annotations
- Personal and Sensitive Information
- Considerations for Using the Data
- Social Impact of Dataset
- Discussion of Biases
- Other Known Limitations
- Additional Information
- Dataset Curators
- Licensing Information
- Citation Information
- Contributions
## Dataset Description
- Homepage: URL
- Repository:
- Paper: [PP4AV: A benchmarking Dataset for Privacy-preserving Autonomous Driving]
- Point of Contact: URL@URL
### Dataset Summary
PP4AV is the first public dataset with faces and license plates annotated with driving scenarios. P4AV provides 3,447 annotated driving images for both faces and license plates. For normal camera data, dataset sampled images from the existing videos in which cameras were mounted in moving vehicles, running around the European cities. The images in PP4AV were sampled from 6 European cities at various times of day, including nighttime. This dataset use the fisheye images from the WoodScape dataset to select 244 images from the front, rear, left, and right cameras for fisheye camera data. PP4AV dataset can be used as a benchmark suite (evaluating dataset) for data anonymization models in autonomous driving.
### Languages
English
## Dataset Structure
### Data Instances
A data point comprises an image and its face and license plate annotations.
### Data Fields
- 'image': A 'PIL.Image.Image' object containing the image. Note that when accessing the image column: 'dataset[0]["image"]' the image file is automatically decoded. Decoding of a large number of image files might take a significant amount of time. Thus it is important to first query the sample index before the '"image"' column, *i.e.* 'dataset[0]["image"]' should always be preferred over 'dataset["image"][0]'
- 'objects': a dictionary of face and license plate bounding boxes present on the image
- 'bbox': the bounding box of each face and license plate (in the yolo format). Basically, each row in annotation '.txt' file for each image '.png' file consists of data in format: '<object-class> <x_center> <y_center> <width> <height>':
- 'object-class': integer number of object from 0 to 1, where 0 indicate face object, and 1 indicate licese plate object
- 'x_center': normalized x-axis coordinate of the center of the bounding box.
'x_center = <absolute_x_center> / <image_width>'
- 'y_center': normalized y-axis coordinate of the center of the bounding box.
'y_center = <absolute_y_center> / <image_height>'
- 'width': normalized width of the bounding box.
'width = <absolute_width> / <image_width>'
- 'height': normalized wheightdth of the bounding box.
'height = <absolute_height> / <image_height>'
- Example lines in YOLO v1.1 format '.txt' annotation file:
' 1 0.716797 0.395833 0.216406 0.147222
0 0.687109 0.379167 0.255469 0.158333
1 0.420312 0.395833 0.140625 0.166667
'
## Dataset Creation
### Source Data
#### Initial Data Collection and Normalization
The objective of PP4AV is to build a benchmark dataset that can be used to evaluate face and license plate detection models for autonomous driving. For normal camera data, we sampled images from the existing videos in which cameras were mounted in moving vehicles, running around the European cities. We focus on sampling data in urban areas rather than highways in order to provide sufficient samples of license plates and pedestrians. The images in PP4AV were sampled from 6 European cities at various times of day, including nighttime. The source data from 6 cities in European was described as follow:
- 'Paris': This subset contains 1450 images of the car driving down a Parisian street during the day. The video frame rate is 30 frames per second. The video is longer than one hour. We cut a shorter video for sampling and annotation. The original video can be found at the following URL:
URL: paris_youtube_video
- 'Netherland day time': This subset consists of 388 images of Hague, Amsterdam city in day time. The image of this subset are sampled from the bellow original video:
URL: netherland_youtube_video
The frame rate of the video is 30 frames per second. We cut a shorter video for sampling and annotation. The original video was longer than a half hour.
- 'Netherland night time': This subset consists of 824 images of Hague, Amsterdam city in night time sampled by the following original video:
URL: netherland_youtube_video
The frame rate of the video is 30 frames per second. We cut a shorter video for sampling and annotation. The original video was longer than a half hour.
- 'Switzerland': This subset consists of 372 images of Switzerland sampled by the following video:
URL: switzerland_youtube_video
The frame rate of the video is 30 frames per second. We cut a shorter video for sampling and annotation. The original video was longer than one hour.
- 'Zurich': This subset consists of 50 images of Zurich city provided by the Cityscapes training set in package leftImg8bit_trainvaltest.zip
- 'Stuttgart': This subset consists of 69 images of Stuttgart city provided by the Cityscapes training set in package leftImg8bit_trainvaltest.zip
- 'Strasbourg': This subset consists of 50 images of Strasbourg city provided by the Cityscapes training set in package leftImg8bit_trainvaltest.zip
We use the fisheye images from the WoodScape dataset to select 244 images from the front, rear, left, and right cameras for fisheye camera data.
The source of fisheye data for sampling is located at WoodScape's Fisheye images.
In total, 3,447 images were selected and annotated in PP4AV.
### Annotations
#### Annotation process
Annotators annotate facial and license plate objects in images. For facial objects, bounding boxes are defined by all detectable human faces from the forehead to the chin to the ears. Faces were labelled with diverse sizes, skin tones, and faces partially obscured by a transparent material, such as a car windshield. For license plate objects, bounding boxes consists of all recognizable license plates with high variability, such as different sizes, countries, vehicle types (motorcycle, automobile, bus, truck), and occlusions by other vehicles. License plates were annotated for vehicles involved in moving traffic. To ensure the quality of annotation, there are two-step process for annotation. In the first phase, two teams of annotators will independently annotate identical image sets. After their annotation output is complete, a merging method based on the IoU scores between the two bounding boxes of the two annotations will be applied. Pairs of annotations with IoU scores above a threshold will be merged and saved as a single annotation. Annotated pairs with IoU scores below a threshold will be considered conflicting. In the second phase, two teams of reviewers will inspect the conflicting pairs of annotations for revision before a second merging method similar to the first is applied. The results of these two phases will be combined to form the final annotation. All work is conducted on the CVAT tool URL
#### Who are the annotators?
Vantix Data Science team
### Personal and Sensitive Information
## Considerations for Using the Data
### Social Impact of Dataset
### Discussion of Biases
### Other Known Limitations
## Additional Information
### Dataset Curators
Linh Trinh
### Licensing Information
Creative Commons Attribution-NonCommercial-NoDerivatives 4.0 International (CC BY-NC-ND 4.0).
### Contributions
Thanks to @khaclinh for adding this dataset.
| [
"# Dataset Card for PP4AV",
"## Table of Contents\n- Table of Contents\n- Dataset Description\n - Dataset Summary\n - Languages\n- Dataset Structure\n - Data Instances\n - Data Fields\n- Dataset Creation\n - Source Data\n - Annotations\n - Personal and Sensitive Information\n- Considerations for Using the Data\n - Social Impact of Dataset\n - Discussion of Biases\n - Other Known Limitations\n- Additional Information\n - Dataset Curators\n - Licensing Information\n - Citation Information\n - Contributions",
"## Dataset Description\n\n- Homepage: URL\n- Repository:\n- Paper: [PP4AV: A benchmarking Dataset for Privacy-preserving Autonomous Driving]\n- Point of Contact: URL@URL",
"### Dataset Summary\n\nPP4AV is the first public dataset with faces and license plates annotated with driving scenarios. P4AV provides 3,447 annotated driving images for both faces and license plates. For normal camera data, dataset sampled images from the existing videos in which cameras were mounted in moving vehicles, running around the European cities. The images in PP4AV were sampled from 6 European cities at various times of day, including nighttime. This dataset use the fisheye images from the WoodScape dataset to select 244 images from the front, rear, left, and right cameras for fisheye camera data. PP4AV dataset can be used as a benchmark suite (evaluating dataset) for data anonymization models in autonomous driving.",
"### Languages\n\nEnglish",
"## Dataset Structure",
"### Data Instances\n\nA data point comprises an image and its face and license plate annotations.",
"### Data Fields\n\n- 'image': A 'PIL.Image.Image' object containing the image. Note that when accessing the image column: 'dataset[0][\"image\"]' the image file is automatically decoded. Decoding of a large number of image files might take a significant amount of time. Thus it is important to first query the sample index before the '\"image\"' column, *i.e.* 'dataset[0][\"image\"]' should always be preferred over 'dataset[\"image\"][0]'\n- 'objects': a dictionary of face and license plate bounding boxes present on the image\n - 'bbox': the bounding box of each face and license plate (in the yolo format). Basically, each row in annotation '.txt' file for each image '.png' file consists of data in format: '<object-class> <x_center> <y_center> <width> <height>':\n - 'object-class': integer number of object from 0 to 1, where 0 indicate face object, and 1 indicate licese plate object\n - 'x_center': normalized x-axis coordinate of the center of the bounding box. \n 'x_center = <absolute_x_center> / <image_width>'\n - 'y_center': normalized y-axis coordinate of the center of the bounding box. \n 'y_center = <absolute_y_center> / <image_height>'\n - 'width': normalized width of the bounding box. \n 'width = <absolute_width> / <image_width>'\n - 'height': normalized wheightdth of the bounding box. \n 'height = <absolute_height> / <image_height>'\n - Example lines in YOLO v1.1 format '.txt' annotation file: \n ' 1 0.716797 0.395833 0.216406 0.147222 \n 0 0.687109 0.379167 0.255469 0.158333 \n 1 0.420312 0.395833 0.140625 0.166667\n '",
"## Dataset Creation",
"### Source Data",
"#### Initial Data Collection and Normalization\n\nThe objective of PP4AV is to build a benchmark dataset that can be used to evaluate face and license plate detection models for autonomous driving. For normal camera data, we sampled images from the existing videos in which cameras were mounted in moving vehicles, running around the European cities. We focus on sampling data in urban areas rather than highways in order to provide sufficient samples of license plates and pedestrians. The images in PP4AV were sampled from 6 European cities at various times of day, including nighttime. The source data from 6 cities in European was described as follow:\n - 'Paris': This subset contains 1450 images of the car driving down a Parisian street during the day. The video frame rate is 30 frames per second. The video is longer than one hour. We cut a shorter video for sampling and annotation. The original video can be found at the following URL:\n URL: paris_youtube_video \n - 'Netherland day time': This subset consists of 388 images of Hague, Amsterdam city in day time. The image of this subset are sampled from the bellow original video: \n URL: netherland_youtube_video \n The frame rate of the video is 30 frames per second. We cut a shorter video for sampling and annotation. The original video was longer than a half hour.\n - 'Netherland night time': This subset consists of 824 images of Hague, Amsterdam city in night time sampled by the following original video: \n URL: netherland_youtube_video \n The frame rate of the video is 30 frames per second. We cut a shorter video for sampling and annotation. The original video was longer than a half hour.\n - 'Switzerland': This subset consists of 372 images of Switzerland sampled by the following video: \n URL: switzerland_youtube_video \n The frame rate of the video is 30 frames per second. We cut a shorter video for sampling and annotation. The original video was longer than one hour.\n - 'Zurich': This subset consists of 50 images of Zurich city provided by the Cityscapes training set in package leftImg8bit_trainvaltest.zip\n - 'Stuttgart': This subset consists of 69 images of Stuttgart city provided by the Cityscapes training set in package leftImg8bit_trainvaltest.zip\n - 'Strasbourg': This subset consists of 50 images of Strasbourg city provided by the Cityscapes training set in package leftImg8bit_trainvaltest.zip\n\nWe use the fisheye images from the WoodScape dataset to select 244 images from the front, rear, left, and right cameras for fisheye camera data. \nThe source of fisheye data for sampling is located at WoodScape's Fisheye images.\n\nIn total, 3,447 images were selected and annotated in PP4AV.",
"### Annotations",
"#### Annotation process\n\nAnnotators annotate facial and license plate objects in images. For facial objects, bounding boxes are defined by all detectable human faces from the forehead to the chin to the ears. Faces were labelled with diverse sizes, skin tones, and faces partially obscured by a transparent material, such as a car windshield. For license plate objects, bounding boxes consists of all recognizable license plates with high variability, such as different sizes, countries, vehicle types (motorcycle, automobile, bus, truck), and occlusions by other vehicles. License plates were annotated for vehicles involved in moving traffic. To ensure the quality of annotation, there are two-step process for annotation. In the first phase, two teams of annotators will independently annotate identical image sets. After their annotation output is complete, a merging method based on the IoU scores between the two bounding boxes of the two annotations will be applied. Pairs of annotations with IoU scores above a threshold will be merged and saved as a single annotation. Annotated pairs with IoU scores below a threshold will be considered conflicting. In the second phase, two teams of reviewers will inspect the conflicting pairs of annotations for revision before a second merging method similar to the first is applied. The results of these two phases will be combined to form the final annotation. All work is conducted on the CVAT tool URL",
"#### Who are the annotators?\n\nVantix Data Science team",
"### Personal and Sensitive Information",
"## Considerations for Using the Data",
"### Social Impact of Dataset",
"### Discussion of Biases",
"### Other Known Limitations",
"## Additional Information",
"### Dataset Curators\n\nLinh Trinh",
"### Licensing Information\n\nCreative Commons Attribution-NonCommercial-NoDerivatives 4.0 International (CC BY-NC-ND 4.0).",
"### Contributions\n\nThanks to @khaclinh for adding this dataset."
] | [
"TAGS\n#task_categories-object-detection #task_ids-face-detection #annotations_creators-expert-generated #language_creators-found #multilinguality-monolingual #size_categories-1K<n<10K #source_datasets-extended #language-English #license-cc-by-nc-nd-4.0 #region-us \n",
"# Dataset Card for PP4AV",
"## Table of Contents\n- Table of Contents\n- Dataset Description\n - Dataset Summary\n - Languages\n- Dataset Structure\n - Data Instances\n - Data Fields\n- Dataset Creation\n - Source Data\n - Annotations\n - Personal and Sensitive Information\n- Considerations for Using the Data\n - Social Impact of Dataset\n - Discussion of Biases\n - Other Known Limitations\n- Additional Information\n - Dataset Curators\n - Licensing Information\n - Citation Information\n - Contributions",
"## Dataset Description\n\n- Homepage: URL\n- Repository:\n- Paper: [PP4AV: A benchmarking Dataset for Privacy-preserving Autonomous Driving]\n- Point of Contact: URL@URL",
"### Dataset Summary\n\nPP4AV is the first public dataset with faces and license plates annotated with driving scenarios. P4AV provides 3,447 annotated driving images for both faces and license plates. For normal camera data, dataset sampled images from the existing videos in which cameras were mounted in moving vehicles, running around the European cities. The images in PP4AV were sampled from 6 European cities at various times of day, including nighttime. This dataset use the fisheye images from the WoodScape dataset to select 244 images from the front, rear, left, and right cameras for fisheye camera data. PP4AV dataset can be used as a benchmark suite (evaluating dataset) for data anonymization models in autonomous driving.",
"### Languages\n\nEnglish",
"## Dataset Structure",
"### Data Instances\n\nA data point comprises an image and its face and license plate annotations.",
"### Data Fields\n\n- 'image': A 'PIL.Image.Image' object containing the image. Note that when accessing the image column: 'dataset[0][\"image\"]' the image file is automatically decoded. Decoding of a large number of image files might take a significant amount of time. Thus it is important to first query the sample index before the '\"image\"' column, *i.e.* 'dataset[0][\"image\"]' should always be preferred over 'dataset[\"image\"][0]'\n- 'objects': a dictionary of face and license plate bounding boxes present on the image\n - 'bbox': the bounding box of each face and license plate (in the yolo format). Basically, each row in annotation '.txt' file for each image '.png' file consists of data in format: '<object-class> <x_center> <y_center> <width> <height>':\n - 'object-class': integer number of object from 0 to 1, where 0 indicate face object, and 1 indicate licese plate object\n - 'x_center': normalized x-axis coordinate of the center of the bounding box. \n 'x_center = <absolute_x_center> / <image_width>'\n - 'y_center': normalized y-axis coordinate of the center of the bounding box. \n 'y_center = <absolute_y_center> / <image_height>'\n - 'width': normalized width of the bounding box. \n 'width = <absolute_width> / <image_width>'\n - 'height': normalized wheightdth of the bounding box. \n 'height = <absolute_height> / <image_height>'\n - Example lines in YOLO v1.1 format '.txt' annotation file: \n ' 1 0.716797 0.395833 0.216406 0.147222 \n 0 0.687109 0.379167 0.255469 0.158333 \n 1 0.420312 0.395833 0.140625 0.166667\n '",
"## Dataset Creation",
"### Source Data",
"#### Initial Data Collection and Normalization\n\nThe objective of PP4AV is to build a benchmark dataset that can be used to evaluate face and license plate detection models for autonomous driving. For normal camera data, we sampled images from the existing videos in which cameras were mounted in moving vehicles, running around the European cities. We focus on sampling data in urban areas rather than highways in order to provide sufficient samples of license plates and pedestrians. The images in PP4AV were sampled from 6 European cities at various times of day, including nighttime. The source data from 6 cities in European was described as follow:\n - 'Paris': This subset contains 1450 images of the car driving down a Parisian street during the day. The video frame rate is 30 frames per second. The video is longer than one hour. We cut a shorter video for sampling and annotation. The original video can be found at the following URL:\n URL: paris_youtube_video \n - 'Netherland day time': This subset consists of 388 images of Hague, Amsterdam city in day time. The image of this subset are sampled from the bellow original video: \n URL: netherland_youtube_video \n The frame rate of the video is 30 frames per second. We cut a shorter video for sampling and annotation. The original video was longer than a half hour.\n - 'Netherland night time': This subset consists of 824 images of Hague, Amsterdam city in night time sampled by the following original video: \n URL: netherland_youtube_video \n The frame rate of the video is 30 frames per second. We cut a shorter video for sampling and annotation. The original video was longer than a half hour.\n - 'Switzerland': This subset consists of 372 images of Switzerland sampled by the following video: \n URL: switzerland_youtube_video \n The frame rate of the video is 30 frames per second. We cut a shorter video for sampling and annotation. The original video was longer than one hour.\n - 'Zurich': This subset consists of 50 images of Zurich city provided by the Cityscapes training set in package leftImg8bit_trainvaltest.zip\n - 'Stuttgart': This subset consists of 69 images of Stuttgart city provided by the Cityscapes training set in package leftImg8bit_trainvaltest.zip\n - 'Strasbourg': This subset consists of 50 images of Strasbourg city provided by the Cityscapes training set in package leftImg8bit_trainvaltest.zip\n\nWe use the fisheye images from the WoodScape dataset to select 244 images from the front, rear, left, and right cameras for fisheye camera data. \nThe source of fisheye data for sampling is located at WoodScape's Fisheye images.\n\nIn total, 3,447 images were selected and annotated in PP4AV.",
"### Annotations",
"#### Annotation process\n\nAnnotators annotate facial and license plate objects in images. For facial objects, bounding boxes are defined by all detectable human faces from the forehead to the chin to the ears. Faces were labelled with diverse sizes, skin tones, and faces partially obscured by a transparent material, such as a car windshield. For license plate objects, bounding boxes consists of all recognizable license plates with high variability, such as different sizes, countries, vehicle types (motorcycle, automobile, bus, truck), and occlusions by other vehicles. License plates were annotated for vehicles involved in moving traffic. To ensure the quality of annotation, there are two-step process for annotation. In the first phase, two teams of annotators will independently annotate identical image sets. After their annotation output is complete, a merging method based on the IoU scores between the two bounding boxes of the two annotations will be applied. Pairs of annotations with IoU scores above a threshold will be merged and saved as a single annotation. Annotated pairs with IoU scores below a threshold will be considered conflicting. In the second phase, two teams of reviewers will inspect the conflicting pairs of annotations for revision before a second merging method similar to the first is applied. The results of these two phases will be combined to form the final annotation. All work is conducted on the CVAT tool URL",
"#### Who are the annotators?\n\nVantix Data Science team",
"### Personal and Sensitive Information",
"## Considerations for Using the Data",
"### Social Impact of Dataset",
"### Discussion of Biases",
"### Other Known Limitations",
"## Additional Information",
"### Dataset Curators\n\nLinh Trinh",
"### Licensing Information\n\nCreative Commons Attribution-NonCommercial-NoDerivatives 4.0 International (CC BY-NC-ND 4.0).",
"### Contributions\n\nThanks to @khaclinh for adding this dataset."
] | [
95,
8,
106,
47,
171,
5,
6,
23,
486,
5,
4,
658,
5,
341,
15,
8,
8,
7,
8,
7,
5,
9,
30,
17
] | [
"passage: TAGS\n#task_categories-object-detection #task_ids-face-detection #annotations_creators-expert-generated #language_creators-found #multilinguality-monolingual #size_categories-1K<n<10K #source_datasets-extended #language-English #license-cc-by-nc-nd-4.0 #region-us \n# Dataset Card for PP4AV## Table of Contents\n- Table of Contents\n- Dataset Description\n - Dataset Summary\n - Languages\n- Dataset Structure\n - Data Instances\n - Data Fields\n- Dataset Creation\n - Source Data\n - Annotations\n - Personal and Sensitive Information\n- Considerations for Using the Data\n - Social Impact of Dataset\n - Discussion of Biases\n - Other Known Limitations\n- Additional Information\n - Dataset Curators\n - Licensing Information\n - Citation Information\n - Contributions## Dataset Description\n\n- Homepage: URL\n- Repository:\n- Paper: [PP4AV: A benchmarking Dataset for Privacy-preserving Autonomous Driving]\n- Point of Contact: URL@URL### Dataset Summary\n\nPP4AV is the first public dataset with faces and license plates annotated with driving scenarios. P4AV provides 3,447 annotated driving images for both faces and license plates. For normal camera data, dataset sampled images from the existing videos in which cameras were mounted in moving vehicles, running around the European cities. The images in PP4AV were sampled from 6 European cities at various times of day, including nighttime. This dataset use the fisheye images from the WoodScape dataset to select 244 images from the front, rear, left, and right cameras for fisheye camera data. PP4AV dataset can be used as a benchmark suite (evaluating dataset) for data anonymization models in autonomous driving.### Languages\n\nEnglish## Dataset Structure### Data Instances\n\nA data point comprises an image and its face and license plate annotations.",
"passage: ### Data Fields\n\n- 'image': A 'PIL.Image.Image' object containing the image. Note that when accessing the image column: 'dataset[0][\"image\"]' the image file is automatically decoded. Decoding of a large number of image files might take a significant amount of time. Thus it is important to first query the sample index before the '\"image\"' column, *i.e.* 'dataset[0][\"image\"]' should always be preferred over 'dataset[\"image\"][0]'\n- 'objects': a dictionary of face and license plate bounding boxes present on the image\n - 'bbox': the bounding box of each face and license plate (in the yolo format). Basically, each row in annotation '.txt' file for each image '.png' file consists of data in format: '<object-class> <x_center> <y_center> <width> <height>':\n - 'object-class': integer number of object from 0 to 1, where 0 indicate face object, and 1 indicate licese plate object\n - 'x_center': normalized x-axis coordinate of the center of the bounding box. \n 'x_center = <absolute_x_center> / <image_width>'\n - 'y_center': normalized y-axis coordinate of the center of the bounding box. \n 'y_center = <absolute_y_center> / <image_height>'\n - 'width': normalized width of the bounding box. \n 'width = <absolute_width> / <image_width>'\n - 'height': normalized wheightdth of the bounding box. \n 'height = <absolute_height> / <image_height>'\n - Example lines in YOLO v1.1 format '.txt' annotation file: \n ' 1 0.716797 0.395833 0.216406 0.147222 \n 0 0.687109 0.379167 0.255469 0.158333 \n 1 0.420312 0.395833 0.140625 0.166667\n '## Dataset Creation### Source Data"
] |
ec76e2bfdd7bfbd9d04b24b5d0cbefb424e0b5c9 | Eric pics | Speedy02/eric | [
"region:us"
] | 2022-09-30T08:20:51+00:00 | {} | 2022-09-30T08:55:02+00:00 | [] | [] | TAGS
#region-us
| Eric pics | [] | [
"TAGS\n#region-us \n"
] | [
6
] | [
"passage: TAGS\n#region-us \n"
] |
56584831fefeb7d6cef37df192c05f4ad8b8fc00 | This dataset contains images for the classification of bees and ants | delima87/beesvsants | [
"region:us"
] | 2022-09-30T08:26:04+00:00 | {} | 2022-09-30T08:34:41+00:00 | [] | [] | TAGS
#region-us
| This dataset contains images for the classification of bees and ants | [] | [
"TAGS\n#region-us \n"
] | [
6
] | [
"passage: TAGS\n#region-us \n"
] |
3191d1da8882b20445722fe811b70533412e2173 | # Dataset Card for "MultiTACRED"
## Dataset Description
- **Homepage:** [https://github.com/DFKI-NLP/MultiTACRED](https://github.com/DFKI-NLP/MultiTACRED)
- **Paper:** [MultiTACRED: A Multilingual Version of the TAC Relation Extraction Dataset](https://arxiv.org/abs/2305.04582)
- **Point of Contact:** See [https://github.com/DFKI-NLP/MultiTACRED](https://github.com/DFKI-NLP/MultiTACRED)
- **Size of downloaded dataset files:** 15.4KB (TACRED-Revisited), 3.7 MB (Re-TACRED)
- **Size of the generated dataset:** 1.7 GB (all languages, all versions)
- **Total amount of disk used:** 1.7 GB (all languages, all versions)
### Dataset Summary
MultiTACRED is a multilingual version of the large-scale [TAC Relation Extraction Dataset](https://nlp.stanford.edu/projects/tacred).
It covers 12 typologically diverse languages from 9 language families, and was created by the
[Speech & Language Technology group of DFKI](https://www.dfki.de/slt) by machine-translating the instances of the
original TACRED dataset and automatically projecting their entity annotations. For details of the original TACRED's
data collection and annotation process, see the [Stanford paper](https://aclanthology.org/D17-1004/). Translations are
syntactically validated by checking the correctness of the XML tag markup. Any translations with an invalid tag
structure, e.g. missing or invalid head or tail tag pairs, are discarded (on average, 2.3% of the instances).
Languages covered are: Arabic, Chinese, Finnish, French, German, Hindi, Hungarian, Japanese, Polish,
Russian, Spanish, Turkish. Intended use is supervised relation classification. Audience - researchers.
Please see [our ACL paper](https://aclanthology.org/2023.acl-long.210/) for full details.
NOTE: This Datasetreader supports a reduced version of the original TACRED JSON format with the following changes:
- Removed fields: stanford_pos, stanford_ner, stanford_head, stanford_deprel, docid
The motivation for this is that we want to support additional languages, for which these fields were not required
or available. The reader expects the specification of a language-specific configuration specifying the variant
(original, revisited or retacred) and the language (as a two-letter iso code).
The DatasetReader changes the offsets of the following fields, to conform with standard Python usage (see
_generate_examples()):
- subj_end to subj_end + 1 (make end offset exclusive)
- obj_end to obj_end + 1 (make end offset exclusive)
NOTE 2: The MultiTACRED dataset offers an additional 'split', namely the backtranslated test data (translated to a
target language and then back to English). To access this split, use dataset['backtranslated_test'].
You can find the TACRED dataset reader for the English version of the dataset at
[https://huggingface.co/datasets/DFKI-SLT/tacred](https://huggingface.co/datasets/DFKI-SLT/tacred).
### Supported Tasks and Leaderboards
- **Tasks:** Relation Classification
- **Leaderboards:** [https://paperswithcode.com/sota/relation-extraction-on-multitacred](https://paperswithcode.com/sota/relation-extraction-on-multitacred)
### Languages
The languages in the dataset are Arabic, German, English, Spanish, Finnish, French, Hindi, Hungarian, Japanese, Polish, Russian, Turkish, and Chinese.
All languages except English are machine-translated using either Deepl's or Google's translation APIs.
## Dataset Structure
### Data Instances
- **Size of downloaded dataset files:** 15.4KB (TACRED-Revisited), 3.7 MB (Re-TACRED)
- **Size of the generated dataset:** 1.7 GB (all languages, all versions)
- **Total amount of disk used:** 1.7 GB (all languages, all versions)
An example of 'train' looks as follows:
```json
{
"id": "61b3a5c8c9a882dcfcd2",
"token": ["Tom", "Thabane", "trat", "im", "Oktober", "letzten", "Jahres", "zurรผck", ",", "um", "die", "All", "Basotho", "Convention", "-LRB-", "ABC", "-RRB-", "zu", "grรผnden", ",", "die", "mit", "17", "Abgeordneten", "das", "Wort", "ergriff", ",", "woraufhin", "der", "konstitutionelle", "Monarch", "Kรถnig", "Letsie", "III.", "das", "Parlament", "auflรถste", "und", "Neuwahlen", "ansetzte", "."],
"relation": "org:founded_by",
"subj_start": 11,
"subj_end": 13,
"obj_start": 0,
"obj_end": 1,
"subj_type": "ORGANIZATION",
"obj_type": "PERSON"
}
```
### Data Fields
The data fields are the same among all splits.
- `id`: the instance id of this sentence, a `string` feature.
- `token`: the list of tokens of this sentence, a `list` of `string` features.
- `relation`: the relation label of this instance, a `string` classification label.
- `subj_start`: the 0-based index of the start token of the relation subject mention, an `รฌnt` feature.
- `subj_end`: the 0-based index of the end token of the relation subject mention, exclusive, an `รฌnt` feature.
- `subj_type`: the NER type of the subject mention, among the types used in the [Stanford NER system](https://stanfordnlp.github.io/CoreNLP/ner.html), a `string` feature.
- `obj_start`: the 0-based index of the start token of the relation object mention, an `รฌnt` feature.
- `obj_end`: the 0-based index of the end token of the relation object mention, exclusive, an `รฌnt` feature.
- `obj_type`: the NER type of the object mention, among 23 fine-grained types used in the [Stanford NER system](https://stanfordnlp.github.io/CoreNLP/ner.html), a `string` feature.
### Data Splits
To miminize dataset bias, TACRED is stratified across years in which the TAC KBP challenge was run.
Languages statistics for the splits differ because not all instances could be translated with the
subject and object entity markup still intact, these were discarded.
| Language | Train | Dev | Test | Backtranslated Test | Translation Engine |
| ----- | ------ | ----- | ---- | ---- | ---- |
| en | 68,124 | 22,631 | 15,509 | - | - |
| ar | 67,736 | 22,502 | 15,425 | 15,425 | Google |
| de | 67,253 | 22,343 | 15,282 | 15,079 | DeepL |
| es | 65,247 | 21,697 | 14,908 | 14,688 | DeepL |
| fi | 66,751 | 22,268 | 15,083 | 14,462 | DeepL |
| fr | 66,856 | 22,298 | 15,237 | 15,088 | DeepL |
| hi | 67,751 | 22,511 | 15,440 | 15,440 | Google |
| hu | 67,766 | 22,519 | 15,436 | 15,436 | Google |
| ja | 61,571 | 20,290 | 13,701 | 12,913 | DeepL |
| pl | 68,124 | 22,631 | 15,509 | 15,509 | Google |
| ru | 66,413 | 21,998 | 14,995 | 14,703 | DeepL |
| tr | 67,749 | 22,510 | 15,429 | 15,429 | Google |
| zh | 65,260 | 21,538 | 14,694 | 14,021 | DeepL |
## Dataset Creation
### Curation Rationale
To enable more research on multilingual Relation Extraction, we generate translations of the TAC relation extraction
dataset using DeepL and Google Translate.
### Source Data
#### Initial Data Collection and Normalization
The instances of this dataset are sentences from the
[original TACRED dataset](https://nlp.stanford.edu/projects/tacred/), which in turn
are sampled from the [corpus](https://catalog.ldc.upenn.edu/LDC2018T03) used in the yearly
[TAC Knowledge Base Population (TAC KBP) challenges](https://tac.nist.gov/2017/KBP/index.html).
#### Who are the source language producers?
Newswire and web texts collected for the [TAC Knowledge Base Population (TAC KBP) challenges](https://tac.nist.gov/2017/KBP/index.html).
### Annotations
#### Annotation process
See the Stanford paper, the TACRED Revisited paper, and the Re-TACRED paper, plus their appendices, for
details on the original annotation process. The translated versions do not change the original labels.
Translations were tokenized with language-specific Spacy models (Spacy 3.1, 'core_news/web_sm' models)
or Trankit (Trankit 1.1.0) when there was no Spacy model for a given language (Hungarian, Turkish, Arabic, Hindi).
#### Who are the annotators?
The original TACRED dataset was annotated by crowd workers, see the [TACRED paper](https://nlp.stanford.edu/pubs/zhang2017tacred.pdf).
### Personal and Sensitive Information
The [authors](https://nlp.stanford.edu/pubs/zhang2017tacred.pdf) of the original TACRED dataset
have not stated measures that prevent collecting sensitive or offensive text. Therefore, we do
not rule out the possible risk of sensitive/offensive content in the translated data.
## Considerations for Using the Data
### Social Impact of Dataset
not applicable
### Discussion of Biases
The dataset is drawn from web and newswire text, and thus reflects any biases of these original
texts, as well as biases introduced by the MT models.
### Other Known Limitations
not applicable
## Additional Information
### Dataset Curators
The dataset was created by members of the
[DFKI SLT team: Leonhard Hennig, Philippe Thomas, Sebastian Mรถller, Gabriel Kressin](https://www.dfki.de/en/web/research/research-departments/speech-and-language-technology/speech-and-language-technology-staff-members)
### Licensing Information
To respect the copyright of the underlying TACRED dataset, MultiTACRED is released via the
Linguistic Data Consortium ([LDC License](https://catalog.ldc.upenn.edu/license/ldc-non-members-agreement.pdf)).
You can download MultiTACRED from the [LDC MultiTACRED webpage](https://catalog.ldc.upenn.edu/TODO).
If you are an LDC member, the access will be free; otherwise, an access fee of $25 is needed.
### Citation Information
The original dataset:
```
@inproceedings{zhang2017tacred,
author = {Zhang, Yuhao and Zhong, Victor and Chen, Danqi and Angeli, Gabor and Manning, Christopher D.},
booktitle = {Proceedings of the 2017 Conference on Empirical Methods in Natural Language Processing (EMNLP 2017)},
title = {Position-aware Attention and Supervised Data Improve Slot Filling},
url = {https://nlp.stanford.edu/pubs/zhang2017tacred.pdf},
pages = {35--45},
year = {2017}
}
```
For the revised version, please also cite:
```
@inproceedings{alt-etal-2020-tacred,
title = "{TACRED} Revisited: A Thorough Evaluation of the {TACRED} Relation Extraction Task",
author = "Alt, Christoph and
Gabryszak, Aleksandra and
Hennig, Leonhard",
booktitle = "Proceedings of the 58th Annual Meeting of the Association for Computational Linguistics",
month = jul,
year = "2020",
address = "Online",
publisher = "Association for Computational Linguistics",
url = "https://www.aclweb.org/anthology/2020.acl-main.142",
doi = "10.18653/v1/2020.acl-main.142",
pages = "1558--1569",
}
```
For the Re-TACRED version, please also cite:
```
@inproceedings{DBLP:conf/aaai/StoicaPP21,
author = {George Stoica and
Emmanouil Antonios Platanios and
Barnab{\'{a}}s P{\'{o}}czos},
title = {Re-TACRED: Addressing Shortcomings of the {TACRED} Dataset},
booktitle = {Thirty-Fifth {AAAI} Conference on Artificial Intelligence, {AAAI}
2021, Thirty-Third Conference on Innovative Applications of Artificial
Intelligence, {IAAI} 2021, The Eleventh Symposium on Educational Advances
in Artificial Intelligence, {EAAI} 2021, Virtual Event, February 2-9,
2021},
pages = {13843--13850},
publisher = {{AAAI} Press},
year = {2021},
url = {https://ojs.aaai.org/index.php/AAAI/article/view/17631},
}
```
### Contributions
Thanks to [@leonhardhennig](https://github.com/leonhardhennig) for adding this dataset. | DFKI-SLT/multitacred | [
"task_categories:text-classification",
"task_ids:multi-class-classification",
"annotations_creators:crowdsourced",
"annotations_creators:expert-generated",
"language_creators:found",
"size_categories:100K<n<1M",
"source_datasets:DFKI-NLP/tacred",
"language:ar",
"language:de",
"language:es",
"language:fi",
"language:fr",
"language:hi",
"language:hu",
"language:ja",
"language:pl",
"language:ru",
"language:tr",
"language:zh",
"license:other",
"relation extraction",
"arxiv:2305.04582",
"region:us"
] | 2022-09-30T10:31:31+00:00 | {"annotations_creators": ["crowdsourced", "expert-generated"], "language_creators": ["found"], "language": ["ar", "de", "es", "fi", "fr", "hi", "hu", "ja", "pl", "ru", "tr", "zh"], "license": "other", "size_categories": ["100K<n<1M"], "source_datasets": ["DFKI-NLP/tacred"], "task_categories": ["text-classification"], "task_ids": ["multi-class-classification"], "paperswithcode_id": "multitacred", "pretty_name": "MultiTACRED - Multilingual TAC Relation Extraction Dataset", "license_details": "https://catalog.ldc.upenn.edu/license/ldc-non-members-agreement.pdf", "tags": ["relation extraction"], "dataset_info": [{"config_name": "original-ar", "features": [{"name": "id", "dtype": "string"}, {"name": "token", "sequence": "string"}, {"name": "subj_start", "dtype": "int32"}, {"name": "subj_end", "dtype": "int32"}, {"name": "subj_type", "dtype": {"class_label": {"names": {"0": "LOCATION", "1": "ORGANIZATION", "2": "PERSON", "3": "DATE", "4": "MONEY", "5": "PERCENT", "6": "TIME", "7": "CAUSE_OF_DEATH", "8": "CITY", "9": "COUNTRY", "10": "CRIMINAL_CHARGE", "11": "EMAIL", "12": "HANDLE", "13": "IDEOLOGY", "14": "NATIONALITY", "15": "RELIGION", "16": "STATE_OR_PROVINCE", "17": "TITLE", "18": "URL", "19": "NUMBER", "20": "ORDINAL", "21": "MISC", "22": "DURATION", "23": "O"}}}}, {"name": "obj_start", "dtype": "int32"}, {"name": "obj_end", "dtype": "int32"}, {"name": "obj_type", "dtype": {"class_label": {"names": {"0": "LOCATION", "1": "ORGANIZATION", "2": "PERSON", "3": "DATE", "4": "MONEY", "5": "PERCENT", "6": "TIME", "7": "CAUSE_OF_DEATH", "8": "CITY", "9": "COUNTRY", "10": "CRIMINAL_CHARGE", "11": "EMAIL", "12": "HANDLE", "13": "IDEOLOGY", "14": "NATIONALITY", "15": "RELIGION", "16": "STATE_OR_PROVINCE", "17": "TITLE", "18": "URL", "19": "NUMBER", "20": "ORDINAL", "21": "MISC", "22": "DURATION", "23": "O"}}}}, {"name": "relation", "dtype": {"class_label": {"names": {"0": "no_relation", "1": "org:alternate_names", "2": "org:city_of_headquarters", "3": "org:country_of_headquarters", "4": "org:dissolved", "5": "org:founded", "6": "org:founded_by", "7": "org:member_of", "8": "org:members", "9": "org:number_of_employees/members", "10": "org:parents", "11": "org:political/religious_affiliation", "12": "org:shareholders", "13": "org:stateorprovince_of_headquarters", "14": "org:subsidiaries", "15": "org:top_members/employees", "16": "org:website", "17": "per:age", "18": "per:alternate_names", "19": "per:cause_of_death", "20": "per:charges", "21": "per:children", "22": "per:cities_of_residence", "23": "per:city_of_birth", "24": "per:city_of_death", "25": "per:countries_of_residence", "26": "per:country_of_birth", "27": "per:country_of_death", "28": "per:date_of_birth", "29": "per:date_of_death", "30": "per:employee_of", "31": "per:origin", "32": "per:other_family", "33": "per:parents", "34": "per:religion", "35": "per:schools_attended", "36": "per:siblings", "37": "per:spouse", "38": "per:stateorprovince_of_birth", "39": "per:stateorprovince_of_death", "40": "per:stateorprovinces_of_residence", "41": "per:title"}}}}], "splits": [{"name": "train", "num_bytes": 32371641, "num_examples": 67736}, {"name": "test", "num_bytes": 6895001, "num_examples": 15425}, {"name": "validation", "num_bytes": 10353930, "num_examples": 22502}, {"name": "backtranslated_test", "num_bytes": 5687302, "num_examples": 15425}], "download_size": 0, "dataset_size": 55307874}, {"config_name": "revisited-ar", "features": [{"name": "id", "dtype": "string"}, {"name": "token", "sequence": "string"}, {"name": "subj_start", "dtype": "int32"}, {"name": "subj_end", "dtype": "int32"}, {"name": "subj_type", "dtype": {"class_label": {"names": {"0": "LOCATION", "1": "ORGANIZATION", "2": "PERSON", "3": "DATE", "4": "MONEY", "5": "PERCENT", "6": "TIME", "7": "CAUSE_OF_DEATH", "8": "CITY", "9": "COUNTRY", "10": "CRIMINAL_CHARGE", "11": "EMAIL", "12": "HANDLE", "13": "IDEOLOGY", "14": "NATIONALITY", "15": "RELIGION", "16": "STATE_OR_PROVINCE", "17": "TITLE", "18": "URL", "19": "NUMBER", "20": "ORDINAL", "21": "MISC", "22": "DURATION", "23": "O"}}}}, {"name": "obj_start", "dtype": "int32"}, {"name": "obj_end", "dtype": "int32"}, {"name": "obj_type", "dtype": {"class_label": {"names": {"0": "LOCATION", "1": "ORGANIZATION", "2": "PERSON", "3": "DATE", "4": "MONEY", "5": "PERCENT", "6": "TIME", "7": "CAUSE_OF_DEATH", "8": "CITY", "9": "COUNTRY", "10": "CRIMINAL_CHARGE", "11": "EMAIL", "12": "HANDLE", "13": "IDEOLOGY", "14": "NATIONALITY", "15": "RELIGION", "16": "STATE_OR_PROVINCE", "17": "TITLE", "18": "URL", "19": "NUMBER", "20": "ORDINAL", "21": "MISC", "22": "DURATION", "23": "O"}}}}, {"name": "relation", "dtype": {"class_label": {"names": {"0": "no_relation", "1": "org:alternate_names", "2": "org:city_of_headquarters", "3": "org:country_of_headquarters", "4": "org:dissolved", "5": "org:founded", "6": "org:founded_by", "7": "org:member_of", "8": "org:members", "9": "org:number_of_employees/members", "10": "org:parents", "11": "org:political/religious_affiliation", "12": "org:shareholders", "13": "org:stateorprovince_of_headquarters", "14": "org:subsidiaries", "15": "org:top_members/employees", "16": "org:website", "17": "per:age", "18": "per:alternate_names", "19": "per:cause_of_death", "20": "per:charges", "21": "per:children", "22": "per:cities_of_residence", "23": "per:city_of_birth", "24": "per:city_of_death", "25": "per:countries_of_residence", "26": "per:country_of_birth", "27": "per:country_of_death", "28": "per:date_of_birth", "29": "per:date_of_death", "30": "per:employee_of", "31": "per:origin", "32": "per:other_family", "33": "per:parents", "34": "per:religion", "35": "per:schools_attended", "36": "per:siblings", "37": "per:spouse", "38": "per:stateorprovince_of_birth", "39": "per:stateorprovince_of_death", "40": "per:stateorprovinces_of_residence", "41": "per:title"}}}}], "splits": [{"name": "train", "num_bytes": 32371641, "num_examples": 67736}, {"name": "test", "num_bytes": 6895001, "num_examples": 15425}, {"name": "validation", "num_bytes": 10353930, "num_examples": 22502}, {"name": "backtranslated_test", "num_bytes": 5687302, "num_examples": 15425}], "download_size": 157165, "dataset_size": 55307874}, {"config_name": "retacred-ar", "features": [{"name": "id", "dtype": "string"}, {"name": "token", "sequence": "string"}, {"name": "subj_start", "dtype": "int32"}, {"name": "subj_end", "dtype": "int32"}, {"name": "subj_type", "dtype": {"class_label": {"names": {"0": "LOCATION", "1": "ORGANIZATION", "2": "PERSON", "3": "DATE", "4": "MONEY", "5": "PERCENT", "6": "TIME", "7": "CAUSE_OF_DEATH", "8": "CITY", "9": "COUNTRY", "10": "CRIMINAL_CHARGE", "11": "EMAIL", "12": "HANDLE", "13": "IDEOLOGY", "14": "NATIONALITY", "15": "RELIGION", "16": "STATE_OR_PROVINCE", "17": "TITLE", "18": "URL", "19": "NUMBER", "20": "ORDINAL", "21": "MISC", "22": "DURATION", "23": "O"}}}}, {"name": "obj_start", "dtype": "int32"}, {"name": "obj_end", "dtype": "int32"}, {"name": "obj_type", "dtype": {"class_label": {"names": {"0": "LOCATION", "1": "ORGANIZATION", "2": "PERSON", "3": "DATE", "4": "MONEY", "5": "PERCENT", "6": "TIME", "7": "CAUSE_OF_DEATH", "8": "CITY", "9": "COUNTRY", "10": "CRIMINAL_CHARGE", "11": "EMAIL", "12": "HANDLE", "13": "IDEOLOGY", "14": "NATIONALITY", "15": "RELIGION", "16": "STATE_OR_PROVINCE", "17": "TITLE", "18": "URL", "19": "NUMBER", "20": "ORDINAL", "21": "MISC", "22": "DURATION", "23": "O"}}}}, {"name": "relation", "dtype": {"class_label": {"names": {"0": "no_relation", "1": "org:alternate_names", "2": "org:city_of_branch", "3": "org:country_of_branch", "4": "org:dissolved", "5": "org:founded", "6": "org:founded_by", "7": "org:member_of", "8": "org:members", "9": "org:number_of_employees/members", "10": "org:political/religious_affiliation", "11": "org:shareholders", "12": "org:stateorprovince_of_branch", "13": "org:top_members/employees", "14": "org:website", "15": "per:age", "16": "per:cause_of_death", "17": "per:charges", "18": "per:children", "19": "per:cities_of_residence", "20": "per:city_of_birth", "21": "per:city_of_death", "22": "per:countries_of_residence", "23": "per:country_of_birth", "24": "per:country_of_death", "25": "per:date_of_birth", "26": "per:date_of_death", "27": "per:employee_of", "28": "per:identity", "29": "per:origin", "30": "per:other_family", "31": "per:parents", "32": "per:religion", "33": "per:schools_attended", "34": "per:siblings", "35": "per:spouse", "36": "per:stateorprovince_of_birth", "37": "per:stateorprovince_of_death", "38": "per:stateorprovinces_of_residence", "39": "per:title"}}}}], "splits": [{"name": "train", "num_bytes": 27777106, "num_examples": 58171}, {"name": "test", "num_bytes": 5950395, "num_examples": 13348}, {"name": "validation", "num_bytes": 8941018, "num_examples": 19480}, {"name": "backtranslated_test", "num_bytes": 4906896, "num_examples": 13348}], "download_size": 3702157, "dataset_size": 47575415}, {"config_name": "original-de", "features": [{"name": "id", "dtype": "string"}, {"name": "token", "sequence": "string"}, {"name": "subj_start", "dtype": "int32"}, {"name": "subj_end", "dtype": "int32"}, {"name": "subj_type", "dtype": {"class_label": {"names": {"0": "LOCATION", "1": "ORGANIZATION", "2": "PERSON", "3": "DATE", "4": "MONEY", "5": "PERCENT", "6": "TIME", "7": "CAUSE_OF_DEATH", "8": "CITY", "9": "COUNTRY", "10": "CRIMINAL_CHARGE", "11": "EMAIL", "12": "HANDLE", "13": "IDEOLOGY", "14": "NATIONALITY", "15": "RELIGION", "16": "STATE_OR_PROVINCE", "17": "TITLE", "18": "URL", "19": "NUMBER", "20": "ORDINAL", "21": "MISC", "22": "DURATION", "23": "O"}}}}, {"name": "obj_start", "dtype": "int32"}, {"name": "obj_end", "dtype": "int32"}, {"name": "obj_type", "dtype": {"class_label": {"names": {"0": "LOCATION", "1": "ORGANIZATION", "2": "PERSON", "3": "DATE", "4": "MONEY", "5": "PERCENT", "6": "TIME", "7": "CAUSE_OF_DEATH", "8": "CITY", "9": "COUNTRY", "10": "CRIMINAL_CHARGE", "11": "EMAIL", "12": "HANDLE", "13": "IDEOLOGY", "14": "NATIONALITY", "15": "RELIGION", "16": "STATE_OR_PROVINCE", "17": "TITLE", "18": "URL", "19": "NUMBER", "20": "ORDINAL", "21": "MISC", "22": "DURATION", "23": "O"}}}}, {"name": "relation", "dtype": {"class_label": {"names": {"0": "no_relation", "1": "org:alternate_names", "2": "org:city_of_headquarters", "3": "org:country_of_headquarters", "4": "org:dissolved", "5": "org:founded", "6": "org:founded_by", "7": "org:member_of", "8": "org:members", "9": "org:number_of_employees/members", "10": "org:parents", "11": "org:political/religious_affiliation", "12": "org:shareholders", "13": "org:stateorprovince_of_headquarters", "14": "org:subsidiaries", "15": "org:top_members/employees", "16": "org:website", "17": "per:age", "18": "per:alternate_names", "19": "per:cause_of_death", "20": "per:charges", "21": "per:children", "22": "per:cities_of_residence", "23": "per:city_of_birth", "24": "per:city_of_death", "25": "per:countries_of_residence", "26": "per:country_of_birth", "27": "per:country_of_death", "28": "per:date_of_birth", "29": "per:date_of_death", "30": "per:employee_of", "31": "per:origin", "32": "per:other_family", "33": "per:parents", "34": "per:religion", "35": "per:schools_attended", "36": "per:siblings", "37": "per:spouse", "38": "per:stateorprovince_of_birth", "39": "per:stateorprovince_of_death", "40": "per:stateorprovinces_of_residence", "41": "per:title"}}}}], "splits": [{"name": "train", "num_bytes": 27810245, "num_examples": 67253}, {"name": "test", "num_bytes": 6043815, "num_examples": 15282}, {"name": "validation", "num_bytes": 9007367, "num_examples": 22343}, {"name": "backtranslated_test", "num_bytes": 5467635, "num_examples": 15079}], "download_size": 0, "dataset_size": 48329062}, {"config_name": "revisited-de", "features": [{"name": "id", "dtype": "string"}, {"name": "token", "sequence": "string"}, {"name": "subj_start", "dtype": "int32"}, {"name": "subj_end", "dtype": "int32"}, {"name": "subj_type", "dtype": {"class_label": {"names": {"0": "LOCATION", "1": "ORGANIZATION", "2": "PERSON", "3": "DATE", "4": "MONEY", "5": "PERCENT", "6": "TIME", "7": "CAUSE_OF_DEATH", "8": "CITY", "9": "COUNTRY", "10": "CRIMINAL_CHARGE", "11": "EMAIL", "12": "HANDLE", "13": "IDEOLOGY", "14": "NATIONALITY", "15": "RELIGION", "16": "STATE_OR_PROVINCE", "17": "TITLE", "18": "URL", "19": "NUMBER", "20": "ORDINAL", "21": "MISC", "22": "DURATION", "23": "O"}}}}, {"name": "obj_start", "dtype": "int32"}, {"name": "obj_end", "dtype": "int32"}, {"name": "obj_type", "dtype": {"class_label": {"names": {"0": "LOCATION", "1": "ORGANIZATION", "2": "PERSON", "3": "DATE", "4": "MONEY", "5": "PERCENT", "6": "TIME", "7": "CAUSE_OF_DEATH", "8": "CITY", "9": "COUNTRY", "10": "CRIMINAL_CHARGE", "11": "EMAIL", "12": "HANDLE", "13": "IDEOLOGY", "14": "NATIONALITY", "15": "RELIGION", "16": "STATE_OR_PROVINCE", "17": "TITLE", "18": "URL", "19": "NUMBER", "20": "ORDINAL", "21": "MISC", "22": "DURATION", "23": "O"}}}}, {"name": "relation", "dtype": {"class_label": {"names": {"0": "no_relation", "1": "org:alternate_names", "2": "org:city_of_headquarters", "3": "org:country_of_headquarters", "4": "org:dissolved", "5": "org:founded", "6": "org:founded_by", "7": "org:member_of", "8": "org:members", "9": "org:number_of_employees/members", "10": "org:parents", "11": "org:political/religious_affiliation", "12": "org:shareholders", "13": "org:stateorprovince_of_headquarters", "14": "org:subsidiaries", "15": "org:top_members/employees", "16": "org:website", "17": "per:age", "18": "per:alternate_names", "19": "per:cause_of_death", "20": "per:charges", "21": "per:children", "22": "per:cities_of_residence", "23": "per:city_of_birth", "24": "per:city_of_death", "25": "per:countries_of_residence", "26": "per:country_of_birth", "27": "per:country_of_death", "28": "per:date_of_birth", "29": "per:date_of_death", "30": "per:employee_of", "31": "per:origin", "32": "per:other_family", "33": "per:parents", "34": "per:religion", "35": "per:schools_attended", "36": "per:siblings", "37": "per:spouse", "38": "per:stateorprovince_of_birth", "39": "per:stateorprovince_of_death", "40": "per:stateorprovinces_of_residence", "41": "per:title"}}}}], "splits": [{"name": "train", "num_bytes": 27810245, "num_examples": 67253}, {"name": "test", "num_bytes": 6043815, "num_examples": 15282}, {"name": "validation", "num_bytes": 9007367, "num_examples": 22343}, {"name": "backtranslated_test", "num_bytes": 5467635, "num_examples": 15079}], "download_size": 157165, "dataset_size": 48329062}, {"config_name": "retacred-de", "features": [{"name": "id", "dtype": "string"}, {"name": "token", "sequence": "string"}, {"name": "subj_start", "dtype": "int32"}, {"name": "subj_end", "dtype": "int32"}, {"name": "subj_type", "dtype": {"class_label": {"names": {"0": "LOCATION", "1": "ORGANIZATION", "2": "PERSON", "3": "DATE", "4": "MONEY", "5": "PERCENT", "6": "TIME", "7": "CAUSE_OF_DEATH", "8": "CITY", "9": "COUNTRY", "10": "CRIMINAL_CHARGE", "11": "EMAIL", "12": "HANDLE", "13": "IDEOLOGY", "14": "NATIONALITY", "15": "RELIGION", "16": "STATE_OR_PROVINCE", "17": "TITLE", "18": "URL", "19": "NUMBER", "20": "ORDINAL", "21": "MISC", "22": "DURATION", "23": "O"}}}}, {"name": "obj_start", "dtype": "int32"}, {"name": "obj_end", "dtype": "int32"}, {"name": "obj_type", "dtype": {"class_label": {"names": {"0": "LOCATION", "1": "ORGANIZATION", "2": "PERSON", "3": "DATE", "4": "MONEY", "5": "PERCENT", "6": "TIME", "7": "CAUSE_OF_DEATH", "8": "CITY", "9": "COUNTRY", "10": "CRIMINAL_CHARGE", "11": "EMAIL", "12": "HANDLE", "13": "IDEOLOGY", "14": "NATIONALITY", "15": "RELIGION", "16": "STATE_OR_PROVINCE", "17": "TITLE", "18": "URL", "19": "NUMBER", "20": "ORDINAL", "21": "MISC", "22": "DURATION", "23": "O"}}}}, {"name": "relation", "dtype": {"class_label": {"names": {"0": "no_relation", "1": "org:alternate_names", "2": "org:city_of_branch", "3": "org:country_of_branch", "4": "org:dissolved", "5": "org:founded", "6": "org:founded_by", "7": "org:member_of", "8": "org:members", "9": "org:number_of_employees/members", "10": "org:political/religious_affiliation", "11": "org:shareholders", "12": "org:stateorprovince_of_branch", "13": "org:top_members/employees", "14": "org:website", "15": "per:age", "16": "per:cause_of_death", "17": "per:charges", "18": "per:children", "19": "per:cities_of_residence", "20": "per:city_of_birth", "21": "per:city_of_death", "22": "per:countries_of_residence", "23": "per:country_of_birth", "24": "per:country_of_death", "25": "per:date_of_birth", "26": "per:date_of_death", "27": "per:employee_of", "28": "per:identity", "29": "per:origin", "30": "per:other_family", "31": "per:parents", "32": "per:religion", "33": "per:schools_attended", "34": "per:siblings", "35": "per:spouse", "36": "per:stateorprovince_of_birth", "37": "per:stateorprovince_of_death", "38": "per:stateorprovinces_of_residence", "39": "per:title"}}}}], "splits": [{"name": "train", "num_bytes": 23935820, "num_examples": 57792}, {"name": "test", "num_bytes": 5219772, "num_examples": 13227}, {"name": "validation", "num_bytes": 7794542, "num_examples": 19365}, {"name": "backtranslated_test", "num_bytes": 4715329, "num_examples": 13046}], "download_size": 3702157, "dataset_size": 41665463}, {"config_name": "original-es", "features": [{"name": "id", "dtype": "string"}, {"name": "token", "sequence": "string"}, {"name": "subj_start", "dtype": "int32"}, {"name": "subj_end", "dtype": "int32"}, {"name": "subj_type", "dtype": {"class_label": {"names": {"0": "LOCATION", "1": "ORGANIZATION", "2": "PERSON", "3": "DATE", "4": "MONEY", "5": "PERCENT", "6": "TIME", "7": "CAUSE_OF_DEATH", "8": "CITY", "9": "COUNTRY", "10": "CRIMINAL_CHARGE", "11": "EMAIL", "12": "HANDLE", "13": "IDEOLOGY", "14": "NATIONALITY", "15": "RELIGION", "16": "STATE_OR_PROVINCE", "17": "TITLE", "18": "URL", "19": "NUMBER", "20": "ORDINAL", "21": "MISC", "22": "DURATION", "23": "O"}}}}, {"name": "obj_start", "dtype": "int32"}, {"name": "obj_end", "dtype": "int32"}, {"name": "obj_type", "dtype": {"class_label": {"names": {"0": "LOCATION", "1": "ORGANIZATION", "2": "PERSON", "3": "DATE", "4": "MONEY", "5": "PERCENT", "6": "TIME", "7": "CAUSE_OF_DEATH", "8": "CITY", "9": "COUNTRY", "10": "CRIMINAL_CHARGE", "11": "EMAIL", "12": "HANDLE", "13": "IDEOLOGY", "14": "NATIONALITY", "15": "RELIGION", "16": "STATE_OR_PROVINCE", "17": "TITLE", "18": "URL", "19": "NUMBER", "20": "ORDINAL", "21": "MISC", "22": "DURATION", "23": "O"}}}}, {"name": "relation", "dtype": {"class_label": {"names": {"0": "no_relation", "1": "org:alternate_names", "2": "org:city_of_headquarters", "3": "org:country_of_headquarters", "4": "org:dissolved", "5": "org:founded", "6": "org:founded_by", "7": "org:member_of", "8": "org:members", "9": "org:number_of_employees/members", "10": "org:parents", "11": "org:political/religious_affiliation", "12": "org:shareholders", "13": "org:stateorprovince_of_headquarters", "14": "org:subsidiaries", "15": "org:top_members/employees", "16": "org:website", "17": "per:age", "18": "per:alternate_names", "19": "per:cause_of_death", "20": "per:charges", "21": "per:children", "22": "per:cities_of_residence", "23": "per:city_of_birth", "24": "per:city_of_death", "25": "per:countries_of_residence", "26": "per:country_of_birth", "27": "per:country_of_death", "28": "per:date_of_birth", "29": "per:date_of_death", "30": "per:employee_of", "31": "per:origin", "32": "per:other_family", "33": "per:parents", "34": "per:religion", "35": "per:schools_attended", "36": "per:siblings", "37": "per:spouse", "38": "per:stateorprovince_of_birth", "39": "per:stateorprovince_of_death", "40": "per:stateorprovinces_of_residence", "41": "per:title"}}}}], "splits": [{"name": "train", "num_bytes": 27586822, "num_examples": 65247}, {"name": "test", "num_bytes": 5941821, "num_examples": 14908}, {"name": "validation", "num_bytes": 8921047, "num_examples": 21697}, {"name": "backtranslated_test", "num_bytes": 5414680, "num_examples": 14688}], "download_size": 0, "dataset_size": 47864370}, {"config_name": "revisited-es", "features": [{"name": "id", "dtype": "string"}, {"name": "token", "sequence": "string"}, {"name": "subj_start", "dtype": "int32"}, {"name": "subj_end", "dtype": "int32"}, {"name": "subj_type", "dtype": {"class_label": {"names": {"0": "LOCATION", "1": "ORGANIZATION", "2": "PERSON", "3": "DATE", "4": "MONEY", "5": "PERCENT", "6": "TIME", "7": "CAUSE_OF_DEATH", "8": "CITY", "9": "COUNTRY", "10": "CRIMINAL_CHARGE", "11": "EMAIL", "12": "HANDLE", "13": "IDEOLOGY", "14": "NATIONALITY", "15": "RELIGION", "16": "STATE_OR_PROVINCE", "17": "TITLE", "18": "URL", "19": "NUMBER", "20": "ORDINAL", "21": "MISC", "22": "DURATION", "23": "O"}}}}, {"name": "obj_start", "dtype": "int32"}, {"name": "obj_end", "dtype": "int32"}, {"name": "obj_type", "dtype": {"class_label": {"names": {"0": "LOCATION", "1": "ORGANIZATION", "2": "PERSON", "3": "DATE", "4": "MONEY", "5": "PERCENT", "6": "TIME", "7": "CAUSE_OF_DEATH", "8": "CITY", "9": "COUNTRY", "10": "CRIMINAL_CHARGE", "11": "EMAIL", "12": "HANDLE", "13": "IDEOLOGY", "14": "NATIONALITY", "15": "RELIGION", "16": "STATE_OR_PROVINCE", "17": "TITLE", "18": "URL", "19": "NUMBER", "20": "ORDINAL", "21": "MISC", "22": "DURATION", "23": "O"}}}}, {"name": "relation", "dtype": {"class_label": {"names": {"0": "no_relation", "1": "org:alternate_names", "2": "org:city_of_headquarters", "3": "org:country_of_headquarters", "4": "org:dissolved", "5": "org:founded", "6": "org:founded_by", "7": "org:member_of", "8": "org:members", "9": "org:number_of_employees/members", "10": "org:parents", "11": "org:political/religious_affiliation", "12": "org:shareholders", "13": "org:stateorprovince_of_headquarters", "14": "org:subsidiaries", "15": "org:top_members/employees", "16": "org:website", "17": "per:age", "18": "per:alternate_names", "19": "per:cause_of_death", "20": "per:charges", "21": "per:children", "22": "per:cities_of_residence", "23": "per:city_of_birth", "24": "per:city_of_death", "25": "per:countries_of_residence", "26": "per:country_of_birth", "27": "per:country_of_death", "28": "per:date_of_birth", "29": "per:date_of_death", "30": "per:employee_of", "31": "per:origin", "32": "per:other_family", "33": "per:parents", "34": "per:religion", "35": "per:schools_attended", "36": "per:siblings", "37": "per:spouse", "38": "per:stateorprovince_of_birth", "39": "per:stateorprovince_of_death", "40": "per:stateorprovinces_of_residence", "41": "per:title"}}}}], "splits": [{"name": "train", "num_bytes": 27586822, "num_examples": 65247}, {"name": "test", "num_bytes": 5941821, "num_examples": 14908}, {"name": "validation", "num_bytes": 8921047, "num_examples": 21697}, {"name": "backtranslated_test", "num_bytes": 5414680, "num_examples": 14688}], "download_size": 157165, "dataset_size": 47864370}, {"config_name": "retacred-es", "features": [{"name": "id", "dtype": "string"}, {"name": "token", "sequence": "string"}, {"name": "subj_start", "dtype": "int32"}, {"name": "subj_end", "dtype": "int32"}, {"name": "subj_type", "dtype": {"class_label": {"names": {"0": "LOCATION", "1": "ORGANIZATION", "2": "PERSON", "3": "DATE", "4": "MONEY", "5": "PERCENT", "6": "TIME", "7": "CAUSE_OF_DEATH", "8": "CITY", "9": "COUNTRY", "10": "CRIMINAL_CHARGE", "11": "EMAIL", "12": "HANDLE", "13": "IDEOLOGY", "14": "NATIONALITY", "15": "RELIGION", "16": "STATE_OR_PROVINCE", "17": "TITLE", "18": "URL", "19": "NUMBER", "20": "ORDINAL", "21": "MISC", "22": "DURATION", "23": "O"}}}}, {"name": "obj_start", "dtype": "int32"}, {"name": "obj_end", "dtype": "int32"}, {"name": "obj_type", "dtype": {"class_label": {"names": {"0": "LOCATION", "1": "ORGANIZATION", "2": "PERSON", "3": "DATE", "4": "MONEY", "5": "PERCENT", "6": "TIME", "7": "CAUSE_OF_DEATH", "8": "CITY", "9": "COUNTRY", "10": "CRIMINAL_CHARGE", "11": "EMAIL", "12": "HANDLE", "13": "IDEOLOGY", "14": "NATIONALITY", "15": "RELIGION", "16": "STATE_OR_PROVINCE", "17": "TITLE", "18": "URL", "19": "NUMBER", "20": "ORDINAL", "21": "MISC", "22": "DURATION", "23": "O"}}}}, {"name": "relation", "dtype": {"class_label": {"names": {"0": "no_relation", "1": "org:alternate_names", "2": "org:city_of_branch", "3": "org:country_of_branch", "4": "org:dissolved", "5": "org:founded", "6": "org:founded_by", "7": "org:member_of", "8": "org:members", "9": "org:number_of_employees/members", "10": "org:political/religious_affiliation", "11": "org:shareholders", "12": "org:stateorprovince_of_branch", "13": "org:top_members/employees", "14": "org:website", "15": "per:age", "16": "per:cause_of_death", "17": "per:charges", "18": "per:children", "19": "per:cities_of_residence", "20": "per:city_of_birth", "21": "per:city_of_death", "22": "per:countries_of_residence", "23": "per:country_of_birth", "24": "per:country_of_death", "25": "per:date_of_birth", "26": "per:date_of_death", "27": "per:employee_of", "28": "per:identity", "29": "per:origin", "30": "per:other_family", "31": "per:parents", "32": "per:religion", "33": "per:schools_attended", "34": "per:siblings", "35": "per:spouse", "36": "per:stateorprovince_of_birth", "37": "per:stateorprovince_of_death", "38": "per:stateorprovinces_of_residence", "39": "per:title"}}}}], "splits": [{"name": "train", "num_bytes": 23707989, "num_examples": 55998}, {"name": "test", "num_bytes": 5139146, "num_examples": 12907}, {"name": "validation", "num_bytes": 7711621, "num_examples": 18788}, {"name": "backtranslated_test", "num_bytes": 4676107, "num_examples": 12722}], "download_size": 3702157, "dataset_size": 41234863}, {"config_name": "original-fi", "features": [{"name": "id", "dtype": "string"}, {"name": "token", "sequence": "string"}, {"name": "subj_start", "dtype": "int32"}, {"name": "subj_end", "dtype": "int32"}, {"name": "subj_type", "dtype": {"class_label": {"names": {"0": "LOCATION", "1": "ORGANIZATION", "2": "PERSON", "3": "DATE", "4": "MONEY", "5": "PERCENT", "6": "TIME", "7": "CAUSE_OF_DEATH", "8": "CITY", "9": "COUNTRY", "10": "CRIMINAL_CHARGE", "11": "EMAIL", "12": "HANDLE", "13": "IDEOLOGY", "14": "NATIONALITY", "15": "RELIGION", "16": "STATE_OR_PROVINCE", "17": "TITLE", "18": "URL", "19": "NUMBER", "20": "ORDINAL", "21": "MISC", "22": "DURATION", "23": "O"}}}}, {"name": "obj_start", "dtype": "int32"}, {"name": "obj_end", "dtype": "int32"}, {"name": "obj_type", "dtype": {"class_label": {"names": {"0": "LOCATION", "1": "ORGANIZATION", "2": "PERSON", "3": "DATE", "4": "MONEY", "5": "PERCENT", "6": "TIME", "7": "CAUSE_OF_DEATH", "8": "CITY", "9": "COUNTRY", "10": "CRIMINAL_CHARGE", "11": "EMAIL", "12": "HANDLE", "13": "IDEOLOGY", "14": "NATIONALITY", "15": "RELIGION", "16": "STATE_OR_PROVINCE", "17": "TITLE", "18": "URL", "19": "NUMBER", "20": "ORDINAL", "21": "MISC", "22": "DURATION", "23": "O"}}}}, {"name": "relation", "dtype": {"class_label": {"names": {"0": "no_relation", "1": "org:alternate_names", "2": "org:city_of_headquarters", "3": "org:country_of_headquarters", "4": "org:dissolved", "5": "org:founded", "6": "org:founded_by", "7": "org:member_of", "8": "org:members", "9": "org:number_of_employees/members", "10": "org:parents", "11": "org:political/religious_affiliation", "12": "org:shareholders", "13": "org:stateorprovince_of_headquarters", "14": "org:subsidiaries", "15": "org:top_members/employees", "16": "org:website", "17": "per:age", "18": "per:alternate_names", "19": "per:cause_of_death", "20": "per:charges", "21": "per:children", "22": "per:cities_of_residence", "23": "per:city_of_birth", "24": "per:city_of_death", "25": "per:countries_of_residence", "26": "per:country_of_birth", "27": "per:country_of_death", "28": "per:date_of_birth", "29": "per:date_of_death", "30": "per:employee_of", "31": "per:origin", "32": "per:other_family", "33": "per:parents", "34": "per:religion", "35": "per:schools_attended", "36": "per:siblings", "37": "per:spouse", "38": "per:stateorprovince_of_birth", "39": "per:stateorprovince_of_death", "40": "per:stateorprovinces_of_residence", "41": "per:title"}}}}], "splits": [{"name": "train", "num_bytes": 25394979, "num_examples": 66751}, {"name": "test", "num_bytes": 5478260, "num_examples": 15083}, {"name": "validation", "num_bytes": 8205629, "num_examples": 22268}, {"name": "backtranslated_test", "num_bytes": 5204235, "num_examples": 14462}], "download_size": 0, "dataset_size": 44283103}, {"config_name": "revisited-fi", "features": [{"name": "id", "dtype": "string"}, {"name": "token", "sequence": "string"}, {"name": "subj_start", "dtype": "int32"}, {"name": "subj_end", "dtype": "int32"}, {"name": "subj_type", "dtype": {"class_label": {"names": {"0": "LOCATION", "1": "ORGANIZATION", "2": "PERSON", "3": "DATE", "4": "MONEY", "5": "PERCENT", "6": "TIME", "7": "CAUSE_OF_DEATH", "8": "CITY", "9": "COUNTRY", "10": "CRIMINAL_CHARGE", "11": "EMAIL", "12": "HANDLE", "13": "IDEOLOGY", "14": "NATIONALITY", "15": "RELIGION", "16": "STATE_OR_PROVINCE", "17": "TITLE", "18": "URL", "19": "NUMBER", "20": "ORDINAL", "21": "MISC", "22": "DURATION", "23": "O"}}}}, {"name": "obj_start", "dtype": "int32"}, {"name": "obj_end", "dtype": "int32"}, {"name": "obj_type", "dtype": {"class_label": {"names": {"0": "LOCATION", "1": "ORGANIZATION", "2": "PERSON", "3": "DATE", "4": "MONEY", "5": "PERCENT", "6": "TIME", "7": "CAUSE_OF_DEATH", "8": "CITY", "9": "COUNTRY", "10": "CRIMINAL_CHARGE", "11": "EMAIL", "12": "HANDLE", "13": "IDEOLOGY", "14": "NATIONALITY", "15": "RELIGION", "16": "STATE_OR_PROVINCE", "17": "TITLE", "18": "URL", "19": "NUMBER", "20": "ORDINAL", "21": "MISC", "22": "DURATION", "23": "O"}}}}, {"name": "relation", "dtype": {"class_label": {"names": {"0": "no_relation", "1": "org:alternate_names", "2": "org:city_of_headquarters", "3": "org:country_of_headquarters", "4": "org:dissolved", "5": "org:founded", "6": "org:founded_by", "7": "org:member_of", "8": "org:members", "9": "org:number_of_employees/members", "10": "org:parents", "11": "org:political/religious_affiliation", "12": "org:shareholders", "13": "org:stateorprovince_of_headquarters", "14": "org:subsidiaries", "15": "org:top_members/employees", "16": "org:website", "17": "per:age", "18": "per:alternate_names", "19": "per:cause_of_death", "20": "per:charges", "21": "per:children", "22": "per:cities_of_residence", "23": "per:city_of_birth", "24": "per:city_of_death", "25": "per:countries_of_residence", "26": "per:country_of_birth", "27": "per:country_of_death", "28": "per:date_of_birth", "29": "per:date_of_death", "30": "per:employee_of", "31": "per:origin", "32": "per:other_family", "33": "per:parents", "34": "per:religion", "35": "per:schools_attended", "36": "per:siblings", "37": "per:spouse", "38": "per:stateorprovince_of_birth", "39": "per:stateorprovince_of_death", "40": "per:stateorprovinces_of_residence", "41": "per:title"}}}}], "splits": [{"name": "train", "num_bytes": 25394979, "num_examples": 66751}, {"name": "test", "num_bytes": 5478260, "num_examples": 15083}, {"name": "validation", "num_bytes": 8205629, "num_examples": 22268}, {"name": "backtranslated_test", "num_bytes": 5204235, "num_examples": 14462}], "download_size": 157165, "dataset_size": 44283103}, {"config_name": "retacred-fi", "features": [{"name": "id", "dtype": "string"}, {"name": "token", "sequence": "string"}, {"name": "subj_start", "dtype": "int32"}, {"name": "subj_end", "dtype": "int32"}, {"name": "subj_type", "dtype": {"class_label": {"names": {"0": "LOCATION", "1": "ORGANIZATION", "2": "PERSON", "3": "DATE", "4": "MONEY", "5": "PERCENT", "6": "TIME", "7": "CAUSE_OF_DEATH", "8": "CITY", "9": "COUNTRY", "10": "CRIMINAL_CHARGE", "11": "EMAIL", "12": "HANDLE", "13": "IDEOLOGY", "14": "NATIONALITY", "15": "RELIGION", "16": "STATE_OR_PROVINCE", "17": "TITLE", "18": "URL", "19": "NUMBER", "20": "ORDINAL", "21": "MISC", "22": "DURATION", "23": "O"}}}}, {"name": "obj_start", "dtype": "int32"}, {"name": "obj_end", "dtype": "int32"}, {"name": "obj_type", "dtype": {"class_label": {"names": {"0": "LOCATION", "1": "ORGANIZATION", "2": "PERSON", "3": "DATE", "4": "MONEY", "5": "PERCENT", "6": "TIME", "7": "CAUSE_OF_DEATH", "8": "CITY", "9": "COUNTRY", "10": "CRIMINAL_CHARGE", "11": "EMAIL", "12": "HANDLE", "13": "IDEOLOGY", "14": "NATIONALITY", "15": "RELIGION", "16": "STATE_OR_PROVINCE", "17": "TITLE", "18": "URL", "19": "NUMBER", "20": "ORDINAL", "21": "MISC", "22": "DURATION", "23": "O"}}}}, {"name": "relation", "dtype": {"class_label": {"names": {"0": "no_relation", "1": "org:alternate_names", "2": "org:city_of_branch", "3": "org:country_of_branch", "4": "org:dissolved", "5": "org:founded", "6": "org:founded_by", "7": "org:member_of", "8": "org:members", "9": "org:number_of_employees/members", "10": "org:political/religious_affiliation", "11": "org:shareholders", "12": "org:stateorprovince_of_branch", "13": "org:top_members/employees", "14": "org:website", "15": "per:age", "16": "per:cause_of_death", "17": "per:charges", "18": "per:children", "19": "per:cities_of_residence", "20": "per:city_of_birth", "21": "per:city_of_death", "22": "per:countries_of_residence", "23": "per:country_of_birth", "24": "per:country_of_death", "25": "per:date_of_birth", "26": "per:date_of_death", "27": "per:employee_of", "28": "per:identity", "29": "per:origin", "30": "per:other_family", "31": "per:parents", "32": "per:religion", "33": "per:schools_attended", "34": "per:siblings", "35": "per:spouse", "36": "per:stateorprovince_of_birth", "37": "per:stateorprovince_of_death", "38": "per:stateorprovinces_of_residence", "39": "per:title"}}}}], "splits": [{"name": "train", "num_bytes": 21807425, "num_examples": 57332}, {"name": "test", "num_bytes": 4724204, "num_examples": 13046}, {"name": "validation", "num_bytes": 7084020, "num_examples": 19278}, {"name": "backtranslated_test", "num_bytes": 4475178, "num_examples": 12480}], "download_size": 3702157, "dataset_size": 38090827}, {"config_name": "original-fr", "features": [{"name": "id", "dtype": "string"}, {"name": "token", "sequence": "string"}, {"name": "subj_start", "dtype": "int32"}, {"name": "subj_end", "dtype": "int32"}, {"name": "subj_type", "dtype": {"class_label": {"names": {"0": "LOCATION", "1": "ORGANIZATION", "2": "PERSON", "3": "DATE", "4": "MONEY", "5": "PERCENT", "6": "TIME", "7": "CAUSE_OF_DEATH", "8": "CITY", "9": "COUNTRY", "10": "CRIMINAL_CHARGE", "11": "EMAIL", "12": "HANDLE", "13": "IDEOLOGY", "14": "NATIONALITY", "15": "RELIGION", "16": "STATE_OR_PROVINCE", "17": "TITLE", "18": "URL", "19": "NUMBER", "20": "ORDINAL", "21": "MISC", "22": "DURATION", "23": "O"}}}}, {"name": "obj_start", "dtype": "int32"}, {"name": "obj_end", "dtype": "int32"}, {"name": "obj_type", "dtype": {"class_label": {"names": {"0": "LOCATION", "1": "ORGANIZATION", "2": "PERSON", "3": "DATE", "4": "MONEY", "5": "PERCENT", "6": "TIME", "7": "CAUSE_OF_DEATH", "8": "CITY", "9": "COUNTRY", "10": "CRIMINAL_CHARGE", "11": "EMAIL", "12": "HANDLE", "13": "IDEOLOGY", "14": "NATIONALITY", "15": "RELIGION", "16": "STATE_OR_PROVINCE", "17": "TITLE", "18": "URL", "19": "NUMBER", "20": "ORDINAL", "21": "MISC", "22": "DURATION", "23": "O"}}}}, {"name": "relation", "dtype": {"class_label": {"names": {"0": "no_relation", "1": "org:alternate_names", "2": "org:city_of_headquarters", "3": "org:country_of_headquarters", "4": "org:dissolved", "5": "org:founded", "6": "org:founded_by", "7": "org:member_of", "8": "org:members", "9": "org:number_of_employees/members", "10": "org:parents", "11": "org:political/religious_affiliation", "12": "org:shareholders", "13": "org:stateorprovince_of_headquarters", "14": "org:subsidiaries", "15": "org:top_members/employees", "16": "org:website", "17": "per:age", "18": "per:alternate_names", "19": "per:cause_of_death", "20": "per:charges", "21": "per:children", "22": "per:cities_of_residence", "23": "per:city_of_birth", "24": "per:city_of_death", "25": "per:countries_of_residence", "26": "per:country_of_birth", "27": "per:country_of_death", "28": "per:date_of_birth", "29": "per:date_of_death", "30": "per:employee_of", "31": "per:origin", "32": "per:other_family", "33": "per:parents", "34": "per:religion", "35": "per:schools_attended", "36": "per:siblings", "37": "per:spouse", "38": "per:stateorprovince_of_birth", "39": "per:stateorprovince_of_death", "40": "per:stateorprovinces_of_residence", "41": "per:title"}}}}], "splits": [{"name": "train", "num_bytes": 29580179, "num_examples": 66856}, {"name": "test", "num_bytes": 6409145, "num_examples": 15237}, {"name": "validation", "num_bytes": 9601199, "num_examples": 22298}, {"name": "backtranslated_test", "num_bytes": 5535658, "num_examples": 15088}], "download_size": 0, "dataset_size": 51126181}, {"config_name": "revisited-fr", "features": [{"name": "id", "dtype": "string"}, {"name": "token", "sequence": "string"}, {"name": "subj_start", "dtype": "int32"}, {"name": "subj_end", "dtype": "int32"}, {"name": "subj_type", "dtype": {"class_label": {"names": {"0": "LOCATION", "1": "ORGANIZATION", "2": "PERSON", "3": "DATE", "4": "MONEY", "5": "PERCENT", "6": "TIME", "7": "CAUSE_OF_DEATH", "8": "CITY", "9": "COUNTRY", "10": "CRIMINAL_CHARGE", "11": "EMAIL", "12": "HANDLE", "13": "IDEOLOGY", "14": "NATIONALITY", "15": "RELIGION", "16": "STATE_OR_PROVINCE", "17": "TITLE", "18": "URL", "19": "NUMBER", "20": "ORDINAL", "21": "MISC", "22": "DURATION", "23": "O"}}}}, {"name": "obj_start", "dtype": "int32"}, {"name": "obj_end", "dtype": "int32"}, {"name": "obj_type", "dtype": {"class_label": {"names": {"0": "LOCATION", "1": "ORGANIZATION", "2": "PERSON", "3": "DATE", "4": "MONEY", "5": "PERCENT", "6": "TIME", "7": "CAUSE_OF_DEATH", "8": "CITY", "9": "COUNTRY", "10": "CRIMINAL_CHARGE", "11": "EMAIL", "12": "HANDLE", "13": "IDEOLOGY", "14": "NATIONALITY", "15": "RELIGION", "16": "STATE_OR_PROVINCE", "17": "TITLE", "18": "URL", "19": "NUMBER", "20": "ORDINAL", "21": "MISC", "22": "DURATION", "23": "O"}}}}, {"name": "relation", "dtype": {"class_label": {"names": {"0": "no_relation", "1": "org:alternate_names", "2": "org:city_of_headquarters", "3": "org:country_of_headquarters", "4": "org:dissolved", "5": "org:founded", "6": "org:founded_by", "7": "org:member_of", "8": "org:members", "9": "org:number_of_employees/members", "10": "org:parents", "11": "org:political/religious_affiliation", "12": "org:shareholders", "13": "org:stateorprovince_of_headquarters", "14": "org:subsidiaries", "15": "org:top_members/employees", "16": "org:website", "17": "per:age", "18": "per:alternate_names", "19": "per:cause_of_death", "20": "per:charges", "21": "per:children", "22": "per:cities_of_residence", "23": "per:city_of_birth", "24": "per:city_of_death", "25": "per:countries_of_residence", "26": "per:country_of_birth", "27": "per:country_of_death", "28": "per:date_of_birth", "29": "per:date_of_death", "30": "per:employee_of", "31": "per:origin", "32": "per:other_family", "33": "per:parents", "34": "per:religion", "35": "per:schools_attended", "36": "per:siblings", "37": "per:spouse", "38": "per:stateorprovince_of_birth", "39": "per:stateorprovince_of_death", "40": "per:stateorprovinces_of_residence", "41": "per:title"}}}}], "splits": [{"name": "train", "num_bytes": 29580179, "num_examples": 66856}, {"name": "test", "num_bytes": 6409145, "num_examples": 15237}, {"name": "validation", "num_bytes": 9601199, "num_examples": 22298}, {"name": "backtranslated_test", "num_bytes": 5535658, "num_examples": 15088}], "download_size": 157165, "dataset_size": 51126181}, {"config_name": "retacred-fr", "features": [{"name": "id", "dtype": "string"}, {"name": "token", "sequence": "string"}, {"name": "subj_start", "dtype": "int32"}, {"name": "subj_end", "dtype": "int32"}, {"name": "subj_type", "dtype": {"class_label": {"names": {"0": "LOCATION", "1": "ORGANIZATION", "2": "PERSON", "3": "DATE", "4": "MONEY", "5": "PERCENT", "6": "TIME", "7": "CAUSE_OF_DEATH", "8": "CITY", "9": "COUNTRY", "10": "CRIMINAL_CHARGE", "11": "EMAIL", "12": "HANDLE", "13": "IDEOLOGY", "14": "NATIONALITY", "15": "RELIGION", "16": "STATE_OR_PROVINCE", "17": "TITLE", "18": "URL", "19": "NUMBER", "20": "ORDINAL", "21": "MISC", "22": "DURATION", "23": "O"}}}}, {"name": "obj_start", "dtype": "int32"}, {"name": "obj_end", "dtype": "int32"}, {"name": "obj_type", "dtype": {"class_label": {"names": {"0": "LOCATION", "1": "ORGANIZATION", "2": "PERSON", "3": "DATE", "4": "MONEY", "5": "PERCENT", "6": "TIME", "7": "CAUSE_OF_DEATH", "8": "CITY", "9": "COUNTRY", "10": "CRIMINAL_CHARGE", "11": "EMAIL", "12": "HANDLE", "13": "IDEOLOGY", "14": "NATIONALITY", "15": "RELIGION", "16": "STATE_OR_PROVINCE", "17": "TITLE", "18": "URL", "19": "NUMBER", "20": "ORDINAL", "21": "MISC", "22": "DURATION", "23": "O"}}}}, {"name": "relation", "dtype": {"class_label": {"names": {"0": "no_relation", "1": "org:alternate_names", "2": "org:city_of_branch", "3": "org:country_of_branch", "4": "org:dissolved", "5": "org:founded", "6": "org:founded_by", "7": "org:member_of", "8": "org:members", "9": "org:number_of_employees/members", "10": "org:political/religious_affiliation", "11": "org:shareholders", "12": "org:stateorprovince_of_branch", "13": "org:top_members/employees", "14": "org:website", "15": "per:age", "16": "per:cause_of_death", "17": "per:charges", "18": "per:children", "19": "per:cities_of_residence", "20": "per:city_of_birth", "21": "per:city_of_death", "22": "per:countries_of_residence", "23": "per:country_of_birth", "24": "per:country_of_death", "25": "per:date_of_birth", "26": "per:date_of_death", "27": "per:employee_of", "28": "per:identity", "29": "per:origin", "30": "per:other_family", "31": "per:parents", "32": "per:religion", "33": "per:schools_attended", "34": "per:siblings", "35": "per:spouse", "36": "per:stateorprovince_of_birth", "37": "per:stateorprovince_of_death", "38": "per:stateorprovinces_of_residence", "39": "per:title"}}}}], "splits": [{"name": "train", "num_bytes": 25484188, "num_examples": 57466}, {"name": "test", "num_bytes": 5553110, "num_examples": 13209}, {"name": "validation", "num_bytes": 8323210, "num_examples": 19341}, {"name": "backtranslated_test", "num_bytes": 4786142, "num_examples": 13078}], "download_size": 3702157, "dataset_size": 44146650}, {"config_name": "original-hi", "features": [{"name": "id", "dtype": "string"}, {"name": "token", "sequence": "string"}, {"name": "subj_start", "dtype": "int32"}, {"name": "subj_end", "dtype": "int32"}, {"name": "subj_type", "dtype": {"class_label": {"names": {"0": "LOCATION", "1": "ORGANIZATION", "2": "PERSON", "3": "DATE", "4": "MONEY", "5": "PERCENT", "6": "TIME", "7": "CAUSE_OF_DEATH", "8": "CITY", "9": "COUNTRY", "10": "CRIMINAL_CHARGE", "11": "EMAIL", "12": "HANDLE", "13": "IDEOLOGY", "14": "NATIONALITY", "15": "RELIGION", "16": "STATE_OR_PROVINCE", "17": "TITLE", "18": "URL", "19": "NUMBER", "20": "ORDINAL", "21": "MISC", "22": "DURATION", "23": "O"}}}}, {"name": "obj_start", "dtype": "int32"}, {"name": "obj_end", "dtype": "int32"}, {"name": "obj_type", "dtype": {"class_label": {"names": {"0": "LOCATION", "1": "ORGANIZATION", "2": "PERSON", "3": "DATE", "4": "MONEY", "5": "PERCENT", "6": "TIME", "7": "CAUSE_OF_DEATH", "8": "CITY", "9": "COUNTRY", "10": "CRIMINAL_CHARGE", "11": "EMAIL", "12": "HANDLE", "13": "IDEOLOGY", "14": "NATIONALITY", "15": "RELIGION", "16": "STATE_OR_PROVINCE", "17": "TITLE", "18": "URL", "19": "NUMBER", "20": "ORDINAL", "21": "MISC", "22": "DURATION", "23": "O"}}}}, {"name": "relation", "dtype": {"class_label": {"names": {"0": "no_relation", "1": "org:alternate_names", "2": "org:city_of_headquarters", "3": "org:country_of_headquarters", "4": "org:dissolved", "5": "org:founded", "6": "org:founded_by", "7": "org:member_of", "8": "org:members", "9": "org:number_of_employees/members", "10": "org:parents", "11": "org:political/religious_affiliation", "12": "org:shareholders", "13": "org:stateorprovince_of_headquarters", "14": "org:subsidiaries", "15": "org:top_members/employees", "16": "org:website", "17": "per:age", "18": "per:alternate_names", "19": "per:cause_of_death", "20": "per:charges", "21": "per:children", "22": "per:cities_of_residence", "23": "per:city_of_birth", "24": "per:city_of_death", "25": "per:countries_of_residence", "26": "per:country_of_birth", "27": "per:country_of_death", "28": "per:date_of_birth", "29": "per:date_of_death", "30": "per:employee_of", "31": "per:origin", "32": "per:other_family", "33": "per:parents", "34": "per:religion", "35": "per:schools_attended", "36": "per:siblings", "37": "per:spouse", "38": "per:stateorprovince_of_birth", "39": "per:stateorprovince_of_death", "40": "per:stateorprovinces_of_residence", "41": "per:title"}}}}], "splits": [{"name": "train", "num_bytes": 47358490, "num_examples": 67751}, {"name": "test", "num_bytes": 10235547, "num_examples": 15440}, {"name": "validation", "num_bytes": 15362616, "num_examples": 22511}, {"name": "backtranslated_test", "num_bytes": 5654198, "num_examples": 15440}], "download_size": 0, "dataset_size": 78610851}, {"config_name": "revisited-hi", "features": [{"name": "id", "dtype": "string"}, {"name": "token", "sequence": "string"}, {"name": "subj_start", "dtype": "int32"}, {"name": "subj_end", "dtype": "int32"}, {"name": "subj_type", "dtype": {"class_label": {"names": {"0": "LOCATION", "1": "ORGANIZATION", "2": "PERSON", "3": "DATE", "4": "MONEY", "5": "PERCENT", "6": "TIME", "7": "CAUSE_OF_DEATH", "8": "CITY", "9": "COUNTRY", "10": "CRIMINAL_CHARGE", "11": "EMAIL", "12": "HANDLE", "13": "IDEOLOGY", "14": "NATIONALITY", "15": "RELIGION", "16": "STATE_OR_PROVINCE", "17": "TITLE", "18": "URL", "19": "NUMBER", "20": "ORDINAL", "21": "MISC", "22": "DURATION", "23": "O"}}}}, {"name": "obj_start", "dtype": "int32"}, {"name": "obj_end", "dtype": "int32"}, {"name": "obj_type", "dtype": {"class_label": {"names": {"0": "LOCATION", "1": "ORGANIZATION", "2": "PERSON", "3": "DATE", "4": "MONEY", "5": "PERCENT", "6": "TIME", "7": "CAUSE_OF_DEATH", "8": "CITY", "9": "COUNTRY", "10": "CRIMINAL_CHARGE", "11": "EMAIL", "12": "HANDLE", "13": "IDEOLOGY", "14": "NATIONALITY", "15": "RELIGION", "16": "STATE_OR_PROVINCE", "17": "TITLE", "18": "URL", "19": "NUMBER", "20": "ORDINAL", "21": "MISC", "22": "DURATION", "23": "O"}}}}, {"name": "relation", "dtype": {"class_label": {"names": {"0": "no_relation", "1": "org:alternate_names", "2": "org:city_of_headquarters", "3": "org:country_of_headquarters", "4": "org:dissolved", "5": "org:founded", "6": "org:founded_by", "7": "org:member_of", "8": "org:members", "9": "org:number_of_employees/members", "10": "org:parents", "11": "org:political/religious_affiliation", "12": "org:shareholders", "13": "org:stateorprovince_of_headquarters", "14": "org:subsidiaries", "15": "org:top_members/employees", "16": "org:website", "17": "per:age", "18": "per:alternate_names", "19": "per:cause_of_death", "20": "per:charges", "21": "per:children", "22": "per:cities_of_residence", "23": "per:city_of_birth", "24": "per:city_of_death", "25": "per:countries_of_residence", "26": "per:country_of_birth", "27": "per:country_of_death", "28": "per:date_of_birth", "29": "per:date_of_death", "30": "per:employee_of", "31": "per:origin", "32": "per:other_family", "33": "per:parents", "34": "per:religion", "35": "per:schools_attended", "36": "per:siblings", "37": "per:spouse", "38": "per:stateorprovince_of_birth", "39": "per:stateorprovince_of_death", "40": "per:stateorprovinces_of_residence", "41": "per:title"}}}}], "splits": [{"name": "train", "num_bytes": 47358490, "num_examples": 67751}, {"name": "test", "num_bytes": 10235547, "num_examples": 15440}, {"name": "validation", "num_bytes": 15362616, "num_examples": 22511}, {"name": "backtranslated_test", "num_bytes": 5654198, "num_examples": 15440}], "download_size": 157165, "dataset_size": 78610851}, {"config_name": "retacred-hi", "features": [{"name": "id", "dtype": "string"}, {"name": "token", "sequence": "string"}, {"name": "subj_start", "dtype": "int32"}, {"name": "subj_end", "dtype": "int32"}, {"name": "subj_type", "dtype": {"class_label": {"names": {"0": "LOCATION", "1": "ORGANIZATION", "2": "PERSON", "3": "DATE", "4": "MONEY", "5": "PERCENT", "6": "TIME", "7": "CAUSE_OF_DEATH", "8": "CITY", "9": "COUNTRY", "10": "CRIMINAL_CHARGE", "11": "EMAIL", "12": "HANDLE", "13": "IDEOLOGY", "14": "NATIONALITY", "15": "RELIGION", "16": "STATE_OR_PROVINCE", "17": "TITLE", "18": "URL", "19": "NUMBER", "20": "ORDINAL", "21": "MISC", "22": "DURATION", "23": "O"}}}}, {"name": "obj_start", "dtype": "int32"}, {"name": "obj_end", "dtype": "int32"}, {"name": "obj_type", "dtype": {"class_label": {"names": {"0": "LOCATION", "1": "ORGANIZATION", "2": "PERSON", "3": "DATE", "4": "MONEY", "5": "PERCENT", "6": "TIME", "7": "CAUSE_OF_DEATH", "8": "CITY", "9": "COUNTRY", "10": "CRIMINAL_CHARGE", "11": "EMAIL", "12": "HANDLE", "13": "IDEOLOGY", "14": "NATIONALITY", "15": "RELIGION", "16": "STATE_OR_PROVINCE", "17": "TITLE", "18": "URL", "19": "NUMBER", "20": "ORDINAL", "21": "MISC", "22": "DURATION", "23": "O"}}}}, {"name": "relation", "dtype": {"class_label": {"names": {"0": "no_relation", "1": "org:alternate_names", "2": "org:city_of_branch", "3": "org:country_of_branch", "4": "org:dissolved", "5": "org:founded", "6": "org:founded_by", "7": "org:member_of", "8": "org:members", "9": "org:number_of_employees/members", "10": "org:political/religious_affiliation", "11": "org:shareholders", "12": "org:stateorprovince_of_branch", "13": "org:top_members/employees", "14": "org:website", "15": "per:age", "16": "per:cause_of_death", "17": "per:charges", "18": "per:children", "19": "per:cities_of_residence", "20": "per:city_of_birth", "21": "per:city_of_death", "22": "per:countries_of_residence", "23": "per:country_of_birth", "24": "per:country_of_death", "25": "per:date_of_birth", "26": "per:date_of_death", "27": "per:employee_of", "28": "per:identity", "29": "per:origin", "30": "per:other_family", "31": "per:parents", "32": "per:religion", "33": "per:schools_attended", "34": "per:siblings", "35": "per:spouse", "36": "per:stateorprovince_of_birth", "37": "per:stateorprovince_of_death", "38": "per:stateorprovinces_of_residence", "39": "per:title"}}}}], "splits": [{"name": "train", "num_bytes": 40764637, "num_examples": 58186}, {"name": "test", "num_bytes": 8839508, "num_examples": 13363}, {"name": "validation", "num_bytes": 13280435, "num_examples": 19488}, {"name": "backtranslated_test", "num_bytes": 4878649, "num_examples": 13363}], "download_size": 3702157, "dataset_size": 67763229}, {"config_name": "original-hu", "features": [{"name": "id", "dtype": "string"}, {"name": "token", "sequence": "string"}, {"name": "subj_start", "dtype": "int32"}, {"name": "subj_end", "dtype": "int32"}, {"name": "subj_type", "dtype": {"class_label": {"names": {"0": "LOCATION", "1": "ORGANIZATION", "2": "PERSON", "3": "DATE", "4": "MONEY", "5": "PERCENT", "6": "TIME", "7": "CAUSE_OF_DEATH", "8": "CITY", "9": "COUNTRY", "10": "CRIMINAL_CHARGE", "11": "EMAIL", "12": "HANDLE", "13": "IDEOLOGY", "14": "NATIONALITY", "15": "RELIGION", "16": "STATE_OR_PROVINCE", "17": "TITLE", "18": "URL", "19": "NUMBER", "20": "ORDINAL", "21": "MISC", "22": "DURATION", "23": "O"}}}}, {"name": "obj_start", "dtype": "int32"}, {"name": "obj_end", "dtype": "int32"}, {"name": "obj_type", "dtype": {"class_label": {"names": {"0": "LOCATION", "1": "ORGANIZATION", "2": "PERSON", "3": "DATE", "4": "MONEY", "5": "PERCENT", "6": "TIME", "7": "CAUSE_OF_DEATH", "8": "CITY", "9": "COUNTRY", "10": "CRIMINAL_CHARGE", "11": "EMAIL", "12": "HANDLE", "13": "IDEOLOGY", "14": "NATIONALITY", "15": "RELIGION", "16": "STATE_OR_PROVINCE", "17": "TITLE", "18": "URL", "19": "NUMBER", "20": "ORDINAL", "21": "MISC", "22": "DURATION", "23": "O"}}}}, {"name": "relation", "dtype": {"class_label": {"names": {"0": "no_relation", "1": "org:alternate_names", "2": "org:city_of_headquarters", "3": "org:country_of_headquarters", "4": "org:dissolved", "5": "org:founded", "6": "org:founded_by", "7": "org:member_of", "8": "org:members", "9": "org:number_of_employees/members", "10": "org:parents", "11": "org:political/religious_affiliation", "12": "org:shareholders", "13": "org:stateorprovince_of_headquarters", "14": "org:subsidiaries", "15": "org:top_members/employees", "16": "org:website", "17": "per:age", "18": "per:alternate_names", "19": "per:cause_of_death", "20": "per:charges", "21": "per:children", "22": "per:cities_of_residence", "23": "per:city_of_birth", "24": "per:city_of_death", "25": "per:countries_of_residence", "26": "per:country_of_birth", "27": "per:country_of_death", "28": "per:date_of_birth", "29": "per:date_of_death", "30": "per:employee_of", "31": "per:origin", "32": "per:other_family", "33": "per:parents", "34": "per:religion", "35": "per:schools_attended", "36": "per:siblings", "37": "per:spouse", "38": "per:stateorprovince_of_birth", "39": "per:stateorprovince_of_death", "40": "per:stateorprovinces_of_residence", "41": "per:title"}}}}], "splits": [{"name": "train", "num_bytes": 26869925, "num_examples": 67766}, {"name": "test", "num_bytes": 5810768, "num_examples": 15436}, {"name": "validation", "num_bytes": 8658082, "num_examples": 22519}, {"name": "backtranslated_test", "num_bytes": 5695172, "num_examples": 15436}], "download_size": 0, "dataset_size": 47033947}, {"config_name": "revisited-hu", "features": [{"name": "id", "dtype": "string"}, {"name": "token", "sequence": "string"}, {"name": "subj_start", "dtype": "int32"}, {"name": "subj_end", "dtype": "int32"}, {"name": "subj_type", "dtype": {"class_label": {"names": {"0": "LOCATION", "1": "ORGANIZATION", "2": "PERSON", "3": "DATE", "4": "MONEY", "5": "PERCENT", "6": "TIME", "7": "CAUSE_OF_DEATH", "8": "CITY", "9": "COUNTRY", "10": "CRIMINAL_CHARGE", "11": "EMAIL", "12": "HANDLE", "13": "IDEOLOGY", "14": "NATIONALITY", "15": "RELIGION", "16": "STATE_OR_PROVINCE", "17": "TITLE", "18": "URL", "19": "NUMBER", "20": "ORDINAL", "21": "MISC", "22": "DURATION", "23": "O"}}}}, {"name": "obj_start", "dtype": "int32"}, {"name": "obj_end", "dtype": "int32"}, {"name": "obj_type", "dtype": {"class_label": {"names": {"0": "LOCATION", "1": "ORGANIZATION", "2": "PERSON", "3": "DATE", "4": "MONEY", "5": "PERCENT", "6": "TIME", "7": "CAUSE_OF_DEATH", "8": "CITY", "9": "COUNTRY", "10": "CRIMINAL_CHARGE", "11": "EMAIL", "12": "HANDLE", "13": "IDEOLOGY", "14": "NATIONALITY", "15": "RELIGION", "16": "STATE_OR_PROVINCE", "17": "TITLE", "18": "URL", "19": "NUMBER", "20": "ORDINAL", "21": "MISC", "22": "DURATION", "23": "O"}}}}, {"name": "relation", "dtype": {"class_label": {"names": {"0": "no_relation", "1": "org:alternate_names", "2": "org:city_of_headquarters", "3": "org:country_of_headquarters", "4": "org:dissolved", "5": "org:founded", "6": "org:founded_by", "7": "org:member_of", "8": "org:members", "9": "org:number_of_employees/members", "10": "org:parents", "11": "org:political/religious_affiliation", "12": "org:shareholders", "13": "org:stateorprovince_of_headquarters", "14": "org:subsidiaries", "15": "org:top_members/employees", "16": "org:website", "17": "per:age", "18": "per:alternate_names", "19": "per:cause_of_death", "20": "per:charges", "21": "per:children", "22": "per:cities_of_residence", "23": "per:city_of_birth", "24": "per:city_of_death", "25": "per:countries_of_residence", "26": "per:country_of_birth", "27": "per:country_of_death", "28": "per:date_of_birth", "29": "per:date_of_death", "30": "per:employee_of", "31": "per:origin", "32": "per:other_family", "33": "per:parents", "34": "per:religion", "35": "per:schools_attended", "36": "per:siblings", "37": "per:spouse", "38": "per:stateorprovince_of_birth", "39": "per:stateorprovince_of_death", "40": "per:stateorprovinces_of_residence", "41": "per:title"}}}}], "splits": [{"name": "train", "num_bytes": 26869925, "num_examples": 67766}, {"name": "test", "num_bytes": 5810768, "num_examples": 15436}, {"name": "validation", "num_bytes": 8658082, "num_examples": 22519}, {"name": "backtranslated_test", "num_bytes": 5695172, "num_examples": 15436}], "download_size": 157165, "dataset_size": 47033947}, {"config_name": "retacred-hu", "features": [{"name": "id", "dtype": "string"}, {"name": "token", "sequence": "string"}, {"name": "subj_start", "dtype": "int32"}, {"name": "subj_end", "dtype": "int32"}, {"name": "subj_type", "dtype": {"class_label": {"names": {"0": "LOCATION", "1": "ORGANIZATION", "2": "PERSON", "3": "DATE", "4": "MONEY", "5": "PERCENT", "6": "TIME", "7": "CAUSE_OF_DEATH", "8": "CITY", "9": "COUNTRY", "10": "CRIMINAL_CHARGE", "11": "EMAIL", "12": "HANDLE", "13": "IDEOLOGY", "14": "NATIONALITY", "15": "RELIGION", "16": "STATE_OR_PROVINCE", "17": "TITLE", "18": "URL", "19": "NUMBER", "20": "ORDINAL", "21": "MISC", "22": "DURATION", "23": "O"}}}}, {"name": "obj_start", "dtype": "int32"}, {"name": "obj_end", "dtype": "int32"}, {"name": "obj_type", "dtype": {"class_label": {"names": {"0": "LOCATION", "1": "ORGANIZATION", "2": "PERSON", "3": "DATE", "4": "MONEY", "5": "PERCENT", "6": "TIME", "7": "CAUSE_OF_DEATH", "8": "CITY", "9": "COUNTRY", "10": "CRIMINAL_CHARGE", "11": "EMAIL", "12": "HANDLE", "13": "IDEOLOGY", "14": "NATIONALITY", "15": "RELIGION", "16": "STATE_OR_PROVINCE", "17": "TITLE", "18": "URL", "19": "NUMBER", "20": "ORDINAL", "21": "MISC", "22": "DURATION", "23": "O"}}}}, {"name": "relation", "dtype": {"class_label": {"names": {"0": "no_relation", "1": "org:alternate_names", "2": "org:city_of_branch", "3": "org:country_of_branch", "4": "org:dissolved", "5": "org:founded", "6": "org:founded_by", "7": "org:member_of", "8": "org:members", "9": "org:number_of_employees/members", "10": "org:political/religious_affiliation", "11": "org:shareholders", "12": "org:stateorprovince_of_branch", "13": "org:top_members/employees", "14": "org:website", "15": "per:age", "16": "per:cause_of_death", "17": "per:charges", "18": "per:children", "19": "per:cities_of_residence", "20": "per:city_of_birth", "21": "per:city_of_death", "22": "per:countries_of_residence", "23": "per:country_of_birth", "24": "per:country_of_death", "25": "per:date_of_birth", "26": "per:date_of_death", "27": "per:employee_of", "28": "per:identity", "29": "per:origin", "30": "per:other_family", "31": "per:parents", "32": "per:religion", "33": "per:schools_attended", "34": "per:siblings", "35": "per:spouse", "36": "per:stateorprovince_of_birth", "37": "per:stateorprovince_of_death", "38": "per:stateorprovinces_of_residence", "39": "per:title"}}}}], "splits": [{"name": "train", "num_bytes": 23084933, "num_examples": 58200}, {"name": "test", "num_bytes": 5011087, "num_examples": 13357}, {"name": "validation", "num_bytes": 7476013, "num_examples": 19495}, {"name": "backtranslated_test", "num_bytes": 4912553, "num_examples": 13357}], "download_size": 3702157, "dataset_size": 40484586}, {"config_name": "original-ja", "features": [{"name": "id", "dtype": "string"}, {"name": "token", "sequence": "string"}, {"name": "subj_start", "dtype": "int32"}, {"name": "subj_end", "dtype": "int32"}, {"name": "subj_type", "dtype": {"class_label": {"names": {"0": "LOCATION", "1": "ORGANIZATION", "2": "PERSON", "3": "DATE", "4": "MONEY", "5": "PERCENT", "6": "TIME", "7": "CAUSE_OF_DEATH", "8": "CITY", "9": "COUNTRY", "10": "CRIMINAL_CHARGE", "11": "EMAIL", "12": "HANDLE", "13": "IDEOLOGY", "14": "NATIONALITY", "15": "RELIGION", "16": "STATE_OR_PROVINCE", "17": "TITLE", "18": "URL", "19": "NUMBER", "20": "ORDINAL", "21": "MISC", "22": "DURATION", "23": "O"}}}}, {"name": "obj_start", "dtype": "int32"}, {"name": "obj_end", "dtype": "int32"}, {"name": "obj_type", "dtype": {"class_label": {"names": {"0": "LOCATION", "1": "ORGANIZATION", "2": "PERSON", "3": "DATE", "4": "MONEY", "5": "PERCENT", "6": "TIME", "7": "CAUSE_OF_DEATH", "8": "CITY", "9": "COUNTRY", "10": "CRIMINAL_CHARGE", "11": "EMAIL", "12": "HANDLE", "13": "IDEOLOGY", "14": "NATIONALITY", "15": "RELIGION", "16": "STATE_OR_PROVINCE", "17": "TITLE", "18": "URL", "19": "NUMBER", "20": "ORDINAL", "21": "MISC", "22": "DURATION", "23": "O"}}}}, {"name": "relation", "dtype": {"class_label": {"names": {"0": "no_relation", "1": "org:alternate_names", "2": "org:city_of_headquarters", "3": "org:country_of_headquarters", "4": "org:dissolved", "5": "org:founded", "6": "org:founded_by", "7": "org:member_of", "8": "org:members", "9": "org:number_of_employees/members", "10": "org:parents", "11": "org:political/religious_affiliation", "12": "org:shareholders", "13": "org:stateorprovince_of_headquarters", "14": "org:subsidiaries", "15": "org:top_members/employees", "16": "org:website", "17": "per:age", "18": "per:alternate_names", "19": "per:cause_of_death", "20": "per:charges", "21": "per:children", "22": "per:cities_of_residence", "23": "per:city_of_birth", "24": "per:city_of_death", "25": "per:countries_of_residence", "26": "per:country_of_birth", "27": "per:country_of_death", "28": "per:date_of_birth", "29": "per:date_of_death", "30": "per:employee_of", "31": "per:origin", "32": "per:other_family", "33": "per:parents", "34": "per:religion", "35": "per:schools_attended", "36": "per:siblings", "37": "per:spouse", "38": "per:stateorprovince_of_birth", "39": "per:stateorprovince_of_death", "40": "per:stateorprovinces_of_residence", "41": "per:title"}}}}], "splits": [{"name": "train", "num_bytes": 31425001, "num_examples": 61571}, {"name": "test", "num_bytes": 6560885, "num_examples": 13701}, {"name": "validation", "num_bytes": 9996196, "num_examples": 20290}, {"name": "backtranslated_test", "num_bytes": 4706581, "num_examples": 12913}], "download_size": 0, "dataset_size": 52688663}, {"config_name": "revisited-ja", "features": [{"name": "id", "dtype": "string"}, {"name": "token", "sequence": "string"}, {"name": "subj_start", "dtype": "int32"}, {"name": "subj_end", "dtype": "int32"}, {"name": "subj_type", "dtype": {"class_label": {"names": {"0": "LOCATION", "1": "ORGANIZATION", "2": "PERSON", "3": "DATE", "4": "MONEY", "5": "PERCENT", "6": "TIME", "7": "CAUSE_OF_DEATH", "8": "CITY", "9": "COUNTRY", "10": "CRIMINAL_CHARGE", "11": "EMAIL", "12": "HANDLE", "13": "IDEOLOGY", "14": "NATIONALITY", "15": "RELIGION", "16": "STATE_OR_PROVINCE", "17": "TITLE", "18": "URL", "19": "NUMBER", "20": "ORDINAL", "21": "MISC", "22": "DURATION", "23": "O"}}}}, {"name": "obj_start", "dtype": "int32"}, {"name": "obj_end", "dtype": "int32"}, {"name": "obj_type", "dtype": {"class_label": {"names": {"0": "LOCATION", "1": "ORGANIZATION", "2": "PERSON", "3": "DATE", "4": "MONEY", "5": "PERCENT", "6": "TIME", "7": "CAUSE_OF_DEATH", "8": "CITY", "9": "COUNTRY", "10": "CRIMINAL_CHARGE", "11": "EMAIL", "12": "HANDLE", "13": "IDEOLOGY", "14": "NATIONALITY", "15": "RELIGION", "16": "STATE_OR_PROVINCE", "17": "TITLE", "18": "URL", "19": "NUMBER", "20": "ORDINAL", "21": "MISC", "22": "DURATION", "23": "O"}}}}, {"name": "relation", "dtype": {"class_label": {"names": {"0": "no_relation", "1": "org:alternate_names", "2": "org:city_of_headquarters", "3": "org:country_of_headquarters", "4": "org:dissolved", "5": "org:founded", "6": "org:founded_by", "7": "org:member_of", "8": "org:members", "9": "org:number_of_employees/members", "10": "org:parents", "11": "org:political/religious_affiliation", "12": "org:shareholders", "13": "org:stateorprovince_of_headquarters", "14": "org:subsidiaries", "15": "org:top_members/employees", "16": "org:website", "17": "per:age", "18": "per:alternate_names", "19": "per:cause_of_death", "20": "per:charges", "21": "per:children", "22": "per:cities_of_residence", "23": "per:city_of_birth", "24": "per:city_of_death", "25": "per:countries_of_residence", "26": "per:country_of_birth", "27": "per:country_of_death", "28": "per:date_of_birth", "29": "per:date_of_death", "30": "per:employee_of", "31": "per:origin", "32": "per:other_family", "33": "per:parents", "34": "per:religion", "35": "per:schools_attended", "36": "per:siblings", "37": "per:spouse", "38": "per:stateorprovince_of_birth", "39": "per:stateorprovince_of_death", "40": "per:stateorprovinces_of_residence", "41": "per:title"}}}}], "splits": [{"name": "train", "num_bytes": 31425001, "num_examples": 61571}, {"name": "test", "num_bytes": 6560885, "num_examples": 13701}, {"name": "validation", "num_bytes": 9996196, "num_examples": 20290}, {"name": "backtranslated_test", "num_bytes": 4706581, "num_examples": 12913}], "download_size": 157165, "dataset_size": 52688663}, {"config_name": "retacred-ja", "features": [{"name": "id", "dtype": "string"}, {"name": "token", "sequence": "string"}, {"name": "subj_start", "dtype": "int32"}, {"name": "subj_end", "dtype": "int32"}, {"name": "subj_type", "dtype": {"class_label": {"names": {"0": "LOCATION", "1": "ORGANIZATION", "2": "PERSON", "3": "DATE", "4": "MONEY", "5": "PERCENT", "6": "TIME", "7": "CAUSE_OF_DEATH", "8": "CITY", "9": "COUNTRY", "10": "CRIMINAL_CHARGE", "11": "EMAIL", "12": "HANDLE", "13": "IDEOLOGY", "14": "NATIONALITY", "15": "RELIGION", "16": "STATE_OR_PROVINCE", "17": "TITLE", "18": "URL", "19": "NUMBER", "20": "ORDINAL", "21": "MISC", "22": "DURATION", "23": "O"}}}}, {"name": "obj_start", "dtype": "int32"}, {"name": "obj_end", "dtype": "int32"}, {"name": "obj_type", "dtype": {"class_label": {"names": {"0": "LOCATION", "1": "ORGANIZATION", "2": "PERSON", "3": "DATE", "4": "MONEY", "5": "PERCENT", "6": "TIME", "7": "CAUSE_OF_DEATH", "8": "CITY", "9": "COUNTRY", "10": "CRIMINAL_CHARGE", "11": "EMAIL", "12": "HANDLE", "13": "IDEOLOGY", "14": "NATIONALITY", "15": "RELIGION", "16": "STATE_OR_PROVINCE", "17": "TITLE", "18": "URL", "19": "NUMBER", "20": "ORDINAL", "21": "MISC", "22": "DURATION", "23": "O"}}}}, {"name": "relation", "dtype": {"class_label": {"names": {"0": "no_relation", "1": "org:alternate_names", "2": "org:city_of_branch", "3": "org:country_of_branch", "4": "org:dissolved", "5": "org:founded", "6": "org:founded_by", "7": "org:member_of", "8": "org:members", "9": "org:number_of_employees/members", "10": "org:political/religious_affiliation", "11": "org:shareholders", "12": "org:stateorprovince_of_branch", "13": "org:top_members/employees", "14": "org:website", "15": "per:age", "16": "per:cause_of_death", "17": "per:charges", "18": "per:children", "19": "per:cities_of_residence", "20": "per:city_of_birth", "21": "per:city_of_death", "22": "per:countries_of_residence", "23": "per:country_of_birth", "24": "per:country_of_death", "25": "per:date_of_birth", "26": "per:date_of_death", "27": "per:employee_of", "28": "per:identity", "29": "per:origin", "30": "per:other_family", "31": "per:parents", "32": "per:religion", "33": "per:schools_attended", "34": "per:siblings", "35": "per:spouse", "36": "per:stateorprovince_of_birth", "37": "per:stateorprovince_of_death", "38": "per:stateorprovinces_of_residence", "39": "per:title"}}}}], "splits": [{"name": "train", "num_bytes": 26944316, "num_examples": 52748}, {"name": "test", "num_bytes": 5627890, "num_examples": 11815}, {"name": "validation", "num_bytes": 8591269, "num_examples": 17470}, {"name": "backtranslated_test", "num_bytes": 4032503, "num_examples": 11138}], "download_size": 3702157, "dataset_size": 45195978}, {"config_name": "original-pl", "features": [{"name": "id", "dtype": "string"}, {"name": "token", "sequence": "string"}, {"name": "subj_start", "dtype": "int32"}, {"name": "subj_end", "dtype": "int32"}, {"name": "subj_type", "dtype": {"class_label": {"names": {"0": "LOCATION", "1": "ORGANIZATION", "2": "PERSON", "3": "DATE", "4": "MONEY", "5": "PERCENT", "6": "TIME", "7": "CAUSE_OF_DEATH", "8": "CITY", "9": "COUNTRY", "10": "CRIMINAL_CHARGE", "11": "EMAIL", "12": "HANDLE", "13": "IDEOLOGY", "14": "NATIONALITY", "15": "RELIGION", "16": "STATE_OR_PROVINCE", "17": "TITLE", "18": "URL", "19": "NUMBER", "20": "ORDINAL", "21": "MISC", "22": "DURATION", "23": "O"}}}}, {"name": "obj_start", "dtype": "int32"}, {"name": "obj_end", "dtype": "int32"}, {"name": "obj_type", "dtype": {"class_label": {"names": {"0": "LOCATION", "1": "ORGANIZATION", "2": "PERSON", "3": "DATE", "4": "MONEY", "5": "PERCENT", "6": "TIME", "7": "CAUSE_OF_DEATH", "8": "CITY", "9": "COUNTRY", "10": "CRIMINAL_CHARGE", "11": "EMAIL", "12": "HANDLE", "13": "IDEOLOGY", "14": "NATIONALITY", "15": "RELIGION", "16": "STATE_OR_PROVINCE", "17": "TITLE", "18": "URL", "19": "NUMBER", "20": "ORDINAL", "21": "MISC", "22": "DURATION", "23": "O"}}}}, {"name": "relation", "dtype": {"class_label": {"names": {"0": "no_relation", "1": "org:alternate_names", "2": "org:city_of_headquarters", "3": "org:country_of_headquarters", "4": "org:dissolved", "5": "org:founded", "6": "org:founded_by", "7": "org:member_of", "8": "org:members", "9": "org:number_of_employees/members", "10": "org:parents", "11": "org:political/religious_affiliation", "12": "org:shareholders", "13": "org:stateorprovince_of_headquarters", "14": "org:subsidiaries", "15": "org:top_members/employees", "16": "org:website", "17": "per:age", "18": "per:alternate_names", "19": "per:cause_of_death", "20": "per:charges", "21": "per:children", "22": "per:cities_of_residence", "23": "per:city_of_birth", "24": "per:city_of_death", "25": "per:countries_of_residence", "26": "per:country_of_birth", "27": "per:country_of_death", "28": "per:date_of_birth", "29": "per:date_of_death", "30": "per:employee_of", "31": "per:origin", "32": "per:other_family", "33": "per:parents", "34": "per:religion", "35": "per:schools_attended", "36": "per:siblings", "37": "per:spouse", "38": "per:stateorprovince_of_birth", "39": "per:stateorprovince_of_death", "40": "per:stateorprovinces_of_residence", "41": "per:title"}}}}], "splits": [{"name": "train", "num_bytes": 26989666, "num_examples": 68124}, {"name": "test", "num_bytes": 5845988, "num_examples": 15509}, {"name": "validation", "num_bytes": 8728082, "num_examples": 22631}, {"name": "backtranslated_test", "num_bytes": 5594933, "num_examples": 15509}], "download_size": 0, "dataset_size": 47158669}, {"config_name": "revisited-pl", "features": [{"name": "id", "dtype": "string"}, {"name": "token", "sequence": "string"}, {"name": "subj_start", "dtype": "int32"}, {"name": "subj_end", "dtype": "int32"}, {"name": "subj_type", "dtype": {"class_label": {"names": {"0": "LOCATION", "1": "ORGANIZATION", "2": "PERSON", "3": "DATE", "4": "MONEY", "5": "PERCENT", "6": "TIME", "7": "CAUSE_OF_DEATH", "8": "CITY", "9": "COUNTRY", "10": "CRIMINAL_CHARGE", "11": "EMAIL", "12": "HANDLE", "13": "IDEOLOGY", "14": "NATIONALITY", "15": "RELIGION", "16": "STATE_OR_PROVINCE", "17": "TITLE", "18": "URL", "19": "NUMBER", "20": "ORDINAL", "21": "MISC", "22": "DURATION", "23": "O"}}}}, {"name": "obj_start", "dtype": "int32"}, {"name": "obj_end", "dtype": "int32"}, {"name": "obj_type", "dtype": {"class_label": {"names": {"0": "LOCATION", "1": "ORGANIZATION", "2": "PERSON", "3": "DATE", "4": "MONEY", "5": "PERCENT", "6": "TIME", "7": "CAUSE_OF_DEATH", "8": "CITY", "9": "COUNTRY", "10": "CRIMINAL_CHARGE", "11": "EMAIL", "12": "HANDLE", "13": "IDEOLOGY", "14": "NATIONALITY", "15": "RELIGION", "16": "STATE_OR_PROVINCE", "17": "TITLE", "18": "URL", "19": "NUMBER", "20": "ORDINAL", "21": "MISC", "22": "DURATION", "23": "O"}}}}, {"name": "relation", "dtype": {"class_label": {"names": {"0": "no_relation", "1": "org:alternate_names", "2": "org:city_of_headquarters", "3": "org:country_of_headquarters", "4": "org:dissolved", "5": "org:founded", "6": "org:founded_by", "7": "org:member_of", "8": "org:members", "9": "org:number_of_employees/members", "10": "org:parents", "11": "org:political/religious_affiliation", "12": "org:shareholders", "13": "org:stateorprovince_of_headquarters", "14": "org:subsidiaries", "15": "org:top_members/employees", "16": "org:website", "17": "per:age", "18": "per:alternate_names", "19": "per:cause_of_death", "20": "per:charges", "21": "per:children", "22": "per:cities_of_residence", "23": "per:city_of_birth", "24": "per:city_of_death", "25": "per:countries_of_residence", "26": "per:country_of_birth", "27": "per:country_of_death", "28": "per:date_of_birth", "29": "per:date_of_death", "30": "per:employee_of", "31": "per:origin", "32": "per:other_family", "33": "per:parents", "34": "per:religion", "35": "per:schools_attended", "36": "per:siblings", "37": "per:spouse", "38": "per:stateorprovince_of_birth", "39": "per:stateorprovince_of_death", "40": "per:stateorprovinces_of_residence", "41": "per:title"}}}}], "splits": [{"name": "train", "num_bytes": 26989666, "num_examples": 68124}, {"name": "test", "num_bytes": 5845988, "num_examples": 15509}, {"name": "validation", "num_bytes": 8728082, "num_examples": 22631}, {"name": "backtranslated_test", "num_bytes": 5594933, "num_examples": 15509}], "download_size": 157165, "dataset_size": 47158669}, {"config_name": "retacred-pl", "features": [{"name": "id", "dtype": "string"}, {"name": "token", "sequence": "string"}, {"name": "subj_start", "dtype": "int32"}, {"name": "subj_end", "dtype": "int32"}, {"name": "subj_type", "dtype": {"class_label": {"names": {"0": "LOCATION", "1": "ORGANIZATION", "2": "PERSON", "3": "DATE", "4": "MONEY", "5": "PERCENT", "6": "TIME", "7": "CAUSE_OF_DEATH", "8": "CITY", "9": "COUNTRY", "10": "CRIMINAL_CHARGE", "11": "EMAIL", "12": "HANDLE", "13": "IDEOLOGY", "14": "NATIONALITY", "15": "RELIGION", "16": "STATE_OR_PROVINCE", "17": "TITLE", "18": "URL", "19": "NUMBER", "20": "ORDINAL", "21": "MISC", "22": "DURATION", "23": "O"}}}}, {"name": "obj_start", "dtype": "int32"}, {"name": "obj_end", "dtype": "int32"}, {"name": "obj_type", "dtype": {"class_label": {"names": {"0": "LOCATION", "1": "ORGANIZATION", "2": "PERSON", "3": "DATE", "4": "MONEY", "5": "PERCENT", "6": "TIME", "7": "CAUSE_OF_DEATH", "8": "CITY", "9": "COUNTRY", "10": "CRIMINAL_CHARGE", "11": "EMAIL", "12": "HANDLE", "13": "IDEOLOGY", "14": "NATIONALITY", "15": "RELIGION", "16": "STATE_OR_PROVINCE", "17": "TITLE", "18": "URL", "19": "NUMBER", "20": "ORDINAL", "21": "MISC", "22": "DURATION", "23": "O"}}}}, {"name": "relation", "dtype": {"class_label": {"names": {"0": "no_relation", "1": "org:alternate_names", "2": "org:city_of_branch", "3": "org:country_of_branch", "4": "org:dissolved", "5": "org:founded", "6": "org:founded_by", "7": "org:member_of", "8": "org:members", "9": "org:number_of_employees/members", "10": "org:political/religious_affiliation", "11": "org:shareholders", "12": "org:stateorprovince_of_branch", "13": "org:top_members/employees", "14": "org:website", "15": "per:age", "16": "per:cause_of_death", "17": "per:charges", "18": "per:children", "19": "per:cities_of_residence", "20": "per:city_of_birth", "21": "per:city_of_death", "22": "per:countries_of_residence", "23": "per:country_of_birth", "24": "per:country_of_death", "25": "per:date_of_birth", "26": "per:date_of_death", "27": "per:employee_of", "28": "per:identity", "29": "per:origin", "30": "per:other_family", "31": "per:parents", "32": "per:religion", "33": "per:schools_attended", "34": "per:siblings", "35": "per:spouse", "36": "per:stateorprovince_of_birth", "37": "per:stateorprovince_of_death", "38": "per:stateorprovinces_of_residence", "39": "per:title"}}}}], "splits": [{"name": "train", "num_bytes": 23161229, "num_examples": 58465}, {"name": "test", "num_bytes": 5044812, "num_examples": 13418}, {"name": "validation", "num_bytes": 7535491, "num_examples": 19584}, {"name": "backtranslated_test", "num_bytes": 4824801, "num_examples": 13418}], "download_size": 3702157, "dataset_size": 40566333}, {"config_name": "original-ru", "features": [{"name": "id", "dtype": "string"}, {"name": "token", "sequence": "string"}, {"name": "subj_start", "dtype": "int32"}, {"name": "subj_end", "dtype": "int32"}, {"name": "subj_type", "dtype": {"class_label": {"names": {"0": "LOCATION", "1": "ORGANIZATION", "2": "PERSON", "3": "DATE", "4": "MONEY", "5": "PERCENT", "6": "TIME", "7": "CAUSE_OF_DEATH", "8": "CITY", "9": "COUNTRY", "10": "CRIMINAL_CHARGE", "11": "EMAIL", "12": "HANDLE", "13": "IDEOLOGY", "14": "NATIONALITY", "15": "RELIGION", "16": "STATE_OR_PROVINCE", "17": "TITLE", "18": "URL", "19": "NUMBER", "20": "ORDINAL", "21": "MISC", "22": "DURATION", "23": "O"}}}}, {"name": "obj_start", "dtype": "int32"}, {"name": "obj_end", "dtype": "int32"}, {"name": "obj_type", "dtype": {"class_label": {"names": {"0": "LOCATION", "1": "ORGANIZATION", "2": "PERSON", "3": "DATE", "4": "MONEY", "5": "PERCENT", "6": "TIME", "7": "CAUSE_OF_DEATH", "8": "CITY", "9": "COUNTRY", "10": "CRIMINAL_CHARGE", "11": "EMAIL", "12": "HANDLE", "13": "IDEOLOGY", "14": "NATIONALITY", "15": "RELIGION", "16": "STATE_OR_PROVINCE", "17": "TITLE", "18": "URL", "19": "NUMBER", "20": "ORDINAL", "21": "MISC", "22": "DURATION", "23": "O"}}}}, {"name": "relation", "dtype": {"class_label": {"names": {"0": "no_relation", "1": "org:alternate_names", "2": "org:city_of_headquarters", "3": "org:country_of_headquarters", "4": "org:dissolved", "5": "org:founded", "6": "org:founded_by", "7": "org:member_of", "8": "org:members", "9": "org:number_of_employees/members", "10": "org:parents", "11": "org:political/religious_affiliation", "12": "org:shareholders", "13": "org:stateorprovince_of_headquarters", "14": "org:subsidiaries", "15": "org:top_members/employees", "16": "org:website", "17": "per:age", "18": "per:alternate_names", "19": "per:cause_of_death", "20": "per:charges", "21": "per:children", "22": "per:cities_of_residence", "23": "per:city_of_birth", "24": "per:city_of_death", "25": "per:countries_of_residence", "26": "per:country_of_birth", "27": "per:country_of_death", "28": "per:date_of_birth", "29": "per:date_of_death", "30": "per:employee_of", "31": "per:origin", "32": "per:other_family", "33": "per:parents", "34": "per:religion", "35": "per:schools_attended", "36": "per:siblings", "37": "per:spouse", "38": "per:stateorprovince_of_birth", "39": "per:stateorprovince_of_death", "40": "per:stateorprovinces_of_residence", "41": "per:title"}}}}], "splits": [{"name": "train", "num_bytes": 36546830, "num_examples": 66413}, {"name": "test", "num_bytes": 7846828, "num_examples": 14995}, {"name": "validation", "num_bytes": 11847712, "num_examples": 21998}, {"name": "backtranslated_test", "num_bytes": 5335337, "num_examples": 14703}], "download_size": 0, "dataset_size": 61576707}, {"config_name": "revisited-ru", "features": [{"name": "id", "dtype": "string"}, {"name": "token", "sequence": "string"}, {"name": "subj_start", "dtype": "int32"}, {"name": "subj_end", "dtype": "int32"}, {"name": "subj_type", "dtype": {"class_label": {"names": {"0": "LOCATION", "1": "ORGANIZATION", "2": "PERSON", "3": "DATE", "4": "MONEY", "5": "PERCENT", "6": "TIME", "7": "CAUSE_OF_DEATH", "8": "CITY", "9": "COUNTRY", "10": "CRIMINAL_CHARGE", "11": "EMAIL", "12": "HANDLE", "13": "IDEOLOGY", "14": "NATIONALITY", "15": "RELIGION", "16": "STATE_OR_PROVINCE", "17": "TITLE", "18": "URL", "19": "NUMBER", "20": "ORDINAL", "21": "MISC", "22": "DURATION", "23": "O"}}}}, {"name": "obj_start", "dtype": "int32"}, {"name": "obj_end", "dtype": "int32"}, {"name": "obj_type", "dtype": {"class_label": {"names": {"0": "LOCATION", "1": "ORGANIZATION", "2": "PERSON", "3": "DATE", "4": "MONEY", "5": "PERCENT", "6": "TIME", "7": "CAUSE_OF_DEATH", "8": "CITY", "9": "COUNTRY", "10": "CRIMINAL_CHARGE", "11": "EMAIL", "12": "HANDLE", "13": "IDEOLOGY", "14": "NATIONALITY", "15": "RELIGION", "16": "STATE_OR_PROVINCE", "17": "TITLE", "18": "URL", "19": "NUMBER", "20": "ORDINAL", "21": "MISC", "22": "DURATION", "23": "O"}}}}, {"name": "relation", "dtype": {"class_label": {"names": {"0": "no_relation", "1": "org:alternate_names", "2": "org:city_of_headquarters", "3": "org:country_of_headquarters", "4": "org:dissolved", "5": "org:founded", "6": "org:founded_by", "7": "org:member_of", "8": "org:members", "9": "org:number_of_employees/members", "10": "org:parents", "11": "org:political/religious_affiliation", "12": "org:shareholders", "13": "org:stateorprovince_of_headquarters", "14": "org:subsidiaries", "15": "org:top_members/employees", "16": "org:website", "17": "per:age", "18": "per:alternate_names", "19": "per:cause_of_death", "20": "per:charges", "21": "per:children", "22": "per:cities_of_residence", "23": "per:city_of_birth", "24": "per:city_of_death", "25": "per:countries_of_residence", "26": "per:country_of_birth", "27": "per:country_of_death", "28": "per:date_of_birth", "29": "per:date_of_death", "30": "per:employee_of", "31": "per:origin", "32": "per:other_family", "33": "per:parents", "34": "per:religion", "35": "per:schools_attended", "36": "per:siblings", "37": "per:spouse", "38": "per:stateorprovince_of_birth", "39": "per:stateorprovince_of_death", "40": "per:stateorprovinces_of_residence", "41": "per:title"}}}}], "splits": [{"name": "train", "num_bytes": 36546830, "num_examples": 66413}, {"name": "test", "num_bytes": 7846828, "num_examples": 14995}, {"name": "validation", "num_bytes": 11847712, "num_examples": 21998}, {"name": "backtranslated_test", "num_bytes": 5335337, "num_examples": 14703}], "download_size": 157165, "dataset_size": 61576707}, {"config_name": "retacred-ru", "features": [{"name": "id", "dtype": "string"}, {"name": "token", "sequence": "string"}, {"name": "subj_start", "dtype": "int32"}, {"name": "subj_end", "dtype": "int32"}, {"name": "subj_type", "dtype": {"class_label": {"names": {"0": "LOCATION", "1": "ORGANIZATION", "2": "PERSON", "3": "DATE", "4": "MONEY", "5": "PERCENT", "6": "TIME", "7": "CAUSE_OF_DEATH", "8": "CITY", "9": "COUNTRY", "10": "CRIMINAL_CHARGE", "11": "EMAIL", "12": "HANDLE", "13": "IDEOLOGY", "14": "NATIONALITY", "15": "RELIGION", "16": "STATE_OR_PROVINCE", "17": "TITLE", "18": "URL", "19": "NUMBER", "20": "ORDINAL", "21": "MISC", "22": "DURATION", "23": "O"}}}}, {"name": "obj_start", "dtype": "int32"}, {"name": "obj_end", "dtype": "int32"}, {"name": "obj_type", "dtype": {"class_label": {"names": {"0": "LOCATION", "1": "ORGANIZATION", "2": "PERSON", "3": "DATE", "4": "MONEY", "5": "PERCENT", "6": "TIME", "7": "CAUSE_OF_DEATH", "8": "CITY", "9": "COUNTRY", "10": "CRIMINAL_CHARGE", "11": "EMAIL", "12": "HANDLE", "13": "IDEOLOGY", "14": "NATIONALITY", "15": "RELIGION", "16": "STATE_OR_PROVINCE", "17": "TITLE", "18": "URL", "19": "NUMBER", "20": "ORDINAL", "21": "MISC", "22": "DURATION", "23": "O"}}}}, {"name": "relation", "dtype": {"class_label": {"names": {"0": "no_relation", "1": "org:alternate_names", "2": "org:city_of_branch", "3": "org:country_of_branch", "4": "org:dissolved", "5": "org:founded", "6": "org:founded_by", "7": "org:member_of", "8": "org:members", "9": "org:number_of_employees/members", "10": "org:political/religious_affiliation", "11": "org:shareholders", "12": "org:stateorprovince_of_branch", "13": "org:top_members/employees", "14": "org:website", "15": "per:age", "16": "per:cause_of_death", "17": "per:charges", "18": "per:children", "19": "per:cities_of_residence", "20": "per:city_of_birth", "21": "per:city_of_death", "22": "per:countries_of_residence", "23": "per:country_of_birth", "24": "per:country_of_death", "25": "per:date_of_birth", "26": "per:date_of_death", "27": "per:employee_of", "28": "per:identity", "29": "per:origin", "30": "per:other_family", "31": "per:parents", "32": "per:religion", "33": "per:schools_attended", "34": "per:siblings", "35": "per:spouse", "36": "per:stateorprovince_of_birth", "37": "per:stateorprovince_of_death", "38": "per:stateorprovinces_of_residence", "39": "per:title"}}}}], "splits": [{"name": "train", "num_bytes": 31523203, "num_examples": 57060}, {"name": "test", "num_bytes": 6793985, "num_examples": 12975}, {"name": "validation", "num_bytes": 10263742, "num_examples": 19052}, {"name": "backtranslated_test", "num_bytes": 4603168, "num_examples": 12724}], "download_size": 3702157, "dataset_size": 53184098}, {"config_name": "original-tr", "features": [{"name": "id", "dtype": "string"}, {"name": "token", "sequence": "string"}, {"name": "subj_start", "dtype": "int32"}, {"name": "subj_end", "dtype": "int32"}, {"name": "subj_type", "dtype": {"class_label": {"names": {"0": "LOCATION", "1": "ORGANIZATION", "2": "PERSON", "3": "DATE", "4": "MONEY", "5": "PERCENT", "6": "TIME", "7": "CAUSE_OF_DEATH", "8": "CITY", "9": "COUNTRY", "10": "CRIMINAL_CHARGE", "11": "EMAIL", "12": "HANDLE", "13": "IDEOLOGY", "14": "NATIONALITY", "15": "RELIGION", "16": "STATE_OR_PROVINCE", "17": "TITLE", "18": "URL", "19": "NUMBER", "20": "ORDINAL", "21": "MISC", "22": "DURATION", "23": "O"}}}}, {"name": "obj_start", "dtype": "int32"}, {"name": "obj_end", "dtype": "int32"}, {"name": "obj_type", "dtype": {"class_label": {"names": {"0": "LOCATION", "1": "ORGANIZATION", "2": "PERSON", "3": "DATE", "4": "MONEY", "5": "PERCENT", "6": "TIME", "7": "CAUSE_OF_DEATH", "8": "CITY", "9": "COUNTRY", "10": "CRIMINAL_CHARGE", "11": "EMAIL", "12": "HANDLE", "13": "IDEOLOGY", "14": "NATIONALITY", "15": "RELIGION", "16": "STATE_OR_PROVINCE", "17": "TITLE", "18": "URL", "19": "NUMBER", "20": "ORDINAL", "21": "MISC", "22": "DURATION", "23": "O"}}}}, {"name": "relation", "dtype": {"class_label": {"names": {"0": "no_relation", "1": "org:alternate_names", "2": "org:city_of_headquarters", "3": "org:country_of_headquarters", "4": "org:dissolved", "5": "org:founded", "6": "org:founded_by", "7": "org:member_of", "8": "org:members", "9": "org:number_of_employees/members", "10": "org:parents", "11": "org:political/religious_affiliation", "12": "org:shareholders", "13": "org:stateorprovince_of_headquarters", "14": "org:subsidiaries", "15": "org:top_members/employees", "16": "org:website", "17": "per:age", "18": "per:alternate_names", "19": "per:cause_of_death", "20": "per:charges", "21": "per:children", "22": "per:cities_of_residence", "23": "per:city_of_birth", "24": "per:city_of_death", "25": "per:countries_of_residence", "26": "per:country_of_birth", "27": "per:country_of_death", "28": "per:date_of_birth", "29": "per:date_of_death", "30": "per:employee_of", "31": "per:origin", "32": "per:other_family", "33": "per:parents", "34": "per:religion", "35": "per:schools_attended", "36": "per:siblings", "37": "per:spouse", "38": "per:stateorprovince_of_birth", "39": "per:stateorprovince_of_death", "40": "per:stateorprovinces_of_residence", "41": "per:title"}}}}], "splits": [{"name": "train", "num_bytes": 26093320, "num_examples": 67749}, {"name": "test", "num_bytes": 5633846, "num_examples": 15429}, {"name": "validation", "num_bytes": 8403271, "num_examples": 22510}, {"name": "backtranslated_test", "num_bytes": 5571104, "num_examples": 15429}], "download_size": 0, "dataset_size": 45701541}, {"config_name": "revisited-tr", "features": [{"name": "id", "dtype": "string"}, {"name": "token", "sequence": "string"}, {"name": "subj_start", "dtype": "int32"}, {"name": "subj_end", "dtype": "int32"}, {"name": "subj_type", "dtype": {"class_label": {"names": {"0": "LOCATION", "1": "ORGANIZATION", "2": "PERSON", "3": "DATE", "4": "MONEY", "5": "PERCENT", "6": "TIME", "7": "CAUSE_OF_DEATH", "8": "CITY", "9": "COUNTRY", "10": "CRIMINAL_CHARGE", "11": "EMAIL", "12": "HANDLE", "13": "IDEOLOGY", "14": "NATIONALITY", "15": "RELIGION", "16": "STATE_OR_PROVINCE", "17": "TITLE", "18": "URL", "19": "NUMBER", "20": "ORDINAL", "21": "MISC", "22": "DURATION", "23": "O"}}}}, {"name": "obj_start", "dtype": "int32"}, {"name": "obj_end", "dtype": "int32"}, {"name": "obj_type", "dtype": {"class_label": {"names": {"0": "LOCATION", "1": "ORGANIZATION", "2": "PERSON", "3": "DATE", "4": "MONEY", "5": "PERCENT", "6": "TIME", "7": "CAUSE_OF_DEATH", "8": "CITY", "9": "COUNTRY", "10": "CRIMINAL_CHARGE", "11": "EMAIL", "12": "HANDLE", "13": "IDEOLOGY", "14": "NATIONALITY", "15": "RELIGION", "16": "STATE_OR_PROVINCE", "17": "TITLE", "18": "URL", "19": "NUMBER", "20": "ORDINAL", "21": "MISC", "22": "DURATION", "23": "O"}}}}, {"name": "relation", "dtype": {"class_label": {"names": {"0": "no_relation", "1": "org:alternate_names", "2": "org:city_of_headquarters", "3": "org:country_of_headquarters", "4": "org:dissolved", "5": "org:founded", "6": "org:founded_by", "7": "org:member_of", "8": "org:members", "9": "org:number_of_employees/members", "10": "org:parents", "11": "org:political/religious_affiliation", "12": "org:shareholders", "13": "org:stateorprovince_of_headquarters", "14": "org:subsidiaries", "15": "org:top_members/employees", "16": "org:website", "17": "per:age", "18": "per:alternate_names", "19": "per:cause_of_death", "20": "per:charges", "21": "per:children", "22": "per:cities_of_residence", "23": "per:city_of_birth", "24": "per:city_of_death", "25": "per:countries_of_residence", "26": "per:country_of_birth", "27": "per:country_of_death", "28": "per:date_of_birth", "29": "per:date_of_death", "30": "per:employee_of", "31": "per:origin", "32": "per:other_family", "33": "per:parents", "34": "per:religion", "35": "per:schools_attended", "36": "per:siblings", "37": "per:spouse", "38": "per:stateorprovince_of_birth", "39": "per:stateorprovince_of_death", "40": "per:stateorprovinces_of_residence", "41": "per:title"}}}}], "splits": [{"name": "train", "num_bytes": 26093320, "num_examples": 67749}, {"name": "test", "num_bytes": 5633846, "num_examples": 15429}, {"name": "validation", "num_bytes": 8403271, "num_examples": 22510}, {"name": "backtranslated_test", "num_bytes": 5571104, "num_examples": 15429}], "download_size": 157165, "dataset_size": 45701541}, {"config_name": "retacred-tr", "features": [{"name": "id", "dtype": "string"}, {"name": "token", "sequence": "string"}, {"name": "subj_start", "dtype": "int32"}, {"name": "subj_end", "dtype": "int32"}, {"name": "subj_type", "dtype": {"class_label": {"names": {"0": "LOCATION", "1": "ORGANIZATION", "2": "PERSON", "3": "DATE", "4": "MONEY", "5": "PERCENT", "6": "TIME", "7": "CAUSE_OF_DEATH", "8": "CITY", "9": "COUNTRY", "10": "CRIMINAL_CHARGE", "11": "EMAIL", "12": "HANDLE", "13": "IDEOLOGY", "14": "NATIONALITY", "15": "RELIGION", "16": "STATE_OR_PROVINCE", "17": "TITLE", "18": "URL", "19": "NUMBER", "20": "ORDINAL", "21": "MISC", "22": "DURATION", "23": "O"}}}}, {"name": "obj_start", "dtype": "int32"}, {"name": "obj_end", "dtype": "int32"}, {"name": "obj_type", "dtype": {"class_label": {"names": {"0": "LOCATION", "1": "ORGANIZATION", "2": "PERSON", "3": "DATE", "4": "MONEY", "5": "PERCENT", "6": "TIME", "7": "CAUSE_OF_DEATH", "8": "CITY", "9": "COUNTRY", "10": "CRIMINAL_CHARGE", "11": "EMAIL", "12": "HANDLE", "13": "IDEOLOGY", "14": "NATIONALITY", "15": "RELIGION", "16": "STATE_OR_PROVINCE", "17": "TITLE", "18": "URL", "19": "NUMBER", "20": "ORDINAL", "21": "MISC", "22": "DURATION", "23": "O"}}}}, {"name": "relation", "dtype": {"class_label": {"names": {"0": "no_relation", "1": "org:alternate_names", "2": "org:city_of_branch", "3": "org:country_of_branch", "4": "org:dissolved", "5": "org:founded", "6": "org:founded_by", "7": "org:member_of", "8": "org:members", "9": "org:number_of_employees/members", "10": "org:political/religious_affiliation", "11": "org:shareholders", "12": "org:stateorprovince_of_branch", "13": "org:top_members/employees", "14": "org:website", "15": "per:age", "16": "per:cause_of_death", "17": "per:charges", "18": "per:children", "19": "per:cities_of_residence", "20": "per:city_of_birth", "21": "per:city_of_death", "22": "per:countries_of_residence", "23": "per:country_of_birth", "24": "per:country_of_death", "25": "per:date_of_birth", "26": "per:date_of_death", "27": "per:employee_of", "28": "per:identity", "29": "per:origin", "30": "per:other_family", "31": "per:parents", "32": "per:religion", "33": "per:schools_attended", "34": "per:siblings", "35": "per:spouse", "36": "per:stateorprovince_of_birth", "37": "per:stateorprovince_of_death", "38": "per:stateorprovinces_of_residence", "39": "per:title"}}}}], "splits": [{"name": "train", "num_bytes": 22386009, "num_examples": 58183}, {"name": "test", "num_bytes": 4857933, "num_examples": 13352}, {"name": "validation", "num_bytes": 7257304, "num_examples": 19488}, {"name": "backtranslated_test", "num_bytes": 4805734, "num_examples": 13352}], "download_size": 3702157, "dataset_size": 39306980}, {"config_name": "original-zh", "features": [{"name": "id", "dtype": "string"}, {"name": "token", "sequence": "string"}, {"name": "subj_start", "dtype": "int32"}, {"name": "subj_end", "dtype": "int32"}, {"name": "subj_type", "dtype": {"class_label": {"names": {"0": "LOCATION", "1": "ORGANIZATION", "2": "PERSON", "3": "DATE", "4": "MONEY", "5": "PERCENT", "6": "TIME", "7": "CAUSE_OF_DEATH", "8": "CITY", "9": "COUNTRY", "10": "CRIMINAL_CHARGE", "11": "EMAIL", "12": "HANDLE", "13": "IDEOLOGY", "14": "NATIONALITY", "15": "RELIGION", "16": "STATE_OR_PROVINCE", "17": "TITLE", "18": "URL", "19": "NUMBER", "20": "ORDINAL", "21": "MISC", "22": "DURATION", "23": "O"}}}}, {"name": "obj_start", "dtype": "int32"}, {"name": "obj_end", "dtype": "int32"}, {"name": "obj_type", "dtype": {"class_label": {"names": {"0": "LOCATION", "1": "ORGANIZATION", "2": "PERSON", "3": "DATE", "4": "MONEY", "5": "PERCENT", "6": "TIME", "7": "CAUSE_OF_DEATH", "8": "CITY", "9": "COUNTRY", "10": "CRIMINAL_CHARGE", "11": "EMAIL", "12": "HANDLE", "13": "IDEOLOGY", "14": "NATIONALITY", "15": "RELIGION", "16": "STATE_OR_PROVINCE", "17": "TITLE", "18": "URL", "19": "NUMBER", "20": "ORDINAL", "21": "MISC", "22": "DURATION", "23": "O"}}}}, {"name": "relation", "dtype": {"class_label": {"names": {"0": "no_relation", "1": "org:alternate_names", "2": "org:city_of_headquarters", "3": "org:country_of_headquarters", "4": "org:dissolved", "5": "org:founded", "6": "org:founded_by", "7": "org:member_of", "8": "org:members", "9": "org:number_of_employees/members", "10": "org:parents", "11": "org:political/religious_affiliation", "12": "org:shareholders", "13": "org:stateorprovince_of_headquarters", "14": "org:subsidiaries", "15": "org:top_members/employees", "16": "org:website", "17": "per:age", "18": "per:alternate_names", "19": "per:cause_of_death", "20": "per:charges", "21": "per:children", "22": "per:cities_of_residence", "23": "per:city_of_birth", "24": "per:city_of_death", "25": "per:countries_of_residence", "26": "per:country_of_birth", "27": "per:country_of_death", "28": "per:date_of_birth", "29": "per:date_of_death", "30": "per:employee_of", "31": "per:origin", "32": "per:other_family", "33": "per:parents", "34": "per:religion", "35": "per:schools_attended", "36": "per:siblings", "37": "per:spouse", "38": "per:stateorprovince_of_birth", "39": "per:stateorprovince_of_death", "40": "per:stateorprovinces_of_residence", "41": "per:title"}}}}], "splits": [{"name": "train", "num_bytes": 26159615, "num_examples": 65260}, {"name": "test", "num_bytes": 5483795, "num_examples": 14694}, {"name": "validation", "num_bytes": 8348430, "num_examples": 21538}, {"name": "backtranslated_test", "num_bytes": 5155679, "num_examples": 14021}], "download_size": 0, "dataset_size": 45147519}, {"config_name": "revisited-zh", "features": [{"name": "id", "dtype": "string"}, {"name": "token", "sequence": "string"}, {"name": "subj_start", "dtype": "int32"}, {"name": "subj_end", "dtype": "int32"}, {"name": "subj_type", "dtype": {"class_label": {"names": {"0": "LOCATION", "1": "ORGANIZATION", "2": "PERSON", "3": "DATE", "4": "MONEY", "5": "PERCENT", "6": "TIME", "7": "CAUSE_OF_DEATH", "8": "CITY", "9": "COUNTRY", "10": "CRIMINAL_CHARGE", "11": "EMAIL", "12": "HANDLE", "13": "IDEOLOGY", "14": "NATIONALITY", "15": "RELIGION", "16": "STATE_OR_PROVINCE", "17": "TITLE", "18": "URL", "19": "NUMBER", "20": "ORDINAL", "21": "MISC", "22": "DURATION", "23": "O"}}}}, {"name": "obj_start", "dtype": "int32"}, {"name": "obj_end", "dtype": "int32"}, {"name": "obj_type", "dtype": {"class_label": {"names": {"0": "LOCATION", "1": "ORGANIZATION", "2": "PERSON", "3": "DATE", "4": "MONEY", "5": "PERCENT", "6": "TIME", "7": "CAUSE_OF_DEATH", "8": "CITY", "9": "COUNTRY", "10": "CRIMINAL_CHARGE", "11": "EMAIL", "12": "HANDLE", "13": "IDEOLOGY", "14": "NATIONALITY", "15": "RELIGION", "16": "STATE_OR_PROVINCE", "17": "TITLE", "18": "URL", "19": "NUMBER", "20": "ORDINAL", "21": "MISC", "22": "DURATION", "23": "O"}}}}, {"name": "relation", "dtype": {"class_label": {"names": {"0": "no_relation", "1": "org:alternate_names", "2": "org:city_of_headquarters", "3": "org:country_of_headquarters", "4": "org:dissolved", "5": "org:founded", "6": "org:founded_by", "7": "org:member_of", "8": "org:members", "9": "org:number_of_employees/members", "10": "org:parents", "11": "org:political/religious_affiliation", "12": "org:shareholders", "13": "org:stateorprovince_of_headquarters", "14": "org:subsidiaries", "15": "org:top_members/employees", "16": "org:website", "17": "per:age", "18": "per:alternate_names", "19": "per:cause_of_death", "20": "per:charges", "21": "per:children", "22": "per:cities_of_residence", "23": "per:city_of_birth", "24": "per:city_of_death", "25": "per:countries_of_residence", "26": "per:country_of_birth", "27": "per:country_of_death", "28": "per:date_of_birth", "29": "per:date_of_death", "30": "per:employee_of", "31": "per:origin", "32": "per:other_family", "33": "per:parents", "34": "per:religion", "35": "per:schools_attended", "36": "per:siblings", "37": "per:spouse", "38": "per:stateorprovince_of_birth", "39": "per:stateorprovince_of_death", "40": "per:stateorprovinces_of_residence", "41": "per:title"}}}}], "splits": [{"name": "train", "num_bytes": 26159615, "num_examples": 65260}, {"name": "test", "num_bytes": 5483795, "num_examples": 14694}, {"name": "validation", "num_bytes": 8348430, "num_examples": 21538}, {"name": "backtranslated_test", "num_bytes": 5155679, "num_examples": 14021}], "download_size": 157165, "dataset_size": 45147519}, {"config_name": "retacred-zh", "features": [{"name": "id", "dtype": "string"}, {"name": "token", "sequence": "string"}, {"name": "subj_start", "dtype": "int32"}, {"name": "subj_end", "dtype": "int32"}, {"name": "subj_type", "dtype": {"class_label": {"names": {"0": "LOCATION", "1": "ORGANIZATION", "2": "PERSON", "3": "DATE", "4": "MONEY", "5": "PERCENT", "6": "TIME", "7": "CAUSE_OF_DEATH", "8": "CITY", "9": "COUNTRY", "10": "CRIMINAL_CHARGE", "11": "EMAIL", "12": "HANDLE", "13": "IDEOLOGY", "14": "NATIONALITY", "15": "RELIGION", "16": "STATE_OR_PROVINCE", "17": "TITLE", "18": "URL", "19": "NUMBER", "20": "ORDINAL", "21": "MISC", "22": "DURATION", "23": "O"}}}}, {"name": "obj_start", "dtype": "int32"}, {"name": "obj_end", "dtype": "int32"}, {"name": "obj_type", "dtype": {"class_label": {"names": {"0": "LOCATION", "1": "ORGANIZATION", "2": "PERSON", "3": "DATE", "4": "MONEY", "5": "PERCENT", "6": "TIME", "7": "CAUSE_OF_DEATH", "8": "CITY", "9": "COUNTRY", "10": "CRIMINAL_CHARGE", "11": "EMAIL", "12": "HANDLE", "13": "IDEOLOGY", "14": "NATIONALITY", "15": "RELIGION", "16": "STATE_OR_PROVINCE", "17": "TITLE", "18": "URL", "19": "NUMBER", "20": "ORDINAL", "21": "MISC", "22": "DURATION", "23": "O"}}}}, {"name": "relation", "dtype": {"class_label": {"names": {"0": "no_relation", "1": "org:alternate_names", "2": "org:city_of_branch", "3": "org:country_of_branch", "4": "org:dissolved", "5": "org:founded", "6": "org:founded_by", "7": "org:member_of", "8": "org:members", "9": "org:number_of_employees/members", "10": "org:political/religious_affiliation", "11": "org:shareholders", "12": "org:stateorprovince_of_branch", "13": "org:top_members/employees", "14": "org:website", "15": "per:age", "16": "per:cause_of_death", "17": "per:charges", "18": "per:children", "19": "per:cities_of_residence", "20": "per:city_of_birth", "21": "per:city_of_death", "22": "per:countries_of_residence", "23": "per:country_of_birth", "24": "per:country_of_death", "25": "per:date_of_birth", "26": "per:date_of_death", "27": "per:employee_of", "28": "per:identity", "29": "per:origin", "30": "per:other_family", "31": "per:parents", "32": "per:religion", "33": "per:schools_attended", "34": "per:siblings", "35": "per:spouse", "36": "per:stateorprovince_of_birth", "37": "per:stateorprovince_of_death", "38": "per:stateorprovinces_of_residence", "39": "per:title"}}}}], "splits": [{"name": "train", "num_bytes": 22440419, "num_examples": 56049}, {"name": "test", "num_bytes": 4717593, "num_examples": 12718}, {"name": "validation", "num_bytes": 7200681, "num_examples": 18642}, {"name": "backtranslated_test", "num_bytes": 4441386, "num_examples": 12127}], "download_size": 3702157, "dataset_size": 38800079}]} | 2024-01-17T09:16:51+00:00 | [
"2305.04582"
] | [
"ar",
"de",
"es",
"fi",
"fr",
"hi",
"hu",
"ja",
"pl",
"ru",
"tr",
"zh"
] | TAGS
#task_categories-text-classification #task_ids-multi-class-classification #annotations_creators-crowdsourced #annotations_creators-expert-generated #language_creators-found #size_categories-100K<n<1M #source_datasets-DFKI-NLP/tacred #language-Arabic #language-German #language-Spanish #language-Finnish #language-French #language-Hindi #language-Hungarian #language-Japanese #language-Polish #language-Russian #language-Turkish #language-Chinese #license-other #relation extraction #arxiv-2305.04582 #region-us
| Dataset Card for "MultiTACRED"
==============================
Dataset Description
-------------------
* Homepage: URL
* Paper: MultiTACRED: A Multilingual Version of the TAC Relation Extraction Dataset
* Point of Contact: See URL
* Size of downloaded dataset files: 15.4KB (TACRED-Revisited), 3.7 MB (Re-TACRED)
* Size of the generated dataset: 1.7 GB (all languages, all versions)
* Total amount of disk used: 1.7 GB (all languages, all versions)
### Dataset Summary
MultiTACRED is a multilingual version of the large-scale TAC Relation Extraction Dataset.
It covers 12 typologically diverse languages from 9 language families, and was created by the
Speech & Language Technology group of DFKI by machine-translating the instances of the
original TACRED dataset and automatically projecting their entity annotations. For details of the original TACRED's
data collection and annotation process, see the Stanford paper. Translations are
syntactically validated by checking the correctness of the XML tag markup. Any translations with an invalid tag
structure, e.g. missing or invalid head or tail tag pairs, are discarded (on average, 2.3% of the instances).
Languages covered are: Arabic, Chinese, Finnish, French, German, Hindi, Hungarian, Japanese, Polish,
Russian, Spanish, Turkish. Intended use is supervised relation classification. Audience - researchers.
Please see our ACL paper for full details.
NOTE: This Datasetreader supports a reduced version of the original TACRED JSON format with the following changes:
* Removed fields: stanford\_pos, stanford\_ner, stanford\_head, stanford\_deprel, docid
The motivation for this is that we want to support additional languages, for which these fields were not required
or available. The reader expects the specification of a language-specific configuration specifying the variant
(original, revisited or retacred) and the language (as a two-letter iso code).
The DatasetReader changes the offsets of the following fields, to conform with standard Python usage (see
\_generate\_examples()):
* subj\_end to subj\_end + 1 (make end offset exclusive)
* obj\_end to obj\_end + 1 (make end offset exclusive)
NOTE 2: The MultiTACRED dataset offers an additional 'split', namely the backtranslated test data (translated to a
target language and then back to English). To access this split, use dataset['backtranslated\_test'].
You can find the TACRED dataset reader for the English version of the dataset at
URL
### Supported Tasks and Leaderboards
* Tasks: Relation Classification
* Leaderboards: URL
### Languages
The languages in the dataset are Arabic, German, English, Spanish, Finnish, French, Hindi, Hungarian, Japanese, Polish, Russian, Turkish, and Chinese.
All languages except English are machine-translated using either Deepl's or Google's translation APIs.
Dataset Structure
-----------------
### Data Instances
* Size of downloaded dataset files: 15.4KB (TACRED-Revisited), 3.7 MB (Re-TACRED)
* Size of the generated dataset: 1.7 GB (all languages, all versions)
* Total amount of disk used: 1.7 GB (all languages, all versions)
An example of 'train' looks as follows:
### Data Fields
The data fields are the same among all splits.
* 'id': the instance id of this sentence, a 'string' feature.
* 'token': the list of tokens of this sentence, a 'list' of 'string' features.
* 'relation': the relation label of this instance, a 'string' classification label.
* 'subj\_start': the 0-based index of the start token of the relation subject mention, an 'รฌnt' feature.
* 'subj\_end': the 0-based index of the end token of the relation subject mention, exclusive, an 'รฌnt' feature.
* 'subj\_type': the NER type of the subject mention, among the types used in the Stanford NER system, a 'string' feature.
* 'obj\_start': the 0-based index of the start token of the relation object mention, an 'รฌnt' feature.
* 'obj\_end': the 0-based index of the end token of the relation object mention, exclusive, an 'รฌnt' feature.
* 'obj\_type': the NER type of the object mention, among 23 fine-grained types used in the Stanford NER system, a 'string' feature.
### Data Splits
To miminize dataset bias, TACRED is stratified across years in which the TAC KBP challenge was run.
Languages statistics for the splits differ because not all instances could be translated with the
subject and object entity markup still intact, these were discarded.
Dataset Creation
----------------
### Curation Rationale
To enable more research on multilingual Relation Extraction, we generate translations of the TAC relation extraction
dataset using DeepL and Google Translate.
### Source Data
#### Initial Data Collection and Normalization
The instances of this dataset are sentences from the
original TACRED dataset, which in turn
are sampled from the corpus used in the yearly
TAC Knowledge Base Population (TAC KBP) challenges.
#### Who are the source language producers?
Newswire and web texts collected for the TAC Knowledge Base Population (TAC KBP) challenges.
### Annotations
#### Annotation process
See the Stanford paper, the TACRED Revisited paper, and the Re-TACRED paper, plus their appendices, for
details on the original annotation process. The translated versions do not change the original labels.
Translations were tokenized with language-specific Spacy models (Spacy 3.1, 'core\_news/web\_sm' models)
or Trankit (Trankit 1.1.0) when there was no Spacy model for a given language (Hungarian, Turkish, Arabic, Hindi).
#### Who are the annotators?
The original TACRED dataset was annotated by crowd workers, see the TACRED paper.
### Personal and Sensitive Information
The authors of the original TACRED dataset
have not stated measures that prevent collecting sensitive or offensive text. Therefore, we do
not rule out the possible risk of sensitive/offensive content in the translated data.
Considerations for Using the Data
---------------------------------
### Social Impact of Dataset
not applicable
### Discussion of Biases
The dataset is drawn from web and newswire text, and thus reflects any biases of these original
texts, as well as biases introduced by the MT models.
### Other Known Limitations
not applicable
Additional Information
----------------------
### Dataset Curators
The dataset was created by members of the
DFKI SLT team: Leonhard Hennig, Philippe Thomas, Sebastian Mรถller, Gabriel Kressin
### Licensing Information
To respect the copyright of the underlying TACRED dataset, MultiTACRED is released via the
Linguistic Data Consortium (LDC License).
You can download MultiTACRED from the LDC MultiTACRED webpage.
If you are an LDC member, the access will be free; otherwise, an access fee of $25 is needed.
The original dataset:
For the revised version, please also cite:
For the Re-TACRED version, please also cite:
### Contributions
Thanks to @leonhardhennig for adding this dataset.
| [
"### Dataset Summary\n\n\nMultiTACRED is a multilingual version of the large-scale TAC Relation Extraction Dataset.\nIt covers 12 typologically diverse languages from 9 language families, and was created by the\nSpeech & Language Technology group of DFKI by machine-translating the instances of the\noriginal TACRED dataset and automatically projecting their entity annotations. For details of the original TACRED's\ndata collection and annotation process, see the Stanford paper. Translations are\nsyntactically validated by checking the correctness of the XML tag markup. Any translations with an invalid tag\nstructure, e.g. missing or invalid head or tail tag pairs, are discarded (on average, 2.3% of the instances).\n\n\nLanguages covered are: Arabic, Chinese, Finnish, French, German, Hindi, Hungarian, Japanese, Polish,\nRussian, Spanish, Turkish. Intended use is supervised relation classification. Audience - researchers.\n\n\nPlease see our ACL paper for full details.\n\n\nNOTE: This Datasetreader supports a reduced version of the original TACRED JSON format with the following changes:\n\n\n* Removed fields: stanford\\_pos, stanford\\_ner, stanford\\_head, stanford\\_deprel, docid\nThe motivation for this is that we want to support additional languages, for which these fields were not required\nor available. The reader expects the specification of a language-specific configuration specifying the variant\n(original, revisited or retacred) and the language (as a two-letter iso code).\n\n\nThe DatasetReader changes the offsets of the following fields, to conform with standard Python usage (see\n\\_generate\\_examples()):\n\n\n* subj\\_end to subj\\_end + 1 (make end offset exclusive)\n* obj\\_end to obj\\_end + 1 (make end offset exclusive)\n\n\nNOTE 2: The MultiTACRED dataset offers an additional 'split', namely the backtranslated test data (translated to a\ntarget language and then back to English). To access this split, use dataset['backtranslated\\_test'].\n\n\nYou can find the TACRED dataset reader for the English version of the dataset at\nURL",
"### Supported Tasks and Leaderboards\n\n\n* Tasks: Relation Classification\n* Leaderboards: URL",
"### Languages\n\n\nThe languages in the dataset are Arabic, German, English, Spanish, Finnish, French, Hindi, Hungarian, Japanese, Polish, Russian, Turkish, and Chinese.\nAll languages except English are machine-translated using either Deepl's or Google's translation APIs.\n\n\nDataset Structure\n-----------------",
"### Data Instances\n\n\n* Size of downloaded dataset files: 15.4KB (TACRED-Revisited), 3.7 MB (Re-TACRED)\n* Size of the generated dataset: 1.7 GB (all languages, all versions)\n* Total amount of disk used: 1.7 GB (all languages, all versions)\n\n\nAn example of 'train' looks as follows:",
"### Data Fields\n\n\nThe data fields are the same among all splits.\n\n\n* 'id': the instance id of this sentence, a 'string' feature.\n* 'token': the list of tokens of this sentence, a 'list' of 'string' features.\n* 'relation': the relation label of this instance, a 'string' classification label.\n* 'subj\\_start': the 0-based index of the start token of the relation subject mention, an 'รฌnt' feature.\n* 'subj\\_end': the 0-based index of the end token of the relation subject mention, exclusive, an 'รฌnt' feature.\n* 'subj\\_type': the NER type of the subject mention, among the types used in the Stanford NER system, a 'string' feature.\n* 'obj\\_start': the 0-based index of the start token of the relation object mention, an 'รฌnt' feature.\n* 'obj\\_end': the 0-based index of the end token of the relation object mention, exclusive, an 'รฌnt' feature.\n* 'obj\\_type': the NER type of the object mention, among 23 fine-grained types used in the Stanford NER system, a 'string' feature.",
"### Data Splits\n\n\nTo miminize dataset bias, TACRED is stratified across years in which the TAC KBP challenge was run.\nLanguages statistics for the splits differ because not all instances could be translated with the\nsubject and object entity markup still intact, these were discarded.\n\n\n\nDataset Creation\n----------------",
"### Curation Rationale\n\n\nTo enable more research on multilingual Relation Extraction, we generate translations of the TAC relation extraction\ndataset using DeepL and Google Translate.",
"### Source Data",
"#### Initial Data Collection and Normalization\n\n\nThe instances of this dataset are sentences from the\noriginal TACRED dataset, which in turn\nare sampled from the corpus used in the yearly\nTAC Knowledge Base Population (TAC KBP) challenges.",
"#### Who are the source language producers?\n\n\nNewswire and web texts collected for the TAC Knowledge Base Population (TAC KBP) challenges.",
"### Annotations",
"#### Annotation process\n\n\nSee the Stanford paper, the TACRED Revisited paper, and the Re-TACRED paper, plus their appendices, for\ndetails on the original annotation process. The translated versions do not change the original labels.\n\n\nTranslations were tokenized with language-specific Spacy models (Spacy 3.1, 'core\\_news/web\\_sm' models)\nor Trankit (Trankit 1.1.0) when there was no Spacy model for a given language (Hungarian, Turkish, Arabic, Hindi).",
"#### Who are the annotators?\n\n\nThe original TACRED dataset was annotated by crowd workers, see the TACRED paper.",
"### Personal and Sensitive Information\n\n\nThe authors of the original TACRED dataset\nhave not stated measures that prevent collecting sensitive or offensive text. Therefore, we do\nnot rule out the possible risk of sensitive/offensive content in the translated data.\n\n\nConsiderations for Using the Data\n---------------------------------",
"### Social Impact of Dataset\n\n\nnot applicable",
"### Discussion of Biases\n\n\nThe dataset is drawn from web and newswire text, and thus reflects any biases of these original\ntexts, as well as biases introduced by the MT models.",
"### Other Known Limitations\n\n\nnot applicable\n\n\nAdditional Information\n----------------------",
"### Dataset Curators\n\n\nThe dataset was created by members of the\nDFKI SLT team: Leonhard Hennig, Philippe Thomas, Sebastian Mรถller, Gabriel Kressin",
"### Licensing Information\n\n\nTo respect the copyright of the underlying TACRED dataset, MultiTACRED is released via the\nLinguistic Data Consortium (LDC License).\nYou can download MultiTACRED from the LDC MultiTACRED webpage.\nIf you are an LDC member, the access will be free; otherwise, an access fee of $25 is needed.\n\n\nThe original dataset:\n\n\nFor the revised version, please also cite:\n\n\nFor the Re-TACRED version, please also cite:",
"### Contributions\n\n\nThanks to @leonhardhennig for adding this dataset."
] | [
"TAGS\n#task_categories-text-classification #task_ids-multi-class-classification #annotations_creators-crowdsourced #annotations_creators-expert-generated #language_creators-found #size_categories-100K<n<1M #source_datasets-DFKI-NLP/tacred #language-Arabic #language-German #language-Spanish #language-Finnish #language-French #language-Hindi #language-Hungarian #language-Japanese #language-Polish #language-Russian #language-Turkish #language-Chinese #license-other #relation extraction #arxiv-2305.04582 #region-us \n",
"### Dataset Summary\n\n\nMultiTACRED is a multilingual version of the large-scale TAC Relation Extraction Dataset.\nIt covers 12 typologically diverse languages from 9 language families, and was created by the\nSpeech & Language Technology group of DFKI by machine-translating the instances of the\noriginal TACRED dataset and automatically projecting their entity annotations. For details of the original TACRED's\ndata collection and annotation process, see the Stanford paper. Translations are\nsyntactically validated by checking the correctness of the XML tag markup. Any translations with an invalid tag\nstructure, e.g. missing or invalid head or tail tag pairs, are discarded (on average, 2.3% of the instances).\n\n\nLanguages covered are: Arabic, Chinese, Finnish, French, German, Hindi, Hungarian, Japanese, Polish,\nRussian, Spanish, Turkish. Intended use is supervised relation classification. Audience - researchers.\n\n\nPlease see our ACL paper for full details.\n\n\nNOTE: This Datasetreader supports a reduced version of the original TACRED JSON format with the following changes:\n\n\n* Removed fields: stanford\\_pos, stanford\\_ner, stanford\\_head, stanford\\_deprel, docid\nThe motivation for this is that we want to support additional languages, for which these fields were not required\nor available. The reader expects the specification of a language-specific configuration specifying the variant\n(original, revisited or retacred) and the language (as a two-letter iso code).\n\n\nThe DatasetReader changes the offsets of the following fields, to conform with standard Python usage (see\n\\_generate\\_examples()):\n\n\n* subj\\_end to subj\\_end + 1 (make end offset exclusive)\n* obj\\_end to obj\\_end + 1 (make end offset exclusive)\n\n\nNOTE 2: The MultiTACRED dataset offers an additional 'split', namely the backtranslated test data (translated to a\ntarget language and then back to English). To access this split, use dataset['backtranslated\\_test'].\n\n\nYou can find the TACRED dataset reader for the English version of the dataset at\nURL",
"### Supported Tasks and Leaderboards\n\n\n* Tasks: Relation Classification\n* Leaderboards: URL",
"### Languages\n\n\nThe languages in the dataset are Arabic, German, English, Spanish, Finnish, French, Hindi, Hungarian, Japanese, Polish, Russian, Turkish, and Chinese.\nAll languages except English are machine-translated using either Deepl's or Google's translation APIs.\n\n\nDataset Structure\n-----------------",
"### Data Instances\n\n\n* Size of downloaded dataset files: 15.4KB (TACRED-Revisited), 3.7 MB (Re-TACRED)\n* Size of the generated dataset: 1.7 GB (all languages, all versions)\n* Total amount of disk used: 1.7 GB (all languages, all versions)\n\n\nAn example of 'train' looks as follows:",
"### Data Fields\n\n\nThe data fields are the same among all splits.\n\n\n* 'id': the instance id of this sentence, a 'string' feature.\n* 'token': the list of tokens of this sentence, a 'list' of 'string' features.\n* 'relation': the relation label of this instance, a 'string' classification label.\n* 'subj\\_start': the 0-based index of the start token of the relation subject mention, an 'รฌnt' feature.\n* 'subj\\_end': the 0-based index of the end token of the relation subject mention, exclusive, an 'รฌnt' feature.\n* 'subj\\_type': the NER type of the subject mention, among the types used in the Stanford NER system, a 'string' feature.\n* 'obj\\_start': the 0-based index of the start token of the relation object mention, an 'รฌnt' feature.\n* 'obj\\_end': the 0-based index of the end token of the relation object mention, exclusive, an 'รฌnt' feature.\n* 'obj\\_type': the NER type of the object mention, among 23 fine-grained types used in the Stanford NER system, a 'string' feature.",
"### Data Splits\n\n\nTo miminize dataset bias, TACRED is stratified across years in which the TAC KBP challenge was run.\nLanguages statistics for the splits differ because not all instances could be translated with the\nsubject and object entity markup still intact, these were discarded.\n\n\n\nDataset Creation\n----------------",
"### Curation Rationale\n\n\nTo enable more research on multilingual Relation Extraction, we generate translations of the TAC relation extraction\ndataset using DeepL and Google Translate.",
"### Source Data",
"#### Initial Data Collection and Normalization\n\n\nThe instances of this dataset are sentences from the\noriginal TACRED dataset, which in turn\nare sampled from the corpus used in the yearly\nTAC Knowledge Base Population (TAC KBP) challenges.",
"#### Who are the source language producers?\n\n\nNewswire and web texts collected for the TAC Knowledge Base Population (TAC KBP) challenges.",
"### Annotations",
"#### Annotation process\n\n\nSee the Stanford paper, the TACRED Revisited paper, and the Re-TACRED paper, plus their appendices, for\ndetails on the original annotation process. The translated versions do not change the original labels.\n\n\nTranslations were tokenized with language-specific Spacy models (Spacy 3.1, 'core\\_news/web\\_sm' models)\nor Trankit (Trankit 1.1.0) when there was no Spacy model for a given language (Hungarian, Turkish, Arabic, Hindi).",
"#### Who are the annotators?\n\n\nThe original TACRED dataset was annotated by crowd workers, see the TACRED paper.",
"### Personal and Sensitive Information\n\n\nThe authors of the original TACRED dataset\nhave not stated measures that prevent collecting sensitive or offensive text. Therefore, we do\nnot rule out the possible risk of sensitive/offensive content in the translated data.\n\n\nConsiderations for Using the Data\n---------------------------------",
"### Social Impact of Dataset\n\n\nnot applicable",
"### Discussion of Biases\n\n\nThe dataset is drawn from web and newswire text, and thus reflects any biases of these original\ntexts, as well as biases introduced by the MT models.",
"### Other Known Limitations\n\n\nnot applicable\n\n\nAdditional Information\n----------------------",
"### Dataset Curators\n\n\nThe dataset was created by members of the\nDFKI SLT team: Leonhard Hennig, Philippe Thomas, Sebastian Mรถller, Gabriel Kressin",
"### Licensing Information\n\n\nTo respect the copyright of the underlying TACRED dataset, MultiTACRED is released via the\nLinguistic Data Consortium (LDC License).\nYou can download MultiTACRED from the LDC MultiTACRED webpage.\nIf you are an LDC member, the access will be free; otherwise, an access fee of $25 is needed.\n\n\nThe original dataset:\n\n\nFor the revised version, please also cite:\n\n\nFor the Re-TACRED version, please also cite:",
"### Contributions\n\n\nThanks to @leonhardhennig for adding this dataset."
] | [
172,
511,
23,
75,
85,
287,
75,
41,
4,
56,
34,
5,
120,
31,
68,
9,
50,
16,
36,
108,
19
] | [
"passage: TAGS\n#task_categories-text-classification #task_ids-multi-class-classification #annotations_creators-crowdsourced #annotations_creators-expert-generated #language_creators-found #size_categories-100K<n<1M #source_datasets-DFKI-NLP/tacred #language-Arabic #language-German #language-Spanish #language-Finnish #language-French #language-Hindi #language-Hungarian #language-Japanese #language-Polish #language-Russian #language-Turkish #language-Chinese #license-other #relation extraction #arxiv-2305.04582 #region-us \n",
"passage: ### Dataset Summary\n\n\nMultiTACRED is a multilingual version of the large-scale TAC Relation Extraction Dataset.\nIt covers 12 typologically diverse languages from 9 language families, and was created by the\nSpeech & Language Technology group of DFKI by machine-translating the instances of the\noriginal TACRED dataset and automatically projecting their entity annotations. For details of the original TACRED's\ndata collection and annotation process, see the Stanford paper. Translations are\nsyntactically validated by checking the correctness of the XML tag markup. Any translations with an invalid tag\nstructure, e.g. missing or invalid head or tail tag pairs, are discarded (on average, 2.3% of the instances).\n\n\nLanguages covered are: Arabic, Chinese, Finnish, French, German, Hindi, Hungarian, Japanese, Polish,\nRussian, Spanish, Turkish. Intended use is supervised relation classification. Audience - researchers.\n\n\nPlease see our ACL paper for full details.\n\n\nNOTE: This Datasetreader supports a reduced version of the original TACRED JSON format with the following changes:\n\n\n* Removed fields: stanford\\_pos, stanford\\_ner, stanford\\_head, stanford\\_deprel, docid\nThe motivation for this is that we want to support additional languages, for which these fields were not required\nor available. The reader expects the specification of a language-specific configuration specifying the variant\n(original, revisited or retacred) and the language (as a two-letter iso code).\n\n\nThe DatasetReader changes the offsets of the following fields, to conform with standard Python usage (see\n\\_generate\\_examples()):\n\n\n* subj\\_end to subj\\_end + 1 (make end offset exclusive)\n* obj\\_end to obj\\_end + 1 (make end offset exclusive)\n\n\nNOTE 2: The MultiTACRED dataset offers an additional 'split', namely the backtranslated test data (translated to a\ntarget language and then back to English). To access this split, use dataset['backtranslated\\_test'].\n\n\nYou can find the TACRED dataset reader for the English version of the dataset at\nURL### Supported Tasks and Leaderboards\n\n\n* Tasks: Relation Classification\n* Leaderboards: URL### Languages\n\n\nThe languages in the dataset are Arabic, German, English, Spanish, Finnish, French, Hindi, Hungarian, Japanese, Polish, Russian, Turkish, and Chinese.\nAll languages except English are machine-translated using either Deepl's or Google's translation APIs.\n\n\nDataset Structure\n-----------------### Data Instances\n\n\n* Size of downloaded dataset files: 15.4KB (TACRED-Revisited), 3.7 MB (Re-TACRED)\n* Size of the generated dataset: 1.7 GB (all languages, all versions)\n* Total amount of disk used: 1.7 GB (all languages, all versions)\n\n\nAn example of 'train' looks as follows:### Data Fields\n\n\nThe data fields are the same among all splits.\n\n\n* 'id': the instance id of this sentence, a 'string' feature.\n* 'token': the list of tokens of this sentence, a 'list' of 'string' features.\n* 'relation': the relation label of this instance, a 'string' classification label.\n* 'subj\\_start': the 0-based index of the start token of the relation subject mention, an 'รฌnt' feature.\n* 'subj\\_end': the 0-based index of the end token of the relation subject mention, exclusive, an 'รฌnt' feature.\n* 'subj\\_type': the NER type of the subject mention, among the types used in the Stanford NER system, a 'string' feature.\n* 'obj\\_start': the 0-based index of the start token of the relation object mention, an 'รฌnt' feature.\n* 'obj\\_end': the 0-based index of the end token of the relation object mention, exclusive, an 'รฌnt' feature.\n* 'obj\\_type': the NER type of the object mention, among 23 fine-grained types used in the Stanford NER system, a 'string' feature.",
"passage: ### Data Splits\n\n\nTo miminize dataset bias, TACRED is stratified across years in which the TAC KBP challenge was run.\nLanguages statistics for the splits differ because not all instances could be translated with the\nsubject and object entity markup still intact, these were discarded.\n\n\n\nDataset Creation\n----------------### Curation Rationale\n\n\nTo enable more research on multilingual Relation Extraction, we generate translations of the TAC relation extraction\ndataset using DeepL and Google Translate.### Source Data#### Initial Data Collection and Normalization\n\n\nThe instances of this dataset are sentences from the\noriginal TACRED dataset, which in turn\nare sampled from the corpus used in the yearly\nTAC Knowledge Base Population (TAC KBP) challenges.#### Who are the source language producers?\n\n\nNewswire and web texts collected for the TAC Knowledge Base Population (TAC KBP) challenges.### Annotations#### Annotation process\n\n\nSee the Stanford paper, the TACRED Revisited paper, and the Re-TACRED paper, plus their appendices, for\ndetails on the original annotation process. The translated versions do not change the original labels.\n\n\nTranslations were tokenized with language-specific Spacy models (Spacy 3.1, 'core\\_news/web\\_sm' models)\nor Trankit (Trankit 1.1.0) when there was no Spacy model for a given language (Hungarian, Turkish, Arabic, Hindi).#### Who are the annotators?\n\n\nThe original TACRED dataset was annotated by crowd workers, see the TACRED paper.### Personal and Sensitive Information\n\n\nThe authors of the original TACRED dataset\nhave not stated measures that prevent collecting sensitive or offensive text. Therefore, we do\nnot rule out the possible risk of sensitive/offensive content in the translated data.\n\n\nConsiderations for Using the Data\n---------------------------------### Social Impact of Dataset\n\n\nnot applicable### Discussion of Biases\n\n\nThe dataset is drawn from web and newswire text, and thus reflects any biases of these original\ntexts, as well as biases introduced by the MT models.### Other Known Limitations\n\n\nnot applicable\n\n\nAdditional Information\n----------------------### Dataset Curators\n\n\nThe dataset was created by members of the\nDFKI SLT team: Leonhard Hennig, Philippe Thomas, Sebastian Mรถller, Gabriel Kressin"
] |
ca9324836eefa4c1d7bc835afcaace6759dc3202 | The data contains three different vehicles from CCSA (https://www.ccsa.gmu.edu/models/):
A Toyota Yaris
A Chevy Silverado
And an ADS vehicle
These vehicles were tested at different speeds, and the binout files were stored.
The car models were used to develop an AI that could estimate a full frontal impact for different cars at different speeds.
This can then be used to predict the force of an impact for an Autonomous car simulator. | holen/Finite_element_crash_data | [
"license:apache-2.0",
"region:us"
] | 2022-09-30T10:43:24+00:00 | {"license": "apache-2.0"} | 2022-09-30T15:35:49+00:00 | [] | [] | TAGS
#license-apache-2.0 #region-us
| The data contains three different vehicles from CCSA (URL
A Toyota Yaris
A Chevy Silverado
And an ADS vehicle
These vehicles were tested at different speeds, and the binout files were stored.
The car models were used to develop an AI that could estimate a full frontal impact for different cars at different speeds.
This can then be used to predict the force of an impact for an Autonomous car simulator. | [] | [
"TAGS\n#license-apache-2.0 #region-us \n"
] | [
14
] | [
"passage: TAGS\n#license-apache-2.0 #region-us \n"
] |
3b04f22b6b00133646c814aac26785a428acdaad |
This is the Faroese Common Crawl corpus. The largest dataset of mono-lingual Faroese text, it was extracted from the Common Crawl.
If you find this dataset useful, please cite
```
@inproceedings{snaebjarnarson-etal-2023-transfer,
title = "{T}ransfer to a Low-Resource Language via Close Relatives: The Case Study on Faroese",
author = "Snรฆbjarnarson, Vรฉsteinn and
Simonsen, Annika and
Glavaลก, Goran and
Vuliฤ, Ivan",
booktitle = "Proceedings of the 24th Nordic Conference on Computational Linguistics (NoDaLiDa)",
month = "may 22--24",
year = "2023",
address = "Tรณrshavn, Faroe Islands",
publisher = {Link{\"o}ping University Electronic Press, Sweden},
}
``` | vesteinn/FC3 | [
"language:fo",
"license:cc",
"region:us"
] | 2022-09-30T11:09:39+00:00 | {"language": ["fo"], "license": "cc", "pretty_name": "FC3"} | 2023-03-23T15:51:34+00:00 | [] | [
"fo"
] | TAGS
#language-Faroese #license-cc #region-us
|
This is the Faroese Common Crawl corpus. The largest dataset of mono-lingual Faroese text, it was extracted from the Common Crawl.
If you find this dataset useful, please cite
| [] | [
"TAGS\n#language-Faroese #license-cc #region-us \n"
] | [
17
] | [
"passage: TAGS\n#language-Faroese #license-cc #region-us \n"
] |
22ed42ff72e12eac2938306f120987e9b3e4c711 |
# Dataset Card for SMG-NFT
## Examples
## Citation
| pking/SMG-NFT | [
"task_categories:text-to-image",
"annotations_creators:machine-generated",
"language_creators:other",
"multilinguality:monolingual",
"size_categories:n<1K",
"language:en",
"license:cc-by-nc-sa-4.0",
"region:us"
] | 2022-09-30T11:20:49+00:00 | {"annotations_creators": ["machine-generated"], "language_creators": ["other"], "language": ["en"], "license": "cc-by-nc-sa-4.0", "multilinguality": ["monolingual"], "size_categories": ["n<1K"], "source_datasets": [], "task_categories": ["text-to-image"], "task_ids": [], "pretty_name": "SMG-NFT", "tags": []} | 2022-10-04T18:31:50+00:00 | [] | [
"en"
] | TAGS
#task_categories-text-to-image #annotations_creators-machine-generated #language_creators-other #multilinguality-monolingual #size_categories-n<1K #language-English #license-cc-by-nc-sa-4.0 #region-us
|
# Dataset Card for SMG-NFT
## Examples
| [
"# Dataset Card for SMG-NFT",
"## Examples"
] | [
"TAGS\n#task_categories-text-to-image #annotations_creators-machine-generated #language_creators-other #multilinguality-monolingual #size_categories-n<1K #language-English #license-cc-by-nc-sa-4.0 #region-us \n",
"# Dataset Card for SMG-NFT",
"## Examples"
] | [
74,
10,
3
] | [
"passage: TAGS\n#task_categories-text-to-image #annotations_creators-machine-generated #language_creators-other #multilinguality-monolingual #size_categories-n<1K #language-English #license-cc-by-nc-sa-4.0 #region-us \n# Dataset Card for SMG-NFT## Examples"
] |
ef1661775d746e0844b299164773db733bdc0bf6 | # Dataset Card for [Dataset Name]
## Table of Contents
- [Table of Contents](#table-of-contents)
- [Dataset Description](#dataset-description)
- [Dataset Summary](#dataset-summary)
- [Supported Tasks and Leaderboards](#supported-tasks-and-leaderboards)
- [Languages](#languages)
- [Dataset Structure](#dataset-structure)
- [Data Instances](#data-instances)
- [Data Fields](#data-fields)
- [Data Splits](#data-splits)
- [Dataset Creation](#dataset-creation)
- [Curation Rationale](#curation-rationale)
- [Source Data](#source-data)
- [Annotations](#annotations)
- [Personal and Sensitive Information](#personal-and-sensitive-information)
- [Considerations for Using the Data](#considerations-for-using-the-data)
- [Social Impact of Dataset](#social-impact-of-dataset)
- [Discussion of Biases](#discussion-of-biases)
- [Other Known Limitations](#other-known-limitations)
- [Additional Information](#additional-information)
- [Dataset Curators](#dataset-curators)
- [Licensing Information](#licensing-information)
- [Citation Information](#citation-information)
- [Contributions](#contributions)
## Dataset Description
- **Homepage:** [The official homepage of Sprรฅkbanken](https://spraakbanken.gu.se/resurser/superlim/)
- **Repository:**
- **Paper:**[SwedishGLUE โ Towards a Swedish Test Set for Evaluating Natural Language Understanding Models](https://gup.ub.gu.se/publication/299130?lang=sv)
- **Leaderboard:** [To be implemented]
- **Point of Contact:**[[email protected]]([email protected])
### Dataset Summary
SuperLim 2.0 is a continuation of SuperLim 1.0, which aims for a standardized suite for evaluation and analysis of Swedish natural language understanding systems. The projects is inspired by the GLUE/SuperGLUE projects from which the name is derived: "lim" is the Swedish translation of "glue".
### Supported Tasks and Leaderboards
[More Information Needed]
### Languages
Swedish
## Dataset Structure
### Data Instances
[More Information Needed]
### Data Fields
[More Information Needed]
### Data Splits
Most datasets have a train, dev and test split. However, there are a few (`supersim`, `sweanalogy` and `swesat-synonyms`) who only have a train and test split. The diagnostic tasks `swediagnostics` and `swewinogender` only have a test split, but they could be evaluated on models trained on `swenli` since they are also NLI-based.
## Dataset Creation
### Curation Rationale
[More Information Needed]
### Source Data
#### Initial Data Collection and Normalization
[More Information Needed]
#### Who are the source language producers?
[More Information Needed]
### Annotations
#### Annotation process
[More Information Needed]
#### Who are the annotators?
[More Information Needed]
### Personal and Sensitive Information
[More Information Needed]
## Considerations for Using the Data
### Social Impact of Dataset
[More Information Needed]
### Discussion of Biases
[More Information Needed]
### Other Known Limitations
[More Information Needed]
## Additional Information
### Dataset Curators
[More Information Needed]
### Licensing Information
[More Information Needed]
### Citation Information
[More Information Needed]
### Contributions
To cite as a whole, use the standard reference. If you use or reference individual resources, cite the references specific for these resources:
Standard reference:
To appear in EMNLP 2023, citation will come soon.
Dataset references:
[More information needed]
Thanks to [Felix Morger](https://github.com/felixhultin) for adding this dataset. | sbx/superlim-2 | [
"task_categories:multiple-choice",
"task_categories:text-classification",
"task_categories:question-answering",
"task_categories:sentence-similarity",
"task_categories:token-classification",
"task_ids:sentiment-analysis",
"task_ids:acceptability-classification",
"task_ids:closed-domain-qa",
"task_ids:word-sense-disambiguation",
"task_ids:coreference-resolution",
"annotations_creators:other",
"language_creators:other",
"multilinguality:monolingual",
"size_categories:unknown",
"language:sv",
"region:us"
] | 2022-09-30T11:21:49+00:00 | {"annotations_creators": ["other"], "language_creators": ["other"], "language": ["sv"], "license": [], "multilinguality": ["monolingual"], "size_categories": ["unknown"], "source_datasets": [], "task_categories": ["multiple-choice", "text-classification", "question-answering", "sentence-similarity", "token-classification"], "task_ids": ["sentiment-analysis", "acceptability-classification", "closed-domain-qa", "word-sense-disambiguation", "coreference-resolution"], "pretty_name": "A standardized suite for evaluation and analysis of Swedish natural language understanding systems.", "tags": []} | 2023-10-12T07:10:39+00:00 | [] | [
"sv"
] | TAGS
#task_categories-multiple-choice #task_categories-text-classification #task_categories-question-answering #task_categories-sentence-similarity #task_categories-token-classification #task_ids-sentiment-analysis #task_ids-acceptability-classification #task_ids-closed-domain-qa #task_ids-word-sense-disambiguation #task_ids-coreference-resolution #annotations_creators-other #language_creators-other #multilinguality-monolingual #size_categories-unknown #language-Swedish #region-us
| # Dataset Card for [Dataset Name]
## Table of Contents
- Table of Contents
- Dataset Description
- Dataset Summary
- Supported Tasks and Leaderboards
- Languages
- Dataset Structure
- Data Instances
- Data Fields
- Data Splits
- Dataset Creation
- Curation Rationale
- Source Data
- Annotations
- Personal and Sensitive Information
- Considerations for Using the Data
- Social Impact of Dataset
- Discussion of Biases
- Other Known Limitations
- Additional Information
- Dataset Curators
- Licensing Information
- Citation Information
- Contributions
## Dataset Description
- Homepage: The official homepage of Sprรฅkbanken
- Repository:
- Paper:SwedishGLUE โ Towards a Swedish Test Set for Evaluating Natural Language Understanding Models
- Leaderboard: [To be implemented]
- Point of Contact:sb-info@URL
### Dataset Summary
SuperLim 2.0 is a continuation of SuperLim 1.0, which aims for a standardized suite for evaluation and analysis of Swedish natural language understanding systems. The projects is inspired by the GLUE/SuperGLUE projects from which the name is derived: "lim" is the Swedish translation of "glue".
### Supported Tasks and Leaderboards
### Languages
Swedish
## Dataset Structure
### Data Instances
### Data Fields
### Data Splits
Most datasets have a train, dev and test split. However, there are a few ('supersim', 'sweanalogy' and 'swesat-synonyms') who only have a train and test split. The diagnostic tasks 'swediagnostics' and 'swewinogender' only have a test split, but they could be evaluated on models trained on 'swenli' since they are also NLI-based.
## Dataset Creation
### Curation Rationale
### Source Data
#### Initial Data Collection and Normalization
#### Who are the source language producers?
### Annotations
#### Annotation process
#### Who are the annotators?
### Personal and Sensitive Information
## Considerations for Using the Data
### Social Impact of Dataset
### Discussion of Biases
### Other Known Limitations
## Additional Information
### Dataset Curators
### Licensing Information
### Contributions
To cite as a whole, use the standard reference. If you use or reference individual resources, cite the references specific for these resources:
Standard reference:
To appear in EMNLP 2023, citation will come soon.
Dataset references:
[More information needed]
Thanks to Felix Morger for adding this dataset. | [
"# Dataset Card for [Dataset Name]",
"## Table of Contents\n- Table of Contents\n- Dataset Description\n - Dataset Summary\n - Supported Tasks and Leaderboards\n - Languages\n- Dataset Structure\n - Data Instances\n - Data Fields\n - Data Splits\n- Dataset Creation\n - Curation Rationale\n - Source Data\n - Annotations\n - Personal and Sensitive Information\n- Considerations for Using the Data\n - Social Impact of Dataset\n - Discussion of Biases\n - Other Known Limitations\n- Additional Information\n - Dataset Curators\n - Licensing Information\n - Citation Information\n - Contributions",
"## Dataset Description\n\n- Homepage: The official homepage of Sprรฅkbanken\n- Repository:\n- Paper:SwedishGLUE โ Towards a Swedish Test Set for Evaluating Natural Language Understanding Models\n- Leaderboard: [To be implemented]\n- Point of Contact:sb-info@URL",
"### Dataset Summary\n\nSuperLim 2.0 is a continuation of SuperLim 1.0, which aims for a standardized suite for evaluation and analysis of Swedish natural language understanding systems. The projects is inspired by the GLUE/SuperGLUE projects from which the name is derived: \"lim\" is the Swedish translation of \"glue\".",
"### Supported Tasks and Leaderboards",
"### Languages\n\nSwedish",
"## Dataset Structure",
"### Data Instances",
"### Data Fields",
"### Data Splits\n\nMost datasets have a train, dev and test split. However, there are a few ('supersim', 'sweanalogy' and 'swesat-synonyms') who only have a train and test split. The diagnostic tasks 'swediagnostics' and 'swewinogender' only have a test split, but they could be evaluated on models trained on 'swenli' since they are also NLI-based.",
"## Dataset Creation",
"### Curation Rationale",
"### Source Data",
"#### Initial Data Collection and Normalization",
"#### Who are the source language producers?",
"### Annotations",
"#### Annotation process",
"#### Who are the annotators?",
"### Personal and Sensitive Information",
"## Considerations for Using the Data",
"### Social Impact of Dataset",
"### Discussion of Biases",
"### Other Known Limitations",
"## Additional Information",
"### Dataset Curators",
"### Licensing Information",
"### Contributions\n\nTo cite as a whole, use the standard reference. If you use or reference individual resources, cite the references specific for these resources:\n \nStandard reference:\n\nTo appear in EMNLP 2023, citation will come soon.\n\nDataset references:\n\n[More information needed]\n\nThanks to Felix Morger for adding this dataset."
] | [
"TAGS\n#task_categories-multiple-choice #task_categories-text-classification #task_categories-question-answering #task_categories-sentence-similarity #task_categories-token-classification #task_ids-sentiment-analysis #task_ids-acceptability-classification #task_ids-closed-domain-qa #task_ids-word-sense-disambiguation #task_ids-coreference-resolution #annotations_creators-other #language_creators-other #multilinguality-monolingual #size_categories-unknown #language-Swedish #region-us \n",
"# Dataset Card for [Dataset Name]",
"## Table of Contents\n- Table of Contents\n- Dataset Description\n - Dataset Summary\n - Supported Tasks and Leaderboards\n - Languages\n- Dataset Structure\n - Data Instances\n - Data Fields\n - Data Splits\n- Dataset Creation\n - Curation Rationale\n - Source Data\n - Annotations\n - Personal and Sensitive Information\n- Considerations for Using the Data\n - Social Impact of Dataset\n - Discussion of Biases\n - Other Known Limitations\n- Additional Information\n - Dataset Curators\n - Licensing Information\n - Citation Information\n - Contributions",
"## Dataset Description\n\n- Homepage: The official homepage of Sprรฅkbanken\n- Repository:\n- Paper:SwedishGLUE โ Towards a Swedish Test Set for Evaluating Natural Language Understanding Models\n- Leaderboard: [To be implemented]\n- Point of Contact:sb-info@URL",
"### Dataset Summary\n\nSuperLim 2.0 is a continuation of SuperLim 1.0, which aims for a standardized suite for evaluation and analysis of Swedish natural language understanding systems. The projects is inspired by the GLUE/SuperGLUE projects from which the name is derived: \"lim\" is the Swedish translation of \"glue\".",
"### Supported Tasks and Leaderboards",
"### Languages\n\nSwedish",
"## Dataset Structure",
"### Data Instances",
"### Data Fields",
"### Data Splits\n\nMost datasets have a train, dev and test split. However, there are a few ('supersim', 'sweanalogy' and 'swesat-synonyms') who only have a train and test split. The diagnostic tasks 'swediagnostics' and 'swewinogender' only have a test split, but they could be evaluated on models trained on 'swenli' since they are also NLI-based.",
"## Dataset Creation",
"### Curation Rationale",
"### Source Data",
"#### Initial Data Collection and Normalization",
"#### Who are the source language producers?",
"### Annotations",
"#### Annotation process",
"#### Who are the annotators?",
"### Personal and Sensitive Information",
"## Considerations for Using the Data",
"### Social Impact of Dataset",
"### Discussion of Biases",
"### Other Known Limitations",
"## Additional Information",
"### Dataset Curators",
"### Licensing Information",
"### Contributions\n\nTo cite as a whole, use the standard reference. If you use or reference individual resources, cite the references specific for these resources:\n \nStandard reference:\n\nTo appear in EMNLP 2023, citation will come soon.\n\nDataset references:\n\n[More information needed]\n\nThanks to Felix Morger for adding this dataset."
] | [
167,
10,
125,
65,
71,
10,
5,
6,
6,
5,
106,
5,
7,
4,
10,
10,
5,
5,
9,
8,
8,
7,
8,
7,
5,
6,
6,
71
] | [
"passage: TAGS\n#task_categories-multiple-choice #task_categories-text-classification #task_categories-question-answering #task_categories-sentence-similarity #task_categories-token-classification #task_ids-sentiment-analysis #task_ids-acceptability-classification #task_ids-closed-domain-qa #task_ids-word-sense-disambiguation #task_ids-coreference-resolution #annotations_creators-other #language_creators-other #multilinguality-monolingual #size_categories-unknown #language-Swedish #region-us \n# Dataset Card for [Dataset Name]## Table of Contents\n- Table of Contents\n- Dataset Description\n - Dataset Summary\n - Supported Tasks and Leaderboards\n - Languages\n- Dataset Structure\n - Data Instances\n - Data Fields\n - Data Splits\n- Dataset Creation\n - Curation Rationale\n - Source Data\n - Annotations\n - Personal and Sensitive Information\n- Considerations for Using the Data\n - Social Impact of Dataset\n - Discussion of Biases\n - Other Known Limitations\n- Additional Information\n - Dataset Curators\n - Licensing Information\n - Citation Information\n - Contributions## Dataset Description\n\n- Homepage: The official homepage of Sprรฅkbanken\n- Repository:\n- Paper:SwedishGLUE โ Towards a Swedish Test Set for Evaluating Natural Language Understanding Models\n- Leaderboard: [To be implemented]\n- Point of Contact:sb-info@URL### Dataset Summary\n\nSuperLim 2.0 is a continuation of SuperLim 1.0, which aims for a standardized suite for evaluation and analysis of Swedish natural language understanding systems. The projects is inspired by the GLUE/SuperGLUE projects from which the name is derived: \"lim\" is the Swedish translation of \"glue\".### Supported Tasks and Leaderboards### Languages\n\nSwedish## Dataset Structure### Data Instances### Data Fields"
] |
3655d3cbaad4028f787282b2ada55967aabac9c1 |
# Dataset Card for NIH Chest X-ray dataset
## Table of Contents
- [Table of Contents](#table-of-contents)
- [Dataset Description](#dataset-description)
- [Dataset Summary](#dataset-summary)
- [Languages](#languages)
- [Dataset Structure](#dataset-structure)
- [Data Instances](#data-instances)
- [Data Fields](#data-fields)
- [Data Splits](#data-splits)
- [Dataset Creation](#dataset-creation)
- [Curation Rationale](#curation-rationale)
- [Source Data](#source-data)
- [Annotations](#annotations)
- [Personal and Sensitive Information](#personal-and-sensitive-information)
- [Considerations for Using the Data](#considerations-for-using-the-data)
- [Social Impact of Dataset](#social-impact-of-dataset)
- [Discussion of Biases](#discussion-of-biases)
- [Other Known Limitations](#other-known-limitations)
- [Additional Information](#additional-information)
- [Dataset Curators](#dataset-curators)
- [Licensing Information](#licensing-information)
- [Citation Information](#citation-information)
- [Contributions](#contributions)
## Dataset Description
- **Homepage:** [NIH Chest X-ray Dataset of 10 Common Thorax Disease Categories](https://nihcc.app.box.com/v/ChestXray-NIHCC/folder/36938765345)
- **Repository:**
- **Paper:** [ChestX-ray8: Hospital-scale Chest X-ray Database and Benchmarks on Weakly-Supervised Classification and Localization of Common Thorax Diseases](https://arxiv.org/abs/1705.02315)
- **Leaderboard:**
- **Point of Contact:** [email protected]
### Dataset Summary
_ChestX-ray dataset comprises 112,120 frontal-view X-ray images of 30,805 unique patients with the text-mined fourteen disease image labels (where each image can have multi-labels), mined from the associated radiological reports using natural language processing. Fourteen common thoracic pathologies include Atelectasis, Consolidation, Infiltration, Pneumothorax, Edema, Emphysema, Fibrosis, Effusion, Pneumonia, Pleural_thickening, Cardiomegaly, Nodule, Mass and Hernia, which is an extension of the 8 common disease patterns listed in our CVPR2017 paper. Note that original radiology reports (associated with these chest x-ray studies) are not meant to be publicly shared for many reasons. The text-mined disease labels are expected to have accuracy >90%.Please find more details and benchmark performance of trained models based on 14 disease labels in our arxiv paper: [1705.02315](https://arxiv.org/abs/1705.02315)_

## Dataset Structure
### Data Instances
A sample from the training set is provided below:
```
{'image_file_path': '/root/.cache/huggingface/datasets/downloads/extracted/95db46f21d556880cf0ecb11d45d5ba0b58fcb113c9a0fff2234eba8f74fe22a/images/00000798_022.png',
'image': <PIL.PngImagePlugin.PngImageFile image mode=L size=1024x1024 at 0x7F2151B144D0>,
'labels': [9, 3]}
```
### Data Fields
The data instances have the following fields:
- `image_file_path` a `str` with the image path
- `image`: A `PIL.Image.Image` object containing the image. Note that when accessing the image column: `dataset[0]["image"]` the image file is automatically decoded. Decoding of a large number of image files might take a significant amount of time. Thus it is important to first query the sample index before the `"image"` column, *i.e.* `dataset[0]["image"]` should **always** be preferred over `dataset["image"][0]`.
- `labels`: an `int` classification label.
<details>
<summary>Class Label Mappings</summary>
```json
{
"No Finding": 0,
"Atelectasis": 1,
"Cardiomegaly": 2,
"Effusion": 3,
"Infiltration": 4,
"Mass": 5,
"Nodule": 6,
"Pneumonia": 7,
"Pneumothorax": 8,
"Consolidation": 9,
"Edema": 10,
"Emphysema": 11,
"Fibrosis": 12,
"Pleural_Thickening": 13,
"Hernia": 14
}
```
</details>
**Label distribution on the dataset:**
| labels | obs | freq |
|:-------------------|------:|-----------:|
| No Finding | 60361 | 0.426468 |
| Infiltration | 19894 | 0.140557 |
| Effusion | 13317 | 0.0940885 |
| Atelectasis | 11559 | 0.0816677 |
| Nodule | 6331 | 0.0447304 |
| Mass | 5782 | 0.0408515 |
| Pneumothorax | 5302 | 0.0374602 |
| Consolidation | 4667 | 0.0329737 |
| Pleural_Thickening | 3385 | 0.023916 |
| Cardiomegaly | 2776 | 0.0196132 |
| Emphysema | 2516 | 0.0177763 |
| Edema | 2303 | 0.0162714 |
| Fibrosis | 1686 | 0.0119121 |
| Pneumonia | 1431 | 0.0101104 |
| Hernia | 227 | 0.00160382 |
### Data Splits
| |train| test|
|-------------|----:|----:|
|# of examples|86524|25596|
**Label distribution by dataset split:**
| labels | ('Train', 'obs') | ('Train', 'freq') | ('Test', 'obs') | ('Test', 'freq') |
|:-------------------|-------------------:|--------------------:|------------------:|-------------------:|
| No Finding | 50500 | 0.483392 | 9861 | 0.266032 |
| Infiltration | 13782 | 0.131923 | 6112 | 0.164891 |
| Effusion | 8659 | 0.082885 | 4658 | 0.125664 |
| Atelectasis | 8280 | 0.0792572 | 3279 | 0.0884614 |
| Nodule | 4708 | 0.0450656 | 1623 | 0.0437856 |
| Mass | 4034 | 0.038614 | 1748 | 0.0471578 |
| Consolidation | 2852 | 0.0272997 | 1815 | 0.0489654 |
| Pneumothorax | 2637 | 0.0252417 | 2665 | 0.0718968 |
| Pleural_Thickening | 2242 | 0.0214607 | 1143 | 0.0308361 |
| Cardiomegaly | 1707 | 0.0163396 | 1069 | 0.0288397 |
| Emphysema | 1423 | 0.0136211 | 1093 | 0.0294871 |
| Edema | 1378 | 0.0131904 | 925 | 0.0249548 |
| Fibrosis | 1251 | 0.0119747 | 435 | 0.0117355 |
| Pneumonia | 876 | 0.00838518 | 555 | 0.0149729 |
| Hernia | 141 | 0.00134967 | 86 | 0.00232012 |
## Dataset Creation
### Curation Rationale
[More Information Needed]
### Source Data
#### Initial Data Collection and Normalization
[More Information Needed]
#### Who are the source language producers?
[More Information Needed]
### Annotations
#### Annotation process
[More Information Needed]
#### Who are the annotators?
[More Information Needed]
### Personal and Sensitive Information
[More Information Needed]
## Considerations for Using the Data
### Social Impact of Dataset
[More Information Needed]
### Discussion of Biases
[More Information Needed]
### Other Known Limitations
[More Information Needed]
## Additional Information
### Dataset Curators
[More Information Needed]
### License and attribution
There are no restrictions on the use of the NIH chest x-ray images. However, the dataset has the following attribution requirements:
- Provide a link to the NIH download site: https://nihcc.app.box.com/v/ChestXray-NIHCC
- Include a citation to the CVPR 2017 paper (see Citation information section)
- Acknowledge that the NIH Clinical Center is the data provider
### Citation Information
```
@inproceedings{Wang_2017,
doi = {10.1109/cvpr.2017.369},
url = {https://doi.org/10.1109%2Fcvpr.2017.369},
year = 2017,
month = {jul},
publisher = {{IEEE}
},
author = {Xiaosong Wang and Yifan Peng and Le Lu and Zhiyong Lu and Mohammadhadi Bagheri and Ronald M. Summers},
title = {{ChestX}-Ray8: Hospital-Scale Chest X-Ray Database and Benchmarks on Weakly-Supervised Classification and Localization of Common Thorax Diseases},
booktitle = {2017 {IEEE} Conference on Computer Vision and Pattern Recognition ({CVPR})}
}
```
### Contributions
Thanks to [@alcazar90](https://github.com/alcazar90) for adding this dataset.
| alkzar90/NIH-Chest-X-ray-dataset | [
"task_categories:image-classification",
"task_ids:multi-class-image-classification",
"annotations_creators:machine-generated",
"annotations_creators:expert-generated",
"language_creators:machine-generated",
"language_creators:expert-generated",
"multilinguality:monolingual",
"size_categories:100K<n<1M",
"language:en",
"license:unknown",
"arxiv:1705.02315",
"region:us"
] | 2022-09-30T11:45:52+00:00 | {"annotations_creators": ["machine-generated", "expert-generated"], "language_creators": ["machine-generated", "expert-generated"], "language": ["en"], "license": ["unknown"], "multilinguality": ["monolingual"], "size_categories": ["100K<n<1M"], "task_categories": ["image-classification"], "task_ids": ["multi-class-image-classification"], "paperswithcode_id": "chestx-ray14", "pretty_name": "NIH-CXR14"} | 2022-11-22T20:10:52+00:00 | [
"1705.02315"
] | [
"en"
] | TAGS
#task_categories-image-classification #task_ids-multi-class-image-classification #annotations_creators-machine-generated #annotations_creators-expert-generated #language_creators-machine-generated #language_creators-expert-generated #multilinguality-monolingual #size_categories-100K<n<1M #language-English #license-unknown #arxiv-1705.02315 #region-us
| Dataset Card for NIH Chest X-ray dataset
========================================
Table of Contents
-----------------
* Table of Contents
* Dataset Description
+ Dataset Summary
+ Languages
* Dataset Structure
+ Data Instances
+ Data Fields
+ Data Splits
* Dataset Creation
+ Curation Rationale
+ Source Data
+ Annotations
+ Personal and Sensitive Information
* Considerations for Using the Data
+ Social Impact of Dataset
+ Discussion of Biases
+ Other Known Limitations
* Additional Information
+ Dataset Curators
+ Licensing Information
+ Citation Information
+ Contributions
Dataset Description
-------------------
* Homepage: NIH Chest X-ray Dataset of 10 Common Thorax Disease Categories
* Repository:
* Paper: ChestX-ray8: Hospital-scale Chest X-ray Database and Benchmarks on Weakly-Supervised Classification and Localization of Common Thorax Diseases
* Leaderboard:
* Point of Contact: rms@URL
### Dataset Summary
*ChestX-ray dataset comprises 112,120 frontal-view X-ray images of 30,805 unique patients with the text-mined fourteen disease image labels (where each image can have multi-labels), mined from the associated radiological reports using natural language processing. Fourteen common thoracic pathologies include Atelectasis, Consolidation, Infiltration, Pneumothorax, Edema, Emphysema, Fibrosis, Effusion, Pneumonia, Pleural\_thickening, Cardiomegaly, Nodule, Mass and Hernia, which is an extension of the 8 common disease patterns listed in our CVPR2017 paper. Note that original radiology reports (associated with these chest x-ray studies) are not meant to be publicly shared for many reasons. The text-mined disease labels are expected to have accuracy >90%.Please find more details and benchmark performance of trained models based on 14 disease labels in our arxiv paper: 1705.02315*

* Acknowledge that the NIH Clinical Center is the data provider
### Contributions
Thanks to @alcazar90 for adding this dataset.
| [
"### Dataset Summary\n\n\n*ChestX-ray dataset comprises 112,120 frontal-view X-ray images of 30,805 unique patients with the text-mined fourteen disease image labels (where each image can have multi-labels), mined from the associated radiological reports using natural language processing. Fourteen common thoracic pathologies include Atelectasis, Consolidation, Infiltration, Pneumothorax, Edema, Emphysema, Fibrosis, Effusion, Pneumonia, Pleural\\_thickening, Cardiomegaly, Nodule, Mass and Hernia, which is an extension of the 8 common disease patterns listed in our CVPR2017 paper. Note that original radiology reports (associated with these chest x-ray studies) are not meant to be publicly shared for many reasons. The text-mined disease labels are expected to have accuracy >90%.Please find more details and benchmark performance of trained models based on 14 disease labels in our arxiv paper: 1705.02315*\n\n\n\n* Acknowledge that the NIH Clinical Center is the data provider",
"### Contributions\n\n\nThanks to @alcazar90 for adding this dataset."
] | [
"TAGS\n#task_categories-image-classification #task_ids-multi-class-image-classification #annotations_creators-machine-generated #annotations_creators-expert-generated #language_creators-machine-generated #language_creators-expert-generated #multilinguality-monolingual #size_categories-100K<n<1M #language-English #license-unknown #arxiv-1705.02315 #region-us \n",
"### Dataset Summary\n\n\n*ChestX-ray dataset comprises 112,120 frontal-view X-ray images of 30,805 unique patients with the text-mined fourteen disease image labels (where each image can have multi-labels), mined from the associated radiological reports using natural language processing. Fourteen common thoracic pathologies include Atelectasis, Consolidation, Infiltration, Pneumothorax, Edema, Emphysema, Fibrosis, Effusion, Pneumonia, Pleural\\_thickening, Cardiomegaly, Nodule, Mass and Hernia, which is an extension of the 8 common disease patterns listed in our CVPR2017 paper. Note that original radiology reports (associated with these chest x-ray studies) are not meant to be publicly shared for many reasons. The text-mined disease labels are expected to have accuracy >90%.Please find more details and benchmark performance of trained models based on 14 disease labels in our arxiv paper: 1705.02315*\n\n\n\n* Acknowledge that the NIH Clinical Center is the data provider",
"### Contributions\n\n\nThanks to @alcazar90 for adding this dataset."
] | [
119,
244,
16,
185,
18,
7,
4,
10,
10,
5,
5,
9,
18,
7,
8,
14,
6,
84,
18
] | [
"passage: TAGS\n#task_categories-image-classification #task_ids-multi-class-image-classification #annotations_creators-machine-generated #annotations_creators-expert-generated #language_creators-machine-generated #language_creators-expert-generated #multilinguality-monolingual #size_categories-100K<n<1M #language-English #license-unknown #arxiv-1705.02315 #region-us \n### Dataset Summary\n\n\n*ChestX-ray dataset comprises 112,120 frontal-view X-ray images of 30,805 unique patients with the text-mined fourteen disease image labels (where each image can have multi-labels), mined from the associated radiological reports using natural language processing. Fourteen common thoracic pathologies include Atelectasis, Consolidation, Infiltration, Pneumothorax, Edema, Emphysema, Fibrosis, Effusion, Pneumonia, Pleural\\_thickening, Cardiomegaly, Nodule, Mass and Hernia, which is an extension of the 8 common disease patterns listed in our CVPR2017 paper. Note that original radiology reports (associated with these chest x-ray studies) are not meant to be publicly shared for many reasons. The text-mined disease labels are expected to have accuracy >90%.Please find more details and benchmark performance of trained models based on 14 disease labels in our arxiv paper: 1705.02315*\n\n\n
- [Table of Contents](#table-of-contents)
- [Dataset Description](#dataset-description)
- [Dataset Summary](#dataset-summary)
- [Supported Tasks and Leaderboards](#supported-tasks-and-leaderboards)
- [Languages](#languages)
- [Dataset Structure](#dataset-structure)
- [Data Instances](#data-instances)
- [Data Fields](#data-fields)
- [Data Splits](#data-splits)
- [Dataset Creation](#dataset-creation)
- [Curation Rationale](#curation-rationale)
- [Source Data](#source-data)
- [Initial Data Collection and Normalization](#initial-data-collection-and-normalization)
- [Who are the source language producers?](#who-are-the-source-language-producers)
- [Annotations](#annotations)
- [Annotation process](#annotation-process)
- [Who are the annotators?](#who-are-the-annotators)
- [Personal and Sensitive Information](#personal-and-sensitive-information)
- [Considerations for Using the Data](#considerations-for-using-the-data)
- [Social Impact of Dataset](#social-impact-of-dataset)
- [Discussion of Biases](#discussion-of-biases)
- [Other Known Limitations](#other-known-limitations)
- [Additional Information](#additional-information)
- [Dataset Curators](#dataset-curators)
- [Licensing Information](#licensing-information)
- [Citation Information](#citation-information)
- [Contributions](#contributions)
## Dataset Description
- **Repository:** https://github.com/TurkuNLP/xlsum-fi
- **Point of Contact:** [Filip Ginter](mailto:[email protected])
### Dataset Summary
This dataset is a DeepL -based machine translation of a part of the English section of the XLSum dataset:[https://github.com/csebuetnlp/xl-sum](https://github.com/csebuetnlp/xl-sum) In the present version, only examples where the full version is at most 10x the summary in length are included. We might translate more later.
### Supported Tasks and Leaderboards
### Languages
- `finnish`
## Dataset Structure
### Data Instances
One example from the `Finnish` dataset is given below in JSON format.
```
{
"id": "technology-17657859",
"url": "https://www.bbc.com/news/technology-17657859",
"title": "Walesin myrskytuulien vuoksi annettu sรครคvaroitus",
"summary": "Tuulet voivat yltyรค Walesissa myrskytuuliin, ja myrskysรครค on luvassa koko maahan tรคllรค viikolla.",
"text": "Met Office on antanut Walesin ja Englannin kattavan keltaisen tuulivaroituksen keskiviikkoillasta kello 21.00 GMT alkaen. Matkustaminen ja sรคhkรถnjakelu todennรคkรถisesti hรคiriintyvรคt, ja varoitus on voimassa torstaihin kello 15:00 asti. Puuskat ovat todennรคkรถisesti nopeudeltaan 88 kilometriรค tunnissa, ja rannikoilla ja kukkuloilla puuskat voivat nousta jopa 70 kilometriin tunnissa, ja lisรคksi voi esiintyรค rankkasateita ja myrskyisiรค sadekuuroja."
}
```
### Data Fields
- 'id': A string representing the article ID, matched to the XLSum dataset original
- 'url': A string representing the article URL as in the original XLSum dataset
- 'title': A string containing the article title, machine-translated to Finnish
- 'summary': A string containing the article summary, machine-translated to Finnish
- 'text' : A string containing the article text, machine-translated to Finnish
### Data Splits
Follows the XLSum dataset.
## Dataset Creation
### Curation Rationale
### Source Data
[BBC News](https://www.bbc.co.uk/ws/languages)
#### Initial Data Collection and Normalization
[Detailed in the paper](https://aclanthology.org/2021.findings-acl.413/) For this present dataset, only English was used as the source and only examples where the full text is at maximum 10x in length compared to the summary are preserved. This 10x cutoff is naturally measured on English.
#### Who are the source language producers?
[Detailed in the paper](https://aclanthology.org/2021.findings-acl.413/)
### Annotations
[Detailed in the paper](https://aclanthology.org/2021.findings-acl.413/) DeepL was used to machine-translate from English to Finnish
#### Annotation process
[Detailed in the paper](https://aclanthology.org/2021.findings-acl.413/)
#### Who are the annotators?
[Detailed in the paper](https://aclanthology.org/2021.findings-acl.413/)
### Personal and Sensitive Information
[More information needed](https://github.com/csebuetnlp/xl-sum)
## Considerations for Using the Data
### Social Impact of Dataset
### Discussion of Biases
### Other Known Limitations
Due to DeepL terms and conditions, this dataset **must not be used for any machine translation work**, namely machine translation system development and evaluation of any kind. In general, we wish you do not pair the original English data with the translations except when working on research unrelated to machine translation, so as not to infringe on the terms and conditions.
## Additional Information
### Dataset Curators
### Licensing Information
Contents of this repository are restricted to only non-commercial research purposes under the [Creative Commons Attribution-NonCommercial-ShareAlike 4.0 International License (CC BY-NC-SA 4.0)](https://creativecommons.org/licenses/by-nc-sa/4.0/). Copyright of the dataset contents belongs to the original copyright holders.
### Citation Information
If you use any of the datasets, models or code modules, please cite the original XL-Sum paper below as well as acknowledge Filip Ginter and the TurkuNLP group for the Finnish machine translated version.
```
@inproceedings{hasan-etal-2021-xl,
title = "{XL}-Sum: Large-Scale Multilingual Abstractive Summarization for 44 Languages",
author = "Hasan, Tahmid and
Bhattacharjee, Abhik and
Islam, Md. Saiful and
Mubasshir, Kazi and
Li, Yuan-Fang and
Kang, Yong-Bin and
Rahman, M. Sohel and
Shahriyar, Rifat",
booktitle = "Findings of the Association for Computational Linguistics: ACL-IJCNLP 2021",
month = aug,
year = "2021",
address = "Online",
publisher = "Association for Computational Linguistics",
url = "https://aclanthology.org/2021.findings-acl.413",
pages = "4693--4703",
}
```
### Contributions
Thanks to the creators of the XLSum dataset! | TurkuNLP/xlsum-fi | [
"task_categories:summarization",
"task_categories:text2text-generation",
"annotations_creators:found",
"language_creators:machine translated",
"multilinguality:monolingual",
"size_categories:10K<n<100K",
"source_datasets:xlsum",
"language:fi",
"license:cc-by-nc-sa-4.0",
"conditional-text-generation",
"region:us"
] | 2022-09-30T12:10:05+00:00 | {"annotations_creators": ["found"], "language_creators": ["machine translated"], "language": ["fi"], "license": ["cc-by-nc-sa-4.0"], "multilinguality": ["monolingual"], "size_categories": ["10K<n<100K"], "source_datasets": ["xlsum"], "task_categories": ["summarization", "text2text-generation"], "task_ids": [], "pretty_name": "XL-Sum-FI", "tags": ["conditional-text-generation"]} | 2022-10-25T05:30:19+00:00 | [] | [
"fi"
] | TAGS
#task_categories-summarization #task_categories-text2text-generation #annotations_creators-found #language_creators-machine translated #multilinguality-monolingual #size_categories-10K<n<100K #source_datasets-xlsum #language-Finnish #license-cc-by-nc-sa-4.0 #conditional-text-generation #region-us
|
# Dataset Card for "XL-Sum-FI"
## Table of Contents
- Dataset Card Creation Guide
- Table of Contents
- Dataset Description
- Dataset Summary
- Supported Tasks and Leaderboards
- Languages
- Dataset Structure
- Data Instances
- Data Fields
- Data Splits
- Dataset Creation
- Curation Rationale
- Source Data
- Initial Data Collection and Normalization
- Who are the source language producers?
- Annotations
- Annotation process
- Who are the annotators?
- Personal and Sensitive Information
- Considerations for Using the Data
- Social Impact of Dataset
- Discussion of Biases
- Other Known Limitations
- Additional Information
- Dataset Curators
- Licensing Information
- Citation Information
- Contributions
## Dataset Description
- Repository: URL
- Point of Contact: Filip Ginter
### Dataset Summary
This dataset is a DeepL -based machine translation of a part of the English section of the XLSum dataset:URL In the present version, only examples where the full version is at most 10x the summary in length are included. We might translate more later.
### Supported Tasks and Leaderboards
### Languages
- 'finnish'
## Dataset Structure
### Data Instances
One example from the 'Finnish' dataset is given below in JSON format.
### Data Fields
- 'id': A string representing the article ID, matched to the XLSum dataset original
- 'url': A string representing the article URL as in the original XLSum dataset
- 'title': A string containing the article title, machine-translated to Finnish
- 'summary': A string containing the article summary, machine-translated to Finnish
- 'text' : A string containing the article text, machine-translated to Finnish
### Data Splits
Follows the XLSum dataset.
## Dataset Creation
### Curation Rationale
### Source Data
BBC News
#### Initial Data Collection and Normalization
Detailed in the paper For this present dataset, only English was used as the source and only examples where the full text is at maximum 10x in length compared to the summary are preserved. This 10x cutoff is naturally measured on English.
#### Who are the source language producers?
Detailed in the paper
### Annotations
Detailed in the paper DeepL was used to machine-translate from English to Finnish
#### Annotation process
Detailed in the paper
#### Who are the annotators?
Detailed in the paper
### Personal and Sensitive Information
More information needed
## Considerations for Using the Data
### Social Impact of Dataset
### Discussion of Biases
### Other Known Limitations
Due to DeepL terms and conditions, this dataset must not be used for any machine translation work, namely machine translation system development and evaluation of any kind. In general, we wish you do not pair the original English data with the translations except when working on research unrelated to machine translation, so as not to infringe on the terms and conditions.
## Additional Information
### Dataset Curators
### Licensing Information
Contents of this repository are restricted to only non-commercial research purposes under the Creative Commons Attribution-NonCommercial-ShareAlike 4.0 International License (CC BY-NC-SA 4.0). Copyright of the dataset contents belongs to the original copyright holders.
If you use any of the datasets, models or code modules, please cite the original XL-Sum paper below as well as acknowledge Filip Ginter and the TurkuNLP group for the Finnish machine translated version.
### Contributions
Thanks to the creators of the XLSum dataset! | [
"# Dataset Card for \"XL-Sum-FI\"",
"## Table of Contents\n- Dataset Card Creation Guide\n - Table of Contents\n - Dataset Description\n - Dataset Summary\n - Supported Tasks and Leaderboards\n - Languages\n - Dataset Structure\n - Data Instances\n - Data Fields\n - Data Splits\n - Dataset Creation\n - Curation Rationale\n - Source Data\n - Initial Data Collection and Normalization\n - Who are the source language producers?\n - Annotations\n - Annotation process\n - Who are the annotators?\n - Personal and Sensitive Information\n - Considerations for Using the Data\n - Social Impact of Dataset\n - Discussion of Biases\n - Other Known Limitations\n - Additional Information\n - Dataset Curators\n - Licensing Information\n - Citation Information\n - Contributions",
"## Dataset Description\n\n- Repository: URL\n- Point of Contact: Filip Ginter",
"### Dataset Summary\n\nThis dataset is a DeepL -based machine translation of a part of the English section of the XLSum dataset:URL In the present version, only examples where the full version is at most 10x the summary in length are included. We might translate more later.",
"### Supported Tasks and Leaderboards",
"### Languages\n\n- 'finnish'",
"## Dataset Structure",
"### Data Instances\n\nOne example from the 'Finnish' dataset is given below in JSON format.",
"### Data Fields\n- 'id': A string representing the article ID, matched to the XLSum dataset original\n- 'url': A string representing the article URL as in the original XLSum dataset\n- 'title': A string containing the article title, machine-translated to Finnish\n- 'summary': A string containing the article summary, machine-translated to Finnish\n- 'text' : A string containing the article text, machine-translated to Finnish",
"### Data Splits\n\nFollows the XLSum dataset.",
"## Dataset Creation",
"### Curation Rationale",
"### Source Data\n\nBBC News",
"#### Initial Data Collection and Normalization\n\nDetailed in the paper For this present dataset, only English was used as the source and only examples where the full text is at maximum 10x in length compared to the summary are preserved. This 10x cutoff is naturally measured on English.",
"#### Who are the source language producers?\n\nDetailed in the paper",
"### Annotations\n\nDetailed in the paper DeepL was used to machine-translate from English to Finnish",
"#### Annotation process\n\nDetailed in the paper",
"#### Who are the annotators?\n\nDetailed in the paper",
"### Personal and Sensitive Information\n\nMore information needed",
"## Considerations for Using the Data",
"### Social Impact of Dataset",
"### Discussion of Biases",
"### Other Known Limitations\n\nDue to DeepL terms and conditions, this dataset must not be used for any machine translation work, namely machine translation system development and evaluation of any kind. In general, we wish you do not pair the original English data with the translations except when working on research unrelated to machine translation, so as not to infringe on the terms and conditions.",
"## Additional Information",
"### Dataset Curators",
"### Licensing Information\n\nContents of this repository are restricted to only non-commercial research purposes under the Creative Commons Attribution-NonCommercial-ShareAlike 4.0 International License (CC BY-NC-SA 4.0). Copyright of the dataset contents belongs to the original copyright holders.\n\n\n\nIf you use any of the datasets, models or code modules, please cite the original XL-Sum paper below as well as acknowledge Filip Ginter and the TurkuNLP group for the Finnish machine translated version.",
"### Contributions\n\nThanks to the creators of the XLSum dataset!"
] | [
"TAGS\n#task_categories-summarization #task_categories-text2text-generation #annotations_creators-found #language_creators-machine translated #multilinguality-monolingual #size_categories-10K<n<100K #source_datasets-xlsum #language-Finnish #license-cc-by-nc-sa-4.0 #conditional-text-generation #region-us \n",
"# Dataset Card for \"XL-Sum-FI\"",
"## Table of Contents\n- Dataset Card Creation Guide\n - Table of Contents\n - Dataset Description\n - Dataset Summary\n - Supported Tasks and Leaderboards\n - Languages\n - Dataset Structure\n - Data Instances\n - Data Fields\n - Data Splits\n - Dataset Creation\n - Curation Rationale\n - Source Data\n - Initial Data Collection and Normalization\n - Who are the source language producers?\n - Annotations\n - Annotation process\n - Who are the annotators?\n - Personal and Sensitive Information\n - Considerations for Using the Data\n - Social Impact of Dataset\n - Discussion of Biases\n - Other Known Limitations\n - Additional Information\n - Dataset Curators\n - Licensing Information\n - Citation Information\n - Contributions",
"## Dataset Description\n\n- Repository: URL\n- Point of Contact: Filip Ginter",
"### Dataset Summary\n\nThis dataset is a DeepL -based machine translation of a part of the English section of the XLSum dataset:URL In the present version, only examples where the full version is at most 10x the summary in length are included. We might translate more later.",
"### Supported Tasks and Leaderboards",
"### Languages\n\n- 'finnish'",
"## Dataset Structure",
"### Data Instances\n\nOne example from the 'Finnish' dataset is given below in JSON format.",
"### Data Fields\n- 'id': A string representing the article ID, matched to the XLSum dataset original\n- 'url': A string representing the article URL as in the original XLSum dataset\n- 'title': A string containing the article title, machine-translated to Finnish\n- 'summary': A string containing the article summary, machine-translated to Finnish\n- 'text' : A string containing the article text, machine-translated to Finnish",
"### Data Splits\n\nFollows the XLSum dataset.",
"## Dataset Creation",
"### Curation Rationale",
"### Source Data\n\nBBC News",
"#### Initial Data Collection and Normalization\n\nDetailed in the paper For this present dataset, only English was used as the source and only examples where the full text is at maximum 10x in length compared to the summary are preserved. This 10x cutoff is naturally measured on English.",
"#### Who are the source language producers?\n\nDetailed in the paper",
"### Annotations\n\nDetailed in the paper DeepL was used to machine-translate from English to Finnish",
"#### Annotation process\n\nDetailed in the paper",
"#### Who are the annotators?\n\nDetailed in the paper",
"### Personal and Sensitive Information\n\nMore information needed",
"## Considerations for Using the Data",
"### Social Impact of Dataset",
"### Discussion of Biases",
"### Other Known Limitations\n\nDue to DeepL terms and conditions, this dataset must not be used for any machine translation work, namely machine translation system development and evaluation of any kind. In general, we wish you do not pair the original English data with the translations except when working on research unrelated to machine translation, so as not to infringe on the terms and conditions.",
"## Additional Information",
"### Dataset Curators",
"### Licensing Information\n\nContents of this repository are restricted to only non-commercial research purposes under the Creative Commons Attribution-NonCommercial-ShareAlike 4.0 International License (CC BY-NC-SA 4.0). Copyright of the dataset contents belongs to the original copyright holders.\n\n\n\nIf you use any of the datasets, models or code modules, please cite the original XL-Sum paper below as well as acknowledge Filip Ginter and the TurkuNLP group for the Finnish machine translated version.",
"### Contributions\n\nThanks to the creators of the XLSum dataset!"
] | [
106,
13,
162,
18,
64,
10,
9,
6,
25,
113,
14,
5,
7,
6,
65,
15,
24,
10,
14,
11,
8,
7,
8,
81,
5,
6,
116,
18
] | [
"passage: TAGS\n#task_categories-summarization #task_categories-text2text-generation #annotations_creators-found #language_creators-machine translated #multilinguality-monolingual #size_categories-10K<n<100K #source_datasets-xlsum #language-Finnish #license-cc-by-nc-sa-4.0 #conditional-text-generation #region-us \n# Dataset Card for \"XL-Sum-FI\"## Table of Contents\n- Dataset Card Creation Guide\n - Table of Contents\n - Dataset Description\n - Dataset Summary\n - Supported Tasks and Leaderboards\n - Languages\n - Dataset Structure\n - Data Instances\n - Data Fields\n - Data Splits\n - Dataset Creation\n - Curation Rationale\n - Source Data\n - Initial Data Collection and Normalization\n - Who are the source language producers?\n - Annotations\n - Annotation process\n - Who are the annotators?\n - Personal and Sensitive Information\n - Considerations for Using the Data\n - Social Impact of Dataset\n - Discussion of Biases\n - Other Known Limitations\n - Additional Information\n - Dataset Curators\n - Licensing Information\n - Citation Information\n - Contributions## Dataset Description\n\n- Repository: URL\n- Point of Contact: Filip Ginter### Dataset Summary\n\nThis dataset is a DeepL -based machine translation of a part of the English section of the XLSum dataset:URL In the present version, only examples where the full version is at most 10x the summary in length are included. We might translate more later.### Supported Tasks and Leaderboards### Languages\n\n- 'finnish'## Dataset Structure### Data Instances\n\nOne example from the 'Finnish' dataset is given below in JSON format."
] |
5e63d4fc3c1140553c27f8db01e881011147b0b6 | This dataset was pushed to Hub through the UI. | Besedo/random-dataset-10000 | [
"region:us"
] | 2022-09-30T12:36:11+00:00 | {} | 2022-09-30T14:27:40+00:00 | [] | [] | TAGS
#region-us
| This dataset was pushed to Hub through the UI. | [] | [
"TAGS\n#region-us \n"
] | [
6
] | [
"passage: TAGS\n#region-us \n"
] |
882bcea9e7a2a6c83e55fee2f9021b4bdf4f95f2 | This dataset was programmatically uploaded to this repo using huggingface-hub Python API | Besedo/random-dataset-1000000 | [
"region:us"
] | 2022-09-30T12:55:38+00:00 | {} | 2022-09-30T14:25:51+00:00 | [] | [] | TAGS
#region-us
| This dataset was programmatically uploaded to this repo using huggingface-hub Python API | [] | [
"TAGS\n#region-us \n"
] | [
6
] | [
"passage: TAGS\n#region-us \n"
] |
27624246741bea210f5f437820169dc2e39d41d4 |
# Dataset Card for MSMARCO - Natural Language Generation Task
## Table of Contents
- [Table of Contents](#table-of-contents)
- [Dataset Description](#dataset-description)
- [Dataset Summary](#dataset-summary)
- [Supported Tasks and Leaderboards](#supported-tasks-and-leaderboards)
- [Languages](#languages)
- [Dataset Structure](#dataset-structure)
- [Data Instances](#data-instances)
- [Data Fields](#data-fields)
- [Data Splits](#data-splits)
- [Dataset Creation](#dataset-creation)
- [Curation Rationale](#curation-rationale)
- [Annotations](#annotations)
- [Additional Information](#additional-information)
- [Licensing Information](#licensing-information)
- [Citation Information](#citation-information)
- [Contributions](#contributions)
## Dataset Description
- **Homepage:** https://microsoft.github.io/msmarco/
- **Repository:** https://github.com/microsoft/MSMARCO-Question-Answering
- **Paper:** https://arxiv.org/abs/1611.09268
- **Leaderboard:** https://microsoft.github.io/msmarco#qnadataset
### Dataset Summary
The original focus of MSMARCO was to provide a corpus for training and testing systems which given a real domain user query systems would then provide the most likley candidate answer and do so in language which was natural and conversational. All questions have been generated from real anonymized Bing user queries which grounds the dataset in a real world problem and can provide researchers real contrainsts their models might be used in. The context passages, from which the answers in the dataset are derived, are extracted from real web documents using the most advanced version of the Bing search engine. The answers to the queries are human generated.
### Supported Tasks and Leaderboards
Question Answering & Natural Language Generation. [Leaderboard](https://microsoft.github.io/msmarco#qnadataset)
### Languages
- English
## Dataset Structure
### Data Instances
```py
{
"query_id":604568,
"query":"what county is columbus city in",
"passages":[
{
"is_selected":0,
"passage_text":"WELCOME TO COLUMBUS! The City of Columbus includes a mix of residential, rural and commercial property. Columbus boasts large tracts of public land, including Carlos Avery Wildlife Management Area and Lamprey Pass.",
"url":"http://www.ci.columbus.mn.us/"
},
{
"is_selected":0,
"passage_text":"The ratio of number of residents in Columbus to the number of sex offenders is 488 to 1. The number of registered sex offenders compared to the number of residents in this city is near the state average. Nearest city with pop. 50,000+: Bloomington, IN (33.3 miles , pop. 69,291).",
"url":"http://www.city-data.com/city/Columbus-Indiana.html"
},
{
"is_selected":0,
"passage_text":"Phone Number: Columbus-Muscogee, the first consolidated city-county in Georgia, began development in 1826, building on ceded Creek Indian territory. Muscogee is the name of a branch of the Creek Nation. Columbus, of course, is named for Christopher Columbus.",
"url":"https://georgia.gov/cities-counties/columbus-muscogee-county"
},
{
"is_selected":1,
"passage_text":"Sponsored Topics. Columbus ( /kษlสmbษs/) is a city in and the county seat of Bartholomew County, Indiana, United States. The population was 44,061 at the 2010 census, and the current mayor is Fred Armstrong. Located approximately 40 miles (64 km) south of Indianapolis, on the east fork of the White River, it is the state's 20th largest city.",
"url":"https://www.mapquest.com/us/in/columbus-282032817"
},
{
"is_selected":0,
"passage_text":"Columbus, Ohio. Columbus (/kษหlสmbษs/; kษ-LUM-bษs) is the capital and largest city of the U.S. state of Ohio. It is the 15th-largest city in the United States, with a population of 850,106 as of 2015 estimates. This makes Columbus the fourth-most populous state capital in the United States, and the third-largest city in the Midwestern United States.",
"url":"https://en.wikipedia.org/wiki/Columbus,_Ohio"
},
{
"is_selected":0,
"passage_text":"Phone Number: Columbus-Muscogee, the first consolidated city-county in Georgia, began development in 1826, building on ceded Creek Indian territory. Muscogee is the name of a branch of the Creek Nation. Columbus, of course, is named for Christopher Columbus.",
"url":"https://georgia.gov/cities-counties/columbus"
},
{
"is_selected":0,
"passage_text":"Latest news from Columbus, IN collected exclusively by city-data.com from local newspapers, TV, and radio stations. Ancestries: American (30.5%), German (13.7%), English (7.7%), Irish (5.3%), European (2.4%), Scottish (1.2%).",
"url":"http://www.city-data.com/city/Columbus-Indiana.html"
},
{
"is_selected":0,
"passage_text":"Columbus, Indiana. 1 Columbus: covered Bridge at Mill Race Park. 2 Columbus: A statue in cloumbus. 3 Columbus. Columbus: Bartholomew County Courthouse. Columbus: Tipton Lakes - A wonderful planned 1 community! Columbus: Barthalomew county memorial for veterans. Columbus: A sculpter called summer storm in 1 columbus. Columbus: Downtown Columbus.",
"url":"http://www.city-data.com/city/Columbus-Indiana.html"
},
{
"is_selected":0,
"passage_text":"The City owns and operates a volunteer fire department through a joint powers agreement with the City of Forest Lake. Police protection is provided through a contract with the Anoka County Sheriffโs Department. Columbus is located within the Forest Lake Area School District (ISD #831).",
"url":"http://www.ci.columbus.mn.us/"
},
{
"is_selected":0,
"passage_text":"Acceptable ID for children: State ID, Birth Certificate, or Health Insurance Card. Effective June 27, 2016, the Franklin County Sheriff's Office will be implementing changes to ensure the safety of inmates, staff, and visitors. Printed materials (magazines, books, pamphlets, leaflets, or catalogues) MUST fit all the below criteria:",
"url":"https://sheriff.franklincountyohio.gov/services/inmate-information.cfm"
}
],
"query_type":"LOCATION",
"answers":[
"Columbus is a city in Bartholomew County."
]
}
```
### Data Fields
- `query_id`: a unique id for each query that is used in evaluation
- `query`: a unique query based on initial Bing usage
- `passages`: a list of 10 passages (`passage_text`), URLs (`url`), and an annotation if they were used to formulate the answer (`is_selected`)
- `query_type`: a basic division of queries based on a trained classifier (`LOCATION`,`NUMERIC`,`PERSON`,`DESCRIPTION`,`ENTITY`)
- `answers`: a list of "well-formed" answers generated by human annotators using natural language
### Data Splits
| **Split** | **Instances** |
|-----------|---------------|
| Train | 153725 |
| Dev | 12467 |
## Dataset Creation
### Curation Rationale
What is the differences between MSMARCO and other MRC datasets?
- Real questions: All questions have been sampled from real anonymized bing queries.
- Real Documents: Most of the URLs that the passages were sourced from contain the full web documents (passages).
- Human Generated Well-Formed Answers: All questions have an answer written by a human in natural language.
### Annotations
#### Annotation process
The MSMARCO dataset is generated by a well oiled pipeline optimized for the highest quality examples. The general process runs as follows:
1. Bing logs are sampled, filtered and anonymized to make sure the queries are both useful to the research community and respectful to bing users and fans.
2. Using the sampled and anonymized queries Bing generates the 10 most relevant passages for the query.
3. Highly trained judges read the query and its related passages and if there is an answer present, the supporting passages are annotated and a natural language answer is generated.
4. A smaller proportion of queries(~17% of overall dataset with 182,887 unique queries) are then passed on to a second round of judges who are asked to verify the answer is correct and rewrite(if possible) the query to be a well formed answer. These answers are designed to be understood without perfect context and are designed with smart speakers/digital assistants in mind.
## Additional Information
### Licensing Information
MS MARCO is licensed under a [Creative Commons Attribution 4.0 International License](http://creativecommons.org/licenses/by/4.0/).
### Citation Information
```
@inproceedings{Bajaj2016Msmarco,
title={MS MARCO: A Human Generated MAchine Reading COmprehension Dataset},
author={Payal Bajaj, Daniel Campos, Nick Craswell, Li Deng, Jianfeng Gao, Xiaodong Liu, Rangan Majumder, Andrew McNamara, Bhaskar Mitra, Tri Nguyen, Mir Rosenberg, Xia Song, Alina Stoica, Saurabh Tiwary, Tong Wang},
booktitle={InCoCo@NIPS},
year={2016}
}
```
### Contributions
Thanks to [@din0s](https://github.com/din0s) for adding this dataset. | din0s/msmarco-nlgen | [
"task_categories:question-answering",
"task_ids:open-domain-qa",
"annotations_creators:expert-generated",
"language_creators:crowdsourced",
"multilinguality:monolingual",
"size_categories:100K<n<1M",
"source_datasets:extended|ms_marco",
"language:en",
"license:cc-by-4.0",
"msmarco",
"natural language generation",
"question answering",
"arxiv:1611.09268",
"region:us"
] | 2022-09-30T13:06:45+00:00 | {"annotations_creators": ["expert-generated"], "language_creators": ["crowdsourced"], "language": ["en"], "license": ["cc-by-4.0"], "multilinguality": ["monolingual"], "size_categories": ["100K<n<1M"], "source_datasets": ["extended|ms_marco"], "task_categories": ["question-answering"], "task_ids": ["open-domain-qa"], "pretty_name": "MSMARCO NLGEN", "tags": ["msmarco", "natural language generation", "question answering"]} | 2022-10-01T11:30:18+00:00 | [
"1611.09268"
] | [
"en"
] | TAGS
#task_categories-question-answering #task_ids-open-domain-qa #annotations_creators-expert-generated #language_creators-crowdsourced #multilinguality-monolingual #size_categories-100K<n<1M #source_datasets-extended|ms_marco #language-English #license-cc-by-4.0 #msmarco #natural language generation #question answering #arxiv-1611.09268 #region-us
| Dataset Card for MSMARCO - Natural Language Generation Task
===========================================================
Table of Contents
-----------------
* Table of Contents
* Dataset Description
+ Dataset Summary
+ Supported Tasks and Leaderboards
+ Languages
* Dataset Structure
+ Data Instances
+ Data Fields
+ Data Splits
* Dataset Creation
+ Curation Rationale
+ Annotations
* Additional Information
+ Licensing Information
+ Citation Information
+ Contributions
Dataset Description
-------------------
* Homepage: URL
* Repository: URL
* Paper: URL
* Leaderboard: URL
### Dataset Summary
The original focus of MSMARCO was to provide a corpus for training and testing systems which given a real domain user query systems would then provide the most likley candidate answer and do so in language which was natural and conversational. All questions have been generated from real anonymized Bing user queries which grounds the dataset in a real world problem and can provide researchers real contrainsts their models might be used in. The context passages, from which the answers in the dataset are derived, are extracted from real web documents using the most advanced version of the Bing search engine. The answers to the queries are human generated.
### Supported Tasks and Leaderboards
Question Answering & Natural Language Generation. Leaderboard
### Languages
* English
Dataset Structure
-----------------
### Data Instances
### Data Fields
* 'query\_id': a unique id for each query that is used in evaluation
* 'query': a unique query based on initial Bing usage
* 'passages': a list of 10 passages ('passage\_text'), URLs ('url'), and an annotation if they were used to formulate the answer ('is\_selected')
* 'query\_type': a basic division of queries based on a trained classifier ('LOCATION','NUMERIC','PERSON','DESCRIPTION','ENTITY')
* 'answers': a list of "well-formed" answers generated by human annotators using natural language
### Data Splits
Dataset Creation
----------------
### Curation Rationale
What is the differences between MSMARCO and other MRC datasets?
* Real questions: All questions have been sampled from real anonymized bing queries.
* Real Documents: Most of the URLs that the passages were sourced from contain the full web documents (passages).
* Human Generated Well-Formed Answers: All questions have an answer written by a human in natural language.
### Annotations
#### Annotation process
The MSMARCO dataset is generated by a well oiled pipeline optimized for the highest quality examples. The general process runs as follows:
1. Bing logs are sampled, filtered and anonymized to make sure the queries are both useful to the research community and respectful to bing users and fans.
2. Using the sampled and anonymized queries Bing generates the 10 most relevant passages for the query.
3. Highly trained judges read the query and its related passages and if there is an answer present, the supporting passages are annotated and a natural language answer is generated.
4. A smaller proportion of queries(~17% of overall dataset with 182,887 unique queries) are then passed on to a second round of judges who are asked to verify the answer is correct and rewrite(if possible) the query to be a well formed answer. These answers are designed to be understood without perfect context and are designed with smart speakers/digital assistants in mind.
Additional Information
----------------------
### Licensing Information
MS MARCO is licensed under a Creative Commons Attribution 4.0 International License.
### Contributions
Thanks to @din0s for adding this dataset.
| [
"### Dataset Summary\n\n\nThe original focus of MSMARCO was to provide a corpus for training and testing systems which given a real domain user query systems would then provide the most likley candidate answer and do so in language which was natural and conversational. All questions have been generated from real anonymized Bing user queries which grounds the dataset in a real world problem and can provide researchers real contrainsts their models might be used in. The context passages, from which the answers in the dataset are derived, are extracted from real web documents using the most advanced version of the Bing search engine. The answers to the queries are human generated.",
"### Supported Tasks and Leaderboards\n\n\nQuestion Answering & Natural Language Generation. Leaderboard",
"### Languages\n\n\n* English\n\n\nDataset Structure\n-----------------",
"### Data Instances",
"### Data Fields\n\n\n* 'query\\_id': a unique id for each query that is used in evaluation\n* 'query': a unique query based on initial Bing usage\n* 'passages': a list of 10 passages ('passage\\_text'), URLs ('url'), and an annotation if they were used to formulate the answer ('is\\_selected')\n* 'query\\_type': a basic division of queries based on a trained classifier ('LOCATION','NUMERIC','PERSON','DESCRIPTION','ENTITY')\n* 'answers': a list of \"well-formed\" answers generated by human annotators using natural language",
"### Data Splits\n\n\n\nDataset Creation\n----------------",
"### Curation Rationale\n\n\nWhat is the differences between MSMARCO and other MRC datasets?\n\n\n* Real questions: All questions have been sampled from real anonymized bing queries.\n* Real Documents: Most of the URLs that the passages were sourced from contain the full web documents (passages).\n* Human Generated Well-Formed Answers: All questions have an answer written by a human in natural language.",
"### Annotations",
"#### Annotation process\n\n\nThe MSMARCO dataset is generated by a well oiled pipeline optimized for the highest quality examples. The general process runs as follows:\n\n\n1. Bing logs are sampled, filtered and anonymized to make sure the queries are both useful to the research community and respectful to bing users and fans.\n2. Using the sampled and anonymized queries Bing generates the 10 most relevant passages for the query.\n3. Highly trained judges read the query and its related passages and if there is an answer present, the supporting passages are annotated and a natural language answer is generated.\n4. A smaller proportion of queries(~17% of overall dataset with 182,887 unique queries) are then passed on to a second round of judges who are asked to verify the answer is correct and rewrite(if possible) the query to be a well formed answer. These answers are designed to be understood without perfect context and are designed with smart speakers/digital assistants in mind.\n\n\nAdditional Information\n----------------------",
"### Licensing Information\n\n\nMS MARCO is licensed under a Creative Commons Attribution 4.0 International License.",
"### Contributions\n\n\nThanks to @din0s for adding this dataset."
] | [
"TAGS\n#task_categories-question-answering #task_ids-open-domain-qa #annotations_creators-expert-generated #language_creators-crowdsourced #multilinguality-monolingual #size_categories-100K<n<1M #source_datasets-extended|ms_marco #language-English #license-cc-by-4.0 #msmarco #natural language generation #question answering #arxiv-1611.09268 #region-us \n",
"### Dataset Summary\n\n\nThe original focus of MSMARCO was to provide a corpus for training and testing systems which given a real domain user query systems would then provide the most likley candidate answer and do so in language which was natural and conversational. All questions have been generated from real anonymized Bing user queries which grounds the dataset in a real world problem and can provide researchers real contrainsts their models might be used in. The context passages, from which the answers in the dataset are derived, are extracted from real web documents using the most advanced version of the Bing search engine. The answers to the queries are human generated.",
"### Supported Tasks and Leaderboards\n\n\nQuestion Answering & Natural Language Generation. Leaderboard",
"### Languages\n\n\n* English\n\n\nDataset Structure\n-----------------",
"### Data Instances",
"### Data Fields\n\n\n* 'query\\_id': a unique id for each query that is used in evaluation\n* 'query': a unique query based on initial Bing usage\n* 'passages': a list of 10 passages ('passage\\_text'), URLs ('url'), and an annotation if they were used to formulate the answer ('is\\_selected')\n* 'query\\_type': a basic division of queries based on a trained classifier ('LOCATION','NUMERIC','PERSON','DESCRIPTION','ENTITY')\n* 'answers': a list of \"well-formed\" answers generated by human annotators using natural language",
"### Data Splits\n\n\n\nDataset Creation\n----------------",
"### Curation Rationale\n\n\nWhat is the differences between MSMARCO and other MRC datasets?\n\n\n* Real questions: All questions have been sampled from real anonymized bing queries.\n* Real Documents: Most of the URLs that the passages were sourced from contain the full web documents (passages).\n* Human Generated Well-Formed Answers: All questions have an answer written by a human in natural language.",
"### Annotations",
"#### Annotation process\n\n\nThe MSMARCO dataset is generated by a well oiled pipeline optimized for the highest quality examples. The general process runs as follows:\n\n\n1. Bing logs are sampled, filtered and anonymized to make sure the queries are both useful to the research community and respectful to bing users and fans.\n2. Using the sampled and anonymized queries Bing generates the 10 most relevant passages for the query.\n3. Highly trained judges read the query and its related passages and if there is an answer present, the supporting passages are annotated and a natural language answer is generated.\n4. A smaller proportion of queries(~17% of overall dataset with 182,887 unique queries) are then passed on to a second round of judges who are asked to verify the answer is correct and rewrite(if possible) the query to be a well formed answer. These answers are designed to be understood without perfect context and are designed with smart speakers/digital assistants in mind.\n\n\nAdditional Information\n----------------------",
"### Licensing Information\n\n\nMS MARCO is licensed under a Creative Commons Attribution 4.0 International License.",
"### Contributions\n\n\nThanks to @din0s for adding this dataset."
] | [
124,
143,
20,
13,
6,
169,
11,
94,
5,
236,
21,
17
] | [
"passage: TAGS\n#task_categories-question-answering #task_ids-open-domain-qa #annotations_creators-expert-generated #language_creators-crowdsourced #multilinguality-monolingual #size_categories-100K<n<1M #source_datasets-extended|ms_marco #language-English #license-cc-by-4.0 #msmarco #natural language generation #question answering #arxiv-1611.09268 #region-us \n### Dataset Summary\n\n\nThe original focus of MSMARCO was to provide a corpus for training and testing systems which given a real domain user query systems would then provide the most likley candidate answer and do so in language which was natural and conversational. All questions have been generated from real anonymized Bing user queries which grounds the dataset in a real world problem and can provide researchers real contrainsts their models might be used in. The context passages, from which the answers in the dataset are derived, are extracted from real web documents using the most advanced version of the Bing search engine. The answers to the queries are human generated.### Supported Tasks and Leaderboards\n\n\nQuestion Answering & Natural Language Generation. Leaderboard### Languages\n\n\n* English\n\n\nDataset Structure\n-----------------### Data Instances### Data Fields\n\n\n* 'query\\_id': a unique id for each query that is used in evaluation\n* 'query': a unique query based on initial Bing usage\n* 'passages': a list of 10 passages ('passage\\_text'), URLs ('url'), and an annotation if they were used to formulate the answer ('is\\_selected')\n* 'query\\_type': a basic division of queries based on a trained classifier ('LOCATION','NUMERIC','PERSON','DESCRIPTION','ENTITY')\n* 'answers': a list of \"well-formed\" answers generated by human annotators using natural language### Data Splits\n\n\n\nDataset Creation\n----------------"
] |
5e3ddde521c24727a134e4825d2927de25784c41 |
# Dataset Card for Lipogram-e
## Table of Contents
- [Table of Contents](#table-of-contents)
- [Dataset Description](#dataset-description)
- [Dataset Summary](#dataset-summary)
- [Supported Tasks and Leaderboards](#supported-tasks-and-leaderboards)
- [Languages](#languages)
- [Dataset Structure](#dataset-structure)
- [Data Instances](#data-instances)
- [Data Fields](#data-fields)
- [Data Splits](#data-splits)
- [Dataset Creation](#dataset-creation)
- [Curation Rationale](#curation-rationale)
- [Source Data](#source-data)
- [Annotations](#annotations)
- [Personal and Sensitive Information](#personal-and-sensitive-information)
- [Considerations for Using the Data](#considerations-for-using-the-data)
- [Social Impact of Dataset](#social-impact-of-dataset)
- [Discussion of Biases](#discussion-of-biases)
- [Other Known Limitations](#other-known-limitations)
- [Additional Information](#additional-information)
- [Dataset Curators](#dataset-curators)
- [Licensing Information](#licensing-information)
- [Citation Information](#citation-information)
- [Contributions](#contributions)
## Dataset Description
- **Homepage**: https://github.com/Hellisotherpeople/Constrained-Text-Generation-Studio
- **Repository**: https://github.com/Hellisotherpeople/Constrained-Text-Generation-Studio
- **Paper** Most Language Models can be Poets too: An AI Writing Assistant
and Constrained Text Generation Studio
- **Leaderboard**: https://github.com/Hellisotherpeople/Constrained-Text-Generation-Studio
- **Point of Contact**: https://www.linkedin.com/in/allen-roush-27721011b/
### Dataset Summary

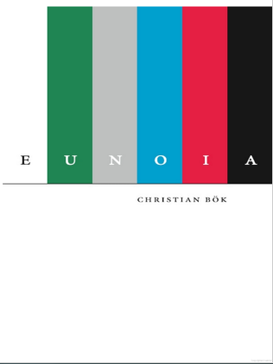

This is a dataset of 3 English books which do not contain the letter "e" in them. This dataset includes all of "Gadsby" by Ernest Vincent Wright, all of "A Void" by Georges Perec, and almost all of "Eunoia" by Christian Bok (except for the single chapter that uses the letter "e" in it)
This dataset is contributed as part of a paper titled "Most Language Models can be Poets too: An AI Writing Assistant and Constrained Text Generation Studio" to appear at COLING 2022.
This dataset and the works within them are examples of Lipograms, which are works where a letter or string is systematically omitted. Lipograms are an example of hard-constrained writing.
### Supported Tasks and Leaderboards
The main task for this dataset is Constrained Text Generation - but all types of language modeling are suitable.
### Languages
English
## Dataset Structure
### Data Instances
Each is extracted directly from the available pdf or epub documents converted to txt using pandoc.
### Data Fields
Text. The name of each work appears before the work starts and again at the end, so the books can be trivially split again if necessary.
### Data Splits
None given. The way I do so in the paper is to extract the final 20% of each book, and concatenate these together. This may not be the most ideal way to do a train/test split, but I couldn't think of a better way. I did not believe randomly sampling was appropriate, but I could be wrong.
## Dataset Creation
### Curation Rationale
One way that we could extract text from datasets that doesn't use the letter "e" in it would be to simply computationally parse through large existing datasets for blocks or sentences which don't have the letter "e" in them. Unfortunately, this is extremely unlikely to lead to coherent or meaningful text. Doing so over increasingly large blocks or spans is likely to result in fewer and fewer examples. While the preparation of such a dataset would be fascinating in its own right - it is more interesting from the perspective of fine-tuning language models to have large scale prose narratives which fulfill the given constraint. This constraint of omitting the letter "e" is attractive because several book length works exist which do this.
### Source Data
#### Initial Data Collection and Normalization
Project Gutenberg
#### Who are the source language producers?
Ernest Vincent Wright
Georges Perec
Christian Bok
### Annotations
#### Annotation process
None
#### Who are the annotators?
n/a
### Personal and Sensitive Information
None
## Considerations for Using the Data
There may be conversion artifacts. I noticed 3 cases of the letter "e" being hallucinated from the pdf conversion of "a void" that I had to fix manually. They were reading special characters as the letter "e", and were not due to the authors making mistakes themselves. This implies that at least a few OCR errors exist.
### Social Impact of Dataset
These books have existed for a awhile now, so it's unlikely that this will have dramatic Social Impact.
### Discussion of Biases
This dataset is 100% biased against the letter "e". There may be biases present in contents of these works. It's recommended to read the books before using this in any non research application to verify that they are not problematic.
### Other Known Limitations
It's possible that more works exist but were not well known enough for the authors to find them and include them. Finding such inclusions would be grounds for iteration of this dataset (e.g. a version 1.1 would be released). The goal of this project is to eventually encompass all book length english language "e" lipograms.
## Additional Information
n/a
### Dataset Curators
Allen Roush
### Licensing Information
MIT
### Citation Information
TBA
### Contributions
Thanks to [@Hellisotherpeople](https://github.com/Hellisotherpeople) for adding this dataset.
| Hellisotherpeople/Lipogram-e | [
"task_categories:text-generation",
"task_categories:fill-mask",
"task_ids:language-modeling",
"task_ids:masked-language-modeling",
"annotations_creators:no-annotation",
"language_creators:expert-generated",
"multilinguality:monolingual",
"size_categories:100K<n<1M",
"source_datasets:original",
"language:en",
"license:mit",
"ctgs",
"CTGS",
"constrained-text-generation",
"lipogram",
"i-hate-the-letter-e",
"region:us"
] | 2022-09-30T16:04:19+00:00 | {"annotations_creators": ["no-annotation"], "language_creators": ["expert-generated"], "language": ["en"], "license": ["mit"], "multilinguality": ["monolingual"], "size_categories": ["100K<n<1M"], "source_datasets": ["original"], "task_categories": ["text-generation", "fill-mask"], "task_ids": ["language-modeling", "masked-language-modeling"], "pretty_name": "Lipogram-e from Most Language Models can be Poets too: An AI Writing Assistant and Constrained Text Generation Studio", "tags": ["ctgs", "CTGS", "constrained-text-generation", "lipogram", "i-hate-the-letter-e"]} | 2022-09-30T17:04:43+00:00 | [] | [
"en"
] | TAGS
#task_categories-text-generation #task_categories-fill-mask #task_ids-language-modeling #task_ids-masked-language-modeling #annotations_creators-no-annotation #language_creators-expert-generated #multilinguality-monolingual #size_categories-100K<n<1M #source_datasets-original #language-English #license-mit #ctgs #CTGS #constrained-text-generation #lipogram #i-hate-the-letter-e #region-us
|
# Dataset Card for Lipogram-e
## Table of Contents
- Table of Contents
- Dataset Description
- Dataset Summary
- Supported Tasks and Leaderboards
- Languages
- Dataset Structure
- Data Instances
- Data Fields
- Data Splits
- Dataset Creation
- Curation Rationale
- Source Data
- Annotations
- Personal and Sensitive Information
- Considerations for Using the Data
- Social Impact of Dataset
- Discussion of Biases
- Other Known Limitations
- Additional Information
- Dataset Curators
- Licensing Information
- Citation Information
- Contributions
## Dataset Description
- Homepage: URL
- Repository: URL
- Paper Most Language Models can be Poets too: An AI Writing Assistant
and Constrained Text Generation Studio
- Leaderboard: URL
- Point of Contact: URL
### Dataset Summary
!Gadsby
!Eunoia
!A Void
This is a dataset of 3 English books which do not contain the letter "e" in them. This dataset includes all of "Gadsby" by Ernest Vincent Wright, all of "A Void" by Georges Perec, and almost all of "Eunoia" by Christian Bok (except for the single chapter that uses the letter "e" in it)
This dataset is contributed as part of a paper titled "Most Language Models can be Poets too: An AI Writing Assistant and Constrained Text Generation Studio" to appear at COLING 2022.
This dataset and the works within them are examples of Lipograms, which are works where a letter or string is systematically omitted. Lipograms are an example of hard-constrained writing.
### Supported Tasks and Leaderboards
The main task for this dataset is Constrained Text Generation - but all types of language modeling are suitable.
### Languages
English
## Dataset Structure
### Data Instances
Each is extracted directly from the available pdf or epub documents converted to txt using pandoc.
### Data Fields
Text. The name of each work appears before the work starts and again at the end, so the books can be trivially split again if necessary.
### Data Splits
None given. The way I do so in the paper is to extract the final 20% of each book, and concatenate these together. This may not be the most ideal way to do a train/test split, but I couldn't think of a better way. I did not believe randomly sampling was appropriate, but I could be wrong.
## Dataset Creation
### Curation Rationale
One way that we could extract text from datasets that doesn't use the letter "e" in it would be to simply computationally parse through large existing datasets for blocks or sentences which don't have the letter "e" in them. Unfortunately, this is extremely unlikely to lead to coherent or meaningful text. Doing so over increasingly large blocks or spans is likely to result in fewer and fewer examples. While the preparation of such a dataset would be fascinating in its own right - it is more interesting from the perspective of fine-tuning language models to have large scale prose narratives which fulfill the given constraint. This constraint of omitting the letter "e" is attractive because several book length works exist which do this.
### Source Data
#### Initial Data Collection and Normalization
Project Gutenberg
#### Who are the source language producers?
Ernest Vincent Wright
Georges Perec
Christian Bok
### Annotations
#### Annotation process
None
#### Who are the annotators?
n/a
### Personal and Sensitive Information
None
## Considerations for Using the Data
There may be conversion artifacts. I noticed 3 cases of the letter "e" being hallucinated from the pdf conversion of "a void" that I had to fix manually. They were reading special characters as the letter "e", and were not due to the authors making mistakes themselves. This implies that at least a few OCR errors exist.
### Social Impact of Dataset
These books have existed for a awhile now, so it's unlikely that this will have dramatic Social Impact.
### Discussion of Biases
This dataset is 100% biased against the letter "e". There may be biases present in contents of these works. It's recommended to read the books before using this in any non research application to verify that they are not problematic.
### Other Known Limitations
It's possible that more works exist but were not well known enough for the authors to find them and include them. Finding such inclusions would be grounds for iteration of this dataset (e.g. a version 1.1 would be released). The goal of this project is to eventually encompass all book length english language "e" lipograms.
## Additional Information
n/a
### Dataset Curators
Allen Roush
### Licensing Information
MIT
TBA
### Contributions
Thanks to @Hellisotherpeople for adding this dataset.
| [
"# Dataset Card for Lipogram-e",
"## Table of Contents\n- Table of Contents\n- Dataset Description\n - Dataset Summary\n - Supported Tasks and Leaderboards\n - Languages\n- Dataset Structure\n - Data Instances\n - Data Fields\n - Data Splits\n- Dataset Creation\n - Curation Rationale\n - Source Data\n - Annotations\n - Personal and Sensitive Information\n- Considerations for Using the Data\n - Social Impact of Dataset\n - Discussion of Biases\n - Other Known Limitations\n- Additional Information\n - Dataset Curators\n - Licensing Information\n - Citation Information\n - Contributions",
"## Dataset Description\n\n- Homepage: URL\n- Repository: URL\n- Paper Most Language Models can be Poets too: An AI Writing Assistant\nand Constrained Text Generation Studio\n- Leaderboard: URL\n- Point of Contact: URL",
"### Dataset Summary\n\n!Gadsby\n!Eunoia\n!A Void\n\nThis is a dataset of 3 English books which do not contain the letter \"e\" in them. This dataset includes all of \"Gadsby\" by Ernest Vincent Wright, all of \"A Void\" by Georges Perec, and almost all of \"Eunoia\" by Christian Bok (except for the single chapter that uses the letter \"e\" in it) \n\nThis dataset is contributed as part of a paper titled \"Most Language Models can be Poets too: An AI Writing Assistant and Constrained Text Generation Studio\" to appear at COLING 2022. \n\nThis dataset and the works within them are examples of Lipograms, which are works where a letter or string is systematically omitted. Lipograms are an example of hard-constrained writing.",
"### Supported Tasks and Leaderboards\n\nThe main task for this dataset is Constrained Text Generation - but all types of language modeling are suitable.",
"### Languages\n\nEnglish",
"## Dataset Structure",
"### Data Instances\n\nEach is extracted directly from the available pdf or epub documents converted to txt using pandoc.",
"### Data Fields\n\nText. The name of each work appears before the work starts and again at the end, so the books can be trivially split again if necessary.",
"### Data Splits\n\nNone given. The way I do so in the paper is to extract the final 20% of each book, and concatenate these together. This may not be the most ideal way to do a train/test split, but I couldn't think of a better way. I did not believe randomly sampling was appropriate, but I could be wrong.",
"## Dataset Creation",
"### Curation Rationale\n\nOne way that we could extract text from datasets that doesn't use the letter \"e\" in it would be to simply computationally parse through large existing datasets for blocks or sentences which don't have the letter \"e\" in them. Unfortunately, this is extremely unlikely to lead to coherent or meaningful text. Doing so over increasingly large blocks or spans is likely to result in fewer and fewer examples. While the preparation of such a dataset would be fascinating in its own right - it is more interesting from the perspective of fine-tuning language models to have large scale prose narratives which fulfill the given constraint. This constraint of omitting the letter \"e\" is attractive because several book length works exist which do this.",
"### Source Data",
"#### Initial Data Collection and Normalization\n\nProject Gutenberg",
"#### Who are the source language producers?\nErnest Vincent Wright\nGeorges Perec\nChristian Bok",
"### Annotations",
"#### Annotation process\n\nNone",
"#### Who are the annotators?\n\nn/a",
"### Personal and Sensitive Information\n\nNone",
"## Considerations for Using the Data\n\nThere may be conversion artifacts. I noticed 3 cases of the letter \"e\" being hallucinated from the pdf conversion of \"a void\" that I had to fix manually. They were reading special characters as the letter \"e\", and were not due to the authors making mistakes themselves. This implies that at least a few OCR errors exist.",
"### Social Impact of Dataset\n\nThese books have existed for a awhile now, so it's unlikely that this will have dramatic Social Impact.",
"### Discussion of Biases\n\nThis dataset is 100% biased against the letter \"e\". There may be biases present in contents of these works. It's recommended to read the books before using this in any non research application to verify that they are not problematic.",
"### Other Known Limitations\n\nIt's possible that more works exist but were not well known enough for the authors to find them and include them. Finding such inclusions would be grounds for iteration of this dataset (e.g. a version 1.1 would be released). The goal of this project is to eventually encompass all book length english language \"e\" lipograms.",
"## Additional Information\nn/a",
"### Dataset Curators\n\nAllen Roush",
"### Licensing Information\n\nMIT\n\n\nTBA",
"### Contributions\n\nThanks to @Hellisotherpeople for adding this dataset."
] | [
"TAGS\n#task_categories-text-generation #task_categories-fill-mask #task_ids-language-modeling #task_ids-masked-language-modeling #annotations_creators-no-annotation #language_creators-expert-generated #multilinguality-monolingual #size_categories-100K<n<1M #source_datasets-original #language-English #license-mit #ctgs #CTGS #constrained-text-generation #lipogram #i-hate-the-letter-e #region-us \n",
"# Dataset Card for Lipogram-e",
"## Table of Contents\n- Table of Contents\n- Dataset Description\n - Dataset Summary\n - Supported Tasks and Leaderboards\n - Languages\n- Dataset Structure\n - Data Instances\n - Data Fields\n - Data Splits\n- Dataset Creation\n - Curation Rationale\n - Source Data\n - Annotations\n - Personal and Sensitive Information\n- Considerations for Using the Data\n - Social Impact of Dataset\n - Discussion of Biases\n - Other Known Limitations\n- Additional Information\n - Dataset Curators\n - Licensing Information\n - Citation Information\n - Contributions",
"## Dataset Description\n\n- Homepage: URL\n- Repository: URL\n- Paper Most Language Models can be Poets too: An AI Writing Assistant\nand Constrained Text Generation Studio\n- Leaderboard: URL\n- Point of Contact: URL",
"### Dataset Summary\n\n!Gadsby\n!Eunoia\n!A Void\n\nThis is a dataset of 3 English books which do not contain the letter \"e\" in them. This dataset includes all of \"Gadsby\" by Ernest Vincent Wright, all of \"A Void\" by Georges Perec, and almost all of \"Eunoia\" by Christian Bok (except for the single chapter that uses the letter \"e\" in it) \n\nThis dataset is contributed as part of a paper titled \"Most Language Models can be Poets too: An AI Writing Assistant and Constrained Text Generation Studio\" to appear at COLING 2022. \n\nThis dataset and the works within them are examples of Lipograms, which are works where a letter or string is systematically omitted. Lipograms are an example of hard-constrained writing.",
"### Supported Tasks and Leaderboards\n\nThe main task for this dataset is Constrained Text Generation - but all types of language modeling are suitable.",
"### Languages\n\nEnglish",
"## Dataset Structure",
"### Data Instances\n\nEach is extracted directly from the available pdf or epub documents converted to txt using pandoc.",
"### Data Fields\n\nText. The name of each work appears before the work starts and again at the end, so the books can be trivially split again if necessary.",
"### Data Splits\n\nNone given. The way I do so in the paper is to extract the final 20% of each book, and concatenate these together. This may not be the most ideal way to do a train/test split, but I couldn't think of a better way. I did not believe randomly sampling was appropriate, but I could be wrong.",
"## Dataset Creation",
"### Curation Rationale\n\nOne way that we could extract text from datasets that doesn't use the letter \"e\" in it would be to simply computationally parse through large existing datasets for blocks or sentences which don't have the letter \"e\" in them. Unfortunately, this is extremely unlikely to lead to coherent or meaningful text. Doing so over increasingly large blocks or spans is likely to result in fewer and fewer examples. While the preparation of such a dataset would be fascinating in its own right - it is more interesting from the perspective of fine-tuning language models to have large scale prose narratives which fulfill the given constraint. This constraint of omitting the letter \"e\" is attractive because several book length works exist which do this.",
"### Source Data",
"#### Initial Data Collection and Normalization\n\nProject Gutenberg",
"#### Who are the source language producers?\nErnest Vincent Wright\nGeorges Perec\nChristian Bok",
"### Annotations",
"#### Annotation process\n\nNone",
"#### Who are the annotators?\n\nn/a",
"### Personal and Sensitive Information\n\nNone",
"## Considerations for Using the Data\n\nThere may be conversion artifacts. I noticed 3 cases of the letter \"e\" being hallucinated from the pdf conversion of \"a void\" that I had to fix manually. They were reading special characters as the letter \"e\", and were not due to the authors making mistakes themselves. This implies that at least a few OCR errors exist.",
"### Social Impact of Dataset\n\nThese books have existed for a awhile now, so it's unlikely that this will have dramatic Social Impact.",
"### Discussion of Biases\n\nThis dataset is 100% biased against the letter \"e\". There may be biases present in contents of these works. It's recommended to read the books before using this in any non research application to verify that they are not problematic.",
"### Other Known Limitations\n\nIt's possible that more works exist but were not well known enough for the authors to find them and include them. Finding such inclusions would be grounds for iteration of this dataset (e.g. a version 1.1 would be released). The goal of this project is to eventually encompass all book length english language \"e\" lipograms.",
"## Additional Information\nn/a",
"### Dataset Curators\n\nAllen Roush",
"### Licensing Information\n\nMIT\n\n\nTBA",
"### Contributions\n\nThanks to @Hellisotherpeople for adding this dataset."
] | [
141,
9,
125,
48,
187,
34,
5,
6,
28,
37,
80,
5,
177,
4,
13,
19,
5,
7,
12,
10,
86,
33,
60,
84,
8,
9,
9,
18
] | [
"passage: TAGS\n#task_categories-text-generation #task_categories-fill-mask #task_ids-language-modeling #task_ids-masked-language-modeling #annotations_creators-no-annotation #language_creators-expert-generated #multilinguality-monolingual #size_categories-100K<n<1M #source_datasets-original #language-English #license-mit #ctgs #CTGS #constrained-text-generation #lipogram #i-hate-the-letter-e #region-us \n# Dataset Card for Lipogram-e## Table of Contents\n- Table of Contents\n- Dataset Description\n - Dataset Summary\n - Supported Tasks and Leaderboards\n - Languages\n- Dataset Structure\n - Data Instances\n - Data Fields\n - Data Splits\n- Dataset Creation\n - Curation Rationale\n - Source Data\n - Annotations\n - Personal and Sensitive Information\n- Considerations for Using the Data\n - Social Impact of Dataset\n - Discussion of Biases\n - Other Known Limitations\n- Additional Information\n - Dataset Curators\n - Licensing Information\n - Citation Information\n - Contributions## Dataset Description\n\n- Homepage: URL\n- Repository: URL\n- Paper Most Language Models can be Poets too: An AI Writing Assistant\nand Constrained Text Generation Studio\n- Leaderboard: URL\n- Point of Contact: URL",
"passage: ### Dataset Summary\n\n!Gadsby\n!Eunoia\n!A Void\n\nThis is a dataset of 3 English books which do not contain the letter \"e\" in them. This dataset includes all of \"Gadsby\" by Ernest Vincent Wright, all of \"A Void\" by Georges Perec, and almost all of \"Eunoia\" by Christian Bok (except for the single chapter that uses the letter \"e\" in it) \n\nThis dataset is contributed as part of a paper titled \"Most Language Models can be Poets too: An AI Writing Assistant and Constrained Text Generation Studio\" to appear at COLING 2022. \n\nThis dataset and the works within them are examples of Lipograms, which are works where a letter or string is systematically omitted. Lipograms are an example of hard-constrained writing.### Supported Tasks and Leaderboards\n\nThe main task for this dataset is Constrained Text Generation - but all types of language modeling are suitable.### Languages\n\nEnglish## Dataset Structure### Data Instances\n\nEach is extracted directly from the available pdf or epub documents converted to txt using pandoc.### Data Fields\n\nText. The name of each work appears before the work starts and again at the end, so the books can be trivially split again if necessary.### Data Splits\n\nNone given. The way I do so in the paper is to extract the final 20% of each book, and concatenate these together. This may not be the most ideal way to do a train/test split, but I couldn't think of a better way. I did not believe randomly sampling was appropriate, but I could be wrong.## Dataset Creation### Curation Rationale\n\nOne way that we could extract text from datasets that doesn't use the letter \"e\" in it would be to simply computationally parse through large existing datasets for blocks or sentences which don't have the letter \"e\" in them. Unfortunately, this is extremely unlikely to lead to coherent or meaningful text. Doing so over increasingly large blocks or spans is likely to result in fewer and fewer examples. While the preparation of such a dataset would be fascinating in its own right - it is more interesting from the perspective of fine-tuning language models to have large scale prose narratives which fulfill the given constraint. This constraint of omitting the letter \"e\" is attractive because several book length works exist which do this.### Source Data#### Initial Data Collection and Normalization\n\nProject Gutenberg#### Who are the source language producers?\nErnest Vincent Wright\nGeorges Perec\nChristian Bok### Annotations#### Annotation process\n\nNone#### Who are the annotators?\n\nn/a### Personal and Sensitive Information\n\nNone"
] |
d3264617542ec95d20eab292cb2b227beacc3c53 | # Dataset Card for "lener_br_finetuning_language_model"
[More Information needed](https://github.com/huggingface/datasets/blob/main/CONTRIBUTING.md#how-to-contribute-to-the-dataset-cards) | luciolrv/lener_br_finetuning_language_model | [
"region:us"
] | 2022-09-30T20:46:00+00:00 | {"dataset_info": {"features": [{"name": "text", "dtype": "string"}], "splits": [{"name": "train", "num_bytes": 1544086, "num_examples": 2659}, {"name": "validation", "num_bytes": 284559, "num_examples": 665}], "download_size": 1013297, "dataset_size": 1828645}} | 2023-06-11T14:57:37+00:00 | [] | [] | TAGS
#region-us
| # Dataset Card for "lener_br_finetuning_language_model"
More Information needed | [
"# Dataset Card for \"lener_br_finetuning_language_model\"\n\nMore Information needed"
] | [
"TAGS\n#region-us \n",
"# Dataset Card for \"lener_br_finetuning_language_model\"\n\nMore Information needed"
] | [
6,
22
] | [
"passage: TAGS\n#region-us \n# Dataset Card for \"lener_br_finetuning_language_model\"\n\nMore Information needed"
] |
c797997d442273e284644de093e2e4ff9419632a |
# Dataset Card for "lmqg/qg_frquad"
***IMPORTANT***: This is a dummy dataset for [lmqg/qg_frquad](https://huggingface.co/datasets/lmqg/qg_frquad). The original FRQuAD requires to fill a form (https://fquad.illuin.tech/) to get the data, and our lmqg/qg_frquad follows FQuAD's license. If you need lmqg/qg_frquad, please first request the access to FQuAD on their website https://fquad.illuin.tech/ . Once you obtain the access, we will add you to our lmqg group so that you can access https://huggingface.co/datasets/lmqg/qg_frquad.
Leave a comment to the [discussion page](https://huggingface.co/datasets/lmqg/qg_frquad_dummy/discussions/1) to request access to the `lmqg/qg_frquad` after being granted FQuAD access!
## Dataset Description
- **Repository:** [https://github.com/asahi417/lm-question-generation](https://github.com/asahi417/lm-question-generation)
- **Paper:** [https://arxiv.org/abs/2210.03992](https://arxiv.org/abs/2210.03992)
- **Point of Contact:** [Asahi Ushio](http://asahiushio.com/)
### Dataset Summary
This is a subset of [QG-Bench](https://github.com/asahi417/lm-question-generation/blob/master/QG_BENCH.md#datasets), a unified question generation benchmark proposed in
["Generative Language Models for Paragraph-Level Question Generation: A Unified Benchmark and Evaluation, EMNLP 2022 main conference"](https://arxiv.org/abs/2210.03992).
This is a modified version of [FQuAD](https://huggingface.co/datasets/fquad) for question generation (QG) task.
Since the original dataset only contains training/validation set, we manually sample test set from training set, which
has no overlap in terms of the paragraph with the training set.
***IMPORTANT NOTE:*** The license of this dataset belongs to [FQuAD](https://fquad.illuin.tech/), so please check the guideline there and request the right to access the dataset [here](https://fquad.illuin.tech/) promptly if you use the datset.
### Supported Tasks and Leaderboards
* `question-generation`: The dataset is assumed to be used to train a model for question generation.
Success on this task is typically measured by achieving a high BLEU4/METEOR/ROUGE-L/BERTScore/MoverScore (see our paper for more in detail).
### Languages
French (fr)
## Dataset Structure
An example of 'train' looks as follows.
```
{
'answer': '16 janvier 1377',
'question': 'Quand est-ce que Grรฉgoire XI arrive ร Rome ?',
'sentence': "Le pape poursuit son voyage jusqu'ร Rome en passant par Corneto oรน il parvient le 6 dรฉcembre 1376, puis il arrive ร Rome le 16 janvier 1377 en remontant le Tibre.",
'paragraph': "Quant ร Catherine, elle part par voie terrestre en passant par Saint-Tropez, Varazze, puis Gรชnes. C'est dans cette derniรจre ville que, selon la Legenda minore, elle aurait de nouveau rencontrรฉ Grรฉgoire XI. Le pape poursuit son voyage jusqu'ร Rome en passant par Corneto oรน il parvient le 6 dรฉcembre 1376, puis il arrive ร Rome le 16 janvier 1377 en remontant le Tibre.",
'sentence_answer': "Le pape poursuit son voyage jusqu'ร Rome en passant par Corneto oรน il parvient le 6 dรฉcembre 1376, puis il arrive ร Rome le <hl> 16 janvier 1377 <hl> en remontant le Tibre.",
'paragraph_answer': "Quant ร Catherine, elle part par voie terrestre en passant par Saint-Tropez, Varazze, puis Gรชnes. C'est dans cette derniรจre ville que, selon la Legenda minore, elle aurait de nouveau rencontrรฉ Grรฉgoire XI. Le pape poursuit son voyage jusqu'ร Rome en passant par Corneto oรน il parvient le 6 dรฉcembre 1376, puis il arrive ร Rome le <hl> 16 janvier 1377 <hl> en remontant le Tibre.",
'paragraph_sentence': "Quant ร Catherine, elle part par voie terrestre en passant par Saint-Tropez, Varazze, puis Gรชnes. C'est dans cette derniรจre ville que, selon la Legenda minore, elle aurait de nouveau rencontrรฉ Grรฉgoire XI. <hl> Le pape poursuit son voyage jusqu'ร Rome en passant par Corneto oรน il parvient le 6 dรฉcembre 1376, puis il arrive ร Rome le 16 janvier 1377 en remontant le Tibre. <hl>"
}
```
The data fields are the same among all splits.
- `question`: a `string` feature.
- `paragraph`: a `string` feature.
- `answer`: a `string` feature.
- `sentence`: a `string` feature.
- `paragraph_answer`: a `string` feature, which is same as the paragraph but the answer is highlighted by a special token `<hl>`.
- `paragraph_sentence`: a `string` feature, which is same as the paragraph but a sentence containing the answer is highlighted by a special token `<hl>`.
- `sentence_answer`: a `string` feature, which is same as the sentence but the answer is highlighted by a special token `<hl>`.
Each of `paragraph_answer`, `paragraph_sentence`, and `sentence_answer` feature is assumed to be used to train a question generation model,
but with different information. The `paragraph_answer` and `sentence_answer` features are for answer-aware question generation and
`paragraph_sentence` feature is for sentence-aware question generation.
## Data Splits
|train|validation|test |
|----:|---------:|----:|
|17543| 3188 |3188 |
## Citation Information
```
@inproceedings{ushio-etal-2022-generative,
title = "{G}enerative {L}anguage {M}odels for {P}aragraph-{L}evel {Q}uestion {G}eneration: {A} {U}nified {B}enchmark and {E}valuation",
author = "Ushio, Asahi and
Alva-Manchego, Fernando and
Camacho-Collados, Jose",
booktitle = "Proceedings of the 2022 Conference on Empirical Methods in Natural Language Processing",
month = dec,
year = "2022",
address = "Abu Dhabi, U.A.E.",
publisher = "Association for Computational Linguistics",
}
``` | lmqg/qg_frquad_dummy | [
"task_categories:text2text-generation",
"multilinguality:monolingual",
"size_categories:10K<n<100K",
"source_datasets:fquad",
"language:fr",
"license:cc-by-4.0",
"question-generation",
"arxiv:2210.03992",
"region:us"
] | 2022-09-30T22:10:39+00:00 | {"language": "fr", "license": "cc-by-4.0", "multilinguality": "monolingual", "size_categories": "10K<n<100K", "source_datasets": "fquad", "task_categories": ["text2text-generation"], "task_ids": [], "pretty_name": "FQuAD for question generation", "tags": ["question-generation"]} | 2022-11-05T03:05:12+00:00 | [
"2210.03992"
] | [
"fr"
] | TAGS
#task_categories-text2text-generation #multilinguality-monolingual #size_categories-10K<n<100K #source_datasets-fquad #language-French #license-cc-by-4.0 #question-generation #arxiv-2210.03992 #region-us
| Dataset Card for "lmqg/qg\_frquad"
==================================
*IMPORTANT*: This is a dummy dataset for lmqg/qg\_frquad. The original FRQuAD requires to fill a form (URL to get the data, and our lmqg/qg\_frquad follows FQuAD's license. If you need lmqg/qg\_frquad, please first request the access to FQuAD on their website URL . Once you obtain the access, we will add you to our lmqg group so that you can access URL
Leave a comment to the discussion page to request access to the 'lmqg/qg\_frquad' after being granted FQuAD access!
Dataset Description
-------------------
* Repository: URL
* Paper: URL
* Point of Contact: Asahi Ushio
### Dataset Summary
This is a subset of QG-Bench, a unified question generation benchmark proposed in
"Generative Language Models for Paragraph-Level Question Generation: A Unified Benchmark and Evaluation, EMNLP 2022 main conference".
This is a modified version of FQuAD for question generation (QG) task.
Since the original dataset only contains training/validation set, we manually sample test set from training set, which
has no overlap in terms of the paragraph with the training set.
*IMPORTANT NOTE:* The license of this dataset belongs to FQuAD, so please check the guideline there and request the right to access the dataset here promptly if you use the datset.
### Supported Tasks and Leaderboards
* 'question-generation': The dataset is assumed to be used to train a model for question generation.
Success on this task is typically measured by achieving a high BLEU4/METEOR/ROUGE-L/BERTScore/MoverScore (see our paper for more in detail).
### Languages
French (fr)
Dataset Structure
-----------------
An example of 'train' looks as follows.
The data fields are the same among all splits.
* 'question': a 'string' feature.
* 'paragraph': a 'string' feature.
* 'answer': a 'string' feature.
* 'sentence': a 'string' feature.
* 'paragraph\_answer': a 'string' feature, which is same as the paragraph but the answer is highlighted by a special token ''.
* 'paragraph\_sentence': a 'string' feature, which is same as the paragraph but a sentence containing the answer is highlighted by a special token ''.
* 'sentence\_answer': a 'string' feature, which is same as the sentence but the answer is highlighted by a special token ''.
Each of 'paragraph\_answer', 'paragraph\_sentence', and 'sentence\_answer' feature is assumed to be used to train a question generation model,
but with different information. The 'paragraph\_answer' and 'sentence\_answer' features are for answer-aware question generation and
'paragraph\_sentence' feature is for sentence-aware question generation.
Data Splits
-----------
| [
"### Dataset Summary\n\n\nThis is a subset of QG-Bench, a unified question generation benchmark proposed in\n\"Generative Language Models for Paragraph-Level Question Generation: A Unified Benchmark and Evaluation, EMNLP 2022 main conference\".\nThis is a modified version of FQuAD for question generation (QG) task.\nSince the original dataset only contains training/validation set, we manually sample test set from training set, which\nhas no overlap in terms of the paragraph with the training set.\n\n\n*IMPORTANT NOTE:* The license of this dataset belongs to FQuAD, so please check the guideline there and request the right to access the dataset here promptly if you use the datset.",
"### Supported Tasks and Leaderboards\n\n\n* 'question-generation': The dataset is assumed to be used to train a model for question generation.\nSuccess on this task is typically measured by achieving a high BLEU4/METEOR/ROUGE-L/BERTScore/MoverScore (see our paper for more in detail).",
"### Languages\n\n\nFrench (fr)\n\n\nDataset Structure\n-----------------\n\n\nAn example of 'train' looks as follows.\n\n\nThe data fields are the same among all splits.\n\n\n* 'question': a 'string' feature.\n* 'paragraph': a 'string' feature.\n* 'answer': a 'string' feature.\n* 'sentence': a 'string' feature.\n* 'paragraph\\_answer': a 'string' feature, which is same as the paragraph but the answer is highlighted by a special token ''.\n* 'paragraph\\_sentence': a 'string' feature, which is same as the paragraph but a sentence containing the answer is highlighted by a special token ''.\n* 'sentence\\_answer': a 'string' feature, which is same as the sentence but the answer is highlighted by a special token ''.\n\n\nEach of 'paragraph\\_answer', 'paragraph\\_sentence', and 'sentence\\_answer' feature is assumed to be used to train a question generation model,\nbut with different information. The 'paragraph\\_answer' and 'sentence\\_answer' features are for answer-aware question generation and\n'paragraph\\_sentence' feature is for sentence-aware question generation.\n\n\nData Splits\n-----------"
] | [
"TAGS\n#task_categories-text2text-generation #multilinguality-monolingual #size_categories-10K<n<100K #source_datasets-fquad #language-French #license-cc-by-4.0 #question-generation #arxiv-2210.03992 #region-us \n",
"### Dataset Summary\n\n\nThis is a subset of QG-Bench, a unified question generation benchmark proposed in\n\"Generative Language Models for Paragraph-Level Question Generation: A Unified Benchmark and Evaluation, EMNLP 2022 main conference\".\nThis is a modified version of FQuAD for question generation (QG) task.\nSince the original dataset only contains training/validation set, we manually sample test set from training set, which\nhas no overlap in terms of the paragraph with the training set.\n\n\n*IMPORTANT NOTE:* The license of this dataset belongs to FQuAD, so please check the guideline there and request the right to access the dataset here promptly if you use the datset.",
"### Supported Tasks and Leaderboards\n\n\n* 'question-generation': The dataset is assumed to be used to train a model for question generation.\nSuccess on this task is typically measured by achieving a high BLEU4/METEOR/ROUGE-L/BERTScore/MoverScore (see our paper for more in detail).",
"### Languages\n\n\nFrench (fr)\n\n\nDataset Structure\n-----------------\n\n\nAn example of 'train' looks as follows.\n\n\nThe data fields are the same among all splits.\n\n\n* 'question': a 'string' feature.\n* 'paragraph': a 'string' feature.\n* 'answer': a 'string' feature.\n* 'sentence': a 'string' feature.\n* 'paragraph\\_answer': a 'string' feature, which is same as the paragraph but the answer is highlighted by a special token ''.\n* 'paragraph\\_sentence': a 'string' feature, which is same as the paragraph but a sentence containing the answer is highlighted by a special token ''.\n* 'sentence\\_answer': a 'string' feature, which is same as the sentence but the answer is highlighted by a special token ''.\n\n\nEach of 'paragraph\\_answer', 'paragraph\\_sentence', and 'sentence\\_answer' feature is assumed to be used to train a question generation model,\nbut with different information. The 'paragraph\\_answer' and 'sentence\\_answer' features are for answer-aware question generation and\n'paragraph\\_sentence' feature is for sentence-aware question generation.\n\n\nData Splits\n-----------"
] | [
79,
166,
80,
295
] | [
"passage: TAGS\n#task_categories-text2text-generation #multilinguality-monolingual #size_categories-10K<n<100K #source_datasets-fquad #language-French #license-cc-by-4.0 #question-generation #arxiv-2210.03992 #region-us \n### Dataset Summary\n\n\nThis is a subset of QG-Bench, a unified question generation benchmark proposed in\n\"Generative Language Models for Paragraph-Level Question Generation: A Unified Benchmark and Evaluation, EMNLP 2022 main conference\".\nThis is a modified version of FQuAD for question generation (QG) task.\nSince the original dataset only contains training/validation set, we manually sample test set from training set, which\nhas no overlap in terms of the paragraph with the training set.\n\n\n*IMPORTANT NOTE:* The license of this dataset belongs to FQuAD, so please check the guideline there and request the right to access the dataset here promptly if you use the datset.### Supported Tasks and Leaderboards\n\n\n* 'question-generation': The dataset is assumed to be used to train a model for question generation.\nSuccess on this task is typically measured by achieving a high BLEU4/METEOR/ROUGE-L/BERTScore/MoverScore (see our paper for more in detail)."
] |
5631a9bd17a096bab2cd02ea23adbf2327db0d91 | # namu.wiki database dump
##
https://namu.wiki/ database dump 2022/03/01<br/>
- 867024 rows
- download size: 3GB
## Usage
```bash
pip install datasets
```
```python
from datasets import load_dataset
dataset = load_dataset("heegyu/namuwiki")
print(dataset["train"][0])
```
```
{'title': '!!์์!!',
'text': '\n[๋ชฉ์ฐจ]\n\n\'\'\'{{{+1 ๏ผ๏ผใใใฃใจ๏ผ๏ผ}}}\'\'\'\n\n== ๊ฐ์ ==\n[[ํ์ผ:3444050440.jpg|width=60%]]\nโฒ[[์ ์ธ๊ณ์์ ๋ฏธ๊ถ 2 ํํ๋๋ฅด๊ธฐ์ฌ|์ ์ธ๊ณ์์ ๋ฏธ๊ถ 2]]์์ ๋ฌ !!์์!!\n\n[[์ธ๊ณ์์ ๋ฏธ๊ถ ์๋ฆฌ์ฆ]]์ ์ ํต์ผ๋ก ๋ฑ์ฅํ๋ ๋์ฌ. [[์ธ๊ณ์์ ๋ฏธ๊ถ 2 ์ ์์ ์ฑ๋ฐฐ|2ํธ]]๋ถํฐ ๋ฑ์ฅํ์ผ๋ฉฐ ํ๋ฅญํ [[์ฌ๋ง ํ๋๊ทธ]]์ ์์์ด๋ค.\n\n์ธ๊ณ์์ ๋ชจํ๊ฐ๋ค์ด ํํํ๋ ๋์ ์ธ ์ํด์ ๊ตฌ์๊ตฌ์์๋ ์ฑ์ทจ/๋ฒ์ฑ/์ฑ๊ตด ํฌ์ธํธ๊ฐ ์์ผ๋ฉฐ, ์ด๋ฅผ ์ํ ์ฑ์ง ์คํฌ์ ํฌ์ํ๋ฉด ์ ํ๋ ์ฑ์ง ๊ธฐํ์์ ๋ณด๋ค ํฐ ์ด๋์ ์ฑ๊ธธ ์ ์๋ค. ๊ทธ๋ฌ๋ ๋ถ๋ฐฐํ ์ ์๋ ์คํฌ ํฌ์ธํธ๋ ํ์ ๋์ด ์๊ธฐ ๋๋ฌธ์ ์ฑ์ง ์คํฌ์ ํฌ์ํ๋ ๋งํผ ์ ํฌ ์คํฌ ๋ ๋ฒจ์ ๋ฎ์์ง๊ฒ ๋๋ค.[* ๋ค๋ง ์ฑ์ง ์์คํ
์ ์ ์ธ๊ณ์ ์๋ฆฌ์ฆ์ ๊ทธ๋ฆฌ๋ชจ์ด ๋ณต์ , ๋ณตํฉ ์ฑ์ง ์คํฌ์ธ ์ผ์์ ๊ฐ, 5ํธ์ ์ข
์กฑ ํน์ ์คํฌ, ํฌ๋ก์ค์ 1๋ ๋ฒจ์ด ๋ง๋ ์ธ ์ฑ์ง ์คํฌ ๋ฑ์ผ๋ก ํธ์์ฑ์ด ์ ์ฐจ ๋์์ ธ์ ์ฑ์ง ์คํฌ ๋๋ฌธ์ ์คํฌ ํธ๋ฆฌ๊ฐ ๋ด๋ ค๊ฐ๋ ์ผ์ ์ ์ ์ค์ด๋ค์๋ค.] !!์์!!์ด ๋ฐ์ํ๋ ๊ณผ์ ์ ์์ฝํ๋ฉด ๋ค์๊ณผ ๊ฐ๋ค.\n\n 1. ์ฑ์ง์ฉ ์บ๋ฆญํฐ๋ค๋ก ์ด๋ฃจ์ด์ง ์ฝํ ํํฐ(ex: [[๋ ์ธ์ (์ธ๊ณ์์ ๋ฏธ๊ถ 2)|๋ ์ธ์ ]] 5๋ช
)๊ฐ ์ํด์ ์
์ฅํ๋ค.\n 1. ํ๋ ์ ํฌ๋ฅผ ํผํด ์ฑ์ง ํฌ์ธํธ์ ๋์ฐฉํ ํ ์ด์ฌํ ์์ดํ
์ ์บ๋ ์ค์...\n 1. \'\'\'!!์์!!\'\'\' ~~๋ผํ๋ ์์๊ฐ ๋ํ๋ฌ๋ค!~~\n ์ด๋ ๋ฑ์ฅํ๋ ๊ฒ์ [[FOE(์ธ๊ณ์์ ๋ฏธ๊ถ ์๋ฆฌ์ฆ)|FOE]]๋ ์๋์ง๋ง \'\'\'ํจ์ฌ ์์ธต์ ๋ฑ์ฅํ๋ ๊ฐ๋ ฅํ ํ๋ ๋ชฌ์คํฐ์ด๋ฉฐ ์ ์ ๊ณต๊ฒฉ์ ๋นํ๊ฒ ๋๋ค!\'\'\'\n 1. \'\'\'์ผ์ ์ฃฝ์\'\'\'(hage)\n\n์ฌ๋ด์ผ๋ก !!์์!!์ ์ ๋๋ 1์ธ์นญ ๋์ ํฌ๋กค๋ฌ์ ์์กฐ [[์์ ๋๋ฆฌ]]์์ ํจ์ ์ ๊ฑด๋๋ ธ์ ๋ ๋์ค๋ ๋์ฌ Oops!(ใใใฃใจ๏ผ)๋ผ๊ณ ํ๋ค.\n\n== ๊ฐ ์ํ์์์ ๋ชจ์ต ==\n=== [[์ธ๊ณ์์ ๋ฏธ๊ถ 2 ์ ์์ ์ฑ๋ฐฐ]] ===\n!!์์!!์ ์
๋ํจ์ ์ฒซ ๋ฑ์ฅํ ์ํ์ด์ ์๋ฆฌ์ฆ ์ค์์๋ ๋ถ์น์ ํ๊ธฐ๋ก ์ ํ์ด ๋ 2ํธ์ด ์ ์ ์ด์๋ค. ๊ทธ์ผ๋ง๋ก ์์ !!์์!! ์ํ์ค ๊ทธ๋๋ก, ๋ฌป์ง๋ ๋ฐ์ง์ง๋ ์๊ณ ์ฑ์งํ ๋๋ง๋ค ์ผ์ ํ๋ฅ ๋ก \'\'\'๊ฐ์ ๋ก\'\'\' ์ ํฌ์ ๋์
ํด์ผ ํ๋ค. ๊ฒ๋ค๊ฐ ์ด๋ด ๋ ์ฐ๋ผ๊ณ ์๋ ๋ ์ธ์ ์ ์คํฌ \'์ํ ๊ฐ์ง(์ค๊ฐ ํ๋ฅ ๋ก ์ ์ ์ ์ ๊ณต๊ฒฉ์ ๋ฌดํจํ)\'๋ ์ ์ ์๋ํ์ง ์๋๋ค!\n\n์ฐธ๊ณ ๋ก 2ํธ์์ ์ฑ์ง ๋์ค !!์์!!์ด ๋ฐ ํ๋ฅ ์ [[http://www.atlusnet.jp/topic/detail/910|๊ณ ์ 1%๋ค.]] [[๋ํํ๋ฅ ์ ๋ฒ์น|๋ฎ์ ๋ณด์ด๋ ํ๋ฅ ์ด์ด๋ ํ๋ ์ด ์ค ํ ๋ฒ์ด๋ผ๋ ์ผ์ด๋๋ ๊ฒ]]์ ๊ฒฝํํ๋ ์ฒด๊ฐ ํ๋ฅ ์ ๊ณ ๋ คํ์ฌ ํ๋ฅ ์ ์ค์ ํ๋ค๊ณ .\n\n=== [[์ธ๊ณ์์ ๋ฏธ๊ถ 3 ์ฑํด์ ๋ด๋ฐฉ์]] ===\n๋คํํ ์ฑ์ง ์ค ๋ฎ์ ํ๋ฅ ๋ก "์ข์ ์์ดํ
์ ์ป์ ์ ์์ ๊ฒ ๊ฐ์ง๋ง... ์ฃผ๋ณ์์ ๋ชฌ์คํฐ๋ค์ ๊ธฐ์ฒ์ด ๋๊ปด์ง๋ค."๋ ๋ฉ์์ง๊ฐ ๋จ๊ณ ์ด๋ ์ด์ด ์ข์ผ๋ฉด ๋ ์ด ์์ดํ
์ ์ป์ ์ ์์ง๋ง ๋ฐ๋์ ๊ฒฝ์ฐ ์ ๊ณผ ์ธ์ฐ๊ฒ ๋๋ ๊ฒ์ผ๋ก ์กฐ์ ๋์๋ค.\n\n=== [[์ธ๊ณ์์ ๋ฏธ๊ถ 4 ์ ์น์ ๊ฑฐ์ ]] ===\n๊ธฐ๋ณธ์ ์ธ ๊ฒ์ 3ํธ๊ณผ ๊ฐ์ง๋ง, 4ํธ์์๋ ์์ง์ด์ง ์๊ณ ์ฑ์งํ ๋๋ ํด์ด ๊ฒฝ๊ณผํ๋๋ก ์กฐ์ ๋์๊ธฐ ๋๋ฌธ์ ์ฃผ๋ณ์ ์๋ FOE๋ฅผ ์๊ณ ์ฑ์ง์ ๋ชฐ๋ํ๋ค๊ฐ FOE์ ๋ถ๋ชํ๋ฉด FOE ๋ฒ์ !!์์!!์ด ๋ฌ๋ค. ๊ทธ๋ฆฌ๊ณ ๋์ด๋ CASUAL๋ก ํ๋ ์ด์, FOE๋ก ์ธํ !!์์!!์ ์ ์ธํ๋ฉด ์ ๋๋ก ๋ฐ์ํ์ง ์๋๋ค.\n\n=== [[์ ์ธ๊ณ์์ ๋ฏธ๊ถ ๋ฐ๋ ๋์์ ์๋
|์ ์ธ๊ณ์์]] [[์ ์ธ๊ณ์์ ๋ฏธ๊ถ 2 ํํ๋๋ฅด๊ธฐ์ฌ|๋ฏธ๊ถ ์๋ฆฌ์ฆ]] ===\n์ฑ์ง ๋ฐฉ์์ด ํ ํด์ผ๋ก ๋๋๋ ๊ตฌ์กฐ[* ์ฑ์ง์ผ๋ก ํ ๋ฒ ์์ดํ
์ ํ๋ํ๋ฉด "๋ค์, (์ฑ์ง ์คํฌ)์ ์ํด..."๊ฐ ๋จ๋ฉด์ ํ๊บผ๋ฒ์ ํ๋๋๋ ๊ตฌ์กฐ.]๋ก ๋ฐ๋ ๋๋ถ์ธ์ง ๊ฐ์ ์กฐ์ฐ๋ก ๋ค์ ํ๊ทํด๋ฒ๋ ธ๋ค(...). ๊ทธ๋๋ง ์ํ ๊ฐ์ง ๋จนํต๊ณผ ๊ฐ์ ๋ฒ๊ทธ์ฑ ๋์ ๋ค์ ์์ ๋์๋ค. ๊ทธ ์ดํ์ ๋์จ [[์ธ๊ณ์์ ๋ฏธ๊ถ 5 ์ค๋ ์ ํ์ ๋]]๊ณผ ์๋ฆฌ์ฆ์ ์ง๋์ฑ ์ํ์ด์ 3DS ๋ง์ง๋ง ์ํ์ธ [[์ธ๊ณ์์ ๋ฏธ๊ถ X]]๋ ๋ง์ฐฌ๊ฐ์ง.\n\n=== [[์ธ๊ณ์์ ๋ฏธ๊ถ X]] ===\n๋ณธ์์ ์ฑ์ง์ ์ ์ธ๊ณ์ ์๋ฆฌ์ฆ์ ๊ฐ์ ๋งค์ปค๋์ฆ์ด๋ผ ๊ตณ์ด ์ธ๊ธํ ํ์๋ ์์ผ๋, ํ์คํธ์ค์ 2ํธ์ !!์์!! ์ํ์ค๋ฅผ ์ฌํํ๋ฉด์ \'\'\'๋ผํ๋ ์์\'\'\'๊ฐ ๋ฑ์ฅํ๋ ํ์คํธ๊ฐ ์กด์ฌํ๋ค.(...) ๊นจ์๊ฐ์ด ์์คํ
๋ฉ์ธ์ง ์ฐฝ์ด ์๋๋ผ ๋ํ์ฐฝ์ ์ด์ฉํด์ ์๋ฒฝ ์ฌํํ ๊ฒ์ด ํฌ์ธํธ.\n\n=== [[ํ๋ฅด์๋ Q ์๋์ฐ ์ค๋ธ ๋ ๋๋ฒ๋ฆฐ์ค]] ===\n์ธ๊ณ์ ์์คํ
์ ๊ธฐ๋ฐ์ผ๋ก ํ [[ํ๋ฅด์๋ ์๋ฆฌ์ฆ]]์์ ์ฝ๋ผ๋ณด ์ํ์ธ ํ๋ฅด์๋ Q์์๋ ๋ฑ์ฅํ๋ค. 3, 4ํธ๊ณผ ๊ฐ์ด ํ์ ์คํฟ์์ ์ฑ์ง ๋์ค ๋ฉ์์ง๊ฐ ๋จ๋ฉฐ, ์คํจํ๋ฉด ํํฐ์ ์ฐธ๊ฐํ๊ณ ์๋ ๋ฉค๋ฒ ์ค ํ ๋ช
์ [[http://nico.ms/sm25683358|!!์์!! ํ๋ ์์ฑ]] ~~๋๋ [[์ฝ๋ก๋ง๋ฃจ|๊ฐ์๋ฆฌ]]~~๊ณผ ํจ๊ป ๊ทธ ๋์ ์ \'๊ฐ์ \'์ธ ๊ฑฐ๋ [[์๋(ํ๋ฅด์๋ ์๋ฆฌ์ฆ)|์๋์ฐ]]๊ฐ ๋ํ๋๋ค.\n\n๊ทธ๋ฌ๋ ๋ด๋น ์ ์ฉ ์คํฌ์ธ ๋ฑ๋ ๋
ธ๋ ค๋ณด๊ธฐ(์ํ ๊ฐ์ง์ ๊ฐ์ ํจ๊ณผ)์ ์ฑ์ง ๋ณด์กฐ ์คํฌ์ ํํฐ์ ์ ํฌ๋ ฅ์ ์ ํ ์ง์ฅ์ ์ฃผ์ง ์์ผ๋ฉฐ, \'๋์์ฌ\'์ ๋ฌ๋ฉด ๊ฑฐ์ ๋ณผ ์ผ์ด ์์ด์ ธ์ ์ด์ค๋ฐ ์ดํ์๋ ์กด์ฌ๊ฐ์ด ๊ธ๊ฒฉํ ์ค์ด๋ ๋ค.\n[[๋ถ๋ฅ:์ธ๊ณ์์ ๋ฏธ๊ถ ์๋ฆฌ์ฆ]]',
'contributors': '110.46.34.123,kirby10,max0243,218.54.117.149,ruby3141,121.165.63.239,iviyuki,1.229.200.194,anatra95,kiri47,175.127.134.2,nickchaos71,chkong1998,kiwitree2,namubot,huwieblusnow',
'namespace': ''}
``` | heegyu/namuwiki | [
"task_categories:other",
"language_creators:other",
"multilinguality:monolingual",
"size_categories:100K<n<1M",
"language:ko",
"license:cc-by-nc-sa-2.0",
"region:us"
] | 2022-09-30T23:40:12+00:00 | {"language_creators": ["other"], "language": ["ko"], "license": "cc-by-nc-sa-2.0", "multilinguality": ["monolingual"], "size_categories": ["100K<n<1M"], "task_categories": ["other"]} | 2022-10-01T01:40:40+00:00 | [] | [
"ko"
] | TAGS
#task_categories-other #language_creators-other #multilinguality-monolingual #size_categories-100K<n<1M #language-Korean #license-cc-by-nc-sa-2.0 #region-us
| # URL database dump
##
URL database dump 2022/03/01<br/>
- 867024 rows
- download size: 3GB
## Usage
| [
"# URL database dump",
"## Usage"
] | [
"TAGS\n#task_categories-other #language_creators-other #multilinguality-monolingual #size_categories-100K<n<1M #language-Korean #license-cc-by-nc-sa-2.0 #region-us \n",
"# URL database dump",
"## Usage"
] | [
60,
5,
3
] | [
"passage: TAGS\n#task_categories-other #language_creators-other #multilinguality-monolingual #size_categories-100K<n<1M #language-Korean #license-cc-by-nc-sa-2.0 #region-us \n# URL database dump## Usage"
] |
Subsets and Splits
No community queries yet
The top public SQL queries from the community will appear here once available.