repo
stringclasses 1
value | number
int64 1
25.3k
| state
stringclasses 2
values | title
stringlengths 1
487
| body
stringlengths 0
234k
⌀ | created_at
stringlengths 19
19
| closed_at
stringlengths 19
19
| comments
stringlengths 0
293k
|
---|---|---|---|---|---|---|---|
transformers | 987 | closed | Generative finetuning | Example script for fine-tuning generative models such as GPT-2 using causal language modeling (CLM). Will eventually cover masked language modeling (MLM) for BERT and RoBERTa as well.
Edit (thom): Added `max_len_single_sentence` and `max_len_sentences_pair` properties to the tokenizer to easily access the max length taking into account the special tokens. | 08-07-2019 21:43:53 | 08-07-2019 21:43:53 | # [Codecov](https://codecov.io/gh/huggingface/pytorch-transformers/pull/987?src=pr&el=h1) Report
> Merging [#987](https://codecov.io/gh/huggingface/pytorch-transformers/pull/987?src=pr&el=desc) into [master](https://codecov.io/gh/huggingface/pytorch-transformers/commit/3566d2791905269b75014e8ea9db322c86f980b2?src=pr&el=desc) will **decrease** coverage by `0.12%`.
> The diff coverage is `77.66%`.
[](https://codecov.io/gh/huggingface/pytorch-transformers/pull/987?src=pr&el=tree)
```diff
@@ Coverage Diff @@
## master #987 +/- ##
==========================================
- Coverage 79.22% 79.09% -0.13%
==========================================
Files 38 42 +4
Lines 6406 6812 +406
==========================================
+ Hits 5075 5388 +313
- Misses 1331 1424 +93
```
| [Impacted Files](https://codecov.io/gh/huggingface/pytorch-transformers/pull/987?src=pr&el=tree) | Coverage Δ | |
|---|---|---|
| [pytorch\_transformers/tokenization\_xlnet.py](https://codecov.io/gh/huggingface/pytorch-transformers/pull/987/diff?src=pr&el=tree#diff-cHl0b3JjaF90cmFuc2Zvcm1lcnMvdG9rZW5pemF0aW9uX3hsbmV0LnB5) | `84.25% <28.57%> (-3.86%)` | :arrow_down: |
| [pytorch\_transformers/tokenization\_bert.py](https://codecov.io/gh/huggingface/pytorch-transformers/pull/987/diff?src=pr&el=tree#diff-cHl0b3JjaF90cmFuc2Zvcm1lcnMvdG9rZW5pemF0aW9uX2JlcnQucHk=) | `93.39% <33.33%> (-1.75%)` | :arrow_down: |
| [pytorch\_transformers/tokenization\_xlm.py](https://codecov.io/gh/huggingface/pytorch-transformers/pull/987/diff?src=pr&el=tree#diff-cHl0b3JjaF90cmFuc2Zvcm1lcnMvdG9rZW5pemF0aW9uX3hsbS5weQ==) | `79.67% <33.33%> (-2.38%)` | :arrow_down: |
| [pytorch\_transformers/tokenization\_utils.py](https://codecov.io/gh/huggingface/pytorch-transformers/pull/987/diff?src=pr&el=tree#diff-cHl0b3JjaF90cmFuc2Zvcm1lcnMvdG9rZW5pemF0aW9uX3V0aWxzLnB5) | `83.03% <42.3%> (-3.28%)` | :arrow_down: |
| [pytorch\_transformers/modeling\_roberta.py](https://codecov.io/gh/huggingface/pytorch-transformers/pull/987/diff?src=pr&el=tree#diff-cHl0b3JjaF90cmFuc2Zvcm1lcnMvbW9kZWxpbmdfcm9iZXJ0YS5weQ==) | `73.52% <73.52%> (ø)` | |
| [...ytorch\_transformers/tests/modeling\_roberta\_test.py](https://codecov.io/gh/huggingface/pytorch-transformers/pull/987/diff?src=pr&el=tree#diff-cHl0b3JjaF90cmFuc2Zvcm1lcnMvdGVzdHMvbW9kZWxpbmdfcm9iZXJ0YV90ZXN0LnB5) | `78.81% <78.81%> (ø)` | |
| [...ch\_transformers/tests/tokenization\_roberta\_test.py](https://codecov.io/gh/huggingface/pytorch-transformers/pull/987/diff?src=pr&el=tree#diff-cHl0b3JjaF90cmFuc2Zvcm1lcnMvdGVzdHMvdG9rZW5pemF0aW9uX3JvYmVydGFfdGVzdC5weQ==) | `90.24% <90.24%> (ø)` | |
| [pytorch\_transformers/tokenization\_roberta.py](https://codecov.io/gh/huggingface/pytorch-transformers/pull/987/diff?src=pr&el=tree#diff-cHl0b3JjaF90cmFuc2Zvcm1lcnMvdG9rZW5pemF0aW9uX3JvYmVydGEucHk=) | `92.45% <92.45%> (ø)` | |
| [pytorch\_transformers/file\_utils.py](https://codecov.io/gh/huggingface/pytorch-transformers/pull/987/diff?src=pr&el=tree#diff-cHl0b3JjaF90cmFuc2Zvcm1lcnMvZmlsZV91dGlscy5weQ==) | `71.22% <0%> (-2.88%)` | :arrow_down: |
| [pytorch\_transformers/modeling\_utils.py](https://codecov.io/gh/huggingface/pytorch-transformers/pull/987/diff?src=pr&el=tree#diff-cHl0b3JjaF90cmFuc2Zvcm1lcnMvbW9kZWxpbmdfdXRpbHMucHk=) | `83.24% <0%> (ø)` | :arrow_up: |
| ... and [5 more](https://codecov.io/gh/huggingface/pytorch-transformers/pull/987/diff?src=pr&el=tree-more) | |
------
[Continue to review full report at Codecov](https://codecov.io/gh/huggingface/pytorch-transformers/pull/987?src=pr&el=continue).
> **Legend** - [Click here to learn more](https://docs.codecov.io/docs/codecov-delta)
> `Δ = absolute <relative> (impact)`, `ø = not affected`, `? = missing data`
> Powered by [Codecov](https://codecov.io/gh/huggingface/pytorch-transformers/pull/987?src=pr&el=footer). Last update [3566d27...a448941](https://codecov.io/gh/huggingface/pytorch-transformers/pull/987?src=pr&el=lastupdated). Read the [comment docs](https://docs.codecov.io/docs/pull-request-comments).
<|||||># [Codecov](https://codecov.io/gh/huggingface/pytorch-transformers/pull/987?src=pr&el=h1) Report
> Merging [#987](https://codecov.io/gh/huggingface/pytorch-transformers/pull/987?src=pr&el=desc) into [master](https://codecov.io/gh/huggingface/pytorch-transformers/commit/e00b4ff1de0591d5093407b16e665e5c86028f04?src=pr&el=desc) will **increase** coverage by `0.04%`.
> The diff coverage is `100%`.
[](https://codecov.io/gh/huggingface/pytorch-transformers/pull/987?src=pr&el=tree)
```diff
@@ Coverage Diff @@
## master #987 +/- ##
==========================================
+ Coverage 79.61% 79.66% +0.04%
==========================================
Files 42 42
Lines 6898 6914 +16
==========================================
+ Hits 5492 5508 +16
Misses 1406 1406
```
| [Impacted Files](https://codecov.io/gh/huggingface/pytorch-transformers/pull/987?src=pr&el=tree) | Coverage Δ | |
|---|---|---|
| [pytorch\_transformers/tokenization\_xlnet.py](https://codecov.io/gh/huggingface/pytorch-transformers/pull/987/diff?src=pr&el=tree#diff-cHl0b3JjaF90cmFuc2Zvcm1lcnMvdG9rZW5pemF0aW9uX3hsbmV0LnB5) | `89.18% <100%> (+0.19%)` | :arrow_up: |
| [pytorch\_transformers/tokenization\_bert.py](https://codecov.io/gh/huggingface/pytorch-transformers/pull/987/diff?src=pr&el=tree#diff-cHl0b3JjaF90cmFuc2Zvcm1lcnMvdG9rZW5pemF0aW9uX2JlcnQucHk=) | `94.88% <100%> (+0.04%)` | :arrow_up: |
| [pytorch\_transformers/tokenization\_transfo\_xl.py](https://codecov.io/gh/huggingface/pytorch-transformers/pull/987/diff?src=pr&el=tree#diff-cHl0b3JjaF90cmFuc2Zvcm1lcnMvdG9rZW5pemF0aW9uX3RyYW5zZm9feGwucHk=) | `33.98% <100%> (+0.37%)` | :arrow_up: |
| [pytorch\_transformers/tokenization\_openai.py](https://codecov.io/gh/huggingface/pytorch-transformers/pull/987/diff?src=pr&el=tree#diff-cHl0b3JjaF90cmFuc2Zvcm1lcnMvdG9rZW5pemF0aW9uX29wZW5haS5weQ==) | `81.81% <100%> (+0.3%)` | :arrow_up: |
| [pytorch\_transformers/tokenization\_gpt2.py](https://codecov.io/gh/huggingface/pytorch-transformers/pull/987/diff?src=pr&el=tree#diff-cHl0b3JjaF90cmFuc2Zvcm1lcnMvdG9rZW5pemF0aW9uX2dwdDIucHk=) | `96.66% <100%> (+0.05%)` | :arrow_up: |
| [pytorch\_transformers/tokenization\_xlm.py](https://codecov.io/gh/huggingface/pytorch-transformers/pull/987/diff?src=pr&el=tree#diff-cHl0b3JjaF90cmFuc2Zvcm1lcnMvdG9rZW5pemF0aW9uX3hsbS5weQ==) | `83.33% <100%> (+0.26%)` | :arrow_up: |
| [pytorch\_transformers/tokenization\_utils.py](https://codecov.io/gh/huggingface/pytorch-transformers/pull/987/diff?src=pr&el=tree#diff-cHl0b3JjaF90cmFuc2Zvcm1lcnMvdG9rZW5pemF0aW9uX3V0aWxzLnB5) | `86.31% <100%> (+0.08%)` | :arrow_up: |
| [pytorch\_transformers/tokenization\_roberta.py](https://codecov.io/gh/huggingface/pytorch-transformers/pull/987/diff?src=pr&el=tree#diff-cHl0b3JjaF90cmFuc2Zvcm1lcnMvdG9rZW5pemF0aW9uX3JvYmVydGEucHk=) | `96.29% <100%> (+0.06%)` | :arrow_up: |
------
[Continue to review full report at Codecov](https://codecov.io/gh/huggingface/pytorch-transformers/pull/987?src=pr&el=continue).
> **Legend** - [Click here to learn more](https://docs.codecov.io/docs/codecov-delta)
> `Δ = absolute <relative> (impact)`, `ø = not affected`, `? = missing data`
> Powered by [Codecov](https://codecov.io/gh/huggingface/pytorch-transformers/pull/987?src=pr&el=footer). Last update [e00b4ff...06510cc](https://codecov.io/gh/huggingface/pytorch-transformers/pull/987?src=pr&el=lastupdated). Read the [comment docs](https://docs.codecov.io/docs/pull-request-comments).
<|||||>@LysandreJik I think we are good to merge this new script for fine-tuning Bert/RoBERTa/GPT and GPT-2, right?<|||||>As a heads-up, when testing this using `--model-type=gpt2` on WikiText-103, I get this:
```
08/23/2019 17:57:44 - WARNING - pytorch_transformers.tokenization_utils - Token indices sequence length is longer than the specified maximum sequence length for this model (119073253 > 1024). Running this sequence through the model will result in indexing errors
Traceback (most recent call last):
File "examples/run_lm_finetuning.py", line 501, in <module>
main()
File "examples/run_lm_finetuning.py", line 450, in main
train_dataset = load_and_cache_examples(args, tokenizer, evaluate=False)
File "examples/run_lm_finetuning.py", line 99, in load_and_cache_examples
dataset = TextDataset(tokenizer, file_path=args.eval_data_file if evaluate else args.train_data_file, block_size=args.block_size)
File "examples/run_lm_finetuning.py", line 75, in __init__
tokenized_text = tokenizer.add_special_tokens_single_sentence(tokenized_text)
File "~/.local/lib/python3.6/site-packages/pytorch_transformers/tokenization_utils.py", line 593, in add_special_tokens_single_sentence
raise NotImplementedError
NotImplementedError
```
I think it is happening because `GPT2Tokenizer` doesn't implement `add_special_tokens_single_sentence`, which is used directly by `run_lm_finetuning.py` in `TextDataset.__init__`.<|||||>Ok, this looks good to me! |
transformers | 986 | closed | Potential bug with gradient clipping when using gradient accumulation in examples | ## ❓ Questions & Help
Hi developpers,
Thanks for the awesome package. I have a question related to the recent major from pytorch_pretrained_bert to pytorch_transformers.
Gradient clipping used to be done inside the optimizer BertAdam and is now done at the same time as gradient computation in `run_squad.py` : https://github.com/huggingface/pytorch-transformers/blob/7729ef738161a0a182b172fcb7c351f6d2b9c50d/examples/run_squad.py#L156
It seems to me like the first accumulated gradients might get clipped several times hence giving more weight to last accumulated gradients :
As an example of my thought here is what happens if we compare what we get if we clip to 1 at each accumulation step instead of at the end of the accumulation for the two gradients [2,0] and [0,2]:
```python
In [1]: import torch
...: from torch.nn.utils import clip_grad_norm_
...: from torch.autograd import Variable
...:
...: x = Variable(torch.FloatTensor([[0],[0]]), requires_grad=True)
...:
...: grad1 = torch.FloatTensor([[2],[0]])
...: grad2 = torch.FloatTensor([[0],[2]])
...:
...: x.grad = grad1
...: clip_grad_norm_(x, 1)
...: print(x.grad)
...:
...: x.grad += grad2
...: clip_grad_norm_(x, 1)
...: print(x.grad)
...:
...: grad1 = torch.FloatTensor([[2],[0]])
...: grad2 = torch.FloatTensor([[0],[2]])
...:
...: x.grad = grad1 + grad2
...: clip_grad_norm_(x, 1)
...: print(x.grad)
...:
...:
tensor([[1.0000],
[0.0000]])
tensor([[0.4472],
[0.8944]])
tensor([[0.7071],
[0.7071]])```
We can see that clipping at each step biased gradient towards the gradient of the second batch:
`python tensor([[0.4472], [0.8944]])`
Instead of getting the balanced expected result : `python tensor([[0.7071], [0.7071]])`
So either I missed something or I think the fix would be to simply move gradient clipping before the call to the optimizer | 08-07-2019 14:59:58 | 08-07-2019 14:59:58 | Hi, indeed we could move the gradient clipping just before the call to the optimizer.
Do you want to send a PR to fix that on `run_squad` and `run_glue`?<|||||>Hi, was this ever implemented? I think it makes the most sense to clip right before an optimizer step. Right now it's implemented in two different ways in [run_lim_finetuning](https://github.com/huggingface/transformers/blob/master/examples/run_lm_finetuning.py) and [run_glue](https://github.com/huggingface/transformers/blob/master/examples/run_glue.py)<|||||>This issue has been automatically marked as stale because it has not had recent activity. It will be closed if no further activity occurs. Thank you for your contributions.
|
transformers | 985 | closed | Unable to read pre-trained model using BertModel.from_pretrained | I am using pre-trained BERT model kept at AWS s3 bucket. When i am trying to read the model using BertModel.from_pretrained It return NONE object. Things are working offline when i download a folder on the same location as my code resides. | 08-07-2019 14:31:51 | 08-07-2019 14:31:51 | Hi, you are trying to download one of your own models kept on a personal AWS s3 bucket or one of our models? What string do you pass to the `from_pretrained` method?<|||||>Thank you for your response,
I am trying to use my own model kept on my personal AWS s3 bucket.
The string to from_pretrained method is the path of folder which contains three files config , vocab and model. I have also tried to zip all files (.tar.gz) and use that file rather then folder but it also didn't work.
Please let me know in case you need more information.
<|||||>Firstly, have you checked that the model in the bucket is reachable?
Secondly, what is the name of the config file?<|||||>Yes its reachable, I can read vocab.txt file .
Name of config file is bert_config.json<|||||>I do have the same (similar) problem, except that I use the pretrained models (e.g. bert-base-uncased"). The script repeatedly downloads the vocab, json and model file and often fails to load the model. Everything is works if I do it on the local machine.
I also tried to download the files locally and unsuccessfully loaded the model directly. (tokenizer failed, model failed, BertConfig worked). Maybe I do something wrong here - example code would help<|||||>This issue has been automatically marked as stale because it has not had recent activity. It will be closed if no further activity occurs. Thank you for your contributions.
|
transformers | 984 | closed | docs: correct number of layers for various xlm models | Hi,
during some NER experiments I found out, that the number of reported layers in the documentation is different compared to the model configuration for some XLM models.
This PR fixes the documentation :) | 08-07-2019 14:24:43 | 08-07-2019 14:24:43 | # [Codecov](https://codecov.io/gh/huggingface/pytorch-transformers/pull/984?src=pr&el=h1) Report
> Merging [#984](https://codecov.io/gh/huggingface/pytorch-transformers/pull/984?src=pr&el=desc) into [master](https://codecov.io/gh/huggingface/pytorch-transformers/commit/7729ef738161a0a182b172fcb7c351f6d2b9c50d?src=pr&el=desc) will **increase** coverage by `0.06%`.
> The diff coverage is `n/a`.
[](https://codecov.io/gh/huggingface/pytorch-transformers/pull/984?src=pr&el=tree)
```diff
@@ Coverage Diff @@
## master #984 +/- ##
==========================================
+ Coverage 79.16% 79.22% +0.06%
==========================================
Files 38 38
Lines 6406 6406
==========================================
+ Hits 5071 5075 +4
+ Misses 1335 1331 -4
```
| [Impacted Files](https://codecov.io/gh/huggingface/pytorch-transformers/pull/984?src=pr&el=tree) | Coverage Δ | |
|---|---|---|
| [pytorch\_transformers/file\_utils.py](https://codecov.io/gh/huggingface/pytorch-transformers/pull/984/diff?src=pr&el=tree#diff-cHl0b3JjaF90cmFuc2Zvcm1lcnMvZmlsZV91dGlscy5weQ==) | `74.1% <0%> (+2.87%)` | :arrow_up: |
------
[Continue to review full report at Codecov](https://codecov.io/gh/huggingface/pytorch-transformers/pull/984?src=pr&el=continue).
> **Legend** - [Click here to learn more](https://docs.codecov.io/docs/codecov-delta)
> `Δ = absolute <relative> (impact)`, `ø = not affected`, `? = missing data`
> Powered by [Codecov](https://codecov.io/gh/huggingface/pytorch-transformers/pull/984?src=pr&el=footer). Last update [7729ef7...39f51cd](https://codecov.io/gh/huggingface/pytorch-transformers/pull/984?src=pr&el=lastupdated). Read the [comment docs](https://docs.codecov.io/docs/pull-request-comments).
<|||||>Thanks Stefan, there were a few other typos in these models details indeed so I'll take care of this in another PR. |
transformers | 983 | closed | Worse performance of gpt2 than gpt | ## ❓ Questions & Help
<!-- A clear and concise description of the question. -->
Hi, I just want to compare the performance of gpt and gpt2 as Language Model to assign Language modeling score. Like #473 , I implement my model as follows:
```
def gpt_score(text, model, tokenizer):
input_ids = torch.tensor(tokenizer.encode(text)).unsqueeze(0) # Batch size 1
input_ids = input_ids.to('cuda')
with torch.no_grad():
outputs = model(input_ids, labels=input_ids)
loss, logits = outputs[:2]
sentence_prob = loss.item()
return sentence_prob
a=['there is a book on the desk',
'there is a rocket on the desk',
'he put an elephant into the fridge', 'he put an apple into the fridge']
tokenizer = OpenAIGPTTokenizer.from_pretrained('openai-gpt')
model = OpenAIGPTLMHeadModel.from_pretrained('openai-gpt')
model.to('cuda')
model.eval()
print([gpt_score(i,model,tokenizer) for i in a])
#config = GPT2Config.from_pretrained('gpt2')
tokenizer = GPT2Tokenizer.from_pretrained('gpt2')
model = GPT2LMHeadModel.from_pretrained('gpt2')
model.to('cuda')
model.eval()
print([gpt_score(i,model,tokenizer) for i in a])
```
And I get the following result:
```
[3.0594890117645264, 4.373698711395264, 5.336375713348389, 4.865700721740723]
[4.475168704986572, 4.266316890716553, 5.423445224761963, 4.562324523925781]
```
It seems that GPT get more sensible result than GPT2, but since gpt2 is literally gpt training with more data, how's that possible?
| 08-07-2019 12:29:32 | 08-07-2019 12:29:32 | @Nealcly Could you try to use the `gpt2-medium` model? It has more layers :)<|||||>Also I'm not sure that your testing procedure is statistically representative :)<|||||>Any new updates on this issue? I am also facing the same question. <|||||>Based on the paper, only the largest model is called gpt2. The smallest model(117m) doesn't guarantee better performance than gpt.<|||||>how to finetune the language model with dataset and then get perplexity scores
<|||||>@anonymous297 please check the [documentation examples](https://huggingface.co/transformers/examples.html#language-model-fine-tuning), in which there's exactly what you're looking for.<|||||>This issue has been automatically marked as stale because it has not had recent activity. It will be closed if no further activity occurs. Thank you for your contributions.
|
transformers | 982 | closed | How to predict masked whole word which was tokenized as sub-words for bert-base-multilingual-cased | ## ❓ Questions & Help
Hello,
I have started working with pytorch-transformers and want to use it to predict masked words in polish. I use ' bert-base-multilingual-cased' pre-trained model and want to predict masked words which very often are tokenized into sub-word.
My question is how can I predict the whole word?
When I predict each token separately the results are poor. especially when I try to concatenate those predicted tokens
Here is sample code showing the problem
```python
import torch
from pytorch_transformers import BertTokenizer, BertModel, BertForMaskedLM
import logging
logging.basicConfig(level=logging.INFO)
USE_GPU = 1
# Device configuration
device = torch.device('cuda' if (torch.cuda.is_available() and USE_GPU) else 'cpu')
# Load pre-trained model tokenizer (vocabulary)
pretrained_model = 'bert-base-multilingual-cased'
tokenizer = BertTokenizer.from_pretrained(pretrained_model)
text = "[CLS] Who was Jim Henson ? [SEP] Jim Henson was a puppeteer [SEP]"
tokenized_text = tokenizer.tokenize(text)
# Mask a token that we will try to predict back with `BertForMaskedLM`
mask1 = 13
mask2 = 14
mask3 = 15
tokenized_text[mask1] = '[MASK]'
tokenized_text[mask2] = '[MASK]'
tokenized_text[mask3] = '[MASK]'
assert tokenized_text == ['[CLS]', 'Who', 'was', 'Jim', 'Hen', '##son', '?', '[SEP]', 'Jim', 'Hen', '##son', 'was', 'a', '[MASK]', '[MASK]', '[MASK]', '[SEP]']
# Convert token to vocabulary indices
indexed_tokens = tokenizer.convert_tokens_to_ids(tokenized_text)
# Define sentence A and B indices associated to 1st and 2nd sentences (see paper)
segments_ids = [0, 0, 0, 0, 0, 0, 0,0, 1, 1, 1, 1, 1, 1, 1,1,1]
# Convert inputs to PyTorch tensors
tokens_tensor = torch.tensor([indexed_tokens])
segments_tensors = torch.tensor([segments_ids])
# Load pre-trained model (weights)
model = BertForMaskedLM.from_pretrained(pretrained_model)
model.eval()
# If you have a GPU, put everything on cuda
tokens_tensor = tokens_tensor.to(device)
segments_tensors = segments_tensors.to(device)
model.to(device)
# Predict all tokens
with torch.no_grad():
outputs = model(tokens_tensor, token_type_ids=segments_tensors)
predictions = outputs[0]
# get predicted tokens
#prediction for mask1
predicted_index = torch.argmax(predictions[0, mask1]).item()
predicted_token = tokenizer.convert_ids_to_tokens([predicted_index])[0]
print(predicted_token) #returns "baseball"
#prediction for mask2
predicted_index = torch.argmax(predictions[0, mask2]).item()
predicted_token = tokenizer.convert_ids_to_tokens([predicted_index])[0]
print(predicted_token) #returns "actor"
#prediction for mask3
predicted_index = torch.argmax(predictions[0, mask3]).item()
predicted_token = tokenizer.convert_ids_to_tokens([predicted_index])[0]
print(predicted_token) # returns "."
```
| 08-07-2019 11:06:23 | 08-07-2019 11:06:23 | Hi I don't have any good solution for your use-case, unfortunately.
There are two "Whole-Word_masking" models for Bert (see the [list here](https://huggingface.co/pytorch-transformers/pretrained_models.html)) that would be better at guessing full words but they are only in English unfortunately.
SpanBert (whose open-sourcing we are still waiting) may also be better but I think they also only trained an English model...<|||||>@thomwolf thanks for the reply.
can you specify what exactly the problem lies in? I know the model is not capable of properly tokenizing polish.
Assuming I have pre-trained model for Polish, or just working with English text how can I predict a sequence of masked two or three tokens side by side<|||||>This issue has been automatically marked as stale because it has not had recent activity. It will be closed if no further activity occurs. Thank you for your contributions.
<|||||>if i want to fine-tune with my dataset,what should i do?<|||||>> if i want to fine-tune with my dataset,what should i do?
Hi tom1125, to fine-tune you can run this script:
export TRAIN_FILE=/path/to/dataset/wiki.train.raw
export TEST_FILE=/path/to/dataset/wiki.test.raw
python run_language_modeling.py \
--output_dir=output \
--model_type=roberta \
--model_name_or_path=roberta-base \
--do_train \
--train_data_file=$TRAIN_FILE \
--do_eval \
--eval_data_file=$TEST_FILE \
--mlm
Explained here: https://huggingface.co/transformers/examples.html#roberta-bert-and-masked-language-modeling |
transformers | 981 | closed | The pre-trained model you are loading is a cased model but you have not set `do_lower_case` to False. | I initialized the tokenizer and the model like
```python
def load_bert_score_model(bert="bert-base-multilingual-cased", num_layers=8):
assert bert in bert_types
tokenizer = BertTokenizer.from_pretrained(bert, do_lower_case=True)
model = BertModel.from_pretrained(bert)
model.eval()
device = 'cuda' if torch.cuda.is_available() else 'cpu'
model.to(device)
# drop unused layers
model.encoder.layer = torch.nn.ModuleList([layer for layer in model.encoder.layer[:num_layers]])
return model, tokenizer
```
so setting the `do_lower_case=True`, but I'm getting this warning:
```
The pre-trained model you are loading is a cased model but you have not set `do_lower_case` to False. We are setting `do_lower_case=False` for you but you may want to check this behavior.
``` | 08-07-2019 09:49:29 | 08-07-2019 09:49:29 | Hi! You seem to be loading a cased model (such as the `bert-base-multilingual-cased`), but you're specifying `do_lower_case` to your tokenizer, which strips accents and lowercases every character.
The model you specified has been trained with uppercase and lowercase characters as well as accent markers, so you should use it with such characters as well. If you're looking at using only lowercase characters, it would be better for you to use an uncased model (such as the `bert-base-multilingual-uncased`).<|||||>@LysandreJik that is correct, thank you. |
transformers | 980 | closed | n/a | 08-07-2019 01:32:05 | 08-07-2019 01:32:05 | ||
transformers | 979 | closed | n/a | 08-06-2019 22:41:56 | 08-06-2019 22:41:56 | Hi @ibeltagy,
Does it train on TPU?<|||||>Not yet, it still has some issues. I will create another PR when it is in good shape. |
|
transformers | 978 | closed | RuntimeError: bool value of Tensor with more than one value is ambiguous | ## ❓ Questions & Help
<!-- Using tokenizer to decode tensor is throwing this error: RuntimeError: bool value of Tensor with more than one value is ambiguous -->
Here's the code I'm trying to run, the tensor itself gets returned, but when I try to decode it I get the error above.
Any ideas? Thanks!
`if __name__ == '__main__':
# main()
model_class, tokenizer_class = MODEL_CLASSES['gpt2']
tokenizer = tokenizer_class.from_pretrained('gpt2')
context_tokens = tokenizer.encode("My favorite first date idea is")
model = model_class.from_pretrained('gpt2')
model.to('cpu')
model.eval()
out = sample_sequence(
model=model,
context=context_tokens,
length=140,
temperature=0.9,
top_k=1,
top_p=0.9
)
text = tokenizer.decode(out)
print(text)`
| 08-06-2019 19:34:53 | 08-06-2019 19:34:53 | Could you please provide more information, especially regarding the `sample_sequence` function and where it is coming from?<|||||>Thanks for the response!
My goal is to wrap the GPT2 model interface in a function so I can input a prompt and output generated text. I'm trying to adapt one of the examples, and I'm getting there, but I wasn't able to find anything on the error specific to Pytorch-Transformers.
Here's the `sample_sequence` function:
def sample_sequence(model, length, context, num_samples=1, temperature=1, top_k=0, top_p=0.0, device='cpu'):
context = torch.tensor(context, dtype=torch.long, device=device)
context = context.unsqueeze(0).repeat(num_samples, 1)
generated = context
with torch.no_grad():
for _ in trange(length):
inputs = {'input_ids': generated}
outputs = model(**inputs)
next_token_logits = outputs[0][0, -1, :] / temperature
filtered_logits = top_k_top_p_filtering(next_token_logits, top_k=top_k, top_p=top_p)
next_token = torch.multinomial(F.softmax(filtered_logits, dim=-1), num_samples=1)
generated = torch.cat((generated, next_token.unsqueeze(0)), dim=1)
return generated<|||||>Your sample sequence function returns a `torch.tensor([[int64]])`, of shape `[batch_size, sequence_length]`. In your specific case it is of size `[1, 146]`.
You cannot feed such an object to the tokenizer for decoding, as it only accepts a list of integers.
You can fetch the list of integers by calling the `tolist()` method on your output, and then feed it to the tokenizer for decoding:
```
out = sample_sequence(
model=model,
context=context_tokens,
length=140,
temperature=0.9,
top_k=1,
top_p=0.9
)
generated_list = out[0].tolist()
text = tokenizer.decode(generated_list)
print(text)
```<|||||>It worked!
This was very helpful, thank you! Just getting familiar with this package, it's awesome! |
transformers | 977 | closed | Fixed typo in migration guide | This PR fixes a minor typo in the migration guide. `weights` was misspelled as `weigths` | 08-06-2019 18:20:57 | 08-06-2019 18:20:57 | # [Codecov](https://codecov.io/gh/huggingface/pytorch-transformers/pull/977?src=pr&el=h1) Report
> Merging [#977](https://codecov.io/gh/huggingface/pytorch-transformers/pull/977?src=pr&el=desc) into [master](https://codecov.io/gh/huggingface/pytorch-transformers/commit/4fc9f9ef54e2ab250042c55b55a2e3c097858cb7?src=pr&el=desc) will **not change** coverage.
> The diff coverage is `n/a`.
[](https://codecov.io/gh/huggingface/pytorch-transformers/pull/977?src=pr&el=tree)
```diff
@@ Coverage Diff @@
## master #977 +/- ##
=======================================
Coverage 79.16% 79.16%
=======================================
Files 38 38
Lines 6406 6406
=======================================
Hits 5071 5071
Misses 1335 1335
```
------
[Continue to review full report at Codecov](https://codecov.io/gh/huggingface/pytorch-transformers/pull/977?src=pr&el=continue).
> **Legend** - [Click here to learn more](https://docs.codecov.io/docs/codecov-delta)
> `Δ = absolute <relative> (impact)`, `ø = not affected`, `? = missing data`
> Powered by [Codecov](https://codecov.io/gh/huggingface/pytorch-transformers/pull/977?src=pr&el=footer). Last update [4fc9f9e...a6f412d](https://codecov.io/gh/huggingface/pytorch-transformers/pull/977?src=pr&el=lastupdated). Read the [comment docs](https://docs.codecov.io/docs/pull-request-comments).
<|||||>Ok, thanks! |
transformers | 976 | closed | Issue: Possibly wrong documentation about labels in BERT classifier | Possibly also elsewhere, but when discussing the proper format of labels for BERT classification, the documentation states the following:
https://github.com/huggingface/pytorch-transformers/blob/44dd941efb602433b7edc29612cbdd0a03bf14dc/pytorch_transformers/modeling_bert.py#L935
However, shouldn't it be `[0, ..., config.num_labels - 1]`? After all, [`CrossEntropyLoss`](https://pytorch.org/docs/stable/nn.html#crossentropyloss) is being used here. | 08-06-2019 14:09:39 | 08-06-2019 14:09:39 | Indeed. @LysandreJik I think it should be `Indices should be in ``[0, ..., config.num_labels-1]`` for classification or torch.floats for regression`, what do you think? |
transformers | 975 | closed | Inconsistant output between pytorch-transformers and pytorch-pretrained-bert | ## 📚 Migration
<!-- Important information -->
Model I am using (GPT, GPT2, XLNet):
Language I am using the model on (English):
The problem arise when using:
* [ ] the official example scripts: (give details)
* [x] my own modified scripts: (give details)
```
def xlnet_score(text, model, tokenizer):
# Tokenized input
tokenized_text = tokenizer.tokenize(text)
# text = "[CLS] Stir the mixture until it is done [SEP]"
sentence_prob = 0
#Sprint(len(tokenized_text))
for masked_index in range(0,len(tokenized_text)):
# Mask a token that we will try to predict back with `BertForMaskedLM`
masked_word = tokenized_text[masked_index]
if masked_word!= "<sep>":
masked_word = tokenized_text[masked_index]
tokenized_text[masked_index] = '<mask>'
# assert tokenized_text == ['[CLS]', 'who', 'was', 'jim', 'henson', '?', '[SEP]', 'jim', '[MASK]', 'was', 'a', 'puppet', '##eer', '[SEP]']
# print (tokenized_text)
input_ids = torch.tensor(tokenizer.convert_tokens_to_ids(tokenized_text)).unsqueeze(0)
index = torch.tensor(tokenizer.convert_tokens_to_ids(masked_word))
perm_mask = torch.zeros((1, input_ids.shape[1], input_ids.shape[1]), dtype=torch.float)
perm_mask[:, :, masked_index] = 1.0 # Previous tokens don't see last token
target_mapping = torch.zeros((1, 1, input_ids.shape[1]), dtype=torch.float) # Shape [1, 1, seq_length] => let's predict one token
target_mapping[0, 0, masked_index] = 1.0 # Our first (and only) prediction will be the last token of the sequence (the masked token)
input_ids = input_ids.to('cuda')
perm_mask = perm_mask.to('cuda')
target_mapping = target_mapping.to('cuda')
index = index.to('cuda')
with torch.no_grad():
outputs = model(input_ids, perm_mask=perm_mask, target_mapping=target_mapping, labels = index)
next_token_logits = outputs[0]
length = len(tokenized_text)
# predict_list = predictions[0, masked_index]
sentence_prob -= next_token_logits.item()
tokenized_text[masked_index] = masked_word
#tokenized_text = tokenized_text.split()
#return math.pow(sentence_prob, 1/(len(tokenized_text)-3))
return sentence_prob/(length-1)
def gpt_score(text, model, tokenizer):
# Tokenized input
# text = "[CLS] I got restricted because Tom reported my reply [SEP]"
input_ids = torch.tensor(tokenizer.encode(text)).unsqueeze(0) # Batch size 1
input_ids = input_ids.to('cuda')
with torch.no_grad():
outputs = model(input_ids, labels=input_ids)
loss, logits = outputs[:2]
# text = "[CLS] Stir the mixture until it is done [SEP]"
sentence_prob = -loss.item()
#return math.pow(sentence_prob, 1/(len(tokenized_text)-3))
return sentence_prob
def gpt2_score(text, model, tokenizer):
# Tokenized input
# text = "[CLS] I got restricted because Tom reported my reply [SEP]"
input_ids = torch.tensor(tokenizer.encode(text)).unsqueeze(0) # Batch size 1
input_ids = input_ids.to('cuda')
with torch.no_grad():
outputs = model(input_ids, labels=input_ids)
loss, logits = outputs[:2]
# text = "[CLS] Stir the mixture until it is done [SEP]"
sentence_prob = -loss.item()
#return math.pow(sentence_prob, 1/(len(tokenized_text)-3))
return sentence_prob
def score(sentence):
tokenize_input = tokenizer.tokenize(sentence)
tensor_input = torch.tensor([tokenizer.convert_tokens_to_ids(tokenize_input)])
tensor_input = tensor_input.to('cuda')
loss=model(tensor_input, labels=tensor_input)[0]
return math.exp(loss)
config = XLNetConfig.from_pretrained('xlnet-base-cased')
tokenizer = XLNetTokenizer.from_pretrained('xlnet-base-cased')
model = XLNetLMHeadModel(config)
model.to('cuda')
model.eval()
a=['there is a book on the desk',
'there is a plane on the desk',
'there is a book under the desk']
print([xlnet_score(i,model,tokenizer) for i in a])
config = OpenAIGPTConfig.from_pretrained('openai-gpt')
tokenizer = OpenAIGPTTokenizer.from_pretrained('openai-gpt')
model = OpenAIGPTLMHeadModel(config)
model.to('cuda')
model.eval()
print([gpt_score(i,model,tokenizer) for i in a])
print([score(i) for i in a])
config = GPT2Config.from_pretrained('gpt2')
tokenizer = GPT2Tokenizer.from_pretrained('gpt2')
model = GPT2LMHeadModel(config)
model.to('cuda')
model.eval()
print([gpt_score(i,model,tokenizer) for i in a])`
```
The tasks I am working on is:
* [ ] an official GLUE/SQUaD task: (give the name)
* [x] my own task or dataset: (give details)
Details of the issue:
<!-- A clear and concise description of the migration issue. If you have code snippets, please provide it here as well. -->
So the issue here is that I try to calculate the perplexity (or loss) of the sentence to determine which sentence makes more sense. However, as #473 shows that we could just retrieve the loss. The scores I get with PyTorch-transformer is different from the scores in that post. For the `def score` function, I literally copy the code in post #473 for comparison.
```
a=['there is a book on the desk',
'there is a plane on the desk',
'there is a book under the desk']
print([model_score(i,model,tokenizer) for i in a])
negative of loss get from XLnet
[-11.915737946828207, -11.859564940134684, -11.996480623881022]
negative of loss get from GPT
[-10.969852447509766, -11.002564430236816, -10.877273559570312]
perplexity get from GPT
[58096.0205576014, 60027.88181824669, 52959.01330928259]
negative of loss get from GPT-2
[-11.469226837158203, -11.445046424865723, -11.510353088378906]
```
Furthermore, as you can see, none of these results above make much sense.
## Environment
* OS: Linux
* Python version: 3.6
* PyTorch version: 1.1.0
* PyTorch Transformers version (or branch):
* Using GPU ? Yes
* Distributed of parallel setup ? No
* Any other relevant information:
## Checklist
- [x] I have read the migration guide in the readme.
- [x] I checked if a related official extension example runs on my machine.
## Additional context
<!-- Add any other context about the problem here. --> | 08-06-2019 10:11:20 | 08-06-2019 10:11:20 | See #954. I got bitten by the same documentation _bug_.<|||||>This issue has been automatically marked as stale because it has not had recent activity. It will be closed if no further activity occurs. Thank you for your contributions.
|
transformers | 974 | closed | Support longer sequences with BertForSequenceClassification | ## ❓ Questions & Help
<!-- A clear and concise description of the question. -->
I am using `BertForSequenceClassification` for solving a regression task. I have a long sequence as an input and the model outputs a float in range [0,1].
Most of my sequences are longer than 512, which is the max sequence length in the current bert pretrained models.
To handle longer sequence you need to split the input with some stride value, As suggested here:
https://github.com/google-research/bert/issues/27#issuecomment-435265194
It seems that it was implemented in the `BertForQuestionAnswering` and the `Squad` example but not in the `BertForSequenceClassification` which i use.
But still, I do not understand how that would really work. I do not understand the use of `start_positions` and `end_positions` enough to implement it on my own.
Given my regression task how do i handle the output of the model for each chunk of my input and get unified output of the model?
| 08-06-2019 09:57:56 | 08-06-2019 09:57:56 | A little question because we are trying to organize the issues better:
- what made you not use the issue templates we have added?<|||||>> A little question because we are trying to organize the issues better:
>
> * what made you not use the issue templates we have added?
Didn't know about it...<|||||>@eladbitton Can you link lines of code to where this was done for `BertForQuestionAnswering` and `SQuAD`? I'd be willing to take a stab at implementation.<|||||>Hey @maxzzze. I was looking at:
https://github.com/huggingface/pytorch-transformers/blob/0d1dad6d5323cf627cb8d7ddd428856ab8475f6b/pytorch_transformers/modeling_bert.py#L1112
Now that i look at it, i am not sure if they implemented it there.
<|||||>This issue has been automatically marked as stale because it has not had recent activity. It will be closed if no further activity occurs. Thank you for your contributions.
<|||||>Same issue here.
Since this package is presented as a "plug n play" solution for this kind of task, that is really unfortunate.<|||||>@eladbitton, I believe the start and end positions in BertForQuestionAnswering are for filtering tokens when computing the loss (since the loss is given by the cross-entropy between the predicted and true distributions of the start token, the latter of which is a one-hot vector; similarly for the end token), not for converting a large sequence into a batch of shorter sequences.
@thomwolf, are there plans to add the functionality mentioned [here](https://github.com/google-research/bert/issues/27#issuecomment-435265194) by Devlin (or would you be able to suggest any alternatives that might work)? |
transformers | 973 | closed | Fix examples of loading pretrained models in docstring | 08-06-2019 03:33:52 | 08-06-2019 03:33:52 | # [Codecov](https://codecov.io/gh/huggingface/pytorch-transformers/pull/973?src=pr&el=h1) Report
> Merging [#973](https://codecov.io/gh/huggingface/pytorch-transformers/pull/973?src=pr&el=desc) into [master](https://codecov.io/gh/huggingface/pytorch-transformers/commit/4fc9f9ef54e2ab250042c55b55a2e3c097858cb7?src=pr&el=desc) will **not change** coverage.
> The diff coverage is `n/a`.
[](https://codecov.io/gh/huggingface/pytorch-transformers/pull/973?src=pr&el=tree)
```diff
@@ Coverage Diff @@
## master #973 +/- ##
=======================================
Coverage 79.16% 79.16%
=======================================
Files 38 38
Lines 6406 6406
=======================================
Hits 5071 5071
Misses 1335 1335
```
| [Impacted Files](https://codecov.io/gh/huggingface/pytorch-transformers/pull/973?src=pr&el=tree) | Coverage Δ | |
|---|---|---|
| [pytorch\_transformers/modeling\_transfo\_xl.py](https://codecov.io/gh/huggingface/pytorch-transformers/pull/973/diff?src=pr&el=tree#diff-cHl0b3JjaF90cmFuc2Zvcm1lcnMvbW9kZWxpbmdfdHJhbnNmb194bC5weQ==) | `57.53% <ø> (ø)` | :arrow_up: |
| [pytorch\_transformers/modeling\_xlnet.py](https://codecov.io/gh/huggingface/pytorch-transformers/pull/973/diff?src=pr&el=tree#diff-cHl0b3JjaF90cmFuc2Zvcm1lcnMvbW9kZWxpbmdfeGxuZXQucHk=) | `79.01% <ø> (ø)` | :arrow_up: |
| [pytorch\_transformers/modeling\_xlm.py](https://codecov.io/gh/huggingface/pytorch-transformers/pull/973/diff?src=pr&el=tree#diff-cHl0b3JjaF90cmFuc2Zvcm1lcnMvbW9kZWxpbmdfeGxtLnB5) | `86.66% <ø> (ø)` | :arrow_up: |
| [pytorch\_transformers/modeling\_bert.py](https://codecov.io/gh/huggingface/pytorch-transformers/pull/973/diff?src=pr&el=tree#diff-cHl0b3JjaF90cmFuc2Zvcm1lcnMvbW9kZWxpbmdfYmVydC5weQ==) | `87.98% <ø> (ø)` | :arrow_up: |
| [pytorch\_transformers/modeling\_openai.py](https://codecov.io/gh/huggingface/pytorch-transformers/pull/973/diff?src=pr&el=tree#diff-cHl0b3JjaF90cmFuc2Zvcm1lcnMvbW9kZWxpbmdfb3BlbmFpLnB5) | `74.76% <ø> (ø)` | :arrow_up: |
| [pytorch\_transformers/modeling\_gpt2.py](https://codecov.io/gh/huggingface/pytorch-transformers/pull/973/diff?src=pr&el=tree#diff-cHl0b3JjaF90cmFuc2Zvcm1lcnMvbW9kZWxpbmdfZ3B0Mi5weQ==) | `75.84% <ø> (ø)` | :arrow_up: |
------
[Continue to review full report at Codecov](https://codecov.io/gh/huggingface/pytorch-transformers/pull/973?src=pr&el=continue).
> **Legend** - [Click here to learn more](https://docs.codecov.io/docs/codecov-delta)
> `Δ = absolute <relative> (impact)`, `ø = not affected`, `? = missing data`
> Powered by [Codecov](https://codecov.io/gh/huggingface/pytorch-transformers/pull/973?src=pr&el=footer). Last update [4fc9f9e...6ec1ee9](https://codecov.io/gh/huggingface/pytorch-transformers/pull/973?src=pr&el=lastupdated). Read the [comment docs](https://docs.codecov.io/docs/pull-request-comments).
<|||||># [Codecov](https://codecov.io/gh/huggingface/pytorch-transformers/pull/973?src=pr&el=h1) Report
> Merging [#973](https://codecov.io/gh/huggingface/pytorch-transformers/pull/973?src=pr&el=desc) into [master](https://codecov.io/gh/huggingface/pytorch-transformers/commit/4fc9f9ef54e2ab250042c55b55a2e3c097858cb7?src=pr&el=desc) will **not change** coverage.
> The diff coverage is `n/a`.
[](https://codecov.io/gh/huggingface/pytorch-transformers/pull/973?src=pr&el=tree)
```diff
@@ Coverage Diff @@
## master #973 +/- ##
=======================================
Coverage 79.16% 79.16%
=======================================
Files 38 38
Lines 6406 6406
=======================================
Hits 5071 5071
Misses 1335 1335
```
| [Impacted Files](https://codecov.io/gh/huggingface/pytorch-transformers/pull/973?src=pr&el=tree) | Coverage Δ | |
|---|---|---|
| [pytorch\_transformers/modeling\_transfo\_xl.py](https://codecov.io/gh/huggingface/pytorch-transformers/pull/973/diff?src=pr&el=tree#diff-cHl0b3JjaF90cmFuc2Zvcm1lcnMvbW9kZWxpbmdfdHJhbnNmb194bC5weQ==) | `57.53% <ø> (ø)` | :arrow_up: |
| [pytorch\_transformers/modeling\_xlnet.py](https://codecov.io/gh/huggingface/pytorch-transformers/pull/973/diff?src=pr&el=tree#diff-cHl0b3JjaF90cmFuc2Zvcm1lcnMvbW9kZWxpbmdfeGxuZXQucHk=) | `79.01% <ø> (ø)` | :arrow_up: |
| [pytorch\_transformers/modeling\_xlm.py](https://codecov.io/gh/huggingface/pytorch-transformers/pull/973/diff?src=pr&el=tree#diff-cHl0b3JjaF90cmFuc2Zvcm1lcnMvbW9kZWxpbmdfeGxtLnB5) | `86.66% <ø> (ø)` | :arrow_up: |
| [pytorch\_transformers/modeling\_bert.py](https://codecov.io/gh/huggingface/pytorch-transformers/pull/973/diff?src=pr&el=tree#diff-cHl0b3JjaF90cmFuc2Zvcm1lcnMvbW9kZWxpbmdfYmVydC5weQ==) | `87.98% <ø> (ø)` | :arrow_up: |
| [pytorch\_transformers/modeling\_openai.py](https://codecov.io/gh/huggingface/pytorch-transformers/pull/973/diff?src=pr&el=tree#diff-cHl0b3JjaF90cmFuc2Zvcm1lcnMvbW9kZWxpbmdfb3BlbmFpLnB5) | `74.76% <ø> (ø)` | :arrow_up: |
| [pytorch\_transformers/modeling\_gpt2.py](https://codecov.io/gh/huggingface/pytorch-transformers/pull/973/diff?src=pr&el=tree#diff-cHl0b3JjaF90cmFuc2Zvcm1lcnMvbW9kZWxpbmdfZ3B0Mi5weQ==) | `75.84% <ø> (ø)` | :arrow_up: |
------
[Continue to review full report at Codecov](https://codecov.io/gh/huggingface/pytorch-transformers/pull/973?src=pr&el=continue).
> **Legend** - [Click here to learn more](https://docs.codecov.io/docs/codecov-delta)
> `Δ = absolute <relative> (impact)`, `ø = not affected`, `? = missing data`
> Powered by [Codecov](https://codecov.io/gh/huggingface/pytorch-transformers/pull/973?src=pr&el=footer). Last update [4fc9f9e...6ec1ee9](https://codecov.io/gh/huggingface/pytorch-transformers/pull/973?src=pr&el=lastupdated). Read the [comment docs](https://docs.codecov.io/docs/pull-request-comments).
<|||||>Great! Thanks a lot @FeiWang96! |
|
transformers | 972 | closed | XLNetForQuestionAnswering - weight pruning | ## 🚀 Feature
Hi guys, first of all, thank you a lot for the great API, I'm using a lot `pytorch-transformers`, you guys are really doing a good job!
I have recently fine-tuned a `XLNetForQuestionAnswering` on SQuAD1.10, results looks good, however the model is taking ~ 2.0 seconds (in a MacBook Pro) to do a forward in a reasonable small "facts/passage" text.
I had some some weight pruning in the past (in a small network), and I was wondering if you guys heard of any paper/idea to do weight pruning in transformer based networks such as BERT or XLNet?
Any other ideas to optimize model forward look for inferencing? I'm thinking to put these model in prod but ~1-2 seconds is still too high.
I'm willing to help and work on this issue, but it will be great if you guys can point some directions on best way to do this?
## Motivation
Currently the forward times of trained `BertForQuestionAnswering` and `XLNetForQuestionAnswering` are too high, I'm searching for options to reduce forward time on QA task for both networks (results below running on a MacBook Pro 2.9GHz Corei7, 16GB RAM):
`BertForQuestionAnswering`: 1.48 s ± 52.4 ms per loop (mean ± std. dev. of 3 runs, 1 loop each)
`XLNetForQuestionAnswering`: 2.14 s ± 45.5 ms per loop (mean ± std. dev. of 3 runs, 1 loop each)
## Additional context
<!-- Add any other context or screenshots about the feature request here. --> | 08-05-2019 19:16:51 | 08-05-2019 19:16:51 | I'm interested in this as well. I've seen similar inference times of nearly 1.5 seconds running BERT for inference on a fine-tuned classification task on TF Serving and would like to improve it without paying for a GPU.
I'm not associated with the following work, but found the paper interesting:
"tranformers.zip: Compressing Transformers with Pruning and Quantization"
http://web.stanford.edu/class/cs224n/reports/custom/15763707.pdf
The open source corresponding to the paper above has been published in a branch of OpenNMT here:
https://github.com/robeld/ERNIE
<|||||>I think we could speed up significantly XLNet by refactoring the tensorflow code to use Embeddings instead of multiplication of static matrices with one-hot vectors as it's currently done in several places. We could also reduce the use of `torch.einsum` and replace them with matrix multiplications. We'll experiment with that in the coming months.<|||||>Might even just dropping in `opt_einsum` as a substitute for the `torch.einsum` be an easy speedup?<|||||>I'm doing some time profiling here, it looks like the time bottleneck in the forward loop of the transformer. In this case my overall forward loop for `XLNetForQuestionAnswering` is taking `2.5 s ± 310 ms per loop (mean ± std. dev. of 3 runs, 1 loop each)`. Please see below a breakdown for each forward step (in seconds). Looks like the large chunk of the time is spent in the chunk of the code below ~2.33 seconds. Will start doing some optimizations on `XLNetRelativeAttention` and `XLNetFeedForward` to see what happens.
```
Causal attention mask: 7e-05
Data mask: 3e-05
Word Embedding: 0.00073
Segment Embedding: 5e-05
___ Pos encoding - 1 : 0.0099
___ Pos encoding - 2 : 0.00012
**___ Pos encoding - 3: 2.33072**
Positional encoding: 2.34084
Prepare output: 0.00025
Transformer time: 2.3420751094818115
```
**___ Pos encoding - 3** - Code chunk
```
new_mems = ()
if mems is None:
mems = [None] * len(self.layer)
attentions = []
hidden_states = []
for i, layer_module in enumerate(self.layer):
# cache new mems
new_mems = new_mems + (self.cache_mem(output_h, mems[i]),)
if self.output_hidden_states:
hidden_states.append((output_h, output_g) if output_g is not None else output_h)
outputs = layer_module(output_h, output_g, attn_mask_h=non_tgt_mask, attn_mask_g=attn_mask,
r=pos_emb, seg_mat=seg_mat, mems=mems[i], target_mapping=target_mapping,
head_mask=head_mask[i])
output_h, output_g = outputs[:2]
if self.output_attentions:
attentions.append(outputs[2])
```<|||||>@MiroFurtado it looks like Torch.Einsum is already as optimized as `opt_einsum` - see attached an example of multiplication of **1024x1024** matrix using `torch.einsum`, `torch.matmul`,`np.einsum` and `opt_einsum`. Looks like in fact `np.einsum` is not optimized after all.
I modified the code to include `opt_einsum` using `contract` and actually it tooked ~3x more! **`5.79 s ± 0 ns per loop (mean ± std. dev. of 1 run, 1 loop each)`**
[Einsum Comparison - Torch Einsum, Matmul, Numpy, Opt Contract](https://drive.google.com/open?id=1Kck35N39sGuU1pKs2NPxAuXcjlNEP8yt)
<|||||>Just FYI, a relevant blog post about this topic, will investigate: https://blog.rasa.com/compressing-bert-for-faster-prediction-2/<|||||>More related information, **freshly** released: https://ai.facebook.com/blog/making-transformer-networks-simpler-and-more-efficient/?refid=52&__tn__=*s-R<|||||>This issue has been automatically marked as stale because it has not had recent activity. It will be closed if no further activity occurs. Thank you for your contributions.
|
transformers | 971 | closed | Brackets are not aligned in the DocString of Bert. | The Brackets in the file https://github.com/huggingface/pytorch-transformers/blob/master/pytorch_transformers/modeling_bert.py#L606 are not aligned, which will cause some highlight mistakes in some editers (i.e. VSCODE).
it should be fixed as : [0, config.max_position_embeddings - 1] | 08-05-2019 17:54:46 | 08-05-2019 17:54:46 | You're right, will fix! cc @LysandreJik |
transformers | 970 | closed | How to use GPT2LMHeadModel for conditional generation | Hi
could you please provide one single example on how to use GPT2LMHeadModel for conditional generation?
thanks
Rabeeh | 08-05-2019 16:27:06 | 08-05-2019 16:27:06 | Hi Rabeeh,
Please take a look at the [run_generation.py](https://github.com/huggingface/pytorch-transformers/blob/master/examples/run_generation.py) example which shows how to do conditional generation with the library's auto-regressive models (GPT/GPT-2/Transformer-XL/XLNet).<|||||>What's cracking Rabeeh,
look, this code makes the trick for GPT2LMHeadModel.
But, as torch.argmax() is used to derive the next word; there is a lot of repetition.
`
from transformers import GPT2LMHeadModel, GPT2Tokenizer
import torch
import argparse
parser = argparse.ArgumentParser()
parser.add_argument('--input', type=str, help='Initial text for GPT2 model', required=True)
parser.add_argument('--length', type=int, help='Amount of new words added to input', required=True, default=20)
args = parser.parse_args()
tokenizer = GPT2Tokenizer.from_pretrained("gpt2")
model = GPT2LMHeadModel.from_pretrained('gpt2')
generated = tokenizer.encode(args.input)
context = torch.tensor([generated])
past = None
for i in range(args.length):
>>>#print("{}=>>{}".format(i,tokenizer.decode(generated)))
>>>output, past = model(context, past=past)
>>>token = torch.argmax(output[0, :])
>>>generated += [token.tolist()]
>>>context = token.unsqueeze(0)
sequence = tokenizer.decode(generated)
print("Final sequence =>>{}".format(sequence))
`
As LysandreJik pointed out, is better to clone the hugginface transformer repo in Git, and go to the examples ---they do it great.<|||||>Hi
Thank you very much, very helpful for me.
On Wed, Jan 29, 2020 at 3:06 PM SaveTheBees-n-Seeds <
[email protected]> wrote:
> What's cracking Rabeeh,
>
> look, this code makes the trick for GPT2LMHeadModel.
> But, as torch.argmax() is used to derive the next word; there is a lot of
> repetition.
>
> `
> from transformers import GPT2LMHeadModel, GPT2Tokenizer
> import torch
> import argparse
> parser = argparse.ArgumentParser()
> parser.add_argument('--input', type=str, help='Initial text for GPT2
> model', required=True)
> parser.add_argument('--length', type=int, help='Amount of new words added
> to input', required=True, default=20)
> args = parser.parse_args()
>
> tokenizer = GPT2Tokenizer.from_pretrained("gpt2")
> model = GPT2LMHeadModel.from_pretrained('gpt2')
>
> generated = tokenizer.encode(args.input)
> context = torch.tensor([generated])
> past = None
>
> for i in range(args.length):
> #print("{}=>>{}".format(i,tokenizer.decode(generated)))
> output, past = model(context, past=past)
> token = torch.argmax(output[0, :])
> generated += [token.tolist()]
> context = token.unsqueeze(0)
>
> sequence = tokenizer.decode(generated)
>
> print("Final sequence =>>{}".format(sequence))
> `
>
> As LysandreJik pointed out, is better to clone the hugginface transformer
> repo in Git, and go to the examples ---they do it great.
>
> —
> You are receiving this because you authored the thread.
> Reply to this email directly, view it on GitHub
> <https://github.com/huggingface/transformers/issues/970?email_source=notifications&email_token=ABP4ZCF2JQTV7W32PHYGAE3RAGEOLA5CNFSM4IJM4LMKYY3PNVWWK3TUL52HS4DFVREXG43VMVBW63LNMVXHJKTDN5WW2ZLOORPWSZGOEKHJRGA#issuecomment-579770520>,
> or unsubscribe
> <https://github.com/notifications/unsubscribe-auth/ABP4ZCE4ZATSJAEF35A7B73RAGEOLANCNFSM4IJM4LMA>
> .
>
|
transformers | 969 | closed | Finetune GPT2 | Hi
According to pytorch-transformers/docs/source/index.rst
There was a run_gpt2.py example which also shows how to finetune GPT2 on the training data.
I was wondernig if you could add this example back, and proving sample script to finetune GPT2.
thanks.
Best regards,
Rabeeh | 08-05-2019 16:03:31 | 08-05-2019 16:03:31 | Hi Rabeeh,
We are currently working on an updated example on fine-tuning generative models, especially GPT-2. The example should be up later this week, keep an eye out!<|||||>Any update on when this example will be available? Thanks!<|||||>Hope this issue won't be closed until the example is done.<|||||>The script is being worked on over at https://github.com/huggingface/pytorch-transformers/pull/987 ([see relevant file here](https://github.com/huggingface/pytorch-transformers/blob/master/examples/run_lm_finetuning.py)). It works for GPT/GPT-2 but it isn't ready for BERT/RoBERTa so we're not releasing it yet.
It shows how to fine-tune GPT-2 using causal language modeling on WikiText-2.<|||||>Any update on when this example will be available? Thanks!
The link of "see relevant file here" is 404<|||||>Oh yes, the script is out.
It was renamed `run_lm_fintuning.py` you can find it in the `examples` folder: https://github.com/huggingface/pytorch-transformers/blob/master/examples/run_lm_finetuning.py
You can use it to fintune GPT, GPT-2, BERT or RoBERTa on your dataset.
Here is an example on how to run it: https://huggingface.co/pytorch-transformers/examples.html#causal-lm-fine-tuning-on-gpt-gpt-2-masked-lm-fine-tuning-on-bert-roberta<|||||>Silly question but how do you know which gpt-2 model is being trained? Does it default to the largest one available. I couldn't find any indication of which size model is being used in the fine tuning script.<|||||>Hi Henry,
Default to the small one.
You can select the size with the `model_name_or_path` argument. Just put in
the argument the relevant shortcut name for the model as listed [here](
https://huggingface.co/transformers/pretrained_models.html).
On Wed, 6 Nov 2019 at 12:35, Henry-E <[email protected]> wrote:
> Silly question but how do you know which gpt-2 model is being trained?
> Does it default to the largest one available. I could find any indication
> of which size model is being used in the fine tuning script.
>
> —
> You are receiving this because you commented.
> Reply to this email directly, view it on GitHub
> <https://github.com/huggingface/transformers/issues/969?email_source=notifications&email_token=ABYDIHNYJ3YQTDE6P6HPCOTQSKTYRA5CNFSM4IJMWQW2YY3PNVWWK3TUL52HS4DFVREXG43VMVBW63LNMVXHJKTDN5WW2ZLOORPWSZGOEDGHRYA#issuecomment-550271200>,
> or unsubscribe
> <https://github.com/notifications/unsubscribe-auth/ABYDIHM7FQGTLI5UPLWJHSTQSKTYRANCNFSM4IJMWQWQ>
> .
>
<|||||>Ah got it, thanks!<|||||>` run_lm_fintuning.py` is no longer available in the examples folder when you clone the transformers repo. Is there a reason for this? It was available a couple of months ago. <|||||>It’s named run_language_modeling.py now<|||||>Great, thanks!<|||||>This may sound silly also, but will `run_lm_fintuning.py` be able to finetune microsoft/DialoGPT model on a custom dataset? Thank you<|||||>Yes, but it's named `run_language_modeling.py` now. |
transformers | 968 | closed | Error when running run_squad.py in colab | Hi I used the below code which was given as an example:
!python -m torch.distributed.launch --nproc_per_node=8 ./examples/run_squad.py \
--model_type bert \
--model_name_or_path bert-large-uncased-whole-word-masking \
--do_train \
--do_eval \
--do_lower_case \
--train_file SQUAD_DIR/train-v1.1.json \
--predict_file SQUAD_DIR/dev-v1.1.json \
--learning_rate 3e-5 \
--num_train_epochs 2 \
--max_seq_length 384 \
--doc_stride 128 \
--output_dir wwm_uncased_finetuned_squad/ \
--per_gpu_eval_batch_size=3 \
--per_gpu_train_batch_size=3 \
and I tested it colab notebook but it throwed some error as following:
Traceback (most recent call last):
File "./examples/run_squad.py", line 527, in <module>
main()
File "./examples/run_squad.py", line 439, in main
torch.distributed.init_process_group(backend='nccl')
File "/usr/local/lib/python3.6/dist-packages/torch/distributed/distributed_c10d.py", line 406, in init_process_group
store, rank, world_size = next(rendezvous(url))
File "/usr/local/lib/python3.6/dist-packages/torch/distributed/rendezvous.py", line 143, in _env_rendezvous_handler
store = TCPStore(master_addr, master_port, world_size, start_daemon)
RuntimeError: Address already in use
THCudaCheck FAIL file=/pytorch/torch/csrc/cuda/Module.cpp line=33 error=10 : invalid device ordinal
Traceback (most recent call last):
File "./examples/run_squad.py", line 527, in <module>
main()
File "./examples/run_squad.py", line 437, in main
torch.cuda.set_device(args.local_rank)
File "/usr/local/lib/python3.6/dist-packages/torch/cuda/__init__.py", line 265, in set_device
torch._C._cuda_setDevice(device)
RuntimeError: cuda runtime error (10) : invalid device ordinal at /pytorch/torch/csrc/cuda/Module.cpp:33
THCudaCheck FAIL file=/pytorch/torch/csrc/cuda/Module.cpp line=33 error=10 : invalid device ordinal
Traceback (most recent call last):
File "./examples/run_squad.py", line 527, in <module>
THCudaCheck FAIL file=/pytorch/torch/csrc/cuda/Module.cpp line=33 error=10 : invalid device ordinal
main()
File "./examples/run_squad.py", line 437, in main
Traceback (most recent call last):
File "./examples/run_squad.py", line 527, in <module>
torch.cuda.set_device(args.local_rank)
File "/usr/local/lib/python3.6/dist-packages/torch/cuda/__init__.py", line 265, in set_device
torch._C._cuda_setDevice(device)
RuntimeError: cuda runtime error (10) : invalid device ordinal at /pytorch/torch/csrc/cuda/Module.cpp:33
main()
File "./examples/run_squad.py", line 437, in main
torch.cuda.set_device(args.local_rank)
File "/usr/local/lib/python3.6/dist-packages/torch/cuda/__init__.py", line 265, in set_device
torch._C._cuda_setDevice(device)
RuntimeError: cuda runtime error (10) : invalid device ordinal at /pytorch/torch/csrc/cuda/Module.cpp:33
THCudaCheck FAIL file=/pytorch/torch/csrc/cuda/Module.cpp line=33 error=10 : invalid device ordinal
Traceback (most recent call last):
File "./examples/run_squad.py", line 527, in <module>
main()
File "./examples/run_squad.py", line 437, in main
torch.cuda.set_device(args.local_rank)
File "/usr/local/lib/python3.6/dist-packages/torch/cuda/__init__.py", line 265, in set_device
torch._C._cuda_setDevice(device)
RuntimeError: cuda runtime error (10) : invalid device ordinal at /pytorch/torch/csrc/cuda/Module.cpp:33
THCudaCheck FAIL file=/pytorch/torch/csrc/cuda/Module.cpp line=33 error=10 : invalid device ordinal
Traceback (most recent call last):
File "./examples/run_squad.py", line 527, in <module>
main()
File "./examples/run_squad.py", line 437, in main
torch.cuda.set_device(args.local_rank)
File "/usr/local/lib/python3.6/dist-packages/torch/cuda/__init__.py", line 265, in set_device
torch._C._cuda_setDevice(device)
RuntimeError: cuda runtime error (10) : invalid device ordinal at /pytorch/torch/csrc/cuda/Module.cpp:33
THCudaCheck FAIL file=/pytorch/torch/csrc/cuda/Module.cpp line=33 error=10 : invalid device ordinal
Traceback (most recent call last):
File "./examples/run_squad.py", line 527, in <module>
main()
File "./examples/run_squad.py", line 437, in main
torch.cuda.set_device(args.local_rank)
File "/usr/local/lib/python3.6/dist-packages/torch/cuda/__init__.py", line 265, in set_device
torch._C._cuda_setDevice(device)
RuntimeError: cuda runtime error (10) : invalid device ordinal at /pytorch/torch/csrc/cuda/Module.cpp:33
THCudaCheck FAIL file=/pytorch/torch/csrc/cuda/Module.cpp line=33 error=10 : invalid device ordinal
Traceback (most recent call last):
File "./examples/run_squad.py", line 527, in <module>
main()
File "./examples/run_squad.py", line 437, in main
torch.cuda.set_device(args.local_rank)
File "/usr/local/lib/python3.6/dist-packages/torch/cuda/__init__.py", line 265, in set_device
torch._C._cuda_setDevice(device)
RuntimeError: cuda runtime error (10) : invalid device ordinal at /pytorch/torch/csrc/cuda/Module.cpp:33
Traceback (most recent call last):
File "/usr/lib/python3.6/runpy.py", line 193, in _run_module_as_main
"__main__", mod_spec)
File "/usr/lib/python3.6/runpy.py", line 85, in _run_code
exec(code, run_globals)
File "/usr/local/lib/python3.6/dist-packages/torch/distributed/launch.py", line 235, in <module>
main()
File "/usr/local/lib/python3.6/dist-packages/torch/distributed/launch.py", line 231, in main
cmd=process.args)
subprocess.CalledProcessError: Command '['/usr/bin/python3', '-u', './examples/run_squad.py', '--local_rank=0', '--model_type', 'bert', '--model_name_or_path', 'bert-large-uncased-whole-word-masking', '--do_train', '--do_eval', '--do_lower_case', '--train_file', 'SQUAD_DIR/train-v1.1.json', '--predict_file', 'SQUAD_DIR/dev-v1.1.json', '--learning_rate', '3e-5', '--num_train_epochs', '2', '--max_seq_length', '384', '--doc_stride', '128', '--output_dir', 'wwm_uncased_finetuned_squad/', '--per_gpu_eval_batch_size=3', '--per_gpu_train_batch_size=3']' returned non-zero exit status 1.
Previously I used other bert package by huggingface (before pytorch-transformers), It worked fine and was fast when used fp16 argument. But after changing it to Pytorch-transformers this is not working.
Can anyone help me in this regard?
| 08-05-2019 14:23:42 | 08-05-2019 14:23:42 | This issue has been automatically marked as stale because it has not had recent activity. It will be closed if no further activity occurs. Thank you for your contributions.
|
transformers | 967 | closed | Unable to load weights properly from tf checkpoint | The function ``` load_tf_weights_in_bert ``` in ``` modeling_bert.py ``` is buggy and throws a lot of attribute errors because of what seems as the pointer pointing to the entire model.
For instance for the variable ```bert/encoder/layer_0/attention/output/dense/kernel ``` it throws an attribute error along the lines of ```Bert model has no attribute weight ``` because the pointer is the model ```bert``` itself whereas the pointer should be ```bert.encoder.layer.0.attention.output.dense```. | 08-05-2019 08:59:44 | 08-05-2019 08:59:44 | This issue has been automatically marked as stale because it has not had recent activity. It will be closed if no further activity occurs. Thank you for your contributions.
<|||||>any updates on this?<|||||>This issue has been automatically marked as stale because it has not had recent activity. It will be closed if no further activity occurs. Thank you for your contributions.
|
transformers | 966 | closed | AttributeError: module 'tensorflow.python.training.training' has no attribute 'list_variables' | TF version 1.1.0:
convert_tf_checkpoint_to_pytorch("../biobert1.1/biobert_v1.1_pubmed/biobert_model.ckpt",
"../biobert1.1/biobert_v1.1_pubmed/bert_config.json",
"../biobert1.1/pytorch_model") | 08-05-2019 08:12:07 | 08-05-2019 08:12:07 | This issue has been automatically marked as stale because it has not had recent activity. It will be closed if no further activity occurs. Thank you for your contributions.
<|||||>You just have to upgrade your tensorflow |
transformers | 965 | closed | How to output a vector | How to use BertModel to output a word's vector which like a vector in word2vec? | 08-05-2019 04:00:22 | 08-05-2019 04:00:22 | Hi, you can use the BertModel to give you the encoded representation of the word ids you have as input. The tensor output by the model’s last layer (of dimension `(batch_size, sequence_length, 768)` for the BertModel) can be considered as the BERT-encoded representation of your input and then be used as input for a downstream task. Is this what you were looking for?<|||||>I want to get the word embedding.
Is the following code correct?
model = BertModel.from_pretrained('ms')
embedding = model.embeddings.word_embeddings
‘ms’ is my pretrained bert model path<|||||>Yes, that works!<|||||>thanks! |
transformers | 964 | closed | RoBERTa: model conversion, inference, tests 🔥 | 08-05-2019 02:07:45 | 08-05-2019 02:07:45 | # [Codecov](https://codecov.io/gh/huggingface/pytorch-transformers/pull/964?src=pr&el=h1) Report
> Merging [#964](https://codecov.io/gh/huggingface/pytorch-transformers/pull/964?src=pr&el=desc) into [master](https://codecov.io/gh/huggingface/pytorch-transformers/commit/7729ef738161a0a182b172fcb7c351f6d2b9c50d?src=pr&el=desc) will **increase** coverage by `0.43%`.
> The diff coverage is `84.71%`.
[](https://codecov.io/gh/huggingface/pytorch-transformers/pull/964?src=pr&el=tree)
```diff
@@ Coverage Diff @@
## master #964 +/- ##
==========================================
+ Coverage 79.16% 79.59% +0.43%
==========================================
Files 38 42 +4
Lines 6406 6845 +439
==========================================
+ Hits 5071 5448 +377
- Misses 1335 1397 +62
```
| [Impacted Files](https://codecov.io/gh/huggingface/pytorch-transformers/pull/964?src=pr&el=tree) | Coverage Δ | |
|---|---|---|
| [pytorch\_transformers/tokenization\_xlnet.py](https://codecov.io/gh/huggingface/pytorch-transformers/pull/964/diff?src=pr&el=tree#diff-cHl0b3JjaF90cmFuc2Zvcm1lcnMvdG9rZW5pemF0aW9uX3hsbmV0LnB5) | `88.99% <100%> (+0.87%)` | :arrow_up: |
| [pytorch\_transformers/tokenization\_bert.py](https://codecov.io/gh/huggingface/pytorch-transformers/pull/964/diff?src=pr&el=tree#diff-cHl0b3JjaF90cmFuc2Zvcm1lcnMvdG9rZW5pemF0aW9uX2JlcnQucHk=) | `95.28% <100%> (+0.13%)` | :arrow_up: |
| [pytorch\_transformers/tokenization\_xlm.py](https://codecov.io/gh/huggingface/pytorch-transformers/pull/964/diff?src=pr&el=tree#diff-cHl0b3JjaF90cmFuc2Zvcm1lcnMvdG9rZW5pemF0aW9uX3hsbS5weQ==) | `83.73% <100%> (+1.68%)` | :arrow_up: |
| [...ytorch\_transformers/tests/tokenization\_xlm\_test.py](https://codecov.io/gh/huggingface/pytorch-transformers/pull/964/diff?src=pr&el=tree#diff-cHl0b3JjaF90cmFuc2Zvcm1lcnMvdGVzdHMvdG9rZW5pemF0aW9uX3hsbV90ZXN0LnB5) | `97.72% <100%> (+0.5%)` | :arrow_up: |
| [...torch\_transformers/tests/tokenization\_bert\_test.py](https://codecov.io/gh/huggingface/pytorch-transformers/pull/964/diff?src=pr&el=tree#diff-cHl0b3JjaF90cmFuc2Zvcm1lcnMvdGVzdHMvdG9rZW5pemF0aW9uX2JlcnRfdGVzdC5weQ==) | `98.66% <100%> (+0.15%)` | :arrow_up: |
| [...orch\_transformers/tests/tokenization\_xlnet\_test.py](https://codecov.io/gh/huggingface/pytorch-transformers/pull/964/diff?src=pr&el=tree#diff-cHl0b3JjaF90cmFuc2Zvcm1lcnMvdGVzdHMvdG9rZW5pemF0aW9uX3hsbmV0X3Rlc3QucHk=) | `97.91% <100%> (+0.41%)` | :arrow_up: |
| [pytorch\_transformers/tokenization\_utils.py](https://codecov.io/gh/huggingface/pytorch-transformers/pull/964/diff?src=pr&el=tree#diff-cHl0b3JjaF90cmFuc2Zvcm1lcnMvdG9rZW5pemF0aW9uX3V0aWxzLnB5) | `86.12% <66.66%> (-0.2%)` | :arrow_down: |
| [pytorch\_transformers/modeling\_roberta.py](https://codecov.io/gh/huggingface/pytorch-transformers/pull/964/diff?src=pr&el=tree#diff-cHl0b3JjaF90cmFuc2Zvcm1lcnMvbW9kZWxpbmdfcm9iZXJ0YS5weQ==) | `73.52% <73.52%> (ø)` | |
| [...ytorch\_transformers/tests/modeling\_roberta\_test.py](https://codecov.io/gh/huggingface/pytorch-transformers/pull/964/diff?src=pr&el=tree#diff-cHl0b3JjaF90cmFuc2Zvcm1lcnMvdGVzdHMvbW9kZWxpbmdfcm9iZXJ0YV90ZXN0LnB5) | `78.81% <78.81%> (ø)` | |
| [...ch\_transformers/tests/tokenization\_roberta\_test.py](https://codecov.io/gh/huggingface/pytorch-transformers/pull/964/diff?src=pr&el=tree#diff-cHl0b3JjaF90cmFuc2Zvcm1lcnMvdGVzdHMvdG9rZW5pemF0aW9uX3JvYmVydGFfdGVzdC5weQ==) | `92.15% <92.15%> (ø)` | |
| ... and [7 more](https://codecov.io/gh/huggingface/pytorch-transformers/pull/964/diff?src=pr&el=tree-more) | |
------
[Continue to review full report at Codecov](https://codecov.io/gh/huggingface/pytorch-transformers/pull/964?src=pr&el=continue).
> **Legend** - [Click here to learn more](https://docs.codecov.io/docs/codecov-delta)
> `Δ = absolute <relative> (impact)`, `ø = not affected`, `? = missing data`
> Powered by [Codecov](https://codecov.io/gh/huggingface/pytorch-transformers/pull/964?src=pr&el=footer). Last update [7729ef7...c4ef103](https://codecov.io/gh/huggingface/pytorch-transformers/pull/964?src=pr&el=lastupdated). Read the [comment docs](https://docs.codecov.io/docs/pull-request-comments).
<|||||>I think RoBERTa is missing in `__init__.py`, so it can't be imported :(<|||||>Would be nice with a modified lm pretraining script to support RoBERTa (ie both removing the NSP task and adding dynamic masking). I might do it in next week.<|||||>@julien-c Does RoBERTa uses token_type_embeddings or token_type_ids as an input? It looks like it doesn't use because token type embeddings matrix has only one row with zeros inside. Am I right?<|||||>@avostryakov You're right.<|||||>@julien-c I modified
MODEL_CLASSES = {...
'roberta': (RobertaConfig, RobertaForSequenceClassification, RobertaTokenizer)} in run_glue.py and it started to train with a parameter "--model_type roberta". I think you can modify run_glue.py too to have an example of roberta usage.<|||||>@avostryakov Yes!! I was about to add this indeed.<|||||>Thanks for this! It would be helpful with entries in `modeling_auto` and `tokenization_auto` as well (just remember to check for `'roberta' in model_name` before `'bert' in model_name` ;) ) |
|
transformers | 963 | closed | Update modeling_bert.py | 08-05-2019 00:58:25 | 08-05-2019 00:58:25 | for win10 cpu<|||||>Ok! |
|
transformers | 962 | closed | Update modeling_xlnet.py | 08-05-2019 00:57:27 | 08-05-2019 00:57:27 | for win10 cpu<|||||>LGTM! |
|
transformers | 961 | closed | Deep learning NLP models for children's story understanding? | I'm working on building NLP systems with common sense reasoning, starting with children's story understanding. I'm very interested in applying the latest pre-trained models here (and maybe Facebook's Roberta too) to a story (not one of the tested datasets like Squad 2.0 and GLUE) for QA, but am not sure how to approach it.
If the answers can be found in the text, will a modified script of run_squad.py be expected to achieve about 90% accuracy? What if the answers need commonsense knowledge and reasoning not explicitly specified in the text?
For example, if we use one of the models (Bert, GPT-2, XLNet, Roberta...) to process the Aesop's story The Fox and the Grapes, will it be able to answer questions such as:
What did the Fox gaze at when his mouth watered?
How many times did the Fox try to get the grapes?
Why did the Fox's mouth water?
Were the grapes sour or ripe?
*****
THE FOX AND THE GRAPES
A Fox one day spied a beautiful bunch of ripe grapes hanging from
a vine trained along the branches of a tree. The grapes seemed
ready to burst with juice, and the Fox's mouth watered as he
gazed longingly at them.
The bunch hung from a high branch, and the Fox had to jump for
it. The first time he jumped he missed it by a long way. So he
walked off a short distance and took a running leap at it, only
to fall short once more. Again and again he tried, but in vain.
Now he sat down and looked at the grapes in disgust.
"What a fool I am," he said. "Here I am wearing myself out to get
a bunch of sour grapes that are not worth gaping for."
And off he walked very, very scornfully.
*****
If not, what do we need to do to be able to answer the questions?
Thanks for any suggestions and thoughts!
| 08-04-2019 17:11:22 | 08-04-2019 17:11:22 | Hi! Your best bet is indeed to use the models that are state-of-the-art on question answering. It is currently a modified version of BERT (see SpanBERT). I cannot tell you what the accuracy would be on your dataset however, as unfortunately, these models are very sensitive to dataset changes. The SQuAD model (fine-tuned on Wikipedia) probably wouldn't get you groundbreaking results.
You can still try it with our BERT model fine-tuned on SQuAD (`bert-large-uncased-whole-word-masking-finetuned-squad`)
If you are looking to increase the accuracy on a specific set of documents (from my understanding you’re focusing on children stories), it might be a good idea to fine-tune your model on a similar dataset. Doing so would probably yield better results on your question answering. cc @thomwolf <|||||>Thank you @LysandreJik for your comment. Can "the models that are state-of-the-art on question answering" here answer questions which require background knowledge and reasoning not explicitly stated in the text?
Yes I'm focusing on children stories. Do you think The Children’s Book Test of the Facebook bAbi project (https://research.fb.com/downloads/babi/) might be a good dataset to fine tune the model on?
Two more questions please: Is there a tutorial on how to prepare a dataset for question answering to fine-tune the Bert model?
If such a dataset is hard to obtain or a lot more data would be needed, would a rule-based method be more practical? <|||||>This issue has been automatically marked as stale because it has not had recent activity. It will be closed if no further activity occurs. Thank you for your contributions.
<|||||>@LysandreJik @thomwolf First of all, thanks a lot for your team's great work on the Swift Core ML implementations of Bert and GPT-2. I just got the chance to try out the BERT-SQuAD iOS sample and it works pretty amazingly if the answer is located in the text, although questions that require some kind of reasoning or answers that are not explicitly stated in the text like motivations or causes/effects are still tough to get right.
Do you think a hybrid approach of using rule-based common sense knowledge and reasoning with the latest deep learning NLP models would be the best way to answer questions which require background knowledge and reasoning not explicitly stated in the text?<|||||>This issue has been automatically marked as stale because it has not had recent activity. It will be closed if no further activity occurs. Thank you for your contributions.
<|||||>SQuAD is extractive question answering so will only give you spans inside the original text<|||||>By the way on common sense reasoning, you can check out this great repo by @atcbosselut: https://github.com/atcbosselut/comet-commonsense<|||||>Thanks @julien-c. I took a brief look at the paper a few months ago and will check out the repo and study the paper more carefully. |
transformers | 960 | closed | Fixing unused weight_decay argument | Currently the L2 regularization is hard-coded to "0.01", even though there is a --weight_decay flag implemented (that is unused). I'm making this flag control the weight decay used for fine-tuning in this script. | 08-04-2019 16:32:05 | 08-04-2019 16:32:05 | # [Codecov](https://codecov.io/gh/huggingface/pytorch-transformers/pull/960?src=pr&el=h1) Report
> Merging [#960](https://codecov.io/gh/huggingface/pytorch-transformers/pull/960?src=pr&el=desc) into [master](https://codecov.io/gh/huggingface/pytorch-transformers/commit/44dd941efb602433b7edc29612cbdd0a03bf14dc?src=pr&el=desc) will **not change** coverage.
> The diff coverage is `n/a`.
[](https://codecov.io/gh/huggingface/pytorch-transformers/pull/960?src=pr&el=tree)
```diff
@@ Coverage Diff @@
## master #960 +/- ##
=======================================
Coverage 79.04% 79.04%
=======================================
Files 34 34
Lines 6242 6242
=======================================
Hits 4934 4934
Misses 1308 1308
```
------
[Continue to review full report at Codecov](https://codecov.io/gh/huggingface/pytorch-transformers/pull/960?src=pr&el=continue).
> **Legend** - [Click here to learn more](https://docs.codecov.io/docs/codecov-delta)
> `Δ = absolute <relative> (impact)`, `ø = not affected`, `? = missing data`
> Powered by [Codecov](https://codecov.io/gh/huggingface/pytorch-transformers/pull/960?src=pr&el=footer). Last update [44dd941...28ba345](https://codecov.io/gh/huggingface/pytorch-transformers/pull/960?src=pr&el=lastupdated). Read the [comment docs](https://docs.codecov.io/docs/pull-request-comments).
<|||||>Indeed, thanks Ethan! |
transformers | 959 | closed | Use the fine-tuned model for another task | Hi, I am currently using this code to research the transferability of those pre-trained models and I wonder how could I apply the fine-tuned parameter of a model to another model. For example, I fine-tuned the **BertForMultipleChoice** and got the **pytorch_model.bin**, and what if I want to use the parameters weight above in the **BertForMaskedLM**.
I believed there should exist a way to do that since they just differ in the linear layer. However, simply use the BertForMaskedLM.from_pretrained method is problematic. | 08-04-2019 13:58:58 | 08-04-2019 13:58:58 | Hi!
If you saved the model `BertForMultipleChoice` to a directory, you can then load the weights for the `BertForMaskedLM` by simply using the `from_pretrained(dir_name)` method. The transformer weights will be re-used by the `BertForMaskedLM` and the weights corresponding to the multiple-choice classifier will be ignored.<|||||>Hi! Thanks for answering me. And this is what I have done at first, which resulted in the following:
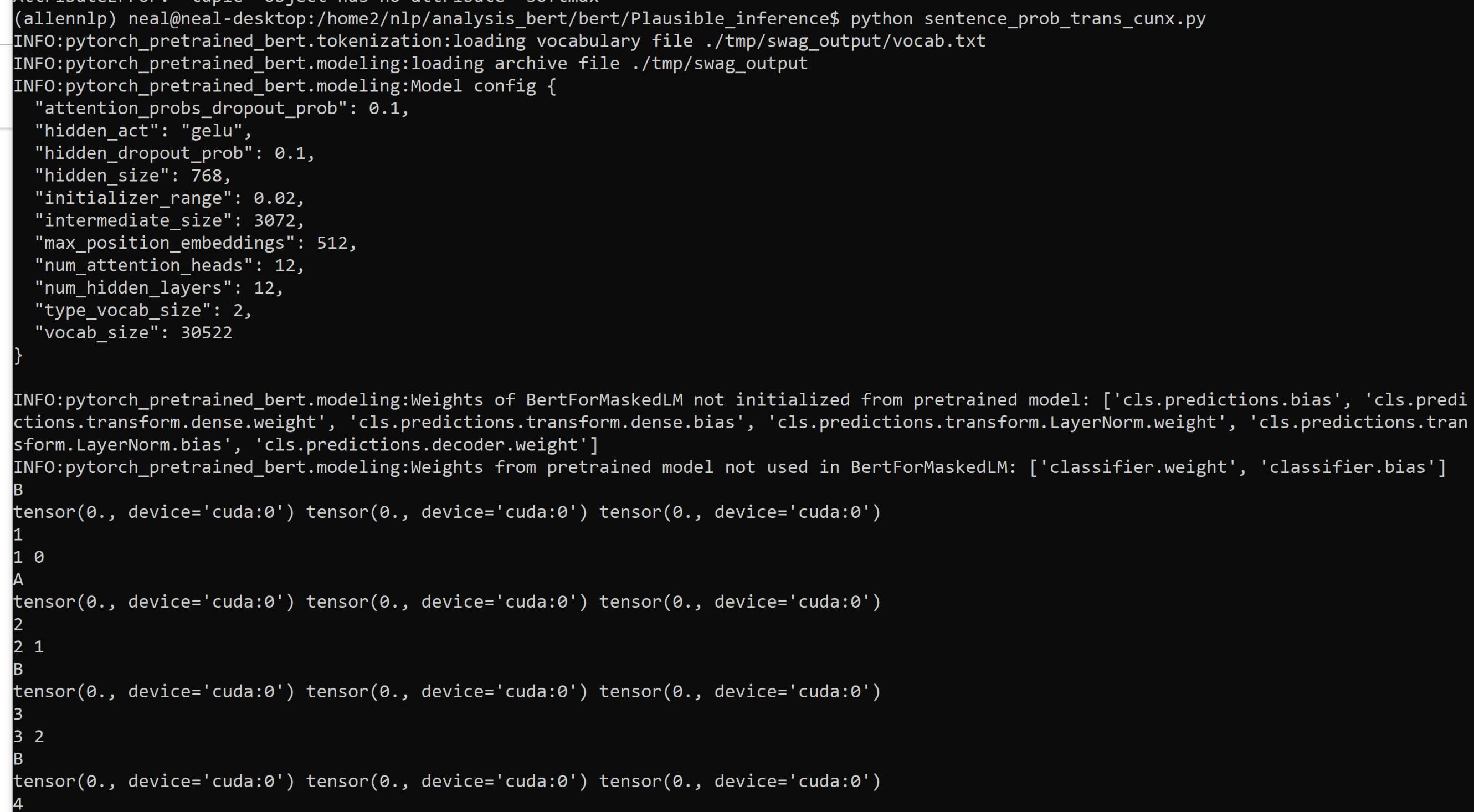
As you can see, the output tensors are all zeros, which seems to be really weird!
Although this might happen, I still want to confirm that I am doing the right thing, I basically calculating each masked word's probability. And some of them are zero which results in the final sentence zero probs.
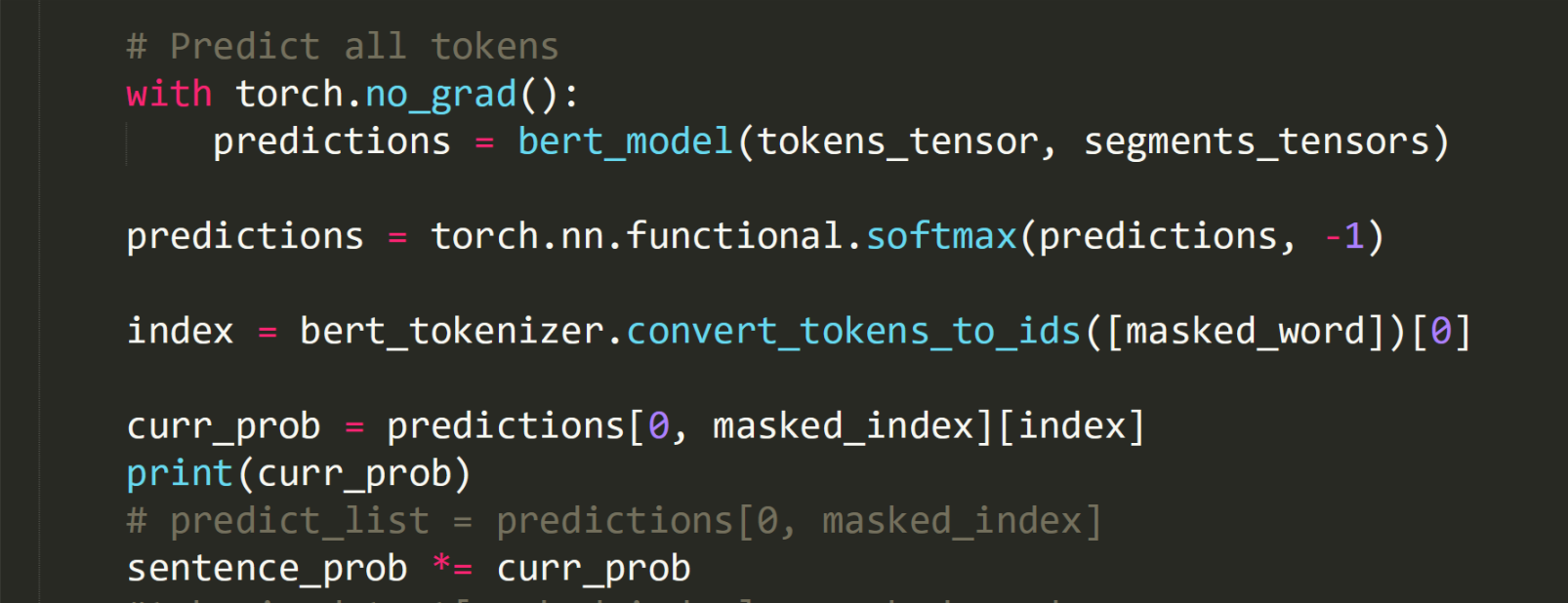
<|||||>Could you share a code snippet that reproduces what you're trying to do so that I can try and see on my side?<|||||>For sure!
```
import torch
from pytorch_pretrained_bert import BertTokenizer, BertModel, BertForMaskedLM
import numpy as np
import math
# OPTIONAL: if you want to have more information on what's happening, activate the logger as follows
import logging
logging.basicConfig(level=logging.INFO)
def predict(text, bert_model, bert_tokenizer):
# Tokenized input
# text = "[CLS] I got restricted because Tom reported my reply [SEP]"
text = "[CLS] " + text + " [SEP]"
tokenized_text = bert_tokenizer.tokenize(text)
# text = "[CLS] Stir the mixture until it is done [SEP]"
#masked_index = 4
sentence_prob = 1
for masked_index in range(1,len(tokenized_text)-1):
# Mask a token that we will try to predict back with `BertForMaskedLM`
masked_word = tokenized_text[masked_index]
#tokenized_text[masked_index] = '[MASK]'
# assert tokenized_text == ['[CLS]', 'who', 'was', 'jim', 'henson', '?', '[SEP]', 'jim', '[MASK]', 'was', 'a', 'puppet', '##eer', '[SEP]']
# print (tokenized_text)
# Convert token to vocabulary indices
indexed_tokens = bert_tokenizer.convert_tokens_to_ids(tokenized_text)
# Define sentence A and B indices associated to 1st and 2nd sentences (see paper)
# segments_ids = [0, 0, 0, 0, 0, 1, 1, 1, 1, 1, 1]
length = len(tokenized_text)
segments_ids = [0 for _ in range(length)]
# Convert inputs to PyTorch tensors
tokens_tensor = torch.tensor([indexed_tokens])
segments_tensors = torch.tensor([segments_ids])
# If you have a GPU, put everything on cuda
tokens_tensor = tokens_tensor.to('cuda')
segments_tensors = segments_tensors.to('cuda')
# Load pre-trained model (weights)
# bert_model = BertForMaskedLM.from_pretrained('bert-large-uncased')
# bert_model.eval()
# If you have a GPU, put everything on cuda
tokens_tensor = tokens_tensor.to('cuda')
segments_tensors = segments_tensors.to('cuda')
bert_model.to('cuda')
# Predict all tokens
with torch.no_grad():
predictions = bert_model(tokens_tensor, segments_tensors)
predictions = torch.nn.functional.softmax(predictions, -1)
index = bert_tokenizer.convert_tokens_to_ids([masked_word])[0]
curr_prob = predictions[0, masked_index][index]
if curr_prob.item()!=0:
#print(curr_prob.item())
sentence_prob *= curr_prob.item()
# predict_list = predictions[0, masked_index]
#tokenized_text[masked_index] = masked_word
#return math.pow(sentence_prob, 1/(len(tokenized_text)-3))
return sentence_prob
# Load pre-trained model tokenizer (vocabulary)
tokenizer = BertTokenizer.from_pretrained('./tmp/swag_output')
# Load pre-trained model (weights)
model = BertForMaskedLM.from_pretrained('./tmp/swag_output')
model.eval()
# prob = predict(sentence_1, bert_model=model, bert_tokenizer=tokenizer)
with open("Sentence4leyang.txt", "r") as f:
file = f.readlines()
num = len(file)
count = 0
curr = 0
for i in file:
label, sentence_1, sentence_2, sentence_3 = i.split("\001")
print (label[0])
prob_1 = predict(sentence_1, bert_model=model, bert_tokenizer=tokenizer)
prob_2 = predict(sentence_2, bert_model=model, bert_tokenizer=tokenizer)
prob_3 = predict(sentence_3, bert_model=model, bert_tokenizer=tokenizer)
answer = max(prob_1, prob_2, prob_3)
print(prob_1, prob_2, prob_3)
```
For the txt file, you could just create some sentences to replace it.
We used the weight after fine-tuning the Bert with official run_swag.py example.<|||||>If you finetuned a `BertForMultipleChoice` and load it in `BertForMaskedLM`some weights will be initialized randomly and not trained.
This is indicated in this part of your output:

If you use this model with un-trained weights you will have random output. You need to train these weights on a down-stream task.<|||||>Hi, Thanks for the response. @thomwolf
However, from my perspective, even if you use the vanilla `Bert-base-uncased` model, the `BertForMaskedLM` still runs perfectly without any random initialization. And I assume `BertForMultipleChoice` is simply the original `Bert-base-uncased` model with an additional linear classifier layer.
Therefore, I think there should be a way to only keep the 'Bert model' but without the linear layer after fine-tuning. I think this feature could be really helpful for researchers to investigate the transferability of the models.<|||||>No unfortunately.
So the model used for pretraining bert and the one we provide on our AWS S3 bucket is `BertForPretraining` which has 2 heads: (i) the masked lm head and (ii) the next sentence prediction head.
`BertForMaskedLM` is a sub-set of `BertForPretraining` which keeps only the first head => all the weights are initialized with pretrained weights if you initialize it from the provided weights, you can use it out-of-the-box.
`BertForMultipleChoice` does NOT have a masked lm head and has instead a multiple-choice head => if you train this model and use it to initialize a `BertForMaskedLM` you won't initialize the language model head.
If you don't remember: just look at the log during model initialization. If it's written `Weights from XXX not initialized from pretrained model` it means you have to train the model before using it.<|||||>We will make the documentation more clear on that.
For your specific use-case, a solution could be to make a model your-self similarly to the way they are made in the library and keep the language modeling head as well as the other heads you want. And then fine-tune the newly added head on your dataset.<|||||>This issue has been automatically marked as stale because it has not had recent activity. It will be closed if no further activity occurs. Thank you for your contributions.
|
transformers | 958 | closed | Fixed small typo | 08-04-2019 06:06:56 | 08-04-2019 06:06:56 | # [Codecov](https://codecov.io/gh/huggingface/pytorch-transformers/pull/958?src=pr&el=h1) Report
> Merging [#958](https://codecov.io/gh/huggingface/pytorch-transformers/pull/958?src=pr&el=desc) into [master](https://codecov.io/gh/huggingface/pytorch-transformers/commit/44dd941efb602433b7edc29612cbdd0a03bf14dc?src=pr&el=desc) will **not change** coverage.
> The diff coverage is `n/a`.
[](https://codecov.io/gh/huggingface/pytorch-transformers/pull/958?src=pr&el=tree)
```diff
@@ Coverage Diff @@
## master #958 +/- ##
=======================================
Coverage 79.04% 79.04%
=======================================
Files 34 34
Lines 6242 6242
=======================================
Hits 4934 4934
Misses 1308 1308
```
------
[Continue to review full report at Codecov](https://codecov.io/gh/huggingface/pytorch-transformers/pull/958?src=pr&el=continue).
> **Legend** - [Click here to learn more](https://docs.codecov.io/docs/codecov-delta)
> `Δ = absolute <relative> (impact)`, `ø = not affected`, `? = missing data`
> Powered by [Codecov](https://codecov.io/gh/huggingface/pytorch-transformers/pull/958?src=pr&el=footer). Last update [44dd941...836e513](https://codecov.io/gh/huggingface/pytorch-transformers/pull/958?src=pr&el=lastupdated). Read the [comment docs](https://docs.codecov.io/docs/pull-request-comments).
<|||||>Nice! |
|
transformers | 957 | closed | total training steps and tokenization in run_glue | Question for total Training Steps:
In run_glue line 78, the total number of training steps is calculated using `t_total = len(train_dataloader) // args.gradient_accumulation_steps * args.num_train_epochs`.
I was wondering if we use gradient accumulation the num_train_epochs in the above code is not actual training epochs instead the actual training epochs may be `args.num_train_epochs/args.gradient_accumulation_steps`. So the total training steps should be `t_total = (len(train_dataloader) // args.gradient_accumulation_steps) * (args.num_train_epochs / args.gradient_accumulation_steps)`. Is my understanding correct?
Question for tokenization:
I saw in your `utils_glue.py`'s `convert_examples_to_features` function, you set `cls_token_at_end=False, pad_on_left=False` , but didn't provide any accesses to change these parameters when users want to fine tune xlnet. Does this will decrease the xlnet fine tuning performance?
Thank you. | 08-03-2019 17:41:25 | 08-03-2019 17:41:25 | |
transformers | 956 | closed | Tokenizer added special token attributes missing | It might not be a bug but I think it would be useful and more consitent behaviour if tokenizers could maintain the added special tokens as attributes after saving and loading a tokenizer. See the following example.
```python
if 'added_tokens.json' in os.listdir('.'):
# loading the saved extended tokenizer
# and trying to reach the added special token
# through the attribute raises an error
tokenizer = XLNetTokenizer.from_pretrained('.')
print(tokenizer.custom_token)
else:
# loading a base tokenizer and extending it with special
# token which is added to the instance attributes
tokenizer = XLNetTokenizer.from_pretrained('xlnet-base-cased')
tokenizer.add_special_tokens({'custom_token': '<custom>'})
# saving the extended tokenizer
tokenizer.save_pretrained('.')
print(tokenizer.custom_token)
```
**1st run result:**
```text
<custom>
```
**2nd run result:**
```text
Traceback (most recent call last):
File "src/_test.py", line 19, in <module>
main()
File "src/_test.py", line 9, in main
print(tokenizer.custom_token)
AttributeError: 'XLNetTokenizer' object has no attribute 'custom_token'
``` | 08-03-2019 08:47:04 | 08-03-2019 08:47:04 | The framework has been updated to store all additional special tokens in `additional_special_tokens` list and custom tokens are no longer available through class attributes. |
transformers | 955 | closed | Fix comment typo | 08-03-2019 04:18:55 | 08-03-2019 04:18:55 | # [Codecov](https://codecov.io/gh/huggingface/pytorch-transformers/pull/955?src=pr&el=h1) Report
> Merging [#955](https://codecov.io/gh/huggingface/pytorch-transformers/pull/955?src=pr&el=desc) into [master](https://codecov.io/gh/huggingface/pytorch-transformers/commit/44dd941efb602433b7edc29612cbdd0a03bf14dc?src=pr&el=desc) will **not change** coverage.
> The diff coverage is `100%`.
[](https://codecov.io/gh/huggingface/pytorch-transformers/pull/955?src=pr&el=tree)
```diff
@@ Coverage Diff @@
## master #955 +/- ##
=======================================
Coverage 79.04% 79.04%
=======================================
Files 34 34
Lines 6242 6242
=======================================
Hits 4934 4934
Misses 1308 1308
```
| [Impacted Files](https://codecov.io/gh/huggingface/pytorch-transformers/pull/955?src=pr&el=tree) | Coverage Δ | |
|---|---|---|
| [pytorch\_transformers/modeling\_bert.py](https://codecov.io/gh/huggingface/pytorch-transformers/pull/955/diff?src=pr&el=tree#diff-cHl0b3JjaF90cmFuc2Zvcm1lcnMvbW9kZWxpbmdfYmVydC5weQ==) | `87.98% <100%> (ø)` | :arrow_up: |
------
[Continue to review full report at Codecov](https://codecov.io/gh/huggingface/pytorch-transformers/pull/955?src=pr&el=continue).
> **Legend** - [Click here to learn more](https://docs.codecov.io/docs/codecov-delta)
> `Δ = absolute <relative> (impact)`, `ø = not affected`, `? = missing data`
> Powered by [Codecov](https://codecov.io/gh/huggingface/pytorch-transformers/pull/955?src=pr&el=footer). Last update [44dd941...a24f830](https://codecov.io/gh/huggingface/pytorch-transformers/pull/955?src=pr&el=lastupdated). Read the [comment docs](https://docs.codecov.io/docs/pull-request-comments).
<|||||>Thanks! |
|
transformers | 954 | closed | Bert model instantiated from BertForMaskedLM.from_pretrained('bert-base-uncased') and BertForMaskedLM(BertConfig.from_pretrained('bert-base-uncased')) give different results | The two different methods for instantiating a model produce different losses.
`from pytorch_transformers import BertForMaskedLM, BertConfig, BertTokenizer
import torch
tokenizer = BertTokenizer.from_pretrained('bert-base-uncased')
input_ids = torch.tensor(tokenizer.encode("Hello, my dog is cute")).unsqueeze(0)
config = BertConfig.from_pretrained('bert-base-uncased')
config_model = BertForMaskedLM(config)
config_model.eval()
with torch.no_grad():
config_outputs = config_model(input_ids, masked_lm_labels=input_ids)
config_loss = config_outputs[0]
print(config_loss.item())
pretrained_model = BertForMaskedLM.from_pretrained('bert-base-uncased')
pretrained_model.eval()
with torch.no_grad():
pretrained_outputs = pretrained_model(input_ids, masked_lm_labels=input_ids)
pretrained_loss = pretrained_outputs[0]
print(pretrained_loss.item())
assert config_loss.item() == pretrained_loss.item()`
The losses produced:
10.574708938598633
1.690806269645691
| 08-03-2019 03:11:00 | 08-03-2019 03:11:00 | Hi, not only they give different results, but also BertModel(BertConfig.from_pretrained('bert-base-uncased')) will give a different result each time you run it. **Other bert models also have this problem**; I think this is a bug. @thomwolf
Following code works well and produce the same result each time you run it.
___________________________
import torch
from pytorch_transformers import BertTokenizer, BertModel, BertConfig
import numpy as np
tokenizer = BertTokenizer.from_pretrained('bert-base-uncased')
model = BertModel.from_pretrained('bert-base-uncased')
model.eval()
input_ids = torch.tensor(tokenizer.encode("Hello, my dog is cute")).unsqueeze(0) # Batch size 1
outputs = model(input_ids)
a = np.squeeze(outputs[0].detach().numpy())
avg = np.mean(a,axis = 0)
print(avg[0])
**Above code will always output -0.2769656.**
___________________________
Following code will produce a different result each time:
__________________________
config = BertConfig.from_pretrained('bert-base-uncased')
tokenizer = BertTokenizer.from_pretrained('bert-base-uncased')
model = BertModel(config)
model.eval()
input_ids = torch.tensor(tokenizer.encode("Hello, my dog is cute")).unsqueeze(0)
outputs = model(input_ids)
last_hidden_states = outputs[0]
a = np.squeeze(last_hidden_states[0].detach().numpy())
avg = np.mean(a,axis = 0)
print(avg[0])<|||||>It seems that BertModel(config) returns a random intialized Bert model with the architecture as the config file indicates, because the __init__ function doesn't load pretrained weights. BertModel.from_pretrained() is the right function to load both model architecture and pretrained weights. It's the same for other bert classes.<|||||>Shouldn't BertConfig.from_pretrained('bert-base-uncased') return a config that loads pretrained weights instead of randomly initialized ones? I thought was the whole point of the example code in the [docs](https://huggingface.co/pytorch-transformers/model_doc/bert.html#bertformaskedlm):
config = BertConfig.from_pretrained('bert-base-uncased')
tokenizer = BertTokenizer.from_pretrained('bert-base-uncased')
model = BertForMaskedLM(config)
input_ids = torch.tensor(tokenizer.encode("Hello, my dog is cute")).unsqueeze(0) # Batch size 1
outputs = model(input_ids, masked_lm_labels=input_ids)
loss, prediction_scores = outputs[:2]<|||||>It should return a config that loads pretrained models; however it does not act like this way.
Sent from Yahoo Mail for iPhone
On Sunday, August 4, 2019, 4:32 PM, Christian Storm <[email protected]> wrote:
Shouldn't BertConfig.from_pretrained('bert-base-uncased') return a config that loads pretrained weights instead of randomly initialized ones? I thought was the whole point of the example code in the docs:
config = BertConfig.from_pretrained('bert-base-uncased')
tokenizer = BertTokenizer.from_pretrained('bert-base-uncased')
model = BertForMaskedLM(config)
input_ids = torch.tensor(tokenizer.encode("Hello, my dog is cute")).unsqueeze(0) # Batch size 1
outputs = model(input_ids, masked_lm_labels=input_ids)
loss, prediction_scores = outputs[:2]
—
You are receiving this because you commented.
Reply to this email directly, view it on GitHub, or mute the thread.
<|||||>You are right, the example in the doc is misleading.
The only way to load pretrained weights in a `model` is to call a `model_class.from_pretrained()` method. I'll fix the doc.<|||||>I've fixed the examples of loading pretrained models in docstrings :-) #973 <|||||>This issue has been automatically marked as stale because it has not had recent activity. It will be closed if no further activity occurs. Thank you for your contributions.
|
transformers | 953 | closed | How to add some parameters in gpt-2 (in attention layer) and initialize the original gpt-2 parameters with pre-trained model and the new introduced parameters randomly? | Hi,
I want to add some weight matrices inside attention layers of gpt-2 model. However, I want to initialize all original parameters with pre-trained gpt-2 and the newly added ones randomly.
Can someone guide me how that's possible or point me to the right direction?
Thanks | 08-02-2019 17:17:05 | 08-02-2019 17:17:05 | You should make a class deriving from `GPT2Model` in which:
- the `__init__` method
* calls its super class `__init__` method (to add the original GPT2 modules),
* you then add the new modules (with names differents from GPT2 original attributes so you don't overwrite over them).
* you call `self.init_weights()` at the end to initalize your weights (check the `init_weights` method in `GPT2PreTrainedModel` to be sure it initialize as you want to)
- the `forward` method has to be written as you want the forward pass to be.
You can then load the pretrained weights and initialize your newly added weights just by doing the usual `model = MyGPT2Model.form_pretrained('gpt2')`.<|||||>Thanks @thomwolf . Just to clarify, does that mean if I need to change the attention layer a little bit, then I have to make three classes derived from ``` GPT2Model``` , ```Block``` ,and ```Attention```? And for that, can I use the original Attention modules inside my forward pass of myAttention?
Should it be something like following?
```
class myAttention(Attention):
def __init__(self, nx, n_ctx, config, scale=False):
super(myAttention, self).__init__()
def forward(): ### my customized forward pass
class myBlock(Block):
def __init__(self, n_ctx, config, scale=False):
super(myBlock, self).__init__()
def forward(...): ### my customized forward pass
class myGPT2Model(GPT2Mode):
def __init__(self, config):
super(myGPT2Model, self).__init__(config)
....
self.apply(self.init_weights)
def forward(...). ### my customized forward pass
```
<|||||>Maybe but it depends on what you put in the `....` parts<|||||>@thomwolf Is it right that I have to have three separate classes each derived from ```GPT2Model```, ```Block``` and ```Attention``` ?
In general, I want to have one additional input to myGPT2Model forward method and I want to incorporate that in the Attention computation.
What I did is I added that aux input to fw of ```myGPT2Model```, I called the block inside myGPT2Model forward with original and aux input,
Then in the myBlock forward method, I called Attention with the two inputs.<|||||>Probably right.
Maybe the easiest in your case would be to copy the `modeling_gpt2` file in whole and modify what you need in the copy.<|||||>This issue has been automatically marked as stale because it has not had recent activity. It will be closed if no further activity occurs. Thank you for your contributions.
|
transformers | 952 | closed | Add 117M and 345M as aliases for pretrained models | This keeps better with the convention in the tensorflow repository. | 08-02-2019 16:18:19 | 08-02-2019 16:18:19 | # [Codecov](https://codecov.io/gh/huggingface/pytorch-transformers/pull/952?src=pr&el=h1) Report
> Merging [#952](https://codecov.io/gh/huggingface/pytorch-transformers/pull/952?src=pr&el=desc) into [master](https://codecov.io/gh/huggingface/pytorch-transformers/commit/44dd941efb602433b7edc29612cbdd0a03bf14dc?src=pr&el=desc) will **not change** coverage.
> The diff coverage is `n/a`.
[](https://codecov.io/gh/huggingface/pytorch-transformers/pull/952?src=pr&el=tree)
```diff
@@ Coverage Diff @@
## master #952 +/- ##
=======================================
Coverage 79.04% 79.04%
=======================================
Files 34 34
Lines 6242 6242
=======================================
Hits 4934 4934
Misses 1308 1308
```
| [Impacted Files](https://codecov.io/gh/huggingface/pytorch-transformers/pull/952?src=pr&el=tree) | Coverage Δ | |
|---|---|---|
| [pytorch\_transformers/modeling\_gpt2.py](https://codecov.io/gh/huggingface/pytorch-transformers/pull/952/diff?src=pr&el=tree#diff-cHl0b3JjaF90cmFuc2Zvcm1lcnMvbW9kZWxpbmdfZ3B0Mi5weQ==) | `75.84% <ø> (ø)` | :arrow_up: |
------
[Continue to review full report at Codecov](https://codecov.io/gh/huggingface/pytorch-transformers/pull/952?src=pr&el=continue).
> **Legend** - [Click here to learn more](https://docs.codecov.io/docs/codecov-delta)
> `Δ = absolute <relative> (impact)`, `ø = not affected`, `? = missing data`
> Powered by [Codecov](https://codecov.io/gh/huggingface/pytorch-transformers/pull/952?src=pr&el=footer). Last update [44dd941...d40e827](https://codecov.io/gh/huggingface/pytorch-transformers/pull/952?src=pr&el=lastupdated). Read the [comment docs](https://docs.codecov.io/docs/pull-request-comments).
<|||||>Thanks, I think we'll stick with `gpt2`, `gpt2-medium` and `gpt2-large` for now.
(also because these number of parameters are actually wrong, the models are respectively 124M and 355M parameters as indicated in the [updated readme of gpt-2](https://github.com/openai/gpt-2#gpt-2)) |
transformers | 951 | closed | run_swag.py should use AdamW | run_swag.py doesn't compile currently, BertAdam is removed (per readme). | 08-02-2019 14:58:50 | 08-02-2019 14:58:50 | # [Codecov](https://codecov.io/gh/huggingface/pytorch-transformers/pull/951?src=pr&el=h1) Report
> Merging [#951](https://codecov.io/gh/huggingface/pytorch-transformers/pull/951?src=pr&el=desc) into [master](https://codecov.io/gh/huggingface/pytorch-transformers/commit/44dd941efb602433b7edc29612cbdd0a03bf14dc?src=pr&el=desc) will **not change** coverage.
> The diff coverage is `n/a`.
[](https://codecov.io/gh/huggingface/pytorch-transformers/pull/951?src=pr&el=tree)
```diff
@@ Coverage Diff @@
## master #951 +/- ##
=======================================
Coverage 79.04% 79.04%
=======================================
Files 34 34
Lines 6242 6242
=======================================
Hits 4934 4934
Misses 1308 1308
```
------
[Continue to review full report at Codecov](https://codecov.io/gh/huggingface/pytorch-transformers/pull/951?src=pr&el=continue).
> **Legend** - [Click here to learn more](https://docs.codecov.io/docs/codecov-delta)
> `Δ = absolute <relative> (impact)`, `ø = not affected`, `? = missing data`
> Powered by [Codecov](https://codecov.io/gh/huggingface/pytorch-transformers/pull/951?src=pr&el=footer). Last update [44dd941...a5e7d11](https://codecov.io/gh/huggingface/pytorch-transformers/pull/951?src=pr&el=lastupdated). Read the [comment docs](https://docs.codecov.io/docs/pull-request-comments).
<|||||># [Codecov](https://codecov.io/gh/huggingface/pytorch-transformers/pull/951?src=pr&el=h1) Report
> Merging [#951](https://codecov.io/gh/huggingface/pytorch-transformers/pull/951?src=pr&el=desc) into [master](https://codecov.io/gh/huggingface/pytorch-transformers/commit/44dd941efb602433b7edc29612cbdd0a03bf14dc?src=pr&el=desc) will **not change** coverage.
> The diff coverage is `n/a`.
[](https://codecov.io/gh/huggingface/pytorch-transformers/pull/951?src=pr&el=tree)
```diff
@@ Coverage Diff @@
## master #951 +/- ##
=======================================
Coverage 79.04% 79.04%
=======================================
Files 34 34
Lines 6242 6242
=======================================
Hits 4934 4934
Misses 1308 1308
```
------
[Continue to review full report at Codecov](https://codecov.io/gh/huggingface/pytorch-transformers/pull/951?src=pr&el=continue).
> **Legend** - [Click here to learn more](https://docs.codecov.io/docs/codecov-delta)
> `Δ = absolute <relative> (impact)`, `ø = not affected`, `? = missing data`
> Powered by [Codecov](https://codecov.io/gh/huggingface/pytorch-transformers/pull/951?src=pr&el=footer). Last update [44dd941...a5e7d11](https://codecov.io/gh/huggingface/pytorch-transformers/pull/951?src=pr&el=lastupdated). Read the [comment docs](https://docs.codecov.io/docs/pull-request-comments).
<|||||>Added a few comments. If you take a look at the `run_glue` and `run_squad` examples, you'll see they are much simpler now in term of optimizer setup. This example could take advantage of the same refactoring if you want to give it a look!<|||||>Thanks for this @jeff-da, we'll close this PR in favor of #1004 for now.
Feel free to re-open if there are other things you would like to change. |
transformers | 950 | closed | CONFIG_NAME and WEIGHTS_NAME are missing in modeling_transfo_xl.py | When I run `convert_transfo_xl_checkpoint_to_pytorch.py`, the following error occurs.
```
Traceback (most recent call last):
File "convert_transfo_xl_checkpoint_to_pytorch.py", line 27, in <module>
from pytorch_transformers.modeling_transfo_xl import (CONFIG_NAME,
ImportError: cannot import name 'CONFIG_NAME'
```
So, in `modeling_transfo_xl.py`, `from .modeling_utils import (PretrainedConfig, PreTrainedModel, add_start_docstrings)` should be `from .modeling_utils import (CONFIG_NAME, WEIGHTS_NAME, PretrainedConfig, PreTrainedModel, add_start_docstrings)`. | 08-02-2019 13:59:39 | 08-02-2019 13:59:39 | Thanks! |
transformers | 949 | closed | <model>ForQuestionAnswering loading non-deterministic weights | I was comparing the weight and bias parameters of two different pre-trained-loaded BertForQuestionAnswering model, and they seem to differ. This causes every instantiation of pre-trained models to have slightly different results.
Compared to #695 where you set the model to eval mode to deactivate dropout layers, the non-deterministic trait seems to come from loading pre-trained models with `BertForQuestionAnswering.from_pretrained("bert-base-uncased")`
To replicate what I'm talking about, you can see below.
```python
model_1 = BertForQuestionAnswering.from_pretrained("bert-base-uncased")
model_2 = BertForQuestionAnswering.from_pretrained("bert-base-uncased")
weights_1 = model_1.state_dict()['qa_outputs.weight']
weights_2 = model_2.state_dict()['qa_outputs.weight']
torch.eq(weights_1, weights_2)
```
This also occurs in XLNetForQuestionAnswering and I was curious to how/why this works that way? | 08-02-2019 13:15:04 | 08-02-2019 13:15:04 | These weights are not pretrained, they are added for fine-tuning the model on a downstream question answering task. You have to train the `qa_output` weights.
They are initialized randomly and so will be different at each run. |
transformers | 948 | closed | How to train BertModel | Hi,
I am trying to train BertModel on my domain-based dataset. Please let me know how to train the BertModel. | 08-02-2019 07:23:21 | 08-02-2019 07:23:21 | Hi, there are examples in the "examples" folder on finetuning language models. Please take a look at [the scripts available here](https://github.com/huggingface/pytorch-transformers/tree/master/examples/lm_finetuning). |
transformers | 947 | closed | [XLNet] Parameters to reproduce SQuAD scores | I'm trying to reproduce the results of XLNet-base on SQuAD 2.0.
From the [README of XLNet](https://github.com/zihangdai/xlnet#results) :
Model | [RACE accuracy](http://www.qizhexie.com/data/RACE_leaderboard.html) | SQuAD1.1 EM | SQuAD2.0 EM
--- | --- | --- | ---
BERT-Large | 72.0 | 84.1 | 78.98
XLNet-Base | | | 80.18
XLNet-Large | **81.75** | **88.95** | **86.12**
---
I ran the example with following hyper-parameters, on a single GPU P100 :
```
python ./examples/run_squad.py \
--model_type xlnet \
--model_name_or_path xlnet-base-cased \
--do_train \
--do_eval \
--train_file squad/train-v1.1.json \
--predict_file squad/dev-v1.1.json \
--learning_rate 3e-5 \
--num_train_epochs 2 \
--max_seq_length 384 \
--doc_stride 128 \
--output_dir ./finetuned_squad_xlnet \
--per_gpu_eval_batch_size 8 \
--per_gpu_train_batch_size 8 \
--save_steps 1000
```
And I got these results :
>{
"exact": 72.88552507095554,
"f1": 80.81417081310839,
"total": 10570,
"HasAns_exact": 72.88552507095554,
"HasAns_f1": 80.81417081310839,
"HasAns_total": 10570
}
It's 8 points lower than the official results.
**What are the parameters needed to reach same score as the official implementation ?**
---
_I open another issue than #822, because my results are not that much off._ | 08-02-2019 05:20:52 | 08-02-2019 05:20:52 | Maybe we can use the same issue so the people following #822 can learn from your experiments as well?<|||||>I'm using xlnet-large-cased.
At first I got
{
"exact": 75.91296121097446,
"f1": 83.19559419987176,
"total": 10570,
"HasAns_exact": 75.91296121097446,
"HasAns_f1": 83.19559419987176,
"HasAns_total": 10570
}
Then I took a look at the XLNet repo and found the current preprocessing in transfomers is a little off. For the XLNet repo, they have P SEP Q SEP CLS, but the preprocessing code in this repo has CLS Q SEP P SEP. I tried to follow the XLNet repo preprocessing code and the hyper parameters in the paper and now I have
{
"exact": 84.37086092715232,
"f1": 92.01817406538726,
"total": 10570,
"HasAns_exact": 84.37086092715232,
"HasAns_f1": 92.01817406538726,
"HasAns_total": 10570
}
Here are my preprocessing code with the changes. Sorry it's a bit messy. I will create a PR next week.
````
# xlnet
cls_token = "[CLS]"
sep_token = "[SEP]"
pad_token = 0
sequence_a_segment_id = 0
sequence_b_segment_id = 1
cls_token_segment_id = 2
# Should this be 4, or it doesn't matter?
pad_token_segment_id = 3
cls_token_at_end = True
mask_padding_with_zero = True
# xlnet
qa_features = []
# unique_id identified unique feature/label pairs. It's different
# from qa_id in that each qa_example can be broken down into
# multiple feature samples if the paragraph length is longer than
# maximum sequence length allowed
query_tokens = tokenizer.tokenize(example.question_text)
if len(query_tokens) > max_question_length:
query_tokens = query_tokens[0:max_question_length]
# map word-piece tokens to original tokens
tok_to_orig_index = []
# map original tokens to corresponding word-piece tokens
orig_to_tok_index = []
all_doc_tokens = []
for (i, token) in enumerate(example.doc_tokens):
orig_to_tok_index.append(len(all_doc_tokens))
sub_tokens = tokenizer.tokenize(token)
for sub_token in sub_tokens:
tok_to_orig_index.append(i)
all_doc_tokens.append(sub_token)
tok_start_position = None
tok_end_position = None
if is_training and example.is_impossible:
tok_start_position = -1
tok_end_position = -1
if is_training and not example.is_impossible:
tok_start_position = orig_to_tok_index[example.start_position]
if example.end_position < len(example.doc_tokens) - 1:
# +1: move the the token after the ending token in
# original tokens
# -1, moves one step back
# these two operations ensures word piece is covered
# when it's part of the original ending token.
tok_end_position = orig_to_tok_index[example.end_position + 1] - 1
else:
tok_end_position = len(all_doc_tokens) - 1
(tok_start_position, tok_end_position) = _improve_answer_span(
all_doc_tokens,
tok_start_position,
tok_end_position,
tokenizer,
example.orig_answer_text,
)
# The -3 accounts for [CLS], [SEP] and [SEP]
max_tokens_for_doc = max_seq_len - len(query_tokens) - 3
# We can have documents that are longer than the maximum sequence length.
# To deal with this we do a sliding window approach, where we take chunks
# of the up to our max length with a stride of `doc_stride`.
_DocSpan = collections.namedtuple("DocSpan", ["start", "length"])
doc_spans = []
start_offset = 0
while start_offset < len(all_doc_tokens):
length = len(all_doc_tokens) - start_offset
if length > max_tokens_for_doc:
length = max_tokens_for_doc
doc_spans.append(_DocSpan(start=start_offset, length=length))
if start_offset + length == len(all_doc_tokens):
break
start_offset += min(length, doc_stride)
for (doc_span_index, doc_span) in enumerate(doc_spans):
if is_training:
unique_id += 1
else:
unique_id += 2
tokens = []
token_to_orig_map = {}
token_is_max_context = {}
segment_ids = []
# p_mask: mask with 1 for token than cannot be in the answer
# (0 for token which can be in an answer)
# Original TF implem also keep the classification token (set to 0), because
# cls token represents prediction for unanswerable question
p_mask = []
# CLS token at the beginning
if not cls_token_at_end:
tokens.append(cls_token)
segment_ids.append(cls_token_segment_id)
p_mask.append(0)
cls_index = 0
# Paragraph
for i in range(doc_span.length):
split_token_index = doc_span.start + i
token_to_orig_map[len(tokens)] = tok_to_orig_index[split_token_index]
## TODO: maybe this can be improved to compute
# is_max_context for each token only once.
is_max_context = _check_is_max_context(doc_spans, doc_span_index, split_token_index)
token_is_max_context[len(tokens)] = is_max_context
tokens.append(all_doc_tokens[split_token_index])
# xlnet
# segment_ids.append(sequence_b_segment_id)
segment_ids.append(sequence_a_segment_id)
# xlnet ends
p_mask.append(0)
paragraph_len = doc_span.length
# xlnet
tokens.append(sep_token)
segment_ids.append(sequence_a_segment_id)
p_mask.append(1)
tokens += query_tokens
segment_ids += [sequence_b_segment_id] * len(query_tokens)
p_mask += [1] * len(query_tokens)
# xlnet ends
# SEP token
tokens.append(sep_token)
segment_ids.append(sequence_b_segment_id)
p_mask.append(1)
# CLS token at the end
if cls_token_at_end:
tokens.append(cls_token)
segment_ids.append(cls_token_segment_id)
p_mask.append(0)
cls_index = len(tokens) - 1 # Index of classification token
input_ids = tokenizer.convert_tokens_to_ids(tokens)
# The mask has 1 for real tokens and 0 for padding tokens. Only real
# tokens are attended to.
input_mask = [1 if mask_padding_with_zero else 0] * len(input_ids)
# Zero-pad up to the sequence length.
if len(input_ids) < max_seq_len:
pad_token_length = max_seq_len - len(input_ids)
pad_mask = 0 if mask_padding_with_zero else 1
input_ids += [pad_token] * pad_token_length
input_mask += [pad_mask] * pad_token_length
segment_ids += [pad_token_segment_id] * pad_token_length
p_mask += [1] * pad_token_length
assert len(input_ids) == max_seq_len
assert len(input_mask) == max_seq_len
assert len(segment_ids) == max_seq_len
assert len(p_mask) == max_seq_len
span_is_impossible = example.is_impossible
start_position = None
end_position = None
if is_training and not span_is_impossible:
# For training, if our document chunk does not contain an annotation
# we throw it out, since there is nothing to predict.
doc_start = doc_span.start
doc_end = doc_span.start + doc_span.length - 1
out_of_span = False
if not (tok_start_position >= doc_start and tok_end_position <= doc_end):
out_of_span = True
if out_of_span:
start_position = 0
end_position = 0
span_is_impossible = True
else:
# +1 for [CLS] token
# +1 for [SEP] token
# xlnet
# doc_offset = len(query_tokens) + 2
doc_offset = 0
# xlnet ends
start_position = tok_start_position - doc_start + doc_offset
end_position = tok_end_position - doc_start + doc_offset
if is_training and span_is_impossible:
start_position = cls_index
end_position = cls_index
```
<|||||>@hlums @Colanim that's amazing, thank you! did you also experiment with SQuAD 2.0? I'm having issues training anything even remotely decent, and deciding whether to answer or not (NoAnswer) seems to be the problem.<|||||>> @hlums @Colanim that's amazing, thank you! did you also experiment with SQuAD 2.0? I'm having issues training anything even remotely decent, and deciding whether to answer or not (NoAnswer) seems to be the problem.
I haven't got a chance to try SQuAD 2.0. My guess is that since the CLS token is needed in SQuAD 2.0 to predict unanswerable questions, when the CLS token is misplaced, the impact on the model performance is bigger. <|||||>This is great @hlums! looking forward to a PR updating the example if you have time<|||||>Updating after I read comments in #1405 carefully.
I've created a local branch with my changes. I will validate it over the weekend.
I'm trying to push my branch to remote and got an access denied error.
This is how I cloned the repo
git clone https://hlums:<my personal access token\>@github.com/huggingface/transformers/
Any one can help? <|||||>@hlums hey you can just fork this repo, make your changes in your version of the repo, and then do a pull request - that should work<|||||>My change is completely independent of data input and preprocessing — it just adjusts a few gemm and batchedGemm calls in the XLNetLayer to be more efficient. I referenced the related issues to give context to the exact f1 scores I was making sure I got on each version of the code. So I believe your PR is very much necessary and important :)
Edit: Original context of the email I replied to as I don't see it here anymore:
@slayton58 , is your change in the modelling code equivalent to changing the order of the tokens in the preprocessing code?<|||||>Thanks for the clarification @slayton58! I figured it out after reading the comments in you PR more carefully. :)<|||||>Thank you guys! I solved the permission denied issue by git clone using ssh instead of https. Not sure why I never had this issue with my company's repos.
Anyway, I forked the repo (https://github.com/hlums/transformers) and pushed my changes to it.
However, I'm still having issue running the run_squad.py script. I'm getting "/data/anaconda/envs/py35/bin/python: Relative module names not supported"
Here are what I did
```
conda install pytorch
cd transformers
pip install --editable .
bash run_squad.sh
```
The content of my bash script is following
```
python -m ./examples/run_squad.py \
--model_type xlnet \
--model_name_or_path xlnet-large-cased \
--do_train \
--do_eval \
--do_lower_case \
--train_file /data/home/hlu/notebooks/NLP/examples/question_answering/train-v1.1.json \
--predict_file /data/home/hlu/notebooks/NLP/examples/question_answering/dev-v1.1.json \
--learning_rate 3e-5 \
--num_train_epochs 2 \
--max_seq_length 384 \
--doc_stride 128 \
--output_dir ./wwm_cased_finetuned_squad/ \
--per_gpu_eval_batch_size=4 \
--per_gpu_train_batch_size=4 \
```<|||||>@hlums
Is your configuration single or multi-GPU?
Using Pytorch==1.3.0 and Transformers=2.1.1?
The reason I ask is that with 2 x 1080Ti NVIDIAs trying to run_squad.py on XLNet & BERT models, I experience data-parallel-run and distributed-performance-reporting (key error) failures. Perhaps you have the solution to either/both?<|||||>@ahotrod I'm using Pytorch 1.2.0. I have 4 NVIDIA V100.
How are you running the script? Are you calling python -m torch.distributed.launch...? Can you try removing torch.distributed.launch? I think it's intended to be used for multi-node training in the way run_squad.py is written, although it can be used for multi-GPU training if we make some changes to run_squad.py. <|||||>@ahotrod I've been seeing key errors only when running eval in distributed -- training is fine (and I've run quite a few full 8xV100 distributed finetunings in the last few weeks), but I have to drop back to `DataParallel` for eval to work.<|||||>@hlums @slayton58 Thank you both for informative, helpful replies.
** Updated, hope I adequately explain my work-around **
I prefer distributed processing for the training speed-up, plus my latest data parallel runs have been loading one of <parameters & buffers> on cuda:1 and shutting down. As recommended I dropped the `do_eval` argument and ran my distributed shell script below, which worked fine. I then ran a `do_eval` script on a single GPU to generate the `predictions_.json` file, which I don't get from a distributed script when including `do_eval` (key error).
Here's my distributed fine-tuning script:
```
SQUAD_DIR=/media/dn/dssd/nlp/transformers/examples/squad1.1
export OMP_NUM_THREADS=6
python -m torch.distributed.launch --nproc_per_node=2 ./run_squad.py \
--model_type xlnet \
--model_name_or_path xlnet-large-cased \
--do_train \
--do_lower_case \
--train_file ${SQUAD_DIR}/train-v1.1.json \
--predict_file ${SQUAD_DIR}/dev-v1.1.json \
--num_train_epochs 3 \
--learning_rate 3e-5 \
--max_seq_length 384 \
--doc_stride 128 \
--save_steps=10000 \
--per_gpu_train_batch_size 1 \
--gradient_accumulation_steps 4 \
--output_dir ./runs/xlnet_large_squad1_dist_X \
```
which maxes-out my 2 x 1080Ti GPUs (0: hybrid, 1: open-frame cooling):
```
***** Running training *****
Num examples = 89993
Num Epochs = 3
Instantaneous batch size per GPU = 1
Total train batch size (w. parallel, distributed & accumulation) = 8
Gradient Accumulation steps = 4
Total optimization steps = 33747
NVIDIA-SMI 430.50 Driver Version: 430.50 CUDA Version: 10.1
0 GeForce GTX 1080Ti
0% 51C P2 256W / 250W | 10166MiB / 11178MiB | 100%
1 GeForce GTX 1080Ti
35% 65C P2 243W / 250W | 10166MiB / 11178MiB | 99%
```
After 3 epochs & ~21 hours, here are the results, similar to @Colanim :
```
***** Running evaluation *****
Num examples = 11057
Batch size = 32
{
"exact": 75.01419110690634,
"f1": 82.13017516396678,
"total": 10570,
"HasAns_exact": 75.01419110690634,
"HasAns_f1": 82.13017516396678,
"HasAns_total": 10570
}
```
generated from my single GPU `do_eval` script pointing to the distributed fine-tuned model (path):
```
CUDA_VISIBLE_DEVICES=0 python run_squad.py \
--model_type xlnet \
--model_name_or_path ${MODEL_PATH} \
--do_eval \
--do_lower_case \
--train_file ${SQUAD_DIR}/train-v1.1.json \
--predict_file ${SQUAD_DIR}/dev-v1.1.json \
--per_gpu_eval_batch_size 32 \
--output_dir ${MODEL_PATH}
```
This model performs well in my Q&A application, but looking forward to @hlums pre-processing code, the imminent RoBERTa-large-SQuAD2.0, and perhaps one-day, ALBERT for the low-resource user that I am.<|||||>OK. Figured out the relative module import issue. Code is running now and should have the PR tomorrow if nothing else goes wrong. <|||||>PR is here #1549. My current result is
{
"exact": 85.45884578997162,
"f1": 92.5974600601065,
"total": 10570,
"HasAns_exact": 85.45884578997162,
"HasAns_f1": 92.59746006010651,
"HasAns_total": 10570
}
Still a few points lower than what's reported in the XLNet paper, but we made some progress. :)<|||||>How to convert
cls_logits: (optional, returned if start_positions or end_positions is not provided)
to probabilities values between 0 to 1?<|||||>This issue has been automatically marked as stale because it has not had recent activity. It will be closed if no further activity occurs. Thank you for your contributions.
|
transformers | 946 | closed | Using memory states with XLNet / TransfoXL | I would like to fine-tune XLNet / TransfoXL for a classification task where I classify each sentence in the context of a large document. Is there an example for how to use the memory states in XLNet and TransfoXL?
This example only uses memory states for inference but there is no example for training:
https://github.com/huggingface/pytorch-transformers/blob/xlnet/examples/single_model_scripts/run_transfo_xl.py
This example doesn't use the memory states:
https://github.com/huggingface/pytorch-transformers/blob/24ed0b9346079da741b952c21966fdc2063292e4/examples/run_xlnet_classifier.py
Naively feeding in the memory states leads to some dimension mismatch at the end of the training epoch:
```
File "/home/lambda/repos/research/trainer/models/xlnet.py", line 86, in forward
mems=new_mems
File "/home/lambda/python-envs/research/lib/python3.6/site-packages/torch/nn/modules/module.py", line 493, in __call__
result = self.forward(*input, **kwargs)
File "/home/lambda/repos/pytorch-transformers/pytorch_transformers/modeling_xlnet.py", line 959, in forward
new_mems = new_mems + (self.cache_mem(output_h, mems[i]),)
File "/home/lambda/repos/pytorch-transformers/pytorch_transformers/modeling_xlnet.py", line 792, in cache_mem
new_mem = torch.cat([prev_mem, curr_out], dim=0)[-self.mem_len:]
RuntimeError: invalid argument 0: Sizes of tensors must match except in dimension 0. Got 14 and 32 in dimension 1 at /pytorch/aten/src/THC/generic/THCTensorMath.cu:71
``` | 08-01-2019 19:22:06 | 08-01-2019 19:22:06 | Which command did you use to "naively" feed in the memory states?
You can just feed the mems that you get from the previous forward pass, but the inputs need to be the continuation of the previous input. So the batch_size, in particular, should stay the same.<|||||>I found this post that talks about how to organize the inputs: https://mlexplained.com/2019/07/04/building-the-transformer-xl-from-scratch/
Is there an example for how to adapt this for classification? Right now the data is organized as `(batch_size x max_seq_length)` and labels are `batch_size`. Each example in the batch represents a sentence, where multiple sentences may come from the same document. For simplicity we can assume they are all from the same document.<|||||>I was looking at doing the same with TransformerXL, but ran into this same issue regarding how to adapt the label vector to work with the data matrix when trying to do classification. I'd appreciate any help from people that have successfully implemented this.<|||||>This issue has been automatically marked as stale because it has not had recent activity. It will be closed if no further activity occurs. Thank you for your contributions.
|
transformers | 945 | closed | _convert_id_to_tokens for XLNet not working | ```
text = self.tokenizer.convert_ids_to_tokens(token_list)
File "/home/lambda/repos/pytorch-transformers/pytorch_transformers/tokenization_utils.py", line 444, in convert_ids_to_tokens
tokens.append(self._convert_id_to_token(index))
File "/home/lambda/repos/pytorch-transformers/pytorch_transformers/tokenization_xlnet.py", line 170, in _convert_id_to_token
token = self.sp_model.IdToPiece(index)
File "/home/lambda/python-envs/research/lib/python3.6/site-packages/sentencepiece.py", line 187, in IdToPiece
return _sentencepiece.SentencePieceProcessor_IdToPiece(self, id)
TypeError: in method 'SentencePieceProcessor_IdToPiece', argument 2 of type 'int'
```
I find that if I explicitly convert ids to integers it works fine. In `tokenization_xlnet.py`
```
def _convert_id_to_token(self, index, return_unicode=True):
"""Converts an index (integer) in a token (string/unicode) using the vocab."""
token = self.sp_model.IdToPiece(int(index))
if six.PY2 and return_unicode and isinstance(token, str):
token = token.decode('utf-8')
return token
``` | 08-01-2019 19:13:25 | 08-01-2019 19:13:25 | Which command can we use to reproduce the behavior?<|||||>Upon further testing, looks like this tokenizer doesn't like numpy arrays, the other ones seem to be fine
```
import numpy as np
from pytorch_transformers import XLNetTokenizer, TransfoXLTokenizer, BertTokenizer
tokenizer = BertTokenizer.from_pretrained('bert-base-uncased')
print(tokenizer.convert_ids_to_tokens(np.array([3, 4, 6, 2356])))
tokenizer = TransfoXLTokenizer.from_pretrained('transfo-xl-wt103')
print(tokenizer.convert_ids_to_tokens(np.array([3, 4, 6, 2356])))
tokenizer = XLNetTokenizer.from_pretrained('xlnet-base-cased')
print(tokenizer.convert_ids_to_tokens(np.array([3, 4, 6, 2356]).tolist()))
print(tokenizer.convert_ids_to_tokens(np.array([3, 4, 6, 2356]))) # Above error
```<|||||>This issue has been automatically marked as stale because it has not had recent activity. It will be closed if no further activity occurs. Thank you for your contributions.
|
transformers | 944 | closed | Missing lines in Readme examples? | 1. In the [example](https://github.com/huggingface/pytorch-transformers#serialization)
```
...
### Do some stuff to our model and tokenizer
# Ex: add new tokens to the vocabulary and embeddings of our model
tokenizer.add_tokens(['[SPECIAL_TOKEN_1]', '[SPECIAL_TOKEN_2]'])
model.resize_token_embeddings(len(tokenizer))
# Train our model
train(model)
...
```
`model.train()` is missing before `train(model)` ?
2. In the [example](https://github.com/huggingface/pytorch-transformers#optimizers-bertadam--openaiadam-are-now-adamw-schedules-are-standard-pytorch-schedules)
```
...
### In PyTorch-Transformers, optimizer and schedules are splitted and instantiated like this:
optimizer = AdamW(model.parameters(), lr=lr, correct_bias=False) # To reproduce BertAdam specific behavior set correct_bias=False
scheduler = WarmupLinearSchedule(optimizer, warmup_steps=num_warmup_steps, t_total=num_total_steps) # PyTorch scheduler
### and used like this:
for batch in train_data:
loss = model(batch)
loss.backward()
scheduler.step()
optimizer.step()
```
`optimizer.zero_grad()` is missing after `optimizer.step()` ? | 08-01-2019 15:21:50 | 08-01-2019 15:21:50 | Thanks<|||||>@thomwolf please, note: the first example hasn't been fixed by the commit.<|||||>Yes, doesn't look like a problem to me. Usually, people put the model in training mode inside the train function (and even inside the training loop I would recommend).<|||||>Ok, got it! |
transformers | 943 | closed | Is pytorch-transformers useful for training from scratch on a custom dataset? | Hello,
I'm looking into the great repo, and I'm wondering if there is a feature that could allow me to train a, let's say, gpt2 model on a custom dataset of sequences.
Is it already provided in your codebase and features ? Otherwise I'll tinker with code on my own.
Thanks in advance and again, great job for the repo which is super useful. | 08-01-2019 13:30:17 | 08-01-2019 13:30:17 | This depends on the model you're interested in. For GPT2, for example, there's a class called `GPT2LMHeadModel` that you could use for pretraining with minimal modifications. For XLNet, the implementation in this repo is missing some key functionality (the permutation generation function and an analogue of the dataset record generator) which you'd have to implement yourself. For the BERT model in this repo, there appears to be a class explicitly designed for this (`BertForPreTraining`). <|||||>Hi, we don't provide efficient scripts for training from scratch but you can have a look at what Microsoft did for instance: https://azure.microsoft.com/en-us/blog/microsoft-makes-it-easier-to-build-popular-language-representation-model-bert-at-large-scale/
They shared all the recipes they used for training a full-scale Bert based on this library. Kudos to them!<|||||>I'd like to see efficient scripts for training from scratch too please. The Azure repo looks interesting, but looks very Azure-specific, and also bert specific. Would be nice to have training scripts within the hugging face repo itself.
(In addition to being able to train standard BERT etc on proprietary data, it would also be nice to be able to easily experiment with training from scratch using variations of the standard BERT etc models, using the existing public datasets).<|||||>@hughperkins
I wrote this post when I modified code to run on (custom) IMDB dataset for BERT model: https://medium.com/dsnet/running-pytorch-transformers-on-custom-datasets-717fd9e10fe2
Not sure if this helps you.<|||||>This issue has been automatically marked as stale because it has not had recent activity. It will be closed if no further activity occurs. Thank you for your contributions.
<|||||>> Hi, we don't provide efficient scripts for training from scratch but you can have a look at what Microsoft did for instance: https://azure.microsoft.com/en-us/blog/microsoft-makes-it-easier-to-build-popular-language-representation-model-bert-at-large-scale/
>
> They shared all the recipes they used for training a full-scale Bert based on this library. Kudos to them!
@thomwolf Indeed this seems very Azure specific and not very helpful. What would be helpful is showing minimal scripts for training transformers, say GPT2, on custom datasets from scratch. Training from scratch is basic requisite functionality for this library to be used in fundamental research as opposed to tweaking / fine-tuning existing results. |
transformers | 942 | closed | Using BERT for predicting masked token | I have a task where I want to obtain better word embeddings for food ingredients. Since I am a bit new to the field of NLP, I have certain fundamental doubts as well which I would love to be corrected upon.
1. I want to get word embeddings so started with Word2Vec. Now, I want to get more contextual representation so using BERT
2. There is no supervised data and so I want to learn embeddings similar to the MASKED training procedure followed in BERT paper itself.
3. I have around 1000 ingredients and each recipe can consist of multiple ingredients.
4. Since BERT works well if we have only one MASKED word, so I would ideally copy the recipe text multiple times and replace ingredients with "MASK" one by one. So, if I have 1 recipe with 5 ingredients, I generate 5 MASKED sentences (`will this lead to overfitting??`)
5. How to handle the case when my ingredient is not part of the BERT vocabulary? Can something be done in that case?
6. Is there some reference where I can start?
I would really appreciate if someone can point out any issues with my assumptions above. | 08-01-2019 10:16:20 | 08-01-2019 10:16:20 | Hi, no need to mask, just input your sequence and keep the hidden-states of the top tokens that correspond to your ingredients.
If your ingredients are not in the vocabulary, they will be split by the tokenizer in sub-word units (totally fine). Then, just use as a representation the mean or the max of the representations for all the sub-word tokens in an ingredient (ex `torch.mean(output[0, 1:3, :], dim=1)` if your ingredient word is made of tokens number 1 and 2 in the first example of the batched input sequence).<|||||>> Hi, no need to mask, just input your sequence and keep the hidden-states of the top tokens that correspond to your ingredients.
>
> If your ingredients are not in the vocabulary, they will be split by the tokenizer in sub-word units (totally fine). Then, just use as a representation the mean or the max of the representations for all the sub-word tokens in an ingredient (ex `torch.mean(output[0, 1:3, :], dim=1)` if your ingredient word is made of tokens number 1 and 2 in the first example of the batched input sequence).
I am trying to figure out how BertForMaskedLM actually works. I saw that in the example, we do not need to mask the input sequence "Hello, my dog is cute". But then in the code, I did not see the random masking taking place either. I am wondering, which word of this input sequence is then masked and where is the ground truth provided?
I am only trying to understand this because I am trying to fine tune the bert model where the task also involves predicting some masked word. And I am trying to figure out how to process the input sequence to signal the "[MASK]" and make the model predict the actual masked out word<|||||>it seems that there is nothing like "run_pretraining.py" in google-research/bert written in tensorflow and the pretrained model is converted from tensorflow, right?<|||||>This issue has been automatically marked as stale because it has not had recent activity. It will be closed if no further activity occurs. Thank you for your contributions.
<|||||>Has anyone figured out exactly how words in BERT are masked for masked LM, or where this occurs in the code? I'm trying to understand if the masked token is initialized randomly for every single epoch. <|||||>That would be related to the training script. If you're using the `run_lm_finetuning.py` script, then [these lines](https://github.com/huggingface/transformers/blob/master/examples/run_lm_finetuning.py#L169-L191) are responsible for the token masking. |
transformers | 941 | closed | Updated model token sizing to replace removed parameter `num_special_… | …tokens`
`num_special_tokens` seems to no longer be implemented. Replaced with `model.resize_token_embeddings(new_num_tokens=len(tokenizer))` which resizes (non-destructively, I think) the embeddings to include the new tokens. | 08-01-2019 10:13:17 | 08-01-2019 10:13:17 | # [Codecov](https://codecov.io/gh/huggingface/pytorch-transformers/pull/941?src=pr&el=h1) Report
> Merging [#941](https://codecov.io/gh/huggingface/pytorch-transformers/pull/941?src=pr&el=desc) into [master](https://codecov.io/gh/huggingface/pytorch-transformers/commit/f2a3eb987e1fc2c85320fc3849c67811f5736b50?src=pr&el=desc) will **not change** coverage.
> The diff coverage is `n/a`.
[](https://codecov.io/gh/huggingface/pytorch-transformers/pull/941?src=pr&el=tree)
```diff
@@ Coverage Diff @@
## master #941 +/- ##
=======================================
Coverage 79.04% 79.04%
=======================================
Files 34 34
Lines 6242 6242
=======================================
Hits 4934 4934
Misses 1308 1308
```
------
[Continue to review full report at Codecov](https://codecov.io/gh/huggingface/pytorch-transformers/pull/941?src=pr&el=continue).
> **Legend** - [Click here to learn more](https://docs.codecov.io/docs/codecov-delta)
> `Δ = absolute <relative> (impact)`, `ø = not affected`, `? = missing data`
> Powered by [Codecov](https://codecov.io/gh/huggingface/pytorch-transformers/pull/941?src=pr&el=footer). Last update [f2a3eb9...c8f622a](https://codecov.io/gh/huggingface/pytorch-transformers/pull/941?src=pr&el=lastupdated). Read the [comment docs](https://docs.codecov.io/docs/pull-request-comments).
<|||||>Hi, I'm also running into this issue. Simply removing the `num_special_tokens=len(special_tokens)` argument seems to resolve the issue, since I'm able to reproduce the scores on the RocStories example.<|||||>This issue has been automatically marked as stale because it has not had recent activity. It will be closed if no further activity occurs. Thank you for your contributions.
|
transformers | 940 | closed | Unexpectedly preprocess when multi-gpu using | When run example/run_squad with more than one gpu, the preprocessor cannot work as expected. For example, the unique_id will not be a serial numbers, then keyerror occurs when writing the result to json file.
https://github.com/huggingface/pytorch-transformers/blob/f2a3eb987e1fc2c85320fc3849c67811f5736b50/examples/utils_squad.py#L511
I do not check there are others unexpectedly behavior or not yet.
I'll update the issue after checking them.
| 08-01-2019 08:44:49 | 08-01-2019 08:44:49 | Why do you think the unique_id is not serial?
Each process should convert ALL the dataset.
Only the PyTorch dataset should be split among processes.
By the way it would be cleaner if the other processes wait for the first process to pre-process the dataset before using the cache so the dataset is only converted once and not several time in parrallel (waste of compute). I'll add this option.<|||||>@thomwolf Hi, thanks for your reply
For example, the unique_id should [1000000, 1000001, 1000002, ...]
However, with multi-process I got [1000000, 100001, 1000004, ....]
I did not check what cause the error.
As a result, when predict the answer with multiple gpu, the key error happened.<|||||>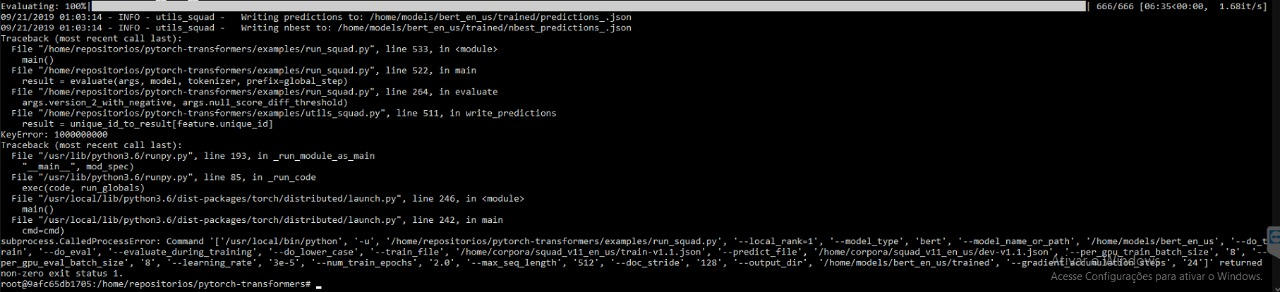
Had the same problema: a KeyError 1000000 after doing a distributed training. Does anyone know how to fix it?<|||||>@ayrtondenner Have the same problem as you when distributed training, after evaluation completes and in writing predictions:
_" File "/media/dn/dssd/nlp/transformers/examples/utils_squad.py", line 511, in write_predictions
result = unique_id_to_result[feature.unique_id]
KeyError: 1000000000"_
Setup: transformers 2.0.0; pytorch 1.2.0; python 3.7.4; NVIDIA 1080Ti x 2
_" python -m torch.distributed.launch --nproc_per_node=2 ./run_squad.py \ "_
Data parallel with the otherwise same shell script works fine producing the results below, but of course takes longer with more limited GPU memory for batch sizes.
Results:
{
"exact": 81.06906338694418,
"f1": 88.57343698391432,
"total": 10570,
"HasAns_exact": 81.06906338694418,
"HasAns_f1": 88.57343698391432,
"HasAns_total": 10570
}
Data parallel shell script:
python ./run_squad.py \
--model_type bert \
--model_name_or_path bert-base-uncased \
--do_train \
--do_eval \
--do_lower_case \
--train_file=${SQUAD_DIR}/train-v1.1.json \
--predict_file=${SQUAD_DIR}/dev-v1.1.json \
--per_gpu_eval_batch_size=8 \
--per_gpu_train_batch_size=8 \
--gradient_accumulation_steps=1 \
--learning_rate=3e-5 \
--num_train_epochs=2 \
--max_seq_length=384 \
--doc_stride=128 \
--adam_epsilon=1e-6 \
--save_steps=2000 \
--output_dir=./runs/bert_base_squad1_ft_2<|||||>The problem is that `evaluate()` distributes the evaluation under DDP:
https://github.com/huggingface/transformers/blob/079bfb32fba4f2b39d344ca7af88d79a3ff27c7c/examples/run_squad.py#L216
Meaning each process collects a subset of `all_results`
but then `write_predictions()` expects `all_results` to have *all the results* 😮
Specifically, `unique_id_to_result` only maps a subset of ids
https://github.com/huggingface/transformers/blob/079bfb32fba4f2b39d344ca7af88d79a3ff27c7c/examples/utils_squad.py#L489-L491
but the code expects an entry for every feature
https://github.com/huggingface/transformers/blob/079bfb32fba4f2b39d344ca7af88d79a3ff27c7c/examples/utils_squad.py#L510-L511
For DDP evaluate to work `all_results` needs to be collected from all the threads. Otherwise don't allow `args.do_eval` and `args.local_rank != -1` at the same time.
edit: or get rid of the `DistributedSampler` and use `SequentialSampler` in all cases. <|||||>Make sense, do you have a fix in mind @immawatson? Happy to welcome a PR that would fix that.<|||||>This issue has been automatically marked as stale because it has not had recent activity. It will be closed if no further activity occurs. Thank you for your contributions.
<|||||>> The problem is that `evaluate()` distributes the evaluation under DDP:
> https://github.com/huggingface/transformers/blob/079bfb32fba4f2b39d344ca7af88d79a3ff27c7c/examples/run_squad.py#L216
>
>
> Meaning each process collects a subset of `all_results`
> but then `write_predictions()` expects `all_results` to have _all the results_ 😮
> Specifically, `unique_id_to_result` only maps a subset of ids
> https://github.com/huggingface/transformers/blob/079bfb32fba4f2b39d344ca7af88d79a3ff27c7c/examples/utils_squad.py#L489-L491
>
>
> but the code expects an entry for every feature
> https://github.com/huggingface/transformers/blob/079bfb32fba4f2b39d344ca7af88d79a3ff27c7c/examples/utils_squad.py#L510-L511
>
> For DDP evaluate to work `all_results` needs to be collected from all the threads. Otherwise don't allow `args.do_eval` and `args.local_rank != -1` at the same time.
> edit: or get rid of the `DistributedSampler` and use `SequentialSampler` in all cases.
That didn't work for me. |
transformers | 939 | closed | Chinese BERT broken | There are still some bug after #860
The same issue is also mention in #903
I'm running on Chinese-Style SQuAD dataset (DRCD).
I can train Chinese-Bert successfully about half year ago.
However, I could not train the model successfully but I can train Multi-Bert successfully.
I'm not able to find out the reasons.
@thomwolf I think there should be more test in this repo as the project is fast growing. | 08-01-2019 08:38:55 | 08-01-2019 08:38:55 | Yes you need to install from master for now. We have not yet done a new release with the fix of #860.<|||||>@thomwolf Not related to this specific issue here, but do you think it makes sense to add the following policy to the newly introduced issue templates: all bug reports should be filed against latest `master` version of PyTorch-Transformers (incl. pip install with git master url) 🤔<|||||>This issue has been automatically marked as stale because it has not had recent activity. It will be closed if no further activity occurs. Thank you for your contributions.
|
transformers | 938 | closed | Performance dramatically drops down after replacing pytorch-pretrained-bert with pytorch-transformers | I am trying to run a baseline model, whose encoder is the pretrained BERT ('bert-base-uncased'). I have tried both versions of this package and found that the performance of the pytorch-transformers-BERT is much worse than pytorch-pretrained-bert-BERT, i.e. the BLeU-4 has dropped from 8. to 2.
Below is my codes, I wanna see if there is some important difference between the two versions that will lead to the drop, or it's the wrong way to call the functions in my codes that causes the bad performance.
(1) pytorch-pretrained-bert:
```python
from pytorch_pretrained_bert import BertModel
pretrained = BertModel.from_pretrained('bert-base-uncased')
enc_outputs, *_ = pretrained(src_seq, token_type_ids=src_sep, output_all_encoded_layers=True)
enc_output = enc_outputs[-1]
```
(2) pytorch-transformers
```python
from pytorch_transformers import BertModel, BertConfig
config = BertConfig.from_pretrained('bert-base-uncased')
config.output_hidden_states = True
pretrained = BertModel(config)
enc_outputs = pretrained(src_seq, token_type_ids=src_sep)
enc_output = enc_outputs[0]
enc_outputs = enc_outputs[2][1:]
``` | 08-01-2019 08:36:01 | 08-01-2019 08:36:01 | Same here. I am finetuning language models on new dataset. Once I change from `pytorch-pretrained-bert` to `pytorch-transformers`, generation quality dramatically drops. <|||||>I have the same problem. I refer to a example of Named Entity Recognition which used pytorch-pretrained-bert. I changed it to pytorch-transformers, but I got a bad F1 score. It's suppsed to be 0.78, I got 0.41. <|||||>I'm also seeing similar problems after the refactoring related to BertForMultipleChoice models (issue here: https://github.com/huggingface/pytorch-transformers/issues/931) <|||||>Similar issue here. Working on custom adaptation of BERT for STS benchmark dataset. Spearman correlation drops by about 2 points (.78 -> .76) after refactoring 0.6.1 to 1.0.0, even though all parameters are the same. If I reload my old models, I still get the old (higher) scores.
I suspect that this might be due to a different linear warmup function used in 0.6.1 (compared to 0.6.2 and 1.0.0), that returns smaller learning rates.<|||||>I think these differences originate from different modifications so it's not really possible to have all of them in one issue like here with no specific description of the setup and condition of each of you.
I've set up templates for the issues to incite people to give more information.
Please re-open separate issues with more details on each setup.
In particular, there is a template called "MIGRATION" which is specifically concerned with giving information on migration issues from pytorch-pretrained-bert.
In the meantime, I will close this issue.<|||||>@YuxiXie @dykang @teng1996 Any updates on this? |
transformers | 937 | closed | Wrong refactoring of mandatory parameters for run_squad.py | When only running evaluation on a squad dev set, it should *not* be mandatory to add a --train_file because only the --predict_file is necessary.
Current script invocation:
```
python run_squad \
--model_type bert \
--model_name_or_path xxx \
--output_dir xxx \
--train_file UNNECESSARY_BUT_MANDATORY \
--predict_file xxx \
--version_2_with_negative \
--do_eval \
--per_gpu_eval_batch_size 2
```
Desired script invocation without --train_file param:
```
python run_squad \
--model_type bert \
--model_name_or_path xxx \
--output_dir xxx \
--predict_file xxx \
--version_2_with_negative \
--do_eval \
--per_gpu_eval_batch_size 2
```
Before refactoring in 50b7e52 the behavior was correct. | 08-01-2019 08:11:17 | 08-01-2019 08:11:17 | indeed, we could remove this<|||||>This issue has been automatically marked as stale because it has not had recent activity. It will be closed if no further activity occurs. Thank you for your contributions.
|
transformers | 936 | closed | XLNet large low accuracy | I was running run_glue.py on one of my classification problems. Using XLNet-base-cased, everything seems to be fine, the classification accuracy converge to around 92%. But using XLNet-large, the accuracy is 89% at the first checkpoint and then drop into 24.85% at the second checkpoint. The data should be ok because I have been running many different algorithms on it.
Some of the logs as below. What could be the possible cause?
```
07/31/2019 05:35:28 - INFO - __main__ - Saving features into cached file /data/_working/sentiment/large/cached_dev_xlnet-large-cased_128_sentimentall
07/31/2019 05:35:29 - INFO - __main__ - ***** Running evaluation *****
07/31/2019 05:35:29 - INFO - __main__ - Num examples = 10000
07/31/2019 05:35:29 - INFO - __main__ - Batch size = 8
07/31/2019 05:38:49 - INFO - __main__ - ***** Eval results *****
07/31/2019 05:38:49 - INFO - __main__ - acc = 0.8902
07/31/2019 05:38:55 - INFO - __main__ - Saving model checkpoint to /data/_working/sentiment/large/output/checkpoint-500000/1250 [03:19<00:00, 6.16it/s]
07/31/2019 13:56:16 - INFO - __main__ - Loading features from cached file /data/_working/sentiment/large/cached_dev_xlnet-large-cased_128_sentimentalls]
07/31/2019 13:56:16 - INFO - __main__ - ***** Running evaluation *****
07/31/2019 13:56:16 - INFO - __main__ - Num examples = 10000
07/31/2019 13:56:16 - INFO - __main__ - Batch size = 8
07/31/2019 13:59:39 - INFO - __main__ - ***** Eval results *****
07/31/2019 13:59:39 - INFO - __main__ - acc = 0.2485
07/31/2019 13:59:44 - INFO - __main__ - Saving model checkpoint to /data/_working/sentiment/large/output/checkpoint-100000/1250 [03:22<00:00, 6.16it/s]
Iteration: 72%|██████████████████████████████████████████████████████████████████▉ | 142278/197500 [23:46:00<9:18:52, 1.65it/s]07/31/2019 22:22:27 - INFO - __main__ - Loading features from cached file /data/_working/sentiment/large/cached_dev_xlnet-large-cased_128_sentimentalls]
07/31/2019 22:22:27 - INFO - __main__ - ***** Running evaluation *****
07/31/2019 22:22:27 - INFO - __main__ - Num examples = 10000
07/31/2019 22:22:27 - INFO - __main__ - Batch size = 8
07/31/2019 22:25:49 - INFO - __main__ - ***** Eval results *****
07/31/2019 22:25:49 - INFO - __main__ - acc = 0.2485
07/31/2019 22:25:55 - INFO - __main__ - Saving model checkpoint to /data/_working/sentiment/large/output/checkpoint-150000/1250 [03:21<00:00, 6.00it/s]
``` | 08-01-2019 05:48:23 | 08-01-2019 05:48:23 | The first thought could be that the learning rate is too high and you overfit.
You probably should try changing the batch size too.
You can have a look at #795 where we discussed similar questions for SST-2. <|||||>This issue has been automatically marked as stale because it has not had recent activity. It will be closed if no further activity occurs. Thank you for your contributions.
|
transformers | 935 | closed | run_glue : Evaluating in every grad_accumulation_step if flag eval during training is true | https://github.com/huggingface/pytorch-transformers/blob/f2a3eb987e1fc2c85320fc3849c67811f5736b50/examples/run_glue.py#L154 | 08-01-2019 05:35:10 | 08-01-2019 05:35:10 | ```
if (step + 1) % args.gradient_accumulation_steps == 0:
scheduler.step() # Update learning rate schedule
optimizer.step()
model.zero_grad()
global_step += 1
if args.local_rank in [-1, 0] and args.logging_steps > 0 and global_step % args.logging_steps == 0:
# Log metrics
if args.local_rank == -1 and args.evaluate_during_training: # Only evaluate when single GPU otherwise metrics may not average well
results = evaluate(args, model, tokenizer)
for key, value in results.items():
tb_writer.add_scalar('eval_{}'.format(key), value, global_step)
```
<|||||>Found the fix. |
transformers | 934 | closed | Feature Request : run_swag with XLNet and XLM | It would be great if the run_swag script too was updated with XLNet and XLM models. They should be similar to BertForMultipleChoice ? | 08-01-2019 02:42:08 | 08-01-2019 02:42:08 | Yes, don't have bandwith for that in the short term. If you want to give it a go feel free.
Closing this issue in favor of the previous one #931 |
transformers | 933 | closed | link to `swift-coreml-transformers` | 08-01-2019 01:10:02 | 08-01-2019 01:10:02 | # [Codecov](https://codecov.io/gh/huggingface/pytorch-transformers/pull/933?src=pr&el=h1) Report
> Merging [#933](https://codecov.io/gh/huggingface/pytorch-transformers/pull/933?src=pr&el=desc) into [master](https://codecov.io/gh/huggingface/pytorch-transformers/commit/f2a3eb987e1fc2c85320fc3849c67811f5736b50?src=pr&el=desc) will **not change** coverage.
> The diff coverage is `n/a`.
[](https://codecov.io/gh/huggingface/pytorch-transformers/pull/933?src=pr&el=tree)
```diff
@@ Coverage Diff @@
## master #933 +/- ##
=======================================
Coverage 79.04% 79.04%
=======================================
Files 34 34
Lines 6242 6242
=======================================
Hits 4934 4934
Misses 1308 1308
```
------
[Continue to review full report at Codecov](https://codecov.io/gh/huggingface/pytorch-transformers/pull/933?src=pr&el=continue).
> **Legend** - [Click here to learn more](https://docs.codecov.io/docs/codecov-delta)
> `Δ = absolute <relative> (impact)`, `ø = not affected`, `? = missing data`
> Powered by [Codecov](https://codecov.io/gh/huggingface/pytorch-transformers/pull/933?src=pr&el=footer). Last update [f2a3eb9...200da37](https://codecov.io/gh/huggingface/pytorch-transformers/pull/933?src=pr&el=lastupdated). Read the [comment docs](https://docs.codecov.io/docs/pull-request-comments).
|
|
transformers | 932 | closed | pip install error: "regex_3/_regex.c:48:10: fatal error: Python.h: No such file or directory" | When pip installing pytorch-pretrained-bert on ubuntu and getting the following error:
```
regex_3/_regex.c:48:10: fatal error: Python.h: No such file or directory
#include "Python.h"
^~~~~~~~~~
compilation terminated.
error: command 'x86_64-linux-gnu-gcc' failed with exit status 1
----------------------------------------
ERROR: Command errored out with exit status 1: /home/.../env/bin/python3.7 -u -c 'import sys, setuptools, tokenize; sys.argv[0] = '"'"'/tmp/pip-install-pogvvuk9/regex/setup.py'"'"'; __file__='"'"'/tmp/pip-install-pogvvuk9/regex/setup.py'"'"';f=getattr(tokenize, '"'"'open'"'"', open)(__file__);code=f.read().replace('"'"'\r\n'"'"', '"'"'\n'"'"');f.close();exec(compile(code, __file__, '"'"'exec'"'"'))' install --record /tmp/pip-record-19j41i0r/install-record.txt --single-version-externally-managed --compile --install-headers /home/.../env/include/site/python3.7/regex Check the logs for full command output.
```
My python version is ```Python 3.7.4 (default, Jul 9 2019, 15:11:16)
[GCC 7.4.0] on linux```.
I'm able to pip install other packages without problems.
Has anyone run into a similar issue? | 08-01-2019 00:09:39 | 08-01-2019 00:09:39 | Hi @seyuboglu I think you need to install the Python Dev package on your distribution. For Ubuntu >= 18.04 this should be possible with `apt install python3.7-dev` :)<|||||>Thank you @stefan-it! That did the trick. Any idea why this is necessary to install pytorch-transformers in particular? <|||||>@stefan-it do you know how to make this happen on on amazon linux2, I have tried a bunch of things am am getting same error. I think i already installed a python3-development package with yum. but when i tried python3.7-dev it said no package located. <|||||>@antleypk Could you try to use `yum install python3-devel` instead?<|||||>Thanks @stefan-it
I solved it last week and should have updated thread.
To anyone else that may come here; this solution is for "amazon linux2"
my current setup.sh scripts looks like this:
sudo yum install python3.x86_64 -y
sudo yum install python3-devel.x86_64 -y
Since getting adding the bottom line I have been able to install every package that I tried to install.
I built the answer based on this solution:
https://stackoverflow.com/questions/43047284/how-to-install-python3-devel-on-red-hat-7
and found this solution based on this original post.
|
transformers | 931 | closed | Updating run_swag script for new pytorch_transformers setup | https://github.com/huggingface/pytorch-transformers/blob/f2a3eb987e1fc2c85320fc3849c67811f5736b50/examples/single_model_scripts/run_swag.py#L35
It appears that WEIGHTS_NAME and CONFIG_NAME have moved out of {pytorch_transformers/pytorch_pretrained_bert}.file_utils (and instead can be imported directly from pytorch_transformers), as shown below :
```
>>> import pytorch_transformers as p
>>> p.__version__
'1.0.0'
>>> from pytorch_transformers.file_utils import WEIGHTS_NAME, CONFIG_NAME
Traceback (most recent call last):
File "<stdin>", line 1, in <module>
ImportError: cannot import name 'WEIGHTS_NAME'
>>> from pytorch_transformers.file_utils import CONFIG_NAME
Traceback (most recent call last):
File "<stdin>", line 1, in <module>
ImportError: cannot import name 'CONFIG_NAME'
>>> from pytorch_transformers import WEIGHTS_NAME
>>>
```
This seems incorrect in the new run_swag script (as shown above) (if true, are there any other important imports that were overlooked here?) | 07-31-2019 22:03:44 | 07-31-2019 22:03:44 | I found a few other issues: 1) the script uses the old BertAdam (instead of AdamW)
https://github.com/huggingface/pytorch-transformers/blob/44dd941efb602433b7edc29612cbdd0a03bf14dc/examples/single_model_scripts/run_swag.py#L431
2) the train and test loops still use the old version of forward, i.e.,
https://github.com/huggingface/pytorch-transformers/blob/44dd941efb602433b7edc29612cbdd0a03bf14dc/examples/single_model_scripts/run_swag.py#L450
https://github.com/huggingface/pytorch-transformers/blob/44dd941efb602433b7edc29612cbdd0a03bf14dc/examples/single_model_scripts/run_swag.py#L525
In the former case, I added the following:
```
#loss = model(input_ids, segment_ids, input_mask, label_ids) # line 450 in train
outputs = model(input_ids, segment_ids, input_mask, label_ids)
loss = outputs[0]
```
And the latter:
```
## tmp_eval_loss = model(input_ids, segment_ids, input_mask, label_ids) ## line 525
output = model(input_ids, segment_ids, input_mask, label_ids)
tmp_eval_loss,logits = output[:2]
```
I'd be curious if the last two versions are correct. I'm actually not able to reproduce the Swag results I was getting before the refactoring (reported here: https://github.com/huggingface/pytorch-transformers/blob/v0.6.2/README.md). Rather than getting around 80%, I'm stuck at around 78%. <|||||>Yes the run_swag script hasn't been updated to the new API yet.
Do you want to give it a look and submit a PR? I don't have plan to work on it in the short-term.<|||||>This issue has been automatically marked as stale because it has not had recent activity. It will be closed if no further activity occurs. Thank you for your contributions.
|
transformers | 930 | closed | Fixing a broken link in the README.md | Fixing the `Quick tour` link. | 07-31-2019 14:18:39 | 07-31-2019 14:18:39 | Thanks Gregory :)<|||||># [Codecov](https://codecov.io/gh/huggingface/pytorch-transformers/pull/930?src=pr&el=h1) Report
> Merging [#930](https://codecov.io/gh/huggingface/pytorch-transformers/pull/930?src=pr&el=desc) into [master](https://codecov.io/gh/huggingface/pytorch-transformers/commit/6b763d04a930e070e4096fefa1bbdb50f0575d52?src=pr&el=desc) will **not change** coverage.
> The diff coverage is `n/a`.
[](https://codecov.io/gh/huggingface/pytorch-transformers/pull/930?src=pr&el=tree)
```diff
@@ Coverage Diff @@
## master #930 +/- ##
=======================================
Coverage 79.04% 79.04%
=======================================
Files 34 34
Lines 6242 6242
=======================================
Hits 4934 4934
Misses 1308 1308
```
------
[Continue to review full report at Codecov](https://codecov.io/gh/huggingface/pytorch-transformers/pull/930?src=pr&el=continue).
> **Legend** - [Click here to learn more](https://docs.codecov.io/docs/codecov-delta)
> `Δ = absolute <relative> (impact)`, `ø = not affected`, `? = missing data`
> Powered by [Codecov](https://codecov.io/gh/huggingface/pytorch-transformers/pull/930?src=pr&el=footer). Last update [6b763d0...4e8c1f6](https://codecov.io/gh/huggingface/pytorch-transformers/pull/930?src=pr&el=lastupdated). Read the [comment docs](https://docs.codecov.io/docs/pull-request-comments).
<|||||># [Codecov](https://codecov.io/gh/huggingface/pytorch-transformers/pull/930?src=pr&el=h1) Report
> Merging [#930](https://codecov.io/gh/huggingface/pytorch-transformers/pull/930?src=pr&el=desc) into [master](https://codecov.io/gh/huggingface/pytorch-transformers/commit/6b763d04a930e070e4096fefa1bbdb50f0575d52?src=pr&el=desc) will **not change** coverage.
> The diff coverage is `n/a`.
[](https://codecov.io/gh/huggingface/pytorch-transformers/pull/930?src=pr&el=tree)
```diff
@@ Coverage Diff @@
## master #930 +/- ##
=======================================
Coverage 79.04% 79.04%
=======================================
Files 34 34
Lines 6242 6242
=======================================
Hits 4934 4934
Misses 1308 1308
```
------
[Continue to review full report at Codecov](https://codecov.io/gh/huggingface/pytorch-transformers/pull/930?src=pr&el=continue).
> **Legend** - [Click here to learn more](https://docs.codecov.io/docs/codecov-delta)
> `Δ = absolute <relative> (impact)`, `ø = not affected`, `? = missing data`
> Powered by [Codecov](https://codecov.io/gh/huggingface/pytorch-transformers/pull/930?src=pr&el=footer). Last update [6b763d0...4e8c1f6](https://codecov.io/gh/huggingface/pytorch-transformers/pull/930?src=pr&el=lastupdated). Read the [comment docs](https://docs.codecov.io/docs/pull-request-comments).
|
transformers | 929 | closed | AttributeError: 'NoneType' object has no attribute 'split' | ---------------------------------------------------------------------------
AttributeError Traceback (most recent call last)
<ipython-input-1-4393ada473d4> in <module>
1 import torch
----> 2 from pytorch_transformers import *
~/anaconda3/envs/python/lib/python3.6/site-packages/pytorch_transformers/__init__.py in <module>
8 from .tokenization_utils import (PreTrainedTokenizer, clean_up_tokenization)
9
---> 10 from .modeling_bert import (BertConfig, BertModel, BertForPreTraining,
11 BertForMaskedLM, BertForNextSentencePrediction,
12 BertForSequenceClassification, BertForMultipleChoice,
~/anaconda3/envs/python/lib/python3.6/site-packages/pytorch_transformers/modeling_bert.py in <module>
222
223 try:
--> 224 from apex.normalization.fused_layer_norm import FusedLayerNorm as BertLayerNorm
225 except ImportError:
226 logger.info("Better speed can be achieved with apex installed from https://www.github.com/nvidia/apex .")
~/anaconda3/envs/python/lib/python3.6/importlib/_bootstrap.py in _find_and_load(name, import_)
~/anaconda3/envs/python/lib/python3.6/importlib/_bootstrap.py in _find_and_load_unlocked(name, import_)
~/anaconda3/envs/python/lib/python3.6/importlib/_bootstrap.py in _load_unlocked(spec)
~/anaconda3/envs/python/lib/python3.6/importlib/_bootstrap.py in _load_backward_compatible(spec)
~/anaconda3/envs/python/lib/python3.6/site-packages/apex-0.1-py3.6.egg/apex/__init__.py in <module>
1 from . import parallel
----> 2 from . import amp
3 from . import fp16_utils
4
5 # For optimizers and normalization there is no Python fallback.
~/anaconda3/envs/python/lib/python3.6/importlib/_bootstrap.py in _find_and_load(name, import_)
~/anaconda3/envs/python/lib/python3.6/importlib/_bootstrap.py in _find_and_load_unlocked(name, import_)
~/anaconda3/envs/python/lib/python3.6/importlib/_bootstrap.py in _load_unlocked(spec)
~/anaconda3/envs/python/lib/python3.6/importlib/_bootstrap.py in _load_backward_compatible(spec)
~/anaconda3/envs/python/lib/python3.6/site-packages/apex-0.1-py3.6.egg/apex/amp/__init__.py in <module>
----> 1 from .amp import init, half_function, float_function, promote_function,\
2 register_half_function, register_float_function, register_promote_function
3 from .handle import scale_loss, disable_casts
4 from .frontend import initialize
5 from ._amp_state import master_params, _amp_state
~/anaconda3/envs/python/lib/python3.6/importlib/_bootstrap.py in _find_and_load(name, import_)
~/anaconda3/envs/python/lib/python3.6/importlib/_bootstrap.py in _find_and_load_unlocked(name, import_)
~/anaconda3/envs/python/lib/python3.6/importlib/_bootstrap.py in _load_unlocked(spec)
~/anaconda3/envs/python/lib/python3.6/importlib/_bootstrap.py in _load_backward_compatible(spec)
~/anaconda3/envs/python/lib/python3.6/site-packages/apex-0.1-py3.6.egg/apex/amp/amp.py in <module>
1 from . import compat, rnn_compat, utils, wrap
2 from .handle import AmpHandle, NoOpHandle
----> 3 from .lists import functional_overrides, torch_overrides, tensor_overrides
4 from ._amp_state import _amp_state
5 from .frontend import *
~/anaconda3/envs/python/lib/python3.6/importlib/_bootstrap.py in _find_and_load(name, import_)
~/anaconda3/envs/python/lib/python3.6/importlib/_bootstrap.py in _find_and_load_unlocked(name, import_)
~/anaconda3/envs/python/lib/python3.6/importlib/_bootstrap.py in _load_unlocked(spec)
~/anaconda3/envs/python/lib/python3.6/importlib/_bootstrap.py in _load_backward_compatible(spec)
~/anaconda3/envs/python/lib/python3.6/site-packages/apex-0.1-py3.6.egg/apex/amp/lists/torch_overrides.py in <module>
67 'baddbmm',
68 'bmm']
---> 69 if utils.get_cuda_version() >= (9, 1, 0):
70 FP16_FUNCS.extend(_bmms)
71 else:
~/anaconda3/envs/python/lib/python3.6/site-packages/apex-0.1-py3.6.egg/apex/amp/utils.py in get_cuda_version()
7
8 def get_cuda_version():
----> 9 return tuple(int(x) for x in torch.version.cuda.split('.'))
10
11 def is_fp_tensor(x):
AttributeError: 'NoneType' object has no attribute 'split' | 07-31-2019 09:42:09 | 07-31-2019 09:42:09 | conda 4.5.12
Python 3.6.8 :: Anaconda, Inc.
torch 1.1.0<|||||>Is it possible you installed the CPU-only version of PyTorch? Which command did you use to install it? Did you do it via conda or pip?<|||||>Seems like a problem related to apex, you should open an issue on NVIDIA's repo.
I'm closing this one for now. |
transformers | 928 | closed | ERNIE 2.0 ? | Latest NLP Language Model.:)
[ERNIE 2.0](https://arxiv.org/pdf/1907.12412.pdf?source=post_page) | 07-31-2019 04:25:52 | 07-31-2019 04:25:52 | This is relevant.
https://medium.com/syncedreview/baidus-ernie-2-0-beats-bert-and-xlnet-on-nlp-benchmarks-51a8c21aa433<|||||>We don't have any plan to add ERNIE in the short-term but if someone wants to do a (clean) PR with this model, happy to have a look and add it to the library.<|||||>This issue has been automatically marked as stale because it has not had recent activity. It will be closed if no further activity occurs. Thank you for your contributions.
|
transformers | 927 | closed | `do_wordpiece_only` argument | A `do_wordpiece_only` argument is referenced [here](https://github.com/huggingface/pytorch-transformers/blob/fec76a481d1ecfbf068d87735dd44ffc26158f6e/pytorch_transformers/tokenization_bert.py#L97) -- does that argument actually exist? I'm not able to find it in the repo anywhere.
Related, is this expected behavior?
```python
>>> tokenizer = BertTokenizer.from_pretrained('bert-base-uncased', never_split=['[unused0]'])
>>> tokenizer.tokenize('[CLS] [unused0] this is a [SEP] test')
['[', 'cl', '##s', ']', '[unused0]', 'this', 'is', 'a', '[', 'sep', ']', 'test']
>>>
>>> tokenizer = BertTokenizer.from_pretrained('bert-base-uncased')
>>> tokenizer.tokenize('[CLS] [unused0] this is a [SEP] test')
['[CLS]', '[', 'unused', '##0', ']', 'this', 'is', 'a', '[SEP]', 'test']
```
I want to be able to use the `[unused*]` tokens in `BertTokenizer`, but it seems like adding them to the `never_split` has some unexpected side effects. Anyone have any ideas on how to set up the tokenizer to use the `[unused*]` tokens? I'd prefer not to have to add the indices in a seperate step after the tokenization if possible.
__Edit:__ Seems like maybe you have to do
```
tokenizer = BertTokenizer.from_pretrained('bert-base-uncased', never_split=['[UNK]', '[SEP]', '[PAD]', '[CLS]', '[MASK]', '[unused0]'])
```
and then behavior makes more sense -- is that right?
Thanks! | 07-31-2019 00:08:19 | 07-31-2019 00:08:19 | Use _additional_special_tokens_ instead.
```
>>> tokenizer = BertTokenizer.from_pretrained('bert-base-uncased', additional_special_tokens=['[unused0]'])
>>> tokenizer.tokenize('[CLS] [unused0] this is a [SEP] test')
['[CLS]', '[unused0]', 'this', 'is', 'a', '[SEP]', 'test']
```
<|||||>This issue has been automatically marked as stale because it has not had recent activity. It will be closed if no further activity occurs. Thank you for your contributions.
|
transformers | 926 | closed | Feature request: roBERTa | Hi, thanks for making a unified framework for all transformer-based models. Just out of curiosity, do you plan to add the roBERTa pre-trained models? Although FairSeq has provided the model, I still prefer using your framework. Thanks again, big fan. 🤗🤗🤗 | 07-30-2019 16:45:02 | 07-30-2019 16:45:02 | See #829 (and thanks for the kind words!) |
transformers | 925 | closed | Torchscipt mode for BertForPreTraining | Hello, i used code from this tutorial https://huggingface.co/pytorch-transformers/torchscript.html
pytorch-transformers==1.0.0
```
from pytorch_pretrained_bert import BertModel, BertTokenizer, BertConfig
import torch
enc = BertTokenizer.from_pretrained("bert-base-uncased")
# Tokenizing input text
text = "[CLS] Who was Jim Henson ? [SEP] Jim Henson was a puppeteer [SEP]"
tokenized_text = enc.tokenize(text)
# Masking one of the input tokens
masked_index = 8
tokenized_text[masked_index] = '[MASK]'
indexed_tokens = enc.convert_tokens_to_ids(tokenized_text)
segments_ids = [0, 0, 0, 0, 0, 0, 0, 1, 1, 1, 1, 1, 1, 1]
# Creating a dummy input
tokens_tensor = torch.tensor([indexed_tokens])
segments_tensors = torch.tensor([segments_ids])
dummy_input = [tokens_tensor, segments_tensors]
# Initializing the model with the torchscript flag
# Flag set to True even though it is not necessary as this model does not have an LM Head.
config = BertConfig(vocab_size_or_config_json_file=32000, hidden_size=768,
num_hidden_layers=12, num_attention_heads=12, intermediate_size=3072, torchscript=True)
# Instantiating the model
model = BertModel(config)
# The model needs to be in evaluation mode
model.eval()
# Creating the trace
traced_model = torch.jit.trace(model, [tokens_tensor, segments_tensors])
torch.jit.save(traced_model, "traced_bert.pt")
```
And then I get an error:
```
---------------------------------------------------------------------------
TypeError Traceback (most recent call last)
<ipython-input-10-d850721ce4cc> in <module>
22 # Flag set to True even though it is not necessary as this model does not have an LM Head.
23 config = BertConfig(vocab_size_or_config_json_file=32000, hidden_size=768,
---> 24 num_hidden_layers=12, num_attention_heads=12, intermediate_size=3072, torchscript=True)
25
26 # Instantiating the model
TypeError: __init__() got an unexpected keyword argument 'torchscript'
```
Then I looked at source code and did not find torchscript argument in constructor. Help me please? | 07-30-2019 14:29:39 | 07-30-2019 14:29:39 | I realised what was the problem. There is an error in documentation.
```from pytorch_pretrained_bert import BertModel, BertTokenizer, BertConfig```
should be
```from pytorch_transformers import BertModel, BertTokenizer, BertConfig```
And also [there](https://github.com/huggingface/pytorch-transformers/blob/master/docs/source/torchscript.rst) is fixed version, but webview documentation looks outdated.<|||||>Indeed. We will update the web documentation, thanks for the report |
transformers | 924 | closed | [RuntimeError: sizes must be non-negative] in run_squad.py using xlnet large model | [RuntimeError: sizes must be non-negative]
run_squad.py in main
global_step, tr_loss = train(args, train_dataset, model, tokenizer)
run_squad.py in train
outputs = model(**inputs)
modeling_xlnet.py
mems_mask = torch.zeros([data_mask.shape[0], mlen, bsz]).to(data_mask), in which
mlen = 0 resulting from "mems = None". | 07-30-2019 11:09:44 | 07-30-2019 11:09:44 | I have encountered a similar problem:
Just copy the code
`
import torch
#from pytorch_pretrained_bert import BertTokenizer, BertModel, BertForMaskedLM
from pytorch_transformers import XLNetLMHeadModel, XLNetTokenizer,XLNetConfig
import numpy as np
import math
config = XLNetConfig.from_pretrained('xlnet-large-cased')
tokenizer = XLNetTokenizer.from_pretrained('xlnet-large-cased')
model = XLNetLMHeadModel(config)
We show how to setup inputs to predict a next token using a bi-directional context.
input_ids = torch.tensor(tokenizer.encode("Hello, my dog is very ")).unsqueeze(0) # We will predict the masked token
perm_mask = torch.zeros((1, input_ids.shape[1], input_ids.shape[1]), dtype=torch.float)
perm_mask[:, :, -1] = 1.0 # Previous tokens don't see last token
target_mapping = torch.zeros((1, 1, input_ids.shape[1]), dtype=torch.float) # Shape [1, 1, seq_length] => let's predict one token
target_mapping[0, 0, -1] = 1.0 # Our first (and only) prediction will be the last token of the sequence (the masked token)
outputs = model(input_ids, perm_mask=perm_mask, target_mapping=target_mapping)
next_token_logits = outputs[0] # Output has shape [target_mapping.size(0), target_mapping.size(1), config.vocab_size]
print(next_token_logits)
`
results the same Runtime Error<|||||>@Nealcly the code you posted runs without any errors for me. @ShuGao0810 Can you both post the full stack trace?<|||||>> @Nealcly the code you posted runs without any errors for me. @ShuGao0810 Can you both post the full stack trace?
Traceback (most recent call last):
File "run_squad.py", line 527, in <module>
main()
File "run_squad.py", line 473, in main
global_step, tr_loss = train(args, train_dataset, model, tokenizer)
File "run_squad.py", line 142, in train
outputs = model(**inputs)
File "/data/anaconda3/lib/python3.6/site-packages/torch/nn/modules/module.py", line 477, in__call__
result = self.forward(*input, **kwargs)
File "/data/anaconda3/lib/python3.6/site-packages/torch/nn/parallel/data_parallel.py", line 123, in forward
outputs = self.parallel_apply(replicas, inputs, kwargs)
File "/data/anaconda3/lib/python3.6/site-packages/torch/nn/parallel/data_parallel.py", line 133, in parallel_apply
return parallel_apply(replicas, inputs, kwargs, self.device_ids[:len(replicas)])
File "/data/anaconda3/lib/python3.6/site-packages/torch/nn/parallel/parallel_apply.py", line 77, in parallel_apply
raise output
File "/data/anaconda3/lib/python3.6/site-packages/torch/nn/parallel/parallel_apply.py", line 53, in _worker
output = module(*input, **kwargs)
File "/data/anaconda3/lib/python3.6/site-packages/torch/nn/modules/module.py", line 477, in__call__
result = self.forward(*input, **kwargs)
File "/data/gaoshu562/SQuAD_v2.0/pytorch_version/xlnet_large/pytorch-transformers-master/pytorch_transformers/modeling_xlnet.py", line 1242, in forward
head_mask=head_mask)
File "/data/anaconda3/lib/python3.6/site-packages/torch/nn/modules/module.py", line 477, in__call__
result = self.forward(*input, **kwargs)
File "/data/gaoshu562/SQuAD_v2.0/pytorch_version/xlnet_large/pytorch-transformers-master/pytorch_transformers/modeling_xlnet.py", line 900, in forward
mems_mask = torch.zeros([data_mask.shape[0], mlen, bsz]).to(data_mask)
RuntimeError: sizes must be non-negative
<|||||>I also run the glue.sh
To be clear: I use Pytorch 0.4.1 Python 3.6.2
It yields the same error:
traceback (most recent call last): | 0/360 [00:00<?, ?it/s]
File "./examples/run_glue.py", line 478, in <module>
main()
File "./examples/run_glue.py", line 432, in main
global_step, tr_loss = train(args, train_dataset, model, tokenizer)
File "./examples/run_glue.py", line 129, in train
outputs = model(**inputs)
File "/home/neal/anaconda3/envs/allennlp/lib/python3.6/site-packages/torch/nn/modules/module.py", line 477, in __call__
result = self.forward(*input, **kwargs)
File "/home/neal/anaconda3/envs/allennlp/lib/python3.6/site-packages/torch/nn/parallel/data_parallel.py", line 123, in forward
outputs = self.parallel_apply(replicas, inputs, kwargs)
File "/home/neal/anaconda3/envs/allennlp/lib/python3.6/site-packages/torch/nn/parallel/data_parallel.py", line 133, in parallel_apply
return parallel_apply(replicas, inputs, kwargs, self.device_ids[:len(replicas)])
File "/home/neal/anaconda3/envs/allennlp/lib/python3.6/site-packages/torch/nn/parallel/parallel_apply.py", line 77, in parallel_apply
raise output
File "/home/neal/anaconda3/envs/allennlp/lib/python3.6/site-packages/torch/nn/parallel/parallel_apply.py", line 53, in _worker
output = module(*input, **kwargs)
File "/home/neal/anaconda3/envs/allennlp/lib/python3.6/site-packages/torch/nn/modules/module.py", line 477, in __call__
result = self.forward(*input, **kwargs)
File "/home/neal/anaconda3/envs/allennlp/lib/python3.6/site-packages/pytorch_transformers/modeling_xlnet.py", line 1129, in forward
head_mask=head_mask)
File "/home/neal/anaconda3/envs/allennlp/lib/python3.6/site-packages/torch/nn/modules/module.py", line 477, in __call__
result = self.forward(*input, **kwargs)
File "/home/neal/anaconda3/envs/allennlp/lib/python3.6/site-packages/pytorch_transformers/modeling_xlnet.py", line 891, in forward
mems_mask = torch.zeros([data_mask.shape[0], mlen, bsz]).to(data_mask)
**RuntimeError: sizes must be non-negative**<|||||>If it’s not too much trouble, try cloning your conda env and replacing your torch version with the latest (1.1.0?), and then running again. <|||||>Yes, I gave a deeper look and we are definitely not compatible anymore with PyTorch 0.4.1 at this point.
Maintaining compatibility would be too difficult and not really worth it so I'll update the readme to remove PyTorch 0.4.1 and indicate we start at PyTorch 1.0.0 from now on. |
transformers | 923 | closed | Don't save model without training (example/run_squad.py bug) | There is a mirror bug in run_squad.py.
The model should not be saved if only do_predict. | 07-30-2019 10:40:43 | 07-30-2019 10:40:43 | # [Codecov](https://codecov.io/gh/huggingface/pytorch-transformers/pull/923?src=pr&el=h1) Report
> Merging [#923](https://codecov.io/gh/huggingface/pytorch-transformers/pull/923?src=pr&el=desc) into [master](https://codecov.io/gh/huggingface/pytorch-transformers/commit/a7b4cfe9194bf93c7044a42c9f1281260ce6279e?src=pr&el=desc) will **decrease** coverage by `0.03%`.
> The diff coverage is `n/a`.
[](https://codecov.io/gh/huggingface/pytorch-transformers/pull/923?src=pr&el=tree)
```diff
@@ Coverage Diff @@
## master #923 +/- ##
==========================================
- Coverage 79.22% 79.19% -0.04%
==========================================
Files 38 38
Lines 6406 6406
==========================================
- Hits 5075 5073 -2
- Misses 1331 1333 +2
```
| [Impacted Files](https://codecov.io/gh/huggingface/pytorch-transformers/pull/923?src=pr&el=tree) | Coverage Δ | |
|---|---|---|
| [pytorch\_transformers/tokenization\_bert.py](https://codecov.io/gh/huggingface/pytorch-transformers/pull/923/diff?src=pr&el=tree#diff-cHl0b3JjaF90cmFuc2Zvcm1lcnMvdG9rZW5pemF0aW9uX2JlcnQucHk=) | `94.17% <0%> (-0.98%)` | :arrow_down: |
------
[Continue to review full report at Codecov](https://codecov.io/gh/huggingface/pytorch-transformers/pull/923?src=pr&el=continue).
> **Legend** - [Click here to learn more](https://docs.codecov.io/docs/codecov-delta)
> `Δ = absolute <relative> (impact)`, `ø = not affected`, `? = missing data`
> Powered by [Codecov](https://codecov.io/gh/huggingface/pytorch-transformers/pull/923?src=pr&el=footer). Last update [a7b4cfe...40aa709](https://codecov.io/gh/huggingface/pytorch-transformers/pull/923?src=pr&el=lastupdated). Read the [comment docs](https://docs.codecov.io/docs/pull-request-comments).
<|||||>Ok, could you fix that also in the `run_glue` example?<|||||>@thomwolf ```run_glue``` is correct.<|||||>@thomwolf I just solved conflicts<|||||>This looks good to me, thanks! |
transformers | 922 | closed | TypeError: 'NoneType' object is not callable | ---------------------------------------------------------------------------
TypeError Traceback (most recent call last)
<ipython-input-7-d477193005ba> in <module>
12 output_attentions=True)
13 input_ids = torch.tensor([tokenizer.encode("Let's see all hidden-states and attentions on this text")])
---> 14 all_hidden_states, all_attentions = model(input_ids)[-2:]
TypeError: 'NoneType' object is not callable | 07-30-2019 07:48:06 | 07-30-2019 07:48:06 | What is the code you are running to get this error?<|||||>I assume it is when trying to run the "quick tour" from the readme. I'm getting the same error and found a similar issue in #712 where the feedback was "usually, this comes from the library not being able to reach AWS S3 servers to download the pretrained weights". However, I also tried running it on Google Colab (with 1Gbit connection) with the same result.
```
ERROR:pytorch_transformers.modeling_utils:Model name 'xlm-mlm-enfr-1024' was not found in model name list (bert-base-uncased, bert-large-uncased, bert-base-cased, bert-large-cased, bert-base-multilingual-uncased, bert-base-multilingual-cased, bert-base-chinese, bert-base-german-cased, bert-large-uncased-whole-word-masking, bert-large-cased-whole-word-masking, bert-large-uncased-whole-word-masking-finetuned-squad, bert-large-cased-whole-word-masking-finetuned-squad, bert-base-cased-finetuned-mrpc). We assumed 'xlm-mlm-enfr-1024' was a path or url but couldn't find any file associated to this path or url.
ERROR:pytorch_transformers.modeling_utils:Model name 'xlm-mlm-enfr-1024' was not found in model name list (bert-base-uncased, bert-large-uncased, bert-base-cased, bert-large-cased, bert-base-multilingual-uncased, bert-base-multilingual-cased, bert-base-chinese, bert-base-german-cased, bert-large-uncased-whole-word-masking, bert-large-cased-whole-word-masking, bert-large-uncased-whole-word-masking-finetuned-squad, bert-large-cased-whole-word-masking-finetuned-squad, bert-base-cased-finetuned-mrpc). We assumed 'xlm-mlm-enfr-1024' was a path or url but couldn't find any file associated to this path or url.
---------------------------------------------------------------------------
TypeError Traceback (most recent call last)
<ipython-input-11-3ed6cc19ece0> in <module>()
41 output_attentions=True)
42 input_ids = torch.tensor([tokenizer.encode("Let's see all hidden-states and attentions on this text")])
---> 43 all_hidden_states, all_attentions = model(input_ids)[-2:]
44
45 # Models are compatible with Torchscript
TypeError: 'NoneType' object is not callable
````<|||||>Yes [bas020](https://github.com/bsa020) I have same error. I ran the same "quick tour".<|||||>@bsa020 I got the same issue in both Google Colab and my PC. Any idea how to solve it?<|||||>As I understand, in the loop
`for model_class, tokenizer_class, pretrained_weights in MODELS:
# Load pretrained model/tokenizer
tokenizer = tokenizer_class.from_pretrained(pretrained_weights)
model = model_class.from_pretrained(pretrained_weights)
# Encode text
input_ids = torch.tensor([tokenizer.encode("Here is some text to encode")])
with torch.no_grad():
last_hidden_states = model(input_ids)[0] # Models outputs are now tuples`
the value of variable `pretrained_weights` is 'xlm-mlm-enfr-1024', not 'bert-base-uncased'. That's why we got error when running
`model = model_class.from_pretrained('bert-base-uncased',
output_hidden_states=True,
output_attentions=True)
input_ids = torch.tensor([tokenizer.encode("Let's see all hidden-states and attentions on this text")])
all_hidden_states, all_attentions = model(input_ids)[-2:]`<|||||>I got the same issue. How to fix the problem??<|||||>@Susan19900316 just change `pretrained_weights` into `bert-base-uncased`<|||||>Done Let me try. Thanks all for help.<|||||>I'm not sure if this issue should be closed before the readme is updated? @rodgzilla @sw-ot-ashishpatel <|||||>This issue has been automatically marked as stale because it has not had recent activity. It will be closed if no further activity occurs. Thank you for your contributions.
|
transformers | 921 | closed | Issues in visualizing a fine tuned model | BertModel finetuned for a sequence classification task does not give expected results on visualisation.
Ideally, the pretrained model should be loaded into BertForSequenceClassification, but that model does not return attentions scores for visualisation.
When loaded into BertModel (0 to 11 layers), I assume the 11th layer (right before classification layer in BertForSequenceClassification) is the right layer to check attention distribution.
But every word is attentive to every other word.
I am wondering what can be the possible reasons and how I can fix it.
Thanks.
<img width="753" alt="Screenshot 2019-07-30 at 11 19 46 AM" src="https://user-images.githubusercontent.com/25073753/62104050-08a2e600-b2bc-11e9-889a-88a6c0c9e2ea.png">
| 07-30-2019 05:48:15 | 07-30-2019 05:48:15 | "But every word is attentive to every other word." --> I don't think that's an error, that's the general way how attention mechanism works. But definitely weights of these attentions to a particular word would vary and based on these weighted attentions and other contextual info. the downstream tasks (entailment, prediction, classification etc.) would be performed. I haven't worked on attention viz yet, but I think checking [BertViz](https://github.com/jessevig/bertviz) repo. or posting your issue over there would be more fruitful. <|||||>I don't know which framework you use for visualizing attention so I can't really help but a way to make the model output attention weights is by loading it like that:
```
model = BertModel.from_pretrained("bert-base-uncased", state_dict=model_state_dict, output_attentions=True).
```
The model will then output a tuple with the last element being the full list of attentions weights (see docstring and doc of the model).<|||||>@thomwolf I see similar results with output_attentions=True.
The output predictions are correct but the attention scores are comparatively higher than in the case with pretrained model ('bert-base-uncased'). This makes me think, if the attention scores extracted are even correct or not for a fine tuned model.
I used [BertViz](https://github.com/jessevig/bertviz/tree/master/bertviz) as a tool for visualization.<|||||>Hi @chikubee , how does the visualization of lower layers look like? First of all, I don't think visualizing final layers is a good idea. I also tried BertVis, and I found that attention weights of higher layers are usually more uniformly distributed, like the screenshot you provided. Although I used it for Transformer encoder-decoder, but I think the phenomenon is similar. Would be interested to see your lower layer visualization.<|||||>This issue has been automatically marked as stale because it has not had recent activity. It will be closed if no further activity occurs. Thank you for your contributions.
|
transformers | 920 | closed | Unigram frequencies in GPT-2 or XLnet? | Question: does the GPT2 or XLnet tokenizer contain unigram frequencies? From the discussion here (https://github.com/huggingface/pytorch-transformers/issues/477), it looks like the tokenizer from TransformerXL has it, but I'm not sure if the same applies for GPT2 and XLnet. If they do contain unigram frequencies, can you point me to the objects in the GPT2/XLnet's tokenizer that have this frequency information? | 07-30-2019 03:21:39 | 07-30-2019 03:21:39 | XLnet tokenizer utilizes SentencePiece and you can use it's score for unigram as relative frequency with something like `math.exp(XLNetTokenizer.sp_model.GetScore(token_id))`.<|||||>Ah perfect. So the raw score gives the unigram log probability, and the exp of it gives the normalised frequency.
How about GPT-2? Is this information saved in the tokenizer?<|||||>As far as I see, GPT-2 tokenizer does not contain frequency information. What's more tokens in dictionary are not ordered according to frequency (so it is not possible to estimate frequency assuming Zipf's distribution), but according to length.<|||||>Many thanks for looking into this. I suppose I have no choice but to mine unigram frequencies myself... (at this point I am looking to use a similar web corpus, such as https://skylion007.github.io/OpenWebTextCorpus/ to do this; if you have any pointers please chime in).<|||||>Hello @jhlau
I was looking for a similar unigram frequency for GPT-2. Would you happen to have acquired (or created) such a list and be willing to share?<|||||>There you go: https://drive.google.com/file/d/1FhObTkvhT46Xy-Vyqi-gku8Ho2c1XvHx/view?usp=sharing
Python3 pickle file. Unigram mined based on the openwebtextcorpus linked above.<|||||>Hi @jhlau
Can you please explain how you mined the unigrams?
I looked at your file (thank you for sharing!) and it seems to me as if the keys are case-sensitive and that there was some method like SentencePieces or BPE used (I noticed keys like "ing" while very common verbs are not included). I'd like to be able to tell the frequency of a word, how should I go about it?
Thank you!<|||||>GPT-2 uses BPE, so the openwebtextcorpus is tokenised with BPE, and then unigram frequencies are collected.<|||||>> There you go: https://drive.google.com/file/d/1FhObTkvhT46Xy-Vyqi-gku8Ho2c1XvHx/view?usp=sharing
>
> Python3 pickle file. Unigram mined based on the openwebtextcorpus linked above.
Hi @jhlau, it seems the link here has expired. It will be quite helpful if you can provide it again. Many thanks!<|||||>@GeassTaiga I no longer have it anymore unfortunately =/ |
transformers | 919 | closed | Code snippet on docs page using old import | This is a documentation issue. I couldn't find where to edit the website source https://huggingface.co/pytorch-transformers/torchscript.html
On that page the code snippet still uses `from pytorch_pretrained_bert import BertModel, BertTokenizer, BertConfig`
The documentation in this repo under [https://github.com/huggingface/pytorch-transformers/blob/master/docs/source/torchscript.rst](url) is correct.
This seems like a simple sync error | 07-29-2019 22:48:31 | 07-29-2019 22:48:31 | Thanks! |
transformers | 918 | closed | Export to Tensorflow not properly implemented | Apologies for going about this backwards. I created a pull request #907 to fix your implementation of converting pytorch weights to tensorflow weights. As explained in the PR, the current implementation puts the weights from the pytorch model into two places in the newly created tensorflow checkpoint. The fix not only reduces the size of the meta file, but also reduces the running time. | 07-29-2019 14:46:13 | 07-29-2019 14:46:13 | Thanks @dhpollack ! |
transformers | 917 | closed | XLNet: Sentence probability/perplexity | Based on my understanding, XLnet can compute sentence probability/perplexity. Is there a example that illustrates how we can do this? I saw one for GPT-2 (https://github.com/huggingface/pytorch-transformers/issues/473), but don't think it'll work exactly the same... | 07-29-2019 05:57:27 | 07-29-2019 05:57:27 | Hi, I want to ask that question too.
Below is my implementation
```
def xlnet_score(text, model, tokenizer):
#text = "<cls>" + text + "<sep>"
# Tokenized input
tokenized_text = tokenizer.tokenize(text)
# text = "[CLS] Stir the mixture until it is done [SEP]"
sentence_prob = 0
#Sprint(len(tokenized_text))
for masked_index in range(0,len(tokenized_text)):
# Mask a token that we will try to predict back with `BertForMaskedLM`
masked_word = tokenized_text[masked_index]
if masked_word!= "<sep>":
masked_word = tokenized_text[masked_index]
tokenized_text[masked_index] = '<mask>'
input_ids = torch.tensor(tokenizer.convert_tokens_to_ids(tokenized_text)).unsqueeze(0)
index = torch.tensor(tokenizer.convert_tokens_to_ids(masked_word))
perm_mask = torch.zeros((1, input_ids.shape[1], input_ids.shape[1]), dtype=torch.float)
perm_mask[:, :, masked_index] = 1.0 # Previous tokens don't see last token
target_mapping = torch.zeros((1, 1, input_ids.shape[1]), dtype=torch.float) # Shape [1, 1, seq_length] => let's predict one token
target_mapping[0, 0, masked_index] = 1.0 # Our first (and only) prediction will be the last token of the sequence (the masked token)
input_ids = input_ids.to('cuda')
perm_mask = perm_mask.to('cuda')
target_mapping = target_mapping.to('cuda')
index = index.to('cuda')
with torch.no_grad():
outputs = model(input_ids, perm_mask=perm_mask, target_mapping=target_mapping, labels = index)
next_token_logits = outputs[0]
length = len(tokenized_text)
sentence_prob += next_token_logits.item()
tokenized_text[masked_index] = masked_word
return sentence_prob/(length)
a=['there is a book on the desk',
'there is a rocket on the desk',
'he put an elephant into the fridge', 'he put an apple into the fridge']
tokenizer = XLNetTokenizer.from_pretrained('xlnet-base-cased')
model = XLNetLMHeadModel.from_pretrained('xlnet-base-cased')
model.to('cuda')
model.eval()
print([xlnet_score(i,model,tokenizer) for i in a])
```
The result, anyway, does not seem to make much sense to me.
So I also want to ask if there is a better way to implement the model.
<|||||>This is how I did it in the end. The important thing is that you need to pad it with a long context before hand (discussed [here](https://medium.com/@amanrusia/xlnet-speaks-comparison-to-gpt-2-ea1a4e9ba39e)), and you need to iterate through the sentence, one word at a time to collect the conditional word probabilities.
```
import torch
from pytorch_transformers import XLNetTokenizer, XLNetLMHeadModel
import numpy as np
from scipy.special import softmax
PADDING_TEXT = """In 1991, the remains of Russian Tsar Nicholas II and his family
(except for Alexei and Maria) are discovered.
The voice of Nicholas's young son, Tsarevich Alexei Nikolaevich, narrates the
remainder of the story. 1883 Western Siberia,
a young Grigori Rasputin is asked by his father and a group of men to perform magic.
Rasputin has a vision and denounces one of the men as a horse thief. Although his
father initially slaps him for making such an accusation, Rasputin watches as the
man is chased outside and beaten. Twenty years later, Rasputin sees a vision of
the Virgin Mary, prompting him to become a priest. Rasputin quickly becomes famous,
with people, even a bishop, begging for his blessing. <eod> """
text = "The dog is very cute."
tokenizer = XLNetTokenizer.from_pretrained('xlnet-large-cased')
model = XLNetLMHeadModel.from_pretrained('xlnet-large-cased')
tokenize_input = tokenizer.tokenize(PADDING_TEXT + text)
tokenize_text = tokenizer.tokenize(text)
sum_lp = 0.0
for max_word_id in range((len(tokenize_input)-len(tokenize_text)), (len(tokenize_input))):
sent = tokenize_input[:]
input_ids = torch.tensor([tokenizer.convert_tokens_to_ids(sent)])
perm_mask = torch.zeros((1, input_ids.shape[1], input_ids.shape[1]), dtype=torch.float)
perm_mask[:, :, max_word_id:] = 1.0
target_mapping = torch.zeros((1, 1, input_ids.shape[1]), dtype=torch.float)
target_mapping[0, 0, max_word_id] = 1.0
with torch.no_grad():
outputs = model(input_ids, perm_mask=perm_mask, target_mapping=target_mapping)
next_token_logits = outputs[0] # Output has shape [target_mapping.size(0), target_mapping.size(1), config.vocab_size]
word_id = tokenizer.convert_tokens_to_ids([tokenize_input[max_word_id]])[0]
predicted_prob = softmax(np.array(next_token_logits[0][-1]))
lp = np.log(predicted_prob[word_id])
sum_lp += lp
print("sentence logprob =", sum_lp)
```<|||||>@jhlau Hi, thanks for sharing your solution. Just wondering if the padded text beforehand is very important for evaluating the sentence scores? What if you use a different text?<|||||>Yes, it is very important. Without the padded text, the sentence probability is pretty much useless. Pretty sure you can use any text, as long as you include the eod tag.<|||||>Hey @jhlau , thank you for sharing this with us!
I have been trying to accelerate the operation of the function by using `mems`, i.e. caching of the hidden states. Since we are The only changes I made are these:
```
model = XLNetLMHeadModel.from_pretrained('xlnet-large-cased', mem_len=1024)
```
, and
```
with torch.no_grad():
outputs = model(input_ids, perm_mask=perm_mask, target_mapping=target_mapping, mems=mems)
mems = outputs[1] # on the first word is none, i.e during first iteration of the for-loop
next_token_logits = outputs[0] # Output has shape [target_mapping.size(0), target_mapping.size(1), config.vocab_size]
predicted_prob = torch.softmax(next_token_logits[0][-1], dim=-1)
```
However, the probabilities for the tokens appear different between the cached and the non-cached version. Do you know if this is actually correct and what could be wrong? Does it actually make sense to cache the intermediate states?
Thanks!<|||||>I don't think you can cache it, since the hidden states are different for every step (which has a different masked word).<|||||>hi @jhlau , wondering if you have a batch-processing version of your script such that people can use as an off-the-shelf tool for evaluating a (big) list of sentences? Thanks very much!<|||||>Unfortunately not. Haven't had the time to look into processing sentences in batch.<|||||>> This is how I did it in the end. The important thing is that you need to pad it with a long context before hand (discussed [here](https://medium.com/@amanrusia/xlnet-speaks-comparison-to-gpt-2-ea1a4e9ba39e)), and you need to iterate through the sentence, one word at a time to collect the conditional word probabilities.
>
> ```
> import torch
> from pytorch_transformers import XLNetTokenizer, XLNetLMHeadModel
> import numpy as np
> from scipy.special import softmax
>
> PADDING_TEXT = """In 1991, the remains of Russian Tsar Nicholas II and his family
> (except for Alexei and Maria) are discovered.
> The voice of Nicholas's young son, Tsarevich Alexei Nikolaevich, narrates the
> remainder of the story. 1883 Western Siberia,
> a young Grigori Rasputin is asked by his father and a group of men to perform magic.
> Rasputin has a vision and denounces one of the men as a horse thief. Although his
> father initially slaps him for making such an accusation, Rasputin watches as the
> man is chased outside and beaten. Twenty years later, Rasputin sees a vision of
> the Virgin Mary, prompting him to become a priest. Rasputin quickly becomes famous,
> with people, even a bishop, begging for his blessing. <eod> """
>
> text = "The dog is very cute."
>
> tokenizer = XLNetTokenizer.from_pretrained('xlnet-large-cased')
> model = XLNetLMHeadModel.from_pretrained('xlnet-large-cased')
>
> tokenize_input = tokenizer.tokenize(PADDING_TEXT + text)
> tokenize_text = tokenizer.tokenize(text)
>
> sum_lp = 0.0
> for max_word_id in range((len(tokenize_input)-len(tokenize_text)), (len(tokenize_input))):
>
> sent = tokenize_input[:]
>
> input_ids = torch.tensor([tokenizer.convert_tokens_to_ids(sent)])
>
> perm_mask = torch.zeros((1, input_ids.shape[1], input_ids.shape[1]), dtype=torch.float)
> perm_mask[:, :, max_word_id:] = 1.0
>
> target_mapping = torch.zeros((1, 1, input_ids.shape[1]), dtype=torch.float)
> target_mapping[0, 0, max_word_id] = 1.0
>
> with torch.no_grad():
> outputs = model(input_ids, perm_mask=perm_mask, target_mapping=target_mapping)
> next_token_logits = outputs[0] # Output has shape [target_mapping.size(0), target_mapping.size(1), config.vocab_size]
>
> word_id = tokenizer.convert_tokens_to_ids([tokenize_input[max_word_id]])[0]
> predicted_prob = softmax(np.array(next_token_logits[0][-1]))
> lp = np.log(predicted_prob[word_id])
>
> sum_lp += lp
>
> print("sentence logprob =", sum_lp)
> ```
@jhlau I selected the link you mentioned but it doesn't talk about the long text for padding. Could you please explain why it is needed or where you found it?<|||||>Hmm I should have cited the github link. Anyway it's explained in his GitHub implementation code README: https://github.com/rusiaaman/XLNet-gen#methodology
(and you can see it in the code, and the dummy text he used)<|||||>@jhlau Do you think this same reasoning could be applied to extract sentence probabilities from BERT?<|||||>@ruanchaves: you can, and I tried it with BERT (left context only for prediction). But the results isn't as good as XLNET (no surprises I supposed since BERT is used to seeing left and right context during training).<|||||>I just found a paper where they use BERT for sentence probabilities (
https://arxiv.org/abs/1905.06655 ). It states that one must train BERT on
the Mask LM task ( without NSP ) before reasonable results can be achieved.<|||||>Looks like they found that scoring sentences based on bidirectional context is better than unidirectional context for speech recognition, and that's a result similar to what we found for scoring sentences for naturalness/fluency: https://arxiv.org/pdf/2004.00881.pdf
(in summary we found that sentence probability (not true probability) computed with bidirectional context with simple normalisation (PenLP in table 2) correlates strongly with human perception of sentence naturalness/fluency) |
transformers | 916 | closed | Avoid i/o in class __init__ methods | Working with model serialization and configs is pretty painful, and we went through a lot of design iterations on this for spaCy.
I think one thing that's definitely unideal in `pytorch_transformers` is that the tokenizers often expect file names in the `__init__` methods. This means that if you're holding the data in memory, you have to first write it to file in order to create the class.
I think it would be nicer to move the load-from-disk part into a method, that could be called after the `__init__`. This wouldn't really change the usage of the classes, since mostly people are using the `.from_pretrained()` class method, but it would make the classes a bit more flexible. | 07-28-2019 11:31:25 | 07-28-2019 11:31:25 | Make sense to me. I'll include that in a coming PR.<|||||>This issue has been automatically marked as stale because it has not had recent activity. It will be closed if no further activity occurs. Thank you for your contributions.
|
transformers | 915 | closed | Wrong layer names for selecting parameters groups (run_openai_gpt.py) | Hi,
In this script [run_openai_gpt.py](https://github.com/huggingface/pytorch-transformers/blob/master/examples/single_model_scripts/run_openai_gpt.py)
Parameter names for selecting param groups are wrong.
Should be:
`
no_decay = no_decay = ['bias', 'ln_1.bias', 'ln_1.weight', 'ln_2.bias', 'ln_2.weight']
` | 07-28-2019 10:01:17 | 07-28-2019 10:01:17 | This issue has been automatically marked as stale because it has not had recent activity. It will be closed if no further activity occurs. Thank you for your contributions.
|
transformers | 914 | closed | Using new pretrained model with it's own vocab.txt file. | I am trying to use SciBert pretrained weights, which has its own vocab , so it's own 'vocab.txt', file.
I think it's fairly straight forward to point the `pytorch_model.bin` but I do not see any options to introduce a new vocab.txt file. | 07-28-2019 01:53:51 | 07-28-2019 01:53:51 | Found the answer
https://github.com/huggingface/pytorch-transformers/issues/69#issuecomment-443215315
you can just do a direct path to it<|||||>Can it work? I tried the solution but didn't work. I put the vocab.txt file under a certain path.<|||||>What error message did you get? Maybe try an absolute path to the file. <|||||>i
> What error message did you get? Maybe try an absolute path to the file.
It only works when you store the vocab.txt in `/tmp` which is the default `cache_dir`<|||||>This issue has been automatically marked as stale because it has not had recent activity. It will be closed if no further activity occurs. Thank you for your contributions.
|
transformers | 913 | closed | Best practices for combining large pretrained models with smaller models? | Hello,
If I were to try to combine a large model (like BERT) with a smaller model (some variation of fully connected, convolutional network with significantly less params and pretraining) by jointly training them and concatenating their outputs for a final classifier, what would be some things I should consider?
For example, should they have different optimizers and learning rates? Should I try to keep the number of params in the smaller model relatively small? What would be some good ways of fusing the output of BERT and the output of the small model besides concatenating?
I'd really appreciate any insight from anyone who's tried something like this or have thought about it. Thank you! | 07-28-2019 01:42:10 | 07-28-2019 01:42:10 | This issue has been automatically marked as stale because it has not had recent activity. It will be closed if no further activity occurs. Thank you for your contributions.
<|||||>@dchang56 Any updates? I am looking to do this as well. <|||||>Hi! I see that you're also doing scientific/medical NLP :)
I sent you an email at your gmail address. |
transformers | 912 | closed | adding vocabulary in OpenAI GPT2 tokenizer issue | Hi,
I am trying to add few vocabulary tokens to the gpt2 tokenizer
but there seems few problems in adding vocab.
Let's say I want to make sequence like
> "__bos__" + sequence A + "__seperator__" + sequence B + "__seperator__" + sequence C + "__eos__"
This means that I have to add "__bos__", "__seperator__", "__eos__" tokens to the tokenizer.
I've found <|endoftext|> token already in the vocab list, but I wanted to use those special
symbols to reflect my special intentions to treat input sequence.
However, when I succesfully added tokens to the vocab list by fixing some of the codes
in the 'tokenization_utils.py' file just like below,
```
# mark this line of code as a comment
# if self.convert_tokens_to_ids(token) == self.convert_tokens_to_ids(self.unk_token):
```
it works fine at the training stage, but the index mapping went totally different in the
evaluation phase.
Should I use random but unused token which already is in the vocab list of the tokenizer
to replace my special tokens?
For example, if there were some random "^&*" token exist in the vocab list,
use that token as my __bos__ token instead.
Anyway, thank you for providing such a legendary libraries opened!
Thank you very much :) | 07-27-2019 07:57:04 | 07-27-2019 07:57:04 | What specifically did you change in `tokenization_utils.py`?
> it works fine at the training stage, but the index mapping went totally different in the
> evaluation phase.
Can you elaborate on what you mean? Perhaps post some output? Is it hanging? Or are you just getting wildly poor performance once you move to eval?
<|||||>@brendanxwhitaker
thanks for asking !! :)
I found solution thanks to #799 ,
the problem was solved by adding
`model.resize_token_embeddings(len(tokenizer))`
line when recalling my model !
The problem was that I skipped over the part
where I had to resize the scale of the vocab to that of
when I add new tokens.
Thank you ! :) |
transformers | 911 | closed | Small fixes | Fix #908 and #901 | 07-26-2019 19:30:11 | 07-26-2019 19:30:11 | # [Codecov](https://codecov.io/gh/huggingface/pytorch-transformers/pull/911?src=pr&el=h1) Report
> Merging [#911](https://codecov.io/gh/huggingface/pytorch-transformers/pull/911?src=pr&el=desc) into [master](https://codecov.io/gh/huggingface/pytorch-transformers/commit/c054b5ee64df1a180417c5e87816879c93f54e17?src=pr&el=desc) will **increase** coverage by `0.01%`.
> The diff coverage is `90.9%`.
[](https://codecov.io/gh/huggingface/pytorch-transformers/pull/911?src=pr&el=tree)
```diff
@@ Coverage Diff @@
## master #911 +/- ##
==========================================
+ Coverage 79.03% 79.04% +0.01%
==========================================
Files 34 34
Lines 6234 6242 +8
==========================================
+ Hits 4927 4934 +7
- Misses 1307 1308 +1
```
| [Impacted Files](https://codecov.io/gh/huggingface/pytorch-transformers/pull/911?src=pr&el=tree) | Coverage Δ | |
|---|---|---|
| [pytorch\_transformers/tokenization\_utils.py](https://codecov.io/gh/huggingface/pytorch-transformers/pull/911/diff?src=pr&el=tree#diff-cHl0b3JjaF90cmFuc2Zvcm1lcnMvdG9rZW5pemF0aW9uX3V0aWxzLnB5) | `86.56% <90.9%> (+0.03%)` | :arrow_up: |
------
[Continue to review full report at Codecov](https://codecov.io/gh/huggingface/pytorch-transformers/pull/911?src=pr&el=continue).
> **Legend** - [Click here to learn more](https://docs.codecov.io/docs/codecov-delta)
> `Δ = absolute <relative> (impact)`, `ø = not affected`, `? = missing data`
> Powered by [Codecov](https://codecov.io/gh/huggingface/pytorch-transformers/pull/911?src=pr&el=footer). Last update [c054b5e...7b6e474](https://codecov.io/gh/huggingface/pytorch-transformers/pull/911?src=pr&el=lastupdated). Read the [comment docs](https://docs.codecov.io/docs/pull-request-comments).
<|||||># [Codecov](https://codecov.io/gh/huggingface/pytorch-transformers/pull/911?src=pr&el=h1) Report
> Merging [#911](https://codecov.io/gh/huggingface/pytorch-transformers/pull/911?src=pr&el=desc) into [master](https://codecov.io/gh/huggingface/pytorch-transformers/commit/c054b5ee64df1a180417c5e87816879c93f54e17?src=pr&el=desc) will **increase** coverage by `0.01%`.
> The diff coverage is `90.9%`.
[](https://codecov.io/gh/huggingface/pytorch-transformers/pull/911?src=pr&el=tree)
```diff
@@ Coverage Diff @@
## master #911 +/- ##
==========================================
+ Coverage 79.03% 79.04% +0.01%
==========================================
Files 34 34
Lines 6234 6242 +8
==========================================
+ Hits 4927 4934 +7
- Misses 1307 1308 +1
```
| [Impacted Files](https://codecov.io/gh/huggingface/pytorch-transformers/pull/911?src=pr&el=tree) | Coverage Δ | |
|---|---|---|
| [pytorch\_transformers/tokenization\_utils.py](https://codecov.io/gh/huggingface/pytorch-transformers/pull/911/diff?src=pr&el=tree#diff-cHl0b3JjaF90cmFuc2Zvcm1lcnMvdG9rZW5pemF0aW9uX3V0aWxzLnB5) | `86.56% <90.9%> (+0.03%)` | :arrow_up: |
------
[Continue to review full report at Codecov](https://codecov.io/gh/huggingface/pytorch-transformers/pull/911?src=pr&el=continue).
> **Legend** - [Click here to learn more](https://docs.codecov.io/docs/codecov-delta)
> `Δ = absolute <relative> (impact)`, `ø = not affected`, `? = missing data`
> Powered by [Codecov](https://codecov.io/gh/huggingface/pytorch-transformers/pull/911?src=pr&el=footer). Last update [c054b5e...7b6e474](https://codecov.io/gh/huggingface/pytorch-transformers/pull/911?src=pr&el=lastupdated). Read the [comment docs](https://docs.codecov.io/docs/pull-request-comments).
|
transformers | 910 | closed | Adding AutoTokenizer and AutoModel classes that automatically detect architecture - Clean up tokenizers | As discussed in #890
Classes that automatically detect the relevant model/config/tokenizer to instantiate based on the`pretrained_model_name_or_path` string provided to `AutoXXX.from_pretrained(pretrained_model_name_or_path)`.
Right now:
- `AutoConfig`
- `AutoTokenizer`
- `AutoModel` (bare models outputting hidden-states)
Missing:
- Tests
- Maybe a few other architectures beside raw models (`AutoModelWithLMHead`, `AutoModelForSequenceClassification`, `AutoModelForTokensClassification`, `AutoModelForQuestionAnswering`)
- Check if we can make hubconfs simpler to maintain using AutoModels.
Additional stuff:
- add a `unk_token` to GPT2 to fix #799
- clean up tokenizers and associated tests | 07-26-2019 17:27:32 | 07-26-2019 17:27:32 | # [Codecov](https://codecov.io/gh/huggingface/pytorch-transformers/pull/910?src=pr&el=h1) Report
> Merging [#910](https://codecov.io/gh/huggingface/pytorch-transformers/pull/910?src=pr&el=desc) into [master](https://codecov.io/gh/huggingface/pytorch-transformers/commit/46cc9dd2b51a152b2e262ec12e40dddd13235aba?src=pr&el=desc) will **increase** coverage by `0.17%`.
> The diff coverage is `91.29%`.
[](https://codecov.io/gh/huggingface/pytorch-transformers/pull/910?src=pr&el=tree)
```diff
@@ Coverage Diff @@
## master #910 +/- ##
=========================================
+ Coverage 79.03% 79.2% +0.17%
=========================================
Files 34 38 +4
Lines 6234 6396 +162
=========================================
+ Hits 4927 5066 +139
- Misses 1307 1330 +23
```
| [Impacted Files](https://codecov.io/gh/huggingface/pytorch-transformers/pull/910?src=pr&el=tree) | Coverage Δ | |
|---|---|---|
| [pytorch\_transformers/modeling\_transfo\_xl.py](https://codecov.io/gh/huggingface/pytorch-transformers/pull/910/diff?src=pr&el=tree#diff-cHl0b3JjaF90cmFuc2Zvcm1lcnMvbW9kZWxpbmdfdHJhbnNmb194bC5weQ==) | `57.53% <ø> (ø)` | :arrow_up: |
| [pytorch\_transformers/modeling\_gpt2.py](https://codecov.io/gh/huggingface/pytorch-transformers/pull/910/diff?src=pr&el=tree#diff-cHl0b3JjaF90cmFuc2Zvcm1lcnMvbW9kZWxpbmdfZ3B0Mi5weQ==) | `75.84% <ø> (ø)` | :arrow_up: |
| [pytorch\_transformers/modeling\_xlm.py](https://codecov.io/gh/huggingface/pytorch-transformers/pull/910/diff?src=pr&el=tree#diff-cHl0b3JjaF90cmFuc2Zvcm1lcnMvbW9kZWxpbmdfeGxtLnB5) | `86.66% <ø> (ø)` | :arrow_up: |
| [pytorch\_transformers/modeling\_bert.py](https://codecov.io/gh/huggingface/pytorch-transformers/pull/910/diff?src=pr&el=tree#diff-cHl0b3JjaF90cmFuc2Zvcm1lcnMvbW9kZWxpbmdfYmVydC5weQ==) | `87.98% <ø> (ø)` | :arrow_up: |
| [pytorch\_transformers/modeling\_openai.py](https://codecov.io/gh/huggingface/pytorch-transformers/pull/910/diff?src=pr&el=tree#diff-cHl0b3JjaF90cmFuc2Zvcm1lcnMvbW9kZWxpbmdfb3BlbmFpLnB5) | `74.76% <ø> (ø)` | :arrow_up: |
| [pytorch\_transformers/modeling\_xlnet.py](https://codecov.io/gh/huggingface/pytorch-transformers/pull/910/diff?src=pr&el=tree#diff-cHl0b3JjaF90cmFuc2Zvcm1lcnMvbW9kZWxpbmdfeGxuZXQucHk=) | `79.01% <ø> (ø)` | :arrow_up: |
| [...transformers/tests/tokenization\_transfo\_xl\_test.py](https://codecov.io/gh/huggingface/pytorch-transformers/pull/910/diff?src=pr&el=tree#diff-cHl0b3JjaF90cmFuc2Zvcm1lcnMvdGVzdHMvdG9rZW5pemF0aW9uX3RyYW5zZm9feGxfdGVzdC5weQ==) | `96.96% <100%> (+0.54%)` | :arrow_up: |
| [...rch\_transformers/tests/tokenization\_openai\_test.py](https://codecov.io/gh/huggingface/pytorch-transformers/pull/910/diff?src=pr&el=tree#diff-cHl0b3JjaF90cmFuc2Zvcm1lcnMvdGVzdHMvdG9rZW5pemF0aW9uX29wZW5haV90ZXN0LnB5) | `97.22% <100%> (+0.44%)` | :arrow_up: |
| [pytorch\_transformers/modeling\_utils.py](https://codecov.io/gh/huggingface/pytorch-transformers/pull/910/diff?src=pr&el=tree#diff-cHl0b3JjaF90cmFuc2Zvcm1lcnMvbW9kZWxpbmdfdXRpbHMucHk=) | `83.16% <100%> (-0.13%)` | :arrow_down: |
| [pytorch\_transformers/tokenization\_xlnet.py](https://codecov.io/gh/huggingface/pytorch-transformers/pull/910/diff?src=pr&el=tree#diff-cHl0b3JjaF90cmFuc2Zvcm1lcnMvdG9rZW5pemF0aW9uX3hsbmV0LnB5) | `88.11% <100%> (ø)` | :arrow_up: |
| ... and [21 more](https://codecov.io/gh/huggingface/pytorch-transformers/pull/910/diff?src=pr&el=tree-more) | |
------
[Continue to review full report at Codecov](https://codecov.io/gh/huggingface/pytorch-transformers/pull/910?src=pr&el=continue).
> **Legend** - [Click here to learn more](https://docs.codecov.io/docs/codecov-delta)
> `Δ = absolute <relative> (impact)`, `ø = not affected`, `? = missing data`
> Powered by [Codecov](https://codecov.io/gh/huggingface/pytorch-transformers/pull/910?src=pr&el=footer). Last update [46cc9dd...0b524b0](https://codecov.io/gh/huggingface/pytorch-transformers/pull/910?src=pr&el=lastupdated). Read the [comment docs](https://docs.codecov.io/docs/pull-request-comments).
|
transformers | 909 | closed | [develop] Convenience args.{train/dev}_file arguments. | Adds Arguments
```
--train_file any_train_file.tsv \
--dev_file any_dev_file.tsv \
```
to use any file for training/dev in the pointed data directory.
Especially handy for evaluation.
Allows for
```
python run_glue.py \
--model_type bert \
--model_name_or_path bert-base-uncased \
--task_name $TASK_NAME \
--do_train \
--do_eval \
--train_file any_train_file.tsv \
--dev_file any_dev_file.tsv \
--do_lower_case \
--data_dir $GLUE_DIR/$TASK_NAME \
--max_seq_length 128 \
--per_gpu_eval_batch_size=8 \
--per_gpu_train_batch_size=8 \
--learning_rate 2e-5 \
--num_train_epochs 3.0 \
--output_dir ./Data/Test/
``` | 07-26-2019 16:52:30 | 07-26-2019 16:52:30 | Why don't give the full path of the train/dev file instead of giving data_dir?<|||||>My thought was not the change much of the argument interface. If we support full path, then the `data_dir` will not be required and considered. So I wasn't sure if that change is the way to go. Sure, we can change it like that as well.
Personally, I do agree that full path for train/dev is more convenient while using. |
transformers | 908 | closed | Cannot inherit from BertPretrainedModel anymore after migrating to pytorch-transformers | Hi,
After I updated my environment today, I cannot run my old code anymore. I think I followed all the steps in migration section of README but still the following code gives me the `NameError: name 'BertPreTrainedModel' is not defined` error. To migrate latest version, I cloned the repository and run `pip install --editable .` command within the directory.
Here is the code:
```python
from pytorch_transformers import *
class BertForMultiLabelSequenceClassification(BertPreTrainedModel):
def __init__(self, config, num_labels=2):
super(BertForMultiLabelSequenceClassification, self).__init__(config)
self.num_labels = num_labels
self.bert = BertModel("bert-base-multilingual-cased")
self.dropout = torch.nn.Dropout(config.hidden_dropout_prob)
self.classifier = torch.nn.Linear(config.hidden_size, num_labels)
self.apply(self.init_bert_weights)
def forward(self, input_ids, token_type_ids=None, attention_mask=None, labels=None):
_, pooled_output = self.bert(input_ids, token_type_ids, attention_mask, output_all_encoded_layers=False)
pooled_output = outputs[-1]
pooled_output = self.dropout(pooled_output)
logits = self.classifier(pooled_output)
return logits
args = {
"train_size": -1,
"val_size": -1,
"bert_model": "bert-base-multilingual-cased",
"do_lower_case":False,
"max_seq_length": 100,
"do_train": True,
"do_eval": True,
"train_batch_size": 32,
"eval_batch_size": 32,
"learning_rate": 3e-5,
"num_train_epochs": 20,
"warmup_proportion": 0.1,
"no_cuda": False,
"local_rank": -1,
"seed": 42,
}
num_labels = 2
model = BertForMultiLabelSequenceClassification.from_pretrained(args['bert-model'],num_labels)
``` | 07-26-2019 16:36:25 | 07-26-2019 16:36:25 | You should do `from pytorch_transformers.modeling_bert import BertPreTrainedModel`
I'll add these to the main `__init__.py`<|||||>Thank you for the answer @thomwolf . It solved that error but now I'm getting another one (which wasn't there when I was using previous versions of the repository): `TypeError: unhashable type: 'BertConfig'` what could be wrong ? <|||||>We need a full error log and more details. |
transformers | 907 | closed | Fix convert to tf | I struggled with this same problem for a long time. The naive `assign` op way, puts all of the weights into both the checkpoint file (`.ckpt.data-XXXXX-of-YYYYY`) and the meta file (`.ckpt.meta`). This is because assign adds an operation to the graph. So basically, you have two instructions in your meta file, one that initializes the variable with random values and then another that assigns the pytorch values to these tensors. But really, you want to initialize everything once with the meta file and then read the data file which should have your pytorch weights in it. Tensorflow hides this functionality deep within it's source code and every answer on stackoverflow tells one to use `assign`. But the `tf.keras.backend.set_value` function does simply replace the weights of a variable. However, this function makes some assumptions about your session and your graph so I had to change your code a bit. Long story short, doing easy things in tensorflow is hard.
So what's the difference?
1. the meta file will be about 1mb instead of 400+ mb
2. the script runs in about 10 seconds instead of 3 minutes
| 07-26-2019 13:35:08 | 07-26-2019 13:35:08 | # [Codecov](https://codecov.io/gh/huggingface/pytorch-transformers/pull/907?src=pr&el=h1) Report
> Merging [#907](https://codecov.io/gh/huggingface/pytorch-transformers/pull/907?src=pr&el=desc) into [master](https://codecov.io/gh/huggingface/pytorch-transformers/commit/46cc9dd2b51a152b2e262ec12e40dddd13235aba?src=pr&el=desc) will **not change** coverage.
> The diff coverage is `n/a`.
[](https://codecov.io/gh/huggingface/pytorch-transformers/pull/907?src=pr&el=tree)
```diff
@@ Coverage Diff @@
## master #907 +/- ##
=======================================
Coverage 79.03% 79.03%
=======================================
Files 34 34
Lines 6234 6234
=======================================
Hits 4927 4927
Misses 1307 1307
```
------
[Continue to review full report at Codecov](https://codecov.io/gh/huggingface/pytorch-transformers/pull/907?src=pr&el=continue).
> **Legend** - [Click here to learn more](https://docs.codecov.io/docs/codecov-delta)
> `Δ = absolute <relative> (impact)`, `ø = not affected`, `? = missing data`
> Powered by [Codecov](https://codecov.io/gh/huggingface/pytorch-transformers/pull/907?src=pr&el=footer). Last update [46cc9dd...09ecf22](https://codecov.io/gh/huggingface/pytorch-transformers/pull/907?src=pr&el=lastupdated). Read the [comment docs](https://docs.codecov.io/docs/pull-request-comments).
<|||||>Ok thanks David! |
transformers | 906 | closed | cuda out of memory | `import torch
from pytorch_transformers import BertTokenizer, BertForSequenceClassification
tokenizer = BertTokenizer.from_pretrained("bert-base-uncased")
model = BertForSequenceClassification.from_pretrained("bert-base-uncased")
import csv
data = []
label = []
with open('Training.csv','r') as file:
reader = csv.reader(file)
for row in reader:
data.append("[CLS] "+row[1]+" [SEP]")
label.append(int(row[2]))
def tokenize_data(data): # for numericalizing the text
for sub in range(len(data)):
data_tokenized = tokenizer.encode(data[sub])
data[sub] = data_tokenized
return data
def make_batches(data): # for making all the sentences into same length
max_len = len(data[-1])
for i in range(len(data)):
if(len(data[i]) < max_len):
iter = max_len - len(data[i])
for j in range(iter):
data[i].append(102)
return data
optim = torch.optim.Adam(model.parameters(), lr=2e-05, betas=(0.9, 0.98), eps=1e-9)
import numpy as np
model = model.cuda()
model.train()
#model = torch.nn.DataParallel(model)
batch_size = 20
for i in range(0,len(data),batch_size):
print(i)
if True:
batch = data[i:i+batch_size]
batch = tokenize_data(batch)
batch.sort(key = lambda x : len(x))
batch = make_batches(batch)
batch = torch.tensor(batch)
target = torch.tensor(label[i:i+batch_size])
inp = batch.cuda()
target = target.cuda()
output = model(inp)
loss = torch.nn.functional.cross_entropy(output[0].view(-1,output[0].size()[-1]),target.contiguous().view(-1))
print(loss)
optim.zero_grad()
model.zero_grad()
loss.backward()
optim.step()
print("success")
`
so the above is my code and whenever i run it ,it give me error saying
`Traceback (most recent call last):
File "classification_using_bert.py", line 49, in <module>
loss.backward()
File "/home/zlabs-nlp/miniconda3/envs/ravienv/lib/python3.7/site-packages/torch/tensor.py", line 107, in backward
torch.autograd.backward(self, gradient, retain_graph, create_graph)
File "/home/zlabs-nlp/miniconda3/envs/ravienv/lib/python3.7/site-packages/torch/autograd/__init__.py", line 93, in backward
allow_unreachable=True) # allow_unreachable flag
RuntimeError: CUDA out of memory. Tried to allocate 42.00 MiB (GPU 0; 10.92 GiB total capacity; 6.34 GiB already allocated; 28.50 MiB free; 392.76 MiB cached)`
CAN ANYONE TEL ME WHAT IS MISTAKE
THANKS IN ADVANCE !!!!!!!!!! | 07-26-2019 08:12:11 | 07-26-2019 08:12:11 | Try to implement gradient accumulation during training, instead of updating parameters in each iteration. Please check this nice and easy-to-follow tutorial by @thomwolf [here](https://medium.com/huggingface/training-larger-batches-practical-tips-on-1-gpu-multi-gpu-distributed-setups-ec88c3e51255) . I used this technique with GPT-2 small, with a dataset of ~350k, with single GPU and it worked completely fine.<|||||>thanks @sajidrahman
i will go through it <|||||>Edit: There is a parameter now for `gradient_accumulation_steps`... this can be adjusted to achieve gradient accumulation? <|||||>The problem is about batch size 20. Batch sizes more than 4 are something that doesn't fit most of (single) gpu's for many models. Check this: https://github.com/huggingface/transformers/issues/2016#issuecomment-561093186 . Some cases you cannot make fit even 1 batch to memory. As @sajidrahman mentioned, [this](https://medium.com/huggingface/training-larger-batches-practical-tips-on-1-gpu-multi-gpu-distributed-setups-ec88c3e51255) is a good point to start.
The issue can be closed if everything is clear?<|||||>This issue has been automatically marked as stale because it has not had recent activity. It will be closed if no further activity occurs. Thank you for your contributions.
<|||||>Even in my case problem was my batch size of 8, worked after changing it to 2. |
transformers | 905 | closed | Bugfix for encoding error during GPT2Tokenizer.from_pretrained('local… | …/path/to/mode')
BUG DESCRIPTION: Loading GPT2-tokenizer from local path with
GPT2Tokenizer.from_pretrained(pretrained_model_name_or_path='local/path/to/model')
returns following error due to encoding error for json.load():
Traceback (most recent call last):
File "/opt/pycharm-2019.1.3/helpers/pydev/pydevd.py", line 1758, in <module>
main()
File "/opt/pycharm-2019.1.3/helpers/pydev/pydevd.py", line 1752, in main
globals = debugger.run(setup['file'], None, None, is_module)
File "/opt/pycharm-2019.1.3/helpers/pydev/pydevd.py", line 1147, in run
pydev_imports.execfile(file, globals, locals) # execute the script
File "/opt/pycharm-2019.1.3/helpers/pydev/_pydev_imps/_pydev_execfile.py", line 18, in execfile
exec(compile(contents+"\n", file, 'exec'), glob, loc)
File "/home/developer/AmI/transfer-learning-conv-ai/pytorch_transformer_evaluation.py", line 24, in <module>
cache_dir=None)
File "/home/developer/AmI/pytorch-transformers/pytorch_transformers/tokenization_utils.py", line 151, in from_pretrained
return cls._from_pretrained(*inputs, **kwargs)
File "/home/developer/AmI/pytorch-transformers/pytorch_transformers/tokenization_utils.py", line 240, in _from_pretrained
tokenizer = cls(*inputs, **kwargs)
File "/home/developer/AmI/pytorch-transformers/pytorch_transformers/tokenization_gpt2.py", line 110, in __init__
self.encoder = json.load(open(vocab_file))
File "/conda/envs/rapids/lib/python3.6/json/__init__.py", line 296, in load
return loads(fp.read(),
File "/conda/envs/rapids/lib/python3.6/encodings/ascii.py", line 26, in decode
return codecs.ascii_decode(input, self.errors)[0]
UnicodeDecodeError: 'ascii' codec can't decode byte 0xc2 in position 840: ordinal not in range(128) | 07-25-2019 20:50:23 | 07-25-2019 20:50:23 | # [Codecov](https://codecov.io/gh/huggingface/pytorch-transformers/pull/905?src=pr&el=h1) Report
> Merging [#905](https://codecov.io/gh/huggingface/pytorch-transformers/pull/905?src=pr&el=desc) into [master](https://codecov.io/gh/huggingface/pytorch-transformers/commit/46cc9dd2b51a152b2e262ec12e40dddd13235aba?src=pr&el=desc) will **increase** coverage by `<.01%`.
> The diff coverage is `100%`.
[](https://codecov.io/gh/huggingface/pytorch-transformers/pull/905?src=pr&el=tree)
```diff
@@ Coverage Diff @@
## master #905 +/- ##
==========================================
+ Coverage 79.03% 79.03% +<.01%
==========================================
Files 34 34
Lines 6234 6235 +1
==========================================
+ Hits 4927 4928 +1
Misses 1307 1307
```
| [Impacted Files](https://codecov.io/gh/huggingface/pytorch-transformers/pull/905?src=pr&el=tree) | Coverage Δ | |
|---|---|---|
| [pytorch\_transformers/tokenization\_gpt2.py](https://codecov.io/gh/huggingface/pytorch-transformers/pull/905/diff?src=pr&el=tree#diff-cHl0b3JjaF90cmFuc2Zvcm1lcnMvdG9rZW5pemF0aW9uX2dwdDIucHk=) | `96.69% <100%> (+0.02%)` | :arrow_up: |
------
[Continue to review full report at Codecov](https://codecov.io/gh/huggingface/pytorch-transformers/pull/905?src=pr&el=continue).
> **Legend** - [Click here to learn more](https://docs.codecov.io/docs/codecov-delta)
> `Δ = absolute <relative> (impact)`, `ø = not affected`, `? = missing data`
> Powered by [Codecov](https://codecov.io/gh/huggingface/pytorch-transformers/pull/905?src=pr&el=footer). Last update [46cc9dd...f8d9977](https://codecov.io/gh/huggingface/pytorch-transformers/pull/905?src=pr&el=lastupdated). Read the [comment docs](https://docs.codecov.io/docs/pull-request-comments).
|
transformers | 904 | closed | AssertionError while using DataParallelModel | Hi,
I'm trying to use _Load Balancing during multi-GPU_ environment. I'm following the tutorial by @thomwolf published at [medium](https://medium.com/huggingface/training-larger-batches-practical-tips-on-1-gpu-multi-gpu-distributed-setups-ec88c3e51255). I'm fine-tuning GPT-2 small for a classification task. Here're the steps I've followed so far:
1. Copy [parallel.py](https://gist.github.com/thomwolf/7e2407fbd5945f07821adae3d9fd1312?source=post_page---------------------------) in local directory
2. Add `from torch.nn.parallel.distributed import DistributedDataParallel` in parallel.py file (otherwise getting an error 'DistributedDataParallel' not found)
3. After loading GPT2Pretrained model, define the parallel model:
```
model = DataParallelModel(model, device_ids=[0, 1])
parallel_loss = DataParallelCriterion(model, device_ids=[0,1])
```
4. Now during training, got the following error. Complete stacktrace is as follows:
> AssertionError Traceback (most recent call last)
> <ipython-input-135-05384873e022> in <module>
> 19
> 20 # losses = model(input_ids, mc_token_ids, lm_labels=lm_labels, mc_labels=mc_labels)
> ---> 21 losses = parallel_loss(input_ids, mc_token_ids, lm_labels=lm_labels, mc_labels=mc_labels)
> 22
> 23 lm_loss, clf_loss = losses
>
> ~/anaconda3/envs/py36/lib/python3.6/site-packages/torch/nn/modules/module.py in __call__(self, *input, **kwargs)
>
> 491 result = self._slow_forward(*input, **kwargs)
> 492 else:
> --> 493 result = self.forward(*input, **kwargs)
> 494 for hook in self._forward_hooks.values():
> 495 hook_result = hook(self, input, result)
>
> ~/github_repos/pytorch-pretrained-BERT/examples/parallel.py in forward(self, inputs, *targets, **kwargs)
>
> 158 return self.module(inputs, *targets[0], **kwargs[0])
> 159 replicas = self.replicate(self.module, self.device_ids[:len(inputs)])
> --> 160 outputs = _criterion_parallel_apply(replicas, inputs, targets, kwargs)
> 161 #return Reduce.apply(*outputs) / len(outputs)
> 162 #return self.gather(outputs, self.output_device).mean()
>
> ~/github_repos/pytorch-pretrained-BERT/examples/parallel.py in _criterion_parallel_apply(modules, inputs, targets, kwargs_tup, devices)
>
> 165
> 166 def _criterion_parallel_apply(modules, inputs, targets, kwargs_tup=None, devices=None):
> --> 167 assert len(modules) == len(inputs)
> 168 assert len(targets) == len(inputs)
> 169 if kwargs_tup:
>
> AssertionError:
From the stacttrace, I'm not sure why module length needs to be equal of inputs length. Am I missing something here? I'm using Python 3.6 with PyTorch version 1.1.0. Any help/pointers will be highly appreciated. Thanks! | 07-25-2019 19:40:06 | 07-25-2019 19:40:06 | You don't need to use this method here because the models have built-in losses computation.
Just feed the labels and you will get the loss back (see the doc/docstrings of the models).<|||||>Hi @thomwolf, thanks for the suggestion. After following your advice, I'm not getting the error anymore, but now I'm a bit confused about the `backward()` pass. Now the **losses** variable contains a list of tensors of loss calculations per gpu and I'm not sure how I can enforce each individual model sitting in each gpu to perform backprop. Following is a sample code of what I've done so far and the sample output:
```
losses:[[tensor(98.5968, device='cuda:0', grad_fn=<NllLossBackward>), tensor(0.7206, device='cuda:0', grad_fn=<BinaryCrossEntropyWithLogitsBackward>)], [tensor(100.5673, device='cuda:1', grad_fn=<NllLossBackward>), tensor(0.6629, device='cuda:1', grad_fn=<BinaryCrossEntropyWithLogitsBackward>)]]
lm_loss: (tensor(98.5968, device='cuda:0', grad_fn=<NllLossBackward>), tensor(100.5673, device='cuda:1', grad_fn=<NllLossBackward>))
clf_loss:(tensor(0.7206, device='cuda:0', grad_fn=<BinaryCrossEntropyWithLogitsBackward>), tensor(0.6629, device='cuda:1', grad_fn=<BinaryCrossEntropyWithLogitsBackward>))
---------------------------------------------------------------------------
AttributeError Traceback (most recent call last)
<ipython-input-55-10ac591c2408> in <module>
26 lm_loss, clf_loss = zip(*losses)
27 print('losses:{} \n lm_loss: {}\nclf_loss:{}\n'.format(losses, lm_loss, clf_loss))
28
---> 29 loss = (args.lm_coef * lm_loss.to(device) + clf_loss.to(device)).to(device)
30
31 print(loss)
AttributeError: 'tuple' object has no attribute 'to'
```
Obviously I can deal with this 'tuple' error, but I'm confused what should I do next with this? Should I call `loss.backward()` per each cuda devices? How will I then gather gradient values in that case? Please excuse me for any naive assumptions I'm making here. Your input would be highly appreciated :)<|||||>This issue has been automatically marked as stale because it has not had recent activity. It will be closed if no further activity occurs. Thank you for your contributions.
<|||||>Hi @thomwolf , I also experience this imbalanced GPU usage when using the trainer function. Can I ask the reason that the DataParallelModel that you discussed on the Medium post, is not applied as default in the trainer function? Thank you. |
transformers | 903 | closed | why the acc of chinese model(bert) is just 0.438 | dataset: XNLI-1.0
i run the dataset of xnli-1.0, but the result is `acc = 0.43855421686746987`, and i run on google bert in tf, the result is `eval_accuracy = 0.7674699`. i use the same epochs and lr, i really don't know why.
i add the dataprocess of xnli ,the same with the version of tf bert:
```
class XnliProcessor(DataProcessor):
"""Processor for the XNLI data set."""
def __init__(self):
self.language = "zh"
def get_train_examples(self, data_dir):
"""See base class."""
lines = self._read_tsv(
os.path.join(data_dir, "multinli",
"multinli.train.%s.tsv" % self.language))
examples = []
for (i, line) in enumerate(lines):
if i == 0:
continue
guid = "train-%d" % (i)
text_a = line[0]
text_b = line[1]
label = line[2]
if label == "contradictory":
label = "contradiction"
examples.append(
InputExample(guid=guid, text_a=text_a, text_b=text_b, label=label))
return examples
def get_dev_examples(self, data_dir):
"""See base class."""
lines = self._read_tsv(os.path.join(data_dir, "xnli.dev.tsv"))
examples = []
for (i, line) in enumerate(lines):
if i == 0:
continue
guid = "dev-%d" % (i)
language = line[0]
if language != self.language:
continue
text_a = line[6]
text_b = line[7]
label = line[1]
examples.append(
InputExample(guid=guid, text_a=text_a, text_b=text_b, label=label))
return examples
def get_labels(self):
"""See base class."""
return ["contradiction", "entailment", "neutral"]
```
and my pytorch command is :
```
python run_glue.py --model_type bert --model_name_or_path bert-base-chinese --task_name XNLI --do_train --do_eval --do_lower_case --data_dir $XNLI_DIR --max_seq_length 128 --per_gpu_eval_batch_size=8 --per_gpu_train_batch_size=8 --learning_rate 5e-5 --num_train_epochs 2.0 --output_dir /tmp/MRPC4/ --overwrite_output_dir --save_steps=1000
```
and my tf common is:
```
python run_classifier.py --task_name=XNLI --do_train=true --do_eval=true --data_dir=$XNLI_DIR --vocab_file=$BERT_BASE_DIR/vocab.txt --bert_config_file=$BERT_BASE_DIR/bert_config.json --init_checkpoint=$BERT_BASE_DIR/bert_model.ckpt --max_seq_length=128 --train_batch_size=32 --learning_rate=5e-5 --num_train_epochs=2.0 --output_dir=/tmp/xnli_output
```
| 07-25-2019 11:40:18 | 07-25-2019 11:40:18 | i have the same issue as you. Do you have a good solution for it?<|||||>I met the same problem in multi-labels classification task, have no idea about the problem!<|||||>@zsk423200 Maybe you can try the "bert-base-multilingual-cased-pytorch_model" , it's performance seems better a lot than the pure Chinese ver in my task, just a temporal solu.<|||||>This issue has been automatically marked as stale because it has not had recent activity. It will be closed if no further activity occurs. Thank you for your contributions.
|
transformers | 902 | closed | Torchscript Trace slower with C++ runtime environment. | I traced the BERT model from PyTorchTransformers library and getting the following results for 10 iterations.
a) Using Python runtime for running the forward: 979,292 µs
```
import time
model = torch.jit.load('models_backup/2_2.pt')
x = torch.randint(2000, (1, 14), dtype=torch.long, device='cpu')
start = time.time()
for i in range(10):
model(x)
end = time.time()
print((end - start)*1000000, "µs")
```
b) Using C++ runtime for running the forward: 3,333,758 µs which is almost 3x of what Python
```
torch::Tensor x = torch::randint(index_max, {1, inputsize}, torch::dtype(torch::kInt64).device(torch::kCPU));
input.push_back(x);
#endif
// Execute the model and turn its output into a tensor.
auto outputs = module->forward(input).toTuple();
auto start = chrono::steady_clock::now();
for (int16_t i = 0; i<10; ++i)
{
outputs = module->forward(input).toTuple();
}
auto end = chrono::steady_clock::now();
cout << "Elapsed time in microseconds : "
<< chrono::duration_cast<chrono::microseconds>(end - start).count()
<< " µs" << endl;
```
@thomwolf any suggestions on what am I missing ? | 07-25-2019 11:06:57 | 07-25-2019 11:06:57 | 2 possible reasons:
1. the first time you run `forward` will do some preheating work, maybe you should exclude the first run.
2. try exclude `toTuple`
According to my experience, jit with python or c++ will cost almost the same time.<|||||>@Meteorix Forward is called once before the loop, are you talking about something else.
Excluding `toTuple` doesn't help. <|||||>This issue has been automatically marked as stale because it has not had recent activity. It will be closed if no further activity occurs. Thank you for your contributions.
|
transformers | 901 | closed | bug: it is broken to use tokenizer path | run run_glue.py with the parameter of tokenizer_name:
`--tokenizer_name=/path/bert-base-chinese-vocab.txt`
but get the error:
```
Traceback (most recent call last):
File "run_glue.py", line 485, in <module>
main()
File "run_glue.py", line 418, in main
tokenizer = tokenizer_class.from_pretrained(args.tokenizer_name if args.tokenizer_name else args.model_name_or_path, do_lower_case=args.do_lower_case)
File "/opt/conda/lib/python3.6/site-packages/pytorch_transformers/tokenization_bert.py", line 200, in from_pretrained
return super(BertTokenizer, cls)._from_pretrained(pretrained_model_name_or_path, *inputs, **kwargs)
File "/opt/conda/lib/python3.6/site-packages/pytorch_transformers/tokenization_utils.py", line 234, in _from_pretrained
special_tokens_map = json.load(open(special_tokens_map_file, encoding="utf-8"))
File "/opt/conda/lib/python3.6/json/__init__.py", line 299, in load
parse_constant=parse_constant, object_pairs_hook=object_pairs_hook, **kw)
File "/opt/conda/lib/python3.6/json/__init__.py", line 354, in loads
return _default_decoder.decode(s)
File "/opt/conda/lib/python3.6/json/decoder.py", line 339, in decode
obj, end = self.raw_decode(s, idx=_w(s, 0).end())
File "/opt/conda/lib/python3.6/json/decoder.py", line 357, in raw_decode
raise JSONDecodeError("Expecting value", s, err.value) from None
json.decoder.JSONDecodeError: Expecting value: line 1 column 2 (char 1)
```
i debug the variable of resolved_vocab_files, has the same value:
```
{'added_tokens_file': '/home/zhoushengkai/script/NLP/pytorch-transformers/pytorch_transformers/vocab_files/bert-base-chinese-vocab.txt', 'special_tokens_map_file': '/home/zhoushengkai/script/NLP/pytorch-transformers/pytorch_transformers/vocab_files/bert-base-chinese-vocab.txt', 'vocab_file': '/home/zhoushengkai/script/NLP/pytorch-transformers/pytorch_transformers/vocab_files/bert-base-chinese-vocab.txt'}
```
| 07-25-2019 09:44:26 | 07-25-2019 09:44:26 | Had the same issue when passing the exact path of the vocabulary file. Fixed it by just passing the name of the directory that contains the vocabulary file (in my case it was `vocab.txt`).<|||||>Good catch.
For non-BPE models with a single vocabulary file (Bert, XLNet, Transformer-XL) we can fix this workflow so you can provide a direct path.
Updating this. |
transformers | 900 | closed | SpanBERT support | Hi,
I think the new *SpanBERT* model should also be supported in `pytorch-transformers` 😅
> We present SpanBERT, a pre-training method that is designed to better represent and predict spans of text.
Paper can be found [here](https://arxiv.org/abs/1907.10529).
Model is currently not released yet, I'll update this issue here whenever the model is available :) | 07-25-2019 09:02:22 | 07-25-2019 09:02:22 | are we going to get this? :) thanks :)<|||||>Fyi https://github.com/mandarjoshi90/coref#pretrained-coreference-models describes how to obtain the coreference models that should contain SpanBERT.
<|||||>@ArneBinder Thanks for that hint!
I downloaded the *SpanBERT* (base) model. Unfortunately, the TF checkpoint conversion throws the following error message:
```bash
INFO:pytorch_transformers.modeling_bert:Loading TF weight width_scores/output_weights/Adam_1 with shape [3000, 1]
INFO:pytorch_transformers.modeling_bert:Skipping antecedent_distance_emb
Traceback (most recent call last):
File "/usr/local/bin/pytorch_transformers", line 11, in <module>
load_entry_point('pytorch-transformers', 'console_scripts', 'pytorch_transformers')()
File "/mnt/pytorch-transformers/pytorch_transformers/__main__.py", line 30, in main
convert_tf_checkpoint_to_pytorch(TF_CHECKPOINT, TF_CONFIG, PYTORCH_DUMP_OUTPUT)
File "/mnt/pytorch-transformers/pytorch_transformers/convert_tf_checkpoint_to_pytorch.py", line 36, in convert_tf_checkpoint_to_pytorch
load_tf_weights_in_bert(model, config, tf_checkpoint_path)
File "/mnt/pytorch-transformers/pytorch_transformers/modeling_bert.py", line 111, in load_tf_weights_in_bert
assert pointer.shape == array.shape
File "/usr/local/lib/python3.6/dist-packages/torch/nn/modules/module.py", line 591, in __getattr__
type(self).__name__, name))
AttributeError: 'BertForPreTraining' object has no attribute 'shape'
```
I think some variables must be skipped, so a debugging session is unavoidable 😅 <|||||>Hi @stefan-it, the SpanBERT authors shared their (~`pytorch-transformers`-compatible) weights with us, so if you'd be interested we can send them your way so you can experiment/integrate them here.
Let me know!<|||||>@julien-c this would be awesome 🤗 I would really like to do some experiments (mainly NER and PoS tagging) - would be great if you can share the weights (my mail is `[email protected]`) - thank you in advance :heart: <|||||>Hi @julien-c, I would also like to receive the spanbert pytorch-compatible weights for semantic tasks like coref. could you send it to me too? my mail is [email protected]. many thanks.<|||||>You can have a look here, the official implementation has just been released: https://github.com/facebookresearch/SpanBERT<|||||>Well, two preliminary experiments (SpanBERT base) on CoNLL-2003 show a difference of ~7.8% compared to a BERT (base, cased) model 😱 So maybe this has something to do with the named entity masking 🤔 But I'll investigate that further this weekend...<|||||>Update on that: I tried SpanBERT for PoS tagging and the results are pretty close to DistilBERT. Here's one run over the Universal Dependencies v1.2:
| Model | Dev | Test
| ---------------------------------------------------------- | --------- | ---------
| RoBERTa (large) | **97.80** | **97.75**
| SpanBERT (large) | 96.48 | 96.61
| BERT (large, cased) | 97.35 | 97.20
| DistilBERT (uncased) | 96.64 | 96.70
| [Plank et. al (2016)](https://arxiv.org/abs/1604.05529) | - | 95.52
| [Yasunaga et. al (2017)](https://arxiv.org/abs/1711.04903) | - | 95.82<|||||>This issue has been automatically marked as stale because it has not had recent activity. It will be closed if no further activity occurs. Thank you for your contributions.
|
transformers | 899 | closed | Fixed import to use torchscript flag. | 07-25-2019 08:56:42 | 07-25-2019 08:56:42 | # [Codecov](https://codecov.io/gh/huggingface/pytorch-transformers/pull/899?src=pr&el=h1) Report
> Merging [#899](https://codecov.io/gh/huggingface/pytorch-transformers/pull/899?src=pr&el=desc) into [master](https://codecov.io/gh/huggingface/pytorch-transformers/commit/067923d3267325f525f4e46f357360c191ba562e?src=pr&el=desc) will **not change** coverage.
> The diff coverage is `n/a`.
[](https://codecov.io/gh/huggingface/pytorch-transformers/pull/899?src=pr&el=tree)
```diff
@@ Coverage Diff @@
## master #899 +/- ##
=======================================
Coverage 79.03% 79.03%
=======================================
Files 34 34
Lines 6234 6234
=======================================
Hits 4927 4927
Misses 1307 1307
```
------
[Continue to review full report at Codecov](https://codecov.io/gh/huggingface/pytorch-transformers/pull/899?src=pr&el=continue).
> **Legend** - [Click here to learn more](https://docs.codecov.io/docs/codecov-delta)
> `Δ = absolute <relative> (impact)`, `ø = not affected`, `? = missing data`
> Powered by [Codecov](https://codecov.io/gh/huggingface/pytorch-transformers/pull/899?src=pr&el=footer). Last update [067923d...e1e2ab3](https://codecov.io/gh/huggingface/pytorch-transformers/pull/899?src=pr&el=lastupdated). Read the [comment docs](https://docs.codecov.io/docs/pull-request-comments).
|
|
transformers | 898 | closed | fp16 is still has the problem | hello, as mentioned in #868 and #871 ,fp16 is broken, and you have fixed in the master once, but i am afraid it also has problem, DP is need after the amp.initialize() too. i review the code of apex, found that amp do not support the model of parallel type:
```
def check_models(models):
for model in models:
parallel_type = None
if isinstance(model, torch.nn.parallel.DistributedDataParallel):
parallel_type = "torch.nn.parallel.DistributedDataParallel"
if isinstance(model, apex_DDP):
parallel_type = "apex.parallel.DistributedDataParallel"
if isinstance(model, torch.nn.parallel.DataParallel):
parallel_type = "torch.nn.parallel.DataParallel"
if parallel_type is not None:
raise RuntimeError("Incoming model is an instance of {}. ".format(parallel_type) +
"Parallel wrappers should only be applied to the model(s) AFTER \n"
"the model(s) have been returned from amp.initialize.")
```
and the other question is that after i fixed the problem and can run with fp16, but i found it speed the same time and gpu memory, why?
| 07-25-2019 07:02:21 | 07-25-2019 07:02:21 | yes, I fixed it in #896 and waiting for author to merge..
but I still can't figure out why fp16 didn't save memory and didn't speed up....<|||||>Merged<|||||>close |
transformers | 897 | closed | Fix FileNotFoundError when running on SQuAD-v1.1 | At "utils_squad_evaluate.py" line 291, no matter version_2_with_negative is True or False, it tries to load "output_null_log_odds_file" which is not saved when version_2_with_negative is False. | 07-25-2019 06:10:57 | 07-25-2019 06:10:57 | Duplicate to #882 |
transformers | 896 | closed | fix multi-gpu training bug when using fp16 | multi-gpu training (orch.nn.DataParallel) should also be after apex fp16 initialization. | 07-25-2019 05:15:19 | 07-25-2019 05:15:19 | Thanks, can you update `run_squad` similarly?<|||||>> Thanks, can you update `run_squad` similarly?
updated already.<|||||># [Codecov](https://codecov.io/gh/huggingface/pytorch-transformers/pull/896?src=pr&el=h1) Report
> Merging [#896](https://codecov.io/gh/huggingface/pytorch-transformers/pull/896?src=pr&el=desc) into [master](https://codecov.io/gh/huggingface/pytorch-transformers/commit/067923d3267325f525f4e46f357360c191ba562e?src=pr&el=desc) will **not change** coverage.
> The diff coverage is `n/a`.
[](https://codecov.io/gh/huggingface/pytorch-transformers/pull/896?src=pr&el=tree)
```diff
@@ Coverage Diff @@
## master #896 +/- ##
=======================================
Coverage 79.03% 79.03%
=======================================
Files 34 34
Lines 6234 6234
=======================================
Hits 4927 4927
Misses 1307 1307
```
------
[Continue to review full report at Codecov](https://codecov.io/gh/huggingface/pytorch-transformers/pull/896?src=pr&el=continue).
> **Legend** - [Click here to learn more](https://docs.codecov.io/docs/codecov-delta)
> `Δ = absolute <relative> (impact)`, `ø = not affected`, `? = missing data`
> Powered by [Codecov](https://codecov.io/gh/huggingface/pytorch-transformers/pull/896?src=pr&el=footer). Last update [067923d...f0aeb7a](https://codecov.io/gh/huggingface/pytorch-transformers/pull/896?src=pr&el=lastupdated). Read the [comment docs](https://docs.codecov.io/docs/pull-request-comments).
<|||||>Thanks a lot! |
transformers | 895 | closed | fix a bug of saving added tokens | Refer to the code that loads `added_tokens.json`:
`added_tok_encoder = json.load(open(added_tokens_file, encoding="utf-8"))`
We can see that `added_tokens_encoder` should be saved in `added_tokens.json`. But the original code saved `added_tokens_decoder`.
| 07-25-2019 04:50:27 | 07-25-2019 04:50:27 | # [Codecov](https://codecov.io/gh/huggingface/pytorch-transformers/pull/895?src=pr&el=h1) Report
> Merging [#895](https://codecov.io/gh/huggingface/pytorch-transformers/pull/895?src=pr&el=desc) into [master](https://codecov.io/gh/huggingface/pytorch-transformers/commit/067923d3267325f525f4e46f357360c191ba562e?src=pr&el=desc) will **not change** coverage.
> The diff coverage is `0%`.
[](https://codecov.io/gh/huggingface/pytorch-transformers/pull/895?src=pr&el=tree)
```diff
@@ Coverage Diff @@
## master #895 +/- ##
=======================================
Coverage 79.03% 79.03%
=======================================
Files 34 34
Lines 6234 6234
=======================================
Hits 4927 4927
Misses 1307 1307
```
| [Impacted Files](https://codecov.io/gh/huggingface/pytorch-transformers/pull/895?src=pr&el=tree) | Coverage Δ | |
|---|---|---|
| [pytorch\_transformers/tokenization\_utils.py](https://codecov.io/gh/huggingface/pytorch-transformers/pull/895/diff?src=pr&el=tree#diff-cHl0b3JjaF90cmFuc2Zvcm1lcnMvdG9rZW5pemF0aW9uX3V0aWxzLnB5) | `86.53% <0%> (ø)` | :arrow_up: |
------
[Continue to review full report at Codecov](https://codecov.io/gh/huggingface/pytorch-transformers/pull/895?src=pr&el=continue).
> **Legend** - [Click here to learn more](https://docs.codecov.io/docs/codecov-delta)
> `Δ = absolute <relative> (impact)`, `ø = not affected`, `? = missing data`
> Powered by [Codecov](https://codecov.io/gh/huggingface/pytorch-transformers/pull/895?src=pr&el=footer). Last update [067923d...c9a7b29](https://codecov.io/gh/huggingface/pytorch-transformers/pull/895?src=pr&el=lastupdated). Read the [comment docs](https://docs.codecov.io/docs/pull-request-comments).
<|||||>Thanks, that was fixed in #893 |
transformers | 894 | closed | Sequence length more than 512 | Hi,
My dataset has sequence with more than 512 words and when use wordpieces sequence length goes beyond 512. How to handle this issue with BERT ?
Regards
Tapas | 07-25-2019 03:11:39 | 07-25-2019 03:11:39 | https://github.com/google-research/bert/issues/27#issuecomment-435265194<|||||>This issue has been automatically marked as stale because it has not had recent activity. It will be closed if no further activity occurs. Thank you for your contributions.
|
transformers | 893 | closed | make save_pretrained do the right thing with added tokens | right now it's dumping the *decoder* when it should be dumping the *encoder*. and then (for obvious reasons) you get an error when you try to load "from_pretrained" using that dump.
this PR fixes that. | 07-24-2019 23:56:03 | 07-24-2019 23:56:03 | # [Codecov](https://codecov.io/gh/huggingface/pytorch-transformers/pull/893?src=pr&el=h1) Report
> Merging [#893](https://codecov.io/gh/huggingface/pytorch-transformers/pull/893?src=pr&el=desc) into [master](https://codecov.io/gh/huggingface/pytorch-transformers/commit/067923d3267325f525f4e46f357360c191ba562e?src=pr&el=desc) will **not change** coverage.
> The diff coverage is `0%`.
[](https://codecov.io/gh/huggingface/pytorch-transformers/pull/893?src=pr&el=tree)
```diff
@@ Coverage Diff @@
## master #893 +/- ##
=======================================
Coverage 79.03% 79.03%
=======================================
Files 34 34
Lines 6234 6234
=======================================
Hits 4927 4927
Misses 1307 1307
```
| [Impacted Files](https://codecov.io/gh/huggingface/pytorch-transformers/pull/893?src=pr&el=tree) | Coverage Δ | |
|---|---|---|
| [pytorch\_transformers/tokenization\_utils.py](https://codecov.io/gh/huggingface/pytorch-transformers/pull/893/diff?src=pr&el=tree#diff-cHl0b3JjaF90cmFuc2Zvcm1lcnMvdG9rZW5pemF0aW9uX3V0aWxzLnB5) | `86.53% <0%> (ø)` | :arrow_up: |
------
[Continue to review full report at Codecov](https://codecov.io/gh/huggingface/pytorch-transformers/pull/893?src=pr&el=continue).
> **Legend** - [Click here to learn more](https://docs.codecov.io/docs/codecov-delta)
> `Δ = absolute <relative> (impact)`, `ø = not affected`, `? = missing data`
> Powered by [Codecov](https://codecov.io/gh/huggingface/pytorch-transformers/pull/893?src=pr&el=footer). Last update [067923d...ae152ce](https://codecov.io/gh/huggingface/pytorch-transformers/pull/893?src=pr&el=lastupdated). Read the [comment docs](https://docs.codecov.io/docs/pull-request-comments).
<|||||>Indeed, thanks Joel! |
transformers | 892 | closed | How to add new special token | I noticed the never_split functionality is no longer used to keep track of special tokens to never split on. If I wanted to add a new special token like '[NEW]' so the tokenizer never splits it, how should I go about doing that? (I've already manually added it to vocab.txt by replacing an unused token with [NEW]. Now I just need to not split it) | 07-24-2019 23:20:38 | 07-24-2019 23:20:38 | How did you add a new vocab.txt file? <|||||>I actually figured it out. I manually replaced one of the unused tokens in the vocab file with [NEW] and added "additiona_special_tokens": "[NEW]" to the special_tokens.json file in the same directory as the vocab.txt file. It works, but I realized that adding new tokens without the ability to do further pretraining isn't all that useful, especially given small dataset size. I decided not to do it. |
transformers | 891 | closed | BERT: run_squad.py falling over after eval | I'm having an issue fine-tuning BERT with run.squad.py, as it falls over at the end of the evaluation stage. I'm fine tuning on SQuAD v1.1. Has anyone else encountered the same issue, or is able to point out where I'm going wrong?
`python run_squad.py \
--model_type bert \
--model_name_or_path bert-base-uncased \
--do_train \
--do_eval \
--do_lower_case \
--overwrite_output_dir \
--train_file $TRAIN_FILE \
--predict_file $PREDICT_FILE \
--learning_rate 2e-5 \
--num_train_epochs 1.0 \
--max_seq_length 384 \
--per_gpu_eval_batch_size=12 \
--per_gpu_train_batch_size=12 \
--output_dir /content/SQuAD_for_bert/models/bert_base_uncased_finetuned_script/`
Which completes fine-tuning the model, and then fits the eval set but returns:
`Evaluating: 100% 257/257 [01:29<00:00, 2.86it/s]
07/22/2019 02:17:18 - INFO - utils_squad - Writing predictions to: /content/SQuAD_for_bert/models/bert_base_uncased_finetuned_script/predictions_.json
07/22/2019 02:17:18 - INFO - utils_squad - Writing nbest to: /content/SQuAD_for_bert/models/bert_base_uncased_finetuned_script/nbest_predictions_.json
Traceback (most recent call last):
File "run_squad.py", line 521, in <module>
main()
File "run_squad.py", line 510, in main
result = evaluate(args, model, tokenizer, prefix=global_step)
File "run_squad.py", line 257, in evaluate
results = evaluate_on_squad(evaluate_options)
File "/content/SQuAD_for_bert/utils_squad_evaluate.py", line 291, in main
with open(OPTS.na_prob_file) as f:
FileNotFoundError: [Errno 2] No such file or directory: '/content/SQuAD_for_bert/models/bert_base_uncased_finetuned_script/null_odds_.json'`
(Running this in Google Colab - in case that is of any relevance).
Any help would be greatly appreciated - thanks! | 07-24-2019 23:19:46 | 07-24-2019 23:19:46 | Fixed in #882 |
transformers | 890 | closed | PreTrainedTokenizer.from_pretrained should be more general | I'm trying to implement a general interface to any of these transformer models in AllenNLP. I would love to be able to do something like `PreTrainedTokenizer.from_pretrained(model_name)`, and have this work for any model name across any of your implemented models. It looks like what needs to happen for this is to detect which underlying model is being requested, and pass off to that class's `_from_pretrained` method. Does this make sense? In particular, I think the thing that needs to change is here: https://github.com/huggingface/pytorch-transformers/blob/067923d3267325f525f4e46f357360c191ba562e/pytorch_transformers/tokenization_utils.py#L149-L151
It could be changed to something like:
```python
@classmethod
def from_pretrained(cls, pretrained_model_name_or_path, *inputs, **kwargs):
if 'bert' in pretrained_model_name_or_path:
# import BertTokenizer here to avoid circular dependencies
return BertTokenizer._from_pretrained(pretrained_model_name_or_path, *inputs, **kwargs)
# other cases here
# default to existing behavior if we can't detect the model class
return cls._from_pretrained(*inputs, **kwargs)
```
If this looks right to you, I can put together an initial PR for this.
It would be super helpful if there were similar functionality for models, too, but I haven't gotten far enough yet to worry about how exactly that would work =). | 07-24-2019 20:56:49 | 07-24-2019 20:56:49 | Yes, this is a nice idea, I was thinking about implementing something like this for another reason (simplifying the task of maintaining `torch.hub` configuration files).
Regarding the library architecture, I think it's better to make a new (very simple) class, something like `AutoTokenizer` in a new file `tokenizer_auto.py` deriving from `PreTrainedTokenizer` (no need to avoid circular dependencies in this case).
Then the idea would be to make a new file `modeling_auto.py` as well with something like `AutoModel`, pretty much like `AutoTokenizer`, and `AutoModelForSequenceClassification`, `AutoModelForQuestionAnswering` that would encapsulate standard architectures on top of each model.
Maybe the `AutoXXX` is not the best name, also thought about `GenericXXX` or `UniversalXXX` but they convey meanings that could be misleading.<|||||>`{Generic,Universal,Auto}` Tokenizer and Model interfaces would be awesome (I'm also highly interested in that, as I'm currently working on Flair to add support for all six architectures) :heart: <|||||>Ok, I've hacked something together for an internal hackathon this week. I'll see if I can pick this up the way you suggest next week, if no one else gets to it first. I also don't know much about your PR requirements, so if someone who's more familiar with this repo wants to pick it up, I wouldn't complain =).<|||||>This issue has been automatically marked as stale because it has not had recent activity. It will be closed if no further activity occurs. Thank you for your contributions.
|
transformers | 889 | closed | Increased number of hidden states returned from transformers in latest release | I noticed an (undocumented?) change in the latest release: namely that transformers now include the pre-encoder input vector in the list returned when `output_hidden_states` is True.
For example, the `hidden_states` output from `BertEncoder` now returns a length-13 list of tensors, whereas it used to return a length-12 list for each of Bert's 12 encoder modules
The change does seem to be intentional, as we have the following line in the tests
https://github.com/huggingface/pytorch-transformers/blob/067923d3267325f525f4e46f357360c191ba562e/pytorch_transformers/tests/modeling_common_test.py#L244
Personally I'm not affected, I just wanted to double-check that this was intended behavior | 07-24-2019 19:57:32 | 07-24-2019 19:57:32 | Yes, this is the initial embedding layer (i.e. this is layers "0" through 12 or 24). There are a number of small changes that haven't been migrated into the documentation yet. |
transformers | 888 | closed | Update docs for parameter rename | small fix: OpenAIGPTLMHeadModel now accepts `labels` instead of `lm_labels`
| 07-24-2019 18:30:21 | 07-24-2019 18:30:21 | # [Codecov](https://codecov.io/gh/huggingface/pytorch-transformers/pull/888?src=pr&el=h1) Report
> Merging [#888](https://codecov.io/gh/huggingface/pytorch-transformers/pull/888?src=pr&el=desc) into [master](https://codecov.io/gh/huggingface/pytorch-transformers/commit/067923d3267325f525f4e46f357360c191ba562e?src=pr&el=desc) will **not change** coverage.
> The diff coverage is `n/a`.
[](https://codecov.io/gh/huggingface/pytorch-transformers/pull/888?src=pr&el=tree)
```diff
@@ Coverage Diff @@
## master #888 +/- ##
=======================================
Coverage 79.03% 79.03%
=======================================
Files 34 34
Lines 6234 6234
=======================================
Hits 4927 4927
Misses 1307 1307
```
| [Impacted Files](https://codecov.io/gh/huggingface/pytorch-transformers/pull/888?src=pr&el=tree) | Coverage Δ | |
|---|---|---|
| [pytorch\_transformers/modeling\_openai.py](https://codecov.io/gh/huggingface/pytorch-transformers/pull/888/diff?src=pr&el=tree#diff-cHl0b3JjaF90cmFuc2Zvcm1lcnMvbW9kZWxpbmdfb3BlbmFpLnB5) | `74.76% <ø> (ø)` | :arrow_up: |
------
[Continue to review full report at Codecov](https://codecov.io/gh/huggingface/pytorch-transformers/pull/888?src=pr&el=continue).
> **Legend** - [Click here to learn more](https://docs.codecov.io/docs/codecov-delta)
> `Δ = absolute <relative> (impact)`, `ø = not affected`, `? = missing data`
> Powered by [Codecov](https://codecov.io/gh/huggingface/pytorch-transformers/pull/888?src=pr&el=footer). Last update [067923d...66b15f7](https://codecov.io/gh/huggingface/pytorch-transformers/pull/888?src=pr&el=lastupdated). Read the [comment docs](https://docs.codecov.io/docs/pull-request-comments).
<|||||>Yes, thanks @rococode! |
Subsets and Splits
No community queries yet
The top public SQL queries from the community will appear here once available.