title
stringlengths 1
100
| titleSlug
stringlengths 3
77
| Java
int64 0
1
| Python3
int64 1
1
| content
stringlengths 28
44.4k
| voteCount
int64 0
3.67k
| question_content
stringlengths 65
5k
| question_hints
stringclasses 970
values |
---|---|---|---|---|---|---|---|
[Java/Python 3] Sort by trimmed string. | query-kth-smallest-trimmed-number | 1 | 1 | \n```java\n public int[] smallestTrimmedNumbers(String[] nums, int[][] queries) {\n int n = queries.length, j = 0;\n int[] ans = new int[n];\n Map<Integer, String[][]> trimmed = new HashMap<>();\n for (int[] q : queries) {\n int k = q[0] - 1;\n int trim = q[1];\n if (!trimmed.containsKey(trim)) {\n String[][] arr = new String[nums.length][2];\n int i = 0;\n for (String num : nums) {\n int sz = num.length();\n arr[i] = new String[]{num.substring(sz - trim), "" + i++};\n }\n Arrays.sort(arr, Comparator.comparing(a -> a[0]));\n trimmed.put(trim, arr);\n }\n ans[j++] = Integer.parseInt(trimmed.get(trim)[k][1]);\n }\n return ans; \n }\n```\n\n```python\n def smallestTrimmedNumbers(self, nums: List[str], queries: List[List[int]]) -> List[int]:\n ans, trimmed = [], {}\n for k, trim in queries:\n trimmed.setdefault(trim, sorted([(num[-trim :], i) for i, num in enumerate(nums)]))\n ans.append(trimmed[trim][k - 1][1])\n return ans\n``` | 14 | You are given a **0-indexed** array of strings `nums`, where each string is of **equal length** and consists of only digits.
You are also given a **0-indexed** 2D integer array `queries` where `queries[i] = [ki, trimi]`. For each `queries[i]`, you need to:
* **Trim** each number in `nums` to its **rightmost** `trimi` digits.
* Determine the **index** of the `kith` smallest trimmed number in `nums`. If two trimmed numbers are equal, the number with the **lower** index is considered to be smaller.
* Reset each number in `nums` to its original length.
Return _an array_ `answer` _of the same length as_ `queries`, _where_ `answer[i]` _is the answer to the_ `ith` _query._
**Note**:
* To trim to the rightmost `x` digits means to keep removing the leftmost digit, until only `x` digits remain.
* Strings in `nums` may contain leading zeros.
**Example 1:**
**Input:** nums = \[ "102 ", "473 ", "251 ", "814 "\], queries = \[\[1,1\],\[2,3\],\[4,2\],\[1,2\]\]
**Output:** \[2,2,1,0\]
**Explanation:**
1. After trimming to the last digit, nums = \[ "2 ", "3 ", "1 ", "4 "\]. The smallest number is 1 at index 2.
2. Trimmed to the last 3 digits, nums is unchanged. The 2nd smallest number is 251 at index 2.
3. Trimmed to the last 2 digits, nums = \[ "02 ", "73 ", "51 ", "14 "\]. The 4th smallest number is 73.
4. Trimmed to the last 2 digits, the smallest number is 2 at index 0.
Note that the trimmed number "02 " is evaluated as 2.
**Example 2:**
**Input:** nums = \[ "24 ", "37 ", "96 ", "04 "\], queries = \[\[2,1\],\[2,2\]\]
**Output:** \[3,0\]
**Explanation:**
1. Trimmed to the last digit, nums = \[ "4 ", "7 ", "6 ", "4 "\]. The 2nd smallest number is 4 at index 3.
There are two occurrences of 4, but the one at index 0 is considered smaller than the one at index 3.
2. Trimmed to the last 2 digits, nums is unchanged. The 2nd smallest number is 24.
**Constraints:**
* `1 <= nums.length <= 100`
* `1 <= nums[i].length <= 100`
* `nums[i]` consists of only digits.
* All `nums[i].length` are **equal**.
* `1 <= queries.length <= 100`
* `queries[i].length == 2`
* `1 <= ki <= nums.length`
* `1 <= trimi <= nums[i].length`
**Follow up:** Could you use the **Radix Sort Algorithm** to solve this problem? What will be the complexity of that solution? | null |
Heap of size K for Kth smallest number|Python3|Similar to Kth smallest Element|Easy to understand | query-kth-smallest-trimmed-number | 0 | 1 | \nPlease upvote if you liked this\n\n**Note** :We have stored -ve index , because if we have case like in heap , we have [[4,2],[4,3]] . We want to return 3 , but if we perfrom heapq.heappop(). We will get result 2.\n```\n\nclass Solution:\n def smallestTrimmedNumbers(self, nums: List[str], queries: List[List[int]]) -> List[int]:\n answer = []\n for x,y in queries:\n li = []\n heapq.heapify(li)\n for i in range(len(nums)):\n \n n1 = nums[i][len(nums[i])-y:len(nums[i])]\n n1 = int(n1)\n \n if len(li) < x:\n heapq.heappush(li,[-1*n1,-1*i])\n \n else:\n if n1<-1*li[0][0]:\n heapq.heappop(li)\n heapq.heappush(li,[-1*n1,-1*i])\n \n answer.append(-1*heapq.heappop(li)[1]) \n \n \n \n \n \n return answer\n \n``` | 3 | You are given a **0-indexed** array of strings `nums`, where each string is of **equal length** and consists of only digits.
You are also given a **0-indexed** 2D integer array `queries` where `queries[i] = [ki, trimi]`. For each `queries[i]`, you need to:
* **Trim** each number in `nums` to its **rightmost** `trimi` digits.
* Determine the **index** of the `kith` smallest trimmed number in `nums`. If two trimmed numbers are equal, the number with the **lower** index is considered to be smaller.
* Reset each number in `nums` to its original length.
Return _an array_ `answer` _of the same length as_ `queries`, _where_ `answer[i]` _is the answer to the_ `ith` _query._
**Note**:
* To trim to the rightmost `x` digits means to keep removing the leftmost digit, until only `x` digits remain.
* Strings in `nums` may contain leading zeros.
**Example 1:**
**Input:** nums = \[ "102 ", "473 ", "251 ", "814 "\], queries = \[\[1,1\],\[2,3\],\[4,2\],\[1,2\]\]
**Output:** \[2,2,1,0\]
**Explanation:**
1. After trimming to the last digit, nums = \[ "2 ", "3 ", "1 ", "4 "\]. The smallest number is 1 at index 2.
2. Trimmed to the last 3 digits, nums is unchanged. The 2nd smallest number is 251 at index 2.
3. Trimmed to the last 2 digits, nums = \[ "02 ", "73 ", "51 ", "14 "\]. The 4th smallest number is 73.
4. Trimmed to the last 2 digits, the smallest number is 2 at index 0.
Note that the trimmed number "02 " is evaluated as 2.
**Example 2:**
**Input:** nums = \[ "24 ", "37 ", "96 ", "04 "\], queries = \[\[2,1\],\[2,2\]\]
**Output:** \[3,0\]
**Explanation:**
1. Trimmed to the last digit, nums = \[ "4 ", "7 ", "6 ", "4 "\]. The 2nd smallest number is 4 at index 3.
There are two occurrences of 4, but the one at index 0 is considered smaller than the one at index 3.
2. Trimmed to the last 2 digits, nums is unchanged. The 2nd smallest number is 24.
**Constraints:**
* `1 <= nums.length <= 100`
* `1 <= nums[i].length <= 100`
* `nums[i]` consists of only digits.
* All `nums[i].length` are **equal**.
* `1 <= queries.length <= 100`
* `queries[i].length == 2`
* `1 <= ki <= nums.length`
* `1 <= trimi <= nums[i].length`
**Follow up:** Could you use the **Radix Sort Algorithm** to solve this problem? What will be the complexity of that solution? | null |
Python Commented Code ( Insertion + Customization for storing index) | query-kth-smallest-trimmed-number | 0 | 1 | ```\nclass Solution:\n def smallestTrimmedNumbers(self, nums: List[str], queries: List[List[int]]) -> List[int]:\n a=[] #to store answer of queries\n for q in queries: \n ia=q[0] #kth smallest value to be returned\n t=q[1] #trim index\n temp=[]\n for n in nums:\n temp.append(int(n[-1*t:])) #using slicing just take last t elements\n \n temp1=[[temp[0],0]] #another list that will store sorted elements along with index\n for i in range(1,len(temp)):\n key=temp[i]\n j=len(temp1)-1\n while j>=0 and key<temp1[j][0]: #using insertion sort, insert elements to new list also store index\n j-=1\n temp1.insert(j+1,[key,i])\n \n a.append(temp1[ia-1][1]) #append required index element \n \n return a\n``` | 2 | You are given a **0-indexed** array of strings `nums`, where each string is of **equal length** and consists of only digits.
You are also given a **0-indexed** 2D integer array `queries` where `queries[i] = [ki, trimi]`. For each `queries[i]`, you need to:
* **Trim** each number in `nums` to its **rightmost** `trimi` digits.
* Determine the **index** of the `kith` smallest trimmed number in `nums`. If two trimmed numbers are equal, the number with the **lower** index is considered to be smaller.
* Reset each number in `nums` to its original length.
Return _an array_ `answer` _of the same length as_ `queries`, _where_ `answer[i]` _is the answer to the_ `ith` _query._
**Note**:
* To trim to the rightmost `x` digits means to keep removing the leftmost digit, until only `x` digits remain.
* Strings in `nums` may contain leading zeros.
**Example 1:**
**Input:** nums = \[ "102 ", "473 ", "251 ", "814 "\], queries = \[\[1,1\],\[2,3\],\[4,2\],\[1,2\]\]
**Output:** \[2,2,1,0\]
**Explanation:**
1. After trimming to the last digit, nums = \[ "2 ", "3 ", "1 ", "4 "\]. The smallest number is 1 at index 2.
2. Trimmed to the last 3 digits, nums is unchanged. The 2nd smallest number is 251 at index 2.
3. Trimmed to the last 2 digits, nums = \[ "02 ", "73 ", "51 ", "14 "\]. The 4th smallest number is 73.
4. Trimmed to the last 2 digits, the smallest number is 2 at index 0.
Note that the trimmed number "02 " is evaluated as 2.
**Example 2:**
**Input:** nums = \[ "24 ", "37 ", "96 ", "04 "\], queries = \[\[2,1\],\[2,2\]\]
**Output:** \[3,0\]
**Explanation:**
1. Trimmed to the last digit, nums = \[ "4 ", "7 ", "6 ", "4 "\]. The 2nd smallest number is 4 at index 3.
There are two occurrences of 4, but the one at index 0 is considered smaller than the one at index 3.
2. Trimmed to the last 2 digits, nums is unchanged. The 2nd smallest number is 24.
**Constraints:**
* `1 <= nums.length <= 100`
* `1 <= nums[i].length <= 100`
* `nums[i]` consists of only digits.
* All `nums[i].length` are **equal**.
* `1 <= queries.length <= 100`
* `queries[i].length == 2`
* `1 <= ki <= nums.length`
* `1 <= trimi <= nums[i].length`
**Follow up:** Could you use the **Radix Sort Algorithm** to solve this problem? What will be the complexity of that solution? | null |
Python O(m * n) solution based on O(m) counting sort | query-kth-smallest-trimmed-number | 0 | 1 | Algorithm itself is simple to understand, using counting-based sort instead of comparison-based sort to get rid of the O(lgm) part in time complexity. \n\nReferenced the super concise implementation from this post: https://leetcode.com/problems/query-kth-smallest-trimmed-number/discuss/2292577/Python-O(mnlogm).-Sort-among-single-digit-instead-of-entire-suffix-for-each-rank\n\nP.S. This algorithm has better asymptotic time complexity than most solutions shared, while I never tried them myself, I suspect "slower" sorting like insertion sort might perform better at small input data in real world (e.g. <= 100 for this problem).\n\n```\nclass Solution:\n def smallestTrimmedNumbers(self, nums: List[str], queries: List[List[int]]) -> List[int]:\n def countingSort(indices, pos):\n count = [0] * 10\n for idx in indices:\n count[ord(nums[idx][pos]) - ord(\'0\')] += 1\n start_pos = list(accumulate([0] + count, add))\n result = [None] * len(indices)\n for idx in indices:\n digit = ord(nums[idx][pos]) - ord(\'0\')\n result[start_pos[digit]] = idx\n start_pos[digit] += 1\n return result\n \n n = len(nums)\n m = len(nums[0])\n suffix_ordered = [list(range(n))]\n for i in range(m - 1, -1, -1):\n suffix_ordered.append(countingSort(suffix_ordered[-1], i))\n return [suffix_ordered[t][k-1] for k, t in queries]\n```\n | 4 | You are given a **0-indexed** array of strings `nums`, where each string is of **equal length** and consists of only digits.
You are also given a **0-indexed** 2D integer array `queries` where `queries[i] = [ki, trimi]`. For each `queries[i]`, you need to:
* **Trim** each number in `nums` to its **rightmost** `trimi` digits.
* Determine the **index** of the `kith` smallest trimmed number in `nums`. If two trimmed numbers are equal, the number with the **lower** index is considered to be smaller.
* Reset each number in `nums` to its original length.
Return _an array_ `answer` _of the same length as_ `queries`, _where_ `answer[i]` _is the answer to the_ `ith` _query._
**Note**:
* To trim to the rightmost `x` digits means to keep removing the leftmost digit, until only `x` digits remain.
* Strings in `nums` may contain leading zeros.
**Example 1:**
**Input:** nums = \[ "102 ", "473 ", "251 ", "814 "\], queries = \[\[1,1\],\[2,3\],\[4,2\],\[1,2\]\]
**Output:** \[2,2,1,0\]
**Explanation:**
1. After trimming to the last digit, nums = \[ "2 ", "3 ", "1 ", "4 "\]. The smallest number is 1 at index 2.
2. Trimmed to the last 3 digits, nums is unchanged. The 2nd smallest number is 251 at index 2.
3. Trimmed to the last 2 digits, nums = \[ "02 ", "73 ", "51 ", "14 "\]. The 4th smallest number is 73.
4. Trimmed to the last 2 digits, the smallest number is 2 at index 0.
Note that the trimmed number "02 " is evaluated as 2.
**Example 2:**
**Input:** nums = \[ "24 ", "37 ", "96 ", "04 "\], queries = \[\[2,1\],\[2,2\]\]
**Output:** \[3,0\]
**Explanation:**
1. Trimmed to the last digit, nums = \[ "4 ", "7 ", "6 ", "4 "\]. The 2nd smallest number is 4 at index 3.
There are two occurrences of 4, but the one at index 0 is considered smaller than the one at index 3.
2. Trimmed to the last 2 digits, nums is unchanged. The 2nd smallest number is 24.
**Constraints:**
* `1 <= nums.length <= 100`
* `1 <= nums[i].length <= 100`
* `nums[i]` consists of only digits.
* All `nums[i].length` are **equal**.
* `1 <= queries.length <= 100`
* `queries[i].length == 2`
* `1 <= ki <= nums.length`
* `1 <= trimi <= nums[i].length`
**Follow up:** Could you use the **Radix Sort Algorithm** to solve this problem? What will be the complexity of that solution? | null |
asy-4-line-soln👁️ | well-explained✅ | Microsoft🔥 | minimum-deletions-to-make-array-divisible | 0 | 1 | # Please upvote if it is helpful ^_^\n*6Companies30days #ReviseWithArsh Challenge 2023\nDay3\nQ14. Deletions to make an array divisible.*\n\n**Intuition: *gcd***\n\n\n# Code\n**Python3:**\n```\nclass Solution:\n def minOperations(self, nums: List[int], numsDivide: List[int]) -> int:\n gc=0\n for i in numsDivide:\n gc=int(math.gcd(gc,i))\n nums.sort()\n for i in range(len(nums)):\n if gc%nums[i]==0:\n return i\n return -1\n\n``` | 1 | You are given two positive integer arrays `nums` and `numsDivide`. You can delete any number of elements from `nums`.
Return _the **minimum** number of deletions such that the **smallest** element in_ `nums` _**divides** all the elements of_ `numsDivide`. If this is not possible, return `-1`.
Note that an integer `x` divides `y` if `y % x == 0`.
**Example 1:**
**Input:** nums = \[2,3,2,4,3\], numsDivide = \[9,6,9,3,15\]
**Output:** 2
**Explanation:**
The smallest element in \[2,3,2,4,3\] is 2, which does not divide all the elements of numsDivide.
We use 2 deletions to delete the elements in nums that are equal to 2 which makes nums = \[3,4,3\].
The smallest element in \[3,4,3\] is 3, which divides all the elements of numsDivide.
It can be shown that 2 is the minimum number of deletions needed.
**Example 2:**
**Input:** nums = \[4,3,6\], numsDivide = \[8,2,6,10\]
**Output:** -1
**Explanation:**
We want the smallest element in nums to divide all the elements of numsDivide.
There is no way to delete elements from nums to allow this.
**Constraints:**
* `1 <= nums.length, numsDivide.length <= 105`
* `1 <= nums[i], numsDivide[i] <= 109` | null |
[Java/Python 3] 2 methods about GCD w/ brief explanation and analysis. | minimum-deletions-to-make-array-divisible | 1 | 1 | "divide all numbers in `numsDivide`" implies "divide the gcd of `numsDivide`". Therefore, we can design an algorithm as follows:\n\n----\n\n**Method 1:**\n1. Compute the gcd of `numsDivide`;\n2. Traverse `nums` to find the smallest number that can divide the gcd;; If fails, return -1\n3. Traverse `nums` to count how many numbers are less than the afore-mentioned smallest number.\n\n```java\n public int minOperations(int[] nums, int[] numsDivide) {\n int g = IntStream.of(numsDivide).reduce(numsDivide[0], (a, b) -> gcd(a, b));\n int minOp = 0, smallest = Integer.MAX_VALUE;\n for (int num : nums) {\n if (g % num == 0) {\n smallest = Math.min(smallest, num);\n }\n }\n for (int num : nums) {\n if (num < smallest) {\n ++minOp;\n }\n }\n return smallest == Integer.MAX_VALUE ? -1 : minOp;\n }\n private int gcd(int a, int b) {\n while (b > 0) {\n int tmp = a;\n a = b;\n b = tmp % b;\n }\n return a;\n }\n```\n\n```python\n def minOperations(self, nums: List[int], numsDivide: List[int]) -> int:\n \n def gcd(a, b) -> int:\n while b > 0:\n a, b = b, a % b\n return a\n \n g = functools.reduce(gcd, numsDivide)\n smallest = min([num for num in nums if g % num == 0], default = inf)\n return -1 if smallest == inf else sum(smallest > num for num in nums)\n```\n\n**Analysis:**\n\nTime: `O(m + n + log(max(numsDivide)))`, \nspace: Java code - `O(1)`, Python 3 code -`O(m)`, where `m = nums.length, n = numsDivide.length`.\n\n----\n\n\n\n**Method 2: Sort**\n\n *Sort nums:*\n \n1. Compute the gcd of `numsDivide`;\n2. Sort `nums`;\n3. Traverse `nums` to check one by one till locating the smallest number that can divide the gcd; If fails, return -1.\n\n\n\n```java\n public int minOperations(int[] nums, int[] numsDivide) {\n int g = IntStream.of(numsDivide).reduce(numsDivide[0], (a, b) -> gcd(a, b));\n int minOp = 0;\n Arrays.sort(nums);\n for (int i = 0; i < nums.length; ++i) {\n if (g % nums[i] == 0) {\n return i;\n }\n }\n return -1;\n }\n private int gcd(int a, int b) {\n while (b > 0) {\n int tmp = a;\n a = b;\n b = tmp % b;\n }\n return a;\n }\n```\n```python\n def minOperations(self, nums: List[int], numsDivide: List[int]) -> int:\n \n def gcd(a, b) -> int:\n while b > 0:\n a, b = b, a % b\n return a\n \n g = functools.reduce(gcd, numsDivide)\n nums.sort()\n for i, num in enumerate(nums):\n if g % num == 0:\n return i \n return -1\n```\n\n----\n\n**Use TreeMap**\n\n```java\n public int minOperations(int[] nums, int[] numsDivide) {\n int g = numsDivide[0];\n for (int n : numsDivide) {\n g = gcd(g, n);\n }\n TreeMap<Integer, Integer> cnt = new TreeMap<>();\n for (int num : nums) {\n cnt.merge(num, 1, Integer::sum);\n }\n int minOp = 0;\n for (var entry : cnt.entrySet()) {\n if (g % entry.getKey() == 0) {\n return minOp;\n }\n minOp += entry.getValue();\n }\n return -1;\n }\n private int gcd(int a, int b) {\n while (b > 0) {\n int tmp = a;\n a = b;\n b = tmp % b;\n }\n return a;\n }\n```\n\n```python\n def minOperations(self, nums: List[int], numsDivide: List[int]) -> int:\n \n def gcd(a, b) -> int:\n while b > 0:\n a, b = b, a % b\n return a\n \n g = reduce(gcd, numsDivide)\n cnt = Counter(nums)\n min_op = 0\n for c in sorted(cnt):\n if g % c == 0:\n return min_op\n min_op += cnt[c]\n return -1\n```\n\n**Analysis:**\n\nTime: `O(m * logm + n + log(max(numsDivide)))`, space: `O(m)` - including sorting space, where `m = nums.length, n = numsDivide.length`. | 10 | You are given two positive integer arrays `nums` and `numsDivide`. You can delete any number of elements from `nums`.
Return _the **minimum** number of deletions such that the **smallest** element in_ `nums` _**divides** all the elements of_ `numsDivide`. If this is not possible, return `-1`.
Note that an integer `x` divides `y` if `y % x == 0`.
**Example 1:**
**Input:** nums = \[2,3,2,4,3\], numsDivide = \[9,6,9,3,15\]
**Output:** 2
**Explanation:**
The smallest element in \[2,3,2,4,3\] is 2, which does not divide all the elements of numsDivide.
We use 2 deletions to delete the elements in nums that are equal to 2 which makes nums = \[3,4,3\].
The smallest element in \[3,4,3\] is 3, which divides all the elements of numsDivide.
It can be shown that 2 is the minimum number of deletions needed.
**Example 2:**
**Input:** nums = \[4,3,6\], numsDivide = \[8,2,6,10\]
**Output:** -1
**Explanation:**
We want the smallest element in nums to divide all the elements of numsDivide.
There is no way to delete elements from nums to allow this.
**Constraints:**
* `1 <= nums.length, numsDivide.length <= 105`
* `1 <= nums[i], numsDivide[i] <= 109` | null |
Python3 || GCD and heap, 6 lines, w/ explanation || T/M: 56%/100% | minimum-deletions-to-make-array-divisible | 0 | 1 | ```\nclass Solution:\n # From number theory, we know that an integer num divides each\n # integer in a list if and only if num divides the list\'s gcd.\n # \n # Our plan here is to:\n # \u2022 find the gcd of numDivide\n # \u2022 heapify(nums) and count the popped elements that do not\n # divide the gcd.\n # \u2022 return that count when and if a popped element eventually\n # divides the gcd. If that never happens, return -1 \n \n def minOperations(self, nums: List[int], numsDivide: List[int]) -> int:\n\t\n g, ans = gcd(*numsDivide), 0 # <-- find gcd (using * operator)\n\n heapify(nums) # <-- create heap\n\n while nums: # <-- pop and count\n\n if not g%heappop(nums): return ans # <-- found a divisor? return count\n else: ans+= 1 # <-- if not, increment the count\n\n return -1 # <-- no divisors found\n\t\t\n#--------------------------------------------------\nclass Solution: # version w/o heap. Seems to run slower\n def minOperations(self, nums: List[int], numsDivide: List[int]) -> int:\n\t\n g = gcd(*numsDivide)\n nums.sort()\n\n for i,num in enumerate(nums):\n if not g%num: return i\n\n return -1 | 3 | You are given two positive integer arrays `nums` and `numsDivide`. You can delete any number of elements from `nums`.
Return _the **minimum** number of deletions such that the **smallest** element in_ `nums` _**divides** all the elements of_ `numsDivide`. If this is not possible, return `-1`.
Note that an integer `x` divides `y` if `y % x == 0`.
**Example 1:**
**Input:** nums = \[2,3,2,4,3\], numsDivide = \[9,6,9,3,15\]
**Output:** 2
**Explanation:**
The smallest element in \[2,3,2,4,3\] is 2, which does not divide all the elements of numsDivide.
We use 2 deletions to delete the elements in nums that are equal to 2 which makes nums = \[3,4,3\].
The smallest element in \[3,4,3\] is 3, which divides all the elements of numsDivide.
It can be shown that 2 is the minimum number of deletions needed.
**Example 2:**
**Input:** nums = \[4,3,6\], numsDivide = \[8,2,6,10\]
**Output:** -1
**Explanation:**
We want the smallest element in nums to divide all the elements of numsDivide.
There is no way to delete elements from nums to allow this.
**Constraints:**
* `1 <= nums.length, numsDivide.length <= 105`
* `1 <= nums[i], numsDivide[i] <= 109` | null |
GCD and heaps | Python3 | Explained | minimum-deletions-to-make-array-divisible | 0 | 1 | 1. Calculate the GCD of numsDivide array\n2. heapify the nums array \n3. Pop elements from the nums array until it is not dividing the GCD in step 1\n\n```\nclass Solution:\n def minOperations(self, nums: List[int], numsDivide: List[int]) -> int:\n \n def GcdOfArray(arr, idx):\n if idx == len(arr)-1:\n return arr[idx]\n\n a = arr[idx]\n b = GcdOfArray(arr,idx+1)\n\n return math.gcd(a, b)\n \n c = GcdOfArray(numsDivide, 0)\n \n heapq.heapify(nums)\n res = 0\n while nums and (c % nums[0] != 0):\n res += 1\n heapq.heappop(nums)\n return res if len(nums) else -1\n```\n | 0 | You are given two positive integer arrays `nums` and `numsDivide`. You can delete any number of elements from `nums`.
Return _the **minimum** number of deletions such that the **smallest** element in_ `nums` _**divides** all the elements of_ `numsDivide`. If this is not possible, return `-1`.
Note that an integer `x` divides `y` if `y % x == 0`.
**Example 1:**
**Input:** nums = \[2,3,2,4,3\], numsDivide = \[9,6,9,3,15\]
**Output:** 2
**Explanation:**
The smallest element in \[2,3,2,4,3\] is 2, which does not divide all the elements of numsDivide.
We use 2 deletions to delete the elements in nums that are equal to 2 which makes nums = \[3,4,3\].
The smallest element in \[3,4,3\] is 3, which divides all the elements of numsDivide.
It can be shown that 2 is the minimum number of deletions needed.
**Example 2:**
**Input:** nums = \[4,3,6\], numsDivide = \[8,2,6,10\]
**Output:** -1
**Explanation:**
We want the smallest element in nums to divide all the elements of numsDivide.
There is no way to delete elements from nums to allow this.
**Constraints:**
* `1 <= nums.length, numsDivide.length <= 105`
* `1 <= nums[i], numsDivide[i] <= 109` | null |
[Java/Python 3] 3 conditionals w/ brief explanation and analysis. | best-poker-hand | 1 | 1 | 1. If all characters in `suits` are same, then it is `Flush`;\n2. If there are at least `3` values in `ranks` are same, it is `Three of a Kind`;\n3. If there are`2` values in `ranks` are same, it is `Pair`;\n4. Otherwise, it is `High Card`.\n\n```java\n public String bestHand(int[] r, char[] s) {\n int[] cnt = new int[14];\n IntStream.of(r).forEach(i -> ++cnt[i]);\n int max = IntStream.of(cnt).max().getAsInt();\n if (s[0] == s[1] && s[1] == s[2] && s[2] == s[3] && s[3] == s[4]) {\n return "Flush";\n }else if (max >= 3) {\n return "Three of a Kind";\n }else if (max == 2) {\n return "Pair";\n }else {\n return "High Card";\n }\n }\n```\n```python\n def bestHand(self, ranks: List[int], suits: List[str]) -> str:\n if len(set(suits)) == 1:\n return "Flush"\n match max(Counter(ranks).values()):\n case 3 | 4 | 5:\n return "Three of a Kind"\n case 2:\n return "Pair"\n case _:\n return "High Card"\n```\nor \n\n```python\n def bestHand(self, ranks: List[int], suits: List[str]) -> str:\n cnt = Counter(ranks)\n if len(set(suits)) == 1:\n return "Flush"\n elif max(cnt.values()) >= 3:\n return "Three of a Kind"\n elif max(cnt.values()) == 2:\n return "Pair"\n else:\n return "High Card"\n```\n\n**Analysis:**\n\nTime: `O(r + s)`, where `r = ranks.length, s = suits.length`;\nspace: \njava code - `O(range)`, where `range` is the upper bound of the `ranks`\nPython 3 - `O(# of distinct values in ranks)` | 10 | You are given an integer array `ranks` and a character array `suits`. You have `5` cards where the `ith` card has a rank of `ranks[i]` and a suit of `suits[i]`.
The following are the types of **poker hands** you can make from best to worst:
1. `"Flush "`: Five cards of the same suit.
2. `"Three of a Kind "`: Three cards of the same rank.
3. `"Pair "`: Two cards of the same rank.
4. `"High Card "`: Any single card.
Return _a string representing the **best** type of **poker hand** you can make with the given cards._
**Note** that the return values are **case-sensitive**.
**Example 1:**
**Input:** ranks = \[13,2,3,1,9\], suits = \[ "a ", "a ", "a ", "a ", "a "\]
**Output:** "Flush "
**Explanation:** The hand with all the cards consists of 5 cards with the same suit, so we have a "Flush ".
**Example 2:**
**Input:** ranks = \[4,4,2,4,4\], suits = \[ "d ", "a ", "a ", "b ", "c "\]
**Output:** "Three of a Kind "
**Explanation:** The hand with the first, second, and fourth card consists of 3 cards with the same rank, so we have a "Three of a Kind ".
Note that we could also make a "Pair " hand but "Three of a Kind " is a better hand.
Also note that other cards could be used to make the "Three of a Kind " hand.
**Example 3:**
**Input:** ranks = \[10,10,2,12,9\], suits = \[ "a ", "b ", "c ", "a ", "d "\]
**Output:** "Pair "
**Explanation:** The hand with the first and second card consists of 2 cards with the same rank, so we have a "Pair ".
Note that we cannot make a "Flush " or a "Three of a Kind ".
**Constraints:**
* `ranks.length == suits.length == 5`
* `1 <= ranks[i] <= 13`
* `'a' <= suits[i] <= 'd'`
* No two cards have the same rank and suit. | null |
Python | Easy & Understanding Solution | best-poker-hand | 0 | 1 | ```\nclass Solution:\n def bestHand(self, ranks: List[int], suits: List[str]) -> str:\n if(len(set(suits))==1):\n return "Flush"\n \n mp={}\n \n for i in range(5):\n if(ranks[i] not in mp):\n mp[ranks[i]]=1\n else:\n mp[ranks[i]]+=1\n \n for val in mp:\n if(mp[val]>=3):\n return "Three of a Kind"\n elif(mp[val]==2):\n return "Pair"\n \n return "High Card"\n\t\t\n\t\t | 1 | You are given an integer array `ranks` and a character array `suits`. You have `5` cards where the `ith` card has a rank of `ranks[i]` and a suit of `suits[i]`.
The following are the types of **poker hands** you can make from best to worst:
1. `"Flush "`: Five cards of the same suit.
2. `"Three of a Kind "`: Three cards of the same rank.
3. `"Pair "`: Two cards of the same rank.
4. `"High Card "`: Any single card.
Return _a string representing the **best** type of **poker hand** you can make with the given cards._
**Note** that the return values are **case-sensitive**.
**Example 1:**
**Input:** ranks = \[13,2,3,1,9\], suits = \[ "a ", "a ", "a ", "a ", "a "\]
**Output:** "Flush "
**Explanation:** The hand with all the cards consists of 5 cards with the same suit, so we have a "Flush ".
**Example 2:**
**Input:** ranks = \[4,4,2,4,4\], suits = \[ "d ", "a ", "a ", "b ", "c "\]
**Output:** "Three of a Kind "
**Explanation:** The hand with the first, second, and fourth card consists of 3 cards with the same rank, so we have a "Three of a Kind ".
Note that we could also make a "Pair " hand but "Three of a Kind " is a better hand.
Also note that other cards could be used to make the "Three of a Kind " hand.
**Example 3:**
**Input:** ranks = \[10,10,2,12,9\], suits = \[ "a ", "b ", "c ", "a ", "d "\]
**Output:** "Pair "
**Explanation:** The hand with the first and second card consists of 2 cards with the same rank, so we have a "Pair ".
Note that we cannot make a "Flush " or a "Three of a Kind ".
**Constraints:**
* `ranks.length == suits.length == 5`
* `1 <= ranks[i] <= 13`
* `'a' <= suits[i] <= 'd'`
* No two cards have the same rank and suit. | null |
Python || easy colution | best-poker-hand | 0 | 1 | ```\nclass Solution:\n def bestHand(self, ranks: List[int], suits: List[str]) -> str:\n N = len(ranks)\n statistic = defaultdict(int)\n\n letter_freq = 0\n number_freq = 0\n\n for i in range(N):\n statistic[ranks[i]] += 1\n statistic[suits[i]] += 1\n letter_freq = max(letter_freq, statistic[suits[i]])\n number_freq = max(number_freq, statistic[ranks[i]])\n\n if letter_freq >=5:\n return "Flush"\n\n if number_freq >=3:\n return "Three of a Kind"\n if number_freq >= 2:\n return "Pair"\n\n return "High Card"\n```\n\nor by using counter\n\n```\nclass Solution:\n def bestHand(self, ranks: List[int], suits: List[str]) -> str:\n N = len(ranks)\n statistic = defaultdict(int)\n\n letter_freq = Counter(suits).most_common(1)\n number_freq = Counter(ranks).most_common(1)\n \n if letter_freq[0][1] >=5:\n return "Flush"\n\n if number_freq[0][1] >=3:\n return "Three of a Kind"\n if number_freq[0][1] >= 2:\n return "Pair"\n\n return "High Card"\n``` | 1 | You are given an integer array `ranks` and a character array `suits`. You have `5` cards where the `ith` card has a rank of `ranks[i]` and a suit of `suits[i]`.
The following are the types of **poker hands** you can make from best to worst:
1. `"Flush "`: Five cards of the same suit.
2. `"Three of a Kind "`: Three cards of the same rank.
3. `"Pair "`: Two cards of the same rank.
4. `"High Card "`: Any single card.
Return _a string representing the **best** type of **poker hand** you can make with the given cards._
**Note** that the return values are **case-sensitive**.
**Example 1:**
**Input:** ranks = \[13,2,3,1,9\], suits = \[ "a ", "a ", "a ", "a ", "a "\]
**Output:** "Flush "
**Explanation:** The hand with all the cards consists of 5 cards with the same suit, so we have a "Flush ".
**Example 2:**
**Input:** ranks = \[4,4,2,4,4\], suits = \[ "d ", "a ", "a ", "b ", "c "\]
**Output:** "Three of a Kind "
**Explanation:** The hand with the first, second, and fourth card consists of 3 cards with the same rank, so we have a "Three of a Kind ".
Note that we could also make a "Pair " hand but "Three of a Kind " is a better hand.
Also note that other cards could be used to make the "Three of a Kind " hand.
**Example 3:**
**Input:** ranks = \[10,10,2,12,9\], suits = \[ "a ", "b ", "c ", "a ", "d "\]
**Output:** "Pair "
**Explanation:** The hand with the first and second card consists of 2 cards with the same rank, so we have a "Pair ".
Note that we cannot make a "Flush " or a "Three of a Kind ".
**Constraints:**
* `ranks.length == suits.length == 5`
* `1 <= ranks[i] <= 13`
* `'a' <= suits[i] <= 'd'`
* No two cards have the same rank and suit. | null |
Python 1-liner. Functional programming. | number-of-zero-filled-subarrays | 0 | 1 | # Approach\nTL;DR, same as [Official solution](https://leetcode.com/problems/number-of-zero-filled-subarrays/editorial/) but written in a declarative and functional way.\n\n# Complexity\n- Time complexity: $$O(n)$$\n\n- Space complexity: $$O(1)$$\n\nwhere, `n is the length of nums`.\n\n# Code\n```python\nclass Solution:\n def zeroFilledSubarray(self, nums: list[int]) -> int:\n return sum(accumulate(nums, lambda a, x: 0 if x else a + 1, initial=0))\n\n\n``` | 6 | Given an integer array `nums`, return _the number of **subarrays** filled with_ `0`.
A **subarray** is a contiguous non-empty sequence of elements within an array.
**Example 1:**
**Input:** nums = \[1,3,0,0,2,0,0,4\]
**Output:** 6
**Explanation:**
There are 4 occurrences of \[0\] as a subarray.
There are 2 occurrences of \[0,0\] as a subarray.
There is no occurrence of a subarray with a size more than 2 filled with 0. Therefore, we return 6.
**Example 2:**
**Input:** nums = \[0,0,0,2,0,0\]
**Output:** 9
**Explanation:**
There are 5 occurrences of \[0\] as a subarray.
There are 3 occurrences of \[0,0\] as a subarray.
There is 1 occurrence of \[0,0,0\] as a subarray.
There is no occurrence of a subarray with a size more than 3 filled with 0. Therefore, we return 9.
**Example 3:**
**Input:** nums = \[2,10,2019\]
**Output:** 0
**Explanation:** There is no subarray filled with 0. Therefore, we return 0.
**Constraints:**
* `1 <= nums.length <= 105`
* `-109 <= nums[i] <= 109` | null |
Python Readable - Simple Intuition | number-of-zero-filled-subarrays | 0 | 1 | # Intuition\n<!-- Describe your first thoughts on how to solve this problem. -->\n1. What exactly are zero filled subarrays?\n2. What are you trying to find with zero filled subarrays? -> [0], [0,0], [0,0,0] ...\n3. Once you have found array [0,0,0] or [0,0], how many subarrays exist in this subarray? -> Math Trick sum of Integers\n\n# Approach\n<!-- Describe your approach to solving the problem. -->\n1. Initialise zeros = 0 to track continuous zeros.\n2. Iterate through nums:\n2. if num == 0, it means we can 1 to the count of total zeros.\n3. if num != 0, sum the current total zeros and reset `zeros = 0`\n\n# Complexity\n- Time complexity: O(N) \n<!-- Add your time complexity here, e.g. $$O(n)$$ -->\n\n- Space complexity: O(1)\n<!-- Add your space complexity here, e.g. $$O(n)$$ -->\n\n# Code\n```\nclass Solution:\n def zeroFilledSubarray(self, nums: List[int]) -> int:\n\n def sumOfIntegers(num):\n return num*(1 + num)//2\n\n res = zeros = 0\n for i in nums:\n if i != 0: \n res += sumOfIntegers(zeros)\n zeros = 0\n continue\n zeros += 1\n \n return res if not zeros else res + sumOfIntegers(zeros)\n\n```\n**Optimised and Elegant**\nCredits to @blue_sky5\n\n**Intuition**\n1. This extends off the intuition of sumOfIntegers. What is the underlying way to sum numbers?\n-> sum(3) = 1 + 2 + 3. ------------> [0,0,0]\n-> sum(4) = 1 + 2 + 3 + 4. --------> [0,0,0,0]\n-> sum(x) = 1 + 2 + 3 + ... + x ----> [0,0, ..., 0]\n\nWhen you see a 0, add it to counter. If its not 0, reset. \nAdd counter to res after every iteration.\n\n*Google Triangular Numbers*\n\n```\nclass Solution:\n def zeroFilledSubarray(self, nums: List[int]) -> int:\n\n res = zeros = 0\n\n for num in nums:\n if num == 0: \n zeros += 1\n else:\n zeros = 0\n res += zeros\n \n return res\n\n``` | 2 | Given an integer array `nums`, return _the number of **subarrays** filled with_ `0`.
A **subarray** is a contiguous non-empty sequence of elements within an array.
**Example 1:**
**Input:** nums = \[1,3,0,0,2,0,0,4\]
**Output:** 6
**Explanation:**
There are 4 occurrences of \[0\] as a subarray.
There are 2 occurrences of \[0,0\] as a subarray.
There is no occurrence of a subarray with a size more than 2 filled with 0. Therefore, we return 6.
**Example 2:**
**Input:** nums = \[0,0,0,2,0,0\]
**Output:** 9
**Explanation:**
There are 5 occurrences of \[0\] as a subarray.
There are 3 occurrences of \[0,0\] as a subarray.
There is 1 occurrence of \[0,0,0\] as a subarray.
There is no occurrence of a subarray with a size more than 3 filled with 0. Therefore, we return 9.
**Example 3:**
**Input:** nums = \[2,10,2019\]
**Output:** 0
**Explanation:** There is no subarray filled with 0. Therefore, we return 0.
**Constraints:**
* `1 <= nums.length <= 105`
* `-109 <= nums[i] <= 109` | null |
Unveil Logic with Crazy Solution | number-of-zero-filled-subarrays | 0 | 1 | \n\n# Superb Logic---->O(N) \n```\nclass Solution:\n def zeroFilledSubarray(self, nums: List[int]) -> int:\n answer=count=0\n for i in nums:\n if i==0: count+=1\n else:count=0\n answer+=count\n return answer\n\n```\n# please upvote me it would encourage me alot\n | 2 | Given an integer array `nums`, return _the number of **subarrays** filled with_ `0`.
A **subarray** is a contiguous non-empty sequence of elements within an array.
**Example 1:**
**Input:** nums = \[1,3,0,0,2,0,0,4\]
**Output:** 6
**Explanation:**
There are 4 occurrences of \[0\] as a subarray.
There are 2 occurrences of \[0,0\] as a subarray.
There is no occurrence of a subarray with a size more than 2 filled with 0. Therefore, we return 6.
**Example 2:**
**Input:** nums = \[0,0,0,2,0,0\]
**Output:** 9
**Explanation:**
There are 5 occurrences of \[0\] as a subarray.
There are 3 occurrences of \[0,0\] as a subarray.
There is 1 occurrence of \[0,0,0\] as a subarray.
There is no occurrence of a subarray with a size more than 3 filled with 0. Therefore, we return 9.
**Example 3:**
**Input:** nums = \[2,10,2019\]
**Output:** 0
**Explanation:** There is no subarray filled with 0. Therefore, we return 0.
**Constraints:**
* `1 <= nums.length <= 105`
* `-109 <= nums[i] <= 109` | null |
O(n) time complexity and O(n) space complexity | number-of-zero-filled-subarrays | 0 | 1 | \n\n\n# Intuition\nThe code aims to find the number of subarrays filled with 0 in the given integer array nums. It does this by iterating through the array and identifying the subarrays filled with 0. The intuition behind the solution is to keep track of contiguous sequences of zeros and then calculate the number of subarrays filled with 0 in each sequence.\n\n# Approach\nThe code uses a simple approach to solve the problem. It maintains an auxiliary list called aux to keep track of contiguous sequences of zeros in the input array nums. The variable resp is used to store the count of subarrays filled with 0.\n\nThe code iterates through the nums array. If the current element is 0, it is appended to the aux list. When a non-zero element is encountered, the code checks if the aux list is not empty. If it is not empty, it means we have a contiguous sequence of zeros, and the code calculates the number of subarrays that can be formed from this sequence using the formula n * (n + 1) / 2, where n is the length of the sequence. The result is added to the resp variable. After that, the aux list is reset to an empty list.\n\nAt the end of the iteration, there might be a remaining sequence of zeros in the aux list. If this is the case, the code performs the same calculation and adds it to the resp variable.\n\nFinally, the function returns the resp, which represents the total number of subarrays filled with 0 in the given array nums.\n\n# Complexity\n- Time complexity:\nThe code iterates through the nums array once, where n is the number of elements in the array. Each element is processed once, and all other operations (appending to aux, calculating resp, resetting aux) take constant time.\n\n- Space complexity:\nThe space complexity is determined by the aux list, which can store up to n elements in the worst case.\n\n# Code\n```\nclass Solution:\n def zeroFilledSubarray(self, nums: List[int]) -> int:\n aux = []\n resp = 0\n for i in range(len(nums)):\n if nums[i] == 0:\n aux.append(nums[i])\n else:\n if len(aux) > 0:\n n = len(aux)\n resp += n*(n+1)/2\n aux = []\n if len(aux) > 0:\n n = len(aux)\n resp += n*(n+1)/2\n return int(resp)\n``` | 1 | Given an integer array `nums`, return _the number of **subarrays** filled with_ `0`.
A **subarray** is a contiguous non-empty sequence of elements within an array.
**Example 1:**
**Input:** nums = \[1,3,0,0,2,0,0,4\]
**Output:** 6
**Explanation:**
There are 4 occurrences of \[0\] as a subarray.
There are 2 occurrences of \[0,0\] as a subarray.
There is no occurrence of a subarray with a size more than 2 filled with 0. Therefore, we return 6.
**Example 2:**
**Input:** nums = \[0,0,0,2,0,0\]
**Output:** 9
**Explanation:**
There are 5 occurrences of \[0\] as a subarray.
There are 3 occurrences of \[0,0\] as a subarray.
There is 1 occurrence of \[0,0,0\] as a subarray.
There is no occurrence of a subarray with a size more than 3 filled with 0. Therefore, we return 9.
**Example 3:**
**Input:** nums = \[2,10,2019\]
**Output:** 0
**Explanation:** There is no subarray filled with 0. Therefore, we return 0.
**Constraints:**
* `1 <= nums.length <= 105`
* `-109 <= nums[i] <= 109` | null |
python3 || dp||easy to understand|| get the pattern|| dictionary|| | number-of-zero-filled-subarrays | 0 | 1 | \'\'\':\n\n\n\t\t\t\t\tthe pattern is here: {1:1,2:3,3:6,4:10,5:15,6:21} ={number of zeros:answer}\n\t\t\t\t\tif the question is [0,0] the answer becomes 3.\n\t\t\t\t\tbut if the question becomes like this [0,0,1,0,0] the answer becomes 6 which means 3+3\n\t\t\t\t\t[0,0] = 3 , [0,0] = 3 , [0,0,1,0,0] = 3+3 = 6\n\t\t\n\'\'\'\n\n\'\'\'\n\n\t\tclass Solution:\n\t\t\n\t\t\t\tdef zeroFilledSubarray(self, nums: List[int]) -> int:\n\t\t\t\t\t"""pattern :1:1,2:3,3:6,4:10,5:15,6:21"""\n\n\t\t\t\t\tdp = [0 for i in range(max(100,len(nums)+1))]\n\t\t\t\t\tdp[1] = 1\n\t\t\t\t\tdp[2] = 3\n\t\t\t\t\tfor i in range(3,len(nums)+1):\n\t\t\t\t\t\tdp[i] = dp[i-1]+i\n\t\t\t\t\tres = 0\n\t\t\t\t\tcount = 0\n\t\t\t\t\tfor i in nums:\n\t\t\t\t\t\tif i ==0:\n\t\t\t\t\t\t\tcount +=1\n\t\t\t\t\t\telse:\n\t\t\t\t\t\t\tres+= dp[count]\n\t\t\t\t\t\t\tcount = 0\n\t\t\t\t\tres+=dp[count]\n\t\t\t\t\treturn res\n\n\'\'\' | 1 | Given an integer array `nums`, return _the number of **subarrays** filled with_ `0`.
A **subarray** is a contiguous non-empty sequence of elements within an array.
**Example 1:**
**Input:** nums = \[1,3,0,0,2,0,0,4\]
**Output:** 6
**Explanation:**
There are 4 occurrences of \[0\] as a subarray.
There are 2 occurrences of \[0,0\] as a subarray.
There is no occurrence of a subarray with a size more than 2 filled with 0. Therefore, we return 6.
**Example 2:**
**Input:** nums = \[0,0,0,2,0,0\]
**Output:** 9
**Explanation:**
There are 5 occurrences of \[0\] as a subarray.
There are 3 occurrences of \[0,0\] as a subarray.
There is 1 occurrence of \[0,0,0\] as a subarray.
There is no occurrence of a subarray with a size more than 3 filled with 0. Therefore, we return 9.
**Example 3:**
**Input:** nums = \[2,10,2019\]
**Output:** 0
**Explanation:** There is no subarray filled with 0. Therefore, we return 0.
**Constraints:**
* `1 <= nums.length <= 105`
* `-109 <= nums[i] <= 109` | null |
easiest two approaches loop using in python3 | number-of-zero-filled-subarrays | 0 | 1 | # Intuition\n<!-- Describe your first thoughts on how to solve this problem. -->\n\n# Approach\n<!-- Describe your approach to solving the problem. -->\n\n# Complexity\n- Time complexity:\n<!-- Add your time complexity here, e.g. $$O(n)$$ -->\n\n- Space complexity:\n<!-- Add your space complexity here, e.g. $$O(n)$$ -->\n\n# Code\n```\nclass Solution:\n def zeroFilledSubarray(self, nums: List[int]) -> int:\n # approach 1\n count,result=0,0\n for i in range(len(nums)):\n if nums[i]==0:\n count+=1\n else:\n count=0\n result=result+count\n return result\n```\n```\nclass Solution:\n def zeroFilledSubarray(self, nums: List[int]) -> int:\n # approach 2\n n=len(nums)\n i=0\n ans=0\n while i<n:\n l=0\n if nums[i]==0:\n while i<n and nums[i]==0:\n l+=1\n i+=1\n else:\n i+=1\n ans+=int(((l)*(l+1))/2)\n return ans\n \n \n\n\n``` | 1 | Given an integer array `nums`, return _the number of **subarrays** filled with_ `0`.
A **subarray** is a contiguous non-empty sequence of elements within an array.
**Example 1:**
**Input:** nums = \[1,3,0,0,2,0,0,4\]
**Output:** 6
**Explanation:**
There are 4 occurrences of \[0\] as a subarray.
There are 2 occurrences of \[0,0\] as a subarray.
There is no occurrence of a subarray with a size more than 2 filled with 0. Therefore, we return 6.
**Example 2:**
**Input:** nums = \[0,0,0,2,0,0\]
**Output:** 9
**Explanation:**
There are 5 occurrences of \[0\] as a subarray.
There are 3 occurrences of \[0,0\] as a subarray.
There is 1 occurrence of \[0,0,0\] as a subarray.
There is no occurrence of a subarray with a size more than 3 filled with 0. Therefore, we return 9.
**Example 3:**
**Input:** nums = \[2,10,2019\]
**Output:** 0
**Explanation:** There is no subarray filled with 0. Therefore, we return 0.
**Constraints:**
* `1 <= nums.length <= 105`
* `-109 <= nums[i] <= 109` | null |
DEAR MJ | design-a-number-container-system | 0 | 1 | # Intuition\n<!-- Describe your first thoughts on how to solve this problem. -->\nusing two hashmap(dictionary, set) and renew the min index of the value each time we call the method \'change\'\n\n# Approach\n<!-- Describe your approach to solving the problem. -->\ndescription is in the code\n\n# Complexity\n- Time complexity:\n<!-- Add your time complexity here, e.g. $$O(n)$$ -->\nchange:\n - if the set of prev_value is not empty after removal, we have to find min value from the set and it takes O(N)\n - all other operation takes O(1) because we are using hashtables\nfind: O(1)\n\n- Space complexity:\n<!-- Add your space complexity here, e.g. $$O(n)$$ -->\n\n\n# Code\n```\nclass NumberContainers:\n\n def __init__(self):\n # {value: [\'min index\', set(indices at which the value is taking place)]}\n self.val_dict = {}\n # {index: value that is taking place at the index}\n self.idx_dict = {}\n\n def change(self, index: int, number: int) -> None:\n # if there is already a number at the index,\n if index in self.idx_dict:\n # we call the number \'prev_value\'\n prev_value = self.idx_dict[index]\n # if prev_value is same as the given number, we do nothing\n if prev_value == number:\n return\n # remove the index from the set of indices of the prev_value\n self.val_dict[prev_value][1].remove(index)\n # if the set is empty, we assign \'infinity\' to min index of the prev_value\n # because it is useful when we compare the current min index and the new index\n if not self.val_dict[prev_value][1]:\n self.val_dict[prev_value][0] = sys.maxsize\n # if the set is not empty after removal, we assign min value of the set to the min index\n else:\n self.val_dict[prev_value][0] = min(self.val_dict[prev_value][1])\n\n # now we\'re done with the prev_value\n # let\'s handle the new number and the index\n\n # set the number to the idx_dict[index]\n self.idx_dict[index] = number\n\n # if the number has already been filled into this container,\n if number in self.val_dict:\n # renew the min index\n # (if the min index == sys.maxsize,\n # it will automatically assign new index to the min index)\n self.val_dict[number][0] = min(self.val_dict[number][0], index)\n # add new index to the set\n self.val_dict[number][1].add(index)\n # else we should make new list of [new index, {new index}]\n else:\n self.val_dict[number] = [index, {index}]\n\n\n def find(self, number: int) -> int:\n # if there is no index that is filled by number\n if number not in self.val_dict or not self.val_dict[number][1]:\n return -1\n # it will return the smallest index for the given number\n return self.val_dict[number][0]\n \n\n\n# Your NumberContainers object will be instantiated and called as such:\n# obj = NumberContainers()\n# obj.change(index,number)\n# param_2 = obj.find(number)\n``` | 1 | Design a number container system that can do the following:
* **Insert** or **Replace** a number at the given index in the system.
* **Return** the smallest index for the given number in the system.
Implement the `NumberContainers` class:
* `NumberContainers()` Initializes the number container system.
* `void change(int index, int number)` Fills the container at `index` with the `number`. If there is already a number at that `index`, replace it.
* `int find(int number)` Returns the smallest index for the given `number`, or `-1` if there is no index that is filled by `number` in the system.
**Example 1:**
**Input**
\[ "NumberContainers ", "find ", "change ", "change ", "change ", "change ", "find ", "change ", "find "\]
\[\[\], \[10\], \[2, 10\], \[1, 10\], \[3, 10\], \[5, 10\], \[10\], \[1, 20\], \[10\]\]
**Output**
\[null, -1, null, null, null, null, 1, null, 2\]
**Explanation**
NumberContainers nc = new NumberContainers();
nc.find(10); // There is no index that is filled with number 10. Therefore, we return -1.
nc.change(2, 10); // Your container at index 2 will be filled with number 10.
nc.change(1, 10); // Your container at index 1 will be filled with number 10.
nc.change(3, 10); // Your container at index 3 will be filled with number 10.
nc.change(5, 10); // Your container at index 5 will be filled with number 10.
nc.find(10); // Number 10 is at the indices 1, 2, 3, and 5. Since the smallest index that is filled with 10 is 1, we return 1.
nc.change(1, 20); // Your container at index 1 will be filled with number 20. Note that index 1 was filled with 10 and then replaced with 20.
nc.find(10); // Number 10 is at the indices 2, 3, and 5. The smallest index that is filled with 10 is 2. Therefore, we return 2.
**Constraints:**
* `1 <= index, number <= 109`
* At most `105` calls will be made **in total** to `change` and `find`. | null |
[Java/Python 3] TreeSet/SortedList w/ brief explanation and analysis. | design-a-number-container-system | 1 | 1 | **Q & A**\n\nQ1: How many third party packages are pre installed on leetcode server? And where to get such list?\nA1: Please see [What are the environments for the programming languages?](https://support.leetcode.com/hc/en-us/articles/360011833974-What-are-the-environments-for-the-programming-languages-).\n\nQ2: Why `find` is `O(logn)` since the values are sorted?\nA2: \n\n\n1) In Python 3 code `SortedList[i]` is actually `SortedList.__getitem__`, which cost time `O(logn)`. Please refer to [source code](https://grantjenks.com/docs/sortedcontainers/_modules/sortedcontainers/sortedlist.html#SortedList.__getitem__) for more details.\n2) In Java code, the `TreeSet` is implemented based on `TreeMap`, which is implemented using Red-Black Tree. [Red-Black Tree](https://en.m.wikipedia.org/wiki/Red%E2%80%93black_tree) is a self-balancing binary search tree, and any search operation cost `O(logn)`. Hence the `TreeSet.first()` cost `O(logn)`.\n\n\n**End of Q & A**\n\n----\n\nSince the problem request that the smallest index of a given `number` be returned, a `TreeSet/SortedList` is a reasonable choice.\n\nTherefore, we can use `2` `HashMap/dict` to store `number/indices` and `index/number` bindings respectively.\n\nNote: For each call of `change`, we first check if there has already been a `number` filled at the `index`: if yes, discard the old value at the `index` first, then update/insert the new value.\n\n```java\n private Map<Integer, TreeSet<Integer>> numToIndices = new HashMap<>();\n private Map<Integer, Integer> indexToNum = new HashMap<>();\n\n public NumberContainers() {}\n \n public void change(int index, int number) {\n if (indexToNum.containsKey(index)) {\n int old = indexToNum.get(index);\n numToIndices.get(old).remove(index);\n if (numToIndices.get(old).isEmpty()) {\n numToIndices.remove(old);\n }\n }\n indexToNum.put(index, number);\n numToIndices.computeIfAbsent(number, s -> new TreeSet<>()).add(index);\n }\n \n public int find(int number) {\n if (numToIndices.containsKey(number)) {\n return numToIndices.get(number).first();\n }\n return -1;\n }\n```\n```python\nfrom sortedcontainers import SortedList\n\nclass NumberContainers:\n\n def __init__(self):\n self.num_to_indices = defaultdict(SortedList)\n self.index_to_num = {}\n\n def change(self, index: int, number: int) -> None:\n if index in self.index_to_num:\n old = self.index_to_num[index]\n self.num_to_indices[old].discard(index)\n if not self.num_to_indices[old]:\n del self.num_to_indices[old]\n self.num_to_indices[number].add(index)\n self.index_to_num[index] = number\n \n\n def find(self, number: int) -> int:\n if number in self.num_to_indices:\n return self.num_to_indices[number][0]\n return -1\n```\n\n**Analysis:**\n\nOverall space: `O(n)`\n\nTime for both `change` and `find`: `O(logn)`, where `n` is the total count of numbers in the container. | 44 | Design a number container system that can do the following:
* **Insert** or **Replace** a number at the given index in the system.
* **Return** the smallest index for the given number in the system.
Implement the `NumberContainers` class:
* `NumberContainers()` Initializes the number container system.
* `void change(int index, int number)` Fills the container at `index` with the `number`. If there is already a number at that `index`, replace it.
* `int find(int number)` Returns the smallest index for the given `number`, or `-1` if there is no index that is filled by `number` in the system.
**Example 1:**
**Input**
\[ "NumberContainers ", "find ", "change ", "change ", "change ", "change ", "find ", "change ", "find "\]
\[\[\], \[10\], \[2, 10\], \[1, 10\], \[3, 10\], \[5, 10\], \[10\], \[1, 20\], \[10\]\]
**Output**
\[null, -1, null, null, null, null, 1, null, 2\]
**Explanation**
NumberContainers nc = new NumberContainers();
nc.find(10); // There is no index that is filled with number 10. Therefore, we return -1.
nc.change(2, 10); // Your container at index 2 will be filled with number 10.
nc.change(1, 10); // Your container at index 1 will be filled with number 10.
nc.change(3, 10); // Your container at index 3 will be filled with number 10.
nc.change(5, 10); // Your container at index 5 will be filled with number 10.
nc.find(10); // Number 10 is at the indices 1, 2, 3, and 5. Since the smallest index that is filled with 10 is 1, we return 1.
nc.change(1, 20); // Your container at index 1 will be filled with number 20. Note that index 1 was filled with 10 and then replaced with 20.
nc.find(10); // Number 10 is at the indices 2, 3, and 5. The smallest index that is filled with 10 is 2. Therefore, we return 2.
**Constraints:**
* `1 <= index, number <= 109`
* At most `105` calls will be made **in total** to `change` and `find`. | null |
Heap + Hashmap Python3 Solution | design-a-number-container-system | 0 | 1 | ```\nclass NumberContainers:\n\n def __init__(self):\n self.num_indices = defaultdict(list)\n self.num_at_index = {}\n \n\n def change(self, index: int, number: int) -> None:\n self.num_at_index[index] = number\n heapq.heappush(self.num_indices[number], index)\n \n\n def find(self, number: int) -> int:\n while self.num_indices[number] and self.num_at_index[self.num_indices[number][0]] != number:\n heapq.heappop(self.num_indices[number])\n \n return self.num_indices[number][0] if len(self.num_indices[number]) > 0 else -1\n \n\n\n# Your NumberContainers object will be instantiated and called as such:\n# obj = NumberContainers()\n# obj.change(index,number)\n# param_2 = obj.find(number)\n``` | 1 | Design a number container system that can do the following:
* **Insert** or **Replace** a number at the given index in the system.
* **Return** the smallest index for the given number in the system.
Implement the `NumberContainers` class:
* `NumberContainers()` Initializes the number container system.
* `void change(int index, int number)` Fills the container at `index` with the `number`. If there is already a number at that `index`, replace it.
* `int find(int number)` Returns the smallest index for the given `number`, or `-1` if there is no index that is filled by `number` in the system.
**Example 1:**
**Input**
\[ "NumberContainers ", "find ", "change ", "change ", "change ", "change ", "find ", "change ", "find "\]
\[\[\], \[10\], \[2, 10\], \[1, 10\], \[3, 10\], \[5, 10\], \[10\], \[1, 20\], \[10\]\]
**Output**
\[null, -1, null, null, null, null, 1, null, 2\]
**Explanation**
NumberContainers nc = new NumberContainers();
nc.find(10); // There is no index that is filled with number 10. Therefore, we return -1.
nc.change(2, 10); // Your container at index 2 will be filled with number 10.
nc.change(1, 10); // Your container at index 1 will be filled with number 10.
nc.change(3, 10); // Your container at index 3 will be filled with number 10.
nc.change(5, 10); // Your container at index 5 will be filled with number 10.
nc.find(10); // Number 10 is at the indices 1, 2, 3, and 5. Since the smallest index that is filled with 10 is 1, we return 1.
nc.change(1, 20); // Your container at index 1 will be filled with number 20. Note that index 1 was filled with 10 and then replaced with 20.
nc.find(10); // Number 10 is at the indices 2, 3, and 5. The smallest index that is filled with 10 is 2. Therefore, we return 2.
**Constraints:**
* `1 <= index, number <= 109`
* At most `105` calls will be made **in total** to `change` and `find`. | null |
Python easy understanding solution | shortest-impossible-sequence-of-rolls | 0 | 1 | For example, k = 3, rolls = [1, 1, 2, 2, 3, 1]\nUse a set to record if all possible numbers has appeared at least once.\nIn this case, when iterate to 3 in [1, 1, 2, 2, **3**, 1], all possible numbers (1-3) has appeared at least once,\nso you must at least take 1 number from interval [1, 1, 2, 2, 3], in practice, take 3.\n\nIn the remain array [1], it cannot fill the set again. It means there exists a number you can take to make the sequence impossible, in this case, take either 2 or 3. So both [3, 2] and [3, 3] are impossible array.\n\n```\nclass Solution:\n def shortestSequence(self, rolls: List[int], k: int) -> int:\n s = set()\n res = 0\n \n for r in rolls:\n s.add(r)\n if len(s) == k: # All possible number has appeared once.\n res += 1 # So you must "at least" use one more place to store it.\n s.clear() # Clear the set.\n \n \n return res + 1 | 1 | You are given an integer array `rolls` of length `n` and an integer `k`. You roll a `k` sided dice numbered from `1` to `k`, `n` times, where the result of the `ith` roll is `rolls[i]`.
Return _the length of the **shortest** sequence of rolls that **cannot** be taken from_ `rolls`.
A **sequence of rolls** of length `len` is the result of rolling a `k` sided dice `len` times.
**Note** that the sequence taken does not have to be consecutive as long as it is in order.
**Example 1:**
**Input:** rolls = \[4,2,1,2,3,3,2,4,1\], k = 4
**Output:** 3
**Explanation:** Every sequence of rolls of length 1, \[1\], \[2\], \[3\], \[4\], can be taken from rolls.
Every sequence of rolls of length 2, \[1, 1\], \[1, 2\], ..., \[4, 4\], can be taken from rolls.
The sequence \[1, 4, 2\] cannot be taken from rolls, so we return 3.
Note that there are other sequences that cannot be taken from rolls.
**Example 2:**
**Input:** rolls = \[1,1,2,2\], k = 2
**Output:** 2
**Explanation:** Every sequence of rolls of length 1, \[1\], \[2\], can be taken from rolls.
The sequence \[2, 1\] cannot be taken from rolls, so we return 2.
Note that there are other sequences that cannot be taken from rolls but \[2, 1\] is the shortest.
**Example 3:**
**Input:** rolls = \[1,1,3,2,2,2,3,3\], k = 4
**Output:** 1
**Explanation:** The sequence \[4\] cannot be taken from rolls, so we return 1.
Note that there are other sequences that cannot be taken from rolls but \[4\] is the shortest.
**Constraints:**
* `n == rolls.length`
* `1 <= n <= 105`
* `1 <= rolls[i] <= k <= 105` | null |
Beats 77.4% | first-letter-to-appear-twice | 0 | 1 | \n\n# Code\n```\nclass Solution:\n def repeatedCharacter(self, s: str) -> str:\n l=\'\'\n for i in range(len(s)):\n if s[i] in l:\n return s[i]\n else:\n l=l+s[i]\n return l \n``` | 1 | Given a string `s` consisting of lowercase English letters, return _the first letter to appear **twice**_.
**Note**:
* A letter `a` appears twice before another letter `b` if the **second** occurrence of `a` is before the **second** occurrence of `b`.
* `s` will contain at least one letter that appears twice.
**Example 1:**
**Input:** s = "abccbaacz "
**Output:** "c "
**Explanation:**
The letter 'a' appears on the indexes 0, 5 and 6.
The letter 'b' appears on the indexes 1 and 4.
The letter 'c' appears on the indexes 2, 3 and 7.
The letter 'z' appears on the index 8.
The letter 'c' is the first letter to appear twice, because out of all the letters the index of its second occurrence is the smallest.
**Example 2:**
**Input:** s = "abcdd "
**Output:** "d "
**Explanation:**
The only letter that appears twice is 'd' so we return 'd'.
**Constraints:**
* `2 <= s.length <= 100`
* `s` consists of lowercase English letters.
* `s` has at least one repeated letter. | null |
🔥100% EASY TO UNDERSTAND/SIMPLE/CLEAN🔥 | first-letter-to-appear-twice | 0 | 1 | ```\nclass Solution:\n def repeatedCharacter(self, s: str) -> str:\n seenAlready = []\n for c in s:\n if c not in seenAlready:\n seenAlready.append(c)\n else:\n return c | 0 | Given a string `s` consisting of lowercase English letters, return _the first letter to appear **twice**_.
**Note**:
* A letter `a` appears twice before another letter `b` if the **second** occurrence of `a` is before the **second** occurrence of `b`.
* `s` will contain at least one letter that appears twice.
**Example 1:**
**Input:** s = "abccbaacz "
**Output:** "c "
**Explanation:**
The letter 'a' appears on the indexes 0, 5 and 6.
The letter 'b' appears on the indexes 1 and 4.
The letter 'c' appears on the indexes 2, 3 and 7.
The letter 'z' appears on the index 8.
The letter 'c' is the first letter to appear twice, because out of all the letters the index of its second occurrence is the smallest.
**Example 2:**
**Input:** s = "abcdd "
**Output:** "d "
**Explanation:**
The only letter that appears twice is 'd' so we return 'd'.
**Constraints:**
* `2 <= s.length <= 100`
* `s` consists of lowercase English letters.
* `s` has at least one repeated letter. | null |
✅Python || Map || Easy Approach | first-letter-to-appear-twice | 0 | 1 | Algorithm:\n(1) use setS to store the letter have ever seen;\n(2) one pass the input string:\n(3) if x is already in setS:\n\t\tx is what we need (it is the first occurrence twice).\n(4) else (x is not in setS):\n\t\twe put the x into the setS to store it.\n```\nclass Solution:\n def repeatedCharacter(self, s: str) -> str:\n \n setS = set()\n\n for x in s:\n if x in setS:\n return x\n else:\n setS.add(x)\n \n``` | 19 | Given a string `s` consisting of lowercase English letters, return _the first letter to appear **twice**_.
**Note**:
* A letter `a` appears twice before another letter `b` if the **second** occurrence of `a` is before the **second** occurrence of `b`.
* `s` will contain at least one letter that appears twice.
**Example 1:**
**Input:** s = "abccbaacz "
**Output:** "c "
**Explanation:**
The letter 'a' appears on the indexes 0, 5 and 6.
The letter 'b' appears on the indexes 1 and 4.
The letter 'c' appears on the indexes 2, 3 and 7.
The letter 'z' appears on the index 8.
The letter 'c' is the first letter to appear twice, because out of all the letters the index of its second occurrence is the smallest.
**Example 2:**
**Input:** s = "abcdd "
**Output:** "d "
**Explanation:**
The only letter that appears twice is 'd' so we return 'd'.
**Constraints:**
* `2 <= s.length <= 100`
* `s` consists of lowercase English letters.
* `s` has at least one repeated letter. | null |
Python Simple Code (Hash Table , Set, List) | first-letter-to-appear-twice | 0 | 1 | **If you got help from this,... Plz Upvote .. it encourage me**\n\n# Code\n> # HashTable\n```\nclass Solution:\n def repeatedCharacter(self, s: str) -> str:\n d = {}\n for char in s:\n if char in d:\n return char\n else:\n d[char] = 1\n\n \n```\n\n> # Set\n```\nclass Solution:\n def repeatedCharacter(self, s: str) -> str:\n seen = set()\n for c in s:\n if c in seen:\n return c\n seen.add(c)\n\n\n```\n> # List\n```\nclass Solution:\n def repeatedCharacter(self, s: str) -> str:\n l = []\n for char in s:\n if char in l:\n return char\n else:\n l.append(char)\n\n\n``` | 3 | Given a string `s` consisting of lowercase English letters, return _the first letter to appear **twice**_.
**Note**:
* A letter `a` appears twice before another letter `b` if the **second** occurrence of `a` is before the **second** occurrence of `b`.
* `s` will contain at least one letter that appears twice.
**Example 1:**
**Input:** s = "abccbaacz "
**Output:** "c "
**Explanation:**
The letter 'a' appears on the indexes 0, 5 and 6.
The letter 'b' appears on the indexes 1 and 4.
The letter 'c' appears on the indexes 2, 3 and 7.
The letter 'z' appears on the index 8.
The letter 'c' is the first letter to appear twice, because out of all the letters the index of its second occurrence is the smallest.
**Example 2:**
**Input:** s = "abcdd "
**Output:** "d "
**Explanation:**
The only letter that appears twice is 'd' so we return 'd'.
**Constraints:**
* `2 <= s.length <= 100`
* `s` consists of lowercase English letters.
* `s` has at least one repeated letter. | null |
Python Elegant & Short | O(n) time & O(1) space | Set | first-letter-to-appear-twice | 0 | 1 | \tdef repeatedCharacter(self, s: str) -> str:\n\t\tseen = set()\n\n\t\tfor c in s:\n\t\t\tif c in seen:\n\t\t\t\treturn c\n\t\t\tseen.add(c)\n | 3 | Given a string `s` consisting of lowercase English letters, return _the first letter to appear **twice**_.
**Note**:
* A letter `a` appears twice before another letter `b` if the **second** occurrence of `a` is before the **second** occurrence of `b`.
* `s` will contain at least one letter that appears twice.
**Example 1:**
**Input:** s = "abccbaacz "
**Output:** "c "
**Explanation:**
The letter 'a' appears on the indexes 0, 5 and 6.
The letter 'b' appears on the indexes 1 and 4.
The letter 'c' appears on the indexes 2, 3 and 7.
The letter 'z' appears on the index 8.
The letter 'c' is the first letter to appear twice, because out of all the letters the index of its second occurrence is the smallest.
**Example 2:**
**Input:** s = "abcdd "
**Output:** "d "
**Explanation:**
The only letter that appears twice is 'd' so we return 'd'.
**Constraints:**
* `2 <= s.length <= 100`
* `s` consists of lowercase English letters.
* `s` has at least one repeated letter. | null |
Python | Easy Solution✅ | first-letter-to-appear-twice | 0 | 1 | ```\ndef repeatedCharacter(self, s: str) -> str:\n st = set()\n for i in range(len(s)): # s = "abccbaacz"\n if s[i] in st: # condition will be true when i = 3\n return s[i] # return c\n else:\n st.add(s[i]) # st = {a,b,c}\n``` | 4 | Given a string `s` consisting of lowercase English letters, return _the first letter to appear **twice**_.
**Note**:
* A letter `a` appears twice before another letter `b` if the **second** occurrence of `a` is before the **second** occurrence of `b`.
* `s` will contain at least one letter that appears twice.
**Example 1:**
**Input:** s = "abccbaacz "
**Output:** "c "
**Explanation:**
The letter 'a' appears on the indexes 0, 5 and 6.
The letter 'b' appears on the indexes 1 and 4.
The letter 'c' appears on the indexes 2, 3 and 7.
The letter 'z' appears on the index 8.
The letter 'c' is the first letter to appear twice, because out of all the letters the index of its second occurrence is the smallest.
**Example 2:**
**Input:** s = "abcdd "
**Output:** "d "
**Explanation:**
The only letter that appears twice is 'd' so we return 'd'.
**Constraints:**
* `2 <= s.length <= 100`
* `s` consists of lowercase English letters.
* `s` has at least one repeated letter. | null |
Easiest python solution to understand for beginners | equal-row-and-column-pairs | 0 | 1 | # Intuition\n<!-- Describe your first thoughts on how to solve this problem. -->\nThe problem aims to count the number of equal pairs in the given grid. One way to approach this is to compare each row in the grid with the first row and check if they are equal.\n# Approach\n<!-- Describe your approach to solving the problem. -->\n1.Store the first row of the grid in the variable column_starts_with.\n\n2.Initialize an empty list called columns to store the columns of the grid.\n\n3.Iterate through each row in the grid.\n\n4.For each row, iterate through each element and append it to the corresponding column in the columns list using the enumerate function.\n\n5.After iterating through all the rows, the columns list contains the columns of the grid.\n\n6.Initialize a variable called equal_pairs to keep track of the count of equal pairs.\n\n7.Iterate through each row in the grid.\n\n8.For each row, iterate through each element in the column_starts_with list.\n\n9.Check if the first element of the row is equal to the element in the corresponding column.\n\n10.If the condition is met, compare the entire row with the column to determine if they are equal.\n\n11.If the row is equal to the column, increment the count of equal_pairs.\nFinally, return the count of equal_pairs.\n# Complexity\n- Time complexity:$$O(n^2)$$\n<!-- Add your time complexity here, e.g. $$O(n)$$ -->\n\n- Space complexity:$$O(n^2)$$\n<!-- Add your space complexity here, e.g. $$O(n)$$ -->\n\n# Code\n```\nclass Solution:\n def equalPairs(self, grid: List[List[int]]) -> int:\n # Store the first row of the grid\n column_starts_with = grid[0]\n\n # Initialize a list to store the columns of the grid\n columns = [[] for _ in column_starts_with]\n \n # Iterate through each row of the grid\n for row in grid:\n # Iterate through each element in the row and append it to the corresponding column\n for j, element in enumerate(row):\n columns[j].append(element)\n \n # Initialize a variable to count the equal pairs\n equal_pairs = 0\n\n # Iterate through each row of the grid\n for row in grid:\n # Iterate through each element in the first row\n for j, element in enumerate(column_starts_with):\n # Check if the first element of the row is equal to the element in the corresponding column\n if row[0] == element:\n # Check if the entire row is equal to the column\n if row == columns[j]:\n equal_pairs += 1\n \n return equal_pairs\n\n\n``` | 4 | Given a **0-indexed** `n x n` integer matrix `grid`, _return the number of pairs_ `(ri, cj)` _such that row_ `ri` _and column_ `cj` _are equal_.
A row and column pair is considered equal if they contain the same elements in the same order (i.e., an equal array).
**Example 1:**
**Input:** grid = \[\[3,2,1\],\[1,7,6\],\[2,7,7\]\]
**Output:** 1
**Explanation:** There is 1 equal row and column pair:
- (Row 2, Column 1): \[2,7,7\]
**Example 2:**
**Input:** grid = \[\[3,1,2,2\],\[1,4,4,5\],\[2,4,2,2\],\[2,4,2,2\]\]
**Output:** 3
**Explanation:** There are 3 equal row and column pairs:
- (Row 0, Column 0): \[3,1,2,2\]
- (Row 2, Column 2): \[2,4,2,2\]
- (Row 3, Column 2): \[2,4,2,2\]
**Constraints:**
* `n == grid.length == grid[i].length`
* `1 <= n <= 200`
* `1 <= grid[i][j] <= 105` | null |
Understandable solution with python3 | equal-row-and-column-pairs | 0 | 1 | # Intuition\n<!-- Describe your first thoughts on how to solve this problem. -->\n\n# Approach\n<!-- Describe your approach to solving the problem. -->\n\n# Complexity\n- Time complexity:\n<!-- Add your time complexity here, e.g. $$O(n)$$ -->\n\n- Space complexity:\n<!-- Add your space complexity here, e.g. $$O(n)$$ -->\n\n# Code\n```\nclass Solution:\n def equalPairs(self, grid: List[List[int]]) -> int:\n a=0\n b=[]\n for i in range(len(grid)):\n c=[]\n for j in range(len(grid)):\n c.append(grid[j][i])\n b.append(c)\n d=0\n for i in range(len(grid)):\n for j in range(0,len(grid[i])):\n if(grid[i]==b[j]):\n d+=1\n return d\n \n\n\n \n\n \n``` | 1 | Given a **0-indexed** `n x n` integer matrix `grid`, _return the number of pairs_ `(ri, cj)` _such that row_ `ri` _and column_ `cj` _are equal_.
A row and column pair is considered equal if they contain the same elements in the same order (i.e., an equal array).
**Example 1:**
**Input:** grid = \[\[3,2,1\],\[1,7,6\],\[2,7,7\]\]
**Output:** 1
**Explanation:** There is 1 equal row and column pair:
- (Row 2, Column 1): \[2,7,7\]
**Example 2:**
**Input:** grid = \[\[3,1,2,2\],\[1,4,4,5\],\[2,4,2,2\],\[2,4,2,2\]\]
**Output:** 3
**Explanation:** There are 3 equal row and column pairs:
- (Row 0, Column 0): \[3,1,2,2\]
- (Row 2, Column 2): \[2,4,2,2\]
- (Row 3, Column 2): \[2,4,2,2\]
**Constraints:**
* `n == grid.length == grid[i].length`
* `1 <= n <= 200`
* `1 <= grid[i][j] <= 105` | null |
Python3 Solution | equal-row-and-column-pairs | 0 | 1 | \n```\nclass Solution:\n def equalPairs(self, grid: List[List[int]]) -> int:\n counter=collections.Counter()\n for row in grid:\n counter[tuple(row)]+=1\n\n\n ans=0\n n=len(grid)\n for i in range(n):\n now=[]\n for j in range(n):\n now.append(grid[j][i])\n\n ans+=counter[tuple(now)]\n\n return ans \n``` | 1 | Given a **0-indexed** `n x n` integer matrix `grid`, _return the number of pairs_ `(ri, cj)` _such that row_ `ri` _and column_ `cj` _are equal_.
A row and column pair is considered equal if they contain the same elements in the same order (i.e., an equal array).
**Example 1:**
**Input:** grid = \[\[3,2,1\],\[1,7,6\],\[2,7,7\]\]
**Output:** 1
**Explanation:** There is 1 equal row and column pair:
- (Row 2, Column 1): \[2,7,7\]
**Example 2:**
**Input:** grid = \[\[3,1,2,2\],\[1,4,4,5\],\[2,4,2,2\],\[2,4,2,2\]\]
**Output:** 3
**Explanation:** There are 3 equal row and column pairs:
- (Row 0, Column 0): \[3,1,2,2\]
- (Row 2, Column 2): \[2,4,2,2\]
- (Row 3, Column 2): \[2,4,2,2\]
**Constraints:**
* `n == grid.length == grid[i].length`
* `1 <= n <= 200`
* `1 <= grid[i][j] <= 105` | null |
Easy & Clear Solution | equal-row-and-column-pairs | 0 | 1 | \n\n# Code\n```\nclass Solution:\n def equalPairs(self, grid: List[List[int]]) -> int:\n sumrow=[]\n sumcol=[]\n n=len(grid)\n for i in range(n):\n sumrow.append(sum(grid[i]))\n s=0\n for j in range(n):\n s+=grid[j][i]\n sumcol.append(s)\n \n res=0\n for i in range(n):\n for j in range(n):\n if sumrow[i]==sumcol[j]:\n test=True\n for k in range(n):\n if grid[i][k]!=grid[k][j]:\n test=False\n break\n if test:\n res+=1\n return res\n\n``` | 5 | Given a **0-indexed** `n x n` integer matrix `grid`, _return the number of pairs_ `(ri, cj)` _such that row_ `ri` _and column_ `cj` _are equal_.
A row and column pair is considered equal if they contain the same elements in the same order (i.e., an equal array).
**Example 1:**
**Input:** grid = \[\[3,2,1\],\[1,7,6\],\[2,7,7\]\]
**Output:** 1
**Explanation:** There is 1 equal row and column pair:
- (Row 2, Column 1): \[2,7,7\]
**Example 2:**
**Input:** grid = \[\[3,1,2,2\],\[1,4,4,5\],\[2,4,2,2\],\[2,4,2,2\]\]
**Output:** 3
**Explanation:** There are 3 equal row and column pairs:
- (Row 0, Column 0): \[3,1,2,2\]
- (Row 2, Column 2): \[2,4,2,2\]
- (Row 3, Column 2): \[2,4,2,2\]
**Constraints:**
* `n == grid.length == grid[i].length`
* `1 <= n <= 200`
* `1 <= grid[i][j] <= 105` | null |
Java✔ || C++✔ ||Python✔ || Easy To Understand | equal-row-and-column-pairs | 1 | 1 | # Guys Please Vote up )):\nTo solve this problem, we can iterate through each row and column of the grid and check if they contain the same elements in the same order. If they do, we increment a counter. Finally, we return the value of the counter.\n\n# Approach\nHere\'s the approach to solve the problem:\n\n Initialize a variable count to 0 to keep track of the number of equal row-column pairs.\n Iterate through each row ri from 0 to n-1:\n a. Iterate through each column cj from 0 to n-1:\n i. Check if the row ri and column cj are equal by comparing the corresponding elements.\n ii. If they are equal, increment the count variable by 1.\n Return the value of count.\n\n# Complexity\n- Time complexity:\nThe time complexity of this approach is O(n^3) because we have three nested loops: one for iterating through rows, one for iterating through columns, and one for comparing the elements. \n\n- Space complexity:\nThe space complexity is O(1) since we are using a constant amount of extra space.\n\n# Java Code\n```\nclass Solution {\n public int equalPairs(int[][] grid) {\n int pair=0;\n int temp=0;\n int row=0;\n while(temp<=grid.length-1)\n {\n HashMap<Integer,Integer> map=new HashMap<>();\n for(int j=0;j<grid.length;j++)\n {\n map.put(j,grid[row][j]);\n }\n for(int i=0;i<grid.length;i++)\n {\n int curr=0;\n for(int k=0;k<grid.length;k++)\n {\n if(map.get(k)!=grid[k][i])\n {\n curr=0;\n break;\n }\n else\n curr=1;\n }\n pair+=curr;\n }\n row++;\n temp++;\n }\n return pair;\n }\n}\n```\n# C++ Code\n```\n\nclass Solution {\npublic:\n int equalPairs(vector<vector<int>>& grid) {\n map<vector<int>, int> hashmap;\n int ans = 0;\n int row = grid.size();\n int col = grid[0].size();\n for (int i=0; i<row; i++) {\n hashmap[grid[i]]++;\n }\n for (int j=0; j<col; j++) {\n vector<int> curr;\n for (int i=0; i<row; i++) {\n curr.emplace_back(grid[i][j]);\n }\n ans += hashmap[curr];\n }\n return ans;\n }\n};\n```\n# Python3 Code\n```\nclass Solution:\n def equalPairs(self, grid: List[List[int]]) -> int:\n m = defaultdict(int)\n cnt = 0\n\n for row in grid:\n m[str(row)] += 1\n \n for i in range(len(grid[0])):\n col = []\n for j in range(len(grid)):\n col.append(grid[j][i])\n cnt += m[str(col)]\n return cnt\n\n```\n | 57 | Given a **0-indexed** `n x n` integer matrix `grid`, _return the number of pairs_ `(ri, cj)` _such that row_ `ri` _and column_ `cj` _are equal_.
A row and column pair is considered equal if they contain the same elements in the same order (i.e., an equal array).
**Example 1:**
**Input:** grid = \[\[3,2,1\],\[1,7,6\],\[2,7,7\]\]
**Output:** 1
**Explanation:** There is 1 equal row and column pair:
- (Row 2, Column 1): \[2,7,7\]
**Example 2:**
**Input:** grid = \[\[3,1,2,2\],\[1,4,4,5\],\[2,4,2,2\],\[2,4,2,2\]\]
**Output:** 3
**Explanation:** There are 3 equal row and column pairs:
- (Row 0, Column 0): \[3,1,2,2\]
- (Row 2, Column 2): \[2,4,2,2\]
- (Row 3, Column 2): \[2,4,2,2\]
**Constraints:**
* `n == grid.length == grid[i].length`
* `1 <= n <= 200`
* `1 <= grid[i][j] <= 105` | null |
✅☑[C++/C/Java/Python/JavaScript] || 2 Approaches || Beats 100% || EXPLAINED🔥 | design-a-food-rating-system | 1 | 1 | # PLEASE UPVOTE IF IT HELPED\n\n---\n\n\n# Approaches\n*(Also explained in the code)*\n\n#### ***Approaches 1(Maps with Queue)***\n1. **Food Class:** Represents a food item with its rating and name.\n\n1. **FoodRatings Class:** Manages food ratings and cuisines using maps and priority queues.\n\n - **foodRatingMap:** Stores food names mapped to their ratings.\n - **foodCuisineMap:** Maps food names to their respective cuisines.\n - **cuisineFoodMap:** Organizes food items in priority queues according to their ratings within their cuisines.\n1. **Functionalities:**\n\n - Initializes and updates food ratings and cuisines.\n - Retrieves the highest-rated food item from a specified cuisine, ensuring its current rating matches the stored rating.\n\n\n# Complexity\n- Time complexity:\n $$O(nlogn+mlog(n+m))\n\n$$\n \n\n- Space complexity:\n $$O(n+m)$$\n \n\n\n# Code\n```C++ []\nclass Food {\npublic:\n // Store the food\'s rating.\n int foodRating;\n // Store the food\'s name.\n string foodName;\n\n Food(int foodRating, string foodName) {\n this->foodRating = foodRating;\n this->foodName = foodName;\n }\n\n // Overload the less than operator for comparison\n bool operator<(const Food& other) const {\n // If food ratings are same sort on the basis of their name. (lexographically smaller name food will be on top)\n if (foodRating == other.foodRating) {\n return foodName > other.foodName;\n }\n // Sort on the basis of food rating. (bigger rating food will be on top)\n return foodRating < other.foodRating;\n }\n};\n\nclass FoodRatings {\n // Map food with its rating.\n unordered_map<string, int> foodRatingMap;\n // Map food with cuisine it belongs to.\n unordered_map<string, string> foodCuisineMap;\n \n // Store all food of a cusine in priority queue (to sort them on ratings/name)\n // Priority queue element -> Food: (foodRating, foodName)\n unordered_map<string, priority_queue<Food>> cuisineFoodMap;\n\npublic:\n FoodRatings(vector<string>& foods, vector<string>& cuisines, vector<int>& ratings) {\n for (int i = 0; i < foods.size(); ++i) {\n // Store \'rating\' and \'cuisine\' of current \'food\' in \'foodRatingMap\' and \'foodCuisineMap\' maps.\n foodRatingMap[foods[i]] = ratings[i];\n foodCuisineMap[foods[i]] = cuisines[i];\n // Insert the \'(rating, name)\' element in current cuisine\'s priority queue.\n cuisineFoodMap[cuisines[i]].push(Food(ratings[i], foods[i]));\n }\n } \n \n void changeRating(string food, int newRating) {\n // Update food\'s rating in \'foodRating\' map.\n foodRatingMap[food] = newRating;\n // Insert the \'(new rating, name)\' element in respective cuisine\'s priority queue.\n auto cuisineName = foodCuisineMap[food];\n cuisineFoodMap[cuisineName].push(Food(newRating, food));\n }\n \n string highestRated(string cuisine) {\n // Get the highest rated \'food\' of \'cuisine\'.\n auto highestRated = cuisineFoodMap[cuisine].top();\n \n // If latest rating of \'food\' doesn\'t match with \'rating\' on which it was sorted in priority queue,\n // then we discard this element of the priority queue.\n while (foodRatingMap[highestRated.foodName] != highestRated.foodRating) {\n cuisineFoodMap[cuisine].pop();\n highestRated = cuisineFoodMap[cuisine].top();\n }\n // Return name of the highest rated \'food\' of \'cuisine\'.\n return highestRated.foodName;\n }\n};\n\n\n```\n```C []\nstruct Food {\n int foodRating;\n char foodName[50];\n};\n\n// Struct to represent FoodRatings\nstruct FoodRatings {\n char foods[100][50];\n char cuisines[100][50];\n int ratings[100];\n int size;\n};\n\nvoid initializeFoodRatings(struct FoodRatings *fr) {\n fr->size = 0;\n}\n\nvoid addFoodRating(struct FoodRatings *fr, char foodName[], char cuisine[], int rating) {\n strcpy(fr->foods[fr->size], foodName);\n strcpy(fr->cuisines[fr->size], cuisine);\n fr->ratings[fr->size] = rating;\n fr->size++;\n}\n\nchar* highestRated(struct FoodRatings *fr, char cuisine[]) {\n int maxRating = -1;\n char* highestRatedFood = "";\n\n for (int i = 0; i < fr->size; ++i) {\n if (strcmp(fr->cuisines[i], cuisine) == 0 && fr->ratings[i] > maxRating) {\n maxRating = fr->ratings[i];\n highestRatedFood = fr->foods[i];\n }\n }\n\n return highestRatedFood;\n}\n\nvoid changeRating(struct FoodRatings *fr, char foodName[], int newRating) {\n for (int i = 0; i < fr->size; ++i) {\n if (strcmp(fr->foods[i], foodName) == 0) {\n fr->ratings[i] = newRating;\n break;\n }\n }\n}\n\n\n\n\n```\n```Java []\nclass Food implements Comparable<Food> {\n // Store the food\'s rating.\n public int foodRating;\n // Store the food\'s name.\n public String foodName;\n\n public Food(int foodRating, String foodName) {\n this.foodRating = foodRating;\n this.foodName = foodName;\n }\n\n // Implement the compareTo method for comparison\n @Override\n public int compareTo(Food other) {\n // If food ratings are the same, sort based on their names (lexicographically smaller name food will be on top)\n if (foodRating == other.foodRating) {\n return foodName.compareTo(other.foodName);\n }\n // Sort based on food rating (bigger rating food will be on top)\n return -1 * Integer.compare(foodRating, other.foodRating);\n }\n}\n\nclass FoodRatings {\n // Map food with its rating.\n private Map<String, Integer> foodRatingMap;\n // Map food with the cuisine it belongs to.\n private Map<String, String> foodCuisineMap;\n \n // Store all food of a cuisine in a priority queue (to sort them on ratings/name)\n // Priority queue element -> Food: (foodRating, foodName)\n private Map<String, PriorityQueue<Food>> cuisineFoodMap;\n\n public FoodRatings(String[] foods, String[] cuisines, int[] ratings) {\n foodRatingMap = new HashMap<>();\n foodCuisineMap = new HashMap<>();\n cuisineFoodMap = new HashMap<>();\n\n for (int i = 0; i < foods.length; ++i) {\n // Store \'rating\' and \'cuisine\' of the current \'food\' in \'foodRatingMap\' and \'foodCuisineMap\' maps.\n foodRatingMap.put(foods[i], ratings[i]);\n foodCuisineMap.put(foods[i], cuisines[i]);\n // Insert the \'(rating, name)\' element into the current cuisine\'s priority queue.\n cuisineFoodMap.computeIfAbsent(cuisines[i], k -> new PriorityQueue<>()).add(new Food(ratings[i], foods[i]));\n }\n } \n \n public void changeRating(String food, int newRating) {\n // Update food\'s rating in the \'foodRating\' map.\n foodRatingMap.put(food, newRating);\n // Insert the \'(new food rating, food name)\' element into the respective cuisine\'s priority queue.\n String cuisineName = foodCuisineMap.get(food);\n cuisineFoodMap.get(cuisineName).add(new Food(newRating, food));\n }\n \n public String highestRated(String cuisine) {\n // Get the highest rated \'food\' of \'cuisine\'.\n Food highestRated = cuisineFoodMap.get(cuisine).peek();\n \n // If the latest rating of \'food\' doesn\'t match with the \'rating\' on which it was sorted in the priority queue,\n // then we discard this element from the priority queue.\n while (foodRatingMap.get(highestRated.foodName) != highestRated.foodRating) {\n cuisineFoodMap.get(cuisine).poll();\n highestRated = cuisineFoodMap.get(cuisine).peek();\n }\n \n // Return the name of the highest-rated \'food\' of \'cuisine\'.\n return highestRated.foodName;\n }\n}\n\n\n```\n```python3 []\nclass Food:\n def __init__(self, food_rating, food_name):\n # Store the food\'s rating.\n self.food_rating = food_rating\n # Store the food\'s name.\n self.food_name = food_name\n\n def __lt__(self, other):\n # Overload the less than operator for comparison.\n # If food ratings are the same, sort based on their name (lexicographically smaller name food will be on top).\n if self.food_rating == other.food_rating:\n return self.food_name < other.food_name\n # Sort based on food rating (bigger rating food will be on top).\n return self.food_rating > other.food_rating\n\nclass FoodRatings:\n def __init__(self, foods: List[str], cuisines: List[str], ratings: List[int]):\n # Map food with its rating.\n self.food_rating_map = {}\n # Map food with the cuisine it belongs to.\n self.food_cuisine_map = {}\n # Store all food of a cuisine in a priority queue (to sort them on ratings/name).\n # Priority queue element -> Food: (food_rating, food_name)\n self.cuisine_food_map = defaultdict(list)\n\n for i in range(len(foods)):\n # Store \'rating\' and \'cuisine\' of the current \'food\' in \'food_rating_map\' and \'food_cuisine_map\' maps.\n self.food_rating_map[foods[i]] = ratings[i]\n self.food_cuisine_map[foods[i]] = cuisines[i]\n # Insert the \'(rating, name)\' element into the current cuisine\'s priority queue.\n heapq.heappush(self.cuisine_food_map[cuisines[i]], Food(ratings[i], foods[i]))\n\n def changeRating(self, food: str, newRating: int) -> None:\n # Update food\'s rating in \'food_rating\' map.\n self.food_rating_map[food] = newRating\n # Insert the \'(new rating, name)\' element in the respective cuisine\'s priority queue.\n cuisineName = self.food_cuisine_map[food]\n heapq.heappush(self.cuisine_food_map[cuisineName], Food(newRating, food))\n\n def highestRated(self, cuisine: str) -> str:\n # Get the highest rated \'food\' of \'cuisine\'.\n highest_rated = self.cuisine_food_map[cuisine][0]\n\n # If the latest rating of \'food\' doesn\'t match with the \'rating\' on which it was sorted in the priority queue,\n # then we discard this element from the priority queue.\n while self.food_rating_map[highest_rated.food_name] != highest_rated.food_rating:\n heapq.heappop(self.cuisine_food_map[cuisine])\n highest_rated = self.cuisine_food_map[cuisine][0]\n\n # Return the name of the highest-rated \'food\' of \'cuisine\'.\n return highest_rated.food_name\n\n```\n\n```javascript []\nclass Food {\n constructor(foodRating, foodName) {\n this.foodRating = foodRating;\n this.foodName = foodName;\n }\n\n // Overload the less than operator for comparison\n compareTo(other) {\n if (this.foodRating === other.foodRating) {\n return this.foodName.localeCompare(other.foodName) > 0;\n }\n return this.foodRating < other.foodRating;\n }\n}\n\nclass FoodRatings {\n constructor(foods, cuisines, ratings) {\n this.foodRatingMap = new Map();\n this.foodCuisineMap = new Map();\n this.cuisineFoodMap = new Map();\n\n for (let i = 0; i < foods.length; ++i) {\n this.foodRatingMap.set(foods[i], ratings[i]);\n this.foodCuisineMap.set(foods[i], cuisines[i]);\n\n if (!this.cuisineFoodMap.has(cuisines[i])) {\n this.cuisineFoodMap.set(cuisines[i], []);\n }\n this.cuisineFoodMap.get(cuisines[i]).push(new Food(ratings[i], foods[i]));\n }\n\n for (const [cuisine, foods] of this.cuisineFoodMap) {\n foods.sort((a, b) => a.compareTo(b));\n }\n }\n\n changeRating(food, newRating) {\n this.foodRatingMap.set(food, newRating);\n const cuisineName = this.foodCuisineMap.get(food);\n const foods = this.cuisineFoodMap.get(cuisineName);\n\n for (const foodObj of foods) {\n if (foodObj.foodName === food) {\n foodObj.foodRating = newRating;\n foods.sort((a, b) => a.compareTo(b));\n break;\n }\n }\n }\n\n highestRated(cuisine) {\n const foods = this.cuisineFoodMap.get(cuisine);\n if (foods.length === 0) {\n return \'\';\n }\n\n while (this.foodRatingMap.get(foods[0].foodName) !== foods[0].foodRating) {\n foods.shift();\n }\n return foods[0].foodName;\n }\n}\n\n\n```\n\n---\n#### ***Approaches 2(Maps with Sets)***\n1. **foodRatingMap:** Maps each food item to its corresponding rating.\n1. **foodCuisineMap:** Maps each food item to the cuisine it belongs to.\n1. **cuisineFoodMap:** Associates each cuisine with a set of food items sorted by their ratings.\n###### **Functions:**\n\n1. **FoodRatings:** Constructor that initializes food ratings and cuisines, stores them in respective maps, and populates `cuisineFoodMap` with food items sorted by ratings.\n1. **changeRating:** Updates a food item\'s rating by modifying `foodRatingMap` and the corresponding set in `cuisineFoodMap`.\n1. **highestRated:** Returns the highest-rated food item for a given cuisine based on the first element in the set sorted by ratings.\n\n# Complexity\n- Time complexity:\n $$O((n+m)logn)\n\n$$\n \n\n- Space complexity:\n $$O(n)$$\n \n\n\n# Code\n```C++ []\nclass FoodRatings {\n // Map food with its rating.\n unordered_map<string, int> foodRatingMap;\n // Map food with cuisine it belongs to.\n unordered_map<string, string> foodCuisineMap;\n\n // Store all food of a cusine in set (to sort them on ratings/name)\n // Set element -> Pair: (-1 * foodRating, foodName)\n unordered_map<string, set<pair<int, string>>> cuisineFoodMap;\n\npublic:\n FoodRatings(vector<string>& foods, vector<string>& cuisines, vector<int>& ratings) {\n for (int i = 0; i < foods.size(); ++i) {\n // Store \'rating\' and \'cuisine\' of current \'food\' in \'foodRatingMap\' and \'foodCuisineMap\' maps.\n foodRatingMap[foods[i]] = ratings[i];\n foodCuisineMap[foods[i]] = cuisines[i];\n // Insert the \'(-1 * rating, name)\' element in current cuisine\'s set.\n cuisineFoodMap[cuisines[i]].insert({ -ratings[i], foods[i] });\n }\n } \n\n void changeRating(string food, int newRating) {\n // Fetch cuisine name for food.\n auto cuisineName = foodCuisineMap[food];\n\n // Find and delete the element from the respective cuisine\'s set.\n auto oldElementIterator = cuisineFoodMap[cuisineName].find({ -foodRatingMap[food], food });\n cuisineFoodMap[cuisineName].erase(oldElementIterator);\n\n // Update food\'s rating in \'foodRating\' map.\n foodRatingMap[food] = newRating;\n // Insert the \'(-1 * new rating, name)\' element in respective cuisine\'s set.\n cuisineFoodMap[cuisineName].insert({ -newRating, food });\n }\n \n string highestRated(string cuisine) {\n auto highestRated = *cuisineFoodMap[cuisine].begin();\n // Return name of the highest rated \'food\' of \'cuisine\'.\n return highestRated.second;\n }\n};\n\n\n```\n```C []\n// Define Pair struct to simulate std::pair in C++\ntypedef struct Pair {\n int first;\n char second[50];\n} Pair;\n\n// Define Set struct to simulate set<pair<int, string>> in C++\ntypedef struct Set {\n Pair elements[100]; // Assuming a maximum of 100 elements in the set\n int size;\n} Set;\n\n// Define the FoodRatings struct to simulate the class in C++\ntypedef struct FoodRatings {\n // Map food with its rating\n int foodRatingMap[100]; // Assuming a maximum of 100 food items\n // Map food with cuisine it belongs to\n char foodCuisineMap[100][50]; // Assuming a maximum of 100 food items and 50-character cuisine names\n\n // Store all food of a cuisine in a set (to sort them on ratings/name)\n Set cuisineFoodMap[100]; // Assuming a maximum of 100 cuisines\n int mapSize; // Size of the cuisineFoodMap\n} FoodRatings;\n\nvoid initFoodRatings(FoodRatings* foodRatings, char foods[][50], char cuisines[][50], int* ratings, int size) {\n foodRatings->mapSize = 0;\n for (int i = 0; i < size; ++i) {\n // Store \'rating\' and \'cuisine\' of current \'food\' in \'foodRatingMap\' and \'foodCuisineMap\' maps\n foodRatings->foodRatingMap[i] = ratings[i];\n strcpy(foodRatings->foodCuisineMap[i], cuisines[i]);\n // Insert the \'(-1 * rating, name)\' element in current cuisine\'s set\n foodRatings->cuisineFoodMap[foodRatings->mapSize].elements[0].first = -ratings[i];\n strcpy(foodRatings->cuisineFoodMap[foodRatings->mapSize].elements[0].second, foods[i]);\n foodRatings->cuisineFoodMap[foodRatings->mapSize].size++;\n foodRatings->mapSize++;\n }\n}\n\nvoid changeRating(FoodRatings* foodRatings, char* food, int newRating) {\n // Fetch cuisine name for food\n char cuisineName[50];\n for (int i = 0; i < 100; ++i) {\n if (strcmp(foodRatings->foodCuisineMap[i], food) == 0) {\n strcpy(cuisineName, foodRatings->foodCuisineMap[i]);\n break;\n }\n }\n\n // Find and delete the element from the respective cuisine\'s set\n int idx;\n for (int i = 0; i < foodRatings->cuisineFoodMap->size; ++i) {\n if (strcmp(foodRatings->cuisineFoodMap[i].elements[i].second, food) == 0) {\n idx = i;\n break;\n }\n }\n for (int i = idx; i < foodRatings->cuisineFoodMap->size - 1; ++i) {\n foodRatings->cuisineFoodMap[i] = foodRatings->cuisineFoodMap[i + 1];\n }\n foodRatings->cuisineFoodMap->size--;\n\n // Update food\'s rating in \'foodRating\' map\n for (int i = 0; i < 100; ++i) {\n if (strcmp(foodRatings->foodCuisineMap[i], food) == 0) {\n foodRatings->foodRatingMap[i] = newRating;\n break;\n }\n }\n\n // Insert the \'(-1 * new rating, name)\' element in respective cuisine\'s set\n foodRatings->cuisineFoodMap[foodRatings->mapSize].elements[0].first = -newRating;\n strcpy(foodRatings->cuisineFoodMap[foodRatings->mapSize].elements[0].second, food);\n foodRatings->cuisineFoodMap[foodRatings->mapSize].size++;\n foodRatings->mapSize++;\n}\n\nchar* highestRated(FoodRatings* foodRatings, char* cuisine) {\n // Return name of the highest rated \'food\' of \'cuisine\'\n return foodRatings->cuisineFoodMap->elements[0].second;\n}\n\n\n\n```\n```Java []\n\nclass FoodRatings {\n // Map food with its rating.\n private Map<String, Integer> foodRatingMap = new HashMap<>();\n // Map food with cuisine it belongs to.\n private Map<String, String> foodCuisineMap = new HashMap<>();\n\n // Store all food of a cuisine in set (to sort them on ratings/name)\n // Set element -> Pair: (-1 * foodRating, foodName)\n private Map<String, TreeSet<Pair<Integer, String>>> cuisineFoodMap = new HashMap<>();\n\n public FoodRatings(String[] foods, String[] cuisines, int[] ratings) {\n for (int i = 0; i < foods.length; ++i) {\n // Store \'rating\' and \'cuisine\' of current \'food\' in \'foodRatingMap\' and \'foodCuisineMap\' maps.\n foodRatingMap.put(foods[i], ratings[i]);\n foodCuisineMap.put(foods[i], cuisines[i]);\n\n // Insert the \'(-1 * rating, name)\' element in the current cuisine\'s set.\n cuisineFoodMap\n .computeIfAbsent(cuisines[i], k -> new TreeSet<>((a, b) -> {\n int compareByRating = Integer.compare(a.getKey(), b.getKey());\n if (compareByRating == 0) {\n // If ratings are equal, compare by food name (in ascending order).\n return a.getValue().compareTo(b.getValue());\n }\n return compareByRating;\n }))\n .add(new Pair(-ratings[i], foods[i]));\n }\n }\n\n public void changeRating(String food, int newRating) {\n // Fetch cuisine name for food.\n String cuisineName = foodCuisineMap.get(food);\n\n // Find and delete the element from the respective cuisine\'s set.\n TreeSet<Pair<Integer, String>> cuisineSet = cuisineFoodMap.get(cuisineName);\n Pair<Integer, String> oldElement = new Pair<>(-foodRatingMap.get(food), food);\n cuisineSet.remove(oldElement);\n\n // Update food\'s rating in \'foodRating\' map.\n foodRatingMap.put(food, newRating);\n // Insert the \'(-1 * new rating, name)\' element in the respective cuisine\'s set.\n cuisineSet.add(new Pair<>(-newRating, food));\n }\n\n public String highestRated(String cuisine) {\n Pair<Integer, String> highestRated = cuisineFoodMap.get(cuisine).first();\n // Return name of the highest rated \'food\' of \'cuisine\'.\n return highestRated.getValue();\n }\n}\n\n```\n```python3 []\nfrom sortedcontainers import SortedSet\n\nclass FoodRatings:\n def __init__(self, foods: List[str], cuisines: List[str], ratings: List[int]):\n # Map food with its rating.\n self.food_rating_map = {}\n # Map food with cuisine it belongs to.\n self.food_cuisine_map = {}\n\n # Store all food of a cuisine in a set (to sort them on ratings/name)\n # Set element -> Tuple: (-1 * food_rating, food_name)\n self.cuisine_food_map = defaultdict(SortedSet)\n\n for i in range(len(foods)):\n # Store \'rating\' and \'cuisine\' of the current \'food\' in \'food_rating_map\' and \'food_cuisine_map\' maps.\n self.food_rating_map[foods[i]] = ratings[i]\n self.food_cuisine_map[foods[i]] = cuisines[i]\n # Insert the \'(-1 * rating, name)\' element in the current cuisine\'s set.\n self.cuisine_food_map[cuisines[i]].add((-ratings[i], foods[i]))\n\n def changeRating(self, food: str, newRating: int) -> None:\n # Fetch cuisine name for food.\n cuisine_name = self.food_cuisine_map[food]\n\n # Find and delete the element from the respective cuisine\'s set.\n old_element = (-self.food_rating_map[food], food)\n self.cuisine_food_map[cuisine_name].remove(old_element)\n\n # Update food\'s rating in \'food_rating\' map.\n self.food_rating_map[food] = newRating\n # Insert the \'(-1 * new rating, name)\' element in the respective cuisine\'s set.\n self.cuisine_food_map[cuisine_name].add((-newRating, food))\n\n def highestRated(self, cuisine: str) -> str:\n highest_rated = self.cuisine_food_map[cuisine][0]\n # Return name of the highest-rated \'food\' of \'cuisine\'.\n return highest_rated[1]\n\n```\n\n```javascript []\n\n// In JavaScript, the equivalent implementation using objects and arrays would be:\nclass FoodRatings {\n constructor(foods, cuisines, ratings) {\n this.foodRatingMap = {};\n this.foodCuisineMap = {};\n this.cuisineFoodMap = {};\n\n for (let i = 0; i < foods.length; ++i) {\n this.foodRatingMap[foods[i]] = ratings[i];\n this.foodCuisineMap[foods[i]] = cuisines[i];\n\n if (!this.cuisineFoodMap[cuisines[i]]) {\n this.cuisineFoodMap[cuisines[i]] = [];\n }\n this.cuisineFoodMap[cuisines[i]].push({ rating: -ratings[i], name: foods[i] });\n }\n for (let key in this.cuisineFoodMap) {\n this.cuisineFoodMap[key].sort((a, b) => a.rating - b.rating);\n }\n }\n\n changeRating(food, newRating) {\n const cuisineName = this.foodCuisineMap[food];\n const cuisineArr = this.cuisineFoodMap[cuisineName];\n\n const idx = cuisineArr.findIndex(item => item.name === food);\n cuisineArr.splice(idx, 1);\n\n this.foodRatingMap[food] = newRating;\n this.cuisineFoodMap[cuisineName].push({ rating: -newRating, name: food });\n this.cuisineFoodMap[cuisineName].sort((a, b) => a.rating - b.rating);\n }\n\n highestRated(cuisine) {\n return this.cuisineFoodMap[cuisine][0].name;\n }\n}\n\n\n```\n\n---\n\n\n# PLEASE UPVOTE IF IT HELPED\n\n---\n---\n\n\n--- | 59 | Design a food rating system that can do the following:
* **Modify** the rating of a food item listed in the system.
* Return the highest-rated food item for a type of cuisine in the system.
Implement the `FoodRatings` class:
* `FoodRatings(String[] foods, String[] cuisines, int[] ratings)` Initializes the system. The food items are described by `foods`, `cuisines` and `ratings`, all of which have a length of `n`.
* `foods[i]` is the name of the `ith` food,
* `cuisines[i]` is the type of cuisine of the `ith` food, and
* `ratings[i]` is the initial rating of the `ith` food.
* `void changeRating(String food, int newRating)` Changes the rating of the food item with the name `food`.
* `String highestRated(String cuisine)` Returns the name of the food item that has the highest rating for the given type of `cuisine`. If there is a tie, return the item with the **lexicographically smaller** name.
Note that a string `x` is lexicographically smaller than string `y` if `x` comes before `y` in dictionary order, that is, either `x` is a prefix of `y`, or if `i` is the first position such that `x[i] != y[i]`, then `x[i]` comes before `y[i]` in alphabetic order.
**Example 1:**
**Input**
\[ "FoodRatings ", "highestRated ", "highestRated ", "changeRating ", "highestRated ", "changeRating ", "highestRated "\]
\[\[\[ "kimchi ", "miso ", "sushi ", "moussaka ", "ramen ", "bulgogi "\], \[ "korean ", "japanese ", "japanese ", "greek ", "japanese ", "korean "\], \[9, 12, 8, 15, 14, 7\]\], \[ "korean "\], \[ "japanese "\], \[ "sushi ", 16\], \[ "japanese "\], \[ "ramen ", 16\], \[ "japanese "\]\]
**Output**
\[null, "kimchi ", "ramen ", null, "sushi ", null, "ramen "\]
**Explanation**
FoodRatings foodRatings = new FoodRatings(\[ "kimchi ", "miso ", "sushi ", "moussaka ", "ramen ", "bulgogi "\], \[ "korean ", "japanese ", "japanese ", "greek ", "japanese ", "korean "\], \[9, 12, 8, 15, 14, 7\]);
foodRatings.highestRated( "korean "); // return "kimchi "
// "kimchi " is the highest rated korean food with a rating of 9.
foodRatings.highestRated( "japanese "); // return "ramen "
// "ramen " is the highest rated japanese food with a rating of 14.
foodRatings.changeRating( "sushi ", 16); // "sushi " now has a rating of 16.
foodRatings.highestRated( "japanese "); // return "sushi "
// "sushi " is the highest rated japanese food with a rating of 16.
foodRatings.changeRating( "ramen ", 16); // "ramen " now has a rating of 16.
foodRatings.highestRated( "japanese "); // return "ramen "
// Both "sushi " and "ramen " have a rating of 16.
// However, "ramen " is lexicographically smaller than "sushi ".
**Constraints:**
* `1 <= n <= 2 * 104`
* `n == foods.length == cuisines.length == ratings.length`
* `1 <= foods[i].length, cuisines[i].length <= 10`
* `foods[i]`, `cuisines[i]` consist of lowercase English letters.
* `1 <= ratings[i] <= 108`
* All the strings in `foods` are **distinct**.
* `food` will be the name of a food item in the system across all calls to `changeRating`.
* `cuisine` will be a type of cuisine of **at least one** food item in the system across all calls to `highestRated`.
* At most `2 * 104` calls **in total** will be made to `changeRating` and `highestRated`. | null |
【Video】Give me 5 minutes - How we think about a solution | design-a-food-rating-system | 1 | 1 | # Intuition\nI like sushi.\n\n---\n\n# Solution Video\n\nhttps://youtu.be/F6RAuglUOSg\n\n\u25A0 Timeline of the video\n\n`0:04` Explain constructor\n`1:21` Why -rating instead of rating?\n`4:09` Talk about changeRating\n`5:19` Time Complexity and Space Complexity\n`6:36` Step by step algorithm of my solution code\n`6:39` Python Tips - SortedList\n\n### \u2B50\uFE0F\u2B50\uFE0F Don\'t forget to subscribe to my channel! \u2B50\uFE0F\u2B50\uFE0F\n\n**\u25A0 Subscribe URL**\nhttp://www.youtube.com/channel/UC9RMNwYTL3SXCP6ShLWVFww?sub_confirmation=1\n\nSubscribers: 3,505\nMy first goal is 10,000 (It\'s far from done \uD83D\uDE05)\nThank you for your support!\n\n---\n\n# Approach\n\n## How we think about a solution\n\nI think it would be easier to understand while looking at the solution code below.\n\nLet\'s talk about constructor.\n\nWe initialize food information and sorted cuisine list. Food information is used when we change rating. We have data like this\n\n```\nself.food_info[food] = (cuisine, rating)\n```\n- Why we get food, cuisine and rating together?\n\nThat\'s because when we return the highest rated food in `highestRated`, there is possiblity that mulitple foods have the same highest rating. In that case the description says "If there is a tie, return the item with the lexicographically smaller name". That\'s why there is a case where we have to compare alphabets. We should have 3 information together.\n\nTo achive that, sorted cuisine also have\n```\n(-rating, food)\n```\n\n- Why `-rating` instead of `rating`?\n\nLet\'s think about this case\n\n```\nfood, rating\nsushi, 8\nmiso, 14\nramen, 14\n```\nSorted list sorts data from small to large.\n\nIf we add (rating, food) to sorted cuisine list, we will get this list\n```\n\'japanese\': SortedList([(8, \'sushi\'), (14, \'miso\'), (14, \'ramen\')])\n```\nIf we add (-rating, food) to sorted cuisine list, we will get this list\n```\n\'japanese\': SortedList([(-14, \'miso\'), (-14, \'ramen\'), (-8, \'sushi\')])\n```\n\nWe want to return the highest rating food with $$O(1)$$ time. In that case, we have two choices.\n\n**Return the first data or the last data.**\n\nBut problem is there is possiblity that we have the same rating with different foods(`14` in this case). \n\nIn the first case, we will return `ramen`. But that is wrong answer because as I told you, the description says "If there is a tie, return the item with the lexicographically smaller name". If we follow the rule, we should return `miso` because `m` (= miso) is smaller than `r` (= ramen). we need to iterate through the list. That is not $$O(1)$$ time.\n\nOn the other hand, look at the second case. `miso` is the first data, because if we convert positive highest rating to negative, the number become the smallest. And Sorted list sorts all data lexicographically, so all we have to do is just return the first food in the list.\n\nWhen we implement `highestRated`, we can do like this.\n```\ndef highestRated(self, cuisine: str) -> str:\n return self.sorted_cuisine[cuisine][0][1]\n```\n\nSummary of constructor\n\n---\n\n\u2B50\uFE0F Points\n\nCreate `food_info` with `HashMap` and add all data\n```\nself.food_info[food] = (cuisine, rating)\n```\n\nCreate `sorted_cuisine` with `defaultdict(SortedList)` and add all data\n```\nself.sorted_cuisine[cuisine].add((-rating, food))\n```\n\n---\n\nLet\'s talk about `changeRating`.\n\n`food_info` has `cuisine` and `current rating`. Take them before update them with `new rating`.\n\nSince we have the same rating with different foods, so remove current rating food with `(-old_rating, food)` from sorted cuisine list, so that we can definitely remove the target food, because constraint says "All the strings in foods are distinct". `old_rating` may be the same but food name is definitely different, so combination of `(-old_rating, food)` is actually unique.\n\nThen add negetive new rating and food to sorted cuisine list.\n\nEasy!\uD83D\uDE04\nLet\'s see a real algorithm!\n\nToday, I don\'t have enogugh time again. So could someone help me convert Python or Java to JavaScript or C++? I want to create a video before I go out.\n\n---\n\n# Complexity\n\nThis is based on Python.\n\n### Init:\n\n- Time Complexity: $$O(N * log(N))$$ where N is the number of foods. The primary operation contributing to this time complexity is the insertion of elements into the SortedList, which has a logarithmic time complexity.\n\n- Space Complexity: $$O(N)$$ for storing food_info and sorted_cuisine.\n\n### changeRating:\n\n- Time Complexity: $$O(log(N))$$ where N is the number of foods in the specific cuisine. This is the time complexity of removing and adding elements to the SortedList.\n\n- Space Complexity: $$O(1)$$. The space used is constant.\n\n### highestRated:\n\n- Time Complexity: $$O(1)$$. Accessing the first element of the SortedList takes constant time.\n\n- Space Complexity: $$O(1)$$. The space used is constant.\n\n```python []\nfrom sortedcontainers import SortedList\n\nclass FoodRatings:\n def __init__(self, foods: List[str], cuisines: List[str], ratings: List[int]):\n self.food_info = {}\n self.sorted_cuisine = defaultdict(SortedList)\n \n for food, cuisine, rating in zip(foods, cuisines, ratings):\n self.food_info[food] = (cuisine, rating)\n self.sorted_cuisine[cuisine].add((-rating, food))\n\n def changeRating(self, food: str, newRating: int) -> None:\n cuisine, old_rating = self.food_info[food]\n self.food_info[food] = (cuisine, newRating)\n self.sorted_cuisine[cuisine].discard((-old_rating, food))\n self.sorted_cuisine[cuisine].add((-newRating, food))\n\n def highestRated(self, cuisine: str) -> str:\n return self.sorted_cuisine[cuisine][0][1]\n```\n```java []\nclass FoodRatings {\n private Map<String, Pair<String, Integer>> foodInfo = new HashMap<>();\n private Map<String, SortedSet<Pair<Integer, String>>> sortedCuisine = new HashMap<>();\n\n public FoodRatings(String[] foods, String[] cuisines, int[] ratings) {\n for (int i = 0; i < foods.length; i++) {\n String food = foods[i];\n String cuisine = cuisines[i];\n int rating = ratings[i];\n\n foodInfo.put(food, new Pair<>(cuisine, rating));\n sortedCuisine\n .computeIfAbsent(cuisine, k -> new TreeSet<>(Comparator\n .<Pair<Integer, String>, Integer>comparing(Pair::getKey)\n .reversed()\n .thenComparing(Pair::getValue)))\n .add(new Pair<>(rating, food));\n } \n }\n \n public void changeRating(String food, int newRating) {\n Pair<String, Integer> info = foodInfo.get(food);\n String cuisine = info.getKey();\n int oldRating = info.getValue();\n\n SortedSet<Pair<Integer, String>> sortedList = sortedCuisine.get(cuisine);\n sortedList.remove(new Pair<>(oldRating, food));\n sortedList.add(new Pair<>(newRating, food));\n\n foodInfo.put(food, new Pair<>(cuisine, newRating)); \n }\n \n public String highestRated(String cuisine) {\n SortedSet<Pair<Integer, String>> sortedList = sortedCuisine.get(cuisine);\n return sortedList.isEmpty() ? "" : sortedList.first().getValue(); \n }\n}\n\n```\n\n## Step by step algorithm\n\nThis is based on Python.\n\n### `__init__` Function:\n1. **Initialize Data Structures:**\n - Create an empty dictionary `food_info` to store information about each food (cuisine and rating).\n - Create a defaultdict of SortedList named `sorted_cuisine` to store foods sorted by rating for each cuisine.\n\n2. **Iterate Through Input Lists:**\n - Use a zip operation to iterate over `foods`, `cuisines`, and `ratings` simultaneously.\n - For each triplet (food, cuisine, rating):\n - Add an entry to `food_info` mapping food to a tuple of cuisine and rating.\n - Add an entry to `sorted_cuisine` mapping cuisine to a SortedList of pairs (-rating, food).\n\n### `changeRating` Function:\n1. **Get Current Information:**\n - Retrieve the current cuisine and old rating for the specified food from `food_info`.\n\n2. **Update Food Information:**\n - Update the entry in `food_info` for the specified food with the same cuisine and the new rating.\n\n3. **Update SortedList:**\n - Remove the pair (-old_rating, food) from the SortedList associated with the cuisine in `sorted_cuisine`.\n - Add the pair (-newRating, food) to the same SortedList.\n\n### `highestRated` Function:\n1. **Get Highest Rated Food:**\n - Retrieve the first element (highest rated) from the SortedList associated with the specified cuisine in `sorted_cuisine`.\n\n2. **Return Food Name:**\n - Return the food name from the retrieved pair.\n\n### Summary:\n- `__init__` initializes data structures by populating `food_info` and `sorted_cuisine` with the provided input lists.\n- `changeRating` updates the rating for a specified food, modifying both `food_info` and the associated SortedList in `sorted_cuisine`.\n- `highestRated` returns the name of the highest-rated food for a specified cuisine by accessing the first element of the associated SortedList.\n\nThis algorithm ensures that food information and cuisine ratings are efficiently stored and updated, and the highest-rated food can be quickly retrieved.\n\n\n---\n\n## Python Tips - SortedList\n\n`SortedList` is a sorted container type in Python provided by the `sortedcontainers` library. It\'s an alternative to Python\'s built-in `list` or `collections.deque` with the added benefit of maintaining the elements in sorted order. This makes it efficient for tasks that require frequent insertion, deletion, and access operations while keeping the collection sorted.\n\nHere are some key features and characteristics of `SortedList`:\n\n1. **Sorted Order:**\n - Elements in a `SortedList` are always kept in sorted order. When you iterate through the elements, they are returned in sorted order.\n\n2. **Efficient Insertions and Deletions:**\n - Inserting an element into a `SortedList` has a time complexity of O(log N), where N is the number of elements.\n - Deleting an element from a `SortedList` also has a time complexity of O(log N).\n\n3. **Fast Access to Sorted Elements:**\n - Accessing elements by index or retrieving the minimum/maximum element has a time complexity of O(1).\n\n4. **Duplicates Handling:**\n - `SortedList` allows duplicate elements, and they are placed next to each other in sorted order.\n\n5. **Custom Comparisons:**\n - You can provide custom comparison functions to define the sorting order.\n\n6. **Built-in Operations:**\n - `SortedList` supports various set-like and list-like operations, such as union, intersection, difference, indexing, slicing, and more.\n\nHere is a simple example of using `SortedList`:\n\n```python\nfrom sortedcontainers import SortedList\n\n# Create a SortedList\nsorted_list = SortedList([3, 1, 4, 1, 5, 9, 2, 6, 5, 3, 5])\n\n# Access elements\nprint(sorted_list) # Output: SortedList([1, 1, 2, 3, 3, 4, 5, 5, 5, 6, 9])\nprint(sorted_list[2]) # Output: 2\n\n# Insert and delete elements\nsorted_list.add(0)\nsorted_list.discard(3)\nprint(sorted_list) # Output: SortedList([0, 1, 1, 2, 4, 5, 5, 5, 6, 9])\n\n# Custom comparison\ncustom_sorted_list = SortedList([3, 1, 4, 1, 5, 9, 2, 6, 5, 3, 5], key=lambda x: -x)\nprint(custom_sorted_list) # Output: SortedList([9, 6, 5, 5, 5, 4, 3, 3, 2, 1, 1])\n```\n\nKeep in mind that `SortedList` may have a slight performance overhead compared to a regular `list` for very small datasets, so it\'s often chosen when the collection needs to be frequently modified and accessed in sorted order.\n\n\n---\n\nThank you for reading my post.\n\u2B50\uFE0F Please upvote it and don\'t forget to subscribe to my channel!\n\n\u25A0 Subscribe URL\nhttp://www.youtube.com/channel/UC9RMNwYTL3SXCP6ShLWVFww?sub_confirmation=1\n\n\u25A0 Twitter\nhttps://twitter.com/CodingNinjaAZ\n\n### My next daily coding challenge post and video.\n\npost\nhttps://leetcode.com/problems/maximum-product-difference-between-two-pairs/solutions/4418270/video-give-me-5-minutes-how-we-think-about-a-solution/\n\nvideo\nhttps://youtu.be/DMzedO_tGr8\n\n\u25A0 Timeline of the video\n\n`0:05` Explain solution formula\n`0:47` Talk about the first smallest and the second smallest\n`3:32` Talk about the first biggest and the second biggest\n`5:11` coding\n`8:10` Time Complexity and Space Complexity\n`8:26` Step by step algorithm of my solution code\n\n### My previous daily coding challenge post and video.\n\npost\nhttps://leetcode.com/problems/valid-anagram/solutions/4410317/video-give-me-5-minutes-4-solutions-how-we-think-about-a-solution/\n\nvideo\nhttps://youtu.be/UR9geRGBvhk\n\n\u25A0 Timeline of the video\n\n`0:03` Explain Solution 1\n`2:52` Coding of Solution 1\n`4:38` Time Complexity and Space Complexity of Solution 1\n`5:04` Step by step algorithm of my solution code of Solution 1\n`5:11` Explain Solution 2\n`8:20` Coding of Solution 2\n`10:10` Time Complexity and Space Complexity of Solution 2\n`10:21` Step by step algorithm of my solution code of Solution 2\n`10:38` Coding of Solution 3\n`12:18` Time Complexity and Space Complexity of Solution 3\n`13:15` Coding of Solution 4\n`13:37` Time Complexity and Space Complexity of Solution 4\n`13:52` Step by step algorithm of my solution code of Solution 3\n\n\n | 82 | Design a food rating system that can do the following:
* **Modify** the rating of a food item listed in the system.
* Return the highest-rated food item for a type of cuisine in the system.
Implement the `FoodRatings` class:
* `FoodRatings(String[] foods, String[] cuisines, int[] ratings)` Initializes the system. The food items are described by `foods`, `cuisines` and `ratings`, all of which have a length of `n`.
* `foods[i]` is the name of the `ith` food,
* `cuisines[i]` is the type of cuisine of the `ith` food, and
* `ratings[i]` is the initial rating of the `ith` food.
* `void changeRating(String food, int newRating)` Changes the rating of the food item with the name `food`.
* `String highestRated(String cuisine)` Returns the name of the food item that has the highest rating for the given type of `cuisine`. If there is a tie, return the item with the **lexicographically smaller** name.
Note that a string `x` is lexicographically smaller than string `y` if `x` comes before `y` in dictionary order, that is, either `x` is a prefix of `y`, or if `i` is the first position such that `x[i] != y[i]`, then `x[i]` comes before `y[i]` in alphabetic order.
**Example 1:**
**Input**
\[ "FoodRatings ", "highestRated ", "highestRated ", "changeRating ", "highestRated ", "changeRating ", "highestRated "\]
\[\[\[ "kimchi ", "miso ", "sushi ", "moussaka ", "ramen ", "bulgogi "\], \[ "korean ", "japanese ", "japanese ", "greek ", "japanese ", "korean "\], \[9, 12, 8, 15, 14, 7\]\], \[ "korean "\], \[ "japanese "\], \[ "sushi ", 16\], \[ "japanese "\], \[ "ramen ", 16\], \[ "japanese "\]\]
**Output**
\[null, "kimchi ", "ramen ", null, "sushi ", null, "ramen "\]
**Explanation**
FoodRatings foodRatings = new FoodRatings(\[ "kimchi ", "miso ", "sushi ", "moussaka ", "ramen ", "bulgogi "\], \[ "korean ", "japanese ", "japanese ", "greek ", "japanese ", "korean "\], \[9, 12, 8, 15, 14, 7\]);
foodRatings.highestRated( "korean "); // return "kimchi "
// "kimchi " is the highest rated korean food with a rating of 9.
foodRatings.highestRated( "japanese "); // return "ramen "
// "ramen " is the highest rated japanese food with a rating of 14.
foodRatings.changeRating( "sushi ", 16); // "sushi " now has a rating of 16.
foodRatings.highestRated( "japanese "); // return "sushi "
// "sushi " is the highest rated japanese food with a rating of 16.
foodRatings.changeRating( "ramen ", 16); // "ramen " now has a rating of 16.
foodRatings.highestRated( "japanese "); // return "ramen "
// Both "sushi " and "ramen " have a rating of 16.
// However, "ramen " is lexicographically smaller than "sushi ".
**Constraints:**
* `1 <= n <= 2 * 104`
* `n == foods.length == cuisines.length == ratings.length`
* `1 <= foods[i].length, cuisines[i].length <= 10`
* `foods[i]`, `cuisines[i]` consist of lowercase English letters.
* `1 <= ratings[i] <= 108`
* All the strings in `foods` are **distinct**.
* `food` will be the name of a food item in the system across all calls to `changeRating`.
* `cuisine` will be a type of cuisine of **at least one** food item in the system across all calls to `highestRated`.
* At most `2 * 104` calls **in total** will be made to `changeRating` and `highestRated`. | null |
Using maps || c++ || java || python | design-a-food-rating-system | 1 | 1 | # Intuition\n<!-- Describe your first thoughts on how to solve this problem. -->\n\n# Approach\n<!-- Describe your approach to solving the problem. -->\n\n# Complexity\n- Time complexity:\n\n Construction (__init__ method): O(N log M)\n\n N is the number of foods.\n M is the maximum number of foods for a particular cuisine.\n The loop over each food involves heap insertions, each taking O(log M) time.\n Change Rating (changeRating method): O(log M)\n\n Updating the heap involves inserting a new element, which takes O(log M) time.\n M is the maximum number of foods for a particular cuisine.\n Highest Rated (highestRated method): O(log M)\n\n Accessing the highest-rated food involves popping elements from the heap until a valid element is found, each taking O(log M) time.\n M is the maximum number of foods for a particular cuisine.\n\n\n- Space complexity:\n Construction (__init__ method): O(N + M)\n\n N is the number of foods.\n M is the maximum number of foods for a particular cuisine.\n The space complexity is dominated by the heap and hash map structures.\n Change Rating (changeRating method): O(1)\n\n The space used for updating the heap and hash map is constant.\n Highest Rated (highestRated method): O(1)\n\n The space used for accessing the highest-rated food is constant.\n```c++ []\nclass FoodRatings {\n map<string, pair<string, int>> foodToCuisineAndRating;\n map<string, map<int, set<string>>> cuisineToRatingAndFoods;\n\npublic:\n FoodRatings(vector<string>& foods, vector<string>& cuisines, vector<int>& ratings) {\n for (int i = 0; i < foods.size(); i++) {\n foodToCuisineAndRating[foods[i]] = {cuisines[i], ratings[i]};\n cuisineToRatingAndFoods[cuisines[i]][ratings[i]].insert(foods[i]);\n }\n }\n\n void changeRating(string food, int newRating) {\n auto foodInfo = foodToCuisineAndRating.find(food);\n string cuisine = foodInfo->second.first;\n int oldRating = foodInfo->second.second;\n foodInfo->second.second = newRating;\n\n cuisineToRatingAndFoods[cuisine][oldRating].erase(food);\n if (cuisineToRatingAndFoods[cuisine][oldRating].empty())\n cuisineToRatingAndFoods[cuisine].erase(oldRating);\n\n cuisineToRatingAndFoods[cuisine][newRating].insert(food);\n }\n\n string highestRated(string cuisine) {\n return *cuisineToRatingAndFoods[cuisine].rbegin()->second.begin();\n }\n};\n```\n```python []\nclass FoodRatings:\n\n def __init__(self, foods: List[str], cuisines: List[str], ratings: List[int]):\n\n self.cuisine_heap_lookup = {}\n\n self.cuisine_hash_lookup = {}\n\n self.cuisine_reverse_lookup = {}\n \n for cuisine in cuisines:\n self.cuisine_heap_lookup[cuisine] = []\n self.cuisine_hash_lookup[cuisine] = {}\n \n n = len(foods)\n for index in range(n):\n heap_lookup = self.cuisine_heap_lookup[cuisines[index]]\n hash_lookup = self.cuisine_hash_lookup[cuisines[index]]\n \n self.cuisine_reverse_lookup[foods[index]] = cuisines[index]\n heapq.heappush(heap_lookup, [-ratings[index], foods[index]])\n hash_lookup[foods[index]] = ratings[index]\n\n def changeRating(self, food: str, newRating: int) -> None:\n \n cuisine = self.cuisine_reverse_lookup[food]\n heap_lookup = self.cuisine_heap_lookup[cuisine]\n hash_lookup = self.cuisine_hash_lookup[cuisine]\n \n heapq.heappush(heap_lookup, [-newRating, food])\n hash_lookup[food] = newRating\n \n def highestRated(self, cuisine: str) -> str:\n heap_lookup = self.cuisine_heap_lookup[cuisine]\n hash_lookup = self.cuisine_hash_lookup[cuisine]\n \n while heap_lookup and -heap_lookup[0][0] != hash_lookup[heap_lookup[0][1]]:\n heapq.heappop(heap_lookup)\n \n return heap_lookup[0][1]\n\n\n```\n```java []\nclass FoodRatings {\n class Pair implements Comparable<Pair> {\n String foodName;\n int rating;\n\n Pair(String name, int rate) {\n foodName = name;\n rating = rate;\n }\n\n public int compareTo(Pair obj) {\n if (this.rating != obj.rating)\n return obj.rating - this.rating;\n\n return this.foodName.compareTo(obj.foodName);\n }\n }\n\n Map<String, PriorityQueue<Pair>> cuisineToRatingsMap;\n Map<String, Integer> foodNameToIndex;\n Map<String, String> foodToCuisine;\n Pair[] foodRatingsArray;\n\n public FoodRatings(String[] foods, String[] cuisines, int[] ratings) {\n int n = foods.length;\n\n cuisineToRatingsMap = new HashMap<>();\n foodNameToIndex = new HashMap<>();\n foodToCuisine = new HashMap<>();\n\n foodRatingsArray = new Pair[n];\n\n for (int i = 0; i < n; i++) {\n foodRatingsArray[i] = new Pair(foods[i], ratings[i]);\n\n foodNameToIndex.put(foods[i], i);\n foodToCuisine.put(foods[i], cuisines[i]);\n\n if (!cuisineToRatingsMap.containsKey(cuisines[i]))\n cuisineToRatingsMap.put(cuisines[i], new PriorityQueue<>());\n\n PriorityQueue<Pair> temp = cuisineToRatingsMap.get(cuisines[i]);\n temp.offer(foodRatingsArray[i]);\n cuisineToRatingsMap.put(cuisines[i], temp);\n }\n }\n\n public void changeRating(String food, int newRating) {\n int position = foodNameToIndex.get(food);\n\n cuisineToRatingsMap.get(foodToCuisine.get(food)).remove(foodRatingsArray[position]);\n foodRatingsArray[position].rating = newRating;\n cuisineToRatingsMap.get(foodToCuisine.get(food)).offer(foodRatingsArray[position]);\n }\n\n public String highestRated(String cuisine) {\n return cuisineToRatingsMap.get(cuisine).peek().foodName;\n }\n}\n\n```\n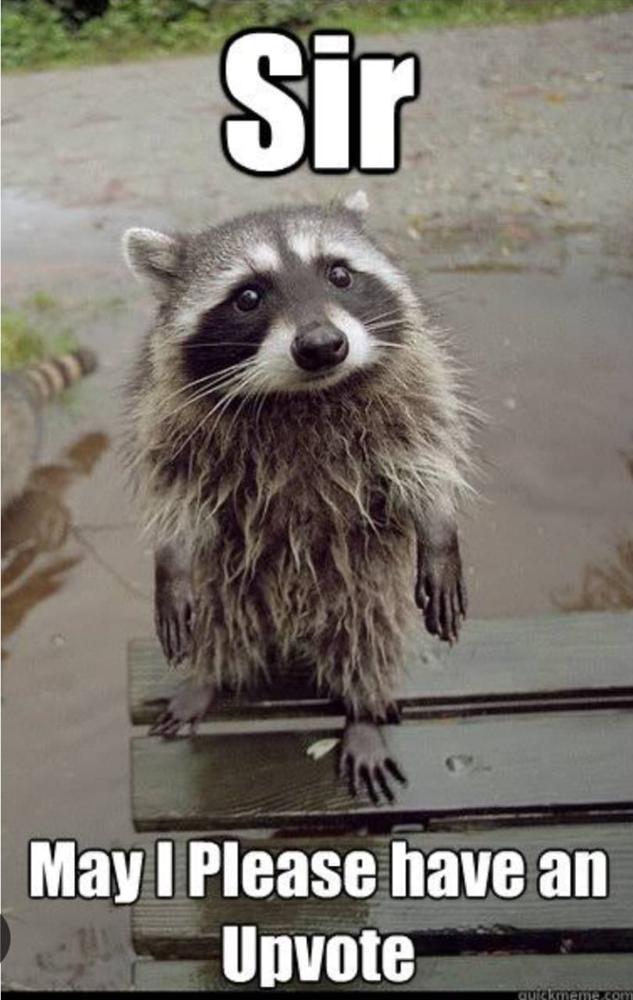\n | 52 | Design a food rating system that can do the following:
* **Modify** the rating of a food item listed in the system.
* Return the highest-rated food item for a type of cuisine in the system.
Implement the `FoodRatings` class:
* `FoodRatings(String[] foods, String[] cuisines, int[] ratings)` Initializes the system. The food items are described by `foods`, `cuisines` and `ratings`, all of which have a length of `n`.
* `foods[i]` is the name of the `ith` food,
* `cuisines[i]` is the type of cuisine of the `ith` food, and
* `ratings[i]` is the initial rating of the `ith` food.
* `void changeRating(String food, int newRating)` Changes the rating of the food item with the name `food`.
* `String highestRated(String cuisine)` Returns the name of the food item that has the highest rating for the given type of `cuisine`. If there is a tie, return the item with the **lexicographically smaller** name.
Note that a string `x` is lexicographically smaller than string `y` if `x` comes before `y` in dictionary order, that is, either `x` is a prefix of `y`, or if `i` is the first position such that `x[i] != y[i]`, then `x[i]` comes before `y[i]` in alphabetic order.
**Example 1:**
**Input**
\[ "FoodRatings ", "highestRated ", "highestRated ", "changeRating ", "highestRated ", "changeRating ", "highestRated "\]
\[\[\[ "kimchi ", "miso ", "sushi ", "moussaka ", "ramen ", "bulgogi "\], \[ "korean ", "japanese ", "japanese ", "greek ", "japanese ", "korean "\], \[9, 12, 8, 15, 14, 7\]\], \[ "korean "\], \[ "japanese "\], \[ "sushi ", 16\], \[ "japanese "\], \[ "ramen ", 16\], \[ "japanese "\]\]
**Output**
\[null, "kimchi ", "ramen ", null, "sushi ", null, "ramen "\]
**Explanation**
FoodRatings foodRatings = new FoodRatings(\[ "kimchi ", "miso ", "sushi ", "moussaka ", "ramen ", "bulgogi "\], \[ "korean ", "japanese ", "japanese ", "greek ", "japanese ", "korean "\], \[9, 12, 8, 15, 14, 7\]);
foodRatings.highestRated( "korean "); // return "kimchi "
// "kimchi " is the highest rated korean food with a rating of 9.
foodRatings.highestRated( "japanese "); // return "ramen "
// "ramen " is the highest rated japanese food with a rating of 14.
foodRatings.changeRating( "sushi ", 16); // "sushi " now has a rating of 16.
foodRatings.highestRated( "japanese "); // return "sushi "
// "sushi " is the highest rated japanese food with a rating of 16.
foodRatings.changeRating( "ramen ", 16); // "ramen " now has a rating of 16.
foodRatings.highestRated( "japanese "); // return "ramen "
// Both "sushi " and "ramen " have a rating of 16.
// However, "ramen " is lexicographically smaller than "sushi ".
**Constraints:**
* `1 <= n <= 2 * 104`
* `n == foods.length == cuisines.length == ratings.length`
* `1 <= foods[i].length, cuisines[i].length <= 10`
* `foods[i]`, `cuisines[i]` consist of lowercase English letters.
* `1 <= ratings[i] <= 108`
* All the strings in `foods` are **distinct**.
* `food` will be the name of a food item in the system across all calls to `changeRating`.
* `cuisine` will be a type of cuisine of **at least one** food item in the system across all calls to `highestRated`.
* At most `2 * 104` calls **in total** will be made to `changeRating` and `highestRated`. | null |
Python3 Solution | design-a-food-rating-system | 0 | 1 | \n```\nfrom sortedcontainers import SortedList\nclass FoodRatings:\n\n def __init__(self, foods: List[str], cuisines: List[str], ratings: List[int]):\n self.ratings={}\n self.cuisine={}\n self.by_cuisine=collections.defaultdict(lambda:SortedList())\n for food,cuisine,rating in zip(foods,cuisines,ratings):\n self.cuisine[food]=cuisine\n self.ratings[food]=rating\n self.by_cuisine[self.cuisine[food]].add((-rating,food))\n\n\n def changeRating(self, food: str, newRating: int) -> None:\n prevRating=-self.ratings[food]\n self.ratings[food]=newRating\n self.by_cuisine[self.cuisine[food]].remove((prevRating,food))\n self.by_cuisine[self.cuisine[food]].add((-newRating,food))\n\n def highestRated(self, cuisine: str) -> str:\n return self.by_cuisine[cuisine][0][1]\n\n\n# Your FoodRatings object will be instantiated and called as such:\n# obj = FoodRatings(foods, cuisines, ratings)\n# obj.changeRating(food,newRating)\n# param_2 = obj.highestRated(cuisine)\n``` | 5 | Design a food rating system that can do the following:
* **Modify** the rating of a food item listed in the system.
* Return the highest-rated food item for a type of cuisine in the system.
Implement the `FoodRatings` class:
* `FoodRatings(String[] foods, String[] cuisines, int[] ratings)` Initializes the system. The food items are described by `foods`, `cuisines` and `ratings`, all of which have a length of `n`.
* `foods[i]` is the name of the `ith` food,
* `cuisines[i]` is the type of cuisine of the `ith` food, and
* `ratings[i]` is the initial rating of the `ith` food.
* `void changeRating(String food, int newRating)` Changes the rating of the food item with the name `food`.
* `String highestRated(String cuisine)` Returns the name of the food item that has the highest rating for the given type of `cuisine`. If there is a tie, return the item with the **lexicographically smaller** name.
Note that a string `x` is lexicographically smaller than string `y` if `x` comes before `y` in dictionary order, that is, either `x` is a prefix of `y`, or if `i` is the first position such that `x[i] != y[i]`, then `x[i]` comes before `y[i]` in alphabetic order.
**Example 1:**
**Input**
\[ "FoodRatings ", "highestRated ", "highestRated ", "changeRating ", "highestRated ", "changeRating ", "highestRated "\]
\[\[\[ "kimchi ", "miso ", "sushi ", "moussaka ", "ramen ", "bulgogi "\], \[ "korean ", "japanese ", "japanese ", "greek ", "japanese ", "korean "\], \[9, 12, 8, 15, 14, 7\]\], \[ "korean "\], \[ "japanese "\], \[ "sushi ", 16\], \[ "japanese "\], \[ "ramen ", 16\], \[ "japanese "\]\]
**Output**
\[null, "kimchi ", "ramen ", null, "sushi ", null, "ramen "\]
**Explanation**
FoodRatings foodRatings = new FoodRatings(\[ "kimchi ", "miso ", "sushi ", "moussaka ", "ramen ", "bulgogi "\], \[ "korean ", "japanese ", "japanese ", "greek ", "japanese ", "korean "\], \[9, 12, 8, 15, 14, 7\]);
foodRatings.highestRated( "korean "); // return "kimchi "
// "kimchi " is the highest rated korean food with a rating of 9.
foodRatings.highestRated( "japanese "); // return "ramen "
// "ramen " is the highest rated japanese food with a rating of 14.
foodRatings.changeRating( "sushi ", 16); // "sushi " now has a rating of 16.
foodRatings.highestRated( "japanese "); // return "sushi "
// "sushi " is the highest rated japanese food with a rating of 16.
foodRatings.changeRating( "ramen ", 16); // "ramen " now has a rating of 16.
foodRatings.highestRated( "japanese "); // return "ramen "
// Both "sushi " and "ramen " have a rating of 16.
// However, "ramen " is lexicographically smaller than "sushi ".
**Constraints:**
* `1 <= n <= 2 * 104`
* `n == foods.length == cuisines.length == ratings.length`
* `1 <= foods[i].length, cuisines[i].length <= 10`
* `foods[i]`, `cuisines[i]` consist of lowercase English letters.
* `1 <= ratings[i] <= 108`
* All the strings in `foods` are **distinct**.
* `food` will be the name of a food item in the system across all calls to `changeRating`.
* `cuisine` will be a type of cuisine of **at least one** food item in the system across all calls to `highestRated`.
* At most `2 * 104` calls **in total** will be made to `changeRating` and `highestRated`. | null |
Short and Intuitive Python solution | design-a-food-rating-system | 0 | 1 | # Intuition\n<!-- Describe your first thoughts on how to solve this problem. -->\n\n# Approach\n<!-- Describe your approach to solving the problem. -->\n\n# Complexity\n- Time complexity:\n<!-- Add your time complexity here, e.g. $$O(n)$$ -->\n\n- Space complexity:\n<!-- Add your space complexity here, e.g. $$O(n)$$ -->\n\n# Code\n```\nfrom sortedcontainers import SortedSet # f.or better time complexity\nclass FoodRatings:\n def __init__(self, foods: List[str], cuisines: List[str], ratings: List[int]):\n self.map1 = defaultdict(SortedSet) # cuisine -> (rating, food)\n self.map2 = defaultdict(list) # food -> (cuisine, rating)\n for x, y, z in zip(foods, cuisines, ratings):\n self.map1[y].add((-z, x))\n self.map2[x].append((y, z))\n\n def changeRating(self, food: str, newRating: int) -> None:\n cuisine, rating = self.map2[food][0]\n self.map1[cuisine].remove((-rating, food))\n self.map1[cuisine].add((-newRating, food))\n self.map2[food][0] = (cuisine, newRating)\n\n def highestRated(self, cuisine: str) -> str:\n return self.map1[cuisine][0][1]\n\n``` | 2 | Design a food rating system that can do the following:
* **Modify** the rating of a food item listed in the system.
* Return the highest-rated food item for a type of cuisine in the system.
Implement the `FoodRatings` class:
* `FoodRatings(String[] foods, String[] cuisines, int[] ratings)` Initializes the system. The food items are described by `foods`, `cuisines` and `ratings`, all of which have a length of `n`.
* `foods[i]` is the name of the `ith` food,
* `cuisines[i]` is the type of cuisine of the `ith` food, and
* `ratings[i]` is the initial rating of the `ith` food.
* `void changeRating(String food, int newRating)` Changes the rating of the food item with the name `food`.
* `String highestRated(String cuisine)` Returns the name of the food item that has the highest rating for the given type of `cuisine`. If there is a tie, return the item with the **lexicographically smaller** name.
Note that a string `x` is lexicographically smaller than string `y` if `x` comes before `y` in dictionary order, that is, either `x` is a prefix of `y`, or if `i` is the first position such that `x[i] != y[i]`, then `x[i]` comes before `y[i]` in alphabetic order.
**Example 1:**
**Input**
\[ "FoodRatings ", "highestRated ", "highestRated ", "changeRating ", "highestRated ", "changeRating ", "highestRated "\]
\[\[\[ "kimchi ", "miso ", "sushi ", "moussaka ", "ramen ", "bulgogi "\], \[ "korean ", "japanese ", "japanese ", "greek ", "japanese ", "korean "\], \[9, 12, 8, 15, 14, 7\]\], \[ "korean "\], \[ "japanese "\], \[ "sushi ", 16\], \[ "japanese "\], \[ "ramen ", 16\], \[ "japanese "\]\]
**Output**
\[null, "kimchi ", "ramen ", null, "sushi ", null, "ramen "\]
**Explanation**
FoodRatings foodRatings = new FoodRatings(\[ "kimchi ", "miso ", "sushi ", "moussaka ", "ramen ", "bulgogi "\], \[ "korean ", "japanese ", "japanese ", "greek ", "japanese ", "korean "\], \[9, 12, 8, 15, 14, 7\]);
foodRatings.highestRated( "korean "); // return "kimchi "
// "kimchi " is the highest rated korean food with a rating of 9.
foodRatings.highestRated( "japanese "); // return "ramen "
// "ramen " is the highest rated japanese food with a rating of 14.
foodRatings.changeRating( "sushi ", 16); // "sushi " now has a rating of 16.
foodRatings.highestRated( "japanese "); // return "sushi "
// "sushi " is the highest rated japanese food with a rating of 16.
foodRatings.changeRating( "ramen ", 16); // "ramen " now has a rating of 16.
foodRatings.highestRated( "japanese "); // return "ramen "
// Both "sushi " and "ramen " have a rating of 16.
// However, "ramen " is lexicographically smaller than "sushi ".
**Constraints:**
* `1 <= n <= 2 * 104`
* `n == foods.length == cuisines.length == ratings.length`
* `1 <= foods[i].length, cuisines[i].length <= 10`
* `foods[i]`, `cuisines[i]` consist of lowercase English letters.
* `1 <= ratings[i] <= 108`
* All the strings in `foods` are **distinct**.
* `food` will be the name of a food item in the system across all calls to `changeRating`.
* `cuisine` will be a type of cuisine of **at least one** food item in the system across all calls to `highestRated`.
* At most `2 * 104` calls **in total** will be made to `changeRating` and `highestRated`. | null |
Heap-Based Solution (Python) | design-a-food-rating-system | 0 | 1 | # Code\n```\nfrom heapq import heappush, heappop\nfrom collections import defaultdict\n\nclass FoodRatings:\n\n def __init__(self, foods: List[str], cuisines: List[str], ratings: List[int]):\n self._cuisines = cuisines\n self._ratings = ratings\n self._heaps = defaultdict(list)\n for i, (f, c, r) in enumerate(zip(foods, cuisines, ratings)):\n heappush(self._heaps[c], (-r, f, i))\n\n self._food_idxs = {f: i for i, f in enumerate(foods)}\n\n def changeRating(self, food: str, newRating: int) -> None:\n idx = self._food_idxs[food]\n self._ratings[idx] = newRating\n cuisine = self._cuisines[idx]\n heappush(self._heaps[cuisine], (-newRating, food, idx))\n\n def highestRated(self, cuisine: str) -> str:\n neg_rating, food, idx = self._heaps[cuisine][0]\n while -neg_rating != self._ratings[idx]:\n heappop(self._heaps[cuisine])\n neg_rating, food, idx = self._heaps[cuisine][0]\n\n return food\n\n``` | 1 | Design a food rating system that can do the following:
* **Modify** the rating of a food item listed in the system.
* Return the highest-rated food item for a type of cuisine in the system.
Implement the `FoodRatings` class:
* `FoodRatings(String[] foods, String[] cuisines, int[] ratings)` Initializes the system. The food items are described by `foods`, `cuisines` and `ratings`, all of which have a length of `n`.
* `foods[i]` is the name of the `ith` food,
* `cuisines[i]` is the type of cuisine of the `ith` food, and
* `ratings[i]` is the initial rating of the `ith` food.
* `void changeRating(String food, int newRating)` Changes the rating of the food item with the name `food`.
* `String highestRated(String cuisine)` Returns the name of the food item that has the highest rating for the given type of `cuisine`. If there is a tie, return the item with the **lexicographically smaller** name.
Note that a string `x` is lexicographically smaller than string `y` if `x` comes before `y` in dictionary order, that is, either `x` is a prefix of `y`, or if `i` is the first position such that `x[i] != y[i]`, then `x[i]` comes before `y[i]` in alphabetic order.
**Example 1:**
**Input**
\[ "FoodRatings ", "highestRated ", "highestRated ", "changeRating ", "highestRated ", "changeRating ", "highestRated "\]
\[\[\[ "kimchi ", "miso ", "sushi ", "moussaka ", "ramen ", "bulgogi "\], \[ "korean ", "japanese ", "japanese ", "greek ", "japanese ", "korean "\], \[9, 12, 8, 15, 14, 7\]\], \[ "korean "\], \[ "japanese "\], \[ "sushi ", 16\], \[ "japanese "\], \[ "ramen ", 16\], \[ "japanese "\]\]
**Output**
\[null, "kimchi ", "ramen ", null, "sushi ", null, "ramen "\]
**Explanation**
FoodRatings foodRatings = new FoodRatings(\[ "kimchi ", "miso ", "sushi ", "moussaka ", "ramen ", "bulgogi "\], \[ "korean ", "japanese ", "japanese ", "greek ", "japanese ", "korean "\], \[9, 12, 8, 15, 14, 7\]);
foodRatings.highestRated( "korean "); // return "kimchi "
// "kimchi " is the highest rated korean food with a rating of 9.
foodRatings.highestRated( "japanese "); // return "ramen "
// "ramen " is the highest rated japanese food with a rating of 14.
foodRatings.changeRating( "sushi ", 16); // "sushi " now has a rating of 16.
foodRatings.highestRated( "japanese "); // return "sushi "
// "sushi " is the highest rated japanese food with a rating of 16.
foodRatings.changeRating( "ramen ", 16); // "ramen " now has a rating of 16.
foodRatings.highestRated( "japanese "); // return "ramen "
// Both "sushi " and "ramen " have a rating of 16.
// However, "ramen " is lexicographically smaller than "sushi ".
**Constraints:**
* `1 <= n <= 2 * 104`
* `n == foods.length == cuisines.length == ratings.length`
* `1 <= foods[i].length, cuisines[i].length <= 10`
* `foods[i]`, `cuisines[i]` consist of lowercase English letters.
* `1 <= ratings[i] <= 108`
* All the strings in `foods` are **distinct**.
* `food` will be the name of a food item in the system across all calls to `changeRating`.
* `cuisine` will be a type of cuisine of **at least one** food item in the system across all calls to `highestRated`.
* At most `2 * 104` calls **in total** will be made to `changeRating` and `highestRated`. | null |
python | O(nlogn) sorting + binary search solution explained | number-of-excellent-pairs | 0 | 1 | For me, the key insight into this problem was the realization that the test we were provided to determine if a pair is excellent can be greatly simplified. The alternate test the simplification leads to can then be reverse to quickly construct and count excellent pairs.\n\n## Checking if a Pair is Excellent\nBefore discussing how to simplify the test, I want to define a couple functions which will be helpful in this discussion:\n* **bits(n) = {the set of all \'1\' bits in the binary representation of n}**\n* **f(m, n) = |bits(m | n)| + |bits(m & n)|**\nwhere |A| is the number of elements in the set A, | is bitwise OR, and & is bitwise AND\n(also note that a pair (m, n) is excellent if f(m, n) >= k)\n\n\nNext, for a bit to be in bits(m | n) it must either be in bits(m) or bits(n). This means that **bits(m | n) = bits(m) \u222A bits(n)**. From here we can compute an alternate method to compute the size of this set, since **|bits(m) \u222A bits(n)| = |bits(m)| + |bits(n)| - |bits(m) \u2229 bits(n)|**. (basically, this means that the size of the set (bits(m) \u222A bits(n)) is the size of bits(m) plus the size of bits(n) minus any double-counting)\n\nThen, for a bit to be in bits(m & n) it must be in both bits(m) and bits(n). Or, in set notation: **bits(m & n) = bits(m) \u2229 bits(n)**. This means that **|bits(m & n)| = |bits(m) \u2229 bits(n)|**.\n\nSubbing these results into our original expression for f(m, n) gives:\n\n**f(m, n) = |bits(m | n)| + |bits(m & n)| = |bits(m)| + |bits(n)| - |bits(m) \u2229 bits(n)| + |bits(m) \u2229 bits(n)|**\n\nAnd finally, the intersection terms cancel out, leaving:\n\n**f(m, n) = |bits(m)| + |bits(n)|**\n\nThis equation is nice, because it implies that f(m, n) doesn\'t include any difficult interations between the bits of m and n, instead it only depends on how many \'1\' bits are in m and n separately, which is a much easier function to work with.\n\n## The Algorithm\nPrecomputation: O(nlogn)\n1. Throw out any duplicates since we only care about unique pairs.\n2. Compute the number of \'1\' bits for each number in nums. I\'ll call the resulting list *B*.\n3. Count the number of occurences of each value *b* in *B*. This will give us a list *counts* which contains tuples of (# bits, occurences).\n4. Sort *counts* for easier lookup later (either with a bianry sort, or a 2-pointer approach).\n5. Compute the reversed prefix sum of the occurences. This is useful, because if a collection of numbers with i bits and a collection of numbers with j bits has k or more bits, then every collection of numbers with more than j bits will also form valid pairs with numbers with i bits. So pre-computing this avoids expensive re-computation later. In my code I called this array *sums* for lack of a better name.\n\nPair Counting: O(nlogn)\n1. Select a *(b, c) = (# bits, occurences)* item from *counts*.\n2. Use a bisection search on counts to find the index *i* of the smallest existing group of numbers which for excellent pairs with numbers containing *b* bits (i.e. the smalles *i* such that b + counts[i][0] >= k).\n3. Compute the number of valid pairs which can be made where the first number has *b* bits using the expression: *c\\*sums[i]* (i.e. to make a valid group you can choose any number with *b* bits, and any number with at least *k - b* bits, so the number of pairs which start with *b* bits is equal to the number of numbers with *b* bits times the number of numbers with at least *k - b* bits).\n4. Repeat steps 1-3 for all items in *counts*, summing the result.\n\n## Code\nFirst Run Performance: 1102 ms / 32 MB\nTime Complexity: O(nlogn)\nSpace Complexity: O(n)\n\'\'\'\n\n\tclass Solution:\n\t\tdef countExcellentPairs(self, nums: List[int], k: int) -> int:\n\t\t\t# Count the Number of Bits in Each Unique Number - O(n)\n\t\t\t# Tally the Number of Times Each Bit Count Occurs - O(n)\n\t\t\t# Sort the (bit count, tally) pairs by bit count - O(nlogn)\n\t\t\tcounts = sorted(Counter(map(int.bit_count, set(nums))).items()) # (I am fully aware that this line of code is really doing too much work)\n\n\t\t\t# Compute the Reversed Prefix Sum of the Tallies (i.e. sums[i] is how many numbers have at least counts[i][0] \'1\' bits) - O(n)\n\t\t\tsums = [0]*len(counts)\n\t\t\tsums[-1] = counts[-1][1]\n\t\t\tfor i in range(len(sums) - 2, -1, -1):\n\t\t\t\tsums[i] += counts[i][1] + sums[i + 1]\n\n\t\t\t# Choose Each Number as the First Number of a Pair - O(nlogn)\n\t\t\tpairs = 0\n\t\t\tfor n, c in counts:\n\t\t\t\t# Find the Smallest Number Which Forms a Valid Pair - O(logn)\n\t\t\t\ti = bisect_left(counts, k - n, key = lambda x: x[0])\n\n\t\t\t\t# Check if Any Pairs Can be Formed\n\t\t\t\tif i < len(sums):\n\t\t\t\t\t# Compute the Number of Pairs Which Start With the Given Collection of Numbers\n\t\t\t\t\tpairs += c*sums[i]\n\n\t\t\t# Return the Number of Pairs\n\t\t\treturn pairs\n\'\'\'\n\n## Notes\nThe pair counting can also be done with a 2-pointer approach, which reduces the time complexity for that section to O(n), since each pointer makes exactly 1 pass through *counts*. | 4 | You are given a **0-indexed** positive integer array `nums` and a positive integer `k`.
A pair of numbers `(num1, num2)` is called **excellent** if the following conditions are satisfied:
* **Both** the numbers `num1` and `num2` exist in the array `nums`.
* The sum of the number of set bits in `num1 OR num2` and `num1 AND num2` is greater than or equal to `k`, where `OR` is the bitwise **OR** operation and `AND` is the bitwise **AND** operation.
Return _the number of **distinct** excellent pairs_.
Two pairs `(a, b)` and `(c, d)` are considered distinct if either `a != c` or `b != d`. For example, `(1, 2)` and `(2, 1)` are distinct.
**Note** that a pair `(num1, num2)` such that `num1 == num2` can also be excellent if you have at least **one** occurrence of `num1` in the array.
**Example 1:**
**Input:** nums = \[1,2,3,1\], k = 3
**Output:** 5
**Explanation:** The excellent pairs are the following:
- (3, 3). (3 AND 3) and (3 OR 3) are both equal to (11) in binary. The total number of set bits is 2 + 2 = 4, which is greater than or equal to k = 3.
- (2, 3) and (3, 2). (2 AND 3) is equal to (10) in binary, and (2 OR 3) is equal to (11) in binary. The total number of set bits is 1 + 2 = 3.
- (1, 3) and (3, 1). (1 AND 3) is equal to (01) in binary, and (1 OR 3) is equal to (11) in binary. The total number of set bits is 1 + 2 = 3.
So the number of excellent pairs is 5.
**Example 2:**
**Input:** nums = \[5,1,1\], k = 10
**Output:** 0
**Explanation:** There are no excellent pairs for this array.
**Constraints:**
* `1 <= nums.length <= 105`
* `1 <= nums[i] <= 109`
* `1 <= k <= 60` | When should you use your armor ability? It is always optimal to use your armor ability on the level where you take the most amount of damage. |
[Python3] Sorting Hamming Weights + Binary Search With Detailed Explanations | number-of-excellent-pairs | 0 | 1 | I have to say that the problem description is a little bit confusing, so let\'s clarify it together.\n\n**Observations & Explanations**\n1. The problem asks for the number of **distinct** excellent pairs, therefore duplicated `num` in the `nums` array does not matter and we only need to look at unique `num` appeared in the array.\n2. The excellent pair **can** include a `num` paired with itself.\n3. For any pair of bits `(b1, b2)`, where `b1` and `b2` can take `0` or `1`, we have `(b1 OR b2) + (b1 AND b2) = b1 + b2`. Specifically,\n* \t`(0 OR 0) + (0 AND 0) = 0 + 0 = 0 = 0 + 0`;\n* \t`(0 OR 1) + (0 AND 1) = 1 + 0 = 1 = 0 + 1`;\n* \t`(1 OR 0) + (1 AND 0) = 1 + 0 = 1 = 1 + 0`;\n* \t`(1 OR 1) + (1 AND 1) = 1 + 1 = 2 = 1 + 1`.\n4. From above, we have that for any pair `(num1, num2)`, the sum of the number of set bits in `num1 OR num2` and `num1 AND num2` is the same as the number of set bits in `num1` and `num2` themselves. Therefore, we don\'t actually need to calculate the `OR/AND` operations but just need to store the hamming weight, `hamming`, for each of the unique `num` in the `nums` array.\n5. After we have the `hamming` array, the problem essentially becomes a "Two Sum Greater Than K" type of problem, where we can sort the `hamming` array first, and then use a binary search to get the number of "Excellent Pairs" in the original `nums` array.\n\n**Complexity Analysis**\nTime: `O(NlogN + NlogM)`, where `M = max(nums)`.\nSpace: `O(N)`, for storing the hamming weights;\n\n**Solution**\nPlease upvote if you find this solution helpful. Thanks!\n```\nclass Solution:\n def countExcellentPairs(self, nums: List[int], k: int) -> int:\n hamming = sorted([self.hammingWeight(num) for num in set(nums)])\n ans = 0\n for h in hamming:\n ans += len(hamming) - bisect.bisect_left(hamming, k - h)\n return ans\n \n def hammingWeight(self, n):\n ans = 0\n while n:\n n &= (n - 1)\n ans += 1\n return ans\n```\n\n**Remark 1**: Above is a solution (~2000 ms) implemented with binary search after sorting the `hamming` array. One can also use two pointers to get the number of "Excellent Pairs" in the original `nums` array. However, the time complexity would not change since sorting the `hamming` array requires `O(NlogN)` time complexity.\n\n**Remark 2**: Shorter and faster solution (1435 ms) using Python 3.10\'s [new built-in method](https://docs.python.org/3/library/stdtypes.html#int.bit_count) `int.bit_count()`.\n```\nclass Solution:\n def countExcellentPairs(self, nums: List[int], k: int) -> int:\n hamming = sorted([num.bit_count() for num in set(nums)])\n ans = 0\n for h in hamming:\n ans += len(hamming) - bisect.bisect_left(hamming, k - h)\n return ans\n```\n\n**Remark 3**: Another short and fast solution (1129 ms) using `Counter` (hashmap).\n```\nclass Solution:\n def countExcellentPairs(self, nums: List[int], k: int) -> int:\n hamming = Counter([num.bit_count() for num in set(nums)])\n ans = 0\n for h1 in hamming:\n for h2 in hamming:\n if h1 + h2 < k:\n continue\n ans += hamming[h1] * hamming[h2]\n return ans\n``` | 22 | You are given a **0-indexed** positive integer array `nums` and a positive integer `k`.
A pair of numbers `(num1, num2)` is called **excellent** if the following conditions are satisfied:
* **Both** the numbers `num1` and `num2` exist in the array `nums`.
* The sum of the number of set bits in `num1 OR num2` and `num1 AND num2` is greater than or equal to `k`, where `OR` is the bitwise **OR** operation and `AND` is the bitwise **AND** operation.
Return _the number of **distinct** excellent pairs_.
Two pairs `(a, b)` and `(c, d)` are considered distinct if either `a != c` or `b != d`. For example, `(1, 2)` and `(2, 1)` are distinct.
**Note** that a pair `(num1, num2)` such that `num1 == num2` can also be excellent if you have at least **one** occurrence of `num1` in the array.
**Example 1:**
**Input:** nums = \[1,2,3,1\], k = 3
**Output:** 5
**Explanation:** The excellent pairs are the following:
- (3, 3). (3 AND 3) and (3 OR 3) are both equal to (11) in binary. The total number of set bits is 2 + 2 = 4, which is greater than or equal to k = 3.
- (2, 3) and (3, 2). (2 AND 3) is equal to (10) in binary, and (2 OR 3) is equal to (11) in binary. The total number of set bits is 1 + 2 = 3.
- (1, 3) and (3, 1). (1 AND 3) is equal to (01) in binary, and (1 OR 3) is equal to (11) in binary. The total number of set bits is 1 + 2 = 3.
So the number of excellent pairs is 5.
**Example 2:**
**Input:** nums = \[5,1,1\], k = 10
**Output:** 0
**Explanation:** There are no excellent pairs for this array.
**Constraints:**
* `1 <= nums.length <= 105`
* `1 <= nums[i] <= 109`
* `1 <= k <= 60` | When should you use your armor ability? It is always optimal to use your armor ability on the level where you take the most amount of damage. |
Python Solution | Brute Force | Optimized | O(N) | number-of-excellent-pairs | 0 | 1 | ```\nclass Solution:\n def countExcellentPairs(self, nums: List[int], k: int) -> int:\n count=0\n n=len(nums)\n \n # Brute Force\n # s=set()\n # for i in range(n):\n # for j in range(n):\n # a=nums[i] | nums[j]\n # b=nums[i] & nums[j]\n # a_count=bin(a).count(\'1\')\n # b_count=bin(b).count(\'1\')\n # if a_count+b_count>=k and (nums[i], nums[j]) not in s:\n # s.add((nums[i], nums[j]))\n # count+=1\n # return count\n \n arr=[]\n for num in set(nums):\n arr.append(bin(num).count(\'1\'))\n arr.sort()\n l=0\n r=len(arr)-1\n ans=0\n while l<=r:\n if arr[l]+arr[r]>=k:\n ans+=(r-l)*2 + 1\n r-=1\n else:\n l+=1\n return ans\n``` | 1 | You are given a **0-indexed** positive integer array `nums` and a positive integer `k`.
A pair of numbers `(num1, num2)` is called **excellent** if the following conditions are satisfied:
* **Both** the numbers `num1` and `num2` exist in the array `nums`.
* The sum of the number of set bits in `num1 OR num2` and `num1 AND num2` is greater than or equal to `k`, where `OR` is the bitwise **OR** operation and `AND` is the bitwise **AND** operation.
Return _the number of **distinct** excellent pairs_.
Two pairs `(a, b)` and `(c, d)` are considered distinct if either `a != c` or `b != d`. For example, `(1, 2)` and `(2, 1)` are distinct.
**Note** that a pair `(num1, num2)` such that `num1 == num2` can also be excellent if you have at least **one** occurrence of `num1` in the array.
**Example 1:**
**Input:** nums = \[1,2,3,1\], k = 3
**Output:** 5
**Explanation:** The excellent pairs are the following:
- (3, 3). (3 AND 3) and (3 OR 3) are both equal to (11) in binary. The total number of set bits is 2 + 2 = 4, which is greater than or equal to k = 3.
- (2, 3) and (3, 2). (2 AND 3) is equal to (10) in binary, and (2 OR 3) is equal to (11) in binary. The total number of set bits is 1 + 2 = 3.
- (1, 3) and (3, 1). (1 AND 3) is equal to (01) in binary, and (1 OR 3) is equal to (11) in binary. The total number of set bits is 1 + 2 = 3.
So the number of excellent pairs is 5.
**Example 2:**
**Input:** nums = \[5,1,1\], k = 10
**Output:** 0
**Explanation:** There are no excellent pairs for this array.
**Constraints:**
* `1 <= nums.length <= 105`
* `1 <= nums[i] <= 109`
* `1 <= k <= 60` | When should you use your armor ability? It is always optimal to use your armor ability on the level where you take the most amount of damage. |
Python Simple Solution | number-of-excellent-pairs | 0 | 1 | ```\nclass Solution:\n def countExcellentPairs(self, nums: List[int], k: int) -> int:\n nums.sort()\n mapp = defaultdict(set)\n ans = 0\n last = None\n for i in nums:\n if i==last:\n continue\n b = format(i,\'b\').count(\'1\')\n mapp[b].add(i)\n t = k-b\n for j in range(max(0,t),31):\n ans+=len(mapp[j])*2\n if i in mapp[j]:\n ans-=1\n last = i\n return ans\n``` | 2 | You are given a **0-indexed** positive integer array `nums` and a positive integer `k`.
A pair of numbers `(num1, num2)` is called **excellent** if the following conditions are satisfied:
* **Both** the numbers `num1` and `num2` exist in the array `nums`.
* The sum of the number of set bits in `num1 OR num2` and `num1 AND num2` is greater than or equal to `k`, where `OR` is the bitwise **OR** operation and `AND` is the bitwise **AND** operation.
Return _the number of **distinct** excellent pairs_.
Two pairs `(a, b)` and `(c, d)` are considered distinct if either `a != c` or `b != d`. For example, `(1, 2)` and `(2, 1)` are distinct.
**Note** that a pair `(num1, num2)` such that `num1 == num2` can also be excellent if you have at least **one** occurrence of `num1` in the array.
**Example 1:**
**Input:** nums = \[1,2,3,1\], k = 3
**Output:** 5
**Explanation:** The excellent pairs are the following:
- (3, 3). (3 AND 3) and (3 OR 3) are both equal to (11) in binary. The total number of set bits is 2 + 2 = 4, which is greater than or equal to k = 3.
- (2, 3) and (3, 2). (2 AND 3) is equal to (10) in binary, and (2 OR 3) is equal to (11) in binary. The total number of set bits is 1 + 2 = 3.
- (1, 3) and (3, 1). (1 AND 3) is equal to (01) in binary, and (1 OR 3) is equal to (11) in binary. The total number of set bits is 1 + 2 = 3.
So the number of excellent pairs is 5.
**Example 2:**
**Input:** nums = \[5,1,1\], k = 10
**Output:** 0
**Explanation:** There are no excellent pairs for this array.
**Constraints:**
* `1 <= nums.length <= 105`
* `1 <= nums[i] <= 109`
* `1 <= k <= 60` | When should you use your armor ability? It is always optimal to use your armor ability on the level where you take the most amount of damage. |
Make Array Zero by Subtracting ... | make-array-zero-by-subtracting-equal-amounts | 0 | 1 | \n# Approach\n<!-- Describe your approach to solving the problem. -->\nThis is just a greedy approach as they said to pick an element which should be minimum ...\nSort the input array and then decrement the corresponding value everytime in the array and then continue the process till it gets zero..\n\n# Code\n```\nclass Solution:\n def minimumOperations(self, nums: List[int]) -> int:\n c=0\n i=0\n nums.sort()\n while i<len(nums) and nums[i]==0:\n i+=1\n #iterate over the entire array till the last element equals to zero....\n while nums[-1]!=0:\n value=nums[i]\n for x in range(i,len(nums)):\n nums[x]=nums[x]-value\n if(nums[x]==0):\n i+=1\n #i+=1\n print(nums)\n c+=1\n return c\n``` | 1 | You are given a non-negative integer array `nums`. In one operation, you must:
* Choose a positive integer `x` such that `x` is less than or equal to the **smallest non-zero** element in `nums`.
* Subtract `x` from every **positive** element in `nums`.
Return _the **minimum** number of operations to make every element in_ `nums` _equal to_ `0`.
**Example 1:**
**Input:** nums = \[1,5,0,3,5\]
**Output:** 3
**Explanation:**
In the first operation, choose x = 1. Now, nums = \[0,4,0,2,4\].
In the second operation, choose x = 2. Now, nums = \[0,2,0,0,2\].
In the third operation, choose x = 2. Now, nums = \[0,0,0,0,0\].
**Example 2:**
**Input:** nums = \[0\]
**Output:** 0
**Explanation:** Each element in nums is already 0 so no operations are needed.
**Constraints:**
* `1 <= nums.length <= 100`
* `0 <= nums[i] <= 100` | null |
Take it easy!! | make-array-zero-by-subtracting-equal-amounts | 0 | 1 | # Intuition\n<!-- Describe your first thoughts on how to solve this problem. -->\nThe goal is to find the minimum number of operations to make all elements in the given list unique. The operations involve removing duplicates and the number zero.\n\n# Approach\n<!-- Describe your approach to solving the problem. -->\nThe approach here involves using a set to get unique elements from the list and then removing the element \'0\' from the set. The length of the resulting set will give the minimum number of operations required.\n\n# Complexity\nTime complexity: \nO(n) - The time complexity is linear, where \nn is the length of the input list. This is because creating a set and discarding an element in a set both have linear time complexity.\n\nSpace complexity: \nO(n) - The space complexity is also linear as it depends on the number of unique elements in the input list. The set is used to store unique elements, and in the worst case, it could be as large as the input list.\n\n# Code\n```\nclass Solution:\n def minimumOperations(self, nums: List[int]) -> int:\n res=(set(nums))\n res.discard(0)\n return len(res)\n\n\n \n``` | 2 | You are given a non-negative integer array `nums`. In one operation, you must:
* Choose a positive integer `x` such that `x` is less than or equal to the **smallest non-zero** element in `nums`.
* Subtract `x` from every **positive** element in `nums`.
Return _the **minimum** number of operations to make every element in_ `nums` _equal to_ `0`.
**Example 1:**
**Input:** nums = \[1,5,0,3,5\]
**Output:** 3
**Explanation:**
In the first operation, choose x = 1. Now, nums = \[0,4,0,2,4\].
In the second operation, choose x = 2. Now, nums = \[0,2,0,0,2\].
In the third operation, choose x = 2. Now, nums = \[0,0,0,0,0\].
**Example 2:**
**Input:** nums = \[0\]
**Output:** 0
**Explanation:** Each element in nums is already 0 so no operations are needed.
**Constraints:**
* `1 <= nums.length <= 100`
* `0 <= nums[i] <= 100` | null |
Set | make-array-zero-by-subtracting-equal-amounts | 0 | 1 | Each unique number (except 0) needs one operation.\n\n**Python 3**\n```python\nclass Solution:\n def minimumOperations(self, nums: List[int]) -> int:\n return len(set(nums) - {0})\n```\n**C++**\n```cpp\nint minimumOperations(vector<int>& nums) {\n return set<int>(begin(nums), end(nums)).size() - (count(begin(nums), end(nums), 0) > 0);\n}\n``` | 28 | You are given a non-negative integer array `nums`. In one operation, you must:
* Choose a positive integer `x` such that `x` is less than or equal to the **smallest non-zero** element in `nums`.
* Subtract `x` from every **positive** element in `nums`.
Return _the **minimum** number of operations to make every element in_ `nums` _equal to_ `0`.
**Example 1:**
**Input:** nums = \[1,5,0,3,5\]
**Output:** 3
**Explanation:**
In the first operation, choose x = 1. Now, nums = \[0,4,0,2,4\].
In the second operation, choose x = 2. Now, nums = \[0,2,0,0,2\].
In the third operation, choose x = 2. Now, nums = \[0,0,0,0,0\].
**Example 2:**
**Input:** nums = \[0\]
**Output:** 0
**Explanation:** Each element in nums is already 0 so no operations are needed.
**Constraints:**
* `1 <= nums.length <= 100`
* `0 <= nums[i] <= 100` | null |
Beginner-friendly || Simple solution with Binary Search in Python3 / TypeScript | maximum-number-of-groups-entering-a-competition | 0 | 1 | # Intuition\nThe problem description is the following:\n- there\'s a list of students with `grades`\n- our goal find the **maximum count of student groups**\n\nThe groups need to follow these rules:\n1. `i` group must have size **less** than in `i+1` group\n2. sum of `grades` in `i` group must be **less** that in `i+1`\n\nThere\'re some ways to calculate the number of groups, that include **linear**, **log** and **constant time** expenses. \nWe\'d like to focus on **Binary Search**.\n\n```\n# Here is the concept, that\'s well known as Solution Space.\n# Solution Space is the range of possible variants, that\'s existing\n# for some input.\n# However in most cases we\'re only interesting in threshold or \n# extremum values such as MAX/MIN, that\'s fit \n# a someone\'s condition.\n```\n\nThe first step is to set **boundaries**, in which we\'re going to search the optimal solution.\n\n```\nleft = 1 # because the starter point is one existing group \n# (base case [1], or [1, 2])\nright = 10e5 # as the maximum constraint of students, \n# this could be a larger one\n```\n\nThen we do the **regular binary search**, defining `mid` and **check**, does the input **fit the constraint?**\nIf it\'s then, shrink the **right boundary**, otherwise shrink the **left**.\n\n# Approach\n1. set `left` and `right` constraints\n2. define `check` function, that accepts `k` and calculate number of groups by [Pascal\'s Triangle formula](https://en.wikipedia.org/wiki/Pascal%27s_triangle). \n3. do the regular **Binary Search**\n4. return `int(left) - 1`, because it finds `groups + 1` count\n\n# Complexity\n- Time complexity: **O(log n)** to perform the **Binary Search**\n\n- Space complexity: **O(1)**, we don\'t allocate extra space.\n\n# Code in Python3\n```\nclass Solution:\n def maximumGroups(self, grades: List[int]) -> int:\n def check(k):\n return ((k * (k + 1)) // 2) > len(grades) \n\n left, right = 1, 10e5\n\n while left <= right:\n mid = (left + right) // 2\n\n if check(mid):\n right = mid - 1\n else:\n left = mid + 1\n\n return int(left) - 1 \n```\n\n# Code in TypeScript\n```\nfunction maximumGroups(grades: number[]): number {\n const check = (k) => Math.floor((k * (k + 1)) / 2) > grades.length\n\n let left = 1\n let right = 10e5\n\n while (left <= right) {\n const mid = Math.floor((left + right) / 2)\n\n if (check(mid)) right = mid - 1\n else left = mid + 1\n }\n\n return left - 1\n}; \n``` | 1 | You are given a positive integer array `grades` which represents the grades of students in a university. You would like to enter **all** these students into a competition in **ordered** non-empty groups, such that the ordering meets the following conditions:
* The sum of the grades of students in the `ith` group is **less than** the sum of the grades of students in the `(i + 1)th` group, for all groups (except the last).
* The total number of students in the `ith` group is **less than** the total number of students in the `(i + 1)th` group, for all groups (except the last).
Return _the **maximum** number of groups that can be formed_.
**Example 1:**
**Input:** grades = \[10,6,12,7,3,5\]
**Output:** 3
**Explanation:** The following is a possible way to form 3 groups of students:
- 1st group has the students with grades = \[12\]. Sum of grades: 12. Student count: 1
- 2nd group has the students with grades = \[6,7\]. Sum of grades: 6 + 7 = 13. Student count: 2
- 3rd group has the students with grades = \[10,3,5\]. Sum of grades: 10 + 3 + 5 = 18. Student count: 3
It can be shown that it is not possible to form more than 3 groups.
**Example 2:**
**Input:** grades = \[8,8\]
**Output:** 1
**Explanation:** We can only form 1 group, since forming 2 groups would lead to an equal number of students in both groups.
**Constraints:**
* `1 <= grades.length <= 105`
* `1 <= grades[i] <= 105` | null |
[Python] Group students into groups of 1,2,3 ... K | Concise Solution | maximum-number-of-groups-entering-a-competition | 0 | 1 | # Intuition\nThe 2 constraints given are that each group should have more students than the previous group and the sum of grades should be more. \n\nIf we group students into groups of 1,2,3...K on a sorted array, that should take care of both the conditions. We don\'t need to explicitly sort it as we don\'t need to output the grades in each group, rather we are just asked the total max number of groups.\n\nThe formula can be further simplified as : -\n\n1 + 2 + 3 ... K <= N -> Equation (1)\n\nIn this the 1st group has 1 person, 2nd group has 2 persons, 3rd group has 3 persons and Kth group has K persons and the sum of persons in all the groups should be less than or equal to N i.e. the total number of students.\n\nThe LHS of equation (1) can be simplifies as sum of first K natural numbers\n=> ( k * (K+1) ) // 2.\n\n# Code\n```\nclass Solution:\n def maximumGroups(self, grades: List[int]) -> int:\n N, k = len(grades), 1\n while k * (k+1) // 2 <= N: \n k += 1\n return k-1\n\n\n\n\n\n\n\n``` | 1 | You are given a positive integer array `grades` which represents the grades of students in a university. You would like to enter **all** these students into a competition in **ordered** non-empty groups, such that the ordering meets the following conditions:
* The sum of the grades of students in the `ith` group is **less than** the sum of the grades of students in the `(i + 1)th` group, for all groups (except the last).
* The total number of students in the `ith` group is **less than** the total number of students in the `(i + 1)th` group, for all groups (except the last).
Return _the **maximum** number of groups that can be formed_.
**Example 1:**
**Input:** grades = \[10,6,12,7,3,5\]
**Output:** 3
**Explanation:** The following is a possible way to form 3 groups of students:
- 1st group has the students with grades = \[12\]. Sum of grades: 12. Student count: 1
- 2nd group has the students with grades = \[6,7\]. Sum of grades: 6 + 7 = 13. Student count: 2
- 3rd group has the students with grades = \[10,3,5\]. Sum of grades: 10 + 3 + 5 = 18. Student count: 3
It can be shown that it is not possible to form more than 3 groups.
**Example 2:**
**Input:** grades = \[8,8\]
**Output:** 1
**Explanation:** We can only form 1 group, since forming 2 groups would lead to an equal number of students in both groups.
**Constraints:**
* `1 <= grades.length <= 105`
* `1 <= grades[i] <= 105` | null |
Python 3 O(N) | maximum-number-of-groups-entering-a-competition | 0 | 1 | # Intuition\n<!-- Describe your first thoughts on how to solve this problem. -->\n\n# Approach\n<!-- Describe your approach to solving the problem. -->\n\n# Complexity\n- Time complexity:\n- O(N) and \n<!-- Add your time complexity here, e.g. $$O(n)$$ -->\n\n- Space complexity:\n- O(1)\n<!-- Add your space complexity here, e.g. $$O(n)$$ -->\n\n# Code\n```\nclass Solution:\n def maximumGroups(self, grades: List[int]) -> int:\n n=len(grades)\n k=0\n while n>=k+1:\n k+=1\n n-=k\n return k\n \n```\nTiem complexcity : O(1)\n`\nreturn (int)(sqrt(len(grades) * 2 + 0.25) - 0.5)\n` | 1 | You are given a positive integer array `grades` which represents the grades of students in a university. You would like to enter **all** these students into a competition in **ordered** non-empty groups, such that the ordering meets the following conditions:
* The sum of the grades of students in the `ith` group is **less than** the sum of the grades of students in the `(i + 1)th` group, for all groups (except the last).
* The total number of students in the `ith` group is **less than** the total number of students in the `(i + 1)th` group, for all groups (except the last).
Return _the **maximum** number of groups that can be formed_.
**Example 1:**
**Input:** grades = \[10,6,12,7,3,5\]
**Output:** 3
**Explanation:** The following is a possible way to form 3 groups of students:
- 1st group has the students with grades = \[12\]. Sum of grades: 12. Student count: 1
- 2nd group has the students with grades = \[6,7\]. Sum of grades: 6 + 7 = 13. Student count: 2
- 3rd group has the students with grades = \[10,3,5\]. Sum of grades: 10 + 3 + 5 = 18. Student count: 3
It can be shown that it is not possible to form more than 3 groups.
**Example 2:**
**Input:** grades = \[8,8\]
**Output:** 1
**Explanation:** We can only form 1 group, since forming 2 groups would lead to an equal number of students in both groups.
**Constraints:**
* `1 <= grades.length <= 105`
* `1 <= grades[i] <= 105` | null |
✅Python || Math || Two Easy Approaches | maximum-number-of-groups-entering-a-competition | 0 | 1 | Math:\nWe have x students.\nThere are 1 student in 1st group,\n\t\t\t\t2 students in 2nd group,\n\t\t\t\t3 students in 3rd group,\n\t\t\t\t4 students in 4th group,\n\t\t\t\t...\n\t\t\t\tn students in n-th group\n\t\t\t\nWe have n * (n + 1) / 2 students in the first n groups totally,\nwe have (n + 1) * (n + 2) / 2 students in the first (n + 1) groups totally.\n\nNow we have \n```\nn * (n + 1) / 2 < x < (n + 1) * (n + 2) / 2\n```\nWe got:\n```\n((8 * x + 1) ** 0.5 - 3) / 2 < n < ((8 * x + 1) ** 0.5 - 1) / 2\n```\nHence, \n```\nn = int( ((8 * x + 1) ** 0.5 - 1) / 2)\n```\n\nImplement:\n```\nclass Solution:\n def maximumGroups(self, grades: List[int]) -> int:\n \n x = len(grades)\n n = 0.5 * ((8 * x + 1) ** 0.5 - 1)\n ans = int(n)\n \n return ans\n```\n\nAnother Solution (more readable):\n```\nclass Solution:\n def maximumGroups(self, grades: List[int]) -> int:\n \n sortedGrades = sorted(grades)\n\n groupNums = 0\n total = 0\n while total <= len(sortedGrades):\n groupNums += 1\n total += groupNums\n return groupNums - 1\n``` | 4 | You are given a positive integer array `grades` which represents the grades of students in a university. You would like to enter **all** these students into a competition in **ordered** non-empty groups, such that the ordering meets the following conditions:
* The sum of the grades of students in the `ith` group is **less than** the sum of the grades of students in the `(i + 1)th` group, for all groups (except the last).
* The total number of students in the `ith` group is **less than** the total number of students in the `(i + 1)th` group, for all groups (except the last).
Return _the **maximum** number of groups that can be formed_.
**Example 1:**
**Input:** grades = \[10,6,12,7,3,5\]
**Output:** 3
**Explanation:** The following is a possible way to form 3 groups of students:
- 1st group has the students with grades = \[12\]. Sum of grades: 12. Student count: 1
- 2nd group has the students with grades = \[6,7\]. Sum of grades: 6 + 7 = 13. Student count: 2
- 3rd group has the students with grades = \[10,3,5\]. Sum of grades: 10 + 3 + 5 = 18. Student count: 3
It can be shown that it is not possible to form more than 3 groups.
**Example 2:**
**Input:** grades = \[8,8\]
**Output:** 1
**Explanation:** We can only form 1 group, since forming 2 groups would lead to an equal number of students in both groups.
**Constraints:**
* `1 <= grades.length <= 105`
* `1 <= grades[i] <= 105` | null |
Straighforward python code (no algorithms) beats >90% | find-closest-node-to-given-two-nodes | 0 | 1 | # Intuition\nPretty straightforward. Im still new to algorithms, so I thought I would brute force it.\n\n# Approach\nKeep 2 SETS (or lists) to record the nodes travelled by nodes 1 and 2. \n\n-If any of the nodes\' value is in **its** set, it means it has been to this exact point before and thus is following a cyclic path.(You dont need to record a cyclic path more than once right?)\n\n-If any of the nodes reaches -1, it has reached the end of its path\n\nKeep recording the nodes till any of these conditions are satisfied.If both have finished recording with no common nodes, return -1\n\nif while recording the nodes, node1 happens to be in node2\'s set or node2 happens to be in node1\'s set, return the node value. If node1 in set2 **and** node2 in set1, return the smallest value\n\n# Complexity\n- Time complexity:\nO(n)\n\n- Space complexity:\nO(n)\n\n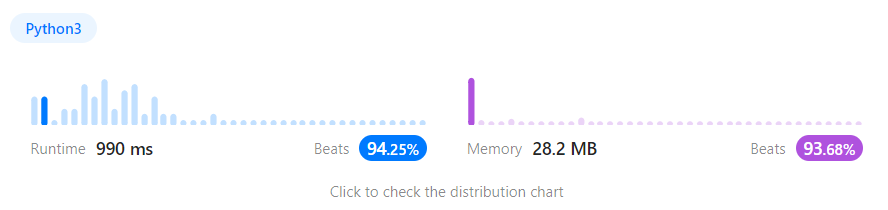\n\n\n# Code\n```\nclass Solution:\n def closestMeetingNode(self, edges: List[int], node1: int, node2: int) -> int:\n #These sets will keep track of its respective node; like browsing history ;)\n visited_1=set()\n visited_2=set()\n \n while node1!=-1 or node2!=-1:\n #record only if node is not cyclic or not at its destination\n if node1!=-1 and node1 not in visited_1:\n if node1 in visited_2:\n if node2 in visited_1:\n #if the code is here,there are 2 common values so return minimum\n return min(node1,node2)\n #if code is here then node2 NOT in visited_1 so just return node1\n return node1\n #here we record our existing node value and move to the next node\n visited_1.add(node1)\n node1=edges[node1]\n \n\n if node2!=-1 and node2 not in visited_2:\n if node2 in visited_1:\n #if the code is here,it means that node1 was not in visited2 (or it would have gone to ther other loop)\n return node2\n visited_2.add(node2)\n node2=edges[node2]\n if (node1 in visited_1 or node1==-1) and (node2 in visited_2 or node2==-1):\n break\n #if code is here, both paths have terminated with no common node\n return -1\n \n``` | 3 | You are given a **directed** graph of `n` nodes numbered from `0` to `n - 1`, where each node has **at most one** outgoing edge.
The graph is represented with a given **0-indexed** array `edges` of size `n`, indicating that there is a directed edge from node `i` to node `edges[i]`. If there is no outgoing edge from `i`, then `edges[i] == -1`.
You are also given two integers `node1` and `node2`.
Return _the **index** of the node that can be reached from both_ `node1` _and_ `node2`_, such that the **maximum** between the distance from_ `node1` _to that node, and from_ `node2` _to that node is **minimized**_. If there are multiple answers, return the node with the **smallest** index, and if no possible answer exists, return `-1`.
Note that `edges` may contain cycles.
**Example 1:**
**Input:** edges = \[2,2,3,-1\], node1 = 0, node2 = 1
**Output:** 2
**Explanation:** The distance from node 0 to node 2 is 1, and the distance from node 1 to node 2 is 1.
The maximum of those two distances is 1. It can be proven that we cannot get a node with a smaller maximum distance than 1, so we return node 2.
**Example 2:**
**Input:** edges = \[1,2,-1\], node1 = 0, node2 = 2
**Output:** 2
**Explanation:** The distance from node 0 to node 2 is 2, and the distance from node 2 to itself is 0.
The maximum of those two distances is 2. It can be proven that we cannot get a node with a smaller maximum distance than 2, so we return node 2.
**Constraints:**
* `n == edges.length`
* `2 <= n <= 105`
* `-1 <= edges[i] < n`
* `edges[i] != i`
* `0 <= node1, node2 < n` | null |
Python sol with DFS and Defaultdict | find-closest-node-to-given-two-nodes | 0 | 1 | # Intuition\n<!-- Describe your first thoughts on how to solve this problem. -->\n\n# Approach\n<!-- Describe your approach to solving the problem. -->\n\n# Complexity\n- Time complexity:\n<!-- Add your time complexity here, e.g. $$O(n)$$ -->\n\n- Space complexity:\n<!-- Add your space complexity here, e.g. $$O(n)$$ -->\n\n# Code\n```\nclass Solution:\n def closestMeetingNode(self, edges: List[int], node1: int, node2: int) -> int:\n reachable1 = defaultdict(int)\n reachable2 = defaultdict(int)\n reachable1[node1] = 0\n curr = node1\n steps = 0\n while edges[curr] != -1:\n curr = edges[curr]\n steps += 1\n if curr in reachable1:\n break\n reachable1[curr] = steps\n \n reachable2[node2] = 0\n curr = node2\n steps = 0\n while edges[curr] != -1:\n curr = edges[curr]\n steps += 1\n if curr in reachable2:\n break\n reachable2[curr] = steps\n result = float(\'inf\')\n result_node = -1\n for node in reachable1.keys():\n if node in reachable2:\n if result > max(reachable1[node], reachable2[node]):\n result = max(reachable1[node], reachable2[node])\n result_node = node\n elif result == max(reachable1[node], reachable2[node]):\n result_node = min(node, result_node)\n \n return result_node\n\n``` | 2 | You are given a **directed** graph of `n` nodes numbered from `0` to `n - 1`, where each node has **at most one** outgoing edge.
The graph is represented with a given **0-indexed** array `edges` of size `n`, indicating that there is a directed edge from node `i` to node `edges[i]`. If there is no outgoing edge from `i`, then `edges[i] == -1`.
You are also given two integers `node1` and `node2`.
Return _the **index** of the node that can be reached from both_ `node1` _and_ `node2`_, such that the **maximum** between the distance from_ `node1` _to that node, and from_ `node2` _to that node is **minimized**_. If there are multiple answers, return the node with the **smallest** index, and if no possible answer exists, return `-1`.
Note that `edges` may contain cycles.
**Example 1:**
**Input:** edges = \[2,2,3,-1\], node1 = 0, node2 = 1
**Output:** 2
**Explanation:** The distance from node 0 to node 2 is 1, and the distance from node 1 to node 2 is 1.
The maximum of those two distances is 1. It can be proven that we cannot get a node with a smaller maximum distance than 1, so we return node 2.
**Example 2:**
**Input:** edges = \[1,2,-1\], node1 = 0, node2 = 2
**Output:** 2
**Explanation:** The distance from node 0 to node 2 is 2, and the distance from node 2 to itself is 0.
The maximum of those two distances is 2. It can be proven that we cannot get a node with a smaller maximum distance than 2, so we return node 2.
**Constraints:**
* `n == edges.length`
* `2 <= n <= 105`
* `-1 <= edges[i] < n`
* `edges[i] != i`
* `0 <= node1, node2 < n` | null |
📌Python3 || ⚡915 ms, faster than 98.28% of Python3 | find-closest-node-to-given-two-nodes | 0 | 1 | \n\n# Code\n```\nclass Solution:\n def closestMeetingNode(self, edges: List[int], node1: int, node2: int) -> int:\n curr = [(node1 , 1), (node2 , 2)]\n v = [0] * len(edges)\n node = inf \n while curr:\n new = []\n f = False\n for a,w in curr:\n if v[a] == 0:\n if edges[a] != -1:\n new.append((edges[a] , w))\n v[a] = w \n elif v[a] != w:\n f = True\n node = min(a,node)\n if f:\n return node\n curr = new \n\n return -1 \n``` | 1 | You are given a **directed** graph of `n` nodes numbered from `0` to `n - 1`, where each node has **at most one** outgoing edge.
The graph is represented with a given **0-indexed** array `edges` of size `n`, indicating that there is a directed edge from node `i` to node `edges[i]`. If there is no outgoing edge from `i`, then `edges[i] == -1`.
You are also given two integers `node1` and `node2`.
Return _the **index** of the node that can be reached from both_ `node1` _and_ `node2`_, such that the **maximum** between the distance from_ `node1` _to that node, and from_ `node2` _to that node is **minimized**_. If there are multiple answers, return the node with the **smallest** index, and if no possible answer exists, return `-1`.
Note that `edges` may contain cycles.
**Example 1:**
**Input:** edges = \[2,2,3,-1\], node1 = 0, node2 = 1
**Output:** 2
**Explanation:** The distance from node 0 to node 2 is 1, and the distance from node 1 to node 2 is 1.
The maximum of those two distances is 1. It can be proven that we cannot get a node with a smaller maximum distance than 1, so we return node 2.
**Example 2:**
**Input:** edges = \[1,2,-1\], node1 = 0, node2 = 2
**Output:** 2
**Explanation:** The distance from node 0 to node 2 is 2, and the distance from node 2 to itself is 0.
The maximum of those two distances is 2. It can be proven that we cannot get a node with a smaller maximum distance than 2, so we return node 2.
**Constraints:**
* `n == edges.length`
* `2 <= n <= 105`
* `-1 <= edges[i] < n`
* `edges[i] != i`
* `0 <= node1, node2 < n` | null |
A 2 pass solution in Python (BFS + loop once to find common nodes) | find-closest-node-to-given-two-nodes | 0 | 1 | # Intuition\n1. Problem refers to graph (probably BFS/DFS solution)\n2. Mention of only one direction outedge clarifies we don\'t need to search for multiple paths\n3. What we are trying to find here is essentially a mid-point between 2 nodes. \n4. Two options\n- Do the 2 pass solution outlined below\n- Start search from both nodes at the same time, keep track of nodes visited, the first node we see that both search paths have visited, that is the node we want.\n\n\n# Approach\n1. Collect distances of all nodes from each of the two nodes\n2. Look for common nodes between these two sets of distances keeping track of best distance so far and the index of node for that best distance.\n\n\n# Complexity\n- Time complexity:\nO(n) : 2 pass to build distance dict, 1 pass to determine best distance\n\n\n# Space complexity:\nWe use the dictionary to maintain distances, which is also O(n)\n\n# Code\n```\nclass Solution:\n def closestMeetingNode(self, edges: List[int], node1: int, node2: int) -> int:\n\n\n def collect_distances(next_node):\n distance_from_node= dict() \n seen = set()\n distance = 0\n while True:\n seen.add(next_node)\n distance_from_node[next_node] = distance\n next_node = edges[next_node]\n if next_node in seen:\n break\n if next_node == -1:\n break\n distance += 1\n return distance_from_node\n\n\n distance_from_node_1 = collect_distances(node1)\n distance_from_node_2 = collect_distances(node2)\n\n import math\n best_distance = math.inf\n mid_node = -1\n for n in range(0, len(edges)):\n if n in distance_from_node_1 and n in distance_from_node_2:\n next_distance = max(distance_from_node_1[n], distance_from_node_2[n])\n if best_distance > next_distance:\n best_distance = next_distance\n mid_node = n\n return mid_node\n\n\n\n\n\n \n \n\n\n\n\n\n\n``` | 1 | You are given a **directed** graph of `n` nodes numbered from `0` to `n - 1`, where each node has **at most one** outgoing edge.
The graph is represented with a given **0-indexed** array `edges` of size `n`, indicating that there is a directed edge from node `i` to node `edges[i]`. If there is no outgoing edge from `i`, then `edges[i] == -1`.
You are also given two integers `node1` and `node2`.
Return _the **index** of the node that can be reached from both_ `node1` _and_ `node2`_, such that the **maximum** between the distance from_ `node1` _to that node, and from_ `node2` _to that node is **minimized**_. If there are multiple answers, return the node with the **smallest** index, and if no possible answer exists, return `-1`.
Note that `edges` may contain cycles.
**Example 1:**
**Input:** edges = \[2,2,3,-1\], node1 = 0, node2 = 1
**Output:** 2
**Explanation:** The distance from node 0 to node 2 is 1, and the distance from node 1 to node 2 is 1.
The maximum of those two distances is 1. It can be proven that we cannot get a node with a smaller maximum distance than 1, so we return node 2.
**Example 2:**
**Input:** edges = \[1,2,-1\], node1 = 0, node2 = 2
**Output:** 2
**Explanation:** The distance from node 0 to node 2 is 2, and the distance from node 2 to itself is 0.
The maximum of those two distances is 2. It can be proven that we cannot get a node with a smaller maximum distance than 2, so we return node 2.
**Constraints:**
* `n == edges.length`
* `2 <= n <= 105`
* `-1 <= edges[i] < n`
* `edges[i] != i`
* `0 <= node1, node2 < n` | null |
Python BFS solution Fully Commented | find-closest-node-to-given-two-nodes | 0 | 1 | # Approach\n<!-- Describe your approach to solving the problem. -->\nUse **Breadth First Search** twice to figure out the minimum distance from both ```node1``` and ```node2``` to every node.\n# Complexity\nLet $$n$$ be the number of nodes.\n- Time complexity: $$O(n)$$\n<!-- Add your time complexity here, e.g. $$O(n)$$ -->\n\n- Space complexity: $$O(n)$$\n<!-- Add your space complexity here, e.g. $$O(n)$$ -->\n\n# Code\n```\nfrom collections import deque\nclass Solution:\n def closestMeetingNode(self, edges: List[int], node1: int, node2: int) -> int:\n n = len(edges)\n # run bfs on with starting nodes on node1 and node2\n # record the min distance using cost1 and cost2\n def bfs(root, cost):\n queue = deque([(root, 0)])\n seen = {root} # to mark visited nodes\n while queue:\n node, step = queue.popleft()\n cost[node] = min(cost[node], step) # record min distance between root and node\n if edges[node] != -1 and edges[node] not in seen:\n seen.add(edges[node])\n queue.append((edges[node], step + 1))\n # initialise with large number\n cost1 = [100000]*n \n cost2 = [100000]*n\n bfs(node1, cost1)\n bfs(node2, cost2)\n # calculate the max of the two distances\n # find the minimum among them and return the node value\n ans = -1 # initialise with -1 so if there is no possible answer, return -1\n cost = 100000\n for i in range(n):\n if cost > max(cost1[i], cost2[i]):\n cost = max(cost1[i], cost2[i])\n ans = i\n return ans\n``` | 1 | You are given a **directed** graph of `n` nodes numbered from `0` to `n - 1`, where each node has **at most one** outgoing edge.
The graph is represented with a given **0-indexed** array `edges` of size `n`, indicating that there is a directed edge from node `i` to node `edges[i]`. If there is no outgoing edge from `i`, then `edges[i] == -1`.
You are also given two integers `node1` and `node2`.
Return _the **index** of the node that can be reached from both_ `node1` _and_ `node2`_, such that the **maximum** between the distance from_ `node1` _to that node, and from_ `node2` _to that node is **minimized**_. If there are multiple answers, return the node with the **smallest** index, and if no possible answer exists, return `-1`.
Note that `edges` may contain cycles.
**Example 1:**
**Input:** edges = \[2,2,3,-1\], node1 = 0, node2 = 1
**Output:** 2
**Explanation:** The distance from node 0 to node 2 is 1, and the distance from node 1 to node 2 is 1.
The maximum of those two distances is 1. It can be proven that we cannot get a node with a smaller maximum distance than 1, so we return node 2.
**Example 2:**
**Input:** edges = \[1,2,-1\], node1 = 0, node2 = 2
**Output:** 2
**Explanation:** The distance from node 0 to node 2 is 2, and the distance from node 2 to itself is 0.
The maximum of those two distances is 2. It can be proven that we cannot get a node with a smaller maximum distance than 2, so we return node 2.
**Constraints:**
* `n == edges.length`
* `2 <= n <= 105`
* `-1 <= edges[i] < n`
* `edges[i] != i`
* `0 <= node1, node2 < n` | null |
EASY PYTHON SOLUTION || BFS TRAVERSAL | find-closest-node-to-given-two-nodes | 0 | 1 | # Intuition\n<!-- Describe your first thoughts on how to solve this problem. -->\n\n# Approach\n<!-- Describe your approach to solving the problem. -->\n\n# Complexity\n- Time complexity:\n<!-- Add your time complexity here, e.g. $$O(n)$$ -->\n\n- Space complexity:\n<!-- Add your space complexity here, e.g. $$O(n)$$ -->\n\n# Code\n```\nclass Solution:\n def closestMeetingNode(self, edges: List[int], node1: int, node2: int) -> int:\n n=len(edges)\n dst1=[float("infinity")]*n\n dst2=[float("infinity")]*n\n\n dst1[node1]=0\n st=[(node1,0)]\n while st:\n x,d=st.pop(0)\n v=edges[x]\n if v!=-1 and dst1[v]==float("infinity"):\n dst1[v]=d+1\n st.append((v,d+1))\n\n dst2[node2]=0\n st=[(node2,0)]\n while st:\n x,d=st.pop(0)\n v=edges[x]\n if v!=-1 and dst2[v]==float("infinity"):\n dst2[v]=d+1\n st.append((v,d+1))\n \n lst=[max(dst1[i],dst2[i]) for i in range(n)]\n mn=0\n for i in range(n):\n if lst[i]<lst[mn]:\n mn=i\n if lst[mn]==float("infinity"):\n return -1\n return mn\n``` | 1 | You are given a **directed** graph of `n` nodes numbered from `0` to `n - 1`, where each node has **at most one** outgoing edge.
The graph is represented with a given **0-indexed** array `edges` of size `n`, indicating that there is a directed edge from node `i` to node `edges[i]`. If there is no outgoing edge from `i`, then `edges[i] == -1`.
You are also given two integers `node1` and `node2`.
Return _the **index** of the node that can be reached from both_ `node1` _and_ `node2`_, such that the **maximum** between the distance from_ `node1` _to that node, and from_ `node2` _to that node is **minimized**_. If there are multiple answers, return the node with the **smallest** index, and if no possible answer exists, return `-1`.
Note that `edges` may contain cycles.
**Example 1:**
**Input:** edges = \[2,2,3,-1\], node1 = 0, node2 = 1
**Output:** 2
**Explanation:** The distance from node 0 to node 2 is 1, and the distance from node 1 to node 2 is 1.
The maximum of those two distances is 1. It can be proven that we cannot get a node with a smaller maximum distance than 1, so we return node 2.
**Example 2:**
**Input:** edges = \[1,2,-1\], node1 = 0, node2 = 2
**Output:** 2
**Explanation:** The distance from node 0 to node 2 is 2, and the distance from node 2 to itself is 0.
The maximum of those two distances is 2. It can be proven that we cannot get a node with a smaller maximum distance than 2, so we return node 2.
**Constraints:**
* `n == edges.length`
* `2 <= n <= 105`
* `-1 <= edges[i] < n`
* `edges[i] != i`
* `0 <= node1, node2 < n` | null |
Python3 || Beats 91.90% || Short solution | longest-cycle-in-a-graph | 0 | 1 | 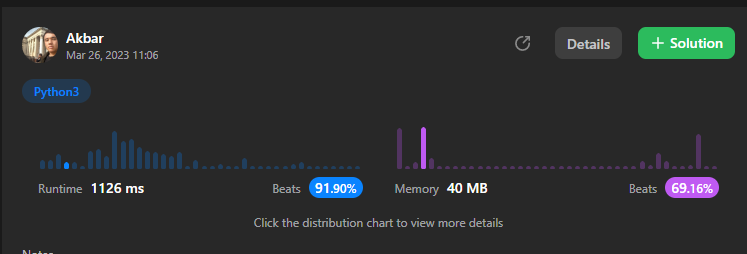\n# Please UPVOTE\uD83D\uDE0A\n\n## Python3\n```\nclass Solution:\n def longestCycle(self, edges: List[int]) -> int:\n n=len(edges)\n bl=[0]*n\n mp=defaultdict(int)\n mx=-1\n for i in range(n):\n if(bl[i]==0):\n x=i\n l=0\n st=set()\n while x>-1 and bl[x]==0:\n bl[x]=1\n mp[x]=l\n l+=1\n st.add(x)\n x=edges[x]\n if(x!=-1 and x in st): mx=max(mx,l-mp[x])\n return mx\n``` | 24 | You are given a **directed** graph of `n` nodes numbered from `0` to `n - 1`, where each node has **at most one** outgoing edge.
The graph is represented with a given **0-indexed** array `edges` of size `n`, indicating that there is a directed edge from node `i` to node `edges[i]`. If there is no outgoing edge from node `i`, then `edges[i] == -1`.
Return _the length of the **longest** cycle in the graph_. If no cycle exists, return `-1`.
A cycle is a path that starts and ends at the **same** node.
**Example 1:**
**Input:** edges = \[3,3,4,2,3\]
**Output:** 3
**Explanation:** The longest cycle in the graph is the cycle: 2 -> 4 -> 3 -> 2.
The length of this cycle is 3, so 3 is returned.
**Example 2:**
**Input:** edges = \[2,-1,3,1\]
**Output:** -1
**Explanation:** There are no cycles in this graph.
**Constraints:**
* `n == edges.length`
* `2 <= n <= 105`
* `-1 <= edges[i] < n`
* `edges[i] != i` | null |
simple solution using arival time. | longest-cycle-in-a-graph | 0 | 1 | \n```\nclass Solution:\n def longestCycle(self, edges: List[int]) -> int:\n v=[0]*len(edges)\n ans=-1\n for i in range(len(edges)):\n t=1\n c=i\n while c>=0:\n if v[c]!=0:\n if v[c][0]==i:\n ans=max(ans,t-v[c][1])\n break\n else:\n v[c]=[i,t]\n t+=1\n c=edges[c]\n return ans\n\n\n``` | 2 | You are given a **directed** graph of `n` nodes numbered from `0` to `n - 1`, where each node has **at most one** outgoing edge.
The graph is represented with a given **0-indexed** array `edges` of size `n`, indicating that there is a directed edge from node `i` to node `edges[i]`. If there is no outgoing edge from node `i`, then `edges[i] == -1`.
Return _the length of the **longest** cycle in the graph_. If no cycle exists, return `-1`.
A cycle is a path that starts and ends at the **same** node.
**Example 1:**
**Input:** edges = \[3,3,4,2,3\]
**Output:** 3
**Explanation:** The longest cycle in the graph is the cycle: 2 -> 4 -> 3 -> 2.
The length of this cycle is 3, so 3 is returned.
**Example 2:**
**Input:** edges = \[2,-1,3,1\]
**Output:** -1
**Explanation:** There are no cycles in this graph.
**Constraints:**
* `n == edges.length`
* `2 <= n <= 105`
* `-1 <= edges[i] < n`
* `edges[i] != i` | null |
[Python3] Really fast! Easy to Understand!!! Untraditional DFS | longest-cycle-in-a-graph | 0 | 1 | # Intuition\n<!-- Describe your first thoughts on how to solve this problem. -->\n\n# Approach\n<!-- Describe your approach to solving the problem. -->\n\n# Complexity\n- Time complexity:\n<!-- Add your time complexity here, e.g. $$O(n)$$ -->\n\n- Space complexity:\n<!-- Add your space complexity here, e.g. $$O(n)$$ -->\n\n# Code\n```\nclass Solution:\n def longestCycle(self, edges: List[int]) -> int:\n n=len(edges)\n visited=[0 for i in range(n)]\n visit=0\n maxlen=-1\n for i in range(n):\n if visited[i]==1:\n continue\n cycle=[i]\n while visited[cycle[-1]]!=1:\n visited[cycle[-1]]=1\n cycle.append(edges[cycle[-1]])\n visit+=1\n if cycle[-1]==-1:\n break\n if cycle[-1]==-1:\n continue\n end = cycle[-1]\n m=len(cycle)\n #print(cycle)\n #print(visited,visit)\n for i in cycle:\n m-=1\n if i==end:\n if m==0:\n break\n maxlen=max(maxlen,m)\n break\n return maxlen\n\n \n\n \n``` | 1 | You are given a **directed** graph of `n` nodes numbered from `0` to `n - 1`, where each node has **at most one** outgoing edge.
The graph is represented with a given **0-indexed** array `edges` of size `n`, indicating that there is a directed edge from node `i` to node `edges[i]`. If there is no outgoing edge from node `i`, then `edges[i] == -1`.
Return _the length of the **longest** cycle in the graph_. If no cycle exists, return `-1`.
A cycle is a path that starts and ends at the **same** node.
**Example 1:**
**Input:** edges = \[3,3,4,2,3\]
**Output:** 3
**Explanation:** The longest cycle in the graph is the cycle: 2 -> 4 -> 3 -> 2.
The length of this cycle is 3, so 3 is returned.
**Example 2:**
**Input:** edges = \[2,-1,3,1\]
**Output:** -1
**Explanation:** There are no cycles in this graph.
**Constraints:**
* `n == edges.length`
* `2 <= n <= 105`
* `-1 <= edges[i] < n`
* `edges[i] != i` | null |
Python iterative BFS Solution | O(n) solution | longest-cycle-in-a-graph | 0 | 1 | ```\n def longestCycle(self, edges: List[int]) -> int:\n dq = deque();\n res,n = 0,len(edges) \n visitedglobal = [ 0 for i in range(0,n)]\n visited = defaultdict(int)\n for i in range(0,n):\n if(visitedglobal[i] == 0 and edges[i] != -1 ):\n visited.clear()\n visited[i] = 1\n visitedglobal[i] =1\n dq.append(i)\n depth = 1\n while(len(dq)>0):\n size = len(dq)\n while(size):\n size-=1\n v = dq.popleft()\n if(edges[v] == -1 ):\n pass\n elif(visited[edges[v]] == 0 ):\n if( visitedglobal[edges[v]] ==1):\n continue;\n visited[edges[v]]=depth+1\n visitedglobal[edges[v]] =1\n dq.append(edges[v])\n elif(res < depth+1-visited[edges[v]] ):\n res = depth+1-visited[edges[v]]\n depth+=1\n return res if res >0 else -1\n\n``` | 1 | You are given a **directed** graph of `n` nodes numbered from `0` to `n - 1`, where each node has **at most one** outgoing edge.
The graph is represented with a given **0-indexed** array `edges` of size `n`, indicating that there is a directed edge from node `i` to node `edges[i]`. If there is no outgoing edge from node `i`, then `edges[i] == -1`.
Return _the length of the **longest** cycle in the graph_. If no cycle exists, return `-1`.
A cycle is a path that starts and ends at the **same** node.
**Example 1:**
**Input:** edges = \[3,3,4,2,3\]
**Output:** 3
**Explanation:** The longest cycle in the graph is the cycle: 2 -> 4 -> 3 -> 2.
The length of this cycle is 3, so 3 is returned.
**Example 2:**
**Input:** edges = \[2,-1,3,1\]
**Output:** -1
**Explanation:** There are no cycles in this graph.
**Constraints:**
* `n == edges.length`
* `2 <= n <= 105`
* `-1 <= edges[i] < n`
* `edges[i] != i` | null |
[Python] Topological Sort, Daily Challenge Mar., day 26 | longest-cycle-in-a-graph | 0 | 1 | # Intuition\nuse topological sort to remove outermost nodes step by step,\nwe remove outermost node by setting **edges[outermost_node] = -1**\n\nthen, we can iterate `edges` again and union remain connected components\n\n- if every connected component\'s size is 1, it means there is NO cycle\n- else we just return max size of connected component\n\n# Complexity\n- Time complexity:\n$$O(E+V)$$ = $$O(n)$$\n\n- Space complexity:\n$$O(n)$$\n\n# Code\n```\nclass Solution:\n def longestCycle(self, edges: List[int]) -> int:\n n = len(edges)\n indegree = [0] * n\n graph = defaultdict(list)\n\n for u, v in enumerate(edges):\n if v == -1: continue\n graph[u].append(v)\n indegree[v] += 1\n\n queue = deque([node for node, indeg in enumerate(indegree) if indeg == 0])\n while queue:\n for _ in range(len(queue)):\n node = queue.popleft()\n edges[node] = -1\n\n for nei in graph[node]:\n indegree[nei] -= 1\n if indegree[nei] == 0:\n queue.append(nei)\n \n parent = list(range(n))\n rank = [1] * n\n\n def find(x):\n if parent[x] != x:\n parent[x] = find(parent[x])\n return parent[x]\n\n for u, v in enumerate(edges):\n if v == -1: continue\n pu, pv = find(u), find(v)\n if pu == pv: continue\n if rank[pu] <= rank[pv]:\n parent[pv] = pu\n rank[pu] += rank[pv]\n else:\n parent[pu] = pv\n rank[pv] += rank[pu]\n mx = max(rank)\n return mx if mx != 1 else -1\n``` | 1 | You are given a **directed** graph of `n` nodes numbered from `0` to `n - 1`, where each node has **at most one** outgoing edge.
The graph is represented with a given **0-indexed** array `edges` of size `n`, indicating that there is a directed edge from node `i` to node `edges[i]`. If there is no outgoing edge from node `i`, then `edges[i] == -1`.
Return _the length of the **longest** cycle in the graph_. If no cycle exists, return `-1`.
A cycle is a path that starts and ends at the **same** node.
**Example 1:**
**Input:** edges = \[3,3,4,2,3\]
**Output:** 3
**Explanation:** The longest cycle in the graph is the cycle: 2 -> 4 -> 3 -> 2.
The length of this cycle is 3, so 3 is returned.
**Example 2:**
**Input:** edges = \[2,-1,3,1\]
**Output:** -1
**Explanation:** There are no cycles in this graph.
**Constraints:**
* `n == edges.length`
* `2 <= n <= 105`
* `-1 <= edges[i] < n`
* `edges[i] != i` | null |
python3 Solution | longest-cycle-in-a-graph | 0 | 1 | \n```\nclass Solution:\n def longestCycle(self, edges: List[int]) -> int:\n n=len(edges)\n visit=set()\n ranks=[float(\'inf\')]*n\n\n def t(i,rank):\n if i in visit or edges[i]==-1:\n return -1\n\n if ranks[i]<rank:\n return rank-ranks[i]\n\n ranks[i]=rank\n\n val=t(edges[i],rank+1)\n visit.add(i)\n\n return val\n\n return max(t(i,0) for i in range(n)) \n``` | 1 | You are given a **directed** graph of `n` nodes numbered from `0` to `n - 1`, where each node has **at most one** outgoing edge.
The graph is represented with a given **0-indexed** array `edges` of size `n`, indicating that there is a directed edge from node `i` to node `edges[i]`. If there is no outgoing edge from node `i`, then `edges[i] == -1`.
Return _the length of the **longest** cycle in the graph_. If no cycle exists, return `-1`.
A cycle is a path that starts and ends at the **same** node.
**Example 1:**
**Input:** edges = \[3,3,4,2,3\]
**Output:** 3
**Explanation:** The longest cycle in the graph is the cycle: 2 -> 4 -> 3 -> 2.
The length of this cycle is 3, so 3 is returned.
**Example 2:**
**Input:** edges = \[2,-1,3,1\]
**Output:** -1
**Explanation:** There are no cycles in this graph.
**Constraints:**
* `n == edges.length`
* `2 <= n <= 105`
* `-1 <= edges[i] < n`
* `edges[i] != i` | null |
[Python 3] Kahn's Algorithm | longest-cycle-in-a-graph | 0 | 1 | ```\nclass Solution:\n def longestCycle(self, edges: List[int]) -> int:\n n = len(edges)\n indeg = Counter(edges)\n\n stack = [node for node in range(n) if indeg[node] == 0]\n inCycle = {node for node in range(n)}\n\n while stack:\n cur = stack.pop()\n inCycle.remove(cur)\n\n nei = edges[cur]\n if nei == -1:\n continue\n indeg[nei] -= 1\n if indeg[nei] == 0:\n stack.append(nei)\n\n def dfs(s):\n stack = [s]\n seen.add(s)\n res = 0\n\n while stack:\n res += 1\n cur = stack.pop()\n\n nei = edges[cur]\n if nei not in seen:\n seen.add(nei)\n stack.append(nei)\n\n return res\n\n seen = set()\n res = 0\n\n for i in inCycle:\n if i not in seen:\n res = max(res, dfs(i))\n\n return res if res else -1\n``` | 5 | You are given a **directed** graph of `n` nodes numbered from `0` to `n - 1`, where each node has **at most one** outgoing edge.
The graph is represented with a given **0-indexed** array `edges` of size `n`, indicating that there is a directed edge from node `i` to node `edges[i]`. If there is no outgoing edge from node `i`, then `edges[i] == -1`.
Return _the length of the **longest** cycle in the graph_. If no cycle exists, return `-1`.
A cycle is a path that starts and ends at the **same** node.
**Example 1:**
**Input:** edges = \[3,3,4,2,3\]
**Output:** 3
**Explanation:** The longest cycle in the graph is the cycle: 2 -> 4 -> 3 -> 2.
The length of this cycle is 3, so 3 is returned.
**Example 2:**
**Input:** edges = \[2,-1,3,1\]
**Output:** -1
**Explanation:** There are no cycles in this graph.
**Constraints:**
* `n == edges.length`
* `2 <= n <= 105`
* `-1 <= edges[i] < n`
* `edges[i] != i` | null |
Hashmap Python3 | merge-similar-items | 0 | 1 | \n\n# Code\n```\nclass Solution:\n def mergeSimilarItems(self, items1: List[List[int]], items2: List[List[int]]) -> List[List[int]]:\n a, c, b = defaultdict(int), [], items1 + items2\n for j in b:\n if j[0] not in a: a[j[0]] = j[1]\n else: a[j[0]] = a[j[0]] + j[1]\n for i in sorted(a): c.append([i,a[i]])\n return c\n \n``` | 1 | You are given two 2D integer arrays, `items1` and `items2`, representing two sets of items. Each array `items` has the following properties:
* `items[i] = [valuei, weighti]` where `valuei` represents the **value** and `weighti` represents the **weight** of the `ith` item.
* The value of each item in `items` is **unique**.
Return _a 2D integer array_ `ret` _where_ `ret[i] = [valuei, weighti]`_,_ _with_ `weighti` _being the **sum of weights** of all items with value_ `valuei`.
**Note:** `ret` should be returned in **ascending** order by value.
**Example 1:**
**Input:** items1 = \[\[1,1\],\[4,5\],\[3,8\]\], items2 = \[\[3,1\],\[1,5\]\]
**Output:** \[\[1,6\],\[3,9\],\[4,5\]\]
**Explanation:**
The item with value = 1 occurs in items1 with weight = 1 and in items2 with weight = 5, total weight = 1 + 5 = 6.
The item with value = 3 occurs in items1 with weight = 8 and in items2 with weight = 1, total weight = 8 + 1 = 9.
The item with value = 4 occurs in items1 with weight = 5, total weight = 5.
Therefore, we return \[\[1,6\],\[3,9\],\[4,5\]\].
**Example 2:**
**Input:** items1 = \[\[1,1\],\[3,2\],\[2,3\]\], items2 = \[\[2,1\],\[3,2\],\[1,3\]\]
**Output:** \[\[1,4\],\[2,4\],\[3,4\]\]
**Explanation:**
The item with value = 1 occurs in items1 with weight = 1 and in items2 with weight = 3, total weight = 1 + 3 = 4.
The item with value = 2 occurs in items1 with weight = 3 and in items2 with weight = 1, total weight = 3 + 1 = 4.
The item with value = 3 occurs in items1 with weight = 2 and in items2 with weight = 2, total weight = 2 + 2 = 4.
Therefore, we return \[\[1,4\],\[2,4\],\[3,4\]\].
**Example 3:**
**Input:** items1 = \[\[1,3\],\[2,2\]\], items2 = \[\[7,1\],\[2,2\],\[1,4\]\]
**Output:** \[\[1,7\],\[2,4\],\[7,1\]\]
**Explanation:**
The item with value = 1 occurs in items1 with weight = 3 and in items2 with weight = 4, total weight = 3 + 4 = 7.
The item with value = 2 occurs in items1 with weight = 2 and in items2 with weight = 2, total weight = 2 + 2 = 4.
The item with value = 7 occurs in items2 with weight = 1, total weight = 1.
Therefore, we return \[\[1,7\],\[2,4\],\[7,1\]\].
**Constraints:**
* `1 <= items1.length, items2.length <= 1000`
* `items1[i].length == items2[i].length == 2`
* `1 <= valuei, weighti <= 1000`
* Each `valuei` in `items1` is **unique**.
* Each `valuei` in `items2` is **unique**. | null |
Simple solution. Beats 100% in Python Using hash map. | merge-similar-items | 0 | 1 | # Intuition\n<!-- Describe your first thoughts on how to solve this problem. -->\n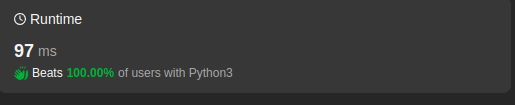\n\n# Approach\n<!-- Describe your approach to solving the problem. -->\n1. Create a hash map from the first array. (value: weight).\n2. Traverse through the second array, modifying values in the hash map.\n3. Traverse through the hash map by key and value, adding a list of [value, weight] pairs to the result list.\n4. Sort the result list.\n# Complexity\n- Time complexity:$$O(n)$$ (avarage) or $$O(N*log(n))$$ (worst)\n<!-- Add your time complexity here, e.g. $$O(n)$$ -->\n\n- Space complexity:$$O(n)$$\n<!-- Add your space complexity here, e.g. $$O(n)$$ -->\n\n# Code\n```python []\nclass Solution:\n def mergeSimilarItems(self, items1: list[list[int]], items2: list[list[int]]) -> list[list[int]]:\n m1 = {value: weight for value, weight in items1}\n ret = []\n \n for item in items2:\n value, weight = item\n \n if not m1.get(value):\n m1[value] = weight\n else:\n m1[value] += weight\n \n for value, weight in m1.items():\n ret.append([value, weight])\n \n ret.sort()\n\n return ret\n```\n```javascript []\n/**\n * @param {number[][]} items1\n * @param {number[][]} items2\n * @return {number[][]}\n */\nvar mergeSimilarItems = function(items1, items2) {\n const m1 = new Map(items1.map(([value, weight]) => [value, weight]));\n const ret = [];\n\n for (const [value, weight] of items2) {\n if (!m1.has(value)) {\n m1.set(value, weight);\n } else {\n m1.set(value, m1.get(value) + weight);\n }\n }\n\n for (const [value, weight] of m1.entries()) {\n ret.push([value, weight]);\n }\n\n ret.sort((a, b) => a[0] - b[0]); // Sort by value\n\n return ret;\n};\n```\n | 0 | You are given two 2D integer arrays, `items1` and `items2`, representing two sets of items. Each array `items` has the following properties:
* `items[i] = [valuei, weighti]` where `valuei` represents the **value** and `weighti` represents the **weight** of the `ith` item.
* The value of each item in `items` is **unique**.
Return _a 2D integer array_ `ret` _where_ `ret[i] = [valuei, weighti]`_,_ _with_ `weighti` _being the **sum of weights** of all items with value_ `valuei`.
**Note:** `ret` should be returned in **ascending** order by value.
**Example 1:**
**Input:** items1 = \[\[1,1\],\[4,5\],\[3,8\]\], items2 = \[\[3,1\],\[1,5\]\]
**Output:** \[\[1,6\],\[3,9\],\[4,5\]\]
**Explanation:**
The item with value = 1 occurs in items1 with weight = 1 and in items2 with weight = 5, total weight = 1 + 5 = 6.
The item with value = 3 occurs in items1 with weight = 8 and in items2 with weight = 1, total weight = 8 + 1 = 9.
The item with value = 4 occurs in items1 with weight = 5, total weight = 5.
Therefore, we return \[\[1,6\],\[3,9\],\[4,5\]\].
**Example 2:**
**Input:** items1 = \[\[1,1\],\[3,2\],\[2,3\]\], items2 = \[\[2,1\],\[3,2\],\[1,3\]\]
**Output:** \[\[1,4\],\[2,4\],\[3,4\]\]
**Explanation:**
The item with value = 1 occurs in items1 with weight = 1 and in items2 with weight = 3, total weight = 1 + 3 = 4.
The item with value = 2 occurs in items1 with weight = 3 and in items2 with weight = 1, total weight = 3 + 1 = 4.
The item with value = 3 occurs in items1 with weight = 2 and in items2 with weight = 2, total weight = 2 + 2 = 4.
Therefore, we return \[\[1,4\],\[2,4\],\[3,4\]\].
**Example 3:**
**Input:** items1 = \[\[1,3\],\[2,2\]\], items2 = \[\[7,1\],\[2,2\],\[1,4\]\]
**Output:** \[\[1,7\],\[2,4\],\[7,1\]\]
**Explanation:**
The item with value = 1 occurs in items1 with weight = 3 and in items2 with weight = 4, total weight = 3 + 4 = 7.
The item with value = 2 occurs in items1 with weight = 2 and in items2 with weight = 2, total weight = 2 + 2 = 4.
The item with value = 7 occurs in items2 with weight = 1, total weight = 1.
Therefore, we return \[\[1,7\],\[2,4\],\[7,1\]\].
**Constraints:**
* `1 <= items1.length, items2.length <= 1000`
* `items1[i].length == items2[i].length == 2`
* `1 <= valuei, weighti <= 1000`
* Each `valuei` in `items1` is **unique**.
* Each `valuei` in `items2` is **unique**. | null |
[Java/Python 3] TreeMap, w/ brief explanation and analysis. | merge-similar-items | 1 | 1 | Use TreeMap to accumulate the weights of items with same value.\n\n```java\n public List<List<Integer>> mergeSimilarItems(int[][] items1, int[][] items2) {\n TreeMap<Integer, Integer> cnt = new TreeMap<>();\n for (int[] it : items1) {\n cnt.merge(it[0], it[1], Integer::sum);\n }\n for (int[] it : items2) {\n cnt.merge(it[0], it[1], Integer::sum);\n }\n List<List<Integer>> ans = new ArrayList<>();\n for (var e : cnt.entrySet()) {\n ans.add(Arrays.asList(e.getKey(), e.getValue()));\n }\n return ans;\n }\n```\n\nJava 8 Stream code - inspired by **@climberig**\n\n```java\n public List<List<Integer>> mergeSimilarItems(int[][] items1, int[][] items2) {\n TreeMap<Integer, Integer> cnt = new TreeMap<>();\n Stream.of(items1).forEach(item -> cnt.put(item[0], item[1]));\n Stream.of(items2).forEach(item -> cnt.merge(item[0], item[1], Integer::sum));\n return cnt.entrySet().stream().map(e -> Arrays.asList(e.getKey(), e.getValue())).collect(Collectors.toList());\n }\n```\n\n----\n\nUse `Counter` to accumulate the weights of items with same value, then sort the `(key value)` mappings.\n```python\n def mergeSimilarItems(self, items1: List[List[int]], items2: List[List[int]]) -> List[List[int]]:\n cnt = Counter()\n for v, w in items1 + items2:\n cnt[v] += w\n return sorted(cnt.items())\n```\n**@stefan4trivia**\'s very clean code:\n```python\n def mergeSimilarItems(self, items1: List[List[int]], items2: List[List[int]]) -> List[List[int]]:\n cnt = Counter(dict(items1))\n cnt += Counter(dict(items2))\n return sorted(cnt.items())\n```\n1 liner - inspired by **@stefan4trivia**:\n\n```python\n def mergeSimilarItems(self, items1: List[List[int]], items2: List[List[int]]) -> List[List[int]]:\n return sorted((Counter(dict(items1)) + Counter(dict(items2))).items())\n```\n\n**Analysis:**\n\nTime: `O(nlogn)`, space: `O(n)`, where `n = items1.length + items2.length`. | 32 | You are given two 2D integer arrays, `items1` and `items2`, representing two sets of items. Each array `items` has the following properties:
* `items[i] = [valuei, weighti]` where `valuei` represents the **value** and `weighti` represents the **weight** of the `ith` item.
* The value of each item in `items` is **unique**.
Return _a 2D integer array_ `ret` _where_ `ret[i] = [valuei, weighti]`_,_ _with_ `weighti` _being the **sum of weights** of all items with value_ `valuei`.
**Note:** `ret` should be returned in **ascending** order by value.
**Example 1:**
**Input:** items1 = \[\[1,1\],\[4,5\],\[3,8\]\], items2 = \[\[3,1\],\[1,5\]\]
**Output:** \[\[1,6\],\[3,9\],\[4,5\]\]
**Explanation:**
The item with value = 1 occurs in items1 with weight = 1 and in items2 with weight = 5, total weight = 1 + 5 = 6.
The item with value = 3 occurs in items1 with weight = 8 and in items2 with weight = 1, total weight = 8 + 1 = 9.
The item with value = 4 occurs in items1 with weight = 5, total weight = 5.
Therefore, we return \[\[1,6\],\[3,9\],\[4,5\]\].
**Example 2:**
**Input:** items1 = \[\[1,1\],\[3,2\],\[2,3\]\], items2 = \[\[2,1\],\[3,2\],\[1,3\]\]
**Output:** \[\[1,4\],\[2,4\],\[3,4\]\]
**Explanation:**
The item with value = 1 occurs in items1 with weight = 1 and in items2 with weight = 3, total weight = 1 + 3 = 4.
The item with value = 2 occurs in items1 with weight = 3 and in items2 with weight = 1, total weight = 3 + 1 = 4.
The item with value = 3 occurs in items1 with weight = 2 and in items2 with weight = 2, total weight = 2 + 2 = 4.
Therefore, we return \[\[1,4\],\[2,4\],\[3,4\]\].
**Example 3:**
**Input:** items1 = \[\[1,3\],\[2,2\]\], items2 = \[\[7,1\],\[2,2\],\[1,4\]\]
**Output:** \[\[1,7\],\[2,4\],\[7,1\]\]
**Explanation:**
The item with value = 1 occurs in items1 with weight = 3 and in items2 with weight = 4, total weight = 3 + 4 = 7.
The item with value = 2 occurs in items1 with weight = 2 and in items2 with weight = 2, total weight = 2 + 2 = 4.
The item with value = 7 occurs in items2 with weight = 1, total weight = 1.
Therefore, we return \[\[1,7\],\[2,4\],\[7,1\]\].
**Constraints:**
* `1 <= items1.length, items2.length <= 1000`
* `items1[i].length == items2[i].length == 2`
* `1 <= valuei, weighti <= 1000`
* Each `valuei` in `items1` is **unique**.
* Each `valuei` in `items2` is **unique**. | null |
Python Easy Solution | merge-similar-items | 0 | 1 | # Code\n```\nclass Solution:\n def mergeSimilarItems(self, items1: List[List[int]], items2: List[List[int]]) -> List[List[int]]:\n items1.sort()\n items2.sort()\n res=[]\n i,j=0,0\n while(i<len(items1) and j<len(items2)):\n if items1[i][0]==items2[j][0]:\n res.append([items1[i][0],items1[i][1]+items2[j][1]])\n i+=1\n j+=1\n elif items1[i][0]<items2[j][0]:\n res.append(items1[i])\n i+=1\n elif items1[i][0]>items2[j][0]:\n res.append(items2[j])\n j+=1\n \n if i==len(items1):\n while(j<len(items2)):\n res.append(items2[j])\n j+=1\n \n if j==len(items2):\n while(i<len(items1)):\n res.append(items1[i])\n i+=1\n \n return res\n \n``` | 1 | You are given two 2D integer arrays, `items1` and `items2`, representing two sets of items. Each array `items` has the following properties:
* `items[i] = [valuei, weighti]` where `valuei` represents the **value** and `weighti` represents the **weight** of the `ith` item.
* The value of each item in `items` is **unique**.
Return _a 2D integer array_ `ret` _where_ `ret[i] = [valuei, weighti]`_,_ _with_ `weighti` _being the **sum of weights** of all items with value_ `valuei`.
**Note:** `ret` should be returned in **ascending** order by value.
**Example 1:**
**Input:** items1 = \[\[1,1\],\[4,5\],\[3,8\]\], items2 = \[\[3,1\],\[1,5\]\]
**Output:** \[\[1,6\],\[3,9\],\[4,5\]\]
**Explanation:**
The item with value = 1 occurs in items1 with weight = 1 and in items2 with weight = 5, total weight = 1 + 5 = 6.
The item with value = 3 occurs in items1 with weight = 8 and in items2 with weight = 1, total weight = 8 + 1 = 9.
The item with value = 4 occurs in items1 with weight = 5, total weight = 5.
Therefore, we return \[\[1,6\],\[3,9\],\[4,5\]\].
**Example 2:**
**Input:** items1 = \[\[1,1\],\[3,2\],\[2,3\]\], items2 = \[\[2,1\],\[3,2\],\[1,3\]\]
**Output:** \[\[1,4\],\[2,4\],\[3,4\]\]
**Explanation:**
The item with value = 1 occurs in items1 with weight = 1 and in items2 with weight = 3, total weight = 1 + 3 = 4.
The item with value = 2 occurs in items1 with weight = 3 and in items2 with weight = 1, total weight = 3 + 1 = 4.
The item with value = 3 occurs in items1 with weight = 2 and in items2 with weight = 2, total weight = 2 + 2 = 4.
Therefore, we return \[\[1,4\],\[2,4\],\[3,4\]\].
**Example 3:**
**Input:** items1 = \[\[1,3\],\[2,2\]\], items2 = \[\[7,1\],\[2,2\],\[1,4\]\]
**Output:** \[\[1,7\],\[2,4\],\[7,1\]\]
**Explanation:**
The item with value = 1 occurs in items1 with weight = 3 and in items2 with weight = 4, total weight = 3 + 4 = 7.
The item with value = 2 occurs in items1 with weight = 2 and in items2 with weight = 2, total weight = 2 + 2 = 4.
The item with value = 7 occurs in items2 with weight = 1, total weight = 1.
Therefore, we return \[\[1,7\],\[2,4\],\[7,1\]\].
**Constraints:**
* `1 <= items1.length, items2.length <= 1000`
* `items1[i].length == items2[i].length == 2`
* `1 <= valuei, weighti <= 1000`
* Each `valuei` in `items1` is **unique**.
* Each `valuei` in `items2` is **unique**. | null |
[ Python ] ✅✅ Simple Python Solution Using HashMap 🥳✌👍 | merge-similar-items | 0 | 1 | # If You like the Solution, Don\'t Forget To UpVote Me, Please UpVote! \uD83D\uDD3C\uD83D\uDE4F\n# Runtime: 138 ms, faster than 15.38% of Python3 online submissions for Merge Similar Items.\n# Memory Usage: 14.8 MB, less than 53.85% of Python3 online submissions for Merge Similar Items.\n\n\tclass Solution:\n\t\tdef mergeSimilarItems(self, items1: List[List[int]], items2: List[List[int]]) -> List[List[int]]:\n\n\t\t\tmerge_item = items1 + items2\n\n\t\t\td = defaultdict(int)\n\n\t\t\tfor i in merge_item:\n\t\t\t\tvalue,weight = i\n\t\t\t\td[value] = d[value] + weight\n\n\t\t\tresult = []\n\n\t\t\tfor j in sorted(d):\n\t\t\t\tresult.append([j,d[j]])\n\n\t\t\treturn result\n\n# Thank You \uD83E\uDD73\u270C\uD83D\uDC4D | 11 | You are given two 2D integer arrays, `items1` and `items2`, representing two sets of items. Each array `items` has the following properties:
* `items[i] = [valuei, weighti]` where `valuei` represents the **value** and `weighti` represents the **weight** of the `ith` item.
* The value of each item in `items` is **unique**.
Return _a 2D integer array_ `ret` _where_ `ret[i] = [valuei, weighti]`_,_ _with_ `weighti` _being the **sum of weights** of all items with value_ `valuei`.
**Note:** `ret` should be returned in **ascending** order by value.
**Example 1:**
**Input:** items1 = \[\[1,1\],\[4,5\],\[3,8\]\], items2 = \[\[3,1\],\[1,5\]\]
**Output:** \[\[1,6\],\[3,9\],\[4,5\]\]
**Explanation:**
The item with value = 1 occurs in items1 with weight = 1 and in items2 with weight = 5, total weight = 1 + 5 = 6.
The item with value = 3 occurs in items1 with weight = 8 and in items2 with weight = 1, total weight = 8 + 1 = 9.
The item with value = 4 occurs in items1 with weight = 5, total weight = 5.
Therefore, we return \[\[1,6\],\[3,9\],\[4,5\]\].
**Example 2:**
**Input:** items1 = \[\[1,1\],\[3,2\],\[2,3\]\], items2 = \[\[2,1\],\[3,2\],\[1,3\]\]
**Output:** \[\[1,4\],\[2,4\],\[3,4\]\]
**Explanation:**
The item with value = 1 occurs in items1 with weight = 1 and in items2 with weight = 3, total weight = 1 + 3 = 4.
The item with value = 2 occurs in items1 with weight = 3 and in items2 with weight = 1, total weight = 3 + 1 = 4.
The item with value = 3 occurs in items1 with weight = 2 and in items2 with weight = 2, total weight = 2 + 2 = 4.
Therefore, we return \[\[1,4\],\[2,4\],\[3,4\]\].
**Example 3:**
**Input:** items1 = \[\[1,3\],\[2,2\]\], items2 = \[\[7,1\],\[2,2\],\[1,4\]\]
**Output:** \[\[1,7\],\[2,4\],\[7,1\]\]
**Explanation:**
The item with value = 1 occurs in items1 with weight = 3 and in items2 with weight = 4, total weight = 3 + 4 = 7.
The item with value = 2 occurs in items1 with weight = 2 and in items2 with weight = 2, total weight = 2 + 2 = 4.
The item with value = 7 occurs in items2 with weight = 1, total weight = 1.
Therefore, we return \[\[1,7\],\[2,4\],\[7,1\]\].
**Constraints:**
* `1 <= items1.length, items2.length <= 1000`
* `items1[i].length == items2[i].length == 2`
* `1 <= valuei, weighti <= 1000`
* Each `valuei` in `items1` is **unique**.
* Each `valuei` in `items2` is **unique**. | null |
✅Python || Easy Approaches | merge-similar-items | 0 | 1 | ```\nclass Solution:\n def mergeSimilarItems(self, items1: List[List[int]], items2: List[List[int]]) -> List[List[int]]:\n \n \n hashset = {}\n\n for i in range(len(items1)):\n if items1[i][0] in hashset:\n hashset[items1[i][0]] += items1[i][1]\n else:\n hashset[items1[i][0]] = items1[i][1]\n\n for i in range(len(items2)):\n if items2[i][0] in hashset:\n hashset[items2[i][0]] += items2[i][1]\n else:\n hashset[items2[i][0]] = items2[i][1]\n \n ans = []\n\n for i in sorted(hashset):\n ans.append([i, hashset[i]])\n \n return ans\n``` | 5 | You are given two 2D integer arrays, `items1` and `items2`, representing two sets of items. Each array `items` has the following properties:
* `items[i] = [valuei, weighti]` where `valuei` represents the **value** and `weighti` represents the **weight** of the `ith` item.
* The value of each item in `items` is **unique**.
Return _a 2D integer array_ `ret` _where_ `ret[i] = [valuei, weighti]`_,_ _with_ `weighti` _being the **sum of weights** of all items with value_ `valuei`.
**Note:** `ret` should be returned in **ascending** order by value.
**Example 1:**
**Input:** items1 = \[\[1,1\],\[4,5\],\[3,8\]\], items2 = \[\[3,1\],\[1,5\]\]
**Output:** \[\[1,6\],\[3,9\],\[4,5\]\]
**Explanation:**
The item with value = 1 occurs in items1 with weight = 1 and in items2 with weight = 5, total weight = 1 + 5 = 6.
The item with value = 3 occurs in items1 with weight = 8 and in items2 with weight = 1, total weight = 8 + 1 = 9.
The item with value = 4 occurs in items1 with weight = 5, total weight = 5.
Therefore, we return \[\[1,6\],\[3,9\],\[4,5\]\].
**Example 2:**
**Input:** items1 = \[\[1,1\],\[3,2\],\[2,3\]\], items2 = \[\[2,1\],\[3,2\],\[1,3\]\]
**Output:** \[\[1,4\],\[2,4\],\[3,4\]\]
**Explanation:**
The item with value = 1 occurs in items1 with weight = 1 and in items2 with weight = 3, total weight = 1 + 3 = 4.
The item with value = 2 occurs in items1 with weight = 3 and in items2 with weight = 1, total weight = 3 + 1 = 4.
The item with value = 3 occurs in items1 with weight = 2 and in items2 with weight = 2, total weight = 2 + 2 = 4.
Therefore, we return \[\[1,4\],\[2,4\],\[3,4\]\].
**Example 3:**
**Input:** items1 = \[\[1,3\],\[2,2\]\], items2 = \[\[7,1\],\[2,2\],\[1,4\]\]
**Output:** \[\[1,7\],\[2,4\],\[7,1\]\]
**Explanation:**
The item with value = 1 occurs in items1 with weight = 3 and in items2 with weight = 4, total weight = 3 + 4 = 7.
The item with value = 2 occurs in items1 with weight = 2 and in items2 with weight = 2, total weight = 2 + 2 = 4.
The item with value = 7 occurs in items2 with weight = 1, total weight = 1.
Therefore, we return \[\[1,7\],\[2,4\],\[7,1\]\].
**Constraints:**
* `1 <= items1.length, items2.length <= 1000`
* `items1[i].length == items2[i].length == 2`
* `1 <= valuei, weighti <= 1000`
* Each `valuei` in `items1` is **unique**.
* Each `valuei` in `items2` is **unique**. | null |
One Liner | merge-similar-items | 0 | 1 | **Python 3**\n```python\nclass Solution:\n def mergeSimilarItems(self, i1: List[List[int]], i2: List[List[int]]) -> List[List[int]]:\n return sorted((Counter({i[0] : i[1] for i in i1}) + Counter({i[0] : i[1] for i in i2})).items())\n``` | 10 | You are given two 2D integer arrays, `items1` and `items2`, representing two sets of items. Each array `items` has the following properties:
* `items[i] = [valuei, weighti]` where `valuei` represents the **value** and `weighti` represents the **weight** of the `ith` item.
* The value of each item in `items` is **unique**.
Return _a 2D integer array_ `ret` _where_ `ret[i] = [valuei, weighti]`_,_ _with_ `weighti` _being the **sum of weights** of all items with value_ `valuei`.
**Note:** `ret` should be returned in **ascending** order by value.
**Example 1:**
**Input:** items1 = \[\[1,1\],\[4,5\],\[3,8\]\], items2 = \[\[3,1\],\[1,5\]\]
**Output:** \[\[1,6\],\[3,9\],\[4,5\]\]
**Explanation:**
The item with value = 1 occurs in items1 with weight = 1 and in items2 with weight = 5, total weight = 1 + 5 = 6.
The item with value = 3 occurs in items1 with weight = 8 and in items2 with weight = 1, total weight = 8 + 1 = 9.
The item with value = 4 occurs in items1 with weight = 5, total weight = 5.
Therefore, we return \[\[1,6\],\[3,9\],\[4,5\]\].
**Example 2:**
**Input:** items1 = \[\[1,1\],\[3,2\],\[2,3\]\], items2 = \[\[2,1\],\[3,2\],\[1,3\]\]
**Output:** \[\[1,4\],\[2,4\],\[3,4\]\]
**Explanation:**
The item with value = 1 occurs in items1 with weight = 1 and in items2 with weight = 3, total weight = 1 + 3 = 4.
The item with value = 2 occurs in items1 with weight = 3 and in items2 with weight = 1, total weight = 3 + 1 = 4.
The item with value = 3 occurs in items1 with weight = 2 and in items2 with weight = 2, total weight = 2 + 2 = 4.
Therefore, we return \[\[1,4\],\[2,4\],\[3,4\]\].
**Example 3:**
**Input:** items1 = \[\[1,3\],\[2,2\]\], items2 = \[\[7,1\],\[2,2\],\[1,4\]\]
**Output:** \[\[1,7\],\[2,4\],\[7,1\]\]
**Explanation:**
The item with value = 1 occurs in items1 with weight = 3 and in items2 with weight = 4, total weight = 3 + 4 = 7.
The item with value = 2 occurs in items1 with weight = 2 and in items2 with weight = 2, total weight = 2 + 2 = 4.
The item with value = 7 occurs in items2 with weight = 1, total weight = 1.
Therefore, we return \[\[1,7\],\[2,4\],\[7,1\]\].
**Constraints:**
* `1 <= items1.length, items2.length <= 1000`
* `items1[i].length == items2[i].length == 2`
* `1 <= valuei, weighti <= 1000`
* Each `valuei` in `items1` is **unique**.
* Each `valuei` in `items2` is **unique**. | null |
Python one-line solution but extremely slow | merge-similar-items | 0 | 1 | # Intuition\n<!-- Describe your first thoughts on how to solve this problem. -->\nUsed a bunch of list comprensions\n\n# Code\n```\nclass Solution:\n def mergeSimilarItems(self, items1: List[List[int]], items2: List[List[int]]) -> List[List[int]]:\n return sorted([[v1, w1 + w2] for v1, w1 in items1 for v2, w2 in items2 if v1 == v2] + [item for item in items1 if item[0] not in (v for v, w in items2)] + [item for item in items2 if item[0] not in (v for v, w in items1)])\n \n``` | 1 | You are given two 2D integer arrays, `items1` and `items2`, representing two sets of items. Each array `items` has the following properties:
* `items[i] = [valuei, weighti]` where `valuei` represents the **value** and `weighti` represents the **weight** of the `ith` item.
* The value of each item in `items` is **unique**.
Return _a 2D integer array_ `ret` _where_ `ret[i] = [valuei, weighti]`_,_ _with_ `weighti` _being the **sum of weights** of all items with value_ `valuei`.
**Note:** `ret` should be returned in **ascending** order by value.
**Example 1:**
**Input:** items1 = \[\[1,1\],\[4,5\],\[3,8\]\], items2 = \[\[3,1\],\[1,5\]\]
**Output:** \[\[1,6\],\[3,9\],\[4,5\]\]
**Explanation:**
The item with value = 1 occurs in items1 with weight = 1 and in items2 with weight = 5, total weight = 1 + 5 = 6.
The item with value = 3 occurs in items1 with weight = 8 and in items2 with weight = 1, total weight = 8 + 1 = 9.
The item with value = 4 occurs in items1 with weight = 5, total weight = 5.
Therefore, we return \[\[1,6\],\[3,9\],\[4,5\]\].
**Example 2:**
**Input:** items1 = \[\[1,1\],\[3,2\],\[2,3\]\], items2 = \[\[2,1\],\[3,2\],\[1,3\]\]
**Output:** \[\[1,4\],\[2,4\],\[3,4\]\]
**Explanation:**
The item with value = 1 occurs in items1 with weight = 1 and in items2 with weight = 3, total weight = 1 + 3 = 4.
The item with value = 2 occurs in items1 with weight = 3 and in items2 with weight = 1, total weight = 3 + 1 = 4.
The item with value = 3 occurs in items1 with weight = 2 and in items2 with weight = 2, total weight = 2 + 2 = 4.
Therefore, we return \[\[1,4\],\[2,4\],\[3,4\]\].
**Example 3:**
**Input:** items1 = \[\[1,3\],\[2,2\]\], items2 = \[\[7,1\],\[2,2\],\[1,4\]\]
**Output:** \[\[1,7\],\[2,4\],\[7,1\]\]
**Explanation:**
The item with value = 1 occurs in items1 with weight = 3 and in items2 with weight = 4, total weight = 3 + 4 = 7.
The item with value = 2 occurs in items1 with weight = 2 and in items2 with weight = 2, total weight = 2 + 2 = 4.
The item with value = 7 occurs in items2 with weight = 1, total weight = 1.
Therefore, we return \[\[1,7\],\[2,4\],\[7,1\]\].
**Constraints:**
* `1 <= items1.length, items2.length <= 1000`
* `items1[i].length == items2[i].length == 2`
* `1 <= valuei, weighti <= 1000`
* Each `valuei` in `items1` is **unique**.
* Each `valuei` in `items2` is **unique**. | null |
BASIC APPROACH || BRUTE FORCE || PYTHON3 | merge-similar-items | 0 | 1 | # BASIC APPROACH || BRUTE FORCE || PYTHON3\n\n# Code\n```\nclass Solution:\n def mergeSimilarItems(self, items1: List[List[int]], items2: List[List[int]]) -> List[List[int]]:\n ans=[]\n dicc={}\n\n for i in range(len(items1)):\n if items1[i][0] not in dicc:\n dicc[items1[i][0]]=items1[i][1]\n else:\n dicc[items1[i][0]]+=items1[i][1]\n \n for j in range(len(items2)):\n if items2[j][0] not in dicc:\n dicc[items2[j][0]]=items2[j][1]\n else:\n dicc[items2[j][0]]+=items2[j][1]\n \n for k,v in dicc.items():\n ans.append([k,v])\n ans.sort()\n \n return ans\n \n``` | 1 | You are given two 2D integer arrays, `items1` and `items2`, representing two sets of items. Each array `items` has the following properties:
* `items[i] = [valuei, weighti]` where `valuei` represents the **value** and `weighti` represents the **weight** of the `ith` item.
* The value of each item in `items` is **unique**.
Return _a 2D integer array_ `ret` _where_ `ret[i] = [valuei, weighti]`_,_ _with_ `weighti` _being the **sum of weights** of all items with value_ `valuei`.
**Note:** `ret` should be returned in **ascending** order by value.
**Example 1:**
**Input:** items1 = \[\[1,1\],\[4,5\],\[3,8\]\], items2 = \[\[3,1\],\[1,5\]\]
**Output:** \[\[1,6\],\[3,9\],\[4,5\]\]
**Explanation:**
The item with value = 1 occurs in items1 with weight = 1 and in items2 with weight = 5, total weight = 1 + 5 = 6.
The item with value = 3 occurs in items1 with weight = 8 and in items2 with weight = 1, total weight = 8 + 1 = 9.
The item with value = 4 occurs in items1 with weight = 5, total weight = 5.
Therefore, we return \[\[1,6\],\[3,9\],\[4,5\]\].
**Example 2:**
**Input:** items1 = \[\[1,1\],\[3,2\],\[2,3\]\], items2 = \[\[2,1\],\[3,2\],\[1,3\]\]
**Output:** \[\[1,4\],\[2,4\],\[3,4\]\]
**Explanation:**
The item with value = 1 occurs in items1 with weight = 1 and in items2 with weight = 3, total weight = 1 + 3 = 4.
The item with value = 2 occurs in items1 with weight = 3 and in items2 with weight = 1, total weight = 3 + 1 = 4.
The item with value = 3 occurs in items1 with weight = 2 and in items2 with weight = 2, total weight = 2 + 2 = 4.
Therefore, we return \[\[1,4\],\[2,4\],\[3,4\]\].
**Example 3:**
**Input:** items1 = \[\[1,3\],\[2,2\]\], items2 = \[\[7,1\],\[2,2\],\[1,4\]\]
**Output:** \[\[1,7\],\[2,4\],\[7,1\]\]
**Explanation:**
The item with value = 1 occurs in items1 with weight = 3 and in items2 with weight = 4, total weight = 3 + 4 = 7.
The item with value = 2 occurs in items1 with weight = 2 and in items2 with weight = 2, total weight = 2 + 2 = 4.
The item with value = 7 occurs in items2 with weight = 1, total weight = 1.
Therefore, we return \[\[1,7\],\[2,4\],\[7,1\]\].
**Constraints:**
* `1 <= items1.length, items2.length <= 1000`
* `items1[i].length == items2[i].length == 2`
* `1 <= valuei, weighti <= 1000`
* Each `valuei` in `items1` is **unique**.
* Each `valuei` in `items2` is **unique**. | null |
🔥 Python || Detailed Explanation ✅ || Faster Than 100% ✅|| Less than 100% ✅ || Simple || MATH | count-number-of-bad-pairs | 0 | 1 | **Appreciate if you could upvote this solution**\n\n\nMethod: `math`\n\nFirst, we know that the number of pair combinations for nums is `nCr`\nAlso, the condition can transform from `i-j != num[j]-num[i]` to `num[i]-i != num[j]-j` via basic calculation.\nThus, the result should be `nCr - num_of_pairs(num[i]-i == num[j]-j)`\nThen, we can use a `dict` to store the duplication counts.\n\nFor example, `nums = [4, 1, 3, 3, 5, 5]`\nThen, transforming `nums` to `[4, 0, 1, 0, 1, 0]`\nand the duplication count dict equals to\n```\n{\n\t0: 3 // the values stored in indexes 1, 3 and 5 are 0 \n\t1: 2 // the values stored in indexes 2 and 4 are 1\n\t4: 1 // the values stored in index 0 is 4\n}\n```\nThus, the pair combinations for `num[i]-i == num[j]-j` is \n```\n3C2 (for value 0) + 2C1 (for value 1) + 0 (value 4 is not duplicated)\n```\nTherefore, the total number of bad pairs is `6C2 - 3C2 - 2C1 = 11`\n<br/>\nCode:\n```\nclass Solution:\n def countBadPairs(self, nums: List[int]) -> int:\n nums_len = len(nums)\n count_dict = dict()\n for i in range(nums_len):\n nums[i] -= i\n if nums[i] not in count_dict:\n count_dict[nums[i]] = 0\n count_dict[nums[i]] += 1\n \n count = 0\n for key in count_dict:\n count += math.comb(count_dict[key], 2)\n return math.comb(nums_len, 2) - count\n```\n\n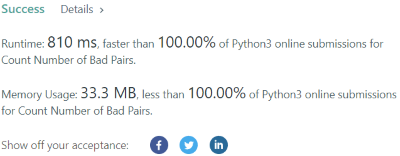\n<br/>\n**Time complexity**:\n - O(n) (ignoring the calculation of combination )\n\n**Space complexity**:\n - O(1) (ignoring the calculation of combination )\n<br/>\n | 39 | You are given a **0-indexed** integer array `nums`. A pair of indices `(i, j)` is a **bad pair** if `i < j` and `j - i != nums[j] - nums[i]`.
Return _the total number of **bad pairs** in_ `nums`.
**Example 1:**
**Input:** nums = \[4,1,3,3\]
**Output:** 5
**Explanation:** The pair (0, 1) is a bad pair since 1 - 0 != 1 - 4.
The pair (0, 2) is a bad pair since 2 - 0 != 3 - 4, 2 != -1.
The pair (0, 3) is a bad pair since 3 - 0 != 3 - 4, 3 != -1.
The pair (1, 2) is a bad pair since 2 - 1 != 3 - 1, 1 != 2.
The pair (2, 3) is a bad pair since 3 - 2 != 3 - 3, 1 != 0.
There are a total of 5 bad pairs, so we return 5.
**Example 2:**
**Input:** nums = \[1,2,3,4,5\]
**Output:** 0
**Explanation:** There are no bad pairs.
**Constraints:**
* `1 <= nums.length <= 105`
* `1 <= nums[i] <= 109` | null |
[Java/Python 3] Count good pairs, w/ brief explanation and analysis. | count-number-of-bad-pairs | 1 | 1 | \nGood pairs have same `nums[i] - i` value, so we can use HashMap to count the number of elements with same hash, `nums[i] - i`, then count the good pairs; Then use total pairs minus good pairs.\n\n```java\n public long countBadPairs(int[] nums) {\n long n = nums.length;\n Map<Integer, Integer> good = new HashMap<>();\n for (int i = 0; i < n; ++i) {\n good.merge(nums[i] - i, 1, Integer::sum);\n }\n long bad = n * (n - 1) / 2;\n for (int v : good.values()) {\n bad -= v * (v - 1L) / 2;\n }\n return bad;\n }\n```\n\n```python\n def countBadPairs(self, nums: List[int]) -> int:\n good, n = Counter(), len(nums)\n for i, num in enumerate(nums):\n good[num - i] += 1\n return (n - 1) * n // 2 - sum((v - 1) * v // 2 for v in good.values())\n```\n\n**Analysis:**\n\nTime: `O(n)`, space: `O(n)`, where `n = nums.length`.\n | 5 | You are given a **0-indexed** integer array `nums`. A pair of indices `(i, j)` is a **bad pair** if `i < j` and `j - i != nums[j] - nums[i]`.
Return _the total number of **bad pairs** in_ `nums`.
**Example 1:**
**Input:** nums = \[4,1,3,3\]
**Output:** 5
**Explanation:** The pair (0, 1) is a bad pair since 1 - 0 != 1 - 4.
The pair (0, 2) is a bad pair since 2 - 0 != 3 - 4, 2 != -1.
The pair (0, 3) is a bad pair since 3 - 0 != 3 - 4, 3 != -1.
The pair (1, 2) is a bad pair since 2 - 1 != 3 - 1, 1 != 2.
The pair (2, 3) is a bad pair since 3 - 2 != 3 - 3, 1 != 0.
There are a total of 5 bad pairs, so we return 5.
**Example 2:**
**Input:** nums = \[1,2,3,4,5\]
**Output:** 0
**Explanation:** There are no bad pairs.
**Constraints:**
* `1 <= nums.length <= 105`
* `1 <= nums[i] <= 109` | null |
✅Python || O(n) || Count || Easy Approaches | count-number-of-bad-pairs | 0 | 1 | ```\nclass Solution:\n def countBadPairs(self, nums: List[int]) -> int:\n\n n = len(nums)\n res = []\n for i in range(n):\n res.append(nums[i] - i)\n\n a = Counter(res)\n ans = n * (n - 1) // 2\n for x in a:\n if a[x] > 1:\n ans -= a[x] * (a[x] - 1) // 2\n \n return ans\n``` | 2 | You are given a **0-indexed** integer array `nums`. A pair of indices `(i, j)` is a **bad pair** if `i < j` and `j - i != nums[j] - nums[i]`.
Return _the total number of **bad pairs** in_ `nums`.
**Example 1:**
**Input:** nums = \[4,1,3,3\]
**Output:** 5
**Explanation:** The pair (0, 1) is a bad pair since 1 - 0 != 1 - 4.
The pair (0, 2) is a bad pair since 2 - 0 != 3 - 4, 2 != -1.
The pair (0, 3) is a bad pair since 3 - 0 != 3 - 4, 3 != -1.
The pair (1, 2) is a bad pair since 2 - 1 != 3 - 1, 1 != 2.
The pair (2, 3) is a bad pair since 3 - 2 != 3 - 3, 1 != 0.
There are a total of 5 bad pairs, so we return 5.
**Example 2:**
**Input:** nums = \[1,2,3,4,5\]
**Output:** 0
**Explanation:** There are no bad pairs.
**Constraints:**
* `1 <= nums.length <= 105`
* `1 <= nums[i] <= 109` | null |
Prettie prettie... prettie good solution in Python. | task-scheduler-ii | 0 | 1 | # Intuition\nKeep a dictionary of the format `{task: earliest day it can be completed}`. \nIterate through `tasks` and increment the current `day` by 1. \n\n# Approach\nIterate thru the list `tasks`. You can consider one task per day. \nIf `day` is behind the earliest day you can complete the `task`, then fast forward `day` variable to the value given in the dictionary. Remember to update the dictionary to reset the clock. \n\n\n# Complexity\n- Time complexity: $$O(n)$$ -- two traversals across `tasks`. \n\n- Space complexity: $$O(n)$$ -- create dictionary `start_day`.\n\n```\nclass Solution:\n def taskSchedulerII(self, tasks: List[int], space: int) -> int:\n start_day = {task:0 for task in tasks}\n day = 0\n for task in tasks:\n day += 1\n\n # if the current day is too early to complete the task, \n # fast forward the day to the earliest day you can.\n if day < start_day[task]:\n day = start_day[task] \n \n # update the earliest day you can complete the task.\n start_day[task] = day + space + 1\n\n return day\n\n\n\n``` | 1 | You are given a **0-indexed** array of positive integers `tasks`, representing tasks that need to be completed **in order**, where `tasks[i]` represents the **type** of the `ith` task.
You are also given a positive integer `space`, which represents the **minimum** number of days that must pass **after** the completion of a task before another task of the **same** type can be performed.
Each day, until all tasks have been completed, you must either:
* Complete the next task from `tasks`, or
* Take a break.
Return _the **minimum** number of days needed to complete all tasks_.
**Example 1:**
**Input:** tasks = \[1,2,1,2,3,1\], space = 3
**Output:** 9
**Explanation:**
One way to complete all tasks in 9 days is as follows:
Day 1: Complete the 0th task.
Day 2: Complete the 1st task.
Day 3: Take a break.
Day 4: Take a break.
Day 5: Complete the 2nd task.
Day 6: Complete the 3rd task.
Day 7: Take a break.
Day 8: Complete the 4th task.
Day 9: Complete the 5th task.
It can be shown that the tasks cannot be completed in less than 9 days.
**Example 2:**
**Input:** tasks = \[5,8,8,5\], space = 2
**Output:** 6
**Explanation:**
One way to complete all tasks in 6 days is as follows:
Day 1: Complete the 0th task.
Day 2: Complete the 1st task.
Day 3: Take a break.
Day 4: Take a break.
Day 5: Complete the 2nd task.
Day 6: Complete the 3rd task.
It can be shown that the tasks cannot be completed in less than 6 days.
**Constraints:**
* `1 <= tasks.length <= 105`
* `1 <= tasks[i] <= 109`
* `1 <= space <= tasks.length` | null |
Python || Easily Understood ✅ || Faster Than 98% || Simple || O(n) | task-scheduler-ii | 0 | 1 | ```\nimport math\nclass Solution:\n def taskSchedulerII(self, tasks: List[int], space: int) -> int:\n count_dict = {}\n total_days = 0\n for task in tasks:\n if task not in count_dict:\n count_dict[task] = -math.inf\n total_days = max(total_days + 1, count_dict[task] + space + 1)\n count_dict[task] = total_days\n return total_days\n```\n**Time complexity**: O(n)\n**Space complexity**: O(n) | 19 | You are given a **0-indexed** array of positive integers `tasks`, representing tasks that need to be completed **in order**, where `tasks[i]` represents the **type** of the `ith` task.
You are also given a positive integer `space`, which represents the **minimum** number of days that must pass **after** the completion of a task before another task of the **same** type can be performed.
Each day, until all tasks have been completed, you must either:
* Complete the next task from `tasks`, or
* Take a break.
Return _the **minimum** number of days needed to complete all tasks_.
**Example 1:**
**Input:** tasks = \[1,2,1,2,3,1\], space = 3
**Output:** 9
**Explanation:**
One way to complete all tasks in 9 days is as follows:
Day 1: Complete the 0th task.
Day 2: Complete the 1st task.
Day 3: Take a break.
Day 4: Take a break.
Day 5: Complete the 2nd task.
Day 6: Complete the 3rd task.
Day 7: Take a break.
Day 8: Complete the 4th task.
Day 9: Complete the 5th task.
It can be shown that the tasks cannot be completed in less than 9 days.
**Example 2:**
**Input:** tasks = \[5,8,8,5\], space = 2
**Output:** 6
**Explanation:**
One way to complete all tasks in 6 days is as follows:
Day 1: Complete the 0th task.
Day 2: Complete the 1st task.
Day 3: Take a break.
Day 4: Take a break.
Day 5: Complete the 2nd task.
Day 6: Complete the 3rd task.
It can be shown that the tasks cannot be completed in less than 6 days.
**Constraints:**
* `1 <= tasks.length <= 105`
* `1 <= tasks[i] <= 109`
* `1 <= space <= tasks.length` | null |
✅Python || Easy Approach | task-scheduler-ii | 0 | 1 | ```\nclass Solution:\n def taskSchedulerII(self, tasks: List[int], space: int) -> int:\n \n ans = 0\n hashset = {}\n n = len(tasks)\n \n for x in set(tasks):\n hashset[x] = 0\n \n i = 0\n while i <= n - 1:\n flag = ans - hashset[tasks[i]]\n if flag >= 0:\n ans += 1\n hashset[tasks[i]] = ans + space\n i += 1\n else:\n ans += -flag\n \n return ans\n``` | 2 | You are given a **0-indexed** array of positive integers `tasks`, representing tasks that need to be completed **in order**, where `tasks[i]` represents the **type** of the `ith` task.
You are also given a positive integer `space`, which represents the **minimum** number of days that must pass **after** the completion of a task before another task of the **same** type can be performed.
Each day, until all tasks have been completed, you must either:
* Complete the next task from `tasks`, or
* Take a break.
Return _the **minimum** number of days needed to complete all tasks_.
**Example 1:**
**Input:** tasks = \[1,2,1,2,3,1\], space = 3
**Output:** 9
**Explanation:**
One way to complete all tasks in 9 days is as follows:
Day 1: Complete the 0th task.
Day 2: Complete the 1st task.
Day 3: Take a break.
Day 4: Take a break.
Day 5: Complete the 2nd task.
Day 6: Complete the 3rd task.
Day 7: Take a break.
Day 8: Complete the 4th task.
Day 9: Complete the 5th task.
It can be shown that the tasks cannot be completed in less than 9 days.
**Example 2:**
**Input:** tasks = \[5,8,8,5\], space = 2
**Output:** 6
**Explanation:**
One way to complete all tasks in 6 days is as follows:
Day 1: Complete the 0th task.
Day 2: Complete the 1st task.
Day 3: Take a break.
Day 4: Take a break.
Day 5: Complete the 2nd task.
Day 6: Complete the 3rd task.
It can be shown that the tasks cannot be completed in less than 6 days.
**Constraints:**
* `1 <= tasks.length <= 105`
* `1 <= tasks[i] <= 109`
* `1 <= space <= tasks.length` | null |
[Java/Python 3] Use HashMap to store task and next available day, w/ brief explanation and analysis. | task-scheduler-ii | 1 | 1 | Traverse `tasks`, for each task `t`, compute the days needed and update the next available day for `t`.\n\n```java\n public long taskSchedulerII(int[] tasks, int space) {\n Map<Integer, Long> nextAvailableDay = new HashMap<>();\n long days = 0;\n for (int t : tasks) {\n days = Math.max(nextAvailableDay.getOrDefault(t, 0L), days + 1);\n nextAvailableDay.put(t, days + space + 1);\n }\n return days;\n }\n```\n\n```python\n def taskSchedulerII(self, tasks: List[int], space: int) -> int:\n next_available_day, days = {}, 0\n for t in tasks:\n days = max(next_available_day.get(t, 0), days + 1)\n next_available_day[t] = days + 1 + space\n return days\n```\n\n**Analysis:**\n\nTime & space: `O(n)`, where `n = tasks.length`. | 13 | You are given a **0-indexed** array of positive integers `tasks`, representing tasks that need to be completed **in order**, where `tasks[i]` represents the **type** of the `ith` task.
You are also given a positive integer `space`, which represents the **minimum** number of days that must pass **after** the completion of a task before another task of the **same** type can be performed.
Each day, until all tasks have been completed, you must either:
* Complete the next task from `tasks`, or
* Take a break.
Return _the **minimum** number of days needed to complete all tasks_.
**Example 1:**
**Input:** tasks = \[1,2,1,2,3,1\], space = 3
**Output:** 9
**Explanation:**
One way to complete all tasks in 9 days is as follows:
Day 1: Complete the 0th task.
Day 2: Complete the 1st task.
Day 3: Take a break.
Day 4: Take a break.
Day 5: Complete the 2nd task.
Day 6: Complete the 3rd task.
Day 7: Take a break.
Day 8: Complete the 4th task.
Day 9: Complete the 5th task.
It can be shown that the tasks cannot be completed in less than 9 days.
**Example 2:**
**Input:** tasks = \[5,8,8,5\], space = 2
**Output:** 6
**Explanation:**
One way to complete all tasks in 6 days is as follows:
Day 1: Complete the 0th task.
Day 2: Complete the 1st task.
Day 3: Take a break.
Day 4: Take a break.
Day 5: Complete the 2nd task.
Day 6: Complete the 3rd task.
It can be shown that the tasks cannot be completed in less than 6 days.
**Constraints:**
* `1 <= tasks.length <= 105`
* `1 <= tasks[i] <= 109`
* `1 <= space <= tasks.length` | null |
【Video】Beat 99% solution with Python, JavaScript, Java and C++ | minimum-replacements-to-sort-the-array | 1 | 1 | # Intuition\nThe most important point is to think about divisible case and not divisible case for number of elements. This Python solution beats 99%.\n\n---\n\n\n# Solution Video\n\n### Please subscribe to my channel from here. I have 252 videos as of August 30th, 2023.\n\n**\u25A0 Subscribe URL**\nhttp://www.youtube.com/channel/UC9RMNwYTL3SXCP6ShLWVFww?sub_confirmation=1\n\nhttps://youtu.be/WaIcjuIGR78\n\n### In the video, the steps of approach below are visualized using diagrams and drawings. I\'m sure you understand the solution easily!\n---\n\n# Approach\nThis is based on Python. Other might be different a bit.\n\n1. **Initialization:**\n - Initialize `current_largest` with the last element of the input array `nums`.\n - Initialize `total_replacements` as 0 to keep track of the total number of replacements.\n\n2. **Iterate Through Array in Reverse:**\n - Start iterating through the input array `nums` in reverse order, from the second-to-last element to the first.\n\n3. **Check If Current Element is Less Than or Equal to `current_largest`:**\n - Compare the current element `nums[i]` with `current_largest`.\n - If `nums[i]` is less than or equal to `current_largest`, update `current_largest` with the value of `nums[i]` and continue to the next iteration.\n\n4. **Calculate Elements When `current_largest` Doesn\'t Divide `nums[i]`:**\n - If `nums[i]` is greater than `current_largest` and is not divisible by `current_largest`, calculate the number of elements needed to represent `nums[i]`.\n - Calculate `num_elements` by dividing `nums[i]` by `current_largest` and adding 1 to ensure that the sum of elements becomes equal to or greater than `nums[i]`.\n\n5. **Calculate Updated `current_largest`:**\n - Update `current_largest` with the result of dividing `nums[i]` by `num_elements`. This ensures that the largest element you can create is less than or equal to the current largest element.\n\n6. **Calculate Elements When `current_largest` Divides `nums[i]`:**\n - If `nums[i]` is greater than `current_largest` and is divisible by `current_largest`, calculate the number of elements needed to represent `nums[i]`.\n - Calculate `num_elements` by dividing `nums[i]` by `current_largest`.\n\n7. **Calculate Replacements and Update Total:**\n - Calculate the number of replacements needed for the current element by subtracting 1 from `num_elements`.\n - Add this value to the `total_replacements` count.\n\n8. **Return `total_replacements`:**\n - After iterating through all elements, return the final count of `total_replacements`.\n\nThis algorithm traverses the array in reverse, calculating the number of replacements required for each element to make the array sorted in non-decreasing order. It optimally divides elements into smaller pieces when necessary to achieve the desired order.\n\n# Complexity\n- Time complexity: O(N)\n\n- Space complexity: O(1)\n\n```python []\nclass Solution:\n def minimumReplacement(self, nums: List[int]) -> int:\n current_largest = nums[-1]\n total_replacements = 0\n \n for i in range(len(nums) - 1, -1, -1):\n if nums[i] <= current_largest:\n current_largest = nums[i]\n continue\n\n if nums[i] % current_largest:\n num_elements = nums[i] // current_largest + 1\n current_largest = nums[i] // num_elements\n else:\n num_elements = nums[i] // current_largest\n \n total_replacements += num_elements - 1\n \n return total_replacements\n```\n```javascript []\n/**\n * @param {number[]} nums\n * @return {number}\n */\nvar minimumReplacement = function(nums) {\n let currentLargest = nums[nums.length - 1];\n let totalReplacements = 0;\n \n for (let i = nums.length - 1; i >= 0; i--) {\n if (nums[i] <= currentLargest) {\n currentLargest = nums[i];\n continue;\n }\n\n let numElements;\n if (nums[i] % currentLargest) {\n numElements = Math.floor(nums[i] / currentLargest) + 1;\n currentLargest = Math.floor(nums[i] / numElements);\n } else {\n numElements = Math.floor(nums[i] / currentLargest);\n }\n \n totalReplacements += numElements - 1;\n }\n \n return totalReplacements; \n};\n```\n```java []\nclass Solution {\n public long minimumReplacement(int[] nums) {\n int currentLargest = nums[nums.length - 1];\n long totalReplacements = 0;\n \n for (int i = nums.length - 1; i >= 0; i--) {\n if (nums[i] <= currentLargest) {\n currentLargest = nums[i];\n continue;\n }\n\n int numElements;\n if (nums[i] % currentLargest != 0) {\n numElements = nums[i] / currentLargest + 1;\n currentLargest = nums[i] / numElements;\n } else {\n numElements = nums[i] / currentLargest;\n }\n \n totalReplacements += numElements - 1;\n }\n \n return totalReplacements; \n }\n}\n```\n```C++ []\nclass Solution {\npublic:\n long long minimumReplacement(vector<int>& nums) {\n int currentLargest = nums[nums.size() - 1];\n long totalReplacements = 0;\n \n for (int i = nums.size() - 1; i >= 0; i--) {\n if (nums[i] <= currentLargest) {\n currentLargest = nums[i];\n continue;\n }\n\n int numElements;\n if (nums[i] % currentLargest != 0) {\n numElements = nums[i] / currentLargest + 1;\n currentLargest = nums[i] / numElements;\n } else {\n numElements = nums[i] / currentLargest;\n }\n \n totalReplacements += numElements - 1;\n }\n \n return totalReplacements; \n }\n};\n```\n | 15 | You are given a **0-indexed** integer array `nums`. In one operation you can replace any element of the array with **any two** elements that **sum** to it.
* For example, consider `nums = [5,6,7]`. In one operation, we can replace `nums[1]` with `2` and `4` and convert `nums` to `[5,2,4,7]`.
Return _the minimum number of operations to make an array that is sorted in **non-decreasing** order_.
**Example 1:**
**Input:** nums = \[3,9,3\]
**Output:** 2
**Explanation:** Here are the steps to sort the array in non-decreasing order:
- From \[3,9,3\], replace the 9 with 3 and 6 so the array becomes \[3,3,6,3\]
- From \[3,3,6,3\], replace the 6 with 3 and 3 so the array becomes \[3,3,3,3,3\]
There are 2 steps to sort the array in non-decreasing order. Therefore, we return 2.
**Example 2:**
**Input:** nums = \[1,2,3,4,5\]
**Output:** 0
**Explanation:** The array is already in non-decreasing order. Therefore, we return 0.
**Constraints:**
* `1 <= nums.length <= 105`
* `1 <= nums[i] <= 109` | null |
C++/Python Math explains Greedy||beats 98.50% | minimum-replacements-to-sort-the-array | 0 | 1 | # Intuition\n<!-- Describe your first thoughts on how to solve this problem. -->\nOnly the number at the end of the array is surely unchanged. Use backward iteration. Compare the last & current numbers. Proceed the division\n$$\ncurr=q*last+r\n$$\nand compute the number of operations and update the value for last.\n# Approach\n<!-- Describe your approach to solving the problem. -->\n3 case to consider\n1. case q=0: last=r (in this case curr!=0 =>r!=0)\n2. case r=0: use (q-1) ops and keep last (spitting curr into q-times last needs (q-1) ops)\n3. case q!=0 and r!=0: use q ops, last-=ceil((last-r)/(q+1)) (Split curr into (q+1) numbers)\n\n# Why in case 3 last-=ceil((last-r)/(q+1))?\nAccording to the division fomula\n$$\ncurr=q*last+r\\\\\n=(q+1)last-(last-r)\n$$\nq times operations will be taken. Among these $q+1$ numbers, some manipulation will be done. The value of $(last - r)$ should be evenly distributed among $(q + 1)$ numbers as the subtrahend, with the resulting smaller value being equal to \n$last - ceil((last - r) / (q + 1))$.\n\nLet\'s consider the testcase ```[7,6,15,6,11,14,10] ```\n```\ni=5-> op+=1 q=1 r=4 last=7\ni=4-> op+=1 q=1 r=4 last=5\ni=3-> op+=1 q=1 r=1 last=3\ni=2-> op+=4 q=5 r=0 last=3\ni=1-> op+=1 q=2 r=0 last=3\ni=0-> op+=2 q=2 r=1 last=2\nop=10\n```\n# 2nd Approach without if clauses\n\nTo fasten the code performance is to minimize the use of if clauses. The process in the iteration is slightly modified as follows:\n1. Perform int division ```q0=(curr-1)/last```\n2. ```op+=q0``` (Note if r=0 then q0=q+1, all if clauses can be saved)\n3. Update last by int division ```last=curr/(q0+1)```( which is the same value in 1st approach and just computed in other way)\n\nSo, neither if clause nor the info on remainder are used.\nThe process for the testcase ```[7,6,15,6,11,14,10] ```\n```\n q0=1 last=7\n q0=1 last=5\n q0=1 last=3\n q0=4 last=3\n q0=1 last=3\n q0=2 last=2\nop=10\n```\n# Complexity\n- Time complexity:\n<!-- Add your time complexity here, e.g. $$O(n)$$ -->\n$$O(n)$$\n- Space complexity:\n<!-- Add your space complexity here, e.g. $$O(n)$$ -->\n$$O(1)$$\n\n# 1st approach Code beats Beats 66.7% runtime 94 ms\n```\nclass Solution {\npublic:\n long long minimumReplacement(vector<int>& nums) {\n long long op=0;\n int last=nums.back();\n for(int i=nums.size()-2; i>=0; i--){\n auto [q, r]=div(nums[i], last);//curr=q*last+r\n if (q==0){\n last=r;\n }\n else if (r==0){ //[last, last, ..., last] use (q-1) ops\n op+=(q-1);\n // cout<<(q-1);\n }\n else{//curr=(q+1)last-(last-r)\n op+=q;\n // cout<<q;\n last-=(last-r+q)/(q+1);//-=ceil((last-r)/(q+1))\n }\n // cout<<"->"<<last<<"\\n"; \n }\n return op;\n }\n};\n```\n# Python code using 1 approach\n```\nclass Solution:\n def minimumReplacement(self, nums: List[int]) -> int:\n op=0\n n=len(nums)\n last=nums[n-1]\n for i in range(n-2, -1, -1):\n q, r=divmod(nums[i], last)\n if q==0:\n last=r\n elif r==0:\n op+=(q-1)\n else:\n op+=q\n last-=((last-r+q)//(q+1)) #-=ceil((last-r)/(q+1))\n return op\n```\n# C++ code using 2nd approach beats 98.50% runtime 74 ms\n```\nclass Solution {\npublic:\n long long minimumReplacement(vector<int>& nums) {\n long long op=0;\n int last=nums.back();\n for(int i=nums.size()-2; i>=0; i--){\n int q0=(nums[i]-1)/last;\n op+=q0;\n last=nums[i]/(q0+1);\n // cout<<" q0="<<q0<<" last="<<last<<"\\n"; \n }\n // cout<<"op="<<op<<endl;\n return op;\n }\n};\n```\n | 7 | You are given a **0-indexed** integer array `nums`. In one operation you can replace any element of the array with **any two** elements that **sum** to it.
* For example, consider `nums = [5,6,7]`. In one operation, we can replace `nums[1]` with `2` and `4` and convert `nums` to `[5,2,4,7]`.
Return _the minimum number of operations to make an array that is sorted in **non-decreasing** order_.
**Example 1:**
**Input:** nums = \[3,9,3\]
**Output:** 2
**Explanation:** Here are the steps to sort the array in non-decreasing order:
- From \[3,9,3\], replace the 9 with 3 and 6 so the array becomes \[3,3,6,3\]
- From \[3,3,6,3\], replace the 6 with 3 and 3 so the array becomes \[3,3,3,3,3\]
There are 2 steps to sort the array in non-decreasing order. Therefore, we return 2.
**Example 2:**
**Input:** nums = \[1,2,3,4,5\]
**Output:** 0
**Explanation:** The array is already in non-decreasing order. Therefore, we return 0.
**Constraints:**
* `1 <= nums.length <= 105`
* `1 <= nums[i] <= 109` | null |
[Python] greedy O(n) explained | minimum-replacements-to-sort-the-array | 0 | 1 | When iterating from the back of the array, each encountered value should not exceed the previous one (the array should be non-decreasing). Once this condition fails, a correspodning number `n` should be divided into a sum of elements, each one not exceeding `m` (i.e., the previous element from the back). The minimal numbers of such elements is `d = ceil(n/m)`. After making the division and replacing the original number `n` with a list of its summands, the algorithm should continue, however, this time with all further elements not exceeding the minimal of obtained summands. The best (i.e., maximal) value of the minimal summand is `floor(n/d)`.\n\nFor example, in the list `[...,13,5]` the best way (i.e., with the maximum value of the minimal summand) to replace `15` would be `[...,4,4,5,5]`. All other divisions, e.g., `[...,3,5,5,5]` are worse because they decrease the value of the minimum summand, thus, potentially increasing the number of operations on subsequent steps.\n\n```python\nclass Solution:\n def minimumReplacement(self, nums: List[int]) -> int:\n\n m = inf\n ops = 0\n\n for n in reversed(nums): \n if n < m: \n m = n\n continue\n d = (n-1) // m + 1 # number of summands \n ops += d-1 # number of operations\n m = n // d # smallest of d summands \n \n return ops\n``` | 7 | You are given a **0-indexed** integer array `nums`. In one operation you can replace any element of the array with **any two** elements that **sum** to it.
* For example, consider `nums = [5,6,7]`. In one operation, we can replace `nums[1]` with `2` and `4` and convert `nums` to `[5,2,4,7]`.
Return _the minimum number of operations to make an array that is sorted in **non-decreasing** order_.
**Example 1:**
**Input:** nums = \[3,9,3\]
**Output:** 2
**Explanation:** Here are the steps to sort the array in non-decreasing order:
- From \[3,9,3\], replace the 9 with 3 and 6 so the array becomes \[3,3,6,3\]
- From \[3,3,6,3\], replace the 6 with 3 and 3 so the array becomes \[3,3,3,3,3\]
There are 2 steps to sort the array in non-decreasing order. Therefore, we return 2.
**Example 2:**
**Input:** nums = \[1,2,3,4,5\]
**Output:** 0
**Explanation:** The array is already in non-decreasing order. Therefore, we return 0.
**Constraints:**
* `1 <= nums.length <= 105`
* `1 <= nums[i] <= 109` | null |
Greedy Python Solution with Thought Process || One For Loop + Two If Statements | minimum-replacements-to-sort-the-array | 0 | 1 | # Code\n```\nclass Solution:\n def minimumReplacement(self, nums: List[int]) -> int:\n N = len(nums)\n splits = 0\n \n for idx in reversed(range(1, N)):\n left, curr = nums[idx - 1], nums[idx]\n \n if left > curr:\n quotient = left // curr\n remainder = left % curr\n \n if remainder == 0:\n nums[idx - 1] = curr\n splits += quotient - 1\n else:\n nums[idx - 1] = left // (quotient + 1)\n splits += quotient\n \n return splits\n```\n\n# Intuition\nWe don\'t have a way to rearrange numbers. The only thing we can do is split a number until it falls below the number to the right of it. There\'s also an objectively optimal way to split each number. Thus, a greedy approach might just be enough.\n\n# Approach\nWe\'ll start from the end:\n```\nfor idx in reversed(range(1, N)):\n left, curr = nums[idx - 1], nums[idx]\n```\nIf we ever encounter a number that\'s larger than it should be, we split it into parts. We want to split numbers in a such a way that we maximize the leftmost splitted part. This way, we give the most breathing room for the numbers further down left.\n\nFor example, we would prefer to split the subsequence [9, 5] into [3,3,3,5] rather than [1,4,4,5].\n\nWe cannot make the leftmost splitted part bigger than the rest of the parts without breaking the sorted property. The best we can do to maximize the leftmost number is by splitting as evenly as we possibly can:\n\n```\n if left > curr:\n quotient = left // curr\n remainder = left % curr\n \n if remainder == 0:\n nums[idx - 1] = curr\n splits += quotient - 1\n else:\n nums[idx - 1] = left // (quotient + 1)\n splits += quotient\n```\n\nNotice that the numbers in between our splits don\'t matter. This will save us the from having to insert the other splitted parts back into the array.\n\nFor example, when we split 14 in [14, 5] to [4,5,5,5], the two new 5\'s are irrelevant. Only the leftmost 4 will be relevant for the rest of the algorithm. We\'ll "split" 14, but only keep it\'s leftmost part 4, so our algorithm would treat it as [4, 5].\n\n# Complexity\n- Time complexity:\nO(n)\n\n- Space complexity:\nO(1)\n | 3 | You are given a **0-indexed** integer array `nums`. In one operation you can replace any element of the array with **any two** elements that **sum** to it.
* For example, consider `nums = [5,6,7]`. In one operation, we can replace `nums[1]` with `2` and `4` and convert `nums` to `[5,2,4,7]`.
Return _the minimum number of operations to make an array that is sorted in **non-decreasing** order_.
**Example 1:**
**Input:** nums = \[3,9,3\]
**Output:** 2
**Explanation:** Here are the steps to sort the array in non-decreasing order:
- From \[3,9,3\], replace the 9 with 3 and 6 so the array becomes \[3,3,6,3\]
- From \[3,3,6,3\], replace the 6 with 3 and 3 so the array becomes \[3,3,3,3,3\]
There are 2 steps to sort the array in non-decreasing order. Therefore, we return 2.
**Example 2:**
**Input:** nums = \[1,2,3,4,5\]
**Output:** 0
**Explanation:** The array is already in non-decreasing order. Therefore, we return 0.
**Constraints:**
* `1 <= nums.length <= 105`
* `1 <= nums[i] <= 109` | null |
✅Easy to understand🔥||✅Full Explanation🔥Done in few steps🔥||O(n) Solution | minimum-replacements-to-sort-the-array | 1 | 1 | # Problem Understanding:\nThe problem requires us to determine the **minimum number of operations** needed to make an array **sorted** in non-decreasing order. Each operation involves **replacing** an element with **two elements** that sum to it.\n\n# Logic Used in the problem\nThe logic revolves around the idea of iteratively choosing the **optimal replacement strategy** for each element to ensure non-decreasing order while **minimizing the number of operations**. The division operation is used to determine how many times an element needs to be divided to fit within the constraints of non-decreasing order. This approach **cleverly balances the need for replacement operations** with the goal of keeping the array sorted with minimal changes.\n<!-- Describe your first thoughts on how to solve this problem. -->\n\n# Approach\n1. **Traverse** the array in reverse order.\n2. For each element, check if it is **greater** than the **previously** encountered element (last).\n3. If the current element is greater, **calculate the number of times** it needs to be divided to become less than or equal to the last element.\n4. **Update** the last element to the value that the **current element** needs to be divided by.\n5.**Add** the calculated number of operations (divisions - 1) to the **total count** of operations.\n6. **Repeat** these steps for all elements in the array.\n<!-- Describe your approach to solving the problem. -->\n\n# SMALL REQUEST : If you found this post even remotely helpful, be kind enough to smash a upvote. I will be grateful.I will be motivated\uD83D\uDE0A\uD83D\uDE0A\n\n# Complexity\n- Time complexity: O(n)\n<!-- Add your time complexity here, e.g. $$O(n)$$ -->\n\n- Space complexity: O(1)\n<!-- Add your space complexity here, e.g. $$O(n)$$ -->\n\n# Code\n```C++ []\nclass Solution {\npublic:\n long long minimumReplacement(vector<int>& nums) {\n int n = nums.size();\n int last = nums[n-1]; // Initialize \'last\' with the last element\n long long ans = 0; // Initialize the total operations count\n\n // Traverse the array in reverse order\n for (int i = n - 2; i >= 0; --i) {\n if (nums[i] > last) { // If the current element needs replacement\n int t = nums[i] / last; // Calculate how many times the element needs to be divided\n if (nums[i] % last) t++; // If there\'s a remainder, increment \'t\'\n last = nums[i] / t; // Update \'last\' for the next comparison\n ans += t - 1; // Add (t - 1) to \'ans\' for the number of operations\n } else {\n last = nums[i]; // Update \'last\' without replacement\n }\n }\n return ans; // Return the total number of operations\n }\n\n};\n```\n```python3 []\nclass Solution:\n def minimumReplacement(self, nums: List[int]) -> int:\n n = len(nums)\n last = nums[n - 1] # Initialize \'last\' with the last element\n ans = 0 # Initialize the total operations count\n\n # Traverse the array in reverse order\n for i in range(n - 2, -1, -1):\n if nums[i] > last: # If the current element needs replacement\n t = nums[i] // last # Calculate how many times the element needs to be divided\n if nums[i] % last:\n t += 1 # If there\'s a remainder, increment \'t\'\n last = nums[i] // t # Update \'last\' for the next comparison\n ans += t - 1 # Add (t - 1) to \'ans\' for the number of operations\n else:\n last = nums[i] # Update \'last\' without replacement\n return ans # Return the total number of operations\n\n```\n```Java []\nclass Solution {\n public long minimumReplacement(int[] nums) {\n int n = nums.length;\n int last = nums[n - 1]; // Initialize \'last\' with the last element\n long ans = 0; // Initialize the total operations count\n\n // Traverse the array in reverse order\n for (int i = n - 2; i >= 0; --i) {\n if (nums[i] > last) { // If the current element needs replacement\n int t = nums[i] / last; // Calculate how many times the element needs to be divided\n if (nums[i] % last != 0) {\n t++; // If there\'s a remainder, increment \'t\'\n }\n last = nums[i] / t; // Update \'last\' for the next comparison\n ans += t - 1; // Add (t - 1) to \'ans\' for the number of operations\n } else {\n last = nums[i]; // Update \'last\' without replacement\n }\n }\n return ans; // Return the total number of operations\n }\n}\n\n```\n```javascript []\n/**\n * @param {number[]} nums\n * @return {number}\n */\nvar minimumReplacement = function(nums) {\n const n = nums.length;\n let last = nums[n - 1]; // Initialize \'last\' with the last element\n let ans = 0; // Initialize the total operations count\n\n // Traverse the array in reverse order\n for (let i = n - 2; i >= 0; --i) {\n if (nums[i] > last) { // If the current element needs replacement\n let t = Math.floor(nums[i] / last); // Calculate how many times the element needs to be divided\n if (nums[i] % last !== 0) {\n t++; // If there\'s a remainder, increment \'t\'\n }\n last = Math.floor(nums[i] / t); // Update \'last\' for the next comparison\n ans += t - 1; // Add (t - 1) to \'ans\' for the number of operations\n } else {\n last = nums[i]; // Update \'last\' without replacement\n }\n }\n return ans; // Return the total number of operations\n};\n\n\n```\n# SMALL REQUEST : If you found this post even remotely helpful, be kind enough to smash a upvote. I will be grateful.I will be motivated\uD83D\uDE0A\uD83D\uDE0A\n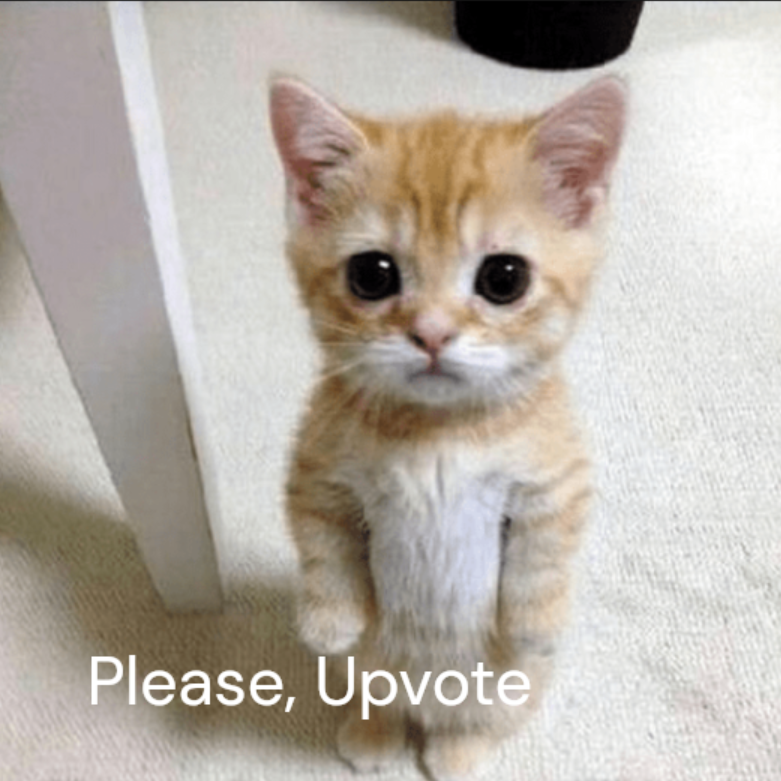\n | 330 | You are given a **0-indexed** integer array `nums`. In one operation you can replace any element of the array with **any two** elements that **sum** to it.
* For example, consider `nums = [5,6,7]`. In one operation, we can replace `nums[1]` with `2` and `4` and convert `nums` to `[5,2,4,7]`.
Return _the minimum number of operations to make an array that is sorted in **non-decreasing** order_.
**Example 1:**
**Input:** nums = \[3,9,3\]
**Output:** 2
**Explanation:** Here are the steps to sort the array in non-decreasing order:
- From \[3,9,3\], replace the 9 with 3 and 6 so the array becomes \[3,3,6,3\]
- From \[3,3,6,3\], replace the 6 with 3 and 3 so the array becomes \[3,3,3,3,3\]
There are 2 steps to sort the array in non-decreasing order. Therefore, we return 2.
**Example 2:**
**Input:** nums = \[1,2,3,4,5\]
**Output:** 0
**Explanation:** The array is already in non-decreasing order. Therefore, we return 0.
**Constraints:**
* `1 <= nums.length <= 105`
* `1 <= nums[i] <= 109` | null |
Python3 Solution | minimum-replacements-to-sort-the-array | 0 | 1 | \n```\nclass Solution:\n def minimumReplacement(self, nums: List[int]) -> int:\n ans=0\n prev=10**9+1\n for x in nums[::-1]:\n d=ceil(x/prev)\n ans+=d-1\n prev=x//d\n\n return ans \n``` | 2 | You are given a **0-indexed** integer array `nums`. In one operation you can replace any element of the array with **any two** elements that **sum** to it.
* For example, consider `nums = [5,6,7]`. In one operation, we can replace `nums[1]` with `2` and `4` and convert `nums` to `[5,2,4,7]`.
Return _the minimum number of operations to make an array that is sorted in **non-decreasing** order_.
**Example 1:**
**Input:** nums = \[3,9,3\]
**Output:** 2
**Explanation:** Here are the steps to sort the array in non-decreasing order:
- From \[3,9,3\], replace the 9 with 3 and 6 so the array becomes \[3,3,6,3\]
- From \[3,3,6,3\], replace the 6 with 3 and 3 so the array becomes \[3,3,3,3,3\]
There are 2 steps to sort the array in non-decreasing order. Therefore, we return 2.
**Example 2:**
**Input:** nums = \[1,2,3,4,5\]
**Output:** 0
**Explanation:** The array is already in non-decreasing order. Therefore, we return 0.
**Constraints:**
* `1 <= nums.length <= 105`
* `1 <= nums[i] <= 109` | null |
python3 solution | minimum-replacements-to-sort-the-array | 0 | 1 | # Complexity\n- Time complexity:\nO(n)\n- Space complexity:\nO(1)\n# Code\n```\nclass Solution:\n def minimumReplacement(self, nums: List[int]) -> int:\n res=0\n for i in range(len(nums)-2, -1, -1):\n if nums[i] <= nums[i+1]: continue\n # We are using ceiling division method to count the possible even ways to break the number\n ceil_division_break_count = (nums[i]+nums[i+1]-1)//nums[i+1]\n res += ceil_division_break_count - 1\n nums[i] //= ceil_division_break_count\n return res\n``` | 1 | You are given a **0-indexed** integer array `nums`. In one operation you can replace any element of the array with **any two** elements that **sum** to it.
* For example, consider `nums = [5,6,7]`. In one operation, we can replace `nums[1]` with `2` and `4` and convert `nums` to `[5,2,4,7]`.
Return _the minimum number of operations to make an array that is sorted in **non-decreasing** order_.
**Example 1:**
**Input:** nums = \[3,9,3\]
**Output:** 2
**Explanation:** Here are the steps to sort the array in non-decreasing order:
- From \[3,9,3\], replace the 9 with 3 and 6 so the array becomes \[3,3,6,3\]
- From \[3,3,6,3\], replace the 6 with 3 and 3 so the array becomes \[3,3,3,3,3\]
There are 2 steps to sort the array in non-decreasing order. Therefore, we return 2.
**Example 2:**
**Input:** nums = \[1,2,3,4,5\]
**Output:** 0
**Explanation:** The array is already in non-decreasing order. Therefore, we return 0.
**Constraints:**
* `1 <= nums.length <= 105`
* `1 <= nums[i] <= 109` | null |
Python: Observation. How to reach the solution | minimum-replacements-to-sort-the-array | 0 | 1 | # Observation\nSearching the minimum cost with a constraint(non-decreasing order form) can be tackled with DP, BS(Binary Search), or Greedy. \n\nHowever, the absence of a clear boundary and the unsorted series(num) hint us that it\'s not a BS problem. Also, The lack of a clear monotonic order(must do A before B) within the problem makes DP infeasible.\n\nNevertheless, we can identify a recursive relationship that holds potential. Given a sorted array `A` and a new value `x`, we can take two apporaches.\n\n1. Append `x` on `A`: We must ensure `x` is greater than `A[-1]`, otherwise we divide `A[-1]` and recursively fix the `A`.\n2. Preppend `x` on `A`: We must ensure `x` must be equal or smaller than `A[0]`, otherwise we divide `x`.\n\nThe second approach is much easier and computation efficient. Consequently, I have chosen to implement the solution using this second method.\n\n# Intuition\nFollow the second approach in Observation.\n\nThe way we divide `x` is intuitive: it\'s optimal to divide `x` as evenly as possible.\n\n# Code\n```\nclass Solution:\n def minimumReplacement(self, A: List[int]) -> int:\n res = 0\n t = A[-1] # threshold\n for x in A[:-1][::-1]:\n if x > t:\n div = x // t\n if x % t:\n div += 1\n res += div - 1\n t = x // div\n else:\n t = x\n return res\n\n\n``` | 1 | You are given a **0-indexed** integer array `nums`. In one operation you can replace any element of the array with **any two** elements that **sum** to it.
* For example, consider `nums = [5,6,7]`. In one operation, we can replace `nums[1]` with `2` and `4` and convert `nums` to `[5,2,4,7]`.
Return _the minimum number of operations to make an array that is sorted in **non-decreasing** order_.
**Example 1:**
**Input:** nums = \[3,9,3\]
**Output:** 2
**Explanation:** Here are the steps to sort the array in non-decreasing order:
- From \[3,9,3\], replace the 9 with 3 and 6 so the array becomes \[3,3,6,3\]
- From \[3,3,6,3\], replace the 6 with 3 and 3 so the array becomes \[3,3,3,3,3\]
There are 2 steps to sort the array in non-decreasing order. Therefore, we return 2.
**Example 2:**
**Input:** nums = \[1,2,3,4,5\]
**Output:** 0
**Explanation:** The array is already in non-decreasing order. Therefore, we return 0.
**Constraints:**
* `1 <= nums.length <= 105`
* `1 <= nums[i] <= 109` | null |
✅Easy Solution🔥Python/C#/C++/Java/C🔥Explain Line by Line🔥 | minimum-replacements-to-sort-the-array | 1 | 1 | # Problem\n---\nThe problem statement describes an array manipulation task where you are given an array nums, and you can perform operations that involve replacing an element of the array with two elements that sum up to the original element. The goal is to sort the array in non-decreasing order using the minimum number of these operations.\n\nThe given solution is implemented in Python and provides a method minimumReplacement that takes a list of integers (nums) as input and returns an integer representing the minimum number of operations required to sort the array.\n\n\n# Solution\n---\n1. Initialize variables:\n\n- n: The length of the input array nums.\n- ops: A counter to keep track of the number of operations performed.\n- prev: A variable to keep track of the previously processed element.\n\n2. Start iterating over the array in reverse (from the second-to-last element to the first element):\n\n- Compare the current element nums[i] with the prev element.\n- If nums[i] is greater than prev, this means the current element needs to be reduced. To do this, find an integer k that, when multiplied by prev, is just greater than or equal to nums[i]. This ensures that after the operation, the element becomes smaller or equal to prev.\n- Increment the ops counter by k - 1 because you are performing one operation but splitting it into k parts.\n- Update prev to be nums[i] // k. This is because after the operation, you want the current element to be equal to prev, and since you\'ve divided it into k parts, each part should be nums[i] // k.\n- If nums[i] is not greater than prev, simply update prev to be nums[i].\n\n\n3. After iterating through the entire array, the ops counter will represent the minimum number of operations required to sort the array in non-decreasing order. Return this value.\n\nIn summary, the solution iterates through the array in reverse, replacing elements with smaller elements using a specific strategy to minimize the number of operations. It keeps track of the required operations using the ops counter and returns the final count.\n\nThe solution seems correct and efficient in solving the given problem. It leverages the idea of using the available operations to adjust the elements in such a way that the array becomes sorted.\n\n---\n\n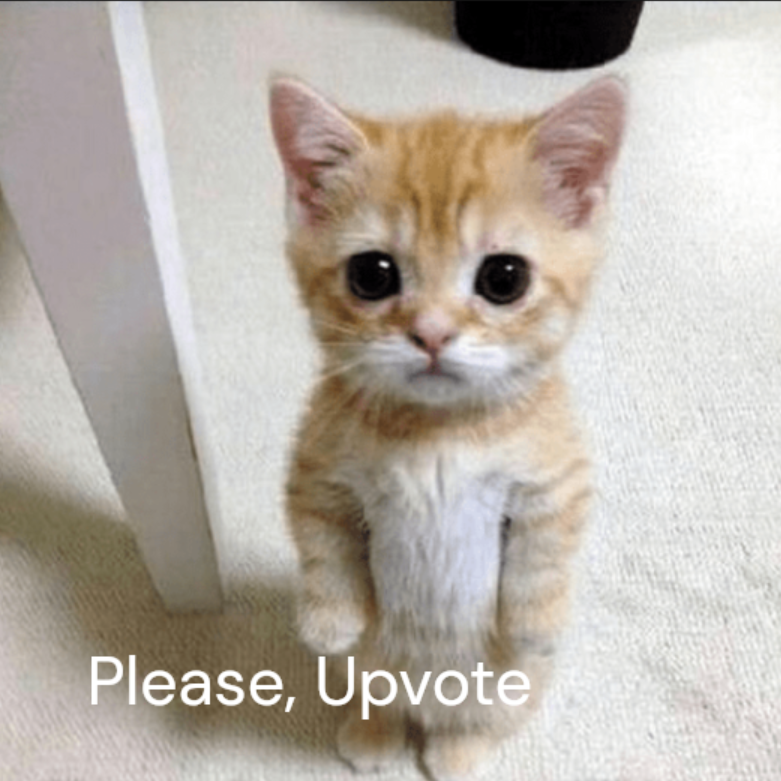\n\n---\n\n```Python3 []\nclass Solution:\n def minimumReplacement(self, nums: List[int]) -> int:\n n = len(nums)\n ops = 0\n\n prev = nums[n - 1]\n\n for i in range(n - 2, -1, -1):\n if nums[i] > prev:\n k = math.ceil(nums[i] / prev)\n ops += k - 1\n prev = nums[i] // k\n else:\n prev = nums[i]\n return ops\n```\n```python []\nclass Solution:\n def minimumReplacement(self, nums: List[int]) -> int:\n n = len(nums)\n ops = 0\n\n prev = nums[n - 1]\n\n for i in range(n - 2, -1, -1):\n if nums[i] > prev:\n k = math.ceil(nums[i] / prev)\n ops += k - 1\n prev = nums[i] // k\n else:\n prev = nums[i]\n return ops\n```\n```C# []\npublic class Solution {\n public long MinimumReplacement(int[] nums) {\n long ans = 0;\n int lastOne = nums[nums.Length - 1];\n for(int i = nums.Length - 2; i >= 0; i--){\n if(nums[i] > lastOne){\n int times = nums[i] / lastOne;\n ans += times - 1;\n int rest = nums[i] % lastOne;\n if(rest != 0){\n lastOne = nums[i] / (times + 1); \n ans++;\n }\n }else{\n lastOne = nums[i];\n }\n }\n return ans;\n }\n}\n```\n```C++ []\nclass Solution {\npublic:\n long long minimumReplacement(std::vector<int>& nums) {\n int n = nums.size();\n long long ops = 0;\n\n int prev = nums[n - 1];\n\n for (int i = n - 2; i >= 0; i--) {\n if (nums[i] > prev) {\n int k = std::ceil(nums[i] / static_cast<double>(prev));\n ops += k - 1;\n prev = nums[i] / k;\n } else {\n prev = nums[i];\n }\n }\n return ops;\n }\n};\n```\n```Java []\nclass Solution {\n public long minimumReplacement(int[] nums) {\n int n = nums.length;\n long ops = 0;\n\n int prev = nums[n - 1];\n\n for (int i = n - 2; i >= 0; i--) {\n if (nums[i] > prev) {\n int k = (int) Math.ceil(nums[i] / (double) prev);\n ops += k - 1;\n prev = nums[i] / k;\n } else {\n prev = nums[i];\n }\n }\n return ops;\n }\n}\n``` | 12 | You are given a **0-indexed** integer array `nums`. In one operation you can replace any element of the array with **any two** elements that **sum** to it.
* For example, consider `nums = [5,6,7]`. In one operation, we can replace `nums[1]` with `2` and `4` and convert `nums` to `[5,2,4,7]`.
Return _the minimum number of operations to make an array that is sorted in **non-decreasing** order_.
**Example 1:**
**Input:** nums = \[3,9,3\]
**Output:** 2
**Explanation:** Here are the steps to sort the array in non-decreasing order:
- From \[3,9,3\], replace the 9 with 3 and 6 so the array becomes \[3,3,6,3\]
- From \[3,3,6,3\], replace the 6 with 3 and 3 so the array becomes \[3,3,3,3,3\]
There are 2 steps to sort the array in non-decreasing order. Therefore, we return 2.
**Example 2:**
**Input:** nums = \[1,2,3,4,5\]
**Output:** 0
**Explanation:** The array is already in non-decreasing order. Therefore, we return 0.
**Constraints:**
* `1 <= nums.length <= 105`
* `1 <= nums[i] <= 109` | null |
✅ 99.17% O(n) Greedy with Dynamic Upper Bound Adjustment | minimum-replacements-to-sort-the-array | 1 | 1 | # Interview Guide - Minimum Replacements to Sort the Array: \n\n## Problem Understanding\n\n**Description**: \nAt its core, this problem is an intricate dance between array manipulation and greedy optimization. You\'re handed an integer array `nums` and given the ability to perform a unique, transformative operation: replace an element with two elements whose sum equals the original element. The goal is deceptively simple yet intriguing\u2014transform this array into a non-decreasing sequence while minimizing the number of these replacement operations.\n\n## Key Points to Consider\n\n### 1. Understand the Constraints\n- Array length: $$1 \\leq \\text{nums.length} \\leq 10^5$$\n- Array elements: $$1 \\leq \\text{nums[i]} \\leq 10^9$$\n \n### 2. Focus on the Unusual\n- The operation to replace a number with two numbers that sum to it is unusual but vital. Recognize that this operation allows us to control the \'granularity\' of our array, breaking down larger numbers into more manageable, smaller ones.\n\n### 3. Contextualizing the Greedy Approach\n- The problem invites a greedy algorithm, not just as a means but as a strategic pathway. We work from right to left, maintaining a dynamic upper bound (`prev_bound`) that serves as our guiding star. This is crucial, as it enables us to make localized, optimal choices that contribute to a global optimum.\n\n### 4. The Magic of Dynamic Upper Bound\n- The concept of a dynamic upper bound (`prev_bound`) is key. As you move from the last element towards the first, this variable adapts to reflect the largest possible number you can place at the current position without requiring further replacements. It\u2019s like having an ever-changing speed limit that you must adhere to but want to get as close to as possible. \n\n### 5. Importance of Reverse Iteration\n- Why do we iterate from the end? Because the last element acts as a natural, immutable upper bound. It sets the stage for the rest of the elements, offering an immediate reference for how small the preceding elements need to be.\n\n### 6. Explain Your Thought Process\n- The algorithm employs a greedy approach, iterating from the rightmost to the leftmost element. An upper bound (`prev_bound`) is maintained and updated dynamically as the algorithm progresses.\n- For each element, the algorithm calculates how many times it should be divided (`no_of_times`) to maintain a non-decreasing order and minimize the number of operations.\n\n---\n\n# More than Live Coding:\nhttps://youtu.be/c7NZJRt89dc?si=MP5lvbp9IbzczVKt\n\n---\n\n# Approach: Greedy Algorithm with Dynamic Upper Bound Adjustment\n\n### Intuition and Logic Behind the Solution\n\nThe problem asks us to convert an array into a non-decreasing sorted array by replacing any element with two elements that sum to it. Essentially, we\'re breaking down larger numbers into smaller ones to sort the array.\n\n#### The Trick\n\nThe trick lies in two observations:\n1. The last element never needs to be replaced because any number can be broken down into smaller components.\n2. The optimal strategy is to replace a number with smaller numbers that are as large as possible but still smaller than the preceding number. This ensures fewer replacements in subsequent steps.\n\n#### Why Greedy?\n\nThe greedy approach comes into play when we decide which numbers to replace and how to replace them. We want to make sure that we replace as few numbers as possible and that the new numbers are as large as possible without being larger than the next number in the sorted sequence. This is why we initialize `prev_bound` with the last element of the array; it\'s the largest possible value we can get without needing to replace the last element.\n\n### Step-by-step Explanation\n\n#### Initialization\n- We start by initializing an `operations` counter to zero. This will keep track of the minimum number of operations required.\n- We also initialize `prev_bound` with the last element of the array, which serves as our dynamic upper bound for the current iteration.\n\n#### Main Algorithm\n1. **Iterate in Reverse**: We loop through the array in reverse order, skipping the last element.\n - **Calculate `no_of_times`**: For the current element (`num`), we calculate how many times it needs to be replaced in order to be smaller than or equal to `prev_bound`. The formula `(num + prev_bound - 1) // prev_bound` is a neat trick to handle both the cases when `num` is divisible by `prev_bound` and when it isn\'t.\n - **Update Operations**: We then increment the `operations` counter by `no_of_times - 1` (the `-1` is because one of the replaced numbers will occupy the original spot, so it\'s not a \'new\' operation).\n - **Update `prev_bound`**: We set the new `prev_bound` as `num // no_of_times`. This ensures that in the next iteration, we are replacing numbers in the most optimal way possible.\n\n#### Wrap-up\n- Finally, the function returns the `operations` counter, which holds the minimum number of operations required to sort the array.\n\n### Complexity Analysis\nThe time complexity is $$O(N)$$, where $$N$$ is the length of the array, because we traverse the array once. There\'s no additional space used, so the space complexity is $$O(1)$$.\n\n---\n\n# Performance\n\n| Language | Runtime (ms) | Memory (MB) |\n|------------|--------------|-------------|\n| Java | 5 | 54.2 |\n| Rust | 13 | 4 |\n| JavaScript | 72 | 50.7 |\n| C++ | 75 | 54.6 |\n| Go | 90 | 10.6 |\n| C# | 168 | 48.6 |\n| PHP | 183 | 32.3 |\n| Python3 | 448 | 31.4 |\n\n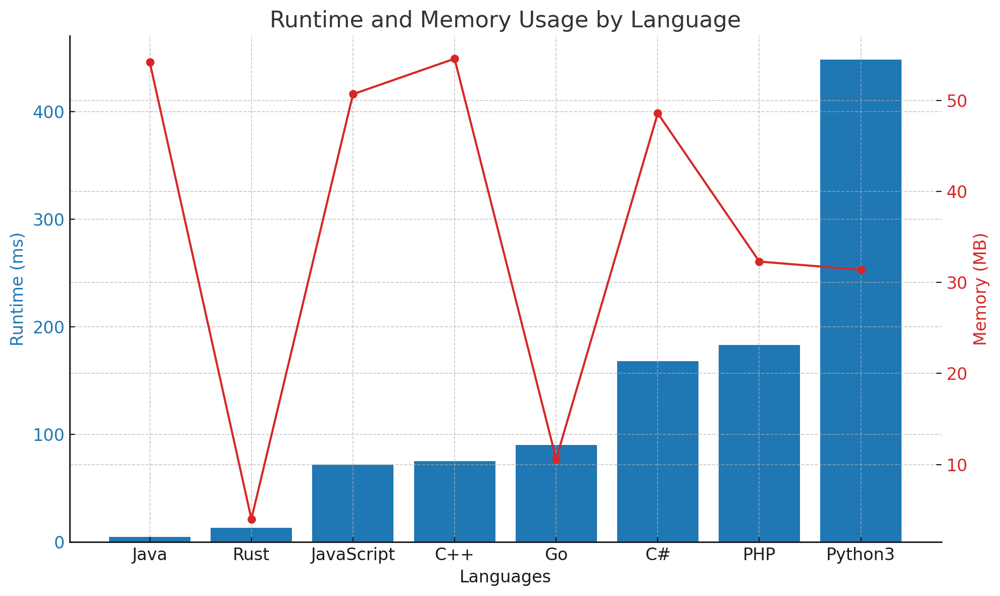\n\n\n# Code\n``` Python []\nclass Solution:\n def minimumReplacement(self, nums: List[int]) -> int:\n operations = 0\n prev_bound = nums[-1]\n\n for num in reversed(nums[:-1]):\n no_of_times = (num + prev_bound - 1) // prev_bound\n operations += no_of_times - 1\n prev_bound = num // no_of_times\n \n return operations\n```\n``` C++ []\nclass Solution {\npublic:\n long long minimumReplacement(std::vector<int>& nums) {\n long long operations = 0;\n long long prev_bound = nums.back();\n \n for (auto it = nums.rbegin() + 1; it != nums.rend(); ++it) {\n long long num = *it;\n long long no_of_times = (num + prev_bound - 1) / prev_bound;\n operations += no_of_times - 1;\n prev_bound = num / no_of_times;\n }\n \n return operations;\n }\n};\n```\n``` Rust []\nimpl Solution {\n pub fn minimum_replacement(nums: Vec<i32>) -> i64 {\n let mut operations: i64 = 0;\n let mut prev_bound: i64 = *nums.last().unwrap() as i64;\n \n for &num in nums.iter().rev().skip(1) {\n let num: i64 = num as i64;\n let no_of_times: i64 = (num + prev_bound - 1) / prev_bound;\n operations += no_of_times - 1;\n prev_bound = num / no_of_times;\n }\n \n operations\n }\n}\n```\n``` Go []\nfunc minimumReplacement(nums []int) int64 {\n var operations int64 = 0\n var prev_bound int64 = int64(nums[len(nums) - 1])\n \n for i := len(nums) - 2; i >= 0; i-- {\n var num int64 = int64(nums[i])\n var no_of_times int64 = (num + prev_bound - 1) / prev_bound\n operations += no_of_times - 1\n prev_bound = num / no_of_times\n }\n \n return operations\n}\n```\n``` Java []\npublic class Solution {\n public long minimumReplacement(int[] nums) {\n long operations = 0;\n long prev_bound = nums[nums.length - 1];\n \n for (int i = nums.length - 2; i >= 0; i--) {\n long num = nums[i];\n long no_of_times = (num + prev_bound - 1) / prev_bound;\n operations += no_of_times - 1;\n prev_bound = num / no_of_times;\n }\n \n return operations;\n }\n}\n```\n``` JavaScript []\n/**\n * @param {number[]} nums\n * @return {number}\n */\nvar minimumReplacement = function(nums) {\n let operations = 0;\n let prev_bound = nums[nums.length - 1];\n \n for (let i = nums.length - 2; i >= 0; i--) {\n let num = nums[i];\n let no_of_times = Math.ceil(num / prev_bound);\n operations += no_of_times - 1;\n prev_bound = Math.floor(num / no_of_times);\n }\n \n return operations;\n};\n```\n``` PHP []\nclass Solution {\n function minimumReplacement($nums) {\n $operations = 0;\n $prev_bound = end($nums);\n \n for ($i = count($nums) - 2; $i >= 0; $i--) {\n $num = $nums[$i];\n $no_of_times = intdiv($num + $prev_bound - 1, $prev_bound);\n $operations += $no_of_times - 1;\n $prev_bound = intdiv($num, $no_of_times);\n }\n \n return $operations;\n }\n}\n```\n``` C# []\npublic class Solution {\n public long MinimumReplacement(int[] nums) {\n long operations = 0;\n long prev_bound = nums[^1];\n \n for (int i = nums.Length - 2; i >= 0; i--) {\n long num = nums[i];\n long no_of_times = (num + prev_bound - 1) / prev_bound;\n operations += no_of_times - 1;\n prev_bound = num / no_of_times;\n }\n \n return operations;\n }\n}\n```\nEvery line of code brings you one step closer to becoming an expert. Whether you value brevity or clarity, each approach has its merits. So jump in, explore, and don\'t hesitate to share your unique insights. Your perspective might be the key to someone else\'s breakthrough. Happy Coding! \uD83D\uDE80\uD83D\uDCA1\uD83C\uDF1F | 47 | You are given a **0-indexed** integer array `nums`. In one operation you can replace any element of the array with **any two** elements that **sum** to it.
* For example, consider `nums = [5,6,7]`. In one operation, we can replace `nums[1]` with `2` and `4` and convert `nums` to `[5,2,4,7]`.
Return _the minimum number of operations to make an array that is sorted in **non-decreasing** order_.
**Example 1:**
**Input:** nums = \[3,9,3\]
**Output:** 2
**Explanation:** Here are the steps to sort the array in non-decreasing order:
- From \[3,9,3\], replace the 9 with 3 and 6 so the array becomes \[3,3,6,3\]
- From \[3,3,6,3\], replace the 6 with 3 and 3 so the array becomes \[3,3,3,3,3\]
There are 2 steps to sort the array in non-decreasing order. Therefore, we return 2.
**Example 2:**
**Input:** nums = \[1,2,3,4,5\]
**Output:** 0
**Explanation:** The array is already in non-decreasing order. Therefore, we return 0.
**Constraints:**
* `1 <= nums.length <= 105`
* `1 <= nums[i] <= 109` | null |
[Java/Python 3] HashSet O(n) codes w/ analysis. | number-of-arithmetic-triplets | 1 | 1 | Credit to **@dms6** for the improvement from 2 pass to 1 pass.\n\n```java\n public int arithmeticTriplets(int[] nums, int diff) {\n int cnt = 0;\n Set<Integer> seen = new HashSet<>();\n for (int num : nums) {\n if (seen.contains(num - diff) && seen.contains(num - diff * 2)) {\n ++cnt;\n }\n seen.add(num);\n }\n return cnt;\n }\n```\n\n```python\n def arithmeticTriplets(self, nums: List[int], diff: int) -> int:\n seen = set()\n cnt = 0\n for num in nums:\n if num - diff in seen and num - diff * 2 in seen:\n cnt += 1\n seen.add(num)\n return cnt\n```\n\nMake the above shorter: - Credit to **@SunnyvaleCA**\n\n```python\n def arithmeticTriplets(self, nums: List[int], diff: int) -> int:\n seen = set(nums)\n return sum(num - diff in seen and num - diff * 2 in seen for num in seen)\n```\n\nOr 1 liner (time `O(n ^ 2)`:\n```python\n def arithmeticTriplets(self, nums: List[int], diff: int) -> int:\n return sum( n+diff in nums and n+diff*2 in nums for n in nums )\n```\n**Analysis:**\n\nTime & space: `O(n)`, where `n = nums.length`. | 75 | You are given a **0-indexed**, **strictly increasing** integer array `nums` and a positive integer `diff`. A triplet `(i, j, k)` is an **arithmetic triplet** if the following conditions are met:
* `i < j < k`,
* `nums[j] - nums[i] == diff`, and
* `nums[k] - nums[j] == diff`.
Return _the number of unique **arithmetic triplets**._
**Example 1:**
**Input:** nums = \[0,1,4,6,7,10\], diff = 3
**Output:** 2
**Explanation:**
(1, 2, 4) is an arithmetic triplet because both 7 - 4 == 3 and 4 - 1 == 3.
(2, 4, 5) is an arithmetic triplet because both 10 - 7 == 3 and 7 - 4 == 3.
**Example 2:**
**Input:** nums = \[4,5,6,7,8,9\], diff = 2
**Output:** 2
**Explanation:**
(0, 2, 4) is an arithmetic triplet because both 8 - 6 == 2 and 6 - 4 == 2.
(1, 3, 5) is an arithmetic triplet because both 9 - 7 == 2 and 7 - 5 == 2.
**Constraints:**
* `3 <= nums.length <= 200`
* `0 <= nums[i] <= 200`
* `1 <= diff <= 50`
* `nums` is **strictly** increasing. | null |
✅Python || Easy Approach | number-of-arithmetic-triplets | 0 | 1 | ```\nclass Solution:\n def arithmeticTriplets(self, nums: List[int], diff: int) -> int:\n \n ans = 0\n n = len(nums)\n for i in range(n):\n if nums[i] + diff in nums and nums[i] + 2 * diff in nums:\n ans += 1\n \n return ans\n``` | 37 | You are given a **0-indexed**, **strictly increasing** integer array `nums` and a positive integer `diff`. A triplet `(i, j, k)` is an **arithmetic triplet** if the following conditions are met:
* `i < j < k`,
* `nums[j] - nums[i] == diff`, and
* `nums[k] - nums[j] == diff`.
Return _the number of unique **arithmetic triplets**._
**Example 1:**
**Input:** nums = \[0,1,4,6,7,10\], diff = 3
**Output:** 2
**Explanation:**
(1, 2, 4) is an arithmetic triplet because both 7 - 4 == 3 and 4 - 1 == 3.
(2, 4, 5) is an arithmetic triplet because both 10 - 7 == 3 and 7 - 4 == 3.
**Example 2:**
**Input:** nums = \[4,5,6,7,8,9\], diff = 2
**Output:** 2
**Explanation:**
(0, 2, 4) is an arithmetic triplet because both 8 - 6 == 2 and 6 - 4 == 2.
(1, 3, 5) is an arithmetic triplet because both 9 - 7 == 2 and 7 - 5 == 2.
**Constraints:**
* `3 <= nums.length <= 200`
* `0 <= nums[i] <= 200`
* `1 <= diff <= 50`
* `nums` is **strictly** increasing. | null |
Python | Easy | O(n) Solution | number-of-arithmetic-triplets | 0 | 1 | ```\nclass Solution:\n def arithmeticTriplets(self, nums: List[int], diff: int) -> int:\n dict_ = {}\n for num in nums:\n dict_[num] = dict_.get(num,0) + 1 ##To keep the count of each num\'s occurence\n \n count = 0\n for num in nums:\n if dict_.get(num+diff) and dict_.get(num+diff*2): #Check if any number with 3 and 6 more than present in dictionary\n count += 1\n return count\n``` | 1 | You are given a **0-indexed**, **strictly increasing** integer array `nums` and a positive integer `diff`. A triplet `(i, j, k)` is an **arithmetic triplet** if the following conditions are met:
* `i < j < k`,
* `nums[j] - nums[i] == diff`, and
* `nums[k] - nums[j] == diff`.
Return _the number of unique **arithmetic triplets**._
**Example 1:**
**Input:** nums = \[0,1,4,6,7,10\], diff = 3
**Output:** 2
**Explanation:**
(1, 2, 4) is an arithmetic triplet because both 7 - 4 == 3 and 4 - 1 == 3.
(2, 4, 5) is an arithmetic triplet because both 10 - 7 == 3 and 7 - 4 == 3.
**Example 2:**
**Input:** nums = \[4,5,6,7,8,9\], diff = 2
**Output:** 2
**Explanation:**
(0, 2, 4) is an arithmetic triplet because both 8 - 6 == 2 and 6 - 4 == 2.
(1, 3, 5) is an arithmetic triplet because both 9 - 7 == 2 and 7 - 5 == 2.
**Constraints:**
* `3 <= nums.length <= 200`
* `0 <= nums[i] <= 200`
* `1 <= diff <= 50`
* `nums` is **strictly** increasing. | null |
Hashset n-diff and n-2*diff | number-of-arithmetic-triplets | 0 | 1 | # Intuition\n<!-- Describe your first thoughts on how to solve this problem. -->\n\n# Approach\n<!-- Describe your approach to solving the problem. -->\n\n# Complexity\n- Time complexity:\n<!-- Add your time complexity here, e.g. $$O(n)$$ -->\n\n- Space complexity:\n<!-- Add your space complexity here, e.g. $$O(n)$$ -->\n\n# Code\n```\nclass Solution:\n def arithmeticTriplets(self, nums: List[int], diff: int) -> int:\n seen=set()\n c=0\n for n in nums:\n seen.add(n)\n if n-diff in seen and n-2*diff in seen:\n c+=1\n return c\n \n \n``` | 2 | You are given a **0-indexed**, **strictly increasing** integer array `nums` and a positive integer `diff`. A triplet `(i, j, k)` is an **arithmetic triplet** if the following conditions are met:
* `i < j < k`,
* `nums[j] - nums[i] == diff`, and
* `nums[k] - nums[j] == diff`.
Return _the number of unique **arithmetic triplets**._
**Example 1:**
**Input:** nums = \[0,1,4,6,7,10\], diff = 3
**Output:** 2
**Explanation:**
(1, 2, 4) is an arithmetic triplet because both 7 - 4 == 3 and 4 - 1 == 3.
(2, 4, 5) is an arithmetic triplet because both 10 - 7 == 3 and 7 - 4 == 3.
**Example 2:**
**Input:** nums = \[4,5,6,7,8,9\], diff = 2
**Output:** 2
**Explanation:**
(0, 2, 4) is an arithmetic triplet because both 8 - 6 == 2 and 6 - 4 == 2.
(1, 3, 5) is an arithmetic triplet because both 9 - 7 == 2 and 7 - 5 == 2.
**Constraints:**
* `3 <= nums.length <= 200`
* `0 <= nums[i] <= 200`
* `1 <= diff <= 50`
* `nums` is **strictly** increasing. | null |
FASTEST, EASY PYTHON SOLUTION | reachable-nodes-with-restrictions | 0 | 1 | # Intuition\n<!-- Describe your first thoughts on how to solve this problem. -->\n\n# Approach\n<!-- Describe your approach to solving the problem. -->\n\n# Complexity\n- Time complexity: $$O(N+2E)$$\n<!-- Add your time complexity here, e.g. $$O(n)$$ -->\n\n- Space complexity: $$O(N*E)$$\n<!-- Add your space complexity here, e.g. $$O(n)$$ -->\n\n# Code\n```\nclass Solution:\n def reachableNodes(self, n: int, edges: List[List[int]], restricted: List[int]) -> int:\n st=[0]\n adj=[[] for _ in range(n)]\n for i,j in edges:\n adj[i].append(j)\n adj[j].append(i)\n visited=[0]*n\n visited[0]=1\n for i in restricted:\n visited[i]=-1\n ct=0\n while st:\n x=st.pop(0)\n ct+=1\n for i in adj[x]:\n if visited[i]==0:\n st.append(i)\n visited[i]=1\n return ct\n\n``` | 2 | There is an undirected tree with `n` nodes labeled from `0` to `n - 1` and `n - 1` edges.
You are given a 2D integer array `edges` of length `n - 1` where `edges[i] = [ai, bi]` indicates that there is an edge between nodes `ai` and `bi` in the tree. You are also given an integer array `restricted` which represents **restricted** nodes.
Return _the **maximum** number of nodes you can reach from node_ `0` _without visiting a restricted node._
Note that node `0` will **not** be a restricted node.
**Example 1:**
**Input:** n = 7, edges = \[\[0,1\],\[1,2\],\[3,1\],\[4,0\],\[0,5\],\[5,6\]\], restricted = \[4,5\]
**Output:** 4
**Explanation:** The diagram above shows the tree.
We have that \[0,1,2,3\] are the only nodes that can be reached from node 0 without visiting a restricted node.
**Example 2:**
**Input:** n = 7, edges = \[\[0,1\],\[0,2\],\[0,5\],\[0,4\],\[3,2\],\[6,5\]\], restricted = \[4,2,1\]
**Output:** 3
**Explanation:** The diagram above shows the tree.
We have that \[0,5,6\] are the only nodes that can be reached from node 0 without visiting a restricted node.
**Constraints:**
* `2 <= n <= 105`
* `edges.length == n - 1`
* `edges[i].length == 2`
* `0 <= ai, bi < n`
* `ai != bi`
* `edges` represents a valid tree.
* `1 <= restricted.length < n`
* `1 <= restricted[i] < n`
* All the values of `restricted` are **unique**. | null |
Basic DFS with Restrictions!!😸 | reachable-nodes-with-restrictions | 0 | 1 | # Intuition\n<!-- Describe your first thoughts on how to solve this problem. -->\n\n# Approach\n<!-- Describe your approach to solving the problem. -->\n\n# Complexity\n- Time complexity:\n<!-- Add your time complexity here, e.g. $$O(n)$$ -->\n\n- Space complexity:\n<!-- Add your space complexity here, e.g. $$O(n)$$ -->\n\n# Code\n```\nfrom collections import defaultdict\nclass Solution:\n def reachableNodes(self, n: int, edges: List[List[int]], restricted: List[int]) -> int:\n graph = defaultdict(list)\n for u, v in edges:\n graph[u].append(v)\n graph[v].append(u)\n visited = set() # set to keep track of visited nodes\n restricted = set(restricted) # convert it to set so that retrieval is faster in comparison to list .. else it may fail the last test case where n = 1000\n\n ans = 1 # ans = 1, as the starting node is always reachable\n #simple dfs traversal code \n def dfs(start):\n nonlocal ans # Allow modification of the \'ans\' variable in the outer scope\n visited.add(start) \n for neighbor in graph[start]:\n # Check if the neighbor is not visited and not restricted\n if neighbor not in visited and neighbor not in restricted:\n ans += 1 \n dfs(neighbor) \n dfs(0)\n return ans\n\n``` | 1 | There is an undirected tree with `n` nodes labeled from `0` to `n - 1` and `n - 1` edges.
You are given a 2D integer array `edges` of length `n - 1` where `edges[i] = [ai, bi]` indicates that there is an edge between nodes `ai` and `bi` in the tree. You are also given an integer array `restricted` which represents **restricted** nodes.
Return _the **maximum** number of nodes you can reach from node_ `0` _without visiting a restricted node._
Note that node `0` will **not** be a restricted node.
**Example 1:**
**Input:** n = 7, edges = \[\[0,1\],\[1,2\],\[3,1\],\[4,0\],\[0,5\],\[5,6\]\], restricted = \[4,5\]
**Output:** 4
**Explanation:** The diagram above shows the tree.
We have that \[0,1,2,3\] are the only nodes that can be reached from node 0 without visiting a restricted node.
**Example 2:**
**Input:** n = 7, edges = \[\[0,1\],\[0,2\],\[0,5\],\[0,4\],\[3,2\],\[6,5\]\], restricted = \[4,2,1\]
**Output:** 3
**Explanation:** The diagram above shows the tree.
We have that \[0,5,6\] are the only nodes that can be reached from node 0 without visiting a restricted node.
**Constraints:**
* `2 <= n <= 105`
* `edges.length == n - 1`
* `edges[i].length == 2`
* `0 <= ai, bi < n`
* `ai != bi`
* `edges` represents a valid tree.
* `1 <= restricted.length < n`
* `1 <= restricted[i] < n`
* All the values of `restricted` are **unique**. | null |
python -bfs solution | reachable-nodes-with-restrictions | 0 | 1 | # Intuition\n<!-- Describe your first thoughts on how to solve this problem. -->\n\n# Approach\n<!-- Describe your approach to solving the problem. -->\n\n# Complexity\n- Time complexity:\n<!-- Add your time complexity here, e.g. $$O(n)$$ -->\n\n- Space complexity:\n<!-- Add your space complexity here, e.g. $$O(n)$$ -->\n\n# Code\n```\nfrom collections import defaultdict\nclass Solution:\n def reachableNodes(self, n: int, edges: List[List[int]], restricted: List[int]) -> int:\n\n #building a undirected graph\n graph=collections.defaultdict(list)\n def buildgraph(edge):\n for x in edge:\n graph[x[0]].append(x[1])\n graph[x[1]].append(x[0])\n restricted=set(restricted)\n buildgraph(edges)\n\n #breadth first search of a graph\n count=0\n q=[]\n q.append(0)\n visited=[True]*n\n while q:\n cur=q[0]\n q.remove(cur)\n #if not visited,taken into account\n if visited[cur]:\n count+=1\n visited[cur]=False\n for x in graph[cur]:\n if visited[x] and x not in restricted :\n q.append(x)\n return count\n``` | 1 | There is an undirected tree with `n` nodes labeled from `0` to `n - 1` and `n - 1` edges.
You are given a 2D integer array `edges` of length `n - 1` where `edges[i] = [ai, bi]` indicates that there is an edge between nodes `ai` and `bi` in the tree. You are also given an integer array `restricted` which represents **restricted** nodes.
Return _the **maximum** number of nodes you can reach from node_ `0` _without visiting a restricted node._
Note that node `0` will **not** be a restricted node.
**Example 1:**
**Input:** n = 7, edges = \[\[0,1\],\[1,2\],\[3,1\],\[4,0\],\[0,5\],\[5,6\]\], restricted = \[4,5\]
**Output:** 4
**Explanation:** The diagram above shows the tree.
We have that \[0,1,2,3\] are the only nodes that can be reached from node 0 without visiting a restricted node.
**Example 2:**
**Input:** n = 7, edges = \[\[0,1\],\[0,2\],\[0,5\],\[0,4\],\[3,2\],\[6,5\]\], restricted = \[4,2,1\]
**Output:** 3
**Explanation:** The diagram above shows the tree.
We have that \[0,5,6\] are the only nodes that can be reached from node 0 without visiting a restricted node.
**Constraints:**
* `2 <= n <= 105`
* `edges.length == n - 1`
* `edges[i].length == 2`
* `0 <= ai, bi < n`
* `ai != bi`
* `edges` represents a valid tree.
* `1 <= restricted.length < n`
* `1 <= restricted[i] < n`
* All the values of `restricted` are **unique**. | null |
Simple python BFS solution, beats 86.82% and memory beats 91.56% | reachable-nodes-with-restrictions | 0 | 1 | # Intuition\nBFS\n\n# Approach\n<!-- Describe your approach to solving the problem. -->\n\n# Complexity\n- Time complexity:\n$$O(n)$$\n- Space complexity:\n$$O(n)$$\n\n# Code\n```\nclass Solution:\n def reachableNodes(self, n: int, edges: List[List[int]], restricted: List[int]) -> int:\n adj_list = defaultdict(list)\n for x,y in edges:\n adj_list[x].append(y)\n adj_list[y].append(x)\n \n que = deque()\n que.append(0)\n result = 0\n visited = set()\n for node in restricted:\n visited.add(node)\n\n while que:\n cur = que.popleft()\n if cur in visited:\n continue \n visited.add(cur)\n result += 1\n for node in adj_list[cur]:\n que.append(node)\n \n return result\n\n\n\n\n``` | 1 | There is an undirected tree with `n` nodes labeled from `0` to `n - 1` and `n - 1` edges.
You are given a 2D integer array `edges` of length `n - 1` where `edges[i] = [ai, bi]` indicates that there is an edge between nodes `ai` and `bi` in the tree. You are also given an integer array `restricted` which represents **restricted** nodes.
Return _the **maximum** number of nodes you can reach from node_ `0` _without visiting a restricted node._
Note that node `0` will **not** be a restricted node.
**Example 1:**
**Input:** n = 7, edges = \[\[0,1\],\[1,2\],\[3,1\],\[4,0\],\[0,5\],\[5,6\]\], restricted = \[4,5\]
**Output:** 4
**Explanation:** The diagram above shows the tree.
We have that \[0,1,2,3\] are the only nodes that can be reached from node 0 without visiting a restricted node.
**Example 2:**
**Input:** n = 7, edges = \[\[0,1\],\[0,2\],\[0,5\],\[0,4\],\[3,2\],\[6,5\]\], restricted = \[4,2,1\]
**Output:** 3
**Explanation:** The diagram above shows the tree.
We have that \[0,5,6\] are the only nodes that can be reached from node 0 without visiting a restricted node.
**Constraints:**
* `2 <= n <= 105`
* `edges.length == n - 1`
* `edges[i].length == 2`
* `0 <= ai, bi < n`
* `ai != bi`
* `edges` represents a valid tree.
* `1 <= restricted.length < n`
* `1 <= restricted[i] < n`
* All the values of `restricted` are **unique**. | null |
[Java/Python 3] BFS and DFS codes w/ brief explanation and analysis. | reachable-nodes-with-restrictions | 1 | 1 | 1. Use a `HashSet` to hold the restricted nodes and then visited nodes; \n2. Do BFS/DFS to explore the tree, and upon completing exploration the `HashSet` contains all reachable and all restricted nodes. \n3. We can use the size of the restricted to deduct the size of `HashSet` to get the number of the reachable ones.\n\n**BFS**\n\n```java\n public int reachableNodes(int n, int[][] edges, int[] restricted) {\n Set<Integer> seen = new HashSet<>();\n for (int r : restricted) {\n seen.add(r);\n }\n Map<Integer, Set<Integer>> g = new HashMap<>();\n for (int[] e : edges) {\n g.computeIfAbsent(e[0], s -> new HashSet<>()).add(e[1]);\n g.computeIfAbsent(e[1], s -> new HashSet<>()).add(e[0]);\n }\n Queue<Integer> q = new LinkedList<>();\n q.offer(0);\n while (!q.isEmpty()) {\n int node = q.poll();\n seen.add(node);\n for (int kid : g.getOrDefault(node, Collections.emptySet())) {\n if (seen.add(kid)) {\n q.offer(kid);\n }\n }\n }\n return seen.size() - restricted.length;\n }\n```\n**@hj96998** provides the following comments:\n\nSome explanation of the BFS python version of codes:\n\nPoint #1 : defaultdict\na sub-class of the dictionary class that returns a dictionary-like object.\nOne smart thing here is the type is set so that the values have type set. Since we only need to make a map of how nodes connect.\nPoint #2: deque\na double-ended queue in which elements can be both inserted and deleted from either the left or the right end of the queue.\n\nFollowed by BFS algorithm and return length of visited nodes without restricted nodes\n\n\n```python\n def reachableNodes(self, n: int, edges: List[List[int]], restricted: List[int]) -> int:\n seen = set(restricted)\n g = defaultdict(set)\n for a, b in edges:\n g[a].add(b)\n g[b].add(a)\n dq = deque([0])\n while dq:\n node = dq.popleft()\n seen.add(node)\n for kid in g[node]:\n if kid not in seen:\n seen.add(kid)\n dq.append(kid)\n return len(seen) - len(restricted) \n```\n\n----\n\n**DFS**\n\n```java\n public int reachableNodes(int n, int[][] edges, int[] restricted) {\n Map<Integer, Set<Integer>> g = new HashMap<>();\n for (int[] e : edges) {\n g.computeIfAbsent(e[0], s -> new HashSet<>()).add(e[1]);\n g.computeIfAbsent(e[1], s -> new HashSet<>()).add(e[0]);\n }\n Set<Integer> seen = new HashSet<>();\n for (int r : restricted) {\n seen.add(r);\n }\n dfs(g, seen, 0);\n return seen.size() - restricted.length;\n }\n private void dfs(Map<Integer, Set<Integer>> g, Set<Integer> seen, int node) {\n if (seen.add(node)) {\n for (int kid : g.getOrDefault(node, Collections.emptySet())) {\n dfs(g, seen, kid);\n }\n }\n }\n```\n```python\n def reachableNodes(self, n: int, edges: List[List[int]], restricted: List[int]) -> int:\n \n def dfs(node: int) -> None:\n if node not in seen:\n seen.add(node)\n for kid in g[node]:\n dfs(kid)\n \n seen = set(restricted)\n g = defaultdict(set)\n for a, b in edges:\n g[a].add(b)\n g[b].add(a) \n dfs(0)\n return len(seen) - len(restricted) \n```\n\n**Analysis for both DFS and BFS codes:**\n\nTime & space: `O(V + E)`, where `V = # of nodes, E = # of edges`.\n | 17 | There is an undirected tree with `n` nodes labeled from `0` to `n - 1` and `n - 1` edges.
You are given a 2D integer array `edges` of length `n - 1` where `edges[i] = [ai, bi]` indicates that there is an edge between nodes `ai` and `bi` in the tree. You are also given an integer array `restricted` which represents **restricted** nodes.
Return _the **maximum** number of nodes you can reach from node_ `0` _without visiting a restricted node._
Note that node `0` will **not** be a restricted node.
**Example 1:**
**Input:** n = 7, edges = \[\[0,1\],\[1,2\],\[3,1\],\[4,0\],\[0,5\],\[5,6\]\], restricted = \[4,5\]
**Output:** 4
**Explanation:** The diagram above shows the tree.
We have that \[0,1,2,3\] are the only nodes that can be reached from node 0 without visiting a restricted node.
**Example 2:**
**Input:** n = 7, edges = \[\[0,1\],\[0,2\],\[0,5\],\[0,4\],\[3,2\],\[6,5\]\], restricted = \[4,2,1\]
**Output:** 3
**Explanation:** The diagram above shows the tree.
We have that \[0,5,6\] are the only nodes that can be reached from node 0 without visiting a restricted node.
**Constraints:**
* `2 <= n <= 105`
* `edges.length == n - 1`
* `edges[i].length == 2`
* `0 <= ai, bi < n`
* `ai != bi`
* `edges` represents a valid tree.
* `1 <= restricted.length < n`
* `1 <= restricted[i] < n`
* All the values of `restricted` are **unique**. | null |
Python begineer friendly solution || Easy to understand || Recursive Solution | reachable-nodes-with-restrictions | 0 | 1 | ```\nclass Solution:\n def reachableNodes(self, n: int, edges: List[List[int]], restricted: List[int]) -> int:\n\t\t# stores final ans\n self.ans = 0\n\t\t\n\t\t# adjacency list for graph\n self.adj = [[] for i in range(n)]\n for pair in edges:\n self.adj[pair[0]].append(pair[1])\n self.adj[pair[1]].append(pair[0])\n\t\t\t\n\t\t# start visiting nodes from 0, pass the restriction as set for fast lookup, and a set for track of visited nodes\n self.find(0,set(restricted),set())\n return self.ans\n \n def find(self,idx,rest,visited):\n\t\t# if node is visited or is in restriction return\n if idx in visited or idx in rest:\n return\n\t\t\t\n\t\t# mark current node as visited and increment the counter\n visited.add(idx)\n self.ans += 1\n\t\t\n\t\t# traverse the neighbours of the current node recursively\n for elm in self.adj[idx]:\n self.find(elm,rest,visited)\n``` | 1 | There is an undirected tree with `n` nodes labeled from `0` to `n - 1` and `n - 1` edges.
You are given a 2D integer array `edges` of length `n - 1` where `edges[i] = [ai, bi]` indicates that there is an edge between nodes `ai` and `bi` in the tree. You are also given an integer array `restricted` which represents **restricted** nodes.
Return _the **maximum** number of nodes you can reach from node_ `0` _without visiting a restricted node._
Note that node `0` will **not** be a restricted node.
**Example 1:**
**Input:** n = 7, edges = \[\[0,1\],\[1,2\],\[3,1\],\[4,0\],\[0,5\],\[5,6\]\], restricted = \[4,5\]
**Output:** 4
**Explanation:** The diagram above shows the tree.
We have that \[0,1,2,3\] are the only nodes that can be reached from node 0 without visiting a restricted node.
**Example 2:**
**Input:** n = 7, edges = \[\[0,1\],\[0,2\],\[0,5\],\[0,4\],\[3,2\],\[6,5\]\], restricted = \[4,2,1\]
**Output:** 3
**Explanation:** The diagram above shows the tree.
We have that \[0,5,6\] are the only nodes that can be reached from node 0 without visiting a restricted node.
**Constraints:**
* `2 <= n <= 105`
* `edges.length == n - 1`
* `edges[i].length == 2`
* `0 <= ai, bi < n`
* `ai != bi`
* `edges` represents a valid tree.
* `1 <= restricted.length < n`
* `1 <= restricted[i] < n`
* All the values of `restricted` are **unique**. | null |
Python3 BFS and DFS | reachable-nodes-with-restrictions | 0 | 1 | BFS\n\n```python\n def reachableNodes(self, n: int, edges: List[List[int]], restricted: List[int]) -> int:\n\t\tgraph = defaultdict(list)\n visited = [False]*n\n queue = deque([0])\n res = 0\n \n for u in restricted:\n visited[u] = True\n \n for u,v in edges:\n graph[u].append(v)\n graph[v].append(u)\n \n while queue:\n res += len(queue)\n for _ in range(len(queue)):\n u = queue.popleft()\n visited[u] = True\n queue.extend([v for v in graph[u] if not visited[v]])\n \n return res\n```\n\nDFS\n```python\n def reachableNodes(self, n: int, edges: List[List[int]], restricted: List[int]) -> int:\n\t\tgraph = defaultdict(list)\n visited = [False]*n\n \n for u in restricted:\n visited[u] = True\n \n for u,v in edges:\n graph[u].append(v)\n graph[v].append(u)\n \n def dfs(u=0):\n if visited[u]: return 0\n visited[u] = True\n return sum((dfs(v) for v in graph[u]), 1)\n \n return dfs()\n``` | 2 | There is an undirected tree with `n` nodes labeled from `0` to `n - 1` and `n - 1` edges.
You are given a 2D integer array `edges` of length `n - 1` where `edges[i] = [ai, bi]` indicates that there is an edge between nodes `ai` and `bi` in the tree. You are also given an integer array `restricted` which represents **restricted** nodes.
Return _the **maximum** number of nodes you can reach from node_ `0` _without visiting a restricted node._
Note that node `0` will **not** be a restricted node.
**Example 1:**
**Input:** n = 7, edges = \[\[0,1\],\[1,2\],\[3,1\],\[4,0\],\[0,5\],\[5,6\]\], restricted = \[4,5\]
**Output:** 4
**Explanation:** The diagram above shows the tree.
We have that \[0,1,2,3\] are the only nodes that can be reached from node 0 without visiting a restricted node.
**Example 2:**
**Input:** n = 7, edges = \[\[0,1\],\[0,2\],\[0,5\],\[0,4\],\[3,2\],\[6,5\]\], restricted = \[4,2,1\]
**Output:** 3
**Explanation:** The diagram above shows the tree.
We have that \[0,5,6\] are the only nodes that can be reached from node 0 without visiting a restricted node.
**Constraints:**
* `2 <= n <= 105`
* `edges.length == n - 1`
* `edges[i].length == 2`
* `0 <= ai, bi < n`
* `ai != bi`
* `edges` represents a valid tree.
* `1 <= restricted.length < n`
* `1 <= restricted[i] < n`
* All the values of `restricted` are **unique**. | null |
🦀🐍🐹 Rust + Go + Python & DP | check-if-there-is-a-valid-partition-for-the-array | 0 | 1 | **Intuition** \uD83E\uDDE0\n\nHey folks! Ever tried to slice an array into special subarrays, where the magic rules are:\n\n1. Two identical numbers sitting side by side, chilling.\n2. Three identical numbers just hanging out together.\n3. Or, three numbers increasing by one - like they\'re climbing stairs!\n\nOur task today? Simple (or is it? \uD83D\uDE09). We need to see if a given array can be split into these magical subarrays. And guess what? I\'ve got not one, not two, but three programming languages ready to tackle this challenge. \uD83D\uDE80 Let\'s jump in!\n\n**Approach** \uD83E\uDDD0\n\nSo, how do we know if a subarray is magical? Dynamic Programming (DP) to the rescue! We\'re going to use a window approach to keep track of the validity of our partitions. At every step, we\'ll just peek at the current number and at most, the two preceding ones. That\'s all we need!\n\n1. If the current number is the same as the previous one and the partition up to the previous number was valid, then... magic!\n2. If the current number and the two before it are identical and the partition two steps back was valid, then... even more magic!\n3. If the numbers are like climbing stairs and the partition two steps back was valid, then... you guessed it, magic!\n\nWith every step, our window slides, capturing the magic of the array.\n\n**Complexity** \u23F3\n\n- **Time complexity:** \\(O(n)\\) - Just a single loop through the numbers.\n- **Space complexity:** \\(O(1)\\) - We only need a fixed-size window.\n\n**Code** \uD83D\uDDA5\uFE0F\n\n\uD83E\uDD80 **Rust**:\n\n```\nimpl Solution {\n pub fn valid_partition(nums: Vec<i32>) -> bool {\n let n = nums.len();\n if n == 1 { return false; }\n\n let mut dp = vec![true, false, if n > 1 { nums[0] == nums[1] } else { false }];\n\n for i in 2..n {\n let current_dp = (nums[i] == nums[i-1] && dp[1]) || \n (nums[i] == nums[i-1] && nums[i] == nums[i-2] && dp[0]) ||\n (nums[i] - nums[i-1] == 1 && nums[i-1] - nums[i-2] == 1 && dp[0]);\n dp[0] = dp[1];\n dp[1] = dp[2];\n dp[2] = current_dp;\n }\n dp[2]\n }\n}\n```\n\n\uD83D\uDC0D **Python**:\n\n```\nclass Solution:\n def validPartition(self, nums: List[int]) -> bool:\n n = len(nums) \n if n == 1: \n return False \n dp = [True, False, nums[0] == nums[1] if n > 1 else False] \n for i in range(2, n): \n current_dp = (nums[i] == nums[i-1] and dp[1]) or \\\n (nums[i] == nums[i-1] == nums[i-2] and dp[0]) or \\\n (nums[i] - nums[i-1] == 1 and nums[i-1] - nums[i-2] == 1 and dp[0])\n dp[0], dp[1], dp[2] = dp[1], dp[2], current_dp \n return dp[2]\n```\n\n\uD83D\uDE80 **Go**:\n\n```\nfunc validPartition(nums []int) bool {\n n := len(nums)\n if n == 1 { return false }\n\n dp := []bool{true, false, false}\n if n > 1 {\n dp[2] = nums[0] == nums[1]\n }\n\n for i := 2; i < n; i++ {\n current_dp := (nums[i] == nums[i-1] && dp[1]) || \n (nums[i] == nums[i-1] && nums[i] == nums[i-2] && dp[0]) ||\n (nums[i] - nums[i-1] == 1 && nums[i-1] - nums[i-2] == 1 && dp[0])\n dp[0], dp[1], dp[2] = dp[1], dp[2], current_dp\n }\n return dp[2]\n}\n```\n\nHang tight, put on your coding hats, and let\'s make some magic happen! And if you\'re feeling the vibes, don\'t forget to like, share, and subscribe for more coding adventures! \u270C\uFE0F\uD83C\uDF0C\uD83C\uDFA9 | 4 | You are given a **0-indexed** integer array `nums`. You have to partition the array into one or more **contiguous** subarrays.
We call a partition of the array **valid** if each of the obtained subarrays satisfies **one** of the following conditions:
1. The subarray consists of **exactly** `2` equal elements. For example, the subarray `[2,2]` is good.
2. The subarray consists of **exactly** `3` equal elements. For example, the subarray `[4,4,4]` is good.
3. The subarray consists of **exactly** `3` consecutive increasing elements, that is, the difference between adjacent elements is `1`. For example, the subarray `[3,4,5]` is good, but the subarray `[1,3,5]` is not.
Return `true` _if the array has **at least** one valid partition_. Otherwise, return `false`.
**Example 1:**
**Input:** nums = \[4,4,4,5,6\]
**Output:** true
**Explanation:** The array can be partitioned into the subarrays \[4,4\] and \[4,5,6\].
This partition is valid, so we return true.
**Example 2:**
**Input:** nums = \[1,1,1,2\]
**Output:** false
**Explanation:** There is no valid partition for this array.
**Constraints:**
* `2 <= nums.length <= 105`
* `1 <= nums[i] <= 106` | How can we use precalculation to efficiently calculate the average difference at an index? Create a prefix and/or suffix sum array. |
Subsets and Splits
No saved queries yet
Save your SQL queries to embed, download, and access them later. Queries will appear here once saved.