Add paper abstract
#1
by
nielsr
HF Staff
- opened
README.md
CHANGED
@@ -1,19 +1,19 @@
|
|
1 |
---
|
2 |
-
license: mit
|
3 |
-
pipeline_tag: image-text-to-text
|
4 |
-
library_name: transformers
|
5 |
base_model:
|
6 |
-
|
7 |
-
|
8 |
-
base_model_relation: finetune
|
9 |
datasets:
|
10 |
-
|
11 |
-
|
12 |
language:
|
13 |
-
|
|
|
|
|
|
|
14 |
tags:
|
15 |
-
|
16 |
-
|
|
|
17 |
---
|
18 |
|
19 |
# VisualPRM-8B
|
@@ -36,7 +36,6 @@ We introduce VisualPRM, an advanced multimodal Process Reward Model (PRM) with 8
|
|
36 |
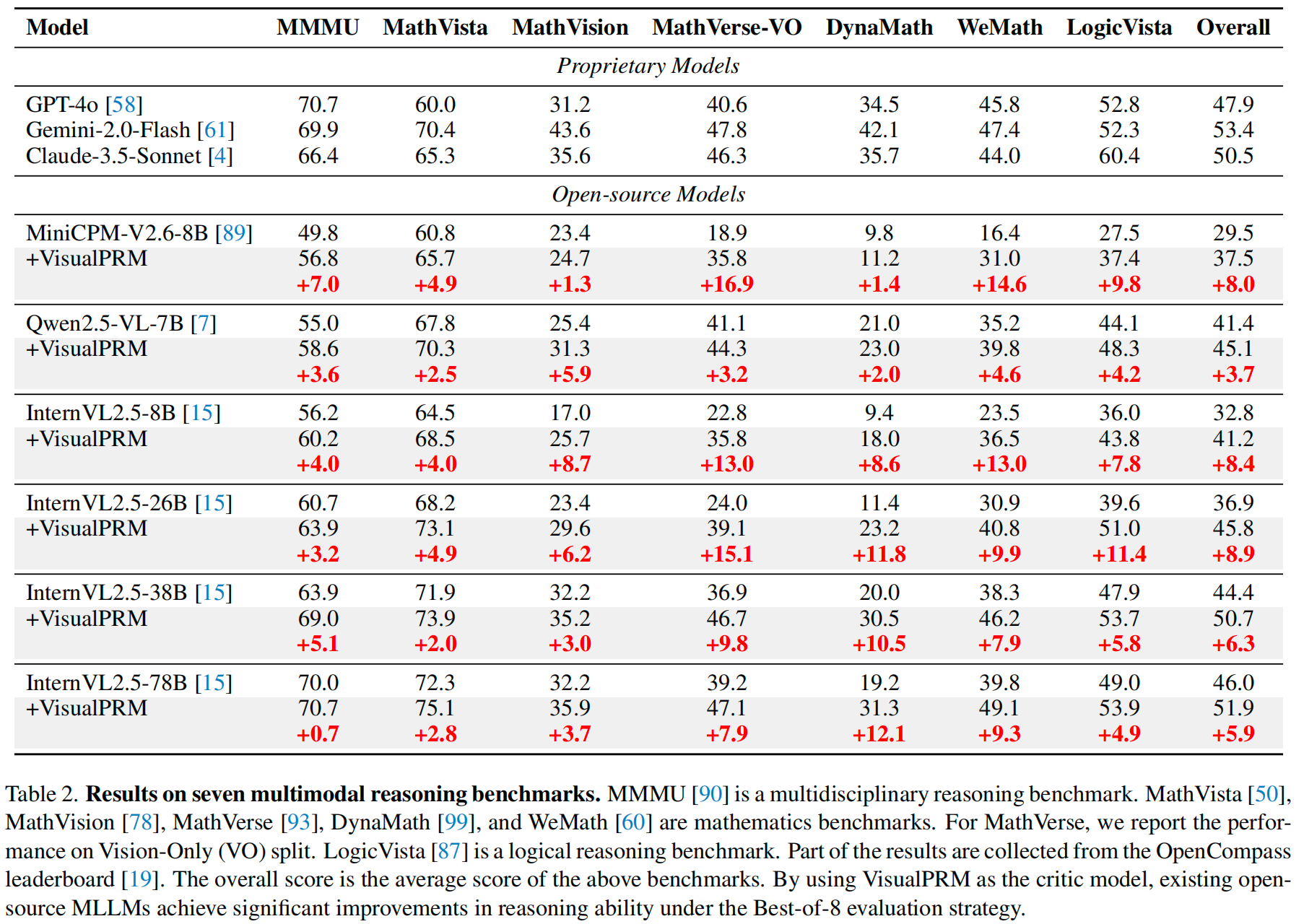
|
37 |

|
38 |
|
39 |
-
|
40 |
## Inference with Transformers
|
41 |
|
42 |
```python
|
@@ -205,3 +204,7 @@ If you find this project useful in your research, please consider citing:
|
|
205 |
year={2024}
|
206 |
}
|
207 |
```
|
|
|
|
|
|
|
|
|
|
1 |
---
|
|
|
|
|
|
|
2 |
base_model:
|
3 |
+
- OpenGVLab/InternVL2_5-8B
|
4 |
+
- OpenGVLab/InternVL2_5-8B-MPO
|
|
|
5 |
datasets:
|
6 |
+
- OpenGVLab/MMPR-v1.1
|
7 |
+
- OpenGVLab/VisualPRM400K
|
8 |
language:
|
9 |
+
- multilingual
|
10 |
+
library_name: transformers
|
11 |
+
license: mit
|
12 |
+
pipeline_tag: image-text-to-text
|
13 |
tags:
|
14 |
+
- internvl
|
15 |
+
- custom_code
|
16 |
+
base_model_relation: finetune
|
17 |
---
|
18 |
|
19 |
# VisualPRM-8B
|
|
|
36 |
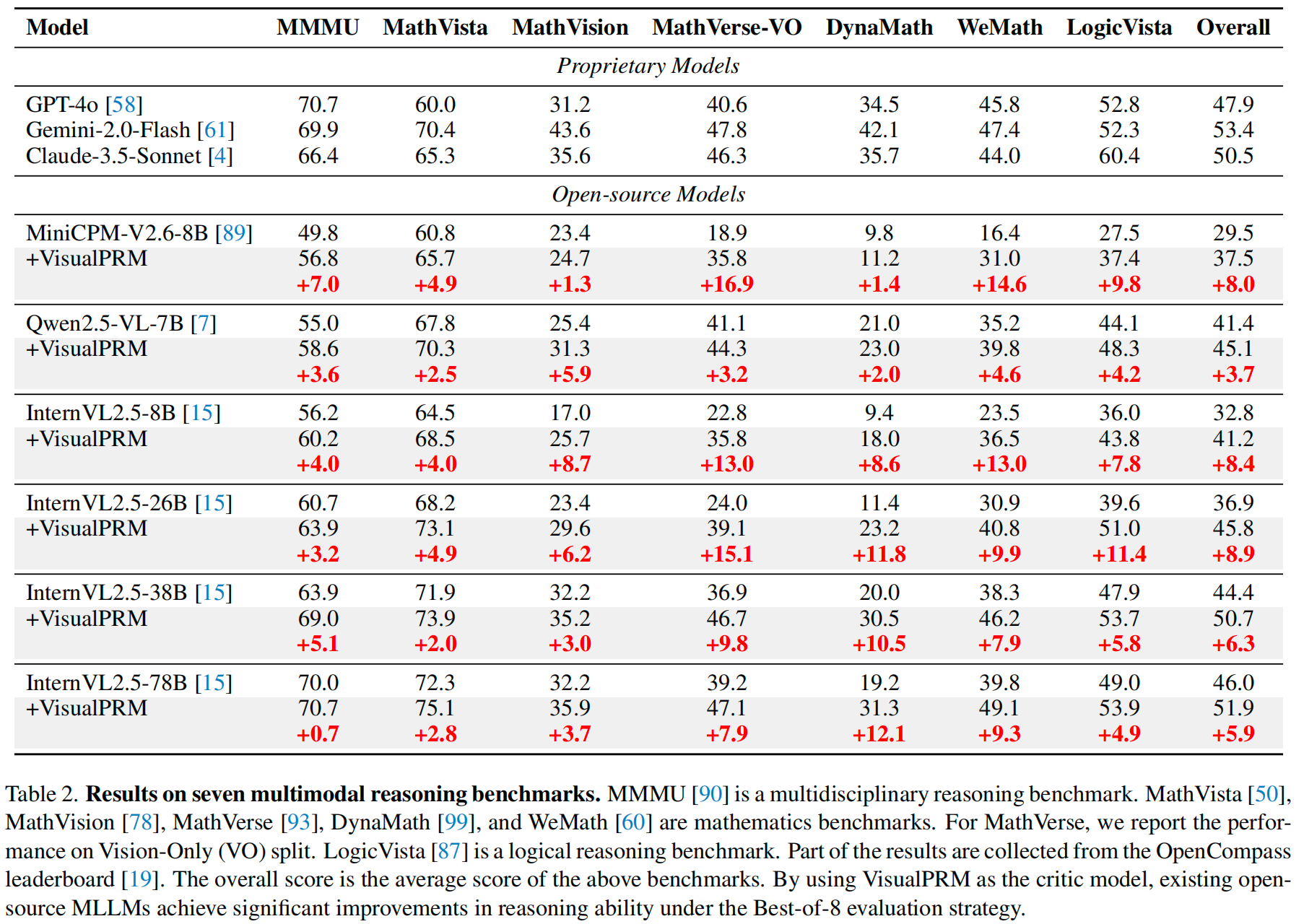
|
37 |

|
38 |
|
|
|
39 |
## Inference with Transformers
|
40 |
|
41 |
```python
|
|
|
204 |
year={2024}
|
205 |
}
|
206 |
```
|
207 |
+
|
208 |
+
## Abstract
|
209 |
+
|
210 |
+
We introduce VisualPRM, an advanced multimodal Process Reward Model (PRM) with 8B parameters, which improves the reasoning abilities of existing Multimodal Large Language Models (MLLMs) across different model scales and families with Best-of-N (BoN) evaluation strategies. **Specifically, our model improves the reasoning performance of three types of MLLMs and four different model scales. Even when applied to the highly capable InternVL2.5-78B, it achieves a 5.9-point improvement across seven multimodal reasoning benchmarks.** Experimental results show that our model exhibits superior performance compared to Outcome Reward Models and Self-Consistency during BoN evaluation. To facilitate the training of multimodal PRMs, we construct a multimodal process supervision dataset VisualPRM400K using an automated data pipeline. For the evaluation of multimodal PRMs, we propose VisualProcessBench, a benchmark with human-annotated step-wise correctness labels, to measure the abilities of PRMs to detect erroneous steps in multimodal reasoning tasks. We hope that our work can inspire more future research and contribute to the development of MLLMs.
|