File size: 5,321 Bytes
c487467 5c7782a c487467 5c7782a c487467 5c7782a f02e576 5c7782a |
1 2 3 4 5 6 7 8 9 10 11 12 13 14 15 16 17 18 19 20 21 22 23 24 25 26 27 28 29 30 31 32 33 34 35 36 37 38 39 40 41 42 43 44 45 46 47 48 49 50 51 52 53 54 55 56 57 58 59 60 61 62 63 64 65 66 67 68 69 70 71 72 73 74 75 76 77 78 79 80 81 82 83 84 85 86 87 88 89 90 91 92 93 94 95 96 97 98 99 100 101 102 103 104 105 106 107 108 109 110 111 112 113 114 115 116 117 118 119 120 121 122 123 124 125 126 127 128 129 130 131 132 133 134 135 136 137 138 139 140 141 142 143 144 145 146 147 148 149 150 151 152 153 154 155 156 157 158 159 160 161 162 163 164 165 166 167 168 169 170 |
---
pipeline_tag: text-generation
inference: true
widget:
- text: '<commit_before>def has_close_elements(numbers: List[float], threshold: float) -> bool:\n for idx, elem in enumerate(numbers):\n for idx2, elem2 in enumerate(numbers):\n if idx != idx2:\n distance = elem - elem2\n if distance < threshold:\n return True\n\n return False<commit_message>Fix bugs in has_close_elements.<commit_after>'
example_title: Fix has_close_elements
group: Python
license: bigcode-openrail-m
datasets:
- bigcode/commits-8129-v2
metrics:
- code_eval
library_name: transformers
tags:
- code
model-index:
- name: SantaCoderPack
results:
- task:
type: text-generation
dataset:
type: bigcode/humanevalpack
name: HumanEvalFix Python
metrics:
- name: pass@1
type: pass@1
value: 3.2
verified: false
- task:
type: text-generation
dataset:
type: bigcode/humanevalpack
name: HumanEvalFix JavaScript
metrics:
- name: pass@1
type: pass@1
value: 4.9
verified: false
- task:
type: text-generation
dataset:
type: bigcode/humanevalpack
name: HumanEvalFix Java
metrics:
- name: pass@1
type: pass@1
value: 1.8
verified: false
- task:
type: text-generation
dataset:
type: bigcode/humanevalpack
name: HumanEvalFix Go
metrics:
- name: pass@1
type: pass@1
value: 3.6
verified: false
- task:
type: text-generation
dataset:
type: bigcode/humanevalpack
name: HumanEvalFix C++
metrics:
- name: pass@1
type: pass@1
value: 4.2
verified: false
- task:
type: text-generation
dataset:
type: bigcode/humanevalpack
name: HumanEvalFix Rust
metrics:
- name: pass@1
type: pass@1
value: 1.7
verified: false
- task:
type: text-generation
dataset:
type: bigcode/humanevalpack
name: HumanEvalFix Average
metrics:
- name: pass@1
type: pass@1
value: 3.3
verified: false
---
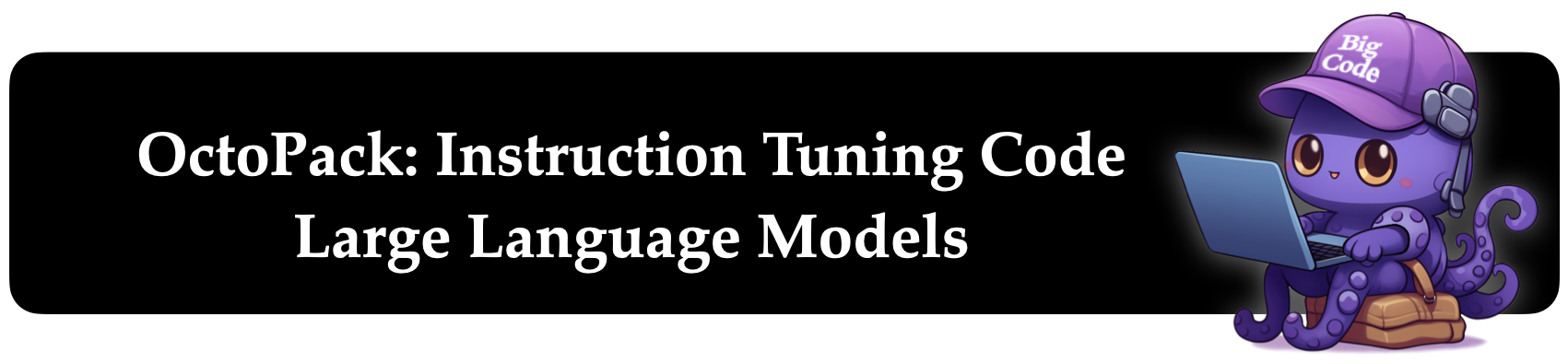
# Table of Contents
1. [Model Summary](#model-summary)
2. [Use](#use)
3. [Training](#training)
4. [Citation](#citation)
# Model Summary
SantaCoderPack is an pre-trained model with the same architecture of SantaCoder on
<th><a href=https://huggingface.co/datasets/bigcode/commitpack>CommitPack</a> using this format: <commit_before>code_before<commit_msg>message<commit_after>
- **Repository:** [bigcode/octopack](https://github.com/bigcode-project/octopack)
- **Paper:** [TODO]()
- **Languages:** Python, JavaScript, Java, C++, Go, Rust
- **SantaCoderPack:**
<table>
<tr>
<th>Data</t>
<th><a href=https://huggingface.co/datasets/bigcode/commitpack>CommitPack</a></th>
<td>4TB of GitHub commits across 350 programming languages</td>
</tr>
<tr>
<th>Model</t>
<th><a href=https://huggingface.co/bigcode/octocoder>SantaCoderPack</a></th>
<td>SantaCoderPack (1.1B parameters) pre-trained on CommitPack</td>
</tr>
<tr>
<th>Evaluation </t>
<th><a href=https://huggingface.co/datasets/bigcode/humanevalpack>HumanEvalPack/HumanEvalFix</a></th>
<td>Extension of OpenAI's HumanEval to HumanEvalFix</td>
</tr>
</table>
# Use
## Intended use
The model follows instructions provided in the input. We recommend prefacing your input with "<commit_before>def has_close_elements(numbers: List[float], threshold: float) -> bool:\n for idx, elem in enumerate(numbers):\n for idx2, elem2 in enumerate(numbers):\n if idx != idx2:\n distance = elem - elem2\n if distance < threshold:\n return True\n\n return False<commit_message>Fix bugs in has_close_elements.<commit_after>"
**Feel free to share your generations in the Community tab!**
## Generation
```python
# pip install -q transformers
from transformers import AutoModelForCausalLM, AutoTokenizer
checkpoint = "bigcode/santacoderpack"
device = "cuda" # for GPU usage or "cpu" for CPU usage
tokenizer = AutoTokenizer.from_pretrained(checkpoint)
model = AutoModelForCausalLM.from_pretrained(checkpoint).to(device)
inputs = tokenizer.encode("Q<commit_before>def has_close_elements(numbers: List[float], threshold: float) -> bool:\n for idx, elem in enumerate(numbers):\n for idx2, elem2 in enumerate(numbers):\n if idx != idx2:\n distance = elem - elem2\n if distance < threshold:\n return True\n\n return False<commit_message>Fix bugs in has_close_elements.<commit_after>", return_tensors="pt").to(device)
outputs = model.generate(inputs)
print(tokenizer.decode(outputs[0]))
```
# Training
## Model
- **Architecture:** GPT-2 model with multi-query attention
- **Steps:** 250k pretraining
- **Pretraining tokens:** 131B
- **Precision:** bfloat16
## Hardware
- **Pretraining:**
- **GPUs:** 32 Tesla A100
- **Training time:** 15 days
## Software
- **Orchestration:** [Megatron-LM/Transformers](https://github.com/bigcode-project/santacoderpack#training)
- **Neural networks:** [PyTorch](https://github.com/pytorch/pytorch)
# Citation
TODO |